- Tools and Resources
- Customer Services
- Communication and Culture
- Communication and Social Change
- Communication and Technology
- Communication Theory
- Critical/Cultural Studies
- Gender (Gay, Lesbian, Bisexual and Transgender Studies)
- Health and Risk Communication
- Intergroup Communication
- International/Global Communication
- Interpersonal Communication
- Journalism Studies
- Language and Social Interaction
- Mass Communication
- Media and Communication Policy
- Organizational Communication
- Political Communication
- Rhetorical Theory
- Share This Facebook LinkedIn Twitter

Article contents
Big data and communication research.
- Ralph Schroeder Ralph Schroeder Oxford Internet Institute, University of Oxford
- https://doi.org/10.1093/acrefore/9780190228613.013.276
- Published online: 22 November 2016
Communication research has recently had an influx of groundbreaking findings based on big data. Examples include not only analyses of Twitter, Wikipedia, and Facebook, but also of search engine and smartphone uses. These can be put together under the label “digital media.” This article reviews some of the main findings of this research, emphasizing how big data findings contribute to existing theories and findings in communication research, which have so far been lacking. To do this, an analytical framework will be developed concerning the sources of digital data and how they relate to the pertinent media. This framework shows how data sources support making statements about the relation between digital media and social change. It is also possible to distinguish between a number of subfields that big data studies contribute to, including political communication, social network analysis, and mobile communication.
One of the major challenges is that most of this research does not fall into the two main traditions in the study of communication, mass and interpersonal communication. This is readily apparent for media like Twitter and Facebook, where messages are often distributed in groups rather than broadcast or shared between only two people. This challenge also applies, for example, to the use of search engines, where the technology can tailor results to particular users or groups (this has been labeled the “filter bubble” effect). The framework is used to locate and integrate big data findings in the landscape of communication research, and thus to provide a guide to this emerging area.
- communication
- social media
- microblogging
- mobile phones
- information seeking
- communication and critical studies
Introduction
Communication research has recently seen a large number of publications with groundbreaking findings based on big data. Examples include analyses of microblogging services (Twitter), online information sources (Wikipedia), social network sites (Facebook), search engine behavior (Google), and smartphone uses. The main reason why big data research has become so prominent is that new sources of digital data have become available that were not previously accessible to researchers. At the same time, the question of just how accessible these sources are, since most of these data come from commercial companies, is perhaps the single most important challenge to this otherwise burgeoning of research. The second important challenge, but one which may be resolved in due course, is that most of this research does not fall into the two main traditions in the study of communication, mass and interpersonal communication. That is because digital media are often in between the two, as when Facebook users share news among their groups of friends, or when Twitter hashtags are created for particular events so that they create an audience around the event rather than, again, being part of one-to-one or broadcast communication.
This second challenge will be a theme below, so it is worth spelling out further at the start: on Twitter and Facebook, people look at links based on what their friends or followers post or on their feeds, which are based partly on the people in their networks. This means that even if the link comes from a traditional news source, it is often shared among groups rather than being broadcast or exchanged between only two people. The same applies to search engines, which can be seen as gatekeepers inasmuch as they tailor content to particular audiences (Pariser, 2011 ), even if, unlike traditional mass media, they do not themselves produce content. Another example is Wikipedia, which, despite being one of the most popular websites, does not easily fall into existing categories of communication or of existing sources of information such as those produced by academic researchers or accredited professionals. The lack of a model or theory for digital or social media represents a problem for communication research generally, but makes it particularly difficult to fit big data findings into existing traditions of communications and media.
Some of the main findings in this new area of research are reviewed first. Particular emphasis is placed on how big data findings contribute to particular theories or research agendas in communication studies. It covers, in turn, research about information seeking (Wikipedia), social network sites (Facebook), the Web as a source of online information, microblogging (Twitter), search engines, and mobile phones. The discussion of these data sources will touch on a number of communication subfields to which big data studies contribute, including political communication and mobile communication. Finally, there are pointers to future directions in this area, leading to the question of how these new findings will contribute to communications research. More broadly, the conclusion also puts big data in a wider perspective: how are big data changing not just communications research, but also the role of media in society at large?
This overview will necessarily be selective: there are too many studies in this rapidly growing area for a comprehensive review (but see Ekbia et al., 2015 ; Golder & Macy, 2014 ; and the “Discussion of the Literature” and “Further Reading” below). Instead, it will be useful to highlight findings from a number of studies which represent a wide range of new digital media. In each case, we can ask: How do the data sources provide new insights? What are the main findings? Where can the findings be located in relation to existing research? And how can these findings be built upon, and what are their limitations? With this, we can begin with Wikipedia.
Wikipedia and Other Information Sources
Wikipedia has been widely researched apart from studies using big data approaches: a recent review counted almost 3,000 papers about Wikipedia by 2013 (Bar-Ilan & Aharony, 2014 ). Most big data studies focus on Wikipedia entries and on the process of collaboration. Very few studies, in contrast, have examined who reads or accesses Wikipedia, which is arguably just as important for communication research. This is so particularly since Wikipedia is the only noncommercial website that is consistently among the top ten most frequently accessed websites worldwide ( Alexa ). Wikipedia is used across the world and exists in many languages ( List of Wikipedias ), though there are competitors. The main alternative to using Wikipedia, at least in mainland China, is Baidu Baike , a Chinese-language online encyclopedia based on the Wikipedia model and developed under the auspices of the Chinese search engine company Baidu. In mainland China, Baidu Baike is more commonly used than the Chinese-language version of Wikipedia, which was banned for several years (Liao, 2009 ). Outside mainland China, among the large Chinese-speaking population outside the People’s Republic, however, Chinese Wikipedia is more popular than Baidu Baike. One reason to mention this is that if we have big data findings about Wikipedia, either for the English-speaking version or for the Chinese or other language versions, it is worth bearing in mind that Wikipedia is used very widely, but not universally.
Wikipedia is an important source of big data because it is openly accessible to researchers—which makes it more reliable as a data source than proprietary sources. In addition to openness, the research in this case can be built upon (replicability), which is a criterion for valid scientific knowledge (a point that is discussed further in the conclusion). In this respect, it is useful to take a quick detour to consider the criticisms of a widely referenced study that used Google Trends to attempt to predict flu outbreaks. In the study (Ginsberg et al., 2009 ), researchers claimed that flu could be predicted by analyzing how often and where “flu” and related search terms were being sought on the Google search engine. Lazer, Kennedy, King, and Vespignani ( 2014 ) criticized this study for its methodology, but also on the grounds that Google’s “black box” did not allow researchers to ascertain how Google Trends are arrived at.
How does this relate to Wikipedia? Another group of researchers went on to show that accessing articles about flu and other diseases on Wikipedia can predict disease outbreaks more accurately than Google Trends (Generous, Fairchild, Deshpande, Del Valle, & Priedhorsky, 2014 , see also McIver & Brownstein, 2014 ). (Tellingly, and echoing the point made earlier about online encyclopedias in China as against the world-at-large, the one disease that could not be predicted is Ebola, which mainly affects people in West Africa, where Internet penetration is low, while Wikipedia articles about Ebola were mainly accessed in rich countries: in rich countries, Internet use is widespread, but Ebola is exceedingly rare.) What the comparison between disease prediction using Google Flu Trends and Wikipedia highlights is that knowledge derived from Wikipedia is open and can be built upon. Indeed, the Wikipedia researchers in this case have made their datasets available and have encouraged others to replicate and improve upon or criticize their results.
This brings us to another feature of big data studies, which is that it is often not known who the users are that leave digital traces. This point also applies to studies of Wikipedia. However, West, Weber, and Castillo ( 2012 ) had access to log data about the behavior of people who edited Wikipedia and who used the Yahoo! tool bar in their web browser. They studied these Wikipedia contributors who use Yahoo! to analyze the kinds of information that they seek. This allowed them to link the edits the contributors made to Wikipedia with the kind of knowledge they brought to the task, based on their browsing (in this case, Yahoo!) behavior. What they found, among other things, is that contributors to the entertainment-related part of Wikipedia, which makes up seven of the ten largest categories of article topics (West et al., 2012 , section 6), look for more information on these topics than those who are not editors; put differently, editors seem to be more expert than others in the sense that they seek more information. Or again, when they break down this expertise into “science, business, and humanities” as against “entertainment-related” editors, they find that the former are more “generalist,” whereas the latter are “from editors immersed primarily in popular culture.” These findings make a start on building up a picture of what kinds of people contribute with what kind of knowledge to Wikipedia (it can be added that the authors of the study argue that Yahoo! users are unlikely to be different from people using other browsers). Perhaps we can also make inferences based on these findings about what kind of knowledge and information different types of people are interested in more generally.
Wikipedia is thus an excellent source of digital data because data from Wikipedia are available to anyone and it is possible to understand how they were generated. But Wikipedia is also a rather special digital medium, used mainly for finding information—unlike other media, such as television, which push information toward the user (recall a major point that “big data” pertains to media that are neither mass nor interpersonal). Yet the role of information seeking in everyday life is not well understood (but see Aspray & Hayes, 2011 ; Savolainen, 2008 ). In the case of Wikipedia, Waller ( 2011 ) analyzed “The search queries that took Australian Internet users to Wikipedia” (as the title of her paper has it). To do this, she had access to log data from the marketing company Hitwise Experian for Australian Internet users (again, we can note that big data studies often depend on commercial data sources). Almost all visitors to Wikipedia (93%), she found, came from Google. Interestingly, she also found that the entries that people sought were quite diverse: of the 600,000 search queries that took users to Wikipedia, at least 400,000 appear only once. And if we recall that Wikipedia is one of the world’s most popular websites, it is notable how diverse the information is that people are looking for. In terms of the content that people looked for (Waller analyzed a subset of queries and classified them), 36% pertained to popular culture such as movie or music stars (mostly American) and 2% to high culture. Or again, 7% pertained to science and 6% to history. At the same time, she found only minor, though significant, differences among the population in terms of income and other demographic characteristics of which groups sought what kind of content. And again, it is worth stressing that this kind of access to data (user demographics) is rare in academic big data studies.
To summarize, from the point of communication research, as opposed to research about the nature of Wikipedia entries or about online collaboration, two questions we might want to ask are: Who reads Wikipedia? What does this source of knowledge provide that other sources do not? What many big data studies have focused on is a topic that we have data about: Who contributes to Wikipedia, and how do they collaborate? These are important topics, but surely an equally important one is who makes use of Wikipedia, and about that there are also abundant data (there is a website, www.stats.grok , where data can be obtained about which Wikipedia entries are accessed). Yet this is a topic about which we know little, perhaps because researchers have found it more interesting to examine how knowledge is produced than how it is consumed. In any event, big data studies using Wikipedia provide findings about one of the most popular sources of online information, though these are hard to fit into theories of “mass” or interpersonal media (and in this case, information found on Wikipedia is mainly sought via Google, which again goes beyond traditional theories of communication). Another key point in this case is that big data research is often done on topics which can readily be analyzed (the nature of entries and how collaboration works), rather than topics which may be of broader interest to communications scholars (what information people look for on Wikipedia). Finally, Wikipedia is a noncommercial platform that makes its data freely available to researchers and is transparent about how the data were produced. As we shall see, this feature sets Wikipedia apart from most other sources of big data.
Facebook and Other Social Network Sites
Research about Facebook has led to two major controversies. The first concerned the possibility of de-anonymizing the data and was thus about privacy. It was triggered by an early study which, though not necessarily on the scale of “big data,” is mentioned here because it raised the issue not just of privacy but also of whether this data source could be made publicly available. The study analyzed students’ online ties (their Facebook “friends”) and their offline ties in relation to their cultural “tastes” (Lewis, Kaufman, Gonzalez, Wimmer, & Christakis, 2008 ). The research caused a stir when it was discovered that the university where the study was done, and perhaps the students, could be identified (Zimmer, 2010 ), which led the researchers to not make the data available to others.
The second controversy also involved questions of privacy, but in addition raised an issue distinctly related to big data. In this case, researchers experimented by dividing Facebook users, overall almost 700,000 of them, into two groups (Kramer, Guillory, & Hancock, 2014 ): one group of users had more positive words introduced into their newsfeeds, the other group was exposed to more negative words. The researchers then measured whether these users subsequently, in the light of the two “treatments,” themselves posted more positive or negative words. They found that indeed they did so, confirming “social contagion.” The issue raised in this case is whether Facebook, and academic researchers in particular who took part in the study, should carry out experiments that manipulate Facebook users (Schroeder, 2014b ). What we see here is that, regardless of the findings, the insights gained could be used to influence Facebook users’ communication patterns.
It is rare in communications research, especially at the scale of hundreds of thousands of people as opposed to small groups in a laboratory, to be able to influence people’s behaviors in this way. Another study that illustrates how social network sites influence behavior was done by Bond et al. ( 2012 ), who tested whether different types of messages from Facebook users in the United States urging their friends to vote could lead more of them to vote. Indeed, it was found (among other things) that a message from close friends (friends of friends, or two degrees of separation) had more powerful effect in increasing voter turnout than messages from more distant friends. The authors of the study argue that this kind of influence or mobilization of potential voters via social network sites could play an important role in close elections.
Yet another line of inquiry about Facebook has been about whether “friends” who share content also share political views or political ideologies. Bakshy, Messing, and Adamic ( 2015 ) investigated this question for more than ten million American Facebook users, and found that Facebook friends are ideologically quite diverse, which is partly because their ties reflect offline networks such as family, school, and work—in contrast with Twitter users, who share common interests or topics but not necessarily offline ties, and who are therefore more ideologically polarized (Conover et al., 2011 ).
A more recent study (Settle et al., 2016 ) examined the content of Facebook messages, and in particular “status updates,” which most (73%, according to Hampton, Goulet, Rainie, & Purcell, 2011 ) Facebook users make at least once a week. It can be added that 60% of Americans use Facebook and 66% use it for civic or political activity (Rainie, Smith, Schlozman, Brady, & Verba, 2012 ). Using machine learning techniques, the researchers separated out content that was political in nature in relation to the US presidential election in 2008 and the Health Care reform debate in 2009 . They were able to show that political messages closely track major events (in the case of the election, these included the party conventions, the election itself, and the inauguration; and in the case of the health care debate, the shift in the discussion from using the term “health care reform” to “Obamacare”). They could also see peaks and troughs in the use of emotional language. Again, given the large number of Americans and others who exchange political messages on Facebook, these are important findings.
At the same time, Facebook is only one of several social network sites, even if it is the dominant one in the United States and across most of the world. Yet there are many who do not use Facebook, which may impact the validity of these studies. Further, again, there are parts of the world where Facebook is almost nonexistent, such as in China (because the government has banned it), but also in Russia, where a rival social network site service (VKontakte) has become dominant. This again raises the issue for studies of Facebook users (as we also saw in the case of Chinese Wikipedia) concerning what part of the population these studies represent. And as mentioned, the question of the extent to which Facebook users accept being analyzed, even if their privacy and anonymity are safeguarded, and whether the data can be made available to other researchers will continue to be challenges. In the meantime, findings about how people behave on this popular social media platform, and how they influence each other in particular, are bound to remain growing areas of research.
The World Wide Web as a Source of Online Information
The Web is less associated with communication research using big data than the other digital media discussed here. This is partly because there are surprisingly few studies of what the Web can tell us about offline social relations generally (but see Bruegger & Schroeder, 2017 ). Studies of information seeking via Wikipedia (see “ Wikipedia and Other Information Sources ”) or search engines (see “ Search Engines ”) are of course aspects of studies of the Web, and in this sense yield insights into society. Yet the Web can also be seen as an entity in itself, which tells about what kind of information can be found online, how this information is organized or interlinked, and how it reflects (or reflects in a distorted way) wider changes in society.
One big data approach to the shape of the Web has been to test whether what is accessed online reflects offline political or cultural or linguistic borders. This is an interesting question because it has often been claimed that the Web is a unique medium insofar as it can be accessed from anywhere—unlike traditional media that are confined, for example, by national broadcasting regulations or by the reach of transmitters and the like. In other cases, most notably in China, it has been argued conversely that the government and its censorship regime ring-fence the Web, making it into a cultural resource whose reach is circumscribed by the state. Both ideas are misleading, as Taneja and Wu ( 2014 ) have shown: first, the Web in China is no less densely bounded off from the Web than other non-English-speaking large clusters on the Web. The way that Taneja and Wu arrive at this finding is by examining traffic to the top 1,000 websites (which together receive more than a 99% share of attention globally), and then grouping these into sites that receive shared attention. Shared attention is defined as when, if someone clicks on one site, they also visit another (after controlling for the statistical chance of covisiting). In the case of China, apart from language, it may be that the state’s active information and communication technology policy has promoted a Chinese-centric web, as in other cases like Korea. But the Chinese Web is not as straightforwardly or uniquely circumscribed by a wall of censorship as is commonly thought. Instead, it may simply be that Chinese citizens, like those of other nations, are primarily interested in content produced in China.
Wu and Taneja ( 2015 ) have extended this analysis to argue that the “thickening” of the Web has changed over time, such that whereas in 2009 a global/US cluster was most central on the Web and at the same time the largest, in 2011 it was overtaken by a Chinese cluster, and there was no longer a global/US cluster, but rather in second place was a US/English cluster followed by a global cluster. The same two clusters occupied the top two spots by size in 2013 , but the global cluster (of websites that are not language specific such as Mozilla and Facebook) had slipped to eighth place (India was ninth and Germany tenth), followed by a number of other clusters including sites in Japan and Russia, but also Spanish-language sites and those in Brazil and France. What we see here is the evolution of the Web becoming more oriented toward the global South (Spanish-language sites and sites in Brazil and also India). We also see, with time, that websites of “global” status have become fewer in number among the world’s top 1,000 sites, and we see language playing an increasing role. State policies promoting information and communication technologies are one factor here, and shared language another. Whatever the most important factors may turn out to be, the Web is not becoming a single whole, but rather a series of clusters: linguistic, and those that develop due to the policies of states and sites promoting shared interests such as commerce or personal relations. (It can be added that Taneja and Wu used data from ComScore, a company that analyzes web traffic, to arrive at their findings.)
Again, against the background of this kind of large-scale analysis of the shape of the Web, as with Wikipedia and other sources of online information, we would need to know how people use the Web in everyday life. But such research on how people search for information is still thin on the ground (Aspray & Hayes, 2011 ; Savolainen, 2008 ), particularly in relation to how people find information on the Web (Rieh, 2004 ; Schroeder, 2014a ). A major issue that has not yet been resolved in communication studies is where to “put” information seeking in general. A simple way to grasp this point is to ask: where did people seek information before the advent of the Web, say, in the mid-1990s? (The same point could be raised, of course, in relation to Wikipedia, and search engine behavior.) They might have consulted a book version encyclopedia instead of Wikipedia, a travel agent instead of a travel website, a pamphlet instead of a blog, and so on. Yet these “media” were also not much studied. What makes the Web different is that it contains all of this information, but also that none of these uses of the Web fit easily into categories in the study of offline behavior or that of other digital media—or indeed into the categories of mass and interpersonal communication. What these uses do fit is the subject studied by the discipline of information science, but that is a discipline with which communication scholars barely overlap (and information science rarely examines ordinary everyday behavior). In any event, the Web, in view of the fact that it is a large and accessible source of data and increasingly important in peoples’ lives, is bound to grow as a basis for big data research.
Twitter and Microblogging
Twitter has been among the most commonly used sources of academic research using big data techniques. One criticism that is often made of studies of Twitter (as of other social media, as we have seen) is that it is not known who the users are, and hence what part of the population—perhaps a very skewed one—they represent? Barberá and Rivero ( 2014 ) addressed the problem of representativeness for Twitter users by analyzing all tweets in relation to the two candidates in the Spanish legislative elections of 2011 and the American presidential elections of 2012 , in each case for 70 days before the election. They found that Twitter users are disproportionately male, somewhat biased toward urban areas, and “highly polarized: Users with clear ideological leaning are much more active and generate a majority of the content” ( 2014 , p. 3). Since they were also able to identify the follower networks of these users, they could show to what extent it mattered that a small number of users generated the majority of the content—in terms of the “reach” of their tweets. What we can see here is that one way to overcome the problem of the representativeness of Twitter users is to focus on Twitter uses for specific purposes. What we also see, however, is that this way of overcoming an unrepresentative population takes considerable effort, including finding the network of users. Further, even when this effort has been made, it will still be necessary to think about the larger context of how Twitter differs from other media, in this case during elections, especially since compared with, say, television, the role of Twitter is more protean.
Another study that gets close to who the users are, though with a different approach, was done by Bastos and Mercea ( 2015 ). They analyzed 20 million tweets in 2009–2013 for 193 political hashtags (such as those related to the Occupy movement or the protests in Iran). Then they focused on those Twitter users who frequently tweeted across several of these hashtags. These they labeled “serial activists” and interviewed 21 of them, as well as reconstructing their networks and those of their followers. What they found was that these serial activists are highly interconnected with each other, even across language barriers (which they overcome partly through peer support, and partly by means of Google Translate). But they were also able to identify who these activists were: urban adults who were much older (average age 45) than average Twitter users, typically on a low income, with a high proportion of IT professionals, and who often lived in cities that had longer periods of Occupy movement camps. The serial activists were found to be motivated by idealism (“expressive” rather than “instrumental” motives) and most of them had also participated in offline protests. Further, they displayed long-term commitment rather than short bursts of activity, refuting the idea that Twitter represents a shallow or “clicktivist” political commitment. What is interesting here is that “big” data can be combined with qualitative “small” data to show not only how political activists are located in larger social networks, but also their characteristics and motivations. This approach is useful in view of the fact that large-scale analyses of political and other forms of communication often show only highly abstract patterns of activity or patterns in networks that may be difficult to relate to what people actually do (in this case, how they are involved in political protest) and how they actually work together and interact.
A different approach of how to put Twitter in a larger context can be illustrated by reference to the study by Neuman, Guggenheim, Mo Jang, and Bae ( 2014 ). They examined agenda setting in traditional news media compared with online news media by reference to data from newspapers and television over several decades and more recently Twitter, blogs, and discussion forums. They asked: Do social media (in this case) change agenda setting compared with traditional media? Among their findings: “Social media are more responsive to public order and social issues and less responsive to the abstractions of economics and foreign affairs” ( 2014 , p. 7). This finding has interesting implications since it suggests that what journalists are interested in differs from what people are interested in when they generate content themselves (see also Boczkowski & Mitchelstein, 2013 ). Here we therefore have a unique study which both compares new and traditional media and specifically tests an existing theory in media and communications—agenda setting—with the interesting result that old and new media are different, even if, as the authors point out, it is increasingly difficult to tell them apart.
Like other digital media, Twitter is global, and yet it is variably used across the globe and competes with other microblogging services. In China, it is officially banned, even if there are many mainland Chinese who still use it (Sullivan, 2012 ). It is also an evolving medium, used by protest movements and journalists, for example, but also by ordinary people as a means of sending short messages in the manner that text messages are sent via phones. Again, although some studies exist (Duggan, 2015 ), relatively little is known about who the users are. And as with the other media and data sources discussed here, Twitter illustrates the issue of publicly versus commercially available data (Puschmann & Burgess 2013 ): Twitter makes a certain nonrepresentative sample of data available publicly (1% or so, but Twitter’s policies have changed over time and also vary by topic, and sometimes up to 10% can be obtained) via an application programming interface (API), and many academic studies have used this “sample” as opposed to the whole dataset (the “firehose,” only available for purchase). But there has been much discussion about the extent to which 1% is a biased sample, and González-Bailón, Wang, Rivero, Borge-Holthoefer, and Moreno ( 2014 ) have shown, by means of an examination of Twitter during a political protest, that a 1% sample (which they compared with the complete dataset) is highly problematic in terms of drawing valid conclusions about protest messages. Nevertheless, Twitter has been widely used to study political communication; hashtags related to protests or mentions of parties to predict elections have been analyzed, among others (Jungherr, 2015 ). It is likely Twitter will continue to be a popular source for research, if only because it is relatively easily accessible.
Search Engines
As we have seen, what information people search for has been a subject of great interest, and big data are abundant in this case, even if, as we saw with the Google flu trends study, this research is often difficult to replicate due to the proprietary and hence “blackboxed” nature of the data. Anderegg and Goldsmith ( 2014 ) examined a different topic using Google Trends: attitudes to climate change. They did this by focusing on two events which have been labeled “climategate” scandals: one concerned emails from climate researchers that were leaked and allegedly showed that they had “covered up” results that downplayed the threat of climate change. The other was a similar alleged misrepresentation of results about melting glaciers arising from a report of the Intergovernmental Panel on Climate Change (IPCC). These two events in late 2009 and early 2010 were widely covered in the media and could have been expected to lead to a shift toward greater skepticism about climate change. Anderegg and Goldsmith examined trends in searches using Google Trends for keywords related to climate change for these events. They found that, although these events led to a spike in searches that indicate increased skepticism about climate change, this effect was transient, lasting only a couple of months (for example, searches for “global warming hoax” spiked during and shortly after the events, but normalized thereafter). What the authors also found, however, was “a strong decline in public attention to climate change since 2007 ” and up to 2013 (Anderegg & Goldsmith, 2014 , p. 6). This finding should be seen in the context that the Web has come to be the single most important source of scientific information, at least in the United States (Horrigan, 2006 ).
Another interesting question is what people search for when they use search engines—in general. Waller’s study ( 2011 ), discussed earlier, had access to the logs of Australian Internet users, among whom almost 90% use Google. She found that e-commerce and popular culture topics accounted for almost half of all queries. She also had access to the demographics of the users (as mentioned, from the company Hitwise Experian), and found that queries did not differ across different groups. Further, most queries (48%) were not really queries in the sense of looking for information at all; they were “navigational” searches where users had a specific website in mind (such as Facebook or the BBC) and merely used a search engine to get to the site.
Along similar lines, Segev and Ahituv ( 2010 ) studied the 150–200 most popular searches on Google and Yahoo! across 21 countries, finding results similar to Waller’s studies of Australians, such as the preponderance of popular culture or entertainment searches. What these studies of search-engine behavior show is that a picture can be built up of people’s information interests as indicated by how they seek information about various topics. Again, if these findings can be put into context, including how they relate to which search engines are most popular where, and how people use search engines in combination with other sources, then it will be possible to build up a rich picture of people’s social behavior—in this case, neither interpersonal or mass communication behavior, but information-seeking behavior.
Mobile Phones
There have been many studies about how often people connect with others and across what kinds of distances via telephones (Fischer, 1992 ), and recently on a large scale via mobile phones (Licoppe, 2004 ). Ling, Bjelland, Sundsøy, and Campbell( 2014 ) showed that our regular and most frequent contact via mobile phones, both text and voice, is nevertheless with a small number of people. They analyzed mobile call records in Norway for a three-month period from the dominant mobile operator in the country and found that most connections are with a small group of people that are close by: “the mobile phone . . . is used in the maintenance of everyday routines with a relatively limited number of people in a relatively limited physical sphere of action . . . the stronger is our tie . . . the closer they are likely to be geographically” ( 2014 , p. 288). Like Fischer for the landline telephone, they thus disconfirm the often mooted idea of “the death of distance” or of a “global village.” They could also distinguish between rural populations, where “the largest proportion of calls is to those who are less than 1 km away,” and urban ones, where “the preponderance of calls goes to people who are more than 1, but less than 24, km distant” ( 2014 , p. 288). This is a counterintuitive finding, since it might be expected that rural people’s calls would be to more distant people and vice versa. However, if we think of the distances that urban people typically drive, also to get to work, and the age of urban and rural populations, the findings make sense (and may have implications for transport and mobile phone operator charging policies, among other things).
How mobile phones, as smartphones, are being used to access the Internet is still not well understood. Perhaps the difference between the smartphones and the Internet is becoming blurred, though Napoli and Obar ( 2015 ) argue that mobile phone users represent an “underclass” because of the much more limited functionality of mobile Internet as opposed to access via desktop or laptop computer. They do this by reviewing studies that show that desktop or laptop computers are more useful for content creation and complex tasks while smartphones are mainly used for more passive and constrained ones. Nevertheless, this argument is counterintuitive, since young people in high-income countries in particular use smartphones ever more to do a wide variety of things. Still, it is important to remember, as Donner ( 2015 ) points out, that these affluent and highly skilled smartphone users are a small minority worldwide. Moreover, affluent users almost invariably also have Internet access via laptops and other devices such as tablets, as well as having high-bandwidth connections at a (relatively) low cost, so even if their smartphone uses are constrained, they can combine them with doing more demanding tasks on other devices. Users in low-income countries in Southeast Asia and Africa, in contrast, have a “metered mindset,” with scarce bandwidth which is (relatively) very expensive and thus used frugally. These users “dip and sip,” rather than “surf and browse,” as Donner puts it, and they are also likely to have far more limited skills and uses restricted by the affordances of their smartphones. This new digital divide may close over time, but the difference between the vast majority of smartphone-only users and a minority of users with multiple devices is also likely to remain a deep fault line for many decades to come.
In any event, it can be foreseen that big data studies of mobile phones, and mobile Internet use, will become much more prominent in the future, partly because in large parts of the world, including India and China, this is the dominant way in which the Internet is accessed. Put differently, the vast majority of Internet users, globally, will for the most part access the Internet via a mobile device, and they may never have access to a laptop or desktop computer. This will be the population that will be of greatest interest to social scientists of all types, and hence smartphones as a source of big data, including the location of phone users, will become ever more important. At the same time, this type of data, which includes geographical location, is obviously more sensitive than other media or communications data.
It is also true that in this case, as in others, access to commercial data is a precondition for carrying out studies like those by Ling et al. ( 2014 ) and Licoppe ( 2004 ). In these two cases, researchers had access to the phone network studied, Telenor and France Telecom, respectively. Further, as elsewhere, one issue in carrying out big data studies concerns the territory covered by the service provider—or rather, several service providers in most cases. This challenge also applies when mobile phones are studied for the purpose of crisis communication and disease control (see Bengtsson, Lu, Thorson, Garfield, & Von Schreeb, 2011 , for a study of the movement of people during the Haiti earthquake). Nevertheless, an advantage of this type of data—when it is available—is that it provides a particularly rich source. Boase and Ling ( 2013 ) showed that log data about mobile phone use are more accurate than self-report (though again, log data may be difficult to obtain since they are owned by mobile phone operators, unless researchers collect them from users themselves).
The contrast between log data and surveying people shows the advantages of obtaining “digital footprints” as against asking people about their uses of digital media: obtaining data directly cuts out the potential biases of user self-report. On the other hand, a mobile phone, like any digital device, could be used by more than one person, or one person could use several devices, and these are just some of the errors that could be avoided by asking people directly. Multimethod studies that combine log data and self-reports or interviews may be the way forward here, though they are obviously more resource intensive. Still, they can overcome the problem that digital data are more revealing about user behavior in some senses and less so in others.
As we have seen, there are many areas in which big data are being used in communication research, and the review here has been able to give only a flavor of this rapidly expanding area. At this point it will be useful to revisit the question of why this area has garnered so much attention, and also ask: What are its prospects? Big data approaches in communication research (and in other areas of knowledge) take social science in the direction of being more quantitative and statistical, and thus more scientific and more powerful, and it is important to spell out why. Quantitative social science is of course nothing new (Porter, 2008 ). Nor are efforts to introduce digital tools and data into research (Meyer & Schroeder, 2015 ). What is new in big data research are the data sources, which provide access to readily manipulable (computable) data. Social science data in the past have been hard to come by, mainly requiring face-to-face interviews or telephone surveys, and digital data are often fraught with difficulties in the case of proprietary and/or sensitive data. Still, an important point here is that the availability of data is a precondition for the growth of social scientific knowledge: data provide an independent means to check or verify (or falsify) results; they are the raw material that allows researchers to build on each other’s work. Having more of these materials, about an aspect of our social lives that is itself rapidly growing, means that this area of research is bound to continue to thrive.
This point can be made differently, by defining data—at least inasmuch as data are part of scientific knowledge. Data belong to (in the nonlegal sense of being a property of) the object under investigation; taking data comes before interpreting them; and data are the most atomic or divisible useful units of analysis (Schroeder, 2014b ). The definition of “big” data, data on a scale and with a scope that is a leap beyond what was previously available in relation to a given phenomenon, thus relates directly to the availability of this raw material, especially in a form that can be readily manipulated or computed (and all the studies using big data in this article certainly meet this definition). No wonder then that communication research, and social research, has recently seen a surge of studies in this area, especially as the software tools to handle these data have also recently proliferated (see Bright, 2017 ). The caveats, that the data need to be such as to meet the criteria of science, of being open to validation and replication, do not need to be stressed again as they have been amply discussed above. Yet often, these criteria are not met, so that while quantitative or scientific knowledge is rapidly advancing in one sense, it also rests on uncertain foundations in another (though again, Wikipedia is a counterexample, and there are others, such as data about the Web and its links). Further, the validity of having only samples of big data, as we saw in the case of samples of Twitter data, can be subject to rigorous investigation too.
As an aside, it can be mentioned that another feature of scientific advance that often applies to big data research is that studies build and improve on each other; that is, “high-consensus rapid-discovery” science (Collins, 1994 ; Schroeder, 2007 ): examples include how Wikipedia disease prediction has outperformed Google Flu Trends research (as we have seen) or how Wikipedia results (Mestyán, Yasseri, & Kertész, 2013 ) have bested Twitter research (Asur & Huberman, 2010 ) in predicting movie box office success. It can also be noted, however, that big data in communication research are still largely in a phase of high task uncertainty and low mutual dependence (Whitley, 2000 ): that is, researchers are exploring many new domains, often without a sense of how this research may contribute to cumulation (Rule, 1997 ). Hence there is a need, in communication studies and in other areas of social science, for dialogue across the various disciplines that are pursuing research based on new sources of digital data. In this respect it may have been noticed that among the studies that have been discussed, many have not been done by communication researchers or social scientists but rather by, for example, computer scientists and researchers in the commercial sector.
Whether the social sciences should be more scientific has of course been a matter of contention. Suffice it to say here that big data approaches can be combined with other, qualitative or mixed methods approaches, as we have seen. Yet the more powerful insights based on these data sources have also been limited, again, for other reasons. First, many studies are not generalizable (or they cannot be built upon) because the data come from proprietary social media or from mobile phones. This means that the findings cannot be replicated, since the data are not accessible to other researchers—or it is not known how the data were generated in the first place. Second, big data findings are often of limited significance because they are aimed at short-term practical goals, such as when big data are analyzed for marketing purposes so that findings may not apply beyond a particular marketing campaign and the specific population being targeted or the products being sold (and in this case, the question of whether the findings are scientific may be moot). Third, studies may be limited because the source of digital data covers only a part of the world’s population, even if it is large one, for reasons of language or censorship or because a particular platform is only one of several popular ones. Fourth, and this is the reason that has been emphasized here, many digital data sources are being investigated in different directions without a sense of how findings fit into the larger picture of communication research. The first two are practical problems, and the third pertains to the scope of the study. Yet the fourth, which is a question of making an effort in the direction of theorizing, synthesizing, and integrating findings, can be overcome (indeed, the foregoing has pointed to ways of doing so).
Important questions for the future of this area thus include how to compare traditional and new media (the Neuman et al., 2014 , study was highlighted as a particularly good example). And in view of the fact that there is such a proliferation of new media, it must be established how these fit into people’s overall media “diets,” and with what effects. Further, it would be useful know about the demographic and other characteristics of the users of the new digital media (the objects of study to which the data “belong”), and how data shed light not just on the specific social or media behaviors related to the devices they use but also on the larger dynamics of the role of these media in society. Again, much has been said earlier about the fact that these new media have different user populations, partly limited by language, geography, uneven access to the Internet, and other factors. How can these be compared with traditional media, which are often studied at the national or regional levels, and again, how can the sum total of traditional and new media be aggregated into an overall understanding of the role of media within and across societies? These are ambitious questions, but ultimately big data research will no longer be a specialized subfield, but will become part of the larger advance of social scientific knowledge, albeit an ever-growing part because of the increasing amounts of digital data available about social interaction.
Big data studies will thus also require new theories since digital media uses are changing rapidly. Traditional analog media are steadily declining and being displaced by digital media. One consequence (mentioned briefly at the outset) is that digital media constitute a shift away from interpersonal communication (one to one) and mass communication (one to many) toward interaction at levels between the two, as when content is shared on Twitter and Facebook or search engine results are tailored to a particular group. New media can be targeted to audiences or content shared by users such that they are not aimed at mass audiences nor limited to interactions between individuals (again, Twitter and Facebook, but also Google search results, are examples). This does not mean that traditional media are dying out; rather, they are slowly fading as people use digital media more. These new digital media add another layer to how the world is becoming mediated and take another step in the ongoing process whereby technologies tether us more closely to information and to each other (Schroeder, 2010 ).
If it is possible to make progress in the challenge of locating big data research findings and integrating them within what we know about the role of media (Neuman, 2016 ), it should not be forgotten that big data research on digital media—for the reasons mentioned (data is often proprietary), but also because of the usefulness of this knowledge—is mainly carried out outside academia (Savage & Burrows, 2007 , 2009 ), primarily for marketing and advertising, as well as for policy and government purposes. Audience “engagement,” market shares, health and transportation, public opinion—these and other aspects of life are increasingly measured in a quantitative way and treated as commercial assets or means of governing. Big data here are part of a broader move toward more scientific, quantitative, and data-driven approaches, not just in communication research but also in the study of politics, policy, and economics. Yet these studies have little value to social scientific knowledge about media unless they can be validated and built upon. Much therefore depends on the data sources. Parks has therefore compared the current situation of communication researchers with what has happened in the past with biomedical researchers who use commercial data: “Communication researchers may have to contend with the fact that companies will grant access only to data that they believe will reflect positively on upon their commercial interests. They will discover, as biomedical researchers have, that sponsorship and assistance often comes with strings” (Parks, 2014 , p. 360). Perhaps; though it is worth pointing out that unlike data in biomedical research, data from social media and mobile phones soon lose their value, so that there may reasons for commercial and other actors to share at least older data.
There is thus a broader societal context to big data research, which is that communications and social science research is only a small part of the research effort. Big data research is much more widespread in the commercial sector and in government and other organizations, where it is used for practical purposes—social engineering, if you like. The main effect, in the United States, Europe, and elsewhere, is that consumer marketing becomes more effective. Another main area of application is public opinion measurement. However, here the context becomes important: in China, for example, this type of research can be used not just to get feedback from the population but also for systematic surveillance purposes (of course, China is not alone in this, but the preconditions for more powerful such uses are perhaps unique to China, at least on a large scale; see Stockmann, 2013 ).
These kinds of nonacademic—social engineering—uses of big data research will expand and continue to bring benefits (marketing, governance) and dangers (surveillance, manipulation). In the meantime, it is worth remembering that even if the benefits of new digital data sources continue to grow and proliferate for academic or scientific knowledge, and even with the growing role of social media and other digital media, the findings will be limited by the extent to which digital data shed light on user behavior. And while these findings will grow, again, they should fit into broader knowledge about people’s media uses and patterns of social interaction. In this respect, the problem that new digital media often do not fit the established paradigms of mass versus interpersonal communication can be seen as a useful opportunity to develop new theories of communication rather than as a limitation.
Discussion of the Literature
Big data research is still too new to have established a body of literature. A number of reviews of this research have been produced, such as Ekbia et al. ( 2015 ) and Golder and Macy ( 2014 ). In relation to communication research in particular, the book by Neuman ( 2016 ) is about theories of the Internet generally, but discusses big data on a number of occasions in relation to existing communication theories. Jungherr ( 2015 ) provides an overview of Twitter research in politics. Schroeder and Taylor ( 2015 ) give an overview of Wikipedia research. Evans and Aceves ( 2016 ) review the various automated techniques for analyzing texts. There is also an extensive literature about the ethical, legal, and social implications of big data, as opposed to big data in academic research (though the two sometimes intersect); an overview of these can be found in Pasquale ( 2015 ).
Acknowledgments
Thank you for the helpful comments from Cornelius Puschmann.
Further Reading
Those interested in big data in communication research may wish to obtain overviews, also in relation to particular data sources (see “ Discussion of the Literature ”). Apart from this, publications about big data in communication research are quite disparate, and interested readers may want to pursue works related to particular types of social media (for example, microblogs such as Twitter or social network sites like Facebook), or they may want to explore particular areas of communication (political communication, marketing, and crisis communication are examples) or particular methods (social network analysis, sentiment analysis, or experiments). An interesting analysis of how big data techniques can be applied at different scales—from individuals, to larger units such as cities and nation-states, all the way to the global level, can be found in Eagle and Greene ( 2014 ), though this book does not focus specifically on communication research. The journal Big Data and Society is devoted to social science research in this area, and there is a special issue of the Journal of Communication (April 2014) specifically devoted to big data and communication research.
- Anderegg, W. R. , & Goldsmith, G. R. (2014). Public interest in climate change over the past decade and the effects of the “climategate” media event. Environmental Research Letters , 9 (5), 054005.
- Aspray, W. , & Hayes, B. (Eds.). (2011). Everyday information: The evolution of information seeking in America . Cambridge, MA: MIT Press.
- Asur, S. , & Huberman, B. A. (2010). Predicting the future with social media. Proceedings of the 2010 IEEE/WIC/ACM International Conference on Web intelligence and intelligent agent technology . Vol. 1, pp. 492–499. Washington, DC: IEEE Computer Society.
- Bakshy, E. , Messing, S. , & Adamic, L. A. (2015). Exposure to ideologically diverse news and opinion on Facebook. Science , 348 (6239), 1130–1132.
- Barberá, P. , & Rivero, G. (2014). Understanding the political representativeness of Twitter users. Social Science Computer Review , 33 (6), 712–729.
- Bar-Ilan, J. , & Aharony, N. (2014). Twelve years of Wikipedia research. WebSci ’14: Proceedings of the 2014 Conference on Web Science (pp. 243–244). New York, NY: ACM.
- Bastos, M. T. , & Mercea, D. (2015). Serial activists: Political Twitter beyond influentials and the twittertariat. New Media and Society , 18(10), 2359-2378.
- Bengtsson, L. , Lu, X. , Thorson, A. , Garfield, R. , & Von Schreeb, J. (2011). Improved response to disasters and outbreaks by tracking population movements with mobile phone network data: A post-earthquake geospatial study in Haiti. PLoS Med , 8 (8), e1001083.
- Boase, J. , & Ling, R. (2013). Measuring mobile phone use: Self‐report versus log data. Journal of Computer‐Mediated Communication , 18 (4), 508–519.
- Boczkowski, P. , & Mitchelstein, E. (2013). The news gap: When the information preferences of the media and the public diverge . Cambridge, MA: MIT Press.
- Bond, R. M. , Fariss, C. J. , Jones, J. J. , Kramer, A. D. I. , Marlow, C. , Settle, J. E. , & Fowler, J. H. (2012). A 61-million-person experiment in social influence and political mobilization. Nature , 489 , 295–298.
- Bright, J. (2017). “Big social science”: Doing big data in the social sciences. In N. Fielding , R. M. Lee , & G. Blank (Eds.), Handbook of Online Research Methods (chapter 12). London, UK: SAGE.
- Bruegger, N. , & Schroeder, R. (Eds.). (2017). The Web as history . London, UK: UCL Press.
- Collins, R. (1994). Why the social sciences won’t become high-consensus, rapid-discovery science. Sociological Forum , 9 (2), 155–177.
- Conover, M. , Ratkiewicz, J. , Francisco, M. R. , Gonçalves, B. , Flammini, A. , & Menczer, F. (2011). Political polarization on Twitter. Proceedings of the Fifth International AAAI Conference on Weblogs and Social Media , 133, 89–96. Palo Alto, CA: AAAI.
- Donner, J. (2015). After access: Inclusion, development, and a more mobile Internet. Cambridge, MA: MIT Press.
- Duggan, M. (2015). Mobile messaging and social media 2015. Pew Research Center, Internet, Science, and Tech , March 17–April 12.
- Eagle, N. , & Greene, K. (2014). Reality mining: Using big data to engineer a better world . Cambridge, MA: MIT Press.
- Ekbia, H. , Mattioli, M. , Kouper, I. , Arave, G. , Ghazinejad, A. , Bowman, T. , & Sugimoto, C. R. (2015). Big data, bigger dilemmas: A critical review. Journal of the Association for Information Science and Technology , 66 (8), 1523–1545.
- Evans, J. & Aceves, P. (2016). Machine translation: Mining text for social theory . Annual Review of Sociology , 42 : 18.1–18.30.
- Fischer, C. (1992). America calling: A social history of the telephone to 1940 . Berkeley: University of California Press.
- Generous, N. , Fairchild, G. , Deshpande, A. , Del Valle, S. Y. , & Priedhorsky, R. (2014). Global disease monitoring and forecasting with Wikipedia. PLoS Computational Biology , 10 (11), e1003892.
- Ginsberg, J. , Mohebbi, M. , Patel, R. S. , Brammer, L. , Smolinski, M. , & Brilliant, L. (2009). Detecting influenza epidemics using search engine query data. Nature , 457 (7232), 1012–1014.
- Golder, S. , & Macy, M. (2014). Digital footprints: Opportunities and challenges for online social research. Annual Review of Sociology , 40 , 6.1–6.24.
- González-Bailón S. , Wang, N. , Rivero, A. , Borge-Holthoefer, J. , & Moreno Y. (2014) Assessing the bias in samples of large online networks. Social Networks , 38 , 16–27.
- Hampton, K. , Goulet, L. S. , Rainie, L. , & Purcell, K. (2011). Social networking sites and our lives. Pew Research Center, Internet, Science, and Tech , June 16.
- Horrigan, J. B. (2006). The Internet as a resource for news and information science. Pew Pew Research Center, Internet, Science, and Tech , November 20. http://www.pewinternet.org/2006/11/20/the-internet-as-a-resource-for-news-and-information-about-science/ .
- Jungherr, A. (2015). Analyzing political communication with digital trace data: The role of twitter messages in social science research . London, UK: Springer.
- Kramer, A. , Guillory, J. , & Hancock, J. (2014). Experimental evidence of massive-scale emotional contagion through social networks. Proceedings of the National Academy of Sciences , 111 (24), 8788–8790.
- Lazer, D. , Kennedy, R. , King, G. , & Vespignani, A. (2014). The parable of Google flu: Traps in big data analysis. Science , 343 (6176), 1203–1205.
- Lewis, K. , Kaufman, J. , Gonzalez, M. , Wimmer, A. , & Christakis, N. (2008). Tastes, ties, and time: A new social network dataset using Facebook.com. Social Networks , 30 (4), 330–342.
- Liao, H.-T. (2009). Conflict and consensus in the Chinese version of Wikipedia. IEEE Technology and Society Magazine , 28 (2), 49–56.
- Licoppe, C. (2004). “Connected” presence: The emergence of a new repertoire for managing social relationships in a changing communication technoscape. Environment and Planning D: Society and Space , 22 (1), 135–156.
- Ling, R. , Bjelland, J. , Sundsøy, P. R. , & Campbell, S. W. (2014). Small circles: Mobile telephony and the cultivation of the private sphere. Information Society , 30 (4), 282–291.
- McIver, D. , & Brownstein, J. (2014). Wikipedia usage estimates prevalence of influenza-like illness in the United States in near real-time. PLoS Computational Biology , 10 (4), e1003581.
- Mestyán, M. , Yasseri, T. , & Kertész, J. (2013). Early prediction of movie box office success based on Wikipedia activity big data. PLoS ONE , 8 (8), e71226.
- Meyer, E. T. , & Schroeder, R. (2015). Knowledge machines: Digital transformations of the sciences and humanities . Cambridge, MA: MIT Press.
- Napoli, P. , & Obar, J. (2015). The emerging mobile Internet underclass: A critique of mobile Internet access. Information Society , 30 (5), 323–334.
- Neuman, W. R. (2016). The digital difference: Media technology and the theory of communication effects. Cambridge, MA: Harvard University Press.
- Neuman, W. R. , Guggenheim, L. , Mo Jang, S. , & Bae, S. Y. (2014). The dynamics of public attention: Agenda-setting theory meets big data. Journal of Communication , 64 , 193–214.
- Pariser, E. (2011). The filter bubble: What the Internet is hiding from you . London, UK: Penguin.
- Parks, M. (2014). Big data in communication research: Its contents and discontents. Journal of Communication , 64 , 355–360.
- Pasquale, F. (2015). The black box society: The secret algorithms that control money and information . Cambridge, MA: Harvard University Press.
- Porter, T. (2008). Statistics and statistical methods. In T. Porter & D. Ross (Eds.), The modern social sciences (pp. 238–250) . Cambridge, UK: Cambridge University Press.
- Puschmann, C. , & Burgess, J. (2013). The politics of Twitter data. In K. Weller , A. Bruns , J. Burgess , M., Mahrt , & C. Puschmann (Eds.), Twitter and society (pp. 43–54) . Oxford, UK: Peter Lang.
- Rainie, L. , Smith, A. , Schlozman, K. L. , Brady, H. , & Verba, S. (2012). Social media and political engagement. Pew Research Center, Internet, Science, and Tech , October 19.
- Rieh, S. Y. (2004). On the Web at home: Information seeking and Web searching in the home environment. Journal of the American Society for Information Science and Technology , 55 (8), 743–753.
- Rule, J. (1997). Theory and progress in social science . Cambridge, UK: Cambridge University Press.
- Savage, M. , & Burrows, R. (2007). The coming crisis of empirical sociology. Sociology , 41 (5), 885–899.
- Savage, M. , & Burrows, R. (2009). Some further reflections on the coming crisis of empirical sociology. Sociology , 43 (4), 762–772.
- Savolainen, R. (2008). Everyday information practices: A social phenomenological perspective . Lanham, MD: Scarecrow.
- Schroeder, R. (2007). Rethinking science, technology and social change. Stanford, CA: Stanford University Press.
- Schroeder, R. (2010). Mobile phones and the inexorable advance of multimodal connectedness. New Media and Society , 12 (1), 75–90.
- Schroeder, R. (2014a). Does Google shape what we know? Prometheus: Critical Studies in Innovation , 32 (2), 145–160.
- Schroeder, R. (2014b). Big data and the brave new world of social media research. Big Data and Society , July–December, 1–11.
- Schroeder, R. , & Taylor, L. (2015). Big data and Wikipedia research: Social science knowledge across disciplinary divides. Information, Communication, and Society , 18 (9), 1039–1056.
- Segev, E. , & Ahituv, N. (2010). Popular searches in Google and Yahoo!: A “digital divide” in information uses? The Information Society , 26 (1), 17–37.
- Settle, J. E. , Fariss, C. J. , Bond, R. M. , Jones, J. J. , Fowler, J. H. , Coviello, L. , . . . Marlow, C. (2016). Quantifying political discussion from the universe of Facebook status updates . Social Science Research Network .
- Stockmann, D. (2013). Media commercialization and authoritarian rule in China. Cambridge, UK: Cambridge University Press.
- Sullivan, J. (2012). A tale of two microblogs in China. Media, Culture, and Society , 34 (6), 773–783.
- Taneja, H. , & Wu, A. X. (2014). Does the Great Firewall really isolate the Chinese? Integrating access blockage with cultural factors to explain Web user behavior. Information Society , 30 (5), 297–309.
- Waller, V. (2011). The search queries that took Australian Internet users to Wikipedia . Information Research , 16 (2).
- West, R. , Weber, I. , & Castillo, C. (2012). Drawing a data-driven portrait of Wikipedia editors. Proceedings of the Eighth Annual International Symposium on Wikis and Open Collaboration—WikiSym ’12 . New York: ACM.
- Whitley, R. (2000). The intellectual and social organization of the sciences . 2nd ed. Oxford, UK: Oxford University Press.
- Wu, A. X. , & Taneja, H. (2015). Reimagining Internet geographies: A user-centric ethnological mapping of the world wide web. Journal of Computer-Mediated Communication , 21 (3), 230–246.
- Zimmer, M. (2010). “But the data is already public”: On the ethics of research in Facebook. Ethics and Information Technology , 12 (4), 313–325.
Related Articles
- Selective Avoidance and Exposure
- Policies for Online Search
- Internet Neutrality
- Blogging, Microblogging, and Exposure to Health and Risk Messages
- Grounded Theory Methodology
- Meta-Analysis in Health and Risk Messaging
- Big Data's Role in Health and Risk Messaging
- Political Economy of the Media
- Digital Cultures and Critical Studies
- Media Technologies in Communication and Critical Cultural Studies
Printed from Oxford Research Encyclopedias, Communication. Under the terms of the licence agreement, an individual user may print out a single article for personal use (for details see Privacy Policy and Legal Notice).
date: 12 July 2024
- Cookie Policy
- Privacy Policy
- Legal Notice
- Accessibility
- [91.193.111.216]
- 91.193.111.216
Character limit 500 /500
Innovative Data Communication Technologies and Application
Proceedings of ICIDCA 2021
- Conference proceedings
- © 2022
- Jennifer S. Raj 0 ,
- Khaled Kamel 1 ,
- Pavel Lafata 2
Department of Electronics and Communication Engineering, Gnanamani College of Technology, Namakkal, India
You can also search for this editor in PubMed Google Scholar
Department of Computer Science, Texas Southern University, Houston, USA
Department of telecommunication engg, czech technical university in prague, prague, czech republic.
- Presents research works in the field of data communication
- Provides original works presented at ICIDCA 2021 held in Coimbatore, India
- Serves as a reference for researchers and practitioners in academia and industry
Part of the book series: Lecture Notes on Data Engineering and Communications Technologies (LNDECT, volume 96)
78k Accesses
105 Citations
1 Altmetric
This is a preview of subscription content, log in via an institution to check access.
Access this book
- Available as EPUB and PDF
- Read on any device
- Instant download
- Own it forever
- Compact, lightweight edition
- Dispatched in 3 to 5 business days
- Free shipping worldwide - see info
Tax calculation will be finalised at checkout
Other ways to access
Licence this eBook for your library
Institutional subscriptions
About this book
This book presents the latest research in the fields of computational intelligence, ubiquitous computing models, communication intelligence, communication security, machine learning, informatics, mobile computing, cloud computing, and big data analytics. The best selected papers, presented at the International Conference on Innovative Data Communication Technologies and Application (ICIDCA 2021), are included in the book. The book focuses on the theory, design, analysis, implementation, and application of distributed systems and networks.
Similar content being viewed by others
Related Technologies
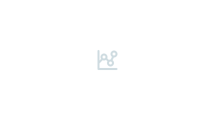
Ubiquitous information service networks and technology based on the convergence of communications, computing and control
A survey of iot key enabling and future technologies: 5g, mobile iot, sematic web and applications.
- Communication Intelligence
- Data Computing
- Big Data Analytics
- Mobile Computing
- Network Security
- ICIDCA 2021
Table of contents (78 papers)
Front matter, mobile application for tour planning using augmented reality.
- P. Babar, A. Chaudhari, S. Deshmukh, A. Mhaisgawali
Wrapper-Naive Bayes Approach to Perform Efficient Customer Behavior Prediction
- R. Sıva Subramanıan, D. Prabha, B. Maheswari, J. Aswini
ECG Acquisition Analysis on Smartphone Through Bluetooth and Wireless Communication
- Renuka Vijay Kapse, Alka S. Barhatte
Enhanced Security of User Authentication on Doctor E-Appointment System
- Md Arif Hassan, Monirul Islam Pavel, Dewan Ahmed Muhtasim, S. M. Kamal Hussain Shahi, Farzana Iasmin Rumpa
Enhanced Shadow Removal for Surveillance Systems
- P. Jishnu, B. Rajathilagam
Vehicle Speed Estimation and Tracking Using Deep Learning and Computer Vision
- B. Sathyabama, Ashutosh Devpura, Mayank Maroti, Rishabh Singh Rajput
Transparent Blockchain-Based Electronic Voting System: A Smart Voting Using Ethereum
- Md. Tarequl Islam, Md. Sabbir Hasan, Abu Sayed Sikder, Md. Selim Hossain, Mir Mohammad Azad
Usage of Machine Learning Algorithms to Detect Intrusion
- S. Sandeep Kumar, Vasantham Vijay Kumar, N. Raghavendra Sai, M. Jogendra Kumar
Speech Emotion Analyzer
- Siddhant Samyak, Apoorve Gupta, Tushar Raj, Amruth Karnam, H. R. Mamatha
A Review of Security Concerns in Smart Grid
- Jagdish Chandra Pandey, Mala Kalra
An Efficient Email Spam Detection Utilizing Machine Learning Approaches
- G. Ravi Kumar, P. Murthuja, G. Anjan Babu, K. Nagamani
Malware Prediction Analysis Using AI Techniques with the Effective Preprocessing and Dimensionality Reduction
- S. Harini, Aswathy Ravikumar, Nailesh Keshwani
Statistical Test to Analyze Gene Microarray
- M. C. S. Sreejitha, P. Sai Priyanka, S. Meghana, Nalini Sampath
An Analysis on Classification Models to Predict Possibility for Type 2 Diabetes of a Patient
- Ch. V. Raghavendran, G. Naga Satish, N. S. L. Kumar Kurumeti, Shaik Mahaboob Basha
Preserve Privacy on Streaming Data During the Process of Mining Using User Defined Delta Value
- Paresh Solanki, Sanjay Garg, Hitesh Chhikaniwala
A Neural Network based Social Distance Detection
- Sirisha Alamanda, Malthumkar Sanjana, Gopasi Sravani
An Automated Attendance System Through Multiple Face Detection and Recognition Methods
- K. Meena, J. N. Swaminathan, T. Rajendiran, S. Sureshkumar, N. Mohamed Imtiaz
Building a Robust Distributed Voting System Using Consortium Blockchain
- Rohit Rajesh Chougule, Swapnil Shrikant Kesur, Atharwa Ajay Adawadkar, Nandinee L. Mudegol
Analysis on the Effectiveness of Transfer Learned Features for X-ray Image Retrieval
- Gokul Krishnan, O. K. Sikha
Editors and Affiliations
Jennifer S. Raj
Khaled Kamel
Pavel Lafata
About the editors
Dr. Jennifer S Raj received the Ph.D. degree from Anna University and master’s degree in communication System from SRM University, India. Currently, she is working in the Department of ECE, Gnanamani College of Technology, Namakkal, India. She is a life member of ISTE, India. She has been serving as an organizing chair and a program chair of several international conferences and in the program committees of several international conferences. She is the book reviewer for Tata McGraw Hill publication and published more than fifty research articles in the journals and IEEE conferences. Her interests are in wireless healthcare informatics and body area sensor networks.
Dr. Khaled Kamel A is currently a professor of Computer Science at TSU. He worked as a full-time faculty and administrator for 22 years at the University of Louisville Engineering School. He was a professor and the chair of the Computer Engineering and Computer Science Department from August 1987 to January 2001. He also was the founding dean of the College of IT at the United Arab Emirates University and the College of CS & IT at the Abu Dhabi University. Dr. Kamel received a B.S. in electrical engineering from Cairo University, a B.S. in mathematics from Ain Shams University, an M.S. in CS from Waterloo University, and a Ph.D. in ECE from the University of Cincinnati. Dr. Kamel worked as a principle investigator on several government and industry grants. He also supervised over 100 graduate research master and doctoral students in the past 25 years. His current research interest is more interdisciplinary in nature but focuses on the use of IT in industry and systems. Dr. Kamel’s area of expertise is computer control, sensory fusion, and distributed computing.
Bibliographic Information
Book Title : Innovative Data Communication Technologies and Application
Book Subtitle : Proceedings of ICIDCA 2021
Editors : Jennifer S. Raj, Khaled Kamel, Pavel Lafata
Series Title : Lecture Notes on Data Engineering and Communications Technologies
DOI : https://doi.org/10.1007/978-981-16-7167-8
Publisher : Springer Singapore
eBook Packages : Intelligent Technologies and Robotics , Intelligent Technologies and Robotics (R0)
Copyright Information : The Editor(s) (if applicable) and The Author(s), under exclusive license to Springer Nature Singapore Pte Ltd. 2022
Softcover ISBN : 978-981-16-7166-1 Published: 25 February 2022
eBook ISBN : 978-981-16-7167-8 Published: 24 February 2022
Series ISSN : 2367-4512
Series E-ISSN : 2367-4520
Edition Number : 1
Number of Pages : XX, 1052
Number of Illustrations : 121 b/w illustrations, 461 illustrations in colour
Topics : Computational Intelligence , Computer Systems Organization and Communication Networks , Statistics, general
- Publish with us
Policies and ethics
- Find a journal
- Track your research
A Brief Study on Data Communication and Computer Networks
7 Pages Posted: 4 Sep 2021
Saideep Sunkari
Kakatiya Institute of Technology and Science, Warangal
Date Written: August 13, 2021
A computer network, sometimes known as a data network, is a kind of telecommunications network that enables computers to communicate with one another. Data is passed between networked computing devices through data links in computer networks. Cable or wireless media are used to establish connections (network links) between nodes. The Internet is the most well-known computer network. Network nodes are computer devices that originate, transport, and terminate data on a network. Hosts such as personal computers, phones, servers, and networking gear are examples of nodes. When one device can share information with another device, whether or not they have a direct connection, they are said to be networked together. Access to the World Wide Web, shared usage of application and storage servers, printers, and fax machines, and the use of email and instant messaging apps are all supported via computer networks. The physical medium used to carry signals, the communications protocols used to arrange network traffic, the network's scale, topology, and organizational intent all vary across computer networks.
Keywords: Computer Networks, Network Classifications, Local Area Network, Coaxial Cables, Network Security, Physical Layer
Suggested Citation: Suggested Citation
Saideep Sunkari (Contact Author)
Kakatiya institute of technology and science, warangal ( email ).
Yerragattu Hillock Hasanparthy Warangal, IN 506015 India
Do you have a job opening that you would like to promote on SSRN?
Paper statistics, related ejournals, information systems ejournal.
Subscribe to this fee journal for more curated articles on this topic
Communication & Technology eJournal
IEEE Account
- Change Username/Password
- Update Address
Purchase Details
- Payment Options
- Order History
- View Purchased Documents
Profile Information
- Communications Preferences
- Profession and Education
- Technical Interests
- US & Canada: +1 800 678 4333
- Worldwide: +1 732 981 0060
- Contact & Support
- About IEEE Xplore
- Accessibility
- Terms of Use
- Nondiscrimination Policy
- Privacy & Opting Out of Cookies
A not-for-profit organization, IEEE is the world's largest technical professional organization dedicated to advancing technology for the benefit of humanity. © Copyright 2024 IEEE - All rights reserved. Use of this web site signifies your agreement to the terms and conditions.
Request More Info
Fill out the form below and a member of our team will reach out right away!
" * " indicates required fields
Data Science
What Is Data Communication? Components & Benefits
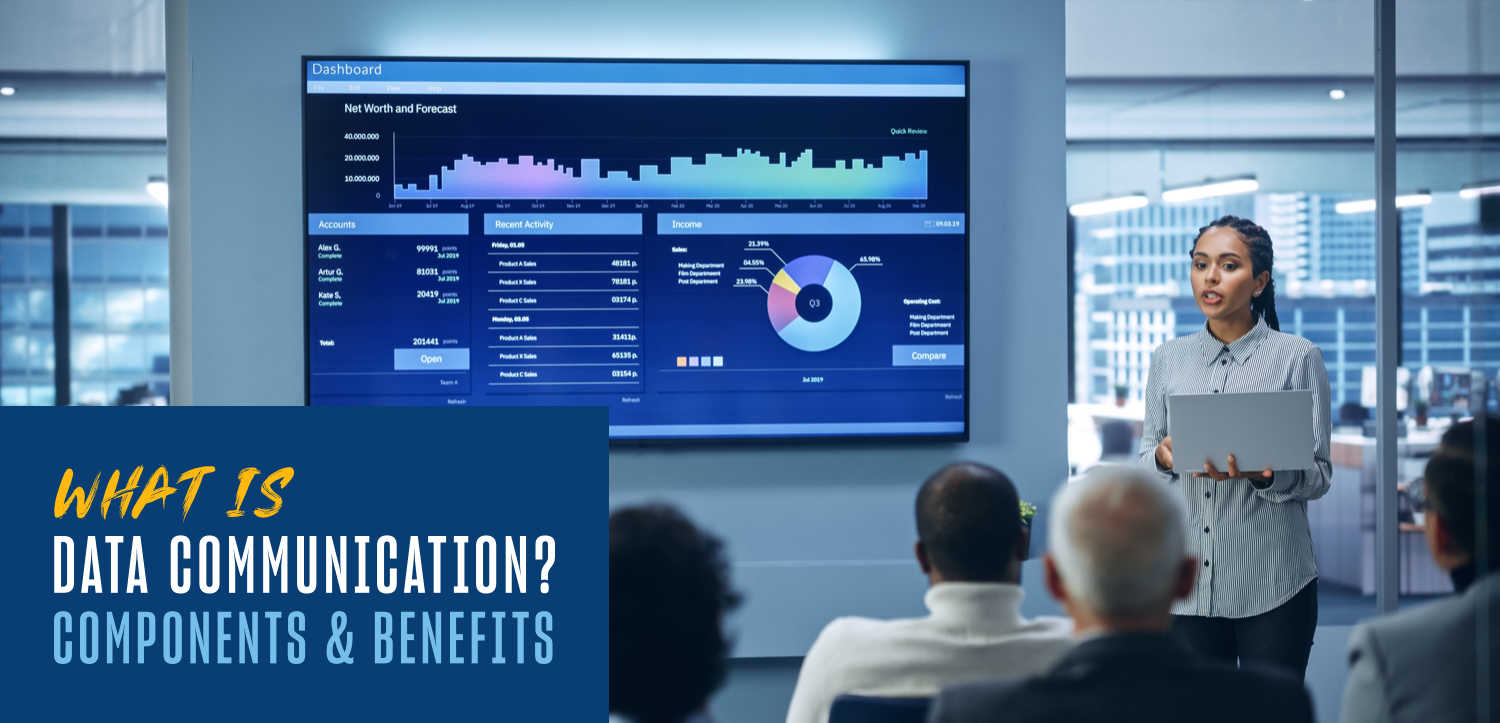
- Data Communication
- Storytelling
- Future Implications
Our modern, connected world hinges on data communication. It’s the fundamental basis that allows our devices to interact, providing us with instant and convenient access to information like never before.
However, the innovations we’ve seen in the last 50 years since the development of the first wireless networks and the creation of the internet are just the tip of the iceberg. The possible technologies we could witness within the next few years are fertile ground for those looking to create new business models, which is a major driving factor of why the University of San Diego developed the Master of Science in Applied Data Science program .
Before diving into detail about what may be on the horizon, it’s important to take a step back to understand the basic models of data communication and its general benefits. These insights will provide some helpful context on where we might be headed and might inspire you to develop the next great innovation in data communication.
What Is Data Communication?
Data communication is functionally similar to what we think of as “regular” communication, which is simply a sender transmitting a message to a destination. Data communication specifically refers to the process of using computing and communication technologies to transfer data (the message) from a sender to a receiver — or even back and forth between participating parties. The concept encompasses technologies like telecommunications, computer networking and radio/satellite communication.
Modern data networks all provide the same basic functions of transferring data from sender to receiver, but each network can use different network hardware and software to achieve these ends. Communication between devices adheres to industrial communications protocols, which is the set of rules that define how data is exchanged. Today’s data communications protocols are defined and are managed by interconnected bodies, including private businesses, standards-making organizations, regulatory agencies and common carriers.
4 Benefits of Data Communication
Before we can get into the benefits of data communication, it’s important to separate the concept of connectivity from communications. Connectivity is the capability of connecting one party to another. The benefits that arise from those connections depend on who’s connecting to whom — or to what.
We can think of it along three types of connections:
- Person to Person — such as when you call someone on a cell phone or have a chat session.
- Person to Machine — whenever you access information from a computer or automated system.
- Machine to Machine — when devices transfer information directly between each other.
Rather than being confined to these simple interactions, you can think of connectivity as a continuum. For example, when you text with someone else, the following steps occur:
- A person connects to a machine to send a message.
- The machine connects to another machine to deliver the message.
- The second machine translates and displays the message to another person.
- That person responds, and the process repeats, enabling a person-to-person connection.
The one thing that remains true in all of these connections is that some kind of information is transmitted, whether it’s retrieving a report from an archive, uploading data to a cloud server or holding a meeting in a Zoom call. Here’s a video that talks about the basics of connectivity in a little more detail.
Now, when we talk about data communications and networking, what we’re usually talking about are specific platforms. A data communications platform is essentially any technology that — whether it’s a cellphone, a laptop or the internet itself — enables connectivity. Today, data communication has become as ubiquitous as electricity itself, which has brought some incredible advances:
Instant communications. All of our modern digital communications, from email and instant messaging to video calls and TikTok, are all built on data communication networks. You can instantly connect with anyone in the world — or broadcast a message to thousands of people.
Greater business efficiency. Data communications has revolutionized how businesses interact with data. More effective ways of collecting and processing data leads to greater insights, which allow businesses to streamline productions, reduce expenses and improve operational efficiencies.
Innovations in automation. The Internet of Things (IoT) enables even more connection between different devices, allowing for new types of automation. For example, when we think of self-driving cars, providing them the ability to directly connect with other cars on the road over a 5G network makes the concept much more workable than trying to rely entirely on cameras and other sensors to determine positioning.
Smart monitoring systems. Sensors in wearable devices allow for advanced human health monitoring, which can transmit real-time data on someone’s condition or send alerts in an emergency. Wider applications include the development of smart cities that can offer improved traffic conditions, waste management, energy consumption and more.
Components of Data Communication
There are several components of data communication, but to keep things relatively brief, we should look at three of the most important elements: communication functionality, network models and standards of communication.
Communication Functionality
We’ve talked about the nature of communication being between a sender and a receiver. As data platforms have advanced, there has been increased functionality in how senders and receivers communicate:
- Simplex communications , which were the first and simplest means of communication where the transmission of data goes only in one direction. Simplex is still used in one-way data communication mediums such as radio stations and TV broadcasts.
- Half-duplex communication , where information can go both ways, but not at the same time. An example would be a CB radio, where a receiver has to wait for the system to be clear before responding.
- Full-duplex data communications models accommodate simultaneous two-way communication of data. The landline telephone is the most widely known means of full-duplex communication.
- Serial data communications is what we think of when we talk about networking. Data is packaged into units and then sent serially to the receiver by the sender. Once it is received, the units are reassembled to recreate the original data.
Network Models
Serial data communications rely upon networks to transmit data. The two most important network models are the Open Systems Interconnection Reference (OSI) model and the Internet model:
- The Open Systems Interconnection Reference model was developed by the Open System Interconnection Subcommittee in 1984. The OSI model consists of seven layers: the physical layer, data link layer, network layer, transport layer, session layer, presentation layer and application layer. Though it isn’t widely used today, it still has value as a foundational understanding of networking.
- The Internet model , though actually older than OSI, is the network model that has arisen to be the dominant model for all current hardware and software. Also referred to as the Transfer Control Protocol/Internet Protocol (TCP/IP) model , it combines the top three OSI layers into a single layer, making it a five-layer model consisting of a physical layer, data link layer, network layer, transport layer and application layer. The Internet model allows different independent networks to connect to one another and together, creating what we know as the global internet.
Standards of Communication
Standards define a set of rules known as protocols, which ensure that the software used in the different layers of the network models are compatible. Without standards, it would be virtually impossible for computers to communicate with each other. With standards, all hardware and software can communicate if they conform to the same specifications.
Previously, standards of communications were set by telecommunications standards bodies specific to different countries. Today, the Third Generation Partner Project (3GPP) initiative unites seven telecommunications standard development organizations — Japan’s Association of Radio Industries and Businesses (ARIB) and Telecommunication Technology Committee (TTC), Alliance for Telecommunications Industry Solutions (ATIS), China Communications Standards Association (CCSA), European Telecommunications Standards Institute (ETSI), Telecommunications Standards Development Society, India (TSDSI) and Korea’s Telecommunications Technology Association (TTA) — to establish a converging set of standards to maintain the global communications network.
All of these fundamentals essentially underpin what is covered in USD’s Master of Applied Data Science program’s foundational coursework , providing a comprehensive introduction to data science principles, including network models and communication standards critical to global data systems. The program covers predictive modeling, machine learning, data engineering and the use of cloud computing, equipping students with the necessary skills to develop advanced data-driven solutions.
Data Science Data Communication & Storytelling
While the term “data communication” often pertains to the transmission of data across technical networks utilizing various models and standards, within the field of data science it assumes a distinctly different role. Here, data communication — or more aptly, data science communication and storytelling — is central to the effectiveness and impact of data science.
Data Science Communication Defined
Data science communication involves the articulate and strategic relay of complex data insights to varied audiences, ensuring that these insights are both understood and actionable. This form of communication is crucial as it transcends the mere presentation of data, elevating it to strategic storytelling that engages stakeholders and drives business decisions.
Karen Church , Vice President of Research, Analytics and Data Science at Intercom, underscores the importance of this skill set : “I believe that communication is one of the most critical skills in data science…the ability to communicate effectively is just as important, if not more so, to driving real impact in data science.” Through effective data science communication, methodologies are impactfully applied across different platforms and environments.
Key Aspects of Effective Data Science Communication
Understanding and translating business requirements.
Data scientists must adeptly convert high-level business objectives into specific, data-driven tasks. Church notes, “Communication helps you understand and translate business requirements into specific data problems and research questions.” This alignment is vital for ensuring that data science projects fulfill organizational goals.
Framing or Reframing Problems
Accurately defining the data science problem is crucial as it ensures that the analysis addresses the right questions. Church explains the importance of problem framing: “Framing a problem in a way that a whole group or team can get a shared understanding of it, rally around it and take action on it [is essential].” Effective communication aids in clarifying the scope and nature of problems, thereby optimizing the focus and resources of data science initiatives.
Collaborating and Influencing
Data science frequently necessitates collaboration across various technical and non-technical stakeholders. Church asserts, “Communication plays a vital role in facilitating effective collaboration and helps influence and persuade others to take action or drive to a decision.” This collaborative process is imperative for seamlessly integrating data science into business strategies.

Presenting Results and Insights Effectively
The ability to communicate findings clearly and compellingly is critical. Data storytelling, which integrates data, visuals and narratives, is a pivotal technique highlighted by Church for making data insights accessible and impactful to stakeholders.
By distinguishing data science communication from traditional data communication, professionals are equipped to analyze, interpret and integrate these insights into the operational fabric of organizations. This specialized form of communication is integral to the creation of robust, data-driven solutions that significantly influence business outcomes and strategic decisions.
Future of Data Communication
Today’s revolutionary data communication capabilities are why the modern era is defined as “the information age.” While recent advances make it challenging to predict the exact trajectory of data communications and networking, here are some trends and developments that are likely to shape the near future:
New Applications of Data Communication Networks
The improving capabilities of wired and wireless communication networks, including 5G and IoT ( Internet of Things ), are enabling new applications such as self-driving vehicles, mixed and augmented reality and remote communication advancements that facilitate complex tasks like remote surgery. Beyond these high-end applications, the acceleration of network speeds continues to solidify cloud computing as a baseline technology for corporate computing networks. Widespread cloud adoption is expected to enhance computational efficiency across all industries.
Investment in Green Technologies
Digital platforms enable greater operational efficiency and reduced reliance on physical resources, but they must be developed sustainably. For instance, smart electricity grids are essential for the electrification of transportation, including the next generation of electric vehicles. This push towards greener and more efficient computing power will likely necessitate the creation of adaptive logical private networks, enhanced two-way data communication networks and the use of AI to optimize resource distribution.
Geopolitical Dynamics and Technological Restrictions
Although the last decade has seen enhanced international cooperation and the establishment of global standards, geopolitical tensions could lead to new restrictions or bans on certain technologies. Examples include the US and EU’s prohibitions on Huawei’s network equipment and discussions around a potential TikTok ban in the US.
Integration of Communication Networks with Cloud Computing
For businesses pursuing digital transformation, merging telecommunication networks with cloud services represents a leap forward. Software will automatically configure these combined networks to tackle business challenges with remarkable precision. Future advancements could feature ultra-wideband, low-latency networks for real-time uses like controlling drone fleets, powering augmented reality displays and enabling interactions within the metaverse. Additionally, we may see a significant expansion in Internet addresses to enhance IoT connectivity in remote areas and the creation of nanonetworks for detailed, local monitoring and data collection.
The true transformative impacts of technologies such as 5G are more substantial than just faster cell phones and enhanced service, which telecom companies roll out swiftly for quick profits. For instance, a merger between telecommunications and data networks, which would make telecom networks programmable, could enable mobile phones to access wireless networks anywhere there is a phone signal. With the full deployment of 5G — and looking toward 6G — we can expect an integration of wired, wireless and satellite communications into a comprehensive network that facilitates entirely new applications.
As we keep pushing the limits of data communication, we must understand what drives these advancements. If you are interested in advancing your career in the world of data, consider how USD’s 100% online MS in Applied Data Science program can help prepare you for success.
Choosing the right educational path is essential to succeed in data science and prepare for future challenges. Download our eBook, 7 Questions to Ask Before Selecting an Applied Data Science Master’s Degree Program , to learn how to select an option that will keep you at the forefront of data science.
Be Sure To Share This Article
- Share on Twitter
- Share on Facebook
- Share on LinkedIn
Considering Earning Your Master’s in Data Science?
Free checklist helps you compare programs, select one that’s ideal for you.
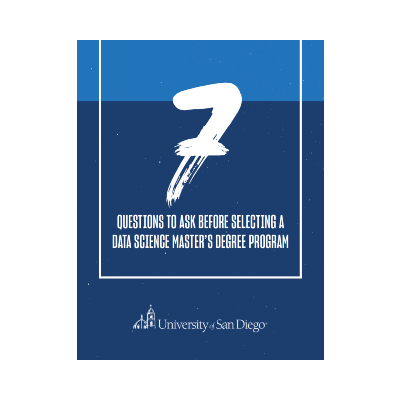
- Master of Science in Applied Data Science
Related Posts
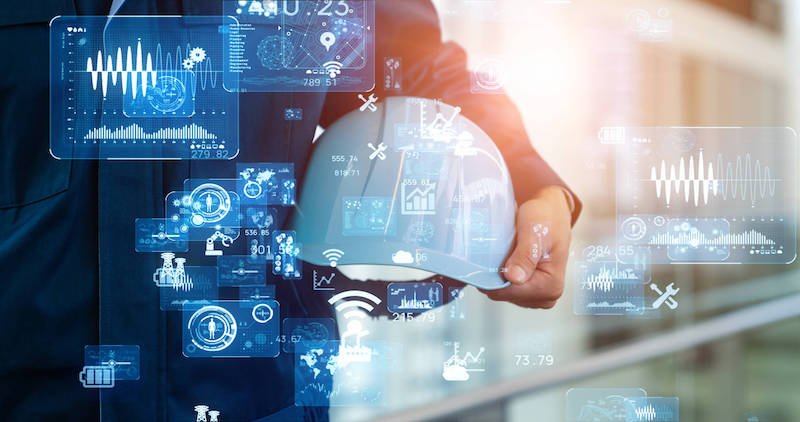
Artificial Intelligence
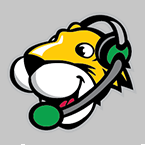
Ask a Librarian
How can I help you today?
A live human is ready to help.
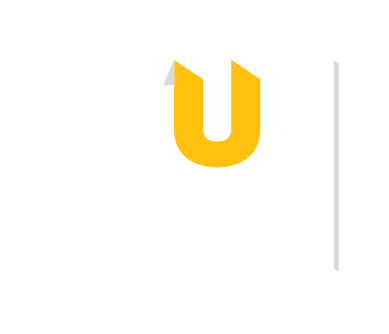
Find & Cite | Research Help | Collections | Services | About
- Cook Library
- Research Guides
Communication Studies
- Research Methods & Data Collection
- Getting Started
- Background Research
- How to Search a Database
- How to Find a Book
- Equity, Diversity & Inclusion Resources
- Associations

These journals directly relate to qualitative & quantitative research methods used in the social sciences. Cook Library has access to them through the databases linked on the "Finding Communication Studies Research" page on this guide, but you are able to search specific journals using the links below.
- Social Science Research Social Science Research publishes papers devoted to quantitative social science research and methodology. The journal features articles that illustrate the use of quantitative methods to empirically test social science theory.
- Journal of Ethnographic & Qualitative Research Journal of Ethnographic & Qualitative Research (JEQR) is a quarterly, peer-reviewed periodical, publishing scholarly articles that address topics relating directly to empirical qualitative research and conceptual articles addressing topics related to qualitative.
- International Journal of Qualitative Methods The International Journal of Qualitative Methods is the peer-reviewed interdisciplinary open access journal of the International Institute for Qualitative Methodology (IIQM) at the University of Alberta, Canada. The journal, established in 2002, is an eclectic international forum for insights, innovations and advances in methods and study designs using qualitative or mixed methods research.
- Communication Methods & Measures The aims of Communication Methods and Measures are to bring developments in methodology, both qualitative and quantitative, to the attention of communication scholars, to provide an outlet for discussion and dissemination of methodological tools and approaches to researchers across the field, to comment on practices with suggestions for improvement in both research design and analysis, and to introduce new methods of measurement useful to communication scientists or improvements on existing methods.
Quick Reference
- Sage Encyclopedia of Communication Research Methods This web-based encyclopedia is searchable by keyword or subject.
- How to...Use ethnographical methods & participant observation This guide from Emerald Publishing describes types of ethnographic research methods and how to apply them.
Measures & Instruments
- << Previous: Finding Communication Studies Research
- Next: How to Search a Database >>
- Last Updated: May 22, 2024 3:44 PM
- URL: https://towson.libguides.com/commstudies
Information and communication technologies for global research
Service areas.
- Cyberinfrastructure
- Identity and Risk Management
- Clinical Research Technology
RDCT provides data management and network services for customers with remote operations. We specialize in support for accurate data collection, management and validation. And our extensive experience in clinical trials and biomedical research give us a unique skill set for corporate communications and data integrity.
Search Icon
Events See all →
Driskell and friends.

The Arthur Ross Gallery presents the work of artist, scholar, and curator David Driskell and explores his relationships with other artists. Friends include: Romare Bearden, Elizabeth Catlett, Jacob Lawrence, Keith Morrison, James Porter, and Hale Woodruff.
6:00 a.m. - 1:00 p.m.
Arthur Ross Gallery, 220 S. 34th St.
Garden Jams

5:00 p.m. - 8:00 p.m.
Penn Museum, 3260 South St.
July 2024 Wellness Walk

8:00 a.m. - 9:00 a.m.
Benjamin Franklin Statue by College Hall
ICA Summer 2024 Opening Celebration
7:00 p.m. - 10:00 p.m.
Institute of Contemporary Art, 118 S. 36th St.
Education, Business, & Law
New books from Wharton faculty
The latest installments of the wharton school’s faculty research podcast, ‘ripple effect,’ showcases recent books on leadership, customer service, immigration, and the power of data..
Wharton’s faculty podcast, “ Ripple Effect ,” introduces its “Meet the Author” series, highlighting recent books published by experts at the Wharton School . The June series examines leadership, customer service, immigration, and the power of data.
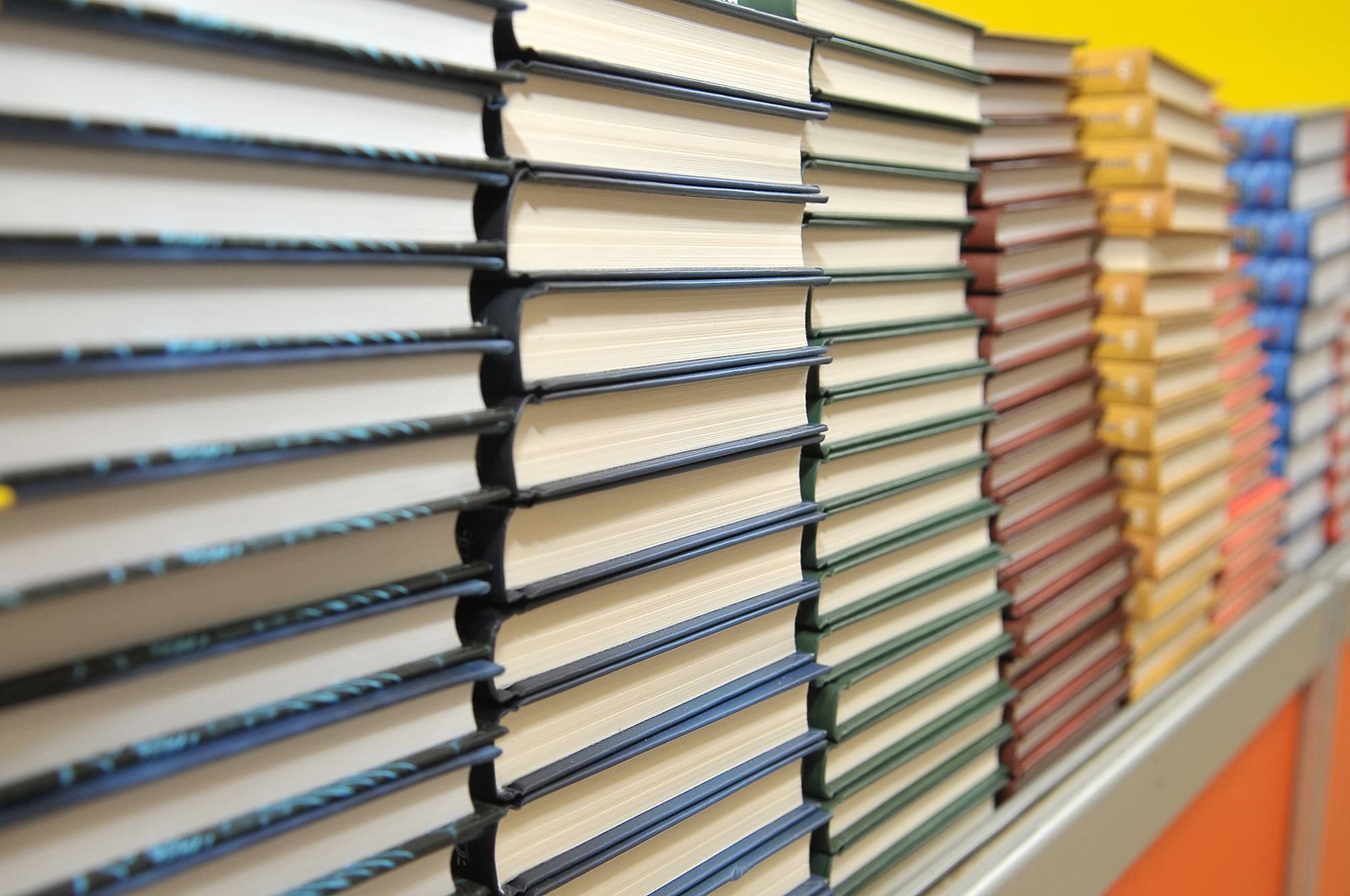
Alberto I. Duran President’s Distinguished Professor Cait Lamberton talks about her new book, “Marketplace Dignity,” which explains why customers want firms to treat them with respect and dignity above anything else.
“We asked consumers to rank the importance of different things that they can experience in their interactions with a firm. Dignity always comes in second. It comes in second to the objective value that you get from your interaction with a firm,” says Lamberton. “But what falls way down the list are the things that we talk about all the time, like whether the firm is sustainable, whether the firm aligns with my political values. What’s second is whether I’m treated with respect. That comes up in the data over and over and over again.”
With political instability rising around the world, now is the time for business leaders to develop a comprehensive strategy to mitigate risk. Vice Dean and Faculty Director of the Environmental, Social and Governance Initiative Witold Henisz explains how in his new book, “Geostrategy by Design.”
“There’s a risk of a greater resurgence of something like the Cold War, where we can’t be sourcing certain products. We’re concerned about the ownership of who owns what company, whether it be TikTok or whether it be semiconductors,” says Henisz. “National identity really matters, and that’s got to be part of the equation. It’s not a new system, but it’s a new set of questions that are going to affect the system in every aspect of global companies’ operations.”
Immigrants have long been cast as outsiders who take jobs and damage the economy. Max and Bernice Garchik Family Presidential Associate Professor Zeke Hernandez dispels these stereotypes and others in his new book, “The Truth About Immigration: Why Successful Societies Welcome Newcomers.”
“We have a lot of research showing that where immigrants go, firms make investment. And these effects last for generations. Where there’s a lot of, say, Germans, historically, a lot of German investment will follow. It’s true for Mexican firms. It’s true for Korean firms. It’s true for every nationality,” says Hernandez. “This is what I call the investment-immigration-jobs triangle. Immigrants arrive, they foster investment from their home countries, those investments create jobs.”
Rather than mining data for insights, companies need to think more strategically about the decisions they need to make, and use data to help them get there. Co-director of AI at Wharton and the Sebastian S. Kresge Professor of Marketing Stefano Puntoni says that’s the focus of “Decision-driven Analytics,” his new book co-authored with Bart De Langhe.
“Nobody argues that using data to make decisions is a bad idea. We need data to make good decisions. What we argue in the book is that the emphasis is wrong,” says Puntoni. “And that the complexity and investments required to get the data systems in place, and all that complex, technical work that has to be done has attracted a lot of attention. At some point, people end up in a situation where we are looking at data and trying to find a purpose for it, rather than looking at the questions that they need to answer and find data for that purpose.”
For a full list of podcast episodes, visit the “ Ripple Effect ” website.
To Penn’s Class of 2024: ‘The world needs you’
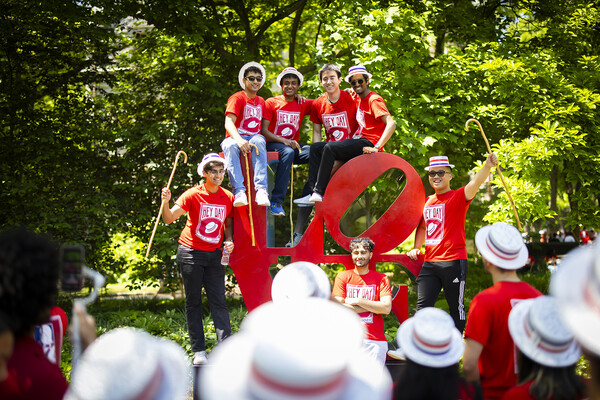
Campus & Community
Class of 2025 relishes time together at Hey Day
An iconic tradition at Penn, third-year students were promoted to senior status.
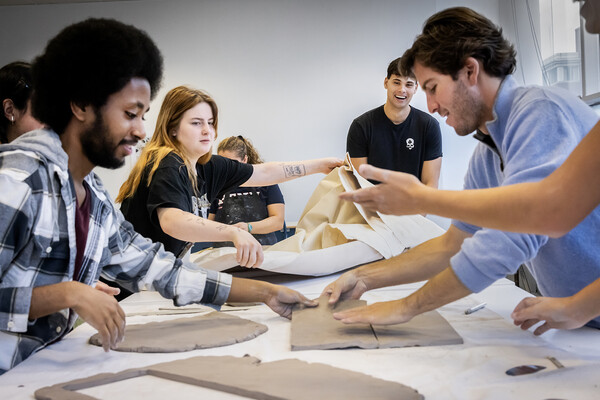
Arts, Humanities, & Social Sciences
Picturing artistic pursuits
Hundreds of undergraduates take classes in the fine arts each semester, among them painting and drawing, ceramics and sculpture, printmaking and animation, photography and videography. The courses, through the School of Arts & Sciences and the Stuart Weitzman School of Design, give students the opportunity to immerse themselves in an art form in a collaborative way.
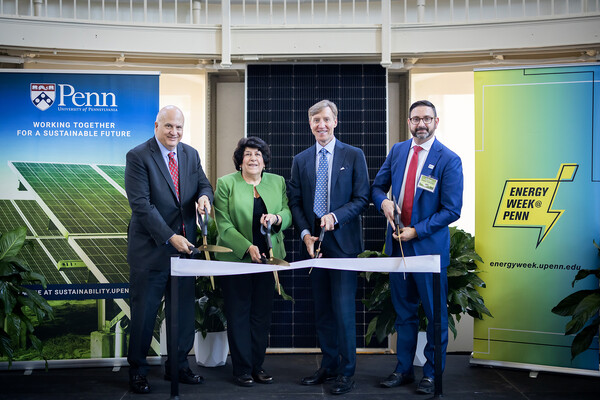
Penn celebrates operation and benefits of largest solar power project in Pennsylvania
Solar production has begun at the Great Cove I and II facilities in central Pennsylvania, the equivalent of powering 70% of the electricity demand from Penn’s academic campus and health system in the Philadelphia area.
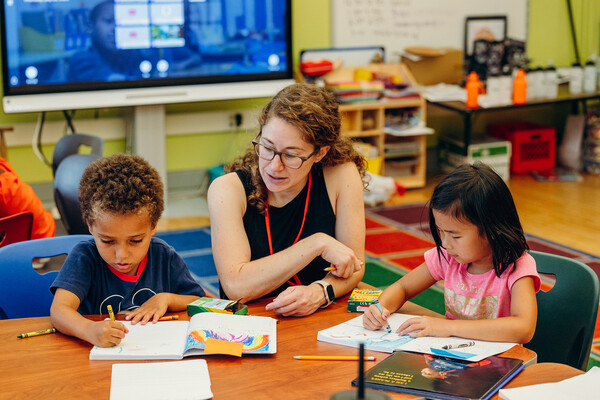
Investing in future teachers and educational leaders
The Empowerment Through Education Scholarship Program at Penn’s Graduate School of Education is helping to prepare and retain teachers and educational leaders.
Thank you for visiting nature.com. You are using a browser version with limited support for CSS. To obtain the best experience, we recommend you use a more up to date browser (or turn off compatibility mode in Internet Explorer). In the meantime, to ensure continued support, we are displaying the site without styles and JavaScript.
- View all journals
- Explore content
- About the journal
- Publish with us
- Sign up for alerts
- Open access
- Published: 10 July 2024
Multi-scale lidar measurements suggest miombo woodlands contain substantially more carbon than thought
- Miro Demol ORCID: orcid.org/0000-0002-5492-2874 1 ,
- Naikoa Aguilar-Amuchastegui ORCID: orcid.org/0000-0002-5072-0079 2 ,
- Gabija Bernotaite ORCID: orcid.org/0009-0004-5550-1109 1 ,
- Mathias Disney ORCID: orcid.org/0000-0002-2407-4026 3 , 4 ,
- Laura Duncanson ORCID: orcid.org/0000-0003-4031-3493 5 ,
- Elise Elmendorp ORCID: orcid.org/0009-0005-0088-6803 1 ,
- Andres Espejo ORCID: orcid.org/0009-0009-4021-1666 2 ,
- Allister Furey 1 ,
- Steven Hancock ORCID: orcid.org/0000-0001-5659-6964 6 ,
- Johannes Hansen ORCID: orcid.org/0000-0003-0743-1332 1 ,
- Harold Horsley ORCID: orcid.org/0009-0005-0361-7609 1 ,
- Sara Langa 7 ,
- Mengyu Liang ORCID: orcid.org/0000-0003-3877-8056 5 ,
- Annabel Locke ORCID: orcid.org/0009-0007-9885-191X 1 ,
- Virgílio Manjate 8 ,
- Francisco Mapanga 9 ,
- Hamidreza Omidvar ORCID: orcid.org/0000-0001-8124-7264 1 ,
- Ashleigh Parsons 1 ,
- Elitsa Peneva-Reed ORCID: orcid.org/0000-0002-4570-4701 2 ,
- Thomas Perry ORCID: orcid.org/0009-0003-9506-4225 1 ,
- Beisit L. Puma Vilca ORCID: orcid.org/0000-0003-2169-9108 1 ,
- Pedro Rodríguez-Veiga ORCID: orcid.org/0000-0003-4845-4215 1 , 10 ,
- Chloe Sutcliffe 1 ,
- Robin Upham ORCID: orcid.org/0000-0002-4172-3358 1 ,
- Benoît de Walque ORCID: orcid.org/0009-0000-2738-556X 1 &
- Andrew Burt ORCID: orcid.org/0000-0002-4209-8101 1
Communications Earth & Environment volume 5 , Article number: 366 ( 2024 ) Cite this article
545 Accesses
33 Altmetric
Metrics details
- Climate-change ecology
- Climate sciences
- Forest ecology
Miombo woodlands are integral to livelihoods across southern Africa, biodiversity in the region, and the global carbon cycle, making accurate and precise monitoring of their state and change essential. Here, we assembled a terrestrial and airborne lidar dataset covering 50 kha of intact and degraded miombo woodlands, and generated aboveground biomass estimates with low uncertainty via direct 3D measurements of forest structure. We found 1.71 ± 0.09 TgC was stored in aboveground biomass across this landscape, between 1.5 and 2.2 times more than the 0.79–1.14 TgC estimated by conventional methods. This difference is in part owing to the systematic underestimation of large trees by allometry. If these results were extrapolated across Africa’s miombo woodlands, their carbon stock would potentially require an upward revision of approximately 3.7 PgC, implying we currently underestimate their carbon sequestration and emissions potential, and disincentivise their protection and restoration.
Similar content being viewed by others
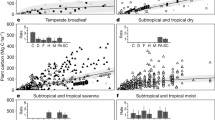
Mapping carbon accumulation potential from global natural forest regrowth
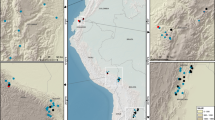
Mature Andean forests as globally important carbon sinks and future carbon refuges
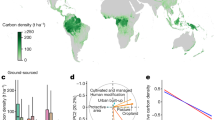
Integrated global assessment of the natural forest carbon potential
Introduction.
Miombo woodlands, the dry tropical forests spanning large areas of southern Africa, directly support many millions of livelihoods in various ways including supply of plant-based materials, fertile soils for agriculture, and grazing lands 1 . These ecosystems also hold cultural and spiritual significance, provide habitat for substantial plant and animal biodiversity, and regulate both the climate and water resources 2 . These landscapes, however, are changing because of human activities, with cover reducing from approximately 2.7 to 1.9 million km 2 between 1980 and 2020 3 . Owing to both their importance and dynamic nature, it is therefore crucial to monitor how the world’s miombo woodlands are changing.
One essential climate variable that requires accurate and precise monitoring is the aboveground biomass (AGB) and carbon stored in these woodlands 4 . Any uncertainty that exists in the quantification of these stocks has consequences, particularly regarding misinformed policy and decision making towards them, as well as the misallocation of funding and resources 5 , 6 . Carbon markets for example, through programmes such as Reducing Emissions from Deforestation and Degradation (REDD+) 7 , require low uncertainty in estimates of carbon stocks if they are to properly incentivise direct climate benefits, and co-benefits including biodiversity and ecosystem services, by safeguarding these woodlands. Further, intended outcomes from international climate agreements towards greenhouse gas emissions reductions, such as the Paris Agreement including individual countries’ Nationally Determined Contributions, are premised on forest carbon accounting with low uncertainty 8 . That is, both high accuracy and precision, quantitatively expressed as a bias and variance, respectively, are usually important for any estimate of forest AGB stocks in these contexts. Whilst accuracy is the principal concern in accounting (systematic over- or under-estimation commensurately misleads understanding of forest carbon sequestration and emissions potential) 9 , precise estimates are also important, including from the requirement to detect change over time (it can be problematic to interpret differences between observations with low precision) 10 . This is particularly the case for miombo woodlands given the aforementioned pace of their anthropogenic change.
The conventional approach to quantifying region-scale forest AGB stocks across miombo woodlands, and forests generally, within the context of UNFCCC- and IPCC-compliant greenhouse gas inventories, sees the combination of activity data and emissions factors (EF): remotely sensed estimates of forest area are multiplied by values of expected AGB per unit area of forest 11 . These expected values, based on in-situ measurements, might be generated from National Forest Inventories (NFI), or alternatively, where such data are unavailable, taken from the literature, such as IPCC defaults 12 . While this overall approach can be readily implemented it does have limitations, including: (i) restricted ability to describe AGB variations within forest types; (ii) EFs not being representative of the forest in question; and (iii) failing to detect change beyond binary transition between forest and non-forest (e.g. degradation).
For example, when focusing solely on the EF, and ignoring immediate questions surrounding the representativeness of applying a single value to any particular region of miombo woodland, uncertainties arise from the methods used to gather the in-situ data from the forest plots underlying the EFs themselves 13 . A ubiquitous feature of such measurements is the application of allometric models to estimate individual tree AGB. These models characterise the correlations that exist between tree shape and mass, enabling AGB estimation from more readily-measurable predictor variables such as stem diameter and tree height 14 . Such allometrics are themselves calibrated using hard-won destructive weighing measurements collected from a limited number of harvested trees that then must represent the entire variability of the specific taxa or region where that model is subsequently applied.
Uncertainties in allometric-derived AGB predictions therefore arise from the selection, measurement and modelling of these calibration trees, and the measurement of the predictor variables of any out-of-sample tree 15 . Several studies have explored the precision of allometric predictions of tropical and subtropical forests, where the expectation is that uncertainties range from 10 to 40% of the estimate itself at the hectare-scale 16 , 17 . Research has also explored their accuracy, with a particular focus on the selection and modelling of allometric calibration data 18 , which are routinely heavily skewed towards small trees owing to their relative ease of harvesting. It has been hypothesised that this, combined with inadequate statistical methods, might cause biased AGB predictions for underrepresented larger trees 19 . Concurrently, independent lidar-based methods for AGB estimation have shown large differences versus allometry, estimating up to 1.77 times greater stocks at the plot-scale 20 . These potential uncertainties in allometric predictions are problematic as they would propagate directly into derived EFs and their aforementioned applications.
Here, we present the first (to our knowledge) mapping of region-scale AGB stocks generated entirely independent of the above conventional methods, including activity data, EFs and allometrics, using 3D multi-scale lidar (MSL) data acquired across 50 kha of forests in and around Gilé National Park, Mozambique (Fig. 1 ). The continuous region of interest (ROI) where these data were collected was selected such that it ranged from intact forests, secondary forests in various states of degradation, through to clearland, resulting in data that could reasonably be considered representative of miombo woodland landscapes more widely. Across the ROI, the MSL dataset (approximately 450 billion measurements) comprised helicopter-based airborne laser scanning (ALS) across its entirety, unoccupied aerial vehicle laser scanning (UAV-LS) from six 300 ha sections, and terrestrial laser scanning (TLS) and conventional forest inventory measurements from six coincident 1 ha plots.
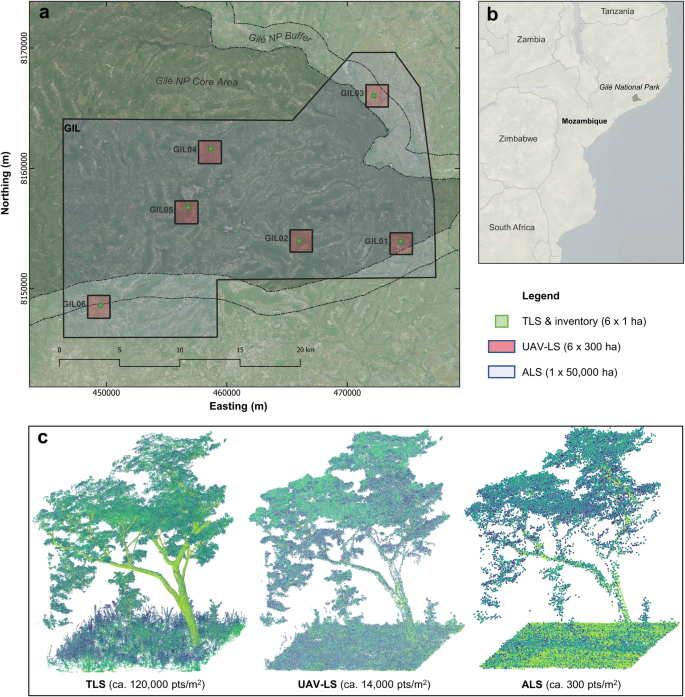
a Approximately 450 billion laser scanning measurements were acquired in a 50 kha region of interest (ROI) located across the southeast corner of the park, capturing core area, buffer zone and beyond, such that the ROI encompassed intact miombo woodlands through to clearland (CRS is EPSG:32737). Helicopter-based airborne laser scanning (ALS) data were collected across its entirety, whilst slow-flying unoccupied aerial vehicle laser scanning (UAV-LS) data were acquired across six 300 ha sections. Terrestrial laser scanning (TLS) and conventional inventory data were collected in six 1 ha plots coinciding with these sections. b Location of Gilé National Park in wider Mozambique. c Example of coincident TLS, UAV-LS and ALS point clouds from a 10 m 2 section of forest (coloured by reflectance). Map data © 2024 Microsoft.
An inverted pyramid approach was used to estimate AGB stocks and uncertainties across the ROI from these MSL data (Fig. 2 ), whereby each layer calibrated the next, commencing with the TLS point clouds that estimated individual tree AGB via both quantitative structural models (QSMs) explicitly reconstructing whole-tree woody architecture and volume 21 (Fig. 3 ), and estimates of basic woody tissue density 22 . These estimates were themselves calibrated and validated using a literature sample of destructive measurements 23 . TLS-derived AGB was gridded and used to train extreme gradient boosting machine learning models 24 using predictor variables retrieved from the UAV-LS point clouds that describe forest structure 25 , 26 (e.g., canopy height, tree fractional cover, and voxel occupancy rates describing the 3D distribution of woody plant material), with the step repeated to upscale to the ALS. The optimisation and performance of these models was evaluated using spatial cross-validation methods 27 , with meaningful confidence intervals capturing uncertainties arising from both the QSM-derived training data and the upscaling itself, providing a robust understanding of the uncertainty in AGB predictions.
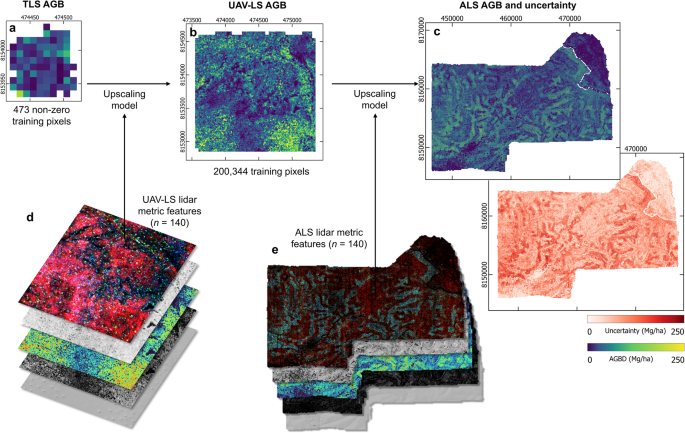
a TLS-derived estimates of gridded AGB (10 m resolution) were generated for the six 1 ha plots (Fig. 1a ) via quantitative structural models describing the woody architecture and volume of individual trees (Fig. 3a ). b Upscaled AGB across the six 300 ha sections was estimated through gradient boosting machine learning, using TLS estimates as training data, and metrics of forest structure retrieved from the UAV-LS data as predictor variables. c This upscaling step was repeated to produce AGB estimates across the ROI, and also shown here are the uncertainties associated with each pixel prediction. Models were evaluated using spatial cross-validation methods, and uncertainty quantification captured components arising from both the upscaling and the underlying TLS training data. d , e Examples of the predictor variables generated from the UAV-LS and ALS point clouds including but not limited to canopy height, tree fractional cover and voxel occupancy rates (a proxy for the 3D distribution of woody volume).
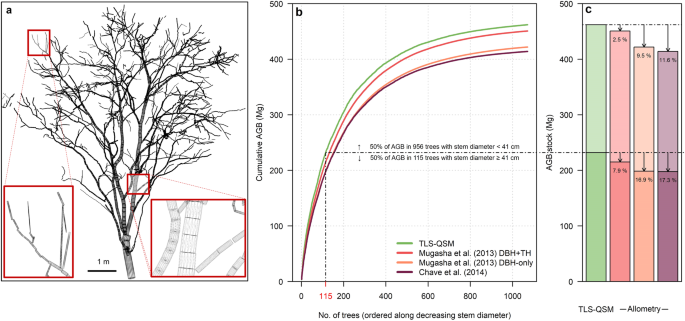
a Illustration of one quantitative structural model derived from the TLS point clouds (here of a Pterocarpus angolensis ) that, coupled with species-specific basic woody tissue density, enables estimation of tree-scale AGB. b The cumulative distribution of TLS- and allometric-derived AGB across the 1,071 trees matched in both the TLS and inventory data across the six 1 ha plots (Fig. 1 ), ordered by decreasing stem diameter. Allometric estimates were generated from three appropriate models: two miombo woodland specific models 29 (predictor variables: stem diameter only [red], and stem diameter and tree height [orange]) and a pan-tropical allometry 30 (predictor variables: stem diameter, tree height and basic woody tissue density [purple]). c Summed AGB estimates across these trees, whereby the percentage decrease between TLS- and allometric estimates is shown. The dotted lines show the contribution to AGB from the 115 largest trees (by stem diameter), where it can be seen that: (i) these ~11% of trees contributed ~50% of summed AGB, and (ii) allometric estimates were systematically smaller than TLS counterparts.
These new MSL-derived AGB estimates provide insights into the accuracy and precision of current best practices, and show that between 51 and 118% more AGB is stored across these miombo woodlands than conventional methods suggest. This is first demonstrated at the tree-scale by directly comparing TLS and allometric estimates for 1000+ trees (Fig. 3 ). We then show how these tree-level discrepancies, in part, translate into large differences at the region-scale, by comparing MSL-derived AGB stocks across the 50 kha ROI with counterparts estimated from both activity data and EFs (Fig. 4 ), and more direct mapping methods, using AGB products from the NASA GEDI spaceborne lidar mission 28 (Fig. 5 ). In the discussion, we explore the likely drivers of these differences, and examine these results in the context of miombo woodlands and global change more widely, particularly the consequences for their protection and restoration.
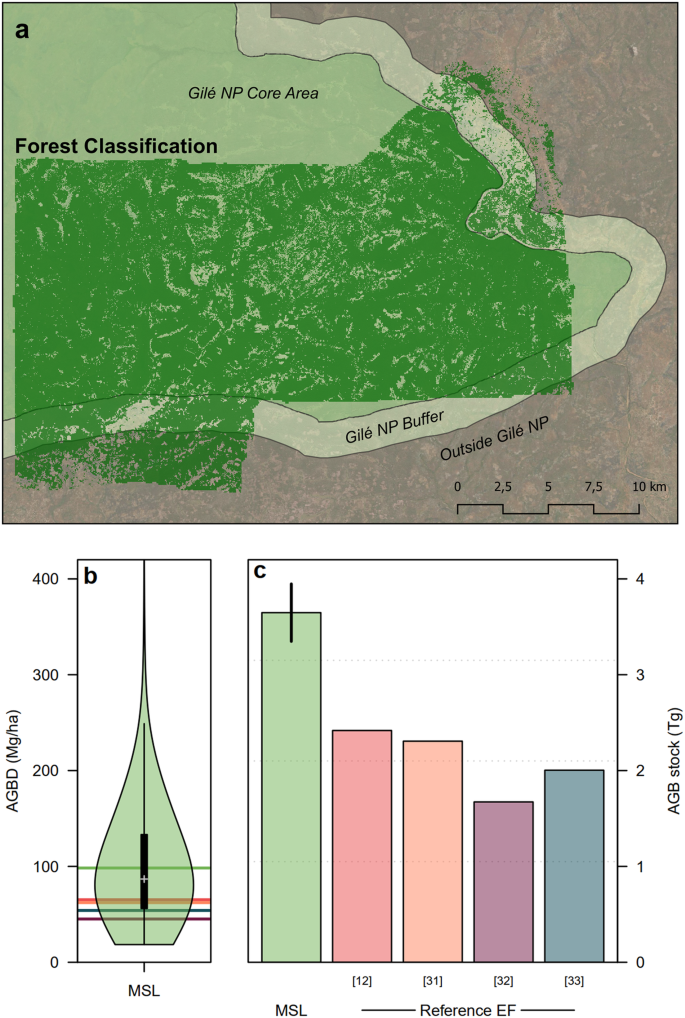
a MSL-derived forest/non-forest map across the 50 kha region of interest, overlaid on the boundaries of Gilé National Park, generated by thresholding tree fractional cover greater than or equal to 30% using a canopy threshold of 5 m at 10 m resolution. b Distribution and mean (green) of MSL-derived aboveground biomass density predictions (Fig. 2 ) for pixels considered forested, versus a representative selection of four EFs (red, orange, purple and blue) taken from IPCC defaults, Mozambique’s Forest Reference Emission Levels, and literature on miombo woodlands 12 , 31 , 32 , 33 . c Summed AGB stocks across the ROI (including uncertainty in the MSL-derived estimate), whereby these EFs were combined with the FNF map. It is observed that the MSL approach estimated AGB stocks between 51 and 118% larger than these conventional methods, with a mean increase of 74%. Map data © 2024 Microsoft.
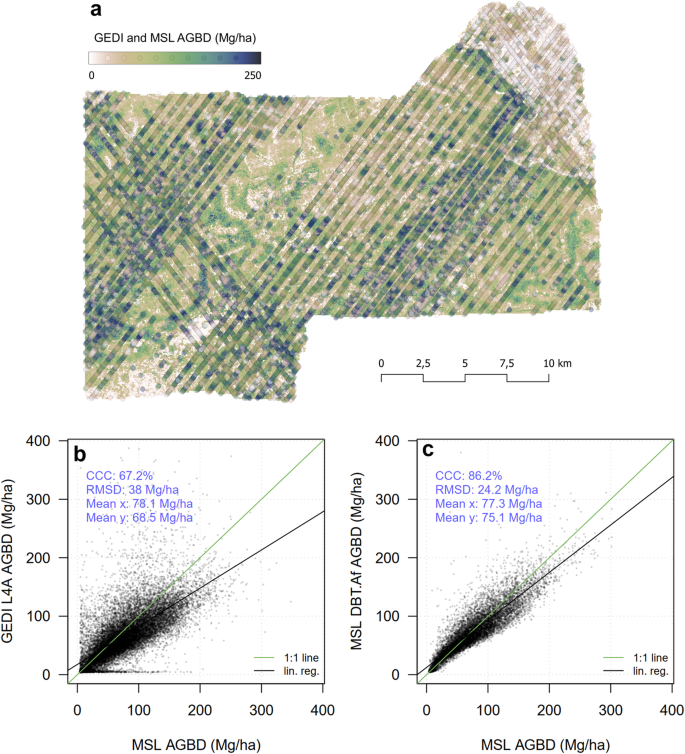
a Illustration of the 18,611 GEDI footprints available across the ROI overlaid on the MSL-derived predictions (Fig. 2 ). b Comparison between AGB density estimated via MSL and GEDI methods for these footprints. GEDI estimates are derived from a model for deciduous broadleaved trees in Africa (DBT.Af) using aboveground relative heights as predictor variables 34 . It is observed that MSL predictions are generally larger for densities greater than 50 Mg/ha. c This difference was examined by simulating GEDI waveforms and retrieving GEDI-perceived relative height metrics from the ALS data 35 , that were subsequently used to generate estimates of AGB density from the DBT.Af model. This subplot compares these estimates with MSL-derived AGB, whereby they are typically larger for lower densities and smaller for higher densities. That is, the agreement between MSL- and GEDI-derived AGB is part owing to these differences offsetting one another. Both scatter plots comprise an identity line (green), linear regression with free intercept (black) and statistics including concordance correlation coefficient (CCC), and root mean square difference (RMSD).
Divergence between small and large trees
A total of 1071 individual trees were explicitly matched in both the TLS and inventory data, with their TLS-derived AGB summing to 462.0 Mg, compared with 450.8, 421.9, and 414.0 Mg (2.5%, 9.5%, and 11.6% smaller, respectively) predicted from two miombo woodland-specific and one widely-used pan-tropical allometric models, respectively 29 , 30 (Fig. 3b, c ). Approximately 50% of AGB was stored in the largest 115 trees by stem diameter (i.e., 11% of trees). Here, the differences in AGB predictions between both methods were more marked, summing to 232.0 Mg vs. 215.0, 198.6 Mg, and 197.8 Mg (7.9%, 16.9% and 17.3% smaller, respectively). That is, a systematic trend was observed whereby allometric predictions produced similar estimates for small trees, but smaller estimates for large trees.
Region-scale differences
MSL-derived AGB (Fig. 2c ) across the ROI summed to 3.85 Tg ± 11.0% (uncertainty expressed as 90% confidence intervals), with uncertainty at individual pixel-level (10 m resolution) averaging 60.4%. This AGB estimate reduced to 3.65 Tg, with average AGB density of 98.4 Mg/ha when considering forested area only (37 kha, derived via a MSL-based forest/non-forest mask defined as tree fractional cover greater than or equal to 30% using a canopy threshold of 5 m at 10 m resolution 31 ). Estimated AGB densities were 99.2, 100.3, and 86.5 Mg/ha for core park, buffer zone and beyond, respectively. Conventional AGB estimates via activity data and EFs ranged from 1.67 to 2.42 Tg (mean: 2.10 Tg) across the ROI (Fig. 4c ), generated using the same mask, and four representative EF values of 65.2, 62.2, 45.1, and 54.0 Mg/ha (IPCC default for African subtropical dry forests, Mozambique’s Forest Reference Emission Levels, and literature on miombo woodlands, respectively 12 , 31 , 32 , 33 ).
Comparison with GEDI
Overall, there was some agreement between MSL- and GEDI-derived AGB, with mean densities of 78.1 and 68.5 Mg/ha, respectively, for the 18,611 GEDI footprints available across the ROI, although it was observed that MSL estimates were generally larger for densities greater than 50 Mg/ha (Fig. 5b ). These differences were explored by considering the African deciduous broadleaf forests model underlying GEDI-derived AGB (predictor variables: aboveground relative heights) 34 , and simulating GEDI-perceived waveforms and metrics from the ALS data 35 . This provided insight into the performance of this model, whose predictions were typically larger for lower densities ( < 50 Mg/ha) and vice versa for higher densities (Fig. 5c ) compared to MSL counterparts. That is, the overall agreement was in part owing to differences at high and low densities offsetting one another.
Here, we presented the first region-scale mapping of forest AGB stocks driven by direct 3D measurements of forest structure, independent of conventional methods, including allometrics. Importantly, these estimates have a credible estimate of uncertainty being 11.0% of the region-scale AGB estimate itself. We note that even with these first-of-their-kind MSL measurements capturing samples of the structure of each individual tree across the 50 kha region, pixel-level (10 m resolution) uncertainty frequently exceeded 60% (averaging out to approximately 36% and 27% when aggregating to 30 and 100 m resolution, respectively). That is, these miombo woodlands exhibit pronounced structural and woody tissue density variation, part of which remained uncaptured by either the MSL data themselves, or more likely, the developed processing methods. These maps then, are likely inappropriate for small-scale applications such as individual tree AGB estimation 36 , but suitable for enabling accurate local and regional carbon accounting through calibration and validation of Earth observation instrumentation such as GEDI, and the upcoming ESA BIOMASS mission 37 .
The principal insight from these MSL methods is that 51–118% more AGB is stored across the ROI than predicted by conventional methods. A key driver of these differences was observed at the tree-level, where TLS-derived AGB estimates were systematically greater than allometric counterparts for large trees. This was also reflected in the GEDI analysis, where the AGB model for African deciduous broadleaf forests 34 , itself underpinned by allometry, generally predicted lower values than MSL-derived methods for higher densities ( > 50 Mg/ha). The importance of this point is magnified when considering the disproportionate contribution of large trees to upscaled AGB, as observed here (i.e., 11% of trees contributed greater than 50% of AGB across the six 1 ha stands) and described in literature 38 . We cannot definitively state whether this is due to over- or under-estimation of either method, or some mixture thereof, as accompanying destructive harvest data were not acquired. However, our TLS-derived estimates were calibrated using a representative sample of destructive measurements from the literature 23 . The trend for allometric and TLS methods to produce biased and unbiased estimates for large trees, respectively, is consistent with studies where estimates from both methods were coincident with destructive measurements across various forests 39 , 40 .
The cause of this potential systematic underestimation remains an open question. One possibility however, is that all widely-used allometrics are modelled via log-transformed linear regression 41 , and then applied to trees of all sizes (oftentimes caveated that any given model should not be used to predict an out-of-sample tree if its size falls outside the range of the calibration data 42 ). It is implicitly assumed then, that model parameters are as equally suitable for large trees, as they are for small trees, within the context of the accuracy of predictions. However, the underlying calibration data are, as a rule, skewed towards smaller trees, often necessarily because of the increasing difficulty of harvesting larger trees 43 . For example, for the miombo woodland specific and pan-tropical models considered here 29 , 30 , the median stem diameter of the 167 and 4004 trees comprising the calibration data was 30 and 15 cm (mean: 35 and 24 cm), respectively. This compares with a median and mean stem diameter of 48 and 50 cm for the largest 10% of trees across the six 1 ha stands. The nature of linear regression whereby each observation is usually assigned equal weight 44 , therefore suggests these aggregate models are unlikely to be representative of large trees, thus leading to biased predictions if this parameter invariance assumption is invalid 19 . Owing to the aforementioned context of large trees driving AGB distributions, and limited study on this subject 15 , 18 , 20 , an argument from parsimony would be that large tree allometric predictions are less certain than small tree estimates, unless proven otherwise.
The MSL methods used here provide capabilities to resolve this issue and further enhance conventional methods. Whilst the airborne components presented here are at a more experimental stage, TLS methods are closer to operational readiness and less cost prohibitive. These data can be collected from 1 ha plots, within days, using sampling protocols complying with adopted good practices 45 . Data processing methods including segmentation and structural modelling are complex, but substantial progress has been made in recent years, particularly on both automation 46 , and the avoidance of overestimating the volume of smaller trees and high-order branches 47 . TLS methods can be deployed in two ways: first, and most direct, estimating plot-scale AGB by summing contributions from individually modelled trees. This, applied across NFI networks for example, would enable updated EFs to be generated. Second, improving existing allometric models by augmenting their calibration datasets 48 . The key here would be generating uniform datasets (i.e., across tree size) through the addition of larger trees. This is appealing as it would both reduce the uncertainty in highly-practicable allometric methods, and leverage the value of historic datasets. However, appending calibration datasets with TLS-derived AGB observations is non-trivial. Assumptions in linear regression include that the mean of the distribution of error in the dependent variable (i.e., AGB) is zero, and ideally errors are not autocorrelated or heteroscedastic 44 . Therefore, such efforts require thoughtful undertaking.
Returning then to the region-scale predictions, while the divergence between TLS- and allometric-derived AGB explains part of the overall difference, they are also driven by the selected EFs. That is, these values are not only underpinned by allometrics, but also the sampling pattern of their underlying field plots, and how that differs from the composition of the ROI considered here. This was partially unpicked by stratifying the ROI into core park, buffer zone and beyond, where it was observed that AGB densities were still an increase of 75%, 77%, and 53% more than the mean of the selected EFs. An important contributor here is the long-tail of large MSL-derived AGB observations driven by aforementioned large trees (e.g., predictions greater than 150 Mg/ha contributed 36% to the total 3.65 Tg), and the statistical likelihood these would be undersampled by randomly distributed field plots.
The question remains then: what are the implications of these observed differences in AGB stocks for our understanding of miombo woodlands? That largely depends on the transferability of our results to the world’s 1.9 million km 2 of these forests 3 . The ROI where data were collected was deliberately positioned to capture as much of the range of states and successions, and therefore variance in AGB, across the wider region as possible. This capture of structural and taxonomic variation is illustrated by the inventory measurements across the six 1 ha plots (Table S1 ), where stem count, stem diameter and basal area ranged from 56 to 349, 10 to 75 cm and 1.9 to 20.9 m 2 /ha, respectively, and that 81 of the estimated 334 unique species across miombo woodlands were observed, including from the dominant Brachystegia and Julbernardia genera 1 . Further, tree fractional cover across the 50 kha ranged from 0 to 1 with a mean of 0.59. These traits coincide with the ranges observed more widely across the continent 49 , so we therefore suggest it is not unreasonable to consider these sampled forests as being at least somewhat representative of miombo woodlands more broadly.
Speculatively then, if we were to extrapolate our results across the world’s miombo woodlands, they potentially store in the region of 3.7 PgC more carbon in their AGB than currently estimated, assuming the mean of the considered EFs (56.6 Mg/ha) is uplifted by 74% (assuming 47% carbon content). It is also noteworthy that MSL methods detected an additional 0.20 Tg AGB stored across the ROI in land classified as non-forest, potentially increasing this delta still further, and emphasising that fragments of miombo woodlands have the potential to store significant quantities of carbon 50 .
Whilst such extrapolation requires additional data for confirmation, the magnitude of this difference suggests our understanding of the role these forests play in global change requires a rethink, considering this overall increase is approaching the current annual global atmospheric increase (5.1 PgC/yr) 51 . That is, these forests could have a more potent ability to sequester carbon from afforestation and reforestation efforts, albeit equally the reverse, that their loss leads to increased emissions. Finally, an uplift in the carbon density of these forests per unit area could correspond to a proportional factor increase of 1.5 to 2.2 in their value on carbon markets, thus better incentivising their protection and restoration, and disincentivizing the value extracted from their deforestation 52 .
Materials and methods
Site description.
The 50 kha region of interest (ROI) where data were collected (Fig. 1a ) was located on the southeastern border of Gilé National Park, Zambezia Province, Mozambique. The forests here feature woodlands, riverine forests, and wooded savannas, dominated by trees from the Brachystegia and Julbernardia genera, characteristic of the more broad classification of miombo woodland 1 . Mean annual precipitation is between 800 and 1000 mm, with a dry season May–October 53 . Mean monthly temperature varies from low teens to high thirties, the terrain is largely flat, and soils comprise sandy loam and sandy clay 54 .
Study design
The ROI was positioned such that it covered core park, buffer zone and beyond (Fig. 4a ). The dataset (Fig. 1a ) comprised airborne laser scanning (ALS) data across the entirety of the ROI (designation: GIL), unoccupied aerial vehicle laser scanning (UAV-LS) data from six 300 ha sections (designation: GIL01 to GIL06), and terrestrial laser scanning (TLS) measurements and inventory data from six 1 ha plots coincident with these sections (designation: GIL01-01 to GIL06-01). These sections and plots were strategically located to capture variations in forest state, succession, structure, and taxonomy across the ROI.
Data collection
Data were acquired between June-November 2022. The six 100 × 100 m planimetric plots were established and inventoried using RAINFOR protocol 55 . Measurements of each tree inside these plots with stem diameter ≥ 10 cm included: (i) stem diameter via a circumference/diameter tape, (ii) point of measurement of the stem diameter (either 1.3 m above ground or 0.5 m above-buttress), (iii) taxonomic identity determined by a single trained botanist, and (iv) x-y coordinates using eye-estimation.
TLS data were collected in GIL01-01 through GIL06-01 using a RIEGL VZ-400i laser scanner. Sampling followed established protocol 56 . That is, in accordance with the CEOS Aboveground Woody Biomass Product Validation Good Practices Protocol 45 . In particular, scans were acquired from 121 locations at 10 m intervals across each plot, with upright and tilt scans acquired at each location to capture a complete sample of the scene. The instrument pulse repetition rate was 300 kHz and the angular step between sequentially fired pulses was 0.04 degrees. The laser pulse had a wavelength, pulse width, beam divergence and exit footprint diameter of 1550 nm, 3.0 ns, 0.30 mrad, and 7.0 mm, respectively. Coarse georeferencing of scans was generated from an onboard GNSS receiver obtaining real-time differential corrections from a nearby static Emlid Reach RS2 GNSS receiver.
UAV-LS data were acquired across GIL01 through GIL06 using a RIEGL VUX-120 and Trimble Applanix APX-20 GNSS/INS kinematic laser scanning system mounted on a hybrid-electric drone, in a 50 m double-gridded configuration at 5.0 m/s velocity with an aboveground level of 100 m. The instrument pulse repetition rate, field of view and scan rate were 1800 kHz, 90 degrees and 315 lines per second, respectively. The laser pulse had a wavelength, pulse width, beam divergence and exit footprint diameter of 1550 nm, 3.0 ns, 0.38 mrad and 5.7 mm, respectively.
ALS data were acquired across GIL using the same kinematic laser scanning system mounted on a helicopter, in a 127 m spaced parallel line configuration at 41.2 m/s velocity with an aboveground level of 160 m. In this configuration, the pulse repetition rate, field of view and scan rate were 1200 kHz, 100 degrees and 396 lines per second, respectively. A nearby static Stonex S900A GNSS receiver collected observables throughout UAV-LS and ALS data acquisition for georeferencing purposes.
Data preprocessing
Inventory data (1406 trees) were manually digitised from field sheets, with accuracy assessed via a second operator digitising a randomly selected 5% subset. Errors in taxonomic identity were resolved using the Taxonomic Name Resolution Service 57 . Estimates of basic woody tissue density were derived from the mean of entries available in the Global Wood Density Database 58 , whereby attribution was made if possible at the species-, or else genus-level (84.4 and 14.2% of the trees, respectively). If no taxonomic attribution could be made, basal area-weighted plot average wood densities were used (1.4%).
TLS data were co-registered into georeferenced (EPSG: 32737) tiled point clouds (Fig. 1c ) using RIEGL RiSCAN Pro (v2.15) via its Automatic Registration 2 and Multi Station Adjustment 2 modules. Airborne mission trajectories were refined to survey-grade accuracy and precision via Applanix POSPac UAV (v8.8) using GNSS observables from the base station, whose absolute positioning was refined using the AUSPOS service 59 . Lidar data were united with these trajectories and merged into georeferenced tiled point clouds (Fig. 1c ) using RIEGL RiPROCESS (v1.9.2.2) including its RiUNITE (v1.0.3.3) and RiPRECISION (v1.4.2) modules. TLS point cloud georeferencing was refined by aligning with the UAV-LS data using an iterative closest point algorithm implemented in CloudCompare (v2.12.4). Noise in TLS, UAV-LS and ALS point clouds was labelled using reflectance and deviation thresholding 60 , and statistical outlier filtering. Incompletely sampled tiles were discarded based on point density and morphological erosion.
Data processing
TLS-derived tree-scale aboveground biomass (AGB) estimates were generated in six steps. First, point clouds representing individual trees either inside, or part of whose AGB fell inside the plot, were segmented from the tiled point clouds (1,339 trees). This was undertaken both manually in CloudCompare (v2.12.4) and using the Forest Structural Complexity Tool 46 . Second, point clouds were manually linked with inventory data via stem maps, whereby due to edge effects around the plot (i.e. trees with stem base inside but crown partly growing outside the plot, and vice versa) and multi-stemmed trees, there were somewhat fewer point clouds than census entries (1339 vs 1406 respectively). Third, leafy material, which had distinctively lower apparent reflectance than the woody material, was segmented from point clouds via thresholding based on evaluating single-tree reflectance histograms. Fourth, quantitative structural models (QSMs) (Fig. 3a ) were constructed for woody point clouds using TreeQSM 21 (v2.4.1). QSMs were inspected by eye, and validated via comparison to the input point clouds (i.e., point-to-cylinder distances). Fifth, potential overestimations of small branch volume arising from wind, co-registration error, properties of the laser pulse itself, or some mixture thereof, were negated using a post-processing step based on metabolic scaling theory 61 . This limited the maximum diameter of third order and above branches to half the diameter of their parent, and the diameter of cylinders intra-branch to no more than their parent. This was validated by comparing open-access data from previous studies where destructive data were available 23 . Sixth, AGB was estimated from QSM-derived volume and basic woody tissue density, the latter obtained from the established link between point clouds and inventory data.
Metrics describing forest structure (Fig. 2d, e ) were generated from the UAV-LS and ALS point clouds at 10 m resolution unless stated otherwise. The metrics, described in 25 , and retrieved using methods similar to those implemented in lidR 62 , comprised: digital terrain and canopy height models (1 m), relative height, tree fractional cover, canopy height rugosity, fixed and variable gap fraction, canopy closure, canopy ratio, z-entropy, skewness and kurtosis. Additionally, voxel-based metrics describing the 3D distribution of woody plant material were also retrieved 26 . This was undertaken by segmenting leafy material via reflectance thresholding, and partitioning the UAV-LS and ALS point clouds into voxels with 0.1 and 0.5 m edge length, respectively. Volumes of each voxel comprising at least one point were then aggregated in 3D grids of 1 m and 5 m resolution, respectively.
Aboveground biomass modelling
TLS-derived gridded estimates of AGB across GIL01-01 through GIL06-01 (Fig. 2a ) were estimated by constructing a georeferenced 10mgrid across each plot, decomposing each QSM into its constituent cylinders, and allocating volume to respective cells. Numerical approximation estimated the quantity of intra-cylinder volume assigned to multiple cells. Cells were considered valid only when all AGB from trees with stem diameter ≥10 cm was captured. This included contributions from trees outside the plot whose stem or crown only partially fell inside the cell in question. For such trees, QSMs were produced with the encroaching cylinder volumes attributed to the relevant cell, assuming a wood density equal to the plot basal area-weighted wood density. In total, 568 cells, of which 473 were non-zero, were created with biomass contributions from 1,259 QSMs.
Gridded estimates of AGB (10 m resolution) across GIL01 through GIL06 (Fig. 2b ) were retrieved using extreme gradient boosting machine learning via XGBoost 63 (v1.6.2), which has previously been applied to AGB modelling 24 . The TLS-derived gridded estimates of AGB were used as training data, and the spatially coincident UAV-LS-derived forest structure metrics were used as predictor variables. The choice of metric and the produced biomass map resolution (10 m) was informed by balancing having plentiful training pixels (i.e. higher resolution) versus the information contained within each pixel to predict biomass, using a rule of thumb that there be at least ten times more training pixels than features. Optimised hyperparameters and feature selection were found by minimising the root mean square error of validation folds within a spatial cross-validation framework 27 using GIL01-01 through GIL06-01 as separate folds. For this, a random grid search of the following six hyperparameters was undertaken: (i) learning rate (a step shrinkage weight; used to make the boosting process more conservative and reduce the risk of overfitting); (ii) minimum loss reduction (a decision parameter to make a partition on a leaf node of the tree); (iii) maximum depth of a tree (a proxy for the complexity, and hence overfitting risk, of the model); (iv) minimum child weight (minimum sum of the hessian required in each child, with higher values again resulting in more conservative models); (v) subsample of the training instances (fraction sampled from the training data prior to tree growing); and (vi) column sampling ratio (fraction of the features to be subsampled). Further performance metrics were also generated, including bias, and two based on the log of the accuracy ratio: (i) median symmetric accuracy, and (ii) symmetric signed percentage bias. These two metrics are well-suited to assessing predictions potentially spanning several orders of magnitude 64 .
Gridded estimates of AGB (10 m resolution) across GIL (Fig. 2c ) were retrieved by repeating this step, where the UAV-LS-derived gridded estimates of AGB were the training data, and the spatially coincident ALS-derived forest structure metrics were the predictor variables. Fig. S 1 and Fig. S 2 present cross-validation statistics, the weight and gain assigned to each predictor variable, and scatter plots illustrating predicted versus reference AGB, for the UAV-LS and ALS models, respectively.
A direct TLS-to-ALS upscaling model was also tested (i.e., skipping the intermediate UAV-LS layer). The direct model was slightly more biased (cross-validation bias with TLS labels: −3.63%) and less accurate (cross-validation RMSE with TLS labels: 62.6 Mg/ha), thus not considered further in this study. Further methodological improvements to the MSL workflow could encompass more sophisticated lidar features, perhaps tailored to the extreme high point density of UAV-LS, that are likely to correlate more with AGB than the ones used in our study. Additionally, spatially explicit models, such as convolutional neural networks, can take into account spatial context rather than only the pixel values themselves. Here, spatial information is only used in the cross-validation of the XGBoost models.
Uncertainty quantification
Uncertainty in TLS-derived tree-scale AGB arises from the underlying point cloud itself, quantitative structural modelling and basic woody tissue density estimation 43 . Uncertainty from these sources was implicitly captured by modelling the expected distribution of error using existing data where TLS-derived AGB estimates were available alongside reference measurements derived from destructive harvesting and weighing (391 trees from 111 species) 23 . To increase the representativity of these harvested trees to the ROI, the dataset was subsetted to contain only trees with stem diameter <75 cm, scanned in leaf-on conditions, and to exclude trees from boreal and temperate regions ( n = 174). The error distribution was modelled via the mean and variance of residuals, as a function of stem diameter, using linear and non-linear quantile regression, respectively. The appropriate mean residual was subtracted from the raw AGB estimate for each tree to remove bias known to arise in TLS-derived volume estimates, especially in smaller branches 47 , complementing the QSM postprocessing based on metabolic scaling. Uncertainty in TLS-derived gridded AGB was derived as a volume-weighted combination of tree-scale AGB uncertainty, by modelling the true AGB of each tree as following a Gaussian distribution dependent on its TLS-estimated AGB, and independent of all other trees. This assumption of independence refers only to effects causing a discrepancy between TLS-estimated AGB and true AGB (i.e., imperfections in lidar scanning, QSM reconstruction and wood density assignment) and does not neglect spatial correlation of true AGB (e.g., arising from the effect of trees on each other), which is inherited by TLS-estimated AGB, but remains an approximation since spatial correlation may exist in some residual effects such as wind noise. Consequently, the only modelled source of covariance is due to a given tree spanning more than one pixel. This enabled calculation of the full pixel covariance matrix, capturing both the marginal uncertainty in each pixel and the correlation between pixels.
Uncertainty in UAV-LS-derived gridded estimates of AGB was modelled as the sum of two independent components: measurement variance and model variance. Measurement variance was estimated using a Monte Carlo random sampling approach. 100 samples of each of the six TLS-derived gridded AGB estimates were generated from their underlying multivariate Gaussian distribution, described above. Each set of six gridded AGB estimates was used to train a separate XGBoost model, producing 100 gridded predictions of AGB for each UAV-LS section, the sample variance across which was taken as the measurement variance, per pixel. Model variance was estimated as a zero-intercept linear function of predicted pixel AGB, with slope calibrated from the cross-validation data described in the previous section. Uncertainty in ALS-derived gridded estimates of AGB (Fig. 2c ) was estimated by repeating this process, using the 100 UAV-LS-derived gridded AGB estimates as training data to a further ensemble of 100 XGBoost models. Uncertainty is expressed as 90% confidence intervals throughout.
Conventional methods
Allometric-derived tree-scale AGB estimates were produced by three allometric models. These models are widely used for carbon stock estimation in the region. First, the pan-tropical allometry described in 30 that considers the predictor variables stem diameter, tree height and basic woody tissue density, itself calibrated from the harvest of 4,004 trees across the tropics and subtropics. Second, two miombo woodland specific allometries described in 29 , themselves calibrated from the harvest of 167 trees in Tanzania, that consider: (i) stem diameter only, and (ii) stem diameter and tree height. Tree height was derived from TLS data. Individual trees with non-matching stem diameters between the inventory and TLS were excluded from the tree-level AGB comparison (i.e., stem diameters with >5 cm difference; n = 188) to ensure potential errors in linking both data sets were omitted.
A selection of four representative emission factors (EFs) were gathered to enable conventional region-scale estimation of AGB. We used values from: (i) the IPCC default for African subtropical dry forest described in 12 (using a combination of L- and C-band radar); (ii) Mozambique’s Forest Reference Emission Levels 31 for semi-deciduous forest including Miombo (based on the country’s National Forest Inventory and the allometrics described in 29 ); (iii) specific to Zambezia province described in 32 (obtained from L-band radar and a network of forest plots using the allometrics of 30 ); and (iv) Mozambique-wide described in 33 (destructive harvesting coupled to 27 ha forest inventory).
GEDI L2A (version 2) and L4A (version 2.1) products (i.e., relative height metrics and AGB density, respectively) were downloaded from NASA’s Earthdata 65 . These data were filtered to only include observations between day-of-year 275 and 365 (to match seasonality) from the years 2018–2022, with a sensitivity greater than 90%, spatially overlapping with the ALS data, and with 98% relative heights under 35 m. This resulted in 18,611 GEDI observations (Fig. 5a ) from the available 72,101. Coincident MSL-derived AGBD was retrieved by simulating a circle at the GEDI footprint coordinates (12.5 m radius), and performing a weighted average extraction from the gridded 10 m resolution lidar-derived AGB predictions. No geospatial aligning of GEDI footprints with the ALS data was applied.
Additionally, we tested the influence of the AGB model underpinning GEDI’s L4 product in the region (i.e. the African dry broadleaf forest model (DBT.Af) 34 ). Since relative heights retrieved from the ALS data (calculated from the height distributions within a point cloud) are not comparable to GEDI-derived relative height (derived from a waveform from a single laser pulse), we simulated the ALS data into GEDI-perceived waveforms for 10,000 randomly distributed 25 m diameter point cloud sections within the ROI using gediRat 35 . These resulting simulated waveforms were directly comparable with GEDI’s waveforms and thus were used as input to GEDI’s DBT.Af model, to predict AGBD.
Data availability
Gridded estimates of AGB and its uncertainty (10 m resolution) from the TLS, UAV-LS and ALS data, and gridded metrics of forest structure (10 m resolution) from the UAV-LS and ALS data, for the section GIL04, and the data to produce the graphs and charts, are available at https://doi.org/10.5281/zenodo.11072918 . These data are distributed under the terms of the Creative Commons Attribution-NonCommercial 4.0 International License (CC BY-NC 4.0).
Code availability
Semantic and instance segmentation was undertaken using a modified version of FSCT 46 . Quantitative structural models were generated using a modified version of TreeQSM 21 . Forest structure metrics were retrieved via a C + + implementation of lidR 62 . Extreme gradient boosting models were constructed using XGBoost 63 . These underlying packages are available at https://github.com/SKrisanski/FSCT , https://github.com/InverseTampere/TreeQSM , https://github.com/r-lidar/lidR and https://github.com/dmlc/xgboost , respectively.
Abbot, J. et al. The Miombo in transition: woodlands and welfare in Africa . The Miombo in Transition: Woodlands and Welfare in Africa (Center for International Forestry Research CIFOR, 1996). https://doi.org/10.17528/cifor/000465 .
Ryan, C. M. et al. Ecosystem services from southern African woodlands and their future under global change. Philos. Trans. R. Soc. B Biol. Sci . 371 , 20150312 (2016).
Ribeiro, N. S., Silva de Miranda, P. L. & Timberlake, J. Biogeography and Ecology of Miombo Woodlands. in Miombo Woodlands in a Changing Environment: Securing the Resilience and Sustainability of People and Woodlands (eds. Ribeiro, N. S., Katerere, Y., Chirwa, P. W. & Grundy, I. M.) 9–53 (Springer International Publishing, 2020). https://doi.org/10.1007/978-3-030-50104-4_2 .
Herold, M. et al. The role and need for space-based forest biomass-related measurements in environmental management and policy. Surv. Geophys. 40 , 757–778 (2019).
Article Google Scholar
Houghton, R. A. Aboveground forest biomass and the global carbon balance. Glob. Chang. Biol. 11 , 945–958 (2005).
Pan, Y. et al. A large and persistent carbon sink in the World’s forests. Science 333 , 988–993 (2011).
Article CAS Google Scholar
Yanai, R. D. et al. Improving uncertainty in forest carbon accounting for REDD+ mitigation efforts. Environ. Res. Lett. 15 , 124002 (2020).
Grassi, G. et al. The key role of forests in meeting climate targets requires science for credible mitigation. Nat. Clim. Chang. 7 , 220–226 (2017).
Clark, D. B. & Kellner, J. R. Tropical forest biomass estimation and the fallacy of misplaced concreteness. J. Veg. Sci. 23 , 1191–1196 (2012).
Giménez, M. G. et al. Assessment of Innovative Technologies and Their Readiness for Remote Sensing-Based Estimation of Forest Carbon Stocks and Dynamics . Report no. 160649, 1-41. http://documents.worldbank.org/curated/en/305171624007704483/Assessment-of-Innovative-Technologies-and-Their-Readiness-for-Remote-Sensing-Based-Estimation-of-Forest-Carbon-Stocks-and-Dynamics (2021).
Espejo, A. et al. Integration of remote-sensing and ground-based observations for estimation of emissions and removals of greenhouse gases in forests: Methods and guidance from the Global Forest Observations Initiative . https://www.reddcompass.org/mgd/resources/GFOI-MGD-3.1-en.pdf (2020).
Rozendaal, D. M. A. et al. Aboveground forest biomass varies across continents, ecological zones and successional stages: refined IPCC default values for tropical and subtropical forests. Environ. Res. Lett. 17 , 014047 (2022).
Maniatis, D. & Mollicone, D. Options for sampling and stratification for national forest inventories to implement REDD+ under the UNFCCC. Carbon Balance Manag. 5 , 9 (2010).
Brown, S., Gillespie, A. J. R. & Lugo, A. E. Biomass estimation methods for tropical forests with applications to forest inventory data. For. Sci. 35 , 881–902 (1989).
Google Scholar
Burt, A. et al. Assessment of bias in pan-tropical biomass predictions. Front. For. Glob. Chang . 3 , 12 (2020).
Réjou-Méchain, M., Tanguy, A., Piponiot, C., Chave, J. & Hérault, B. Biomass: an R package for estimating above-ground biomass and its uncertainty in tropical forests. Methods Ecol. Evol. 8 , 1163–1167 (2017).
Picard, N., Boyemba Bosela, F. & Rossi, V. Reducing the error in biomass estimates strongly depends on model selection. Ann. For. Sci. 72 , 811–823 (2015).
Picard, N., Rutishauser, E., Ploton, P., Ngomanda, A. & Henry, M. Should tree biomass allometry be restricted to power models?. For. Ecol. Manage. 353 , 156–163 (2015).
Zhou, X. et al. Dynamic allometric scaling of tree biomass and size. Nat. Plants 7 , 42–49 (2021).
Calders, K. et al. Laser scanning reveals potential underestimation of biomass carbon in temperate forest. Ecol. Solut. Evid. 3 , 1–30 (2022).
Raumonen, P. et al. Fast automatic precision tree models from terrestrial laser scanner data. Remote Sens. 5 , 491–520 (2013).
Chave, J. et al. Towards a worldwide wood economics spectrum. Ecol. Lett. 12 , 351–366 (2009).
Demol, M. et al. Estimating forest above‐ground biomass with terrestrial laser scanning: Current status and future directions. Methods Ecol. Evol. 13 , 1628–1639 (2022).
Li, Y., Li, M., Li, C. & Liu, Z. Forest aboveground biomass estimation using Landsat 8 and Sentinel-1A data with machine learning algorithms. Sci. Rep. 10 , 1–12 (2020).
McNicol, I. M. et al. To what extent can UAV photogrammetry replicate UAV LiDAR to determine forest structure? A test in two contrasting tropical forests. J. Geophys. Res. Biogeosci. 126 , 1–17 (2021).
Whelan, A. W., Cannon, J. B., Bigelow, S. W., Rutledge, B. T. & Sánchez Meador, A. J. Improving generalized models of forest structure in complex forest types using area- and voxel-based approaches from lidar. Remote Sens. Environ. 284 , 113362 (2023).
Ploton, P. et al. Spatial validation reveals poor predictive performance of large-scale ecological mapping models. Nat. Commun. 11 , 1–11 (2020).
Dubayah, R. et al. GEDI launches a new era of biomass inference from space. Environ. Res. Lett. 17 , 095001 (2022).
Mugasha, W. A. et al. Allometric models for prediction of above- and belowground biomass of trees in the miombo woodlands of Tanzania. For. Ecol. Manage. 310 , 87–101 (2013).
Chave, J. et al. Improved allometric models to estimate the aboveground biomass of tropical trees. Glob. Chang. Biol. 20 , 3177–3190 (2014).
Governo de Moçambique. Mozambique’s Forest Reference Emission Level for Reducing Emissions from Deforestation in Natural Forests . https://redd.unfccc.int/media/2018_frel_submission_mozambique.pdf (2018).
McNicol, I. M., Ryan, C. M. & Mitchard, E. T. A. Carbon losses from deforestation and widespread degradation offset by extensive growth in African woodlands. Nat. Commun. 9 , 3045 (2018).
Ryan, C. M., Williams, M. & Grace, J. Above- and belowground carbon stocks in a miombo woodland landscape of mozambique. Biotropica 43 , 423–432 (2011).
Kellner, J. R., Armston, J. & Duncanson, L. Algorithm theoretical basis document for GEDI footprint aboveground biomass density. Earth Sp. Sci. 10 , 1–20 (2023).
Hancock, S. et al. The GEDI simulator: a large-footprint waveform lidar simulator for calibration and validation of spaceborne missions. Earth Sp. Sci. 6 , 294–310 (2019).
Mugabowindekwe, M. et al. Nation-wide mapping of tree-level aboveground carbon stocks in Rwanda. Nat. Clim. Chang. 13 , 91–97 (2023).
Quegan, S. et al. The European Space Agency BIOMASS mission: Measuring forest above-ground biomass from space. Remote Sens. Environ. 227 , 44–60 (2019).
Slik, J. W. F. et al. Large trees drive forest aboveground biomass variation in moist lowland forests across the tropics. Glob. Ecol. Biogeogr. 22 , 1261–1271 (2013).
Calders, K. et al. Nondestructive estimates of above-ground biomass using terrestrial laser scanning. Methods Ecol. Evol. 6 , 198–208 (2015).
Gonzalez de Tanago, J. et al. Estimation of above‐ground biomass of large tropical trees with terrestrial LiDAR. Methods Ecol. Evol. 9 , 223–234 (2018).
Kerkhoff, A. J. & Enquist, B. J. Multiplicative by nature: why logarithmic transformation is necessary in allometry. J. Theor. Biol. 257 , 519–521 (2009).
Chave, J. et al. Error propagation and scaling for tropical forest biomass estimates. Philos. Trans. R. Soc. London. Ser. B Biol. Sci. 359 , 409–420 (2004).
Burt, A. et al. New insights into large tropical tree mass and structure from direct harvest and terrestrial lidar. R. Soc. Open Sci. 8 , 201458 (2021).
Hayashi, F. Econometrics . (Princeton University Press, 2000).
Duncanson, L. et al. Aboveground woody biomass product validation good practices protocol. Version 1.0. Good Pract. Satell. Deriv. L. Prod. Valid . 1–236 https://doi.org/10.5067/doc/ceoswgcv/lpv/agb.001 (2021).
Krisanski, S., Taskhiri, M. S., Gonzalez Aracil, S., Herries, D. & Turner, P. Sensor agnostic semantic segmentation of structurally diverse and complex forest point clouds using deep learning. Remote Sens. 13 , 1413 (2021).
Abegg, M., Bösch, R., Kükenbrink, D. & Morsdorf, F. Tree volume estimation with terrestrial laser scanning — Testing for bias in a 3D virtual environment. Agric. For. Meteorol. 331 , 109348 (2023).
Momo Takoudjou, S. et al. Using terrestrial laser scanning data to estimate large tropical trees biomass and calibrate allometric models: A comparison with traditional destructive approach. Methods Ecol. Evol. 9 , 905–916 (2018).
The SEOSAW partnership A network to understand the changing socio‐ecology of the southern African woodlands (SEOSAW): challenges, benefits, and methods. Plants People Planet 3 , 249–267 (2021).
Reiner, F. et al. More than one quarter of Africa’s tree cover is found outside areas previously classified as forest. Nat. Commun. 14 , 2258 (2023).
Friedlingstein, P. et al. Global carbon budget 2021. Earth Syst. Sci. Data 14 , 1917–2005 (2022).
Austin, K. G. et al. The economic costs of planting, preserving, and managing the world’s forests to mitigate climate change. Nat. Commun. 11 , 5946 (2020).
Fusari, A., Lamarque, F., Chardonnet, P. & Boulet, H. Reserva Nacional do Gilé: Plano de Maneio 2012 − 2021 . 1–143. https://www.biofund.org.mz/wp-content/uploads/2015/03/PM-RNG-2012-2021.pdf (2010).
Montfort, F. et al. Regeneration capacities of woody species biodiversity and soil properties in Miombo woodland after slash-and-burn agriculture in Mozambique. For. Ecol. Manage. 488 , 119039 (2021).
Phillips, O., Baker, T., Feldpausch, T. R. & Brienen, R. RAINFOR: Field manual for plot establishment and remeasurement. 1–30. RAINFOR, PAN-AMAZONIA Project https://forestplots.net/upload/ManualsEnglish/RAINFOR_field_manual_EN.pdf (2021).
Wilkes, P. et al. Data acquisition considerations for Terrestrial Laser Scanning of forest plots. Remote Sens. Environ. 196 , 140–153 (2017).
Boyle, B. et al. The taxonomic name resolution service: an online tool for automated standardization of plant names. BMC Bioinf. 14 , 16 (2013).
Zanne, A. E. et al. Data from: Towards a worldwide wood economics spectrum. Dryad https://doi.org/10.5061/dryad.234 (2009).
Janssen, V. & McElroy, S. A Practical Guide to AUSPOS. Proceedings of the 25th Association of Public Authority Surveyors Conference , 3–28 (2022).
Pfennigbauer, M. & Ullrich, A. Improving quality of laser scanning data acquisition through calibrated amplitude and pulse deviation measurement. in Laser Radar Technology and Applications XV (eds. Turner, M. D. & Kamerman, G. W.) vol. 7684 76841F (2010).
West, G. B., Brown, J. H. & Enquist, B. J. A general model for the structure and allometry of plant vascular systems. Nature 400 , 664–667 (1999).
Roussel, J. R. et al. lidR: An R package for analysis of Airborne Laser Scanning (ALS) data. Remote Sens. Environ. 251 , 112061 (2020).
Chen, T. & Guestrin, C. XGBoost: A scalable tree boosting system. Proc. ACM SIGKDD Int. Conf. Knowl. Discov. Data Min . 785–794 (2016).
Morley, S. K., Brito, T. V. & Welling, D. T. Measures of model performance based on the log accuracy ratio. Sp. Weather 16 , 69–88 (2018).
Dubayah, R. et al. GEDI L4A footprint level aboveground biomass density. Version 2.1. https://doi.org/10.3334/ORNLDAAC/2056 (2022).
Download references
Acknowledgements
We gratefully acknowledge the following people and institutions for enabling and assisting with data collection: Aristides Muhate, Muri Soares, Alismo Herculano, and Délfio Mapsanganhe from the Monitoring, Reporting and Verification Unit of the National Fund for Sustainable Development; João Juvencio Muchanga, Thomas Buruwate, and Jose Zavale from Gilé National Park; Theo Kerr and Veronica Leitold from the University of Maryland; Selemane Momade Sualei and Elsa Carlos Jahar, chiefs of Namahipi and Malema village, respectively, and Edimur Alfonso Chambuca, Angel Musseia, João Wate, Alexandre Francisco Mulualua, Magido Martinho Uatelauane, Ali Fereira Marques, Artur Bernaldo Camoeis, Francisco Simão Waite, and Samuel Jaime Maqueia. We thank Gilé National Park and the villages of Malema and Namahipi for permission to acquire data on their land. We acknowledge funding from the World Bank through the Forest Carbon Partnership Facility. We acknowledge funding from the Government of Mozambique through the Monitoring, Reporting and Verification Unit of the National Fund for Sustainable Development. This work was supported by Innovate UK (project number 10004871).
Author information
Authors and affiliations.
Sylvera Ltd, London, UK
Miro Demol, Gabija Bernotaite, Elise Elmendorp, Allister Furey, Johannes Hansen, Harold Horsley, Annabel Locke, Hamidreza Omidvar, Ashleigh Parsons, Thomas Perry, Beisit L. Puma Vilca, Pedro Rodríguez-Veiga, Chloe Sutcliffe, Robin Upham, Benoît de Walque & Andrew Burt
The World Bank Group, Washington, DC, USA
Naikoa Aguilar-Amuchastegui, Andres Espejo & Elitsa Peneva-Reed
Department of Geography, University College London, London, UK
Mathias Disney
NERC National Centre for Earth Observation (NCEO), Leicester, UK
Department of Geographical Sciences, University of Maryland, College Park, USA
Laura Duncanson & Mengyu Liang
School of GeoSciences, University of Edinburgh, Edinburgh, UK
Steven Hancock
Independent researcher, Maputo, Mozambique
Independent researcher, Boane, Mozambique
Virgílio Manjate
Independent researcher, Inhaca, Mozambique
Francisco Mapanga
School of Geography, Geology and the Environment, University of Leicester, Leicester, UK
Pedro Rodríguez-Veiga
You can also search for this author in PubMed Google Scholar
Contributions
Study design: M. Demol, A.E., A.F., H.O., A.P., E.P.-R., A.B. Data acquisition: E.E., H.H., S.L., M.L., V.M., F.M., B.L.P.V., C.S., B.W. Data analysis: M. Demol, G.B., E.E., H.H., A.L., A.P., T.P., B.L.P.V., C.S., R.U., B.W., A.B. Interpretation of results: M. Demol, N.A.-A., G.B., M. Disney, L.D., S.H., J.H., M.L., P.R.-V., R.U., A.B. Writing—original draft: M. Demol, A.B. Writing—review & editing: all authors.
Corresponding author
Correspondence to Miro Demol .
Ethics declarations
Competing interests.
M. Demol, G.B., E.E., A.F., J.H., H.H., A.L., H.O., A.P., T.P., B.L.P.V., P.R.-V., C.S., R.U., B.W., and A.B. are employees and/or shareowners of Sylvera Ltd. N.A.-A., A.E., and E.P.-R. are employees of the World Bank’s Forest Carbon Partnership Facility. The interpretation of the results reflects the position of the authors and has not been endorsed by the World Bank. All other authors have no competing interests to declare.
Peer review
Peer review information.
Communications Earth & Environment thanks the anonymous reviewers for their contribution to the peer review of this work. A peer review file is available.
Additional information
Publisher’s note Springer Nature remains neutral with regard to jurisdictional claims in published maps and institutional affiliations.
Supplementary information
Peer review file, supplementary information, rights and permissions.
The opinions expressed in this article are those of the authors and do not necessarily reflect the views of the The World Bank, its Board of Directors, or the countries they represent. Open Access This article is licensed under the terms of the Creative Commons Attribution 3.0 IGO License, which permits use, sharing, adaptation, distribution and reproduction in any medium or format, as long as you give appropriate credit to the The World Bank, provide a link to the Creative Commons licence and indicate if changes were made. The use of the The World Bank’s name, and the use of the The World Bank’s logo, shall be subject to a separate written licence agreement between the The World Bank and the user and is not authorized as part of this CC-IGO licence. Note that the link provided below includes additional terms and conditions of the licence. The images or other third party material in this article are included in the article’s Creative Commons licence, unless indicated otherwise in a credit line to the material. If material is not included in the article’s Creative Commons licence and your intended use is not permitted by statutory regulation or exceeds the permitted use, you will need to obtain permission directly from the copyright holder. To view a copy of this licence, visit http://creativecommons.org/licenses/by/3.0/igo/ .
Reprints and permissions
About this article
Cite this article.
Demol, M., Aguilar-Amuchastegui, N., Bernotaite, G. et al. Multi-scale lidar measurements suggest miombo woodlands contain substantially more carbon than thought. Commun Earth Environ 5 , 366 (2024). https://doi.org/10.1038/s43247-024-01448-x
Download citation
Received : 07 November 2023
Accepted : 15 May 2024
Published : 10 July 2024
DOI : https://doi.org/10.1038/s43247-024-01448-x
Share this article
Anyone you share the following link with will be able to read this content:
Sorry, a shareable link is not currently available for this article.
Provided by the Springer Nature SharedIt content-sharing initiative
By submitting a comment you agree to abide by our Terms and Community Guidelines . If you find something abusive or that does not comply with our terms or guidelines please flag it as inappropriate.
Quick links
- Explore articles by subject
- Guide to authors
- Editorial policies
Sign up for the Nature Briefing: Anthropocene newsletter — what matters in anthropocene research, free to your inbox weekly.

Deploying ML for Voice Safety
Our mission is to connect a billion people with optimism and civility, which will require us to help people feel truly together with one another. For 3D immersive worlds, much like in the physical world, few things are more authentic or powerful than the human voice in forging lasting friendships and connections. But how do we scale the immersiveness and richness of voice communication on Roblox while keeping our community safe and civil?
In this blog, we’ll share how we brought to life Real-time Safety, an end-to-end machine learning (ML) model—operating at a scale of millions of minutes of voice activity per day—that detects policy violations in voice communication more accurately than human moderation. The outputs from this system are fed into another model, which determines the appropriate consequences. The consequence model triggers notifications for people who have violated our policies, initially with warnings and then with more drastic actions if the behavior persists.
This end-to-end Real-time Safety system was an audacious goal as, to our knowledge, no one else in the industry was delivering multilingual, near real-time voice safety features to their users. Voice classification depends on both audio style, including volume and tone, and content, including the words spoken. We are excited to share how we developed this system from essentially no prior automation—effectively zero labeled data and no models—going from zero to 60 for real-time voice safety.
And finally, we are excited to share our first open-source model , which is one of our voice safety models. In open sourcing this model and making it available for commercial use, we hope to provide an industry baseline for policy violation detection that can accelerate the development of newer ML models for voice safety. This open-source model is our first version, and we’ve since made significant improvements that we are currently testing.
Overcoming Data Scarcity
We began our ML efforts as many companies do—by assessing the quality of available data for training and evaluating our models. The ideal dataset pairing would include voice utterance along with a high-quality labeled safety categorization for that utterance. However, when we started, we had almost no large-scale human-labeled real-world data. To train a high-quality voice safety detection model using a supervised approach, we needed thousands of audio hours of labeled data for each language we supported, which would have taken years to gather and would’ve been prohibitively resource and time intensive.
Instead of relying on thousands of hours of hand-labeled data, we developed several more efficient methods:
Machine-labeled data for training. Instead of getting stuck on the pursuit of perfect hand-labeled data for training, we opted for a large volume of training data from machine labeling of voice utterances. Using large amounts of machine-labeled data with weak supervision generated training models that were robust to some noise in the labels. The keys to making this approach work were access to great open-source speech-to-text libraries and years of experience using ML to detect Community Standards violations in people’s textual communications. This machine labeling approach allowed us to label the volume of training data we needed for our models in weeks instead of years.
Human-labeled data for evaluation. Although high-quality yet imperfect machine-labeled data was good enough to train a highly performant model, we didn’t trust machine labels to perform the final validation of the resulting model. The next question, then, was where we could get enough human-labeled data for evaluation. Luckily, while it was impossible to gather enough human-labeled data for training in a timely way, it was possible to gather enough for evaluation of our model using our in-house moderators, who were already classifying abuse reports from people on Roblox to manually issue consequences. This allowed us to enjoy the best of both worlds: machine-labeled training data that was good and plentiful enough to produce a highly performant model, and human-labeled evaluation data that was much smaller in volume but more than enough to give us confidence that the model truly worked.
Another area where we faced data scarcity was in policy violation categories where we have very low prevalence, such as references to drugs and alcohol or self-harm. To address this issue, we combined several low-prevalence categories into an “other” category. As a result, our eventual model could identify the categories of profanity, bullying, discrimination, dating, and “other.” In order to understand these “other” categories, so we can better protect our community and ensure safe and civil discourse on Roblox, we will continue monitoring these for more examples. Over time, the subcategories in “other” will also become named categories as the number of training examples in those subcategories reaches a critical mass.
Machine Labeling Pipeline for Training Data
We designed a fully automatic machine labeling pipeline for extracting high-quality labels from voice chat sequences. Our pipeline consists of three stages:
Audio chunk splitting. The first stage of the pipeline involves splitting the audio into chunks, or shorter segments, wherever we detect periods of silence between sentences. It allows us to identify and label policy-violating content more efficiently.
Audio transcription. The second stage of the pipeline consists of transcribing these audio chunks into text using an automatic speech recognition (ASR) model. We use publicly available open source ASR models.
Text classification. The final stage of the pipeline involves classifying the transcribed text using our in-house text filter. This filter is designed to detect and block inappropriate content in text-based communications. We adapted the filter to work with the transcribed audio data, allowing us to label the audio chunks with policy-violation classes and keywords. The text filter is an ensemble model trained on human-labeled policy-violating text data comprising an extended DistilBERT model and regular expression rules.
It’s important to note that this pipeline was used only for generating training data for our ultimate production model. You might wonder, however, why train a model at all if there’s already a pipeline here that generates the labels we are looking for? The answer is efficiency—we need to be incredibly accurate, in far less time. At Roblox scale, invoking the ASR to transcribe all voice communications would be prohibitively slow and resource intensive. However, a compact ML model trained from this data, specifically designed to detect policy violations in voice communications without doing a full transcription, is equally accurate, yet significantly faster and can be used at Roblox scale.
Scaling the Machine Labeling Pipeline
With most large AI initiatives, the mechanism to obtain quality training data is itself a production ML system , which needs to be created from scratch. For this project, we needed to develop our machine labeling pipeline as a first-class production system with 24/7 uptime and the ability to scale to thousands of concurrent CPU or equivalent numbers of GPU. We implemented a training data cluster with thousands of CPU cores that automatically process the incoming audio streams in parallel to generate machine labels. This system had to run flawlessly for maximal throughput, and any mistakes or downtime could result in days or weeks of lost time in training data generation.
Below is a high-level overview of the architecture that supported the scale we needed to machine label tens of thousands of audio hours in a matter of just weeks. The key takeaway here was that investing in queues at key points in our processing allowed us to remove bottlenecks by horizontally scaling worker threads across many machines. These worker threads performed the audio chunk splitting, audio transcription, and text classification steps mentioned in the previous section.
ML Architecture
A central requirement for our model search was low latency, i.e., near real-time speeds for model inference, which led us to architectures that directly operate on raw audio and return a score. We use Transformer-based architectures, which work very well for sequence summarization and are very successful in the industry for natural language processing (NLP) and audio modeling. Our challenge was to find a sweet spot that balances complexity with low-latency inference—i.e., handling multiple languages plus accents, robustness to background noise, and audio quality, while satisfying our product latency constraints.
Model Selection
An immediate design question was to determine the size of the context window needed to train the Transformer models. We looked at the histogram of length of utterances in voice chat data across several days of usage and determined that a 15-second window provided the trade-off between latency and sufficient context needed for classification. We use “no-violation” as a category to detect the absence of policy violations. Given that a single audio clip can embody multiple types of violations, the task inherently becomes multilabel rather than a conventional multiclass classification problem. We fine-tuned the entire network, including head layers for this task, with binary cross-entropy (BCE) loss.
Caption: Histogram of voice utterances from chat data, showing that 75 percent of utterances are less than 15 seconds.
We evaluated several popular open source encoder models from the audio research community and narrowed down our choices to WavLM and Whisper . Our first experiment was to fine-tune the pretrained WavLM base+ with 2,300 hours of Roblox machine-labeled voice data and evaluate the classification results on two real-world eval datasets. We obtained very encouraging classification results (see Model Evaluation, below), but found that the latency was larger than our thresholds for production deployment. As a follow-up, we implemented a custom version of the WavLM architecture with fewer Transformer layers and trained an end-to-end model from scratch on 7,000 hours of Roblox machine-labeled voice data. This model produces robust classifications in conversational settings and was more compact compared with the fine-tuned model. Our final model candidate used a student-teacher distillation setup, with a Whisper encoder as the teacher network and the WavLM end-to-end architecture as the student network. When we trained it on 4,000 hours of audio, we saw classification accuracies similar to the fine-tuned model but with a substantial improvement in latency and reduced model size. The image below summarizes the model parameters for the three experiments described above. We continue to iterate the data sampling strategies, evaluation strategies, and model hyperparameters as we extend the models for multilingual voice safety classification.
|
|
|
| |
| 2300h | 96M parameters | 102 ms | 9.80 |
| 7071h | 52M parameters | 83 ms | 12.08 |
| 4080h | 48M parameters | 50 ms | 19.95 |
Model Optimization
We employed standard industry methods, including quantizing selected Transformer layers to achieve a more than 25 percent speedup without compromising quality. Switching the feature extraction stage to MFCC inputs combined with convolutional neural networks (CNNs) instead of only CNNs also resulted in greater than 40 percent speedups during inference. Additionally, introducing a voice activity detection (VAD) model as a preprocessing step significantly increased the robustness of the overall pipeline, especially for users with noisy microphones. VAD allowed us to filter out noise and apply our safety pipeline only when we detect human speech in the audio, which reduced the overall volume of inference by approximately 10 percent and provided higher-quality inputs to our system.
Model Evaluation
Although we used many different datasets and metrics for evaluation, we can share how our voice classifier performed on an English-language dataset with high policy violation prevalence (such as what we would find in voice abuse reports from users). This dataset was 100 percent human labeled by our moderators. When we combined all violation types (profanity, bullying, dating, etc.) into a single binary category, we observed a PR-AUC (area under precision-recall curve) score of over 0.95, as shown below. This means that on this evaluation dataset, the classifier can typically catch a great majority of violations without falsely flagging too many non-violations.
The strong evaluation results above, however, do not necessarily translate directly across all use cases. For example, in the case of our notifications about policy-violating speech, the classifier is evaluating all Roblox voice chats and finding a lower prevalence of violations, and there is a greater chance of false-positive results. In the case of voice abuse reports, the classifier is evaluating only speech that has been flagged for potential violations, so the prevalence is higher. Still, the results above were encouraging enough for us to initiate experiments with the classifier in production (at conservative thresholds) to notify users about their policy-violating language. The results of these experiments greatly exceeded our expectations.
What’s Next?
By leveraging our own CPU infrastructure and carefully designing the pipeline for large scale, we were able to successfully deploy this model at Roblox scale. During peak hours, the model is successfully serving over 2,000 requests per second (the majority of which contain no violations). We have also observed a significant reduction in policy-violating behavior on the platform due to the use of the model for notifying people about policy-violating language. In particular, from our initial rollout, we are seeing a 15.3 percent reduction in severe-level voice abuse reports and an 11.4 percent decrease in violations per minute of speech.
We are extending our models with multilingual training data, which allows us to deploy a single classification model across the platform to handle several languages as well as language mixing. We are also exploring new multitask architectures for identifying select keywords in addition to the classification objective without resorting to full ASR. The detection of these keywords in addition to violation labels improves the quality of the classification and provides an opportunity to give people context while issuing consequences.
The research described here was a joint effort across many teams at Roblox. This was a great display of our core value of respecting the community and a great collaboration across multiple disciplines.
We've detected unusual activity from your computer network
To continue, please click the box below to let us know you're not a robot.
Why did this happen?
Please make sure your browser supports JavaScript and cookies and that you are not blocking them from loading. For more information you can review our Terms of Service and Cookie Policy .
For inquiries related to this message please contact our support team and provide the reference ID below.

COMMENTS
Thinking and communicating with data visualizations is critical for an educated public (Börner et al., 2019).Science education standards require students to use visualizations to understand relationships, to reason about scientific models, and to communicate data to others (National Governors Association Center for Best Practices and Council of Chief State School Officers, 2010; National ...
Information data can be represented in different forms such as text, numbers, images, audio, and video. A network, as discussed throughout this chapter, is a set of devices (often referred to as nodes) that are connected by communication links.A node is a computer, printer, or any device that can send and/or receive data generated by other nodes on the network.
Explore the latest full-text research PDFs, articles, conference papers, preprints and more on DATA COMMUNICATIONS. Find methods information, sources, references or conduct a literature review on ...
Summary. Communication research has recently had an influx of groundbreaking findings based on big data. Examples include not only analyses of Twitter, Wikipedia, and Facebook, but also of search engine and smartphone uses. These can be put together under the label "digital media.". This article reviews some of the main findings of this ...
Part of an innovative journal, this section explores high-quality fundamental and applied research in the area of data science for communication.
Thus, data stemming from digital communication is an important source of insights for retailers, manufacturers, and service firms alike. This article discusses emerging trends and recent advances in digital communication research, as well as its future opportunities for retail practice and research.
Communication Research (CR), peer-reviewed and published bi-monthly, has provided researchers and practitioners with the most up-to-date, comprehensive and important research on communication and its related fields.It publishes articles that explore the processes, antecedents, and consequences of communication in a broad range of societal systems.
Data communication has been with us for a long time. Smoke signals, drum beats, and semaphore signals are examples that are commonly given; indeed, semaphore relay may be regarded as the first modern communication network [1]. ... Toward a National Research Network, Computer and Science and Technology Board, National Research Council, National ...
Presents outstanding research papers presented at GUCON 2019. Discusses new findings in data communication and networks. Offers a valuable reference guide for researchers and practitioners in academia and industry. Part of the book series: Advances in Intelligent Systems and Computing (AISC, volume 1049) 15k Accesses. 1 Altmetric.
Data communication networks deal with the transfer of data between two points. Data originates at the source and is finally delivered to the destination, which is also called a sink. It can also be classified according to the type of medium over which the signal propagates.
Presents research works in the field of data communication; Provides original works presented at ICIDCA 2021 held in Coimbatore, India ... His current research interest is more interdisciplinary in nature but focuses on the use of IT in industry and systems. Dr. Kamel's area of expertise is computer control, sensory fusion, and distributed ...
Data communication is the process of transferring data from one place to another or between two locations. It allows electronic and digital data to move between two networks, no matter where the two are located geographically, what the data contains, or what format they are in. A common example of data communication is connecting your laptop to ...
Extracting scientific data from published research is a complex task required specialised tools. Here the authors present a scheme based on large language models to automatise the retrieval of ...
The article was prepared bas d on literature review, research reports and an an lysis of seco dary s ur es. It also utlines the practical implications. The consideratio s provided are the basis for further activities and empirical research. Keywords: Big Data, rketing communication, man gement, data processing and llectio , ommunication ma ...
A lack of bandwidth can present several problems for. networking and data communication. Network congestion, where th e bandwidth is not enough to handle the volume of. traffic, is one of the mai ...
Abstract. A computer network, sometimes known as a data network, is a kind of telecommunications network that enables computers to communicate with one another. Data is passed between networked computing devices through data links in computer networks. Cable or wireless media are used to establish connections (network links) between nodes.
Data communications Abstract: The author describes how events in the consumer market and the business world have recently shaped (and for the next few years will continue to shape) this area far more than technological breakthroughs. The need for real-time delivery of much higher volumes of data to meet the video and audio requirements of ...
Explore the latest full-text research PDFs, articles, conference papers, preprints and more on DATA COMMUNICATIONS. Find methods information, sources, references or conduct a literature review on ...
4 Benefits of Data Communication. Before we can get into the benefits of data communication, it's important to separate the concept of connectivity from communications. Connectivity is the capability of connecting one party to another. The benefits that arise from those connections depend on who's connecting to whom — or to what.
This web-based encyclopedia is searchable by keyword or subject. Research Methods in Communication by Alison Jane Pickard. Call Number: eBook. ISBN: 9781783300235. Publication Date: 2017. This eBook is full of ideas, use the "search within" function or scan the table of contents to get started. How to...Use ethnographical methods ...
Clinical Research Technology. RDCT provides data management and network services for customers with remote operations. We specialize in support for accurate data collection, management and validation. And our extensive experience in clinical trials and biomedical research give us a unique skill set for corporate communications and data integrity.
Electronics Letters is an interdisciplinary, rapid-communication journal covering the latest developments in all electronic and electrical engineering related fields. Electronics Letters: Vol 60, No 14
The SEC's investigations uncovered pervasive and longstanding uses of unapproved communication methods, known as off-channel communications, at all 16 firms. As described in the SEC's orders, the broker-dealer firms admitted that, from at least 2019 or 2020, their employees communicated through personal text messages about the business of ...
The latest installments of The Wharton School's faculty research podcast, 'Ripple Effect,' showcases recent books on leadership, customer service, immigration, and the power of data. Wharton's faculty podcast, " Ripple Effect ," introduces its "Meet the Author" series, highlighting recent books published by experts at the ...
1. AI for scientific discovery: While artificial intelligence (AI) has been used in research for many years, advances in deep learning, generative AI and foundation models are revolutionizing the scientific discovery process.AI will enable researchers to make unprecedented connections and advancements in understanding diseases, proposing new materials, and enhancing knowledge of the human body ...
Data Representation Data representation is defined as the methods used to represent information in computers. Different types of data can be stored in the computer system. This includes numeric ...
These data were filtered to only include observations between day-of-year 275 and 365 (to match seasonality) from the years 2018-2022, with a sensitivity greater than 90%, spatially overlapping ...
Caption: Histogram of voice utterances from chat data, showing that 75 percent of utterances are less than 15 seconds. We evaluated several popular open source encoder models from the audio research community and narrowed down our choices to WavLM and Whisper. Our first experiment was to fine-tune the pretrained WavLM base+ with 2,300 hours of ...
The Indian government rejected a Citigroup report claiming that the country will struggle to create enough jobs even if the economy grows at 7% annually.. India's Ministry of Labour and ...
The data communication is made easy using the networks. But still the data communication &. networking technology no fully matured. There are issues from several areas starting from the. designing ...