Causal Research: Definition, Design, Tips, Examples
Appinio Research · 21.02.2024 · 34min read
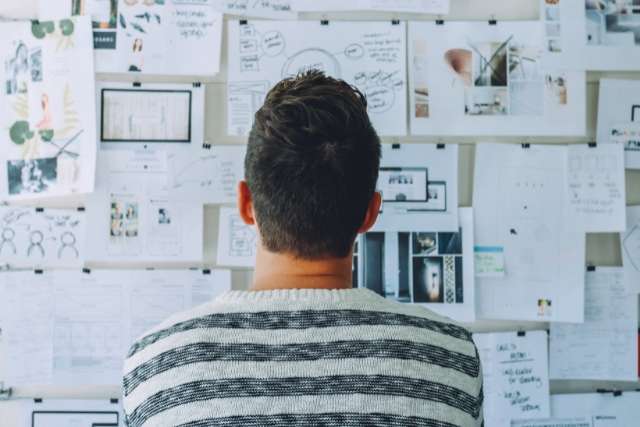
Ever wondered why certain events lead to specific outcomes? Understanding causality—the relationship between cause and effect—is crucial for unraveling the mysteries of the world around us. In this guide on causal research, we delve into the methods, techniques, and principles behind identifying and establishing cause-and-effect relationships between variables. Whether you're a seasoned researcher or new to the field, this guide will equip you with the knowledge and tools to conduct rigorous causal research and draw meaningful conclusions that can inform decision-making and drive positive change.

What is Causal Research?
Causal research is a methodological approach used in scientific inquiry to investigate cause-and-effect relationships between variables. Unlike correlational or descriptive research, which merely examine associations or describe phenomena, causal research aims to determine whether changes in one variable cause changes in another variable.
Importance of Causal Research
Understanding the importance of causal research is crucial for appreciating its role in advancing knowledge and informing decision-making across various fields. Here are key reasons why causal research is significant:
- Establishing Causality: Causal research enables researchers to determine whether changes in one variable directly cause changes in another variable. This helps identify effective interventions, predict outcomes, and inform evidence-based practices.
- Guiding Policy and Practice: By identifying causal relationships, causal research provides empirical evidence to support policy decisions, program interventions, and business strategies. Decision-makers can use causal findings to allocate resources effectively and address societal challenges.
- Informing Predictive Modeling : Causal research contributes to the development of predictive models by elucidating causal mechanisms underlying observed phenomena. Predictive models based on causal relationships can accurately forecast future outcomes and trends.
- Advancing Scientific Knowledge: Causal research contributes to the cumulative body of scientific knowledge by testing hypotheses, refining theories, and uncovering underlying mechanisms of phenomena. It fosters a deeper understanding of complex systems and phenomena.
- Mitigating Confounding Factors: Understanding causal relationships allows researchers to control for confounding variables and reduce bias in their studies. By isolating the effects of specific variables, researchers can draw more valid and reliable conclusions.
Causal Research Distinction from Other Research
Understanding the distinctions between causal research and other types of research methodologies is essential for researchers to choose the most appropriate approach for their study objectives. Let's explore the differences and similarities between causal research and descriptive, exploratory, and correlational research methodologies .
Descriptive vs. Causal Research
Descriptive research focuses on describing characteristics, behaviors, or phenomena without manipulating variables or establishing causal relationships. It provides a snapshot of the current state of affairs but does not attempt to explain why certain phenomena occur.
Causal research , on the other hand, seeks to identify cause-and-effect relationships between variables by systematically manipulating independent variables and observing their effects on dependent variables. Unlike descriptive research, causal research aims to determine whether changes in one variable directly cause changes in another variable.
Similarities:
- Both descriptive and causal research involve empirical observation and data collection.
- Both types of research contribute to the scientific understanding of phenomena, albeit through different approaches.
Differences:
- Descriptive research focuses on describing phenomena, while causal research aims to explain why phenomena occur by identifying causal relationships.
- Descriptive research typically uses observational methods, while causal research often involves experimental designs or causal inference techniques to establish causality.
Exploratory vs. Causal Research
Exploratory research aims to explore new topics, generate hypotheses, or gain initial insights into phenomena. It is often conducted when little is known about a subject and seeks to generate ideas for further investigation.
Causal research , on the other hand, is concerned with testing hypotheses and establishing cause-and-effect relationships between variables. It builds on existing knowledge and seeks to confirm or refute causal hypotheses through systematic investigation.
- Both exploratory and causal research contribute to the generation of knowledge and theory development.
- Both types of research involve systematic inquiry and data analysis to answer research questions.
- Exploratory research focuses on generating hypotheses and exploring new areas of inquiry, while causal research aims to test hypotheses and establish causal relationships.
- Exploratory research is more flexible and open-ended, while causal research follows a more structured and hypothesis-driven approach.
Correlational vs. Causal Research
Correlational research examines the relationship between variables without implying causation. It identifies patterns of association or co-occurrence between variables but does not establish the direction or causality of the relationship.
Causal research , on the other hand, seeks to establish cause-and-effect relationships between variables by systematically manipulating independent variables and observing their effects on dependent variables. It goes beyond mere association to determine whether changes in one variable directly cause changes in another variable.
- Both correlational and causal research involve analyzing relationships between variables.
- Both types of research contribute to understanding the nature of associations between variables.
- Correlational research focuses on identifying patterns of association, while causal research aims to establish causal relationships.
- Correlational research does not manipulate variables, while causal research involves systematically manipulating independent variables to observe their effects on dependent variables.
How to Formulate Causal Research Hypotheses?
Crafting research questions and hypotheses is the foundational step in any research endeavor. Defining your variables clearly and articulating the causal relationship you aim to investigate is essential. Let's explore this process further.
1. Identify Variables
Identifying variables involves recognizing the key factors you will manipulate or measure in your study. These variables can be classified into independent, dependent, and confounding variables.
- Independent Variable (IV): This is the variable you manipulate or control in your study. It is the presumed cause that you want to test.
- Dependent Variable (DV): The dependent variable is the outcome or response you measure. It is affected by changes in the independent variable.
- Confounding Variables: These are extraneous factors that may influence the relationship between the independent and dependent variables, leading to spurious correlations or erroneous causal inferences. Identifying and controlling for confounding variables is crucial for establishing valid causal relationships.
2. Establish Causality
Establishing causality requires meeting specific criteria outlined by scientific methodology. While correlation between variables may suggest a relationship, it does not imply causation. To establish causality, researchers must demonstrate the following:
- Temporal Precedence: The cause must precede the effect in time. In other words, changes in the independent variable must occur before changes in the dependent variable.
- Covariation of Cause and Effect: Changes in the independent variable should be accompanied by corresponding changes in the dependent variable. This demonstrates a consistent pattern of association between the two variables.
- Elimination of Alternative Explanations: Researchers must rule out other possible explanations for the observed relationship between variables. This involves controlling for confounding variables and conducting rigorous experimental designs to isolate the effects of the independent variable.
3. Write Clear and Testable Hypotheses
Hypotheses serve as tentative explanations for the relationship between variables and provide a framework for empirical testing. A well-formulated hypothesis should be:
- Specific: Clearly state the expected relationship between the independent and dependent variables.
- Testable: The hypothesis should be capable of being empirically tested through observation or experimentation.
- Falsifiable: There should be a possibility of proving the hypothesis false through empirical evidence.
For example, a hypothesis in a study examining the effect of exercise on weight loss could be: "Increasing levels of physical activity (IV) will lead to greater weight loss (DV) among participants (compared to those with lower levels of physical activity)."
By formulating clear hypotheses and operationalizing variables, researchers can systematically investigate causal relationships and contribute to the advancement of scientific knowledge.
Causal Research Design
Designing your research study involves making critical decisions about how you will collect and analyze data to investigate causal relationships.
Experimental vs. Observational Designs
One of the first decisions you'll make when designing a study is whether to employ an experimental or observational design. Each approach has its strengths and limitations, and the choice depends on factors such as the research question, feasibility , and ethical considerations.
- Experimental Design: In experimental designs, researchers manipulate the independent variable and observe its effects on the dependent variable while controlling for confounding variables. Random assignment to experimental conditions allows for causal inferences to be drawn. Example: A study testing the effectiveness of a new teaching method on student performance by randomly assigning students to either the experimental group (receiving the new teaching method) or the control group (receiving the traditional method).
- Observational Design: Observational designs involve observing and measuring variables without intervention. Researchers may still examine relationships between variables but cannot establish causality as definitively as in experimental designs. Example: A study observing the association between socioeconomic status and health outcomes by collecting data on income, education level, and health indicators from a sample of participants.
Control and Randomization
Control and randomization are crucial aspects of experimental design that help ensure the validity of causal inferences.
- Control: Controlling for extraneous variables involves holding constant factors that could influence the dependent variable, except for the independent variable under investigation. This helps isolate the effects of the independent variable. Example: In a medication trial, controlling for factors such as age, gender, and pre-existing health conditions ensures that any observed differences in outcomes can be attributed to the medication rather than other variables.
- Randomization: Random assignment of participants to experimental conditions helps distribute potential confounders evenly across groups, reducing the likelihood of systematic biases and allowing for causal conclusions. Example: Randomly assigning patients to treatment and control groups in a clinical trial ensures that both groups are comparable in terms of baseline characteristics, minimizing the influence of extraneous variables on treatment outcomes.
Internal and External Validity
Two key concepts in research design are internal validity and external validity, which relate to the credibility and generalizability of study findings, respectively.
- Internal Validity: Internal validity refers to the extent to which the observed effects can be attributed to the manipulation of the independent variable rather than confounding factors. Experimental designs typically have higher internal validity due to their control over extraneous variables. Example: A study examining the impact of a training program on employee productivity would have high internal validity if it could confidently attribute changes in productivity to the training intervention.
- External Validity: External validity concerns the extent to which study findings can be generalized to other populations, settings, or contexts. While experimental designs prioritize internal validity, they may sacrifice external validity by using highly controlled conditions that do not reflect real-world scenarios. Example: Findings from a laboratory study on memory retention may have limited external validity if the experimental tasks and conditions differ significantly from real-life learning environments.
Types of Experimental Designs
Several types of experimental designs are commonly used in causal research, each with its own strengths and applications.
- Randomized Control Trials (RCTs): RCTs are considered the gold standard for assessing causality in research. Participants are randomly assigned to experimental and control groups, allowing researchers to make causal inferences. Example: A pharmaceutical company testing a new drug's efficacy would use an RCT to compare outcomes between participants receiving the drug and those receiving a placebo.
- Quasi-Experimental Designs: Quasi-experimental designs lack random assignment but still attempt to establish causality by controlling for confounding variables through design or statistical analysis . Example: A study evaluating the effectiveness of a smoking cessation program might compare outcomes between participants who voluntarily enroll in the program and a matched control group of non-enrollees.
By carefully selecting an appropriate research design and addressing considerations such as control, randomization, and validity, researchers can conduct studies that yield credible evidence of causal relationships and contribute valuable insights to their field of inquiry.
Causal Research Data Collection
Collecting data is a critical step in any research study, and the quality of the data directly impacts the validity and reliability of your findings.
Choosing Measurement Instruments
Selecting appropriate measurement instruments is essential for accurately capturing the variables of interest in your study. The choice of measurement instrument depends on factors such as the nature of the variables, the target population , and the research objectives.
- Surveys : Surveys are commonly used to collect self-reported data on attitudes, opinions, behaviors, and demographics . They can be administered through various methods, including paper-and-pencil surveys, online surveys, and telephone interviews.
- Observations: Observational methods involve systematically recording behaviors, events, or phenomena as they occur in natural settings. Observations can be structured (following a predetermined checklist) or unstructured (allowing for flexible data collection).
- Psychological Tests: Psychological tests are standardized instruments designed to measure specific psychological constructs, such as intelligence, personality traits, or emotional functioning. These tests often have established reliability and validity.
- Physiological Measures: Physiological measures, such as heart rate, blood pressure, or brain activity, provide objective data on bodily processes. They are commonly used in health-related research but require specialized equipment and expertise.
- Existing Databases: Researchers may also utilize existing datasets, such as government surveys, public health records, or organizational databases, to answer research questions. Secondary data analysis can be cost-effective and time-saving but may be limited by the availability and quality of data.
Ensuring accurate data collection is the cornerstone of any successful research endeavor. With the right tools in place, you can unlock invaluable insights to drive your causal research forward. From surveys to tests, each instrument offers a unique lens through which to explore your variables of interest.
At Appinio , we understand the importance of robust data collection methods in informing impactful decisions. Let us empower your research journey with our intuitive platform, where you can effortlessly gather real-time consumer insights to fuel your next breakthrough. Ready to take your research to the next level? Book a demo today and see how Appinio can revolutionize your approach to data collection!
Book a Demo
Sampling Techniques
Sampling involves selecting a subset of individuals or units from a larger population to participate in the study. The goal of sampling is to obtain a representative sample that accurately reflects the characteristics of the population of interest.
- Probability Sampling: Probability sampling methods involve randomly selecting participants from the population, ensuring that each member of the population has an equal chance of being included in the sample. Common probability sampling techniques include simple random sampling , stratified sampling, and cluster sampling .
- Non-Probability Sampling: Non-probability sampling methods do not involve random selection and may introduce biases into the sample. Examples of non-probability sampling techniques include convenience sampling, purposive sampling, and snowball sampling.
The choice of sampling technique depends on factors such as the research objectives, population characteristics, resources available, and practical constraints. Researchers should strive to minimize sampling bias and maximize the representativeness of the sample to enhance the generalizability of their findings.
Ethical Considerations
Ethical considerations are paramount in research and involve ensuring the rights, dignity, and well-being of research participants. Researchers must adhere to ethical principles and guidelines established by professional associations and institutional review boards (IRBs).
- Informed Consent: Participants should be fully informed about the nature and purpose of the study, potential risks and benefits, their rights as participants, and any confidentiality measures in place. Informed consent should be obtained voluntarily and without coercion.
- Privacy and Confidentiality: Researchers should take steps to protect the privacy and confidentiality of participants' personal information. This may involve anonymizing data, securing data storage, and limiting access to identifiable information.
- Minimizing Harm: Researchers should mitigate any potential physical, psychological, or social harm to participants. This may involve conducting risk assessments, providing appropriate support services, and debriefing participants after the study.
- Respect for Participants: Researchers should respect participants' autonomy, diversity, and cultural values. They should seek to foster a trusting and respectful relationship with participants throughout the research process.
- Publication and Dissemination: Researchers have a responsibility to accurately report their findings and acknowledge contributions from participants and collaborators. They should adhere to principles of academic integrity and transparency in disseminating research results.
By addressing ethical considerations in research design and conduct, researchers can uphold the integrity of their work, maintain trust with participants and the broader community, and contribute to the responsible advancement of knowledge in their field.
Causal Research Data Analysis
Once data is collected, it must be analyzed to draw meaningful conclusions and assess causal relationships.
Causal Inference Methods
Causal inference methods are statistical techniques used to identify and quantify causal relationships between variables in observational data. While experimental designs provide the most robust evidence for causality, observational studies often require more sophisticated methods to account for confounding factors.
- Difference-in-Differences (DiD): DiD compares changes in outcomes before and after an intervention between a treatment group and a control group, controlling for pre-existing trends. It estimates the average treatment effect by differencing the changes in outcomes between the two groups over time.
- Instrumental Variables (IV): IV analysis relies on instrumental variables—variables that affect the treatment variable but not the outcome—to estimate causal effects in the presence of endogeneity. IVs should be correlated with the treatment but uncorrelated with the error term in the outcome equation.
- Regression Discontinuity (RD): RD designs exploit naturally occurring thresholds or cutoff points to estimate causal effects near the threshold. Participants just above and below the threshold are compared, assuming that they are similar except for their proximity to the threshold.
- Propensity Score Matching (PSM): PSM matches individuals or units based on their propensity scores—the likelihood of receiving the treatment—creating comparable groups with similar observed characteristics. Matching reduces selection bias and allows for causal inference in observational studies.
Assessing Causality Strength
Assessing the strength of causality involves determining the magnitude and direction of causal effects between variables. While statistical significance indicates whether an observed relationship is unlikely to occur by chance, it does not necessarily imply a strong or meaningful effect.
- Effect Size: Effect size measures the magnitude of the relationship between variables, providing information about the practical significance of the results. Standard effect size measures include Cohen's d for mean differences and odds ratios for categorical outcomes.
- Confidence Intervals: Confidence intervals provide a range of values within which the actual effect size is likely to lie with a certain degree of certainty. Narrow confidence intervals indicate greater precision in estimating the true effect size.
- Practical Significance: Practical significance considers whether the observed effect is meaningful or relevant in real-world terms. Researchers should interpret results in the context of their field and the implications for stakeholders.
Handling Confounding Variables
Confounding variables are extraneous factors that may distort the observed relationship between the independent and dependent variables, leading to spurious or biased conclusions. Addressing confounding variables is essential for establishing valid causal inferences.
- Statistical Control: Statistical control involves including confounding variables as covariates in regression models to partially out their effects on the outcome variable. Controlling for confounders reduces bias and strengthens the validity of causal inferences.
- Matching: Matching participants or units based on observed characteristics helps create comparable groups with similar distributions of confounding variables. Matching reduces selection bias and mimics the randomization process in experimental designs.
- Sensitivity Analysis: Sensitivity analysis assesses the robustness of study findings to changes in model specifications or assumptions. By varying analytical choices and examining their impact on results, researchers can identify potential sources of bias and evaluate the stability of causal estimates.
- Subgroup Analysis: Subgroup analysis explores whether the relationship between variables differs across subgroups defined by specific characteristics. Identifying effect modifiers helps understand the conditions under which causal effects may vary.
By employing rigorous causal inference methods, assessing the strength of causality, and addressing confounding variables, researchers can confidently draw valid conclusions about causal relationships in their studies, advancing scientific knowledge and informing evidence-based decision-making.
Causal Research Examples
Examples play a crucial role in understanding the application of causal research methods and their impact across various domains. Let's explore some detailed examples to illustrate how causal research is conducted and its real-world implications:
Example 1: Software as a Service (SaaS) User Retention Analysis
Suppose a SaaS company wants to understand the factors influencing user retention and engagement with their platform. The company conducts a longitudinal observational study, collecting data on user interactions, feature usage, and demographic information over several months.
- Design: The company employs an observational cohort study design, tracking cohorts of users over time to observe changes in retention and engagement metrics. They use analytics tools to collect data on user behavior , such as logins, feature usage, session duration, and customer support interactions.
- Data Collection: Data is collected from the company's platform logs, customer relationship management (CRM) system, and user surveys. Key metrics include user churn rates, active user counts, feature adoption rates, and Net Promoter Scores ( NPS ).
- Analysis: Using statistical techniques like survival analysis and regression modeling, the company identifies factors associated with user retention, such as feature usage patterns, onboarding experiences, customer support interactions, and subscription plan types.
- Findings: The analysis reveals that users who engage with specific features early in their lifecycle have higher retention rates, while those who encounter usability issues or lack personalized onboarding experiences are more likely to churn. The company uses these insights to optimize product features, improve onboarding processes, and enhance customer support strategies to increase user retention and satisfaction.
Example 2: Business Impact of Digital Marketing Campaign
Consider a technology startup launching a digital marketing campaign to promote its new product offering. The company conducts an experimental study to evaluate the effectiveness of different marketing channels in driving website traffic, lead generation, and sales conversions.
- Design: The company implements an A/B testing design, randomly assigning website visitors to different marketing treatment conditions, such as Google Ads, social media ads, email campaigns, or content marketing efforts. They track user interactions and conversion events using web analytics tools and marketing automation platforms.
- Data Collection: Data is collected on website traffic, click-through rates, conversion rates, lead generation, and sales revenue. The company also gathers demographic information and user feedback through surveys and customer interviews to understand the impact of marketing messages and campaign creatives .
- Analysis: Utilizing statistical methods like hypothesis testing and multivariate analysis, the company compares key performance metrics across different marketing channels to assess their effectiveness in driving user engagement and conversion outcomes. They calculate return on investment (ROI) metrics to evaluate the cost-effectiveness of each marketing channel.
- Findings: The analysis reveals that social media ads outperform other marketing channels in generating website traffic and lead conversions, while email campaigns are more effective in nurturing leads and driving sales conversions. Armed with these insights, the company allocates marketing budgets strategically, focusing on channels that yield the highest ROI and adjusting messaging and targeting strategies to optimize campaign performance.
These examples demonstrate the diverse applications of causal research methods in addressing important questions, informing policy decisions, and improving outcomes in various fields. By carefully designing studies, collecting relevant data, employing appropriate analysis techniques, and interpreting findings rigorously, researchers can generate valuable insights into causal relationships and contribute to positive social change.
How to Interpret Causal Research Results?
Interpreting and reporting research findings is a crucial step in the scientific process, ensuring that results are accurately communicated and understood by stakeholders.
Interpreting Statistical Significance
Statistical significance indicates whether the observed results are unlikely to occur by chance alone, but it does not necessarily imply practical or substantive importance. Interpreting statistical significance involves understanding the meaning of p-values and confidence intervals and considering their implications for the research findings.
- P-values: A p-value represents the probability of obtaining the observed results (or more extreme results) if the null hypothesis is true. A p-value below a predetermined threshold (typically 0.05) suggests that the observed results are statistically significant, indicating that the null hypothesis can be rejected in favor of the alternative hypothesis.
- Confidence Intervals: Confidence intervals provide a range of values within which the true population parameter is likely to lie with a certain degree of confidence (e.g., 95%). If the confidence interval does not include the null value, it suggests that the observed effect is statistically significant at the specified confidence level.
Interpreting statistical significance requires considering factors such as sample size, effect size, and the practical relevance of the results rather than relying solely on p-values to draw conclusions.
Discussing Practical Significance
While statistical significance indicates whether an effect exists, practical significance evaluates the magnitude and meaningfulness of the effect in real-world terms. Discussing practical significance involves considering the relevance of the results to stakeholders and assessing their impact on decision-making and practice.
- Effect Size: Effect size measures the magnitude of the observed effect, providing information about its practical importance. Researchers should interpret effect sizes in the context of their field and the scale of measurement (e.g., small, medium, or large effect sizes).
- Contextual Relevance: Consider the implications of the results for stakeholders, policymakers, and practitioners. Are the observed effects meaningful in the context of existing knowledge, theory, or practical applications? How do the findings contribute to addressing real-world problems or informing decision-making?
Discussing practical significance helps contextualize research findings and guide their interpretation and application in practice, beyond statistical significance alone.
Addressing Limitations and Assumptions
No study is without limitations, and researchers should transparently acknowledge and address potential biases, constraints, and uncertainties in their research design and findings.
- Methodological Limitations: Identify any limitations in study design, data collection, or analysis that may affect the validity or generalizability of the results. For example, sampling biases , measurement errors, or confounding variables.
- Assumptions: Discuss any assumptions made in the research process and their implications for the interpretation of results. Assumptions may relate to statistical models, causal inference methods, or theoretical frameworks underlying the study.
- Alternative Explanations: Consider alternative explanations for the observed results and discuss their potential impact on the validity of causal inferences. How robust are the findings to different interpretations or competing hypotheses?
Addressing limitations and assumptions demonstrates transparency and rigor in the research process, allowing readers to critically evaluate the validity and reliability of the findings.
Communicating Findings Clearly
Effectively communicating research findings is essential for disseminating knowledge, informing decision-making, and fostering collaboration and dialogue within the scientific community.
- Clarity and Accessibility: Present findings in a clear, concise, and accessible manner, using plain language and avoiding jargon or technical terminology. Organize information logically and use visual aids (e.g., tables, charts, graphs) to enhance understanding.
- Contextualization: Provide context for the results by summarizing key findings, highlighting their significance, and relating them to existing literature or theoretical frameworks. Discuss the implications of the findings for theory, practice, and future research directions.
- Transparency: Be transparent about the research process, including data collection procedures, analytical methods, and any limitations or uncertainties associated with the findings. Clearly state any conflicts of interest or funding sources that may influence interpretation.
By communicating findings clearly and transparently, researchers can facilitate knowledge exchange, foster trust and credibility, and contribute to evidence-based decision-making.
Causal Research Tips
When conducting causal research, it's essential to approach your study with careful planning, attention to detail, and methodological rigor. Here are some tips to help you navigate the complexities of causal research effectively:
- Define Clear Research Questions: Start by clearly defining your research questions and hypotheses. Articulate the causal relationship you aim to investigate and identify the variables involved.
- Consider Alternative Explanations: Be mindful of potential confounding variables and alternative explanations for the observed relationships. Take steps to control for confounders and address alternative hypotheses in your analysis.
- Prioritize Internal Validity: While external validity is important for generalizability, prioritize internal validity in your study design to ensure that observed effects can be attributed to the manipulation of the independent variable.
- Use Randomization When Possible: If feasible, employ randomization in experimental designs to distribute potential confounders evenly across experimental conditions and enhance the validity of causal inferences.
- Be Transparent About Methods: Provide detailed descriptions of your research methods, including data collection procedures, analytical techniques, and any assumptions or limitations associated with your study.
- Utilize Multiple Methods: Consider using a combination of experimental and observational methods to triangulate findings and strengthen the validity of causal inferences.
- Be Mindful of Sample Size: Ensure that your sample size is adequate to detect meaningful effects and minimize the risk of Type I and Type II errors. Conduct power analyses to determine the sample size needed to achieve sufficient statistical power.
- Validate Measurement Instruments: Validate your measurement instruments to ensure that they are reliable and valid for assessing the variables of interest in your study. Pilot test your instruments if necessary.
- Seek Feedback from Peers: Collaborate with colleagues or seek feedback from peer reviewers to solicit constructive criticism and improve the quality of your research design and analysis.
Conclusion for Causal Research
Mastering causal research empowers researchers to unlock the secrets of cause and effect, shedding light on the intricate relationships between variables in diverse fields. By employing rigorous methods such as experimental designs, causal inference techniques, and careful data analysis, you can uncover causal mechanisms, predict outcomes, and inform evidence-based practices. Through the lens of causal research, complex phenomena become more understandable, and interventions become more effective in addressing societal challenges and driving progress. In a world where understanding the reasons behind events is paramount, causal research serves as a beacon of clarity and insight. Armed with the knowledge and techniques outlined in this guide, you can navigate the complexities of causality with confidence, advancing scientific knowledge, guiding policy decisions, and ultimately making meaningful contributions to our understanding of the world.
How to Conduct Causal Research in Minutes?
Introducing Appinio , your gateway to lightning-fast causal research. As a real-time market research platform, we're revolutionizing how companies gain consumer insights to drive data-driven decisions. With Appinio, conducting your own market research is not only easy but also thrilling. Experience the excitement of market research with Appinio, where fast, intuitive, and impactful insights are just a click away.
Here's why you'll love Appinio:
- Instant Insights: Say goodbye to waiting days for research results. With our platform, you'll go from questions to insights in minutes, empowering you to make decisions at the speed of business.
- User-Friendly Interface: No need for a research degree here! Our intuitive platform is designed for anyone to use, making complex research tasks simple and accessible.
- Global Reach: Reach your target audience wherever they are. With access to over 90 countries and the ability to define precise target groups from 1200+ characteristics, you'll gather comprehensive data to inform your decisions.
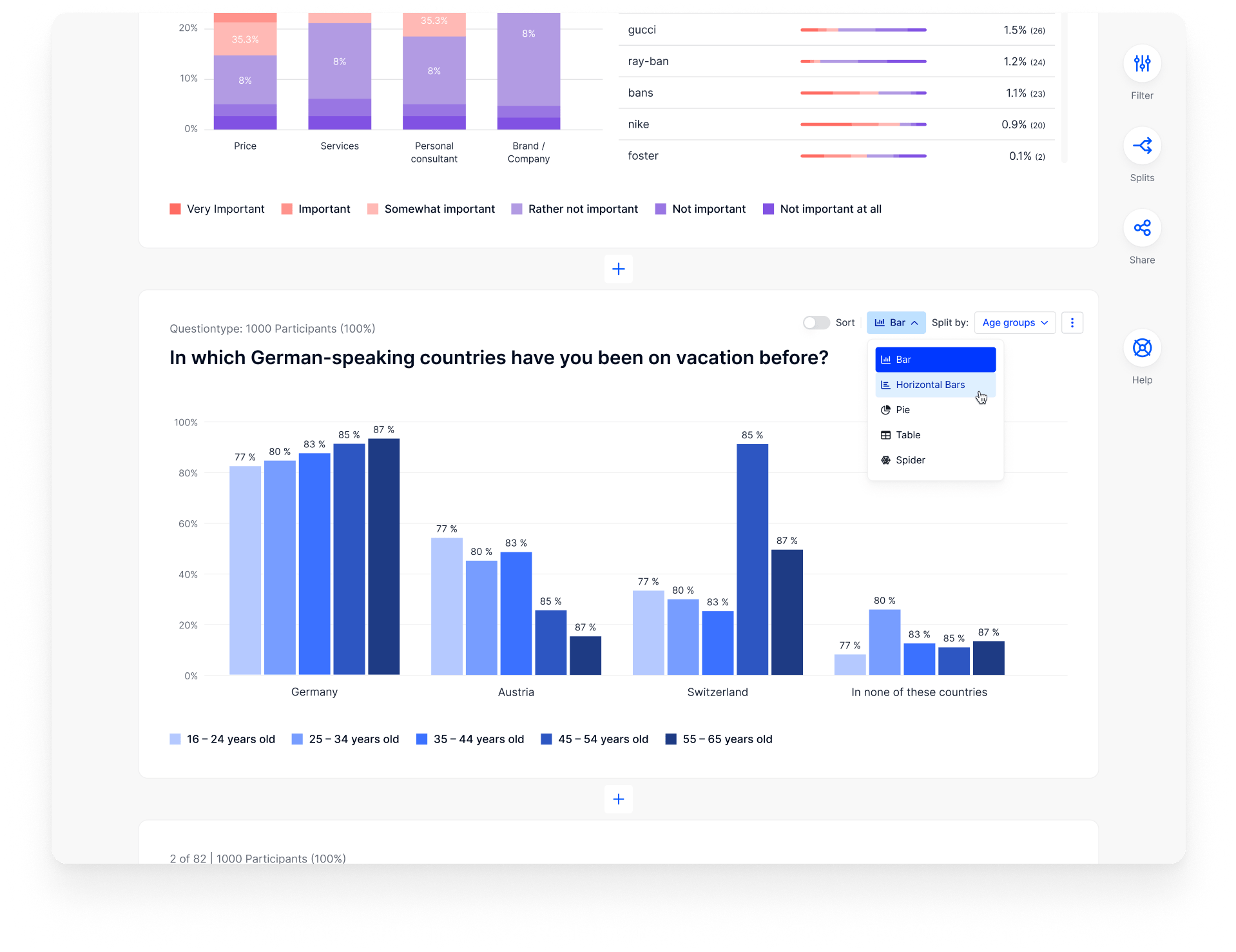
Get free access to the platform!
Join the loop 💌
Be the first to hear about new updates, product news, and data insights. We'll send it all straight to your inbox.
Get the latest market research news straight to your inbox! 💌
Wait, there's more
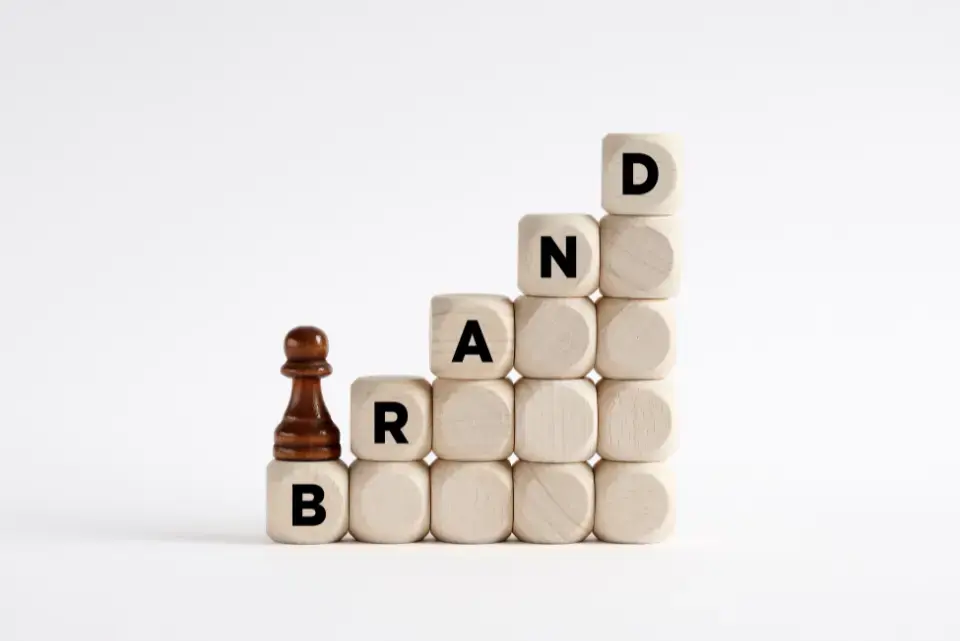
26.06.2024 | 35min read
Brand Development: Definition, Process, Strategies, Examples
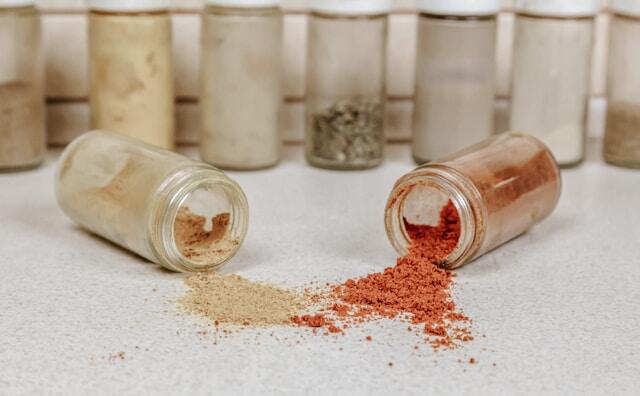
18.06.2024 | 7min read
Future Flavors: How Burger King nailed Concept Testing with Appinio's Predictive Insights
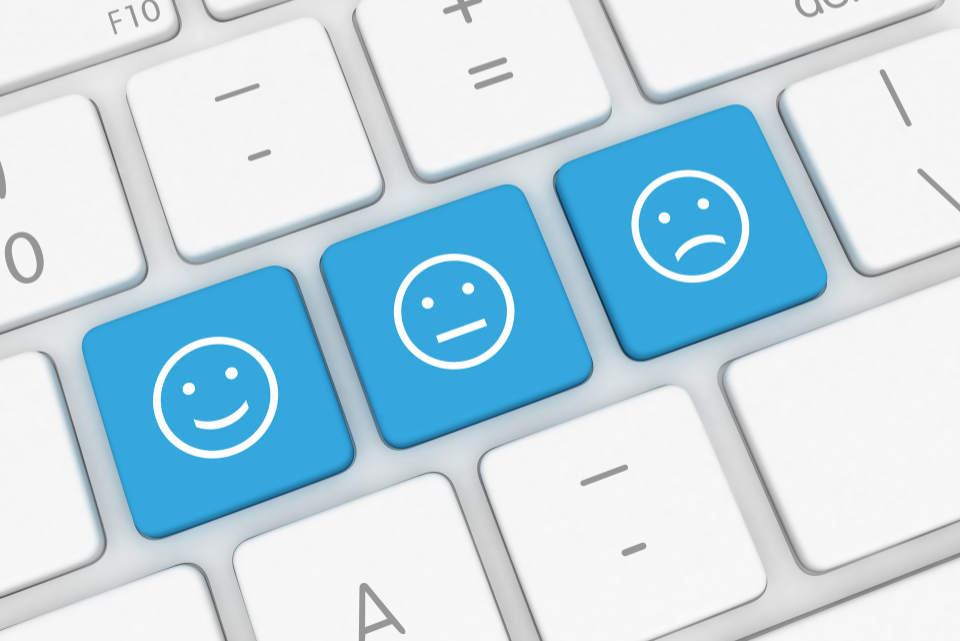
18.06.2024 | 32min read
What is a Pulse Survey? Definition, Types, Questions
Root out friction in every digital experience, super-charge conversion rates, and optimize digital self-service
Uncover insights from any interaction, deliver AI-powered agent coaching, and reduce cost to serve
Increase revenue and loyalty with real-time insights and recommendations delivered to teams on the ground
Know how your people feel and empower managers to improve employee engagement, productivity, and retention
Take action in the moments that matter most along the employee journey and drive bottom line growth
Whatever they’re are saying, wherever they’re saying it, know exactly what’s going on with your people
Get faster, richer insights with qual and quant tools that make powerful market research available to everyone
Run concept tests, pricing studies, prototyping + more with fast, powerful studies designed by UX research experts
Track your brand performance 24/7 and act quickly to respond to opportunities and challenges in your market
Explore the platform powering Experience Management
- Free Account
- Product Demos
- For Digital
- For Customer Care
- For Human Resources
- For Researchers
- Financial Services
- All Industries
Popular Use Cases
- Customer Experience
- Employee Experience
- Net Promoter Score
- Voice of Customer
- Customer Success Hub
- Product Documentation
- Training & Certification
- XM Institute
- Popular Resources
- Customer Stories
- Artificial Intelligence
Market Research
- Partnerships
- Marketplace
The annual gathering of the experience leaders at the world’s iconic brands building breakthrough business results, live in Salt Lake City.
- English/AU & NZ
- Español/Europa
- Español/América Latina
- Português Brasileiro
- REQUEST DEMO
- Experience Management
- Causal Research
Try Qualtrics for free
Causal research: definition, examples and how to use it.
16 min read Causal research enables market researchers to predict hypothetical occurrences & outcomes while improving existing strategies. Discover how this research can decrease employee retention & increase customer success for your business.
What is causal research?
Causal research, also known as explanatory research or causal-comparative research, identifies the extent and nature of cause-and-effect relationships between two or more variables.
It’s often used by companies to determine the impact of changes in products, features, or services process on critical company metrics. Some examples:
- How does rebranding of a product influence intent to purchase?
- How would expansion to a new market segment affect projected sales?
- What would be the impact of a price increase or decrease on customer loyalty?
To maintain the accuracy of causal research, ‘confounding variables’ or influences — e.g. those that could distort the results — are controlled. This is done either by keeping them constant in the creation of data, or by using statistical methods. These variables are identified before the start of the research experiment.
As well as the above, research teams will outline several other variables and principles in causal research:
- Independent variables
The variables that may cause direct changes in another variable. For example, the effect of truancy on a student’s grade point average. The independent variable is therefore class attendance.
- Control variables
These are the components that remain unchanged during the experiment so researchers can better understand what conditions create a cause-and-effect relationship.
This describes the cause-and-effect relationship. When researchers find causation (or the cause), they’ve conducted all the processes necessary to prove it exists.
- Correlation
Any relationship between two variables in the experiment. It’s important to note that correlation doesn’t automatically mean causation. Researchers will typically establish correlation before proving cause-and-effect.
- Experimental design
Researchers use experimental design to define the parameters of the experiment — e.g. categorizing participants into different groups.
- Dependent variables
These are measurable variables that may change or are influenced by the independent variable. For example, in an experiment about whether or not terrain influences running speed, your dependent variable is the terrain.
Why is causal research useful?
It’s useful because it enables market researchers to predict hypothetical occurrences and outcomes while improving existing strategies. This allows businesses to create plans that benefit the company. It’s also a great research method because researchers can immediately see how variables affect each other and under what circumstances.
Also, once the first experiment has been completed, researchers can use the learnings from the analysis to repeat the experiment or apply the findings to other scenarios. Because of this, it’s widely used to help understand the impact of changes in internal or commercial strategy to the business bottom line.
Some examples include:
- Understanding how overall training levels are improved by introducing new courses
- Examining which variations in wording make potential customers more interested in buying a product
- Testing a market’s response to a brand-new line of products and/or services
So, how does causal research compare and differ from other research types?
Well, there are a few research types that are used to find answers to some of the examples above:
1. Exploratory research
As its name suggests, exploratory research involves assessing a situation (or situations) where the problem isn’t clear. Through this approach, researchers can test different avenues and ideas to establish facts and gain a better understanding.
Researchers can also use it to first navigate a topic and identify which variables are important. Because no area is off-limits, the research is flexible and adapts to the investigations as it progresses.
Finally, this approach is unstructured and often involves gathering qualitative data, giving the researcher freedom to progress the research according to their thoughts and assessment. However, this may make results susceptible to researcher bias and may limit the extent to which a topic is explored.
2. Descriptive research
Descriptive research is all about describing the characteristics of the population, phenomenon or scenario studied. It focuses more on the “what” of the research subject than the “why”.
For example, a clothing brand wants to understand the fashion purchasing trends amongst buyers in California — so they conduct a demographic survey of the region, gather population data and then run descriptive research. The study will help them to uncover purchasing patterns amongst fashion buyers in California, but not necessarily why those patterns exist.
As the research happens in a natural setting, variables can cross-contaminate other variables, making it harder to isolate cause and effect relationships. Therefore, further research will be required if more causal information is needed.
Get started on your market research journey with Strategic Research
How is causal research different from the other two methods above?
Well, causal research looks at what variables are involved in a problem and ‘why’ they act a certain way. As the experiment takes place in a controlled setting (thanks to controlled variables) it’s easier to identify cause-and-effect amongst variables.
Furthermore, researchers can carry out causal research at any stage in the process, though it’s usually carried out in the later stages once more is known about a particular topic or situation.
Finally, compared to the other two methods, causal research is more structured, and researchers can combine it with exploratory and descriptive research to assist with research goals.
Summary of three research types
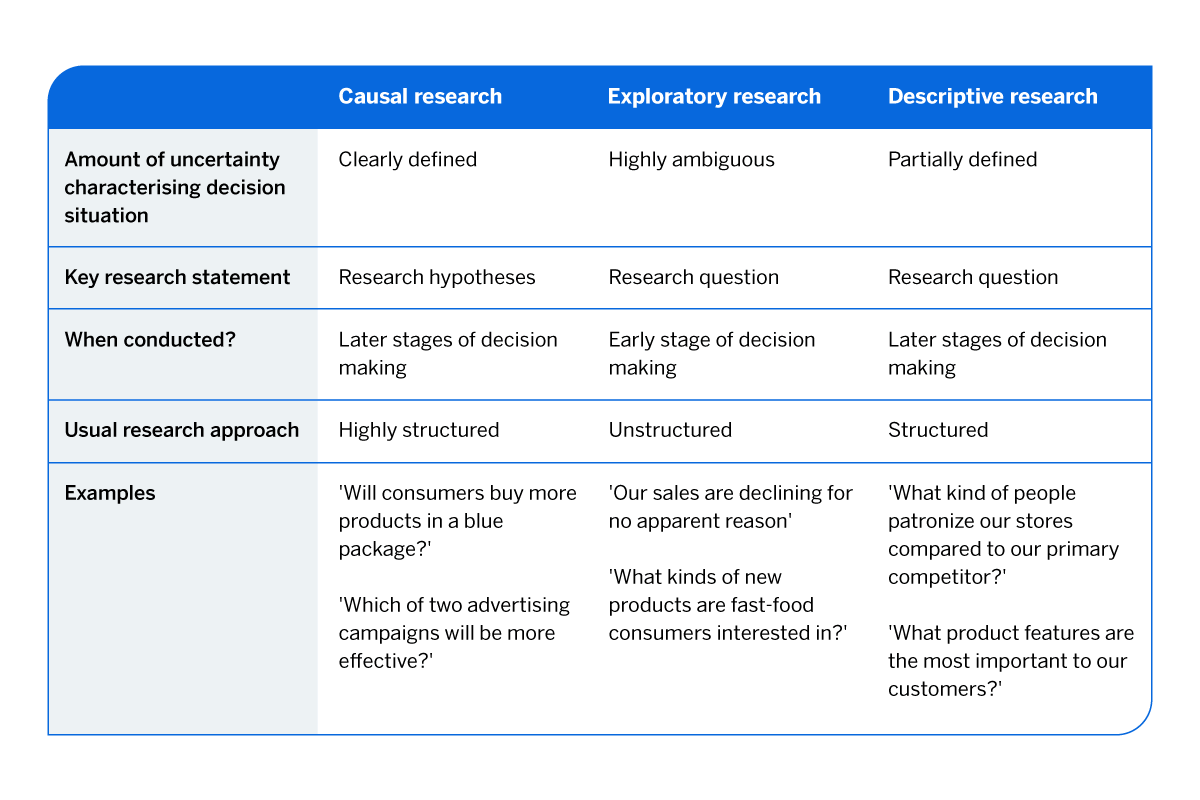
What are the advantages of causal research?
- Improve experiences
By understanding which variables have positive impacts on target variables (like sales revenue or customer loyalty), businesses can improve their processes, return on investment, and the experiences they offer customers and employees.
- Help companies improve internally
By conducting causal research, management can make informed decisions about improving their employee experience and internal operations. For example, understanding which variables led to an increase in staff turnover.
- Repeat experiments to enhance reliability and accuracy of results
When variables are identified, researchers can replicate cause-and-effect with ease, providing them with reliable data and results to draw insights from.
- Test out new theories or ideas
If causal research is able to pinpoint the exact outcome of mixing together different variables, research teams have the ability to test out ideas in the same way to create viable proof of concepts.
- Fix issues quickly
Once an undesirable effect’s cause is identified, researchers and management can take action to reduce the impact of it or remove it entirely, resulting in better outcomes.
What are the disadvantages of causal research?
- Provides information to competitors
If you plan to publish your research, it provides information about your plans to your competitors. For example, they might use your research outcomes to identify what you are up to and enter the market before you.
- Difficult to administer
Causal research is often difficult to administer because it’s not possible to control the effects of extraneous variables.
- Time and money constraints
Budgetary and time constraints can make this type of research expensive to conduct and repeat. Also, if an initial attempt doesn’t provide a cause and effect relationship, the ROI is wasted and could impact the appetite for future repeat experiments.
- Requires additional research to ensure validity
You can’t rely on just the outcomes of causal research as it’s inaccurate. It’s best to conduct other types of research alongside it to confirm its output.
- Trouble establishing cause and effect
Researchers might identify that two variables are connected, but struggle to determine which is the cause and which variable is the effect.
- Risk of contamination
There’s always the risk that people outside your market or area of study could affect the results of your research. For example, if you’re conducting a retail store study, shoppers outside your ‘test parameters’ shop at your store and skew the results.
How can you use causal research effectively?
To better highlight how you can use causal research across functions or markets, here are a few examples:
Market and advertising research
A company might want to know if their new advertising campaign or marketing campaign is having a positive impact. So, their research team can carry out a causal research project to see which variables cause a positive or negative effect on the campaign.
For example, a cold-weather apparel company in a winter ski-resort town may see an increase in sales generated after a targeted campaign to skiers. To see if one caused the other, the research team could set up a duplicate experiment to see if the same campaign would generate sales from non-skiers. If the results reduce or change, then it’s likely that the campaign had a direct effect on skiers to encourage them to purchase products.
Improving customer experiences and loyalty levels
Customers enjoy shopping with brands that align with their own values, and they’re more likely to buy and present the brand positively to other potential shoppers as a result. So, it’s in your best interest to deliver great experiences and retain your customers.
For example, the Harvard Business Review found that an increase in customer retention rates by 5% increased profits by 25% to 95%. But let’s say you want to increase your own, how can you identify which variables contribute to it?Using causal research, you can test hypotheses about which processes, strategies or changes influence customer retention. For example, is it the streamlined checkout? What about the personalized product suggestions? Or maybe it was a new solution that solved their problem? Causal research will help you find out.
Improving problematic employee turnover rates
If your company has a high attrition rate, causal research can help you narrow down the variables or reasons which have the greatest impact on people leaving. This allows you to prioritize your efforts on tackling the issues in the right order, for the best positive outcomes.
For example, through causal research, you might find that employee dissatisfaction due to a lack of communication and transparency from upper management leads to poor morale, which in turn influences employee retention.
To rectify the problem, you could implement a routine feedback loop or session that enables your people to talk to your company’s C-level executives so that they feel heard and understood.
How to conduct causal research first steps to getting started are:
1. Define the purpose of your research
What questions do you have? What do you expect to come out of your research? Think about which variables you need to test out the theory.
2. Pick a random sampling if participants are needed
Using a technology solution to support your sampling, like a database, can help you define who you want your target audience to be, and how random or representative they should be.
3. Set up the controlled experiment
Once you’ve defined which variables you’d like to measure to see if they interact, think about how best to set up the experiment. This could be in-person or in-house via interviews, or it could be done remotely using online surveys.
4. Carry out the experiment
Make sure to keep all irrelevant variables the same, and only change the causal variable (the one that causes the effect) to gather the correct data. Depending on your method, you could be collecting qualitative or quantitative data, so make sure you note your findings across each regularly.
5. Analyze your findings
Either manually or using technology, analyze your data to see if any trends, patterns or correlations emerge. By looking at the data, you’ll be able to see what changes you might need to do next time, or if there are questions that require further research.
6. Verify your findings
Your first attempt gives you the baseline figures to compare the new results to. You can then run another experiment to verify your findings.
7. Do follow-up or supplemental research
You can supplement your original findings by carrying out research that goes deeper into causes or explores the topic in more detail. One of the best ways to do this is to use a survey. See ‘Use surveys to help your experiment’.
Identifying causal relationships between variables
To verify if a causal relationship exists, you have to satisfy the following criteria:
- Nonspurious association
A clear correlation exists between one cause and the effect. In other words, no ‘third’ that relates to both (cause and effect) should exist.
- Temporal sequence
The cause occurs before the effect. For example, increased ad spend on product marketing would contribute to higher product sales.
- Concomitant variation
The variation between the two variables is systematic. For example, if a company doesn’t change its IT policies and technology stack, then changes in employee productivity were not caused by IT policies or technology.
How surveys help your causal research experiments?
There are some surveys that are perfect for assisting researchers with understanding cause and effect. These include:
- Employee Satisfaction Survey – An introductory employee satisfaction survey that provides you with an overview of your current employee experience.
- Manager Feedback Survey – An introductory manager feedback survey geared toward improving your skills as a leader with valuable feedback from your team.
- Net Promoter Score (NPS) Survey – Measure customer loyalty and understand how your customers feel about your product or service using one of the world’s best-recognized metrics.
- Employee Engagement Survey – An entry-level employee engagement survey that provides you with an overview of your current employee experience.
- Customer Satisfaction Survey – Evaluate how satisfied your customers are with your company, including the products and services you provide and how they are treated when they buy from you.
- Employee Exit Interview Survey – Understand why your employees are leaving and how they’ll speak about your company once they’re gone.
- Product Research Survey – Evaluate your consumers’ reaction to a new product or product feature across every stage of the product development journey.
- Brand Awareness Survey – Track the level of brand awareness in your target market, including current and potential future customers.
- Online Purchase Feedback Survey – Find out how well your online shopping experience performs against customer needs and expectations.
That covers the fundamentals of causal research and should give you a foundation for ongoing studies to assess opportunities, problems, and risks across your market, product, customer, and employee segments.
If you want to transform your research, empower your teams and get insights on tap to get ahead of the competition, maybe it’s time to leverage Qualtrics CoreXM.
Qualtrics CoreXM provides a single platform for data collection and analysis across every part of your business — from customer feedback to product concept testing. What’s more, you can integrate it with your existing tools and services thanks to a flexible API.
Qualtrics CoreXM offers you as much or as little power and complexity as you need, so whether you’re running simple surveys or more advanced forms of research, it can deliver every time.
Get started on your market research journey with CoreXM
Related resources
Market intelligence 10 min read, marketing insights 11 min read, ethnographic research 11 min read, qualitative vs quantitative research 13 min read, qualitative research questions 11 min read, qualitative research design 12 min read, primary vs secondary research 14 min read, request demo.
Ready to learn more about Qualtrics?
What is causal research design?
Last updated
14 May 2023
Reviewed by
Short on time? Get an AI generated summary of this article instead
Examining these relationships gives researchers valuable insights into the mechanisms that drive the phenomena they are investigating.
Organizations primarily use causal research design to identify, determine, and explore the impact of changes within an organization and the market. You can use a causal research design to evaluate the effects of certain changes on existing procedures, norms, and more.
This article explores causal research design, including its elements, advantages, and disadvantages.
Analyze your causal research
Dovetail streamlines causal research analysis to help you uncover and share actionable insights
- Components of causal research
You can demonstrate the existence of cause-and-effect relationships between two factors or variables using specific causal information, allowing you to produce more meaningful results and research implications.
These are the key inputs for causal research:
The timeline of events
Ideally, the cause must occur before the effect. You should review the timeline of two or more separate events to determine the independent variables (cause) from the dependent variables (effect) before developing a hypothesis.
If the cause occurs before the effect, you can link cause and effect and develop a hypothesis .
For instance, an organization may notice a sales increase. Determining the cause would help them reproduce these results.
Upon review, the business realizes that the sales boost occurred right after an advertising campaign. The business can leverage this time-based data to determine whether the advertising campaign is the independent variable that caused a change in sales.
Evaluation of confounding variables
In most cases, you need to pinpoint the variables that comprise a cause-and-effect relationship when using a causal research design. This uncovers a more accurate conclusion.
Co-variations between a cause and effect must be accurate, and a third factor shouldn’t relate to cause and effect.
Observing changes
Variation links between two variables must be clear. A quantitative change in effect must happen solely due to a quantitative change in the cause.
You can test whether the independent variable changes the dependent variable to evaluate the validity of a cause-and-effect relationship. A steady change between the two variables must occur to back up your hypothesis of a genuine causal effect.
- Why is causal research useful?
Causal research allows market researchers to predict hypothetical occurrences and outcomes while enhancing existing strategies. Organizations can use this concept to develop beneficial plans.
Causal research is also useful as market researchers can immediately deduce the effect of the variables on each other under real-world conditions.
Once researchers complete their first experiment, they can use their findings. Applying them to alternative scenarios or repeating the experiment to confirm its validity can produce further insights.
Businesses widely use causal research to identify and comprehend the effect of strategic changes on their profits.
- How does causal research compare and differ from other research types?
Other research types that identify relationships between variables include exploratory and descriptive research .
Here’s how they compare and differ from causal research designs:
Exploratory research
An exploratory research design evaluates situations where a problem or opportunity's boundaries are unclear. You can use this research type to test various hypotheses and assumptions to establish facts and understand a situation more clearly.
You can also use exploratory research design to navigate a topic and discover the relevant variables. This research type allows flexibility and adaptability as the experiment progresses, particularly since no area is off-limits.
It’s worth noting that exploratory research is unstructured and typically involves collecting qualitative data . This provides the freedom to tweak and amend the research approach according to your ongoing thoughts and assessments.
Unfortunately, this exposes the findings to the risk of bias and may limit the extent to which a researcher can explore a topic.
This table compares the key characteristics of causal and exploratory research:
|
|
|
Main research statement | Research hypotheses | Research question |
Amount of uncertainty characterizing decision situation | Clearly defined | Highly ambiguous |
Research approach | Highly structured | Unstructured |
When you conduct it | Later stages of decision-making | Early stages of decision-making |
Descriptive research
This research design involves capturing and describing the traits of a population, situation, or phenomenon. Descriptive research focuses more on the " what " of the research subject and less on the " why ."
Since descriptive research typically happens in a real-world setting, variables can cross-contaminate others. This increases the challenge of isolating cause-and-effect relationships.
You may require further research if you need more causal links.
This table compares the key characteristics of causal and descriptive research.
|
|
|
Main research statement | Research hypotheses | Research question |
Amount of uncertainty characterizing decision situation | Clearly defined | Partially defined |
Research approach | Highly structured | Structured |
When you conduct it | Later stages of decision-making | Later stages of decision-making |
Causal research examines a research question’s variables and how they interact. It’s easier to pinpoint cause and effect since the experiment often happens in a controlled setting.
Researchers can conduct causal research at any stage, but they typically use it once they know more about the topic.
In contrast, causal research tends to be more structured and can be combined with exploratory and descriptive research to help you attain your research goals.
- How can you use causal research effectively?
Here are common ways that market researchers leverage causal research effectively:
Market and advertising research
Do you want to know if your new marketing campaign is affecting your organization positively? You can use causal research to determine the variables causing negative or positive impacts on your campaign.
Improving customer experiences and loyalty levels
Consumers generally enjoy purchasing from brands aligned with their values. They’re more likely to purchase from such brands and positively represent them to others.
You can use causal research to identify the variables contributing to increased or reduced customer acquisition and retention rates.
Could the cause of increased customer retention rates be streamlined checkout?
Perhaps you introduced a new solution geared towards directly solving their immediate problem.
Whatever the reason, causal research can help you identify the cause-and-effect relationship. You can use this to enhance your customer experiences and loyalty levels.
Improving problematic employee turnover rates
Is your organization experiencing skyrocketing attrition rates?
You can leverage the features and benefits of causal research to narrow down the possible explanations or variables with significant effects on employees quitting.
This way, you can prioritize interventions, focusing on the highest priority causal influences, and begin to tackle high employee turnover rates.
- Advantages of causal research
The main benefits of causal research include the following:
Effectively test new ideas
If causal research can pinpoint the precise outcome through combinations of different variables, researchers can test ideas in the same manner to form viable proof of concepts.
Achieve more objective results
Market researchers typically use random sampling techniques to choose experiment participants or subjects in causal research. This reduces the possibility of exterior, sample, or demography-based influences, generating more objective results.
Improved business processes
Causal research helps businesses understand which variables positively impact target variables, such as customer loyalty or sales revenues. This helps them improve their processes, ROI, and customer and employee experiences.
Guarantee reliable and accurate results
Upon identifying the correct variables, researchers can replicate cause and effect effortlessly. This creates reliable data and results to draw insights from.
Internal organization improvements
Businesses that conduct causal research can make informed decisions about improving their internal operations and enhancing employee experiences.
- Disadvantages of causal research
Like any other research method, casual research has its set of drawbacks that include:
Extra research to ensure validity
Researchers can't simply rely on the outcomes of causal research since it isn't always accurate. There may be a need to conduct other research types alongside it to ensure accurate output.
Coincidence
Coincidence tends to be the most significant error in causal research. Researchers often misinterpret a coincidental link between a cause and effect as a direct causal link.
Administration challenges
Causal research can be challenging to administer since it's impossible to control the impact of extraneous variables .
Giving away your competitive advantage
If you intend to publish your research, it exposes your information to the competition.
Competitors may use your research outcomes to identify your plans and strategies to enter the market before you.
- Causal research examples
Multiple fields can use causal research, so it serves different purposes, such as.
Customer loyalty research
Organizations and employees can use causal research to determine the best customer attraction and retention approaches.
They monitor interactions between customers and employees to identify cause-and-effect patterns. That could be a product demonstration technique resulting in higher or lower sales from the same customers.
Example: Business X introduces a new individual marketing strategy for a small customer group and notices a measurable increase in monthly subscriptions.
Upon getting identical results from different groups, the business concludes that the individual marketing strategy resulted in the intended causal relationship.
Advertising research
Businesses can also use causal research to implement and assess advertising campaigns.
Example: Business X notices a 7% increase in sales revenue a few months after a business introduces a new advertisement in a certain region. The business can run the same ad in random regions to compare sales data over the same period.
This will help the company determine whether the ad caused the sales increase. If sales increase in these randomly selected regions, the business could conclude that advertising campaigns and sales share a cause-and-effect relationship.
Educational research
Academics, teachers, and learners can use causal research to explore the impact of politics on learners and pinpoint learner behavior trends.
Example: College X notices that more IT students drop out of their program in their second year, which is 8% higher than any other year.
The college administration can interview a random group of IT students to identify factors leading to this situation, including personal factors and influences.
With the help of in-depth statistical analysis, the institution's researchers can uncover the main factors causing dropout. They can create immediate solutions to address the problem.
Is a causal variable dependent or independent?
When two variables have a cause-and-effect relationship, the cause is often called the independent variable. As such, the effect variable is dependent, i.e., it depends on the independent causal variable. An independent variable is only causal under experimental conditions.
What are the three criteria for causality?
The three conditions for causality are:
Temporality/temporal precedence: The cause must precede the effect.
Rationality: One event predicts the other with an explanation, and the effect must vary in proportion to changes in the cause.
Control for extraneous variables: The covariables must not result from other variables.
Is causal research experimental?
Causal research is mostly explanatory. Causal studies focus on analyzing a situation to explore and explain the patterns of relationships between variables.
Further, experiments are the primary data collection methods in studies with causal research design. However, as a research design, causal research isn't entirely experimental.
What is the difference between experimental and causal research design?
One of the main differences between causal and experimental research is that in causal research, the research subjects are already in groups since the event has already happened.
On the other hand, researchers randomly choose subjects in experimental research before manipulating the variables.
Should you be using a customer insights hub?
Do you want to discover previous research faster?
Do you share your research findings with others?
Do you analyze research data?
Start for free today, add your research, and get to key insights faster
Editor’s picks
Last updated: 18 April 2023
Last updated: 27 February 2023
Last updated: 6 February 2023
Last updated: 6 October 2023
Last updated: 5 February 2023
Last updated: 16 April 2023
Last updated: 9 March 2023
Last updated: 12 December 2023
Last updated: 11 March 2024
Last updated: 4 July 2024
Last updated: 6 March 2024
Last updated: 5 March 2024
Last updated: 13 May 2024
Latest articles
Related topics, .css-je19u9{-webkit-align-items:flex-end;-webkit-box-align:flex-end;-ms-flex-align:flex-end;align-items:flex-end;display:-webkit-box;display:-webkit-flex;display:-ms-flexbox;display:flex;-webkit-flex-direction:row;-ms-flex-direction:row;flex-direction:row;-webkit-box-flex-wrap:wrap;-webkit-flex-wrap:wrap;-ms-flex-wrap:wrap;flex-wrap:wrap;-webkit-box-pack:center;-ms-flex-pack:center;-webkit-justify-content:center;justify-content:center;row-gap:0;text-align:center;max-width:671px;}@media (max-width: 1079px){.css-je19u9{max-width:400px;}.css-je19u9>span{white-space:pre;}}@media (max-width: 799px){.css-je19u9{max-width:400px;}.css-je19u9>span{white-space:pre;}} decide what to .css-1kiodld{max-height:56px;display:-webkit-box;display:-webkit-flex;display:-ms-flexbox;display:flex;-webkit-align-items:center;-webkit-box-align:center;-ms-flex-align:center;align-items:center;}@media (max-width: 1079px){.css-1kiodld{display:none;}} build next, decide what to build next.

Users report unexpectedly high data usage, especially during streaming sessions.

Users find it hard to navigate from the home page to relevant playlists in the app.

It would be great to have a sleep timer feature, especially for bedtime listening.

I need better filters to find the songs or artists I’m looking for.
Log in or sign up
Get started for free
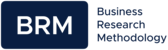
Causal Research (Explanatory research)
Causal research, also known as explanatory research is conducted in order to identify the extent and nature of cause-and-effect relationships. Causal research can be conducted in order to assess impacts of specific changes on existing norms, various processes etc.
Causal studies focus on an analysis of a situation or a specific problem to explain the patterns of relationships between variables. Experiments are the most popular primary data collection methods in studies with causal research design.
The presence of cause cause-and-effect relationships can be confirmed only if specific causal evidence exists. Causal evidence has three important components:
1. Temporal sequence . The cause must occur before the effect. For example, it would not be appropriate to credit the increase in sales to rebranding efforts if the increase had started before the rebranding.
2. Concomitant variation . The variation must be systematic between the two variables. For example, if a company doesn’t change its employee training and development practices, then changes in customer satisfaction cannot be caused by employee training and development.
3. Nonspurious association . Any covarioaton between a cause and an effect must be true and not simply due to other variable. In other words, there should be no a ‘third’ factor that relates to both, cause, as well as, effect.
The table below compares the main characteristics of causal research to exploratory and descriptive research designs: [1]
Amount of uncertainty characterising decision situation | Clearly defined | Highly ambiguous | Partially defined |
Key research statement | Research hypotheses | Research question | Research question |
When conducted? | Later stages of decision making | Early stage of decision making | Later stages of decision making |
Usual research approach | Highly structured | Unstructured | Structured |
Examples | ‘Will consumers buy more products in a blue package?’ ‘Which of two advertising campaigns will be more effective?’ | ‘Our sales are declining for no apparent reason’ ‘What kinds of new products are fast-food consumers interested in?’ | ‘What kind of people patronize our stores compared to our primary competitor?’ ‘What product features are the most important to our customers?’ |
Main characteristics of research designs
Examples of Causal Research (Explanatory Research)
The following are examples of research objectives for causal research design:
- To assess the impacts of foreign direct investment on the levels of economic growth in Taiwan
- To analyse the effects of re-branding initiatives on the levels of customer loyalty
- To identify the nature of impact of work process re-engineering on the levels of employee motivation
Advantages of Causal Research (Explanatory Research)
- Causal studies may play an instrumental role in terms of identifying reasons behind a wide range of processes, as well as, assessing the impacts of changes on existing norms, processes etc.
- Causal studies usually offer the advantages of replication if necessity arises
- This type of studies are associated with greater levels of internal validity due to systematic selection of subjects
Disadvantages of Causal Research (Explanatory Research)
- Coincidences in events may be perceived as cause-and-effect relationships. For example, Punxatawney Phil was able to forecast the duration of winter for five consecutive years, nevertheless, it is just a rodent without intellect and forecasting powers, i.e. it was a coincidence.
- It can be difficult to reach appropriate conclusions on the basis of causal research findings. This is due to the impact of a wide range of factors and variables in social environment. In other words, while casualty can be inferred, it cannot be proved with a high level of certainty.
- It certain cases, while correlation between two variables can be effectively established; identifying which variable is a cause and which one is the impact can be a difficult task to accomplish.
My e-book, The Ultimate Guide to Writing a Dissertation in Business Studies: a step by step assistance contains discussions of theory and application of research designs. The e-book also explains all stages of the research process starting from the selection of the research area to writing personal reflection. Important elements of dissertations such as research philosophy , research approach , methods of data collection , data analysis and sampling are explained in this e-book in simple words.
John Dudovskiy
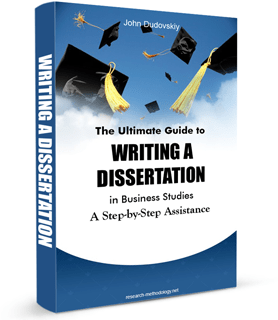
[1] Source: Zikmund, W.G., Babin, J., Carr, J. & Griffin, M. (2012) “Business Research Methods: with Qualtrics Printed Access Card” Cengage Learning
- Skip to main content
- Skip to primary sidebar
- Skip to footer
- QuestionPro

- Solutions Industries Gaming Automotive Sports and events Education Government Travel & Hospitality Financial Services Healthcare Cannabis Technology Use Case NPS+ Communities Audience Contactless surveys Mobile LivePolls Member Experience GDPR Positive People Science 360 Feedback Surveys
- Resources Blog eBooks Survey Templates Case Studies Training Help center

Home Market Research Research Tools and Apps
Causal Research: What it is, Tips & Examples
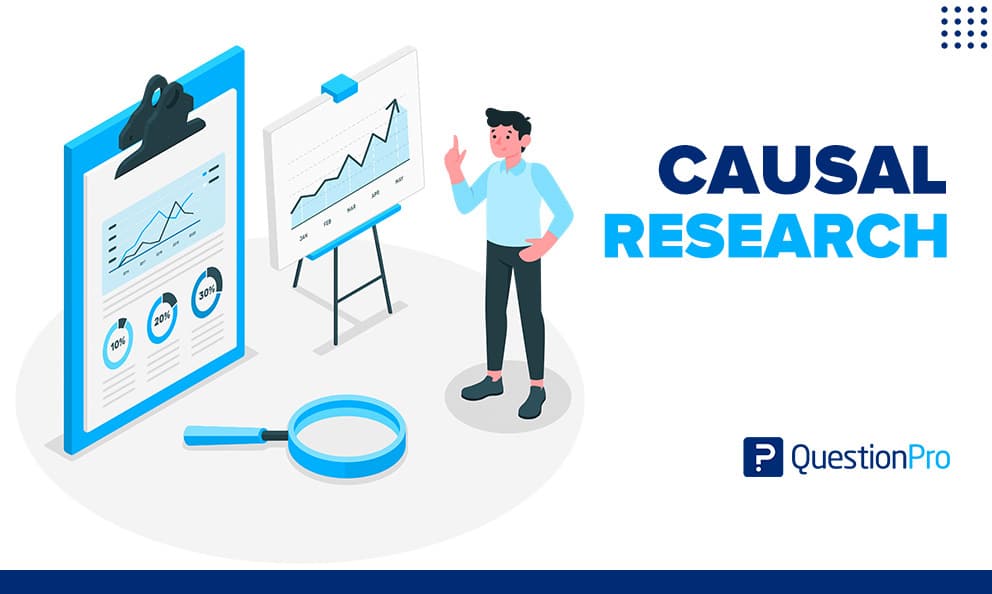
Causal research is classified as conclusive research since it attempts to build a cause-and-effect link between two variables. This research is mainly used to determine the cause of particular behavior. We can use this research to determine what changes occur in an independent variable due to a change in the dependent variable.
It can assist you in evaluating marketing activities, improving internal procedures, and developing more effective business plans. Understanding how one circumstance affects another may help you determine the most effective methods for satisfying your business needs.
LEARN ABOUT: Behavioral Research
This post will explain causal research, define its essential components, describe its benefits and limitations, and provide some important tips.
Content Index
What is causal research?
Temporal sequence, non-spurious association, concomitant variation, the advantages, the disadvantages, causal research examples, causal research tips.
Causal research is also known as explanatory research . It’s a type of research that examines if there’s a cause-and-effect relationship between two separate events. This would occur when there is a change in one of the independent variables, which is causing changes in the dependent variable.
You can use causal research to evaluate the effects of particular changes on existing norms, procedures, and so on. This type of research examines a condition or a research problem to explain the patterns of interactions between variables.
LEARN ABOUT: Research Process Steps
Components of causal research
Only specific causal information can demonstrate the existence of cause-and-effect linkages. The three key components of causal research are as follows:
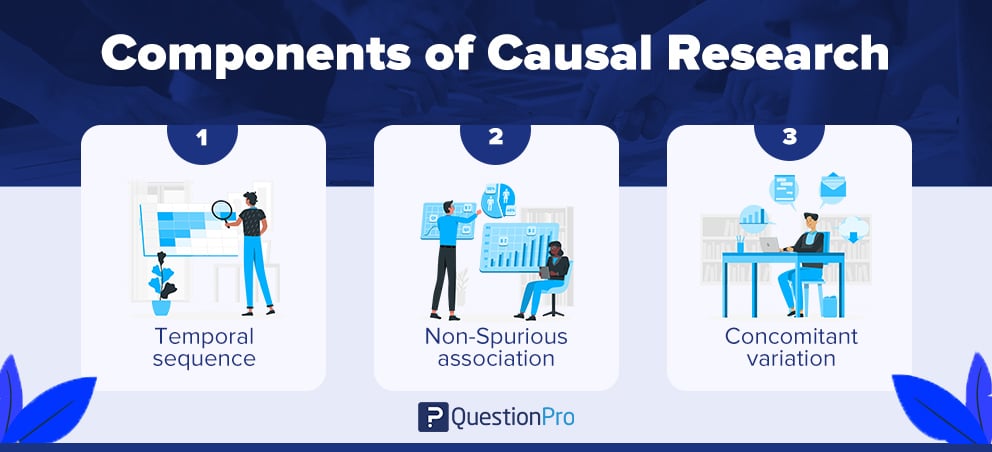
Prior to the effect, the cause must occur. If the cause occurs before the appearance of the effect, the cause and effect can only be linked. For example, if the profit increase occurred before the advertisement aired, it cannot be linked to an increase in advertising spending.
Linked fluctuations between two variables are only allowed if there is no other variable that is related to both cause and effect. For example, a notebook manufacturer has discovered a correlation between notebooks and the autumn season. They see that during this season, more people buy notebooks because students are buying them for the upcoming semester.
During the summer, the company launched an advertisement campaign for notebooks. To test their assumption, they can look up the campaign data to see if the increase in notebook sales was due to the student’s natural rhythm of buying notebooks or the advertisement.
Concomitant variation is defined as a quantitative change in effect that happens solely as a result of a quantitative change in the cause. This means that there must be a steady change between the two variables. You can examine the validity of a cause-and-effect connection by seeing if the independent variable causes a change in the dependent variable.
For example, if any company does not make an attempt to enhance sales by acquiring skilled employees or offering training to them, then the hire of experienced employees cannot be credited for an increase in sales. Other factors may have contributed to the increase in sales.
Causal Research Advantages and Disadvantages
Causal or explanatory research has various advantages for both academics and businesses. As with any other research method, it has a few disadvantages that researchers should be aware of. Let’s look at some of the advantages and disadvantages of this research design .
- Helps in the identification of the causes of system processes. This allows the researcher to take the required steps to resolve issues or improve outcomes.
- It provides replication if it is required.
- Causal research assists in determining the effects of changing procedures and methods.
- Subjects are chosen in a methodical manner. As a result, it is beneficial for improving internal validity .
- The ability to analyze the effects of changes on existing events, processes, phenomena, and so on.
- Finds the sources of variable correlations, bridging the gap in correlational research .
- It is not always possible to monitor the effects of all external factors, so causal research is challenging to do.
- It is time-consuming and might be costly to execute.
- The effect of a large range of factors and variables existing in a particular setting makes it difficult to draw results.
- The most major error in this research is a coincidence. A coincidence between a cause and an effect can sometimes be interpreted as a direction of causality.
- To corroborate the findings of the explanatory research , you must undertake additional types of research. You can’t just make conclusions based on the findings of a causal study.
- It is sometimes simple for a researcher to see that two variables are related, but it can be difficult for a researcher to determine which variable is the cause and which variable is the effect.
Since different industries and fields can carry out causal comparative research , it can serve many different purposes. Let’s discuss 3 examples of causal research:
Advertising Research
Companies can use causal research to enact and study advertising campaigns. For example, six months after a business debuts a new ad in a region. They see a 5% increase in sales revenue.
To assess whether the ad has caused the lift, they run the same ad in randomly selected regions so they can compare sales data across regions over another six months. When sales pick up again in these regions, they can conclude that the ad and sales have a valuable cause-and-effect relationship.
LEARN ABOUT: Ad Testing
Customer Loyalty Research
Businesses can use causal research to determine the best customer retention strategies. They monitor interactions between associates and customers to identify patterns of cause and effect, such as a product demonstration technique leading to increased or decreased sales from the same customers.
For example, a company implements a new individual marketing strategy for a small group of customers and sees a measurable increase in monthly subscriptions. After receiving identical results from several groups, they concluded that the one-to-one marketing strategy has the causal relationship they intended.
Educational Research
Learning specialists, academics, and teachers use causal research to learn more about how politics affects students and identify possible student behavior trends. For example, a university administration notices that more science students drop out of their program in their third year, which is 7% higher than in any other year.
They interview a random group of science students and discover many factors that could lead to these circumstances, including non-university components. Through the in-depth statistical analysis, researchers uncover the top three factors, and management creates a committee to address them in the future.
Causal research is frequently the last type of research done during the research process and is considered definitive. As a result, it is critical to plan the research with specific parameters and goals in mind. Here are some tips for conducting causal research successfully:
1. Understand the parameters of your research
Identify any design strategies that change the way you understand your data. Determine how you acquired data and whether your conclusions are more applicable in practice in some cases than others.
2. Pick a random sampling strategy
Choosing a technique that works best for you when you have participants or subjects is critical. You can use a database to generate a random list, select random selections from sorted categories, or conduct a survey.
3. Determine all possible relations
Examine the different relationships between your independent and dependent variables to build more sophisticated insights and conclusions.
To summarize, causal or explanatory research helps organizations understand how their current activities and behaviors will impact them in the future. This is incredibly useful in a wide range of business scenarios. This research can ensure the outcome of various marketing activities, campaigns, and collaterals. Using the findings of this research program, you will be able to design more successful business strategies that take advantage of every business opportunity.
At QuestionPro, we offer all kinds of necessary tools for researchers to carry out their projects. It can help you get the most out of your data by guiding you through the process.
MORE LIKE THIS
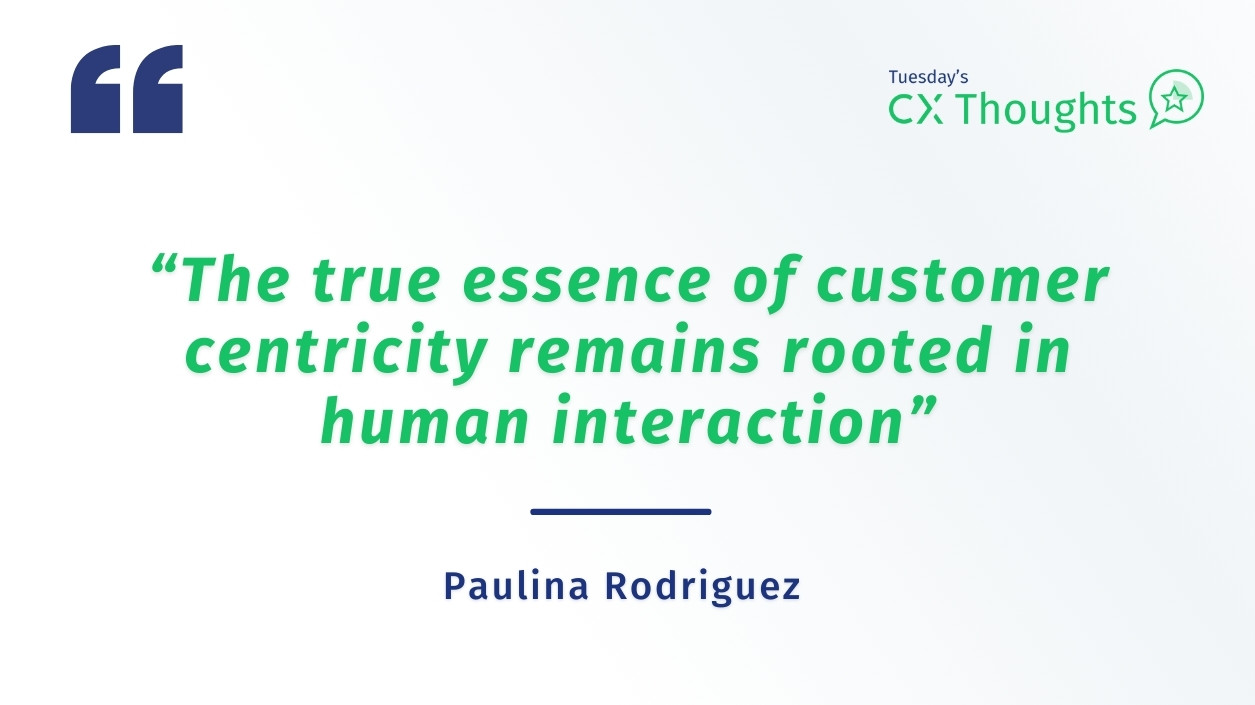
CX Shenanigans: Booth Duty and Beyond — Tuesday CX Thoughts
Jul 9, 2024
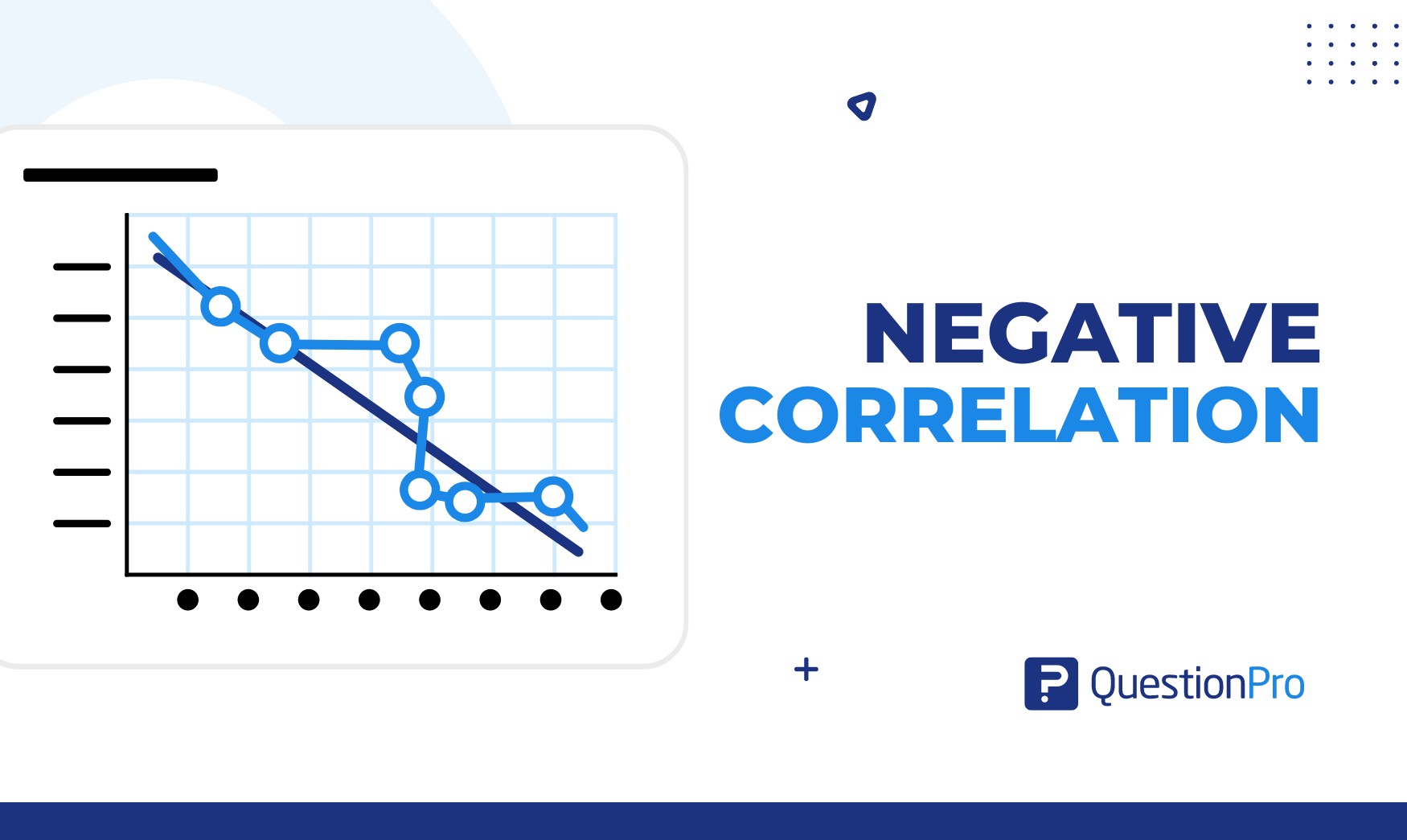
Negative Correlation: Definition, Examples + How to Find It?
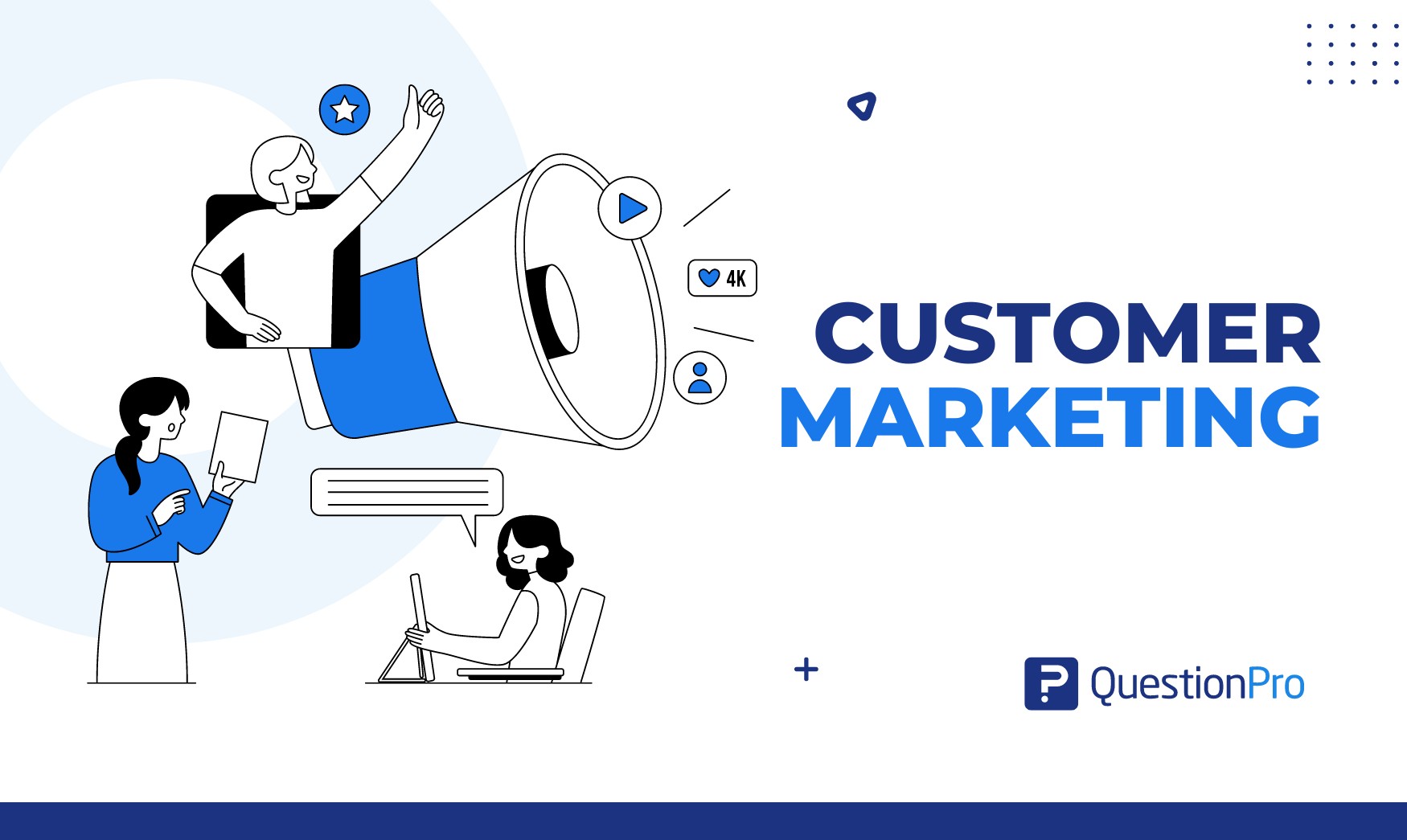
Customer Marketing: The Best Kept Secret of Big Brands
Jul 8, 2024
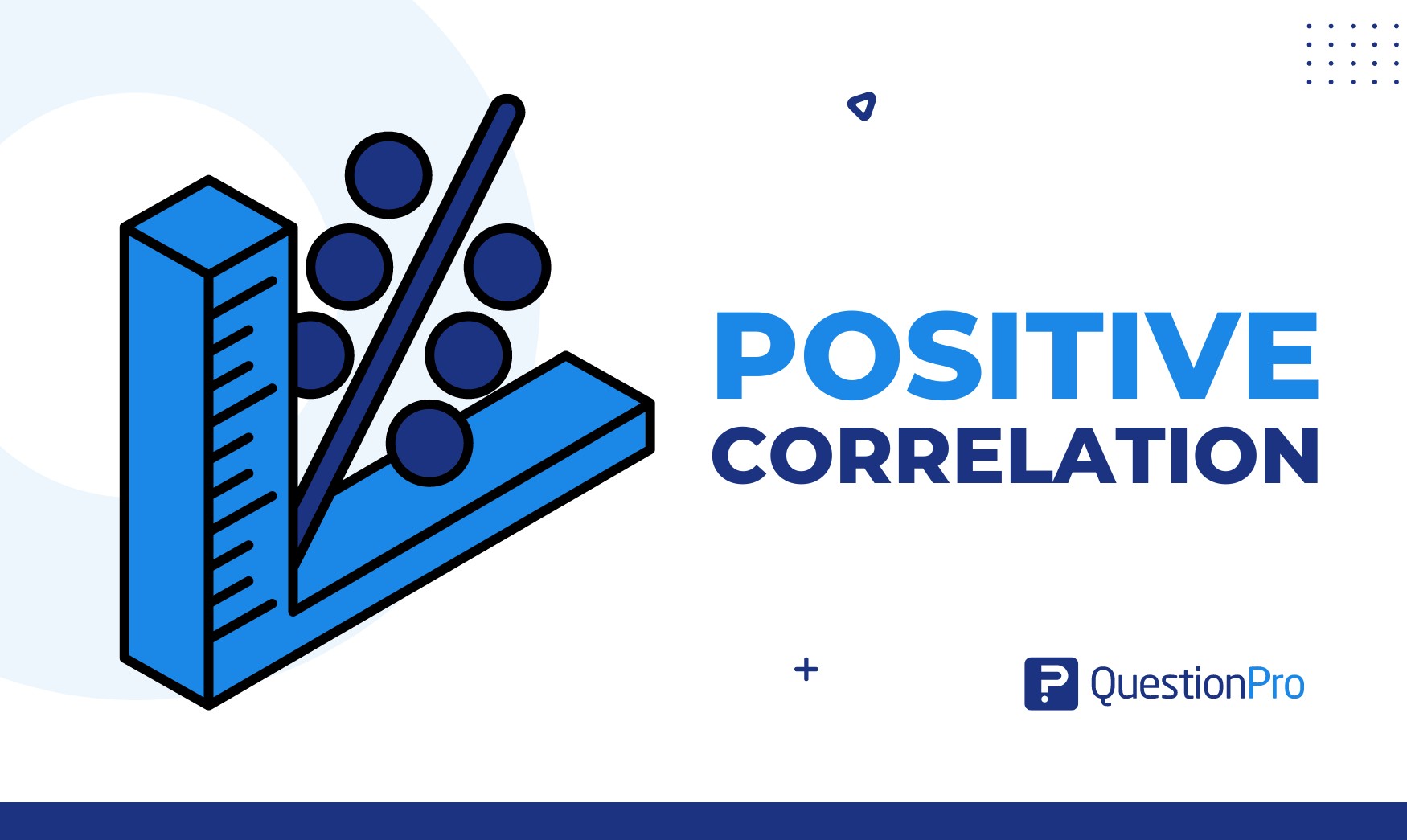
Positive Correlation: What It Is, Importance & How It Works
Jul 5, 2024
Other categories
- Academic Research
- Artificial Intelligence
- Assessments
- Brand Awareness
- Case Studies
- Communities
- Consumer Insights
- Customer effort score
- Customer Engagement
- Customer Experience
- Customer Loyalty
- Customer Research
- Customer Satisfaction
- Employee Benefits
- Employee Engagement
- Employee Retention
- Friday Five
- General Data Protection Regulation
- Insights Hub
- Life@QuestionPro
- Market Research
- Mobile diaries
- Mobile Surveys
- New Features
- Online Communities
- Question Types
- Questionnaire
- QuestionPro Products
- Release Notes
- Research Tools and Apps
- Revenue at Risk
- Survey Templates
- Training Tips
- Tuesday CX Thoughts (TCXT)
- Uncategorized
- What’s Coming Up
- Workforce Intelligence
- What is Causal Research? Definition + Key Elements

Cause-and-effect relationships happen in all aspects of life, from business to medicine, to marketing, to education, and so much more. They are the invisible threads that connect both our actions and inactions to their outcomes.
Causal research is the type of research that investigates cause-and-effect relationships. It is more comprehensive than descriptive research, which just talks about how things affect each other.
Let’s take a closer look at how you can use informal research to gain insight into your research results and make more informed decisions.

Defining Causal Research
Causal research investigates why one variable (the independent variable) is causing things to change in another ( the dependent variable).
For example, a causal research study about the cause-and-effect relationship between smoking and the prevalence of lung cancer. Smoking prevalence would be the independent variable, while lung cancer prevalence would be the dependent variable.
You would establish that smoking causes lung cancer by modulating the independent variable (smoking) and observing the effects on the dependent variable (lung cancer).
What’s the Difference Between Correlation and Causation
Correlation simply means that two variables are related to each other. But it does not necessarily mean that one variable causes changes in the other.
For example, let’s say there is a correlation between high coffee sales and low ice cream sales. This does not mean that people are not buying ice cream because they prefer coffee.
Both of these variables correlate because they’re influenced by the same factor: cold weather.
The Need for Causal Research

The major reason for investigating causal relationships between variables is better decision-making , which leads to developing effective solutions to complex problems. Here’s a breakdown of how it works:
- Decision-Making
Causal research enables us to figure out how variables relate to each other and how a change in one variable affects another. This helps us make better decisions about resource allocation, problem-solving, and achieving our goals.
In business, for example, customer satisfaction (independent variable) directly impacts sales (dependent variable). If customers are happy with your product or service, they’re more likely to keep returning and recommending it to their friends, which translates into more sales.
- Developing Effective Solutions to Problems
Understanding the causes of a problem, allows you to develop more effective solutions to address it. For example, medical causal research enables you to understand symptoms better, create new prevention strategies, and provide more effective treatment for illnesses.

Examples of Where Causal Relationships Are Critical
Here are a couple of ways you can leverage causal research:
- Policy-making : Causal research informs policy decisions about issues such as education, healthcare, and the environment. Let’s say causal research shows that the availability of junk food in schools directly impacts the prevalence of obesity in teenagers. This would inform the decision to incorporate more healthy food options in schools.
- Marketing strategies : Causal research studies allow you to identify factors that influence customer behavior to develop effective marketing strategies. For example, you can use causal research to reach and attract your target audience with the right content.
- Product development : Causal research enables you to create successful products by understanding users’ pain points and providing products that meet these needs.

Key Elements of Causal Research
Let’s take a deep dive into what it takes to design and conduct a causal study:
- Control and Experimental Groups
In a controlled study, the researchers randomly put people into one of two groups: the control group, who don’t get the treatment, or the experimental group, who do.
Having a control group allows you to compare the effects of the treatment to the effects of no treatment. It enables you to rule out the possibility that any changes in the dependent variable are due to factors other than the treatment.
- Independent variable : The independent variable is the variable that affects the dependent variable. It is the variable that you alter to see the effect on the dependent variable.
- Dependent variable : The dependent variable is the variable that is affected by the independent variable. This is what you measure to see the impact of the independent variable.
An Illustration of How Independent vs Dependent Variable Works in Causal Research
Here’s an illustration to help you understand how to differentiate and use variables in causal research:
Let’s say you want to investigate “ the effect of dieting on weight loss ”, dieting would be the independent variable, and weight loss would be the dependent variable. Next, you would vary the independent variable (dieting) by assigning some participants to a restricted diet and others to a control group.
You will see the cause-and-effect relationship between dieting and weight loss by measuring the dependent variable (weight loss) in both groups.
Skip the setup hassle! Get a head start on your research with our ready-to-use Experimental Research Survey Template
Research Designs for Establishing Causality
There are several ways to investigate the relationship between variables, but here are the most common:
A. Experimental Design
Experimental designs are the gold standard for establishing causality. In an experimental design, the researcher randomly assigns participants to either a control group or an experimental group. The control group does not receive the treatment, while the experimental group does.
Pros of experimental designs :
- Highly rigorous
- Explicitly establishes causality
- Strictly controls for extraneous variables
- Time-consuming and expensive
- Difficult to implement in real-world settings
- Not always ethical
B. Quasi-Experimental Design
A quasi-experimental design attempts to determine the causal relationship without fully randomizing the participant distribution into groups. The primary reason for this is ethical or practical considerations.
Different types of quasi-experimental designs
- Time series design : This design involves collecting data over time on the same group of participants. You see the cause-and-effect relationship by identifying the changes in the dependent variable that coincide with changes in the independent variable.
- Nonequivalent control group design : This design involves comparing an experimental group to a control group that is not randomly assigned. The differences between the two groups explain the cause-and-effect relationship.
- Interrupted time series design : Unlike the time series that measures changes over time, this introduces treatment at a specific point in time. You figure out the relationship between treatment and the dependent variable by looking for any changes that occurred at the time the treatment was introduced.
Pros of quasi-experimental designs
- Cost-effective
- More feasible to implement in real-world settings
- More ethical than experimental designs
- Not as thorough as experimental designs
- May not accurately establish causality
- More susceptible to bias
Establishing Causality without Experiments
Using experiments to determine the cause-and-effect relationship between each dependent variable and the independent variable can be time-consuming and expensive. As a result, the following are cost-effective methods for establishing a causal relationship:
- Longitudinal Studies
Long-term studies are observational studies that follow the same participants or groups over a long period. This way, you can see changes in variables you’re studying over time, and establish a causal relationship between them.
For example, you can use a longitudinal study to determine the effect of a new education program on student performance. You then track students’ academic performance over the years to see if the program improved student performance.
Challenges of Longitudinal Studies
One of the biggest problems of longitudinal studies is confounding variables. These are factors that are related to both the independent variable and the dependent variable.
Confounding variables can make it hard to isolate the cause of an independent variable’s effect. Using the earlier example, if you’re looking at how new educational programs affect student success, you need to make sure you’re controlling for factors such as students’ socio-economic background and their prior academic performance.
- Instrumental Variables (IV) Analysis
Instrumental variable analysis (IV) is a statistical approach that enables you to estimate causal effects in observational studies. An instrumental variable is a variable that is correlated with the independent variable but is not correlated with the dependent variable except through the independent variable.
For example, in academic achievement research, an instrumental variable could be the distance to the nearest college. This variable is correlated with family income but doesn’t correlate with academic achievement except through family income.
Challenges of Instrumental Variables (IV) Analysis
A primary limitation of IV analysis is that it can be challenging to find a good instrumental variable. IV analysis can also be very sensitive to the assumptions of the model.
Challenges and Pitfalls

It is a powerful tool for solving problems, making better decisions, and advancing human knowledge. However, causal research is not without its challenges and pitfalls.
- Confounding Variables
A confounding variable is a variable that correlates with both the independent and dependent variables, and it can make it difficult to isolate the causal effect of the independent variable.
For example, let’s say you are interested in the causal effect of smoking on lung cancer. If you simply compare smokers to nonsmokers, you may find that smokers are more likely to get lung cancer.
However, the relationship between smoking and lung cancer may be confounded by other factors, such as age, socioeconomic status, or exposure to secondhand smoke. These other factors may be responsible for the increased risk of lung cancer in smokers, rather than smoking itself.
Unlock the research secrets that top professionals use: Get the facts you need about Desk Research here
Strategy to Control for Confounding Variables
Confounding variables can lead to misleading results and make it difficult to determine the cause-and-effect between variables. Here are some strategies that allow you to control for confounding variables and improve the reliability of causal research findings:
- Randomized Controlled Trial (RCT)
In an RCT, participants are randomly assigned to either the treatment group or the control group. This ensures that the two groups are comparable on all confounding variables, except for the treatment itself.
- Statistical Methods
Using statistical methods such as multivariate regression analysis allows you to control for multiple confounding variables simultaneously.
Reverse Causation
Reverse Causation is when the relationship between the cause and effect of variables is reversed.
For example, let’s say you want to find a correlation between education and income. You’d expect people with higher levels of education to earn more, right?
Well, what if it’s the other way around? What if people with higher income are only more college-educated because they can afford it and lower-income people can’t?
Strategy to Control for Reverse Causation
Here are some ways to prevent and mitigate the effect of reverse causation:
- Longitudinal study
A longitudinal study follows the same individuals or groups over time. This allows researchers to see how changes in one variable (e.g., education) are associated with changes in another variable (e.g., income) over time.
- Instrumental Variables Analysis
Instrumental variables analysis is a statistical technique that estimates the causal effect of a variable when there is reverse causation.
Real-World Applications
Causal research allows us to identify the root causes of problems and develop solutions that work. Here are some examples of the real-world applications of causal research:
- Healthcare Research:
Causal research enables healthcare professionals to figure out what causes diseases and how to treat them.
For example, medical researchers can use casual research to figure out if a drug or treatment is effective for a specific condition. It also helps determine what causes certain diseases.
Randomized controlled trials (RCTs) are widely regarded as the standard for determining causal relationships in healthcare research. They have been used to determine the effects of multiple medical interventions, such as the effectiveness of new drugs and vaccines, surgery, as well as lifestyle changes on health.
- Public Policy Impact
Causal research can also be used to inform public policy decisions. For example, a causal study showed that early childhood education for disadvantaged children improved their academic performance and reduced their likelihood of dropping out. This has been leveraged to support policies that increase early childhood education access.
You can also use causal research to see if existing policies are working. For example, a causal study proves that giving ex-offenders job training reduces their chances of reoffending. The governments would be motivated to set up, fund, and mandate ex-offenders to take training programs.
Understanding causal effects helps us make informed decisions across different fields such as health, business, lifestyle, public policy, and more. But, this research method has its challenges and limitations.
Using the best practices and strategies in this guide can help you mitigate the limitations of causal research. Start your journey to seamlessly collecting valid data for your research with Formplus .
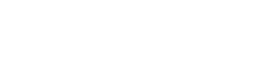
Connect to Formplus, Get Started Now - It's Free!
- casual research
- research design
- Moradeke Owa
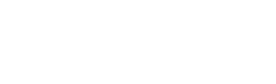
You may also like:
43 Market Research Terminologies You Need To Know
Introduction Market research is a process of gathering information to determine the needs, wants, or behaviors of consumers or...

Projective Techniques In Surveys: Definition, Types & Pros & Cons
Introduction When you’re conducting a survey, you need to find out what people think about things. But how do you get an accurate and...
Desk Research: Definition, Types, Application, Pros & Cons
If you are looking for a way to conduct a research study while optimizing your resources, desk research is a great option. Desk research...
Writing Research Proposals: Tips, Examples & Mistakes
In this article, we’ll discover several tips for writing an effective research proposal and common pitfalls you should look out for.
Formplus - For Seamless Data Collection
Collect data the right way with a versatile data collection tool. try formplus and transform your work productivity today..

An official website of the United States government
The .gov means it’s official. Federal government websites often end in .gov or .mil. Before sharing sensitive information, make sure you’re on a federal government site.
The site is secure. The https:// ensures that you are connecting to the official website and that any information you provide is encrypted and transmitted securely.
- Publications
- Account settings
Preview improvements coming to the PMC website in October 2024. Learn More or Try it out now .
- Advanced Search
- Journal List
- Dtsch Arztebl Int
- v.117(7); 2020 Feb
Methods for Evaluating Causality in Observational Studies
Emilio a.l.gianicolo.
1 Institute for Medical Biostatistics, Epidemiology and Informatics (IMBEI), University Medical Center of the Johannes Gutenberg University of Mainz
2 Institute of Clinical Physiology of the Italian National Research Council, Lecce, Italy
Martin Eichler
3 Technical University Dresden, University Hospital Carl Gustav Carus, Medical Clinic 1, Dresden
Oliver Muensterer
4 Department of Pediatric Surgery, Faculty of Medicine, Johannes Gutenberg University of Mainz
Konstantin Strauch
5 Institute of Genetic Epidemiology, Helmholtz Zentrum München—German Research Center for Environmental Health, Neuherberg; Chair of Genetic Epidemiology, Institute for Medical Information Processing, Biometry, and Epidemiology, Ludwig-Maximilians-Universität, München
Maria Blettner
In clinical medical research, causality is demonstrated by randomized controlled trials (RCTs). Often, however, an RCT cannot be conducted for ethical reasons, and sometimes for practical reasons as well. In such cases, knowledge can be derived from an observational study instead. In this article, we present two methods that have not been widely used in medical research to date.
The methods of assessing causal inferences in observational studies are described on the basis of publications retrieved by a selective literature search.
Two relatively new approaches—regression-discontinuity methods and interrupted time series—can be used to demonstrate a causal relationship under certain circumstances. The regression-discontinuity design is a quasi-experimental approach that can be applied if a continuous assignment variable is used with a threshold value. Patients are assigned to different treatment schemes on the basis of the threshold value. For assignment variables that are subject to random measurement error, it is assumed that, in a small interval around a threshold value, e.g., cholesterol values of 160 mg/dL, subjects are assigned essentially at random to one of two treatment groups. If patients with a value above the threshold are given a certain treatment, those with values below the threshold can serve as control group. Interrupted time series are a special type of regression-discontinuity design in which time is the assignment variable, and the threshold is a cutoff point. This is often an external event, such as the imposition of a smoking ban. A before-and-after comparison can be used to determine the effect of the intervention (e.g., the smoking ban) on health parameters such as the frequency of cardiovascular disease.
The approaches described here can be used to derive causal inferences from observational studies. They should only be applied after the prerequisites for their use have been carefully checked.
The fact that correlation does not imply causality was frequently mentioned in 2019 in the public debate on the effects of diesel emission exposure ( 1 , 2 ). This truism is well known and generally acknowledged. A more difficult question is how causality can be unambiguously defined and demonstrated ( box 1 ) . According to the eighteenth-century philosopher David Hume, causality is present when two conditions are satisfied: 1) B always follows A—in which case, A is called a “sufficient cause” of B; 2) if A does not occur, then B does not occur—in which case, A is called a “necessary cause” of B ( 3 ). These strict logical criteria are only rarely met in the medical field. In the context of exposure to diesel emissions, they would be met only if fine-particle exposure always led to lung cancer, and lung cancer never occurred without prior fine-particle exposure. Of course, neither of these is true. So what is biological, medical, or epidemiological causality? In medicine, causality is generally expressed in probabilistic terms, i.e. exposure to a risk factor such as cigarette smoking or diesel emissions increases the probability of a disease, e.g., lung cancer. The same understanding of causality applies to the effects of treatment: for instance, a certain type of chemotherapy increases the likelihood of survival in patients with a diagnosis of cancer, but does not guarantee it.
Causality in epidemiological observational studies (modified from Parascondola and Weed [34])
- ausality as production: A produces B. Causality is to be distinguished from mere temporal sequence. It does not suffice to note that A is always followed by B; rather, A must in some way produce, lead to, or create B. However, it remains unclear what ’producing’, ‘leading to’, or ‘creating’ exactly means. On a practical level, the notion of production is what is illustrated in the diagrams of cause-and-effect relationships that are commonly seen in medical publications.
- Sufficient and necessary causes: A is a sufficient cause of B if B always happens when A has happened. A is a necessary cause of B if B only happens when A has happened. Although these relationships are logically clear and seemingly simple, this type of deterministic causality is hardly ever found in real-life scientific research. Thus, smoking is neither a sufficient nor a necessary cause of lung cancer. Smoking is not always followed by lung cancer (not a sufficient cause), and lung cancer can occur in the absence of tobacco exposure (not a necessary cause, either).
- Sufficient component cause: This notion was developed in response to the definitions of sufficient and necessary causes. In this approach, it is assumed that multiple causes act together to produce an effect where no single one of them could do so alone. There can also be different combinations of causes that produce the same effect.
- Probabilistic causality: In this scenario, the cause (A) increases the probability (P) that the effect (B) will occur: in symbols, P (B | A) > (B | not A). Sufficient and necessary causes, as defined above in ( 2 ), are only those extreme cases in which P (B | A) = 1 and P (B | not A) = 0, respectively. When these probabilities take on values that are neither 0 nor 1, causality is no longer deterministic, but rather probabilistic (stochastic). There is no assumption that a cause must be followed by an effect. This viewpoint corresponds to the method of proceeding in statistically oriented scientific disciplines.
- Causal inference: This is the determination that a causal relationship exists between two types of event. Causal inferences are made by analyzing the changes in the effect that arise when there are changes in the cause. Causal inference goes beyond the mere assertion of an association and is connected to a number of specific concepts: some that have been widely discussed recently are counterfactuals, potential outcomes, causal diagrams, and structural equation models ( 36 , 37 ).
- Triangulation: Not all questions can be answered with an experiment or a randomized controlled trial. Alternatively, methodological pluralism is needed, or, as it is now sometimes called, triangulation: confidence in a finding increases when the same finding is arrived at from multiple data sets, multiple scientific disciplines, multiple theories, and/or multiple methods ( 35 ).
- The criterion of consequentiality: The claim that a causal relationship exists has consequences on a societal level (taking action or not taking action). Olsen has called for the formulation of a criterion to determine when action should be taken and when not ( 7 ).
In many scientific disciplines, causality must be demonstrated by an experiment. In clinical medical research, this purpose is achieved with a randomized controlled trial (RCT) ( 4 ). An RCT, however, often cannot be conducted for either ethical or practical reasons. If a risk factor such as exposure to diesel emissions is to be studied, persons cannot be randomly allocated to exposure or non-exposure. Nor is any randomization possible if the research question is whether or not an accident associated with an exposure, such as the Chernobyl nuclear reactor disaster, increased the frequency of illness or death. The same applies when a new law or regulation, e.g., a smoking ban, is introduced.
When no experiment can be conducted, observational studies need to be performed. The object under study—i.e., the possible cause—cannot be varied in a targeted and controlled way; instead, the effect this factor has on a target variable, such as a particular illness, is observed and documented.
Several publications in epidemiology have dealt with the ways in which causality can be inferred in the absence of an experiment, starting with the classic work of Bradford Hill and the nine aspects of causality (viewpoints) that he proposed ( box 2 ) ( 5 ) and continuing up to the present ( 6 , 7 ).
The Bradford Hill criteria for causality (modified from [5])
- Strength: the stronger the observed association between two variables, the less likely it is due to chance.
- Consistency: the association has been observed in multiple studies, populations at risk, places, and times, and by different researchers.
- Specificity: it is a strong argument for causality when a specific population suffers from a specific disease.
- Temporality: the effect must be temporally subsequent to the cause.
- Biological gradient: the association displays a dose–response effect, e.g., the incidence of lung cancer is greater when more cigarettes are smoked per day.
- Plausibility: a plausible mechanism linking the cause to the effect is helpful, but not absolutely required. What is biologically plausible depends upon the state-of-the-art knowledge of the time.
- Coherence: the causal interpretation of the data should not conflict with biological knowledge about the disease.
- Experiment: experimental evidence should be adduced in support, if possible.
- Analogy: an association speaks for causality if similar causes are already known to have similar effects.
Aside from the statistical uncertainty that always arises when only a sample of an affected population is studied, rather than its entirety ( 8 ), the main obstacle to the study of putative causal relationships comes from confounding variables (“confounders”). These are so named because they can, depending on the circumstances, either obscure a true effect or simulate an effect that is, in fact, not present ( 9 ). Age, for example, is a confounder in the study of the association between occupational radiation exposure and cataract ( 10 ), because both cumulative radiation exposure and the risk of cataract rise with increasing age.
The various statistical methods of dealing with known confounders in the analysis of epidemiological data have already been presented in other articles in this series ( 9 , 11 , 12 ). In the current article, we discuss two new approaches that have not been widely applied in medical and epidemiological research to date.
Methods of evaluating causal inferences in observational studies
The main advantage of an RCT is randomization, i.e., the random allocation of the units of observation (patients) to treatment groups. Potential confounders, whether known or unknown, are thereby distributed to the treatment groups at random as well, although differences between groups may arise through sample variance. Whenever randomization is not possible, the effect of confounders must be taken into account in the planning of the study and in data analysis, as well as in the interpretation of study findings.
Classic methods of dealing with confounders in study planning are stratification and matching ( 13 , 14 ), as well as so-called propensity score matching (PSM) ( 11 ).
The best-known and most commonly used method of data analysis is regression analysis, e.g., linear, logistic, or Cox regression ( 15 ). This method is based on a mathematical model created in order to explain the probability that any particular outcome will arise as the combined result of the known confounders and the effect under study.
Regression analyses are used in the analysis of clinical or epidemiological data and are found in all commonly used statistical software packages. However, they are often used inappropriately because the prerequisites for their correct application have not been checked. They should not be used, for example, if the sample is too small, if the number of variables is too large, or if a correlation between the model variables makes the results uninterpretable ( 16 ).
Regression-discontinuity methods
Regression-discontinuity methods have been little used in medical research to date, but they can be helpful in the study of cause-and-effect relationships from observational data ( 17 ). Regression-discontinuity design is a quasi-experimental approach ( box 3 ) that was developed in educational psychology in the 1960s ( 18 ). It can be used when a threshold value of a continuous variable (the “assignment variable”) determines the treatment regimen to which each patient in the study is assigned ( box 4 ) .
Terms used to characterize experiments ( 18 )
- Experiment/trial A study in which an intervention is deliberately introduced in order to observe an effect.
- Randomized experiment/trial An experiment in which persons, patients, or other units of observation are randomly assigned to one of two or more treatment groups (or intervention groups).
- Quasi-experiment An experiment in which the units of observation are not randomly assigned to the treatment/intervention groups.
- Natural experiment A study in which a natural event (e.g., an earthquake) is compared with a comparison scenario.
- Non-experimental observational study A study in which the size and direction of the association between two variables is determined.
In the simplest case, that of a linear regression, the parameters in the following model are to be estimated:
y i = ß 0 + ß 1 z i + ß 2 (x i - x c ) + e i,
i from 1 to N represents the statistical units
y is the outcome
ß 0 is the y-intercept
z is a dichotomous variable (0, ) indicating whether the patient was treated ( 1 ) or not treated (0)
x is the assignment variable
x c is the threshold
ß 1 is the effect of treatment
ß 2 is the regression coefficient of the assignment variable
e is the random error
A possible assignment variable could be, for example, the serum cholesterol level: consider a study in which patients with a cholesterol level of 160 mg/dL or above are assigned to receive a therapy. Since the cholesterol level (the assignment variable) is subject to random measurement error, it can be assumed that patients whose level of cholesterol is close to the threshold (160 mg/dL) are randomly assigned to the different treatment regimens. Thus, in a small interval around the threshold value, the assignment of patients to treatment groups can effectively be considered random ( 18 ). This sample of patients with near-threshold measurements can thus be used for the analysis of treatment efficacy. For this line of argument to be valid, it must truly be the case that the value being measured is subject to measuring error, and that there is practically no difference between persons with measured values slightly below or slightly above threshold. Treatment allocation in this narrow range can be considered quasi-random.
This method can be applied if the following prerequisites are met:
- The assignment variable is a continuous variable that is measured before the treatment is provided. If the assignment variable is totally independent of the outcome and has no biological, medical, or epidemiological significance, the method is theoretically equivalent to an RCT ( 19 ).
- The treatment must not affect the assignment variable ( 18 ).
- The patients in the two treatment groups with near-threshold values of the assignment variable must be shown to be similar in their baseline properties, i.e., covariables, including possible confounders. This can be demonstrated either with statistical techniques or graphically ( 20 ).
- The range of the assignment variable in the vicinity of the threshold must be optimally set: it must be large enough to yield samples of adequate size in the treatment groups, yet small enough that the effect of the assignment variable itself does not alter the outcome being studied. Methods of choosing this range appropriately are available in the literature ( 21 , 22 ).
- The treatment can be decided upon solely on the basis of the assignment variable (deterministic regression-discontinuity methods), or on the basis of other clinical factors (fuzzy regression-discontinuity methods).
Example 1: The one-year mortality of neonates as a function of the intensity of medical and nursing care was to be studied, where the intensity of care was determined by a birth-weight threshold: infants with very low birth weight (<1500 g) (group A) were cared for more intensively than heavier infants (group B) ( 23 ). The question to be answered was whether the greater intensity of care in group A led to a difference in mortality between the two groups. It was assumed that children with birth weight near the threshold are identical in all other respects, and that their assignment to group A or group B is quasi-random, because the measured value (birth weight) is subject to a relatively small error. Thus, for example, one might compare children weighing 1450–1500 g to those weighing 1501–1550 g at birth to study whether, and how, a greater intensity of care affects mortality.
In this example, it is assumed that the variable “birth weight” has a random measuring error, and thus that neonates whose (true) weight is near the threshold will be randomly allocated to one or the other category. But birth weight itself is an important factor affecting infant mortality, with lower birth weight associated with higher mortality ( 23 ); thus, the interval taken around the threshold for the purpose of this study had to be kept narrow. The study, in fact, showed that the children treated more intensively because their birth weight was just below threshold had a lower mortality than those treated less intensively because their birth weight was just above threshold.
Example 2: A regression-discontinuity design was used to evaluate the effect of a measure taken by the Canadian government: the introduction of a minimum age of 19 years for alcohol consumption. The researchers compared the number of alcohol-related disorders and of violent attacks, accidents, and suicides under the influence of alcohol in the months leading up to (group A) and subsequent to (group B) the 19 th birthday of the persons involved. It was found that persons in group B had a greater number of alcohol-related inpatient treatments and emergency hospitalizations than persons in group A. With the aid of this quasi-experimental approach, the researchers were able to demonstrate the success of the measure ( 24 ). It may be assumed that the two groups differed only with respect to age, and not with respect to any other property affecting alcohol consumption.
Interrupted time series
Interrupted time series are a special type of regression-discontinuity design in which time is the assignment variable. The cutoff point is often an external event that is unambiguously identifiable as having occurred at a certain point in time, e.g., an industrial accident or a change in the law. A before-and-after comparison is made in which the analysis must still take adequate account of any relevant secular trends and seasonal fluctuations ( box 5 ) .
In the simplest case of a study involving an interrupted time series, the temporal sequence is analyzed with a piecewise regression. The following model is used to study both a shift in slope and a shift in the level of an outcome before and after an intervention, e.g., the introduction of a law banning smoking ( figure 2 ):
y = ß 0 + ß 1 × time + ß 2 × intervention + ß 3 × time × intervention + e,
y is the outcome, e.g., cardiovascular diseases
intervention is a dummy variable for the time before (0) and after (1) the intervention (e.g., smoking ban)
time is the time since the beginning of the study
ß 0 is the baseline incidence of cardiovascular diseases
ß 1 is the slope in the incidence of cardiovascular diseases over time before the introduction of the smoking ban
ß 2 is the change in the incidence level of cardiovascular diseases after the introduction of the smoking ban (level effect)
ß 3 is the change in the slope over time (cf. ß 1 ) after the introduction of the smoking ban (slope effect)
The prerequisites for the use of this method must be met ( 18 , 25 ):
- Interrupted time series are valid only if a single intervention took place in the period of the study.
- The time before the intervention must be clearly distinguishable from the time after the intervention.
- There is no required minimum number of data points, but studies with only a small number of data points or small effect sizes must be interpreted with caution. The power of a study is greatest when the number of data points before the intervention equals the number after the intervention ( 26 ).
- Although the equation in Box 5 has a linear specification, polynomial and other nonlinear regression models can be used as well. Meticulous study of the temporal sequence is very important when a nonlinear model is used.
- If an observation at time t —e.g., the monthly incidence of cardiovascular diseases—is correlated with previous observations (autoregression), then the appropriate statistical techniques must be used (autoregressive integrated moving average [ARIMA] models).
Example 1: In one study, the rates of acute hospitalization for cardiovascular diseases before and after the temporary closure of Heathrow Airport because of volcanic ash were determined to investigate the putative effect of aircraft noise ( 27 ). The intervention (airport closure) took place from 15 to 20 April 2010. The hospitalization rate was found to have decreased among persons living in the urban area with the most aircraft noise. The number of observation points was too low, however, to show a causal link conclusively.
Example 2: In another study, the rates of hospitalization before and after the implementation of a smoking ban (the intervention) in public areas in Italy were determined ( 28 ). The intervention occurred in January 2004 (the cutoff time). The number of hospitalizations for acute coronary events was measured from January 2002 to November 2006 ( figure 1 ) . The analysis took account of seasonal dependence, and an effect modification for two age groups—persons under age 70 and persons aged 70 and up—was determined as well. The hospitalization rate declined in the former group, but not the latter.

Age-standardized hospitalization rates for acute coronary events (ACE) in persons under age 70 before and after the implementation of a smoking ban in public places in Italy, studied with the corresponding methods ( 30 ). The observed and predicted rates are shown (circles and solid lines, respectively). The dashed lines show the seasonally adjusted trend in ACE before and after the introduction of the nationwide smoking ban.
The necessary distinction between causality and correlation is often emphasized in scientific discussions, yet it is often not applied strictly enough. Furthermore, causality in medicine and epidemiology is mostly probabilistic in nature, i.e., an intervention alters the probability that the event under study will take place. A good illustration of this principle is offered by research on the effects of radiation, in which a strict distinction is maintained between deterministic radiation damage on the one hand, and probabilistic (stochastic) radiation damage on the other ( 29 ). Deterministic radiation damage—radiation-induced burns or death—arises with certainty whenever a subject receives a certain radiation dose (usually a high one). On the other hand, the risk of cancer-related mortality after radiation exposure is a stochastic matter. Epidemiological observations and biological experiments should be evaluated in tandem to strengthen conclusions about probabilistic causality ( box 1 ) .
While RCTs still retain their importance as the gold standard of clinical research, they cannot always be carried out. Some indispensable knowledge can only be obtained from observational studies. Confounding factors must be eliminated, or at least accounted for, early on when such studies are planned. Moreover, the data that are obtained must be carefully analyzed. And, finally, a single observational study hardly ever suffices to establish a causal relationship.
In this article, we have presented two newer methods that are relatively simple and which, therefore, could easily be used more widely in medical and epidemiological research ( 30 ). Either one should be used only after the prerequisites for its applicability have been meticulously checked. In regression-discontinuity methods, the assumption of continuity must be verified: in other words, it must be checked whether other properties of the treatment and control groups are the same, or at least equally balanced. The rules of group assignment and the role played by the continuous assignment variable must be known as well. Regression-discontinuity methods can generate causal conclusions, but any such conclusion will not be generalizable if the treatment effects are heterogeneous over the range of the assignment variable. The estimate of effect size is applicable only in a small, predefined interval around the threshold value. It must also be checked whether the outcome and the assignment variable are in a linear relationship, and whether there is any interaction between the treatment and assignment variables that needs to be considered.
In the analysis of interrupted time series, the assumption of continuity must be tested as well. Furthermore, the method is valid only if the occurrence of any other intervention at the same time point as the one under study can be ruled out ( 20 ). Finally, the type of temporal sequence must be considered, and more complex statistical methods must be applied, as needed, to take such phenomena as autoregression into account.
Observational studies often suggest causal relationships that will then be either supported or rejected after further studies and experiments. Knowledge of the effects of radiation exposure was derived, at first, mainly from observations on victims of the Hiroshima and Nagasaki atomic bomb explosions ( 31 ). These findings were reinforced by further epidemiological studies on other populations exposed to radiation (e.g., through medical procedures or as an occupational hazard), by physical considerations, and by biological experiments ( 32 ). A classic example from the mid-19 th century is the observational study by Snow ( 33 ): until then, the biological cause of cholera was unknown. Snow found that there had to be a causal relationship between the contamination of a well and a subsequent outbreak of cholera. This new understanding led to improved hygienic measures, which did, indeed, prevent infection with the cholera pathogen. Cases such as these prove that it is sometimes reasonable to take action on the basis of an observational study alone ( 6 ). They also demonstrate, however, that further studies are necessary for the definitive establishment of a causal relationship.

The effect of a smoking ban on the incidence of cardiovascular diseases
Key messages
- Causal inferences can be drawn from observational studies, as long as certain conditions are met.
- Confounding variables are a major impediment to the demonstration of causal links, as they can either obscure or mimic such a link.
- Random assignment leads to the even distribution of known and unknown confounders among the intervention groups that are being compared in the study.
- In the regression-discontinuity method, it is assumed that the assignment of patients to treatment groups is random with, in a small range of the assignment variable around the threshold, with the result that the confounders are randomly distributed as well.
- The interrupted time series is a variant of the regression-discontinuity method in which a given point in time splits the subjects into a before group and an after group, with random distribution of confounders to the two groups.
Acknowledgments
Translated from the original German by Ethan Taub, M.D.
Conflict of interest statement The authors state that they have no conflict of interest.
Have a language expert improve your writing
Run a free plagiarism check in 10 minutes, generate accurate citations for free.
- Knowledge Base
Methodology
- Explanatory Research | Definition, Guide, & Examples
Explanatory Research | Definition, Guide, & Examples
Published on December 3, 2021 by Tegan George and Julia Merkus. Revised on November 20, 2023.
Explanatory research is a research method that explores why something occurs when limited information is available. It can help you increase your understanding of a given topic, ascertain how or why a particular phenomenon is occurring, and predict future occurrences.
Explanatory research can also be explained as a “cause and effect” model, investigating patterns and trends in existing data that haven’t been previously investigated. For this reason, it is often considered a type of causal research .
Table of contents
When to use explanatory research, explanatory research questions, explanatory research data collection, explanatory research data analysis, step-by-step example of explanatory research, explanatory vs. exploratory research, advantages and disadvantages of explanatory research, other interesting articles, frequently asked questions about explanatory research.
Explanatory research is used to investigate how or why a phenomenon takes place. Therefore, this type of research is often one of the first stages in the research process, serving as a jumping-off point for future research. While there is often data available about your topic, it’s possible the particular causal relationship you are interested in has not been robustly studied.
Explanatory research helps you analyze these patterns, formulating hypotheses that can guide future endeavors. If you are seeking a more complete understanding of a relationship between variables, explanatory research is a great place to start. However, keep in mind that it will likely not yield conclusive results.
You analyzed their final grades and noticed that the students who take your course in the first semester always obtain higher grades than students who take the same course in the second semester.
Prevent plagiarism. Run a free check.
Explanatory research answers “why” and “how” questions, leading to an improved understanding of a previously unresolved problem or providing clarity for related future research initiatives.
Here are a few examples:
- Why do undergraduate students obtain higher average grades in the first semester than in the second semester?
- How does marital status affect labor market participation?
- Why do multilingual individuals show more risky behavior during business negotiations than monolingual individuals?
- How does a child’s ability to delay immediate gratification predict success later in life?
- Why are teens more likely to litter in a highly littered area than in a clean area?
After choosing your research question, there is a variety of options for research and data collection methods to choose from.
A few of the most common research methods include:
- Literature reviews
- Interviews and focus groups
- Pilot studies
- Observations
- Experiments
The method you choose depends on several factors, including your timeline, budget, and the structure of your question. If there is already a body of research on your topic, a literature review is a great place to start. If you are interested in opinions and behavior, consider an interview or focus group format. If you have more time or funding available, an experiment or pilot study may be a good fit for you.
In order to ensure you are conducting your explanatory research correctly, be sure your analysis is definitively causal in nature, and not just correlated.
Always remember the phrase “correlation doesn’t mean causation.” Correlated variables are merely associated with one another: when one variable changes, so does the other. However, this isn’t necessarily due to a direct or indirect causal link.
Causation means that changes in the independent variable bring about changes in the dependent variable. In other words, there is a direct cause-and-effect relationship between variables.
Causal evidence must meet three criteria:
- Temporal : What you define as the “cause” must precede what you define as the “effect.”
- Variation : Intervention must be systematic between your independent variable and dependent variable.
- Non-spurious : Be careful that there are no mitigating factors or hidden third variables that confound your results.
Correlation doesn’t imply causation, but causation always implies correlation. In order to get conclusive causal results, you’ll need to conduct a full experimental design .
Receive feedback on language, structure, and formatting
Professional editors proofread and edit your paper by focusing on:
- Academic style
- Vague sentences
- Style consistency
See an example
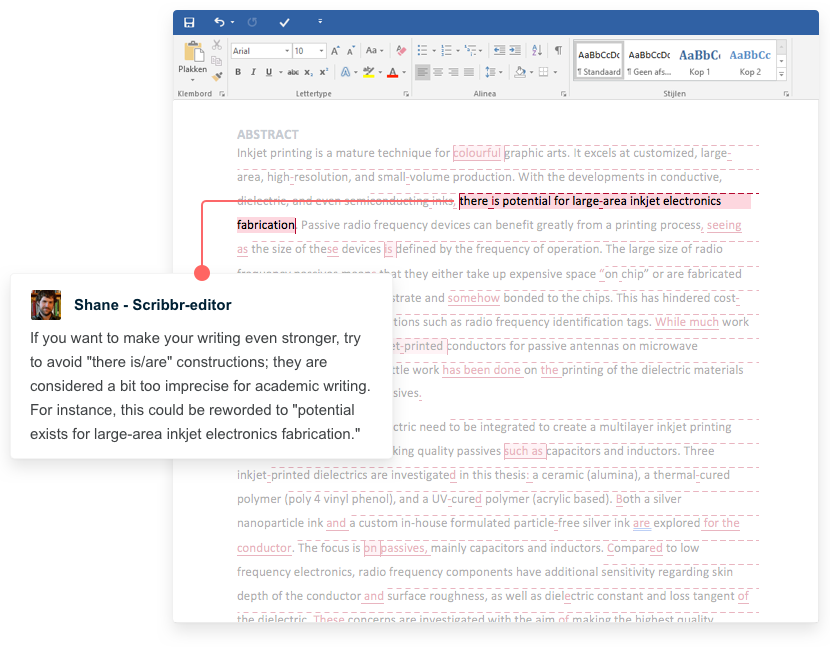
Your explanatory research design depends on the research method you choose to collect your data . In most cases, you’ll use an experiment to investigate potential causal relationships. We’ll walk you through the steps using an example.
Step 1: Develop the research question
The first step in conducting explanatory research is getting familiar with the topic you’re interested in, so that you can develop a research question .
Let’s say you’re interested in language retention rates in adults.
You are interested in finding out how the duration of exposure to language influences language retention ability later in life.
Step 2: Formulate a hypothesis
The next step is to address your expectations. In some cases, there is literature available on your subject or on a closely related topic that you can use as a foundation for your hypothesis . In other cases, the topic isn’t well studied, and you’ll have to develop your hypothesis based on your instincts or on existing literature on more distant topics.
You phrase your expectations in terms of a null (H 0 ) and alternative hypothesis (H 1 ):
- H 0 : The duration of exposure to a language in infancy does not influence language retention in adults who were adopted from abroad as children.
- H 1 : The duration of exposure to a language in infancy has a positive effect on language retention in adults who were adopted from abroad as children.
Step 3: Design your methodology and collect your data
Next, decide what data collection and data analysis methods you will use and write them up. After carefully designing your research, you can begin to collect your data.
You compare:
- Adults who were adopted from Colombia between 0 and 6 months of age.
- Adults who were adopted from Colombia between 6 and 12 months of age.
- Adults who were adopted from Colombia between 12 and 18 months of age.
- Monolingual adults who have not been exposed to a different language.
During the study, you test their Spanish language proficiency twice in a research design that has three stages:
- Pre-test : You conduct several language proficiency tests to establish any differences between groups pre-intervention.
- Intervention : You provide all groups with 8 hours of Spanish class.
- Post-test : You again conduct several language proficiency tests to establish any differences between groups post-intervention.
You made sure to control for any confounding variables , such as age, gender, proficiency in other languages, etc.
Step 4: Analyze your data and report results
After data collection is complete, proceed to analyze your data and report the results.
You notice that:
- The pre-exposed adults showed higher language proficiency in Spanish than those who had not been pre-exposed. The difference is even greater for the post-test.
- The adults who were adopted between 12 and 18 months of age had a higher Spanish language proficiency level than those who were adopted between 0 and 6 months or 6 and 12 months of age, but there was no difference found between the latter two groups.
To determine whether these differences are significant, you conduct a mixed ANOVA. The ANOVA shows that all differences are not significant for the pre-test, but they are significant for the post-test.
Step 5: Interpret your results and provide suggestions for future research
As you interpret the results, try to come up with explanations for the results that you did not expect. In most cases, you want to provide suggestions for future research.
However, this difference is only significant after the intervention (the Spanish class.)
You decide it’s worth it to further research the matter, and propose a few additional research ideas:
- Replicate the study with a larger sample
- Replicate the study for other maternal languages (e.g. Korean, Lingala, Arabic)
- Replicate the study for other language aspects, such as nativeness of the accent
It can be easy to confuse explanatory research with exploratory research. If you’re in doubt about the relationship between exploratory and explanatory research, just remember that exploratory research lays the groundwork for later explanatory research.
Exploratory research questions often begin with “what”. They are designed to guide future research and do not usually have conclusive results. Exploratory research is often utilized as a first step in your research process, to help you focus your research question and fine-tune your hypotheses.
Explanatory research questions often start with “why” or “how”. They help you study why and how a previously studied phenomenon takes place.
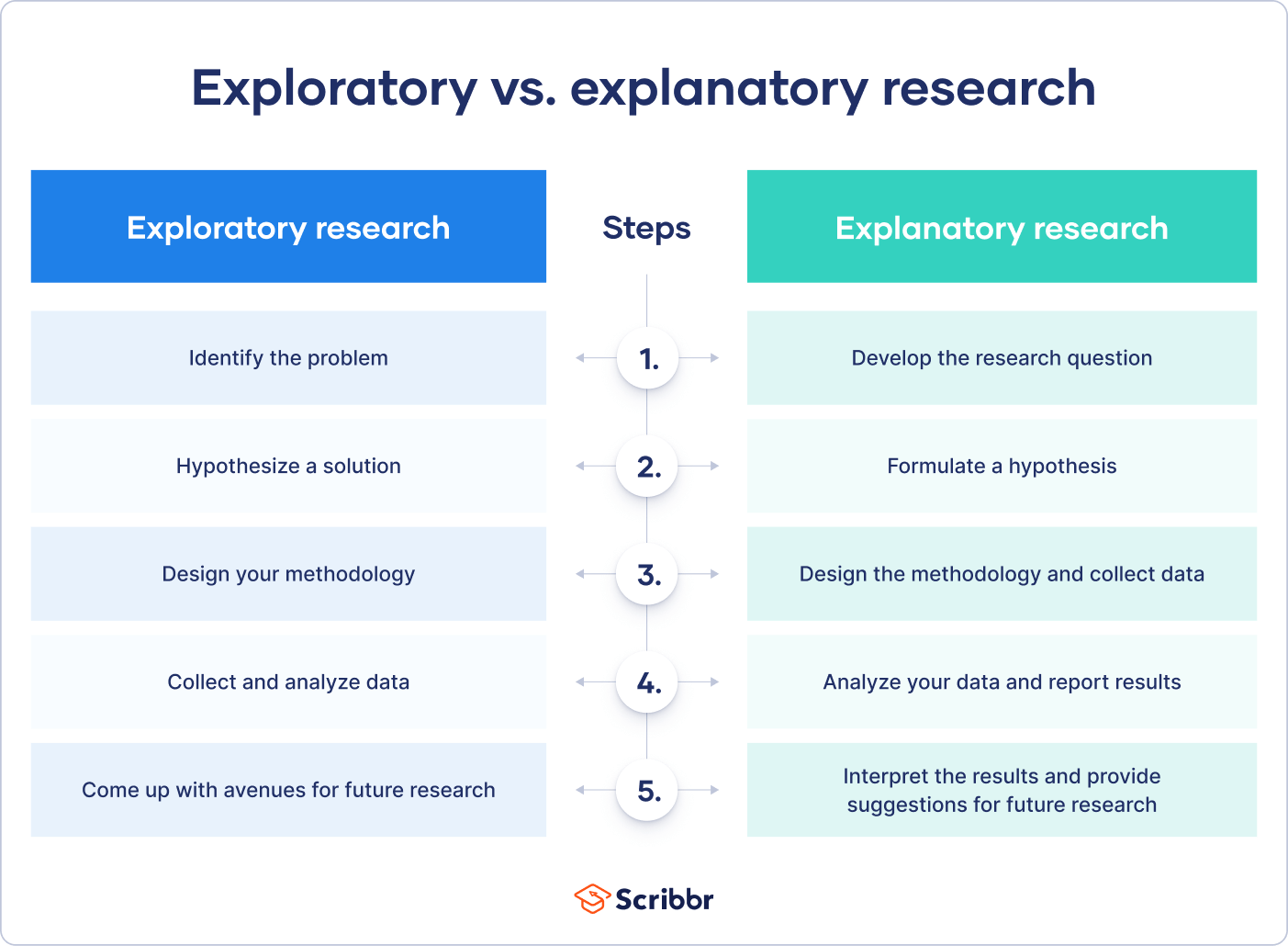
Like any other research design , explanatory research has its trade-offs: while it provides a unique set of benefits, it also has significant downsides:
- It gives more meaning to previous research. It helps fill in the gaps in existing analyses and provides information on the reasons behind phenomena.
- It is very flexible and often replicable , since the internal validity tends to be high when done correctly.
- As you can often use secondary research, explanatory research is often very cost- and time-effective, allowing you to utilize pre-existing resources to guide your research prior to committing to heavier analyses.
Disadvantages
- While explanatory research does help you solidify your theories and hypotheses, it usually lacks conclusive results.
- Results can be biased or inadmissible to a larger body of work and are not generally externally valid . You will likely have to conduct more robust (often quantitative ) research later to bolster any possible findings gleaned from explanatory research.
- Coincidences can be mistaken for causal relationships , and it can sometimes be challenging to ascertain which is the causal variable and which is the effect.
If you want to know more about statistics , methodology , or research bias , make sure to check out some of our other articles with explanations and examples.
- Normal distribution
- Degrees of freedom
- Null hypothesis
- Discourse analysis
- Control groups
- Mixed methods research
- Non-probability sampling
- Quantitative research
- Ecological validity
Research bias
- Rosenthal effect
- Implicit bias
- Cognitive bias
- Selection bias
- Negativity bias
- Status quo bias
Explanatory research is a research method used to investigate how or why something occurs when only a small amount of information is available pertaining to that topic. It can help you increase your understanding of a given topic.
Exploratory research aims to explore the main aspects of an under-researched problem, while explanatory research aims to explain the causes and consequences of a well-defined problem.
Explanatory research is used to investigate how or why a phenomenon occurs. Therefore, this type of research is often one of the first stages in the research process , serving as a jumping-off point for future research.
Quantitative research deals with numbers and statistics, while qualitative research deals with words and meanings.
Quantitative methods allow you to systematically measure variables and test hypotheses . Qualitative methods allow you to explore concepts and experiences in more detail.
Cite this Scribbr article
If you want to cite this source, you can copy and paste the citation or click the “Cite this Scribbr article” button to automatically add the citation to our free Citation Generator.
George, T. & Merkus, J. (2023, November 20). Explanatory Research | Definition, Guide, & Examples. Scribbr. Retrieved July 10, 2024, from https://www.scribbr.com/methodology/explanatory-research/
Is this article helpful?
Tegan George
Other students also liked, exploratory research | definition, guide, & examples, what is a research design | types, guide & examples, qualitative vs. quantitative research | differences, examples & methods, get unlimited documents corrected.
✔ Free APA citation check included ✔ Unlimited document corrections ✔ Specialized in correcting academic texts
- USC Libraries
- Research Guides
Organizing Your Social Sciences Research Paper
- Types of Research Designs
- Purpose of Guide
- Design Flaws to Avoid
- Independent and Dependent Variables
- Glossary of Research Terms
- Reading Research Effectively
- Narrowing a Topic Idea
- Broadening a Topic Idea
- Extending the Timeliness of a Topic Idea
- Academic Writing Style
- Applying Critical Thinking
- Choosing a Title
- Making an Outline
- Paragraph Development
- Research Process Video Series
- Executive Summary
- The C.A.R.S. Model
- Background Information
- The Research Problem/Question
- Theoretical Framework
- Citation Tracking
- Content Alert Services
- Evaluating Sources
- Primary Sources
- Secondary Sources
- Tiertiary Sources
- Scholarly vs. Popular Publications
- Qualitative Methods
- Quantitative Methods
- Insiderness
- Using Non-Textual Elements
- Limitations of the Study
- Common Grammar Mistakes
- Writing Concisely
- Avoiding Plagiarism
- Footnotes or Endnotes?
- Further Readings
- Generative AI and Writing
- USC Libraries Tutorials and Other Guides
- Bibliography
Introduction
Before beginning your paper, you need to decide how you plan to design the study .
The research design refers to the overall strategy and analytical approach that you have chosen in order to integrate, in a coherent and logical way, the different components of the study, thus ensuring that the research problem will be thoroughly investigated. It constitutes the blueprint for the collection, measurement, and interpretation of information and data. Note that the research problem determines the type of design you choose, not the other way around!
De Vaus, D. A. Research Design in Social Research . London: SAGE, 2001; Trochim, William M.K. Research Methods Knowledge Base. 2006.
General Structure and Writing Style
The function of a research design is to ensure that the evidence obtained enables you to effectively address the research problem logically and as unambiguously as possible . In social sciences research, obtaining information relevant to the research problem generally entails specifying the type of evidence needed to test the underlying assumptions of a theory, to evaluate a program, or to accurately describe and assess meaning related to an observable phenomenon.
With this in mind, a common mistake made by researchers is that they begin their investigations before they have thought critically about what information is required to address the research problem. Without attending to these design issues beforehand, the overall research problem will not be adequately addressed and any conclusions drawn will run the risk of being weak and unconvincing. As a consequence, the overall validity of the study will be undermined.
The length and complexity of describing the research design in your paper can vary considerably, but any well-developed description will achieve the following :
- Identify the research problem clearly and justify its selection, particularly in relation to any valid alternative designs that could have been used,
- Review and synthesize previously published literature associated with the research problem,
- Clearly and explicitly specify hypotheses [i.e., research questions] central to the problem,
- Effectively describe the information and/or data which will be necessary for an adequate testing of the hypotheses and explain how such information and/or data will be obtained, and
- Describe the methods of analysis to be applied to the data in determining whether or not the hypotheses are true or false.
The research design is usually incorporated into the introduction of your paper . You can obtain an overall sense of what to do by reviewing studies that have utilized the same research design [e.g., using a case study approach]. This can help you develop an outline to follow for your own paper.
NOTE: Use the SAGE Research Methods Online and Cases and the SAGE Research Methods Videos databases to search for scholarly resources on how to apply specific research designs and methods . The Research Methods Online database contains links to more than 175,000 pages of SAGE publisher's book, journal, and reference content on quantitative, qualitative, and mixed research methodologies. Also included is a collection of case studies of social research projects that can be used to help you better understand abstract or complex methodological concepts. The Research Methods Videos database contains hours of tutorials, interviews, video case studies, and mini-documentaries covering the entire research process.
Creswell, John W. and J. David Creswell. Research Design: Qualitative, Quantitative, and Mixed Methods Approaches . 5th edition. Thousand Oaks, CA: Sage, 2018; De Vaus, D. A. Research Design in Social Research . London: SAGE, 2001; Gorard, Stephen. Research Design: Creating Robust Approaches for the Social Sciences . Thousand Oaks, CA: Sage, 2013; Leedy, Paul D. and Jeanne Ellis Ormrod. Practical Research: Planning and Design . Tenth edition. Boston, MA: Pearson, 2013; Vogt, W. Paul, Dianna C. Gardner, and Lynne M. Haeffele. When to Use What Research Design . New York: Guilford, 2012.
Action Research Design
Definition and Purpose
The essentials of action research design follow a characteristic cycle whereby initially an exploratory stance is adopted, where an understanding of a problem is developed and plans are made for some form of interventionary strategy. Then the intervention is carried out [the "action" in action research] during which time, pertinent observations are collected in various forms. The new interventional strategies are carried out, and this cyclic process repeats, continuing until a sufficient understanding of [or a valid implementation solution for] the problem is achieved. The protocol is iterative or cyclical in nature and is intended to foster deeper understanding of a given situation, starting with conceptualizing and particularizing the problem and moving through several interventions and evaluations.
What do these studies tell you ?
- This is a collaborative and adaptive research design that lends itself to use in work or community situations.
- Design focuses on pragmatic and solution-driven research outcomes rather than testing theories.
- When practitioners use action research, it has the potential to increase the amount they learn consciously from their experience; the action research cycle can be regarded as a learning cycle.
- Action research studies often have direct and obvious relevance to improving practice and advocating for change.
- There are no hidden controls or preemption of direction by the researcher.
What these studies don't tell you ?
- It is harder to do than conducting conventional research because the researcher takes on responsibilities of advocating for change as well as for researching the topic.
- Action research is much harder to write up because it is less likely that you can use a standard format to report your findings effectively [i.e., data is often in the form of stories or observation].
- Personal over-involvement of the researcher may bias research results.
- The cyclic nature of action research to achieve its twin outcomes of action [e.g. change] and research [e.g. understanding] is time-consuming and complex to conduct.
- Advocating for change usually requires buy-in from study participants.
Coghlan, David and Mary Brydon-Miller. The Sage Encyclopedia of Action Research . Thousand Oaks, CA: Sage, 2014; Efron, Sara Efrat and Ruth Ravid. Action Research in Education: A Practical Guide . New York: Guilford, 2013; Gall, Meredith. Educational Research: An Introduction . Chapter 18, Action Research. 8th ed. Boston, MA: Pearson/Allyn and Bacon, 2007; Gorard, Stephen. Research Design: Creating Robust Approaches for the Social Sciences . Thousand Oaks, CA: Sage, 2013; Kemmis, Stephen and Robin McTaggart. “Participatory Action Research.” In Handbook of Qualitative Research . Norman Denzin and Yvonna S. Lincoln, eds. 2nd ed. (Thousand Oaks, CA: SAGE, 2000), pp. 567-605; McNiff, Jean. Writing and Doing Action Research . London: Sage, 2014; Reason, Peter and Hilary Bradbury. Handbook of Action Research: Participative Inquiry and Practice . Thousand Oaks, CA: SAGE, 2001.
Case Study Design
A case study is an in-depth study of a particular research problem rather than a sweeping statistical survey or comprehensive comparative inquiry. It is often used to narrow down a very broad field of research into one or a few easily researchable examples. The case study research design is also useful for testing whether a specific theory and model actually applies to phenomena in the real world. It is a useful design when not much is known about an issue or phenomenon.
- Approach excels at bringing us to an understanding of a complex issue through detailed contextual analysis of a limited number of events or conditions and their relationships.
- A researcher using a case study design can apply a variety of methodologies and rely on a variety of sources to investigate a research problem.
- Design can extend experience or add strength to what is already known through previous research.
- Social scientists, in particular, make wide use of this research design to examine contemporary real-life situations and provide the basis for the application of concepts and theories and the extension of methodologies.
- The design can provide detailed descriptions of specific and rare cases.
- A single or small number of cases offers little basis for establishing reliability or to generalize the findings to a wider population of people, places, or things.
- Intense exposure to the study of a case may bias a researcher's interpretation of the findings.
- Design does not facilitate assessment of cause and effect relationships.
- Vital information may be missing, making the case hard to interpret.
- The case may not be representative or typical of the larger problem being investigated.
- If the criteria for selecting a case is because it represents a very unusual or unique phenomenon or problem for study, then your interpretation of the findings can only apply to that particular case.
Case Studies. Writing@CSU. Colorado State University; Anastas, Jeane W. Research Design for Social Work and the Human Services . Chapter 4, Flexible Methods: Case Study Design. 2nd ed. New York: Columbia University Press, 1999; Gerring, John. “What Is a Case Study and What Is It Good for?” American Political Science Review 98 (May 2004): 341-354; Greenhalgh, Trisha, editor. Case Study Evaluation: Past, Present and Future Challenges . Bingley, UK: Emerald Group Publishing, 2015; Mills, Albert J. , Gabrielle Durepos, and Eiden Wiebe, editors. Encyclopedia of Case Study Research . Thousand Oaks, CA: SAGE Publications, 2010; Stake, Robert E. The Art of Case Study Research . Thousand Oaks, CA: SAGE, 1995; Yin, Robert K. Case Study Research: Design and Theory . Applied Social Research Methods Series, no. 5. 3rd ed. Thousand Oaks, CA: SAGE, 2003.
Causal Design
Causality studies may be thought of as understanding a phenomenon in terms of conditional statements in the form, “If X, then Y.” This type of research is used to measure what impact a specific change will have on existing norms and assumptions. Most social scientists seek causal explanations that reflect tests of hypotheses. Causal effect (nomothetic perspective) occurs when variation in one phenomenon, an independent variable, leads to or results, on average, in variation in another phenomenon, the dependent variable.
Conditions necessary for determining causality:
- Empirical association -- a valid conclusion is based on finding an association between the independent variable and the dependent variable.
- Appropriate time order -- to conclude that causation was involved, one must see that cases were exposed to variation in the independent variable before variation in the dependent variable.
- Nonspuriousness -- a relationship between two variables that is not due to variation in a third variable.
- Causality research designs assist researchers in understanding why the world works the way it does through the process of proving a causal link between variables and by the process of eliminating other possibilities.
- Replication is possible.
- There is greater confidence the study has internal validity due to the systematic subject selection and equity of groups being compared.
- Not all relationships are causal! The possibility always exists that, by sheer coincidence, two unrelated events appear to be related [e.g., Punxatawney Phil could accurately predict the duration of Winter for five consecutive years but, the fact remains, he's just a big, furry rodent].
- Conclusions about causal relationships are difficult to determine due to a variety of extraneous and confounding variables that exist in a social environment. This means causality can only be inferred, never proven.
- If two variables are correlated, the cause must come before the effect. However, even though two variables might be causally related, it can sometimes be difficult to determine which variable comes first and, therefore, to establish which variable is the actual cause and which is the actual effect.
Beach, Derek and Rasmus Brun Pedersen. Causal Case Study Methods: Foundations and Guidelines for Comparing, Matching, and Tracing . Ann Arbor, MI: University of Michigan Press, 2016; Bachman, Ronet. The Practice of Research in Criminology and Criminal Justice . Chapter 5, Causation and Research Designs. 3rd ed. Thousand Oaks, CA: Pine Forge Press, 2007; Brewer, Ernest W. and Jennifer Kubn. “Causal-Comparative Design.” In Encyclopedia of Research Design . Neil J. Salkind, editor. (Thousand Oaks, CA: Sage, 2010), pp. 125-132; Causal Research Design: Experimentation. Anonymous SlideShare Presentation; Gall, Meredith. Educational Research: An Introduction . Chapter 11, Nonexperimental Research: Correlational Designs. 8th ed. Boston, MA: Pearson/Allyn and Bacon, 2007; Trochim, William M.K. Research Methods Knowledge Base. 2006.
Cohort Design
Often used in the medical sciences, but also found in the applied social sciences, a cohort study generally refers to a study conducted over a period of time involving members of a population which the subject or representative member comes from, and who are united by some commonality or similarity. Using a quantitative framework, a cohort study makes note of statistical occurrence within a specialized subgroup, united by same or similar characteristics that are relevant to the research problem being investigated, rather than studying statistical occurrence within the general population. Using a qualitative framework, cohort studies generally gather data using methods of observation. Cohorts can be either "open" or "closed."
- Open Cohort Studies [dynamic populations, such as the population of Los Angeles] involve a population that is defined just by the state of being a part of the study in question (and being monitored for the outcome). Date of entry and exit from the study is individually defined, therefore, the size of the study population is not constant. In open cohort studies, researchers can only calculate rate based data, such as, incidence rates and variants thereof.
- Closed Cohort Studies [static populations, such as patients entered into a clinical trial] involve participants who enter into the study at one defining point in time and where it is presumed that no new participants can enter the cohort. Given this, the number of study participants remains constant (or can only decrease).
- The use of cohorts is often mandatory because a randomized control study may be unethical. For example, you cannot deliberately expose people to asbestos, you can only study its effects on those who have already been exposed. Research that measures risk factors often relies upon cohort designs.
- Because cohort studies measure potential causes before the outcome has occurred, they can demonstrate that these “causes” preceded the outcome, thereby avoiding the debate as to which is the cause and which is the effect.
- Cohort analysis is highly flexible and can provide insight into effects over time and related to a variety of different types of changes [e.g., social, cultural, political, economic, etc.].
- Either original data or secondary data can be used in this design.
- In cases where a comparative analysis of two cohorts is made [e.g., studying the effects of one group exposed to asbestos and one that has not], a researcher cannot control for all other factors that might differ between the two groups. These factors are known as confounding variables.
- Cohort studies can end up taking a long time to complete if the researcher must wait for the conditions of interest to develop within the group. This also increases the chance that key variables change during the course of the study, potentially impacting the validity of the findings.
- Due to the lack of randominization in the cohort design, its external validity is lower than that of study designs where the researcher randomly assigns participants.
Healy P, Devane D. “Methodological Considerations in Cohort Study Designs.” Nurse Researcher 18 (2011): 32-36; Glenn, Norval D, editor. Cohort Analysis . 2nd edition. Thousand Oaks, CA: Sage, 2005; Levin, Kate Ann. Study Design IV: Cohort Studies. Evidence-Based Dentistry 7 (2003): 51–52; Payne, Geoff. “Cohort Study.” In The SAGE Dictionary of Social Research Methods . Victor Jupp, editor. (Thousand Oaks, CA: Sage, 2006), pp. 31-33; Study Design 101. Himmelfarb Health Sciences Library. George Washington University, November 2011; Cohort Study. Wikipedia.
Cross-Sectional Design
Cross-sectional research designs have three distinctive features: no time dimension; a reliance on existing differences rather than change following intervention; and, groups are selected based on existing differences rather than random allocation. The cross-sectional design can only measure differences between or from among a variety of people, subjects, or phenomena rather than a process of change. As such, researchers using this design can only employ a relatively passive approach to making causal inferences based on findings.
- Cross-sectional studies provide a clear 'snapshot' of the outcome and the characteristics associated with it, at a specific point in time.
- Unlike an experimental design, where there is an active intervention by the researcher to produce and measure change or to create differences, cross-sectional designs focus on studying and drawing inferences from existing differences between people, subjects, or phenomena.
- Entails collecting data at and concerning one point in time. While longitudinal studies involve taking multiple measures over an extended period of time, cross-sectional research is focused on finding relationships between variables at one moment in time.
- Groups identified for study are purposely selected based upon existing differences in the sample rather than seeking random sampling.
- Cross-section studies are capable of using data from a large number of subjects and, unlike observational studies, is not geographically bound.
- Can estimate prevalence of an outcome of interest because the sample is usually taken from the whole population.
- Because cross-sectional designs generally use survey techniques to gather data, they are relatively inexpensive and take up little time to conduct.
- Finding people, subjects, or phenomena to study that are very similar except in one specific variable can be difficult.
- Results are static and time bound and, therefore, give no indication of a sequence of events or reveal historical or temporal contexts.
- Studies cannot be utilized to establish cause and effect relationships.
- This design only provides a snapshot of analysis so there is always the possibility that a study could have differing results if another time-frame had been chosen.
- There is no follow up to the findings.
Bethlehem, Jelke. "7: Cross-sectional Research." In Research Methodology in the Social, Behavioural and Life Sciences . Herman J Adèr and Gideon J Mellenbergh, editors. (London, England: Sage, 1999), pp. 110-43; Bourque, Linda B. “Cross-Sectional Design.” In The SAGE Encyclopedia of Social Science Research Methods . Michael S. Lewis-Beck, Alan Bryman, and Tim Futing Liao. (Thousand Oaks, CA: 2004), pp. 230-231; Hall, John. “Cross-Sectional Survey Design.” In Encyclopedia of Survey Research Methods . Paul J. Lavrakas, ed. (Thousand Oaks, CA: Sage, 2008), pp. 173-174; Helen Barratt, Maria Kirwan. Cross-Sectional Studies: Design Application, Strengths and Weaknesses of Cross-Sectional Studies. Healthknowledge, 2009. Cross-Sectional Study. Wikipedia.
Descriptive Design
Descriptive research designs help provide answers to the questions of who, what, when, where, and how associated with a particular research problem; a descriptive study cannot conclusively ascertain answers to why. Descriptive research is used to obtain information concerning the current status of the phenomena and to describe "what exists" with respect to variables or conditions in a situation.
- The subject is being observed in a completely natural and unchanged natural environment. True experiments, whilst giving analyzable data, often adversely influence the normal behavior of the subject [a.k.a., the Heisenberg effect whereby measurements of certain systems cannot be made without affecting the systems].
- Descriptive research is often used as a pre-cursor to more quantitative research designs with the general overview giving some valuable pointers as to what variables are worth testing quantitatively.
- If the limitations are understood, they can be a useful tool in developing a more focused study.
- Descriptive studies can yield rich data that lead to important recommendations in practice.
- Appoach collects a large amount of data for detailed analysis.
- The results from a descriptive research cannot be used to discover a definitive answer or to disprove a hypothesis.
- Because descriptive designs often utilize observational methods [as opposed to quantitative methods], the results cannot be replicated.
- The descriptive function of research is heavily dependent on instrumentation for measurement and observation.
Anastas, Jeane W. Research Design for Social Work and the Human Services . Chapter 5, Flexible Methods: Descriptive Research. 2nd ed. New York: Columbia University Press, 1999; Given, Lisa M. "Descriptive Research." In Encyclopedia of Measurement and Statistics . Neil J. Salkind and Kristin Rasmussen, editors. (Thousand Oaks, CA: Sage, 2007), pp. 251-254; McNabb, Connie. Descriptive Research Methodologies. Powerpoint Presentation; Shuttleworth, Martyn. Descriptive Research Design, September 26, 2008; Erickson, G. Scott. "Descriptive Research Design." In New Methods of Market Research and Analysis . (Northampton, MA: Edward Elgar Publishing, 2017), pp. 51-77; Sahin, Sagufta, and Jayanta Mete. "A Brief Study on Descriptive Research: Its Nature and Application in Social Science." International Journal of Research and Analysis in Humanities 1 (2021): 11; K. Swatzell and P. Jennings. “Descriptive Research: The Nuts and Bolts.” Journal of the American Academy of Physician Assistants 20 (2007), pp. 55-56; Kane, E. Doing Your Own Research: Basic Descriptive Research in the Social Sciences and Humanities . London: Marion Boyars, 1985.
Experimental Design
A blueprint of the procedure that enables the researcher to maintain control over all factors that may affect the result of an experiment. In doing this, the researcher attempts to determine or predict what may occur. Experimental research is often used where there is time priority in a causal relationship (cause precedes effect), there is consistency in a causal relationship (a cause will always lead to the same effect), and the magnitude of the correlation is great. The classic experimental design specifies an experimental group and a control group. The independent variable is administered to the experimental group and not to the control group, and both groups are measured on the same dependent variable. Subsequent experimental designs have used more groups and more measurements over longer periods. True experiments must have control, randomization, and manipulation.
- Experimental research allows the researcher to control the situation. In so doing, it allows researchers to answer the question, “What causes something to occur?”
- Permits the researcher to identify cause and effect relationships between variables and to distinguish placebo effects from treatment effects.
- Experimental research designs support the ability to limit alternative explanations and to infer direct causal relationships in the study.
- Approach provides the highest level of evidence for single studies.
- The design is artificial, and results may not generalize well to the real world.
- The artificial settings of experiments may alter the behaviors or responses of participants.
- Experimental designs can be costly if special equipment or facilities are needed.
- Some research problems cannot be studied using an experiment because of ethical or technical reasons.
- Difficult to apply ethnographic and other qualitative methods to experimentally designed studies.
Anastas, Jeane W. Research Design for Social Work and the Human Services . Chapter 7, Flexible Methods: Experimental Research. 2nd ed. New York: Columbia University Press, 1999; Chapter 2: Research Design, Experimental Designs. School of Psychology, University of New England, 2000; Chow, Siu L. "Experimental Design." In Encyclopedia of Research Design . Neil J. Salkind, editor. (Thousand Oaks, CA: Sage, 2010), pp. 448-453; "Experimental Design." In Social Research Methods . Nicholas Walliman, editor. (London, England: Sage, 2006), pp, 101-110; Experimental Research. Research Methods by Dummies. Department of Psychology. California State University, Fresno, 2006; Kirk, Roger E. Experimental Design: Procedures for the Behavioral Sciences . 4th edition. Thousand Oaks, CA: Sage, 2013; Trochim, William M.K. Experimental Design. Research Methods Knowledge Base. 2006; Rasool, Shafqat. Experimental Research. Slideshare presentation.
Exploratory Design
An exploratory design is conducted about a research problem when there are few or no earlier studies to refer to or rely upon to predict an outcome . The focus is on gaining insights and familiarity for later investigation or undertaken when research problems are in a preliminary stage of investigation. Exploratory designs are often used to establish an understanding of how best to proceed in studying an issue or what methodology would effectively apply to gathering information about the issue.
The goals of exploratory research are intended to produce the following possible insights:
- Familiarity with basic details, settings, and concerns.
- Well grounded picture of the situation being developed.
- Generation of new ideas and assumptions.
- Development of tentative theories or hypotheses.
- Determination about whether a study is feasible in the future.
- Issues get refined for more systematic investigation and formulation of new research questions.
- Direction for future research and techniques get developed.
- Design is a useful approach for gaining background information on a particular topic.
- Exploratory research is flexible and can address research questions of all types (what, why, how).
- Provides an opportunity to define new terms and clarify existing concepts.
- Exploratory research is often used to generate formal hypotheses and develop more precise research problems.
- In the policy arena or applied to practice, exploratory studies help establish research priorities and where resources should be allocated.
- Exploratory research generally utilizes small sample sizes and, thus, findings are typically not generalizable to the population at large.
- The exploratory nature of the research inhibits an ability to make definitive conclusions about the findings. They provide insight but not definitive conclusions.
- The research process underpinning exploratory studies is flexible but often unstructured, leading to only tentative results that have limited value to decision-makers.
- Design lacks rigorous standards applied to methods of data gathering and analysis because one of the areas for exploration could be to determine what method or methodologies could best fit the research problem.
Cuthill, Michael. “Exploratory Research: Citizen Participation, Local Government, and Sustainable Development in Australia.” Sustainable Development 10 (2002): 79-89; Streb, Christoph K. "Exploratory Case Study." In Encyclopedia of Case Study Research . Albert J. Mills, Gabrielle Durepos and Eiden Wiebe, editors. (Thousand Oaks, CA: Sage, 2010), pp. 372-374; Taylor, P. J., G. Catalano, and D.R.F. Walker. “Exploratory Analysis of the World City Network.” Urban Studies 39 (December 2002): 2377-2394; Exploratory Research. Wikipedia.
Field Research Design
Sometimes referred to as ethnography or participant observation, designs around field research encompass a variety of interpretative procedures [e.g., observation and interviews] rooted in qualitative approaches to studying people individually or in groups while inhabiting their natural environment as opposed to using survey instruments or other forms of impersonal methods of data gathering. Information acquired from observational research takes the form of “ field notes ” that involves documenting what the researcher actually sees and hears while in the field. Findings do not consist of conclusive statements derived from numbers and statistics because field research involves analysis of words and observations of behavior. Conclusions, therefore, are developed from an interpretation of findings that reveal overriding themes, concepts, and ideas. More information can be found HERE .
- Field research is often necessary to fill gaps in understanding the research problem applied to local conditions or to specific groups of people that cannot be ascertained from existing data.
- The research helps contextualize already known information about a research problem, thereby facilitating ways to assess the origins, scope, and scale of a problem and to gage the causes, consequences, and means to resolve an issue based on deliberate interaction with people in their natural inhabited spaces.
- Enables the researcher to corroborate or confirm data by gathering additional information that supports or refutes findings reported in prior studies of the topic.
- Because the researcher in embedded in the field, they are better able to make observations or ask questions that reflect the specific cultural context of the setting being investigated.
- Observing the local reality offers the opportunity to gain new perspectives or obtain unique data that challenges existing theoretical propositions or long-standing assumptions found in the literature.
What these studies don't tell you
- A field research study requires extensive time and resources to carry out the multiple steps involved with preparing for the gathering of information, including for example, examining background information about the study site, obtaining permission to access the study site, and building trust and rapport with subjects.
- Requires a commitment to staying engaged in the field to ensure that you can adequately document events and behaviors as they unfold.
- The unpredictable nature of fieldwork means that researchers can never fully control the process of data gathering. They must maintain a flexible approach to studying the setting because events and circumstances can change quickly or unexpectedly.
- Findings can be difficult to interpret and verify without access to documents and other source materials that help to enhance the credibility of information obtained from the field [i.e., the act of triangulating the data].
- Linking the research problem to the selection of study participants inhabiting their natural environment is critical. However, this specificity limits the ability to generalize findings to different situations or in other contexts or to infer courses of action applied to other settings or groups of people.
- The reporting of findings must take into account how the researcher themselves may have inadvertently affected respondents and their behaviors.
Historical Design
The purpose of a historical research design is to collect, verify, and synthesize evidence from the past to establish facts that defend or refute a hypothesis. It uses secondary sources and a variety of primary documentary evidence, such as, diaries, official records, reports, archives, and non-textual information [maps, pictures, audio and visual recordings]. The limitation is that the sources must be both authentic and valid.
- The historical research design is unobtrusive; the act of research does not affect the results of the study.
- The historical approach is well suited for trend analysis.
- Historical records can add important contextual background required to more fully understand and interpret a research problem.
- There is often no possibility of researcher-subject interaction that could affect the findings.
- Historical sources can be used over and over to study different research problems or to replicate a previous study.
- The ability to fulfill the aims of your research are directly related to the amount and quality of documentation available to understand the research problem.
- Since historical research relies on data from the past, there is no way to manipulate it to control for contemporary contexts.
- Interpreting historical sources can be very time consuming.
- The sources of historical materials must be archived consistently to ensure access. This may especially challenging for digital or online-only sources.
- Original authors bring their own perspectives and biases to the interpretation of past events and these biases are more difficult to ascertain in historical resources.
- Due to the lack of control over external variables, historical research is very weak with regard to the demands of internal validity.
- It is rare that the entirety of historical documentation needed to fully address a research problem is available for interpretation, therefore, gaps need to be acknowledged.
Howell, Martha C. and Walter Prevenier. From Reliable Sources: An Introduction to Historical Methods . Ithaca, NY: Cornell University Press, 2001; Lundy, Karen Saucier. "Historical Research." In The Sage Encyclopedia of Qualitative Research Methods . Lisa M. Given, editor. (Thousand Oaks, CA: Sage, 2008), pp. 396-400; Marius, Richard. and Melvin E. Page. A Short Guide to Writing about History . 9th edition. Boston, MA: Pearson, 2015; Savitt, Ronald. “Historical Research in Marketing.” Journal of Marketing 44 (Autumn, 1980): 52-58; Gall, Meredith. Educational Research: An Introduction . Chapter 16, Historical Research. 8th ed. Boston, MA: Pearson/Allyn and Bacon, 2007.
Longitudinal Design
A longitudinal study follows the same sample over time and makes repeated observations. For example, with longitudinal surveys, the same group of people is interviewed at regular intervals, enabling researchers to track changes over time and to relate them to variables that might explain why the changes occur. Longitudinal research designs describe patterns of change and help establish the direction and magnitude of causal relationships. Measurements are taken on each variable over two or more distinct time periods. This allows the researcher to measure change in variables over time. It is a type of observational study sometimes referred to as a panel study.
- Longitudinal data facilitate the analysis of the duration of a particular phenomenon.
- Enables survey researchers to get close to the kinds of causal explanations usually attainable only with experiments.
- The design permits the measurement of differences or change in a variable from one period to another [i.e., the description of patterns of change over time].
- Longitudinal studies facilitate the prediction of future outcomes based upon earlier factors.
- The data collection method may change over time.
- Maintaining the integrity of the original sample can be difficult over an extended period of time.
- It can be difficult to show more than one variable at a time.
- This design often needs qualitative research data to explain fluctuations in the results.
- A longitudinal research design assumes present trends will continue unchanged.
- It can take a long period of time to gather results.
- There is a need to have a large sample size and accurate sampling to reach representativness.
Anastas, Jeane W. Research Design for Social Work and the Human Services . Chapter 6, Flexible Methods: Relational and Longitudinal Research. 2nd ed. New York: Columbia University Press, 1999; Forgues, Bernard, and Isabelle Vandangeon-Derumez. "Longitudinal Analyses." In Doing Management Research . Raymond-Alain Thiétart and Samantha Wauchope, editors. (London, England: Sage, 2001), pp. 332-351; Kalaian, Sema A. and Rafa M. Kasim. "Longitudinal Studies." In Encyclopedia of Survey Research Methods . Paul J. Lavrakas, ed. (Thousand Oaks, CA: Sage, 2008), pp. 440-441; Menard, Scott, editor. Longitudinal Research . Thousand Oaks, CA: Sage, 2002; Ployhart, Robert E. and Robert J. Vandenberg. "Longitudinal Research: The Theory, Design, and Analysis of Change.” Journal of Management 36 (January 2010): 94-120; Longitudinal Study. Wikipedia.
Meta-Analysis Design
Meta-analysis is an analytical methodology designed to systematically evaluate and summarize the results from a number of individual studies, thereby, increasing the overall sample size and the ability of the researcher to study effects of interest. The purpose is to not simply summarize existing knowledge, but to develop a new understanding of a research problem using synoptic reasoning. The main objectives of meta-analysis include analyzing differences in the results among studies and increasing the precision by which effects are estimated. A well-designed meta-analysis depends upon strict adherence to the criteria used for selecting studies and the availability of information in each study to properly analyze their findings. Lack of information can severely limit the type of analyzes and conclusions that can be reached. In addition, the more dissimilarity there is in the results among individual studies [heterogeneity], the more difficult it is to justify interpretations that govern a valid synopsis of results. A meta-analysis needs to fulfill the following requirements to ensure the validity of your findings:
- Clearly defined description of objectives, including precise definitions of the variables and outcomes that are being evaluated;
- A well-reasoned and well-documented justification for identification and selection of the studies;
- Assessment and explicit acknowledgment of any researcher bias in the identification and selection of those studies;
- Description and evaluation of the degree of heterogeneity among the sample size of studies reviewed; and,
- Justification of the techniques used to evaluate the studies.
- Can be an effective strategy for determining gaps in the literature.
- Provides a means of reviewing research published about a particular topic over an extended period of time and from a variety of sources.
- Is useful in clarifying what policy or programmatic actions can be justified on the basis of analyzing research results from multiple studies.
- Provides a method for overcoming small sample sizes in individual studies that previously may have had little relationship to each other.
- Can be used to generate new hypotheses or highlight research problems for future studies.
- Small violations in defining the criteria used for content analysis can lead to difficult to interpret and/or meaningless findings.
- A large sample size can yield reliable, but not necessarily valid, results.
- A lack of uniformity regarding, for example, the type of literature reviewed, how methods are applied, and how findings are measured within the sample of studies you are analyzing, can make the process of synthesis difficult to perform.
- Depending on the sample size, the process of reviewing and synthesizing multiple studies can be very time consuming.
Beck, Lewis W. "The Synoptic Method." The Journal of Philosophy 36 (1939): 337-345; Cooper, Harris, Larry V. Hedges, and Jeffrey C. Valentine, eds. The Handbook of Research Synthesis and Meta-Analysis . 2nd edition. New York: Russell Sage Foundation, 2009; Guzzo, Richard A., Susan E. Jackson and Raymond A. Katzell. “Meta-Analysis Analysis.” In Research in Organizational Behavior , Volume 9. (Greenwich, CT: JAI Press, 1987), pp 407-442; Lipsey, Mark W. and David B. Wilson. Practical Meta-Analysis . Thousand Oaks, CA: Sage Publications, 2001; Study Design 101. Meta-Analysis. The Himmelfarb Health Sciences Library, George Washington University; Timulak, Ladislav. “Qualitative Meta-Analysis.” In The SAGE Handbook of Qualitative Data Analysis . Uwe Flick, editor. (Los Angeles, CA: Sage, 2013), pp. 481-495; Walker, Esteban, Adrian V. Hernandez, and Micheal W. Kattan. "Meta-Analysis: It's Strengths and Limitations." Cleveland Clinic Journal of Medicine 75 (June 2008): 431-439.
Mixed-Method Design
- Narrative and non-textual information can add meaning to numeric data, while numeric data can add precision to narrative and non-textual information.
- Can utilize existing data while at the same time generating and testing a grounded theory approach to describe and explain the phenomenon under study.
- A broader, more complex research problem can be investigated because the researcher is not constrained by using only one method.
- The strengths of one method can be used to overcome the inherent weaknesses of another method.
- Can provide stronger, more robust evidence to support a conclusion or set of recommendations.
- May generate new knowledge new insights or uncover hidden insights, patterns, or relationships that a single methodological approach might not reveal.
- Produces more complete knowledge and understanding of the research problem that can be used to increase the generalizability of findings applied to theory or practice.
- A researcher must be proficient in understanding how to apply multiple methods to investigating a research problem as well as be proficient in optimizing how to design a study that coherently melds them together.
- Can increase the likelihood of conflicting results or ambiguous findings that inhibit drawing a valid conclusion or setting forth a recommended course of action [e.g., sample interview responses do not support existing statistical data].
- Because the research design can be very complex, reporting the findings requires a well-organized narrative, clear writing style, and precise word choice.
- Design invites collaboration among experts. However, merging different investigative approaches and writing styles requires more attention to the overall research process than studies conducted using only one methodological paradigm.
- Concurrent merging of quantitative and qualitative research requires greater attention to having adequate sample sizes, using comparable samples, and applying a consistent unit of analysis. For sequential designs where one phase of qualitative research builds on the quantitative phase or vice versa, decisions about what results from the first phase to use in the next phase, the choice of samples and estimating reasonable sample sizes for both phases, and the interpretation of results from both phases can be difficult.
- Due to multiple forms of data being collected and analyzed, this design requires extensive time and resources to carry out the multiple steps involved in data gathering and interpretation.
Burch, Patricia and Carolyn J. Heinrich. Mixed Methods for Policy Research and Program Evaluation . Thousand Oaks, CA: Sage, 2016; Creswell, John w. et al. Best Practices for Mixed Methods Research in the Health Sciences . Bethesda, MD: Office of Behavioral and Social Sciences Research, National Institutes of Health, 2010Creswell, John W. Research Design: Qualitative, Quantitative, and Mixed Methods Approaches . 4th edition. Thousand Oaks, CA: Sage Publications, 2014; Domínguez, Silvia, editor. Mixed Methods Social Networks Research . Cambridge, UK: Cambridge University Press, 2014; Hesse-Biber, Sharlene Nagy. Mixed Methods Research: Merging Theory with Practice . New York: Guilford Press, 2010; Niglas, Katrin. “How the Novice Researcher Can Make Sense of Mixed Methods Designs.” International Journal of Multiple Research Approaches 3 (2009): 34-46; Onwuegbuzie, Anthony J. and Nancy L. Leech. “Linking Research Questions to Mixed Methods Data Analysis Procedures.” The Qualitative Report 11 (September 2006): 474-498; Tashakorri, Abbas and John W. Creswell. “The New Era of Mixed Methods.” Journal of Mixed Methods Research 1 (January 2007): 3-7; Zhanga, Wanqing. “Mixed Methods Application in Health Intervention Research: A Multiple Case Study.” International Journal of Multiple Research Approaches 8 (2014): 24-35 .
Observational Design
This type of research design draws a conclusion by comparing subjects against a control group, in cases where the researcher has no control over the experiment. There are two general types of observational designs. In direct observations, people know that you are watching them. Unobtrusive measures involve any method for studying behavior where individuals do not know they are being observed. An observational study allows a useful insight into a phenomenon and avoids the ethical and practical difficulties of setting up a large and cumbersome research project.
- Observational studies are usually flexible and do not necessarily need to be structured around a hypothesis about what you expect to observe [data is emergent rather than pre-existing].
- The researcher is able to collect in-depth information about a particular behavior.
- Can reveal interrelationships among multifaceted dimensions of group interactions.
- You can generalize your results to real life situations.
- Observational research is useful for discovering what variables may be important before applying other methods like experiments.
- Observation research designs account for the complexity of group behaviors.
- Reliability of data is low because seeing behaviors occur over and over again may be a time consuming task and are difficult to replicate.
- In observational research, findings may only reflect a unique sample population and, thus, cannot be generalized to other groups.
- There can be problems with bias as the researcher may only "see what they want to see."
- There is no possibility to determine "cause and effect" relationships since nothing is manipulated.
- Sources or subjects may not all be equally credible.
- Any group that is knowingly studied is altered to some degree by the presence of the researcher, therefore, potentially skewing any data collected.
Atkinson, Paul and Martyn Hammersley. “Ethnography and Participant Observation.” In Handbook of Qualitative Research . Norman K. Denzin and Yvonna S. Lincoln, eds. (Thousand Oaks, CA: Sage, 1994), pp. 248-261; Observational Research. Research Methods by Dummies. Department of Psychology. California State University, Fresno, 2006; Patton Michael Quinn. Qualitiative Research and Evaluation Methods . Chapter 6, Fieldwork Strategies and Observational Methods. 3rd ed. Thousand Oaks, CA: Sage, 2002; Payne, Geoff and Judy Payne. "Observation." In Key Concepts in Social Research . The SAGE Key Concepts series. (London, England: Sage, 2004), pp. 158-162; Rosenbaum, Paul R. Design of Observational Studies . New York: Springer, 2010;Williams, J. Patrick. "Nonparticipant Observation." In The Sage Encyclopedia of Qualitative Research Methods . Lisa M. Given, editor.(Thousand Oaks, CA: Sage, 2008), pp. 562-563.
Philosophical Design
Understood more as an broad approach to examining a research problem than a methodological design, philosophical analysis and argumentation is intended to challenge deeply embedded, often intractable, assumptions underpinning an area of study. This approach uses the tools of argumentation derived from philosophical traditions, concepts, models, and theories to critically explore and challenge, for example, the relevance of logic and evidence in academic debates, to analyze arguments about fundamental issues, or to discuss the root of existing discourse about a research problem. These overarching tools of analysis can be framed in three ways:
- Ontology -- the study that describes the nature of reality; for example, what is real and what is not, what is fundamental and what is derivative?
- Epistemology -- the study that explores the nature of knowledge; for example, by what means does knowledge and understanding depend upon and how can we be certain of what we know?
- Axiology -- the study of values; for example, what values does an individual or group hold and why? How are values related to interest, desire, will, experience, and means-to-end? And, what is the difference between a matter of fact and a matter of value?
- Can provide a basis for applying ethical decision-making to practice.
- Functions as a means of gaining greater self-understanding and self-knowledge about the purposes of research.
- Brings clarity to general guiding practices and principles of an individual or group.
- Philosophy informs methodology.
- Refine concepts and theories that are invoked in relatively unreflective modes of thought and discourse.
- Beyond methodology, philosophy also informs critical thinking about epistemology and the structure of reality (metaphysics).
- Offers clarity and definition to the practical and theoretical uses of terms, concepts, and ideas.
- Limited application to specific research problems [answering the "So What?" question in social science research].
- Analysis can be abstract, argumentative, and limited in its practical application to real-life issues.
- While a philosophical analysis may render problematic that which was once simple or taken-for-granted, the writing can be dense and subject to unnecessary jargon, overstatement, and/or excessive quotation and documentation.
- There are limitations in the use of metaphor as a vehicle of philosophical analysis.
- There can be analytical difficulties in moving from philosophy to advocacy and between abstract thought and application to the phenomenal world.
Burton, Dawn. "Part I, Philosophy of the Social Sciences." In Research Training for Social Scientists . (London, England: Sage, 2000), pp. 1-5; Chapter 4, Research Methodology and Design. Unisa Institutional Repository (UnisaIR), University of South Africa; Jarvie, Ian C., and Jesús Zamora-Bonilla, editors. The SAGE Handbook of the Philosophy of Social Sciences . London: Sage, 2011; Labaree, Robert V. and Ross Scimeca. “The Philosophical Problem of Truth in Librarianship.” The Library Quarterly 78 (January 2008): 43-70; Maykut, Pamela S. Beginning Qualitative Research: A Philosophic and Practical Guide . Washington, DC: Falmer Press, 1994; McLaughlin, Hugh. "The Philosophy of Social Research." In Understanding Social Work Research . 2nd edition. (London: SAGE Publications Ltd., 2012), pp. 24-47; Stanford Encyclopedia of Philosophy . Metaphysics Research Lab, CSLI, Stanford University, 2013.
Sequential Design
- The researcher has a limitless option when it comes to sample size and the sampling schedule.
- Due to the repetitive nature of this research design, minor changes and adjustments can be done during the initial parts of the study to correct and hone the research method.
- This is a useful design for exploratory studies.
- There is very little effort on the part of the researcher when performing this technique. It is generally not expensive, time consuming, or workforce intensive.
- Because the study is conducted serially, the results of one sample are known before the next sample is taken and analyzed. This provides opportunities for continuous improvement of sampling and methods of analysis.
- The sampling method is not representative of the entire population. The only possibility of approaching representativeness is when the researcher chooses to use a very large sample size significant enough to represent a significant portion of the entire population. In this case, moving on to study a second or more specific sample can be difficult.
- The design cannot be used to create conclusions and interpretations that pertain to an entire population because the sampling technique is not randomized. Generalizability from findings is, therefore, limited.
- Difficult to account for and interpret variation from one sample to another over time, particularly when using qualitative methods of data collection.
Betensky, Rebecca. Harvard University, Course Lecture Note slides; Bovaird, James A. and Kevin A. Kupzyk. "Sequential Design." In Encyclopedia of Research Design . Neil J. Salkind, editor. (Thousand Oaks, CA: Sage, 2010), pp. 1347-1352; Cresswell, John W. Et al. “Advanced Mixed-Methods Research Designs.” In Handbook of Mixed Methods in Social and Behavioral Research . Abbas Tashakkori and Charles Teddle, eds. (Thousand Oaks, CA: Sage, 2003), pp. 209-240; Henry, Gary T. "Sequential Sampling." In The SAGE Encyclopedia of Social Science Research Methods . Michael S. Lewis-Beck, Alan Bryman and Tim Futing Liao, editors. (Thousand Oaks, CA: Sage, 2004), pp. 1027-1028; Nataliya V. Ivankova. “Using Mixed-Methods Sequential Explanatory Design: From Theory to Practice.” Field Methods 18 (February 2006): 3-20; Bovaird, James A. and Kevin A. Kupzyk. “Sequential Design.” In Encyclopedia of Research Design . Neil J. Salkind, ed. Thousand Oaks, CA: Sage, 2010; Sequential Analysis. Wikipedia.
Systematic Review
- A systematic review synthesizes the findings of multiple studies related to each other by incorporating strategies of analysis and interpretation intended to reduce biases and random errors.
- The application of critical exploration, evaluation, and synthesis methods separates insignificant, unsound, or redundant research from the most salient and relevant studies worthy of reflection.
- They can be use to identify, justify, and refine hypotheses, recognize and avoid hidden problems in prior studies, and explain data inconsistencies and conflicts in data.
- Systematic reviews can be used to help policy makers formulate evidence-based guidelines and regulations.
- The use of strict, explicit, and pre-determined methods of synthesis, when applied appropriately, provide reliable estimates about the effects of interventions, evaluations, and effects related to the overarching research problem investigated by each study under review.
- Systematic reviews illuminate where knowledge or thorough understanding of a research problem is lacking and, therefore, can then be used to guide future research.
- The accepted inclusion of unpublished studies [i.e., grey literature] ensures the broadest possible way to analyze and interpret research on a topic.
- Results of the synthesis can be generalized and the findings extrapolated into the general population with more validity than most other types of studies .
- Systematic reviews do not create new knowledge per se; they are a method for synthesizing existing studies about a research problem in order to gain new insights and determine gaps in the literature.
- The way researchers have carried out their investigations [e.g., the period of time covered, number of participants, sources of data analyzed, etc.] can make it difficult to effectively synthesize studies.
- The inclusion of unpublished studies can introduce bias into the review because they may not have undergone a rigorous peer-review process prior to publication. Examples may include conference presentations or proceedings, publications from government agencies, white papers, working papers, and internal documents from organizations, and doctoral dissertations and Master's theses.
Denyer, David and David Tranfield. "Producing a Systematic Review." In The Sage Handbook of Organizational Research Methods . David A. Buchanan and Alan Bryman, editors. ( Thousand Oaks, CA: Sage Publications, 2009), pp. 671-689; Foster, Margaret J. and Sarah T. Jewell, editors. Assembling the Pieces of a Systematic Review: A Guide for Librarians . Lanham, MD: Rowman and Littlefield, 2017; Gough, David, Sandy Oliver, James Thomas, editors. Introduction to Systematic Reviews . 2nd edition. Los Angeles, CA: Sage Publications, 2017; Gopalakrishnan, S. and P. Ganeshkumar. “Systematic Reviews and Meta-analysis: Understanding the Best Evidence in Primary Healthcare.” Journal of Family Medicine and Primary Care 2 (2013): 9-14; Gough, David, James Thomas, and Sandy Oliver. "Clarifying Differences between Review Designs and Methods." Systematic Reviews 1 (2012): 1-9; Khan, Khalid S., Regina Kunz, Jos Kleijnen, and Gerd Antes. “Five Steps to Conducting a Systematic Review.” Journal of the Royal Society of Medicine 96 (2003): 118-121; Mulrow, C. D. “Systematic Reviews: Rationale for Systematic Reviews.” BMJ 309:597 (September 1994); O'Dwyer, Linda C., and Q. Eileen Wafford. "Addressing Challenges with Systematic Review Teams through Effective Communication: A Case Report." Journal of the Medical Library Association 109 (October 2021): 643-647; Okoli, Chitu, and Kira Schabram. "A Guide to Conducting a Systematic Literature Review of Information Systems Research." Sprouts: Working Papers on Information Systems 10 (2010); Siddaway, Andy P., Alex M. Wood, and Larry V. Hedges. "How to Do a Systematic Review: A Best Practice Guide for Conducting and Reporting Narrative Reviews, Meta-analyses, and Meta-syntheses." Annual Review of Psychology 70 (2019): 747-770; Torgerson, Carole J. “Publication Bias: The Achilles’ Heel of Systematic Reviews?” British Journal of Educational Studies 54 (March 2006): 89-102; Torgerson, Carole. Systematic Reviews . New York: Continuum, 2003.
- << Previous: Purpose of Guide
- Next: Design Flaws to Avoid >>
- Last Updated: Jul 3, 2024 10:07 AM
- URL: https://libguides.usc.edu/writingguide
Tell cause from effect: models and evaluation
- Regular Paper
- Published: 21 July 2017
- Volume 4 , pages 99–112, ( 2017 )
Cite this article
- Jing Song 1 ,
- Satoshi Oyama 1 &
- Masahito Kurihara 1
4938 Accesses
Explore all metrics
Causal relationships differ from statistical relationships, and distinguishing cause from effect is a fundamental scientific problem that has attracted the interest of many researchers. Among causal discovery problems, discovering bivariate causal relationships is a special case. Causal relationships between two variables (“X causes Y” or “Y causes X”) belong to the same Markov equivalence class, and the well-known independence tests and conditional independence tests cannot distinguish directed acyclic graphs in the same Markov equivalence class. We empirically evaluated the performance of three state-of-the-art models for causal discovery in the bivariate case using both simulation and real-world data: the additive-noise model (ANM), the post-nonlinear (PNL) model, and the information geometric causal inference (IGCI) model. The performance metrics were accuracy, area under the ROC curve, and time to make a decision. The IGCI model was the fastest in terms of algorithm efficiency even when the dataset was large, while the PNL model took the most time to make a decision. In terms of decision accuracy, the IGCI model was susceptible to noise and thus performed well only under low-noise conditions. The PNL model was the most robust to noise. Simulation experiments showed that the IGCI model was the most susceptible to “confounding,” while the ANM and PNL models were able to avoid the effects of confounding to some degree.
Similar content being viewed by others
The Effect of Noise Level on the Accuracy of Causal Discovery Methods with Additive Noise Models
On the Relations of Theoretical Foundations of Different Causal Inference Algorithms
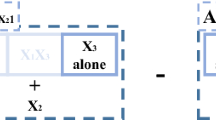
Data-driven discovery of causal interactions
Avoid common mistakes on your manuscript.
1 Introduction
People are generally more concerned with causal relationships between variables than with statistical relationships between variables, and the concept of causality has been widely discussed [ 9 , 12 ]. The best way to demonstrate a causal relationship between variables is to conduct a controlled randomized experiment. However, real-world experiments are often expensive, unethical, or even impossible. Many researchers working in various fields (economics, sociology, machine learning, etc.) are thus using statistical methods to analyze causal relationships between variables [ 2 , 3 , 16 , 19 , 21 , 25 , 31 , 37 , 48 , 55 ].
Possible relationships between X and Y. a Independent. b Feedback. c X causes Y. d Y causes X. e “common cause.” f “selection bias”
Directed acyclic graphs (DAGs) have been used to formalize the concept of causality [ 29 ]. Although a conditional independence test cannot tell the full story of a causal relationship, it can be used to exclude irrelevant relationships between variables [ 29 , 44 ]. However, a conditional independence test is impossible when there are only two variables. Several models have been proposed to solve this problem [ 20 , 38 , 46 , 47 , 49 ]. For two variables X and Y, there are at least six possible relationships between them (Fig. 1 ). The top two diagrams show the independent case and the feedback case, respectively. The middle two show the two possible causal relationships between X and Y: “X causes Y” and “Y causes X.” The bottom two show the “common cause” case and the “selection bias” case. Unobserved variables Z are “confounders” Footnote 1 for causal discovery between X and Y. The existence of “confounders” Footnote 2 creates a spurious correlation between X and Y. Distinguishing spurious correlation due to unobserved confounders from actual causality remains a challenging task in the field of causal discovery. Many models are based on the assumption that no unobserved confounders exist. Footnote 3
In the work reported here, we experimentally compared the performance of three state-of-the-art causal discovery model, the additive-noise model (ANM) [ 15 ], the post-nonlinear (PNL) model [ 54 ], and the information geometric causal inference (IGCI) model [ 22 ]. We used three metrics: accuracy, area under the ROC curve (AUC), and time to make a decision. This paper is an extended and revised version of our conference paper [ 23 ]. It includes new AUC results and the updated time to make a decision for the PNL model. It also describes typical examples of model failure and discusses the reasons for failure. Finally, it describes new experiments on the responses of the models to spurious correlation caused by confounders using simulation and real-world data.
In Sect. 2 , we discuss related work in the field of causal discovery. In Sect. 3 , we briefly describe the three models. In Sect. 4 , we describe the dataset we used and the implementations of the three models. In Sect. 5 , we present the results and give a detailed analysis of the performances of the three models. We conclude in Sect. 6 by summarizing the strengths and weaknesses of the three models and mentioning future tasks.
2 Related work
Temporal information is useful for causal discovery modeling [ 30 , 32 ]. Granger [ 7 ] proposed detecting the causal direction of time series data on the basis of the temporal ordering of the variables and used linear systems to make it more operational. He formulated the definition of causality in terms of conditional independence relations [ 8 ]. Chen et al. extended the linear stochastic systems he proposed [ 7 ] to work on nonlinear systems [ 1 ]. Shajarisales et al. [ 39 ] proposed using the spectral independence criterion (SIC) for causal inference from time series data and a mechanism different from that used in Granger causality and compared the two methods. For Granger causality [ 7 ] and extended Granger causality [ 1 ], temporal information is needed. When discovering causal relationship from time series data, the data resolution might be different from the true causal frequency. Gong et al. [ 6 ] discussed this issue and showed that using non-Gaussian of data can help identify the underlying model under some conditions.
Shimizu et al. [ 41 ] proposed using a linear non-Gaussian acyclic model (LiNGAM for short) to detect the causal direction of variables without the need for temporal information. LiNGAM works when the causal relationship between variables is linear, the distributions of disturbance variables are non-Gaussian, and the network structure can be expressed using a DAG. Several extensions of LiNGAM have been proposed [ 14 , 18 , 40 , 42 ].
LiNGAM is based on the assumption of linear relationships between variables. Hoyer et al. [ 15 ] proposed using an additive- noise model (ANM) to deal with nonlinear relationships. If the regression function is linear, ANM works in the same way as LiNGAM. Zhang et al. [ 52 , 53 , 54 , 56 ] proposed using a PNL model that takes into account the nonlinear effect of causes, inner additive noise, and external sensor distortion. The ANM and PNL model are briefly introduced in the following section.
While the above models are based on structural equation modeling (SEM), which requires structural constraints on the data generating process, another research direction is based on the assumption that independent mechanisms in nature generate causes and effects. The idea is that the shortest description of joint distribution \(p(\mathrm {cause, effect})\) can be expressed by \(p(\mathrm {cause})p(\mathrm {effect|cause})\) . Compared with factorization into p (cause) p (effect|cause), p (effect) p (cause|effect) has lower total complexity. Although comparing total complexity is an intuitive idea, Kolmogorov complexity and algorithmic information could be used to measure it [ 19 ].
Janzing et al. [ 4 , 22 ] proposed IGCI to infer asymmetry between cause and effect through the complexity loss of distributions. The IGCI model is briefly introduced in the following section. Zhang et al. [ 57 ] proposed using a bootstrap-based approach to detect causal direction. It is based on the assumption that the parameters of the causes involved in the causality data generation process are exogenous to those of the cause to the effect. Stegle et al. [ 45 ] proposed using a probabilistic latent variable model (GPI) to distinguish between cause and effect using standard Bayesian model selection.
In addition to the above studies on the causal relationship between two variables, there have been several reviews. Spirtes et al. discussed the main concepts of causal discovery and introduced several models based on SEM [ 43 ]. Eberhardt [ 5 ] discussed the fundamental assumptions of causal discovery and gave an overview of related causal discovery methods. Several methods have been proposed for deciding the causal direction between two variables, and specific methods have been compared. However, as far as we know, there has been little discussion of how to fairly compare methods based on different assumptions. In the work described above, accuracy was usually used as the evaluation metric. Another commonly used metric for a binary classifier is the AUC. Compared with accuracy, the ROC curve can show the trade-off between the true-positive rate (TPR) and the false-positive rate (FPR) of a binary classifier. In our framework for causal discovery models, we use AUC as an evaluation metric. We have used it to obtain several new insights. We also used the time to make a decision as an evaluation metric since it may become a performance bottleneck when dealing with big data.
We used the ANM, the PNL model, and the IGCI model in the comparison experiments. The ANM and PNL model define how causality data are generated in nature through SEM. The assumption of additive noise is enlightening. The IGCI model finds the asymmetry between cause and effect through the complexity loss of distributions. The assumption of IGCI is intuitive and how well it works needs to be further researched.
The additive-noise model of Hoyer et al. [ 15 ] is based on two assumptions: (1) the observed effects (Y) can be expressed using functional models of the cause (X) and additive noise (N) (Eq. 1 ); (2) the cause and additive noise are independent. If f () is a linear function and the noise has a non-Gaussian distribution, the ANM works in the same way as LiNGAM [ 41 ]. The model is learned by performing regression in both directions and testing the independence between the assumed cause and noise (residuals) for each direction. The decision rule is to choose the direction with the less dependence as the true causal direction. The ANM cannot handle the linear Gaussian case since the data can fit the model in both directions, so the asymmetry between cause and effect disappears. Gretton et al. improved the algorithm and extended the ANM to work even in the linear Gaussian case [ 50 ]. The improved model also works more efficiently in the multivariate case.
3.2 PNL model
In the post-nonlinear model of Zhang et al. [ 53 , 54 ], effects are nonlinear transformations of causes with some inner additive noise, followed by external nonlinear distortion (Eq. 2 ). From Eq. 2 , we obtain \(N={f}^{-1}(Y)-g(X)\) , where X and Y are the two observed variables representing cause and effect, respectively. To identify the cause and effect, a particular type of constrained nonlinear ICA [ 17 , 53 ] is performed to extract two components that are as independent as possible. The two extracted components are the assumed cause and corresponding additive noise, respectively. The identification method of the model is described elsewhere ([ 53 ], Section 4). The identifiability of the causal direction inferred by the PNL model has been proven [ 54 ]. The PNL model can identify the causal direction of data generated in accordance with the model except for the five situations described in Table 1 in [ 54 ].
The IGCI model [ 4 , 22 ] is based on the hypothesis that if “X causes Y,” the marginal distribution p ( x ) and the conditional distribution p ( y | x ) are independent in a particular way. The IGCI model gives an information-theoretic view of additive noise and defines independence by using orthogonality. With ANM [ 15 ], if there is no additive noise, inference is impossible, while it is possible with the IGCI model.
The IGCI model determines the causal direction on the basis of complexity loss. According to IGCI, the choice of p ( x ) is independent of the choice of f for the relationship \(y=f(x)+n\) . Let \(\nu _{x}\) and \(\nu _{y}\) be the reference distributions Footnote 4 for X and Y.
is the KL-distance between \(P _{x}\) and \(\nu _{x}\) . \(D(P_{x} \left| \right| \nu _{x})\) works as a feature of the complexity of the distribution. The complexity loss from X to Y is given by
The decision rule of the IGCI model is that if \(V_{X\rightarrow Y} < 0\) , infer “X causes Y,” and if \(V_{X\rightarrow Y} > 0\) , infer “Y causes X.” This rule is rather theoretical. An applicable and explicit form for the reference measure is entropy-based IGCI or slope-based IGCI.
Entropy-based IGCI:
where \(\psi ()\) is the digamma function Footnote 5 and m is the number of data points.
Slope-based IGCI:
These explicit forms are simpler, and we can see that the two calculation methods coincide. The calculation does not take much time even when dealing with a large amount of data. However, the IGCI model assumes that the causal process is noiseless and may perform poorly under high-noise conditions. We discuss the performance of the three models in Sect. 5 .
4 Experiments
Here we describe the dataset used in our experiments and the implementation of each model.
4.1 Dataset
We used the cause effect pairs (CEP) [ 27 ] dataset, which contains 97 pairs of real-world causal variables with the cause and effect labeled for each pair. The dataset is publicly available online [ 27 ]. Some of the data were collected from the UCI machine learning repository [ 24 ]. The data come from various fields, including geography, biology, physics, and economics. The dataset also contains time series data. Most of the data are noisy. An appendix in [ 28 ] contains a detailed description of each pair of variables.
We used 91 of the pairs in our experiments since some of the data (e.g., pair0052) contain multi-dimensional variables. Footnote 6 The 91 pairs are listed in Table 4 in “Appendix.” Some contain the same variables collected in different countries or at different times. Footnote 7 The variables range in size from 126 to 16,382. Footnote 8 The variety of data types in the CEP dataset makes causal analysis using real-world data challenging.
4.2 Implementation
We implemented the three models following the original work [ 15 , 22 , 53 ]. A brief introduction is given blow.
ANM Using the reported experimental settings [ 15 ], we performed Gaussian processes for machine learning regression [ 33 , 34 , 35 ]. We then used the Hilbert–Schmidt Independence Criterion (HSIC) [ 10 ] to test the independence between the assumed cause and residuals. The dataset used had been labeled with the true causal direction for each pair with no cases of independence or feedback. Using the decision rule of ANM, we determined that the direction with the greater independence was the true causal direction.
PNL Model We used a particular type of constrained nonlinear ICA to extract the two components that would be the cause and noise if the model had been learned in the correct direction. The nonlinearities of g () and \(f^{-1}()\) in Eq. 2 were modeled using multilayer perceptrons. By minimizing the mutual information between the two output components, we made the output as independent as possible. After extracting two independent components, we tested their independence by using the HSIC [ 10 , 11 ]. Finally, in the same way as for the ANM, we determined that the direction with the greater independence was the correct one.
IGCI \(\mathrm {(entropy, uniform)}\) Compared with the first two models, the implementation of the IGCI (entropy, uniform) model was simpler. We used reported equations ( 3 , 4 ) to calculate \(\hat{V}_{X\rightarrow Y}\) and \(\hat{V}_{Y\rightarrow X}\) and determined that the direction in which entropy decreased was the correct direction. If \(\hat{V}_{X\rightarrow Y}<0\) , the inferred causal direction was “X causes Y”; otherwise, it was “Y causes X.” For the IGCI model, the data should be normalized before calculating \(\hat{V}_{X\rightarrow Y}\) and \(\hat{V}_{Y\rightarrow X}\) . In accordance with the reported experimental results, we used the uniform distribution as the reference distribution because of its good performance. For the repetitive data in the dataset, we set \(\log 0=0\) .
IGCI \(\mathrm {(slope, uniform)}\) The implementation of the IGCI (slope, uniform) model was similar to that of the IGCI (entropy, uniform) one. We used Eq. 5 to calculate \(\hat{V}_{X\rightarrow Y}\) and \(\hat{V}_{Y\rightarrow X}\) and determined that the direction with a negative value was the correct one. For the same reason as above, we normalized the data to [0,1] before calculating \(\hat{V}_{X\rightarrow Y}\) and \(\hat{V}_{Y\rightarrow X}\) . To make Eq. 5 meaningful, we filtered out the repetitive data.
Here, we first compare model accuracy for different decision rates. Footnote 9 We changed the threshold and calculated the corresponding decision rate and accuracy for each model. The accuracy of the models for different decision rates has been compared in the original study [ 4 ]. Compared with [ 4 ], we used more real-world data in our experiments. Besides, how accuracy changed under different decision rates was showed. Our results are consistent with those shown of Mooij et al. [ 26 ]. The performance of the models for different decision rates is discussed in Sect. 5.1 .
Accuracy of three models for different decision rates. Decision rate changed when threshold was changed: the larger the threshold, the smaller the decision rate. In an ideal case, the accuracy of each model should improve with a decrease in the decision rate
Since causal discovery models in the bivariate case make a decision between two choices, we can regard these models as binary classifiers and evaluate them using AUC. We previously divided the data into two groups (inferred as “X causes Y” and inferred as “Y causes X”) and evaluated the performance of each model for each group [ 23 ]. Here we give the results for the entire (undivided) dataset.
Finally, we compare model efficiency by using the average time needed to make a decision. This is described in Sect. 5.3 .
5.1 Accuracy for different decision rates
We calculated the accuracy of each model for different decision rates using Eqs. 6 and 7 . The results are plotted in (Fig. 2 ). The decision rate changed when the threshold was changed. The larger the threshold, the more stringent the decision rule. In an ideal situation, accuracy decreases as the decision rate increases, with the starting point at 1.0. However, the results with real-world data were not perfect because the data were noisy.
As shown in Fig. 2 , the accuracy started from 1.0 for the ANM and IGCI and from 0.0 for the PNL model. This means that the PNL model made an incorrect decision when it had the highest confidence. Although the accuracies of the IGCI models started from 1.0, they dropped sharply when the decision rate was between 0 and 0.2. The reasons for this are discussed in detail in Sect. 5.4 . After reaching a minimum, the accuracies increased almost continuously and stabilized. The accuracy of the ANM was more stable than those of the other models. When all decisions had been made, the model accuracies were ranked IGCI > ANM > PNL.
5.2 Area under ROC curve (AUC)
Besides calculating the accuracy of the three models for different decision rates, we used the AUC to evaluate their performance. Some of the experimental results were presented in our conference paper [ 23 ], for which the dataset was divided into two groups: inferred as “X causes Y” and inferred as “Y causes X.” Here we present updated experimental results for the entire dataset.
The following steps were taken to get the experimental results:
Set X as the cause and Y as the effect in the input data.
Set \({V}_{X \rightarrow Y}\) and \({V}_{Y \rightarrow X}\) to be the outputs.
Calculate the absolute value of the difference between \({V}_{X \rightarrow Y}\) and \({V}_{Y \rightarrow X}\) (Eq. 8 ) and map \(V_{\mathrm {diff}}\) to [0,1].
Assign a positive label to the pairs inferred as “X causes Y” and a negative one to the pairs inferred as “Y causes X.”
Use \(V_{\mathrm {diff}}\) and the labels assigned in step 4 to calculate the true-positive rate (TPR) and false-positive rate (FPR) for different thresholds.
Plot the ROC curve and calculate the corresponding AUC value.
In step (1), instead of dividing the data into two groups as done previously [ 23 ], we set the input matrix so that the first column was the cause and the second column was the effect. Then, if the inferred causal direction for a pair was “X causes Y,” a positive label was assigned to that pair; otherwise, a negative label was assigned.
In step (3), we used the absolute value of the difference between \({V}_{X \rightarrow Y}\) and \({V}_{Y \rightarrow X}\) as the “confidence” of the model when making a decision. The larger the \(V_{\mathrm {diff}}\) , the greater the confidence. We did not use division because, if one of \({V}_{X \rightarrow Y}\) and \({V}_{Y \rightarrow X}\) was very small, the division result would be very large. We mapped \(V_{\mathrm {diff}}\) to [0,1] to make the calculation more convenient. In this way, \(V_{\mathrm {diff}}\) could be used in the same way as the output of a binary classifier. For causal discovery, the larger the \(V_{\mathrm {diff}}\) , the greater the confidence in the decision. At the same time, more punishment should be given when the decision is incorrect.
In step (4), we labeled the data in accordance with the inferred causal direction. Since the correct label for all the pairs was “X causes Y,” if the inferred result for a pair was “Y causes X,” it was assigned a negative label.
In step (5), we used the normalized \(V_{\mathrm {diff}}\) and the label assigned in step (4) to calculate TPR and FPR for different thresholds. We plotted TPR and FPR to get the ROC curve and calculated the corresponding AUC value.
The ROC results are plotted in Fig. 3 . The corresponding AUC values are shown in Table 1 . Different from the results shown in Fig. 2 , both IGCI models performed poorly in terms of AUC. The AUC values for IGCI were smaller than 0.5, which means their performances were even worse than that of a random classifier. However, as described in Sect. 5.1 , when we used different decision rates, the IGCI models had better performance.
We checked the decisions made by the IGCI models and found that they made several incorrect decisions when the threshold was large. Such decisions with a large threshold are punished severely when using the AUC metric. As shown in Fig. 2 , although the accuracies of the IGCI models started from 1.0, they dropped sharply when the decision rate was between 0 and 0.2. An incorrect decision with a low decision rate was not punished much when evaluating accuracy for different decision rates. However, for the AUC, an incorrect decision when the threshold was large was punished more than when the threshold was small. For these reasons, the starting point of the ROC curve for the IGCI models in Fig. 3 was shifted to the right, making the AUC less than 0.5. In Sect. 5.4 , we will discuss why the IGCI models failed.
ROC of three models. Four graphs are shown because IGCI has two explicit forms
5.3 Algorithm efficiency
Besides comparing the accuracy and ROC of the three models, we also compared the average time for the algorithm to make a decision. Footnote 10 We performed the experiment on the MATLAB platform with an Intel Core i7-4770 3.40 GHz CPU and 8 GB memory. From Table 2 , we can see that the IGCI models were the most efficient one, while the PNL model was the least efficient. The ANM was in the middle. The longer time to make a decision for the PNL model was due to the estimation procedure of \({f}^{-1}\) and g in Eq. 2 .
5.4 Typical examples of model failure
5.4.1 discretization.
In Sect. 5.1 , we explained that the PNL model gives an incorrect decision when the threshold is set the highest, i.e., the accuracy for different decision rates starts from 0.0. We investigated the reason for the PNL model making an incorrect decision when the threshold was the highest. We found that it happens due to the discretization of data. A scatter plot for a pair of variables (pair0070) is shown in Fig. 4 . The data have two variables. Variable \({x}_{1}\) is a value between 0 and 14 reflecting the features of an artificial face. It is used to decide whether the face is that of a man or a woman. A value of 0 means very female, and a value of 14 means very male. Variable \({x}_{2}\) is a value of 0 (female) or 1 (male) reflecting the gender decision. Since variable \({x}_{2}\) has only two values, no matter what nonlinear transformation is made to \({x}_{2}\) , the transformation result is two discretized values. According to the mechanism of the PNL model, \(x_{2}\) with two discretized values is inferred to be the cause since the independency is larger if \({x}_{2}\) is the cause. In fact, for this pair of variables, all three models made incorrect decisions in our experiments. For the ANM, the discretization of data makes regression analysis difficult. And the poor regression results negatively affect testing of the independence between the assumed cause and residuals using HSIC. For IGCI, to make Eqs. 3 and 5 meaningful, the repetitive data have to be filtered out. This means that only a few data points are actually used in the final IGCI calculation.
Example of discretized data in CEP dataset. Variable \({x}_{1}\) is between 0 (very female) and 14 (very male), reflecting features of artificial face. Variable \({x}_{2}\) is 0 (female) or 1 (male), reflecting gender decision
5.4.2 Noisiness
In Sect. 5.1 , we showed that IGCI had good performance in general. However, its accuracy dropped sharply when the decision rate was between 0 and 0.2. The reason for this is that it made incorrect decisions with high confidence when dealing with pair0056-pair00 63. These eight pairs contain much noise, which degraded model performance. Moreover, there were outliers for the eight pairs, which greatly affected the decision result. A scatter plot for one example pair is shown in Fig. 5 (pair0056). It shows that the two variables have relatively small correlation and that there are outliers in the data. The calculation method used in IGCI is such that these kinds of outliers affect the inference result more than the other data points. The incorrect decisions that IGCI made about pair0056-pair0063 account for the small AUC value for the IGCI models given in Sect. 5.2 . The ANM also made an incorrect inference about these pairs. This is because noise and outliers make overfitting more likely to occur for these variables. For these noisy pairs, the PNL model had the best performance.
Example scatter plot of noisy data in CEP. Variable \({x}_{1}\) is female life expectancy at birth for 20002005. Variable \({x}_{2}\) is latitude of birth country’s capital (data for China, Russia, and Canada were removed)
5.5 Response to spurious correlation caused by “confounding”
A causal relationship differs from a statistical one, and a statistical relationship is usually not enough to explain a causal one. Even if we observe that two variables are highly correlated, we cannot say that they have a causal relationship. As shown in Table 4 in “Appendix,” the causal direction of the variables in CEP is obvious by common sense. The dataset has been collected for evaluating causal discovery models, and the causal direction of most pairs is obvious from knowledge. However, the relationships of variables that are of general interest in the real world are usually more controversial, e.g., smoking and lung cancer, for which the existence of confounding is usually a bone of contention. A good causal discovery model for two variables should have the ability to avoid the effect of “confounding” to some degree. To test how the ANM, the PNL model, and IGCI perform when dealing with spurious correlation caused by confounding, we first simulated the “common cause” case shown in Fig. 1 . We controlled the data generating process to simulate different degrees of confounding. In addition to simulation, we used real-world data from the CEP to evaluate model performance when dealing with real-world data.
Scatter plots of generated data. a a/b: 0.1, b a/b: 1, c a/b: 10, d a/b: 100, e a/b: 1000
Estimated results of IGCI for generated data. Inference was “Y causes X” when estimated result was larger than 0
Test statistics for PNL model for generated data
5.5.1 Simulation
We conducted simulation experiments of the “common cause” confounding case shown in Fig. 1 . We generated data using two equations: \(x=a \times z^{3}+b \times n_{1}\) and \(y=a \times z+b \times n_{2}\) , where \(z,n_{1},n_{2} \in U(0,1)\) . We used the quotient a / b to control the degree of confounding. There was no direct causal relationship between variables x and y except for the various degrees of confounding. Scatter plots of the data generated using five different quotients are shown in Fig. 6 .
We used the generated data to test the performance of the three models. We performed ten random experiments for each quotient and calculated the average of the inferred results. The experimental results are shown in Figs. 7 , 8 , and Table 3 . For IGCI, when the degree of confounding was low, the mean of the estimated results Footnote 11 was almost zero. Each estimated result got a positive or negative value randomly. As the degree of confounding increased, the estimated results tended to approve “Y causes X.” For the PNL model, the independence assumptions for both directions were accepted when \(a/b=0.1, 1, 10\) ( \(\alpha =0.01\) ) and the means of the test statistics (Equation 4 in [ 11 ]) were almost equal. When \(a/b=100\) , the PNL model rejected the independence assumption for direction “X causes Y” and accepted that “Y causes X.” When a/b was even larger, the independence assumptions were rejected for both directions, especially that of “X causes Y.” For the ANM, when the degree of confounding was low, the independence assumptions were accepted for both directions. When the degree was high, the independence assumptions were rejected for both directions, especially “X causes Y.” From these results, we can see that the IGCI is the most susceptible of the three models tested to confounding, while the PNL model and the ANM can avoid the effect of confounding to a certain extent.
5.5.2 Real-world data
In addition to the simulation experiments described above, we conducted experiments using real-world data. The generation of real- world data can be very complex, which increases the difficulty of the causal discovery task. Here we describe the “common cause” and “selection bias” cases (Fig. 1 ).
Common cause case Although data for “common cause” are not included in the CEP dataset, some CEP pairs contain the same cause, as shown in “Appendix.” We combined data containing the same cause to obtain pairs of variables, such as “length and diameter.” Footnote 12 A scatter plot of the results is shown in Fig. 9 for the pair “length, diameter.” For the ANM, the p value for the forward direction, “length causes diameter,” was \(8.28 \times 10^{-5}\) while that for the backward direction was \(1.05 \times 10^{-3}\) . For the PNL model, the independence test statistic was \(1.11 \times 10^{-3}\) for the forward direction and \(9.50 \times 10^{-4}\) for the backward direction. For IGCI, the result estimated by calculating the entropy was 0.2197, while the other was 0.056. For this pair, although the tendency was not strong, the three models tended to approve “diameter is the cause of length.”
Results for “common cause” case with real-world data. Variable \(x_{1}\) : “length”; variable \(x_{2}\) : “diameter”
Selection bias case Although there is no causal relationship between X and Y in the selection bias case, independence does not hold between X and Y when conditioned on variable Z. This is the well-known Berkson paradox. Footnote 13 We used the variables “altitude and longitude” (Fig. 10 ) contained in CEP to test how the three models perform when dealing with the “selection bias” case. The variables were obtained by combining “cause: altitude, effect: temperature” and “cause: longitude, effect: temperature.” The data came from 349 weather stations in Germany [ 27 ]. A scatter plot of the results is shown in Fig. 10 . For the ANM, the p value for the forward direction, “altitude causes longitude,” was \(4.21 \times 10^{-2}\) while that for the backward direction was \(8.73 \times 10^{-2}\) . The independence assumptions were accepted for both directions although “longitude causes altitude” was favored. For the PNL model, the test statistic was \(2.46 \times 10^{-3}\) for the forward direction and \(3.30 \times 10^{-3}\) for the backward direction. The independence assumptions were accepted for both directions, and the independence test results were similar. For IGCI, the result estimated by calculating the entropy was 1.2742 and that estimated by calculating the slope was 1.9032. Both results were positive, and the estimated causal direction was “longitude causes altitude.” Although there should be no causal relationship between “altitude” and “longitude,” it was hard for the three models to determine that from the limited amount of observational data available.
For most cases of causal discovery in the real world, only limited observational data can be obtained, and in some cases the data are incomplete. Moreover, for the case of two variables, the causal sufficiency assumption [ 36 ] is easily violated if there is an unobserved common cause. The limited amount of data and unobserved confounders make causal discovery in the bivariate case challenging.
Results for “selection bias” case with real-world data. Variable \(x_{1}\) : “altitude”; variable \(x_{2}\) : “longitude”
6 Conclusion
We compared three state-of-the-art models (ANM, PNL model, IGCI) for causal discovery in the binary case using simulation and real-world data. Testing using different decision rates showed that the three models had similar accuracies when all the decisions were made. To check whether the decisions made were reasonable, we used a binary classifier metric: the area under the ROC curve (AUC). The IGCI model had a small AUC value because it made several incorrect decisions when the threshold was high. Compared with those of the other models, the accuracy of the ANM was relatively stable. A comparison of the time to make a decision showed that IGCI was the fastest even when the dataset was large. The PNL model took the most time to make a decision.
Of the three models, the IGCI one had the best performance when there was little noise and the data were not discretized much. Improving the performance of the IGCI model when there is much noise and how to deal with discretized data are future tasks. Although the performance of the ANM was relatively stable, overfitting should be avoided for ANM because it will negatively affect the subsequent independence test. Of the three models, the PNL model is the most generalized one as it takes into account the nonlinear effect of causes, inner additive noise, and external sensor distortion. However, estimation procedure of g () and \({f}^{-1}()\) is a lengthy procedure. Finally, testing the responses of the models to “confounding” showed that the ANM and the PNL model can avoid the effect of “confounding” to some degree, while IGCI is the most susceptible to confounding.
For the definition of “confounding,” please refer to [ 13 , 51 ].
The number of “confounders” is not limited to one.
There have been some efforts to deal with confounders. For example, Shimizu et al. [ 41 ] extended the linear non-Gaussian acyclic model to detect causal direction when there are “common causes” [ 14 , 40 ].
Reference distributions are used to measure the complexity of \(P_{x}\) and \(P_{y}\) . In [ 22 ], non-informative distributions like uniform and Gaussian ones are recommended.
https://en.wikipedia.org/wiki/Digamma_function .
The three models we evaluated cannot deal with multi-dimensional data.
Country and time information is not included in the table.
To avoid overfitting, we limited the size to 500 or less.
Since all three models have two outputs, e.g., \(\hat{V}_{X\rightarrow Y}\) , \(\hat{V}_{Y\rightarrow X}\) corresponding to the two possible causal directions, we set thresholds based on the absolute difference between them \(|\hat{V}_{X \rightarrow Y}-\hat{V}_{Y \rightarrow X}|\) for use in deciding each decision rate and model accuracy.
Compared to our previous report [ 23 ], we reduced the program output so that the PNL model works faster. We have updated the results for time to make a decision for the PNL model accordingly.
We used the difference between \(\hat{V}_{X\rightarrow Y}\) and \(\hat{V}_{Y\rightarrow X}\) (Eqs. 4 , 5 ) as the estimated result. For IGCI, \(\hat{V}_{X\rightarrow Y}\) is the opposite of \(\hat{V}_{Y\rightarrow X}\) if there is no repetitive data. Thus, we can infer that the correct causal direction is \(X\rightarrow Y\) if the estimated result is negative and that \(Y\rightarrow X\) is correct if the estimated result is positive.
The pair “length, diameter” was created from “cause: rings (abalone), effect: length” and “cause: rings (abalone), effect: diameter” using data for abalone [ 24 ].
https://en.wikipedia.org/wiki/Berkson’s_paradox .
Chen, Y., Rangarajan, G., Feng, J., Ding, M.: Analyzing multiple nonlinear time series with extended granger causality. Phys. Lett. A 324 (1), 26–35 (2004)
Article MathSciNet MATH Google Scholar
Chen, Z., Zhang, K., Chan, L.: Causal discovery with scale-mixture model for spatiotemporal variance dependencies. In: Advances in Neural Information Processing Systems, pp. 1727–1735 (2012)
Chen, Z., Zhang, K., Chan, L., Schölkopf, B.: Causal discovery via reproducing kernel hilbert space embeddings. Neural Comput. 26 (7), 1484–1517 (2014)
Article MathSciNet Google Scholar
Daniusis, P., Janzing, D., Mooij, J., Zscheischler, J., Steudel, B., Zhang, K., Schölkopf, B.: Inferring deterministic causal relations. arXiv preprint arXiv:1203.3475 (2012)
Eberhardt, F.: Introduction to the foundations of causal discovery. Int. J. Data Sci. Anal., 1–11 (2017)
Gong, M., Zhang, K., Schoelkopf, B., Tao, D., Geiger, P.: Discovering temporal causal relations from subsampled data. In: Proceedings of 32th International Conference on Machine Learning (ICML 2015) (2015)
Granger, C.W.: Investigating causal relations by econometric models and cross-spectral methods. Econom. J. Econom. Soc. 37 , 424–438 (1969)
MATH Google Scholar
Granger, C.W.: Testing for causality: a personal viewpoint. J. Econ. Dyn. Control 2 , 329–352 (1980)
Granger, C.W.: Some recent development in a concept of causality. J. Econom. 39 (1), 199–211 (1988)
Gretton, A., Herbrich, R., Smola, A., Bousquet, O., Schölkopf, B.: Kernel methods for measuring independence. J. Mach. Learn. Res. 6 (Dec), 2075–2129 (2005)
MathSciNet MATH Google Scholar
Gretton, A., Fukumizu, K., Teo, C.H., Song, L., Schölkopf, B., Smola, A.J., et al.: A kernel statistical test of independence. NIPS 20 , 585–592 (2008)
Google Scholar
Halpern, J.Y.: A modification of the halpern-pearl definition of causality. arXiv preprint arXiv:1505.00162 (2015)
Howards, P.P., Schisterman, E.F., Poole, C., Kaufman, J.S., Weinberg, C.R.: “Toward a clearer definition of confounding” revisited with directed acyclic graphs. Am. J. Epidemiol. 176 (6), 506–511 (2012)
Article Google Scholar
Hoyer, P.O., Shimizu, S., Kerminen, A.J., Palviainen, M.: Estimation of causal effects using linear non-gaussian causal models with hidden variables. Int. J. Approx. Reason. 49 (2), 362–378 (2008)
Hoyer, P.O., Janzing, D., Mooij, J.M., Peters, J., Schölkopf, B.: Nonlinear causal discovery with additive noise models. In: Advances in neural information processing systems, pp. 689–696 (2009)
Huang, B., Zhang, K., Schölkopf, B.: Identification of time-dependent causal model: A gaussian process treatment. In: The 24th International Joint Conference on Artificial Intelligence, Machine Learning Track, pp. 3561–3568. Buenos, Argentina (2015)
Hyvärinen, A., Oja, E.: Independent component analysis: algorithms and applications. Neural Netw. 13 (4), 411–430 (2000)
Hyvärinen, A., Zhang, K., Shimizu, S., Hoyer, P.O.: Estimation of a structural vector autoregression model using non-gaussianity. J. Mach. Learn. Res. 11 (May), 1709–1731 (2010)
Janzing, D., Scholkopf, B.: Causal inference using the algorithmic markov condition. IEEE Trans. Inf. Theory 56 (10), 5168–5194 (2010)
Janzing, D., Hoyer, P.O., Schölkopf, B.: Telling cause from effect based on high-dimensional observations. arXiv preprint arXiv:0909.4386 (2009)
Janzing, D., MPG, T., Schölkopf, B.: Causality: Objectives and assessment (2010)
Janzing, D., Mooij, J., Zhang, K., Lemeire, J., Zscheischler, J., Daniušis, P., Steudel, B., Schölkopf, B.: Information-geometric approach to inferring causal directions. Artif. Intell. 182 , 1–31 (2012)
Jing, S., Satoshi, O., Haruhiko, S., Masahito, K.: Evaluation of causal discovery models in bivariate case using real world data. In: Proceedings of the International MultiConference of Engineers and Computer Scientists 2016, pp. 291–296 (2016)
Lichman, M.: UCI machine learning repository. http://archive.ics.uci.edu/ml (2013)
Lopez-Paz, D., Muandet, K., Schölkopf, B., Tolstikhin, I.: Towards a learning theory of cause-effect inference. In: Proceedings of the 32nd International Conference on Machine Learning. JMLR: W&CP, Lille, France (2015)
Mooij, J.M., Janzing, D., Schölkopf, B.: Distinguishing between cause and effect. In: NIPS Causality: Objectives and Assessment, pp. 147–156 (2010)
Mooij J.M., Janzing, D., Zscheischler, J., Schölkopf, B.: Cause effect pairs repository. https://webdav.tuebingen.mpg.de/cause-effect/ (2014a)
Mooij, J.M., Peters, J., Janzing, D., Zscheischler, J., Schölkopf, B.: Distinguishing cause from effect using observational data: methods and benchmarks. arXiv preprint arXiv:1412.3773 (2014b)
Pearl, J., et al.: Models, reasoning and inference (2000)
Peters, J., Janzing, D., Gretton, A., Schölkopf, B.: Detecting the direction of causal time series. In: Proceedings of the 26th Annual International Conference on Machine Learning, pp. 801–808. ACM, New York (2009)
Peters, J., Mooij, J., Janzing, D., Schölkopf, B.: Identifiability of causal graphs using functional models. arXiv preprint arXiv:1202.3757 (2012)
Peters, J., Janzing, D., Schölkopf, B.: Causal inference on time series using restricted structural equation models. In: Advances in Neural Information Processing Systems, pp. 154–162 (2013)
Rasmussen, C.E.: Gaussian Processes for Machine Learning. MIT press, Cambridge (2006)
Rasmussen, C.E., Nickisch, H.: Gaussian processes for machine learning (gpml) toolbox. J. Mach. Learn. Res. 11 (Nov), 3011–3015 (2010a)
Rasmussen, C.E., Nickisch, H.: GPML code. http://www.gaussianprocess.org/gpml/code/matlab/doc/ (2010b)
Scheines, R.: An introduction to causal inference (1997)
Schölkopf, B., Janzing, D., Peters, J., Sgouritsa, E., Zhang, K., Mooij, J.: On causal and anticausal learning. arXiv preprint arXiv:1206.6471 (2012)
Sgouritsa, E., Janzing, D., Hennig, P., Schölkopf, B.: Inference of cause and effect with unsupervised inverse regression. In: AISTATS (2015)
Shajarisales, N., Janzing, D., Schoelkopf, B., Besserve, M.: Telling cause from effect in deterministic linear dynamical systems. arXiv preprint arXiv:1503.01299 (2015)
Shimizu, S., Bollen, K.: Bayesian estimation of causal direction in acyclic structural equation models with individual-specific confounder variables and non-gaussian distributions. J. Mach. Learn. Res. 15 (1), 2629–2652 (2014)
Shimizu, S., Hoyer, P.O., Hyvärinen, A., Kerminen, A.: A linear non-gaussian acyclic model for causal discovery. J. Mach. Learn. Res. 7 (Oct), 2003–2030 (2006)
Shimizu, S., Inazumi, T., Sogawa, Y., Hyvärinen, A., Kawahara, Y., Washio, T., Hoyer, P.O., Bollen, K.: Directlingam: a direct method for learning a linear non-gaussian structural equation model. J. Mach. Learn. Res. 12 (Apr), 1225–1248 (2011)
Spirtes, P., Zhang, K.: Causal discovery and inference: concepts and recent methodological advances. Appl. Inform. 3 , 3 (2016)
Spirtes, P., Glymour, C.N., Scheines, R.: Causation, Prediction, and Search. MIT press, Cambridge (2000)
Stegle, O., Janzing, D., Zhang, K., Mooij, J.M., Schölkopf, B.: Probabilistic latent variable models for distinguishing between cause and effect. In: Advances in Neural Information Processing Systems, pp. 1687–1695 (2010)
Sun, X., Janzing, D., Schölkopf, B.: Causal inference by choosing graphs with most plausible markov kernels. In: ISAIM (2006)
Sun, X., Janzing, D., Schölkopf, B.: Distinguishing between cause and effect via kernel-based complexity measures for conditional distributions. In: ESANN, pp. 441–446 (2007a)
Sun, X., Janzing, D., Schölkopf, B., Fukumizu, K.: A kernel-based causal learning algorithm. In: Proceedings of the 24th international conference on Machine learning, pp. 855–862. ACM, New York (2007b)
Sun, X., Janzing, D., Schölkopf, B.: Causal reasoning by evaluating the complexity of conditional densities with kernel methods. Neurocomputing 71 (7), 1248–1256 (2008)
Tillman, R.E., Gretton, A., Spirtes, P.: Nonlinear directed acyclic structure learning with weakly additive noise models. In: Advances in Neural Information Processing Systems, pp. 1847–1855 (2009)
Weinberg, C.R.: Toward a clearer definition of confounding. Am. J. Epidemiol. 137 (1), 1–8 (1993)
Zhang, K., Chan, L.W.: Extensions of ICA for causality discovery in the Hong Kong stock market. In: International Conference on Neural Information Processing, pp. 400–409. Springer, Berlin (2006)
Zhang, K., Hyvärinen, A.: Distinguishing causes from effects using nonlinear acyclic causal models. In: Journal of Machine Learning Research, Workshop and Conference Proceedings (NIPS 2008 Causality Workshop), vol. 6, pp. 157–164 (2008)
Zhang, K., Hyvärinen, A.: On the identifiability of the post-nonlinear causal model. In: Proceedings of the Twenty-fifth Conference on Uncertainty in Artificial Intelligence, pp. 647–655. AUAI Press, Corvallis (2009)
Zhang, K., Schölkopf, B., Janzing, D.: Invariant gaussian process latent variable models and application in causal discovery. arXiv preprint arXiv:1203.3534 (2012)
Zhang, K., Wang, Z., Schölkopf, B.: On estimation of functional causal models: post-nonlinear causal model as an example. In: 2013 IEEE 13th International Conference on Data Mining Workshops, pp. 139–146. IEEE (2013)
Zhang, K., Zhang, J., Schölkopf, B.: Distinguishing cause from effect based on exogeneity. arXiv preprint arXiv:1504.05651 (2015)
Download references
Acknowledgements
We thank the anonymous reviewers for their helpful comments to improve the paper. The work was supported in part by a Grant-in-Aid for Scientific Research (15K12148) from the Japan Society for the Promotion of Science.
Author information
Authors and affiliations.
Graduate School of Information Science and Technology, Hokkaido University, Sapporo, Japan
Jing Song, Satoshi Oyama & Masahito Kurihara
You can also search for this author in PubMed Google Scholar
Corresponding author
Correspondence to Jing Song .
Additional information
This paper is an revised and extended version of our conference paper [ 23 ].

Appendix: Pairs of variables used in experiment
See Table 4 .
Rights and permissions
Reprints and permissions
About this article
Song, J., Oyama, S. & Kurihara, M. Tell cause from effect: models and evaluation. Int J Data Sci Anal 4 , 99–112 (2017). https://doi.org/10.1007/s41060-017-0063-0
Download citation
Received : 20 July 2016
Accepted : 30 June 2017
Published : 21 July 2017
Issue Date : September 2017
DOI : https://doi.org/10.1007/s41060-017-0063-0
Share this article
Anyone you share the following link with will be able to read this content:
Sorry, a shareable link is not currently available for this article.
Provided by the Springer Nature SharedIt content-sharing initiative
- Causal discovery
- Time to make a decision
- Find a journal
- Publish with us
- Track your research
- Privacy Policy

Home » Explanatory Research – Types, Methods, Guide
Explanatory Research – Types, Methods, Guide
Table of Contents

Explanatory Research
Definition :
Explanatory research is a type of research that aims to uncover the underlying causes and relationships between different variables. It seeks to explain why a particular phenomenon occurs and how it relates to other factors.
This type of research is typically used to test hypotheses or theories and to establish cause-and-effect relationships. Explanatory research often involves collecting data through surveys , experiments , or other empirical methods, and then analyzing that data to identify patterns and correlations. The results of explanatory research can provide a better understanding of the factors that contribute to a particular phenomenon and can help inform future research or policy decisions.
Types of Explanatory Research
There are several types of explanatory research, each with its own approach and focus. Some common types include:
Experimental Research
This involves manipulating one or more variables to observe the effect on other variables. It allows researchers to establish a cause-and-effect relationship between variables and is often used in natural and social sciences.
Quasi-experimental Research
This type of research is similar to experimental research but lacks full control over the variables. It is often used in situations where it is difficult or impossible to manipulate certain variables.
Correlational Research
This type of research aims to identify relationships between variables without manipulating them. It involves measuring and analyzing the strength and direction of the relationship between variables.
Case study Research
This involves an in-depth investigation of a specific case or situation. It is often used in social sciences and allows researchers to explore complex phenomena and contexts.
Historical Research
This involves the systematic study of past events and situations to understand their causes and effects. It is often used in fields such as history and sociology.
Survey Research
This involves collecting data from a sample of individuals through structured questionnaires or interviews. It allows researchers to investigate attitudes, behaviors, and opinions.
Explanatory Research Methods
There are several methods that can be used in explanatory research, depending on the research question and the type of data being collected. Some common methods include:
Experiments
In experimental research, researchers manipulate one or more variables to observe their effect on other variables. This allows them to establish a cause-and-effect relationship between the variables.
Surveys are used to collect data from a sample of individuals through structured questionnaires or interviews. This method can be used to investigate attitudes, behaviors, and opinions.
Correlational studies
This method aims to identify relationships between variables without manipulating them. It involves measuring and analyzing the strength and direction of the relationship between variables.
Case studies
Case studies involve an in-depth investigation of a specific case or situation. This method is often used in social sciences and allows researchers to explore complex phenomena and contexts.
Secondary Data Analysis
This method involves analyzing data that has already been collected by other researchers or organizations. It can be useful when primary data collection is not feasible or when additional data is needed to support research findings.
Data Analysis Methods
Explanatory research data analysis methods are used to explore the relationships between variables and to explain how they interact with each other. Here are some common data analysis methods used in explanatory research:
Correlation Analysis
Correlation analysis is used to identify the strength and direction of the relationship between two or more variables. This method is particularly useful when exploring the relationship between quantitative variables.
Regression Analysis
Regression analysis is used to identify the relationship between a dependent variable and one or more independent variables. This method is particularly useful when exploring the relationship between a dependent variable and several predictor variables.
Path Analysis
Path analysis is a method used to examine the direct and indirect relationships between variables. It is particularly useful when exploring complex relationships between variables.
Structural Equation Modeling (SEM)
SEM is a statistical method used to test and validate theoretical models of the relationships between variables. It is particularly useful when exploring complex models with multiple variables and relationships.
Factor Analysis
Factor analysis is used to identify underlying factors that contribute to the variation in a set of variables. This method is particularly useful when exploring relationships between multiple variables.
Content Analysis
Content analysis is used to analyze qualitative data by identifying themes and patterns in text, images, or other forms of data. This method is particularly useful when exploring the meaning and context of data.
Applications of Explanatory Research
The applications of explanatory research include:
- Social sciences: Explanatory research is commonly used in social sciences to investigate the causes and effects of social phenomena, such as the relationship between poverty and crime, or the impact of social policies on individuals or communities.
- Marketing : Explanatory research can be used in marketing to understand the reasons behind consumer behavior, such as why certain products are preferred over others or why customers choose to purchase from certain brands.
- Healthcare : Explanatory research can be used in healthcare to identify the factors that contribute to disease or illness, as well as the effectiveness of different treatments and interventions.
- Education : Explanatory research can be used in education to investigate the causes of academic achievement or failure, as well as the factors that influence teaching and learning processes.
- Business : Explanatory research can be used in business to understand the factors that contribute to the success or failure of different strategies, as well as the impact of external factors, such as economic or political changes, on business operations.
- Public policy: Explanatory research can be used in public policy to evaluate the effectiveness of policies and programs, as well as to identify the factors that contribute to social problems or inequalities.
Explanatory Research Question
An explanatory research question is a type of research question that seeks to explain the relationship between two or more variables, and to identify the underlying causes of that relationship. The goal of explanatory research is to test hypotheses or theories about the relationship between variables, and to gain a deeper understanding of complex phenomena.
Examples of explanatory research questions include:
- What is the relationship between sleep quality and academic performance among college students, and what factors contribute to this relationship?
- How do environmental factors, such as temperature and humidity, affect the spread of infectious diseases?
- What are the factors that contribute to the success or failure of small businesses in a particular industry, and how do these factors interact with each other?
- How do different teaching strategies impact student engagement and learning outcomes in the classroom?
- What is the relationship between social support and mental health outcomes among individuals with chronic illnesses, and how does this relationship vary across different populations?
Examples of Explanatory Research
Here are a few Real-Time Examples of explanatory research:
- Exploring the factors influencing customer loyalty: A business might conduct explanatory research to determine which factors, such as product quality, customer service, or price, have the greatest impact on customer loyalty. This research could involve collecting data through surveys, interviews, or other means and analyzing it using methods such as correlation or regression analysis.
- Understanding the causes of crime: Law enforcement agencies might conduct explanatory research to identify the factors that contribute to crime in a particular area. This research could involve collecting data on factors such as poverty, unemployment, drug use, and social inequality and analyzing it using methods such as regression analysis or structural equation modeling.
- Investigating the effectiveness of a new medical treatment: Medical researchers might conduct explanatory research to determine whether a new medical treatment is effective and which variables, such as dosage or patient age, are associated with its effectiveness. This research could involve conducting clinical trials and analyzing data using methods such as path analysis or SEM.
- Exploring the impact of social media on mental health : Researchers might conduct explanatory research to determine whether social media use has a positive or negative impact on mental health and which variables, such as frequency of use or type of social media, are associated with mental health outcomes. This research could involve collecting data through surveys or interviews and analyzing it using methods such as factor analysis or content analysis.
When to use Explanatory Research
Here are some situations where explanatory research might be appropriate:
- When exploring a new or complex phenomenon: Explanatory research can be used to understand the mechanisms of a new or complex phenomenon and to identify the variables that are most strongly associated with it.
- When testing a theoretical model: Explanatory research can be used to test a theoretical model of the relationships between variables and to validate or modify the model based on empirical data.
- When identifying the causal relationships between variables: Explanatory research can be used to identify the causal relationships between variables and to determine which variables have the greatest impact on the outcome of interest.
- When conducting program evaluation: Explanatory research can be used to evaluate the effectiveness of a program or intervention and to identify the factors that contribute to its success or failure.
- When making informed decisions: Explanatory research can be used to provide a basis for informed decision-making in business, government, or other contexts by identifying the factors that contribute to a particular outcome.
How to Conduct Explanatory Research
Here are the steps to conduct explanatory research:
- Identify the research problem: Clearly define the research question or problem you want to investigate. This should involve identifying the variables that you want to explore, and the potential relationships between them.
- Conduct a literature review: Review existing research on the topic to gain a deeper understanding of the variables and relationships you plan to explore. This can help you develop a hypothesis or research questions to guide your study.
- Develop a research design: Decide on the research design that best suits your study. This may involve collecting data through surveys, interviews, experiments, or observations.
- Collect and analyze data: Collect data from your selected sample and analyze it using appropriate statistical methods to identify any significant relationships between variables.
- Interpret findings: Interpret the results of your analysis in light of your research question or hypothesis. Identify any patterns or relationships between variables, and discuss the implications of your findings for the wider field of study.
- Draw conclusions: Draw conclusions based on your analysis and identify any areas for further research. Make recommendations for future research or policy based on your findings.
Purpose of Explanatory Research
The purpose of explanatory research is to identify and explain the relationships between different variables, as well as to determine the causes of those relationships. This type of research is often used to test hypotheses or theories, and to explore complex phenomena that are not well understood.
Explanatory research can help to answer questions such as “why” and “how” by providing a deeper understanding of the underlying causes and mechanisms of a particular phenomenon. For example, explanatory research can be used to determine the factors that contribute to a particular health condition, or to identify the reasons why certain marketing strategies are more effective than others.
The main purpose of explanatory research is to gain a deeper understanding of a particular phenomenon, with the goal of developing more effective solutions or interventions to address the problem. By identifying the underlying causes and mechanisms of a phenomenon, explanatory research can help to inform decision-making, policy development, and best practices in a wide range of fields, including healthcare, social sciences, business, and education
Advantages of Explanatory Research
Here are some advantages of explanatory research:
- Provides a deeper understanding: Explanatory research aims to uncover the underlying causes and mechanisms of a particular phenomenon, providing a deeper understanding of complex phenomena that is not possible with other research designs.
- Test hypotheses or theories: Explanatory research can be used to test hypotheses or theories by identifying the relationships between variables and determining the causes of those relationships.
- Provides insights for decision-making: Explanatory research can provide insights that can inform decision-making in a wide range of fields, from healthcare to business.
- Can lead to the development of effective solutions: By identifying the underlying causes of a problem, explanatory research can help to develop more effective solutions or interventions to address the problem.
- Can improve the validity of research: By identifying and controlling for potential confounding variables, explanatory research can improve the validity and reliability of research findings.
- Can be used in combination with other research designs : Explanatory research can be used in combination with other research designs, such as exploratory or descriptive research, to provide a more comprehensive understanding of a phenomenon.
Limitations of Explanatory Research
Here are some limitations of explanatory research:
- Limited generalizability: Explanatory research typically involves studying a specific sample, which can limit the generalizability of findings to other populations or settings.
- Time-consuming and resource-intensive: Explanatory research can be time-consuming and resource-intensive, particularly if it involves collecting and analyzing large amounts of data.
- Limited scope: Explanatory research is typically focused on a narrow research question or hypothesis, which can limit its scope in comparison to other research designs such as exploratory or descriptive research.
- Limited control over variables: Explanatory research can be limited by the researcher’s ability to control for all possible variables that may influence the relationship between variables of interest.
- Potential for bias: Explanatory research can be subject to various types of bias, such as selection bias, measurement bias, and recall bias, which can influence the validity of research findings.
- Ethical considerations: Explanatory research may involve the use of invasive or risky procedures, which can raise ethical concerns and require careful consideration of the potential risks and benefits of the study.
About the author
Muhammad Hassan
Researcher, Academic Writer, Web developer
You may also like

Case Study – Methods, Examples and Guide

Triangulation in Research – Types, Methods and...

Ethnographic Research -Types, Methods and Guide

One-to-One Interview – Methods and Guide

Qualitative Research Methods

Mixed Methods Research – Types & Analysis

Community Blog
Keep up-to-date on postgraduate related issues with our quick reads written by students, postdocs, professors and industry leaders.
Types of Research – Explained with Examples

- By DiscoverPhDs
- October 2, 2020
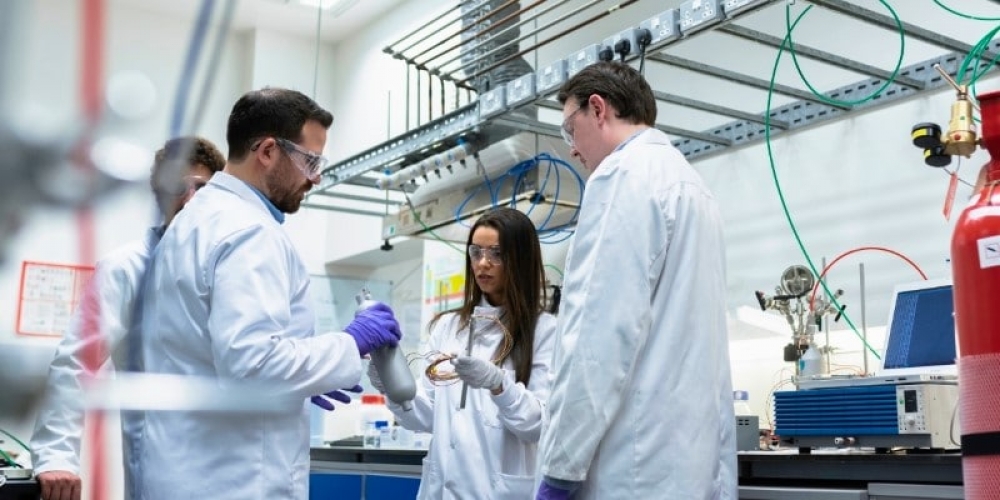
Types of Research
Research is about using established methods to investigate a problem or question in detail with the aim of generating new knowledge about it.
It is a vital tool for scientific advancement because it allows researchers to prove or refute hypotheses based on clearly defined parameters, environments and assumptions. Due to this, it enables us to confidently contribute to knowledge as it allows research to be verified and replicated.
Knowing the types of research and what each of them focuses on will allow you to better plan your project, utilises the most appropriate methodologies and techniques and better communicate your findings to other researchers and supervisors.
Classification of Types of Research
There are various types of research that are classified according to their objective, depth of study, analysed data, time required to study the phenomenon and other factors. It’s important to note that a research project will not be limited to one type of research, but will likely use several.
According to its Purpose
Theoretical research.
Theoretical research, also referred to as pure or basic research, focuses on generating knowledge , regardless of its practical application. Here, data collection is used to generate new general concepts for a better understanding of a particular field or to answer a theoretical research question.
Results of this kind are usually oriented towards the formulation of theories and are usually based on documentary analysis, the development of mathematical formulas and the reflection of high-level researchers.
Applied Research
Here, the goal is to find strategies that can be used to address a specific research problem. Applied research draws on theory to generate practical scientific knowledge, and its use is very common in STEM fields such as engineering, computer science and medicine.
This type of research is subdivided into two types:
- Technological applied research : looks towards improving efficiency in a particular productive sector through the improvement of processes or machinery related to said productive processes.
- Scientific applied research : has predictive purposes. Through this type of research design, we can measure certain variables to predict behaviours useful to the goods and services sector, such as consumption patterns and viability of commercial projects.
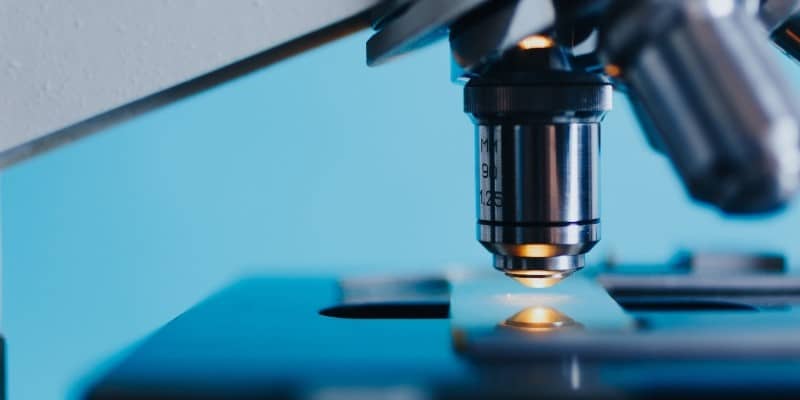
According to your Depth of Scope
Exploratory research.
Exploratory research is used for the preliminary investigation of a subject that is not yet well understood or sufficiently researched. It serves to establish a frame of reference and a hypothesis from which an in-depth study can be developed that will enable conclusive results to be generated.
Because exploratory research is based on the study of little-studied phenomena, it relies less on theory and more on the collection of data to identify patterns that explain these phenomena.
Descriptive Research
The primary objective of descriptive research is to define the characteristics of a particular phenomenon without necessarily investigating the causes that produce it.
In this type of research, the researcher must take particular care not to intervene in the observed object or phenomenon, as its behaviour may change if an external factor is involved.
Explanatory Research
Explanatory research is the most common type of research method and is responsible for establishing cause-and-effect relationships that allow generalisations to be extended to similar realities. It is closely related to descriptive research, although it provides additional information about the observed object and its interactions with the environment.
Correlational Research
The purpose of this type of scientific research is to identify the relationship between two or more variables. A correlational study aims to determine whether a variable changes, how much the other elements of the observed system change.
According to the Type of Data Used
Qualitative research.
Qualitative methods are often used in the social sciences to collect, compare and interpret information, has a linguistic-semiotic basis and is used in techniques such as discourse analysis, interviews, surveys, records and participant observations.
In order to use statistical methods to validate their results, the observations collected must be evaluated numerically. Qualitative research, however, tends to be subjective, since not all data can be fully controlled. Therefore, this type of research design is better suited to extracting meaning from an event or phenomenon (the ‘why’) than its cause (the ‘how’).
Quantitative Research
Quantitative research study delves into a phenomena through quantitative data collection and using mathematical, statistical and computer-aided tools to measure them . This allows generalised conclusions to be projected over time.

According to the Degree of Manipulation of Variables
Experimental research.
It is about designing or replicating a phenomenon whose variables are manipulated under strictly controlled conditions in order to identify or discover its effect on another independent variable or object. The phenomenon to be studied is measured through study and control groups, and according to the guidelines of the scientific method.
Non-Experimental Research
Also known as an observational study, it focuses on the analysis of a phenomenon in its natural context. As such, the researcher does not intervene directly, but limits their involvement to measuring the variables required for the study. Due to its observational nature, it is often used in descriptive research.
Quasi-Experimental Research
It controls only some variables of the phenomenon under investigation and is therefore not entirely experimental. In this case, the study and the focus group cannot be randomly selected, but are chosen from existing groups or populations . This is to ensure the collected data is relevant and that the knowledge, perspectives and opinions of the population can be incorporated into the study.
According to the Type of Inference
Deductive investigation.
In this type of research, reality is explained by general laws that point to certain conclusions; conclusions are expected to be part of the premise of the research problem and considered correct if the premise is valid and the inductive method is applied correctly.
Inductive Research
In this type of research, knowledge is generated from an observation to achieve a generalisation. It is based on the collection of specific data to develop new theories.
Hypothetical-Deductive Investigation
It is based on observing reality to make a hypothesis, then use deduction to obtain a conclusion and finally verify or reject it through experience.

According to the Time in Which it is Carried Out
Longitudinal study (also referred to as diachronic research).
It is the monitoring of the same event, individual or group over a defined period of time. It aims to track changes in a number of variables and see how they evolve over time. It is often used in medical, psychological and social areas .
Cross-Sectional Study (also referred to as Synchronous Research)
Cross-sectional research design is used to observe phenomena, an individual or a group of research subjects at a given time.
According to The Sources of Information
Primary research.
This fundamental research type is defined by the fact that the data is collected directly from the source, that is, it consists of primary, first-hand information.
Secondary research
Unlike primary research, secondary research is developed with information from secondary sources, which are generally based on scientific literature and other documents compiled by another researcher.
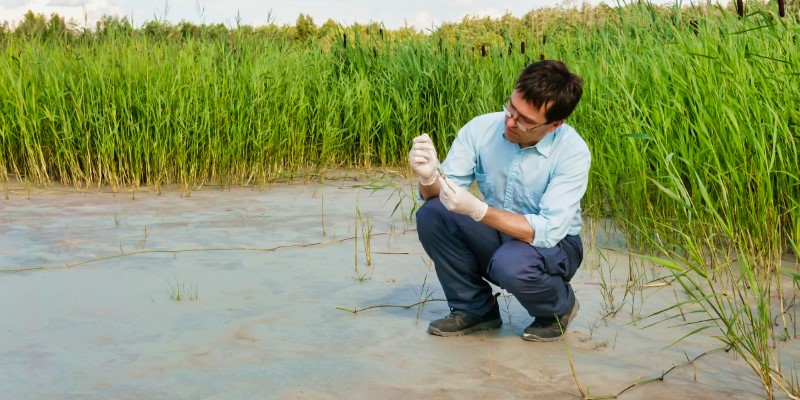
According to How the Data is Obtained
Documentary (cabinet).
Documentary research, or secondary sources, is based on a systematic review of existing sources of information on a particular subject. This type of scientific research is commonly used when undertaking literature reviews or producing a case study.
Field research study involves the direct collection of information at the location where the observed phenomenon occurs.
From Laboratory
Laboratory research is carried out in a controlled environment in order to isolate a dependent variable and establish its relationship with other variables through scientific methods.
Mixed-Method: Documentary, Field and/or Laboratory
Mixed research methodologies combine results from both secondary (documentary) sources and primary sources through field or laboratory research.
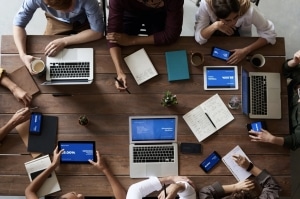
Learning how to effectively collaborate with others is an important skill for anyone in academia to develop.

The term rationale of research means the reason for performing the research study in question.

Are you always finding yourself working on sections of your research tasks right up until your deadlines? Are you still finding yourself distracted the moment
Join thousands of other students and stay up to date with the latest PhD programmes, funding opportunities and advice.
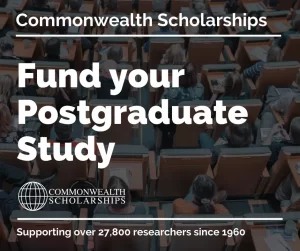
Browse PhDs Now

Self-plagiarism is when you try and pass off work that you’ve previously done as something that is completely new.
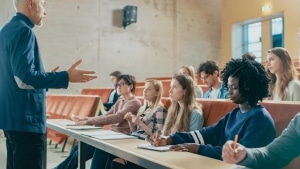
You’ll come across many academics with PhD, some using the title of Doctor and others using Professor. This blog post helps you understand the differences.

Sabrina’s in the third year of her PhD at The University of Adelaide. Her esearch combines molecular techniques, data analysis, and next generation sequencing to investigate modifications on RNAs in plants.

Charlene is a 5th year PhD candidate at the University of Wisconsin-Madison. She studies depression and neuroticism in people with Temporal Lobe Epilepsy (TLE) using MR Imaging and behavioural tests.
Join Thousands of Students
Establishing Cause and Effect
A central goal of most research is the identification of causal relationships, or demonstrating that a particular independent variable (the cause) has an effect on the dependent variable of interest (the effect). The three criteria for establishing cause and effect – association, time ordering (or temporal precedence), and non-spuriousness – are familiar to most researchers from courses in research methods or statistics. While the classic examples used to illustrate these criteria may imply that establishing cause and effect is straightforward, it is often one of the most challenging aspects of designing research studies for implementation in real world conditions.
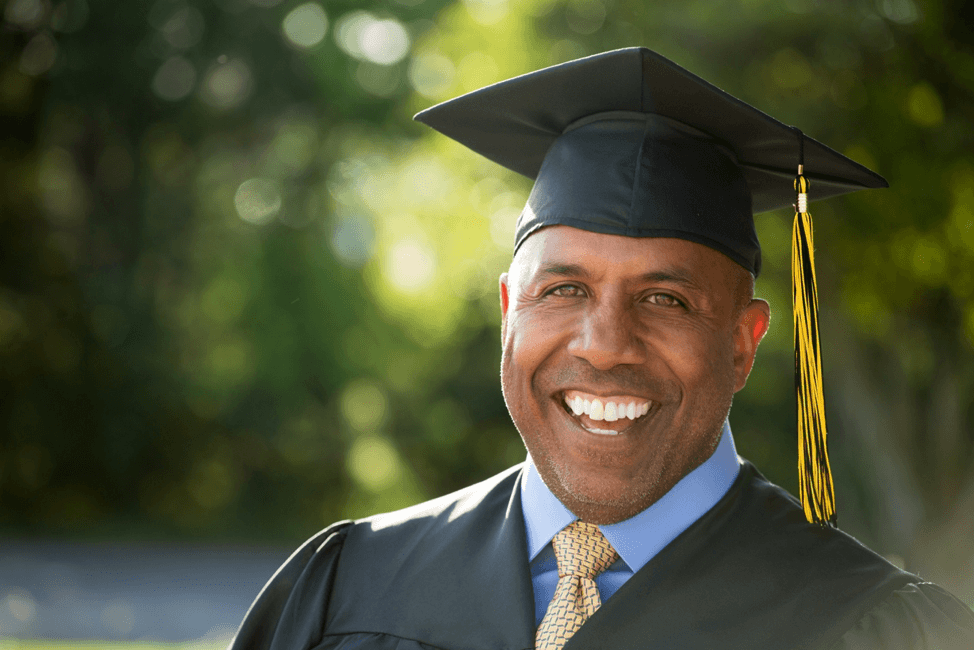
Discover How We Assist to Edit Your Dissertation Chapters
Aligning theoretical framework, gathering articles, synthesizing gaps, articulating a clear methodology and data plan, and writing about the theoretical and practical implications of your research are part of our comprehensive dissertation editing services.
- Bring dissertation editing expertise to chapters 1-5 in timely manner.
- Track all changes, then work with you to bring about scholarly writing.
- Ongoing support to address committee feedback, reducing revisions.
The first step in establishing causality is demonstrating association; simply put, is there a relationship between the independent variable and the dependent variable? If both variables are numeric, this can be established by looking at the correlation between the two to determine if they appear to convey. A common example is the relationship between education and income: in general, individuals with more years of education are also likely to earn higher incomes. Cross tabulation, which cross-classifies the distributions of two categorical variables, can also be used to examination association. For example, we may observe that 60% of Protestants support the death penalty while only 35% of Catholics do so, establishing an association between denomination and attitudes toward capital punishment. There is ongoing debate regarding just how closely associated variables must be to make a causal claim, but in general researchers are more concerned with the statistical significance of an association (whether it is likely to exist in the population) than with the actual strength of the association.
Once an association has been established, our attention turns to determining the time order of the variables of interest. In order for the independent variable to cause the dependent variable, logic dictates that the independent variable must occur first in time; in short, the cause must come before the effect. This time ordering is easy to ensure in an experimental design where the researcher carefully controls exposure to the treatment (which would be the independent variable) and then measures the outcome of interest (the dependent variable). In cross-sectional designs the time ordering can be much more difficult to determine, especially when the relationship between variables could reasonably go in the opposite direction. For example, although education usually precedes income, it is possible that individuals who are making a good living may finally have the money necessary to return to school. Determining time ordering thus may involve using logic, existing research, and common sense when a controlled experimental design is not possible. In any case, researchers must be very careful about specifying the hypothesized direction of the relationship between the variables and provide evidence (either theoretical or empirical) to support their claim.
The third criterion for causality is also the most troublesome, as it requires that alternative explanations for the observed relationship between two variables be ruled out. This is termed non-spuriousness, which simply means “not false.” A spurious or false relationship exists when what appears to be an association between the two variables is actually caused by a third extraneous variable. Classic examples of spuriousness include the relationship between children’s shoe sizes and their academic knowledge: as shoe size increases so does knowledge, but of course both are also strongly related to age. Another well-known example is the relationship between the number of fire fighters that respond to a fire and the amount of damage that results – clearly, the size of the fire determines both, so it is inaccurate to say that more fire fighters cause greater damage. Though these examples seem straightforward, researchers in the fields of psychology, education, and the social sciences often face much greater challenges in ruling out spurious relationships simply because there are so many other factors that might influence the relationship between two variables. Appropriate study design (using experimental procedures whenever possible), careful data collection and use of statistical controls, and triangulation of many data sources are all essential when seeking to establish non-spurious relationships between variables.
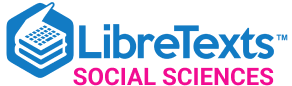
- school Campus Bookshelves
- menu_book Bookshelves
- perm_media Learning Objects
- login Login
- how_to_reg Request Instructor Account
- hub Instructor Commons
Margin Size
- Download Page (PDF)
- Download Full Book (PDF)
- Periodic Table
- Physics Constants
- Scientific Calculator
- Reference & Cite
- Tools expand_more
- Readability
selected template will load here
This action is not available.
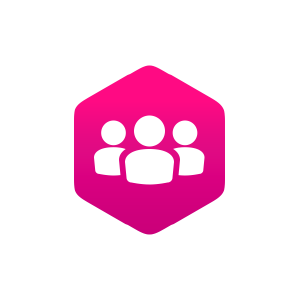
4.01: Types of research
- Last updated
- Save as PDF
- Page ID 127954
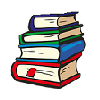
- Rebecca L. Mauldin
- University of Texas at Arlington via Mavs Open Press
\( \newcommand{\vecs}[1]{\overset { \scriptstyle \rightharpoonup} {\mathbf{#1}} } \)
\( \newcommand{\vecd}[1]{\overset{-\!-\!\rightharpoonup}{\vphantom{a}\smash {#1}}} \)
\( \newcommand{\id}{\mathrm{id}}\) \( \newcommand{\Span}{\mathrm{span}}\)
( \newcommand{\kernel}{\mathrm{null}\,}\) \( \newcommand{\range}{\mathrm{range}\,}\)
\( \newcommand{\RealPart}{\mathrm{Re}}\) \( \newcommand{\ImaginaryPart}{\mathrm{Im}}\)
\( \newcommand{\Argument}{\mathrm{Arg}}\) \( \newcommand{\norm}[1]{\| #1 \|}\)
\( \newcommand{\inner}[2]{\langle #1, #2 \rangle}\)
\( \newcommand{\Span}{\mathrm{span}}\)
\( \newcommand{\id}{\mathrm{id}}\)
\( \newcommand{\kernel}{\mathrm{null}\,}\)
\( \newcommand{\range}{\mathrm{range}\,}\)
\( \newcommand{\RealPart}{\mathrm{Re}}\)
\( \newcommand{\ImaginaryPart}{\mathrm{Im}}\)
\( \newcommand{\Argument}{\mathrm{Arg}}\)
\( \newcommand{\norm}[1]{\| #1 \|}\)
\( \newcommand{\Span}{\mathrm{span}}\) \( \newcommand{\AA}{\unicode[.8,0]{x212B}}\)
\( \newcommand{\vectorA}[1]{\vec{#1}} % arrow\)
\( \newcommand{\vectorAt}[1]{\vec{\text{#1}}} % arrow\)
\( \newcommand{\vectorB}[1]{\overset { \scriptstyle \rightharpoonup} {\mathbf{#1}} } \)
\( \newcommand{\vectorC}[1]{\textbf{#1}} \)
\( \newcommand{\vectorD}[1]{\overrightarrow{#1}} \)
\( \newcommand{\vectorDt}[1]{\overrightarrow{\text{#1}}} \)
\( \newcommand{\vectE}[1]{\overset{-\!-\!\rightharpoonup}{\vphantom{a}\smash{\mathbf {#1}}}} \)
Learning Objectives
- Differentiate between exploratory, descriptive, and explanatory research
A recent news story about college students’ addictions to electronic gadgets (Lisk, 2011) describes findings from some research by Professor Susan Moeller and colleagues from the University of Maryland . The story raises a number of interesting questions. Just what sorts of gadgets are students addicted to? How do these addictions work? Why do they exist, and who is most likely to experience them?
Social science research is great for answering just these sorts of questions. But in order to answer our questions well, we must take care in designing our research projects. In this chapter, we’ll consider what aspects of a research project should be considered at the beginning, including specifying the goals of the research, the components that are common across most research projects, and a few other considerations.

One of the first things to think about when designing a research project is what you hope to accomplish, in very general terms, by conducting the research. What do you hope to be able to say about your topic? Do you hope to gain a deep understanding of whatever phenomenon it is that you’re studying, or would you rather have a broad, but perhaps less deep, understanding? Do you want your research to be used by policymakers or others to shape social life, or is this project more about exploring your curiosities? Your answers to each of these questions will shape your research design.
Exploration, description, and explanation
You’ll need to decide in the beginning phases whether your research will be exploratory, descriptive, or explanatory. Each has a different purpose, so how you design your research project will be determined in part by this decision.
Researchers conducting exploratory research are typically at the early stages of examining their topics. These sorts of projects are usually conducted when a researcher wants to test the feasibility of conducting a more extensive study and to figure out the “lay of the land” with respect to the particular topic. Perhaps very little prior research has been conducted on this subject. If this is the case, a researcher may wish to do some exploratory work to learn what method to use in collecting data, how best to approach research subjects, or even what sorts of questions are reasonable to ask. A researcher wanting to simply satisfy her own curiosity about a topic could also conduct exploratory research. In the case of the study of college students’ addictions to their electronic gadgets, a researcher conducting exploratory research on this topic may simply wish to learn more about students’ use of these gadgets. Because these addictions seemed to be a relatively new phenomenon, an exploratory study of the topic made sense as an initial first step toward understanding it.
It is important to note that exploratory designs do not make sense for topic areas with a lot of existing research. For example, the question “What are common interventions for parents who neglect their children?” would not make much sense as a research question. One could simply look at journal articles and textbooks to see what interventions are commonly used with this population. Exploratory questions are best suited to topics that have not been studied. Students may sometimes say there is not much literature on their chosen topic, when there is in fact a large body of literature on that topic. However, that said, there are a few students each semester who pick a topic for which there is little existing research. Perhaps, if you were looking at child neglect interventions for parents who identify as transgender or parents who are refugees from the Syrian civil war, less would be known about child neglect for those specific populations. In that case, an exploratory design would make sense as there is less literature to guide your study.
Descriptive research is used to describe or define a particular phenomenon. For example, a social work researcher may want to understand what it means to be a first-generation college student or a resident in a psychiatric group home. In this case, descriptive research would be an appropriate strategy. A descriptive study of college students’ addictions to their electronic gadgets, for example, might aim to describe patterns in how many hours students use gadgets or which sorts of gadgets students tend to use most regularly.
Researchers at the Princeton Review conduct descriptive research each year when they set out to provide students and their parents with information about colleges and universities around the United States. They describe the social life at a school, the cost of admission, and student-to-faculty ratios (to name just a few of the categories reported). Although students and parents may be able to obtain much of this information on their own, having access to the data gathered by a team of researchers is much more convenient and less time consuming.

Social workers often rely on descriptive research to tell them about their service area. Keeping track of the number of children receiving foster care services, their demographic makeup (e.g., race, gender), and length of time in care are excellent examples of descriptive research. On a more macro-level, the Centers for Disease Control provides a remarkable amount of descriptive research on mental and physical health conditions. In fact, descriptive research has many useful applications, and you probably rely on findings from descriptive research without even being aware that that is what you are doing.
Finally, social work researchers often aim to explain why particular phenomena work in the way that they do. Research that answers “why” questions is referred to as explanatory research. In this case, the researcher is trying to identify the causes and effects of whatever phenomenon she is studying. An explanatory study of college students’ addictions to their electronic gadgets might aim to understand why students become addicted. Does it have anything to do with their family histories? With their other extracurricular hobbies and activities? With whom they spend their time? An explanatory study could answer these kinds of questions.
There are numerous examples of explanatory social scientific investigations. For example, in one study, Dominique Simons and Sandy Wurtele (2010) sought to discover whether receiving corporal punishment from parents led children to turn to violence in solving their interpersonal conflicts with other children. In their study of 102 families with children between the ages of 3 and 7, the researchers found that experiencing frequent spanking did, in fact, result in children being more likely to accept aggressive problem-solving techniques. Another example of explanatory research can be seen in Robert Faris and Diane Felmlee’s (2011) research on the connections between popularity and bullying. From their study of 8th, 9th, and 10th graders in 19 North Carolina schools, they found that aggression increased as adolescents’ popularity increased. (This pattern was found until adolescents reached the top 2% in the popularity ranks. After that, aggression declines).
The choice between descriptive, exploratory, and explanatory research should be made with your research question in mind. What does your question ask? Are you trying to learn the basics about a new area, establish a clear “why” relationship, or define or describe an activity or concept? In the next section, we will explore how each type of research is associated with different methods, paradigms, and forms of logic.
Key Takeaways
- Exploratory research is usually conducted when a researcher has just begun an investigation and wishes to understand the topic generally.
- Descriptive research is research that aims to describe or define the topic at hand.
- Explanatory research is research that aims to explain why particular phenomena work in the way that they do.
- Descriptive research- research that describes or define a particular phenomenon
- Explanatory research- explains why particular phenomena work in the way that they do, answers “why” questions
- Exploratory research- conducted during the early stages of a project, usually when a researcher wants to test the feasibility of conducting a more extensive study
Image attributions
Pencil by kaboompics CC-0
Two men and one woman in a photo by Rawpixel.com CC-0
- Search Menu
Sign in through your institution
- Browse content in Arts and Humanities
- Browse content in Archaeology
- Historical Archaeology
- Browse content in Architecture
- History of Architecture
- Browse content in Art
- History of Art
- Browse content in Classical Studies
- Classical Literature
- Religion in the Ancient World
- Browse content in History
- Colonialism and Imperialism
- History by Period
- Intellectual History
- Military History
- Political History
- Regional and National History
- Social and Cultural History
- Theory, Methods, and Historiography
- Browse content in Literature
- Literary Studies (European)
- Literary Studies (Romanticism)
- Literary Studies - World
- Literary Studies (19th Century)
- Literary Studies (African American Literature)
- Literary Studies (Early and Medieval)
- Literary Studies (Poetry and Poets)
- Literary Studies (Women's Writing)
- Literary Theory and Cultural Studies
- Mythology and Folklore
- Shakespeare Studies and Criticism
- Media Studies
- Browse content in Music
- Musical Structures, Styles, and Techniques
- Musicology and Music History
- Browse content in Philosophy
- Aesthetics and Philosophy of Art
- History of Western Philosophy
- Metaphysics
- Moral Philosophy
- Philosophy of Science
- Philosophy of Language
- Philosophy of Religion
- Social and Political Philosophy
- Browse content in Religion
- Biblical Studies
- Christianity
- History of Religion
- Judaism and Jewish Studies
- Religious Studies
- Society and Culture
- Browse content in Law
- Company and Commercial Law
- Comparative Law
- Constitutional and Administrative Law
- Criminal Law
- History of Law
- Browse content in Science and Mathematics
- Browse content in Biological Sciences
- Aquatic Biology
- Biochemistry
- Ecology and Conservation
- Evolutionary Biology
- Genetics and Genomics
- Molecular and Cell Biology
- Zoology and Animal Sciences
- Browse content in Computer Science
- Programming Languages
- Environmental Science
- History of Science and Technology
- Browse content in Mathematics
- Applied Mathematics
- History of Mathematics
- Mathematical Education
- Mathematical Finance
- Mathematical Analysis
- Numerical and Computational Mathematics
- Probability and Statistics
- Pure Mathematics
- Browse content in Neuroscience
- Cognition and Behavioural Neuroscience
- Development of the Nervous System
- Browse content in Physics
- Astronomy and Astrophysics
- Biological and Medical Physics
- Computational Physics
- Condensed Matter Physics
- History of Physics
- Mathematical and Statistical Physics
- Browse content in Psychology
- Cognitive Neuroscience
- Social Psychology
- Browse content in Social Sciences
- Browse content in Anthropology
- Anthropology of Religion
- Regional Anthropology
- Social and Cultural Anthropology
- Browse content in Business and Management
- Business History
- Industry Studies
- International Business
- Knowledge Management
- Public and Nonprofit Management
- Criminology and Criminal Justice
- Browse content in Economics
- Asian Economics
- Behavioural Economics and Neuroeconomics
- Econometrics and Mathematical Economics
- Economic History
- Economic Development and Growth
- Financial Markets
- Financial Institutions and Services
- History of Economic Thought
- International Economics
- Macroeconomics and Monetary Economics
- Microeconomics
- Browse content in Education
- Higher and Further Education
- Browse content in Politics
- Asian Politics
- Comparative Politics
- Conflict Politics
- Environmental Politics
- International Relations
- Political Sociology
- Political Economy
- Political Theory
- Public Policy
- Security Studies
- UK Politics
- US Politics
- Browse content in Regional and Area Studies
- Middle Eastern Studies
- Native American Studies
- Browse content in Social Work
- Social Work and Crime and Justice
- Browse content in Sociology
- Comparative and Historical Sociology
- Economic Sociology
- Gender and Sexuality
- Health, Illness, and Medicine
- Migration Studies
- Occupations, Professions, and Work
- Population and Demography
- Race and Ethnicity
- Social Theory
- Social Movements and Social Change
- Social Research and Statistics
- Social Stratification, Inequality, and Mobility
- Sociology of Religion
- Sociology of Education
- Urban and Rural Studies
- Reviews and Awards
- Journals on Oxford Academic
- Books on Oxford Academic

- < Previous chapter
- Next chapter >
3 Causes-of-Effects versus Effects-of-Causes
- Published: September 2012
- Cite Icon Cite
- Permissions Icon Permissions
This chapter examines two approaches used in social science research: the “causes-of-effects” approach and the “effects-of-causes” approach. The quantitative and qualitative cultures differ in the extent to which and the ways in which they address causes-of-effects and effects-of-causes questions. Quantitative scholars, who favor the effects-of-causes approach, focus on estimating the average effects of particular variables within populations or samples. By contrast, qualitative scholars employ individual case analysis to explain outcomes as well as the effects of particular causal factors. The chapter first considers the type of research question addressed by both quantitative and qualitative researchers before discussing the use of within-case analysis by the latter to investigate individual cases versus cross-case analysis by the former to elucidate central tendencies in populations. It also describes the complementarities between qualitative and quantitative research that make mixed-method research possible.
Personal account
- Sign in with email/username & password
- Get email alerts
- Save searches
- Purchase content
- Activate your purchase/trial code
- Add your ORCID iD
Institutional access
Sign in with a library card.
- Sign in with username/password
- Recommend to your librarian
- Institutional account management
- Get help with access
Access to content on Oxford Academic is often provided through institutional subscriptions and purchases. If you are a member of an institution with an active account, you may be able to access content in one of the following ways:
IP based access
Typically, access is provided across an institutional network to a range of IP addresses. This authentication occurs automatically, and it is not possible to sign out of an IP authenticated account.
Choose this option to get remote access when outside your institution. Shibboleth/Open Athens technology is used to provide single sign-on between your institution’s website and Oxford Academic.
- Click Sign in through your institution.
- Select your institution from the list provided, which will take you to your institution's website to sign in.
- When on the institution site, please use the credentials provided by your institution. Do not use an Oxford Academic personal account.
- Following successful sign in, you will be returned to Oxford Academic.
If your institution is not listed or you cannot sign in to your institution’s website, please contact your librarian or administrator.
Enter your library card number to sign in. If you cannot sign in, please contact your librarian.
Society Members
Society member access to a journal is achieved in one of the following ways:
Sign in through society site
Many societies offer single sign-on between the society website and Oxford Academic. If you see ‘Sign in through society site’ in the sign in pane within a journal:
- Click Sign in through society site.
- When on the society site, please use the credentials provided by that society. Do not use an Oxford Academic personal account.
If you do not have a society account or have forgotten your username or password, please contact your society.
Sign in using a personal account
Some societies use Oxford Academic personal accounts to provide access to their members. See below.
A personal account can be used to get email alerts, save searches, purchase content, and activate subscriptions.
Some societies use Oxford Academic personal accounts to provide access to their members.
Viewing your signed in accounts
Click the account icon in the top right to:
- View your signed in personal account and access account management features.
- View the institutional accounts that are providing access.
Signed in but can't access content
Oxford Academic is home to a wide variety of products. The institutional subscription may not cover the content that you are trying to access. If you believe you should have access to that content, please contact your librarian.
For librarians and administrators, your personal account also provides access to institutional account management. Here you will find options to view and activate subscriptions, manage institutional settings and access options, access usage statistics, and more.
Our books are available by subscription or purchase to libraries and institutions.
Month: | Total Views: |
---|---|
October 2022 | 8 |
November 2022 | 2 |
December 2022 | 2 |
January 2023 | 5 |
February 2023 | 5 |
March 2023 | 4 |
April 2023 | 5 |
May 2023 | 4 |
June 2023 | 11 |
July 2023 | 2 |
August 2023 | 13 |
September 2023 | 8 |
October 2023 | 5 |
November 2023 | 4 |
December 2023 | 5 |
January 2024 | 15 |
February 2024 | 7 |
March 2024 | 4 |
April 2024 | 8 |
May 2024 | 6 |
June 2024 | 7 |
- About Oxford Academic
- Publish journals with us
- University press partners
- What we publish
- New features
- Open access
- Rights and permissions
- Accessibility
- Advertising
- Media enquiries
- Oxford University Press
- Oxford Languages
- University of Oxford
Oxford University Press is a department of the University of Oxford. It furthers the University's objective of excellence in research, scholarship, and education by publishing worldwide
- Copyright © 2024 Oxford University Press
- Cookie settings
- Cookie policy
- Privacy policy
- Legal notice
This Feature Is Available To Subscribers Only
Sign In or Create an Account
This PDF is available to Subscribers Only
For full access to this pdf, sign in to an existing account, or purchase an annual subscription.
Thank you for visiting nature.com. You are using a browser version with limited support for CSS. To obtain the best experience, we recommend you use a more up to date browser (or turn off compatibility mode in Internet Explorer). In the meantime, to ensure continued support, we are displaying the site without styles and JavaScript.
- View all journals
- Explore content
- About the journal
- Publish with us
- Sign up for alerts
- Open access
- Published: 10 July 2024
Myofibroblasts derived type V collagen promoting tissue mechanical stress and facilitating metastasis and therapy resistance of lung adenocarcinoma cells
- Guangsheng Zhu ORCID: orcid.org/0000-0001-7580-1986 1 na1 ,
- Yanan Wang 1 na1 ,
- Yingjie Wang 1 na1 ,
- Hua Huang 1 ,
- Boshi Li 1 ,
- Peijie Chen 1 ,
- Chen Chen 2 ,
- Hongbing Zhang 1 ,
- Yongwen Li 2 ,
- Hongyu Liu 2 &
- Jun Chen ORCID: orcid.org/0000-0001-9552-4429 1 , 2
Cell Death & Disease volume 15 , Article number: 493 ( 2024 ) Cite this article
Metrics details
- Cancer microenvironment
- Non-small-cell lung cancer
- Prognostic markers
Lung cancer is a leading cause of cancer-related mortality globally, with a dismal 5-year survival rate, particularly for Lung Adenocarcinoma (LUAD). Mechanical changes within the tumor microenvironment, such as extracellular matrix (ECM) remodeling and fibroblast activity, play pivotal roles in cancer progression and metastasis. However, the specific impact of the basement membrane (BM) on the mechanical characteristics of LUAD remains unclear. This study aims to identify BM genes influencing internal mechanical stress in tumors, elucidating their effects on LUAD metastasis and therapy resistance, and exploring strategies to counteract these effects. Using Matrigel overlay and Transwell assays, we found that mechanical stress, mimicked by matrix application, augmented LUAD cell migration and invasion, correlating with ECM alterations and activation of the epithelial-mesenchymal transition (EMT) pathway. Employing machine learning, we developed the SVM_Score model based on relevant BM genes, which accurately predicted LUAD patient prognosis and EMT propensity across multiple datasets. Lower SVM_Scores were associated with worse survival outcomes, elevated cancer-related pathways, increased Tumor Mutation Burden, and higher internal mechanical stress in LUAD tissues. Notably, the SVM_Score was closely linked to COL5A1 expression in myofibroblasts, a key marker of mechanical stress. High COL5A1 expression from myofibroblasts promoted tumor invasiveness and EMT pathway activation in LUAD cells. Additionally, treatment with Sorafenib, which targets COL5A1 secretion, attenuated the tumor-promoting effects of myofibroblast-derived COL5A1, inhibiting LUAD cell proliferation, migration, and enhancing chemosensitivity. In conclusion, this study elucidates the complex interplay between mechanical stress, ECM alterations, and LUAD progression. The SVM_Score emerges as a robust prognostic tool reflecting tumor mechanical characteristics, while Sorafenib intervention targeting COL5A1 secretion presents a promising therapeutic strategy to mitigate LUAD aggressiveness. These findings deepen our understanding of the biomechanical aspects of LUAD and offer insights for future research and clinical applications.
Introduction
Lung cancer is the most common cancer, accounting for 11.6% of all newly diagnosed malignancies. It is dominated by non-small cell lung cancer (NSCLC), which accounts for approximately 85% of lung cancer cases [ 1 , 2 ]. Lung cancer is the leading cause of male and female cancer deaths in over 90 countries, partly due to its high mortality rate [ 1 ]. Lung adenocarcinoma (LUAD), which is one of the significant NSCLC histology types, has increased in prevalence compared to other lung cancer subtypes [ 3 ], and the 5-year survival rate is only approximately 5% for LUAD.
The changes in the mechanical environment produce deformation and movement effects on tissue cells and lead to complex physiological and pathological physiological changes [ 4 ], including lung cancer. Emerging evidence suggests that mechanical alterations and an abnormal microenvironment may cooperatively drive cancer cell aggression and treatment resistance [ 5 ]. The tumor microenvironment plays an important role during tumor progression and runs throughout [ 6 ]. The pathological changes in the tumor microenvironment, including inflammatory exudation, cell infiltration and contraction, and extracellular matrix remodeling, promote tumor progression while also altering the mechanical characteristics of the tumor [ 7 , 8 ]. Among them, the contraction of fibroblasts and the hardening of the extracellular matrix are essential factors that alter the mechanical characteristics of the tumor microenvironment [ 7 ].
The extracellular matrix (ECM) is involved in a variety of activities, such as regulating cell growth, migration, and differentiation, and also a major tumor-stroma component [ 9 , 10 ]. Meanwhile, the quantity and cross-linking status of ECM components determine tissue stiffness. The physical reorganization of collagen causes the ECM to gradually while the internal stress of the tumor increases. Eventually, due to the hardening of the stroma and the change in stress [ 11 , 12 ], the tumor cell polarity and the intercellular adhesion changes, leading to tumor cell migration along the hardened collagen fibers toward the stroma and facilitating distant metastasis of the tumor [ 11 , 13 ].
The basement membrane (BM), a special ECM arranged on the bottom side of epithelial and endothelial tissues, stabilizes the tissue structure and is essential for biological behaviors such as cell proliferation, differentiation, angiogenesis, and tissue repair [ 14 , 15 ]. In addition, aberrant BM gene expression is thought to be associated with diseases such as tumors. Tumor cells are thought to have a greater ability to migrate and invade when they break through the BM [ 16 ]. Epithelial tissue formation during animal development depends on mechanical force generation and the sensitivity of cells to mechanical stress [ 17 ], and the BM is inevitably subjected to mechanical stress as an extracellular matrix. During malignant tumor progression, tumor cells induce the generation of intracellular contractile forces through adhesion to the pericellular matrix, altering the mechanical stresses in the TME, and an increase in tumor-associated collagen prompts tumor cell adaptation to the mechanical changes induced by the mechanical stresses on the BM [ 18 ]. Mechanical stresses within the tumor stiffen the BM increasing the risk of invasive cancer development. Tumor stromal sitffening may further promote the migration and invasiveness of malignant cells [ 19 ].
In contrast, sorafenib, as an oral multikinase inhibitor, blocks TGF-β signaling, decreasing the expression of collagen and pro-fibrotic genes, resulting in a reduction of tumor-stroma stiffness and a weakening of inter-tumor stress. This inhibits the fibrosis process, reduces the proliferation of fibroblasts and the production of Epithelial-Mesenchymal Transition (EMT), attenuates the accumulation effect of ECM, and inhibits tumor migration and invasion [ 20 , 21 ]. Therefore, sorafenib has the potential to reduce the internal mechanical stress of tumors.
Understanding how changes in the tumor microenvironment’s mechanical characteristics affect tumors’ biological characteristics and clarifying the signaling pathways related to cellular mechanical mechanics is an urgent page to be completed. Simultaneously, BM genes are closely associated with modulating mechanical stress within tumors. Therefore, this study will commence from the perspective of BM genes to investigate the mechanisms by which internal mechanical stress influences tumors.
Cell culture and transfection
H1975 and A549 LUAD cell lines were obtained from the ATCC and cultured in RPMI 1640 and DMEM medium, respectively, supplemented with 10% FBS and maintained at 37 °C in a 5% CO 2 humidified atmosphere. After cell seeding, a layer of extracellular 17 mg/ml Matrigel was overlaid to impart mechanical stress [ 22 ]. To perform the transfection, siRNA explicitly targeting COL5A1 or a scrambled control siRNA (Ribobio,) was transfected using Lipofectamine 2000 (Invitrogen, USA) following the manufacturer’s instructions. The siRNAs targeting COL5A1 were sense: 5′-GGGAUUCCUUCAAGGUUUATT-3′, antisense: 5′-UAAACCUUGAAGGAAUCCCTT-3′.
Western blot
Protein samples were extracted and quantified, and agarose gel electrophoresis was performed using 10% separating gels. Proteins were transferred onto PVDF membranes (Millipore, Billerica, MA, USA) using a semidry transfer system. The membranes were then blocked with 5% skim milk at room temperature for 2 h. The primary antibodies, anti-beta-catenin (Affinity, BF8016, 1:1000), anti-Snail (CST, 3879S, 1:1000), anti-E-cadherin (BD, 810182S, 1:1000), anti-Fibronectin (CST, 26836, 1:1000), anti-Vimentin (Pronteintech, 10366-1-AP, 1:1000), anti-GAPDH(Pronteintech, 60004-1-lg, 1:1000) were incubated with membranes overnight at 4 °C. Subsequently, the secondary antibody was added and(1:5000 dilution; Thermo Fisher Scientific, Inc.) incubated for 1 h at room temperature. The bands were visualized using the Pierce ECL substrate (Thermo Fisher Scientific, Inc.).
Immunofluorescence and immunohistochemistry (IHC)
LUAD cells were seeded on 12-well plates with coverslips, fixed in 4% paraformaldehyde, permeabilized with 0.5% Triton X-100, blocked with 1% BSA, and incubated with the primary antibodies, such as anti-panCK (Abcam, ab7753, 1:1000), anti-COL5A1(Immunoway, YT1030, 1:200), anti-Vimentin (Pronteintech, 10366-1-AP, 1:200), anti-alpha-SMA (Immunoway, Y5053, 1:200)) overnight at 4 °C. After washing, cells were incubated with the fluorescent secondary antibody, and coverslips were mounted using a fluorescence quenching mounting medium with DAPI. Imaging was performed using a fluorescent microscope. IHC staining of paraffin-embedded tissues with primary antibodies (anti-COL5A1(Immunoway, YT1030, 1:200), anti-Vimentin (Pronteintech, 10366-1-AP, 1:200), anti-alpha-SMA (Immunoway, Y5053, 1:200), and anti-Ki67 (Abcam, ab1667, 1:200) were performed and scored according to standard procedures. Two independent pathologists determined the staining score.
Transwell assay
Cell migration and invasion were evaluated using transwell chambers. For the migration assay, cells were seeded into the upper chamber with a serum-free medium, whereas the lower chamber was filled with a medium containing 10% fetal bovine serum. For the Matrigel group, 17 mg/ml Matrigel was added above to the cells to provide additional mechanical stress after planting the cells. After incubation for 24 h, the cells that migrated to the lower chamber were fixed with 4% paraformaldehyde and stained with crystal violet. For the invasion assay, transwell chambers were coated with Matrigel before cell seeding.
Scratch wound-healing assay
The cells were seeded into 6-well plates and cultured to confluence. A scratch wound was created using a sterile 200 μL pipette tip, and the cells were washed with phosphate-buffered saline to remove the debris. Then, the cells were incubated in a serum-free medium, and wound closure was monitored at various time points using an inverted microscope. For the Matrigel group, after planting the cells, 17 mg/ml Matrigel was added to the above cells to provide additional mechanical stress.
Data sources of the bioinformatics analyses and lung cancer tissue collection
Seven data sources were collected in our study, including the Cancer Genome Map (TCGA) database (59 normal and 515 lung adenocarcinoma samples), GSE3141 (58 lung adenocarcinoma samples), GSE26939 (86 lung adenocarcinoma samples), GSE30219 (83 lung adenocarcinoma samples), GSE31210 (226 lung adenocarcinoma samples), GSE50081 (127 lung adenocarcinoma samples), GSE131907 (11 lung adenocarcinoma samples, 25,369 cells) and GSE72094 (398 lung adenocarcinoma samples) and downloaded the transcriptional profiles of bulk RNA sequencing and single-cell RNA sequencing for all tumor samples, as well as relevant clinical information. Two hundred and twenty-two BM-related genes were derived from a recently published study [ 23 ]. To facilitate the smooth progress of the research, we obtained 35 BM-related genes for subsequent research by intersecting the seven studies above and the BM-related genes. Transcript sequencing for 33 LUAD patients was obtained from the Department of Lung Cancer Surgery of Tianjin Medical University General Hospital (TMU), and tumor specimens from ten of those patients were performed with immunohistochemistry (IHC) and polychromatic immunofluorescence. The informed consent was obtained from each patient.
Selection and understanding of candidate BM genes
First, differential expression analysis was performed on normal and lung cancer samples of TCGA-LUAD using the R package “edgR” [ 20 ] and displayed through heat maps and volcanic maps. Somatic mutations with differentially expressed BM genes were visualized using the R package “maftools” [ 24 ]. Then, to further screen for BM core genes, a univariate Cox analysis of variance was applied to determine OS-related genes.
Signature generated from machine learning-based integrative approaches
We have integrated ten machine learning algorithms and 101 algorithm combinations. The comprehensive algorithms include Random Survival Forest, Elastic Network (Enet), Lasso, Ridge, Stepwise Cox, CoxBoost, Cox Partial Least Squares Regression (plsRcox), Supervised Principal Component, Generalized Enhanced Resignation Modeling (GBM), and Survival Support Vector Machine (Survival SVM). The feature generation program is as follows: First, univariate Cox regression in the TCGA-LUAD queue was used to determine prognosis-related BM genes. Then, in the TCGA-LUAD queue, 101 algorithm combinations were performed on relevant BM genes to establish a prediction model based on the left one cross-validation (LOOCV) framework. Subsequently, all models (GSE3141, GSE26939, GSE30219, GSE31210, GSE50081, and GSE72094) were detected in six validation datasets. Finally, the Harrell consistency index (C-index) is calculated on all validation datasets for each model, and the model with the highest average C-index was considered optimal.
Bioinformatics and statistical methods
Immune microenvironment scoring [ 25 ] and immune cell infiltration analysis were performed using the GSVA package [ 26 ]. Drug sensitivity analysis was carried out using the pRRophytic package [ 27 ]. Single-cell analysis was completed by the Seurat package [ 28 ]. Cell Chat analysis was completed by the CellChat package [ 29 ]. An independent sample t-test was used to compare two sample groups. The Wilcoxon test compared multiple groups of samples, and the log-rank test was used to compare two or more survival curves. Essential scripts for implementing machine learning-based integrative procedures in multiple independent datasets are available on the GitHub website ( https://github.com/Zaoqu-Liu/IRLS ). Essential single-cell analysis scripts are available on the GitHub website ( https://github.com/Mcdull8/METArisk ). Statistical significance was defined as a p -value ≤ 0.05. **** denotes a p -value ≤ 0.0001, *** denotes a p -value ≤ 0.001, ** denotes a p -value ≤ 0.01, and * denotes a p -value ≤ 0.05.
Atomic force microscopy(AFM)
The Park NX10 atomic force microscope (manufactured by Park Systems, South Korea) was utilized for AFM measurements on each slice. Based on TL-CONT-NANOSENSORS, cantilever probes with ten μm silicon dioxide spheres were used for detection. Before each experiment, a noncontact calibration method was used to calibrate each probe’s sensitivity and spring constant. All measurements were conducted in force spectroscopy mode, and force-indentation curves were generated from an average of 20 points per sample. All force measurements’ approach and retraction speeds were set at 0.2 μm/s, with a set point force of 2.5 nN and a retraction distance of 0.5 μm. XEI data processing software was used for data analysis. The Young’s modulus was calculated from AFM force curves using the Hertz-Sneddon model to assess tissue stiffness. Young’s modulus data were plotted in GraphPad software, and intergroup differences were tested using t-tests.
Cell proliferation assay
Cell proliferation was evaluated utilizing the Cell Counting Kit-8 (CCK8) assay. Cells were plated in 96-well plates and cultured for 24, 48, and 72 h. At each time point, 10 μL of CCK8 solution was introduced to individual wells and incubated for 2 h. Subsequently, the optical density was quantified at 450 nm using a microplate reader.
Establishment and culture of LUAD tumor organoids
Tumor specimens were dissected into small pieces and incubated in a tissue digestion medium containing Advanced DMEM/F-12 (Gibco), 200 mM GlutaMAX (Gibco), 1 M HEPES (Gibco), 1× Primocin (InvivoGen), 1 mg/ml collagenase IV (Sigma-Aldrich), 100 μg/ml DNase I (AppliChem), 1× B27 (Gibco), 1 mM N-acetyl cysteine (Sigma-Aldrich), and 10 μM Y-27632 (Selleckchem). The cells were resuspended in growth factor-reduced Matrigel (Corning) and seeded in 12-well plates as 50 μl droplets. After 15 min at 37 °C, 1 mL of culture medium containing Advanced DMEM/F-12, 200 mM GlutaMAX, 1 M HEPES, 1× B27 supplement, 1 mM N-acetyl cysteine, 10% RSPO1-conditioned medium, 100 ng/ml FGF-10 (PeproTech), 100 ng/ml Noggin (PeproTech), 500 nM A83-01 (Tocris), and 1× Primocin was added. Organoids were passaged approximately every seven days by dissociation at 37 °C using TrypLE (Gibco).
Isolation and culture of myofibroblasts
Primary myofibroblasts were isolated from tumor specimens using a growth-based method [ 30 ]. During the organ separation process, tissue fragments were generated and co-cultured with fibroblast culture medium containing 10% fetal bovine serum (Gibco), 200 mM GlutaMAX (Gibco), and penicillin/streptomycin (Gibco) in RPMI medium. The culturing cancer-associated fibroblasts (CAFs) success rate was approximately 90%. CAFs were passaged approximately every 8–9 days, and their identity was confirmed through morphological assessment and immunofluorescence staining for α-SMA. CAFs were used for experiments within 4–7 passages after isolation.
Co-culture of tumor organoids and Myofibroblasts
The digestion of organoid into single cells or small aggregates (termed organoid-forming units) was performed using TrypLE Express (Gibco), supplemented with 100 μg/ml of DNase I (from AppliChem) and 10 μM of Y-27632. A 1:1 mixture of organoid-forming units and fibroblasts was prepared. Depending on the growth rate of each organoid system, approximately 2000–3000 organoid-forming units were utilized for every 10 μl of matrix. Cells were seeded into a co-culture matrix in droplet form, consisting of a gelatin solution and 3 mg/ml of collagen I (from Corning). The co-culture system was maintained in a medium containing Advanced DMEM/F12, 200 mM GlutaMAX, 1 M HEPES, 1× B27, 100 ng/ml of FGF-10, 50 ng/ml of EGF (obtained from PeproTech), and 5% RSPO1-conditioned medium [ 31 ].
Mechanical stress upregulates tumor EMT pathway and promotes tumor migration and invasion ability
We added Matrigel to the scratched LUAD cells seeded on 6-well plates to investigate the influence of mechanical stress to the migration and invasive ability of LUAD cells. The A549 and H1975 cells had enhanced migration ability after adding matrix adhesive and were significantly superior in 24-hour migration ability compared to their counterparts maintained without Matrigel (Fig. 1a ). When testing lung adenocarcinoma migration ability through the transwell experiments (Fig. 1b ), we also found that the tumor cells with added Matrigel above achieved stronger migration ability and had statistical significance. Similarly, when testing the invasive ability of lung adenocarcinoma cells through the Transwell experiment, we also found that the two types of tumor cells with added matrix glue achieved significantly stronger invasive ability than their counterparts maintained without Matrigel. (Fig. 1b ).
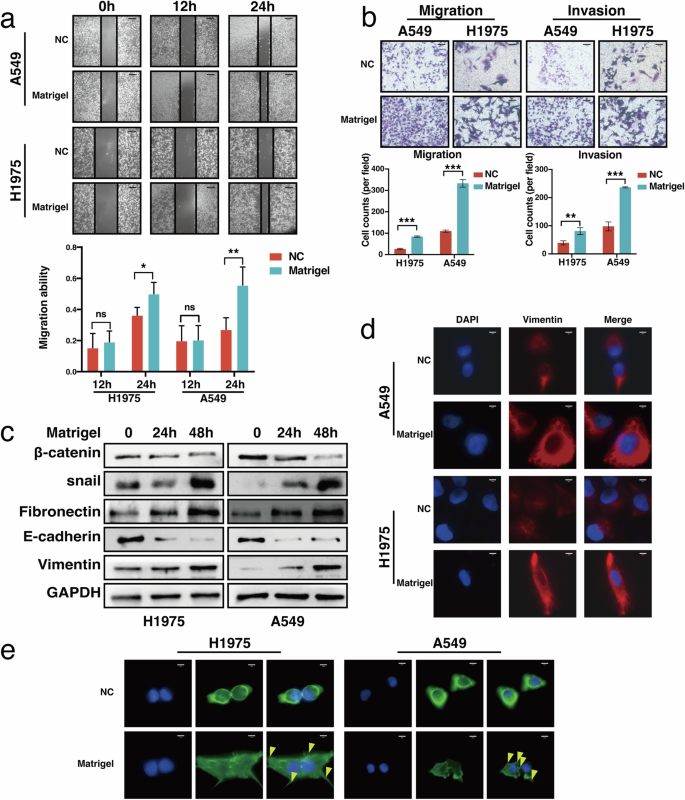
The migration and invasive ability of LUAD cells were tested by scratch wound-healing assay ( a ) and transwell assays ( b ). c EMT marker expression in response to Matrigel addition was measured with Western blot. d Vimentin expression changes were visualized with an immunofluorescence assay. e Cell morphology and b-actin alterations were visualized with Phalloidin Staining (Yellow arrow: tentacles).
The Western blot experiment showed that the EMT pathway of tumor cells was significantly upregulated when adding the matrix gel, manifested as the upregulation of Vimentin and Snail and downregulation of beta-Catenin and E-cadherin (Fig. 1c ). Additionally, the immunofluorescence experiments showed that the tumor cells with matrix glue added significantly upregulated Vimentin (Fig. 1d ). The Phalloidin experiment demonstrated that the tumor cell cultured with matrix gel changed morphology significantly and were no longer round and continuous, with a significant increase in tentacles (Fig. 1e ).
SVM_Score generated based on machine learning effectively predicts the prognosis of lung adenocarcinoma patients
A heat map between normal and lung adenocarcinoma tissues of TCGA-LUAD is shown in Supplementary Fig. 1a . The upste plot then shows 35 BM genes in all seven datasets we used (Supplementary Fig. 1b ). The volcanic map displays the differential genes between normal and lung adenocarcinoma tissues of TCGA-LUAD (Supplementary Fig. 1c ). At the same time, we conducted unit correlation analysis on these 35 BM genes and selected 14 BM genes related to the overall survival of lung adenocarcinoma for subsequent modeling by intersecting with differential gene results (Supplementary Fig. 1d ). The oncoplt showed the mutations status of these 14 genes in lung adenocarcinoma, with the FBN2 gene has the highest mutation rate of 16%. (Supplementary Fig. 1e ).
Our machine learning analysis results indicate that SVM_score, constructed using a Support Vector Machine (SVM), has the highest Concordance Index(C-index), as shown in Fig. 2a . C-index is a statistical measure used to assess a model’s discriminatory power or predictive accuracy, particularly in the context of survival analysis or time-to-event data. A higher C-index indicates a model with good discrimination. Therefore, we selected SVM_score for further analysis. Survival analysis revealed that in TCGA-LUAD (Fig. 2b ), GSE31210 (Fig. 2c ), GSE50081 (Fig. 2d ), GSE3141 (Fig. 2e ), GSE26939 (Fig. 2f ), GSE30219 (Fig. 2g ), and GSE72094 datasets (Fig. 2h ), lower SVM_score values corresponded with a shorter overall survival. Additionally, in TCGA-LUAD, GSE31210, GSE50081, and GSE30219 datasets, lower SVM_score values were associated with shorter progression-free survival (Fig. 2i ).
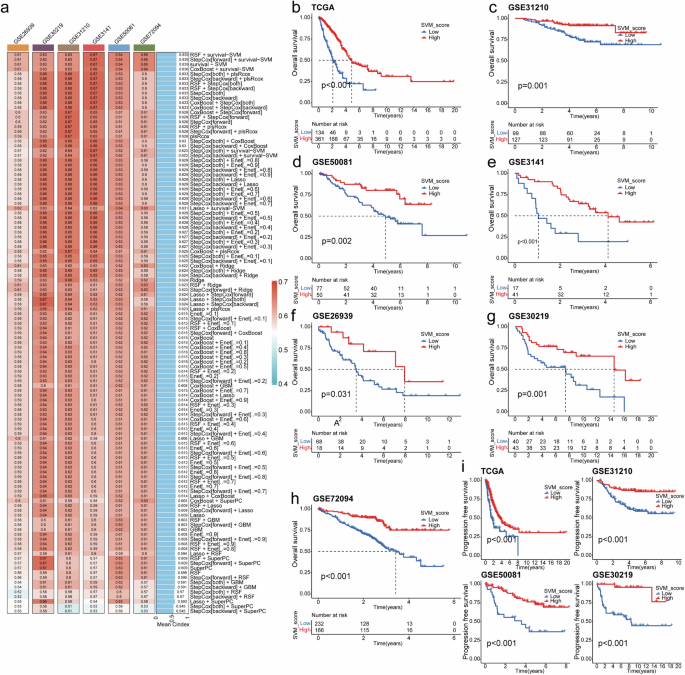
a Build and screen a prognosis prediction model with machine learning algorithms based on BM genes. b – i Kaplan–Meier survival analysis for overall survival based on SVM_Scores in various datasets, including TCGA-LUAD ( b ), GSE31210 ( c ), GSE50081 ( d ), GSE3141 ( e ), GSE26939 ( f ), GSE30219 ( g ), and GSE72094 ( h ). i Progression-free survival analysis based on SVM_Scores in TCGA-LUAD, GSE31210, GSE50081, and GSE30219.
SVM_score demonstrated robust prognostic prediction capabilities in TCGA-LUAD, GSE31210, GSE50081, GSE3141, GSE26939, GSE30219, and GSE72094, with C-index values exceeding 0.6 across all seven datasets (Fig. 3a ). Furthermore, the area under the curve (AUC) values for one-year overall survival, determined by ROC analysis, exceeded 0.7 in TCGA-LUAD (Fig. 3b ), GSE31210 (Fig. 3c ), GSE50081 (Fig. 3d ), GSE3141 (Fig. 3e ), GSE26939 (Fig. 3f ), and GSE30219 (Fig. 3g ), with GSE30219(Fig. 3h ) even surpassing 0.8. The one-year overall survival AUC reached 0.7 when combining all GEO datasets (Fig. 3i ).
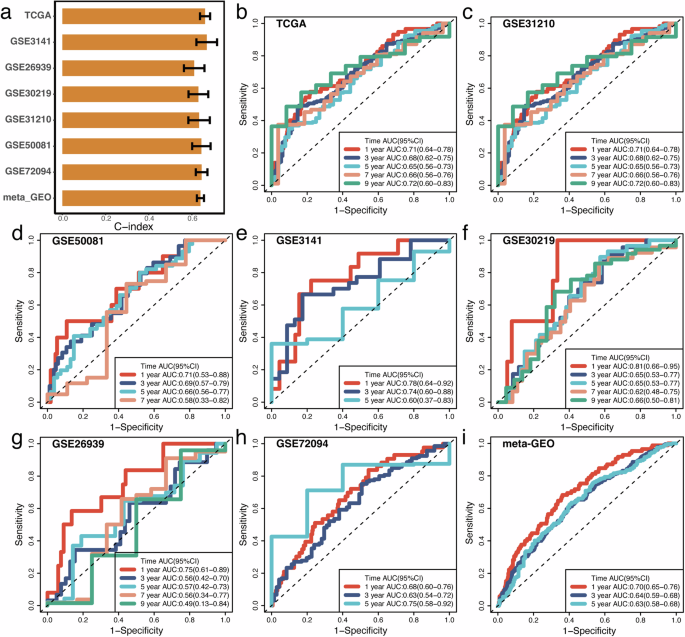
( a ) C-index Values: Comparison of C-index values for SVM_Score and other prognostic indicators, evaluating their effectiveness in predicting patient outcomes. b – i AUC Analysis with SVM_Score in the various datasets, including TCGA-LUAD ( b ), GSE31210 ( c ), GSE50081 ( d ), GSE3141 ( e ), GSE30219 ( f ), GSE26939 ( g ), GSE72094 ( h ) and meta-GEO ( i ).
The c-index of SVM_score, compared to other commonly used clinical factors in the TCGA-LUAD dataset, is slightly lower than the Stage factor but higher than all other factors (Supplementary Fig. 2a ). In the meta-GEO dataset, SVM_score outperforms all other factors, including Stage (Supplementary Fig. 2b ). Similarly, in the GSE31210 dataset, SVM_score surpasses all other factors except for Stage (Supplementary Fig. 2c ). In the GSE50081 dataset, SVM_score outperforms all other factors (Supplementary Fig. 2d ). In the GSE26939 dataset, SVM_score exceeds all other indicators except age (Supplementary Fig. 2e ). Finally, in the GSE30219 (Supplementary Fig. 2f ) and GSE70294 (Supplementary Fig. 2g ) datasets, SVM_score outperforms all other factors.
SVM_Score is associated with tumor proliferation and EMT propensity in TMU LUAD patients
We further investigate the SVM_Score in the LUAD from our institute. In the 33 cases of TMU LUAD patients, the one-year overall survival AUC value for SVM_score reached 0.87 (Fig. 4a ). Although the C-index of SVM_score may not have achieved statistical significance compared to other clinical factors, possibly due to the limited sample size, it demonstrated a stronger trend than all other clinical factors (Fig. 4b ). Survival analysis results revealed that a lower SVM_score was associated with a worse prognosis, both in terms of overall survival (Fig. 4c ) and progression-free survival (Fig. 4d ). We also assessed whether there were differences in SVM_score among various clinical factors, and the results indicated an association between SVM_score and the lymph node metastasis status of lung adenocarcinoma (Fig. 4e ).
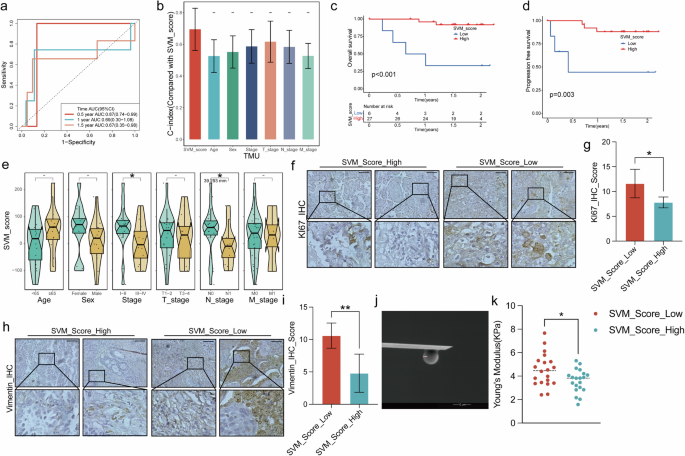
a Using SVM_Score in the performed ROC analysis on overall survival rate for TMU LUAD patients. b Comparison of C-index values and SVM_Score and other clinical factors with TMU LUAD patients. Survival analysis illustrates lower SVM_Score in the TMU LUAD patients associated with poor overall survival ( c ) and progression-free survival ( d ). e Check SVM_Scores in TMU LUAD patients stratified by clinical factors. Representative images of immunohistochemical results in ten TMU LUAD patients with low/high SVM_Scores staining with Ki67 ( f , g ) and Vimentin ( h , i ). Patients with lower SVM_Scores had significantly higher Ki67 ( g ) and Vimentin ( i ). j Electron microscopy scan image of the probe of atomic force microscope. k Comparison of tumor tissue Young’s modulus value between patients with high and low SVM_Scores.
Immunohistochemistry results further demonstrated that patients with lower SVM_score had more tumor cell expression Ki67, indicating a higher degree of malignancy consistent and statistically significant in the ten patients (Fig. 4f–g ). Additionally, tumor tissues from patients with low SVM_score showed increased expression of Vimentin, signifying higher EMT activation pathway and an increased metastasis propensity(Fig. 4h ). Again, this trend was consistent and statistically significant in all the patients (Fig. 4i ).
Atomic force microscopy was used to detect mechanical stress within the tumor tissues. Tumor tissues from patients in the lower SVM_score group had higher internal mechanical stress (Fig. 4j, k ).
The lower SVM_score is associated with lower immunogenicity
We employed GSVA to calculate scores for 25 immune-related pathways (Supplementary Appendix 1 ) in TCGA-LUAD and displayed a heatmap illustrating lower SVM_Scores in tumor tissues associated with reduced immunogenicity (Fig. 5a ). For patients with low SVM_Scores, the degree of activation of immune-related pathways is inversely correlated, indicating a lower level of immune pathway activation. We conducted separate analyses of the correlation between SVM_Scores and 27 immune cell types in TCGA and meta-GEO datasets. The results revealed statistically significant correlations between SVM scores and Activated B cell, effector memory CD8+ T cell, immature B cell, CD56dim natural killer cell, immature dendritic cell, macrophage, mast cell, and MDSC in both datasets (Fig. 5b ).
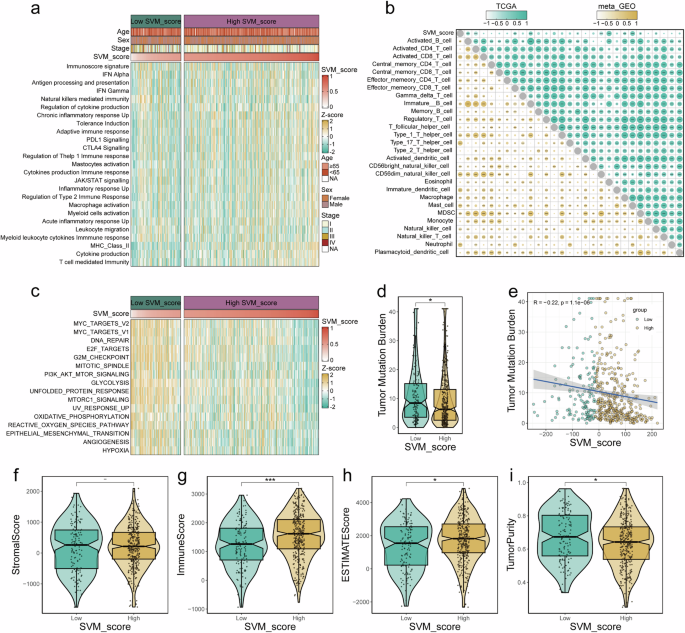
a Heatmap displays the correlation between SVM_Score and immunogenicity in TCGA-LUAD. b Correlation analysis between SVM_Score and immune cell types in TCGA and meta-GEO datasets. c GSVA scores for HALLMARK cancer-related pathways, revealing differences in cancer pathway activity. d Analysis of Tumor Mutation Burden (TMB) in relation to SVM_Score. e Negative correlation between SVM_Score and TMB. The differences between the high and low SVM_Score groups in terms of stromal score ( f ), Immune score ( g ), ESTIMATE score ( h ), and Tumor purity ( i ).
Using GSVA, we calculated scores for 50 HALLMARK cancer-related pathways (Supplementary Appendix 2 ). Our findings demonstrated that patients with lower SVM scores exhibited increased activity in cancer pathways, including the EMT pathway (Fig. 5c ). Furthermore, patients with lower SVM scores had higher Tumor Mutation Burden (TMB) (Fig. 5d ), and SVM scores exhibited a negative correlation with TMB (Fig. 5e ).
We employed ESTIMATE to calculate tumor stromal, immune, estimate, and tumor purity scores. We observed that SVM_Scores were independent of stromal scores (Fig. 5f ). Patients with higher SVM_Scores exhibited higher immune scores (Fig. 5g ) and estimate scores (Fig. 5h ), as well as lower tumor purity (Fig. 5i ).
The expression of COL5A1 of myofibroblasts influences the SVM_Score, while myofibroblasts are intricately associated with the microenvironment
To further investigate SVM_Scores among different cell populations in the LUAD patients, we applied the GSE131907 dataset, which contains single-cell sequencing data from 11 LUAD samples, including 25,369 cells. The t-SNE dimensionality reduction plot displays the types of cells in single-cell sequencing data. (Fig. 6a ). We computed an SVM_Score for each cell population and visualized it on the t-SNE plot (Fig. 6b ). The results showed that the SVM_Score in cancer-associated fibroblasts (CAFs) significantly differed from other cell types (Fig. 6a, b ). The genes contributing to the SVM_Score were also predominantly expressed in CAFs (Fig. 6c ). We presented the expression distribution of the top four genes of SVM_Score, COL5A1, GPC3, OGN, and SLT3, on the overall single-cell dimensionality reduction plot, and they were primarily localized in CAFs (Fig. 6d ). Therefore, we isolated CAFs and performed a separate t-SNE dimensionality reduction (Fig. 6e ). When visualizing the distribution of SVM_Scores, the results indicated that all myofibroblasts have lower SVM_Scores (Fig. 6f ). Moreover, myofibroblasts exhibited significantly lower SVM_Scores than all other CAFs, except for FB-like cells, which could not be statistically analyzed due to their limited numbers (Fig. 6g ). Among the genes contributing to SVM_Scores, COL5A1 showed the highest expression in myofibroblasts (Fig. 6h ). The expression distribution of COL5A1 in CAFs closely resembled the SVM_Score pattern (Fig. 6i ). In both the TCGA-LUAD cohort (Supplementary Fig. 3a ) and the TMU patients (Supplementary Fig. 3b ), COL5A1 is highly expressed in tumor tissues. The findings above regarding COL5A1 suggest that COL5A1 may serve as a pivotal biomarker linking SVM_Scores and mechanical stress.
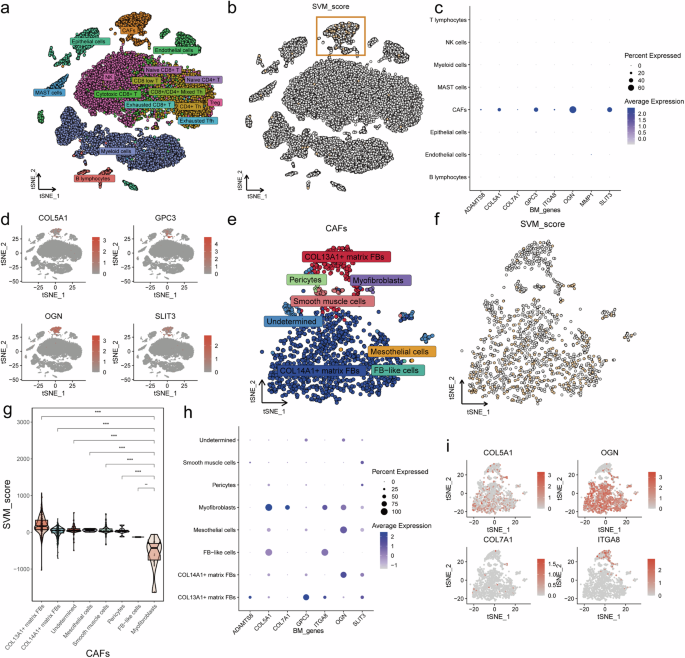
a , b t-SNE dimensionality reduction plot and SVM_Score distribution highlighting cell clustering in different cell types. c Gene expression profile of SVM_Score-associated genes within various cells. d Expression distribution of top genes (COL5A1, GPC3, OGN, and SLT3) across the single-cell dimensionality reduction plot e , f Isolation and Distribution of SVM_Score for CAFs. g Comparison of SVM_Score values in myofibroblasts with other CAF subtypes. h Gene expression profile of SVM_Score-associated genes within CAFs. i Expression distribution of top genes (COL5A1, COL7A1, ITGA8, and OGN) across the CAFs single-cell dimensionality reduction plot.
Following that, we analyzed cellular interactions in the tumor microenvironment, which revealed that myofibroblasts were the most interactive cell type with other cells (Supplementary Fig. 4a ). Furthermore, myofibroblasts exhibited the highest interaction intensity with other cells (Supplementary Fig. 4b ). We also identified that myofibroblasts primarily interact by secreting Macrophage Migration Inhibitory Factor (MIF) and binding to CD74 on the cell surface of other cells, along with CXCR4 or CD44 (Supplementary Fig. 4c ).
Notably, myofibroblasts predominantly interacted with other cells through the MIF signaling pathway (Supplementary Fig. 4d ) and the MK signaling pathway (Supplementary Fig. 4e ). Moving forward, our focus will be directed toward myofibroblasts and COL5A1.
COL5A1 from myofibroblasts increases tumor invasiveness and upregulates the EMT pathway of tumor cells
Furthermore, immunohistochemistry was applied to the ten FFPE samples of the aforementioned TMU LUAD patients. The results indicated that patients with low SVM_Score exhibit higher expression of alpha-SMA (Fig. 7a ), which is used as a marker for activated myofibroblasts, and this trend is consistently observed in all ten patient tissues, with statistical significance (Fig. 7b ). Similarly, the low SVM_Score patients demonstrate increased expression of COL5A1 (Fig. 7c ), which is consistently significant across all ten patient tissues (Fig. 7d ). Our study indicates that increased mechanical stress activates the EMT pathway (Fig. 1c, d ), concurrently highlighting the reported association between the secretion of COL5A1 and tissue mechanical stress [ 32 ]. Therefore, multicolor immunofluorescence analysis was employed to investigate the relationship between COL5A1 and the tumor cell EMT pathway in the tissues of ten lung adenocarcinoma patients. The results revealed that low SVM_score tissues exhibited higher COL5A1 expression, and near the areas with elevated COL5A1 expression, Vimentin was also highly expressed (Fig. 7e ). This trend is consistent across all ten patients and is statistically significant (Fig. 7f, g ). Furthermore, in-depth statistical analysis of multicolor immunofluorescence shows that the number of COL5A1-positive cells is directly proportional to the number of vimentin-positive cells with a p -value less than 0.0001 (Fig. 7h ).
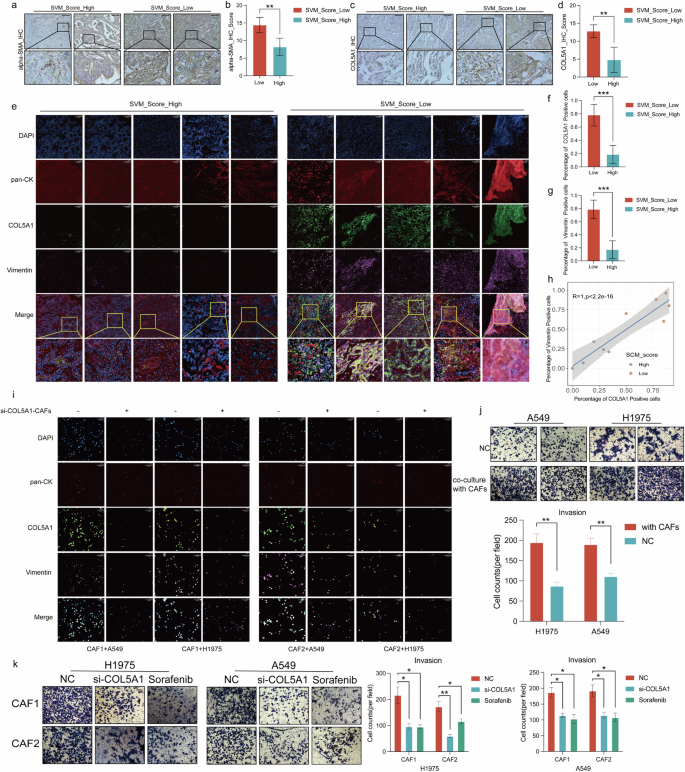
( a – d ) The immunohistochemical results showed that in the tumor tissue of the low SVM_Score group, the expression of alpha-SMA (a, b)was statistically higher than the high SVM_Score; and the expression of COL5A1was also statistically higher ( c , d ). e – g Multicolor immunofluorescence analysis provides insight into elevated COL5A1 and Vimentin expression in tissues from the lower SVM_Score group ( e ). This trend is consistently observed in all ten patients and holds statistical significance ( f , g ). h Pearson correlation analysis showed a strong correlation between the number of COL5A1-positive and vimentin-positive cells. i Co-culturing si-COL5A1 downregulated CAF cell lines with two kinds of lung adenocarcinoma cells revealed changes in surface markers COL5A1 and Vimentin expression. j The Transwell experiment detected changes in the invasive ability of lung adenocarcinoma cells co-cultured with CAFs. k The Transwell experiment detects the invasive ability of lung adenocarcinoma cells co-cultured with CAFs, especially after knocking down COL5A1 of CAFs or using Sorafenib.
Simultaneously, two myofibroblast strains, CAF1 and CAF2 (Supplementary Fig. 5a ), were extracted from the LUAD tumor tissues. Small RNA interference downregulates COL5A1 in these two myofibroblast strains(Supplementary Fig. 5b, c ). When co-cultured with tumor cells, it was observed that tumor cells located near CAFs with reduced COL5A1 expression also exhibited decreased Vimentin expression (Fig. 7i ). We employed Transwell assays to investigate alterations in the invasive capacity of lung adenocarcinoma cells co-cultured with cancer-associated fibroblasts (CAFs). The co-cultivation of lung adenocarcinoma cells with CAFs led to an increase in the invasive ability of lung adenocarcinoma cells (Fig. 7j ). However, when we knocked down COL5A1 in CAFs or treated them with Sorafenib, the invasive capacity of lung adenocarcinoma cells co-cultured with CAFs decreased compared with scrambled transfected CAFs (Fig. 7k ).
Sorafenib attenuates the tumor-promoting effect of COL5A1 from myofibroblast
Previous studies have reported that Sorafenib decreases the expression of collagen and fibronectin genes, ultimately contributing to the reduction of tumor-stroma stiffness and concurrently alleviating intertumoral stress [ 21 , 33 ]. To investigate whether Sorafenib attenuates COL5A1 from myofibroblasts, we first analyzed drug sensitivity in TCGA-LUAD, indicating a direct proportionality between the IC-50 and SVM_Score for Sorafenib, suggesting its potential use in treating patients with a poorer prognosis characterized by low SVM scores (Fig. 8a ). Subsequently, we treated two myofibroblast cell lines with Sorafenib, revealing its inhibitory effect on COL5A1 expression (Fig. 8b ). The IC-50 values for Sorafenib in these two myofibroblast cell lines were 4.133 µM/L and 3.955 µM/L, respectively (Supplementary Fig. 5d ). Therefore, we selected a nonlethal concentration of 200 nM/L of Sorafenib for further treatment. CCK-8 experiments demonstrated that A549 and H1975 cells had IC-50 values of 2.76 µM/L and 1.852 µM/L for cisplatin, respectively (Fig. 8c ). Posttreatment with 200 nM/L Sorafenib, there was minimal change in their IC-50 values for cisplatin (Fig. 8d ).
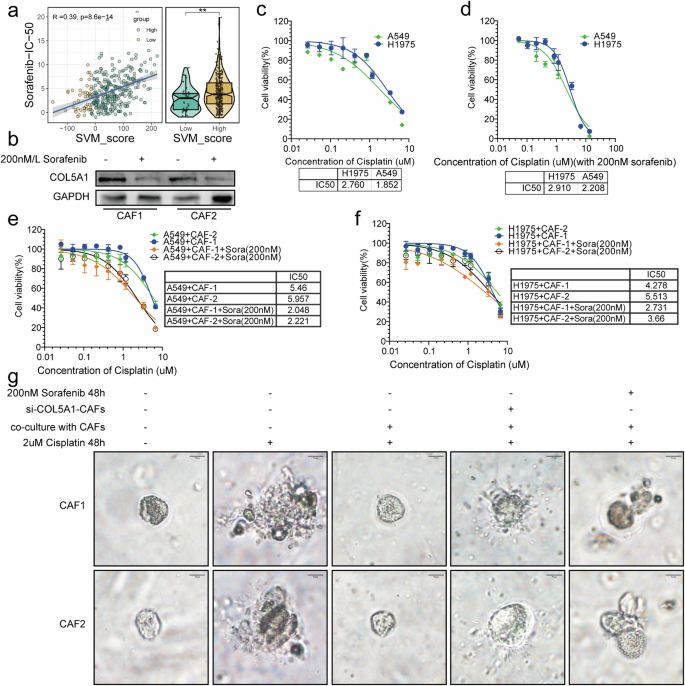
a Correlation between Sorafenib IC-50 values and SVM_Score in TCGA cohort. b Demonstration of the impact of Sorafenib on COL5A1 expression in both CAF cell lines through Western blot. Determination of IC-50 values for cisplatin in A549 and H1975 cells ( c ), highlighting the impact of Sorafenib treatment on cisplatin IC-50 in H1975 cells ( d ). e , f IC-50 values for cisplatin after co-culture with CAF cell lines, with and without Sorafenib treatment, demonstrating the impact of Sorafenib on drug sensitivity. g Analysis of the response of organoids to cisplatin in co-culture with CAF cells and COL5A1-knockdown CAF cells, with and without Sorafenib treatment, to assess changes in drug sensitivity within the tumor microenvironment.
Subsequent co-culturing of A549 and H1975 cells with the two CAF cell lines resulted in a nearly twofold increase in their IC-50 values for cisplatin (Fig. 8e, f ). However, after treatment with 200 nM/L Sorafenib, the IC-50 values reverted to their original levels (Fig. 8e, f ).
Concurrently, we established organoid models using patient-derived tissues from the same patients as the two CAF cell lines. When co-cultured with CAF cells, the organoids resisted cisplatin at 2 μM/L (Fig. 8g ). Conversely, co-culture with COL5A1-knockdown CAF cells rendered the organoids vulnerable to 2 μM/L cisplatin (Fig. 8g ). Similarly, treatment with 200 nM/L Sorafenib did not restore resistance to cisplatin when co-cultured with CAF cells (Fig. 8g ).
The microenvironment of tumor tissues differs from that of normal tissues and is characterized by alterations in both biochemical and physical parameters [ 32 ]. Recent investigations underscore that, alongside biochemical cues, physical signals substantially influence cellular behaviors, such as proliferation and metastatic potential. Solid tumors exhibit distinct stiffening features, with tissue stiffness as an indicator for various human cancer types [ 34 ]. Tissue stiffness, as a biomechanical hallmark of solid tumors, has been the focus of extensive research and is believed to play a pivotal role in modulating diverse tumor characteristics, including growth, metabolism, invasion, metastasis, and therapy resistance [ 35 , 36 ]. Meanwhile, as the major factors determining tissue stiffness, the quantity and cross-linking status of ECM components play crucial roles in malignant transformation and tumor progression. Notably, breast cancer tissue demonstrates a hardness approximately tenfold higher than that of normal breast tissue [ 37 ].
In contrast, the hardness of liver cancer tissue is analogously increased compared to normal liver tissue, owing to the association with chronic liver diseases that lead to hepatocellular carcinoma [ 38 ]. The activation of hepatic stellate cells in response to liver damage contributes to extensive ECM accumulation, thereby facilitating the progression of liver fibrosis, cirrhosis, and hepatocellular carcinoma [ 39 ]. Nevertheless, the precise mechanisms underlying how ECM stiffening propels tumor progression remain to be elucidated.
As demonstrated in our in vitro experiments, elevated mechanical stress remarkably enhances the migratory and invasive capabilities of LUAD cells. This underlines the multifaceted influence of mechanical cues on cancer cell behavior and characteristics, establishing mechanical stress as a pivotal player in tumor development.
To decipher the intricate interplay between genetic factors and the mechanical microenvironment, we introduced the SVM_Score. Constructed using Support Vector Machine analysis of basement membrane (BM)-related genes, this score emerged as a robust prognostic tool. The consistently high C-index across various datasets attests to its reliability and discriminative power. By validating various datasets, SVM_Score demonstrated robust prognostic prediction capabilities, with lower SVM_Score values corresponding to shorter overall and progression-free survival. SVM_Scores provide crucial prognostic insights and signify a paradigm shift in integrating genomic information with mechanical dynamics for personalized treatment strategies. A low SVM_Score also tends to have higher Ki67 positive and increased expression of Vimentin and indicates that SVM_Score is associated with tumor proliferation and EMT propensity in our in-house LUAD patients cohort. We also found that the tissues with different SVM_Scores have different mechanical properties, evidenced by using atomic force microscopy, and low SVM_Scores tend to have higher tissue stiffness. This demonstrated that SVM_Score is a factor that reflects the tissue mechanical characteristics and that the tissue mechanical properties are associated with prognoses and biological behavior, such as migration, invasion, and drug resistance of tumor cells.
By analyzing single-cell sequencing data from LUAD patients, we identified that myofibroblasts from the tumor tissues showed the lowest SVM_Score among all the cell populations. Our investigation revealed that collagen from myofibroblasts, with particular emphasis on COL5A1, plays crucial roles for SVM_Score. By analyzing the FFPE samples from LUAD patients, we found that LUAD tissues with Low SVM_Scores tend to have more myofibroblasts and COL5A1 and high Vimentin expression. Furthermore, in vitro experiment using siRNA knocking down the COL5A1 expression of myofibroblasts and co-culturing with lung adenocarcinoma cells, the Vimentin expression was downregulated, as well as the migration and invasion ability of lung adenocarcinoma cells.
Our research revealed the pivotal role of COL5A1 in influencing the mechanical properties of the tumor microenvironment. Our findings illuminate the intricate relationship between COL5A1, mechanical stress, and the activation of the Epithelial-Mesenchymal Transition (EMT) pathway, offering critical insights into LUAD progression. Both experiments on lung cancer patient tissues and in vitro experiments on lung cancer cells have demonstrated the role of COL5A1 in regulating the mechanical properties of the tumor microenvironment. Meanwhile, this is consistent with previously published research, where COL5A1 is closely related to the mechanical stress of tissues [ 40 ].
Our research found that by inhibiting COL5A1, Sorafenib-treated myofibroblasts inhibited adenocarcinoma cells’ invasion ability and sensitized adenocarcinoma cells to cisplatin. This inhibition of fibrosis makes Sorafenib a promising drug for regulating mechanical stress in tumors and inhibiting tumor malignancy. Therefore, our study provides a theoretical basis for applying Sorafenib to lung cancer.
The strong association between SVM_Scores and patient outcomes underscores the clinical relevance of our findings. Our results indicate that lower SVM_Scores correlate with shorter overall and progression-free survival, outperforming traditional clinical factors in certain datasets. Moreover, the lower immunogenicity observed in tumors with low SVM_Scores suggests a potential avenue for immunotherapeutic interventions.
Identifying COL5A1 as a key biomarker linking SVM_Scores and mechanical stress provides a foundation for further research and potential therapeutic targeting. The comprehensive analysis of cellular interactions within the tumor microenvironment, especially the prominence of myofibroblasts and their intricate communication pathways, opens new avenues for understanding the stromal dynamics in LUAD.
Conclusions
Our study advances the understanding of the intricate interplay between mechanical stress, SVM_Scores, and collagen secretion from myofibroblasts in LUAD. Integrating innovative prognostic tools, mechanistic insights into tumor-stroma interactions, and identifying Sorafenib as a modulator of COL5A1 expression collectively contribute to the evolving landscape of precision oncology. This research lays the groundwork for future investigations to translate these findings into clinically relevant therapeutic strategies for patients with LUAD.
Data availability
The datasets [TCGA dataset] for this study can be found in the [cBioPortal] [ http://www.cbioportal.org/ ]. The datasets [GSE3141 /GSE26939/GSE30219/GSE31210/GSE50081/GSE131907/GSE72094] for this study can be found in the [GEO Datasets] [ https://www.ncbi.nlm.nih.gov ]. The raw sequence data of TMU dataset reported in this paper have been deposited in the Genome Sequence Archive in National Genomics Data Center, China National Center for Bioinformation, Chinese Academy of Sciences (GSA-Human: HRA006222) that are publicly accessible at https://ngdc.cncb.ac.cn/gsa-human . The other data can be obtained from the corresponding author upon reasonable request.
Bray F, Ferlay J, Soerjomataram I, Siegel RL, Torre LA, Jemal A. Global cancer statistics 2018: GLOBOCAN estimates of incidence and mortality worldwide for 36 cancers in 185 countries. CA Cancer J Clin. 2018;68:394–424.
Article PubMed Google Scholar
Friedlaender A, Addeo A, Russo A, Gregorc V, Cortinovis D, Rolfo CD. Targeted therapies in early stage NSCLC: hype or hope? Int J Mol Sci. 2020;21::6329.
Meza R, Meernik C, Jeon J, Cote ML. Lung cancer incidence trends by gender, race and histology in the United States, 1973-2010. PLoS ONE. 2015;10:e0121323 https://doi.org/10.1371/journal.pone.0121323 .
Article CAS PubMed PubMed Central Google Scholar
Kai F, Laklai H, Weaver VM. Force matters: biomechanical regulation of cell invasion and migration in disease. Trends Cell Biol. 2016;26:486–97. https://doi.org/10.1016/j.tcb.2016.03.007 .
Hanahan D, Weinberg RA. Hallmarks of cancer: the next generation. Cell. 2011;144:646–74. https://doi.org/10.1016/j.cell.2011.02.013 . PMID: 21376230.
Article CAS PubMed Google Scholar
Quail DF, Joyce JA. Microenvironmental regulation of tumor progression and metastasis. Nat Med. 2013;19:1423–37. https://doi.org/10.1038/nm.3394 .
Lampi MC, Reinhart-King CA. Targeting extracellular matrix stiffness to attenuate disease: from molecular mechanisms to clinical trials. Sci Transl Med. 2018;10:eaao0475 https://doi.org/10.1126/scitranslmed.aao0475 .
Northcott JM, Dean IS, Mouw JK, Weaver VM. Feeling stress: the mechanics of cancer progression and aggression. Front Cell Dev Biol. 2018;6:17 https://doi.org/10.3389/fcell.2018.00017 .
Article PubMed PubMed Central Google Scholar
Theocharis AD, Skandalis SS, Gialeli C, Karamanos NK. Extracellular matrix structure. Adv Drug Deliv Rev. 2016;97:4–27.
Frantz C, Stewart KM, Weaver VM. The extracellular matrix at a glance. J Cell Sci. 2010;123:4195–200.
Fang M, Yuan J, Peng C, Li Y. Collagen as a double-edged sword in tumor progression. Tumor Biol. 2014;35:2871–82.
Article CAS Google Scholar
Egeblad M, Rasch MG, Weaver VM. Dynamic interplay between the collagen scaffold and tumor evolution. Curr Opin Cell Biol. 2010;22:697–706.
Xu S, Xu H, Wang W, Li S, Li H, Li T, et al. The role of collagen in cancer: from bench to bedside. J Transl Med. 2019;17:309.
Töpfer U. Basement membrane dynamics and mechanics in tissue morphogenesis. Biol Open. 2023;12:bio059980.
Rozario T, Desimone DW. The extracellular matrix in development and morphogenesis: a dynamic view. Dev Biol. 2010;341:126–40.
Sekiguchi R, Yamada KM. Basement membranes in development and disease. Curr Top Dev Biol. 2018;130:143–91.
Töpfer U, Guerra Santillán KY, Fischer-Friedrich E, Dahmann C. Distinct contributions of ECM proteins to basement membrane mechanical properties in Drosophila. Development. 2022;149:dev200456.
Mierke CT. The matrix environmental and cell mechanical properties regulate cell migration and contribute to the invasive phenotype of cancer cells. Rep Prog Phys. 2019;82:064602.
Chang TT, Thakar D, Weaver VM. Force-dependent breaching of the basement membrane. Matrix Biol. 2017;57-58:178–89.
Chen YL, Zhang X, Bai J, Gai L, Ye XL, Zhang L, et al. Sorafenib ameliorates bleomycin-induced pulmonary fibrosis: potential roles in the inhibition of epithelial-mesenchymal transition and fibroblast activation. Cell Death Dis. 2013;4:e665.
Wang W, Qu M, Xu L, Wu X, Gao Z, Gu T, et al. Sorafenib exerts an anti-keloid activity by antagonizing TGF-beta/Smad and MAPK/ERK signaling pathways. J Mol Med. 2016;94:1181–94.
Chrisnandy A, Blondel D, Rezakhani S, Broguiere N, Lutolf MP. Synthetic dynamic hydrogels promote degradation-independent in vitro organogenesis. Nat Mater. 2022;21:479–87. https://doi.org/10.1038/s41563-021-01136-7 .
Jayadev R, Morais MRPT, Ellingford JM, Srinivasan S, Naylor RW, Lawless C, et al. A basement membrane discovery pipeline uncovers network complexity, regulators, and human disease associations. Sci Adv. 2022;8:eabn2265 https://doi.org/10.1126/sciadv.abn2265 .
Subramanian A, Tamayo P, Mootha VK, Mukherjee S, Ebert BL, Gillette MA, et al. Gene set enrichment analysis: a knowledge-based approach for interpreting genome-wide expression profiles. Proc Natl Acad Sci USA. 2005;102:15545–50. https://doi.org/10.1073/pnas.0506580102 .
García-Mulero S, Alonso MH, Pardo J, Santos C, Sanjuan X, Salazar R, et al. Lung metastases share common immune features regardless of primary tumor origin. J Immunother Cancer. 2020;8:e000491 https://doi.org/10.1136/jitc-2019-000491 .
Hänzelmann S, Castelo R, Guinney J. GSVA: gene set variation analysis for microarray and RNA-seq data. BMC Bioinform. 2013;14:7 https://doi.org/10.1186/1471-2105-14-7 .
Article Google Scholar
Geeleher P, Cox N, Huang RS. pRRophetic: an R package for prediction of clinical chemotherapeutic response from tumor gene expression levels. PLoS ONE. 2014;9:e107468 https://doi.org/10.1371/journal.pone.0107468 .
Satija R, Farrell JA, Gennert D, Schier AF, Regev A. Spatial reconstruction of single-cell gene expression data. Nat Biotechnol. 2015;33:495–502. https://doi.org/10.1038/nbt.3192 .
Vu R, Jin S, Sun P, Haensel D, Nguyen QH, Dragan M, et al. Wound healing in aged skin exhibits systems-level alterations in cellular composition and cell-cell communication. Cell Rep. 2022;40:111155 https://doi.org/10.1016/j.celrep.2022.111155
Bachem MG, Schneider E, Gross H, Weidenbach H, Schmid RM, Menke A, et al. Identification, culture, and characterization of pancreatic stellate cells in rats and humans. Gastroenterology. 1998;115:421–32. https://doi.org/10.1016/s0016-5085(98)70209-4 .
Schuth S, Le Blanc S, Krieger TG, Jabs J, Schenk M, Giese NA, et al. Patient-specific modeling of stroma-mediated chemoresistance of pancreatic cancer using a three-dimensional organoid-fibroblast co-culture system. J Exp Clin Cancer Res. 2022;41:312 https://doi.org/10.1186/s13046-022-02519-7 .
Liu Q, Luo Q, Ju Y, Song G. Role of the mechanical microenvironment in cancer development and progression. Cancer Biol Med. 2020;17:282–92. https://doi.org/10.20892/j.issn.2095-3941.2019.0437 .
Khalilgharibi N, Mao Y. To form and function: on the role of basement membrane mechanics in tissue development, homeostasis and disease. Open Biol. 2021;11:200360.
Pang MF, Siedlik MJ, Han S, Stallings-Mann M, Radisky DC, Nelson CM. Tissue stiffness and hypoxia modulate the integrin-linked kinase ILK to control breast cancer stem-like cells. Cancer Res. 2016;76:5277–87. https://doi.org/10.1158/0008-5472.CAN-16-0579 .
Chaudhuri O, Koshy ST, Branco da Cunha C, Shin JW, Verbeke CS, Allison KH, et al. Extracellular matrix stiffness and composition jointly regulate the induction of malignant phenotypes in mammary epithelium. Nat Mater. 2014;13:970–8. https://doi.org/10.1038/nmat4009 .
Jain RK, Martin JD, Stylianopoulos T. The role of mechanical forces in tumor growth and therapy. Annu Rev Biomed Eng. 2014;16:321–46. https://doi.org/10.1146/annurev-bioeng-071813-105259
Lopez JI, Kang I, You WK, McDonald DM, Weaver VM. In situ force mapping of mammary gland transformation. Integr Biol. 2011;3:910–21. https://doi.org/10.1039/c1ib00043h .
Masuzaki R, Tateishi R, Yoshida H, Sato T, Ohki T, Goto T, et al. Assessing liver tumor stiffness by transient elastography. Hepatol Int. 2007;1:394–7. https://doi.org/10.1007/s12072-007-9012-7 .
Sokolović A, Sokolović M, Boers W, Elferink RP, Bosma PJ. Insulin-like growth factor binding protein 5 enhances survival of LX2 human hepatic stellate cells. Fibrogenes Tissue Repair. 2010;3:3 https://doi.org/10.1186/1755-1536-3-3
Yokota T, McCourt J, Ma F, Ren S, Li S, Kim TH, et al. Type V collagen in scar tissue regulates the size of scar after heart injury. Cell. 2020;182:545–562.e23. https://doi.org/10.1016/j.cell.2020.06.030 .
Download references
This work was supported by the National Natural Science Foundation of China (82072595, 82172569, and 61973232), Tianjin Key Medical Discipline (Specialty) Construction Project (TJYXZDXK-061B, TJWJ2022XK005). Tianjin Health Science and Technology Project (ZC20179). Beijing Science and Technology Innovation Medical Development Fund (KC2021-JX-0186-57). Funding sources had no role in study design, data collection, and analysis; in the decision to publish; or in the preparation of the manuscript.
Author information
These authors contributed equally: Guangsheng Zhu, Yanan Wang, Yingjie Wang.
Authors and Affiliations
Department of Lung Cancer Surgery, Tianjin Medical University General Hospital, Tianjin, People’s Republic of China
Guangsheng Zhu, Yanan Wang, Yingjie Wang, Hua Huang, Boshi Li, Peijie Chen, Hongbing Zhang & Jun Chen
Tianjin Key Laboratory of Lung Cancer Metastasis and Tumor Microenvironment, Tianjin Lung Cancer Institute, Tianjin Medical University General Hospital, Tianjin, People’s Republic of China
Chen Chen, Yongwen Li, Hongyu Liu & Jun Chen
You can also search for this author in PubMed Google Scholar
Contributions
GZ, YW and YL analyzed and interpreted the public data. GZ, YW and YW conducted the cell experiments. HH, BL, and PC conducted experiments based on tumor tissue. CC, ZH, and HL collected tumor tissue and performed transcriptome sequencing. GZ, HL and JC were major contributors in writing the manuscript. All authors read and approved the final manuscript.
Corresponding authors
Correspondence to Yongwen Li , Hongyu Liu or Jun Chen .
Ethics declarations
Competing interests.
The authors declare no competing interests.
Ethics approval and consent to participate
The acquisition of tissue from lung adenocarcinoma patients has been approved by the Ethics Committee of Tianjin Medical University General Hospital, and the patients have signed an informed consent form.
Additional information
Publisher’s note Springer Nature remains neutral with regard to jurisdictional claims in published maps and institutional affiliations.
Edited by Nickolai Barlev
Supplementary information
Supplementary figure, supplementary appendix 1, supplementary appendix 2, rights and permissions.
Open Access This article is licensed under a Creative Commons Attribution 4.0 International License, which permits use, sharing, adaptation, distribution and reproduction in any medium or format, as long as you give appropriate credit to the original author(s) and the source, provide a link to the Creative Commons licence, and indicate if changes were made. The images or other third party material in this article are included in the article’s Creative Commons licence, unless indicated otherwise in a credit line to the material. If material is not included in the article’s Creative Commons licence and your intended use is not permitted by statutory regulation or exceeds the permitted use, you will need to obtain permission directly from the copyright holder. To view a copy of this licence, visit http://creativecommons.org/licenses/by/4.0/ .
Reprints and permissions
About this article
Cite this article.
Zhu, G., Wang, Y., Wang, Y. et al. Myofibroblasts derived type V collagen promoting tissue mechanical stress and facilitating metastasis and therapy resistance of lung adenocarcinoma cells. Cell Death Dis 15 , 493 (2024). https://doi.org/10.1038/s41419-024-06873-6
Download citation
Received : 04 March 2024
Revised : 22 June 2024
Accepted : 28 June 2024
Published : 10 July 2024
DOI : https://doi.org/10.1038/s41419-024-06873-6
Share this article
Anyone you share the following link with will be able to read this content:
Sorry, a shareable link is not currently available for this article.
Provided by the Springer Nature SharedIt content-sharing initiative
Quick links
- Explore articles by subject
- Guide to authors
- Editorial policies

- Study protocol
- Open access
- Published: 02 July 2024
Improving medication adherence among persons with cardiovascular disease through m-health and community health worker-led interventions in Kerala; protocol for a type II effectiveness-implementation research-(SHRADDHA-ENDIRA)
- Jaideep C. Menon 1 ,
- Denny John 2 ,
- Aswathy Sreedevi ORCID: orcid.org/0000-0002-6037-9265 3 ,
- Chandrasekhar Janakiram 4 ,
- Akshaya R 3 ,
- Sumithra S 5 ,
- Aravind M S 6 ,
- Mathews Numpeli 7 ,
- Bipin Gopal 8 ,
- Renjini B A 7 ,
- Sajeev P K 9 ,
- Ravivarman Lakshmanasamy 10 &
- Abhishek Kunwar 11
Trials volume 25 , Article number: 437 ( 2024 ) Cite this article
275 Accesses
7 Altmetric
Metrics details
A Correction to this article was published on 09 July 2024
This article has been updated
Cardiovascular disease (CVD) is the leading cause of mortality worldwide, and at present, India has the highest burden of acute coronary syndrome and ST-elevation myocardial infarction (MI). A key reason for poor outcomes is non-adherence to medication.
The intervention is a 2 × 2 factorial design trial applying two interventions individually and in combination with 1:1 allocation ratio: (i) ASHA-led medication adherence initiative comprising of home visits and (ii) m-health intervention using reminders and self-reporting of medication use. This design will lead to four potential experimental conditions: (i) ASHA-led intervention, (ii) m-health intervention, (iii) ASHA and m-health intervention combination, (iv) standard of care. The cluster randomized trial has been chosen as it randomizes communities instead of individuals, avoiding contamination between participants. Subcenters are a natural subset of the health system, and they will be considered as the cluster/unit. The factorial cluster randomized controlled trial (cRCT) will also incorporate a nested health economic evaluation to assess the cost-effectiveness and return on investment (ROI) of the interventions on medication adherence among patients with CVDs. The sample size has been calculated to be 393 individuals per arm with 4–5 subcenters in each arm. A process evaluation to understand the effect of the intervention in terms of acceptability, adoption (uptake), appropriateness, costs, feasibility, fidelity, penetration (integration of a practice within a specific setting), and sustainability will be done.
The effect of different types of intervention alone and in combination will be assessed using a cluster randomized design involving 18 subcenter areas. The trial will explore local knowledge and perceptions and empower people by shifting the onus onto themselves for their medication adherence. The proposal is aligned to the WHO-NCD aims of improving the availability of the affordable basic technologies and essential medicines, training the health workforce and strengthening the capacity of at the primary care level, to address the control of NCDs. The proposal also helps expand the use of digital technologies to increase health service access and efficacy for NCD treatment and may help reduce cost of treatment.
Trial registration
The trial has been registered with the Clinical Trial Registry of India (CTRI), reference number CTRI/2023/10/059095.
Peer Review reports
Administrative information
Note: the numbers in curly brackets in this protocol refer to SPIRIT checklist item numbers. The order of the items has been modified to group similar items (see http://www.equator-network.org/reporting-guidelines/spirit-2013-statement-defining-standard-protocol-items-for-clinical-trials/ ).
Title {1} | SPIRIT guidance: Descriptive title identifying the study design, population, interventions, and, if applicable, trial acronym.
|
Trial registration {2a and 2b}. | SPIRIT guidance: Trial identifier and registry name. If not yet registered, name of intended registry. Item 2b is met if the register used for registration collects all items from the World Health Organization Trial Registration Data Set. |
Protocol version {3} | SPIRIT guidance: Date and version identifier. Version 3. 23 February 2024. |
Funding {4} | SPIRIT guidance: Sources and types of financial, material, and other support. Financial support from WHO, Geneva, Alliance for Health Policy and System research |
Author details {5a} | SPIRIT guidance: Affiliations of protocol contributors. Jaideep C Menon, Professor, Adult Cardiology, AIMS, Kochi Denny John, Adjunct Professor, Ramaiah University of applied Sciences Aswathy S, Professor, Community Medicine Chandrasekhar J, Professor, Public Health Dentistry Akshaya R, Senior Resident, Community Medicine Sumithra S, Senior Lecturer, St John’s research Institute Aravind MS, Research Associate, Public Health, AIMS, Kochi Mathews Numpeli, CHC MO, DHS, Govt of Kerala Bipin Gopal, State nodal Officer- NCDs, Kerala Renjini BA, MO, DHS, Govt of Kerala Sajeev PK, NHM Coordinator, Kalady Ravivarman L, WHO NCD officer, India Country Office Abhishek Kunwar, NPO NCD, WHO India |
Name and contact information for the trial sponsor {5b} | SPIRIT guidance: Name and contact information for the trial sponsor. Dr Sarah Rylance, Medical Officer for Chronic Respiratory Diseases, Focal point for NCD Research and Innovation World Health Organization HQ |
Role of sponsor {5c} | SPIRIT guidance: Role of study sponsor and funders, if any, in study design; collection, management, analysis, and interpretation of data; writing of the report; and the decision to submit the report for publication, including whether they will have ultimate authority over any of these activities. Study sponsor does not have any role in the study design, collection, management, analysis and interpretation of data |
Introduction
Background and rationale {6a}.
Cardiovascular disease (CVD) is the leading cause of mortality worldwide, and at present, India has the highest burden of acute coronary syndrome and ST-elevation myocardial infarction (MI) [ 1 ]. A key reason for poor outcomes is non-adherence to medication. The WHO has reported that non-adherence to drugs in chronic conditions is as high as 50%, and 30% of re-admissions are related to non-compliance to medication. In its 2003 report [ 2 ], WHO states that “increasing the effectiveness of adherence interventions may have a far greater impact on the health of the population than any improvement in specific medical treatment.”
A systematic review published in 2015 on adherence to medication had eleven studies from India reporting adherence rates (using pills taken, prescribed doses taken, changes, etc.) using Morisky Medication Adherence Score (MMAS) in the range of 0–51.2% [ 3 , 4 , 5 , 6 , 7 , 8 ]. The factors associated with non-adherence to medications were forgetfulness, difficulty in remembering, and stopping medication upon feeling better/worse.
Various interventions have been studied to increase medication adherence for cardiovascular disease in India. These include the use of combination therapy or polypill [ 9 , 10 , 11 ], use of community health workers (CHW) for simplified hypertension management with the aid of a smart-phone-based electronic decision support system [ 12 ], “task shifting” interventions to CHWs for CVD risk reduction through behavioral change [ 13 ], improving adherence to drugs, lifestyle changes, and clinical risk markers in patients of acute coronary syndromes [ 14 , 15 ] and use of CHWs and doctors in primary health center (PHC) to assess CVD risk with clinical decision support being provided through an m-health platform by doctors sitting remotely [ 16 ]. Studies have also identified the use of mobile technology by health workers in resource-limited settings for health delivery improvement [ 17 ]. The different studies mentioned have looked at m-health or CHWs alone to improve adherence to medication, lifestyle changes, or as a platform for treatment, with varied results.
We measured adherence in 2064 patients of coronary artery disease (CAD) the ENDIRA cohort using the MMAS-8 in the year 2019. Our results revealed poor adherence to chronic care medications in CAD patients. On an average, only 2.8 of the mandated 4 drugs (beta blocker, ACE Inhibitor /ARB, statin, and anti-platelet) were being taken by patients regularly [ 18 ]. The mean value of MMAS was 4 out of a possible 8, reflecting poor adherence [ 19 ]. A study on the feasibility of an m-health intervention in the same cohort for the prevention and management of CAD revealed that the use and ownership of mobiles was 88% (2015), 92% were willing to receive mobile health advice [ 19 ], 70% preferred voice calls over SMS, 85.9% would send self-recorded blood pressure, weight, and blood glucose to a doctor or community health worker [ 19 ]. Given that the results of our study revealed poor adherence and that use of m-health for CVD was both acceptable and feasible, the obvious next step would be in trying to improve adherence using these resources.
Objectives {7}
Primary objective.
To assess the effectiveness of using m-health and community health worker-led interventions for improving adherence to drugs in patients with cardiovascular disease using m-health and community health worker intervention individually and in combination in comparison to control group.
Secondary objective
To assess the effects of using the interventions (m-health and community health worker-led interventions) for improving adherence to drugs among heart disease patients on implementation outcomes such as acceptability and adoption.
To assess the cardiometabolic risk factors among first degree relatives of patients with heart disease
Trial design {8}
It is a 2 × 2 factorial design trial applying two interventions individually and in combination with a 1:1 allocation ratio. Two interventions are applied individually and in combination: (i) ASHA-led medication adherence initiative comprising of home visits, and (ii) m-health intervention using reminders and self-reporting of medication use. This design will lead to four potential experimental conditions: (i) ASHA-led intervention, (ii) m-health intervention, (iii) ASHA and m-health intervention combination, (iv) standard of care.
Methods: participants, interventions, and outcomes
Study setting {9}.
The study will be implemented in the ENDIRA (Epidemiology of Non-communicable Diseases in Rural Areas) cohort (n-114,064 individuals) which includes 2064 patients with heart disease in whom adherence to drugs for heart disease has already been assessed. The ENDIRA cohort is spread over 5 primary health centers consisting of 18 subcenters where the health details of all individuals have been recorded. In order to avoid contamination in the treatment allocation and its response, at least 10 km of distance among villages will be maintained and they will be clubbed into 4 groups.
The intervention will be implemented in Angamaly block consisting of five local self-government areas namely Mookkannoor, Kalady, Thuravoor, Karukutty, and Manjapra with a population of 18,638, 20,407, 20,475, 26,811, and 14,668 in Ernakulam district [ 20 ] in Kerala state, India, respectively.
Eligibility criteria {10}
The study samples will consist of adult community members with diagnosis of CAD, valvular disease, heart failure, and rhythm disorders in the target areas who provide informed consent.
Eligibility criteria
Diagnosed case of CAD who have received treatment for MI/STEMI/UA or diagnosed using a coronary angiogram or CT coronary angiogram or have undergone revascularization and are on medications.
Other cardiovascular cases such as rhythm disorders, valve disorders, and heart failure identified as pumping disorders by the community will also be a part of the study. Male or female aged 18 years or more will be considered.
Resident of village during the baseline survey.
Has no plans to migrate in next 12 months from the date of initiation of intervention.
Exclusion criteria
Persons who are bedridden and are unable to answer the questions.
Pregnant or lactating mothers
Individuals with cognitive impairment
Who will take informed consent? {26a}
Informed consent will be taken by the accredited social health activist of the area who will be collecting the data. The data collection will be through an application called SHRADDHA (which means care). The participant’s digital signature will be obtained on the tablet.
Additional consent provisions for collection and use of participant data and biological specimens {26b}
Blood samples will be collected to assess random blood sugar and HbA1c among cardiac patients with type 2 DM after obtaining consent. These samples will be tested using point-of-care devices and will not be stored. We will request consent for review of participants’ medical records, and for the collection of blood samples to assess random blood sugar and HbA1c among the cardiac patients with type 2 diabetes. But this trial does not involve collecting biological specimens for storage.
Interventions
Explanation for the choice of comparators {6b}.
Results of our study revealed poor adherence and that use of m-health for CVD was both acceptable and feasible. Various interventions have been studied to increase medication adherence for cardiovascular disease in India such as use of combination therapy or polypill, use of community health workers (CHW) for simplified hypertension management with the aid of a smart phone-based electronic decision support system, so we decided to use factorial study design where study units would be assigned to ASHA and no ASHA group. Following this they would be assigned to m-health and no m-health group. Thus, there are four arms to the study: namely ASHA, ASHA and m-health, m-health, and standard of care.
Intervention description {11a}
The intervention content is prepared after discussion with the stakeholders such as ASHAs, Medical Officers, and patients. Qualitative data would be obtained from unstructured or semi-structured interviews exploring the individual’s understanding of the use of medicines, potential obstacles and incentives to adherence, useful strategies to improve adherence. Interview guide for In-Depth-Interviews and Key-Informant Interviews will be developed after a thorough literature search. In-Depth Interviews will be done with the participants and their relatives to identify individual’s understanding of the use of medicines, potential obstacles and incentives to adherence, useful strategies to improve adherence, and other questions spontaneously raised during the interview. For Key-Informant Interviews, Health care providers such as doctors, the multipurpose health worker, ASHAs, and pharmacists (about 10) will be interviewed till saturation Is reached. Focus group discussions (FGD) will be conducted among adherent CVD patients and nonadherent CVD patients. About 3-4 such FGD will be conducted till data saturation is reached. This will be repeated at endline.
Community health worker directed visits to the house of the patient, where they will explain the use of drugs and the various roles of the different classes of drugs along with taking a pill count and giving health advice and counselling with a PowerPoint on a tablet. The frequency of visits is twice a month for the first 3 months, and once a month for the next 3 (11 visits in all). A schedule of visits with the areas to be highlighted in each visit such as diet, physical activity, tobacco, and alcohol will be prepared and given.
Before the commencement of the intervention training, sessions for community health workers (ASHAs) in the intervention arm will be conducted. This will comprise of three sessions of 6 h each and would include curriculum-based training modules on CVD, HTN, diabetes, dyslipidemia; awareness of the role each of the 4 classes of drugs in AS-CVD plays in secondary prevention; sensitization to the role of adherence in preventing recurrence; sensitization to the side effects of the drugs and counselling skill training. Role of lifestyle changes such as diet, physical activity, tobacco, and alcohol will also be carried out.
The envisaged m-health platform is a two-way system through which messages or jingles (audio clips) could be passed back and forth between the care provider (ASHA, Research assistant, or doctor) and the recipient (patient). Individual patient details gathered and entered on a Tab PC get stored on a central server. The data is anonymized and coded individually, with a 12-digit UID. In clusters where the m-health intervention is planned, individual patients could download and activate an already developed App, which is a free download from the Google play store [Ente app (my app)]. The individual patient would be able to access his personal health record as entered, by way of the App. This App would serve as a two-way channel of communication between the patient and caregiver. In the other clusters, individual patients could download their personal data and the App, with the communication channel remaining blocked.
Bi-monthly reminders via text or audio messages and weekly reminders on taking medicines at the time of a scheduled dose by way of a beep or tune and health advice by way of messages are sent for the first 3 months, followed by monthly reminders of text messages the next 3 and weekly drug reminder tunes.
Community health worker and m-health
Health worker (ASHA)-directed visits to the house of the patient, where they will explain the use of drugs and the various roles of the different classes of drugs along with taking a pill count and giving health advice and counselling. The frequency of visits is twice a month for the first 3 months, and once a month for the next 3. In addition, bi-monthly reminders via text or audio messages and weekly reminders on taking medicines at the time of a scheduled dose by way of a beep or tune and health advice by way of messages are sent for the first 3 months, followed by monthly reminders of text messages the next 3 and weekly drug reminder tunes.
Standard of care (SoC) is patient-initiated physician visit with health advice and treatment as prescribed by the treating doctor.
In all the groups, the patients can visit the doctor in case of any need or emergency.
After completion of baseline survey in all clusters, intervention will be implemented in intervention clusters for 6 months. All the participants in the intervention and control arms will be permitted to use standard treatment for CVD. Community health worker-directed visits to the house of the patient, where they will explain the use of drugs and the various roles of the different classes of drugs along with taking a pill count and giving health advice and counselling. The frequency of visits is twice a month for the first 3 months, and once a month for the next 3 (9 visits in all). Table 1 shows the timepoint for the intervention implementation.
Criteria for discontinuing or modifying allocated interventions {11b}
This is not applicable as the intervention is to improve medication adherence, so there will be no special criteria for discontinuing or modifying allocated intervention.
Strategies to improve adherence to interventions {11c}
The various interventions are for the improvement of adherence as measured by the Morisky Medication Adherence scale [ 21 ].
Relevant concomitant care permitted or prohibited during the trial {11d}
Relevant concomitant care is permitted.
Provisions for post-trial care {30}
This is a non-pharmacological intervention; therefore, there are no specific post trial care provisions.
Outcomes {12}
Primary, secondary, and other outcomes.
The primary outcome is the adherence of patients as measured by Morisky adherence scale [ 21 ] at the beginning of the study, midterm, and at the end. The secondary outcomes include Quality of Life (EuroQOL) [ 22 ], blood pressure, random blood sugar, HbA1c among the cardiac patients with type 2 diabetes, mortality events, and other unintended outcomes will also be recorded. The analysis will include change from baseline. Adherence is chosen as the main outcome as the objective is to study the impact of the various interventions singly and in combination on adherence in comparison to standard of care. Various symptoms, such as dyspnea, fatigue, edema, difficulty sleeping, depression, and chest pain associated with CVD limits activities of daily life [ 23 ]. Therefore, it is important to measure the quality of life before and after the intervention. Metabolic control can result from better adherence to medication and a better awareness of the importance to adhering to medication. Therefore, meeting targets of blood pressure, blood sugar levels, and HbA1c will be considered as secondary outcomes.
Participant timeline {13}
Sample size {14}.
Based on the learnings from the previous study, the rate of missing data due to electronic data collection will be low.
Phases 1 and 2: planning and baseline evaluation
The process of developing the intervention will start with the development of the initial concepts based on the available literature and interaction with healthcare professionals working in the rural areas.
Baseline study
Selection and training of team : The team will deliver the training to the selected project coordinator and the field staff. Field staff (part-time) will be recruited by the investigators on the advice of village head and/or NCD clinic in-charge. He/she should be a member of community preferably the accredited social health activist with an interest in health care and community, willingness to learn, and leadership qualities. A strong commitment to work in the community will be identified as an important criteria for the selection of all the team members. After a sensitization session of the data collectors/field staff, they will be asked to prepare a list of persons with cardiovascular disease including coronary artery disease, valvular disease, arrhythmias, and heart failure. Hands on sessions to download the App and collect data will be provided.
In Phase 2, baseline evaluation will be initiated in the study areas after obtaining the ethics committee approval. Written informed consent will be obtained from the study participants. Participants will receive a participant information sheet (PIS) outlining the rationale for the study, details on interventions, the steps, and protocols to be followed throughout the study, potential side effects and risks, benefits, a confidentiality statement, the option to withdraw from the study at any time, and the investigators’ contact information. The baseline survey performed by ASHAs will be done through a survey app called SHRADDHA. The variables collected would include (1) basic demographic information, including age, income, gender, marital status, religion, and occupation; (2) lifestyle-related factors such as physical activity, tobacco use, and alcohol consumption, dietary factors intake of fruits and vegetables, cooking oil and red meats; (3) disease details including for diabetes, hypertension, dyslipidemia, stroke and CAD, COPD, and surgeries; and (4) current medications. Questions will be explained to each participant to help them get familiar with the contents, instructions for filling them out will be given, and the responses will be recorded. On the home visit, the Field staff/ASHA will also record height and weight, measure sugars with a glucometer, and take a photo of the most recent prescription. All of this will be recorded in the app. Glycosylated hemoglobin will also be measured among the cardiac patients with diabetes using the point of care device called Lumira Dx.
Sample size : Sample size was estimated assuming an improvement of 10% in medication adherence at the end of a 6-month period in either m-health or community health worker-led intervention compared to control group. This 10% improvement will lead to an effect size of 0.4 units in medication adherence through m-health or community health worker-led intervention and an effect size of 0.8 units in combined intervention (m-health and community health worker-led intervention) compared to the control group. The 10% was an assumption considering that large differences are not possible in a community setting and was based on another community-based study which has also used 10% improvement of adherence score [ 24 ].
To observe a difference of 0.4 units in the medication adherence between study groups, with a standard deviation of 1.8, 5% level of significance (adjusted for multiple comparison) and 80% power, the sample size needed will be 238 participants in each of the study groups. After accounting for a design effect (cluster effect) and 10% attrition, the number of participants required per group will be 393, a total of 1572 participants.
Recruitment {15}
Working through the public health system, keeping in mind the proximity of the ASHAs to the community, it is expected that adequate participant enrolment can be achieved. Monitoring and supervision by the project team will assist in timely completion. The time period of recruitment is from February to May, 2024. After the recruitment, the randomization will be done and intervention will be administered for 6 months. Expected to finish by November 2024 and endline assessment in December 2024.
Assignment of interventions: allocation
Sequence generation {16a}.
Allocation of intervention and sequence generation will be as follows. Codes will be randomly assigned to the four interventions (ASHA, m-health, ASHA + m-health, and control groups) namely A, B, C, and D. In the next step, randomization list will be generated using RANDOM ALLOC software. Eighteen subcenters will be randomized into 4 study groups (A, B, C, and D) using different permutations of ABCD. Each subcenter will be allocated random numbers ranging from 1 to 18 using random number generators and random shuffling of this number. Interventions will be allocation to the subcenters in the sequence of random shuffled numbers as per the randomization list.
Concealment mechanism {16b}
It will be an open-label trial as concealment is not possible. However, study site allocation will be done only after completing baseline assessment and agreements with sites to participate.
Implementation {16c}
The allocation sequence will be generated by the Statistician, enrolment will be by the Field coordinator and the Field coordinator will assign subcenter as it is a cluster randomized trial.
Assignment of interventions: blinding
Who will be blinded {17a}.
Data analysts will be blinded. The ASHA workers, patients, and outcome assessors are not blinded.
Procedure for unblinding if needed {17b}
In this study, the ASHA workers, patients, and outcome assessors will not be blinded. Only the data analysts will be blinded. The data analyst will be unblinded if there are any outlier biochemical values which requires immediate action so that the patient can be intimated.
Data collection and management
Plans for assessment and collection of outcomes {18a}.
The primary outcome adherence will be measured by Morisky 8-item adherence questionnaire which has been validated in various countries including India and in various disease conditions. The eight-item Morisky Medication Adherence Scale (MMAS-8) is a structured self-report measure of medication-taking behavior that has been widely used in various cultures [ 25 , 26 , 27 ].It has a maximum score of 8.
The quality of life will be measured by the EuroQol five‐dimensions – 3‐level (EQ5D) which is a versatile quality of life (QOL) instrument with five dimensions (mobility, self‐care, usual activities, pain/discomfort, and anxiety/depression) and a visual analog scale. The questionnaire has also been found to be valid and reliable in various disease conditions including cardiovascular and cancer in India and neighboring countries [ 28 , 29 ].Random blood sugar among the patient and family member will be measured by the ASHA as per standard methods using a glucometer. Blood pressure will also be measured using the electronic Blood pressure will be recorded with the OMRON HEM 7124 automatic blood pressure monitor (Shimogyo-ku, Kyoto, Japan) by measuring upper arm BP. A laboratory technician will measure Glycosylated Haemoglobin using the Lumira Dx point of care device.
Real-time data entry will be monitored, and wherever there are difficulties with using the app, support will be provided by the field coordinator.
Plans to promote participant retention and complete follow-up {18b}
All efforts will be made to retain all participants in the study. As they are also part of the earlier ENDIRA study, there is a good rapport with the study group, local self-government, and frontline health workers. Loss to follow-up may result from migration to their children’s places of living or death or for other reasons. The characteristics of the patients who drop out will be recorded and compared to those who are in the study.
Data management {19}
As the data is collected through the SHRADDHA app, the data will be exported to excel and checked for completion each day. According to the data collected, feedback, and monitoring will be done to ensure correct and complete entries. Duplicate entries will be checked for and removed.
Confidentiality {27}
The data of the patients will be anonymized, and each patient will be assigned a unique id. From the app de-identified anonymized data will be stored in Excel. This will be stored confidentially before, during, and after the trial.
Plans for collection, laboratory evaluation, and storage of biological specimens for genetic or molecular analysis in this trial/future use {33}
In this study, blood samples will be collected to assess random blood sugar and HbA1c. These tests are done using point-of-care devices. The blood samples will not be stored in the current trial.
Statistical methods
Statistical methods for primary and secondary outcomes {20a}.
Several models will be run to test for the main outcomes, implementation outcomes, and related research questions. Mixed linear and logistic effects models as appropriate will be used to identify differences between the groups (ASHA, ASHA and m-health, m-health, control group), where random effects will be used for the clusters and fixed effects will be used for effects of ASHA workers and of m-health. The primary dependent variable in the models will be change in adherence measured by the Morisky scale. Models will also be fitted for the secondary outcomes such as change in blood pressure, random blood sugar, Hba1c levels, and quality of life. Subsequently, covariates such as age, sex, and co-morbidities will be added to the models to adjust for potential confounders.
Interim analyses {21b}
In this study, the intervention is done to improve the medication adherence through health education by ASHA workers, m-health, or both. Since the risk due to the intervention is minimal or none, interim analysis, and stopping guidelines have not been prescribed by the ethical committee and therefore there will not be any stopping guidelines.
Methods for additional analyses (e.g., subgroup analyses) {20b}
Subgroup analysis will not be carried out. However, for the primary and secondary outcome variables, covariates such as age, sex, and co-morbidities will be considered as potential confounders in the mixed effect model analysis.
Methods in analysis to handle protocol non-adherence and any statistical methods to handle missing data {20c}
Nonadherence will be managed by the intention to treat analysis and if there are too many missing data, imputations will be considered. Mixed method analysis will be considered for intention to treat analysis. Also depending on the percentage of data missingness and assumption for data missing in the study variables, appropriate missing data imputation technique will be used.
Plans to give access to the full protocol, participant-level data, and statistical code {31c}
Full protocol can be given. Full dataset can be given with the permission of the Institution, WHO, and Government.
Oversight and monitoring
Composition of the coordinating center and trial steering committee {5d}.
There is only one site for the study; therefore, the coordinating and steering committee will be situated at the site. The coordinating center is the Community Health Centre (CHC). The ASHA’s work is coordinated through the CHC by the National Health mission coordinators. The trial steering committee (TSC) monitors recruitment, communicates, and provides conflict resolution and timely advice. They meet every 6 weeks. Local organization and implementation is taken care of by the NHM coordinators and a responsible person reporting to the Principal Investigator from the Project management group. Trainings and other group meetings are conducted by the project management group. Consent is obtained by the ASHA. Periodic meetings are conducted by the Project management group (investigators) team to monitor progress. The stakeholder groups are apprised of the progress of the trial, role of intervention, and its possible benefit.
Composition of the data monitoring committee, its role and reporting structure {21a}
This study is measuring adherence which is a low-risk intervention; therefore, a data monitoring committee is not required. The project management group meets every 2 weeks. The Trial Steering Group and the independent Data Monitoring and Ethics Committee meet to review conduct throughout the trial period.
Adverse event reporting and harms {22}
As this study is measuring adherence of an intervention, no adverse events or serious adverse events and harms from the intervention are anticipated. But if there are any, they will be reported to relevant regulatory bodies such as Project management group, Trial steering Committee, District Health Authority, and Ethics Committee. Trial deviations will be reported to the ethical committee.
Frequency and plans for auditing trial conduct {23}
The meetings of the Project management group, Trial Steering group, independent data monitoring, and Ethics Committee periodically will also serve to audit the trial.
Plans for communicating important protocol amendments to relevant parties (e.g. trial participants, ethical committees) {25}
Before the start and at the start, there have been some minor modifications which has been updated to the ethical committee and subsequently uploaded in the CTRI.
Dissemination plans {31a}
The results of the study will be published in standard journals. Social media and Stakeholder workshops will be used to disseminate the findings. A lay summary will be shared with all participants.
The present study will promote much needed research and innovation for increasing adherence among patients with cardiovascular disease. The effect of different types of intervention alone and in combination will be assessed using a cluster randomized design involving 18 subcenter areas. This factorial cluster randomized controlled trial will benefit by increasing the drug adherence for NCD using m-health platform and frontline health workers. The trial will explore local knowledge, perceptions and empower people by shifting the onus onto themselves for their medication adherence.
The proposal is aligned to the WHO-NCD aims of improving the availability of affordable basic technologies and essential medicines, improving adherence for non-communicable diseases (NCDs). It also aligns to WHO-NCD aim of training the health workforce and strengthening the capacity of health systems, particularly at the primary care level, to address the control of NCDs. The proposal also helps expand the use of digital technologies to increase health service access and efficacy for NCD treatment and may help reduce the cost of treatment.
The proposal helps implementation of WHO-PEN protocol for Self-Care guidelines including utilizing frontline health workers in improving self-care in patients of heart disease, counselling to improving adherence and self-care, considering patients’ beliefs and concerns about drugs and their effect. The research is also aligned to the WHO-HEARTS package, both by way of A&T of the HEARTS where A- consists of information on CVD medicine and technology procurement, quantification, distribution, management, and handling of supplies at facility level. T- consists of guidance and examples on team-based care and task shifting related to the care of CVD. The research is also aligned with the Sustainable Development Goals (SDG) goal in relation to NCD of reducing by one third premature mortality from non-communicable diseases through prevention and treatment.
There are significant expected implementation challenges to note. First, the trial involves working with the primary clinics providing NCD screening and detection services, and building an effective partnership with the state government of Kerala where the project will be implemented will be crucial for its success. Second, medication nonadherence for patients with chronic diseases is extremely common with 40–50% of patients prescribed medications for management of diabetes and hypertension [ 30 ]. There exist treatment-related barriers, such as treatment complexity, side effects (or fear of side effects), inconvenience, cost, and time, and other barriers such as poor practitioner-patient relationship, aspects of which are beyond the scope of the intervention [ 30 ].
If successful, the medication adherence intervention, using m-health and ASHAs, has the potential to constitute evidence-based practice for improving medication adherence for CVD in India, and in similar developing countries.
Trial status
The current protocol is version 3 dated 23–02-2024. The recruitment began on November 30, 2023 and is expected to be complete by May 30, 2024. The submission has been delayed due to unavoidable circumstances such as elections and heatwave.
Availability of data and materials {29}
The investigators will have access to the final data set. There are no contractual agreements which limit access to investigators. The investigators in the field collect the data and the data is with them. Any data required to support the protocol can be supplied on request.
Change history
09 july 2024.
A Correction to this paper has been published: https://doi.org/10.1186/s13063-024-08323-2
Abbreviations
Angiotensin-converting enzyme
Angiotensin II receptor blocker
Accredited social health activist
- Coronary artery disease
Community health workers
Chronic obstructive pulmonary disease
Cluster randomized control trial
Computed tomography
Clinical Trials Registry—India
Cardiovascular diseases
Epidemiology of Non-Communicable Diseases in Rural Areas
Hypertension
Low-middle-income countries
Mobile Health
Myocardial infarction
Morisky Medication Adherence Score
Non-communicable diseases
Primary health center
Quality of Life
Return on investment
Sustainable Development Goals
Short Message Service
ST elevation myocardial infarction
Unstable angina
World Health Organization
Sreeniwas Kumar A, Sinha N. Cardiovascular disease in India: A 360 degree overview. Medical Journal Armed Forces India. 2020;76(1):1–3.
Article CAS PubMed PubMed Central Google Scholar
Sabaté E, ed. Adherence to Long-Term Therapies: Evidence for Action. Geneva: World Health Organization; 2003.
Baroletti S, Dell’Orfano H. Medication adherence in cardiovascular disease. Circulation. 2010;121:1455–8.
Article PubMed Google Scholar
Prabhakaran D, Jeemon P, Roy A. Cardiovascular disease in India, Current epidemiology and future directions. Circulation. 2016;133:1605–20.
Akeroyd JM, Chan WJ, Kamal AK, Palaniappan L, Virani SS. Adherence to cardiovascular medications in the South Asian population: A systematic review of current evidence and future directions. World J Cardiol. 2015;7(12):938–47.
Article PubMed PubMed Central Google Scholar
Fathima FN, Shanbhag DN, Hegde SKB, Sebastian B, Briguglio S. Cross Sectional Study of Adherence to Prescribed Medications among Individuals Registered at a High Risk Clinic in a Rural Area in Bangalore, India. Indian J Publ Health Research and Development. 2013;4(3):90–4.
Article Google Scholar
Venkatachalam J, Abrahm SB, Singh Z, Stalin P, Sathya GR. Determinants of Patient’s Adherence to Hypertension Medications in a Rural Population of Kancheepuram District inTamilNadu. SouthIndia Indian J Community Med. 2015;40:33–7.
Article CAS Google Scholar
Kumar N, Unnikrishnan B, Thapar R, Mithra P, Kulkarni V, Holla R, Bhagawan D, Mehta I. Factors associated with adherence to antihypertensive treatment among patients attention atertiary care hospital in Mangalore. South India IJCRR. 2014;6:77–85.
Google Scholar
Bahl VK, Jadhav UM, Thacker HP. Management of hypertension with the fixed combination of perindopril and amlodipine in daily clinical practice: results from the STRONG prospective, observational, multicenter study. Am J Cardiovasc Drugs. 2009;9:135–42.
Article CAS PubMed Google Scholar
Soliman EZ, Mendis S, Dissanayake WP, Somasundaram NP, Gunaratne PS, Jayasingne IK, Furberg CD. A Polypill for primary prevention of cardiovascular disease: a feasibility study of the World Health Organization. Trials. 2011;12:3.
Thom S, Poulter N, Field J, Patel A, Prabhakaran D, Stanton A, Grobbee DE, Bots ML, Reddy KS, Cidambi R, et al. Effects of a fixed-dose combination strategy on adherence and risk factors in patients with or at high risk of CVD:theUMPIRE randomized clinical trial. JAMA. 2013;310:918–29.
Ajay VS, Tian M, Chen H, Wu Y, Li X, Dunzhu D, et al. A cluster-randomized controlled trial to evaluate the effects of a simplified cardiovascular management program in Tibet, China and Haryana, India: study design and rationale. BMC Public Health. 2014;14(1):924.
Jeemon P, Narayanan G, Kondal D, et al. Task shifting of frontline community health workers for cardiovascular risk reduction: design and rationale of a cluster randomised controlled trial (DISHAstudy) in India. BMC Public health. 2016;16:264.
Kamath DY, Xavier D, Gupta R, Devereaux PJ, Sigamani A, Hussain T, et al. Rationale and design of a randomized controlled trial evaluating community health worker–based interventions for the secondary prevention of acute coronary syndromes in India (SPREAD). Am Heart J. 2014;168(5):690–7.
Sharma KK, Gupta R, Mathur M, Natani V, Lodha S, Roy S, Xavier D. Non-physician health workers for improving adherence to medications and healthy lifestyle following acute coronary syndrome: 24-month follow-up study. Indian Heart J. 2016;68(6):832–40.
Peiris D, Praveen D, Kishor M, et al. SMART health India: A stepped-wedge, a cluster randomised controlled trial of a community health worker managed mobile health intervention for people assessed at high cardiovascular disease risk in rural India. PLOS One. 2019;14(3):e0213708.
Free C, Phillips G, Watson L, Galli L, Felix L, Edwards P, et al. The effectiveness of mobile-health technologies to improve health care service delivery processes: a systematic review and meta-analysis. PLoS Med. 2013;10(1): e1001363.
Banerjee A, Menon JC, et al. A learning health system for secondary prevention in cardiovascular disease in Kerala using informatics and non-physician health workers (LHSCVD). Indian Heart J. 2018;70:S2.
Feinberg L, Menon JC, et al. Potential for mobile health (m-Health) prevention of cardiovascular diseases in Kerala: A population-based survey. Indian Heart J. 2017;69:182–99.
Angamaly Municipality City Population Census 2011-2024 | Kerala. [cited 2024 Jun 3]. Available from: https://www.census2011.co.in/data/town/803285-angamaly-kerala.html
Janežič A, Locatelli I, Kos M. Criterion validity of 8-item Morisky Medication Adherence Scale in patients with asthma. PLoS ONE. 2017;12(11):e0187835. https://doi.org/10.1371/journal.pone.0187835.PMID:29190693;PMCID:PMC5708647 .
EQ-5D-3LUserguide-23–07.pdf. [cited 2024 Jun 3]. Available from: https://euroqol.org/wp-content/uploads/2023/11/EQ-5D-3LUserguide-23-07.pdf
Heo S, Lennie TA, Okoli C, Moser DK. Quality of life in patients with heart failure: ask the patients. Heart Lung. 2009;38(2):100–8.
Xavier D, Gupta R, Kamath D, Sigamani A, Devereaux PJ, George N, et al. Community health worker-based intervention for adherence to drugs and lifestyle change after acute coronary syndrome: a multicentre, open, randomised controlled trial. Lancet Diabetes Endocrinol. 2016;4(3):244–53.
Surekha A, Fathima FN, Agrawal T, Misquith D. Psychometric Properties of Morisky Medication Adherence Scale (MMAS) in Known Diabetic and Hypertensive Patients in a Rural Population of Kolar District, Karnataka. Indian Journal of Public Health Research & Development. 2016;7(2):250.
Grover A, Oberoi M. Self-reported Morisky eight item medication adherence scale is a reliable and valid measure of compliance to statins in hyperlipidemic patients in India. Indian Heart J. 2020;72(4):319–20.
Okello S, Nasasira B, Muiru ANW, Muyingo A. Validity and Reliability of a Self-Reported Measure of Antihypertensive Medication Adherence in Uganda. PLoS ONE. 2016;11(7):e0158499.
Mahesh PKB, Gunathunga MW, Jayasinghe S, Arnold SM, Senanayake S, Senanayake C, et al. Construct validity and reliability of EQ-5D-3L for stroke survivors in a lower middle-income setting. Ceylon Med J. 2019;64(2):52–8.
Tripathy S, Hansda U, Seth N, Rath S, Rao PB, Mishra TS, et al. Validation of the EuroQol Five-dimensions - Three-Level Quality of Life Instrument in a Classical Indian Language (Odia) and Its Use to Assess Quality of Life and Health Status of Cancer Patients in Eastern India. Indian J Palliat Care. 2015;21(3):282–8.
Kleinsinger F. The Unmet Challenge of Medication Nonadherence. Perm J. 2018;22:18–033. https://doi.org/10.7812/TPP/18-033 .
Download references
Acknowledgements
The authors acknowledge the Accredited Social Health Activists, Dr Naseema Najeeb CHC MO, Dr Sunil Kumar, and Mr Sunny V V.
Funded by WHO NCD Division and NCD Alliance, Geneva.
WHO Reference 2023/1376413–0.
The funding body does not have a role in the design, data collection, analysis, and interpretation of data.
Author information
Authors and affiliations.
Adult Cardiology, AIMS, Amrita Vishwa Vidyapeetham, Kochi, India
Jaideep C. Menon
Ramaiah University of Applied Sciences, Bengaluru, India
Community Medicine, AIMS, Amrita Vishwa Vidyapeetham, Kochi, India
Aswathy Sreedevi & Akshaya R
Public Health Dentistry, Amrita School of Dentistry, Amrita Vishwa Vidyapeetham, Kochi, India
Chandrasekhar Janakiram
StJohn’s Research Institute, Bangalore, India
AIMS, Kochi, India
Aravind M S
MO, DHS, Govt of Kerala, Ernakulam, India
Mathews Numpeli & Renjini B A
NCD, DHS, Govt of Kerala, Kerala, Thiruvananthapuram, India
Bipin Gopal
CHC, Kalady, Kalady, India
India Country Office, New Delhi, India
Ravivarman Lakshmanasamy
NPO NCD, WHO India, New Delhi, India
Abhishek Kunwar
You can also search for this author in PubMed Google Scholar
Contributions
➙JCM is the Chief investigator, conceived the study, led the proposal and protocol development, Funding Acquisition, Methodology, Writing – Original Draft Preparation, Review & Editing
➙DJ—Conceptualization, Methodology, Writing – Original Draft Preparation, Review & Editing
➙AS—Development of proposal, Funding Acquisition, Methodology, Writing – Original Draft Preparation, Review & Editing
➙CJ—Development of proposal, Funding Acquisition, Methodology, Project Administration, Formal Analysis, Writing – Review & Editing
➙AR—Analysis, Project Administration, Supervision, Writing – Original Draft Preparation, Review & Editing
➙SS—Analysis, Sample calculation, Methodology, Visualization, Writing—Review & Editing
➙AMS—Project Administration, Supervision, Writing – Original Draft Preparation, Review & Editing
➙MN—Methodology, Project Administration, Supervision, Writing – Review & Editing
➙BG—Conceptualization, Methodology, Writing – Review & Editing
➙RBA—Project administration, Methodology, Writing – Review & Editing,
➙RL—Investigation, Methodology, Writing – Review & Editing
➙AK—Investigation, Methodology, Writing – Review & Editing
➙All the authors have read and approved the final manuscript.
Corresponding author
Correspondence to Aswathy Sreedevi .
Ethics declarations
Ethics approval and consent to participate {24}.
Ethical Review Board of Amrita Institute of Medical Sciences had approved the study dated 23–02-2024 number ECASM-AIMS-2024–098. Written, informed consent to participate will be obtained from all participants. Ethical approval has been obtained.
Consent for publication {32}
Informed consent has been obtained and the model consent form can be made available. No identifying images or other personal or clinical details of participants are presented here or will be presented in reports of the trial results.
Competing interests {28}
The authors declare that there are no competing interests.
Additional information
Publisher’s note.
Springer Nature remains neutral with regard to jurisdictional claims in published maps and institutional affiliations.
The original online version of this article was revised: Following the publication of the original article, we were notified that the 12th author’s name was incorrectly spelled. Originally published author name: Ravivarman Lakshmanaswamy. Correct name: Ravivarman Lakshmanasamy.
Rights and permissions
Open Access This article is licensed under a Creative Commons Attribution 4.0 International License, which permits use, sharing, adaptation, distribution and reproduction in any medium or format, as long as you give appropriate credit to the original author(s) and the source, provide a link to the Creative Commons licence, and indicate if changes were made. The images or other third party material in this article are included in the article's Creative Commons licence, unless indicated otherwise in a credit line to the material. If material is not included in the article's Creative Commons licence and your intended use is not permitted by statutory regulation or exceeds the permitted use, you will need to obtain permission directly from the copyright holder. To view a copy of this licence, visit http://creativecommons.org/licenses/by/4.0/ . The Creative Commons Public Domain Dedication waiver ( http://creativecommons.org/publicdomain/zero/1.0/ ) applies to the data made available in this article, unless otherwise stated in a credit line to the data.
Reprints and permissions
About this article
Cite this article.
Menon, J.C., John, D., Sreedevi, A. et al. Improving medication adherence among persons with cardiovascular disease through m-health and community health worker-led interventions in Kerala; protocol for a type II effectiveness-implementation research-(SHRADDHA-ENDIRA). Trials 25 , 437 (2024). https://doi.org/10.1186/s13063-024-08244-0
Download citation
Received : 09 May 2024
Accepted : 11 June 2024
Published : 02 July 2024
DOI : https://doi.org/10.1186/s13063-024-08244-0
Share this article
Anyone you share the following link with will be able to read this content:
Sorry, a shareable link is not currently available for this article.
Provided by the Springer Nature SharedIt content-sharing initiative
- Factorial study design
- Valvular disease
- Heart failure
- Implementation Research
- Medication adherence
- Morisky Medication Adherence Scale
ISSN: 1745-6215
- Submission enquiries: Access here and click Contact Us
- General enquiries: [email protected]

Researchers unveil comprehensive youth diabetes dataset and interactive portal to boost research and prevention strategies
A team from the Icahn School of Medicine at Mount Sinai has developed the most comprehensive epidemiological dataset for youth diabetes and prediabetes research, derived from extensive National Health and Nutrition Examination Survey (NHANES) data collected from 1999 to 2018. The dataset, revealed through the newly launched Prediabetes/diabetes in youth ONline Dashboard (POND), aims to ignite a new wave of research into the escalating issue of diabetes among young people.
The newly compiled dataset integrates data on 15,149 youths residing in the United States, aged 12 to 19, covering a range of variables from sociodemographic backgrounds to health statuses, dietary habits, and other lifestyle behaviors relevant to prediabetes and diabetes (preDM/DM). The POND portal invites researchers, health care professionals, and the public to explore these data, facilitating an understanding of factors that may influence youth diabetes risk.
"By providing a detailed view of the risk factors and trends associated with prediabetes and diabetes in our youth, this dataset empowers clinicians and researchers to develop more effective interventions tailored to the needs of this vulnerable population," said Nita Vangeepuram, MD, MPH, Associate Professor of Pediatrics, Population Health Science and Policy, and Environmental Medicine and Climate Science at Icahn Mount Sinai, and clinical expert on the research team.
"The availability of such a comprehensive and accessible dataset is crucial for advancing our understanding of diabetes risk factors in youths," added Gaurav Pandey, PhD, Associate Professor of Genetics and Genomic Sciences, and Artificial Intelligence and Human Health, and co-senior author of the study. "It allows researchers to apply advanced statistical and machine learning methods to uncover patterns underlying this serious disorder that were previously obscured due to a lack of publicly available comprehensive data."
The development of the dataset and the POND web portal by co-first authors Yan Chak Li, MPhil, and Catherine McDonough, MS, underscores Mount Sinai's commitment to accessible, actionable health data and to transparency of the methodology. By allowing users to interact with and analyze this comprehensive dataset, POND serves as a critical tool in the global fight against youth diabetes.
"Our findings have unveiled both established and novel variables linked to youth preDM/DM, emphasizing the hypothesis-generating value of this dataset and its potential to transform future research and develop targeted prevention strategies," added Bian Liu, PhD, Associate Professor of Population Health Science and Policy, and Environmental Medicine and Climate Science, and co-senior author of the study. "It's our hope that POND will not only foster more detailed studies, but also drive innovations in how we manage and prevent diabetes among younger populations."
The urgency of this research is amplified by the anticipated rise in diabetes diagnoses among young people worldwide, marking a significant public health concern. The research team's efforts to streamline and democratize access to critical health data through POND could lead to breakthroughs in how diabetes is understood and addressed in youth populations.
The study was funded by National Institutes of Health grant #s R21DK131555 and R01HG011407.
- Health Policy
- Teen Health
- Personalized Medicine
- Medical Topics
- Diseases and Conditions
- Chronic Illness
- Public Health Education
- Diabetes mellitus type 1
- Diabetes mellitus type 2
- Diabetic diet
- Hyperglycemia
- Erectile dysfunction
- Personalized medicine
Story Source:
Materials provided by The Mount Sinai Hospital / Mount Sinai School of Medicine . Note: Content may be edited for style and length.
Journal Reference :
- Catherine McDonough, Yan Chak Li, Nita Vangeepuram, Bian Liu, Gaurav Pandey. A Comprehensive Youth Diabetes Epidemiological Data Set and Web Portal: Resource Development and Case Studies . JMIR Public Health and Surveillance , 2024; 10: e53330 DOI: 10.2196/53330
Cite This Page :
Explore More
- Cause of Lupus and Possible Way to Reverse It
- Most Complete Dinosaur Found in UK in a Century
- The Origins of Dark Comets
- Gulf Stream Weakening With Climate Change?
- Treating Stroke-Related Brain Injury
- Boost for Battle Against Harmful Bacteria
- 'Missing Middle' Black-Hole in Omega Centauri
- Earliest Plant Farming in East Africa
- How Large-Scale Ocean Circulation Works
- Oceanic Seabirds Are Storm-Chasers
Trending Topics
Strange & offbeat.
Information
- Author Services
Initiatives
You are accessing a machine-readable page. In order to be human-readable, please install an RSS reader.
All articles published by MDPI are made immediately available worldwide under an open access license. No special permission is required to reuse all or part of the article published by MDPI, including figures and tables. For articles published under an open access Creative Common CC BY license, any part of the article may be reused without permission provided that the original article is clearly cited. For more information, please refer to https://www.mdpi.com/openaccess .
Feature papers represent the most advanced research with significant potential for high impact in the field. A Feature Paper should be a substantial original Article that involves several techniques or approaches, provides an outlook for future research directions and describes possible research applications.
Feature papers are submitted upon individual invitation or recommendation by the scientific editors and must receive positive feedback from the reviewers.
Editor’s Choice articles are based on recommendations by the scientific editors of MDPI journals from around the world. Editors select a small number of articles recently published in the journal that they believe will be particularly interesting to readers, or important in the respective research area. The aim is to provide a snapshot of some of the most exciting work published in the various research areas of the journal.
Original Submission Date Received: .
- Active Journals
- Find a Journal
- Proceedings Series
- For Authors
- For Reviewers
- For Editors
- For Librarians
- For Publishers
- For Societies
- For Conference Organizers
- Open Access Policy
- Institutional Open Access Program
- Special Issues Guidelines
- Editorial Process
- Research and Publication Ethics
- Article Processing Charges
- Testimonials
- Preprints.org
- SciProfiles
- Encyclopedia
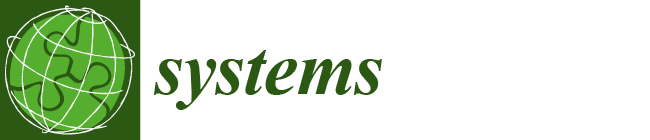
Article Menu
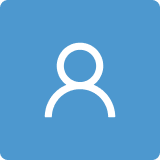
- Subscribe SciFeed
- Recommended Articles
- Google Scholar
- on Google Scholar
- Table of Contents
Find support for a specific problem in the support section of our website.
Please let us know what you think of our products and services.
Visit our dedicated information section to learn more about MDPI.
JSmol Viewer
The impact of scientific and technological information resource utilization on breakthrough innovation in enterprises: the moderating role of strategic aggressiveness.
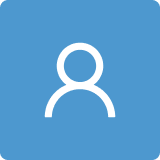
1. Introduction
2. literature review, 2.1. research on scientific and technological information resources, 2.2. research on breakthrough innovation, 2.3. research on strategic aggressiveness, 3. theoretical analysis and hypotheses, 3.1. analysis of the impact of scientific and technological information resource utilization on enterprise breakthrough innovation, 3.2. the moderating role of strategic aggressiveness, 4. data sources and research methods, 4.1. data sources and processing, 4.2. main research variables, 4.3. research methods, 5. research results, 5.1. descriptive statistical analysis of enterprise indicators, 5.2. correlation analysis of indicators, 5.3. the impact of enterprise scientific and technological information resource utilization on breakthrough innovation, 5.4. testing the moderating role of strategic aggressiveness, 5.5. endogeneity and robustness tests, 5.6. heterogeneity analysis, 6. discussion, 6.1. relationship between enterprise utilization of scientific and technological information resources and breakthrough innovation, 6.2. moderating role of strategic aggressiveness in the relationship between utilization of scientific and technological information resources and breakthrough innovation, 6.3. regional and ownership differences in the utilization of scientific and technological information resources and breakthrough innovation, 7. conclusions and implications, 7.1. conclusions, 7.2. implications, 7.2.1. theoretical contributions, 7.2.2. managerial implications, 8. research limitations, author contributions, data availability statement, conflicts of interest.
- Farhana, M.; Swietlicki, D. Dynamic capabilities impact on innovation: Niche market and startups. J. Technol. Manag. Innov. 2020 , 15 , 83–96. [ Google Scholar ] [ CrossRef ]
- Birkle, C.; Pendlebury, D.A.; Schnell, J.; Adams, J. Web of Science as a data source for research on scientific and scholarly activity. Quant. Sci. Stud. 2020 , 1 , 363–376. [ Google Scholar ] [ CrossRef ]
- Meyer, M. Does science push technology? Patents citing scientific literature. Res. Policy 2000 , 29 , 404–434. [ Google Scholar ] [ CrossRef ]
- Vicente-Gomila, J.M.; Artacho-Ramirez, M.A.; Ting, M.; Porter, A.L. Combining tech mining and semantic TRIZ for technology assessment: Dye-sensitized solar cell as a case. Technol. Forecast. Soc. 2021 , 169 , 120826. [ Google Scholar ] [ CrossRef ]
- Wu, L.; Sun, L.; Chang, Q.; Zhang, D.; Qi, P. How do digitalization capabilities enable open innovation in manufacturing enterprises? A multiple case study based on resource integration perspective. Technol. Forecast. Soc. 2022 , 184 , 122019. [ Google Scholar ] [ CrossRef ]
- Wang, X.; Zhai, Y.; Lin, Y.; Wang, F. Mining layered technological information in scientific papers: A semi-supervised method. J. Inf. Sci. 2019 , 45 , 779–793. [ Google Scholar ] [ CrossRef ]
- Yam, R.C.; Lo, W.; Tang, E.P.; Lau, A.K. Analysis of sources of innovation, technological innovation capabilities, and performance: An empirical study of Hong Kong manufacturing industries. Res. Policy 2011 , 40 , 391–402. [ Google Scholar ] [ CrossRef ]
- Chen, J.; Chen, Y.; Vanhaverbeke, W. The influence of scope, depth, and orientation of external technology sources on the innovative performance of Chinese firms. Technovation 2011 , 31 , 362–373. [ Google Scholar ] [ CrossRef ]
- Zhang, G.; Zhao, S.; Xi, Y.; Liu, N.; Xu, X. Relating science and technology resources integration and polarization effect to innovation ability in emerging economies: An empirical study of Chinese enterprises. Technol. Forecast. Soc. 2018 , 135 , 188–198. [ Google Scholar ] [ CrossRef ]
- Gómez, J.; Salazar, I.; Vargas, P. Does information technology improve open innovation performance? An examination of manufacturers in Spain. Inform. Syst. Res. 2017 , 28 , 661–675. [ Google Scholar ] [ CrossRef ]
- Jemala, M. Long-term research on technology innovation in the form of new technology patents. Int. J. Innov. Stud. 2021 , 5 , 148–160. [ Google Scholar ] [ CrossRef ]
- Jin, P.; Mangla, S.K.; Song, M. The power of innovation diffusion: How patent transfer affects urban innovation quality. J. Bus. Res. 2022 , 145 , 414–425. [ Google Scholar ] [ CrossRef ]
- Weinzimmer, L.; Esken, C.A.; Michel, E.J.; McDowell, W.C.; Mahto, R.V. The differential impact of strategic aggressiveness on firm performance: The role of firm size. J. Bus. Res. 2023 , 158 , 113623. [ Google Scholar ] [ CrossRef ]
- Wang, J.; Cao, H. Improving competitive strategic decisions of Chinese coal companies toward green transformation: A hybrid multi-criteria decision-making model. Resour. Policy 2022 , 75 , 102483. [ Google Scholar ] [ CrossRef ]
- Zhang, Y.; Tang, X.; Yang, J. Synergies of Technological and Institutional Innovation Driving Manufacturing Transformation: Insights from Northeast China. J. Knowl. Econ. 2024 , 16 , 1–35. [ Google Scholar ] [ CrossRef ]
- Fan, P.; Watanabe, C. Promoting industrial development through technology policy: Lessons from Japan and China. Technol. Soc. 2006 , 28 , 303–320. [ Google Scholar ] [ CrossRef ]
- Wu, Y.; Ji, Y.; Gu, F.; Guo, J. A collaborative evaluation method of the quality of patent scientific and technological resources. World Pat. Inf. 2021 , 67 , 102074. [ Google Scholar ] [ CrossRef ]
- Yonghui, G.; Jie, L. Policymakers and Policy Evolution of Scientific and Technological Resource Integration in China. J. Knowl. Econ. 2023 , 15 , 1–25. [ Google Scholar ] [ CrossRef ]
- Horton, F.W. Information Resources Management ; Nanjing University Publication: Nanjing, China, 1985. [ Google Scholar ]
- Syuntyurenko, O.V. Determinants of the ineffective use of information resources in scientific and technological activities. Sci. Tech. Inf. Process 2017 , 44 , 159–169. [ Google Scholar ] [ CrossRef ]
- Omona, W.; Ikoja-Odongo, R. Application of information and communication technology (ICT) in health information access and dissemination in Uganda. J. Libr. Inf. Sci. 2006 , 38 , 45–55. [ Google Scholar ] [ CrossRef ]
- Leonardi, P.M. When does technology use enable network change in organizations? A comparative study of feature use and shared affordances. Mis Quart. 2013 , 37 , 749–775. [ Google Scholar ] [ CrossRef ]
- Liu, J.; Chang, H.; Forrest, J.Y.L.; Yang, B. Influence of artificial intelligence on technological innovation: Evidence from the panel data of china’s manufacturing sectors. Technol. Forecast. Soc. 2020 , 158 , 120142. [ Google Scholar ] [ CrossRef ]
- Duncan, N.B. Capturing flexibility of information technology infrastructure: A study of resource characteristics and their measure. J. Manag. Inform. Syst. 1995 , 12 , 37–57. [ Google Scholar ] [ CrossRef ]
- Coccia, M. Deep learning technology for improving cancer care in society: New directions in cancer imaging driven by artificial intelligence. Technol. Soc. 2020 , 60 , 101198. [ Google Scholar ] [ CrossRef ]
- Xie, Z.; Wu, R.; Wang, S. How technological progress affects the carbon emission efficiency? Evidence from national panel quantile regression. J. Clean. Prod. 2021 , 307 , 127133. [ Google Scholar ] [ CrossRef ]
- Ezinwa Nwagwu, W. Creating science and technology information databases for developing and sustaining sub-Saharan Africa’s indigenous knowledge. J. Inf. Sci. 2007 , 33 , 737–751. [ Google Scholar ] [ CrossRef ]
- Lypak, H.; Rzheuskyi, A.; Kunanets, N.; Pasichnyk, V. Formation of a consolidated information resource by means of cloud technologies. In Proceedings of the 2018 International Scientific-Practical Conference Problems of Infocommunications, Kharkiv, Ukraine, 9–12 October 2018; Science and Technology (PIC S&T): Kharkiv, Ukraine, 2018; pp. 157–160. [ Google Scholar ]
- Makinde, O.B.M.; Jiyane, G.V.; Mugwisi, T. Information resources importance and format inclination of Science and Technology researchers. Int. J. Inf. Sci. Manag. 2020 , 18 , 83–96. [ Google Scholar ]
- Fry, J. Scholarly research and information practices: A domain analytic approach. Inform. Process Manag. 2006 , 42 , 299–316. [ Google Scholar ] [ CrossRef ]
- Mikhaylova, A.A.; Mikhaylov, A.S.; Savchina, O.V.; Plotnikova, A.P. Innovation landscape of the Baltic region. Adm. Public. Manag. Rev. 2019 , 33 , 165–180. [ Google Scholar ] [ CrossRef ]
- Jonscher, C. Information resources and economic productivity. Inf. Econ. Policy 1983 , 1 , 13–35. [ Google Scholar ] [ CrossRef ]
- Zhao, Y.; Zhang, L. System Dynamics Modeling and Simulation for Information Resources Allocation in R&D Cooperation. Data Anal. Knowl. Discov. 2011 , 27 , 54–61. [ Google Scholar ]
- Popoola, S.O. The use of information sources and services and its effect on the research output of social scientists in Nigerian universities. Libr. Philos. Pract. 2008 , 183 , 1–10. [ Google Scholar ]
- Sutrisno, S.; Ausat, A.M.A.; Permana, B.; Harahap, M.A.K. Do Information Technology and Human Resources Create Business Performance: A Review. Int. J. Prof. Bus. Rev. 2023 , 8 , 14. [ Google Scholar ] [ CrossRef ]
- Zhao, J. Dual innovation: The road to sustainable development of enterprises. Int. J. Inov. Sci. 2021 , 13 , 423–436. [ Google Scholar ] [ CrossRef ]
- Laplane, A.; Mazzucato, M.; Laplane, A.; Mazzucato, M. Socializing the risks and rewards of public investments: Economic, policy, and legal issues. Res. Policy 2020 , 49 , 100008. [ Google Scholar ] [ CrossRef ]
- Kolade, O.; Adegbile, A.; Sarpong, D. Can university-industry-government collaborations drive a 3D printing revolution in Africa? A triple helix model of technological leapfrogging in additive manufacturing. Technol. Soc. 2022 , 69 , 101960. [ Google Scholar ] [ CrossRef ]
- Lüdeke Freund, F. Sustainable entrepreneurship, innovation, and business models: Integrative framework and propositions for future research. Bus. Strateg. Environ. 2020 , 29 , 665–681. [ Google Scholar ] [ CrossRef ]
- Xia, Q.H.; Zhu, Q. Group Analysis and Paradigm Choice of Breakthrough Innovation for "Specialized, Specialized and New" Enterprises. Foreign Econ. Manag. 2023 , 45 , 20–34. [ Google Scholar ]
- Zhang, J.C.; Long, J. How Digital Technology Adoption Drives Breakthrough Innovation in Business. J. Shanxi Univ. Financ. Econ. 2022 , 44 , 69–83. [ Google Scholar ]
- Bahemia, H.; Sillince, J.; Vanhaverbeke, W. The Timing of Openness in a Radical Innovation Project, a Temporal and Loose Coupling Perspective. Res. Policy 2018 , 47 , 2066–2076. [ Google Scholar ] [ CrossRef ]
- Wang, Y.J.; Xie, W.H.; Wang, T.H.; Cheng, M.H. A Study of the Relationship between Strengths and Weaknesses and Breakthrough Innovation—The Mediating Role of Absorptive Capacity and the Moderating Effects of Environmental Dynamics. Manag. Rev. 2016 , 28 , 111–122. [ Google Scholar ]
- Bi, X.F.; Liu, S.Y.; Fu, S.Z.; Xing, X.H. Does Surplus Smoothing Affect Firms’ Breakthrough Innovation—An External Stakeholder Evaluation Perspective. Account. Res. 2022 , 60 , 91–102. [ Google Scholar ]
- Dess, G.G.; Lumpkin, G.T. Emerging issues in strategy process research. Blackwell Handb. Strateg. Manag. 2005 , 1 , 1–32. [ Google Scholar ]
- Chen, C.S.; Li, D.Y.; Li, C.W.; Yi, C.J. Overseas Chinese Networks, Corporate Strategic Aggressiveness and OFDI ; Huaqiao University: Fujian, China, 2023. [ Google Scholar ]
- Al-Mamary, Y.H.; Alshallaqi, M. Impact of autonomy, innovativeness, risk-taking, proactiveness, and competitive aggressiveness on students’ intention to start a new venture. J. Innov. Knowl. 2022 , 7 , 100239. [ Google Scholar ] [ CrossRef ]
- Luiz, J.M.; Magada, T.; Mukumbuzi, R. Strategic responses to institutional voids (rationalization, aggression, and defensiveness): Institutional complementarity and why the home country matters. Manag. Int. Rev. 2021 , 61 , 681–711. [ Google Scholar ] [ CrossRef ]
- Chih-Yi, S.; Bou-Wen, L. Attack and defense in patent-based competition: A new paradigm of strategic decision-making in the era of the fourth industrial revolution. Technol. Forecast. Soc. 2021 , 167 , 120670. [ Google Scholar ] [ CrossRef ]
- Al-Mamary, Y.H.; Alwaheeb, M.A.; Alshammari, N.G.M.; Abdulrab, M.; Balhareth, H.; Soltane, H.B. The effect of entrepreneurial orientation on financial and non-financial performance in Saudi SMES: A review. J. Crit. Rev. 2020 , 7 , 270–278. [ Google Scholar ]
- Zhou, J.; Li, J.; Jiao, H.; Qiu, H.; Liu, Z. The more funding the better? The moderating role of knowledge stock on the effects of different government-funded research projects on firm innovation in Chinese cultural and creative industries. Technovation 2020 , 92 , 102059. [ Google Scholar ] [ CrossRef ]
- Carayannis, E.G.; Dezi, L.; Gregori, G.; Calo, E. Smart environments and techno-centric and human-centric innovations for Industry and Society 5.0: A quintuple helix innovation system view towards smart, sustainable, and inclusive solutions. J. Knowl. Econ. 2022 , 13 , 926–955. [ Google Scholar ] [ CrossRef ]
- Liu, W.; Tan, R.; Li, Z.; Cao, G.; Yu, F. A patent-based method for monitoring the development of technological innovations based on knowledge diffusion. J. Knowl. Manag. 2021 , 25 , 380–401. [ Google Scholar ] [ CrossRef ]
- Chiu, M.L.; Cheng, T.S.; Lin, C.N. Driving Open Innovation Capability Through New Knowledge Diffusion of Integrating Intrinsic and Extrinsic Motivations in Organizations: Moderator of Individual Absorptive Capacity. J. Knowl. Econ. 2023 , 15 , 3685–3717. [ Google Scholar ] [ CrossRef ]
- Bierly III, P.E.; Damanpour, F.; Santoro, M.D. The application of external knowledge: Organizational conditions for exploration and exploitation. J. Manag. Stud. 2009 , 46 , 481–509. [ Google Scholar ] [ CrossRef ]
- Carnabuci, G.; Bruggeman, J. Knowledge specialization, knowledge brokerage and the uneven growth of technology domains. Soc. Forces 2009 , 88 , 607–641. [ Google Scholar ] [ CrossRef ]
- Fortunato, S.; Bergstrom, C.T.; Börner, K.; Evans, J.A.; Helbing, D.; Milojević, S.; Petersen, A.M.; Radicchi, F.; Sinatra, R.; Uzzi, B.; et al. Science of science. Science 2018 , 359 , eaao0185. [ Google Scholar ] [ CrossRef ] [ PubMed ]
- Dolata, U. Technological innovations and sectoral change: Transformative capacity, adaptability, patterns of change: An analytical framework. Res. Policy 2009 , 38 , 1066–1076. [ Google Scholar ] [ CrossRef ]
- Fang, H.; Huo, Q.; Hatim, K. Can Digital Services Trade Liberalization Improve the Quality of Green Innovation of Enterprises? Evidence from China. Sustainability 2023 , 15 , 6674. [ Google Scholar ] [ CrossRef ]
- Tijssen, R.J. Global and domestic utilization of industrial relevant science: Patent citation analysis of science–technology interactions and knowledge flows. Res. Policy 2001 , 30 , 35–54. [ Google Scholar ] [ CrossRef ]
- Kapoor, R.; Adner, R. What firms make vs. what they know: How firms’ production and knowledge boundaries affect competitive advantage in the face of technological chang. Organ. Sci. 2012 , 23 , 1227–1248. [ Google Scholar ] [ CrossRef ]
- Akpan, I.J.; Soopramanien, D.; Kwak, D.H. Cutting-edge technologies for small business and innovation in the era of COVID-19 global health pandemic. J. Small Bus. Entrep. 2021 , 33 , 607–617. [ Google Scholar ] [ CrossRef ]
- Teece, D.J. Technological innovation and the theory of the firm: The role of enterprise-level knowledge, complementarities, and (dynamic) capabilities. Handb. Econ. Innov. 2010 , 1 , 679–730. [ Google Scholar ]
- Wang, C.; Chin, T.; Lin, J.H. Openness and firm innovation performance: The moderating effect of ambidextrous knowledge search strategy. J. Knowl. Manag. 2020 , 24 , 301–323. [ Google Scholar ] [ CrossRef ]
- Xue, J. Understanding knowledge networks and knowledge flows in high technology clusters: The role of heterogeneity of knowledge contents. Innovation 2018 , 20 , 139–163. [ Google Scholar ] [ CrossRef ]
- Lindelöf, P.; Löfsten, H. Proximity as a resource base for competitive advantage: University–industry links for technology transfer. J. Technol. Transf. 2004 , 29 , 311–326. [ Google Scholar ] [ CrossRef ]
- Tohãnean, D.; Buzatu, A.I.; Baba, C.A.; Georgescu, B. Business model innovation through the use of digital technologies: Managing risks and creating sustainability. Amfiteatru Econ. 2020 , 22 , 758–774. [ Google Scholar ]
- Wang, S.H.; Wang, Z.J.; Tian, Y. A study of the relationship between managerial overconfidence and firms’ investment in technological innovation. Res. Manag. 2013 , 34 , 1–9. [ Google Scholar ]
- Ràfols, I. S&T indicators in the wild: Contextualization and participation for responsible metrics. Res. Eval. 2019 , 28 , 7–22. [ Google Scholar ]
- Cao, Q.; Li, Y.; Peng, H. From university basic research to firm innovation: Diffusion mechanism and boundary conditions under a U-shaped relationship. Technovation 2023 , 123 , 102718. [ Google Scholar ] [ CrossRef ]
- Cotropia, C.A.; Lemley, M.A.; Sampat, B. Do applicant patent citations matter? Res. Policy 2013 , 42 , 844–854. [ Google Scholar ] [ CrossRef ]
- Chen, L. Do patent citations indicate knowledge linkage? The evidence from text similarities between patents and their citations. J. Informetr. 2017 , 11 , 63–79. [ Google Scholar ] [ CrossRef ]
- Lin, R.H.; Wang, L. The Impact of Knowledge Integration Capability on Breakthrough Innovation Based on Exploratory Innovation—The Moderating Role of Firm Absorptive Capacity and Openness to Innovation. Sci. Technol. Manag. Res. 2023 , 43 , 19–27. [ Google Scholar ]
- Jiang, S.Y.; Zhuang, Y.M.; Ding, L. Industry-University-Research Basic Research Cooperation, Financial and Tax Incentive Options, and Firms’ Breakthrough Innovation. Res. Manag. 2021 , 42 , 40–47. [ Google Scholar ]
- Ahuja, G.; Lampert, C.M. Entrepreneurship in the Large Corporation: A Longitudinal Study of How Established Firms Create Breakthrough Inventions. Strateg. Manag. J. 2001 , 21 , 267–294. [ Google Scholar ] [ CrossRef ]
- Bentley, K.A.; Omer, T.C.; Sharp, N.Y. Business strategy, financial reporting irregularities, and audit effort. Contemp. Account. Res. 2013 , 30 , 780–817. [ Google Scholar ] [ CrossRef ]
- Zhang, D.L.; Xu, S.S.; Xue, F.; Wang, H.C. Strategic Aggressiveness and CSR Fulfillment—A Resource Acquisition-Based Perspective. China Soft Sci. 2022 , 8 , 111–123. [ Google Scholar ]
- Song, M.; Wang, S.; Zhang, H. Could environmental regulation and R&D tax incentives affect green product innovation? J. Clean. Prod. 2020 , 258 , 120849. [ Google Scholar ]
- Chen, C.Y.; Lin, S.H.; Chou, L.C.; Chen, K.D. A comparative study of production efficiency in coastal region and non-coastal region in Mainland China: An application of metafrontier model. J. Int. Trade Econ. Dev. 2018 , 27 , 901–916. [ Google Scholar ] [ CrossRef ]
- Nana Yaw Simpson, S. Boards and governance of state-owned enterprises. Corp. Gov. 2014 , 14 , 238–251. [ Google Scholar ] [ CrossRef ]
- Doh, J.P.; Teegen, H. Nongovernmental organizations as institutional actors in international business: Theory and implications. International Business Review. Int. Bus. Rev. 2022 , 11 , 665–684. [ Google Scholar ] [ CrossRef ]
- Wynarczyk, P.; Piperopoulos, P.; McAdam, M. Open innovation in small and medium-sized enterprises: An overview. Int. Small Bus. J. 2013 , 31 , 240–255. [ Google Scholar ] [ CrossRef ]
- Wang, Y.C.; Phillips, F.; Yang, C. Bridging innovation and commercialization to create value: An open innovation study. J. Bus. Res. 2021 , 123 , 255–266. [ Google Scholar ] [ CrossRef ]
- Zou, L.; Cao, X.Z.; Zhu, Y.W. Research on Regional High-Tech Innovation Efficiency and Influence Factors: Evidence from Yangtze River Economic Belt in China. Complexity 2021 , 2021 , 9946098. [ Google Scholar ] [ CrossRef ]
- Meng, M.; Lei, J.; Jiao, J.; Tao, Q. How does strategic flexibility affect bricolage: The moderating role of environmental turbulence. PLoS ONE 2020 , 15 , e0238030. [ Google Scholar ] [ CrossRef ] [ PubMed ]
- Zhang, F.; Yang, B.; Zhu, L. Digital technology usage, strategic flexibility, and business model innovation in traditional manufacturing firms: The moderating role of the institutional environment. Technol. Forecast. Social. Change 2023 , 194 , 122726. [ Google Scholar ] [ CrossRef ]
- Müller, J.M.; Buliga, O.; Voigt, K.I. The role of absorptive capacity and innovation strategy in the design of industry 4.0 business Models-A comparison between SMEs and large enterprises. Eur. Manag. J. 2021 , 39 , 333–343. [ Google Scholar ] [ CrossRef ]
- Ryan Charleton, T.; Galavan, R.J. Multimarket contact between partners and strategic alliance survival. Strateg. Manag. J. 2024 , 2024 , 1–30. [ Google Scholar ]
- Bouncken, R.B.; Kraus, S. Innovation in knowledge-intensive industries: The double-edged sword of coopetition. J. Bus. Res. 2013 , 66 , 2060–2070. [ Google Scholar ] [ CrossRef ]
- Šmejkal, A.; Novotná, M.; Volek, T. Company Investments in the Context of Financial Strategies. Argum. Oecon 2022 , 48 , 164–185. [ Google Scholar ] [ CrossRef ]
- Chen, J.; Wang, L.; Li, Y. Natural resources, urbanization and regional innovation capabilities. Resour. Policy 2020 , 66 , 101643. [ Google Scholar ] [ CrossRef ]
- Ganau, R.; Grandinetti, R. Disentangling regional innovation capability: What really matters? Ind. Innov. 2021 , 28 , 749–772. [ Google Scholar ] [ CrossRef ]
- Hjaltadóttir, R.E.; Makkonen, T.; Mitze, T. Inter-regional innovation cooperation and structural heterogeneity: Does being a rural, or border region, or both, make a difference? J. Rural. Stud. 2020 , 74 , 257–270. [ Google Scholar ] [ CrossRef ]
- Yang, R.; Che, T.; Lai, F. The Impacts of production linkages on cross-regional collaborative innovations: The role of inter-regional network capital. Technol. Forecast. Soc. 2021 , 170 , 120905. [ Google Scholar ] [ CrossRef ]
- Genin, A.L.; Tan, J.; Song, J. State governance and technological innovation in emerging economies: State-owned enterprise restructuration and institutional logic dissonance in China’s high-speed train sector. J. Int. Bus. Stud. 2021 , 52 , 621–645. [ Google Scholar ] [ CrossRef ]
Click here to enlarge figure
Variable Type | Variable Name | Variable Breakdown Name | Symbol |
---|---|---|---|
Independent variable | Utilization of enterprise scientific and technological information resources | Intensity of utilization of enterprise S&T information resources | STIRA_In |
Imbalance of utilization of enterprise S&T information resources | STIRA_Im | ||
Moderating variable | Strategic aggressiveness | Strategic aggressiveness | SA |
Dependent variable | Breakthrough innovation | Enterprise breakthrough innovation performance | BI |
Control variable | Control variables | Enterprise age | Ea |
Enterprise profitability | Ep | ||
Nature of property rights | Npr | ||
Number of R&D Staff | R&Dsn | ||
Investment in R&D | R&Di | ||
Time dummy | Year | ||
Industry dummy | Ind |
Coefficients | ||||
---|---|---|---|---|
(b) | (B) | (b-B) | Sqrt (Diag(V_b-V_B)) | |
FE | RE | Difference | Std. Err. | |
Stira_In | −0.031944 | −0.0329217 | 0.0009777 | 0.0005199 |
Stira_Im | 0.1151972 | 0.1242172 | −0.00902 | 0.0008367 |
Ea | −0.0099359 | −0.0160318 | 0.0060959 | 0.0034465 |
Ep | −2.20 × 10 | 1.07 × 10 | −3.27 × 10 | 1.73 × 10 |
R&Dsn | 0.0219173 | 0.01065 | 0.0112673 | 0.0020405 |
R&Di | 0.1543455 | 0.2717775 | −0.117432 | 0.008623 |
Variable | N | Mean | p50 | SD | Min | Max |
---|---|---|---|---|---|---|
STIRA_In | 14,632 | 6.008 | 6 | 2.549 | 0 | 34 |
STIRA_Im | 14,632 | 2.714 | 2.51 | 2.144 | 0 | 23.784 |
BI | 14,632 | 2.135 | 1.946 | 1.147 | 0.693 | 9.028 |
SA | 6611 | 11.683 | 12 | 3.835 | 0 | 23 |
Ea | 14,632 | 17.352 | 17 | 6.042 | 1 | 63 |
Ep | 14,632 | −13.843 | 11.198 | 1612.435 | −160,034.734 | 4805.529 |
R&Dsn | 14,632 | 3.19 | 4.382 | 2.897 | 0 | 10.485 |
R&Di | 14,150 | 17.855 | 17.726 | 1.311 | 8.007 | 23.491 |
Variable | BI | STIRA_In | STIRA_Im | SA | Ea | Ep | Npr | R&Dsn | R&Di | Mean VIF |
---|---|---|---|---|---|---|---|---|---|---|
BI | 1 | |||||||||
STIRA_In | 0.036 *** | 1 | ||||||||
STIRA_Im | 0.375 *** | 0.353 *** | 1 | |||||||
SA | −0.059 *** | −0.023 * | −0.002 | 1 | ||||||
Ea | 0.097 *** | 0.028 *** | 0.128 *** | −0.137 *** | 1 | |||||
Ep | 0.001 | 0.01 | −0.003 | 0.018 | 0.012 | 1 | ||||
Npr | 0.134 *** | 0.020 ** | 0.017 ** | −0.189 *** | 0.178 *** | 0.005 | 1 | |||
R&Dsn | 0.244 *** | 0.057 *** | 0.265 *** | −0.042 *** | 0.386 *** | 0.003 | 0.085 *** | 1 | ||
R&Di | 0.525 *** | 0.044 *** | 0.225 *** | −0.165 *** | 0.246 *** | −0.027 *** | 0.216 *** | 0.482 *** | 1 | |
VIF | - | 1.17 | 1.27 | 1.08 | 1.12 | 1.01 | 1.08 | 1.36 | 1.27 | 1.17 |
1/VIF | - | 0.857186 | 0.789739 | 0.926606 | 0.894439 | 0.989701 | 0.924842 | 0.734387 | 0.787834 | - |
(1) | (2) | (3) | (4) | (5) | (6) | |
---|---|---|---|---|---|---|
BI | BI | BI | BI | BI | BI | |
STIRA_In | 0.0164 *** | 0.00634 ** | 0.00757 ** | |||
(4.40) | (1.98) | (2.37) | ||||
STIRA_Im | 0.201 *** | 0.150 *** | 0.160 *** | |||
(49.00) | (39.87) | (41.72) | ||||
Ea | −0.00928 *** | −0.00377 ** | −0.0107 *** | −0.00305 ** | ||
(−6.20) | (−2.48) | (−7.51) | (−2.13) | |||
Ep | 0.0000114 ** | 0.00000699 | 0.0000113 ** | 0.00000561 | ||
(2.25) | (1.41) | (2.37) | (1.20) | |||
Npr | 0.0816 *** | 0.0684 *** | 0.110 *** | 0.0676 *** | ||
(4.03) | (3.26) | (5.72) | (3.42) | |||
R&Dsn | 0.000926 | 0.00216 | −0.0194 *** | 0.00213 | ||
(0.27) | (0.46) | (−5.92) | (0.48) | |||
R&Di | 0.463 *** | 0.459 *** | 0.429 *** | 0.417 *** | ||
(63.53) | (59.92) | (61.65) | (57.01) | |||
_cons | 2.037 *** | −6.021 *** | −6.001 *** | 1.590 *** | −5.706 *** | −5.476 *** |
(83.97) | (−47.51) | (−40.30) | (112.21) | (−47.82) | (−38.96) | |
N | 14,632 | 14,150 | 14,150 | 14,632 | 14,150 | 14,150 |
Year | No | No | Yes | No | No | Yes |
Ind | No | No | Yes | No | No | Yes |
R | 0.001 | 0.279 | 0.309 | 0.141 | 0.352 | 0.385 |
adj. R | 0.001 | 0.279 | 0.307 | 0.141 | 0.352 | 0.383 |
(1) | (2) | (3) | (4) | |
---|---|---|---|---|
BI | BI | BI | BI | |
STIRA_In | 0.00757 ** | 0.0522 *** | ||
(2.37) | (5.61) | |||
STIRA_Im | 0.160 *** | 0.264 *** | ||
(41.72) | (18.40) | |||
Ea | −0.00377 ** | 0.0134 *** | −0.00305 ** | 0.0125 *** |
(−2.48) | (4.14) | (−2.13) | (4.10) | |
Ep | 0.00000699 | 0.00311 *** | 0.00000561 | 0.00244 *** |
(1.41) | (4.48) | (1.20) | (3.73) | |
Npr | 0.0684 *** | 0.300 *** | 0.0676 *** | 0.261 *** |
(3.26) | (8.91) | (3.42) | (8.25) | |
R&Dsn | 0.00216 | 0.00213 | ||
(0.46) | (0.48) | |||
R&Di | 0.459 *** | 0.417 *** | ||
(59.92) | (57.01) | |||
STIRA_In * SA | −0.00201 *** | |||
(−3.31) | ||||
STIRA_Im * SA | −0.00503 *** | |||
(−4.75) | ||||
_cons | −6.001 *** | 1.405 *** | −5.476 *** | 1.338 *** |
(−40.30) | (8.71) | (−38.96) | (8.98) | |
N | 14,150 | 6611 | 14,150 | 6611 |
Year | Yes | Yes | Yes | Yes |
Ind | Yes | Yes | Yes | Yes |
R | 0.309 | 0.122 | 0.385 | 0.223 |
adj. R | 0.307 | 0.115 | 0.383 | 0.218 |
-Value | -Value | |||||
TMTIP | 23.22846 | 1.70 | 0.09 | 74.77208 | 6.5 | <0.01 |
Constant Term | 6.001367 | 280.14 | <0.01 | 2.692901 | 149.6 | <0.01 |
N | 14,632 | 14,632 | ||||
R | 0.000 | 0.0028 | ||||
-Value | ||||||
STIRA_In | 0.0164 | 4.40 | <0.01 | |||
STIRA_Im | 0.201 | 49.00 | <0.01 | |||
Constant Term | 2.037 | 83.97 | <0.01 | |||
N | 14,632 | |||||
R | 0.001 |
One Period Lag | Change Sample Size | Add Regional Dummy Variables | Replace Dependent Variable | |||||
---|---|---|---|---|---|---|---|---|
BI | BI | BI | BI | BI | BI | BI | BI | |
L.STIRA_In | 0.00824 ** | |||||||
(2.02) | ||||||||
L.STIRA_Im | 0.0968 *** | |||||||
(19.36) | ||||||||
STIRA_In | 0.0134 *** | 0.00731 ** | 0.0645 *** | |||||
(3.73) | (2.29) | (4.31) | ||||||
STIRA_Im | 0.141 *** | 0.160 *** | 0.326 *** | |||||
(32.31) | (41.68) | (17.28) | ||||||
Ea | −0.00504 *** | −0.00485 *** | −0.00476 ** | −0.00417 ** | −0.00368 ** | −0.00301 ** | −0.0114 | −0.00995 |
(−2.90) | (−2.84) | (−2.50) | (−2.32) | (−2.42) | (−2.10) | (−1.60) | (−1.41) | |
Ep | 0.00000717 | 0.00000665 | 0.00000682 | 0.00000603 | 0.00000688 | 0.00000556 | 0.0000232 | 0.0000215 |
(1.35) | (1.27) | (1.35) | (1.26) | (1.39) | (1.19) | (1.00) | (0.93) | |
Npr | 0.0609 ** | 0.0679 *** | 0.0338 | 0.0323 | 0.0611 *** | 0.0643 *** | 0.474 *** | 0.475 *** |
(2.55) | (2.90) | (1.22) | (1.23) | (2.85) | (3.18) | (4.82) | (4.88) | |
R&Dsn | −0.00367 | −0.00582 | −0.00206 | 0.000857 | 0.00194 | 0.00203 | −0.0608 *** | −0.0604 *** |
(−0.67) | (−1.08) | (−0.36) | (0.16) | (0.41) | (0.46) | (−2.76) | (−2.77) | |
R&Di | 0.495 *** | 0.475 *** | 0.481 *** | 0.435 *** | 0.460 *** | 0.417 *** | 1.264 *** | 1.181 *** |
(55.19) | (53.58) | (45.76) | (43.25) | (59.94) | (57.01) | (35.14) | (32.83) | |
_cons | −6.448 *** | −6.229 *** | −6.346 *** | −5.755 *** | −5.988 *** | −5.471 *** | −18.32 *** | −17.06 *** |
(−36.30) | (−35.78) | (−33.30) | (−31.81) | (−40.16) | (−38.88) | (−26.22) | (−24.65) | |
N | 10,945 | 10,945 | 9028 | 9028 | 14,150 | 14,150 | 14,150 | 14,150 |
Year | Yes | Yes | Yes | Yes | Yes | Yes | Yes | Yes |
Ind | Yes | Yes | Yes | Yes | Yes | Yes | Yes | Yes |
Province | No | No | No | No | Yes | Yes | No | No |
R2 | 0.329 | 0.351 | 0.313 | 0.384 | 0.310 | 0.385 | 0.165 | 0.181 |
adj. R2 | 0.325 | 0.348 | 0.310 | 0.381 | 0.307 | 0.383 | 0.162 | 0.178 |
One Period Lag | Change Sample Size | Add Regional Dummy Variables | Replace Dependent Variable | |||||
---|---|---|---|---|---|---|---|---|
BI | BI | BI | BI | BI | BI | BI | BI | |
L.STIRA_In | 0.00542 * | |||||||
(1.83) | ||||||||
L.STIRA_Im | 0.0859 *** | |||||||
(11.15) | ||||||||
STIRA_In | 0.00268 * | 0.0116 * | 0.0864 ** | |||||
(1.28) | (1.44) | (2.12) | ||||||
STIRA_Im | 0.133 *** | 0.148 *** | 0.409 *** | |||||
(9.47) | (11.59) | (6.13) | ||||||
Ea | 0.00623 ** | 0.00845 *** | 0.00779 ** | 0.00652 ** | 0.00704 ** | 0.00627 ** | 0.0417 *** | 0.0402 *** |
(2.08) | (2.95) | (2.36) | (2.06) | (2.52) | (2.36) | (2.98) | (2.89) | |
Ep | 0.000780 | 0.000379 | 0.00141 * | 0.000904 | 0.000610 | 0.000299 | 0.00367 | 0.00314 |
(1.20) | (0.61) | (1.79) | (1.20) | (1.00) | (0.51) | (1.19) | (1.03) | |
Npr | 0.153 *** | 0.175 *** | 0.127 *** | 0.102 *** | 0.170 *** | 0.143 *** | 0.734 *** | 0.687 *** |
(4.85) | (5.76) | (3.53) | (2.97) | (5.70) | (5.05) | (5.01) | (4.73) | |
R&Dsn | 0.0778 *** | 0.0586 *** | 0.0953 *** | 0.0850 *** | 0.100 *** | 0.0826 *** | 0.412 *** | 0.379 *** |
(4.71) | (3.69) | (4.96) | (4.61) | (6.69) | (5.77) | (5.47) | (5.05) | |
R&Di | 0.484 *** | 0.464 *** | 0.451 *** | 0.416 *** | 0.441 *** | 0.406 *** | 1.105 *** | 1.041 *** |
(31.83) | (31.76) | (23.16) | (22.28) | (33.22) | (31.99) | (16.55) | (15.69) | |
STIRA_In*SA | −0.00134 *** | −0.00137 ** | −0.00192 *** | −0.000944 * | ||||
(−3.47) | (−2.14) | (−3.62) | (−1.35) | |||||
STIRA_Im*SA | −0.00840 *** | −0.000192 * | −0.000787 * | −0.00788 * | ||||
(−16.74) | (1.19) | (−1.84) | (−1.62) | |||||
_cons | −6.569 *** | −6.423 *** | −6.878 *** | −6.242 *** | −6.014 *** | −5.490 *** | −16.57 *** | −15.39 *** |
(−20.39) | (−21.01) | (−21.49) | (−20.36) | (−22.55) | (−21.67) | (−12.37) | (−11.61) | |
N | 5420 | 5420 | 4448 | 4448 | 6539 | 6539 | 14,632 | 14,632 |
Year | Yes | Yes | Yes | Yes | Yes | Yes | Yes | Yes |
Ind | Yes | Yes | Yes | Yes | Yes | Yes | Yes | Yes |
Province | No | No | No | No | Yes | Yes | No | No |
R2 | 0.366 | 0.416 | 0.359 | 0.411 | 0.349 | 0.408 | 0.078 | 0.107 |
adj. R2 | 0.360 | 0.410 | 0.353 | 0.405 | 0.344 | 0.403 | 0.075 | 0.104 |
Non-Coastal | Coastal | Non-Coastal | Coastal | |
---|---|---|---|---|
BI | BI | BI | BI | |
STIRA_In | 0.0179 *** | 0.00288 * | ||
(3.01) | (1.76) | |||
STIRA_Im | 0.143 *** | 0.167 *** | ||
(20.46) | (36.54) | |||
Ea | −0.00584 * | −0.00183 | −0.00424 | −0.00139 |
(−1.77) | (−1.09) | (−1.35) | (−0.88) | |
Ep | −0.000125 | 0.00000834 * | −0.000127 * | 0.00000672 |
(−1.61) | (1.71) | (−1.70) | (1.47) | |
Npr | 0.00133 | 0.00141 | 0.00122 | 0.00157 |
(0.14) | (0.26) | (0.14) | (0.31) | |
R&Dsn | 0.418 *** | 0.489 *** | 0.391 *** | 0.437 *** |
(31.21) | (52.31) | (30.47) | (49.19) | |
R&Di | −5.114 *** | −6.610 *** | −4.819 *** | −5.923 *** |
(−19.91) | (−35.76) | (−19.79) | (−33.99) | |
_cons | 0.0179 *** | 0.00288 * | ||
(3.01) | (1.76) | |||
N | 4122 | 10,028 | 4122 | 10,028 |
Year | Yes | Yes | Yes | Yes |
Ind | Yes | Yes | Yes | Yes |
R2 | 0.319 | 0.317 | 0.381 | 0.398 |
adj. R2 | 0.312 | 0.314 | 0.374 | 0.395 |
Inter-group differences | p = 0.398 > 0.1 | p = 0.0408 < 0.1 |
Non-State | Nationalized | Non-State | Nationalized | |
---|---|---|---|---|
BI | BI | BI | BI | |
STIRA_In | 0.00381 * | 0.0235 *** | ||
(1.13) | (2.79) | |||
STIRA_Im | 0.158 *** | 0.169 *** | ||
(38.61) | (17.37) | |||
Ea | −0.00541 *** | 0.00573 | −0.00463 *** | 0.00655 * |
(−3.36) | (1.38) | (−3.07) | (1.65) | |
Ep | 0.00000734 | 0.000506 | 0.00000571 | 0.000348 |
(1.54) | (0.70) | (1.28) | (0.50) | |
R&Dsn | 0.00185 | 0.0214 | 0.00322 | 0.0129 |
(0.37) | (1.47) | (0.69) | (0.93) | |
R&Di | 0.456 *** | 0.448 *** | 0.409 *** | 0.415 *** |
(49.72) | (28.86) | (47.12) | (27.78) | |
_cons | −5.889 *** | −6.044 *** | −5.318 *** | −5.621 *** |
(−33.80) | (−18.72) | (−32.52) | (−18.24) | |
N | 10,952 | 3198 | 10,952 | 3198 |
Year | Yes | Yes | Yes | Yes |
Ind | Yes | Yes | Yes | Yes |
R2 | 0.268 | 0.379 | 0.356 | 0.431 |
adj. R2 | 0.265 | 0.371 | 0.353 | 0.424 |
Inter-group differences | p = 0.0317 < 0.1 | p = 0.4417 > 0.1 |
The statements, opinions and data contained in all publications are solely those of the individual author(s) and contributor(s) and not of MDPI and/or the editor(s). MDPI and/or the editor(s) disclaim responsibility for any injury to people or property resulting from any ideas, methods, instructions or products referred to in the content. |
Share and Cite
Hou, J.; Yang, X.; Song, H. The Impact of Scientific and Technological Information Resource Utilization on Breakthrough Innovation in Enterprises: The Moderating Role of Strategic Aggressiveness. Systems 2024 , 12 , 248. https://doi.org/10.3390/systems12070248
Hou J, Yang X, Song H. The Impact of Scientific and Technological Information Resource Utilization on Breakthrough Innovation in Enterprises: The Moderating Role of Strategic Aggressiveness. Systems . 2024; 12(7):248. https://doi.org/10.3390/systems12070248
Hou, Jianhua, Xiucai Yang, and Haoyang Song. 2024. "The Impact of Scientific and Technological Information Resource Utilization on Breakthrough Innovation in Enterprises: The Moderating Role of Strategic Aggressiveness" Systems 12, no. 7: 248. https://doi.org/10.3390/systems12070248
Article Metrics
Further information, mdpi initiatives, follow mdpi.
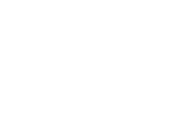
Subscribe to receive issue release notifications and newsletters from MDPI journals

COMMENTS
Explore the fundamentals of causal research, unraveling cause-and-effect relationships to drive informed decisions and insights. ... Both types of research contribute to the scientific understanding of phenomena, albeit through different approaches. ... Exploratory research aims to explore new topics, generate hypotheses, or gain initial ...
Help companies improve internally. By conducting causal research, management can make informed decisions about improving their employee experience and internal operations. For example, understanding which variables led to an increase in staff turnover. Repeat experiments to enhance reliability and accuracy of results.
Types of research can be categorized based on the research aims, the type of data, and the subjects, timescale, and location of the research. ... Exploratory research aims to explore the main aspects of an under-researched problem, ... while experimental research manipulates and controls variables to determine cause and effect.
Causal research is sometimes called an explanatory or analytical study. It delves into the fundamental cause-and-effect connections between two or more variables. Researchers typically observe how changes in one variable affect another related variable. Examining these relationships gives researchers valuable insights into the mechanisms that ...
Causal studies focus on an analysis of a situation or a specific problem to explain the patterns of relationships between variables. Experiments are the most popular primary data collection methods in studies with causal research design. The presence of cause cause-and-effect relationships can be confirmed only if specific causal evidence exists.
Causal research is also known as explanatory research. It's a type of research that examines if there's a cause-and-effect relationship between two separate events. This would occur when there is a change in one of the independent variables, which is causing changes in the dependent variable. You can use causal research to evaluate the ...
Defining Causal Research. Causal research investigates why one variable (the independent variable) is causing things to change in another ( the dependent variable). For example, a causal research study about the cause-and-effect relationship between smoking and the prevalence of lung cancer. Smoking prevalence would be the independent variable ...
Regression-discontinuity methods have been little used in medical research to date, but they can be helpful in the study of cause-and-effect relationships from observational data . Regression-discontinuity design is a quasi-experimental approach ( box 3 ) that was developed in educational psychology in the 1960s ( 18 ).
Causal research, also known as explanatory research, is a method of conducting research that aims to identify the cause-and-effect relationship between situations or variables. This is a valuable research method, as various factors can contribute to observable events, changes, or developments . When conducting explanatory research, there are ...
Nature Methods 7 , 243 ( 2010) Cite this article. The experimental tractability of biological systems makes it possible to explore the idea that causal relationships can be estimated from ...
Explanatory research can also be explained as a "cause and effect" model, investigating patterns and trends in existing data that haven't been previously investigated. ... Exploratory research aims to explore the main aspects of an under-researched problem, while explanatory research aims to explain the causes and consequences of a well ...
Causal research, sometimes referred to as explanatory research, is a type of study that evaluates whether two different situations have a cause-and-effect relationship. Since many alternative factors can contribute to cause-and-effect, researchers design experiments to collect statistical evidence of the connection between the situations.
Permits the researcher to identify cause and effect relationships between variables and to distinguish placebo effects from treatment effects. Experimental research designs support the ability to limit alternative explanations and to infer direct causal relationships in the study. Approach provides the highest level of evidence for single studies.
From Eq. 2, we obtain \(N={f}^{-1}(Y)-g(X)\), where X and Y are the two observed variables representing cause and effect, respectively. To identify the cause and effect, a particular type of constrained nonlinear ICA [17, 53] is performed to extract two components that are as independent as possible. The two extracted components are the assumed ...
It is apparent then how many purposes quantitative research can serve. Some purposes focus on describing or exploring a problem, whereas others are concerned with establishing a cause-and-effect relationship. For each of these purposes (Fig 1), different types of quantitative research can be used to create credible evidence. Let us explore each ...
Definition: Explanatory research is a type of research that aims to uncover the underlying causes and relationships between different variables. It seeks to explain why a particular phenomenon occurs and how it relates to other factors. This type of research is typically used to test hypotheses or theories and to establish cause-and-effect ...
Benefits of researching cause and effect. Here are the advantages of this style of research: Improves user experience: Companies use this form of research to increase their investment return and improve employee and customer experiences. Solves problems quickly: Managers and researchers use the findings from such research to identify the cause ...
Explanatory research is the most common type of research method and is responsible for establishing cause-and-effect relationships that allow generalisations to be extended to similar realities. It is closely related to descriptive research, although it provides additional information about the observed object and its interactions with the ...
Establishing Cause and Effect. A central goal of most research is the identification of causal relationships, or demonstrating that a particular independent variable (the cause) has an effect on the dependent variable of interest (the effect). The three criteria for establishing cause and effect - association, time ordering (or temporal precedence), and non-spuriousness - are familiar to ...
Exploratory research is usually conducted when a researcher has just begun an investigation and wishes to understand the topic generally. Descriptive research is research that aims to describe or define the topic at hand. Explanatory research is research that aims to explain why particular phenomena work in the way that they do.
Let us distinguish two different ways to ask and address causal questions. One can begin with an outcome, i.e., Y, and then work backward to the causes, i.e., X s. The second option works in the other direction; one starts with a potential cause and then asks about its impact on Y.The former procedure is often called the "causes-of-effects" approach, whereas the latter is known as the ...
This type of research will recognize trends and patterns in data, but it does not go so far in its analysis to prove causes for these observed patterns. Cause and effect is not the basis of this type of observational research. The data, relationships, and distributions of variables are studied only. Variables are not manipulated; they are only ...
Lung cancer is a leading cause of cancer-related mortality globally, with a dismal 5-year survival rate, particularly for Lung Adenocarcinoma (LUAD). Mechanical changes within the tumor ...
Cardiovascular disease (CVD) is the leading cause of mortality worldwide, and at present, India has the highest burden of acute coronary syndrome and ST-elevation myocardial infarction (MI). A key reason for poor outcomes is non-adherence to medication. The intervention is a 2 × 2 factorial design trial applying two interventions individually and in combination with 1:1 allocation ratio: (i ...
The dataset, revealed through the newly launched Prediabetes/diabetes in youth ONline Dashboard (POND), aims to ignite a new wave of research into the escalating issue of diabetes among young people.
This study aims to explore the relationship between the utilization of scientific and technological information resources and breakthrough innovation in enterprises, examining the moderating role of strategic aggressiveness in this relationship. Based on an investigation of 438,228 patent data from 2616 Chinese enterprises, we construct a theoretical framework of "strategy-capability ...