6.1 Overview of Non-Experimental Research
Learning objectives.
- Define non-experimental research, distinguish it clearly from experimental research, and give several examples.
- Explain when a researcher might choose to conduct non-experimental research as opposed to experimental research.

What Is Non-Experimental Research?
Non-experimental research is research that lacks the manipulation of an independent variable. Rather than manipulating an independent variable, researchers conducting non-experimental research simply measure variables as they naturally occur (in the lab or real world).
Most researchers in psychology consider the distinction between experimental and non-experimental research to be an extremely important one. This is because although experimental research can provide strong evidence that changes in an independent variable cause differences in a dependent variable, non-experimental research generally cannot. As we will see, however, this inability to make causal conclusions does not mean that non-experimental research is less important than experimental research.
When to Use Non-Experimental Research
As we saw in the last chapter , experimental research is appropriate when the researcher has a specific research question or hypothesis about a causal relationship between two variables—and it is possible, feasible, and ethical to manipulate the independent variable. It stands to reason, therefore, that non-experimental research is appropriate—even necessary—when these conditions are not met. There are many times in which non-experimental research is preferred, including when:
- the research question or hypothesis relates to a single variable rather than a statistical relationship between two variables (e.g., How accurate are people’s first impressions?).
- the research question pertains to a non-causal statistical relationship between variables (e.g., is there a correlation between verbal intelligence and mathematical intelligence?).
- the research question is about a causal relationship, but the independent variable cannot be manipulated or participants cannot be randomly assigned to conditions or orders of conditions for practical or ethical reasons (e.g., does damage to a person’s hippocampus impair the formation of long-term memory traces?).
- the research question is broad and exploratory, or is about what it is like to have a particular experience (e.g., what is it like to be a working mother diagnosed with depression?).
Again, the choice between the experimental and non-experimental approaches is generally dictated by the nature of the research question. Recall the three goals of science are to describe, to predict, and to explain. If the goal is to explain and the research question pertains to causal relationships, then the experimental approach is typically preferred. If the goal is to describe or to predict, a non-experimental approach will suffice. But the two approaches can also be used to address the same research question in complementary ways. For example, Similarly, after his original study, Milgram conducted experiments to explore the factors that affect obedience. He manipulated several independent variables, such as the distance between the experimenter and the participant, the participant and the confederate, and the location of the study (Milgram, 1974) [1] .
Types of Non-Experimental Research
Non-experimental research falls into three broad categories: cross-sectional research, correlational research, and observational research.
First, cross-sectional research involves comparing two or more pre-existing groups of people. What makes this approach non-experimental is that there is no manipulation of an independent variable and no random assignment of participants to groups. Imagine, for example, that a researcher administers the Rosenberg Self-Esteem Scale to 50 American college students and 50 Japanese college students. Although this “feels” like a between-subjects experiment, it is a cross-sectional study because the researcher did not manipulate the students’ nationalities. As another example, if we wanted to compare the memory test performance of a group of cannabis users with a group of non-users, this would be considered a cross-sectional study because for ethical and practical reasons we would not be able to randomly assign participants to the cannabis user and non-user groups. Rather we would need to compare these pre-existing groups which could introduce a selection bias (the groups may differ in other ways that affect their responses on the dependent variable). For instance, cannabis users are more likely to use more alcohol and other drugs and these differences may account for differences in the dependent variable across groups, rather than cannabis use per se.
Cross-sectional designs are commonly used by developmental psychologists who study aging and by researchers interested in sex differences. Using this design, developmental psychologists compare groups of people of different ages (e.g., young adults spanning from 18-25 years of age versus older adults spanning 60-75 years of age) on various dependent variables (e.g., memory, depression, life satisfaction). Of course, the primary limitation of using this design to study the effects of aging is that differences between the groups other than age may account for differences in the dependent variable. For instance, differences between the groups may reflect the generation that people come from (a cohort effect) rather than a direct effect of age. For this reason, longitudinal studies in which one group of people is followed as they age offer a superior means of studying the effects of aging. Once again, cross-sectional designs are also commonly used to study sex differences. Since researchers cannot practically or ethically manipulate the sex of their participants they must rely on cross-sectional designs to compare groups of men and women on different outcomes (e.g., verbal ability, substance use, depression). Using these designs researchers have discovered that men are more likely than women to suffer from substance abuse problems while women are more likely than men to suffer from depression. But, using this design it is unclear what is causing these differences. So, using this design it is unclear whether these differences are due to environmental factors like socialization or biological factors like hormones?
When researchers use a participant characteristic to create groups (nationality, cannabis use, age, sex), the independent variable is usually referred to as an experimenter-selected independent variable (as opposed to the experimenter-manipulated independent variables used in experimental research). Figure 6.1 shows data from a hypothetical study on the relationship between whether people make a daily list of things to do (a “to-do list”) and stress. Notice that it is unclear whether this is an experiment or a cross-sectional study because it is unclear whether the independent variable was manipulated by the researcher or simply selected by the researcher. If the researcher randomly assigned some participants to make daily to-do lists and others not to, then the independent variable was experimenter-manipulated and it is a true experiment. If the researcher simply asked participants whether they made daily to-do lists or not, then the independent variable it is experimenter-selected and the study is cross-sectional. The distinction is important because if the study was an experiment, then it could be concluded that making the daily to-do lists reduced participants’ stress. But if it was a cross-sectional study, it could only be concluded that these variables are statistically related. Perhaps being stressed has a negative effect on people’s ability to plan ahead. Or perhaps people who are more conscientious are more likely to make to-do lists and less likely to be stressed. The crucial point is that what defines a study as experimental or cross-sectional l is not the variables being studied, nor whether the variables are quantitative or categorical, nor the type of graph or statistics used to analyze the data. It is how the study is conducted.
Figure 6.1 Results of a Hypothetical Study on Whether People Who Make Daily To-Do Lists Experience Less Stress Than People Who Do Not Make Such Lists
Second, the most common type of non-experimental research conducted in Psychology is correlational research. Correlational research is considered non-experimental because it focuses on the statistical relationship between two variables but does not include the manipulation of an independent variable. More specifically, in correlational research , the researcher measures two continuous variables with little or no attempt to control extraneous variables and then assesses the relationship between them. As an example, a researcher interested in the relationship between self-esteem and school achievement could collect data on students’ self-esteem and their GPAs to see if the two variables are statistically related. Correlational research is very similar to cross-sectional research, and sometimes these terms are used interchangeably. The distinction that will be made in this book is that, rather than comparing two or more pre-existing groups of people as is done with cross-sectional research, correlational research involves correlating two continuous variables (groups are not formed and compared).
Third, observational research is non-experimental because it focuses on making observations of behavior in a natural or laboratory setting without manipulating anything. Milgram’s original obedience study was non-experimental in this way. He was primarily interested in the extent to which participants obeyed the researcher when he told them to shock the confederate and he observed all participants performing the same task under the same conditions. The study by Loftus and Pickrell described at the beginning of this chapter is also a good example of observational research. The variable was whether participants “remembered” having experienced mildly traumatic childhood events (e.g., getting lost in a shopping mall) that they had not actually experienced but that the researchers asked them about repeatedly. In this particular study, nearly a third of the participants “remembered” at least one event. (As with Milgram’s original study, this study inspired several later experiments on the factors that affect false memories.
The types of research we have discussed so far are all quantitative, referring to the fact that the data consist of numbers that are analyzed using statistical techniques. But as you will learn in this chapter, many observational research studies are more qualitative in nature. In qualitative research , the data are usually nonnumerical and therefore cannot be analyzed using statistical techniques. Rosenhan’s observational study of the experience of people in a psychiatric ward was primarily qualitative. The data were the notes taken by the “pseudopatients”—the people pretending to have heard voices—along with their hospital records. Rosenhan’s analysis consists mainly of a written description of the experiences of the pseudopatients, supported by several concrete examples. To illustrate the hospital staff’s tendency to “depersonalize” their patients, he noted, “Upon being admitted, I and other pseudopatients took the initial physical examinations in a semi-public room, where staff members went about their own business as if we were not there” (Rosenhan, 1973, p. 256) [2] . Qualitative data has a separate set of analysis tools depending on the research question. For example, thematic analysis would focus on themes that emerge in the data or conversation analysis would focus on the way the words were said in an interview or focus group.
Internal Validity Revisited
Recall that internal validity is the extent to which the design of a study supports the conclusion that changes in the independent variable caused any observed differences in the dependent variable. Figure 6.2 shows how experimental, quasi-experimental, and non-experimental (correlational) research vary in terms of internal validity. Experimental research tends to be highest in internal validity because the use of manipulation (of the independent variable) and control (of extraneous variables) help to rule out alternative explanations for the observed relationships. If the average score on the dependent variable in an experiment differs across conditions, it is quite likely that the independent variable is responsible for that difference. Non-experimental (correlational) research is lowest in internal validity because these designs fail to use manipulation or control. Quasi-experimental research (which will be described in more detail in a subsequent chapter) is in the middle because it contains some, but not all, of the features of a true experiment. For instance, it may fail to use random assignment to assign participants to groups or fail to use counterbalancing to control for potential order effects. Imagine, for example, that a researcher finds two similar schools, starts an anti-bullying program in one, and then finds fewer bullying incidents in that “treatment school” than in the “control school.” While a comparison is being made with a control condition, the lack of random assignment of children to schools could still mean that students in the treatment school differed from students in the control school in some other way that could explain the difference in bullying (e.g., there may be a selection effect).

Figure 6.2 Internal Validity of Correlation, Quasi-Experimental, and Experimental Studies. Experiments are generally high in internal validity, quasi-experiments lower, and correlation studies lower still.
Notice also in Figure 6.2 that there is some overlap in the internal validity of experiments, quasi-experiments, and correlational studies. For example, a poorly designed experiment that includes many confounding variables can be lower in internal validity than a well-designed quasi-experiment with no obvious confounding variables. Internal validity is also only one of several validities that one might consider, as noted in Chapter 5.
Key Takeaways
- Non-experimental research is research that lacks the manipulation of an independent variable.
- There are two broad types of non-experimental research. Correlational research that focuses on statistical relationships between variables that are measured but not manipulated, and observational research in which participants are observed and their behavior is recorded without the researcher interfering or manipulating any variables.
- In general, experimental research is high in internal validity, correlational research is low in internal validity, and quasi-experimental research is in between.
- A researcher conducts detailed interviews with unmarried teenage fathers to learn about how they feel and what they think about their role as fathers and summarizes their feelings in a written narrative.
- A researcher measures the impulsivity of a large sample of drivers and looks at the statistical relationship between this variable and the number of traffic tickets the drivers have received.
- A researcher randomly assigns patients with low back pain either to a treatment involving hypnosis or to a treatment involving exercise. She then measures their level of low back pain after 3 months.
- A college instructor gives weekly quizzes to students in one section of his course but no weekly quizzes to students in another section to see whether this has an effect on their test performance.
- Milgram, S. (1974). Obedience to authority: An experimental view . New York, NY: Harper & Row. ↵
- Rosenhan, D. L. (1973). On being sane in insane places. Science, 179 , 250–258. ↵

Share This Book
- Increase Font Size

An official website of the United States government
The .gov means it’s official. Federal government websites often end in .gov or .mil. Before sharing sensitive information, make sure you’re on a federal government site.
The site is secure. The https:// ensures that you are connecting to the official website and that any information you provide is encrypted and transmitted securely.
- Publications
- Account settings
Preview improvements coming to the PMC website in October 2024. Learn More or Try it out now .
- Advanced Search
- Journal List
- v.11(2); 2019 Feb

Planning and Conducting Clinical Research: The Whole Process
Boon-how chew.
1 Family Medicine, Universiti Putra Malaysia, Serdang, MYS
The goal of this review was to present the essential steps in the entire process of clinical research. Research should begin with an educated idea arising from a clinical practice issue. A research topic rooted in a clinical problem provides the motivation for the completion of the research and relevancy for affecting medical practice changes and improvements. The research idea is further informed through a systematic literature review, clarified into a conceptual framework, and defined into an answerable research question. Engagement with clinical experts, experienced researchers, relevant stakeholders of the research topic, and even patients can enhance the research question’s relevance, feasibility, and efficiency. Clinical research can be completed in two major steps: study designing and study reporting. Three study designs should be planned in sequence and iterated until properly refined: theoretical design, data collection design, and statistical analysis design. The design of data collection could be further categorized into three facets: experimental or non-experimental, sampling or census, and time features of the variables to be studied. The ultimate aims of research reporting are to present findings succinctly and timely. Concise, explicit, and complete reporting are the guiding principles in clinical studies reporting.
Introduction and background
Medical and clinical research can be classified in many different ways. Probably, most people are familiar with basic (laboratory) research, clinical research, healthcare (services) research, health systems (policy) research, and educational research. Clinical research in this review refers to scientific research related to clinical practices. There are many ways a clinical research's findings can become invalid or less impactful including ignorance of previous similar studies, a paucity of similar studies, poor study design and implementation, low test agent efficacy, no predetermined statistical analysis, insufficient reporting, bias, and conflicts of interest [ 1 - 4 ]. Scientific, ethical, and moral decadence among researchers can be due to incognizant criteria in academic promotion and remuneration and too many forced studies by amateurs and students for the sake of research without adequate training or guidance [ 2 , 5 - 6 ]. This article will review the proper methods to conduct medical research from the planning stage to submission for publication (Table (Table1 1 ).
a Feasibility and efficiency are considered during the refinement of the research question and adhered to during data collection.
Epidemiologic studies in clinical and medical fields focus on the effect of a determinant on an outcome [ 7 ]. Measurement errors that happen systematically give rise to biases leading to invalid study results, whereas random measurement errors will cause imprecise reporting of effects. Precision can usually be increased with an increased sample size provided biases are avoided or trivialized. Otherwise, the increased precision will aggravate the biases. Because epidemiologic, clinical research focuses on measurement, measurement errors are addressed throughout the research process. Obtaining the most accurate estimate of a treatment effect constitutes the whole business of epidemiologic research in clinical practice. This is greatly facilitated by clinical expertise and current scientific knowledge of the research topic. Current scientific knowledge is acquired through literature reviews or in collaboration with an expert clinician. Collaboration and consultation with an expert clinician should also include input from the target population to confirm the relevance of the research question. The novelty of a research topic is less important than the clinical applicability of the topic. Researchers need to acquire appropriate writing and reporting skills from the beginning of their careers, and these skills should improve with persistent use and regular reviewing of published journal articles. A published clinical research study stands on solid scientific ground to inform clinical practice given the article has passed through proper peer-reviews, revision, and content improvement.
Systematic literature reviews
Systematic literature reviews of published papers will inform authors of the existing clinical evidence on a research topic. This is an important step to reduce wasted efforts and evaluate the planned study [ 8 ]. Conducting a systematic literature review is a well-known important step before embarking on a new study [ 9 ]. A rigorously performed and cautiously interpreted systematic review that includes in-process trials can inform researchers of several factors [ 10 ]. Reviewing the literature will inform the choice of recruitment methods, outcome measures, questionnaires, intervention details, and statistical strategies – useful information to increase the study’s relevance, value, and power. A good review of previous studies will also provide evidence of the effects of an intervention that may or may not be worthwhile; this would suggest either no further studies are warranted or that further study of the intervention is needed. A review can also inform whether a larger and better study is preferable to an additional small study. Reviews of previously published work may yield few studies or low-quality evidence from small or poorly designed studies on certain intervention or observation; this may encourage or discourage further research or prompt consideration of a first clinical trial.
Conceptual framework
The result of a literature review should include identifying a working conceptual framework to clarify the nature of the research problem, questions, and designs, and even guide the latter discussion of the findings and development of possible solutions. Conceptual frameworks represent ways of thinking about a problem or how complex things work the way they do [ 11 ]. Different frameworks will emphasize different variables and outcomes, and their inter-relatedness. Each framework highlights or emphasizes different aspects of a problem or research question. Often, any single conceptual framework presents only a partial view of reality [ 11 ]. Furthermore, each framework magnifies certain elements of the problem. Therefore, a thorough literature search is warranted for authors to avoid repeating the same research endeavors or mistakes. It may also help them find relevant conceptual frameworks including those that are outside one’s specialty or system.
Conceptual frameworks can come from theories with well-organized principles and propositions that have been confirmed by observations or experiments. Conceptual frameworks can also come from models derived from theories, observations or sets of concepts or even evidence-based best practices derived from past studies [ 11 ].
Researchers convey their assumptions of the associations of the variables explicitly in the conceptual framework to connect the research to the literature. After selecting a single conceptual framework or a combination of a few frameworks, a clinical study can be completed in two fundamental steps: study design and study report. Three study designs should be planned in sequence and iterated until satisfaction: the theoretical design, data collection design, and statistical analysis design [ 7 ].
Study designs
Theoretical Design
Theoretical design is the next important step in the research process after a literature review and conceptual framework identification. While the theoretical design is a crucial step in research planning, it is often dealt with lightly because of the more alluring second step (data collection design). In the theoretical design phase, a research question is designed to address a clinical problem, which involves an informed understanding based on the literature review and effective collaboration with the right experts and clinicians. A well-developed research question will have an initial hypothesis of the possible relationship between the explanatory variable/exposure and the outcome. This will inform the nature of the study design, be it qualitative or quantitative, primary or secondary, and non-causal or causal (Figure (Figure1 1 ).

A study is qualitative if the research question aims to explore, understand, describe, discover or generate reasons underlying certain phenomena. Qualitative studies usually focus on a process to determine how and why things happen [ 12 ]. Quantitative studies use deductive reasoning, and numerical statistical quantification of the association between groups on data often gathered during experiments [ 13 ]. A primary clinical study is an original study gathering a new set of patient-level data. Secondary research draws on the existing available data and pooling them into a larger database to generate a wider perspective or a more powerful conclusion. Non-causal or descriptive research aims to identify the determinants or associated factors for the outcome or health condition, without regard for causal relationships. Causal research is an exploration of the determinants of an outcome while mitigating confounding variables. Table Table2 2 shows examples of non-causal (e.g., diagnostic and prognostic) and causal (e.g., intervention and etiologic) clinical studies. Concordance between the research question, its aim, and the choice of theoretical design will provide a strong foundation and the right direction for the research process and path.
A problem in clinical epidemiology is phrased in a mathematical relationship below, where the outcome is a function of the determinant (D) conditional on the extraneous determinants (ED) or more commonly known as the confounding factors [ 7 ]:
For non-causal research, Outcome = f (D1, D2…Dn) For causal research, Outcome = f (D | ED)
A fine research question is composed of at least three components: 1) an outcome or a health condition, 2) determinant/s or associated factors to the outcome, and 3) the domain. The outcome and the determinants have to be clearly conceptualized and operationalized as measurable variables (Table (Table3; 3 ; PICOT [ 14 ] and FINER [ 15 ]). The study domain is the theoretical source population from which the study population will be sampled, similar to the wording on a drug package insert that reads, “use this medication (study results) in people with this disease” [ 7 ].
The interpretation of study results as they apply to wider populations is known as generalization, and generalization can either be statistical or made using scientific inferences [ 16 ]. Generalization supported by statistical inferences is seen in studies on disease prevalence where the sample population is representative of the source population. By contrast, generalizations made using scientific inferences are not bound by the representativeness of the sample in the study; rather, the generalization should be plausible from the underlying scientific mechanisms as long as the study design is valid and nonbiased. Scientific inferences and generalizations are usually the aims of causal studies.
Confounding: Confounding is a situation where true effects are obscured or confused [ 7 , 16 ]. Confounding variables or confounders affect the validity of a study’s outcomes and should be prevented or mitigated in the planning stages and further managed in the analytical stages. Confounders are also known as extraneous determinants in epidemiology due to their inherent and simultaneous relationships to both the determinant and outcome (Figure (Figure2), 2 ), which are usually one-determinant-to-one outcome in causal clinical studies. The known confounders are also called observed confounders. These can be minimized using randomization, restriction, or a matching strategy. Residual confounding has occurred in a causal relationship when identified confounders were not measured accurately. Unobserved confounding occurs when the confounding effect is present as a variable or factor not observed or yet defined and, thus, not measured in the study. Age and gender are almost universal confounders followed by ethnicity and socio-economic status.

Confounders have three main characteristics. They are a potential risk factor for the disease, associated with the determinant of interest, and should not be an intermediate variable between the determinant and the outcome or a precursor to the determinant. For example, a sedentary lifestyle is a cause for acute coronary syndrome (ACS), and smoking could be a confounder but not cardiorespiratory unfitness (which is an intermediate factor between a sedentary lifestyle and ACS). For patients with ACS, not having a pair of sports shoes is not a confounder – it is a correlate for the sedentary lifestyle. Similarly, depression would be a precursor, not a confounder.
Sample size consideration: Sample size calculation provides the required number of participants to be recruited in a new study to detect true differences in the target population if they exist. Sample size calculation is based on three facets: an estimated difference in group sizes, the probability of α (Type I) and β (Type II) errors chosen based on the nature of the treatment or intervention, and the estimated variability (interval data) or proportion of the outcome (nominal data) [ 17 - 18 ]. The clinically important effect sizes are determined based on expert consensus or patients’ perception of benefit. Value and economic consideration have increasingly been included in sample size estimations. Sample size and the degree to which the sample represents the target population affect the accuracy and generalization of a study’s reported effects.
Pilot study: Pilot studies assess the feasibility of the proposed research procedures on small sample size. Pilot studies test the efficiency of participant recruitment with minimal practice or service interruptions. Pilot studies should not be conducted to obtain a projected effect size for a larger study population because, in a typical pilot study, the sample size is small, leading to a large standard error of that effect size. This leads to bias when projected for a large population. In the case of underestimation, this could lead to inappropriately terminating the full-scale study. As the small pilot study is equally prone to bias of overestimation of the effect size, this would lead to an underpowered study and a failed full-scale study [ 19 ].
The Design of Data Collection
The “perfect” study design in the theoretical phase now faces the practical and realistic challenges of feasibility. This is the step where different methods for data collection are considered, with one selected as the most appropriate based on the theoretical design along with feasibility and efficiency. The goal of this stage is to achieve the highest possible validity with the lowest risk of biases given available resources and existing constraints.
In causal research, data on the outcome and determinants are collected with utmost accuracy via a strict protocol to maximize validity and precision. The validity of an instrument is defined as the degree of fidelity of the instrument, measuring what it is intended to measure, that is, the results of the measurement correlate with the true state of an occurrence. Another widely used word for validity is accuracy. Internal validity refers to the degree of accuracy of a study’s results to its own study sample. Internal validity is influenced by the study designs, whereas the external validity refers to the applicability of a study’s result in other populations. External validity is also known as generalizability and expresses the validity of assuming the similarity and comparability between the study population and the other populations. Reliability of an instrument denotes the extent of agreeableness of the results of repeated measurements of an occurrence by that instrument at a different time, by different investigators or in a different setting. Other terms that are used for reliability include reproducibility and precision. Preventing confounders by identifying and including them in data collection will allow statistical adjustment in the later analyses. In descriptive research, outcomes must be confirmed with a referent standard, and the determinants should be as valid as those found in real clinical practice.
Common designs for data collection include cross-sectional, case-control, cohort, and randomized controlled trials (RCTs). Many other modern epidemiology study designs are based on these classical study designs such as nested case-control, case-crossover, case-control without control, and stepwise wedge clustered RCTs. A cross-sectional study is typically a snapshot of the study population, and an RCT is almost always a prospective study. Case-control and cohort studies can be retrospective or prospective in data collection. The nested case-control design differs from the traditional case-control design in that it is “nested” in a well-defined cohort from which information on the cohorts can be obtained. This design also satisfies the assumption that cases and controls represent random samples of the same study base. Table Table4 4 provides examples of these data collection designs.
Additional aspects in data collection: No single design of data collection for any research question as stated in the theoretical design will be perfect in actual conduct. This is because of myriad issues facing the investigators such as the dynamic clinical practices, constraints of time and budget, the urgency for an answer to the research question, and the ethical integrity of the proposed experiment. Therefore, feasibility and efficiency without sacrificing validity and precision are important considerations in data collection design. Therefore, data collection design requires additional consideration in the following three aspects: experimental/non-experimental, sampling, and timing [ 7 ]:
Experimental or non-experimental: Non-experimental research (i.e., “observational”), in contrast to experimental, involves data collection of the study participants in their natural or real-world environments. Non-experimental researches are usually the diagnostic and prognostic studies with cross-sectional in data collection. The pinnacle of non-experimental research is the comparative effectiveness study, which is grouped with other non-experimental study designs such as cross-sectional, case-control, and cohort studies [ 20 ]. It is also known as the benchmarking-controlled trials because of the element of peer comparison (using comparable groups) in interpreting the outcome effects [ 20 ]. Experimental study designs are characterized by an intervention on a selected group of the study population in a controlled environment, and often in the presence of a similar group of the study population to act as a comparison group who receive no intervention (i.e., the control group). Thus, the widely known RCT is classified as an experimental design in data collection. An experimental study design without randomization is referred to as a quasi-experimental study. Experimental studies try to determine the efficacy of a new intervention on a specified population. Table Table5 5 presents the advantages and disadvantages of experimental and non-experimental studies [ 21 ].
a May be an issue in cross-sectional studies that require a long recall to the past such as dietary patterns, antenatal events, and life experiences during childhood.
Once an intervention yields a proven effect in an experimental study, non-experimental and quasi-experimental studies can be used to determine the intervention’s effect in a wider population and within real-world settings and clinical practices. Pragmatic or comparative effectiveness are the usual designs used for data collection in these situations [ 22 ].
Sampling/census: Census is a data collection on the whole source population (i.e., the study population is the source population). This is possible when the defined population is restricted to a given geographical area. A cohort study uses the census method in data collection. An ecologic study is a cohort study that collects summary measures of the study population instead of individual patient data. However, many studies sample from the source population and infer the results of the study to the source population for feasibility and efficiency because adequate sampling provides similar results to the census of the whole population. Important aspects of sampling in research planning are sample size and representation of the population. Sample size calculation accounts for the number of participants needed to be in the study to discover the actual association between the determinant and outcome. Sample size calculation relies on the primary objective or outcome of interest and is informed by the estimated possible differences or effect size from previous similar studies. Therefore, the sample size is a scientific estimation for the design of the planned study.
A sampling of participants or cases in a study can represent the study population and the larger population of patients in that disease space, but only in prevalence, diagnostic, and prognostic studies. Etiologic and interventional studies do not share this same level of representation. A cross-sectional study design is common for determining disease prevalence in the population. Cross-sectional studies can also determine the referent ranges of variables in the population and measure change over time (e.g., repeated cross-sectional studies). Besides being cost- and time-efficient, cross-sectional studies have no loss to follow-up; recall bias; learning effect on the participant; or variability over time in equipment, measurement, and technician. A cross-sectional design for an etiologic study is possible when the determinants do not change with time (e.g., gender, ethnicity, genetic traits, and blood groups).
In etiologic research, comparability between the exposed and the non-exposed groups is more important than sample representation. Comparability between these two groups will provide an accurate estimate of the effect of the exposure (risk factor) on the outcome (disease) and enable valid inference of the causal relation to the domain (the theoretical population). In a case-control study, a sampling of the control group should be taken from the same study population (study base), have similar profiles to the cases (matching) but do not have the outcome seen in the cases. Matching important factors minimizes the confounding of the factors and increases statistical efficiency by ensuring similar numbers of cases and controls in confounders’ strata [ 23 - 24 ]. Nonetheless, perfect matching is neither necessary nor achievable in a case-control study because a partial match could achieve most of the benefits of the perfect match regarding a more precise estimate of odds ratio than statistical control of confounding in unmatched designs [ 25 - 26 ]. Moreover, perfect or full matching can lead to an underestimation of the point estimates [ 27 - 28 ].
Time feature: The timing of data collection for the determinant and outcome characterizes the types of studies. A cross-sectional study has the axis of time zero (T = 0) for both the determinant and the outcome, which separates it from all other types of research that have time for the outcome T > 0. Retrospective or prospective studies refer to the direction of data collection. In retrospective studies, information on the determinant and outcome have been collected or recorded before. In prospective studies, this information will be collected in the future. These terms should not be used to describe the relationship between the determinant and the outcome in etiologic studies. Time of exposure to the determinant, the time of induction, and the time at risk for the outcome are important aspects to understand. Time at risk is the period of time exposed to the determinant risk factors. Time of induction is the time from the sufficient exposure to the risk or causal factors to the occurrence of a disease. The latent period is when the occurrence of a disease without manifestation of the disease such as in “silence” diseases for example cancers, hypertension and type 2 diabetes mellitus which is detected from screening practices. Figure Figure3 3 illustrates the time features of a variable. Variable timing is important for accurate data capture.

The Design of Statistical Analysis
Statistical analysis of epidemiologic data provides the estimate of effects after correcting for biases (e.g., confounding factors) measures the variability in the data from random errors or chance [ 7 , 16 , 29 ]. An effect estimate gives the size of an association between the studied variables or the level of effectiveness of an intervention. This quantitative result allows for comparison and assessment of the usefulness and significance of the association or the intervention between studies. This significance must be interpreted with a statistical model and an appropriate study design. Random errors could arise in the study resulting from unexplained personal choices by the participants. Random error is, therefore, when values or units of measurement between variables change in non-concerted or non-directional manner. Conversely, when these values or units of measurement between variables change in a concerted or directional manner, we note a significant relationship as shown by statistical significance.
Variability: Researchers almost always collect the needed data through a sampling of subjects/participants from a population instead of a census. The process of sampling or multiple sampling in different geographical regions or over different periods contributes to varied information due to the random inclusion of different participants and chance occurrence. This sampling variation becomes the focus of statistics when communicating the degree and intensity of variation in the sampled data and the level of inference in the population. Sampling variation can be influenced profoundly by the total number of participants and the width of differences of the measured variable (standard deviation). Hence, the characteristics of the participants, measurements and sample size are all important factors in planning a study.
Statistical strategy: Statistical strategy is usually determined based on the theoretical and data collection designs. Use of a prespecified statistical strategy (including the decision to dichotomize any continuous data at certain cut-points, sub-group analysis or sensitive analyses) is recommended in the study proposal (i.e., protocol) to prevent data dredging and data-driven reports that predispose to bias. The nature of the study hypothesis also dictates whether directional (one-tailed) or non-directional (two-tailed) significance tests are conducted. In most studies, two-sided tests are used except in specific instances when unidirectional hypotheses may be appropriate (e.g., in superiority or non-inferiority trials). While data exploration is discouraged, epidemiological research is, by nature of its objectives, statistical research. Hence, it is acceptable to report the presence of persistent associations between any variables with plausible underlying mechanisms during the exploration of the data. The statistical methods used to produce the results should be explicitly explained. Many different statistical tests are used to handle various kinds of data appropriately (e.g., interval vs discrete), and/or the various distribution of the data (e.g., normally distributed or skewed). For additional details on statistical explanations and underlying concepts of statistical tests, readers are recommended the references as cited in this sentence [ 30 - 31 ].
Steps in statistical analyses: Statistical analysis begins with checking for data entry errors. Duplicates are eliminated, and proper units should be confirmed. Extremely low, high or suspicious values are confirmed from the source data again. If this is not possible, this is better classified as a missing value. However, if the unverified suspicious data are not obviously wrong, they should be further examined as an outlier in the analysis. The data checking and cleaning enables the analyst to establish a connection with the raw data and to anticipate possible results from further analyses. This initial step involves descriptive statistics that analyze central tendency (i.e., mode, median, and mean) and dispersion (i.e., (minimum, maximum, range, quartiles, absolute deviation, variance, and standard deviation) of the data. Certain graphical plotting such as scatter plot, a box-whiskers plot, histogram or normal Q-Q plot are helpful at this stage to verify data normality in distribution. See Figure Figure4 4 for the statistical tests available for analyses of different types of data.

Once data characteristics are ascertained, further statistical tests are selected. The analytical strategy sometimes involves the transformation of the data distribution for the selected tests (e.g., log, natural log, exponential, quadratic) or for checking the robustness of the association between the determinants and their outcomes. This step is also referred to as inferential statistics whereby the results are about hypothesis testing and generalization to the wider population that the study’s sampled participants represent. The last statistical step is checking whether the statistical analyses fulfill the assumptions of that particular statistical test and model to avoid violation and misleading results. These assumptions include evaluating normality, variance homogeneity, and residuals included in the final statistical model. Other statistical values such as Akaike information criterion, variance inflation factor/tolerance, and R2 are also considered when choosing the best-fitted models. Transforming raw data could be done, or a higher level of statistical analyses can be used (e.g., generalized linear models and mixed-effect modeling). Successful statistical analysis allows conclusions of the study to fit the data.
Bayesian and Frequentist statistical frameworks: Most of the current clinical research reporting is based on the frequentist approach and hypotheses testing p values and confidence intervals. The frequentist approach assumes the acquired data are random, attained by random sampling, through randomized experiments or influences, and with random errors. The distribution of the data (its point estimate and confident interval) infers a true parameter in the real population. The major conceptual difference between Bayesian statistics and frequentist statistics is that in Bayesian statistics, the parameter (i.e., the studied variable in the population) is random and the data acquired is real (true or fix). Therefore, the Bayesian approach provides a probability interval for the parameter. The studied parameter is random because it could vary and be affected by prior beliefs, experience or evidence of plausibility. In the Bayesian statistical approach, this prior belief or available knowledge is quantified into a probability distribution and incorporated into the acquired data to get the results (i.e., the posterior distribution). This uses mathematical theory of Bayes’ Theorem to “turn around” conditional probabilities.
The goal of research reporting is to present findings succinctly and timely via conference proceedings or journal publication. Concise and explicit language use, with all the necessary details to enable replication and judgment of the study applicability, are the guiding principles in clinical studies reporting.
Writing for Reporting
Medical writing is very much a technical chore that accommodates little artistic expression. Research reporting in medicine and health sciences emphasize clear and standardized reporting, eschewing adjectives and adverbs extensively used in popular literature. Regularly reviewing published journal articles can familiarize authors with proper reporting styles and help enhance writing skills. Authors should familiarize themselves with standard, concise, and appropriate rhetoric for the intended audience, which includes consideration for journal reviewers, editors, and referees. However, proper language can be somewhat subjective. While each publication may have varying requirements for submission, the technical requirements for formatting an article are usually available via author or submission guidelines provided by the target journal.
Research reports for publication often contain a title, abstract, introduction, methods, results, discussion, and conclusions section, and authors may want to write each section in sequence. However, best practices indicate the abstract and title should be written last. Authors may find that when writing one section of the report, ideas come to mind that pertains to other sections, so careful note taking is encouraged. One effective approach is to organize and write the result section first, followed by the discussion and conclusions sections. Once these are drafted, write the introduction, abstract, and the title of the report. Regardless of the sequence of writing, the author should begin with a clear and relevant research question to guide the statistical analyses, result interpretation, and discussion. The study findings can be a motivator to propel the author through the writing process, and the conclusions can help the author draft a focused introduction.
Writing for Publication
Specific recommendations on effective medical writing and table generation are available [ 32 ]. One such resource is Effective Medical Writing: The Write Way to Get Published, which is an updated collection of medical writing articles previously published in the Singapore Medical Journal [ 33 ]. The British Medical Journal’s Statistics Notes series also elucidates common and important statistical concepts and usages in clinical studies. Writing guides are also available from individual professional societies, journals, or publishers such as Chest (American College of Physicians) medical writing tips, PLoS Reporting guidelines collection, Springer’s Journal Author Academy, and SAGE’s Research methods [ 34 - 37 ]. Standardized research reporting guidelines often come in the form of checklists and flow diagrams. Table Table6 6 presents a list of reporting guidelines. A full compilation of these guidelines is available at the EQUATOR (Enhancing the QUAlity and Transparency Of health Research) Network website [ 38 ] which aims to improve the reliability and value of medical literature by promoting transparent and accurate reporting of research studies. Publication of the trial protocol in a publicly available database is almost compulsory for publication of the full report in many potential journals.
Graphics and Tables
Graphics and tables should emphasize salient features of the underlying data and should coherently summarize large quantities of information. Although graphics provide a break from dense prose, authors must not forget that these illustrations should be scientifically informative, not decorative. The titles for graphics and tables should be clear, informative, provide the sample size, and use minimal font weight and formatting only to distinguish headings, data entry or to highlight certain results. Provide a consistent number of decimal points for the numerical results, and with no more than four for the P value. Most journals prefer cell-delineated tables created using the table function in word processing or spreadsheet programs. Some journals require specific table formatting such as the absence or presence of intermediate horizontal lines between cells.
Decisions of authorship are both sensitive and important and should be made at an early stage by the study’s stakeholders. Guidelines and journals’ instructions to authors abound with authorship qualifications. The guideline on authorship by the International Committee of Medical Journal Editors is widely known and provides a standard used by many medical and clinical journals [ 39 ]. Generally, authors are those who have made major contributions to the design, conduct, and analysis of the study, and who provided critical readings of the manuscript (if not involved directly in manuscript writing).
Picking a target journal for submission
Once a report has been written and revised, the authors should select a relevant target journal for submission. Authors should avoid predatory journals—publications that do not aim to advance science and disseminate quality research. These journals focus on commercial gain in medical and clinical publishing. Two good resources for authors during journal selection are Think-Check-Submit and the defunct Beall's List of Predatory Publishers and Journals (now archived and maintained by an anonymous third-party) [ 40 , 41 ]. Alternatively, reputable journal indexes such as Thomson Reuters Journal Citation Reports, SCOPUS, MedLine, PubMed, EMBASE, EBSCO Publishing's Electronic Databases are available areas to start the search for an appropriate target journal. Authors should review the journals’ names, aims/scope, and recently published articles to determine the kind of research each journal accepts for publication. Open-access journals almost always charge article publication fees, while subscription-based journals tend to publish without author fees and instead rely on subscription or access fees for the full text of published articles.
Conclusions
Conducting a valid clinical research requires consideration of theoretical study design, data collection design, and statistical analysis design. Proper study design implementation and quality control during data collection ensures high-quality data analysis and can mitigate bias and confounders during statistical analysis and data interpretation. Clear, effective study reporting facilitates dissemination, appreciation, and adoption, and allows the researchers to affect real-world change in clinical practices and care models. Neutral or absence of findings in a clinical study are as important as positive or negative findings. Valid studies, even when they report an absence of expected results, still inform scientific communities of the nature of a certain treatment or intervention, and this contributes to future research, systematic reviews, and meta-analyses. Reporting a study adequately and comprehensively is important for accuracy, transparency, and reproducibility of the scientific work as well as informing readers.
Acknowledgments
The author would like to thank Universiti Putra Malaysia and the Ministry of Higher Education, Malaysia for their support in sponsoring the Ph.D. study and living allowances for Boon-How Chew.
The content published in Cureus is the result of clinical experience and/or research by independent individuals or organizations. Cureus is not responsible for the scientific accuracy or reliability of data or conclusions published herein. All content published within Cureus is intended only for educational, research and reference purposes. Additionally, articles published within Cureus should not be deemed a suitable substitute for the advice of a qualified health care professional. Do not disregard or avoid professional medical advice due to content published within Cureus.
The materials presented in this paper is being organized by the author into a book.

Want to create or adapt books like this? Learn more about how Pressbooks supports open publishing practices.
7.1 Overview of Nonexperimental Research
Learning objectives.
- Define nonexperimental research, distinguish it clearly from experimental research, and give several examples.
- Explain when a researcher might choose to conduct nonexperimental research as opposed to experimental research.
What Is Nonexperimental Research?
Nonexperimental research is research that lacks the manipulation of an independent variable, random assignment of participants to conditions or orders of conditions, or both.
In a sense, it is unfair to define this large and diverse set of approaches collectively by what they are not . But doing so reflects the fact that most researchers in psychology consider the distinction between experimental and nonexperimental research to be an extremely important one. This is because while experimental research can provide strong evidence that changes in an independent variable cause differences in a dependent variable, nonexperimental research generally cannot. As we will see, however, this does not mean that nonexperimental research is less important than experimental research or inferior to it in any general sense.
When to Use Nonexperimental Research
As we saw in Chapter 6 “Experimental Research” , experimental research is appropriate when the researcher has a specific research question or hypothesis about a causal relationship between two variables—and it is possible, feasible, and ethical to manipulate the independent variable and randomly assign participants to conditions or to orders of conditions. It stands to reason, therefore, that nonexperimental research is appropriate—even necessary—when these conditions are not met. There are many ways in which this can be the case.
- The research question or hypothesis can be about a single variable rather than a statistical relationship between two variables (e.g., How accurate are people’s first impressions?).
- The research question can be about a noncausal statistical relationship between variables (e.g., Is there a correlation between verbal intelligence and mathematical intelligence?).
- The research question can be about a causal relationship, but the independent variable cannot be manipulated or participants cannot be randomly assigned to conditions or orders of conditions (e.g., Does damage to a person’s hippocampus impair the formation of long-term memory traces?).
- The research question can be broad and exploratory, or it can be about what it is like to have a particular experience (e.g., What is it like to be a working mother diagnosed with depression?).
Again, the choice between the experimental and nonexperimental approaches is generally dictated by the nature of the research question. If it is about a causal relationship and involves an independent variable that can be manipulated, the experimental approach is typically preferred. Otherwise, the nonexperimental approach is preferred. But the two approaches can also be used to address the same research question in complementary ways. For example, nonexperimental studies establishing that there is a relationship between watching violent television and aggressive behavior have been complemented by experimental studies confirming that the relationship is a causal one (Bushman & Huesmann, 2001). Similarly, after his original study, Milgram conducted experiments to explore the factors that affect obedience. He manipulated several independent variables, such as the distance between the experimenter and the participant, the participant and the confederate, and the location of the study (Milgram, 1974).
Types of Nonexperimental Research
Nonexperimental research falls into three broad categories: single-variable research, correlational and quasi-experimental research, and qualitative research. First, research can be nonexperimental because it focuses on a single variable rather than a statistical relationship between two variables. Although there is no widely shared term for this kind of research, we will call it single-variable research . Milgram’s original obedience study was nonexperimental in this way. He was primarily interested in one variable—the extent to which participants obeyed the researcher when he told them to shock the confederate—and he observed all participants performing the same task under the same conditions. The study by Loftus and Pickrell described at the beginning of this chapter is also a good example of single-variable research. The variable was whether participants “remembered” having experienced mildly traumatic childhood events (e.g., getting lost in a shopping mall) that they had not actually experienced but that the research asked them about repeatedly. In this particular study, nearly a third of the participants “remembered” at least one event. (As with Milgram’s original study, this study inspired several later experiments on the factors that affect false memories.)
As these examples make clear, single-variable research can answer interesting and important questions. What it cannot do, however, is answer questions about statistical relationships between variables. This is a point that beginning researchers sometimes miss. Imagine, for example, a group of research methods students interested in the relationship between children’s being the victim of bullying and the children’s self-esteem. The first thing that is likely to occur to these researchers is to obtain a sample of middle-school students who have been bullied and then to measure their self-esteem. But this would be a single-variable study with self-esteem as the only variable. Although it would tell the researchers something about the self-esteem of children who have been bullied, it would not tell them what they really want to know, which is how the self-esteem of children who have been bullied compares with the self-esteem of children who have not. Is it lower? Is it the same? Could it even be higher? To answer this question, their sample would also have to include middle-school students who have not been bullied.
Research can also be nonexperimental because it focuses on a statistical relationship between two variables but does not include the manipulation of an independent variable, random assignment of participants to conditions or orders of conditions, or both. This kind of research takes two basic forms: correlational research and quasi-experimental research. In correlational research , the researcher measures the two variables of interest with little or no attempt to control extraneous variables and then assesses the relationship between them. A research methods student who finds out whether each of several middle-school students has been bullied and then measures each student’s self-esteem is conducting correlational research. In quasi-experimental research , the researcher manipulates an independent variable but does not randomly assign participants to conditions or orders of conditions. For example, a researcher might start an antibullying program (a kind of treatment) at one school and compare the incidence of bullying at that school with the incidence at a similar school that has no antibullying program.
The final way in which research can be nonexperimental is that it can be qualitative. The types of research we have discussed so far are all quantitative, referring to the fact that the data consist of numbers that are analyzed using statistical techniques. In qualitative research , the data are usually nonnumerical and are analyzed using nonstatistical techniques. Rosenhan’s study of the experience of people in a psychiatric ward was primarily qualitative. The data were the notes taken by the “pseudopatients”—the people pretending to have heard voices—along with their hospital records. Rosenhan’s analysis consists mainly of a written description of the experiences of the pseudopatients, supported by several concrete examples. To illustrate the hospital staff’s tendency to “depersonalize” their patients, he noted, “Upon being admitted, I and other pseudopatients took the initial physical examinations in a semipublic room, where staff members went about their own business as if we were not there” (Rosenhan, 1973, p. 256).
Internal Validity Revisited
Recall that internal validity is the extent to which the design of a study supports the conclusion that changes in the independent variable caused any observed differences in the dependent variable. Figure 7.1 shows how experimental, quasi-experimental, and correlational research vary in terms of internal validity. Experimental research tends to be highest because it addresses the directionality and third-variable problems through manipulation and the control of extraneous variables through random assignment. If the average score on the dependent variable in an experiment differs across conditions, it is quite likely that the independent variable is responsible for that difference. Correlational research is lowest because it fails to address either problem. If the average score on the dependent variable differs across levels of the independent variable, it could be that the independent variable is responsible, but there are other interpretations. In some situations, the direction of causality could be reversed. In others, there could be a third variable that is causing differences in both the independent and dependent variables. Quasi-experimental research is in the middle because the manipulation of the independent variable addresses some problems, but the lack of random assignment and experimental control fails to address others. Imagine, for example, that a researcher finds two similar schools, starts an antibullying program in one, and then finds fewer bullying incidents in that “treatment school” than in the “control school.” There is no directionality problem because clearly the number of bullying incidents did not determine which school got the program. However, the lack of random assignment of children to schools could still mean that students in the treatment school differed from students in the control school in some other way that could explain the difference in bullying.

Experiments are generally high in internal validity, quasi-experiments lower, and correlational studies lower still.
Notice also in Figure 7.1 that there is some overlap in the internal validity of experiments, quasi-experiments, and correlational studies. For example, a poorly designed experiment that includes many confounding variables can be lower in internal validity than a well designed quasi-experiment with no obvious confounding variables.
Key Takeaways
- Nonexperimental research is research that lacks the manipulation of an independent variable, control of extraneous variables through random assignment, or both.
- There are three broad types of nonexperimental research. Single-variable research focuses on a single variable rather than a relationship between variables. Correlational and quasi-experimental research focus on a statistical relationship but lack manipulation or random assignment. Qualitative research focuses on broader research questions, typically involves collecting large amounts of data from a small number of participants, and analyzes the data nonstatistically.
- In general, experimental research is high in internal validity, correlational research is low in internal validity, and quasi-experimental research is in between.
Discussion: For each of the following studies, decide which type of research design it is and explain why.
- A researcher conducts detailed interviews with unmarried teenage fathers to learn about how they feel and what they think about their role as fathers and summarizes their feelings in a written narrative.
- A researcher measures the impulsivity of a large sample of drivers and looks at the statistical relationship between this variable and the number of traffic tickets the drivers have received.
- A researcher randomly assigns patients with low back pain either to a treatment involving hypnosis or to a treatment involving exercise. She then measures their level of low back pain after 3 months.
- A college instructor gives weekly quizzes to students in one section of his course but no weekly quizzes to students in another section to see whether this has an effect on their test performance.
Bushman, B. J., & Huesmann, L. R. (2001). Effects of televised violence on aggression. In D. Singer & J. Singer (Eds.), Handbook of children and the media (pp. 223–254). Thousand Oaks, CA: Sage.
Milgram, S. (1974). Obedience to authority: An experimental view . New York, NY: Harper & Row.
Rosenhan, D. L. (1973). On being sane in insane places. Science, 179 , 250–258.
Research Methods in Psychology Copyright © 2016 by University of Minnesota is licensed under a Creative Commons Attribution-NonCommercial-ShareAlike 4.0 International License , except where otherwise noted.
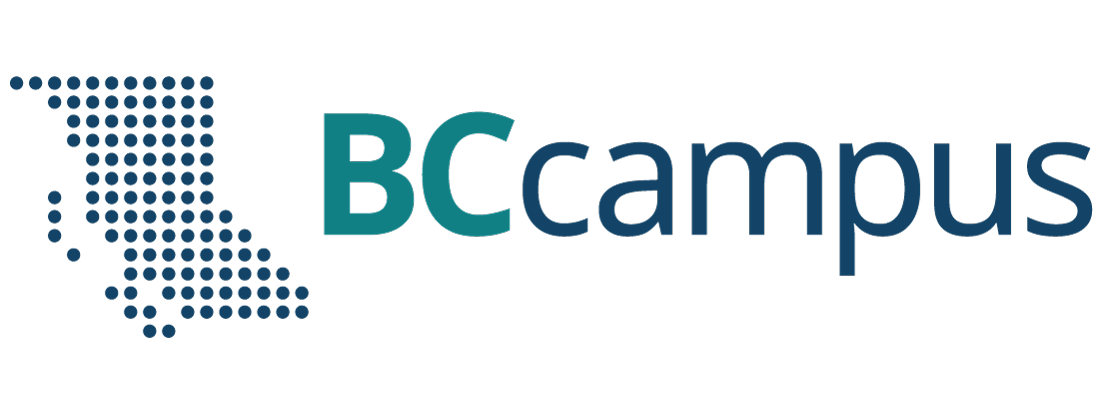
Want to create or adapt books like this? Learn more about how Pressbooks supports open publishing practices.
Chapter 7: Nonexperimental Research
Overview of Nonexperimental Research
Learning Objectives
- Define nonexperimental research, distinguish it clearly from experimental research, and give several examples.
- Explain when a researcher might choose to conduct nonexperimental research as opposed to experimental research.
What Is Nonexperimental Research?
Nonexperimental research is research that lacks the manipulation of an independent variable, random assignment of participants to conditions or orders of conditions, or both.
In a sense, it is unfair to define this large and diverse set of approaches collectively by what they are not . But doing so reflects the fact that most researchers in psychology consider the distinction between experimental and nonexperimental research to be an extremely important one. This distinction is because although experimental research can provide strong evidence that changes in an independent variable cause differences in a dependent variable, nonexperimental research generally cannot. As we will see, however, this inability does not mean that nonexperimental research is less important than experimental research or inferior to it in any general sense.
When to Use Nonexperimental Research
As we saw in Chapter 6 , experimental research is appropriate when the researcher has a specific research question or hypothesis about a causal relationship between two variables—and it is possible, feasible, and ethical to manipulate the independent variable and randomly assign participants to conditions or to orders of conditions. It stands to reason, therefore, that nonexperimental research is appropriate—even necessary—when these conditions are not met. There are many ways in which preferring nonexperimental research can be the case.
- The research question or hypothesis can be about a single variable rather than a statistical relationship between two variables (e.g., How accurate are people’s first impressions?).
- The research question can be about a noncausal statistical relationship between variables (e.g., Is there a correlation between verbal intelligence and mathematical intelligence?).
- The research question can be about a causal relationship, but the independent variable cannot be manipulated or participants cannot be randomly assigned to conditions or orders of conditions (e.g., Does damage to a person’s hippocampus impair the formation of long-term memory traces?).
- The research question can be broad and exploratory, or it can be about what it is like to have a particular experience (e.g., What is it like to be a working mother diagnosed with depression?).
Again, the choice between the experimental and nonexperimental approaches is generally dictated by the nature of the research question. If it is about a causal relationship and involves an independent variable that can be manipulated, the experimental approach is typically preferred. Otherwise, the nonexperimental approach is preferred. But the two approaches can also be used to address the same research question in complementary ways. For example, nonexperimental studies establishing that there is a relationship between watching violent television and aggressive behaviour have been complemented by experimental studies confirming that the relationship is a causal one (Bushman & Huesmann, 2001) [1] . Similarly, after his original study, Milgram conducted experiments to explore the factors that affect obedience. He manipulated several independent variables, such as the distance between the experimenter and the participant, the participant and the confederate, and the location of the study (Milgram, 1974) [2] .
Types of Nonexperimental Research
Nonexperimental research falls into three broad categories: single-variable research, correlational and quasi-experimental research, and qualitative research. First, research can be nonexperimental because it focuses on a single variable rather than a statistical relationship between two variables. Although there is no widely shared term for this kind of research, we will call it single-variable research . Milgram’s original obedience study was nonexperimental in this way. He was primarily interested in one variable—the extent to which participants obeyed the researcher when he told them to shock the confederate—and he observed all participants performing the same task under the same conditions. The study by Loftus and Pickrell described at the beginning of this chapter is also a good example of single-variable research. The variable was whether participants “remembered” having experienced mildly traumatic childhood events (e.g., getting lost in a shopping mall) that they had not actually experienced but that the research asked them about repeatedly. In this particular study, nearly a third of the participants “remembered” at least one event. (As with Milgram’s original study, this study inspired several later experiments on the factors that affect false memories.)
As these examples make clear, single-variable research can answer interesting and important questions. What it cannot do, however, is answer questions about statistical relationships between variables. This detail is a point that beginning researchers sometimes miss. Imagine, for example, a group of research methods students interested in the relationship between children’s being the victim of bullying and the children’s self-esteem. The first thing that is likely to occur to these researchers is to obtain a sample of middle-school students who have been bullied and then to measure their self-esteem. But this design would be a single-variable study with self-esteem as the only variable. Although it would tell the researchers something about the self-esteem of children who have been bullied, it would not tell them what they really want to know, which is how the self-esteem of children who have been bullied compares with the self-esteem of children who have not. Is it lower? Is it the same? Could it even be higher? To answer this question, their sample would also have to include middle-school students who have not been bullied thereby introducing another variable.
Research can also be nonexperimental because it focuses on a statistical relationship between two variables but does not include the manipulation of an independent variable, random assignment of participants to conditions or orders of conditions, or both. This kind of research takes two basic forms: correlational research and quasi-experimental research. In correlational research , the researcher measures the two variables of interest with little or no attempt to control extraneous variables and then assesses the relationship between them. A research methods student who finds out whether each of several middle-school students has been bullied and then measures each student’s self-esteem is conducting correlational research. In quasi-experimental research , the researcher manipulates an independent variable but does not randomly assign participants to conditions or orders of conditions. For example, a researcher might start an antibullying program (a kind of treatment) at one school and compare the incidence of bullying at that school with the incidence at a similar school that has no antibullying program.
The final way in which research can be nonexperimental is that it can be qualitative. The types of research we have discussed so far are all quantitative, referring to the fact that the data consist of numbers that are analyzed using statistical techniques. In qualitative research , the data are usually nonnumerical and therefore cannot be analyzed using statistical techniques. Rosenhan’s study of the experience of people in a psychiatric ward was primarily qualitative. The data were the notes taken by the “pseudopatients”—the people pretending to have heard voices—along with their hospital records. Rosenhan’s analysis consists mainly of a written description of the experiences of the pseudopatients, supported by several concrete examples. To illustrate the hospital staff’s tendency to “depersonalize” their patients, he noted, “Upon being admitted, I and other pseudopatients took the initial physical examinations in a semipublic room, where staff members went about their own business as if we were not there” (Rosenhan, 1973, p. 256). [3] Qualitative data has a separate set of analysis tools depending on the research question. For example, thematic analysis would focus on themes that emerge in the data or conversation analysis would focus on the way the words were said in an interview or focus group.
Internal Validity Revisited
Recall that internal validity is the extent to which the design of a study supports the conclusion that changes in the independent variable caused any observed differences in the dependent variable. Figure 7.1 shows how experimental, quasi-experimental, and correlational research vary in terms of internal validity. Experimental research tends to be highest because it addresses the directionality and third-variable problems through manipulation and the control of extraneous variables through random assignment. If the average score on the dependent variable in an experiment differs across conditions, it is quite likely that the independent variable is responsible for that difference. Correlational research is lowest because it fails to address either problem. If the average score on the dependent variable differs across levels of the independent variable, it could be that the independent variable is responsible, but there are other interpretations. In some situations, the direction of causality could be reversed. In others, there could be a third variable that is causing differences in both the independent and dependent variables. Quasi-experimental research is in the middle because the manipulation of the independent variable addresses some problems, but the lack of random assignment and experimental control fails to address others. Imagine, for example, that a researcher finds two similar schools, starts an antibullying program in one, and then finds fewer bullying incidents in that “treatment school” than in the “control school.” There is no directionality problem because clearly the number of bullying incidents did not determine which school got the program. However, the lack of random assignment of children to schools could still mean that students in the treatment school differed from students in the control school in some other way that could explain the difference in bullying.

Notice also in Figure 7.1 that there is some overlap in the internal validity of experiments, quasi-experiments, and correlational studies. For example, a poorly designed experiment that includes many confounding variables can be lower in internal validity than a well designed quasi-experiment with no obvious confounding variables. Internal validity is also only one of several validities that one might consider, as noted in Chapter 5.
Key Takeaways
- Nonexperimental research is research that lacks the manipulation of an independent variable, control of extraneous variables through random assignment, or both.
- There are three broad types of nonexperimental research. Single-variable research focuses on a single variable rather than a relationship between variables. Correlational and quasi-experimental research focus on a statistical relationship but lack manipulation or random assignment. Qualitative research focuses on broader research questions, typically involves collecting large amounts of data from a small number of participants, and analyses the data nonstatistically.
- In general, experimental research is high in internal validity, correlational research is low in internal validity, and quasi-experimental research is in between.
Discussion: For each of the following studies, decide which type of research design it is and explain why.
- A researcher conducts detailed interviews with unmarried teenage fathers to learn about how they feel and what they think about their role as fathers and summarizes their feelings in a written narrative.
- A researcher measures the impulsivity of a large sample of drivers and looks at the statistical relationship between this variable and the number of traffic tickets the drivers have received.
- A researcher randomly assigns patients with low back pain either to a treatment involving hypnosis or to a treatment involving exercise. She then measures their level of low back pain after 3 months.
- A college instructor gives weekly quizzes to students in one section of his course but no weekly quizzes to students in another section to see whether this has an effect on their test performance.
- Bushman, B. J., & Huesmann, L. R. (2001). Effects of televised violence on aggression. In D. Singer & J. Singer (Eds.), Handbook of children and the media (pp. 223–254). Thousand Oaks, CA: Sage. ↵
- Milgram, S. (1974). Obedience to authority: An experimental view . New York, NY: Harper & Row. ↵
- Rosenhan, D. L. (1973). On being sane in insane places. Science, 179 , 250–258. ↵
Research that lacks the manipulation of an independent variable, random assignment of participants to conditions or orders of conditions, or both.
Research that focuses on a single variable rather than a statistical relationship between two variables.
The researcher measures the two variables of interest with little or no attempt to control extraneous variables and then assesses the relationship between them.
The researcher manipulates an independent variable but does not randomly assign participants to conditions or orders of conditions.
Research Methods in Psychology - 2nd Canadian Edition Copyright © 2015 by Paul C. Price, Rajiv Jhangiani, & I-Chant A. Chiang is licensed under a Creative Commons Attribution-NonCommercial-ShareAlike 4.0 International License , except where otherwise noted.
Share This Book
- Skip to main content
- Skip to primary sidebar
- Skip to footer
- QuestionPro

- Solutions Industries Gaming Automotive Sports and events Education Government Travel & Hospitality Financial Services Healthcare Cannabis Technology Use Case NPS+ Communities Audience Contactless surveys Mobile LivePolls Member Experience GDPR Positive People Science 360 Feedback Surveys
- Resources Blog eBooks Survey Templates Case Studies Training Help center

Home Investigación de mercado
Non-experimental research: What it is, overview & advantages
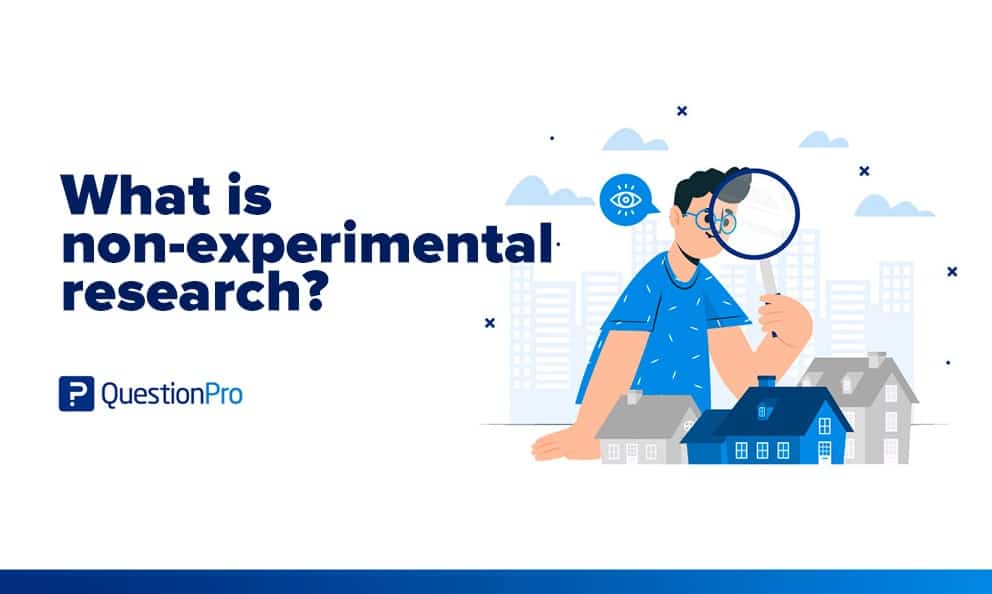
Non-experimental research is the type of research that lacks an independent variable. Instead, the researcher observes the context in which the phenomenon occurs and analyzes it to obtain information.
Unlike experimental research , where the variables are held constant, non-experimental research happens during the study when the researcher cannot control, manipulate or alter the subjects but relies on interpretation or observations to conclude.
This means that the method must not rely on correlations, surveys , or case studies and cannot demonstrate an actual cause and effect relationship.
Characteristics of non-experimental research
Some of the essential characteristics of non-experimental research are necessary for the final results. Let’s talk about them to identify the most critical parts of them.
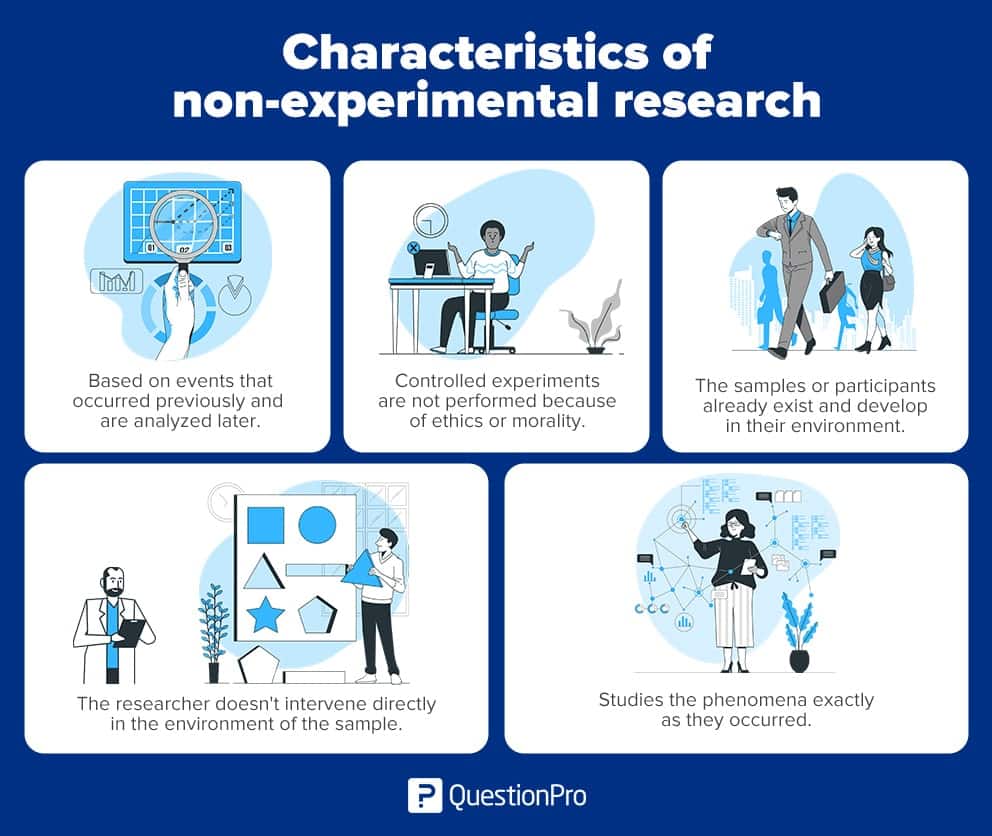
- Most studies are based on events that occurred previously and are analyzed later.
- In this method, controlled experiments are not performed for reasons such as ethics or morality.
- No study samples are created; on the contrary, the samples or participants already exist and develop in their environment.
- The researcher does not intervene directly in the environment of the sample.
- This method studies the phenomena exactly as they occurred.
Types of non-experimental research
Non-experimental research can take the following forms:
Cross-sectional research : Cross-sectional research is used to observe and analyze the exact time of the research to cover various study groups or samples. This type of research is divided into:
- Descriptive: When values are observed where one or more variables are presented.
- Causal: It is responsible for explaining the reasons and relationship that exists between variables in a given time.
Longitudinal research: In a longitudinal study , researchers aim to analyze the changes and development of the relationships between variables over time. Longitudinal research can be divided into:
- Trend: When they study the changes faced by the study group in general.
- Group evolution: When the study group is a smaller sample.
- Panel: It is in charge of analyzing individual and group changes to discover the factor that produces them.
LEARN ABOUT: Quasi-experimental Research
When to use non-experimental research
Non-experimental research can be applied in the following ways:
- When the research question may be about one variable rather than a statistical relationship about two variables.
- There is a non-causal statistical relationship between variables in the research question.
- The research question has a causal research relationship, but the independent variable cannot be manipulated.
- In exploratory or broad research where a particular experience is confronted.
Advantages and disadvantages
Some advantages of non-experimental research are:
- It is very flexible during the research process
- The cause of the phenomenon is known, and the effect it has is investigated.
- The researcher can define the characteristics of the study group.
Among the disadvantages of non-experimental research are:
- The groups are not representative of the entire population.
- Errors in the methodology may occur, leading to research biases .
Non-experimental research is based on the observation of phenomena in their natural environment. In this way, they can be studied later to reach a conclusion.
Difference between experimental and non-experimental research
Experimental research involves changing variables and randomly assigning conditions to participants. As it can determine the cause, experimental research designs are used for research in medicine, biology, and social science.
Experimental research designs have strict standards for control and establishing validity. Although they may need many resources, they can lead to very interesting results.
Non-experimental research, on the other hand, is usually descriptive or correlational without any explicit changes done by the researcher. You simply describe the situation as it is, or describe a relationship between variables. Without any control, it is difficult to determine causal effects. The validity remains a concern in this type of research. However, it’s’ more regarding the measurements instead of the effects.
LEARN MORE: Descriptive Research vs Correlational Research
Whether you should choose experimental research or non-experimental research design depends on your goals and resources. If you need any help with how to conduct research and collect relevant data, or have queries regarding the best approach for your research goals, contact us today! You can create an account with our survey software and avail of 88+ features including dashboard and reporting for free.
Create a free account
MORE LIKE THIS
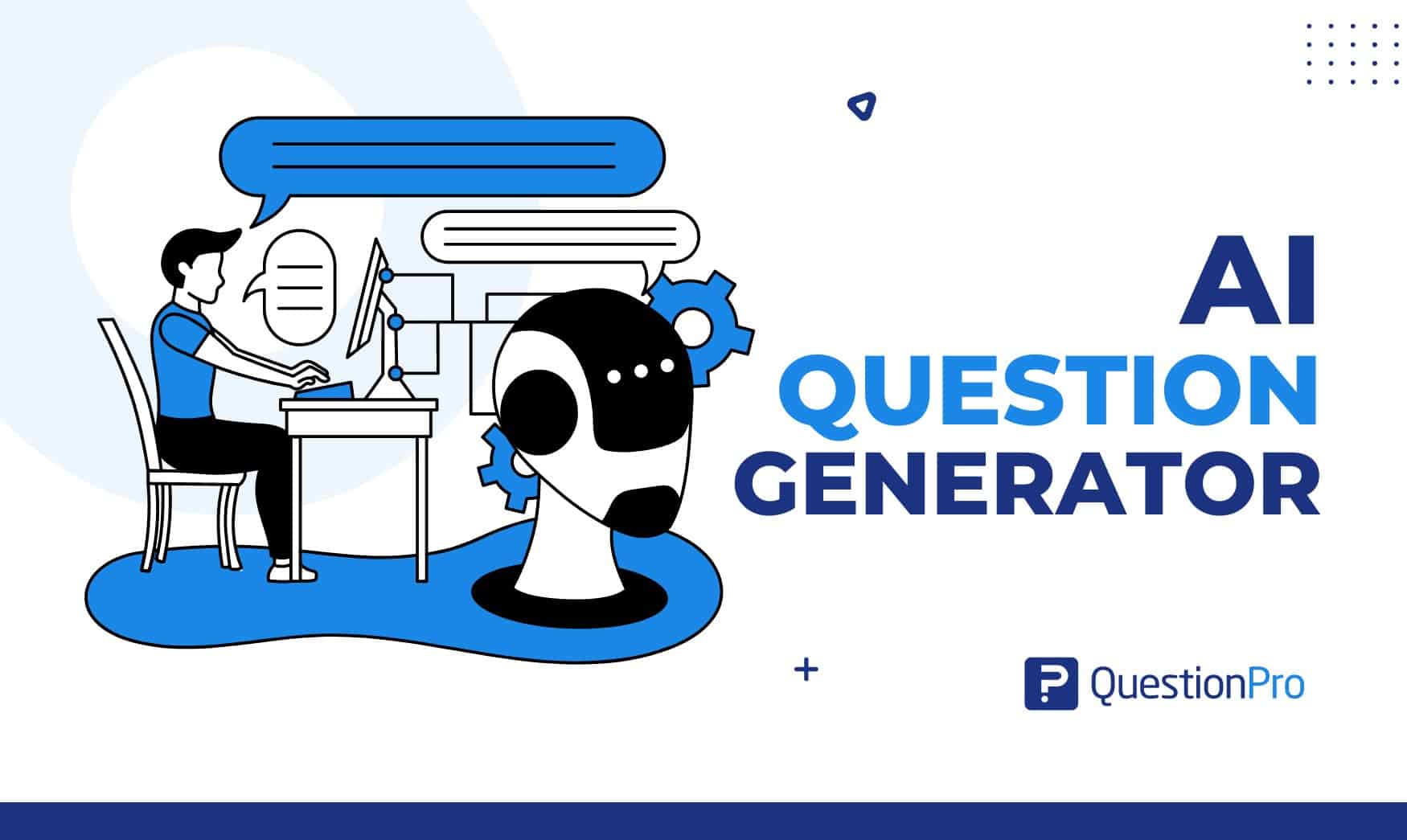
AI Question Generator: Create Easy + Accurate Tests and Surveys
Apr 6, 2024
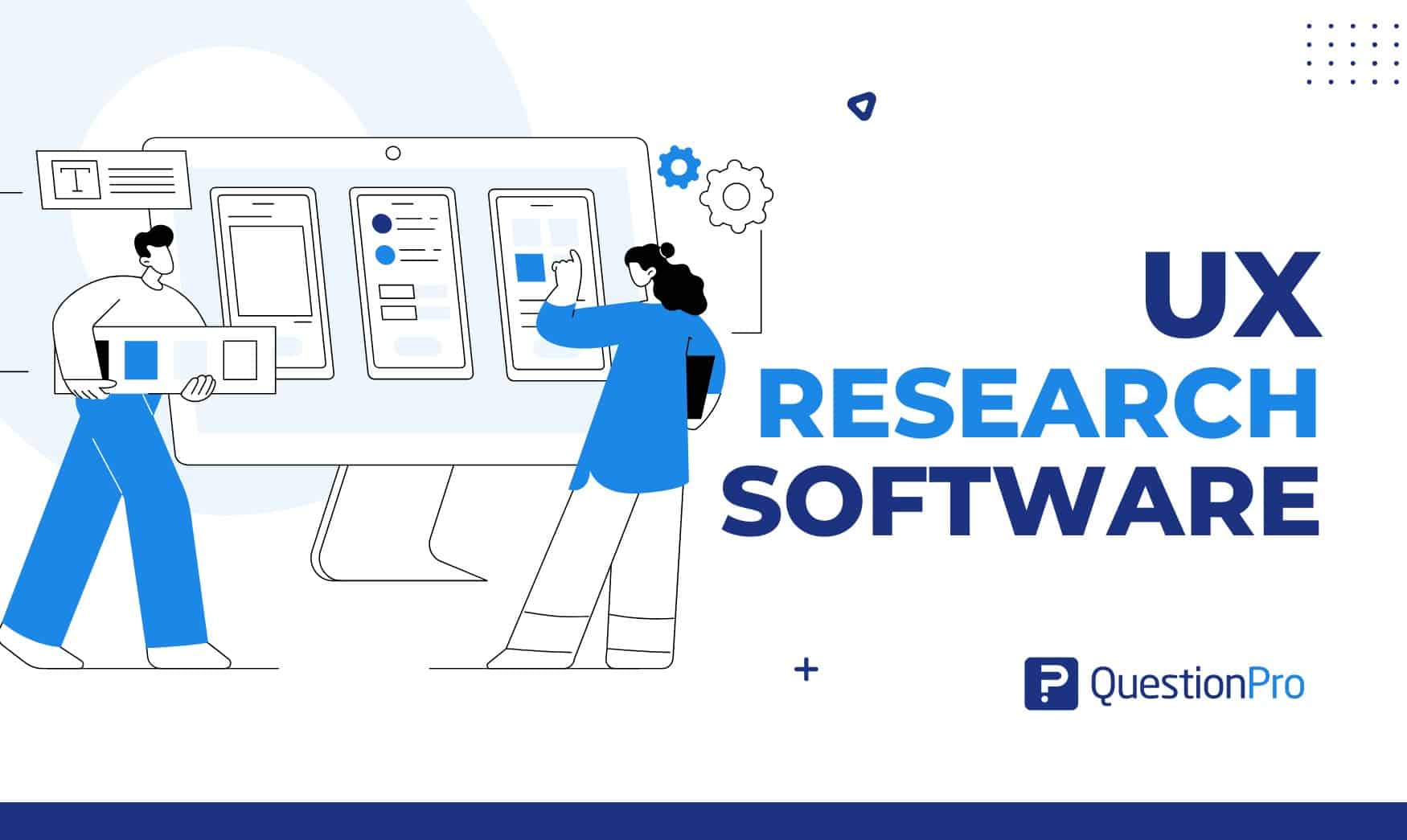
Top 17 UX Research Software for UX Design in 2024
Apr 5, 2024
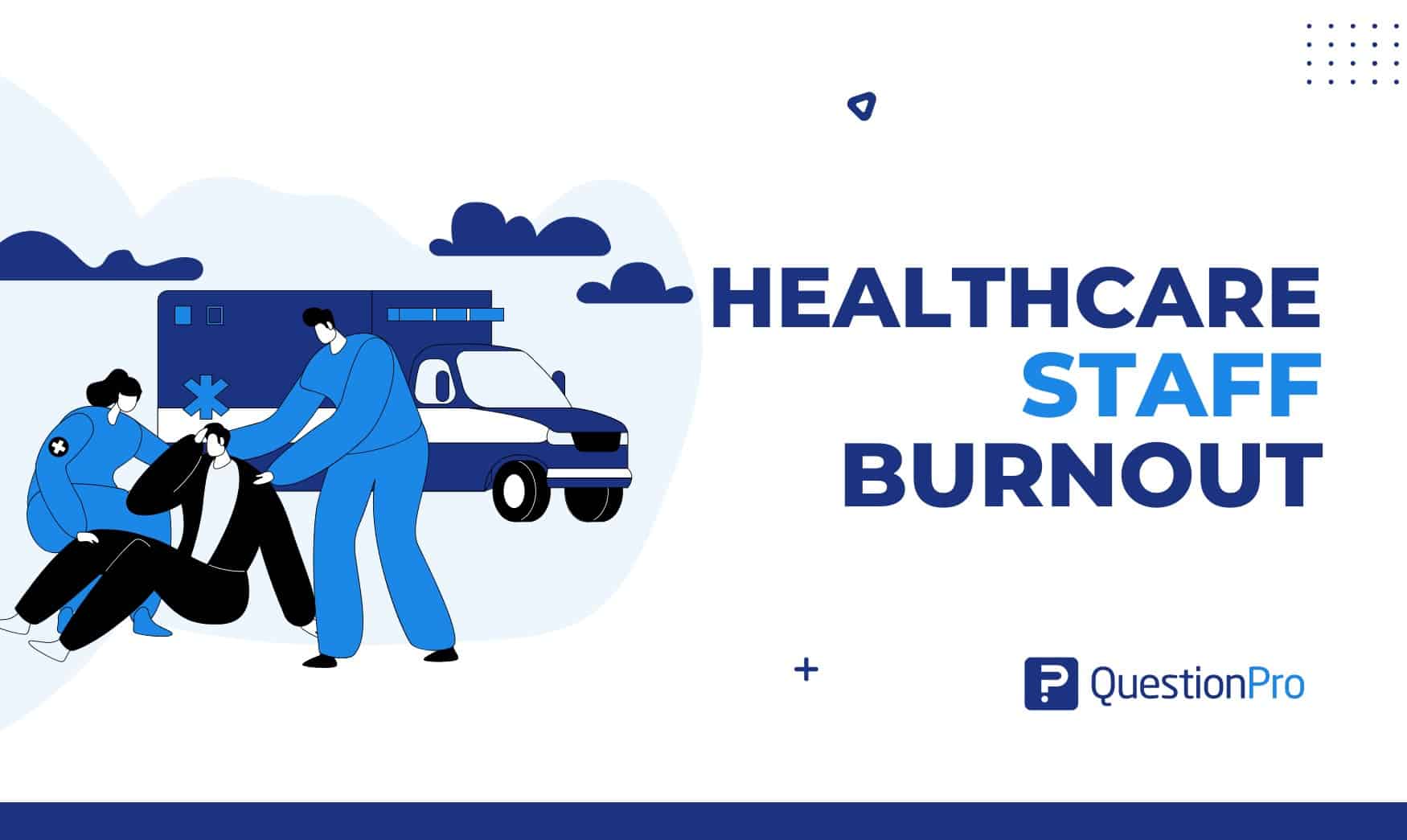
Healthcare Staff Burnout: What it Is + How To Manage It
Apr 4, 2024
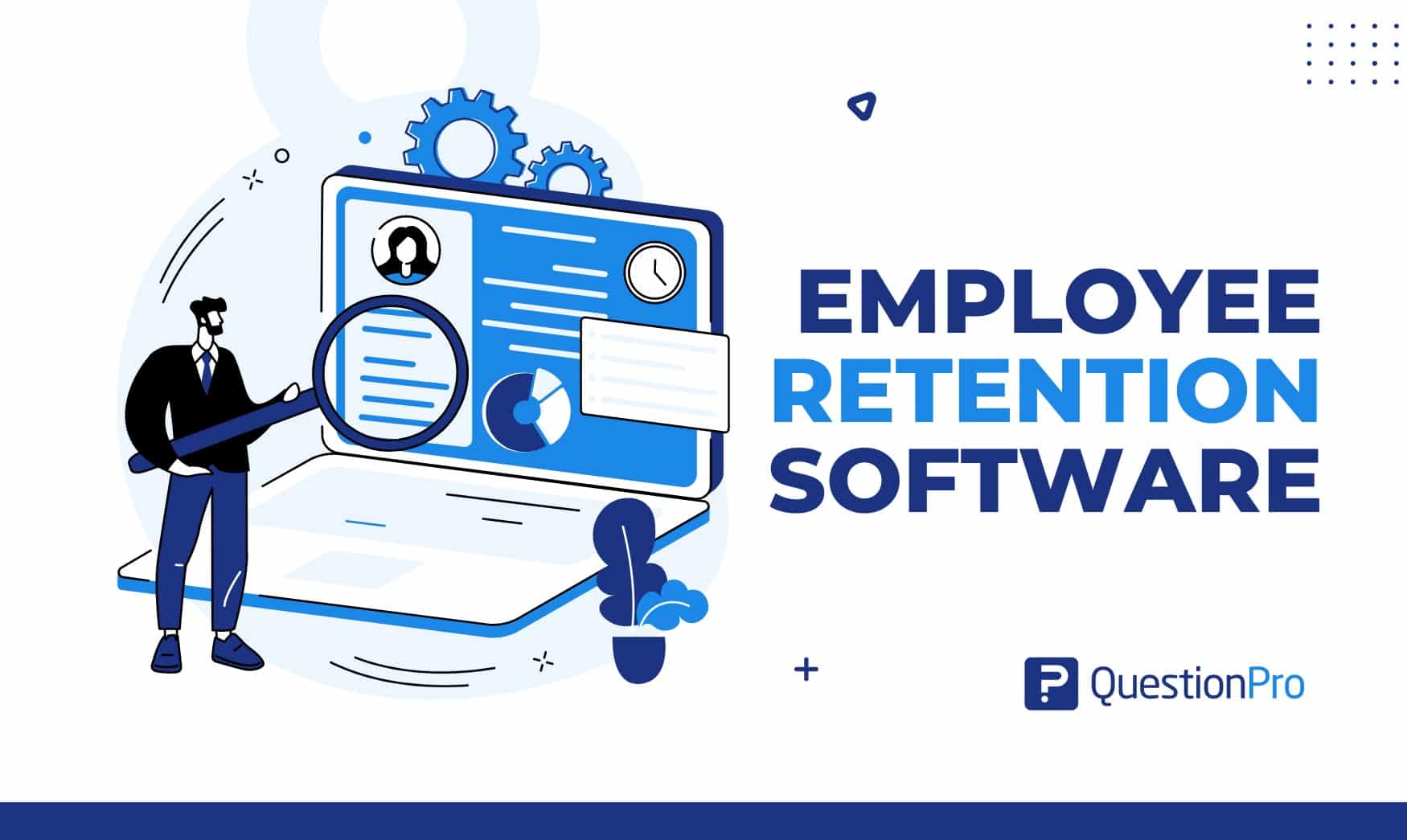
Top 15 Employee Retention Software in 2024
Other categories.
- Academic Research
- Artificial Intelligence
- Assessments
- Brand Awareness
- Case Studies
- Communities
- Consumer Insights
- Customer effort score
- Customer Engagement
- Customer Experience
- Customer Loyalty
- Customer Research
- Customer Satisfaction
- Employee Benefits
- Employee Engagement
- Employee Retention
- Friday Five
- General Data Protection Regulation
- Insights Hub
- Life@QuestionPro
- Market Research
- Mobile diaries
- Mobile Surveys
- New Features
- Online Communities
- Question Types
- Questionnaire
- QuestionPro Products
- Release Notes
- Research Tools and Apps
- Revenue at Risk
- Survey Templates
- Training Tips
- Uncategorized
- Video Learning Series
- What’s Coming Up
- Workforce Intelligence
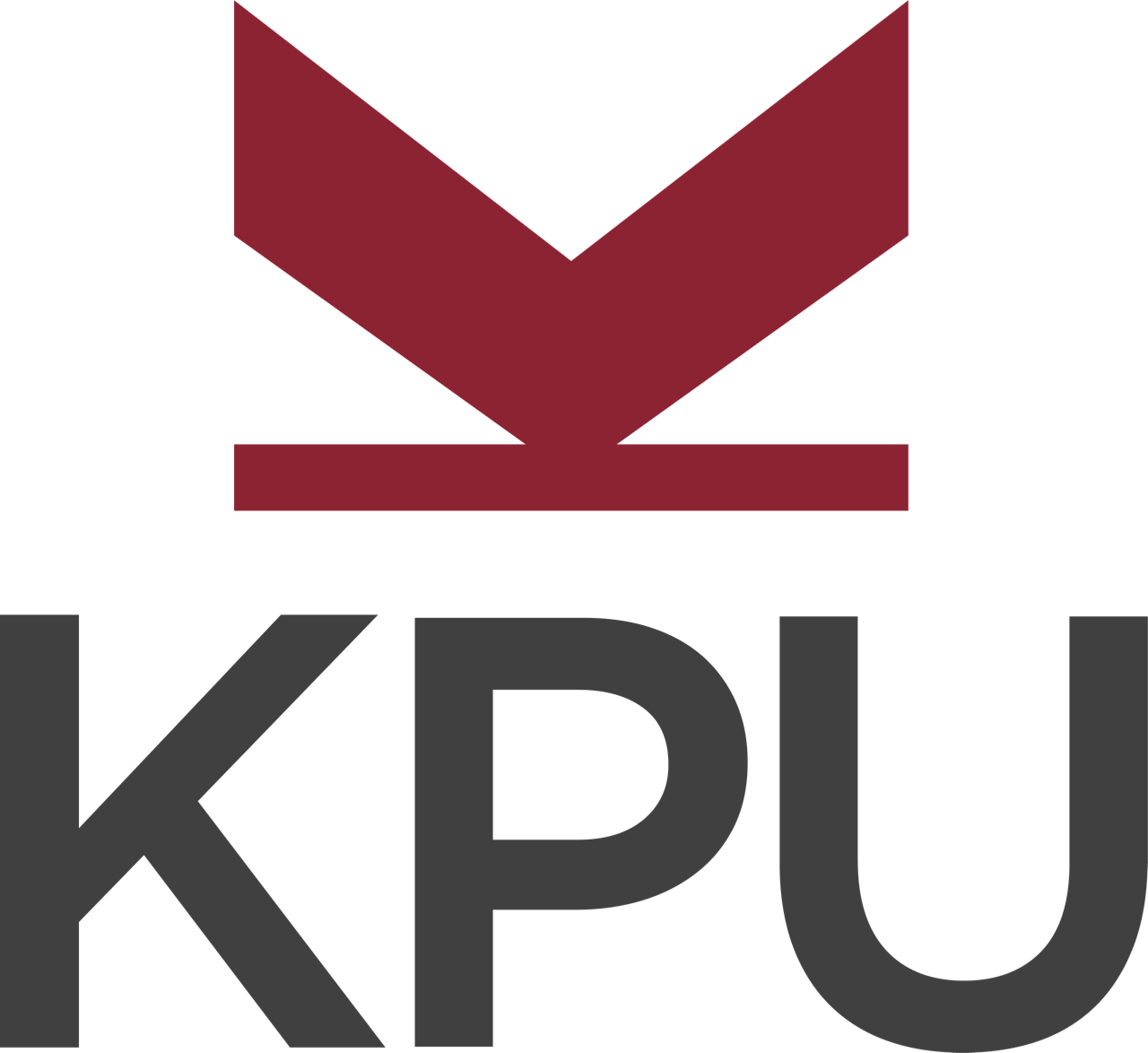
Want to create or adapt books like this? Learn more about how Pressbooks supports open publishing practices.
Non-Experimental Research
What do the following classic studies have in common?
- Stanley Milgram found that about two thirds of his research participants were willing to administer dangerous shocks to another person just because they were told to by an authority figure (Milgram, 1963) [1] .
- Elizabeth Loftus and Jacqueline Pickrell showed that it is relatively easy to “implant” false memories in people by repeatedly asking them about childhood events that did not actually happen to them (Loftus & Pickrell, 1995) [2] .
- John Cacioppo and Richard Petty evaluated the validity of their Need for Cognition Scale—a measure of the extent to which people like and value thinking—by comparing the scores of university professors with those of factory workers (Cacioppo & Petty, 1982) [3] .
- David Rosenhan found that confederates who went to psychiatric hospitals claiming to have heard voices saying things like “empty” and “thud” were labeled as schizophrenic by the hospital staff and kept there even though they behaved normally in all other ways (Rosenhan, 1973) [4] .
The answer for purposes of this chapter is that they are not experiments. In this chapter, we look more closely at non-experimental research. We begin with a general definition of non-experimental research, along with a discussion of when and why non-experimental research is more appropriate than experimental research. We then look separately at two important types of non-experimental research: correlational research and observational research.
- Milgram, S. (1963). Behavioral study of obedience. Journal of Abnormal and Social Psychology, 67 , 371–378. ↵
- Loftus, E. F., & Pickrell, J. E. (1995). The formation of false memories. Psychiatric Annals, 25 , 720–725. ↵
- Cacioppo, J. T., & Petty, R. E. (1982). The need for cognition. Journal of Personality and Social Psychology, 42 , 116–131. ↵
- Rosenhan, D. L. (1973). On being sane in insane places. Science, 179 , 250–258. ↵
Research Methods in Psychology Copyright © 2019 by Rajiv S. Jhangiani, I-Chant A. Chiang, Carrie Cuttler, & Dana C. Leighton is licensed under a Creative Commons Attribution-NonCommercial-ShareAlike 4.0 International License , except where otherwise noted.
Share This Book

Want to create or adapt books like this? Learn more about how Pressbooks supports open publishing practices.
Overview of Non-Experimental Research
Rajiv S. Jhangiani; I-Chant A. Chiang; Carrie Cuttler; and Dana C. Leighton
Learning Objectives
- Define non-experimental research, distinguish it clearly from experimental research, and give several examples.
- Explain when a researcher might choose to conduct non-experimental research as opposed to experimental research.
What Is Non-Experimental Research?
Non-experimental research is research that lacks the manipulation of an independent variable. Rather than manipulating an independent variable, researchers conducting non-experimental research simply measure variables as they naturally occur (in the lab or real world).
Most researchers in psychology consider the distinction between experimental and non-experimental research to be an extremely important one. This is because although experimental research can provide strong evidence that changes in an independent variable cause differences in a dependent variable, non-experimental research generally cannot. As we will see, however, this inability to make causal conclusions does not mean that non-experimental research is less important than experimental research. It is simply used in cases where experimental research is not able to be carried out.
When to Use Non-Experimental Research
As we saw in the last chapter , experimental research is appropriate when the researcher has a specific research question or hypothesis about a causal relationship between two variables—and it is possible, feasible, and ethical to manipulate the independent variable. It stands to reason, therefore, that non-experimental research is appropriate—even necessary—when these conditions are not met. There are many times in which non-experimental research is preferred, including when:
- the research question or hypothesis relates to a single variable rather than a statistical relationship between two variables (e.g., how accurate are people’s first impressions?).
- the research question pertains to a non-causal statistical relationship between variables (e.g., is there a correlation between verbal intelligence and mathematical intelligence?).
- the research question is about a causal relationship, but the independent variable cannot be manipulated or participants cannot be randomly assigned to conditions or orders of conditions for practical or ethical reasons (e.g., does damage to a person’s hippocampus impair the formation of long-term memory traces?).
- the research question is broad and exploratory, or is about what it is like to have a particular experience (e.g., what is it like to be a working mother diagnosed with depression?).
Again, the choice between the experimental and non-experimental approaches is generally dictated by the nature of the research question. Recall the three goals of science are to describe, to predict, and to explain. If the goal is to explain and the research question pertains to causal relationships, then the experimental approach is typically preferred. If the goal is to describe or to predict, a non-experimental approach is appropriate. But the two approaches can also be used to address the same research question in complementary ways. For example, in Milgram’s original (non-experimental) obedience study, he was primarily interested in one variable—the extent to which participants obeyed the researcher when he told them to shock the confederate—and he observed all participants performing the same task under the same conditions. However, Milgram subsequently conducted experiments to explore the factors that affect obedience. He manipulated several independent variables, such as the distance between the experimenter and the participant, the participant and the confederate, and the location of the study (Milgram, 1974) [1] .
Types of Non-Experimental Research
Non-experimental research falls into two broad categories: correlational research and observational research.
The most common type of non-experimental research conducted in psychology is correlational research. Correlational research is considered non-experimental because it focuses on the statistical relationship between two variables but does not include the manipulation of an independent variable. More specifically, in correlational research , the researcher measures two variables with little or no attempt to control extraneous variables and then assesses the relationship between them. As an example, a researcher interested in the relationship between self-esteem and school achievement could collect data on students’ self-esteem and their GPAs to see if the two variables are statistically related.
Observational research is non-experimental because it focuses on making observations of behavior in a natural or laboratory setting without manipulating anything. Milgram’s original obedience study was non-experimental in this way. He was primarily interested in the extent to which participants obeyed the researcher when he told them to shock the confederate and he observed all participants performing the same task under the same conditions. The study by Loftus and Pickrell described at the beginning of this chapter is also a good example of observational research. The variable was whether participants “remembered” having experienced mildly traumatic childhood events (e.g., getting lost in a shopping mall) that they had not actually experienced but that the researchers asked them about repeatedly. In this particular study, nearly a third of the participants “remembered” at least one event. (As with Milgram’s original study, this study inspired several later experiments on the factors that affect false memories).
Cross-Sectional, Longitudinal, and Cross-Sequential Studies
When psychologists wish to study change over time (for example, when developmental psychologists wish to study aging) they usually take one of three non-experimental approaches: cross-sectional, longitudinal, or cross-sequential. Cross-sectional studies involve comparing two or more pre-existing groups of people (e.g., children at different stages of development). What makes this approach non-experimental is that there is no manipulation of an independent variable and no random assignment of participants to groups. Using this design, developmental psychologists compare groups of people of different ages (e.g., young adults spanning from 18-25 years of age versus older adults spanning 60-75 years of age) on various dependent variables (e.g., memory, depression, life satisfaction). Of course, the primary limitation of using this design to study the effects of aging is that differences between the groups other than age may account for differences in the dependent variable. For instance, differences between the groups may reflect the generation that people come from (a cohort effect ) rather than a direct effect of age. For this reason, longitudinal studies , in which one group of people is followed over time as they age, offer a superior means of studying the effects of aging. However, longitudinal studies are by definition more time consuming and so require a much greater investment on the part of the researcher and the participants. A third approach, known as cross-sequential studies , combines elements of both cross-sectional and longitudinal studies. Rather than measuring differences between people in different age groups or following the same people over a long period of time, researchers adopting this approach choose a smaller period of time during which they follow people in different age groups. For example, they might measure changes over a ten year period among participants who at the start of the study fall into the following age groups: 20 years old, 30 years old, 40 years old, 50 years old, and 60 years old. This design is advantageous because the researcher reaps the immediate benefits of being able to compare the age groups after the first assessment. Further, by following the different age groups over time they can subsequently determine whether the original differences they found across the age groups are due to true age effects or cohort effects.
The types of research we have discussed so far are all quantitative, referring to the fact that the data consist of numbers that are analyzed using statistical techniques. But as you will learn in this chapter, many observational research studies are more qualitative in nature. In qualitative research , the data are usually nonnumerical and therefore cannot be analyzed using statistical techniques. Rosenhan’s observational study of the experience of people in psychiatric wards was primarily qualitative. The data were the notes taken by the “pseudopatients”—the people pretending to have heard voices—along with their hospital records. Rosenhan’s analysis consists mainly of a written description of the experiences of the pseudopatients, supported by several concrete examples. To illustrate the hospital staff’s tendency to “depersonalize” their patients, he noted, “Upon being admitted, I and other pseudopatients took the initial physical examinations in a semi-public room, where staff members went about their own business as if we were not there” (Rosenhan, 1973, p. 256) [2] . Qualitative data has a separate set of analysis tools depending on the research question. For example, thematic analysis would focus on themes that emerge in the data or conversation analysis would focus on the way the words were said in an interview or focus group.
Internal Validity Revisited
Recall that internal validity is the extent to which the design of a study supports the conclusion that changes in the independent variable caused any observed differences in the dependent variable. Figure 6.1 shows how experimental, quasi-experimental, and non-experimental (correlational) research vary in terms of internal validity. Experimental research tends to be highest in internal validity because the use of manipulation (of the independent variable) and control (of extraneous variables) help to rule out alternative explanations for the observed relationships. If the average score on the dependent variable in an experiment differs across conditions, it is quite likely that the independent variable is responsible for that difference. Non-experimental (correlational) research is lowest in internal validity because these designs fail to use manipulation or control. Quasi-experimental research (which will be described in more detail in a subsequent chapter) falls in the middle because it contains some, but not all, of the features of a true experiment. For instance, it may fail to use random assignment to assign participants to groups or fail to use counterbalancing to control for potential order effects. Imagine, for example, that a researcher finds two similar schools, starts an anti-bullying program in one, and then finds fewer bullying incidents in that “treatment school” than in the “control school.” While a comparison is being made with a control condition, the inability to randomly assign children to schools could still mean that students in the treatment school differed from students in the control school in some other way that could explain the difference in bullying (e.g., there may be a selection effect).

Notice also in Figure 6.1 that there is some overlap in the internal validity of experiments, quasi-experiments, and correlational (non-experimental) studies. For example, a poorly designed experiment that includes many confounding variables can be lower in internal validity than a well-designed quasi-experiment with no obvious confounding variables. Internal validity is also only one of several validities that one might consider, as noted in Chapter 5.
- Milgram, S. (1974). Obedience to authority: An experimental view . New York, NY: Harper & Row. ↵
- Rosenhan, D. L. (1973). On being sane in insane places. Science, 179 , 250–258. ↵
A research that lacks the manipulation of an independent variable.
Research that is non-experimental because it focuses on the statistical relationship between two variables but does not include the manipulation of an independent variable.
Research that is non-experimental because it focuses on recording systemic observations of behavior in a natural or laboratory setting without manipulating anything.
Studies that involve comparing two or more pre-existing groups of people (e.g., children at different stages of development).
Differences between the groups may reflect the generation that people come from rather than a direct effect of age.
Studies in which one group of people are followed over time as they age.
Studies in which researchers follow people in different age groups in a smaller period of time.
Overview of Non-Experimental Research Copyright © 2022 by Rajiv S. Jhangiani; I-Chant A. Chiang; Carrie Cuttler; and Dana C. Leighton is licensed under a Creative Commons Attribution-NonCommercial-ShareAlike 4.0 International License , except where otherwise noted.
Share This Book
- Experimental Vs Non-Experimental Research: 15 Key Differences

There is a general misconception around research that once the research is non-experimental, then it is non-scientific, making it more important to understand what experimental and experimental research entails. Experimental research is the most common type of research, which a lot of people refer to as scientific research.
Non experimental research, on the other hand, is easily used to classify research that is not experimental. It clearly differs from experimental research, and as such has different use cases.
In this article, we will be explaining these differences in detail so as to ensure proper identification during the research process.
What is Experimental Research?
Experimental research is the type of research that uses a scientific approach towards manipulating one or more control variables of the research subject(s) and measuring the effect of this manipulation on the subject. It is known for the fact that it allows the manipulation of control variables.
This research method is widely used in various physical and social science fields, even though it may be quite difficult to execute. Within the information field, they are much more common in information systems research than in library and information management research.
Experimental research is usually undertaken when the goal of the research is to trace cause-and-effect relationships between defined variables. However, the type of experimental research chosen has a significant influence on the results of the experiment.
Therefore bringing us to the different types of experimental research. There are 3 main types of experimental research, namely; pre-experimental, quasi-experimental, and true experimental research.
Pre-experimental Research
Pre-experimental research is the simplest form of research, and is carried out by observing a group or groups of dependent variables after the treatment of an independent variable which is presumed to cause change on the group(s). It is further divided into three types.
- One-shot case study research
- One-group pretest-posttest research
- Static-group comparison
Quasi-experimental Research
The Quasi type of experimental research is similar to true experimental research, but uses carefully selected rather than randomized subjects. The following are examples of quasi-experimental research:
- Time series
- No equivalent control group design
- Counterbalanced design.
True Experimental Research
True experimental research is the most accurate type, and may simply be called experimental research. It manipulates a control group towards a group of randomly selected subjects and records the effect of this manipulation.
True experimental research can be further classified into the following groups:
- The posttest-only control group
- The pretest-posttest control group
- Solomon four-group
Pros of True Experimental Research
- Researchers can have control over variables.
- It can be combined with other research methods.
- The research process is usually well structured.
- It provides specific conclusions.
- The results of experimental research can be easily duplicated.
Cons of True Experimental Research
- It is highly prone to human error.
- Exerting control over extraneous variables may lead to the personal bias of the researcher.
- It is time-consuming.
- It is expensive.
- Manipulating control variables may have ethical implications.
- It produces artificial results.
What is Non-Experimental Research?
Non-experimental research is the type of research that does not involve the manipulation of control or independent variable. In non-experimental research, researchers measure variables as they naturally occur without any further manipulation.
This type of research is used when the researcher has no specific research question about a causal relationship between 2 different variables, and manipulation of the independent variable is impossible. They are also used when:
- subjects cannot be randomly assigned to conditions.
- the research subject is about a causal relationship but the independent variable cannot be manipulated.
- the research is broad and exploratory
- the research pertains to a non-causal relationship between variables.
- limited information can be accessed about the research subject.
There are 3 main types of non-experimental research , namely; cross-sectional research, correlation research, and observational research.
Cross-sectional Research
Cross-sectional research involves the comparison of two or more pre-existing groups of people under the same criteria. This approach is classified as non-experimental because the groups are not randomly selected and the independent variable is not manipulated.
For example, an academic institution may want to reward its first-class students with a scholarship for their academic excellence. Therefore, each faculty places students in the eligible and ineligible group according to their class of degree.
In this case, the student’s class of degree cannot be manipulated to qualify him or her for a scholarship because it is an unethical thing to do. Therefore, the placement is cross-sectional.
Correlational Research
Correlational type of research compares the statistical relationship between two variables .Correlational research is classified as non-experimental because it does not manipulate the independent variables.
For example, a researcher may wish to investigate the relationship between the class of family students come from and their grades in school. A questionnaire may be given to students to know the average income of their family, then compare it with CGPAs.
The researcher will discover whether these two factors are positively correlated, negatively corrected, or have zero correlation at the end of the research.
Observational Research
Observational research focuses on observing the behavior of a research subject in a natural or laboratory setting. It is classified as non-experimental because it does not involve the manipulation of independent variables.
A good example of observational research is an investigation of the crowd effect or psychology in a particular group of people. Imagine a situation where there are 2 ATMs at a place, and only one of the ATMs is filled with a queue, while the other is abandoned.
The crowd effect infers that the majority of newcomers will also abandon the other ATM.
You will notice that each of these non-experimental research is descriptive in nature. It then suffices to say that descriptive research is an example of non-experimental research.
Pros of Observational Research
- The research process is very close to a real-life situation.
- It does not allow for the manipulation of variables due to ethical reasons.
- Human characteristics are not subject to experimental manipulation.

Cons of Observational Research
- The groups may be dissimilar and nonhomogeneous because they are not randomly selected, affecting the authenticity and generalizability of the study results.
- The results obtained cannot be absolutely clear and error-free.
What Are The Differences Between Experimental and Non-Experimental Research?
- Definitions
Experimental research is the type of research that uses a scientific approach towards manipulating one or more control variables and measuring their defect on the dependent variables, while non-experimental research is the type of research that does not involve the manipulation of control variables.
The main distinction in these 2 types of research is their attitude towards the manipulation of control variables. Experimental allows for the manipulation of control variables while non-experimental research doesn’t.
Examples of experimental research are laboratory experiments that involve mixing different chemical elements together to see the effect of one element on the other while non-experimental research examples are investigations into the characteristics of different chemical elements.
Consider a researcher carrying out a laboratory test to determine the effect of adding Nitrogen gas to Hydrogen gas. It may be discovered that using the Haber process, one can create Nitrogen gas.
Non-experimental research may further be carried out on Ammonia, to determine its characteristics, behaviour, and nature.
There are 3 types of experimental research, namely; experimental research, quasi-experimental research, and true experimental research. Although also 3 in number, non-experimental research can be classified into cross-sectional research, correlational research, and observational research.
The different types of experimental research are further divided into different parts, while non-experimental research types are not further divided. Clearly, these divisions are not the same in experimental and non-experimental research.
- Characteristics
Experimental research is usually quantitative, controlled, and multivariable. Non-experimental research can be both quantitative and qualitative , has an uncontrolled variable, and also a cross-sectional research problem.
The characteristics of experimental research are the direct opposite of that of non-experimental research. The most distinct characteristic element is the ability to control or manipulate independent variables in experimental research and not in non-experimental research.
In experimental research, a level of control is usually exerted on extraneous variables, therefore tampering with the natural research setting. Experimental research settings are usually more natural with no tampering with the extraneous variables.
- Data Collection/Tools
The data used during experimental research is collected through observational study, simulations, and surveys while non-experimental data is collected through observations, surveys, and case studies. The main distinction between these data collection tools is case studies and simulations.
Even at that, similar tools are used differently. For example, an observational study may be used during a laboratory experiment that tests how the effect of a control variable manifests over a period of time in experimental research.
However, when used in non-experimental research, data is collected based on the researcher’s discretion and not through a clear scientific reaction. In this case, we see a difference in the level of objectivity.
The goal of experimental research is to measure the causes and effects of variables present in research, while non-experimental research provides very little to no information about causal agents.
Experimental research answers the question of why something is happening. This is quite different in non-experimental research, as they are more descriptive in nature with the end goal being to describe what .
Experimental research is mostly used to make scientific innovations and find major solutions to problems while non-experimental research is used to define subject characteristics, measure data trends, compare situations and validate existing conditions.
For example, if experimental research results in an innovative discovery or solution, non-experimental research will be conducted to validate this discovery. This research is done for a period of time in order to properly study the subject of research.
Experimental research process is usually well structured and as such produces results with very little to no errors, while non-experimental research helps to create real-life related experiments. There are a lot more advantages of experimental and non-experimental research , with the absence of each of these advantages in the other leaving it at a disadvantage.
For example, the lack of a random selection process in non-experimental research leads to the inability to arrive at a generalizable result. Similarly, the ability to manipulate control variables in experimental research may lead to the personal bias of the researcher.
- Disadvantage
Experimental research is highly prone to human error while the major disadvantage of non-experimental research is that the results obtained cannot be absolutely clear and error-free. In the long run, the error obtained due to human error may affect the results of the experimental research.
Some other disadvantages of experimental research include the following; extraneous variables cannot always be controlled, human responses can be difficult to measure, and participants may also cause bias.
In experimental research, researchers can control and manipulate control variables, while in non-experimental research, researchers cannot manipulate these variables. This cannot be done due to ethical reasons.
For example, when promoting employees due to how well they did in their annual performance review, it will be unethical to manipulate the results of the performance review (independent variable). That way, we can get impartial results of those who deserve a promotion and those who don’t.
Experimental researchers may also decide to eliminate extraneous variables so as to have enough control over the research process. Once again, this is something that cannot be done in non-experimental research because it relates more to real-life situations.
Experimental research is carried out in an unnatural setting because most of the factors that influence the setting are controlled while the non-experimental research setting remains natural and uncontrolled. One of the things usually tampered with during research is extraneous variables.
In a bid to get a perfect and well-structured research process and results, researchers sometimes eliminate extraneous variables. Although sometimes seen as insignificant, the elimination of these variables may affect the research results.
Consider the optimization problem whose aim is to minimize the cost of production of a car, with the constraints being the number of workers and the number of hours they spend working per day.
In this problem, extraneous variables like machine failure rates or accidents are eliminated. In the long run, these things may occur and may invalidate the result.
- Cause-Effect Relationship
The relationship between cause and effect is established in experimental research while it cannot be established in non-experimental research. Rather than establish a cause-effect relationship, non-experimental research focuses on providing descriptive results.
Although it acknowledges the causal variable and its effect on the dependent variables, it does not measure how or the extent to which these dependent variables change. It, however, observes these changes, compares the changes in 2 variables, and describes them.
Experimental research does not compare variables while non-experimental research does. It compares 2 variables and describes the relationship between them.
The relationship between these variables can be positively correlated, negatively correlated or not correlated at all. For example, consider a case whereby the subject of research is a drum, and the control or independent variable is the drumstick.
Experimental research will measure the effect of hitting the drumstick on the drum, where the result of this research will be sound. That is, when you hit a drumstick on a drum, it makes a sound.
Non-experimental research, on the other hand, will investigate the correlation between how hard the drum is hit and the loudness of the sound that comes out. That is, if the sound will be higher with a harder bang, lower with a harder bang, or will remain the same no matter how hard we hit the drum.
- Quantitativeness
Experimental research is a quantitative research method while non-experimental research can be both quantitative and qualitative depending on the time and the situation where it is been used. An example of a non-experimental quantitative research method is correlational research .
Researchers use it to correlate two or more variables using mathematical analysis methods. The original patterns, relationships, and trends between variables are observed, then the impact of one of these variables on the other is recorded along with how it changes the relationship between the two variables.
Observational research is an example of non-experimental research, which is classified as a qualitative research method.
- Cross-section
Experimental research is usually single-sectional while non-experimental research is cross-sectional. That is, when evaluating the research subjects in experimental research, each group is evaluated as an entity.
For example, let us consider a medical research process investigating the prevalence of breast cancer in a certain community. In this community, we will find people of different ages, ethnicities, and social backgrounds.
If a significant amount of women from a particular age are found to be more prone to have the disease, the researcher can conduct further studies to understand the reason behind it. A further study into this will be experimental and the subject won’t be a cross-sectional group.
A lot of researchers consider the distinction between experimental and non-experimental research to be an extremely important one. This is partly due to the fact that experimental research can accommodate the manipulation of independent variables, which is something non-experimental research can not.
Therefore, as a researcher who is interested in using any one of experimental and non-experimental research, it is important to understand the distinction between these two. This helps in deciding which method is better for carrying out particular research.
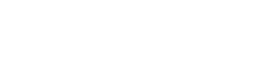
Connect to Formplus, Get Started Now - It's Free!
- examples of experimental research
- non experimental research
- busayo.longe
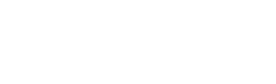
You may also like:
Simpson’s Paradox & How to Avoid it in Experimental Research
In this article, we are going to look at Simpson’s Paradox from its historical point and later, we’ll consider its effect in...

Experimental Research Designs: Types, Examples & Methods
Ultimate guide to experimental research. It’s definition, types, characteristics, uses, examples and methodolgy
Response vs Explanatory Variables: Definition & Examples
In this article, we’ll be comparing the two types of variables, what they both mean and see some of their real-life applications in research
What is Experimenter Bias? Definition, Types & Mitigation
In this article, we will look into the concept of experimental bias and how it can be identified in your research
Formplus - For Seamless Data Collection
Collect data the right way with a versatile data collection tool. try formplus and transform your work productivity today..
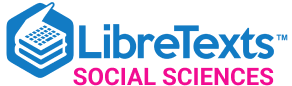
- school Campus Bookshelves
- menu_book Bookshelves
- perm_media Learning Objects
- login Login
- how_to_reg Request Instructor Account
- hub Instructor Commons
- Download Page (PDF)
- Download Full Book (PDF)
- Periodic Table
- Physics Constants
- Scientific Calculator
- Reference & Cite
- Tools expand_more
- Readability
selected template will load here
This action is not available.
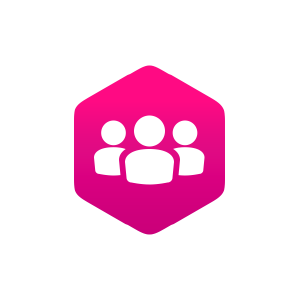
6: Non-Experimental Research
- Last updated
- Save as PDF
- Page ID 19616
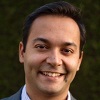
- Rajiv S. Jhangiani, I-Chant A. Chiang, Carrie Cuttler, & Dana C. Leighton
- Kwantlen Polytechnic U., Washington State U., & Texas A&M U.—Texarkana
In this chapter we look more closely at non-experimental research. We begin with a general definition of, non-experimental research, along with a discussion of when and why non-experimental research is more appropriate than experimental research. We then look separately at three important types of non-experimental research: cross-sectional research, correlational research and observational research.
- 6.1: Prelude to Nonexperimental Research What do the following classic studies have in common? Stanley Milgram found that about two thirds of his research participants were willing to administer dangerous shocks to another person just because they were told to by an authority figure (Milgram, 1963). Elizabeth Loftus and Jacqueline Pickrell showed that it is relatively easy to “implant” false memories in people by repeatedly asking them about childhood events that did not actually happen to them (Loftus & Pickrell, 1995).
- 6.2: Overview of Non-Experimental Research Most researchers in psychology consider the distinction between experimental and non-experimental research to be an extremely important one. This is because although experimental research can provide strong evidence that changes in an independent variable cause differences in a dependent variable, non-experimental research generally cannot. As we will see, however, this inability to make causal conclusions does not mean that non-experimental research is less important than experimental research.
- 6.3: Correlational Research Correlational research is a type of non-experimental research in which the researcher measures two variables and assesses the statistical relationship (i.e., the correlation) between them with little or no effort to control extraneous variables. There are many reasons that researchers interested in statistical relationships between variables would choose to conduct a correlational study rather than an experiment.
- 6.4: Complex Correlation As we have already seen, researchers conduct correlational studies rather than experiments when they are interested in noncausal relationships or when they are interested in causal relationships but the independent variable cannot be manipulated for practical or ethical reasons. In this section, we look at some approaches to complex correlational research that involve measuring several variables and assessing the relationships among them.
- 6.5: Qualitative Research Quantitative researchers typically start with a focused research question or hypothesis, collect a small amount of data from a large number of individuals, describe the resulting data using statistical techniques, and draw general conclusions about some large population. Although this method is by far the most common approach to conducting empirical research in psychology, there is an important alternative called qualitative research.
- 6.6: Observational Research Observational research is used to refer to several different types of non-experimental studies in which behavior is systematically observed and recorded. The goal of observational research is to describe a variable or set of variables. The goal is to obtain a snapshot of specific characteristics of an individual, group, or setting. Observational research is non-experimental because nothing is manipulated or controlled, and as such we cannot arrive at causal conclusions using this approach.
- 6.7: Non-Experimental Research (Summary) Key Takeaways and Exercises for the chapter on Non-Experimental Research.
Thumbnail: An example of data produced by data dredging, showing a correlation between the number of letters in a spelling bee's winning word (red curve) and the number of people in the United States killed by venomous spiders (black curve). (CC BY 4.0 International; Tyler Vigen - Spurious Correlations ).
Advertisement
Non-Experimental Comparative Effectiveness Research: How to Plan and Conduct a Good Study
- Pharmacoepidemiology (T Stürmer, Section Editor)
- Published: 04 October 2014
- Volume 1 , pages 206–212, ( 2014 )
Cite this article
- Vera Ehrenstein 1 ,
- Christian F. Christiansen 1 ,
- Morten Schmidt 1 &
- Henrik T. Sørensen 1
3166 Accesses
1 Altmetric
Explore all metrics
Knowledge about the benefit-to-harm balance of alternative treatment options is central to high-quality patient care. In contrast to the traditional hierarchy of evidence, led by randomized designs, the emerging consensus is to move away from judging a study’s validity based only on randomization. Ethical, practical, and financial considerations dictate that most epidemiologic research be non-experimental. That includes studies of effectiveness and safety of treatments. We provide a non-technical overview of essential prerequisites for high-quality comparative effectiveness research from the standpoint of clinical epidemiology, keeping in mind potentially divergent agendas of investigators and other stakeholders. We discuss the essentials of study planning, implementation, and publication of results. Our focus is on non-experimental studies that generate evidence addressing different dimensions of harm–benefit profiles of therapies. Bias minimization strategies, transparency, and independence in reporting are the guiding principles of comparative effectiveness research, whose ultimate goal is to improve patient care and public health.
Similar content being viewed by others
Empirical consequences of current recommendations for the design and interpretation of noninferiority trials.
Scott K. Aberegg, Andrew M. Hersh & Matthew H. Samore
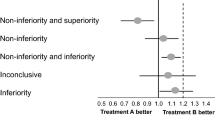
Methodological aspects of superiority, equivalence, and non-inferiority trials
Roumeliotis Stefanos, D.’Arrigo Graziella & Tripepi Giovanni
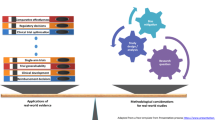
Real-world evidence: the devil is in the detail
Mugdha Gokhale, Til Stürmer & John B. Buse
Avoid common mistakes on your manuscript.
Introduction
Knowledge about the benefit-to-harm balance of alternative treatment options is central to high-quality patient care. Traditionally, the experiment [randomized controlled trial (RCT) or natural experiment] has been at the top of the ‘hierarchy of evidence’ as the gold standard for evidence-based medicine, especially for therapeutic choices [ 1 ]. Bias-reducing features of the RCTs—random treatment assignment with the expectation of zero net confounding at baseline; restriction to uniform patient populations; blinding; and standardized data collection (all combined with underlying statistical theory)—are ways to maximize internal validity. In contrast to the traditional hierarchy of evidence [ 1 ], the emerging consensus among clinical epidemiologists is to move away from judging a study’s validity based only on its design type [ 2 – 5 ]. This consensus arises from an appreciation that some purported benefits of experimental designs are not always realized in practice (e.g., the baseline prognostic balance achieved by randomization is often upset during follow-up). Nor do the internally valid results of RCTs apply in all settings of routine clinical care because of the inevitable validity–generalizability tradeoffs of RCTs [ 2 – 5 , 6 ••, 7 – 9 ]. As well, ethical, practical, and financial considerations dictate that most epidemiologic research be observational, including studies of comparative effectiveness and comparative safety of treatments [ 10 ]. Thus, observational studies comparing treatments are increasingly advocated and implemented [ 6 ••, 11 ]. Novel designs that combine advantages of randomized and non-randomized approaches (such as lowering the tradeoff between internal and external validity in pragmatic trials or reliance on new-user designs [ 12 , 13 ••]) help mitigate the disadvantages of both approaches, aiding the acceptance of non-experimental methods in the clinical research community. Modern design and analytic approaches to reducing or quantifying systematic errors in observational research include propensity score methods, marginal structural models, instrumental variables, external adjustment, and bias analyses [ 2 , 12 , 14 – 19 ]. Choosing and correctly implementing study design is a prerequisite for subsequent valid application of different analytic techniques.
Although clinicians have routinely compared harms and benefits of treatments for their patients in an informal way, the concept of systematic comparative effectiveness research (CER) is relatively new. For example, the 2008 edition of the Dictionary of Epidemiology did not yet contain an entry for CER [ 20 ]. In 2009, the Institute of Medicine defined CER as “generation and synthesis of evidence that compares the benefits or harms of alternative methods to prevent, diagnose, treat, and monitor clinical conditions, or to improve the delivery of care” [ 21 ]. CER thus encompasses studies (1) directly or indirectly comparing safety and/or effectiveness of active treatments for the same indication; (2) carried out in routine clinical practice; and (3) aiming to help clinicians, regulators, and policy makers to make evidence-based decisions. In addition to scientific aims, CER studies initiated outside academic institutions may have explicit practical goals, including formulation of guidelines, standards of care, safety regulations, or reimbursement policies [ 22 ]. Thus, clinical decision making and policy are much more prominent in planning CER studies in non-academic settings than in conventional investigator-initiated studies in academia [ 22 , 23 ].
Guidelines relevant to CER have been published by several authorities [ 8 , 9 , 24 – 28 ], with some of these publications eliciting critique and calls for harmonization [ 29 , 30 •]. Investigators embarking on a CER study should start by consulting the Guidelines for Good Pharmacoepidemiology Practice (GPP), maintained by the International Society for Pharmacoepidemiology (ISPE) [ 30 •]. The Good Research for Comparative Effectiveness (GRACE) principles specify the following questions to be considered when assessing study quality [ 25 ]: (1) whether the study plans (including research questions, main comparisons, outcomes, etc.) were specified before the study was conducted; (2) whether the study was conducted and analyzed in a manner consistent with good practices and reported in sufficient detail for evaluation and replication; and (3) how valid the interpretation of the CER study is for the population of interest, assuming sound methods and appropriate follow-up.
With these questions in mind, we provide a non-technical overview of essential prerequisites for high-quality CER studies from a clinical epidemiology standpoint, keeping in mind the potentially divergent agendas of investigators and other stakeholders. We discuss the essentials of study planning, implementation, and publication of results, focusing on observational studies that generate evidence addressing different dimensions of the harm–benefit profiles of therapies.
Study Planning
The stakeholders and the aim.
The aim of a CER study should be clearly and unambiguously defined and should meet criteria for good research, e.g., the FINER [ 31 ] or PICOTS [ 32 ] criteria. The FINER criteria state that the proposed research should be f easible (in terms of number of patients and sources of data, technical expertise, expenditure of time and money, and manageable scope); i nteresting (to the clinical community as well as the investigator); n ovel (in terms of extending and improving previous research); e thical; and r elevant (to scientific knowledge, clinical health policies, or future research). The parameters for good research to be considered according to PICOTS include the p opulation (condition(s), disease severity and stage, co-morbidities, and patient demographics), the i ntervention (dosage, frequency, and method of administration), c omparator (placebo, usual care, or active control),the o utcome (morbidity, mortality, or quality of life), the t iming (duration of follow-up), and the s etting (primary, specialty, inpatient, and co-interventions).
The CER study proposal should also explicitly list study initiators, sponsors, and other stakeholders, and potential conflicts of interest. Stakeholders are individuals, organizations, or communities who have a direct interest in the process and outcomes of a study [ 22 , 23 , 33 ]. Stakeholders who might be involved in a CER study include industry (in voluntary or regulator-imposed post-authorization safety or effectiveness studies [ 34 •]), regulators (e.g., European Medicines Agency (EMA), US Food and Drug Administration), and governments—in different combinations [ 22 ]. Patient engagement in reviewing merits of research proposals is becoming increasingly common, and may serve to increase relevance to patient care of CER and clinical research [ 35 ].
An investigator contemplating a CER study initiated by a pharmaceutical company should always consider underlying motivations. These could include concern about safety signals emerging from spontaneous reporting, a wish to study disease risk in the general population or in specific groups of patients before a new treatment enters the market, or a regulator-imposed post-authorization monitoring. To eliminate concerns about hidden agendas that might otherwise compromise the integrity of a CER study, any potential conflict of interest among investigators or participating institutions should be fully disclosed.
It is important to note that collaboration with industry does not per se threaten study validity. If there is an agenda (hidden or obvious), university-based researchers are in a better position than for-profit contract research organizations to uphold and enforce principles and procedures protecting study validity. Academically based investigators are backed by institutional mandates for independence and the obligation to publish results of all studies in journals with independent peer review. Unless they are providing direct gainful consultancy services to the pharmaceutical industry, academic researchers are typically salaried employees who do not directly benefit financially from ‘landing’ a lucrative pharmaceutical contract. Since such a contract is executed between institutions rather than individuals, the financial gain of an individual academic investigator is limited (source: Susanne Kudsk, Legal Advisor, Aarhus University, personal communication). As well, conducting a poor study under pressure from a sponsor affects an investigator’s reputation [ 29 ]. If experts from academia refuse to collaborate with industry on CER studies, they may be replaced by potentially less skillful, less scrupulous, or less independent investigators [ 36 ].
The Contract
Collaboration between academic institutions and regulators, government, and/or industry sponsors should be governed by a professional contract, which is crucial for both the researcher and the sponsor. A contract is a formal agreement establishing the ‘rules of the game’: what is to be done, by whom, when, and at what cost. In international environments, the country whose laws will govern the contract should be clearly specified. A contract serves as a master document to be consulted in case of disputes. It should be executed by the researcher’s institution to avoid conflicts and charges of corruption that could arise, were the researcher to receive payment directly from the sponsor.
The type of contract depends on the sponsor’s role. It can take the form of (1) a grant for investigator-initiated studies with no substantial involvement by the sponsor; (2) a cooperative agreement in which the investigator and the sponsor collaborate on the project and both contribute funding and intellectual content; or (3) a contract for sponsor-initiated studies with substantial involvement by the sponsor.
The contract should regulate the interests of both the investigator (and his/her institution) and the sponsor. It should describe the parties, the purpose of the research, the definition of the project, deliverables, schedule, subcontracting, contributions and obligations of the parties, distribution and transfer of rights, confidentiality, and consequences of ending the collaboration.
The contract must ensure that the researcher and the researcher’s institution are free to use the findings in future research and teaching. The researcher also should have the unrestricted right to publish the research findings. In most cases, the sponsor may require a period of time (e.g., 30 or 60 days) to review and comment on a manuscript arising from contract research before submission for publication. Both parties must be willing to negotiate the manuscript’s content and phrasing, but the researcher should have the final say. In special circumstances, the sponsor may postpone publication for up to 6 months, for instance, to apply for a patent. However, this is a rare occasion in CER, in which timely publication of results with a public health impact has high priority. In addition, publication should not be postponed by adverse event reporting, which is usually not possible or appropriate based on aggregate results from a non-experimental CER study using databases [ 30 •].
Assessing Study Feasibility
CER studies are increasingly conducted using secondary data sources, such as healthcare databases, which rely on routine data collected for other purposes. This raises the question whether the data relevant to the study aim are measured or measured well in the candidate data source. A feasibility study conducted ahead of the main effort may help secure data access, estimate study size, or evaluate background rates of the target condition. A feasibility study may also help establish referral and hospitalization patterns to assess the potential role of selection bias or confounding by indication. At our institution, we routinely evaluate the validity of study algorithms before using them in CER studies. For example, we evaluated the validity of an algorithm to identify osteonecrosis of the jaw and serious infections [ 37 – 39 ] before conducting regulator-imposed industry-sponsored comparative safety studies of antiresorptive agents [ 40 ]. While the validity of the algorithm used to identify serious infection was high in hospital records, the algorithm to identify osteonecrosis of the jaw performed poorly and necessitated primary data collection [ 41 ]. Thus, a feasibility study helps estimate whether—and to what extent—existing data must be supplemented with primary data collection. In addition, a pilot study can help in estimating associated costs and in planning appropriate resources. If data from several different databases are to be combined in a CER study, a pilot study may help determine whether all databases measure equally well what they purport to measure. For example, pilot studies may compare estimates of incidence of well-characterized conditions, examine sources of any unexpected variation, and adjust the methodology (see Avillach et al. [ 42 ] and Coloma et al. [ 43 ] for illustration of this approach).
Review of the Skills of Team Members
For a CER study to be well-conducted, the investigator should be mindful of whether the research team covers the spectrum of required expertise and skills. Multidisciplinary CER study teams usually include pharmacoepidemiologists, biostatisticians, pharmacologists, and clinicians. Access to legal advice and project management are also essential to a well-conducted CER study. For multi-institutional studies, it may be efficient to outsource certain administrative or IT tasks. Furthermore, since many comparative effectiveness studies address major and pressing clinical and legal issues, it is important to select participating investigators who can meet tight deadlines without compromising research quality.
International Collaboration
If the required skills and resources are not present within the local team, international collaboration with leading experts in relevant fields can help ensure high quality of a CER study. Moreover, data from a single country/data system may be insufficient to address all study objectives, to achieve sufficient sample size, or to achieve sufficient generalizability. In some instances, collaboration between at least two different countries may be a condition for funding: for example, the EMA routinely requires use of data from two or more EU Member States in its commissioned research [ 44 ]. Finally, investigators whose institutional or national policies proscribe direct collaboration with industry may contribute to CER as subcontractors within international collaborations [ 40 ]. Decisions about the number of required databases can be formalized in the study protocol, as recently described [ 45 ].
Study Implementation
Protocol and statistical analysis plan.
After study feasibility is established, study sources identified, study teams assembled, and the contract signed, a study protocol is developed or finalized as the first step of study implementation. Several guidelines for the structure and components of CER protocols have been proposed [ 13 ••, 27 , 46 , 47 ]. The user guide developed for the United States Agency for Healthcare Research and Quality is comprehensive yet readable and contains contributions by highly reputed experts [ 13 ••].
Protocol writers should strive to create a detailed and transparent guide to the conduct of the study. The protocol must define the primary, secondary, and potential exploratory study objectives. Protocol writing is an iterative process that helps raise and address methodological issues. Protocol-related challenges of studies based on multinational secondary data sources require an adequate description of diverse data systems and measurement of study variables extracted from diverse sources (such as general practice-based databases, claims databases, and/or national registries). These sources may have different mechanisms for generating records, which affect data validity and completeness as well as interpretation of results.
In multinational studies, it is crucial to involve all participants in writing and revising the study protocol. In regulator-imposed post-authorization studies, the marketing authorization holder may initiate writing of the protocol according to prespecified formats, working with data custodians in participating countries to harmonize data-generating mechanisms. The protocol should be reviewed by clinicians with relevant expertise and with experience treating patients in a given health system; by statisticians with practical expertise in data-generating mechanisms, data flow, and data architecture; and by epidemiologists who can foresee the implications of data idiosyncrasies for interpretation of results.
For observational studies, including CER studies, the protocol should contain clear provisions for efforts to rule out methodological threats to validity, including selection bias, information bias, confounding, and chance. Use of automated health records—claims, patient, and disease registries, medical record databases, and insurance databases—has become a mainstay of CER [ 8 , 9 , 25 , 48 ]. Thus, investigators have large amounts of routinely collected data on large numbers of individuals but limited control of data collection. In an era of automated databases, it is essential to consider how selection bias, confounding by indication, data quality, misclassification, and medical surveillance bias, are to be handled [ 49 •]. Some traditional epidemiologic ‘mantras’ [ 50 ] may not apply in CER settings. One example is the dilution of estimates by non-differential misclassification of exposure, frequently invoked to defend ‘conservative estimates’ in studies of non-pharmaceutical exposures. Dilution of estimates in CER studies is, like in any other study, a potential public health hazard if exposure measurement instruments and definitions are so poor that they lower the strength of a safety signal beyond detection, resulting in continued use of a potentially unsafe agent. CER study protocols must specify ways to avoid dilution of the effect by inclusion of outcome measures that have high specificity. Another example is the challenge of confounding by indication when comparing treated with untreated; however, in CER studies comparing two different drugs with the same indication, this problem is often reduced considerably.
The planned statistical analysis should be described in sufficient detail in the study protocol. However, the comprehensive description of statistical procedures may require a separate document, the Statistical Analysis Plan (SAP). As the SAP is a guide for the study statistician, he/she should be involved in its preparation and must approve it. The SAP closely follows the study protocol and is developed after the protocol is finalized. The SAP contains a detailed description of sampling and analytic procedures, and many sections of the SAP will be lifted verbatim for use in the statistical analysis section of the study report or a published paper. Analysis of data from different international sources may be country-based or pooled. Development of common data models is quickly becoming the standard approach. Different approaches to combining international data have been described and are beyond the scope of this paper [ 40 – 43 , 45 , 51 , 52 ••, 53 , 54 ••, 55 , 56 ].
Transparency and methodological rigor are necessary features of the protocol and the SAP. The CER protocol must be in place before the study commences. In some situations, e.g., in some regulator-imposed studies, a protocol must be in place before the drug under study enters the market. By definition, such a protocol is not informed by crucial aspects of real-life drug utilization, including whether the drug will be distributed in inpatient or outpatient settings (and therefore measurable in outpatient prescription databases) and how fast drug uptake occurs. Therefore, amendments to the protocol are often necessary as real-life aspects of drug use become apparent. Protocol amendments should be justified, scientifically sound, agreed-upon by all study stakeholders, and meticulously documented [ 57 ]. CER protocols and all amendments may need approval by a regulator. The EMA publishes the protocols of imposed post-authorization studies in its ENCePP (European Network of Centres for Pharmacoepidemiology and Pharmacovigilance) register of studies [ 58 ]. Researchers should consider registration of any CER study; for example non-ENCePP studies can be registered in the ENCePP registry.
Interacting with the Sponsor
Professional interaction with the sponsor is important in both investigator- and sponsor-initiated studies, depending on contributions agreed on before study initiation. Formal channels of communication (e.g., frequency of investigator meetings, teleconferences, and updates) should be agreed upon in advance. Informal communication with sponsor employees is less regulated. Pharmaceutical companies often have dedicated research, development, and/or safety departments that are separated from the sales department in order to reduce conflicts of interest.
The sponsor may contribute important background knowledge to a CER study, which can be useful in formulating the research question (e.g., nature of potential adverse events from ongoing RCTs). However, during the conduct of the study, communication may be more informative than interactive. While the researcher and the sponsor should share a fundamental interest in improving health for patients, they may have different interests that should be kept in mind during interactions. Respectful communication is required, as research findings should not be influenced by the sponsor. Still, the sponsor may have a particular interest in getting as much information as possible, as research findings may have a major impact on approval, labeling, and sale of the company’s products.
Publication of Results
The publication potential of CER studies is attractive to academia-based researchers and may serve as an important motivator for expert clinicians and methodologists to contribute their efforts. The investigators should be free to publish all results stemming from CER research, and this right should be delineated in the contract. Sponsor employees should co-author the publications, provided they fulfill the authorship criteria [ 59 ]. Several scientific publications may stem from a single CER study, with different author constellations. Even if it seems redundant, it is worth circulating the ICMJE (International Committee of Medical Journal Editors) authorship criteria before drafting a manuscript to ensure that all aspiring authors understand and are prepared to fulfill their expected contributions. Results should be transparently reported and judiciously interpreted, including honest discussion of study limitations. Current reporting guidelines [ 60 ], especially the STROBE (STrengthening the Reporting of OBservational studies in Epidemiology) statement for observational studies, and the upcoming RECORD (REporting of studies Conducted using Observational Routinely collected Data) guidelines for reporting studies conducted using routinely collected data [ 61 •], will help determine the type of information that needs to be included in the planned report.
In conclusion, methodological rigor, clear rules, transparency in communication, and independence in reporting are the guiding principles of observational CER, with the ultimate goal of improving patient care and public health.
Papers of particular interest, published recently, have been highlighted as: • Of importance •• Of major importance
Fletcher RH, Fletcher SW, Fletcher GS. Clinical epidemiology: the essentials. 5th ed. Philadelphia: Wolters Kluwer/Lippincott Williams & Wilkins Health; 2014.
Google Scholar
Hernan MA, Alonso A, Logan R, et al. Observational studies analyzed like randomized experiments: an application to postmenopausal hormone therapy and coronary heart disease. Epidemiology. 2008;19(6):766–79.
Article PubMed PubMed Central Google Scholar
Hernan MA, Hernandez-Diaz S, Robins JM. Randomized trials analyzed as observational studies. Ann Intern Med. 2013;159(8):560–2.
PubMed Google Scholar
Sorensen HT, Lash TL, Rothman KJ. Beyond randomized controlled trials: a critical comparison of trials with nonrandomized studies. Hepatology. 2006;44(5):1075–82.
Article PubMed Google Scholar
Rothman KJ. Six persistent research misconceptions. J Gen Intern Med. 2014;29(7):1060–4.
Sox HC, Goodman SN. The methods of comparative effectiveness research. Annu Rev Public Health. 2012;33:425–45. This review provides a concise and comprehensive overview of methods used in CER and its key elements, with focus on issues relevant in observational settings .
Haynes B. Can it work? Does it work? Is it worth it? BMJ. 1999;319(7211):652–3.
Article PubMed CAS PubMed Central Google Scholar
Dreyer NA. Making observational studies count: shaping the future of comparative effectiveness research. Epidemiology. 2011;22(3):295–7.
Sturmer T, Jonsson Funk M, Poole C, Brookhart MA. Nonexperimental comparative effectiveness research using linked healthcare databases. Epidemiology. 2011;22(3):298–301.
Holve E, Pittman P. A First Look at the Volume and Cost of Comparative Effectiveness Research in the United States. AcademyHealth. 2009. http://www.academyhealth.org/files/publications/CERMonograph09.pdf .
Sox HC. Comparative effectiveness research: a progress report. Ann Intern Med. 2010;153(7):469–72.
Sturmer T, Schneeweiss S, Brookhart MA, Rothman KJ, Avorn J, Glynn RJ. Analytic strategies to adjust confounding using exposure propensity scores and disease risk scores: nonsteroidal antiinflammatory drugs and short-term mortality in the elderly. Am J Epidemiol. 2005;161(9):891–8.
Velentgas P, Dreyer NA, Nourjah P, Smith SR, Torchia MM, editors. Developing a protocol for observational comparative effectiveness research: a user’s guide. AHRQ publication no. 12(13)-EHC099. Rockville: Agency for Healthcare Research and Quality; 2013. http://www.effectivehealthcare.ahrq.gov/Methods-OCER.cfm . A well-referenced, comprehensive, modern, and methodologically sound manual for those writing CER prototols. Of particular value is the reference material to state-of-the art analytic techniques and advice on study design decisions, using real-life examples.
Schneeweiss S. Sensitivity analysis and external adjustment for unmeasured confounders in epidemiologic database studies of therapeutics. Pharmacoepidemiol Drug Saf. 2006;15(5):291–303.
Braitman LE, Rosenbaum PR. Rare outcomes, common treatments: analytic strategies using propensity scores. Ann Intern Med. 2002;137(8):693–5.
Brookhart MA, Rassen JA, Schneeweiss S. Instrumental variable methods in comparative safety and effectiveness research. Pharmacoepidemiol Drug Saf. 2010;19(6):537–54.
Brookhart MA, Sturmer T, Glynn RJ, Rassen J, Schneeweiss S. Confounding control in healthcare database research: challenges and potential approaches. Med Care. 2010;48(6 Suppl):S114–20.
Lash TL, Fox MP, Fink AK. Applying quantitative bias analysis to epidemiologic data. Dordrecht: Springer; 2009.
Book Google Scholar
Garabedian LF, Chu P, Toh S, Zaslavsky AM, Soumerai SB. Potential bias of instrumental variable analyses for observational comparative effectiveness research. Ann Intern Med. 2014;161(2):131–8.
Porta MS, editor. A dictionary of epidemiology. 5th ed. Oxford: Oxford University Press; 2008.
Sox HC, Greenfield S. Comparative effectiveness research: a report from the Institute of Medicine. Ann Intern Med. 2009;151(3):203–5.
Smith SR. Introduction. In: Velentgas P, Dreyer NA, Nourjah P, Smith SR, Torchia MM, editors. Developing a protocol for observational comparative effectiveness research: a user’s guide. AHRQ publication no 12(13)-EHC099. Rockville: Agency for Healthcare Research and Quality; 2013. p. 1–6.
Smith SR. Study objectives and questions. In: Velentgas P, Dreyer NA, Nourjah P, Smith SR, Torchia MM, editors. Developing a protocol for observational comparative effectiveness research: a user’s guide. AHRQ publication no 12(13)-EHC099. Rockville: Agency for Healthcare Research and Quality; 2013. p. 7–20.
Berger ML, Mamdani M, Atkins D, Johnson ML. Good research practices for comparative effectiveness research: defining, reporting and interpreting nonrandomized studies of treatment effects using secondary data sources: the ISPOR Good Research Practices for Retrospective Database Analysis Task Force Report--Part. Value Health. 2009;12(8):1044–52.
Dreyer NA, Schneeweiss S, McNeil BJ, et al. GRACE principles: recognizing high-quality observational studies of comparative effectiveness. Am J Manag Care. 2010;16(6):467–71.
Johnson ML, Crown W, Martin BC, Dormuth CR, Siebert U. Good research practices for comparative effectiveness research: analytic methods to improve causal inference from nonrandomized studies of treatment effects using secondary data sources: the ISPOR Good Research Practices for Retrospective Database Analysis Task Force Report--Part II. Value Health. 2009;12(8):1062–73.
The European Medicines Agency. Guidance for the format and content of the protocol of non-interventional post-authorisation safety studies. http://www.ema.europa.eu/docs/en_GB/document_library/Other/2012/10/WC500133174.pdf . Accessed 28 Jul 2012.
Cox E, Martin BC, Van Staa T, Garbe E, Siebert U, Johnson ML. Good research practices for comparative effectiveness research: approaches to mitigate bias and confounding in the design of nonrandomized studies of treatment effects using secondary data sources: the International Society for Pharmacoeconomics and Outcomes Research Good Research Practices for Retrospective Database Analysis Task Force Report--Part I. Value Health. 2009;12(8):1053–61.
Sturmer T, Carey T, Poole C. ISPOR Health Policy Council proposed good research practices for comparative effectiveness research: benefit or harm? Value Health. 2009;12(8):1042–3.
International Society for Pharmacoepidemiology. Guidelines for Good Pharmacoepidemiology Practices (GPP). https://www.pharmacoepi.org/resources/guidelines_08027.cfm . Accessed 31 May 2014. A current industry standard for conducting pharmacoepidemiology and pharmacovigilance studies.
Hulley SB, Cumming SR, Browner WS, Grady DG, Newman TB. Designing clinical research. 4th ed. Philadelphia: Lippincott, Williams and Wilkins; 2013.
Whitlock EP, Lopez SA, Chang S, Helfand M, Eder M, Floyd N. AHRQ series paper 3: identifying, selecting, and refining topics for comparative effectiveness systematic reviews: AHRQ and the effective health-care program. J Clin Epidemiol. 2010;63(5):491–501.
Deverka PA, Lavallee DC, Desai PJ, et al. Stakeholder participation in comparative effectiveness research: defining a framework for effective engagement. J Comp Eff Res. 2012;1(2):181–94.
European Medicines Agency. Post-authorisation safety studies (PASS). http://www.ema.europa.eu/ema/index.jsp?curl=pages/regulation/document_listing/document_listing_000377.jsp&mid=WC0b01ac058066e979 . Accessed 28 Jul 2014. Guide on type of CER studies from the European regulator.
Fleurence RL, Forsythe LP, Lauer M, et al. Engaging patients and stakeholders in research proposal review: the patient-centered outcomes research institute. Ann Intern Med. 2014;161(2):122–30.
Lash TL. Plenary lecture: The future of epidemiology - where do we go from here? European Congress of Epidemiology (EUROEPI); 11–13 Aug 2013; Aarhus, Denmark.
Bergdahl J, Jarnbring F, Ehrenstein V, et al. Evaluation of an algorithm ascertaining cases of osteonecrosis of the jaw in the Swedish National Patient Register. Clin Epidemiol. 2013;5:1–7.
Gammelager H, Svaerke C, Noerholt SE, et al. Validity of an algorithm to identify osteonecrosis of the jaw in women with postmenopausal osteoporosis in the Danish National Registry of Patients. Clin Epidemiol. 2013;5:263–7.
Holland-Bill L, Xu H, Sørensen HT, et al . Positive predictive value of primary inpatient discharge diagnoses of infection among cancer patients in the Danish National Registry of Patients. Ann Epidemiol. 2014;24(8):593–597.e18
Xue F, Ma H, Stehman-Breen C, et al. Design and methods of a postmarketing pharmacoepidemiology study assessing long-term safety of Prolia® (denosumab) for the treatment of postmenopausal osteoporosis. Pharmacoepidemiol Drug Saf. 2013;22(10):1107–14.
PubMed CAS Google Scholar
Schiodt M, Wexell CL, Herlofson BB, Giltvedt KM, Norholt SE, Ehrenstein V. Existing Data Sources for Clinical Epidemiology: Scandinavian Cohort for Osteonecrosis of the Jaw – Work in Progress and Challenges. Clinical Epidemiol 2014 (in press).
Avillach P, Coloma PM, Gini R, et al. Harmonization process for the identification of medical events in eight European healthcare databases: the experience from the EU-ADR project. J Am Med Inform Assoc. 2013;20(1):184–92.
Coloma PM, Schuemie MJ, Trifiro G, et al. Combining electronic healthcare databases in Europe to allow for large-scale drug safety monitoring: the EU-ADR Project. Pharmacoepidemiol Drug Saf. 2011;20(1):1–11.
Ehrenstein V, Hernandez RK, Ulrichsen SP, et al. Rosiglitazone use and post-discontinuation glycaemic control in two European countries, 2000-2010. BMJ Open. 2013;3(9):e003424.
Maro JC, Brown JS, Kulldorff M. Medical product safety surveillance how many databases to use? Epidemiology. 2013;24(5):692–9.
Berger ML, Dreyer N, Anderson F, Towse A, Sedrakyan A, Normand SL. Prospective observational studies to assess comparative effectiveness: the ISPOR good research practices task force report. Value Health. 2012;15(2):217–30.
ENCePP Guide on Methodological Standards in Pharmacoepidemiology. Section 9.1: Comparative effectiveness research. http://www.encepp.eu/standards_and_guidances/methodologicalGuide9_1.shtml . Accessed 1 Jun 2014.
Schneeweiss S. Developments in post-marketing comparative effectiveness research. Clin Pharmacol Ther. 2007;82(2):143–56.
Article PubMed CAS Google Scholar
Sørensen HT, Baron JA. Medical databases. In: Olsen J, Saracci R, Trichopoulos D, editors. Teaching epidemiology: a guide for teachers in epidemiology, public health and clinical medicine. 4th edn. Oxford: Oxford University Press; (in press). An overview of database research, which has become a CER mainstay.
Lash TL, Fink AK. Re: “Neighborhood environment and loss of physical function in older adults: evidence fro alameda county study” [letter]. Am J Epidemiol. 2003;157(5):472–3.
Gagne JJ, Glynn RJ, Rassen JA, et al. Active safety monitoring of newly marketed medications in a distributed data network: application of a semi-automated monitoring system. Clin Pharmacol Ther. 2012;92(1):80–6.
Gagne JJ, Wang SV, Rassen JA, Schneeweiss S. A modular, prospective, semi-automated drug safety monitoring system for use in a distributed data environment. Pharmacoepidemiol Drug Saf. 2014;23(6):619–27. A guide for conducting drug safety monitoring involving databases from different countries. The authors demonstrate the feasibility of a semi-automated prospective monitoring approach .
Platt R, Davis R, Finkelstein J, et al. Multicenter epidemiologic and health services research on therapeutics in the HMO Research Network Center for Education and Research on Therapeutics. Pharmacoepidemiol Drug Saf. 2001;10(5):373–7.
Toh S, Gagne JJ, Rassen JA, Fireman BH, Kulldorff M, Brown JS. Confounding adjustment in comparative effectiveness research conducted within distributed research networks. Med Care. 2013;51(8 Suppl 3):S4–10. A critical assessment of different confounding adjustment applications for observational CER studies conducted within distributed research networks, including analysis of patient-level data, case-centered logistic regression of risk set data, analysis of aggregated data, and meta-analysis of site-specific effect estimates .
Kieler H, Artama M, Engeland A, et al. Selective serotonin reuptake inhibitors during pregnancy and risk of persistent pulmonary hypertension in the newborn: population based cohort study from the five Nordic countries. BMJ. 2012;344:d8012.
Harcourt SE, Smith GE, Elliot AJ, et al. Use of a large general practice syndromic surveillance system to monitor the progress of the influenza A(H1N1) pandemic 2009 in the UK. Epidemiol Infect. 2012;140(1):100–5.
Chalkidou K, Anderson G. Comparative Effectiveness Research: International Experiences and Implications for the United States. http://www.academyhealth.org/files/publications/CER_International_Experience_09%20(3).pdf . Accessed 1 Jun 2014.
ENCePP. The EU PAS Register. http://www.encepp.eu/encepp_studies/indexRegister.shtml . Accessed 28 Jul 2014.
ICMJE. Defining the role of authors and contributors. http://www.icmje.org/recommendations/browse/roles-and-responsibilities/defining-the-role-of-authors-and-contributors.html . Accessed 22 Jul 2014.
EQUATOR Network: Enhancing the QUAlity and Transparency Of health Research. http://www.equator-network.org/ . Accessed 22 Jul 2014.
Langan SM, Benchimol EI, Guttmann A, et al. Setting the RECORD straight: developing a guideline for the REporting of studies Conducted using Observational Routinely collected Data. Clin Epidemiol. 2013;5:29–31. A guideline specifically addressing issues of reporting results of studies stemming from automated databases .
PubMed PubMed Central Google Scholar
Download references
Acknowledgments
The authors each report being a salaried employee of Aarhus University (Aarhus, Denmark). Aarhus University receives (and administers) research grants from various pharmaceutical companies and the European Medicines Agency. V. Ehrenstein, C.F. Christiansen, M. Schmidt, and H.T. Sørensen do not receive research grants or consultant fees from pharmaceutical companies.
Compliance with Ethics Guidelines
Conflict of interest.
V. Ehrenstein, C.F. Christiansen, M. Schmidt, and H.T. Sørensen all declare no conflicts of interest.
Human and Animal Rights and Informed Consent
All studies by the authors involving animal and/or human subjects were performed after approval by the appropriate institutional review boards. When required, written informed consent was obtained from all participants.
Author information
Authors and affiliations.
Department of Clinical Epidemiology, Aarhus University Hospital, Olof Palmes Allé 43-45, 8200, Aarhus, Denmark
Vera Ehrenstein, Christian F. Christiansen, Morten Schmidt & Henrik T. Sørensen
You can also search for this author in PubMed Google Scholar
Corresponding author
Correspondence to Vera Ehrenstein .
Rights and permissions
Reprints and permissions
About this article
Ehrenstein, V., Christiansen, C.F., Schmidt, M. et al. Non-Experimental Comparative Effectiveness Research: How to Plan and Conduct a Good Study. Curr Epidemiol Rep 1 , 206–212 (2014). https://doi.org/10.1007/s40471-014-0021-5
Download citation
Published : 04 October 2014
Issue Date : December 2014
DOI : https://doi.org/10.1007/s40471-014-0021-5
Share this article
Anyone you share the following link with will be able to read this content:
Sorry, a shareable link is not currently available for this article.
Provided by the Springer Nature SharedIt content-sharing initiative
- Comparative effectiveness research
- Database research
- Epidemiology
- Evidence-based medicine
- Observational research
- Pharmacoepidemiology
- Post-authorization study
- Find a journal
- Publish with us
- Track your research
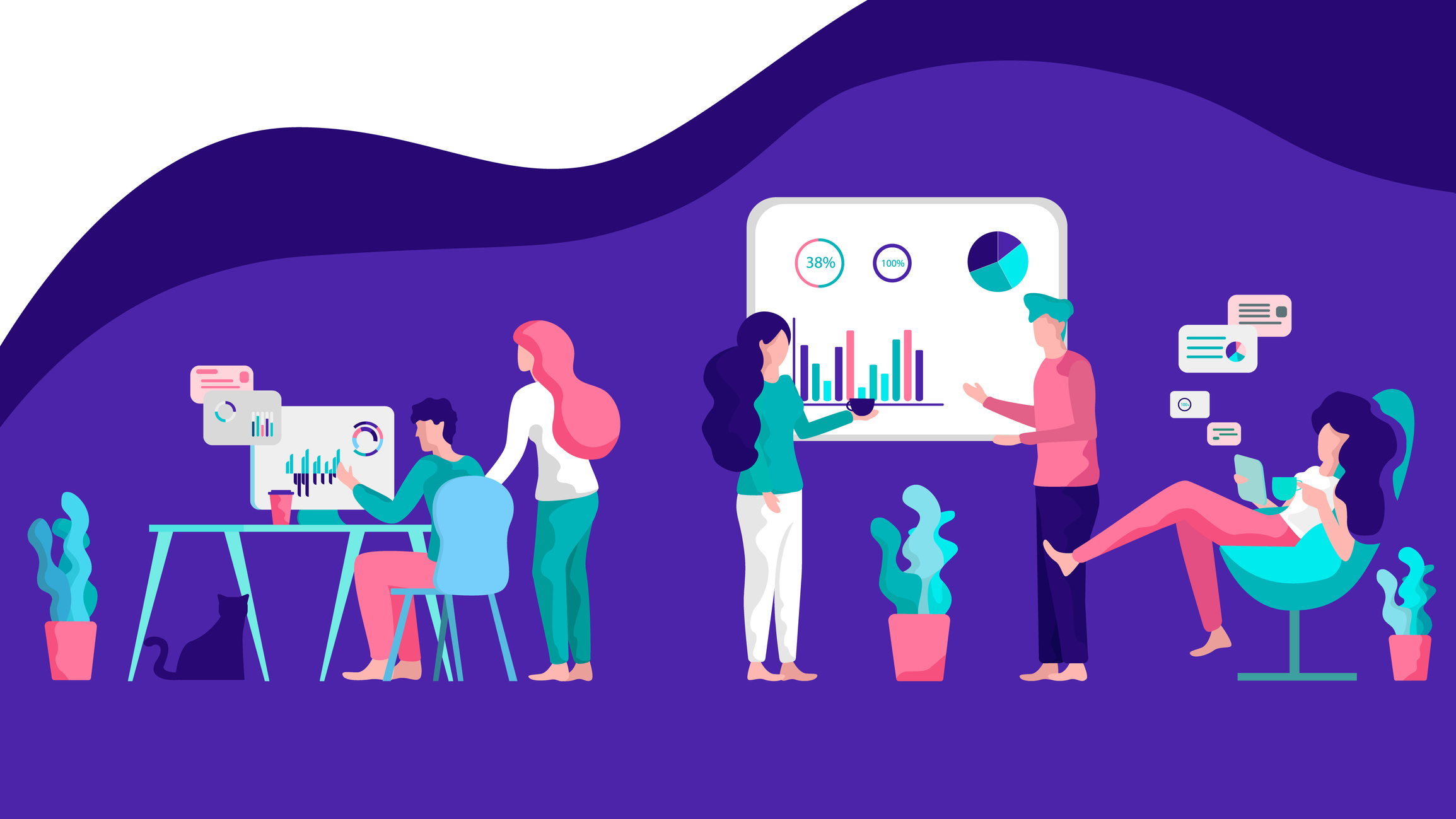
Non-Experimental Research: Overview & Advantages
In traditional experimental research, variables are carefully controlled by researchers in a lab setting. In non-experimental study, there are no variables the observer can directly control.
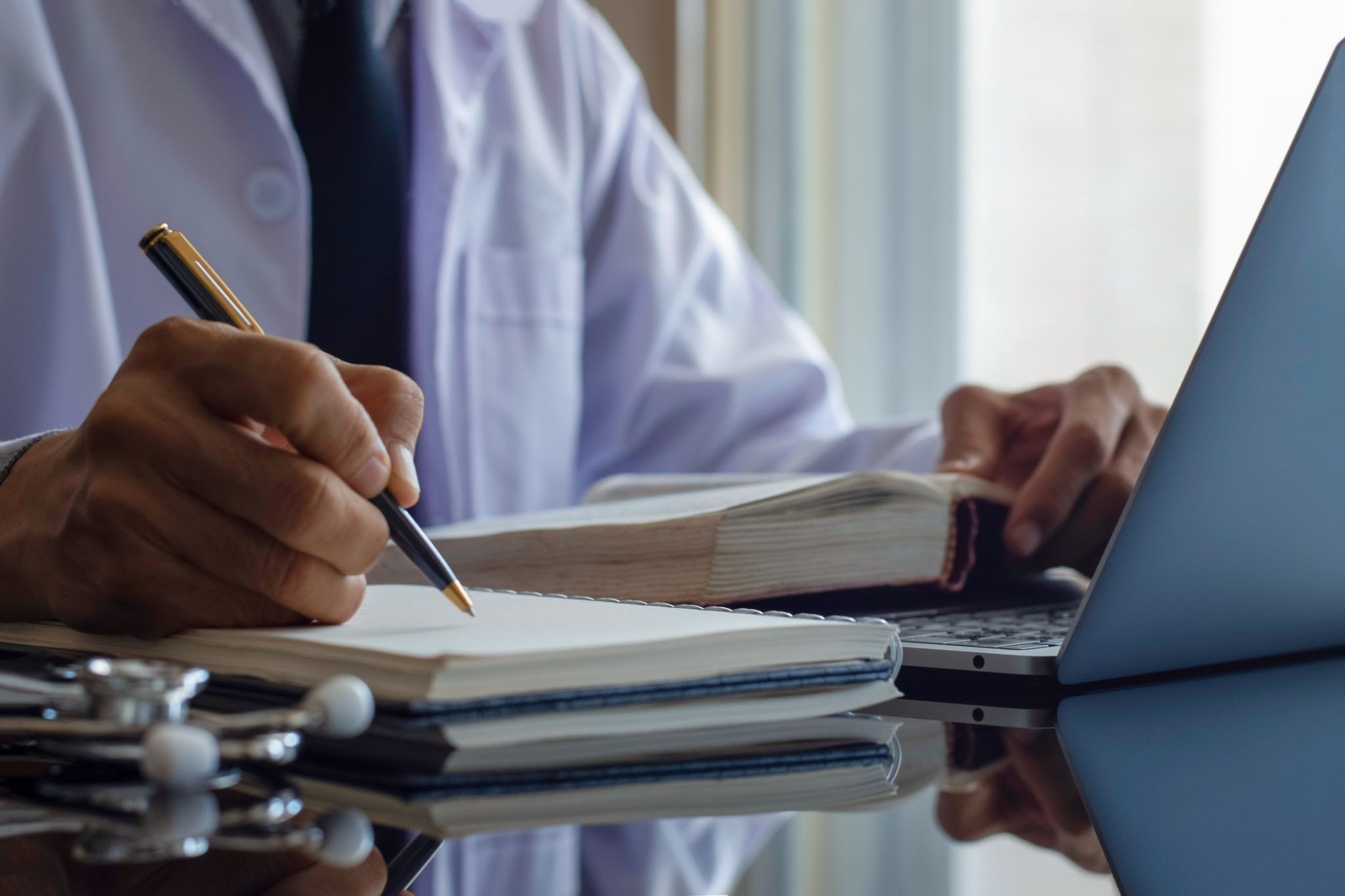
Non-Experimental Research
Non-experimental research gets its name from the fact that there is no independent variable involved in testing. Researchers instead look to take past events and re-examine them; analyzing them for new information and coming to new or supporting conclusions.
In traditional experimental research, variables are carefully controlled by researchers in a lab setting. In non-experimental study, there are no variables the observer can directly control. Instead, researchers are tasked with parsing through established context to come up with their own interpretation of the events. While non-experimental research is limited in use, there are a few key areas where a researcher may find using this kind of methodology is beneficial.
Characteristics of Non-Experimental Research
These key characteristics of non-experimental research set it apart from other common methods:
- The vast majority of these studies are conducted using prior events and past experiences.
- This method is not concerned with establishing links between variables.
- The research collected does not directly influence the events that are being studied.
- This type of testing does not influence or impact the phenomena being studied.
Types of Non-Experimental Research
There are three primary forms of non-experimental research. They are:
Single-Variable Research
Single-variable research involves locating one variable and attempting to discern new meaning from these events. Instead of trying to discern a relationship between two variables, this type of study aims to ganer a deeper understanding of a particular issue - often so that further testing can be completed.
One example of a single-variable research project could involve looking at how high the average person can jump. In this case, researchers would invite participants to make 3 attempts to jump up into the air as high as they could from a standing position; researchers would then average out the 3 attempts into one number. In this case, researchers are not looking to connect the variable jump height with any other piece of information. All the study is concerned about is measuring the average of an individual’s jumps.
Correlational and Quasi-Experimental
Correlational research involves measuring two or more variables of interest while maintaining little or no control over the variables themselves. In the quasi-experimental method, researchers change an independent variable - but will not recruit or control the participants involved in the experiment. An example would be a researcher who starts a campaign urging people to stop smoking in one city - and then comparing those results to cities without a no-smoking program.
Qualitative Research
The qualitative research method seeks to answer complex questions, and involves written documentation of experiences and events. Unlike the quantitative research method, which is concerned with facts and hard data, the qualitative method can be used to gather insights for a breadth of vital topics.
Advantages of Non-Experimental Research
Non-experimental designs can open a number of advantageous research opportunities. The benefits include:
- Non-experimental research can be used to analyze events that have happened in the past.
- The versatility of the model can be used to observe many unique phenomena.
- This method of research is far more affordable than the experimental kind.
Disadvantages of Non-Experimental Research
The limitations of non-experimental research are:
- These limited samples do not represent the larger population.
- The research can only be used to observe a single variable.
- Researcher bias or error in the methodology can lead to inaccurate results.
These disadvantages can be mitigated by applying the non-experimental method to the correct situations.
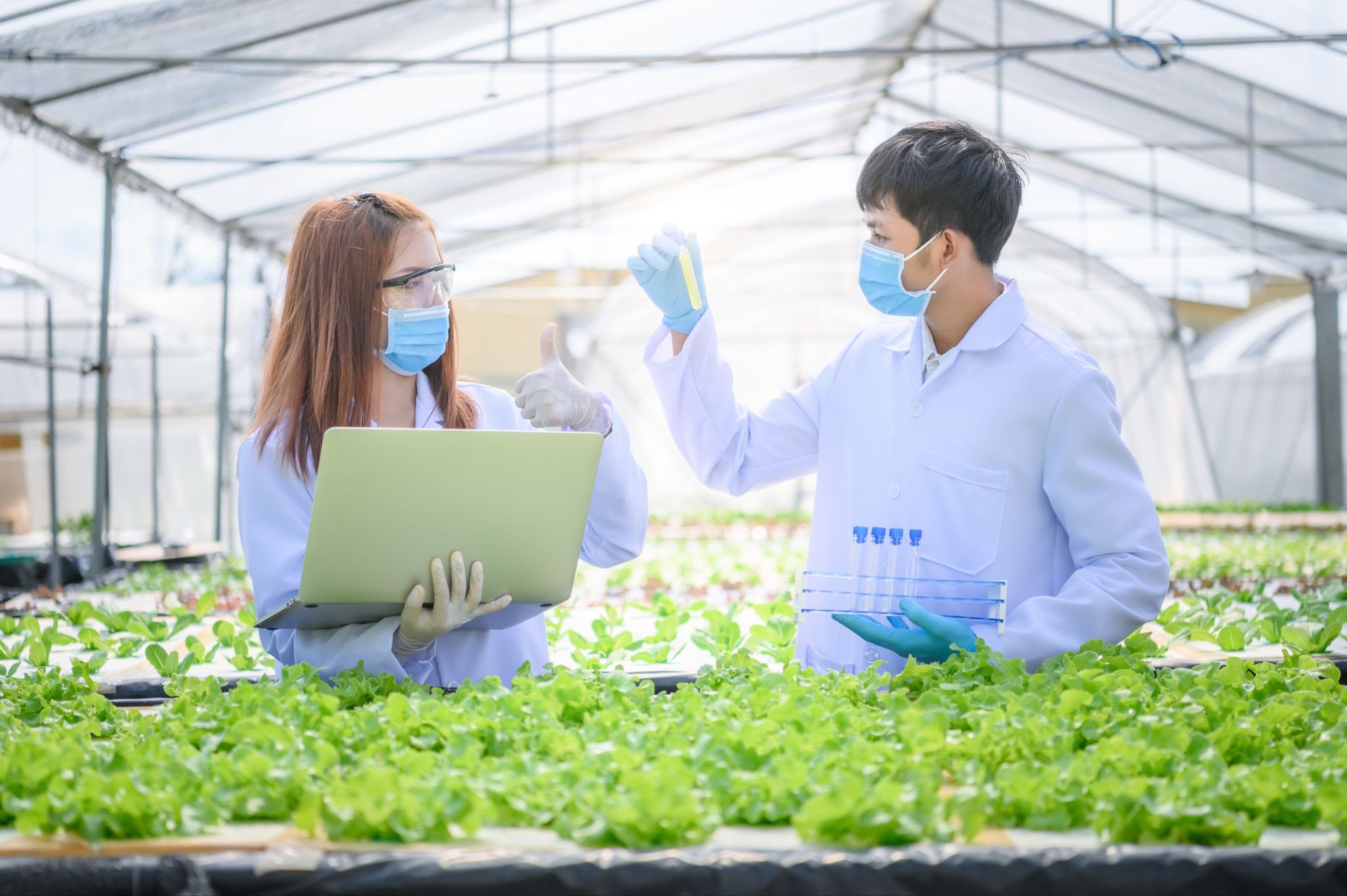
How it is different from experimental research
Experimental research often involves taking two or more variables (independent and dependent) and attempting to develop a causal relationship between them. Experimental designs will be tightly controlled by researchers, and the tests themselves will often be far more intricate and expansive than non-experimental ones.
When to use Non-Experimental Research
Non-experimental research is best suited for situations where you want to observe events that have already happened; or you are only interested in gathering information about one isolated variable.
Experimental designs are far more common in the fields of science: medicine, biology, psychology, and so forth. Non-experimental design often sees use in business, politics, history, and general academia.
Determining when you should use either experimental or non-experimental methods boil down to the purpose of your research.
If the situation calls for direct intervention, then experimental methods offer researchers more tools for changing and measuring independent variables.
The best place to use non-experimental research design is when the question at hand can be answered without altering the independent variable.
About Author
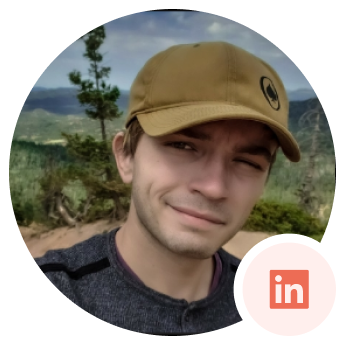
Send Your First Survey Today!
Set up and begin receiving results within minutes. Sign up for free, no contract required.
Helpfull is the easiest way to get feedback from thousands of people in minutes. Whether you're comparing images, text or surveys; our pool of qualified testers give you their real detailed opinion to help you make better decisions and be more informed.
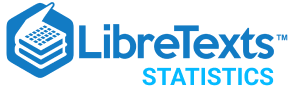
- school Campus Bookshelves
- menu_book Bookshelves
- perm_media Learning Objects
- login Login
- how_to_reg Request Instructor Account
- hub Instructor Commons
- Download Page (PDF)
- Download Full Book (PDF)
- Periodic Table
- Physics Constants
- Scientific Calculator
- Reference & Cite
- Tools expand_more
- Readability
selected template will load here
This action is not available.
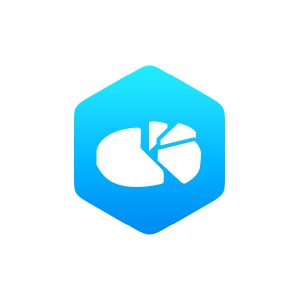
1.11: Experimental and non-experimental research
- Last updated
- Save as PDF
- Page ID 16762
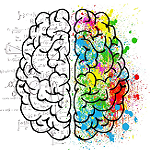
- Matthew J. C. Crump
- Brooklyn College of CUNY
One of the big distinctions that you should be aware of is the distinction between “experimental research” and “non-experimental research”. When we make this distinction, what we’re really talking about is the degree of control that the researcher exercises over the people and events in the study.
Experimental research
The key features of experimental research is that the researcher controls all aspects of the study, especially what participants experience during the study. In particular, the researcher manipulates or varies something (IVs), and then allows the outcome variable (DV) to vary naturally. The idea here is to deliberately vary the something in the world (IVs) to see if it has any causal effects on the outcomes. Moreover, in order to ensure that there’s no chance that something other than the manipulated variable is causing the outcomes, everything else is kept constant or is in some other way “balanced” to ensure that they have no effect on the results. In practice, it’s almost impossible to think of everything else that might have an influence on the outcome of an experiment, much less keep it constant. The standard solution to this is randomization : that is, we randomly assign people to different groups, and then give each group a different treatment (i.e., assign them different values of the predictor variables). We’ll talk more about randomization later in this course, but for now, it’s enough to say that what randomization does is minimize (but not eliminate) the chances that there are any systematic difference between groups.
Let’s consider a very simple, completely unrealistic and grossly unethical example. Suppose you wanted to find out if smoking causes lung cancer. One way to do this would be to find people who smoke and people who don’t smoke, and look to see if smokers have a higher rate of lung cancer. This is not a proper experiment, since the researcher doesn’t have a lot of control over who is and isn’t a smoker. And this really matters: for instance, it might be that people who choose to smoke cigarettes also tend to have poor diets, or maybe they tend to work in asbestos mines, or whatever. The point here is that the groups (smokers and non-smokers) actually differ on lots of things, not just smoking. So it might be that the higher incidence of lung cancer among smokers is caused by something else, not by smoking per se. In technical terms, these other things (e.g. diet) are called “confounds”, and we’ll talk about those in just a moment.
In the meantime, let’s now consider what a proper experiment might look like. Recall that our concern was that smokers and non-smokers might differ in lots of ways. The solution, as long as you have no ethics, is to control who smokes and who doesn’t. Specifically, if we randomly divide participants into two groups, and force half of them to become smokers, then it’s very unlikely that the groups will differ in any respect other than the fact that half of them smoke. That way, if our smoking group gets cancer at a higher rate than the non-smoking group, then we can feel pretty confident that (a) smoking does cause cancer and (b) we’re murderers.
Non-experimental research
Non-experimental research is a broad term that covers “any study in which the researcher doesn’t have quite as much control as they do in an experiment”. Obviously, control is something that scientists like to have, but as the previous example illustrates, there are lots of situations in which you can’t or shouldn’t try to obtain that control. Since it’s grossly unethical (and almost certainly criminal) to force people to smoke in order to find out if they get cancer, this is a good example of a situation in which you really shouldn’t try to obtain experimental control. But there are other reasons too. Even leaving aside the ethical issues, our “smoking experiment” does have a few other issues. For instance, when I suggested that we “force” half of the people to become smokers, I must have been talking about starting with a sample of non-smokers, and then forcing them to become smokers. While this sounds like the kind of solid, evil experimental design that a mad scientist would love, it might not be a very sound way of investigating the effect in the real world. For instance, suppose that smoking only causes lung cancer when people have poor diets, and suppose also that people who normally smoke do tend to have poor diets. However, since the “smokers” in our experiment aren’t “natural” smokers (i.e., we forced non-smokers to become smokers; they didn’t take on all of the other normal, real life characteristics that smokers might tend to possess) they probably have better diets. As such, in this silly example they wouldn’t get lung cancer, and our experiment will fail, because it violates the structure of the “natural” world (the technical name for this is an “artifactual” result; see later).
One distinction worth making between two types of non-experimental research is the difference between quasi-experimental research and case studies . The example I discussed earlier – in which we wanted to examine incidence of lung cancer among smokers and non-smokers, without trying to control who smokes and who doesn’t – is a quasi-experimental design. That is, it’s the same as an experiment, but we don’t control the predictors (IVs). We can still use statistics to analyse the results, it’s just that we have to be a lot more careful.
The alternative approach, case studies, aims to provide a very detailed description of one or a few instances. In general, you can’t use statistics to analyse the results of case studies, and it’s usually very hard to draw any general conclusions about “people in general” from a few isolated examples. However, case studies are very useful in some situations. Firstly, there are situations where you don’t have any alternative: neuropsychology has this issue a lot. Sometimes, you just can’t find a lot of people with brain damage in a specific area, so the only thing you can do is describe those cases that you do have in as much detail and with as much care as you can. However, there’s also some genuine advantages to case studies: because you don’t have as many people to study, you have the ability to invest lots of time and effort trying to understand the specific factors at play in each case. This is a very valuable thing to do. As a consequence, case studies can complement the more statistically-oriented approaches that you see in experimental and quasi-experimental designs. We won’t talk much about case studies in these lectures, but they are nevertheless very valuable tools!
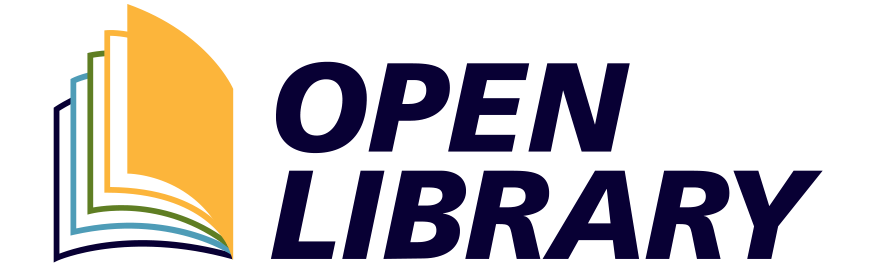
Want to create or adapt books like this? Learn more about how Pressbooks supports open publishing practices.
Overview of Nonexperimental Research
Learning objectives.
- Define nonexperimental research, distinguish it clearly from experimental research, and give several examples.
- Explain when a researcher might choose to conduct nonexperimental research as opposed to experimental research.
What Is Nonexperimental Research?
Nonexperimental research is research that lacks the manipulation of an independent variable, random assignment of participants to conditions or orders of conditions, or both.
In a sense, it is unfair to define this large and diverse set of approaches collectively by what they are not . But doing so reflects the fact that most researchers in psychology consider the distinction between experimental and nonexperimental research to be an extremely important one. This distinction is because although experimental research can provide strong evidence that changes in an independent variable cause differences in a dependent variable, nonexperimental research generally cannot. As we will see, however, this inability does not mean that nonexperimental research is less important than experimental research or inferior to it in any general sense.
When to Use Nonexperimental Research
As we saw in Chapter 6 , experimental research is appropriate when the researcher has a specific research question or hypothesis about a causal relationship between two variables—and it is possible, feasible, and ethical to manipulate the independent variable and randomly assign participants to conditions or to orders of conditions. It stands to reason, therefore, that nonexperimental research is appropriate—even necessary—when these conditions are not met. There are many ways in which preferring nonexperimental research can be the case.
- The research question or hypothesis can be about a single variable rather than a statistical relationship between two variables (e.g., How accurate are people’s first impressions?).
- The research question can be about a noncausal statistical relationship between variables (e.g., Is there a correlation between verbal intelligence and mathematical intelligence?).
- The research question can be about a causal relationship, but the independent variable cannot be manipulated or participants cannot be randomly assigned to conditions or orders of conditions (e.g., Does damage to a person’s hippocampus impair the formation of long-term memory traces?).
- The research question can be broad and exploratory, or it can be about what it is like to have a particular experience (e.g., What is it like to be a working mother diagnosed with depression?).
Again, the choice between the experimental and nonexperimental approaches is generally dictated by the nature of the research question. If it is about a causal relationship and involves an independent variable that can be manipulated, the experimental approach is typically preferred. Otherwise, the nonexperimental approach is preferred. But the two approaches can also be used to address the same research question in complementary ways. For example, nonexperimental studies establishing that there is a relationship between watching violent television and aggressive behaviour have been complemented by experimental studies confirming that the relationship is a causal one (Bushman & Huesmann, 2001) [1] . Similarly, after his original study, Milgram conducted experiments to explore the factors that affect obedience. He manipulated several independent variables, such as the distance between the experimenter and the participant, the participant and the confederate, and the location of the study (Milgram, 1974) [2] .
Types of Nonexperimental Research
Nonexperimental research falls into three broad categories: single-variable research, correlational and quasi-experimental research, and qualitative research. First, research can be nonexperimental because it focuses on a single variable rather than a statistical relationship between two variables. Although there is no widely shared term for this kind of research, we will call it single-variable research . Milgram’s original obedience study was nonexperimental in this way. He was primarily interested in one variable—the extent to which participants obeyed the researcher when he told them to shock the confederate—and he observed all participants performing the same task under the same conditions. The study by Loftus and Pickrell described at the beginning of this chapter is also a good example of single-variable research. The variable was whether participants “remembered” having experienced mildly traumatic childhood events (e.g., getting lost in a shopping mall) that they had not actually experienced but that the research asked them about repeatedly. In this particular study, nearly a third of the participants “remembered” at least one event. (As with Milgram’s original study, this study inspired several later experiments on the factors that affect false memories.)
As these examples make clear, single-variable research can answer interesting and important questions. What it cannot do, however, is answer questions about statistical relationships between variables. This detail is a point that beginning researchers sometimes miss. Imagine, for example, a group of research methods students interested in the relationship between children’s being the victim of bullying and the children’s self-esteem. The first thing that is likely to occur to these researchers is to obtain a sample of middle-school students who have been bullied and then to measure their self-esteem. But this design would be a single-variable study with self-esteem as the only variable. Although it would tell the researchers something about the self-esteem of children who have been bullied, it would not tell them what they really want to know, which is how the self-esteem of children who have been bullied compares with the self-esteem of children who have not. Is it lower? Is it the same? Could it even be higher? To answer this question, their sample would also have to include middle-school students who have not been bullied thereby introducing another variable.
Research can also be nonexperimental because it focuses on a statistical relationship between two variables but does not include the manipulation of an independent variable, random assignment of participants to conditions or orders of conditions, or both. This kind of research takes two basic forms: correlational research and quasi-experimental research. In correlational research , the researcher measures the two variables of interest with little or no attempt to control extraneous variables and then assesses the relationship between them. A research methods student who finds out whether each of several middle-school students has been bullied and then measures each student’s self-esteem is conducting correlational research. In quasi-experimental research , the researcher manipulates an independent variable but does not randomly assign participants to conditions or orders of conditions. For example, a researcher might start an antibullying program (a kind of treatment) at one school and compare the incidence of bullying at that school with the incidence at a similar school that has no antibullying program.
The final way in which research can be nonexperimental is that it can be qualitative. The types of research we have discussed so far are all quantitative, referring to the fact that the data consist of numbers that are analyzed using statistical techniques. In qualitative research , the data are usually nonnumerical and therefore cannot be analyzed using statistical techniques. Rosenhan’s study of the experience of people in a psychiatric ward was primarily qualitative. The data were the notes taken by the “pseudopatients”—the people pretending to have heard voices—along with their hospital records. Rosenhan’s analysis consists mainly of a written description of the experiences of the pseudopatients, supported by several concrete examples. To illustrate the hospital staff’s tendency to “depersonalize” their patients, he noted, “Upon being admitted, I and other pseudopatients took the initial physical examinations in a semipublic room, where staff members went about their own business as if we were not there” (Rosenhan, 1973, p. 256) [3] . Qualitative data has a separate set of analysis tools depending on the research question. For example, thematic analysis would focus on themes that emerge in the data or conversation analysis would focus on the way the words were said in an interview or focus group.
Internal Validity Revisited
Recall that internal validity is the extent to which the design of a study supports the conclusion that changes in the independent variable caused any observed differences in the dependent variable. Figure 7.1 shows how experimental, quasi-experimental, and correlational research vary in terms of internal validity. Experimental research tends to be highest because it addresses the directionality and third-variable problems through manipulation and the control of extraneous variables through random assignment. If the average score on the dependent variable in an experiment differs across conditions, it is quite likely that the independent variable is responsible for that difference. Correlational research is lowest because it fails to address either problem. If the average score on the dependent variable differs across levels of the independent variable, it could be that the independent variable is responsible, but there are other interpretations. In some situations, the direction of causality could be reversed. In others, there could be a third variable that is causing differences in both the independent and dependent variables. Quasi-experimental research is in the middle because the manipulation of the independent variable addresses some problems, but the lack of random assignment and experimental control fails to address others. Imagine, for example, that a researcher finds two similar schools, starts an antibullying program in one, and then finds fewer bullying incidents in that “treatment school” than in the “control school.” There is no directionality problem because clearly the number of bullying incidents did not determine which school got the program. However, the lack of random assignment of children to schools could still mean that students in the treatment school differed from students in the control school in some other way that could explain the difference in bullying.

Notice also in Figure 7.1 that there is some overlap in the internal validity of experiments, quasi-experiments, and correlational studies. For example, a poorly designed experiment that includes many confounding variables can be lower in internal validity than a well designed quasi-experiment with no obvious confounding variables. Internal validity is also only one of several validities that one might consider, as noted in Chapter 5.
Key Takeaways
- Nonexperimental research is research that lacks the manipulation of an independent variable, control of extraneous variables through random assignment, or both.
- There are three broad types of nonexperimental research. Single-variable research focuses on a single variable rather than a relationship between variables. Correlational and quasi-experimental research focus on a statistical relationship but lack manipulation or random assignment. Qualitative research focuses on broader research questions, typically involves collecting large amounts of data from a small number of participants, and analyses the data nonstatistically.
- In general, experimental research is high in internal validity, correlational research is low in internal validity, and quasi-experimental research is in between.
- A researcher conducts detailed interviews with unmarried teenage fathers to learn about how they feel and what they think about their role as fathers and summarizes their feelings in a written narrative.
- A researcher measures the impulsivity of a large sample of drivers and looks at the statistical relationship between this variable and the number of traffic tickets the drivers have received.
- A researcher randomly assigns patients with low back pain either to a treatment involving hypnosis or to a treatment involving exercise. She then measures their level of low back pain after 3 months.
- A college instructor gives weekly quizzes to students in one section of his course but no weekly quizzes to students in another section to see whether this has an effect on their test performance.
- Bushman, B. J., & Huesmann, L. R. (2001). Effects of televised violence on aggression. In D. Singer & J. Singer (Eds.), Handbook of children and the media (pp. 223–254). Thousand Oaks, CA: Sage. ↵
- Milgram, S. (1974). Obedience to authority: An experimental view . New York, NY: Harper & Row. ↵
- Rosenhan, D. L. (1973). On being sane in insane places. Science, 179 , 250–258. ↵
Research Methods in Psychology Copyright © 2015 by Paul C. Price, Rajiv Jhangiani, & I-Chant A. Chiang is licensed under a Creative Commons Attribution-NonCommercial-ShareAlike 4.0 International License , except where otherwise noted.
Share This Book

IMAGES
VIDEO
COMMENTS
Non-experimental research is research that lacks the manipulation of an independent variable. Rather than manipulating an independent variable, researchers conducting non-experimental research simply measure variables as they naturally occur (in the lab or real world). Most researchers in psychology consider the distinction between experimental ...
The pinnacle of non-experimental research is the comparative effectiveness study, which is grouped with other non-experimental study designs such as cross-sectional, case-control, ... Conducting a valid clinical research requires consideration of theoretical study design, data collection design, and statistical analysis design. ...
When to Use Nonexperimental Research. As we saw in Chapter 6 "Experimental Research", experimental research is appropriate when the researcher has a specific research question or hypothesis about a causal relationship between two variables—and it is possible, feasible, and ethical to manipulate the independent variable and randomly assign participants to conditions or to orders of ...
A research methods student who finds out whether each of several middle-school students has been bullied and then measures each student's self-esteem is conducting correlational research. In quasi-experimental research, the researcher manipulates an independent variable but does not randomly assign participants to conditions or orders of ...
Non-experimental research is research that lacks the manipulation of an independent variable. Rather than manipulating an independent variable, researchers conducting non-experimental research simply measure variables as they naturally occur (in the lab or real world). Most researchers in psychology consider the distinction between experimental ...
Non-experimental research is the type of research that lacks an independent variable. Instead, the researcher observes the context in which the phenomenon occurs and analyzes it to obtain information. ... If you need any help with how to conduct research and collect relevant data, or have queries regarding the best approach for your research ...
Non-experimental research is research that lacks the manipulation of an independent variable. Rather than manipulating an independent variable, researchers conducting non-experimental research simply measure variables as they naturally occur (in the lab or real world). Most researchers in psychology consider the distinction between experimental ...
Non-experimental research is research that lacks the manipulation of an independent variable. Rather than manipulating an independent variable, researchers conducting non-experimental research simply measure variables as they naturally occur (in the lab or real world). Most researchers in psychology consider the distinction between experimental ...
A food scientist studies the relationship between the temperature inside people's refrigerators and the amount of bacteria on their food. A social psychologist tells some research participants that they need to hurry over to the next building to complete a study. She tells others that they can take their time.
Non-experimental research is research that lacks the manipulation of an independent variable. Rather than manipulating an independent variable, researchers conducting non-experimental research simply measure variables as they naturally occur (in the lab or real world). Most researchers in social sciences consider the distinction between ...
•Conducting a randomized experiment also forces clarity around the treatment and comparison conditions, outcomes, and timing of measurement -will come back to that! •(Many of the issues are similar to the considerations between probability and non-probability samples in survey research; Mercer et al., POQ, 2017)
Conducting Surveys. 37. Key Takeaways and Exercises. VIII. Quasi-Experimental Research. 38. One-Group Designs. 39. Non-Equivalent Groups Designs. ... We begin with a general definition of non-experimental research, along with a discussion of when and why non-experimental research is more appropriate than experimental research. We then look ...
Non-experimental research is research that lacks the manipulation of an independent variable. Rather than manipulating an independent variable, researchers conducting non-experimental research simply measure variables as they naturally occur (in the lab or real world). Most researchers in psychology consider the distinction between experimental ...
both approaches, aiding the acceptance of non-experimental methods in the clinical research community. Modern design and analytic approaches to reducing or quantifying systematic errors in observational research include propensity score methods, marginal structural models, instrumental variables, externaladjustment,andbiasanalyses[ 2,12,14-19 ...
Non-experimental research is the type of research that does not involve the manipulation of control or independent variable. In non-experimental research, researchers measure variables as they naturally occur without any further manipulation. ... the researcher can conduct further studies to understand the reason behind it. A further study into ...
6.3: Correlational Research. Correlational research is a type of non-experimental research in which the researcher measures two variables and assesses the statistical relationship (i.e., the correlation) between them with little or no effort to control extraneous variables. There are many reasons that researchers interested in statistical ...
Leung and Shek (2018) explain: Experimental research design utilizes the principle of manipulation of the independent variables and examines its cause-and-effect relationship on the dependent variables by controlling the effects of other variables. Usually, the experimenter assigns two or more groups with similar characteristics.
2.5: Experimental and Non-experimental Research. One of the big distinctions that you should be aware of is the distinction between "experimental research" and "non-experimental research". When we make this distinction, what we're really talking about is the degree of control that the researcher exercises over the people and events in ...
Knowledge about the benefit-to-harm balance of alternative treatment options is central to high-quality patient care. In contrast to the traditional hierarchy of evidence, led by randomized designs, the emerging consensus is to move away from judging a study's validity based only on randomization. Ethical, practical, and financial considerations dictate that most epidemiologic research be ...
Non-experimental designs can open a number of advantageous research opportunities. The benefits include: Non-experimental research can be used to analyze events that have happened in the past. The versatility of the model can be used to observe many unique phenomena. This method of research is far more affordable than the experimental kind.
Non-experimental research. Non-experimental research is a broad term that covers "any study in which the researcher doesn't have quite as much control as they do in an experiment". Obviously, control is something that scientists like to have, but as the previous example illustrates, there are lots of situations in which you can't or shouldn't try to obtain that control.
A research methods student who finds out whether each of several middle-school students has been bullied and then measures each student's self-esteem is conducting correlational research. In quasi-experimental research, the researcher manipulates an independent variable but does not randomly assign participants to conditions or orders of ...