- Skip to Content
- Berkeley Academic Guide Home
- Institution Home

Berkeley Berkeley Academic Guide: Academic Guide 2023-24
Biostatistics.

About the Program
Many issues in the health, medical, and biological sciences are addressed by collecting and exploring relevant data. The development and application of techniques to better understand such data is the fundamental concern of the Group in Biostatistics. The program offers training in theory of statistics and biostatistics, the computer implementation of analytic methods, and opportunities to use this knowledge in areas of biological/medical research. The curriculum is taught principally by members of the Division of Biostatistics (School of Public Health) and the Department of Statistics (College of Letters & Science) and provides a wide range of ideas and approaches to the analysis of data.
Established in 1955, the Graduate Group in Biostatistics curriculum offers instruction in statistical theory and computing, as well as opportunities to rigorously apply this knowledge in biological and medical research. The degree programs offered (listed below) are appropriate for students who have either a strong mathematical and statistical background with a focus in the biomedical sciences, or degrees in the biological sciences with a focus in mathematics and statistics. (The MA degree can be obtained under Plan I or Plan II. The PhD dissertation is administered according to Plan B.)
Master of Arts (MA)
The Masters of Arts Degree in Biostatistics is completed in 4 semesters. Candidates for this degree are expected to earn 48 units with courses in biostatistics, statistics, public health, and biology. Students pursuing the MA degree in Biostatistics will be expected, upon completion of the program, to be well-versed in the following areas:
Understand the foundations of statistical inference, e.g., maximum likelihood estimation, regression.
Have grounding in theoretical framework and ability to apply existing estimators in following categories:
Computational statistics
Multivariate analysis
Categorical data analysis
Survival analysis
Longitudinal data analysis
Causal inference
Clinical trials
Statistical genomics
Statistical computing
Have fluency in statistical programming languages for both analysis using classic methods and implementation of novel methods.
Identify and apply sound and pertinent methods to address statistical inference questions in biological, public health, and medical research.
Effectively communicate research findings, orally and in writing.
Doctor of Philosophy (PhD)
All Biostatistics PhD students are required to hold a master's degree in Biostatistics or a related field. The PhD degree requires 4-6 semesters of course work in biostatistics, statistics, and at least one other subject area (e.g., biology, environmental health, epidemiology). There are no unit or course requirements for the PhD, so a program of courses appropriate to a student's background and interests may be developed. Courses cover traditional topics as well as recent advances in biostatistics and in statistics. Those completing the PhD will have acquired a deep knowledge and understanding of these subject areas. Since graduates with doctorates often assume academic careers in research and teaching, a high degree of mastery in research design, theory, methodology, and execution is expected as well as the ability to communicate and present research findings and area of expertise in a clear, understandable manner.
Many doctoral graduates accept faculty positions in schools of public health, medicine, and statistics and/or math departments at colleges and universities, both in the United States and abroad. Some graduates take research positions, including with pharmaceutical companies, hospital research units, non-profits, and within the tech sector.
Biostatistics Doctor of Philosophy (PhD) with Designated Emphasis (DE)
Students enrolled in the UC Berkeley Biostatistics doctoral (PhD) program are eligible to apply for interdisciplinary study in a Designated Emphasis (DE), which we refer to as the Associated Programs. At UC Berkeley, acquiring a DE is like earning a "minor" with a PhD degree. Applications for Designated Emphasis are reviewed on a rolling basis throughout the year. However, students must apply prior to taking the qualifying exam and are strongly encouraged to begin the application process early in the third semester of graduate study. To be accepted to a Designated Emphasis, you must be a PhD candidate in one of the Associated Programs (e.g., Biostatistics). The two DE programs offered in biostatistics are:
- Designated Emphasis in Computational and Genomic Biology (DE-CGB)
- Designated Emphasis in Computational Science and Data Science and Engineering (DE-CSDE)
The goal of the DE-CGB program is to train a new generation of computational biology researchers by enhancing and facilitating interactions between faculty, postdoctoral scholars and students in the Associated Programs through a flexible and integrated research and teaching environment which transcends traditional departmental boundaries. Upon successful completion of all requirements and dissertation, your transcript and diploma will read, "PhD in Biostatistics with a Designated Emphasis in Computational & Genomic Biology."
The DE in Computational Science and Engineering (CSE) promises to bring a new paradigm to interdisciplinary research and education. The team-oriented approach provides our students with a solid foundation in the different facets of genomic research and ensuing competitive edge for the most desirable jobs in academia and industry, which increasingly require interdisciplinary training by combining high-performance computing, mathematical modeling, scientific and engineering theory, and analysis of large scale databases of observations. Upon successful completion of all requirements and dissertation, your transcript and diploma will read, "PhD in Biostatistics with a Designated Emphasis in Computational Science and Engineering."
Visit Group Website
Admission to the University
Applying for graduate admission.
Thank you for considering UC Berkeley for graduate study! UC Berkeley offers more than 120 graduate programs representing the breadth and depth of interdisciplinary scholarship. A complete list of graduate academic departments, degrees offered, and application deadlines can be found on the Graduate Division website .
Prospective students must submit an online application to be considered for admission, in addition to any supplemental materials specific to the program for which they are applying. The online application can be found on the Graduate Division website .
Admission Requirements
The minimum graduate admission requirements are:
A bachelor’s degree or recognized equivalent from an accredited institution;
A satisfactory scholastic average, usually a minimum grade-point average (GPA) of 3.0 (B) on a 4.0 scale; and
Enough undergraduate training to do graduate work in your chosen field.
For a list of requirements to complete your graduate application, please see the Graduate Division’s Admissions Requirements page . It is also important to check with the program or department of interest, as they may have additional requirements specific to their program of study and degree. Department contact information can be found here .
Where to apply?
Visit the Berkeley Graduate Division application page .
Master's Degree Requirements
Unit requirements.
Candidates for this degree are expected to complete 48 units, in four semesters.
New MA Students should take the following courses (for a letter-grade):
Additionally, students should take the following courses:, ma comprehensive examination.
The examination for the MA degree is designed to test a candidate's breadth and depth of understanding and knowledge and the ability to articulate and explain the basic concepts gained from the curriculum. The examination committee consists of two faculty members representing both biostatistics and statistics. Candidates are asked to select three topics and write a description of each. Topics are presented during the exam period of 90 minutes. Examiners are free to ask for clarification or elaboration through requests for more background, detail or examples.
Note that the decision to submit a thesis rather than take the oral comprehensive examination must be made early in the final semester of the program.
Doctoral Degree Requirements
Normative time requirements.
Since there are no unit or course requirements for the PhD, a program of courses appropriate to a student's background and interests may be developed.
All students in the Biostatistics PhD program hold a master's degree in Biostatistics or a related field and those applying for PhD study who do not already hold a masters degree are considered for admission to the Biostatistics MA. This practice does not prolong the time to the doctorate since the first two years of both the MA and PhD programs for students coming from the baccalaureate are identical.
Normative time to advancement: 2-3 years. Normative time in candidacy: 1-2 years. Total normative time: 3-5 years.
Time to Advancement
The qualifying examination.
The oral qualifying examination is scheduled for three hours. The primary purpose of the exam is to test both a candidate's general competence in the field of biostatistics and the ability to apply biostatistical methods to a broad research area. The exam is designed to measure the candidate's breadth of knowledge as well as provide a determination of the candidate's readiness to enter the research phase of study.
To assure the examining committee that the candidate has a firm grasp of both basic areas and a familiarity with current problems in the field, the exam is conducted as follows:
- The candidate is expected to begin with a 30 minute presentation of a proposed dissertation topic that includes a sound research strategy that the candidate can defend.
- Following this presentation, the candidate will be asked to demonstrate an ability to synthesize the methods and techniques learned through course work and to apply this knowledge to areas and problems suggested by the committee members. To achieve this goal, committee members are likely to ask questions that delve into subjects that go beyond the chosen area of dissertation research.
Students are encouraged to take the qualifying exam after they have identified a dissertation adviser and a research topic . In preparation for the exam, the candidate should meet with the chair of the qualifying examination committee to discuss the details of the structure of the exam and any other pertinent issues.
Time in Candidacy
Dissertation.
After completion of course work and the oral comprehensive examination, a doctoral student advances to candidacy for the PhD. Before this is possible, a student must have identified:
- A dissertation topic
- A dissertation adviser
- A committee
Advisers and committee members are particularly interested in working with candidates who have demonstrated an ability to perform original research, and doctoral students are encouraged to explore dissertation research topics early in the program. Topics for research are selected from biostatistics and statistics, public health, biology, computing and other areas (see dissertation topics of some recent graduates).
Contact Information
Graduate group in biostatistics.
2121 Berkeley Way West
Graduate Group Co-Chair
Mark van der Laan, PhD
Lexin Li, PhD
Biostatistics Division Chair
Alan Hubbard
Senior Program Manager
Lauren M Krupa
Print Options
When you print this page, you are actually printing everything within the tabs on the page you are on: this may include all the Related Courses and Faculty, in addition to the Requirements or Overview. If you just want to print information on specific tabs, you're better off downloading a PDF of the page, opening it, and then selecting the pages you really want to print.
The PDF will include all information unique to this page.
Master of Arts in Statistics Program Information
Fall semester.
STAT 201A: Introduction to Probability at an Advanced Level
Distributions in probability and statistics, central limit theorem, Poisson processes, modes of convergence, transformations involving random variables.
STAT 201B: Introduction to Statistics at an Advanced Level
Estimation, confidence intervals, hypothesis testing, linear models, large sample theory, categorical models, decision theory.
STAT 243: Introduction to Statistical Computing
Concepts in statistical programming and statistical computation, including programming principles, data and text manipulation, parallel processing, simulation, numerical linear algebra, and optimization.
Fall Semester Total Units: 12
Spring Semester
STAT 222: Masters of Statistics Capstone Project
The capstone project is part of the masters degree program in statistics. Students engage in professionally-oriented group research under the supervision of a research advisor. The research synthesizes the statistical, computational, economic, and social issues involved in solving complex real-world problems.
STAT 230A: Linear Models
Theory of least squares estimation, interval estimation, and tests under the general linear fixed effects model with normally distributed errors. Large sample theory for non-normal linear models. Two and higher way layouts, residual analysis. Effects of departures from the underlying assumptions. Robust alternatives to least squares.
Approved Elective
Spring Semester Total Units: 12
- Program Requirements
- Comprehensive Exam
- Thesis Option
- Third Semester Option
The program is for full-time students and is designed to be completed in two semesters (fall and spring). In order to obtain the M.A. in Statistics, admitted M.A. students must complete a minimum of 24 units of courses and pass a comprehensive examination.
In the first semester, all students will take intensive graduate courses in probability, theoretical statistics, and statistical computing; the typical courses are STAT 201A, 201B, and 243. In the second semester, students will take an advanced course in modern applied statistics (STAT 230), an elective, and a capstone course. The capstone will consist of a team-based learning experience that will give students the opportunity to work on a real-world problem and carry out a substantial data analysis project. It will culminate with a written report and an oral presentation of findings. The elective will depend on the student’s interests and will be decided in consultation with advisers. For a complete list of courses offered by the department and course descriptions, please visit the academic guide .
All coursework used for the M.A. must be letter graded unless the course is only offered on a satisfactory/unsatisfactory (S/U) basis. The total of S/U units may only be 2
Elective courses are chosen with the guidance and approval of the M.A. program Chair. Generally, the elective must be a graduate level course related to statistics. Such courses can be within the Statistics Department or from other departments. Some examples of popular past electives:
- STAT 248: Analysis of Time Series
- STAT 254: Modern Statistical Prediction and Machine Learning
- STAT 260: Topics in Probability and Statistics
If an elective that you would like to take is not on the list the course can be submitted for department approval. You can submit your request using the Google Form here . If you take an approved elective that is less than 4 units you will need to take another elective and/or approved seminar course to meet the 24 unit minimum requirement for the program. *Approved electives are subject to change.
In extremely rare cases, a thesis option may be considered by the M.A. Chair. Typically, this will be when either the option has been offered to the student at the time of admission, or if the student arrives with substantial progress in research in an area of interest to our faculty. If approved by the M.A. Chair for the thesis option you will not have to take the comprehensive exam.
If approved for the thesis option, you must find three faculty to be on your thesis committee. Though not required, it is strongly encouraged that one of the faculty be from outside the Statistics Department. Both you and the thesis committee chair must agree on the topic of your thesis. Please provide a short description of your thesis topic, the names of your committee members and the signature of your committee chair on the Worksheet for the M.A. in Statistics, Thesis Option . In addition, you will also need to complete Graduate Division’s Application for Candidacy for the Master's Degree (Plan 1 - Thesis)
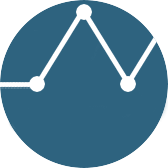
Professional Development
Prepare for a career in statistics with our Professional Development resources. Workshops, alumni insights, and individual advising sessions are available to help you succeed.
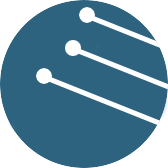
Summer Prep Program
Equip yourself for your M.A. Statistics journey with our intensive 2-week Summer Prep Program. Strengthen your mathematical foundations, explore statistical concepts, and dive into probability theory
Will I be able to take courses other than those that are required?
Course selection will be done in consultation with Statistics Department M.A. Chair and/or committee member. Some students do take additional courses, including courses in other departments, depending on their background and level of preparation. Other professional graduate programs on campus all have their own policies for enrollment in their courses. After appropriate consultation, students will need to check these policies before registering for such courses.
Can I transfer to the PhD program?
There is no transfer arrangement into the PhD program. To gain acceptance into the PhD program, you must apply along with all other applicants, and you will be considered in the same way as other applicants. Students should know that admission to the UC Berkeley Statistics PhD program is highly competitive.
How to Advance to Candidacy
All coursework for the M.A. must be completed by the end of the semester in which you intend to graduate. In order to advancement to candidacy, you need to complete the M.A. Statistics Comprehensive Exam and completed all the required MA coursework. For those approved by the M.A. Program Committee Chair, to apply for the M.A. thesis option, you will have to submit individual application for advancement that lists the proposed committee for the thesis through CalCentral.
If you already have a master's degree from any institution including UC Berkeley and are applying for advancement to candidacy for a master's degree in Statistics, you must:
- Provide a transcript (unofficial one)
Please submit your completed paperwork to the Master's Program Coordinator at 367 Evans Hall for review by the M.A. Program Committee. The department deadline to turn in paperwork is by the end of the third week of the semester in which you plan to graduate.
How do I add/drop classes or change my grading option between letter grading and satisfactory/unsatisfactory (SU)?
Each semester, graduate students have till the Friday of the third week of classes to add/drop courses on Cal Central. If you want to add/drop courses after the third week, you will have to complete a form called the Graduate Petition to Change Class Schedule . After completing the form, please submit it to the Master's Program Coordinator in 375 Evans so that it can be processed. The form can be given in person or put in the MA Program Coordinator's mailbox in 367 Evans. It must be submitted before the last day of classes in each semester, which occurs before the week of final exams.
Student Life
For information about graduate student life and housing options, please visit Resources & Services for Graduate Students and the Statistics Graduate Student Association .
International Students
What is the proportion of international students for this program.
We do not admit students based on national origin and we do not have fixed proportions of domestic and international students. The city of Berkeley and the Department of Statistics have always had a diverse and lively international community.
For questions regarding visas, employment of international students, or any other questions regarding temporary stay in the United States, please visit the Berkeley International Office Website or call them at (510) 642-2818 .
Is the M.A. Statistics Program a STEM OPT eligible program?
Yes, the M.A. Statistics program is eligible for the STEM OPT extension. The program is falls under CIP code "Statistics, General 27.0501" as of July 12, 2023.
Recommended texts
1. applied and theoretical statistics, categorical data.
- ‘‘Categorical Data Analysis’’ by Alan Agresti Well-written, go-to reference for all things involving categorical data.
Causal Inference
More information available through the causal inference reading group and online seminar

Communicating with Data
- ‘‘Communicating with Data The Art of Writing for Data Science’’ by Deborah Nolan and Sara Stoudt
Compositional Data
- ‘‘Compositional Data Analysis’’ by Pawlowsky-Glahn and Buccianti
Linear models
- ‘‘Generalized Linear Models’’ by McCullagh and Nelder Theoretical take on GLMs. Does not have a lot of concrete data examples.
- ‘‘Statistical Models’’ by David A. Freedman Berkeley classic!
- ‘‘Linear Models with R’’ by Julian Faraway Undergraduate-level textbook, has been used previously as a textbook for Stat 151A. Appropriate for beginners to R who would like to learn how to use linear models in practice. Does not cover GLMs.
Experimental Design
- ‘‘Design of Comparative Experiments’’ by Rosemary A Bailey Classic, approachable text, free for download here
Machine Learning (see also Probabilistic Modeling and Sampling)
- ‘‘The Elements of Statistical Learning’’ by Hastie, Tibshirani, and Friedman Comprehensive but superficial coverage of all modern machine learning techniques for handling data. Introduces PCA, EM algorithm, k-means/hierarchical clustering, boosting, classification and regression trees, random forest, neural networks, etc. …the list goes on. Download the book here .
- ‘‘Computer Age Statistical Inference: Algorithms, Evidence, and Data Science’’ by Hastie and Efron.
- ‘‘Pattern Recognition and Machine Learning’’ by Bishop
- ‘‘Bayesian Reasoning and Machine Learning’’ by Barber Available online .
- ‘‘Probabilistic Graphical Models’’ by Koller and Friedman
- ‘‘Deep Learning’’ by Goodfellow, Bengio and Courville
Multiple Testing, Post-Selection Inference and Selective Inference
- ‘‘Multiple Comparisons: theory and methods’’ by Jason Hsu One of many sources in this field of research. Most of the literature comes from research papers.
More information available through online seminar .
Probabilistic Modeling and Sampling (see also Machine Learning)
- ‘‘Monte Carlo Statistical Methods’’ by Robert and Casella A comprehensive text on sampling approaches.
- ‘‘Handbook of Approximate Bayesian Computation’’ by Sisson, Fan and Beaumont
- ‘‘Graphical Models, Exponential Families, and Variational Inference’’ by Wainwright and Jordan Assuming knowledge at the level of Stat 210AB, elucidates how exponential families can be used in large-scale and interpretable probabilistic modeling.
Theory and Foundations
- ‘‘Theoretical Statistics: Topics for a Core Course’’ by Keener The primary text for Stat 210A. Download from SpringerLink .
- ‘‘Theory of Point Estimation’’ by Lehmann and Casella A good reference for Stat 210A, covering estimation.
- ‘‘Testing Statistical Hypotheses’’ by Lehmann and Romano A more advanced reference for Stat 210A, convering testing and a litany of related concepts.
- ‘‘Empirical Processes in M-Estimation’’ by van de Geer
- Some students find this helpful to supplement the material in 210B.
- ‘‘Concentration Inequalities’’ by Boucheron, Lugosi, and Massart This is also useful to supplement 210B material.
2. Probability
Undergraduate level probability.
- ‘‘Probability’’ by Pitman What the majority of Berkeley undergraduates use to learn probability.
- ‘‘Introduction to Probability Theory’’ by Hoel, Port and Stone This text is more mathematically inclined than Pitman’s, and more concise, but not as good at teaching probabilistic thinking.
- ‘‘Probability and Computing’’ by Upfal and Mitzenmacher What students in EECS use to learn about randomized algorithms and applied probability.
Measure Theoretic Probability
- ‘‘Probability: Theory and Examples’’ by Durrett This is the standard text for learning measure theoretic probability. Its style of presentation can be confusing at times, but the aim is to present the material in a manner that emphasizes understanding rather than mathematical clarity. It has become the standard text in Stat 205A and Stat 205B for good reason. Online here .
- ‘‘Foundations of Modern Probability’’ by Olav Kallenberg This epic tome is the ultimate research level reference for fundamental probability. It starts from scratch, building up the appropriate measure theory and then going through all the material found in 205A and 205B before powering on through to stochastic calculus and a variety of other specialized topics. The author put much effort into making every proof as concise as possible, and thus the reader must put in a similar amount of effort to understand the proofs. This might sound daunting, but the rewards are great. This book has sometimes been used as the text for 205A.
- ‘‘Probability and Measure’’ by Billingsley This text is often a useful supplement for students taking 205 who have not previously done measure theory. Download here .
- ‘‘Probability with Martingales’’ by David Williams This delightful and entertaining book is the fastest way to learn measure theoretic probability, but far from the most thorough. A great way to learn the essentials.
Stochastic Calculus
Stochastic Calculus is an advanced topic that interested students can learn by themselves or in a reading group. There are three classic texts:
- ‘‘Continuous Martingales and Brownian Motion’’ by Revuz and Yor
- ‘‘Diffusions, Markov Processes and Martingales (Volumes 1 and 2)’’ by Rogers and Williams
- ‘‘Brownian Motion and Stochastic Calculus’’ by Karatzas and Shreve
Random Walk and Markov Chains
These are indispensable tools of probability. Some nice references are
- ‘‘Markov Chain and Mixing Times’’ by Levin, Peres and Wilmer. Online here .
- ‘‘Markov Chains’’ by Norris Starting with elementary examples, this book gives very good hints on how to think about Markov Chains.
- ‘‘Continuous time Markov Processes’’ by Liggett A theoretical perspective on this important topic in stochastic processes. The text uses Brownian motion as the motivating example.
3. Mathematics
Convex optimization.
- ‘‘Convex Optimization’’ by Boyd and Vandenberghe. Download the book here
- ‘‘Introductory Lectures on Convex Optimization’’ by Nesterov.
Linear Algebra
- ‘‘The Matrix Cookbook’’ by Petersen and Pedersen: ‘‘Matrix identities, relations and approximations. A desktop reference for quick overview of mathematics of matrices.’’ Download here .
- ‘‘Matrix Analysis’’ and ‘‘Topics in Matrix Analysis’’ by Horn and Johnson Second book is more advanced than the first. Everything you need to know about matrix analysis.
Convex Analysis
- ‘‘A course in Convexity’’ by Barvinok. A great book for self study and reference. It starts with the basis of convex analysis, then moves on to duality, Krein-Millman theorem, duality, concentration of measure, ellipsoid method and ends with Minkowski bodies, lattices and integer programming. Fairly theoretical and has many fun exercises.
Measure Theory
- ‘‘Real Analysis and Probability’’ by Dudley Very comprehensive.
- ‘‘Probability and Measure Theory’’ by Ash Nice and easy to digest. Good as companion for 205A
Combinatorics
- ‘‘Enumerative Combinatorics Vol I and II’’ by Richard Stanley. There’s also a course on combinatorics this semester in the math department called Math249: Algebraic Combinatorics. Despite the scary “algebraic” prefix it’s really fun. Download here .
4. Computational Biology
‘big picture’ overview.
- ‘‘Modern Statistics for Modern Biology’’ by Susan Holmes and Wolfgang Huber Accessible ‘data analysis’-focused overview of the field, with numerous motivating examples and plentiful opportunities for hands-on practice. Although written for biologists, can indirectly help with developing an understanding of how to identify problems that impact on biology.
Bioinformatics
- ‘‘Statistical Methods in Bioinformatics’’ by Ewens and Grant Great overview of sequencing technology for the unacquainted.
- ‘‘Computational Genome Analysis: An Introduction’’ by Deonier, Tavaré, and Waterman Great R code examples from computational biology. Discusses the basics, such as the greedy algorithm, etc.
Population Genetics
- ‘‘Probability Models for DNA Sequence Evolution’’ by Rick Durrett
- ‘‘Mathematical Population Genetics’’ by Warren Ewens
5. Computer Science
Numerical analysis.
- ‘‘Numerical Analysis’’ by Burden and Faires This book is a good overview of numerical computation methods for everything you’d need to know about implementing most computational methods you’ll run into in statistics. It is filled with pseudo-code but does use Maple as it’s exemplary language sometimes. It has been a great resource for the Computational Statistics courses (243/244). Depending on what happens with this course, this may be a good place to look when you’re lost in computation.
- ‘‘Introduction to Algorithms’’, Third Edition, by Cormen, Leiserson, Rivest, and Stein. MIT OpenCourseWare 6.046J / 18.410J ‘‘Introduction to Algorithms’’ (SMA 5503) was taught by one of the authors, Prof. Charles Leiserson, in 2005. This is an undergraduate course and this book was used as the textbook
- ‘‘Algorithm Design’’, by Jon Kleinberg and Éva Tardos.
Biostatistics MA
Many issues in the health, medical and biological sciences are addressed by collecting and exploring relevant data. The development and application of techniques to better understand this data is the fundamental concern of our program. We offer training in statistics and biostatistics theory, computer implementation of analytic methods, and opportunities to use this knowledge in areas of biological and medical research.
Berkeley Public Health and UC Berkeley’s Department of Statistics, together with other UC Berkeley departments, offer a broad set of opportunities to satisfy the needs of individual students. In addition, the involvement of faculty from UCSF’s Department of Biostatistics and Epidemiology enriches our instructional and research activities.
Our master’s program is a two-year program consisting of 48 units with courses selected from biostatistics and statistics, public health, and biology.
The oral comprehensive examination is designed to test a candidate’s breadth of understanding and knowledge, as well as the ability to articulate and explain the basic concepts gained from the curriculum. Alternatively, a thesis may be submitted to fulfill requirements. However, the decision to submit a thesis rather than take the oral examination must be made early in the final semester of the program.
Students should take the following courses:
- STAT 201A: Introduction to Probability at an Advanced Level
- STAT 201B: Introduction to Statistics at an Advanced Level
- PH C240A: Introduction to Modern Biostatistical Theory and Practice
In addition to Statistics 201A and 201B and PH C240A, students are expected to take PH252D (Introduction to Causal Inference) and at least two other courses from the following list:
- PH C240B: Biostatistical Methods: Survival Analysis and Causality
- PH 240C: Computational Statistics
- PH 252E: Advanced Topics in Causal Inference
- PH 244: Big Data: A Public Health Perspective
- CS 294.150: Machine Learning and Statistics Meet Biology
- PH C242C: Longitudinal Data Analysis
- PH 290.X: Targeted Learning in Biomedical Big Data
Qualifications
Previous coursework in calculus, linear algebra, and statistics is strongly recommended.
Common undergraduate majors for admitted applicants: Biomedical & biological sciences, mathematics, statistics. Common work experience for admitted applicants: Typical successful applicants have work experience in Research Assistant positions at a health department.
GRE Exemption Criteria
GRE General Test scores are required for admission to the Biostatistics MA program however applicants are exempted from the requirement if they meet all of the following criteria:
- Completed two semesters of calculus for a letter grade and earned a grade of “B” or higher.
- Completed one semester of linear algebra for a letter grade and earned a grade of “B” or higher.
- Completed one semester of statistics for a letter grade and earned a grade of “B” or higher.
- Cumulative undergraduate GPA of 3.0 or higher.
- Overall quantitative/math GPA of 3.0 or higher.
- For international students: TOEFL score of 100 or higher OR IELTS score of 7.0 or higher
Berkeley Public Health also exempts applicants who already hold a doctoral level degree from the GRE requirement.You can find more information on the application instructions page . There is a program page in the Berkeley Graduate Application where you can indicate you meet the criteria for GRE exemption. Applicants who are exempted from the GRE are not at a disadvantage in the application review process.
Some students pursuing the MA degree intend to continue directly into a PhD program, while others take research positions in tech companies, federal agencies, state and local health departments, health care delivery organizations, and private industry. MA students interested in continuing into the UC Berkeley Biostatistics doctoral program immediately following their MA degree should apply to the new degree program through the Online Application for Admission during their second year of study during the normal admissions cycle.
Funding and Fee Remission
Prospective students who are US citizens or permanent residents can find more information about applying for an application fee waiver for the Berkeley Graduate Application. Fees will be waived based on financial need or participation in selected programs described on the linked website. International applicants (non-US citizens or Permanent Residents) are not eligible for application fee waivers.
Some MA and MA/PhD admitted students are made a funding offer as a part of their admission package. These offers depend on funding availability and the applicant pool for that year.
Tuition and fees change each academic year. To view the current tuition and fees, see the fee schedule on the Office of the Registrar website (in the Graduate: Academic section).
Please contact [email protected] if you have any questions about funding opportunities for the biostatistics programs.
Diversity, Equity and Inclusion
The Division of Biostatistics is committed to challenging systemic inequities in the areas of health, medical, and biological sciences, and to advancing the goals of diversity, equity, and inclusivity in Biostatistics and related fields.
Diversity, Equity and Inclusion in Biostatistics
Admissions Statistics
Clinical faculty, emeritus faculty, faculty associated in biostatistics graduate group.
- Peter Bickel PhD Statistics
- David R. Brillinger PhD Statistics
- Perry de Valpine PhD Environmental Science, Policy, and Management
- Haiyan Huang PhD Statistics
- Michael J. Klass PhD Statistics
- Priya Moorjani PhD Molecular & Cell Biology
- Rasmus Nielsen PhD Integrative Biology and Statistics
- Elizabeth Purdom PhD Statistics
- Sophia Rabe-Hesketh PhD Education
- John Rice PhD Statistics
- Yun S. Song PhD Statistics; Electrical Engineering and Computer Sciences
- Bin Yu PhD Statistics
Jump to navigation

- Course Catalog
- Class Schedule
- Undergraduate
2024 Fall ENGLISH 190 001 SEM 001
Statistics PhD
Statistics ma.
- Request Information
- Current Students
- Faculty and Staff
- Scholarships and Awards
- Student News
- Dean’s List
- Doctor of Philosophy (PhD)
- Master of Science (MS)
- Co-Major PhD
- Graduate Admissions FAQ
- Administrative Faculty
- Graduate Students
- Affiliate Faculty
- Emeritus/a Faculty
- Mission Statement
- DEI Information
Spring 2024 Graduate Research Awards from the Department of Statistics
Iowa State University has announced the recipients for the Spring 2024 Graduate Research Excellence Awards. Among these distinguished scholars stand three individuals from the Department of Statistics, whose outstanding research contributions have garnered well-deserved recognition.
Eva Biswas (Nominator: Somak Dutta)
Nomination statement: Eva Biswas has demonstrated outstanding research achievements. She has made remarkable contributions to theory and methods for time series, functional data, Markov random fields, directional statistics, and applications of statistical methods in plant science. She has two published papers, two under major revision, and is working on finishing four more.
Yonghyun Kwon (Nominator: Jae-Kwang Kim)
Nomination statement: Yonghyun has recently completed his PhD. His academic portfolio includes four papers that have been published or accepted in peer-reviewed journals, along with one paper currently under review by the Journal of the American Statistical Association (JASA).
Yunhui Qi (Nominator: Peng Liu)
Nomination statement: Yunhui Qi is a dedicated researcher in statistics and a great collaborator with biologists. She has a genuine interest in research and has made significant advancements in several challenging problems in multi-omics data analysis.
Eligible students are those enrolled at Iowa State University and nearing completion of their thesis or dissertation. Candidates embody excellence in research, academic prowess, and a passion for scholarly exploration, showcasing a profound understanding of their field. With strong analytical skills and a commitment to collaboration, they tackle complex research questions with creativity and rigor. Each recipient receives a commendation letter from the Iowa State President, a certificate of achievement, an honor cord for commencement, and an official notation on their transcript.
Join us in congratulating these exemplary scholars from the Department of Statistics for their achievements and contributions to statistical research.
- Equity & Inclusion
Francisca Vasconcelos chosen as Paul and Daisy Soros New American fellow
Francisca Vasconcelos, a doctoral student in UC Berkeley’s Department of Electrical Engineering and Computer Sciences, has been chosen as a Paul and Daisy Soros fellow.
She will receive up to $90,000 to support her graduate education as part of the Paul and Daisy Soros New American Fellowship, a merit-based program for immigrants and children of immigrants. Vasconcelos, whose parents immigrated from Portugal, is the first Soros fellow with Portuguese heritage.
“This is an incredible, diverse community of immigrants and first-generation Americans that I am excited to interact with and learn from. I am also very grateful for the fellowship funding,” Vasconcelos said. “As the first Portuguese recipient of this fellowship, I am proud to represent Portugal in this international community.”
Vasconcelos is one of two Berkeley students included in the 30-person 2024 cohort from 2,323 applicants. The program has spent more than $80 million since 1998 supporting 805 fellows from 103 countries pursuing U.S. graduate degrees in fields from medicine to law. The fellowship is currently accepting applications to join its 2025 fellows.
Vasconcelos was born in Boston, Mass., and grew up in San Diego, Calif. She graduated from the Massachusetts Institute of Technology in 2020 with a bachelor’s of science in electrical engineering, computer science and physics. She then received the Rhodes Scholarship, allowing her to earn master’s degrees in statistical sciences and the philosophy of physics at the University of Oxford.
After graduating from MIT, Vasconcelos also served as the founding academic director and a lead instructor of the Coding School’s Qubit by Qubit initiative. In that role, she helped teach thousands of students quantum computing fundamentals.
At Berkeley, Vasconcelos is a member of the Berkeley Artificial Intelligence Research Lab and computer science theory group and is advised by EECS professors Michael Jordan and Umesh Vazirani. She studies the intersections of quantum computation and machine learning in order to find impactful ways quantum computers can outperform classical computers.
“Quantum computers have the potential to revolutionize our ability to solve many computational problems of societal import,” said Vasconcelos. “I hope to contribute to that revolution."

IMAGES
VIDEO
COMMENTS
The Statistics PhD program is rigorous, yet welcoming to students with interdisciplinary interests and different levels of preparation. Students in the PhD program take core courses on the theory and application of probability and statistics during their first year. The second year typically includes additional course work and a transition to ...
Statistics at UC Berkeley. We are a community engaged in research and education in probability and statistics. In addition to developing fundamental theory and methodology, we are actively involved in statistical problems that arise in such diverse fields as molecular biology, geophysics, astronomy, AIDS research, neurophysiology, sociology, political science, education, demography, and the U ...
The Statistics MA program prepares students for careers that require statistical skills. It focuses on tackling statistical challenges encountered by industry rather than preparing for a PhD. The program is for full-time students and is designed to be completed in two semesters (fall and spring).
A PhD degree in Biostatistics requires a program of courses selected from biostatistics, statistics, and at least one other subject area (such as environmental health, epidemiology, or genomics), an oral qualifying examination, and a dissertation. Courses cover traditional topics as well as recent advances in biostatistics and statistics.
The Statistical Computing Facility (SCF) is a unit of the Department of Statistics. Its mission is to provide the undergraduate students, graduate students, postdocs, and faculty in the Statistics Department at Berkeley with state-of-the-art computing resources, services, and technical knowledge, supporting them in carrying out cutting-edge ...
Philip B. Stark | Distinguished Professor of Statistics | University of California Berkeley, CA 94720-3860 | stark [at] stat.berkeley.edu | 510-394-5077 | @philipbstark . My research centers on inference problems and uncertainty quantification, especially confidence procedures tailored for specific goals. I've published on causal inference, the U.S. Census, climate, clinical trials, cosmology ...
Course content. This is an introductory Ph.D.-level course in theoretical statistics. It is a fast-paced and demanding course intended to prepare students for research careers in statistics. Statistics is the study of methods that use data to understand the world. Statistical methods are used throughout the natural and social sciences, in ...
Students enrolled in the UC Berkeley Biostatistics doctoral (PhD) program are eligible to apply for interdisciplinary study in a Designated Emphasis (DE), which we refer to as the Associated Programs. At UC Berkeley, acquiring a DE is like earning a "minor" with a PhD degree. Applications for Designated Emphasis are reviewed on a rolling basis ...
The Biostatistics Division has participated in the graduate diversity admissions fair at University of California, Berkeley since 2020. In 2021, we are partnering with the Department of Statistics to launch Zoom panels to introduce the program to undergraduate students who belong to groups historically underrepresented in the graduate programs ...
The program is for full-time students and is designed to be completed in two semesters (fall and spring). In order to obtain the M.A. in Statistics, admitted M.A. students must complete a minimum of 24 units of courses and pass a comprehensive examination. In the first semester, all students will take intensive graduate courses in probability ...
Doctor of Philosophy (PhD) All Biostatistics PhD students are required to hold a master's degree in Biostatistics or a related field. The PhD degree requires 4-6 semesters of course work in biostatistics, statistics, and at least one other subject area (e.g., biology, environmental health, epidemiology). There are no unit or course requirements ...
About. The Department of Statistics at University of California, Berkeley offers the Master of Arts (MA) and Doctor of Philosophy (PhD) degrees. University of California, Berkeley. Berkeley , California , United States. Top 0.1% worldwide. Studyportals University Meta Ranking. 4.5 Read 47 reviews.
April 8, 2024. UC Berkeley graduate programs are again among the best in the nation, topping several categories, according to the 2024 rankings by U.S. News and World Report released late on Monday, April 8 . In all, some 30 Berkeley graduate programs ranked in the Top 10 in the country. Every year, U.S. News ranks professional school programs ...
UC Berkeley Statistics Graduate Student Association. Recommended texts 1. Applied and Theoretical Statistics Categorical Data ''Categorical Data Analysis'' by Alan Agresti Well-written, go-to reference for all things involving categorical data. Causal Inference
Curriculum. Our master's program is a two-year program consisting of 48 units with courses selected from biostatistics and statistics, public health, and biology. The oral comprehensive examination is designed to test a candidate's breadth of understanding and knowledge, as well as the ability to articulate and explain the basic concepts ...
On Tuesday, April 9, eight Berkeley graduate students selected as semi-finalists competed in the annual Grad Slam competition. Each student presented their fascinating research in a manner accessible to the general public in a three-minute "mini TED talk" - offering more than 300 virtual attendees from across the globe a truly educational experience.
Course Catalog. Class Schedule; Course Catalog; Undergraduate; Graduate; Copyright © 2014-24, UC Regents; all rights reserved.
Beyond Academia is a non-profit founded and run by UC Berkeley graduate students, dedicated to empowering PhDs to expand their career options beyond the professoriate. This year, Beyond Academia hosted its 12th annual conference virtually on Thursday, February 22 and Friday, February 23, 2024, drawing over 2,100 registrants from more than 50 countries.
2024 Fall ENGLISH 190 001 SEM 001 | Course Catalog. Class Schedule. Course Catalog. Undergraduate.
The Graduate Division serves more than 13,000 students in over 100 graduate degree programs. We are here to help you from the time you are admitted until you complete your graduate program. ... Working toward obtaining your graduate degree at Berkeley is an exciting and challenging endeavor, but funding your graduate education shouldn't be ...
April 16, 2024. Iowa State University has announced the recipients for the Spring 2024 Graduate Research Excellence Awards. Among these distinguished scholars stand three individuals from the Department of Statistics, whose outstanding research contributions have garnered well-deserved recognition. Eva Biswas (Nominator: Somak Dutta)
Francisca Vasconcelos, a doctoral student in UC Berkeley's Department of Electrical Engineering and Computer Sciences, has been chosen as a Paul and Daisy Soros fellow. She will receive up to $90,000 to support her graduate education as part of the Paul and Daisy Soros New American Fellowship, a merit-based program for immigrants and children of immigrants.