Starbucks_CapstoneProject
Capstone project for the udacity data scientist nanodegree, starbucks capstone challenge, introduction.
Starbucks has provided a dataset that emulates the behavior of customers using the Starbucks rewards mobile app. The Starbucks app provides a way to advertise and share offers with the customers. Customers can also use it to pay at the stores. Starbucks sends different types of advertisement and offers once every few days to their customers. A customer might get one of the following:
- Informational offer (i.e., mere advertisement)
- Discount offer
- Buy one get one free (BOGO) offer
Discount and BOGO offers have a challenge, that is, the customer must make a minimum purchase before it can redeem the offer. Additionally, each offer has an expiration date. In the case of the informational offers, the expiration date is when the customer stop feeling the influence of the advertisement.
The data provided includes the demographics of each customer, the app activity of customers for a period of 30 days. It includes the timestamps of when a costumer received, viewed and completed an offer, when a purchase was done, and the amount of money spent.

Problem Statement
The goal of sending advertisement and offers to customers is to increase the customer purchases. However, it would be naive to send all offers to all customers at the same time. The goal of the project is to take advantage of the transactions and demographics data to determine the offers that should be targeted to different groups of customers.
The following data files have been provided and included in the project’s repo
- portfolio.json contains the details of each offer: duration, reward, type, etc
- profile.json contains demographic information of customer
- transcript.json contains all customers activity: transactions, offers received, offers viewed, and offers completed.
The datasets were cleaned and merged in a way that each row includes customers activity, customers demographics and offers metadata.
Data Analysis
From the datasets, we first note that the simulated data was obtained for a period of 29.75 days, with 306,534 events. Each event represents a transaction, an offer received, an offer viewed, or an offer completed.

The figure shows the distribution of the population based on gender, income and age. We note that around 8,500 customers are male, 6,100 are female and around 200 belong to other gender. In the case of the income, we observe that curve roughly approaches a normal curve with a mean around 65,000 and a standard deviation of 21,000. Finally, the age also seems to approach a normal distribution. It is truncated on the left side of the curve due to the fact that a customer must be 18 years or older to be part of the Starbucks program. The mean of the age is 54 years and the standard deviation is 17.

Now, we observe that the average expense of a single transaction follows a bimodal distribution. The first lobe is centered around $2.5 and the second one around $18. In the case of the distribution of the total expense done by a customer the values seems to decrease exponentially.

When separating the data based on gender, we note that the women make average purchases of $17.5, others of $15 and men of $12. However, we also note that the total amount of money spent by men and women is similar (~85,000) while others spend a fraction of that. This is distributions are directly affected by the number of members of each gender group.

In the case of the average transaction and the total expense, we see that the values increase as the income of the customer increases, which is expected. People that make less than $30,000 have an average transaction of $6 while people making more than $90,000 have transactions of more than $25. In the case of the total expense, the values go from $60 to $180 in the same range of income.

As in the case of income, the spending behavior is similar with age. As the value of age increases so do the average transaction and the total expense values. The average transaction value goes from a value of $8 for people under 20 to a value of $17 for people above
- Similarly, the total expense goes from $80 in a month to almost $140.

The figure above shows the distribution of offers received by customers. We note that each 30,000 discount and bogo offers have been received, and 15,000 informational ones. The latter offer is half of the others since there are only two informational offer and there are four discount and four bogo. Moreover, we note that each offer has been received by around 7,600 customers. This detail is important since the simulated data does not have any bias towards an specific offer, each customer has the same changes of receiving any offer.
Offer Recommendation
Based on the data we have observed, we noticed that different customer groups have different spending habits, that might be influenced by the fact that they received an offer or not. For instance, the correlation coefficient of 0.52 suggest that there is a moderate correlation between the net expense (i.e., total expense - reward received ) and the whether a customer completed an offer or not. Similarly, the correlation with the completion of bogo and discount offers is 0.39 and 0.40 , respectively. Finally, we observe a moderate correlation of 0.38 with the income of the customers.
These correlation indicate that offers should be targeted to customers based on their income or their transaction behavior. To take this into account, a knowledge-based recommendation system was implemented.
Simple System
Initially, we consider a simple system that recommends an offer that has been completed by a group of customers with the highest median of the net expense value. This system assumes a dependency between offer completion and net expense, and aims at maximizing the net expense.
In order to use significant samples, we impose the following conditions:
- Customers with positive net expense.
- Customers with at least 5 transactions completed.
- Only use customers that viewed and completed the offer.
The first condition is due to the fact that some customers received more rewards than the amount of money they spent. This is possible due to the fact that (a) a customer might have multiple offers active at the same time, and (b) a transaction might help multiple offers to reach their goal.
The second condition is necessary so we consider customers engaged with the offer/reward system. Finally, the third condition also targets at considering customer engaged with the system. There are a few customers that completed offers without knowing that they received an offer.
As an example of this simple recommendation system, we call the program and it provides the following list of offers sorted in the order of recommendation.
The output show us that the offer associated to customers with the highest median net expense is B1 , followed by D1 and B2 , the least recommended offer was I2 . Note that the difference in the net expense between the best and worst offers (e.g., B1 and I2 , respectively) is around $55 . We also compared these median values to the customers that do not meet the conditions to be considered part of our recommendation system.
This value is significantly lower to the ones of customers engaged with the offer system.
System with Demographics Consideration
As it is stated before, the net expense variable is also correlated to the income of the customers. So it would make sense to add some filtering to the recommendation system so that it benefits from this data and helps us target more specific users.

The figure shows the average expense of the customers that received, viewed and completed the B2 offer. The received-offer plots consider the customers that received the offer but did not view it. The viewed-offer plots represent the customers that viewed the offer but did not complete it. Finally, the completed-offer plots are for the customers that view and completed the offer.
We note that customers that complete the offer spend significantly more than those that do not. However, for customers that make more than $80,000, the viewed-offer customers spend more in each transaction average than the ones that completed the offer. This might be an indication that a different offer might be a better fit for them.
To incorporate such information to our recommendation system, we add filters that help filter the dataset used in the offer ranking. The filters limit the population based on age, income and gender. For instance, let’s consider two customers that make $95,000 and $100,000, respectively. As we discussed before, the population that makes more than $80,000 might have a different top offer recommended.
The system with filters picks B3 for the customer that makes $95,000 and D2 for the one with $100,000. Let’s take a look a the population net expense plots for the first customer.

Here we note that for an income of $90,000 and $105,000 the average transaction value for completed B3 offers is greater than for viewed offers. In the case of the net expense, the values for completed offers are always greater than the ones for viewed offers.
Finally, in our population analysis, we noted that women make transactions of higher value when compared to men and other genders. Let’s see if that is reflected in our recommendation system.
We note that for men, the offer recommended is the same as the one picked by the simple recommendation system. This might be due to the fact that the total customer population has men as a majority.

In the case of female, the selection changes and it favors D1 , which clearly has higher values of net expense and average transactions. Moreover, when compared with the selection of the simple system, there is a difference of $3.5 in the median net expense.

In the case of other gender, the difference between the simple recommendation system and the one with filters is even higher. We note a difference of $24 between the offers picked by the two systems.
Formal evaluation
In order to formally evaluate these methods, we should pick a control and test groups with real users. The two recommendation systems can be used together. That is, the simple systems should be use for customers that do not provide their personal information, while the one with filters can be used for customers that do.
In this experiment, the control group would be subject to a random distribution of offers in which each user has the same odds of receiving the offer. This distribution mimics the same used to generate the simulated data provided for this analysis. The control group would use the two recommendation systems provided in this project.
For evaluation metrics, we can measure the user engagement by keeping track of the ratio between received offers and viewed-and-completed offers. Also, it would be important to keep track of values that reflect the customer purchase habits such as the net expense, the total number of transactions and the average transaction value. If these recommendation systems are successful we should see a significant improvement in customer/offer engagement as well as an increase in the purchase behavior of the customer.
Conclusions
This project provided a way to implement a real recommendation system from scratch. The process was engaging since it required the implementation of all the steps of the data science methodology. It was interesting as well as difficult to decide what kind of data to use for the analysis. The project could have taken different directions if other aspects of the data were taken into account. For instance, instead of using the net expense and the average transaction value, we could have used the frequency at which offers were completed, or what was the time that customer took to completed an offer after viewing it. This was possible thanks to the timestamps provided in the datasets.
Also, other recommendation systems could have been explored. Modeling the data could have been another choice to recommend offers. This was not picked since the simulated data made a lot of simplifications in comparison to the real app. The mathematical model would have needed considerable adjustments in order to be used in a production systems. Picking metrics and methods than can be used in both the simulated system and the production system was considered in the design.
To improve the recommendation system, we could include other metrics. For instance, the frequency at which offers are completed would add an interesting dimension to the system. Also, our system does not take into account the number of valid offers a customer has a given time. As we noted in our analysis, some customers took advantage of this to maximize the reward received at the least possible expense. To prevent this from happening, the company could limit the number of offers a customer receives, or if the customer has multiple offers, a purchase only helps the completion of a single offer.
![starbucks capstone project udacity github [photo]](https://just-ed.github.io/photo.png)
Edgar Tomeyan
Ruby on rails developer.
Web developer with abilities to design, implement, deploy, test and debug Ruby on Rails applications.
- Phone: +79773030311
- Email: [email protected]
- GitHub: github.com/just-ed
- Ruby, Ruby on Rails
- Git, GitHub
- Heroku, VPS
- Russian - Proficiency
- English - Upper-Intermediate
- Armenian - Beginner
- Self-development
- Playing guitar
- Android ROMs
- Phone: +90 (212) 875 19 08
- E-Mail: [email protected]

- Company Profile
- Company Policy
- Mission and Vision
- Certificates
- Aluminium Windows
- Aluminium Doors
- Aluminium Sliding Elements
- Aluminium Curtain Walls
- Aluminium Skylight Elements
- Aluminium Frames for Safety and Security
- Aluminium Conservatories
- Metal Panel Sheet Claddings
- Aluminium Entrance Frames
- Glass Structures
- Complementary Items
- Lightweight Steel Structures
- Human Resources OPEN

Project Description
Project name:, year of construction:, title area:, contractor:.



For the first time Rosatom Fuel Division supplied fresh nuclear fuel to the world’s only floating nuclear cogeneration plant in the Arctic
The fuel was supplied to the northernmost town of Russia along the Northern Sea Route.
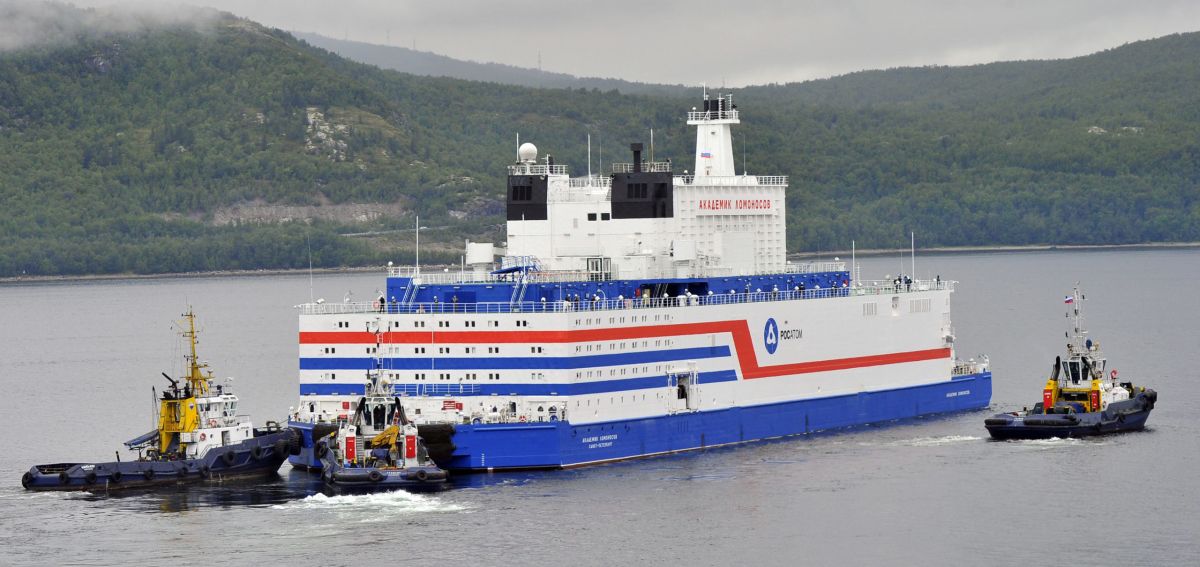
The first in the history of the power plant refueling, that is, the replacement of spent nuclear fuel with fresh one, is planned to begin before 2024. The manufacturer of nuclear fuel for all Russian nuclear icebreakers, as well as the Akademik Lomonosov FNPP, is Machinery Manufacturing Plant, Joint-Stock Company (MSZ JSC), a company of Rosatom Fuel Company TVEL that is based in Elektrostal, Moscow Region.
The FNPP includes two KLT-40S reactors of the icebreaking type. Unlike convenient ground-based large reactors (that require partial replacement of fuel rods once every 12-18 months), in the case of these reactors, the refueling takes place once every few years and includes unloading of the entire reactor core and loading of fresh fuel into the reactor.
The cores of KLT-40 reactors of the Akademik Lomonosov floating power unit have a number of advantages compared to the reference ones: a cassette core was used for the first time in the history of the unit, which made it possible to increase the fuel energy resource to 3-3.5 years between refuelings, and also reduce the fuel component of the electricity cost by one and a half times. The FNPP operating experience formed the basis for the designs of reactors for nuclear icebreakers of the newest series 22220. Three such icebreakers have been launched by now.
For the first time the power units of the Akademik Lomonosov floating nuclear power plant were connected to the grid in December 2019, and put into commercial operation in May 2020. The supply of nuclear fuel from Elektrostal to Pevek and its loading into the second reactor is planned for 2024. The total power of the Akademik Lomonosov FNPP, supplied to the coastal grid of Pevek without thermal energy consumption on shore, is about 76 MW, being about 44 MW in the maximum thermal power supply mode. The FNPP generated 194 million kWh according to the results of 2023. The population of Pevek is just a little more than 4 thousand, while the FNPP has a potential for supplying electricity to a city with a population of up to 100 thousand people. After the FNPP commissioning two goals were achieved. These include first of all the replacement of the retiring capacities of the Bilibino NPP, which has been operating since 1974, as well as the Chaunskaya TPP, which has already been operating for more than 70 years. Secondly, energy is supplied to the main mining companies in western Chukotka in the Chaun-Bilibino energy hub a large ore and metal cluster, including gold mining companies and projects related to the development of the Baimsk ore zone. In September 2023, a 110 kilovolt power transmission line with a length of 490 kilometers was put into operation, connecting the towns of Pevek and Bilibino. The line increased the reliability of energy supply from the FNPP to both Bilibino consumers and mining companies, the largest of which is the Baimsky GOK. The comprehensive development of the Russian Arctic is a national strategic priority. To increase the NSR traffic is of paramount importance for accomplishment of the tasks set in the field of cargo shipping. This logistics corridor is being developed due regular freight voyages, construction of new nuclear-powered icebreakers and modernization of the relevant infrastructure. Rosatom companies are actively involved in this work. Rosatom Fuel Company TVEL (Rosatom Fuel Division) includes companies fabricating nuclear fuel, converting and enriching uranium, manufacturing gas centrifuges, conducting researches and producing designs. As the only nuclear fuel supplier to Russian NPPs, TVEL supplies fuel for a total of 75 power reactors in 15 countries, for research reactors in nine countries, as well as for propulsion reactors of the Russian nuclear fleet. Every sixth power reactor in the world runs on TVEL fuel. Rosatom Fuel Division is the world’s largest producer of enriched uranium and the leader on the global stable isotope market. The Fuel Division is actively developing new businesses in chemistry, metallurgy, energy storage technologies, 3D printing, digital products, and decommissioning of nuclear facilities. TVEL also includes Rosatom integrators for additive technologies and electricity storage systems. Rosenergoatom, Joint-Stock Company is part of Rosatom Electric Power Division and one of the largest companies in the industry acting as an operator of nuclear power plants. It includes, as its branches, 11 operating NPPs, including the FNPP, the Scientific and Technical Center for Emergency Operations at NPPs, Design and Engineering as well as Technological companies. In total, 37 power units with a total installed capacity of over 29.5 GW are in operation at 11 nuclear power plants in Russia. Machinery Manufacturing Plant, Joint-Stock Company (MSZ JSC, Elektrostal) is one of the world’s largest manufacturers of fuel for nuclear power plants. The company produces fuel assemblies for VVER-440, VVER-1000, RBMK-1000, BN-600,800, VK-50, EGP-6; powders and fuel pellets intended for supply to foreign customers. It also produces nuclear fuel for research reactors. The plant belongs to the TVEL Fuel Company of Rosatom.
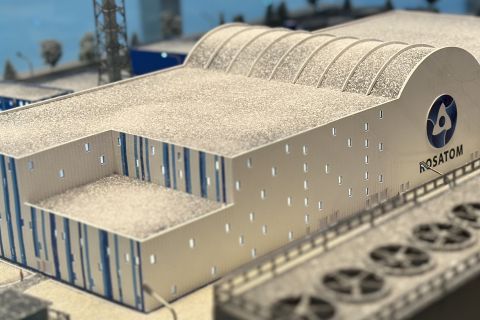
Rosatom obtained a license for the first land-based SMR in Russia
On April 21, Rosenergoatom obtained a license issued by Rostekhnadzor to construct the Yakutsk land-based SMR in the Ust-Yansky District of the Republic of Sakha (Yakutia).
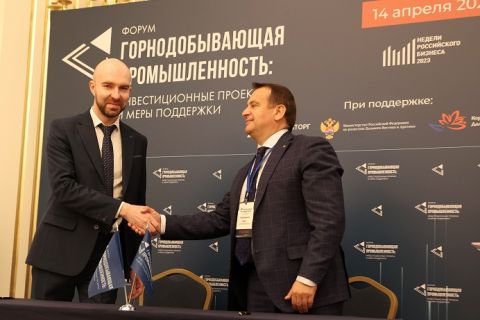
ROSATOM and FEDC agree to cooperate in the construction of Russia's first onshore SNPP
ROSATOM and FEDC have signed a cooperation agreement to build Russia's first onshore SNPP in Yakutia.
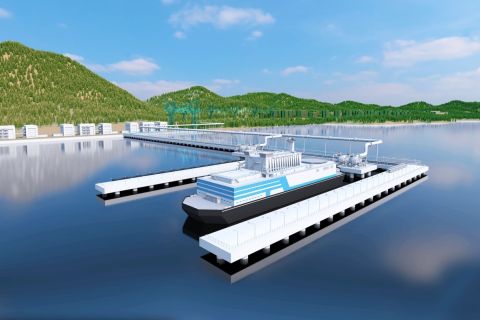
Rosatom develops nuclear fuel for modernized floating power units
Rosatom has completed the development of nuclear fuel for the RITM-200S small modular reactor designed for the upgraded floating power units.
- Popular Professionals
- Design & Planning
- Construction & Renovation
- Finishes & Fixtures
- Landscaping & Outdoor
- Systems & Appliances
- Interior Designers & Decorators
- Architects & Building Designers
- Design-Build Firms
- Kitchen & Bathroom Designers
- General Contractors
- Kitchen & Bathroom Remodelers
- Home Builders
- Roofing & Gutters
- Cabinets & Cabinetry
- Tile & Stone
- Hardwood Flooring Dealers
- Landscape Contractors
- Landscape Architects & Landscape Designers
- Home Stagers
- Swimming Pool Builders
- Lighting Designers and Suppliers
- 3D Rendering
- Sustainable Design
- Basement Design
- Architectural Design
- Universal Design
- Energy-Efficient Homes
- Multigenerational Homes
- House Plans
- Home Remodeling
- Home Additions
- Green Building
- Garage Building
- New Home Construction
- Basement Remodeling
- Stair & Railing Contractors
- Cabinetry & Cabinet Makers
- Roofing & Gutter Contractors
- Window Contractors
- Exterior & Siding Contractors
- Carpet Contractors
- Carpet Installation
- Flooring Contractors
- Wood Floor Refinishing
- Tile Installation
- Custom Countertops
- Quartz Countertops
- Cabinet Refinishing
- Custom Bathroom Vanities
- Finish Carpentry
- Cabinet Repair
- Custom Windows
- Window Treatment Services
- Window Repair
- Fireplace Contractors
- Paint & Wall Covering Dealers
- Door Contractors
- Glass & Shower Door Contractors
- Landscape Construction
- Land Clearing
- Garden & Landscape Supplies
- Deck & Patio Builders
- Deck Repair
- Patio Design
- Stone, Pavers, & Concrete
- Paver Installation
- Driveway & Paving Contractors
- Driveway Repair
- Asphalt Paving
- Garage Door Repair
- Fence Contractors
- Fence Installation
- Gate Repair
- Pergola Construction
- Spa & Pool Maintenance
- Swimming Pool Contractors
- Hot Tub Installation
- HVAC Contractors
- Electricians
- Appliance Services
- Solar Energy Contractors
- Outdoor Lighting Installation
- Landscape Lighting Installation
- Outdoor Lighting & Audio/Visual Specialists
- Home Theater & Home Automation Services
- Handyman Services
- Closet Designers
- Professional Organizers
- Furniture & Accessories Retailers
- Furniture Repair & Upholstery Services
- Specialty Contractors
- Color Consulting
- Wine Cellar Designers & Builders
- Home Inspection
- Custom Artists
- Columbus, OH Painters
- New York City, NY Landscapers
- San Diego, CA Bathroom Remodelers
- Minneapolis, MN Architects
- Portland, OR Tile Installers
- Kansas City, MO Flooring Contractors
- Denver, CO Countertop Installers
- San Francisco, CA New Home Builders
- Rugs & Decor
- Home Improvement
- Kitchen & Tabletop
- Bathroom Vanities
- Bathroom Vanity Lighting
- Bathroom Mirrors
- Bathroom Fixtures
- Nightstands & Bedside Tables
- Kitchen & Dining
- Bar Stools & Counter Stools
- Dining Chairs
- Dining Tables
- Buffets and Sideboards
- Kitchen Fixtures
- Wall Mirrors
- Living Room
- Armchairs & Accent Chairs
- Coffee & Accent Tables
- Sofas & Sectionals
- Media Storage
- Patio & Outdoor Furniture
- Outdoor Lighting
- Ceiling Lighting
- Chandeliers
- Pendant Lighting
- Wall Sconces
- Desks & Hutches
- Office Chairs
- View All Products
- Designer Picks
- Side & End Tables
- Console Tables
- Living Room Sets
- Chaise Lounges
- Ottomans & Poufs
- Bedroom Furniture
- Nightstands
- Bedroom Sets
- Dining Room Sets
- Sideboards & Buffets
- File Cabinets
- Room Dividers
- Furniture Sale
- Trending in Furniture
- View All Furniture
- Bath Vanities
- Single Vanities
- Double Vanities
- Small Vanities
- Transitional Vanities
- Modern Vanities
- Houzz Curated Vanities
- Best Selling Vanities
- Bathroom Vanity Mirrors
- Medicine Cabinets
- Bathroom Faucets
- Bathroom Sinks
- Shower Doors
- Showerheads & Body Sprays
- Bathroom Accessories
- Bathroom Storage
- Trending in Bath
- View All Bath
- Houzz x Jennifer Kizzee
- Houzz x Motivo Home
- How to Choose a Bathroom Vanity
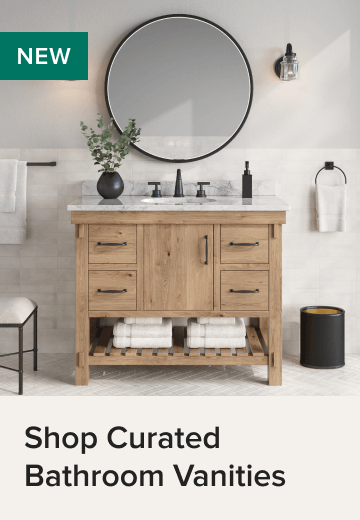
- Patio Furniture
- Outdoor Dining Furniture
- Outdoor Lounge Furniture
- Outdoor Chairs
- Adirondack Chairs
- Outdoor Bar Furniture
- Outdoor Benches
- Wall Lights & Sconces
- Outdoor Flush-Mounts
- Landscape Lighting
- Outdoor Flood & Spot Lights
- Outdoor Decor
- Outdoor Rugs
- Outdoor Cushions & Pillows
- Patio Umbrellas
- Lawn & Garden
- Garden Statues & Yard Art
- Planters & Pots
- Outdoor Sale
- Trending in Outdoor
- View All Outdoor
- 8 x 10 Rugs
- 9 x 12 Rugs
- Hall & Stair Runners
- Home Decor & Accents
- Pillows & Throws
- Decorative Storage
- Faux Florals
- Wall Panels
- Window Treatments
- Curtain Rods
- Blackout Curtains
- Blinds & Shades
- Rugs & Decor Sale
- Trending in Rugs & Decor
- View All Rugs & Decor
- Pendant Lights
- Flush-Mounts
- Ceiling Fans
- Track Lighting
- Wall Lighting
- Swing Arm Wall Lights
- Display Lighting
- Table Lamps
- Floor Lamps
- Lamp Shades
- Lighting Sale
- Trending in Lighting
- View All Lighting
- Bathroom Remodel
- Kitchen Remodel
- Kitchen Faucets
- Kitchen Sinks
- Major Kitchen Appliances
- Cabinet Hardware
- Backsplash Tile
- Mosaic Tile
- Wall & Floor Tile
- Accent, Trim & Border Tile
- Whole House Remodel
- Heating & Cooling
- Building Materials
- Front Doors
- Interior Doors
- Home Improvement Sale
- Trending in Home Improvement
- View All Home Improvement
- Cups & Glassware
- Kitchen & Table Linens
- Kitchen Storage and Org
- Kitchen Islands & Carts
- Food Containers & Canisters
- Pantry & Cabinet Organizers
- Kitchen Appliances
- Gas & Electric Ranges
- Range Hoods & Vents
- Beer & Wine Refrigerators
- Small Kitchen Appliances
- Cookware & Bakeware
- Tools & Gadgets
- Kitchen & Tabletop Sale
- Trending in Kitchen & Tabletop
- View All Kitchen & Tabletop
- Storage & Organization
- Baby & Kids
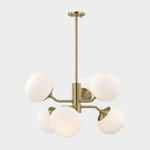
- View all photos
- Dining Room
- Breakfast Nook
- Family Room
- Bed & Bath
- Powder Room
- Storage & Closet
- Outdoor Kitchen
- Bar & Wine
- Wine Cellar
- Home Office
- Popular Design Ideas
- Kitchen Backsplash
- Deck Railing
- Privacy Fence
- Small Closet
- Stories and Guides
- Popular Stories
- Renovation Cost Guides
- Fence Installation Cost Guide
- Window Installation Cost Guide
- Discussions
- Design Dilemmas
- Before & After
- Houzz Research
- View all pros
- View all services
- View all products
- View all sales
- Living Room Chairs
- Dining Room Furniture
- Coffee Tables
- Home Office Furniture
- Join as a Pro
- Interior Design Software
- Project Management
- Custom Website
- Lead Generation
- Invoicing & Billing
- Landscape Contractor Software
- General Contractor Software
- Remodeler Software
- Builder Software
- Roofer Software
- Architect Software
- Takeoff Software
- Lumber & Framing Takeoffs
- Steel Takeoffs
- Concrete Takeoffs
- Drywall Takeoffs
- Insulation Takeoffs
- Stories & Guides
- LATEST FROM HOUZZ
- HOUZZ DISCUSSIONS
- SHOP KITCHEN & DINING
- Kitchen & Dining Furniture
- Sinks & Faucets
- Kitchen Cabinets & Storage
- Knobs & Pulls
- Kitchen Knives
- KITCHEN PHOTOS
- FIND KITCHEN PROS
- Bath Accessories
- Bath Linens
- BATH PHOTOS
- FIND BATH PROS
- SHOP BEDROOM
- Beds & Headboards
- Bedroom Decor
- Closet Storage
- Bedroom Vanities
- BEDROOM PHOTOS
- Kids' Room
- FIND DESIGN PROS
- SHOP LIVING
- Fireplaces & Accessories
- LIVING PHOTOS
- SHOP OUTDOOR
- Pool & Spa
- Backyard Play
- OUTDOOR PHOTOS
- FIND LANDSCAPING PROS
- SHOP LIGHTING
- Bathroom & Vanity
- Flush Mounts
- Kitchen & Cabinet
- Outdoor Wall Lights
- Outdoor Hanging Lights
- Kids' Lighting
- Decorative Accents
- Artificial Flowers & Plants
- Decorative Objects
- Screens & Room Dividers
- Wall Shelves
- About Houzz
- Houzz Credit Cards
- Privacy & Notice
- Cookie Policy
- Your Privacy Choices
- Mobile Apps
- Copyright & Trademark
- For Professionals
- Houzz vs. Houzz Pro
- Houzz Pro vs. Ivy
- Houzz Pro Advertising Reviews
- Houzz Pro 3D Floor Planner Reviews
- Trade Program
- Buttons & Badges
- Your Orders
- Shipping & Delivery
- Return Policy
- Houzz Canada
- Review Professionals
- Suggested Professionals
- Accessibility
- Houzz Support
- COUNTRY COUNTRY
New & Custom Home Builders in Elektrostal'
Location (1).
- Use My Current Location
Popular Locations
- Albuquerque
- Cedar Rapids
- Grand Rapids
- Indianapolis
- Jacksonville
- Kansas City
- Little Rock
- Los Angeles
- Minneapolis
- New Orleans
- Oklahoma City
- Orange County
- Philadelphia
- Portland Maine
- Salt Lake City
- San Francisco
- San Luis Obispo
- Santa Barbara
- Washington D.C.
- Elektrostal', Moscow Oblast, Russia
Professional Category (1)
- Accessory Dwelling Units (ADU)
Featured Reviews for New & Custom Home Builders in Elektrostal'
- Reach out to the pro(s) you want, then share your vision to get the ball rolling.
- Request and compare quotes, then hire the Home Builder that perfectly fits your project and budget limits.
Before choosing a Builder for your residential home project in Elektrostal', there are a few important steps to take:
- Define your project: Outline your desired home type, features, and layout. Provide specific details and preferences to help the builder understand your vision.
- Establish a budget: Develop a comprehensive budget, including construction expenses and material costs. Communicate your budgetary constraints to the builder from the beginning.
- Timeline: Share your estimated timeline or desired completion date.
- Site conditions: Inform the builder about any unique site conditions or challenges.
- Local regulations: Make the builder aware of any building regulations or permits required.
- Land Surveying
What do new home building contractors do?
Questions to ask a prospective custom home builder in elektrostal', moscow oblast, russia:.
If you search for Home Builders near me you'll be sure to find a business that knows about modern design concepts and innovative technologies to meet the evolving needs of homeowners. With their expertise, Home Builders ensure that renovation projects align with clients' preferences and aspirations, delivering personalized and contemporary living spaces.
BUSINESS SERVICES
Connect with us.

IMAGES
VIDEO
COMMENTS
This repository has all the code and report for my Udacity Data Scientist Nanodegree Capstone project. Project Overview : This data set contains simulated data that mimics customer behavior on the Starbucks rewards mobile app.
Project Overview. This project focuses on identifying reward program offers that effectively engage Starbucks' current customers and attract new ones. Starbucks is a data-driven company that invests in obtaining a complete understanding of its customers by utilizing datasets containing customer information, special offers, and transaction data.
Whether a customer created an account on the Starbucks rewards mobile application in 2018 Since the top three features are associated with an customer offer, it may be possible to improve the performance of a random forest model by creating features that describe an offer's success rate as a function of offer difficulty, duration, and reward.
Introducing a Dataset. This data set contains simulated data that mimics customer behavior on the Starbucks rewards mobile app. Once every few days, Starbucks sends out an offer to users of the mobile app. An offer can be merely an advertisement for a drink or an actual offer such as a discount or BOGO (buy one get one free).
Capstone project for the Udacity Data Scientist Nanodegree - MohanCR97/Starbucks_CapstoneProject
Starbucks-Capstone-Project. This is a capstone project for the course completed in response to Udacity- Data Scientist Nanodegree Program. Once every few days, Starbucks sends out an offer to users of the mobile app. An offer can be merely an advertisement for a drink or an actual offer such as a discount or BOGO (buy one get one free).
Udacity Capstone Project Starbucks. Final project for Udacity's Data Scientist Nanodegree. Installation. In order to run the Jupyter notebook you will need to have python 3 installed and the following dependencies: pandas; Sklearn; Numpy; Matplotlib; Seaborn; Project Motivation. Using similated data obtained from Starbucks that mimics how ...
Credits and Acknowledgements. 1. Problem Statement. A data set contains simulated data that mimics customer behaviour on the Starbucks rewards mobile app. Once every few days, Starbucks sends out an offer to users of its mobile app. An offer can be merely an advertisement for a drink or an actual offer such as a discount or BOGO (buy one get ...
This is my capstone project for the Udacity Machine Learning Engineer Nanodegree. The full source code can be found on my GitHub. Udacity partnered with Starbucks Coffee to provide a real-world…
# Starbucks Promotions Project ### This project is the Capstone Project of Udacity's Machine Learning Engineering Nanodegree program.  profile.json — demographic data for each customer.
This is a capstone project of the Udacity data science nanodegree program. The dataset contains simulated data that mimics customer behavior on the Starbucks rewards mobile app. Once every few days, Starbucks sends out an offer to users of the mobile app. An offer can be merely an advertisement for a drink or an actual offer such as a discount ...
The project is popularly known as Starbucks Capstone Project. The project contains simulated data that mimics customer behavior on the Starbucks rewards app. This data collection contains ...
GitHub - kwlwman2/StarbuckCaptoneProject: Udacity Data Science Nanodegree Udacity Data Science Nanodegree The project is the capstone project set up for the completion of Udacity Data Scientist ...
Capstone project for the Udacity Data Scientist Nanodegree View on GitHub Starbucks Capstone Challenge Introduction. Starbucks has provided a dataset that emulates the behavior of customers using the Starbucks rewards mobile app. The Starbucks app provides a way to advertise and share offers with the customers. Customers can also use it to pay ...
jdabel123/Udacity-CapstoneProject-Starbucks Permalink GitHub is home to over 50 million developers working together to host and review code, manage projects, and… github.com
Starbucks Capstone Project. Contribute to pato0301/starbucks_capstone_udacity development by creating an account on GitHub.
Starbucks Capstone Project. This article is a part of Udacity Data Scientist Nanodegree Progam. To see more about my analysis, see my github. Photo by TR on Unsplash
Web developer with abilities to design, implement, deploy, test and debug Ruby on Rails applications.
Adres: BOSB Mermerciler San. Sitesi 4. Cadde No: 7 34520, Beylikdüzü / İstanbul / TÜRKİYE
Secondly, energy is supplied to the main mining companies in western Chukotka in the Chaun-Bilibino energy hub a large ore and metal cluster, including gold mining companies and projects related to the development of the Baimsk ore zone.
House builders are responsible for ensuring that the project sticks as closely as possible to the specified timetable, particularly in the event of change orders. Custom local home builders in Elektrostal', Moscow Oblast, Russia also need interpersonal skills to deal with clients of all types, soothe frazzled nerves, negotiate conflicts and ...