- En español – ExME
- Em português – EME

Systematic reviews vs meta-analysis: what’s the difference?
Posted on 24th July 2023 by Verónica Tanco Tellechea
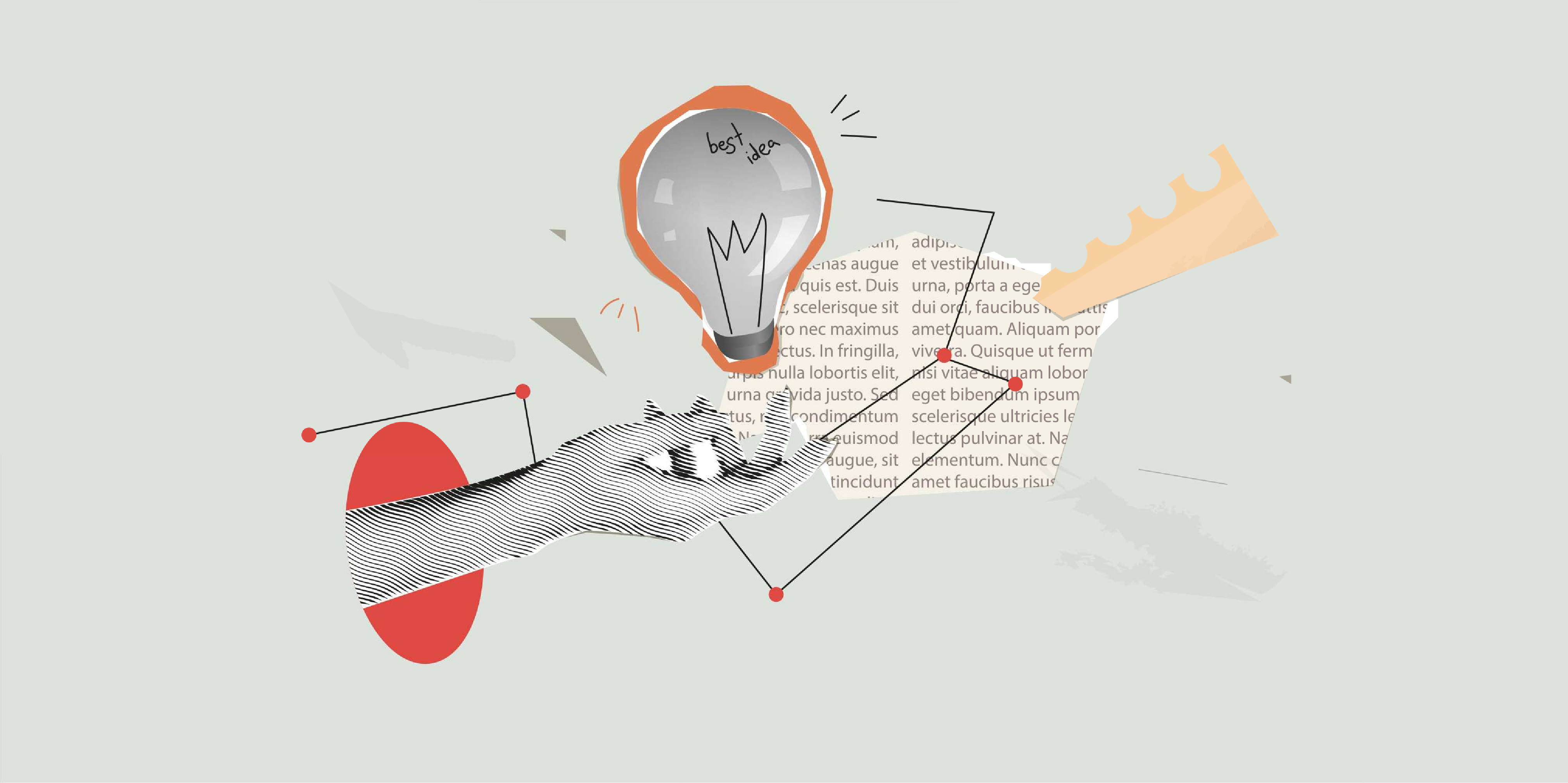
You may hear the terms ‘systematic review’ and ‘meta-analysis being used interchangeably’. Although they are related, they are distinctly different. Learn more in this blog for beginners.
What is a systematic review?
According to Cochrane (1), a systematic review attempts to identify, appraise and synthesize all the empirical evidence to answer a specific research question. Thus, a systematic review is where you might find the most relevant, adequate, and current information regarding a specific topic. In the levels of evidence pyramid , systematic reviews are only surpassed by meta-analyses.
To conduct a systematic review, you will need, among other things:
- A specific research question, usually in the form of a PICO question.
- Pre-specified eligibility criteria, to decide which articles will be included or discarded from the review.
- To follow a systematic method that will minimize bias.
You can find protocols that will guide you from both Cochrane and the Equator Network , among other places, and if you are a beginner to the topic then have a read of an overview about systematic reviews.
What is a meta-analysis?
A meta-analysis is a quantitative, epidemiological study design used to systematically assess the results of previous research (2) . Usually, they are based on randomized controlled trials, though not always. This means that a meta-analysis is a mathematical tool that allows researchers to mathematically combine outcomes from multiple studies.
When can a meta-analysis be implemented?
There is always the possibility of conducting a meta-analysis, yet, for it to throw the best possible results it should be performed when the studies included in the systematic review are of good quality, similar designs, and have similar outcome measures.
Why are meta-analyses important?
Outcomes from a meta-analysis may provide more precise information regarding the estimate of the effect of what is being studied because it merges outcomes from multiple studies. In a meta-analysis, data from various trials are combined and generate an average result (1), which is portrayed in a forest plot diagram. Moreover, meta-analysis also include a funnel plot diagram to visually detect publication bias.
Conclusions
A systematic review is an article that synthesizes available evidence on a certain topic utilizing a specific research question, pre-specified eligibility criteria for including articles, and a systematic method for its production. Whereas a meta-analysis is a quantitative, epidemiological study design used to assess the results of articles included in a systematic-review.
DEFINITION | Synthesis of empirical evidence regarding a specific research question | Statistical tool used with quantitative outcomes of various studies regarding a specific topic |
RESULTS | Synthesizes relevant and current information regarding a specific research question (qualitative). | Merges multiple outcomes from different researches and provides an average result (quantitative). |
Remember: All meta-analyses involve a systematic review, but not all systematic reviews involve a meta-analysis.
If you would like some further reading on this topic, we suggest the following:
The systematic review – a S4BE blog article
Meta-analysis: what, why, and how – a S4BE blog article
The difference between a systematic review and a meta-analysis – a blog article via Covidence
Systematic review vs meta-analysis: what’s the difference? A 5-minute video from Research Masterminds:
- About Cochrane reviews [Internet]. Cochranelibrary.com. [cited 2023 Apr 30]. Available from: https://www.cochranelibrary.com/about/about-cochrane-reviews
- Haidich AB. Meta-analysis in medical research. Hippokratia. 2010;14(Suppl 1):29–37.
Verónica Tanco Tellechea
Leave a reply cancel reply.
Your email address will not be published. Required fields are marked *
Save my name, email, and website in this browser for the next time I comment.
Subscribe to our newsletter
You will receive our monthly newsletter and free access to Trip Premium.
Related Articles
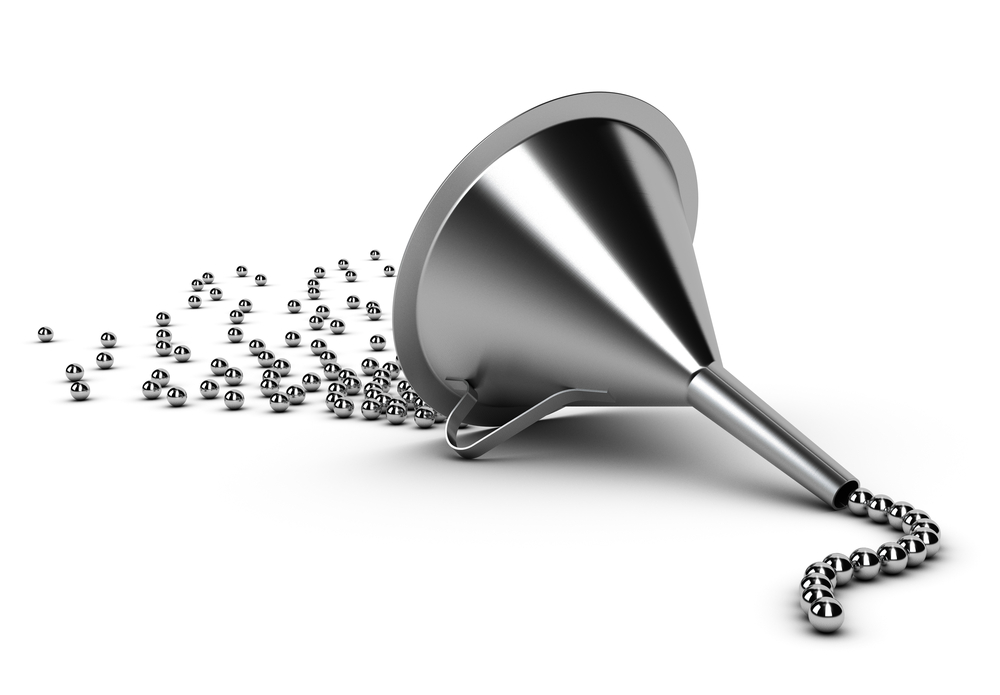
How to read a funnel plot
This blog introduces you to funnel plots, guiding you through how to read them and what may cause them to look asymmetrical.
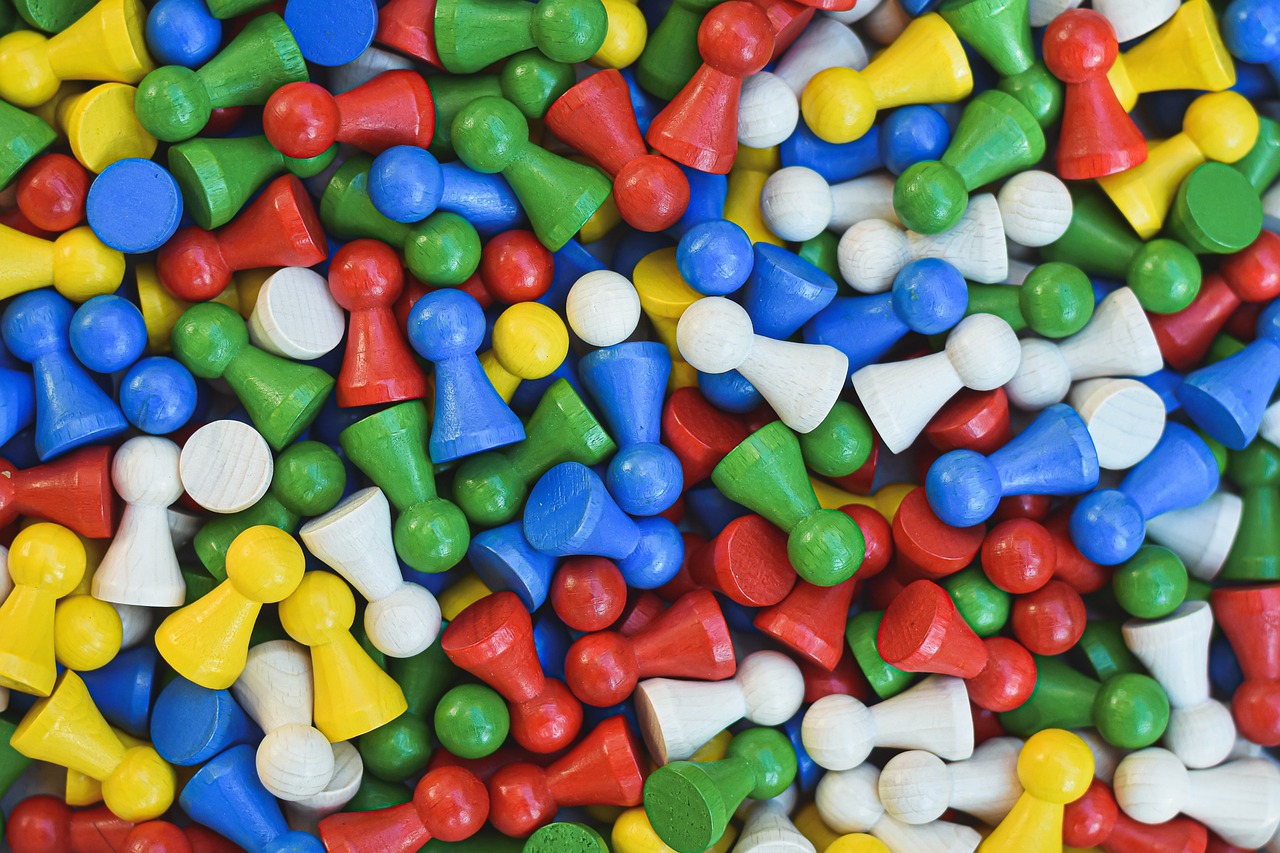
Heterogeneity in meta-analysis
When you bring studies together in a meta-analysis, one of the things you need to consider is the variability in your studies – this is called heterogeneity. This blog presents the three types of heterogeneity, considers the different types of outcome data, and delves a little more into dealing with the variations.
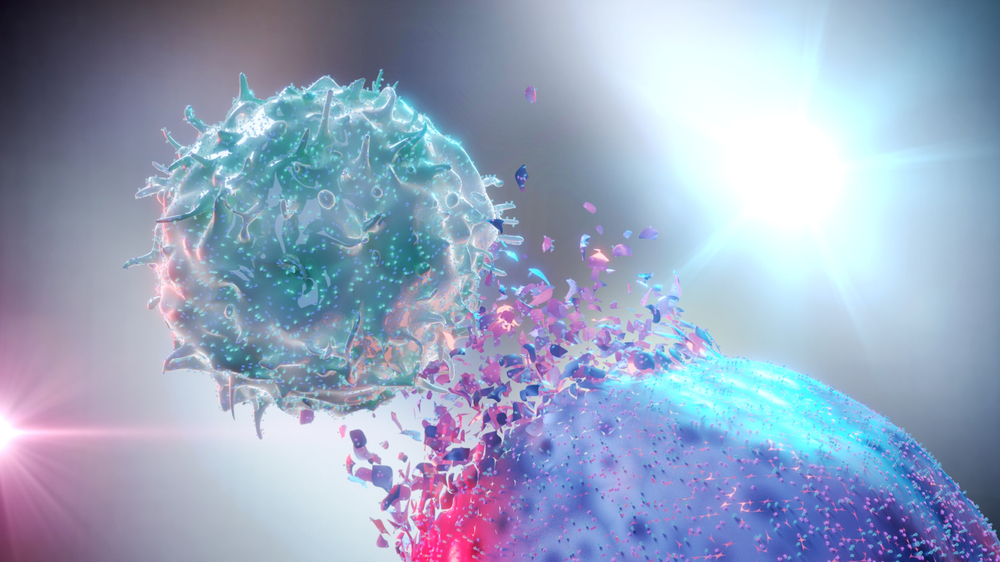
Natural killer cells in glioblastoma therapy
As seen in a previous blog from Davide, modern neuroscience often interfaces with other medical specialities. In this blog, he provides a summary of new evidence about the potential of a therapeutic strategy born at the crossroad between neurology, immunology and oncology.

An official website of the United States government
The .gov means it’s official. Federal government websites often end in .gov or .mil. Before sharing sensitive information, make sure you’re on a federal government site.
The site is secure. The https:// ensures that you are connecting to the official website and that any information you provide is encrypted and transmitted securely.
- Publications
- Account settings
- My Bibliography
- Collections
- Citation manager
Save citation to file
Email citation, add to collections.
- Create a new collection
- Add to an existing collection
Add to My Bibliography
Your saved search, create a file for external citation management software, your rss feed.
- Search in PubMed
- Search in NLM Catalog
- Add to Search
Systematic Reviews and Meta-Analysis: A Guide for Beginners
Affiliation.
- 1 Department of Pediatrics, Advanced Pediatrics Centre, PGIMER, Chandigarh. Correspondence to: Prof Joseph L Mathew, Department of Pediatrics, Advanced Pediatrics Centre, PGIMER Chandigarh. [email protected].
- PMID: 34183469
- PMCID: PMC9065227
- DOI: 10.1007/s13312-022-2500-y
Systematic reviews involve the application of scientific methods to reduce bias in review of literature. The key components of a systematic review are a well-defined research question, comprehensive literature search to identify all studies that potentially address the question, systematic assembly of the studies that answer the question, critical appraisal of the methodological quality of the included studies, data extraction and analysis (with and without statistics), and considerations towards applicability of the evidence generated in a systematic review. These key features can be remembered as six 'A'; Ask, Access, Assimilate, Appraise, Analyze and Apply. Meta-analysis is a statistical tool that provides pooled estimates of effect from the data extracted from individual studies in the systematic review. The graphical output of meta-analysis is a forest plot which provides information on individual studies and the pooled effect. Systematic reviews of literature can be undertaken for all types of questions, and all types of study designs. This article highlights the key features of systematic reviews, and is designed to help readers understand and interpret them. It can also help to serve as a beginner's guide for both users and producers of systematic reviews and to appreciate some of the methodological issues.
PubMed Disclaimer
Similar articles
- The future of Cochrane Neonatal. Soll RF, Ovelman C, McGuire W. Soll RF, et al. Early Hum Dev. 2020 Nov;150:105191. doi: 10.1016/j.earlhumdev.2020.105191. Epub 2020 Sep 12. Early Hum Dev. 2020. PMID: 33036834
- How to review and assess a systematic review and meta-analysis article: a methodological study (secondary publication). Myung SK. Myung SK. J Educ Eval Health Prof. 2023;20:24. doi: 10.3352/jeehp.2023.20.24. Epub 2023 Aug 27. J Educ Eval Health Prof. 2023. PMID: 37619974 Free PMC article.
- How to Critically Appraise and Interpret Systematic Reviews and Meta-Analyses of Diagnostic Accuracy: A User Guide. Frank RA, Salameh JP, Islam N, Yang B, Murad MH, Mustafa R, Leeflang M, Bossuyt PM, Takwoingi Y, Whiting P, Dawit H, Kang SK, Ebrahimzadeh S, Levis B, Hutton B, McInnes MDF. Frank RA, et al. Radiology. 2023 May;307(3):e221437. doi: 10.1148/radiol.221437. Epub 2023 Mar 14. Radiology. 2023. PMID: 36916896 Free PMC article. Review.
- Detecting, quantifying and adjusting for publication bias in meta-analyses: protocol of a systematic review on methods. Mueller KF, Meerpohl JJ, Briel M, Antes G, von Elm E, Lang B, Gloy V, Motschall E, Schwarzer G, Bassler D. Mueller KF, et al. Syst Rev. 2013 Jul 25;2:60. doi: 10.1186/2046-4053-2-60. Syst Rev. 2013. PMID: 23885765 Free PMC article.
- Characteristics, quality and volume of the first 5 months of the COVID-19 evidence synthesis infodemic: a meta-research study. Abbott R, Bethel A, Rogers M, Whear R, Orr N, Shaw L, Stein K, Thompson Coon J. Abbott R, et al. BMJ Evid Based Med. 2022 Jun;27(3):169-177. doi: 10.1136/bmjebm-2021-111710. Epub 2021 Jun 3. BMJ Evid Based Med. 2022. PMID: 34083212 Free PMC article. Review.
- Italian and Middle Eastern adherence to Mediterranean diet in relation to Body Mass Index and non-communicable diseases: nutritional adequacy of simulated weekly food plans. Luconi E, Tosi M, Boracchi P, Colonna I, Rappocciolo E, Ferraretto A, Lorenzini EC. Luconi E, et al. J Transl Med. 2024 Jul 30;22(1):703. doi: 10.1186/s12967-024-05325-1. J Transl Med. 2024. PMID: 39080668 Free PMC article.
- A commentary on 'Efficacy of electroacupuncture in improving postoperative ileus in patients receiving colorectal surgery: a systematic review and meta-analysis'. Zhou M, Ye H, Ma R. Zhou M, et al. Int J Surg. 2024 Aug 1;110(8):5290-5291. doi: 10.1097/JS9.0000000000001564. Int J Surg. 2024. PMID: 38716889 Free PMC article. No abstract available.
- Evidence, Experience, and Eminence: Building Blocks for Pediatric Pulmonology Practice and Research in India. Mathew JL. Mathew JL. Indian J Pediatr. 2023 Jul;90(7):690-692. doi: 10.1007/s12098-023-04649-y. Epub 2023 Jun 2. Indian J Pediatr. 2023. PMID: 37264278 Free PMC article. No abstract available.
- Efficiency, Economy and Excellence: Experimental Exploration of Evidence-based Guideline Development in Resource-Constrained Settings. Mathew JL. Mathew JL. Indian J Pediatr. 2023 Jul;90(7):700-707. doi: 10.1007/s12098-023-04575-z. Epub 2023 Apr 28. Indian J Pediatr. 2023. PMID: 37106227 Free PMC article. Review.
- Sackett D, Strauss S, Richardson W, et al. Evidence-Based Medicine: How to practice and teach EBM. 2nd ed. Churchill Livingstone: 2000.
- Cook DJ, Mulrow CD, Haynes RB. Systematic reviews: Synthesis of best evidence for clinical decisions. Ann Intern Med. 1997;126:376–80. doi: 10.7326/0003-4819-126-5-199703010-00006. - DOI - PubMed
- PennState Eberley College of Science. Lesson 4: Bias and Random Error. Accessed October 01, 2020. Available from: https://online.stat.psu.edu/stat509/node/26/
- Comprehensive Meta-analysis. Accessed October 01, 2020. Available from: https://www.meta-analysis.com/pages/why_do.php?cart=
- National Institute for Health Research. PROSPERO International prospective register of systematic reviews. Accessed October 01, 2020. Available from: https://utas.libguides.com/SystematicReviews/Protocol
Publication types
- Search in MeSH
LinkOut - more resources
Full text sources.
- Europe PubMed Central
- Indian Pediatrics
- PubMed Central

- Citation Manager
NCBI Literature Resources
MeSH PMC Bookshelf Disclaimer
The PubMed wordmark and PubMed logo are registered trademarks of the U.S. Department of Health and Human Services (HHS). Unauthorized use of these marks is strictly prohibited.
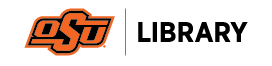
Literature Review, Systematic Review and Meta-analysis
Literature reviews can be a good way to narrow down theoretical interests; refine a research question; understand contemporary debates; and orientate a particular research project. It is very common for PhD theses to contain some element of reviewing the literature around a particular topic. It’s typical to have an entire chapter devoted to reporting the result of this task, identifying gaps in the literature and framing the collection of additional data.
Systematic review is a type of literature review that uses systematic methods to collect secondary data, critically appraise research studies, and synthesise findings. Systematic reviews are designed to provide a comprehensive, exhaustive summary of current theories and/or evidence and published research (Siddaway, Wood & Hedges, 2019) and may be qualitative or qualitative. Relevant studies and literature are identified through a research question, summarised and synthesized into a discrete set of findings or a description of the state-of-the-art. This might result in a ‘literature review’ chapter in a doctoral thesis, but can also be the basis of an entire research project.
Meta-analysis is a specialised type of systematic review which is quantitative and rigorous, often comparing data and results across multiple similar studies. This is a common approach in medical research where several papers might report the results of trials of a particular treatment, for instance. The meta-analysis then statistical techniques to synthesize these into one summary. This can have a high statistical power but care must be taken not to introduce bias in the selection and filtering of evidence.
Whichever type of review is employed, the process is similarly linear. The first step is to frame a question which can guide the review. This is used to identify relevant literature, often through searching subject-specific scientific databases. From these results the most relevant will be identified. Filtering is important here as there will be time constraints that prevent the researcher considering every possible piece of evidence or theoretical viewpoint. Once a concrete evidence base has been identified, the researcher extracts relevant data before reporting the synthesized results in an extended piece of writing.
Literature Review: GO-GN Insights
Sarah Lambert used a systematic review of literature with both qualitative and quantitative phases to investigate the question “How can open education programs be reconceptualised as acts of social justice to improve the access, participation and success of those who are traditionally excluded from higher education knowledge and skills?”
“My PhD research used systematic review, qualitative synthesis, case study and discourse analysis techniques, each was underpinned and made coherent by a consistent critical inquiry methodology and an overarching research question. “Systematic reviews are becoming increasingly popular as a way to collect evidence of what works across multiple contexts and can be said to address some of the weaknesses of case study designs which provide detail about a particular context – but which is often not replicable in other socio-cultural contexts (such as other countries or states.) Publication of systematic reviews that are done according to well defined methods are quite likely to be published in high-ranking journals – my PhD supervisors were keen on this from the outset and I was encouraged along this path. “Previously I had explored social realist authors and a social realist approach to systematic reviews (Pawson on realist reviews) but they did not sufficiently embrace social relations, issues of power, inclusion/exclusion. My supervisors had pushed me to explain what kind of realist review I intended to undertake, and I found out there was a branch of critical realism which was briefly of interest. By getting deeply into theory and trying out ways of combining theory I also feel that I have developed a deeper understanding of conceptual working and the different ways theories can be used at all stagesof research and even how to come up with novel conceptual frameworks.”
Useful references for Systematic Review & Meta-Analysis: Finfgeld-Connett (2014); Lambert (2020); Siddaway, Wood & Hedges (2019)
Research Methods Handbook Copyright © 2020 by Rob Farrow; Francisco Iniesto; Martin Weller; and Rebecca Pitt is licensed under a Creative Commons Attribution 4.0 International License , except where otherwise noted.
Share This Book
Covidence website will be inaccessible as we upgrading our platform on Monday 23rd August at 10am AEST, / 2am CEST/1am BST (Sunday, 15th August 8pm EDT/5pm PDT)
The difference between a systematic review and a meta-analysis
- Best Practice
Home | Blog | Best Practice | The difference between a systematic review and a meta-analysis
Covidence explains the difference between systematic review & meta-analysis.
Systematic review and meta-analysis are two terms that you might see used interchangeably. Each term refers to research about research, but there are important differences!
A systematic review is a piece of work that asks a research question and then answers it by summarising the evidence that meets a set of pre-specified criteria. Some systematic reviews present their results using meta-analysis, a statistical method that combines the results of several trials to generate an average result. Meta-analysis adds value because it can produce a more precise estimate of the effect of a treatment than considering each study individually 🎯.
Let’s take a look at a few related questions that you might have about systematic reviews and meta-analysis.
🙋🏽♂️ What are the stages of a systematic review?
A systematic review starts with a research question and a protocol or research plan. A review team searches for studies to answer the question using a highly sensitive search strategy. The retrieved studies are then screened for eligibility using the inclusion and exclusion criteria (this is done by at least two people working independently). Next, the reviewers extract the relevant data and assess the quality of the included studies. Finally, the review team synthesises the extracted study data (perhaps using meta-analysis) and presents the results. The process is shown in figure 1.
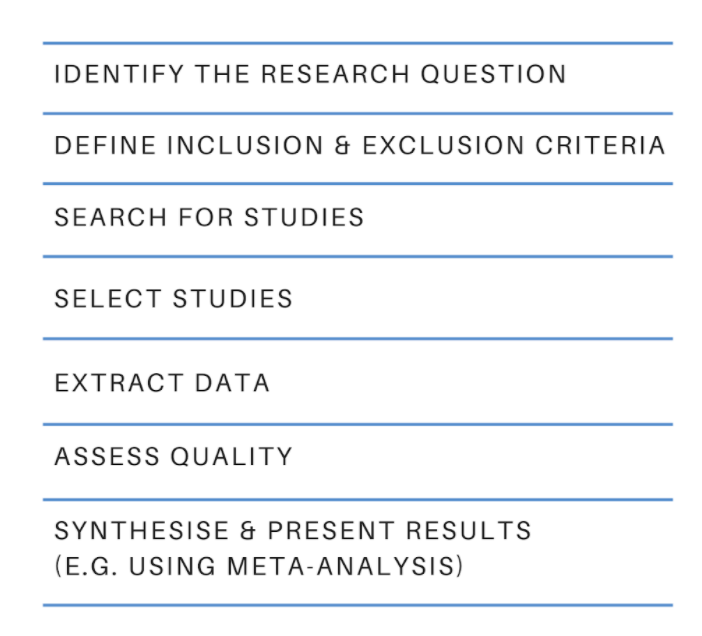
Covidence helps researchers complete systematic review quickly and easily! It supports reviewers with study selection, data extraction and quality assessment. Data exported from Covidence can be saved in Excel for reliable transfer to your choice of data analysis software or, if you’re writing a Cochrane Review, to RevMan 5.
🙋🏻♀️ What does 'systematic' actually mean?
In this context, systematic means that the methods used to search for and analyse the data are
transparent, reproducible and defined before searching begins. This is what differentiates a systematic review from a descriptive review that might be based on, for example, a subset of the literature that the author is familiar with at the time of writing. Systematic reviews strive to be as thorough and rigorous as possible to minimise the bias that would result from cherry-picking studies in a non-systematic way. Systematic reviews sit at the top of the evidence hierarchy because it is widely agreed that studies with rigorous methods are those best able to minimise the risk of bias on the results of the study. This is what makes systematic reviews the most reliable form of evidence (see figure 2).
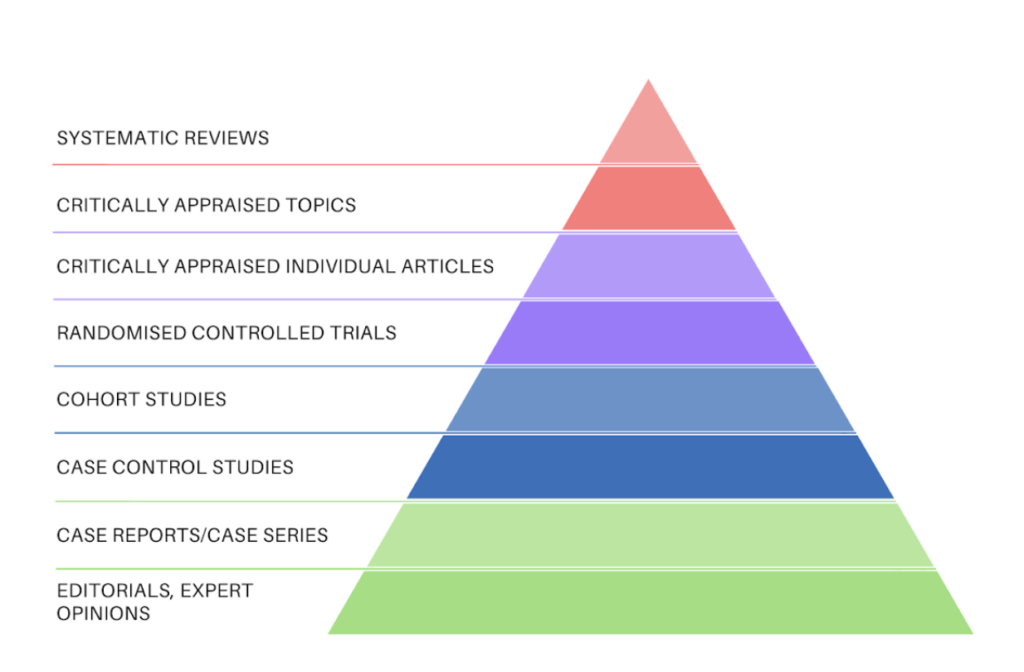
🙋🏾♂️ Why don't all systematic reviews use meta-analysis?
Meta-analysis can improve the precision of an effect estimate. But it can also be misleading if it is performed with data that are not sufficiently similar, or with data whose methodological quality is poor (for example, because the study participants were not properly randomized). So it’s not always appropriate to use meta-analysis and many systematic reviews do not include them. Reviews that do not contain meta-analysis can still synthesise study data to produce something that has greater value than the sum of its parts.
🙋🏾♀️ What does meta-analysis do?
Meta-analysis produces a more precise estimate of treatment effect. There are several types of effect size and the most suitable type is chosen by the review team based on the type of outcomes and interventions under investigation. Typical effect sizes in systematic reviews are the odds ratio, the risk ratio, the weighted mean difference and the standardized mean difference. The results of a meta-analysis are displayed using a forest plot like the one in figure 3.
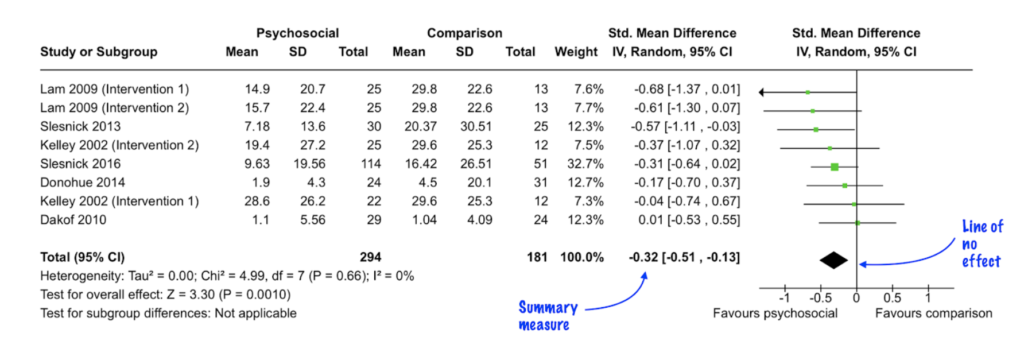
Some meta-analyses also include subgroup analysis or meta-regression. These techniques are used to explore a factor (for example, the age of the study participant) that might influence the relationship between the treatment and the intervention. Plans to analyse the data using these techniques should be described and justified before looking at the data, ideally at the research plan or protocol stage, to avoid introducing bias. Like meta-analysis, subgroup analysis and meta-regression are advisable only in certain circumstances.
Systematic reviewer pro-tip
Think carefully before you plan subgroup analysis or meta-regression and always ask a methodologist for advice
🙋🏼♀️ What are the other ways to synthesise evidence?
Systematic reviews combine study data in a number of ways to reach an overall understanding of the evidence. Meta-analysis is a type of statistical synthesis. Narrative synthesis combines the findings of multiple studies using words. All systematic reviews, including those that use meta-analysis, are likely to contain an element of narrative synthesis by summarising in words the evidence included in the review. But narrative synthesis doesn’t just describe the included studies: it also seeks to explain the gathered evidence, for example by looking at similarities and differences between the study findings and by exploring possible reasons for those similarities and differences in a systematic way. Narrative synthesis should not be confused with narrative review, which is a term sometimes used for a non-systematic review of the literature (for example in a textbook chapter) where there is no systematic attempt to address issues of bias.
There are many types of systematic review . What they all have in common is the use of transparent and reproducible methods that are defined before the search begins. There is no ‘best’ way to synthesise systematic review evidence, and the most suitable approach will depend on factors such as the nature of the review question, the type of intervention and the outcomes of interest.
Covidence is a web-based tool that saves you time at the screening, selection, data extraction and quality assessment stages of your review. It provides easy collaboration across teams and a clear overview of task status, helping you to efficiently complete your review. Sign up for a free trial today! 😀
1 Effectiveness of psychosocial interventions for reducing parental substance misuse – McGovern, R – 2021 | Cochrane Library https://www.cochranelibrary.com/cdsr/doi/10.1002/14651858.CD012823.pub2/full . Accessed 25 March 2021
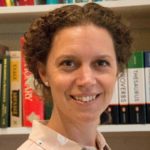
Laura Mellor. Portsmouth, UK
Perhaps you'd also like....
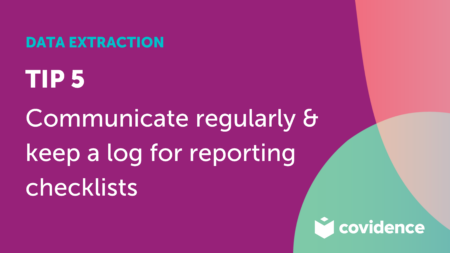
Data Extraction Tip 5: Communicate Regularly
The Covidence Global Scholarship recipients are putting evidence-based research into practice. We caught up with some of the winners to discover the impact of their work and find out more about their experiences.
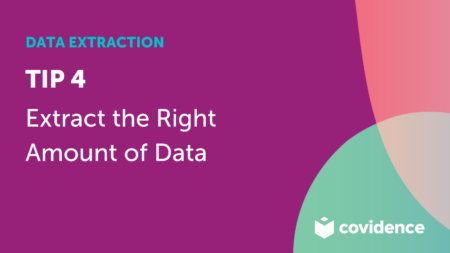
Data Extraction Tip 4: Extract the Right Amount of Data
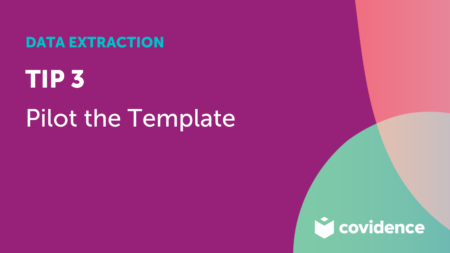
Data Extraction Tip 3: Pilot the Template
Better systematic review management, head office, working for an institution or organisation.
Find out why over 350 of the world’s leading institutions are seeing a surge in publications since using Covidence!
Request a consultation with one of our team members and start empowering your researchers:
By using our site you consent to our use of cookies to measure and improve our site’s performance. Please see our Privacy Policy for more information.
Jump to navigation

Cochrane Training
Chapter 10: analysing data and undertaking meta-analyses.
Jonathan J Deeks, Julian PT Higgins, Douglas G Altman; on behalf of the Cochrane Statistical Methods Group
Key Points:
- Meta-analysis is the statistical combination of results from two or more separate studies.
- Potential advantages of meta-analyses include an improvement in precision, the ability to answer questions not posed by individual studies, and the opportunity to settle controversies arising from conflicting claims. However, they also have the potential to mislead seriously, particularly if specific study designs, within-study biases, variation across studies, and reporting biases are not carefully considered.
- It is important to be familiar with the type of data (e.g. dichotomous, continuous) that result from measurement of an outcome in an individual study, and to choose suitable effect measures for comparing intervention groups.
- Most meta-analysis methods are variations on a weighted average of the effect estimates from the different studies.
- Studies with no events contribute no information about the risk ratio or odds ratio. For rare events, the Peto method has been observed to be less biased and more powerful than other methods.
- Variation across studies (heterogeneity) must be considered, although most Cochrane Reviews do not have enough studies to allow for the reliable investigation of its causes. Random-effects meta-analyses allow for heterogeneity by assuming that underlying effects follow a normal distribution, but they must be interpreted carefully. Prediction intervals from random-effects meta-analyses are a useful device for presenting the extent of between-study variation.
- Many judgements are required in the process of preparing a meta-analysis. Sensitivity analyses should be used to examine whether overall findings are robust to potentially influential decisions.
Cite this chapter as: Deeks JJ, Higgins JPT, Altman DG (editors). Chapter 10: Analysing data and undertaking meta-analyses. In: Higgins JPT, Thomas J, Chandler J, Cumpston M, Li T, Page MJ, Welch VA (editors). Cochrane Handbook for Systematic Reviews of Interventions version 6.4 (updated August 2023). Cochrane, 2023. Available from www.training.cochrane.org/handbook .
10.1 Do not start here!
It can be tempting to jump prematurely into a statistical analysis when undertaking a systematic review. The production of a diamond at the bottom of a plot is an exciting moment for many authors, but results of meta-analyses can be very misleading if suitable attention has not been given to formulating the review question; specifying eligibility criteria; identifying and selecting studies; collecting appropriate data; considering risk of bias; planning intervention comparisons; and deciding what data would be meaningful to analyse. Review authors should consult the chapters that precede this one before a meta-analysis is undertaken.
10.2 Introduction to meta-analysis
An important step in a systematic review is the thoughtful consideration of whether it is appropriate to combine the numerical results of all, or perhaps some, of the studies. Such a meta-analysis yields an overall statistic (together with its confidence interval) that summarizes the effectiveness of an experimental intervention compared with a comparator intervention. Potential advantages of meta-analyses include the following:
- T o improve precision . Many studies are too small to provide convincing evidence about intervention effects in isolation. Estimation is usually improved when it is based on more information.
- To answer questions not posed by the individual studies . Primary studies often involve a specific type of participant and explicitly defined interventions. A selection of studies in which these characteristics differ can allow investigation of the consistency of effect across a wider range of populations and interventions. It may also, if relevant, allow reasons for differences in effect estimates to be investigated.
- To settle controversies arising from apparently conflicting studies or to generate new hypotheses . Statistical synthesis of findings allows the degree of conflict to be formally assessed, and reasons for different results to be explored and quantified.
Of course, the use of statistical synthesis methods does not guarantee that the results of a review are valid, any more than it does for a primary study. Moreover, like any tool, statistical methods can be misused.
This chapter describes the principles and methods used to carry out a meta-analysis for a comparison of two interventions for the main types of data encountered. The use of network meta-analysis to compare more than two interventions is addressed in Chapter 11 . Formulae for most of the methods described are provided in the RevMan Web Knowledge Base under Statistical Algorithms and calculations used in Review Manager (documentation.cochrane.org/revman-kb/statistical-methods-210600101.html), and a longer discussion of many of the issues is available ( Deeks et al 2001 ).
10.2.1 Principles of meta-analysis
The commonly used methods for meta-analysis follow the following basic principles:
- Meta-analysis is typically a two-stage process. In the first stage, a summary statistic is calculated for each study, to describe the observed intervention effect in the same way for every study. For example, the summary statistic may be a risk ratio if the data are dichotomous, or a difference between means if the data are continuous (see Chapter 6 ).

- The combination of intervention effect estimates across studies may optionally incorporate an assumption that the studies are not all estimating the same intervention effect, but estimate intervention effects that follow a distribution across studies. This is the basis of a random-effects meta-analysis (see Section 10.10.4 ). Alternatively, if it is assumed that each study is estimating exactly the same quantity, then a fixed-effect meta-analysis is performed.
- The standard error of the summary intervention effect can be used to derive a confidence interval, which communicates the precision (or uncertainty) of the summary estimate; and to derive a P value, which communicates the strength of the evidence against the null hypothesis of no intervention effect.
- As well as yielding a summary quantification of the intervention effect, all methods of meta-analysis can incorporate an assessment of whether the variation among the results of the separate studies is compatible with random variation, or whether it is large enough to indicate inconsistency of intervention effects across studies (see Section 10.10 ).
- The problem of missing data is one of the numerous practical considerations that must be thought through when undertaking a meta-analysis. In particular, review authors should consider the implications of missing outcome data from individual participants (due to losses to follow-up or exclusions from analysis) (see Section 10.12 ).
Meta-analyses are usually illustrated using a forest plot . An example appears in Figure 10.2.a . A forest plot displays effect estimates and confidence intervals for both individual studies and meta-analyses (Lewis and Clarke 2001). Each study is represented by a block at the point estimate of intervention effect with a horizontal line extending either side of the block. The area of the block indicates the weight assigned to that study in the meta-analysis while the horizontal line depicts the confidence interval (usually with a 95% level of confidence). The area of the block and the confidence interval convey similar information, but both make different contributions to the graphic. The confidence interval depicts the range of intervention effects compatible with the study’s result. The size of the block draws the eye towards the studies with larger weight (usually those with narrower confidence intervals), which dominate the calculation of the summary result, presented as a diamond at the bottom.
Figure 10.2.a Example of a forest plot from a review of interventions to promote ownership of smoke alarms (DiGuiseppi and Higgins 2001). Reproduced with permission of John Wiley & Sons
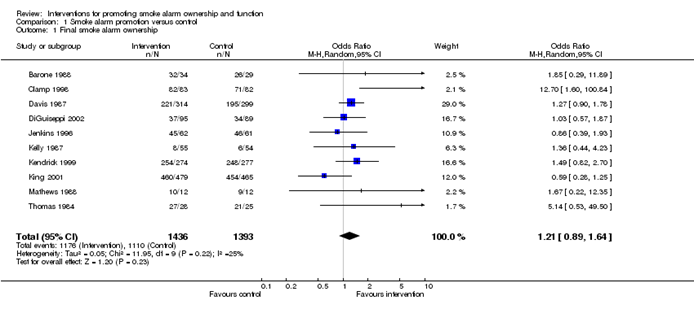
10.3 A generic inverse-variance approach to meta-analysis
A very common and simple version of the meta-analysis procedure is commonly referred to as the inverse-variance method . This approach is implemented in its most basic form in RevMan, and is used behind the scenes in many meta-analyses of both dichotomous and continuous data.
The inverse-variance method is so named because the weight given to each study is chosen to be the inverse of the variance of the effect estimate (i.e. 1 over the square of its standard error). Thus, larger studies, which have smaller standard errors, are given more weight than smaller studies, which have larger standard errors. This choice of weights minimizes the imprecision (uncertainty) of the pooled effect estimate.
10.3.1 Fixed-effect method for meta-analysis
A fixed-effect meta-analysis using the inverse-variance method calculates a weighted average as:

where Y i is the intervention effect estimated in the i th study, SE i is the standard error of that estimate, and the summation is across all studies. The basic data required for the analysis are therefore an estimate of the intervention effect and its standard error from each study. A fixed-effect meta-analysis is valid under an assumption that all effect estimates are estimating the same underlying intervention effect, which is referred to variously as a ‘fixed-effect’ assumption, a ‘common-effect’ assumption or an ‘equal-effects’ assumption. However, the result of the meta-analysis can be interpreted without making such an assumption (Rice et al 2018).
10.3.2 Random-effects methods for meta-analysis
A variation on the inverse-variance method is to incorporate an assumption that the different studies are estimating different, yet related, intervention effects (Higgins et al 2009). This produces a random-effects meta-analysis, and the simplest version is known as the DerSimonian and Laird method (DerSimonian and Laird 1986). Random-effects meta-analysis is discussed in detail in Section 10.10.4 .
10.3.3 Performing inverse-variance meta-analyses
Most meta-analysis programs perform inverse-variance meta-analyses. Usually the user provides summary data from each intervention arm of each study, such as a 2×2 table when the outcome is dichotomous (see Chapter 6, Section 6.4 ), or means, standard deviations and sample sizes for each group when the outcome is continuous (see Chapter 6, Section 6.5 ). This avoids the need for the author to calculate effect estimates, and allows the use of methods targeted specifically at different types of data (see Sections 10.4 and 10.5 ).
When the data are conveniently available as summary statistics from each intervention group, the inverse-variance method can be implemented directly. For example, estimates and their standard errors may be entered directly into RevMan under the ‘Generic inverse variance’ outcome type. For ratio measures of intervention effect, the data must be entered into RevMan as natural logarithms (for example, as a log odds ratio and the standard error of the log odds ratio). However, it is straightforward to instruct the software to display results on the original (e.g. odds ratio) scale. It is possible to supplement or replace this with a column providing the sample sizes in the two groups. Note that the ability to enter estimates and standard errors creates a high degree of flexibility in meta-analysis. It facilitates the analysis of properly analysed crossover trials, cluster-randomized trials and non-randomized trials (see Chapter 23 ), as well as outcome data that are ordinal, time-to-event or rates (see Chapter 6 ).
10.4 Meta-analysis of dichotomous outcomes
There are four widely used methods of meta-analysis for dichotomous outcomes, three fixed-effect methods (Mantel-Haenszel, Peto and inverse variance) and one random-effects method (DerSimonian and Laird inverse variance). All of these methods are available as analysis options in RevMan. The Peto method can only combine odds ratios, whilst the other three methods can combine odds ratios, risk ratios or risk differences. Formulae for all of the meta-analysis methods are available elsewhere (Deeks et al 2001).
Note that having no events in one group (sometimes referred to as ‘zero cells’) causes problems with computation of estimates and standard errors with some methods: see Section 10.4.4 .
10.4.1 Mantel-Haenszel methods
When data are sparse, either in terms of event risks being low or study size being small, the estimates of the standard errors of the effect estimates that are used in the inverse-variance methods may be poor. Mantel-Haenszel methods are fixed-effect meta-analysis methods using a different weighting scheme that depends on which effect measure (e.g. risk ratio, odds ratio, risk difference) is being used (Mantel and Haenszel 1959, Greenland and Robins 1985). They have been shown to have better statistical properties when there are few events. As this is a common situation in Cochrane Reviews, the Mantel-Haenszel method is generally preferable to the inverse variance method in fixed-effect meta-analyses. In other situations the two methods give similar estimates.
10.4.2 Peto odds ratio method
Peto’s method can only be used to combine odds ratios (Yusuf et al 1985). It uses an inverse-variance approach, but uses an approximate method of estimating the log odds ratio, and uses different weights. An alternative way of viewing the Peto method is as a sum of ‘O – E’ statistics. Here, O is the observed number of events and E is an expected number of events in the experimental intervention group of each study under the null hypothesis of no intervention effect.
The approximation used in the computation of the log odds ratio works well when intervention effects are small (odds ratios are close to 1), events are not particularly common and the studies have similar numbers in experimental and comparator groups. In other situations it has been shown to give biased answers. As these criteria are not always fulfilled, Peto’s method is not recommended as a default approach for meta-analysis.
Corrections for zero cell counts are not necessary when using Peto’s method. Perhaps for this reason, this method performs well when events are very rare (Bradburn et al 2007); see Section 10.4.4.1 . Also, Peto’s method can be used to combine studies with dichotomous outcome data with studies using time-to-event analyses where log-rank tests have been used (see Section 10.9 ).
10.4.3 Which effect measure for dichotomous outcomes?
Effect measures for dichotomous data are described in Chapter 6, Section 6.4.1 . The effect of an intervention can be expressed as either a relative or an absolute effect. The risk ratio (relative risk) and odds ratio are relative measures, while the risk difference and number needed to treat for an additional beneficial outcome are absolute measures. A further complication is that there are, in fact, two risk ratios. We can calculate the risk ratio of an event occurring or the risk ratio of no event occurring. These give different summary results in a meta-analysis, sometimes dramatically so.
The selection of a summary statistic for use in meta-analysis depends on balancing three criteria (Deeks 2002). First, we desire a summary statistic that gives values that are similar for all the studies in the meta-analysis and subdivisions of the population to which the interventions will be applied. The more consistent the summary statistic, the greater is the justification for expressing the intervention effect as a single summary number. Second, the summary statistic must have the mathematical properties required to perform a valid meta-analysis. Third, the summary statistic would ideally be easily understood and applied by those using the review. The summary intervention effect should be presented in a way that helps readers to interpret and apply the results appropriately. Among effect measures for dichotomous data, no single measure is uniformly best, so the choice inevitably involves a compromise.
Consistency Empirical evidence suggests that relative effect measures are, on average, more consistent than absolute measures (Engels et al 2000, Deeks 2002, Rücker et al 2009). For this reason, it is wise to avoid performing meta-analyses of risk differences, unless there is a clear reason to suspect that risk differences will be consistent in a particular clinical situation. On average there is little difference between the odds ratio and risk ratio in terms of consistency (Deeks 2002). When the study aims to reduce the incidence of an adverse event, there is empirical evidence that risk ratios of the adverse event are more consistent than risk ratios of the non-event (Deeks 2002). Selecting an effect measure based on what is the most consistent in a particular situation is not a generally recommended strategy, since it may lead to a selection that spuriously maximizes the precision of a meta-analysis estimate.
Mathematical properties The most important mathematical criterion is the availability of a reliable variance estimate. The number needed to treat for an additional beneficial outcome does not have a simple variance estimator and cannot easily be used directly in meta-analysis, although it can be computed from the meta-analysis result afterwards (see Chapter 15, Section 15.4.2 ). There is no consensus regarding the importance of two other often-cited mathematical properties: the fact that the behaviour of the odds ratio and the risk difference do not rely on which of the two outcome states is coded as the event, and the odds ratio being the only statistic which is unbounded (see Chapter 6, Section 6.4.1 ).
Ease of interpretation The odds ratio is the hardest summary statistic to understand and to apply in practice, and many practising clinicians report difficulties in using them. There are many published examples where authors have misinterpreted odds ratios from meta-analyses as risk ratios. Although odds ratios can be re-expressed for interpretation (as discussed here), there must be some concern that routine presentation of the results of systematic reviews as odds ratios will lead to frequent over-estimation of the benefits and harms of interventions when the results are applied in clinical practice. Absolute measures of effect are thought to be more easily interpreted by clinicians than relative effects (Sinclair and Bracken 1994), and allow trade-offs to be made between likely benefits and likely harms of interventions. However, they are less likely to be generalizable.
It is generally recommended that meta-analyses are undertaken using risk ratios (taking care to make a sensible choice over which category of outcome is classified as the event) or odds ratios. This is because it seems important to avoid using summary statistics for which there is empirical evidence that they are unlikely to give consistent estimates of intervention effects (the risk difference), and it is impossible to use statistics for which meta-analysis cannot be performed (the number needed to treat for an additional beneficial outcome). It may be wise to plan to undertake a sensitivity analysis to investigate whether choice of summary statistic (and selection of the event category) is critical to the conclusions of the meta-analysis (see Section 10.14 ).
It is often sensible to use one statistic for meta-analysis and to re-express the results using a second, more easily interpretable statistic. For example, often meta-analysis may be best performed using relative effect measures (risk ratios or odds ratios) and the results re-expressed using absolute effect measures (risk differences or numbers needed to treat for an additional beneficial outcome – see Chapter 15, Section 15.4 . This is one of the key motivations for ‘Summary of findings’ tables in Cochrane Reviews: see Chapter 14 ). If odds ratios are used for meta-analysis they can also be re-expressed as risk ratios (see Chapter 15, Section 15.4 ). In all cases the same formulae can be used to convert upper and lower confidence limits. However, all of these transformations require specification of a value of baseline risk that indicates the likely risk of the outcome in the ‘control’ population to which the experimental intervention will be applied. Where the chosen value for this assumed comparator group risk is close to the typical observed comparator group risks across the studies, similar estimates of absolute effect will be obtained regardless of whether odds ratios or risk ratios are used for meta-analysis. Where the assumed comparator risk differs from the typical observed comparator group risk, the predictions of absolute benefit will differ according to which summary statistic was used for meta-analysis.
10.4.4 Meta-analysis of rare events
For rare outcomes, meta-analysis may be the only way to obtain reliable evidence of the effects of healthcare interventions. Individual studies are usually under-powered to detect differences in rare outcomes, but a meta-analysis of many studies may have adequate power to investigate whether interventions do have an impact on the incidence of the rare event. However, many methods of meta-analysis are based on large sample approximations, and are unsuitable when events are rare. Thus authors must take care when selecting a method of meta-analysis (Efthimiou 2018).
There is no single risk at which events are classified as ‘rare’. Certainly risks of 1 in 1000 constitute rare events, and many would classify risks of 1 in 100 the same way. However, the performance of methods when risks are as high as 1 in 10 may also be affected by the issues discussed in this section. What is typical is that a high proportion of the studies in the meta-analysis observe no events in one or more study arms.
10.4.4.1 Studies with no events in one or more arms
Computational problems can occur when no events are observed in one or both groups in an individual study. Inverse variance meta-analytical methods involve computing an intervention effect estimate and its standard error for each study. For studies where no events were observed in one or both arms, these computations often involve dividing by a zero count, which yields a computational error. Most meta-analytical software routines (including those in RevMan) automatically check for problematic zero counts, and add a fixed value (typically 0.5) to all cells of a 2×2 table where the problems occur. The Mantel-Haenszel methods require zero-cell corrections only if the same cell is zero in all the included studies, and hence need to use the correction less often. However, in many software applications the same correction rules are applied for Mantel-Haenszel methods as for the inverse-variance methods. Odds ratio and risk ratio methods require zero cell corrections more often than difference methods, except for the Peto odds ratio method, which encounters computation problems only in the extreme situation of no events occurring in all arms of all studies.
Whilst the fixed correction meets the objective of avoiding computational errors, it usually has the undesirable effect of biasing study estimates towards no difference and over-estimating variances of study estimates (consequently down-weighting inappropriately their contribution to the meta-analysis). Where the sizes of the study arms are unequal (which occurs more commonly in non-randomized studies than randomized trials), they will introduce a directional bias in the treatment effect. Alternative non-fixed zero-cell corrections have been explored by Sweeting and colleagues, including a correction proportional to the reciprocal of the size of the contrasting study arm, which they found preferable to the fixed 0.5 correction when arm sizes were not balanced (Sweeting et al 2004).
10.4.4.2 Studies with no events in either arm
The standard practice in meta-analysis of odds ratios and risk ratios is to exclude studies from the meta-analysis where there are no events in both arms. This is because such studies do not provide any indication of either the direction or magnitude of the relative treatment effect. Whilst it may be clear that events are very rare on both the experimental intervention and the comparator intervention, no information is provided as to which group is likely to have the higher risk, or on whether the risks are of the same or different orders of magnitude (when risks are very low, they are compatible with very large or very small ratios). Whilst one might be tempted to infer that the risk would be lowest in the group with the larger sample size (as the upper limit of the confidence interval would be lower), this is not justified as the sample size allocation was determined by the study investigators and is not a measure of the incidence of the event.
Risk difference methods superficially appear to have an advantage over odds ratio methods in that the risk difference is defined (as zero) when no events occur in either arm. Such studies are therefore included in the estimation process. Bradburn and colleagues undertook simulation studies which revealed that all risk difference methods yield confidence intervals that are too wide when events are rare, and have associated poor statistical power, which make them unsuitable for meta-analysis of rare events (Bradburn et al 2007). This is especially relevant when outcomes that focus on treatment safety are being studied, as the ability to identify correctly (or attempt to refute) serious adverse events is a key issue in drug development.
It is likely that outcomes for which no events occur in either arm may not be mentioned in reports of many randomized trials, precluding their inclusion in a meta-analysis. It is unclear, though, when working with published results, whether failure to mention a particular adverse event means there were no such events, or simply that such events were not included as a measured endpoint. Whilst the results of risk difference meta-analyses will be affected by non-reporting of outcomes with no events, odds and risk ratio based methods naturally exclude these data whether or not they are published, and are therefore unaffected.
10.4.4.3 Validity of methods of meta-analysis for rare events
Simulation studies have revealed that many meta-analytical methods can give misleading results for rare events, which is unsurprising given their reliance on asymptotic statistical theory. Their performance has been judged suboptimal either through results being biased, confidence intervals being inappropriately wide, or statistical power being too low to detect substantial differences.
In the following we consider the choice of statistical method for meta-analyses of odds ratios. Appropriate choices appear to depend on the comparator group risk, the likely size of the treatment effect and consideration of balance in the numbers of experimental and comparator participants in the constituent studies. We are not aware of research that has evaluated risk ratio measures directly, but their performance is likely to be very similar to corresponding odds ratio measurements. When events are rare, estimates of odds and risks are near identical, and results of both can be interpreted as ratios of probabilities.
Bradburn and colleagues found that many of the most commonly used meta-analytical methods were biased when events were rare (Bradburn et al 2007). The bias was greatest in inverse variance and DerSimonian and Laird odds ratio and risk difference methods, and the Mantel-Haenszel odds ratio method using a 0.5 zero-cell correction. As already noted, risk difference meta-analytical methods tended to show conservative confidence interval coverage and low statistical power when risks of events were low.
At event rates below 1% the Peto one-step odds ratio method was found to be the least biased and most powerful method, and provided the best confidence interval coverage, provided there was no substantial imbalance between treatment and comparator group sizes within studies, and treatment effects were not exceptionally large. This finding was consistently observed across three different meta-analytical scenarios, and was also observed by Sweeting and colleagues (Sweeting et al 2004).
This finding was noted despite the method producing only an approximation to the odds ratio. For very large effects (e.g. risk ratio=0.2) when the approximation is known to be poor, treatment effects were under-estimated, but the Peto method still had the best performance of all the methods considered for event risks of 1 in 1000, and the bias was never more than 6% of the comparator group risk.
In other circumstances (i.e. event risks above 1%, very large effects at event risks around 1%, and meta-analyses where many studies were substantially imbalanced) the best performing methods were the Mantel-Haenszel odds ratio without zero-cell corrections, logistic regression and an exact method. None of these methods is available in RevMan.
Methods that should be avoided with rare events are the inverse-variance methods (including the DerSimonian and Laird random-effects method) (Efthimiou 2018). These directly incorporate the study’s variance in the estimation of its contribution to the meta-analysis, but these are usually based on a large-sample variance approximation, which was not intended for use with rare events. We would suggest that incorporation of heterogeneity into an estimate of a treatment effect should be a secondary consideration when attempting to produce estimates of effects from sparse data – the primary concern is to discern whether there is any signal of an effect in the data.
10.5 Meta-analysis of continuous outcomes
An important assumption underlying standard methods for meta-analysis of continuous data is that the outcomes have a normal distribution in each intervention arm in each study. This assumption may not always be met, although it is unimportant in very large studies. It is useful to consider the possibility of skewed data (see Section 10.5.3 ).
10.5.1 Which effect measure for continuous outcomes?
The two summary statistics commonly used for meta-analysis of continuous data are the mean difference (MD) and the standardized mean difference (SMD). Other options are available, such as the ratio of means (see Chapter 6, Section 6.5.1 ). Selection of summary statistics for continuous data is principally determined by whether studies all report the outcome using the same scale (when the mean difference can be used) or using different scales (when the standardized mean difference is usually used). The ratio of means can be used in either situation, but is appropriate only when outcome measurements are strictly greater than zero. Further considerations in deciding on an effect measure that will facilitate interpretation of the findings appears in Chapter 15, Section 15.5 .
The different roles played in MD and SMD approaches by the standard deviations (SDs) of outcomes observed in the two groups should be understood.
For the mean difference approach, the SDs are used together with the sample sizes to compute the weight given to each study. Studies with small SDs are given relatively higher weight whilst studies with larger SDs are given relatively smaller weights. This is appropriate if variation in SDs between studies reflects differences in the reliability of outcome measurements, but is probably not appropriate if the differences in SD reflect real differences in the variability of outcomes in the study populations.
For the standardized mean difference approach, the SDs are used to standardize the mean differences to a single scale, as well as in the computation of study weights. Thus, studies with small SDs lead to relatively higher estimates of SMD, whilst studies with larger SDs lead to relatively smaller estimates of SMD. For this to be appropriate, it must be assumed that between-study variation in SDs reflects only differences in measurement scales and not differences in the reliability of outcome measures or variability among study populations, as discussed in Chapter 6, Section 6.5.1.2 .
These assumptions of the methods should be borne in mind when unexpected variation of SDs is observed across studies.
10.5.2 Meta-analysis of change scores
In some circumstances an analysis based on changes from baseline will be more efficient and powerful than comparison of post-intervention values, as it removes a component of between-person variability from the analysis. However, calculation of a change score requires measurement of the outcome twice and in practice may be less efficient for outcomes that are unstable or difficult to measure precisely, where the measurement error may be larger than true between-person baseline variability. Change-from-baseline outcomes may also be preferred if they have a less skewed distribution than post-intervention measurement outcomes. Although sometimes used as a device to ‘correct’ for unlucky randomization, this practice is not recommended.
The preferred statistical approach to accounting for baseline measurements of the outcome variable is to include the baseline outcome measurements as a covariate in a regression model or analysis of covariance (ANCOVA). These analyses produce an ‘adjusted’ estimate of the intervention effect together with its standard error. These analyses are the least frequently encountered, but as they give the most precise and least biased estimates of intervention effects they should be included in the analysis when they are available. However, they can only be included in a meta-analysis using the generic inverse-variance method, since means and SDs are not available for each intervention group separately.
In practice an author is likely to discover that the studies included in a review include a mixture of change-from-baseline and post-intervention value scores. However, mixing of outcomes is not a problem when it comes to meta-analysis of MDs. There is no statistical reason why studies with change-from-baseline outcomes should not be combined in a meta-analysis with studies with post-intervention measurement outcomes when using the (unstandardized) MD method. In a randomized study, MD based on changes from baseline can usually be assumed to be addressing exactly the same underlying intervention effects as analyses based on post-intervention measurements. That is to say, the difference in mean post-intervention values will on average be the same as the difference in mean change scores. If the use of change scores does increase precision, appropriately, the studies presenting change scores will be given higher weights in the analysis than they would have received if post-intervention values had been used, as they will have smaller SDs.
When combining the data on the MD scale, authors must be careful to use the appropriate means and SDs (either of post-intervention measurements or of changes from baseline) for each study. Since the mean values and SDs for the two types of outcome may differ substantially, it may be advisable to place them in separate subgroups to avoid confusion for the reader, but the results of the subgroups can legitimately be pooled together.
In contrast, post-intervention value and change scores should not in principle be combined using standard meta-analysis approaches when the effect measure is an SMD. This is because the SDs used in the standardization reflect different things. The SD when standardizing post-intervention values reflects between-person variability at a single point in time. The SD when standardizing change scores reflects variation in between-person changes over time, so will depend on both within-person and between-person variability; within-person variability in turn is likely to depend on the length of time between measurements. Nevertheless, an empirical study of 21 meta-analyses in osteoarthritis did not find a difference between combined SMDs based on post-intervention values and combined SMDs based on change scores (da Costa et al 2013). One option is to standardize SMDs using post-intervention SDs rather than change score SDs. This would lead to valid synthesis of the two approaches, but we are not aware that an appropriate standard error for this has been derived.
A common practical problem associated with including change-from-baseline measures is that the SD of changes is not reported. Imputation of SDs is discussed in Chapter 6, Section 6.5.2.8 .
10.5.3 Meta-analysis of skewed data
Analyses based on means are appropriate for data that are at least approximately normally distributed, and for data from very large trials. If the true distribution of outcomes is asymmetrical, then the data are said to be skewed. Review authors should consider the possibility and implications of skewed data when analysing continuous outcomes (see MECIR Box 10.5.a ). Skew can sometimes be diagnosed from the means and SDs of the outcomes. A rough check is available, but it is only valid if a lowest or highest possible value for an outcome is known to exist. Thus, the check may be used for outcomes such as weight, volume and blood concentrations, which have lowest possible values of 0, or for scale outcomes with minimum or maximum scores, but it may not be appropriate for change-from-baseline measures. The check involves calculating the observed mean minus the lowest possible value (or the highest possible value minus the observed mean), and dividing this by the SD. A ratio less than 2 suggests skew (Altman and Bland 1996). If the ratio is less than 1, there is strong evidence of a skewed distribution.
Transformation of the original outcome data may reduce skew substantially. Reports of trials may present results on a transformed scale, usually a log scale. Collection of appropriate data summaries from the trialists, or acquisition of individual patient data, is currently the approach of choice. Appropriate data summaries and analysis strategies for the individual patient data will depend on the situation. Consultation with a knowledgeable statistician is advised.
Where data have been analysed on a log scale, results are commonly presented as geometric means and ratios of geometric means. A meta-analysis may be then performed on the scale of the log-transformed data; an example of the calculation of the required means and SD is given in Chapter 6, Section 6.5.2.4 . This approach depends on being able to obtain transformed data for all studies; methods for transforming from one scale to the other are available (Higgins et al 2008b). Log-transformed and untransformed data should not be mixed in a meta-analysis.
MECIR Box 10.5.a Relevant expectations for conduct of intervention reviews
Addressing skewed data ( ) | |
| Skewed data are sometimes not summarized usefully by means and standard deviations. While statistical methods are approximately valid for large sample sizes, skewed outcome data can lead to misleading results when studies are small. |
10.6 Combining dichotomous and continuous outcomes
Occasionally authors encounter a situation where data for the same outcome are presented in some studies as dichotomous data and in other studies as continuous data. For example, scores on depression scales can be reported as means, or as the percentage of patients who were depressed at some point after an intervention (i.e. with a score above a specified cut-point). This type of information is often easier to understand, and more helpful, when it is dichotomized. However, deciding on a cut-point may be arbitrary, and information is lost when continuous data are transformed to dichotomous data.
There are several options for handling combinations of dichotomous and continuous data. Generally, it is useful to summarize results from all the relevant, valid studies in a similar way, but this is not always possible. It may be possible to collect missing data from investigators so that this can be done. If not, it may be useful to summarize the data in three ways: by entering the means and SDs as continuous outcomes, by entering the counts as dichotomous outcomes and by entering all of the data in text form as ‘Other data’ outcomes.
There are statistical approaches available that will re-express odds ratios as SMDs (and vice versa), allowing dichotomous and continuous data to be combined (Anzures-Cabrera et al 2011). A simple approach is as follows. Based on an assumption that the underlying continuous measurements in each intervention group follow a logistic distribution (which is a symmetrical distribution similar in shape to the normal distribution, but with more data in the distributional tails), and that the variability of the outcomes is the same in both experimental and comparator participants, the odds ratios can be re-expressed as a SMD according to the following simple formula (Chinn 2000):
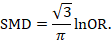
The standard error of the log odds ratio can be converted to the standard error of a SMD by multiplying by the same constant (√3/π=0.5513). Alternatively SMDs can be re-expressed as log odds ratios by multiplying by π/√3=1.814. Once SMDs (or log odds ratios) and their standard errors have been computed for all studies in the meta-analysis, they can be combined using the generic inverse-variance method. Standard errors can be computed for all studies by entering the data as dichotomous and continuous outcome type data, as appropriate, and converting the confidence intervals for the resulting log odds ratios and SMDs into standard errors (see Chapter 6, Section 6.3 ).
10.7 Meta-analysis of ordinal outcomes and measurement scale s
Ordinal and measurement scale outcomes are most commonly meta-analysed as dichotomous data (if so, see Section 10.4 ) or continuous data (if so, see Section 10.5 ) depending on the way that the study authors performed the original analyses.
Occasionally it is possible to analyse the data using proportional odds models. This is the case when ordinal scales have a small number of categories, the numbers falling into each category for each intervention group can be obtained, and the same ordinal scale has been used in all studies. This approach may make more efficient use of all available data than dichotomization, but requires access to statistical software and results in a summary statistic for which it is challenging to find a clinical meaning.
The proportional odds model uses the proportional odds ratio as the measure of intervention effect (Agresti 1996) (see Chapter 6, Section 6.6 ), and can be used for conducting a meta-analysis in advanced statistical software packages (Whitehead and Jones 1994). Estimates of log odds ratios and their standard errors from a proportional odds model may be meta-analysed using the generic inverse-variance method (see Section 10.3.3 ). If the same ordinal scale has been used in all studies, but in some reports has been presented as a dichotomous outcome, it may still be possible to include all studies in the meta-analysis. In the context of the three-category model, this might mean that for some studies category 1 constitutes a success, while for others both categories 1 and 2 constitute a success. Methods are available for dealing with this, and for combining data from scales that are related but have different definitions for their categories (Whitehead and Jones 1994).
10.8 Meta-analysis of counts and rates
Results may be expressed as count data when each participant may experience an event, and may experience it more than once (see Chapter 6, Section 6.7 ). For example, ‘number of strokes’, or ‘number of hospital visits’ are counts. These events may not happen at all, but if they do happen there is no theoretical maximum number of occurrences for an individual. Count data may be analysed using methods for dichotomous data if the counts are dichotomized for each individual (see Section 10.4 ), continuous data (see Section 10.5 ) and time-to-event data (see Section 10.9 ), as well as being analysed as rate data.
Rate data occur if counts are measured for each participant along with the time over which they are observed. This is particularly appropriate when the events being counted are rare. For example, a woman may experience two strokes during a follow-up period of two years. Her rate of strokes is one per year of follow-up (or, equivalently 0.083 per month of follow-up). Rates are conventionally summarized at the group level. For example, participants in the comparator group of a clinical trial may experience 85 strokes during a total of 2836 person-years of follow-up. An underlying assumption associated with the use of rates is that the risk of an event is constant across participants and over time. This assumption should be carefully considered for each situation. For example, in contraception studies, rates have been used (known as Pearl indices) to describe the number of pregnancies per 100 women-years of follow-up. This is now considered inappropriate since couples have different risks of conception, and the risk for each woman changes over time. Pregnancies are now analysed more often using life tables or time-to-event methods that investigate the time elapsing before the first pregnancy.
Analysing count data as rates is not always the most appropriate approach and is uncommon in practice. This is because:
- the assumption of a constant underlying risk may not be suitable; and
- the statistical methods are not as well developed as they are for other types of data.
The results of a study may be expressed as a rate ratio , that is the ratio of the rate in the experimental intervention group to the rate in the comparator group. The (natural) logarithms of the rate ratios may be combined across studies using the generic inverse-variance method (see Section 10.3.3 ). Alternatively, Poisson regression approaches can be used (Spittal et al 2015).
In a randomized trial, rate ratios may often be very similar to risk ratios obtained after dichotomizing the participants, since the average period of follow-up should be similar in all intervention groups. Rate ratios and risk ratios will differ, however, if an intervention affects the likelihood of some participants experiencing multiple events.
It is possible also to focus attention on the rate difference (see Chapter 6, Section 6.7.1 ). The analysis again can be performed using the generic inverse-variance method (Hasselblad and McCrory 1995, Guevara et al 2004).
10.9 Meta-analysis of time-to-event outcomes
Two approaches to meta-analysis of time-to-event outcomes are readily available to Cochrane Review authors. The choice of which to use will depend on the type of data that have been extracted from the primary studies, or obtained from re-analysis of individual participant data.
If ‘O – E’ and ‘V’ statistics have been obtained (see Chapter 6, Section 6.8.2 ), either through re-analysis of individual participant data or from aggregate statistics presented in the study reports, then these statistics may be entered directly into RevMan using the ‘O – E and Variance’ outcome type. There are several ways to calculate these ‘O – E’ and ‘V’ statistics. Peto’s method applied to dichotomous data (Section 10.4.2 ) gives rise to an odds ratio; a log-rank approach gives rise to a hazard ratio; and a variation of the Peto method for analysing time-to-event data gives rise to something in between (Simmonds et al 2011). The appropriate effect measure should be specified. Only fixed-effect meta-analysis methods are available in RevMan for ‘O – E and Variance’ outcomes.
Alternatively, if estimates of log hazard ratios and standard errors have been obtained from results of Cox proportional hazards regression models, study results can be combined using generic inverse-variance methods (see Section 10.3.3 ).
If a mixture of log-rank and Cox model estimates are obtained from the studies, all results can be combined using the generic inverse-variance method, as the log-rank estimates can be converted into log hazard ratios and standard errors using the approaches discussed in Chapter 6, Section 6.8 .
10.10 Heterogeneity
10.10.1 what is heterogeneity.
Inevitably, studies brought together in a systematic review will differ. Any kind of variability among studies in a systematic review may be termed heterogeneity. It can be helpful to distinguish between different types of heterogeneity. Variability in the participants, interventions and outcomes studied may be described as clinical diversity (sometimes called clinical heterogeneity), and variability in study design, outcome measurement tools and risk of bias may be described as methodological diversity (sometimes called methodological heterogeneity). Variability in the intervention effects being evaluated in the different studies is known as statistical heterogeneity , and is a consequence of clinical or methodological diversity, or both, among the studies. Statistical heterogeneity manifests itself in the observed intervention effects being more different from each other than one would expect due to random error (chance) alone. We will follow convention and refer to statistical heterogeneity simply as heterogeneity .
Clinical variation will lead to heterogeneity if the intervention effect is affected by the factors that vary across studies; most obviously, the specific interventions or patient characteristics. In other words, the true intervention effect will be different in different studies.
Differences between studies in terms of methodological factors, such as use of blinding and concealment of allocation sequence, or if there are differences between studies in the way the outcomes are defined and measured, may be expected to lead to differences in the observed intervention effects. Significant statistical heterogeneity arising from methodological diversity or differences in outcome assessments suggests that the studies are not all estimating the same quantity, but does not necessarily suggest that the true intervention effect varies. In particular, heterogeneity associated solely with methodological diversity would indicate that the studies suffer from different degrees of bias. Empirical evidence suggests that some aspects of design can affect the result of clinical trials, although this is not always the case. Further discussion appears in Chapter 7 and Chapter 8 .
The scope of a review will largely determine the extent to which studies included in a review are diverse. Sometimes a review will include studies addressing a variety of questions, for example when several different interventions for the same condition are of interest (see also Chapter 11 ) or when the differential effects of an intervention in different populations are of interest. Meta-analysis should only be considered when a group of studies is sufficiently homogeneous in terms of participants, interventions and outcomes to provide a meaningful summary (see MECIR Box 10.10.a. ). It is often appropriate to take a broader perspective in a meta-analysis than in a single clinical trial. A common analogy is that systematic reviews bring together apples and oranges, and that combining these can yield a meaningless result. This is true if apples and oranges are of intrinsic interest on their own, but may not be if they are used to contribute to a wider question about fruit. For example, a meta-analysis may reasonably evaluate the average effect of a class of drugs by combining results from trials where each evaluates the effect of a different drug from the class.
MECIR Box 10.10.a Relevant expectations for conduct of intervention reviews
( ) | |
| Meta-analyses of very diverse studies can be misleading, for example where studies use different forms of control. Clinical diversity does not indicate necessarily that a meta-analysis should not be performed. However, authors must be clear about the underlying question that all studies are addressing. |
There may be specific interest in a review in investigating how clinical and methodological aspects of studies relate to their results. Where possible these investigations should be specified a priori (i.e. in the protocol for the systematic review). It is legitimate for a systematic review to focus on examining the relationship between some clinical characteristic(s) of the studies and the size of intervention effect, rather than on obtaining a summary effect estimate across a series of studies (see Section 10.11 ). Meta-regression may best be used for this purpose, although it is not implemented in RevMan (see Section 10.11.4 ).
10.10.2 Identifying and measuring heterogeneity
It is essential to consider the extent to which the results of studies are consistent with each other (see MECIR Box 10.10.b ). If confidence intervals for the results of individual studies (generally depicted graphically using horizontal lines) have poor overlap, this generally indicates the presence of statistical heterogeneity. More formally, a statistical test for heterogeneity is available. This Chi 2 (χ 2 , or chi-squared) test is included in the forest plots in Cochrane Reviews. It assesses whether observed differences in results are compatible with chance alone. A low P value (or a large Chi 2 statistic relative to its degree of freedom) provides evidence of heterogeneity of intervention effects (variation in effect estimates beyond chance).
MECIR Box 10.10.b Relevant expectations for conduct of intervention reviews
Assessing statistical heterogeneity ( ) | |
| The presence of heterogeneity affects the extent to which generalizable conclusions can be formed. It is important to identify heterogeneity in case there is sufficient information to explain it and offer new insights. Authors should recognize that there is much uncertainty in measures such as and Tau when there are few studies. Thus, use of simple thresholds to diagnose heterogeneity should be avoided. |
Care must be taken in the interpretation of the Chi 2 test, since it has low power in the (common) situation of a meta-analysis when studies have small sample size or are few in number. This means that while a statistically significant result may indicate a problem with heterogeneity, a non-significant result must not be taken as evidence of no heterogeneity. This is also why a P value of 0.10, rather than the conventional level of 0.05, is sometimes used to determine statistical significance. A further problem with the test, which seldom occurs in Cochrane Reviews, is that when there are many studies in a meta-analysis, the test has high power to detect a small amount of heterogeneity that may be clinically unimportant.
Some argue that, since clinical and methodological diversity always occur in a meta-analysis, statistical heterogeneity is inevitable (Higgins et al 2003). Thus, the test for heterogeneity is irrelevant to the choice of analysis; heterogeneity will always exist whether or not we happen to be able to detect it using a statistical test. Methods have been developed for quantifying inconsistency across studies that move the focus away from testing whether heterogeneity is present to assessing its impact on the meta-analysis. A useful statistic for quantifying inconsistency is:
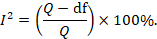
In this equation, Q is the Chi 2 statistic and df is its degrees of freedom (Higgins and Thompson 2002, Higgins et al 2003). I 2 describes the percentage of the variability in effect estimates that is due to heterogeneity rather than sampling error (chance).
Thresholds for the interpretation of the I 2 statistic can be misleading, since the importance of inconsistency depends on several factors. A rough guide to interpretation in the context of meta-analyses of randomized trials is as follows:
- 0% to 40%: might not be important;
- 30% to 60%: may represent moderate heterogeneity*;
- 50% to 90%: may represent substantial heterogeneity*;
- 75% to 100%: considerable heterogeneity*.
*The importance of the observed value of I 2 depends on (1) magnitude and direction of effects, and (2) strength of evidence for heterogeneity (e.g. P value from the Chi 2 test, or a confidence interval for I 2 : uncertainty in the value of I 2 is substantial when the number of studies is small).
10.10.3 Strategies for addressing heterogeneity
Review authors must take into account any statistical heterogeneity when interpreting results, particularly when there is variation in the direction of effect (see MECIR Box 10.10.c ). A number of options are available if heterogeneity is identified among a group of studies that would otherwise be considered suitable for a meta-analysis.
MECIR Box 10.10.c Relevant expectations for conduct of intervention reviews
Considering statistical heterogeneity when interpreting the results ( ) | |
| The presence of heterogeneity affects the extent to which generalizable conclusions can be formed. If a fixed-effect analysis is used, the confidence intervals ignore the extent of heterogeneity. If a random-effects analysis is used, the result pertains to the mean effect across studies. In both cases, the implications of notable heterogeneity should be addressed. It may be possible to understand the reasons for the heterogeneity if there are sufficient studies. |
- Check again that the data are correct. Severe apparent heterogeneity can indicate that data have been incorrectly extracted or entered into meta-analysis software. For example, if standard errors have mistakenly been entered as SDs for continuous outcomes, this could manifest itself in overly narrow confidence intervals with poor overlap and hence substantial heterogeneity. Unit-of-analysis errors may also be causes of heterogeneity (see Chapter 6, Section 6.2 ).
- Do not do a meta -analysis. A systematic review need not contain any meta-analyses. If there is considerable variation in results, and particularly if there is inconsistency in the direction of effect, it may be misleading to quote an average value for the intervention effect.
- Explore heterogeneity. It is clearly of interest to determine the causes of heterogeneity among results of studies. This process is problematic since there are often many characteristics that vary across studies from which one may choose. Heterogeneity may be explored by conducting subgroup analyses (see Section 10.11.3 ) or meta-regression (see Section 10.11.4 ). Reliable conclusions can only be drawn from analyses that are truly pre-specified before inspecting the studies’ results, and even these conclusions should be interpreted with caution. Explorations of heterogeneity that are devised after heterogeneity is identified can at best lead to the generation of hypotheses. They should be interpreted with even more caution and should generally not be listed among the conclusions of a review. Also, investigations of heterogeneity when there are very few studies are of questionable value.
- Ignore heterogeneity. Fixed-effect meta-analyses ignore heterogeneity. The summary effect estimate from a fixed-effect meta-analysis is normally interpreted as being the best estimate of the intervention effect. However, the existence of heterogeneity suggests that there may not be a single intervention effect but a variety of intervention effects. Thus, the summary fixed-effect estimate may be an intervention effect that does not actually exist in any population, and therefore have a confidence interval that is meaningless as well as being too narrow (see Section 10.10.4 ).
- Perform a random-effects meta-analysis. A random-effects meta-analysis may be used to incorporate heterogeneity among studies. This is not a substitute for a thorough investigation of heterogeneity. It is intended primarily for heterogeneity that cannot be explained. An extended discussion of this option appears in Section 10.10.4 .
- Reconsider the effect measure. Heterogeneity may be an artificial consequence of an inappropriate choice of effect measure. For example, when studies collect continuous outcome data using different scales or different units, extreme heterogeneity may be apparent when using the mean difference but not when the more appropriate standardized mean difference is used. Furthermore, choice of effect measure for dichotomous outcomes (odds ratio, risk ratio, or risk difference) may affect the degree of heterogeneity among results. In particular, when comparator group risks vary, homogeneous odds ratios or risk ratios will necessarily lead to heterogeneous risk differences, and vice versa. However, it remains unclear whether homogeneity of intervention effect in a particular meta-analysis is a suitable criterion for choosing between these measures (see also Section 10.4.3 ).
- Exclude studies. Heterogeneity may be due to the presence of one or two outlying studies with results that conflict with the rest of the studies. In general it is unwise to exclude studies from a meta-analysis on the basis of their results as this may introduce bias. However, if an obvious reason for the outlying result is apparent, the study might be removed with more confidence. Since usually at least one characteristic can be found for any study in any meta-analysis which makes it different from the others, this criterion is unreliable because it is all too easy to fulfil. It is advisable to perform analyses both with and without outlying studies as part of a sensitivity analysis (see Section 10.14 ). Whenever possible, potential sources of clinical diversity that might lead to such situations should be specified in the protocol.
10.10.4 Incorporating heterogeneity into random-effects models
The random-effects meta-analysis approach incorporates an assumption that the different studies are estimating different, yet related, intervention effects (DerSimonian and Laird 1986, Borenstein et al 2010). The approach allows us to address heterogeneity that cannot readily be explained by other factors. A random-effects meta-analysis model involves an assumption that the effects being estimated in the different studies follow some distribution. The model represents our lack of knowledge about why real, or apparent, intervention effects differ, by considering the differences as if they were random. The centre of the assumed distribution describes the average of the effects, while its width describes the degree of heterogeneity. The conventional choice of distribution is a normal distribution. It is difficult to establish the validity of any particular distributional assumption, and this is a common criticism of random-effects meta-analyses. The importance of the assumed shape for this distribution has not been widely studied.
To undertake a random-effects meta-analysis, the standard errors of the study-specific estimates (SE i in Section 10.3.1 ) are adjusted to incorporate a measure of the extent of variation, or heterogeneity, among the intervention effects observed in different studies (this variation is often referred to as Tau-squared, τ 2 , or Tau 2 ). The amount of variation, and hence the adjustment, can be estimated from the intervention effects and standard errors of the studies included in the meta-analysis.
In a heterogeneous set of studies, a random-effects meta-analysis will award relatively more weight to smaller studies than such studies would receive in a fixed-effect meta-analysis. This is because small studies are more informative for learning about the distribution of effects across studies than for learning about an assumed common intervention effect.
Note that a random-effects model does not ‘take account’ of the heterogeneity, in the sense that it is no longer an issue. It is always preferable to explore possible causes of heterogeneity, although there may be too few studies to do this adequately (see Section 10.11 ).
10.10.4.1 Fixed or random effects?
A fixed-effect meta-analysis provides a result that may be viewed as a ‘typical intervention effect’ from the studies included in the analysis. In order to calculate a confidence interval for a fixed-effect meta-analysis the assumption is usually made that the true effect of intervention (in both magnitude and direction) is the same value in every study (i.e. fixed across studies). This assumption implies that the observed differences among study results are due solely to the play of chance (i.e. that there is no statistical heterogeneity).
A random-effects model provides a result that may be viewed as an ‘average intervention effect’, where this average is explicitly defined according to an assumed distribution of effects across studies. Instead of assuming that the intervention effects are the same, we assume that they follow (usually) a normal distribution. The assumption implies that the observed differences among study results are due to a combination of the play of chance and some genuine variation in the intervention effects.
The random-effects method and the fixed-effect method will give identical results when there is no heterogeneity among the studies.
When heterogeneity is present, a confidence interval around the random-effects summary estimate is wider than a confidence interval around a fixed-effect summary estimate. This will happen whenever the I 2 statistic is greater than zero, even if the heterogeneity is not detected by the Chi 2 test for heterogeneity (see Section 10.10.2 ).
Sometimes the central estimate of the intervention effect is different between fixed-effect and random-effects analyses. In particular, if results of smaller studies are systematically different from results of larger ones, which can happen as a result of publication bias or within-study bias in smaller studies (Egger et al 1997, Poole and Greenland 1999, Kjaergard et al 2001), then a random-effects meta-analysis will exacerbate the effects of the bias (see also Chapter 13, Section 13.3.5.6 ). A fixed-effect analysis will be affected less, although strictly it will also be inappropriate.
The decision between fixed- and random-effects meta-analyses has been the subject of much debate, and we do not provide a universal recommendation. Some considerations in making this choice are as follows:
- Many have argued that the decision should be based on an expectation of whether the intervention effects are truly identical, preferring the fixed-effect model if this is likely and a random-effects model if this is unlikely (Borenstein et al 2010). Since it is generally considered to be implausible that intervention effects across studies are identical (unless the intervention has no effect at all), this leads many to advocate use of the random-effects model.
- Others have argued that a fixed-effect analysis can be interpreted in the presence of heterogeneity, and that it makes fewer assumptions than a random-effects meta-analysis. They then refer to it as a ‘fixed-effects’ meta-analysis (Peto et al 1995, Rice et al 2018).
- Under any interpretation, a fixed-effect meta-analysis ignores heterogeneity. If the method is used, it is therefore important to supplement it with a statistical investigation of the extent of heterogeneity (see Section 10.10.2 ).
- In the presence of heterogeneity, a random-effects analysis gives relatively more weight to smaller studies and relatively less weight to larger studies. If there is additionally some funnel plot asymmetry (i.e. a relationship between intervention effect magnitude and study size), then this will push the results of the random-effects analysis towards the findings in the smaller studies. In the context of randomized trials, this is generally regarded as an unfortunate consequence of the model.
- A pragmatic approach is to plan to undertake both a fixed-effect and a random-effects meta-analysis, with an intention to present the random-effects result if there is no indication of funnel plot asymmetry. If there is an indication of funnel plot asymmetry, then both methods are problematic. It may be reasonable to present both analyses or neither, or to perform a sensitivity analysis in which small studies are excluded or addressed directly using meta-regression (see Chapter 13, Section 13.3.5.6 ).
- The choice between a fixed-effect and a random-effects meta-analysis should never be made on the basis of a statistical test for heterogeneity.
10.10.4.2 Interpretation of random-effects meta-analyses
The summary estimate and confidence interval from a random-effects meta-analysis refer to the centre of the distribution of intervention effects, but do not describe the width of the distribution. Often the summary estimate and its confidence interval are quoted in isolation and portrayed as a sufficient summary of the meta-analysis. This is inappropriate. The confidence interval from a random-effects meta-analysis describes uncertainty in the location of the mean of systematically different effects in the different studies. It does not describe the degree of heterogeneity among studies, as may be commonly believed. For example, when there are many studies in a meta-analysis, we may obtain a very tight confidence interval around the random-effects estimate of the mean effect even when there is a large amount of heterogeneity. A solution to this problem is to consider a prediction interval (see Section 10.10.4.3 ).
Methodological diversity creates heterogeneity through biases variably affecting the results of different studies. The random-effects summary estimate will only correctly estimate the average intervention effect if the biases are symmetrically distributed, leading to a mixture of over-estimates and under-estimates of effect, which is unlikely to be the case. In practice it can be very difficult to distinguish whether heterogeneity results from clinical or methodological diversity, and in most cases it is likely to be due to both, so these distinctions are hard to draw in the interpretation.
When there is little information, either because there are few studies or if the studies are small with few events, a random-effects analysis will provide poor estimates of the amount of heterogeneity (i.e. of the width of the distribution of intervention effects). Fixed-effect methods such as the Mantel-Haenszel method will provide more robust estimates of the average intervention effect, but at the cost of ignoring any heterogeneity.
10.10.4.3 Prediction intervals from a random-effects meta-analysis
An estimate of the between-study variance in a random-effects meta-analysis is typically presented as part of its results. The square root of this number (i.e. Tau) is the estimated standard deviation of underlying effects across studies. Prediction intervals are a way of expressing this value in an interpretable way.
To motivate the idea of a prediction interval, note that for absolute measures of effect (e.g. risk difference, mean difference, standardized mean difference), an approximate 95% range of normally distributed underlying effects can be obtained by creating an interval from 1.96´Tau below the random-effects mean, to 1.96✕Tau above it. (For relative measures such as the odds ratio and risk ratio, an equivalent interval needs to be based on the natural logarithm of the summary estimate.) In reality, both the summary estimate and the value of Tau are associated with uncertainty. A prediction interval seeks to present the range of effects in a way that acknowledges this uncertainty (Higgins et al 2009). A simple 95% prediction interval can be calculated as:

where M is the summary mean from the random-effects meta-analysis, t k −2 is the 95% percentile of a t -distribution with k –2 degrees of freedom, k is the number of studies, Tau 2 is the estimated amount of heterogeneity and SE( M ) is the standard error of the summary mean.
The term ‘prediction interval’ relates to the use of this interval to predict the possible underlying effect in a new study that is similar to the studies in the meta-analysis. A more useful interpretation of the interval is as a summary of the spread of underlying effects in the studies included in the random-effects meta-analysis.
Prediction intervals have proved a popular way of expressing the amount of heterogeneity in a meta-analysis (Riley et al 2011). They are, however, strongly based on the assumption of a normal distribution for the effects across studies, and can be very problematic when the number of studies is small, in which case they can appear spuriously wide or spuriously narrow. Nevertheless, we encourage their use when the number of studies is reasonable (e.g. more than ten) and there is no clear funnel plot asymmetry.
10.10.4.4 Implementing random-effects meta-analyses
As introduced in Section 10.3.2 , the random-effects model can be implemented using an inverse-variance approach, incorporating a measure of the extent of heterogeneity into the study weights. RevMan implements a version of random-effects meta-analysis that is described by DerSimonian and Laird, making use of a ‘moment-based’ estimate of the between-study variance (DerSimonian and Laird 1986). The attraction of this method is that the calculations are straightforward, but it has a theoretical disadvantage in that the confidence intervals are slightly too narrow to encompass full uncertainty resulting from having estimated the degree of heterogeneity.
For many years, RevMan has implemented two random-effects methods for dichotomous data: a Mantel-Haenszel method and an inverse-variance method. Both use the moment-based approach to estimating the amount of between-studies variation. The difference between the two is subtle: the former estimates the between-study variation by comparing each study’s result with a Mantel-Haenszel fixed-effect meta-analysis result, whereas the latter estimates it by comparing each study’s result with an inverse-variance fixed-effect meta-analysis result. In practice, the difference is likely to be trivial.
There are alternative methods for performing random-effects meta-analyses that have better technical properties than the DerSimonian and Laird approach with a moment-based estimate (Veroniki et al 2016). Most notable among these is an adjustment to the confidence interval proposed by Hartung and Knapp and by Sidik and Jonkman (Hartung and Knapp 2001, Sidik and Jonkman 2002). This adjustment widens the confidence interval to reflect uncertainty in the estimation of between-study heterogeneity, and it should be used if available to review authors. An alternative option to encompass full uncertainty in the degree of heterogeneity is to take a Bayesian approach (see Section 10.13 ).
An empirical comparison of different ways to estimate between-study variation in Cochrane meta-analyses has shown that they can lead to substantial differences in estimates of heterogeneity, but seldom have major implications for estimating summary effects (Langan et al 2015). Several simulation studies have concluded that an approach proposed by Paule and Mandel should be recommended (Langan et al 2017); whereas a comprehensive recent simulation study recommended a restricted maximum likelihood approach, although noted that no single approach is universally preferable (Langan et al 2019). Review authors are encouraged to select one of these options if it is available to them.
10.11 Investigating heterogeneity
10.11.1 interaction and effect modification.
Does the intervention effect vary with different populations or intervention characteristics (such as dose or duration)? Such variation is known as interaction by statisticians and as effect modification by epidemiologists. Methods to search for such interactions include subgroup analyses and meta-regression. All methods have considerable pitfalls.
10.11.2 What are subgroup analyses?
Subgroup analyses involve splitting all the participant data into subgroups, often in order to make comparisons between them. Subgroup analyses may be done for subsets of participants (such as males and females), or for subsets of studies (such as different geographical locations). Subgroup analyses may be done as a means of investigating heterogeneous results, or to answer specific questions about particular patient groups, types of intervention or types of study.
Subgroup analyses of subsets of participants within studies are uncommon in systematic reviews based on published literature because sufficient details to extract data about separate participant types are seldom published in reports. By contrast, such subsets of participants are easily analysed when individual participant data have been collected (see Chapter 26 ). The methods we describe in the remainder of this chapter are for subgroups of studies.
Findings from multiple subgroup analyses may be misleading. Subgroup analyses are observational by nature and are not based on randomized comparisons. False negative and false positive significance tests increase in likelihood rapidly as more subgroup analyses are performed. If their findings are presented as definitive conclusions there is clearly a risk of people being denied an effective intervention or treated with an ineffective (or even harmful) intervention. Subgroup analyses can also generate misleading recommendations about directions for future research that, if followed, would waste scarce resources.
It is useful to distinguish between the notions of ‘qualitative interaction’ and ‘quantitative interaction’ (Yusuf et al 1991). Qualitative interaction exists if the direction of effect is reversed, that is if an intervention is beneficial in one subgroup but is harmful in another. Qualitative interaction is rare. This may be used as an argument that the most appropriate result of a meta-analysis is the overall effect across all subgroups. Quantitative interaction exists when the size of the effect varies but not the direction, that is if an intervention is beneficial to different degrees in different subgroups.
10.11.3 Undertaking subgroup analyses
Meta-analyses can be undertaken in RevMan both within subgroups of studies as well as across all studies irrespective of their subgroup membership. It is tempting to compare effect estimates in different subgroups by considering the meta-analysis results from each subgroup separately. This should only be done informally by comparing the magnitudes of effect. Noting that either the effect or the test for heterogeneity in one subgroup is statistically significant whilst that in the other subgroup is not statistically significant does not indicate that the subgroup factor explains heterogeneity. Since different subgroups are likely to contain different amounts of information and thus have different abilities to detect effects, it is extremely misleading simply to compare the statistical significance of the results.
10.11.3.1 Is the effect different in different subgroups?
Valid investigations of whether an intervention works differently in different subgroups involve comparing the subgroups with each other. It is a mistake to compare within-subgroup inferences such as P values. If one subgroup analysis is statistically significant and another is not, then the latter may simply reflect a lack of information rather than a smaller (or absent) effect. When there are only two subgroups, non-overlap of the confidence intervals indicates statistical significance, but note that the confidence intervals can overlap to a small degree and the difference still be statistically significant.
A formal statistical approach should be used to examine differences among subgroups (see MECIR Box 10.11.a ). A simple significance test to investigate differences between two or more subgroups can be performed (Borenstein and Higgins 2013). This procedure consists of undertaking a standard test for heterogeneity across subgroup results rather than across individual study results. When the meta-analysis uses a fixed-effect inverse-variance weighted average approach, the method is exactly equivalent to the test described by Deeks and colleagues (Deeks et al 2001). An I 2 statistic is also computed for subgroup differences. This describes the percentage of the variability in effect estimates from the different subgroups that is due to genuine subgroup differences rather than sampling error (chance). Note that these methods for examining subgroup differences should be used only when the data in the subgroups are independent (i.e. they should not be used if the same study participants contribute to more than one of the subgroups in the forest plot).
If fixed-effect models are used for the analysis within each subgroup, then these statistics relate to differences in typical effects across different subgroups. If random-effects models are used for the analysis within each subgroup, then the statistics relate to variation in the mean effects in the different subgroups.
An alternative method for testing for differences between subgroups is to use meta-regression techniques, in which case a random-effects model is generally preferred (see Section 10.11.4 ). Tests for subgroup differences based on random-effects models may be regarded as preferable to those based on fixed-effect models, due to the high risk of false-positive results when a fixed-effect model is used to compare subgroups (Higgins and Thompson 2004).
MECIR Box 10.11.a Relevant expectations for conduct of intervention reviews
Comparing subgroups ( ) | |
| Concluding that there is a difference in effect in different subgroups on the basis of differences in the level of statistical significance within subgroups can be very misleading. |
10.11.4 Meta-regression
If studies are divided into subgroups (see Section 10.11.2 ), this may be viewed as an investigation of how a categorical study characteristic is associated with the intervention effects in the meta-analysis. For example, studies in which allocation sequence concealment was adequate may yield different results from those in which it was inadequate. Here, allocation sequence concealment, being either adequate or inadequate, is a categorical characteristic at the study level. Meta-regression is an extension to subgroup analyses that allows the effect of continuous, as well as categorical, characteristics to be investigated, and in principle allows the effects of multiple factors to be investigated simultaneously (although this is rarely possible due to inadequate numbers of studies) (Thompson and Higgins 2002). Meta-regression should generally not be considered when there are fewer than ten studies in a meta-analysis.
Meta-regressions are similar in essence to simple regressions, in which an outcome variable is predicted according to the values of one or more explanatory variables . In meta-regression, the outcome variable is the effect estimate (for example, a mean difference, a risk difference, a log odds ratio or a log risk ratio). The explanatory variables are characteristics of studies that might influence the size of intervention effect. These are often called ‘potential effect modifiers’ or covariates. Meta-regressions usually differ from simple regressions in two ways. First, larger studies have more influence on the relationship than smaller studies, since studies are weighted by the precision of their respective effect estimate. Second, it is wise to allow for the residual heterogeneity among intervention effects not modelled by the explanatory variables. This gives rise to the term ‘random-effects meta-regression’, since the extra variability is incorporated in the same way as in a random-effects meta-analysis (Thompson and Sharp 1999).
The regression coefficient obtained from a meta-regression analysis will describe how the outcome variable (the intervention effect) changes with a unit increase in the explanatory variable (the potential effect modifier). The statistical significance of the regression coefficient is a test of whether there is a linear relationship between intervention effect and the explanatory variable. If the intervention effect is a ratio measure, the log-transformed value of the intervention effect should always be used in the regression model (see Chapter 6, Section 6.1.2.1 ), and the exponential of the regression coefficient will give an estimate of the relative change in intervention effect with a unit increase in the explanatory variable.
Meta-regression can also be used to investigate differences for categorical explanatory variables as done in subgroup analyses. If there are J subgroups, membership of particular subgroups is indicated by using J minus 1 dummy variables (which can only take values of zero or one) in the meta-regression model (as in standard linear regression modelling). The regression coefficients will estimate how the intervention effect in each subgroup differs from a nominated reference subgroup. The P value of each regression coefficient will indicate the strength of evidence against the null hypothesis that the characteristic is not associated with the intervention effect.
Meta-regression may be performed using the ‘metareg’ macro available for the Stata statistical package, or using the ‘metafor’ package for R, as well as other packages.
10.11.5 Selection of study characteristics for subgroup analyses and meta-regression
Authors need to be cautious about undertaking subgroup analyses, and interpreting any that they do. Some considerations are outlined here for selecting characteristics (also called explanatory variables, potential effect modifiers or covariates) that will be investigated for their possible influence on the size of the intervention effect. These considerations apply similarly to subgroup analyses and to meta-regressions. Further details may be obtained elsewhere (Oxman and Guyatt 1992, Berlin and Antman 1994).
10.11.5.1 Ensure that there are adequate studies to justify subgroup analyses and meta-regressions
It is very unlikely that an investigation of heterogeneity will produce useful findings unless there is a substantial number of studies. Typical advice for undertaking simple regression analyses: that at least ten observations (i.e. ten studies in a meta-analysis) should be available for each characteristic modelled. However, even this will be too few when the covariates are unevenly distributed across studies.
10.11.5.2 Specify characteristics in advance
Authors should, whenever possible, pre-specify characteristics in the protocol that later will be subject to subgroup analyses or meta-regression. The plan specified in the protocol should then be followed (data permitting), without undue emphasis on any particular findings (see MECIR Box 10.11.b ). Pre-specifying characteristics reduces the likelihood of spurious findings, first by limiting the number of subgroups investigated, and second by preventing knowledge of the studies’ results influencing which subgroups are analysed. True pre-specification is difficult in systematic reviews, because the results of some of the relevant studies are often known when the protocol is drafted. If a characteristic was overlooked in the protocol, but is clearly of major importance and justified by external evidence, then authors should not be reluctant to explore it. However, such post-hoc analyses should be identified as such.
MECIR Box 10.11.b Relevant expectations for conduct of intervention reviews
Interpreting subgroup analyses ( ) | |
If subgroup analyses are conducted | Selective reporting, or over-interpretation, of particular subgroups or particular subgroup analyses should be avoided. This is a problem especially when multiple subgroup analyses are performed. This does not preclude the use of sensible and honest post hoc subgroup analyses. |
10.11.5.3 Select a small number of characteristics
The likelihood of a false-positive result among subgroup analyses and meta-regression increases with the number of characteristics investigated. It is difficult to suggest a maximum number of characteristics to look at, especially since the number of available studies is unknown in advance. If more than one or two characteristics are investigated it may be sensible to adjust the level of significance to account for making multiple comparisons.
10.11.5.4 Ensure there is scientific rationale for investigating each characteristic
Selection of characteristics should be motivated by biological and clinical hypotheses, ideally supported by evidence from sources other than the included studies. Subgroup analyses using characteristics that are implausible or clinically irrelevant are not likely to be useful and should be avoided. For example, a relationship between intervention effect and year of publication is seldom in itself clinically informative, and if identified runs the risk of initiating a post-hoc data dredge of factors that may have changed over time.
Prognostic factors are those that predict the outcome of a disease or condition, whereas effect modifiers are factors that influence how well an intervention works in affecting the outcome. Confusion between prognostic factors and effect modifiers is common in planning subgroup analyses, especially at the protocol stage. Prognostic factors are not good candidates for subgroup analyses unless they are also believed to modify the effect of intervention. For example, being a smoker may be a strong predictor of mortality within the next ten years, but there may not be reason for it to influence the effect of a drug therapy on mortality (Deeks 1998). Potential effect modifiers may include participant characteristics (age, setting), the precise interventions (dose of active intervention, choice of comparison intervention), how the study was done (length of follow-up) or methodology (design and quality).
10.11.5.5 Be aware that the effect of a characteristic may not always be identified
Many characteristics that might have important effects on how well an intervention works cannot be investigated using subgroup analysis or meta-regression. These are characteristics of participants that might vary substantially within studies, but that can only be summarized at the level of the study. An example is age. Consider a collection of clinical trials involving adults ranging from 18 to 60 years old. There may be a strong relationship between age and intervention effect that is apparent within each study. However, if the mean ages for the trials are similar, then no relationship will be apparent by looking at trial mean ages and trial-level effect estimates. The problem is one of aggregating individuals’ results and is variously known as aggregation bias, ecological bias or the ecological fallacy (Morgenstern 1982, Greenland 1987, Berlin et al 2002). It is even possible for the direction of the relationship across studies be the opposite of the direction of the relationship observed within each study.
10.11.5.6 Think about whether the characteristic is closely related to another characteristic (confounded)
The problem of ‘confounding’ complicates interpretation of subgroup analyses and meta-regressions and can lead to incorrect conclusions. Two characteristics are confounded if their influences on the intervention effect cannot be disentangled. For example, if those studies implementing an intensive version of a therapy happened to be the studies that involved patients with more severe disease, then one cannot tell which aspect is the cause of any difference in effect estimates between these studies and others. In meta-regression, co-linearity between potential effect modifiers leads to similar difficulties (Berlin and Antman 1994). Computing correlations between study characteristics will give some information about which study characteristics may be confounded with each other.
10.11.6 Interpretation of subgroup analyses and meta-regressions
Appropriate interpretation of subgroup analyses and meta-regressions requires caution (Oxman and Guyatt 1992).
- Subgroup comparisons are observational. It must be remembered that subgroup analyses and meta-regressions are entirely observational in their nature. These analyses investigate differences between studies. Even if individuals are randomized to one group or other within a clinical trial, they are not randomized to go in one trial or another. Hence, subgroup analyses suffer the limitations of any observational investigation, including possible bias through confounding by other study-level characteristics. Furthermore, even a genuine difference between subgroups is not necessarily due to the classification of the subgroups. As an example, a subgroup analysis of bone marrow transplantation for treating leukaemia might show a strong association between the age of a sibling donor and the success of the transplant. However, this probably does not mean that the age of donor is important. In fact, the age of the recipient is probably a key factor and the subgroup finding would simply be due to the strong association between the age of the recipient and the age of their sibling.
- Was the analysis pre-specified or post hoc? Authors should state whether subgroup analyses were pre-specified or undertaken after the results of the studies had been compiled (post hoc). More reliance may be placed on a subgroup analysis if it was one of a small number of pre-specified analyses. Performing numerous post-hoc subgroup analyses to explain heterogeneity is a form of data dredging. Data dredging is condemned because it is usually possible to find an apparent, but false, explanation for heterogeneity by considering lots of different characteristics.
- Is there indirect evidence in support of the findings? Differences between subgroups should be clinically plausible and supported by other external or indirect evidence, if they are to be convincing.
- Is the magnitude of the difference practically important? If the magnitude of a difference between subgroups will not result in different recommendations for different subgroups, then it may be better to present only the overall analysis results.
- Is there a statistically significant difference between subgroups? To establish whether there is a different effect of an intervention in different situations, the magnitudes of effects in different subgroups should be compared directly with each other. In particular, statistical significance of the results within separate subgroup analyses should not be compared (see Section 10.11.3.1 ).
- Are analyses looking at within-study or between-study relationships? For patient and intervention characteristics, differences in subgroups that are observed within studies are more reliable than analyses of subsets of studies. If such within-study relationships are replicated across studies then this adds confidence to the findings.
10.11.7 Investigating the effect of underlying risk
One potentially important source of heterogeneity among a series of studies is when the underlying average risk of the outcome event varies between the studies. The underlying risk of a particular event may be viewed as an aggregate measure of case-mix factors such as age or disease severity. It is generally measured as the observed risk of the event in the comparator group of each study (the comparator group risk, or CGR). The notion is controversial in its relevance to clinical practice since underlying risk represents a summary of both known and unknown risk factors. Problems also arise because comparator group risk will depend on the length of follow-up, which often varies across studies. However, underlying risk has received particular attention in meta-analysis because the information is readily available once dichotomous data have been prepared for use in meta-analyses. Sharp provides a full discussion of the topic (Sharp 2001).
Intuition would suggest that participants are more or less likely to benefit from an effective intervention according to their risk status. However, the relationship between underlying risk and intervention effect is a complicated issue. For example, suppose an intervention is equally beneficial in the sense that for all patients it reduces the risk of an event, say a stroke, to 80% of the underlying risk. Then it is not equally beneficial in terms of absolute differences in risk in the sense that it reduces a 50% stroke rate by 10 percentage points to 40% (number needed to treat=10), but a 20% stroke rate by 4 percentage points to 16% (number needed to treat=25).
Use of different summary statistics (risk ratio, odds ratio and risk difference) will demonstrate different relationships with underlying risk. Summary statistics that show close to no relationship with underlying risk are generally preferred for use in meta-analysis (see Section 10.4.3 ).
Investigating any relationship between effect estimates and the comparator group risk is also complicated by a technical phenomenon known as regression to the mean. This arises because the comparator group risk forms an integral part of the effect estimate. A high risk in a comparator group, observed entirely by chance, will on average give rise to a higher than expected effect estimate, and vice versa. This phenomenon results in a false correlation between effect estimates and comparator group risks. There are methods, which require sophisticated software, that correct for regression to the mean (McIntosh 1996, Thompson et al 1997). These should be used for such analyses, and statistical expertise is recommended.
10.11.8 Dose-response analyses
The principles of meta-regression can be applied to the relationships between intervention effect and dose (commonly termed dose-response), treatment intensity or treatment duration (Greenland and Longnecker 1992, Berlin et al 1993). Conclusions about differences in effect due to differences in dose (or similar factors) are on stronger ground if participants are randomized to one dose or another within a study and a consistent relationship is found across similar studies. While authors should consider these effects, particularly as a possible explanation for heterogeneity, they should be cautious about drawing conclusions based on between-study differences. Authors should be particularly cautious about claiming that a dose-response relationship does not exist, given the low power of many meta-regression analyses to detect genuine relationships.
10.12 Missing data
10.12.1 types of missing data.
There are many potential sources of missing data in a systematic review or meta-analysis (see Table 10.12.a ). For example, a whole study may be missing from the review, an outcome may be missing from a study, summary data may be missing for an outcome, and individual participants may be missing from the summary data. Here we discuss a variety of potential sources of missing data, highlighting where more detailed discussions are available elsewhere in the Handbook .
Whole studies may be missing from a review because they are never published, are published in obscure places, are rarely cited, or are inappropriately indexed in databases. Thus, review authors should always be aware of the possibility that they have failed to identify relevant studies. There is a strong possibility that such studies are missing because of their ‘uninteresting’ or ‘unwelcome’ findings (that is, in the presence of publication bias). This problem is discussed at length in Chapter 13 . Details of comprehensive search methods are provided in Chapter 4 .
Some studies might not report any information on outcomes of interest to the review. For example, there may be no information on quality of life, or on serious adverse effects. It is often difficult to determine whether this is because the outcome was not measured or because the outcome was not reported. Furthermore, failure to report that outcomes were measured may be dependent on the unreported results (selective outcome reporting bias; see Chapter 7, Section 7.2.3.3 ). Similarly, summary data for an outcome, in a form that can be included in a meta-analysis, may be missing. A common example is missing standard deviations (SDs) for continuous outcomes. This is often a problem when change-from-baseline outcomes are sought. We discuss imputation of missing SDs in Chapter 6, Section 6.5.2.8 . Other examples of missing summary data are missing sample sizes (particularly those for each intervention group separately), numbers of events, standard errors, follow-up times for calculating rates, and sufficient details of time-to-event outcomes. Inappropriate analyses of studies, for example of cluster-randomized and crossover trials, can lead to missing summary data. It is sometimes possible to approximate the correct analyses of such studies, for example by imputing correlation coefficients or SDs, as discussed in Chapter 23, Section 23.1 , for cluster-randomized studies and Chapter 23,Section 23.2 , for crossover trials. As a general rule, most methodologists believe that missing summary data (e.g. ‘no usable data’) should not be used as a reason to exclude a study from a systematic review. It is more appropriate to include the study in the review, and to discuss the potential implications of its absence from a meta-analysis.
It is likely that in some, if not all, included studies, there will be individuals missing from the reported results. Review authors are encouraged to consider this problem carefully (see MECIR Box 10.12.a ). We provide further discussion of this problem in Section 10.12.3 ; see also Chapter 8, Section 8.5 .
Missing data can also affect subgroup analyses. If subgroup analyses or meta-regressions are planned (see Section 10.11 ), they require details of the study-level characteristics that distinguish studies from one another. If these are not available for all studies, review authors should consider asking the study authors for more information.
Table 10.12.a Types of missing data in a meta-analysis
|
|
Missing studies | Publication bias Search not sufficiently comprehensive |
Missing outcomes | Outcome not measured Selective reporting bias |
Missing summary data | Selective reporting bias Incomplete reporting |
Missing individuals | Lack of intention-to-treat analysis Attrition from the study Selective reporting bias |
Missing study-level characteristics (for subgroup analysis or meta-regression) | Characteristic not measured Incomplete reporting |
MECIR Box 10.12.a Relevant expectations for conduct of intervention reviews
Addressing missing outcome data ( ) | |
| Incomplete outcome data can introduce bias. In most circumstances, authors should follow the principles of intention-to-treat analyses as far as possible (this may not be appropriate for adverse effects or if trying to demonstrate equivalence). Risk of bias due to incomplete outcome data is addressed in the Cochrane risk-of-bias tool. However, statistical analyses and careful interpretation of results are additional ways in which the issue can be addressed by review authors. Imputation methods can be considered (accompanied by, or in the form of, sensitivity analyses). |
10.12.2 General principles for dealing with missing data
There is a large literature of statistical methods for dealing with missing data. Here we briefly review some key concepts and make some general recommendations for Cochrane Review authors. It is important to think why data may be missing. Statisticians often use the terms ‘missing at random’ and ‘not missing at random’ to represent different scenarios.
Data are said to be ‘missing at random’ if the fact that they are missing is unrelated to actual values of the missing data. For instance, if some quality-of-life questionnaires were lost in the postal system, this would be unlikely to be related to the quality of life of the trial participants who completed the forms. In some circumstances, statisticians distinguish between data ‘missing at random’ and data ‘missing completely at random’, although in the context of a systematic review the distinction is unlikely to be important. Data that are missing at random may not be important. Analyses based on the available data will often be unbiased, although based on a smaller sample size than the original data set.
Data are said to be ‘not missing at random’ if the fact that they are missing is related to the actual missing data. For instance, in a depression trial, participants who had a relapse of depression might be less likely to attend the final follow-up interview, and more likely to have missing outcome data. Such data are ‘non-ignorable’ in the sense that an analysis of the available data alone will typically be biased. Publication bias and selective reporting bias lead by definition to data that are ‘not missing at random’, and attrition and exclusions of individuals within studies often do as well.
The principal options for dealing with missing data are:
- analysing only the available data (i.e. ignoring the missing data);
- imputing the missing data with replacement values, and treating these as if they were observed (e.g. last observation carried forward, imputing an assumed outcome such as assuming all were poor outcomes, imputing the mean, imputing based on predicted values from a regression analysis);
- imputing the missing data and accounting for the fact that these were imputed with uncertainty (e.g. multiple imputation, simple imputation methods (as point 2) with adjustment to the standard error); and
- using statistical models to allow for missing data, making assumptions about their relationships with the available data.
Option 2 is practical in most circumstances and very commonly used in systematic reviews. However, it fails to acknowledge uncertainty in the imputed values and results, typically, in confidence intervals that are too narrow. Options 3 and 4 would require involvement of a knowledgeable statistician.
Five general recommendations for dealing with missing data in Cochrane Reviews are as follows:
- Whenever possible, contact the original investigators to request missing data.
- Make explicit the assumptions of any methods used to address missing data: for example, that the data are assumed missing at random, or that missing values were assumed to have a particular value such as a poor outcome.
- Follow the guidance in Chapter 8 to assess risk of bias due to missing outcome data in randomized trials.
- Perform sensitivity analyses to assess how sensitive results are to reasonable changes in the assumptions that are made (see Section 10.14 ).
- Address the potential impact of missing data on the findings of the review in the Discussion section.
10.12.3 Dealing with missing outcome data from individual participants
Review authors may undertake sensitivity analyses to assess the potential impact of missing outcome data, based on assumptions about the relationship between missingness in the outcome and its true value. Several methods are available (Akl et al 2015). For dichotomous outcomes, Higgins and colleagues propose a strategy involving different assumptions about how the risk of the event among the missing participants differs from the risk of the event among the observed participants, taking account of uncertainty introduced by the assumptions (Higgins et al 2008a). Akl and colleagues propose a suite of simple imputation methods, including a similar approach to that of Higgins and colleagues based on relative risks of the event in missing versus observed participants. Similar ideas can be applied to continuous outcome data (Ebrahim et al 2013, Ebrahim et al 2014). Particular care is required to avoid double counting events, since it can be unclear whether reported numbers of events in trial reports apply to the full randomized sample or only to those who did not drop out (Akl et al 2016).
Although there is a tradition of implementing ‘worst case’ and ‘best case’ analyses clarifying the extreme boundaries of what is theoretically possible, such analyses may not be informative for the most plausible scenarios (Higgins et al 2008a).
10.13 Bayesian approaches to meta-analysis
Bayesian statistics is an approach to statistics based on a different philosophy from that which underlies significance tests and confidence intervals. It is essentially about updating of evidence. In a Bayesian analysis, initial uncertainty is expressed through a prior distribution about the quantities of interest. Current data and assumptions concerning how they were generated are summarized in the likelihood . The posterior distribution for the quantities of interest can then be obtained by combining the prior distribution and the likelihood. The likelihood summarizes both the data from studies included in the meta-analysis (for example, 2×2 tables from randomized trials) and the meta-analysis model (for example, assuming a fixed effect or random effects). The result of the analysis is usually presented as a point estimate and 95% credible interval from the posterior distribution for each quantity of interest, which look much like classical estimates and confidence intervals. Potential advantages of Bayesian analyses are summarized in Box 10.13.a . Bayesian analysis may be performed using WinBUGS software (Smith et al 1995, Lunn et al 2000), within R (Röver 2017), or – for some applications – using standard meta-regression software with a simple trick (Rhodes et al 2016).
A difference between Bayesian analysis and classical meta-analysis is that the interpretation is directly in terms of belief: a 95% credible interval for an odds ratio is that region in which we believe the odds ratio to lie with probability 95%. This is how many practitioners actually interpret a classical confidence interval, but strictly in the classical framework the 95% refers to the long-term frequency with which 95% intervals contain the true value. The Bayesian framework also allows a review author to calculate the probability that the odds ratio has a particular range of values, which cannot be done in the classical framework. For example, we can determine the probability that the odds ratio is less than 1 (which might indicate a beneficial effect of an experimental intervention), or that it is no larger than 0.8 (which might indicate a clinically important effect). It should be noted that these probabilities are specific to the choice of the prior distribution. Different meta-analysts may analyse the same data using different prior distributions and obtain different results. It is therefore important to carry out sensitivity analyses to investigate how the results depend on any assumptions made.
In the context of a meta-analysis, prior distributions are needed for the particular intervention effect being analysed (such as the odds ratio or the mean difference) and – in the context of a random-effects meta-analysis – on the amount of heterogeneity among intervention effects across studies. Prior distributions may represent subjective belief about the size of the effect, or may be derived from sources of evidence not included in the meta-analysis, such as information from non-randomized studies of the same intervention or from randomized trials of other interventions. The width of the prior distribution reflects the degree of uncertainty about the quantity. When there is little or no information, a ‘non-informative’ prior can be used, in which all values across the possible range are equally likely.
Most Bayesian meta-analyses use non-informative (or very weakly informative) prior distributions to represent beliefs about intervention effects, since many regard it as controversial to combine objective trial data with subjective opinion. However, prior distributions are increasingly used for the extent of among-study variation in a random-effects analysis. This is particularly advantageous when the number of studies in the meta-analysis is small, say fewer than five or ten. Libraries of data-based prior distributions are available that have been derived from re-analyses of many thousands of meta-analyses in the Cochrane Database of Systematic Reviews (Turner et al 2012).
Box 10.13.a Some potential advantages of Bayesian meta-analysis
Some potential advantages of Bayesian approaches over classical methods for meta-analyses are that they: of various clinical outcome states; ); ); ); and |
Statistical expertise is strongly recommended for review authors who wish to carry out Bayesian analyses. There are several good texts (Sutton et al 2000, Sutton and Abrams 2001, Spiegelhalter et al 2004).
10.14 Sensitivity analyses
The process of undertaking a systematic review involves a sequence of decisions. Whilst many of these decisions are clearly objective and non-contentious, some will be somewhat arbitrary or unclear. For instance, if eligibility criteria involve a numerical value, the choice of value is usually arbitrary: for example, defining groups of older people may reasonably have lower limits of 60, 65, 70 or 75 years, or any value in between. Other decisions may be unclear because a study report fails to include the required information. Some decisions are unclear because the included studies themselves never obtained the information required: for example, the outcomes of those who were lost to follow-up. Further decisions are unclear because there is no consensus on the best statistical method to use for a particular problem.
It is highly desirable to prove that the findings from a systematic review are not dependent on such arbitrary or unclear decisions by using sensitivity analysis (see MECIR Box 10.14.a ). A sensitivity analysis is a repeat of the primary analysis or meta-analysis in which alternative decisions or ranges of values are substituted for decisions that were arbitrary or unclear. For example, if the eligibility of some studies in the meta-analysis is dubious because they do not contain full details, sensitivity analysis may involve undertaking the meta-analysis twice: the first time including all studies and, second, including only those that are definitely known to be eligible. A sensitivity analysis asks the question, ‘Are the findings robust to the decisions made in the process of obtaining them?’
MECIR Box 10.14.a Relevant expectations for conduct of intervention reviews
Sensitivity analysis ( ) | |
| It is important to be aware when results are robust, since the strength of the conclusion may be strengthened or weakened. |
There are many decision nodes within the systematic review process that can generate a need for a sensitivity analysis. Examples include:
Searching for studies:
- Should abstracts whose results cannot be confirmed in subsequent publications be included in the review?
Eligibility criteria:
- Characteristics of participants: where a majority but not all people in a study meet an age range, should the study be included?
- Characteristics of the intervention: what range of doses should be included in the meta-analysis?
- Characteristics of the comparator: what criteria are required to define usual care to be used as a comparator group?
- Characteristics of the outcome: what time point or range of time points are eligible for inclusion?
- Study design: should blinded and unblinded outcome assessment be included, or should study inclusion be restricted by other aspects of methodological criteria?
What data should be analysed?
- Time-to-event data: what assumptions of the distribution of censored data should be made?
- Continuous data: where standard deviations are missing, when and how should they be imputed? Should analyses be based on change scores or on post-intervention values?
- Ordinal scales: what cut-point should be used to dichotomize short ordinal scales into two groups?
- Cluster-randomized trials: what values of the intraclass correlation coefficient should be used when trial analyses have not been adjusted for clustering?
- Crossover trials: what values of the within-subject correlation coefficient should be used when this is not available in primary reports?
- All analyses: what assumptions should be made about missing outcomes? Should adjusted or unadjusted estimates of intervention effects be used?
Analysis methods:
- Should fixed-effect or random-effects methods be used for the analysis?
- For dichotomous outcomes, should odds ratios, risk ratios or risk differences be used?
- For continuous outcomes, where several scales have assessed the same dimension, should results be analysed as a standardized mean difference across all scales or as mean differences individually for each scale?
Some sensitivity analyses can be pre-specified in the study protocol, but many issues suitable for sensitivity analysis are only identified during the review process where the individual peculiarities of the studies under investigation are identified. When sensitivity analyses show that the overall result and conclusions are not affected by the different decisions that could be made during the review process, the results of the review can be regarded with a higher degree of certainty. Where sensitivity analyses identify particular decisions or missing information that greatly influence the findings of the review, greater resources can be deployed to try and resolve uncertainties and obtain extra information, possibly through contacting trial authors and obtaining individual participant data. If this cannot be achieved, the results must be interpreted with an appropriate degree of caution. Such findings may generate proposals for further investigations and future research.
Reporting of sensitivity analyses in a systematic review may best be done by producing a summary table. Rarely is it informative to produce individual forest plots for each sensitivity analysis undertaken.
Sensitivity analyses are sometimes confused with subgroup analysis. Although some sensitivity analyses involve restricting the analysis to a subset of the totality of studies, the two methods differ in two ways. First, sensitivity analyses do not attempt to estimate the effect of the intervention in the group of studies removed from the analysis, whereas in subgroup analyses, estimates are produced for each subgroup. Second, in sensitivity analyses, informal comparisons are made between different ways of estimating the same thing, whereas in subgroup analyses, formal statistical comparisons are made across the subgroups.
10.15 Chapter information
Editors: Jonathan J Deeks, Julian PT Higgins, Douglas G Altman; on behalf of the Cochrane Statistical Methods Group
Contributing authors: Douglas Altman, Deborah Ashby, Jacqueline Birks, Michael Borenstein, Marion Campbell, Jonathan Deeks, Matthias Egger, Julian Higgins, Joseph Lau, Keith O’Rourke, Gerta Rücker, Rob Scholten, Jonathan Sterne, Simon Thompson, Anne Whitehead
Acknowledgements: We are grateful to the following for commenting helpfully on earlier drafts: Bodil Als-Nielsen, Deborah Ashby, Jesse Berlin, Joseph Beyene, Jacqueline Birks, Michael Bracken, Marion Campbell, Chris Cates, Wendong Chen, Mike Clarke, Albert Cobos, Esther Coren, Francois Curtin, Roberto D’Amico, Keith Dear, Heather Dickinson, Diana Elbourne, Simon Gates, Paul Glasziou, Christian Gluud, Peter Herbison, Sally Hollis, David Jones, Steff Lewis, Tianjing Li, Joanne McKenzie, Philippa Middleton, Nathan Pace, Craig Ramsey, Keith O’Rourke, Rob Scholten, Guido Schwarzer, Jack Sinclair, Jonathan Sterne, Simon Thompson, Andy Vail, Clarine van Oel, Paula Williamson and Fred Wolf.
Funding: JJD received support from the National Institute for Health Research (NIHR) Birmingham Biomedical Research Centre at the University Hospitals Birmingham NHS Foundation Trust and the University of Birmingham. JPTH is a member of the NIHR Biomedical Research Centre at University Hospitals Bristol NHS Foundation Trust and the University of Bristol. JPTH received funding from National Institute for Health Research Senior Investigator award NF-SI-0617-10145. The views expressed are those of the author(s) and not necessarily those of the NHS, the NIHR or the Department of Health.
10.16 References
Agresti A. An Introduction to Categorical Data Analysis . New York (NY): John Wiley & Sons; 1996.
Akl EA, Kahale LA, Agoritsas T, Brignardello-Petersen R, Busse JW, Carrasco-Labra A, Ebrahim S, Johnston BC, Neumann I, Sola I, Sun X, Vandvik P, Zhang Y, Alonso-Coello P, Guyatt G. Handling trial participants with missing outcome data when conducting a meta-analysis: a systematic survey of proposed approaches. Systematic Reviews 2015; 4 : 98.
Akl EA, Kahale LA, Ebrahim S, Alonso-Coello P, Schünemann HJ, Guyatt GH. Three challenges described for identifying participants with missing data in trials reports, and potential solutions suggested to systematic reviewers. Journal of Clinical Epidemiology 2016; 76 : 147-154.
Altman DG, Bland JM. Detecting skewness from summary information. BMJ 1996; 313 : 1200.
Anzures-Cabrera J, Sarpatwari A, Higgins JPT. Expressing findings from meta-analyses of continuous outcomes in terms of risks. Statistics in Medicine 2011; 30 : 2967-2985.
Berlin JA, Longnecker MP, Greenland S. Meta-analysis of epidemiologic dose-response data. Epidemiology 1993; 4 : 218-228.
Berlin JA, Antman EM. Advantages and limitations of metaanalytic regressions of clinical trials data. Online Journal of Current Clinical Trials 1994; Doc No 134 .
Berlin JA, Santanna J, Schmid CH, Szczech LA, Feldman KA, Group A-LAITS. Individual patient- versus group-level data meta-regressions for the investigation of treatment effect modifiers: ecological bias rears its ugly head. Statistics in Medicine 2002; 21 : 371-387.
Borenstein M, Hedges LV, Higgins JPT, Rothstein HR. A basic introduction to fixed-effect and random-effects models for meta-analysis. Research Synthesis Methods 2010; 1 : 97-111.
Borenstein M, Higgins JPT. Meta-analysis and subgroups. Prev Sci 2013; 14 : 134-143.
Bradburn MJ, Deeks JJ, Berlin JA, Russell Localio A. Much ado about nothing: a comparison of the performance of meta-analytical methods with rare events. Statistics in Medicine 2007; 26 : 53-77.
Chinn S. A simple method for converting an odds ratio to effect size for use in meta-analysis. Statistics in Medicine 2000; 19 : 3127-3131.
da Costa BR, Nuesch E, Rutjes AW, Johnston BC, Reichenbach S, Trelle S, Guyatt GH, Jüni P. Combining follow-up and change data is valid in meta-analyses of continuous outcomes: a meta-epidemiological study. Journal of Clinical Epidemiology 2013; 66 : 847-855.
Deeks JJ. Systematic reviews of published evidence: Miracles or minefields? Annals of Oncology 1998; 9 : 703-709.
Deeks JJ, Altman DG, Bradburn MJ. Statistical methods for examining heterogeneity and combining results from several studies in meta-analysis. In: Egger M, Davey Smith G, Altman DG, editors. Systematic Reviews in Health Care: Meta-analysis in Context . 2nd edition ed. London (UK): BMJ Publication Group; 2001. p. 285-312.
Deeks JJ. Issues in the selection of a summary statistic for meta-analysis of clinical trials with binary outcomes. Statistics in Medicine 2002; 21 : 1575-1600.
DerSimonian R, Laird N. Meta-analysis in clinical trials. Controlled Clinical Trials 1986; 7 : 177-188.
DiGuiseppi C, Higgins JPT. Interventions for promoting smoke alarm ownership and function. Cochrane Database of Systematic Reviews 2001; 2 : CD002246.
Ebrahim S, Akl EA, Mustafa RA, Sun X, Walter SD, Heels-Ansdell D, Alonso-Coello P, Johnston BC, Guyatt GH. Addressing continuous data for participants excluded from trial analysis: a guide for systematic reviewers. Journal of Clinical Epidemiology 2013; 66 : 1014-1021 e1011.
Ebrahim S, Johnston BC, Akl EA, Mustafa RA, Sun X, Walter SD, Heels-Ansdell D, Alonso-Coello P, Guyatt GH. Addressing continuous data measured with different instruments for participants excluded from trial analysis: a guide for systematic reviewers. Journal of Clinical Epidemiology 2014; 67 : 560-570.
Efthimiou O. Practical guide to the meta-analysis of rare events. Evidence-Based Mental Health 2018; 21 : 72-76.
Egger M, Davey Smith G, Schneider M, Minder C. Bias in meta-analysis detected by a simple, graphical test. BMJ 1997; 315 : 629-634.
Engels EA, Schmid CH, Terrin N, Olkin I, Lau J. Heterogeneity and statistical significance in meta-analysis: an empirical study of 125 meta-analyses. Statistics in Medicine 2000; 19 : 1707-1728.
Greenland S, Robins JM. Estimation of a common effect parameter from sparse follow-up data. Biometrics 1985; 41 : 55-68.
Greenland S. Quantitative methods in the review of epidemiologic literature. Epidemiologic Reviews 1987; 9 : 1-30.
Greenland S, Longnecker MP. Methods for trend estimation from summarized dose-response data, with applications to meta-analysis. American Journal of Epidemiology 1992; 135 : 1301-1309.
Guevara JP, Berlin JA, Wolf FM. Meta-analytic methods for pooling rates when follow-up duration varies: a case study. BMC Medical Research Methodology 2004; 4 : 17.
Hartung J, Knapp G. A refined method for the meta-analysis of controlled clinical trials with binary outcome. Statistics in Medicine 2001; 20 : 3875-3889.
Hasselblad V, McCrory DC. Meta-analytic tools for medical decision making: A practical guide. Medical Decision Making 1995; 15 : 81-96.
Higgins JPT, Thompson SG. Quantifying heterogeneity in a meta-analysis. Statistics in Medicine 2002; 21 : 1539-1558.
Higgins JPT, Thompson SG, Deeks JJ, Altman DG. Measuring inconsistency in meta-analyses. BMJ 2003; 327 : 557-560.
Higgins JPT, Thompson SG. Controlling the risk of spurious findings from meta-regression. Statistics in Medicine 2004; 23 : 1663-1682.
Higgins JPT, White IR, Wood AM. Imputation methods for missing outcome data in meta-analysis of clinical trials. Clinical Trials 2008a; 5 : 225-239.
Higgins JPT, White IR, Anzures-Cabrera J. Meta-analysis of skewed data: combining results reported on log-transformed or raw scales. Statistics in Medicine 2008b; 27 : 6072-6092.
Higgins JPT, Thompson SG, Spiegelhalter DJ. A re-evaluation of random-effects meta-analysis. Journal of the Royal Statistical Society: Series A (Statistics in Society) 2009; 172 : 137-159.
Kjaergard LL, Villumsen J, Gluud C. Reported methodologic quality and discrepancies between large and small randomized trials in meta-analyses. Annals of Internal Medicine 2001; 135 : 982-989.
Langan D, Higgins JPT, Simmonds M. An empirical comparison of heterogeneity variance estimators in 12 894 meta-analyses. Research Synthesis Methods 2015; 6 : 195-205.
Langan D, Higgins JPT, Simmonds M. Comparative performance of heterogeneity variance estimators in meta-analysis: a review of simulation studies. Research Synthesis Methods 2017; 8 : 181-198.
Langan D, Higgins JPT, Jackson D, Bowden J, Veroniki AA, Kontopantelis E, Viechtbauer W, Simmonds M. A comparison of heterogeneity variance estimators in simulated random-effects meta-analyses. Research Synthesis Methods 2019; 10 : 83-98.
Lewis S, Clarke M. Forest plots: trying to see the wood and the trees. BMJ 2001; 322 : 1479-1480.
Lunn DJ, Thomas A, Best N, Spiegelhalter D. WinBUGS - A Bayesian modelling framework: Concepts, structure, and extensibility. Statistics and Computing 2000; 10 : 325-337.
Mantel N, Haenszel W. Statistical aspects of the analysis of data from retrospective studies of disease. Journal of the National Cancer Institute 1959; 22 : 719-748.
McIntosh MW. The population risk as an explanatory variable in research synthesis of clinical trials. Statistics in Medicine 1996; 15 : 1713-1728.
Morgenstern H. Uses of ecologic analysis in epidemiologic research. American Journal of Public Health 1982; 72 : 1336-1344.
Oxman AD, Guyatt GH. A consumers guide to subgroup analyses. Annals of Internal Medicine 1992; 116 : 78-84.
Peto R, Collins R, Gray R. Large-scale randomized evidence: large, simple trials and overviews of trials. Journal of Clinical Epidemiology 1995; 48 : 23-40.
Poole C, Greenland S. Random-effects meta-analyses are not always conservative. American Journal of Epidemiology 1999; 150 : 469-475.
Rhodes KM, Turner RM, White IR, Jackson D, Spiegelhalter DJ, Higgins JPT. Implementing informative priors for heterogeneity in meta-analysis using meta-regression and pseudo data. Statistics in Medicine 2016; 35 : 5495-5511.
Rice K, Higgins JPT, Lumley T. A re-evaluation of fixed effect(s) meta-analysis. Journal of the Royal Statistical Society Series A (Statistics in Society) 2018; 181 : 205-227.
Riley RD, Higgins JPT, Deeks JJ. Interpretation of random effects meta-analyses. BMJ 2011; 342 : d549.
Röver C. Bayesian random-effects meta-analysis using the bayesmeta R package 2017. https://arxiv.org/abs/1711.08683 .
Rücker G, Schwarzer G, Carpenter J, Olkin I. Why add anything to nothing? The arcsine difference as a measure of treatment effect in meta-analysis with zero cells. Statistics in Medicine 2009; 28 : 721-738.
Sharp SJ. Analysing the relationship between treatment benefit and underlying risk: precautions and practical recommendations. In: Egger M, Davey Smith G, Altman DG, editors. Systematic Reviews in Health Care: Meta-analysis in Context . 2nd edition ed. London (UK): BMJ Publication Group; 2001. p. 176-188.
Sidik K, Jonkman JN. A simple confidence interval for meta-analysis. Statistics in Medicine 2002; 21 : 3153-3159.
Simmonds MC, Tierney J, Bowden J, Higgins JPT. Meta-analysis of time-to-event data: a comparison of two-stage methods. Research Synthesis Methods 2011; 2 : 139-149.
Sinclair JC, Bracken MB. Clinically useful measures of effect in binary analyses of randomized trials. Journal of Clinical Epidemiology 1994; 47 : 881-889.
Smith TC, Spiegelhalter DJ, Thomas A. Bayesian approaches to random-effects meta-analysis: a comparative study. Statistics in Medicine 1995; 14 : 2685-2699.
Spiegelhalter DJ, Abrams KR, Myles JP. Bayesian Approaches to Clinical Trials and Health-Care Evaluation . Chichester (UK): John Wiley & Sons; 2004.
Spittal MJ, Pirkis J, Gurrin LC. Meta-analysis of incidence rate data in the presence of zero events. BMC Medical Research Methodology 2015; 15 : 42.
Sutton AJ, Abrams KR, Jones DR, Sheldon TA, Song F. Methods for Meta-analysis in Medical Research . Chichester (UK): John Wiley & Sons; 2000.
Sutton AJ, Abrams KR. Bayesian methods in meta-analysis and evidence synthesis. Statistical Methods in Medical Research 2001; 10 : 277-303.
Sweeting MJ, Sutton AJ, Lambert PC. What to add to nothing? Use and avoidance of continuity corrections in meta-analysis of sparse data. Statistics in Medicine 2004; 23 : 1351-1375.
Thompson SG, Smith TC, Sharp SJ. Investigating underlying risk as a source of heterogeneity in meta-analysis. Statistics in Medicine 1997; 16 : 2741-2758.
Thompson SG, Sharp SJ. Explaining heterogeneity in meta-analysis: a comparison of methods. Statistics in Medicine 1999; 18 : 2693-2708.
Thompson SG, Higgins JPT. How should meta-regression analyses be undertaken and interpreted? Statistics in Medicine 2002; 21 : 1559-1574.
Turner RM, Davey J, Clarke MJ, Thompson SG, Higgins JPT. Predicting the extent of heterogeneity in meta-analysis, using empirical data from the Cochrane Database of Systematic Reviews. International Journal of Epidemiology 2012; 41 : 818-827.
Veroniki AA, Jackson D, Viechtbauer W, Bender R, Bowden J, Knapp G, Kuss O, Higgins JPT, Langan D, Salanti G. Methods to estimate the between-study variance and its uncertainty in meta-analysis. Research Synthesis Methods 2016; 7 : 55-79.
Whitehead A, Jones NMB. A meta-analysis of clinical trials involving different classifications of response into ordered categories. Statistics in Medicine 1994; 13 : 2503-2515.
Yusuf S, Peto R, Lewis J, Collins R, Sleight P. Beta blockade during and after myocardial infarction: an overview of the randomized trials. Progress in Cardiovascular Diseases 1985; 27 : 335-371.
Yusuf S, Wittes J, Probstfield J, Tyroler HA. Analysis and interpretation of treatment effects in subgroups of patients in randomized clinical trials. JAMA 1991; 266 : 93-98.
For permission to re-use material from the Handbook (either academic or commercial), please see here for full details.

The Guide to Literature Reviews
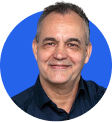
- What is a Literature Review?
- The Purpose of Literature Reviews
- Guidelines for Writing a Literature Review
- How to Organize a Literature Review?
- Software for Literature Reviews
- Using Artificial Intelligence for Literature Reviews
- How to Conduct a Literature Review?
- Common Mistakes and Pitfalls in a Literature Review
- Methods for Literature Reviews
- What is a Systematic Literature Review?
- What is a Narrative Literature Review?
- What is a Descriptive Literature Review?
- What is a Scoping Literature Review?
- What is a Realist Literature Review?
- What is a Critical Literature Review?
- Introduction
What are the differences between a meta-analysis and a literature review?
- How to conduct a meta-analysis?
When to conduct meta-analyses?
- What is an Umbrella Literature Review?
- Differences Between Annotated Bibliographies and Literature Reviews
- Literature Review vs. Theoretical Framework
- How to Write a Literature Review?
- How to Structure a Literature Review?
- How to Make a Cover Page for a Literature Review?
- How to Write an Abstract for a Literature Review?
- How to Write a Literature Review Introduction?
- How to Write the Body of a Literature Review?
- How to Write a Literature Review Conclusion?
- How to Make a Literature Review Bibliography?
- How to Format a Literature Review?
- How Long Should a Literature Review Be?
- Examples of Literature Reviews
- How to Present a Literature Review?
- How to Publish a Literature Review?
Meta-Analysis vs. Literature Review
A meta-analysis is a statistical method used to combine data from multiple independent studies, often conducted as part of comprehensive literature reviews to provide more precise estimates and robust conclusions. Its purpose is to provide a more precise estimate of effect sizes by aggregating results from various studies. Meta-analyses are crucial in evidence-based medicine as they provide robust conclusions based on empirical evidence.
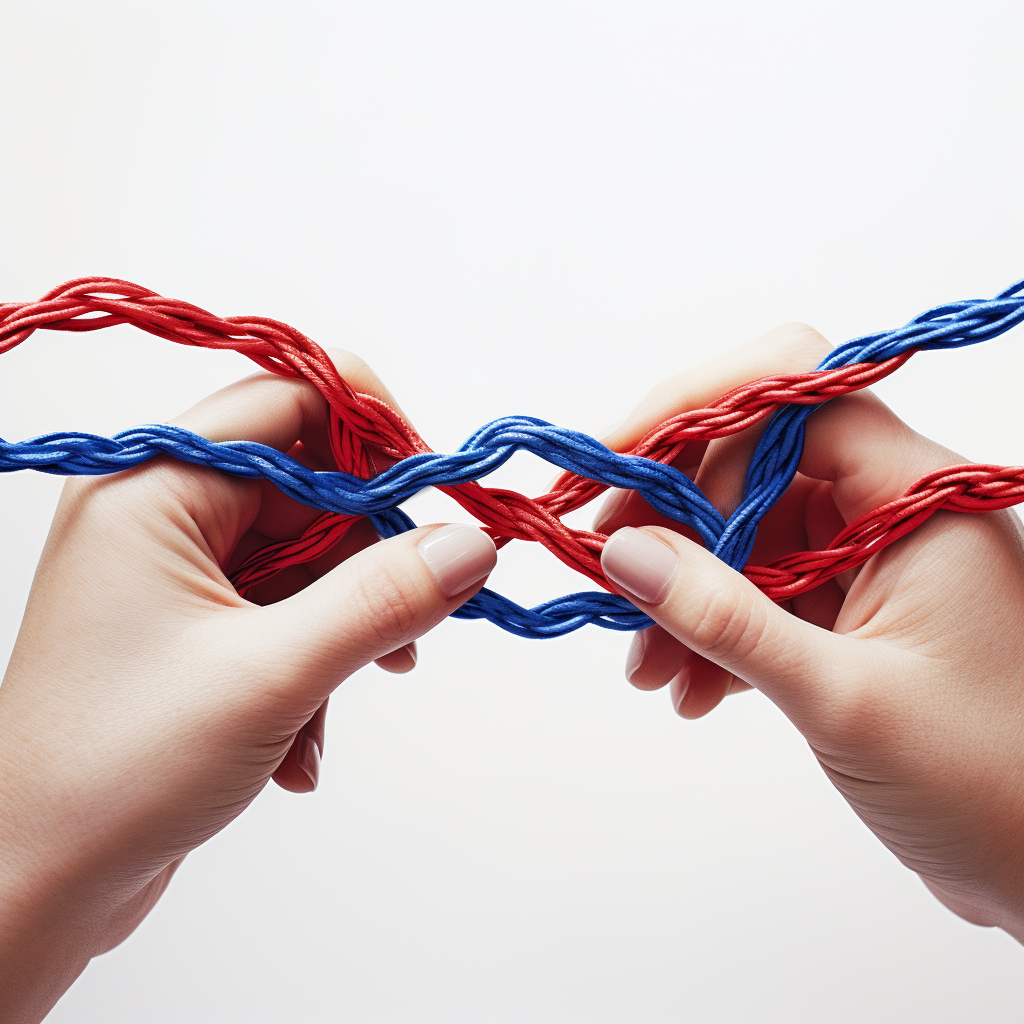
The primary purpose of a meta-analysis is to synthesize quantitative data from multiple studies to arrive at a single conclusion. By combining results, a meta-analysis can increase statistical power, making it possible to detect effects that may be missed in individual studies. It helps to resolve uncertainty when studies disagree and provides a comprehensive understanding of effect size across different contexts and conditions.
Meta-analyses are important because they offer a higher level of evidence than individual studies. They help researchers and practitioners make informed decisions by summarizing the best available evidence. In clinical settings, meta-analyses can guide treatment choices by comparing the effectiveness of different interventions. Meta-analyses help identify gaps in existing research, paving the way for future studies. They are also essential for developing guidelines and policies based on a thorough synthesis of the evidence.
Although meta-analyses are commonly associated with quantitative research , they can also be applied to qualitative research through a process known as meta-synthesis. Meta-synthesis involves systematically reviewing and integrating findings from multiple qualitative studies to draw broader conclusions. This approach allows researchers to combine qualitative data to develop new theories, understand complex phenomena, and gain insights into contextual factors.
Using meta-synthesis, qualitative meta-analyses can help provide a deeper understanding of a research topic by incorporating diverse perspectives and experiences from various studies. This method can reveal patterns and themes that might not be evident in individual qualitative studies, thereby enhancing the richness and depth of the analysis. By combining the strengths of both quantitative and qualitative research, meta-analyses can offer a more comprehensive view of the research landscape, supporting evidence-based practice and informed decision-making.
A meta-analysis literature review combines elements of both meta-analysis and traditional literature review methodologies. It is a comprehensive approach that includes both a qualitative summary and a quantitative synthesis of research findings. It is a hybrid approach that leverages the strengths of both meta-analysis and traditional literature reviews to provide a thorough and nuanced understanding of a research topic. Read this article to find out more about the differences, and when to use it.
A meta-analysis and literature reviews differ in purpose, methodology, and outcomes. The primary purpose of a meta-analysis is to provide a quantitative analysis of data from multiple studies, producing a precise estimate of the effect size through statistical methods. A literature review synthesizes findings to offer an overview of current knowledge, identify gaps, and suggest future research areas. Literature reviews can be systematic, scoping, or narrative reviews among others.
Meta-analyses use systematic methods, including defining inclusion and exclusion criteria, conducting a systematic search, extracting data, and applying statistical methods such as calculating the standardized mean difference or risk ratio. Other reviews, like systematic and scoping reviews, summarize relevant studies without combining results statistically. Narrative reviews provide qualitative summaries and interpretations.
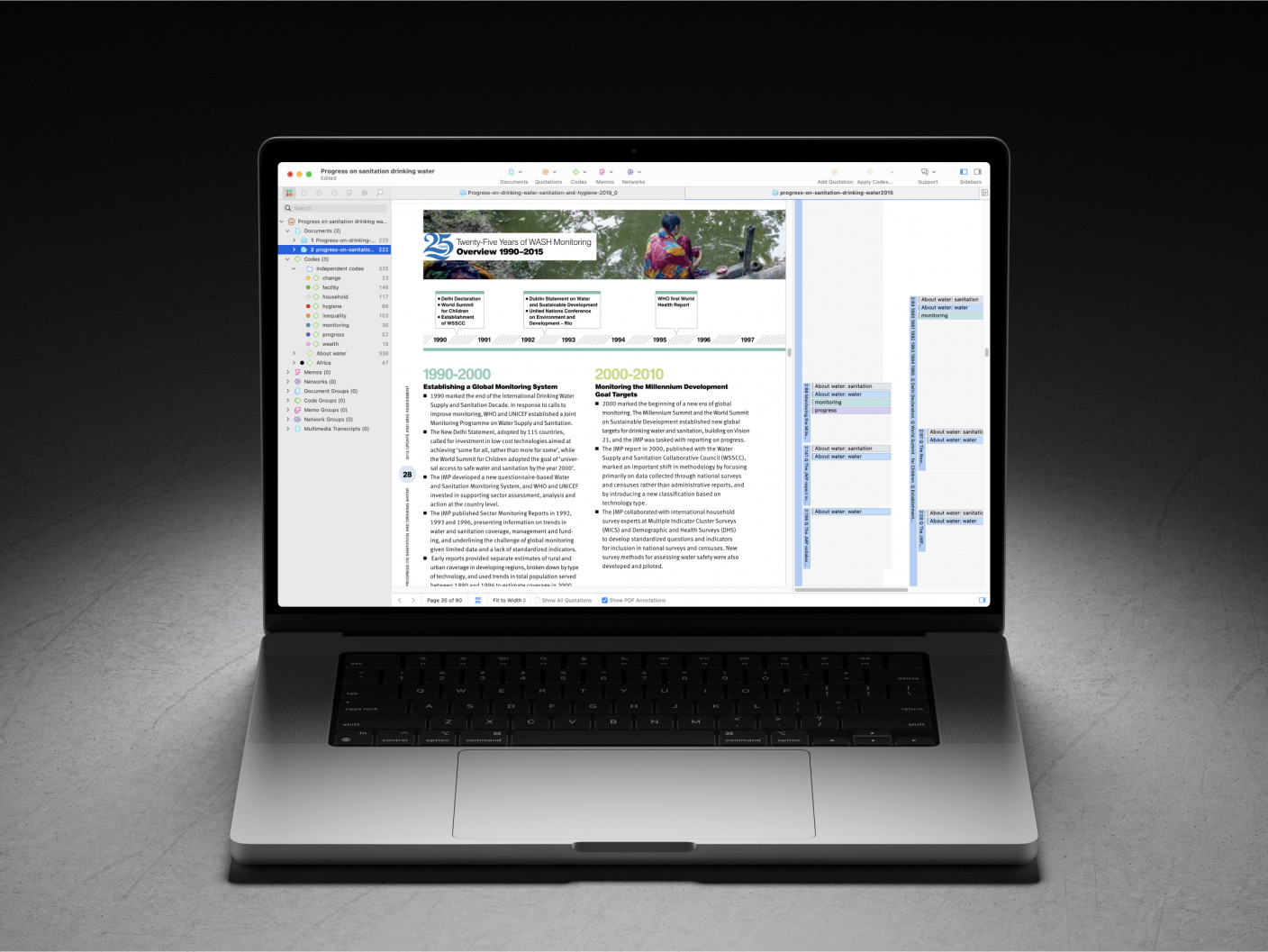
Quality literature reviews start with ATLAS.ti
From searching literature to analyzing it, ATLAS.ti is there for you at every step. See how with a free trial.
The outcome of a meta-analysis is a quantitative synthesis, offering more precise estimates of key variable effect sizes and identifying patterns through subgroup analysis and forest plots. Systematic reviews provide comprehensive literature summaries, highlighting research strengths and weaknesses. Scoping reviews map key concepts and evidence, while narrative reviews offer critical analysis.
Meta-analyses focus on combining quantitative data, often used in fields with substantial empirical evidence and similar research methods. Systematic and scoping reviews have a broader scope, and narrative reviews provide critical insights into theories and concepts. Each review type offers unique benefits, depending on the research goals.
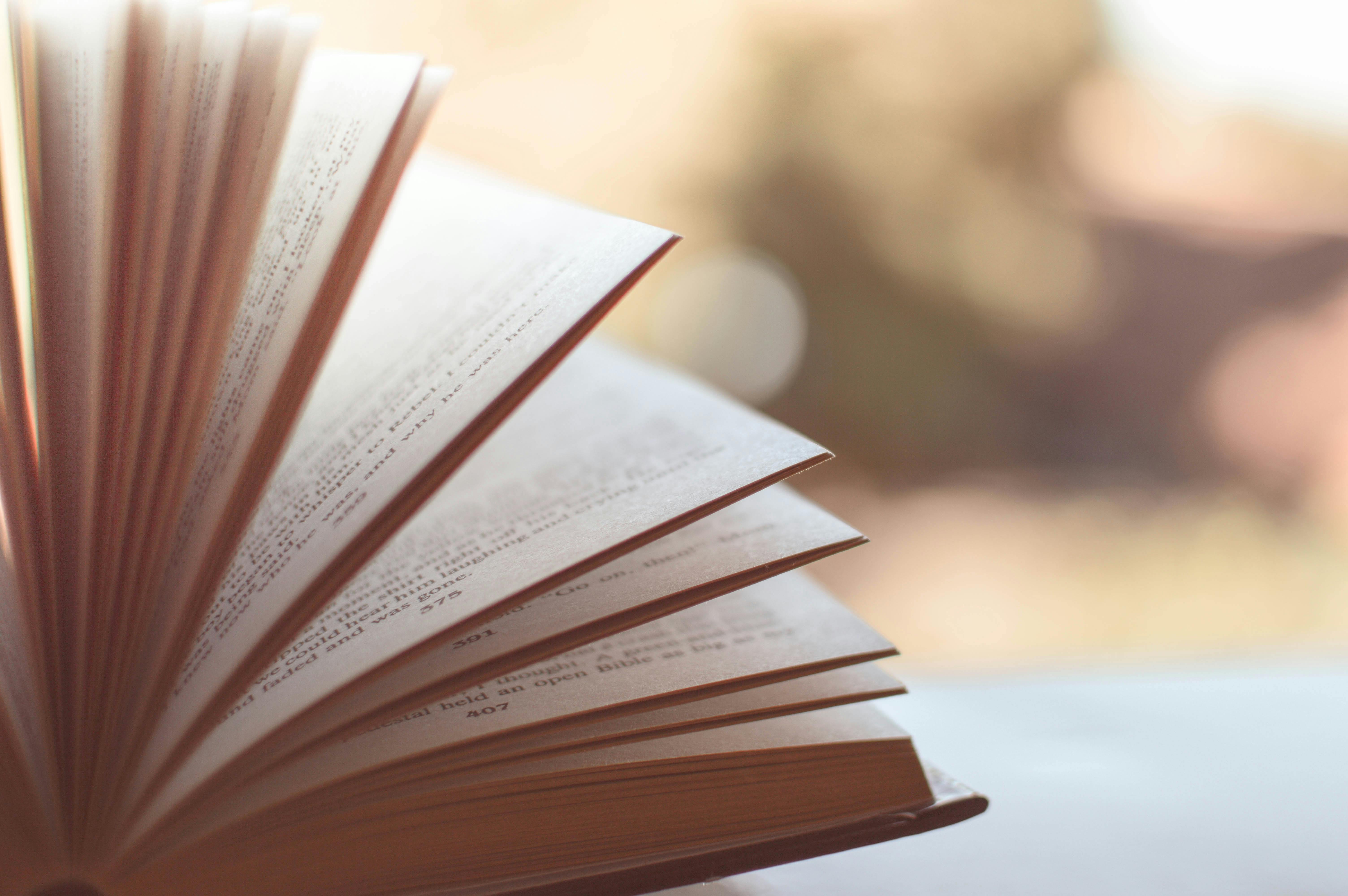
Conducting a meta-analysis involves a systematic and rigorous process. By following established guidelines and best practices, such as those outlined in the Cochrane Handbook for Systematic Reviews of Interventions and the PRISMA statement, researchers can effectively combine data from multiple studies to derive more precise estimates of effect sizes. The following steps provide a structured approach to conducting a meta-analysis, ensuring a comprehensive and reproducible methodology (Higgins & Green, 2011; Moher et al., 2009).
Formulate a research question : Define a specific research question that the meta-analysis will address. This question guides the entire process.
Systematic search : Conduct a systematic search to identify relevant studies. Use scholarly databases to find studies on the same topic.
Inclusion and exclusion criteria : Establish clear inclusion and exclusion criteria to select studies. This ensures that only relevant studies are included.
Data extraction : Extract relevant data from the included studies. Key data points include effect sizes, sample sizes, and study characteristics.
Statistical methods : Use statistical methods to combine data from the studies. Common methods include calculating the standardized mean difference and risk ratio.
Subgroup analysis : Perform subgroup analyses to explore differences among studies. This can help identify factors that influence the overall estimate.
Forest plot : Create a forest plot to visualize the results of the meta-analysis. This plot shows the effect sizes and confidence intervals for each study.
Interpret results : Interpret the results in the context of the existing literature. Discuss the implications of the findings and their relevance to the research question.
Report findings : Write a comprehensive report detailing the methodology, findings, and conclusions. Ensure that the report is clear and reproducible.
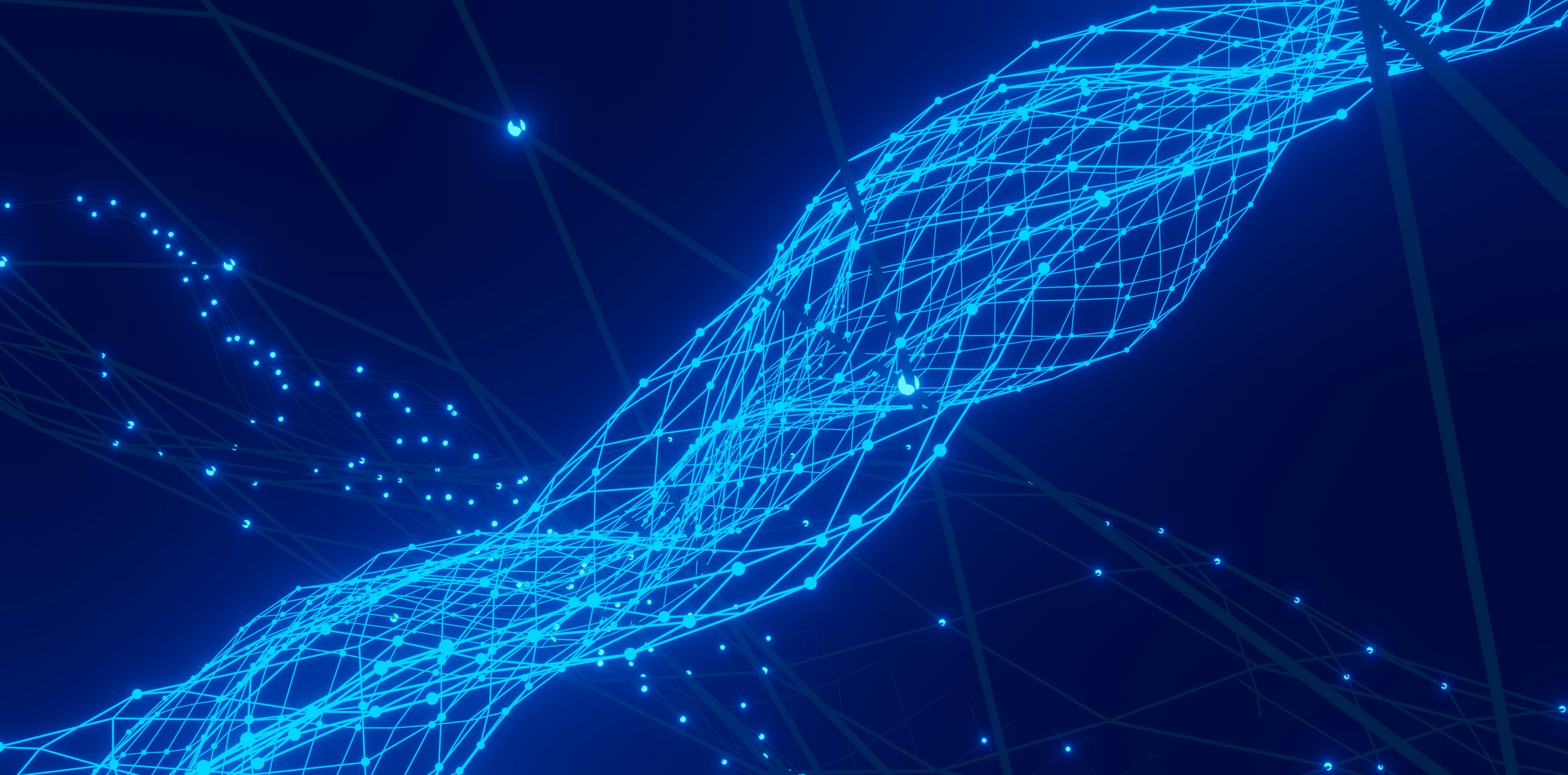
Meta-analyses are conducted to achieve a quantitative analysis of data from multiple studies, providing more precise and robust conclusions. They are particularly useful when individual studies yield conflicting results, as combining data can help resolve discrepancies and offer a clearer understanding of the effect size. Meta-analyses enhance statistical power by aggregating data from studies with small sample sizes, making it possible to detect significant effects that individual studies might miss.
These analyses are crucial for generalizing findings across different populations, settings, or conditions, offering broader insights that are not limited to a single study's context. In evidence-based fields such as medicine, education, and psychology, meta-analyses often include data from randomized controlled trials and observational studies, providing a high level of evidence. This synthesis aids practitioners in making informed decisions and developing effective interventions.
Meta-analyses also help identify patterns, trends, and gaps in existing research. This is achieved through a systematic review attempt and critical analysis of previous studies, guiding future research directions. This supports informed decision-making and the creation of robust clinical practice recommendations. Conducting meta-analyses is essential for advancing knowledge, improving practices, and ensuring that decisions are based on the best available evidence.
Additionally, meta-analyses complement scoping reviews and literature reviews by providing a quantitative analysis of study findings, which literature reviews provide qualitatively. They form a crucial part of the research process , transforming diverse research papers into coherent, actionable insights.
Meta-analyses are powerful methods for synthesizing quantitative data from multiple studies, offering precise estimates and robust conclusions. By combining results, they enhance statistical power and resolve conflicting findings, providing a comprehensive understanding of research topics. Meta-analyses are essential in evidence-based fields, guiding informed decision-making and developing effective interventions. They complement literature reviews by adding a quantitative dimension to the analysis. Meta-synthesis extends the principles of meta-analysis to qualitative research, providing deeper insights and broader perspectives.
Higgins, J. P. T., & Green, S. (Eds.). (2011). Cochrane handbook for systematic reviews of interventions (Version 5.1.0). The Cochrane Collaboration. Available from: www.cochrane-handbook.org
Moher, D., Liberati, A., Tetzlaff, J., Altman, D. G., & The PRISMA Group. (2009). Preferred reporting items for systematic reviews and meta-analyses: The PRISMA statement. PLoS Med, 6(7), e1000097. https://doi.org/10.1371/journal.pmed.1000097
Borenstein, M., Hedges, L. V., Higgins, J. P. T., & Rothstein, H. R. (2009). Introduction to meta-analysis. John Wiley & Sons.
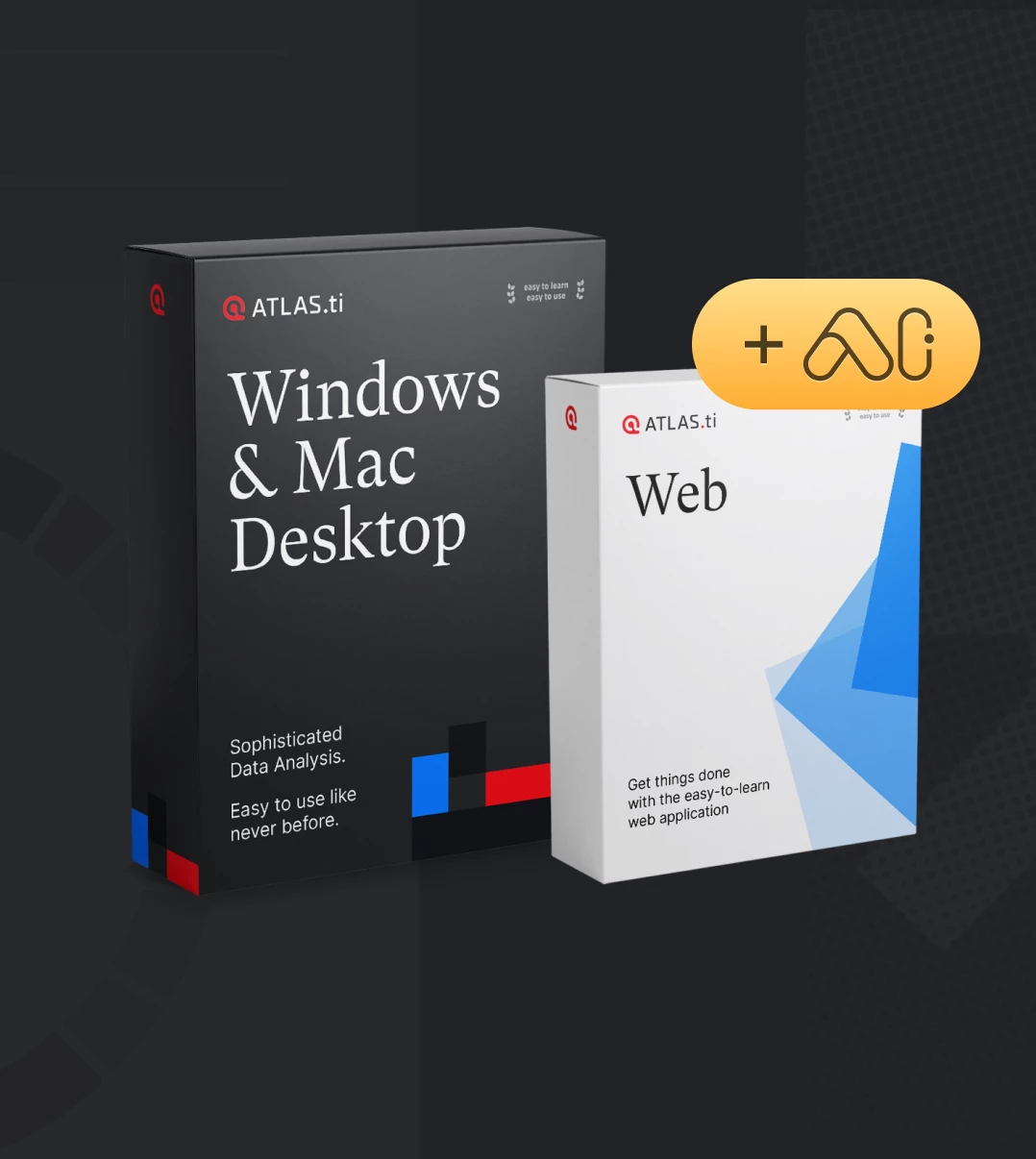
Develop powerful literature reviews with ATLAS.ti
Use our intuitive data analysis platform to make the most of your literature review. Get started with a free trial.


Systematic Reviews
Definition of a systematic review, key differences: a literature review or a systematic review, types of reviews.
- The PICO Framework
- Searching in Bibliographic Databases
- Grey Literature
- Developing a Protocol
- Reference Management
- PRESS - Checklist for Search Strategies
“A systematic review attempts to identify, appraise and synthesize all the empirical evidence that meets pre-specified eligibility criteria to answer a given research question. Researchers conducting systematic reviews use explicit methods aimed at minimizing bias…” - Cochrane Library
A systematic review uses robust methods to reduce bias in the gathering, summarizing, presenting, interpreting, and reporting of the research evidence. The key characteristics of a systematic review are:
- clearly stated objectives;
- pre-defined eligibility criteria;
- explicit, reproducible methodology;
- systematic search of the literature;
- assessment of validity of included studies;
- systematic synthesis and presentation of findings.
Cochrane Library. About Cochrane Reviews | Cochrane Library: https://www.cochranelibrary.com/about/about-cochrane-reviews
As systematic reviews summarise the results of all original studies within a given field, it is commonly regarded as high quality evidence. Referring to the hierarchy of evidence shown below, we can see that as the rigour of scientific method increases, we can be more confident of the reliability and robustness of the methodology used.
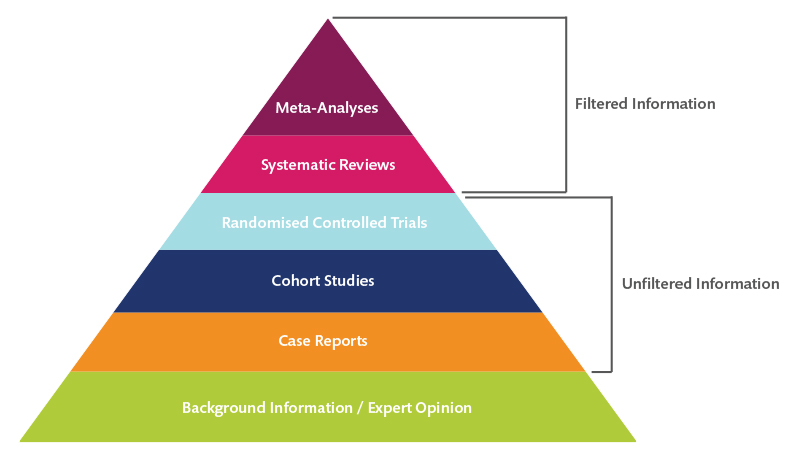
Karolinska Institutet University Library (2022). Systematic reviews [ Evidence Based Pyramid] : https://kib.ki.se/en/search-evaluate/systematic-reviews
There are four essential criteria for a systematic review:
- It should be exhaustive : all relevant literature in a research field should be included.
- A rigorous methodology must be followed throughout – from defining the research question, writing a protocol and searching the literature, to gathering, screening and analysing. The entire process should also be thoroughly documented.
- At least two people should be involved, particularly for screening articles and extracting data.
- Plenty of time resources are needed, but also in terms of availing yourself of others' expertise – for instance in database searching – and tools and software.
For a condensed overview, see the comparison below (from Jesson, Matheson & Lacey, 2011, p. 105 ).
To gain a broad understanding, and description of the field | Tightly specified aim and objectives with a specific review question | |
Big picture | Narrow focus | |
No defined path, allows for creativity and exploration | Transparent process and documented audit trail | |
Searching is probing, moving from one study to another, following up leads | Rigorous and comprehensive search for ALL studies | |
Purposive selection made by the reviewer | Predetermined criteria for including and excluding studies | |
Based on the reviewer's opinion | Checklists to assess the methodological quality of studies | |
Discursive | In tabular format and short summary answers | |
Not necessarily given | Must be presented for transparency |
Karolinska Institutet University Library (2022). Systematic reviews : https://kib.ki.se/en/search-evaluate/systematic-reviews
In an article from 2009, Grant & Booth described 14 review types, for example scoping reviews, and their associated methodologies.
Aims to demonstrate writer has extensively researched literature and critically evaluated its quality. Goes beyond mere description to include degree of analysis and conceptual innovation. Typically results in hypothesis or model. | Seeks to identify most significant items in the field. | No formal quality assessment. Attempts to evaluate according to contribution. | Typically narrative, perhaps conceptual or chronological. | Significant component: seeks to identify conceptual contribution to embody existing or derive new theory. | |
Generic term: published materials that provide examination of recent or current literature. Can cover wide range of subjects at various levels of completeness and comprehensiveness. May include research findings. | May or may not include comprehensive searching. | May or may not include quality assessment. | Typically narrative | Analysis may be chronological, conceptual, thematic, etc. | |
Map out and categorize existing literature from which to commission further reviews and/or primary research by identifying gaps in research literature. | Completeness of searching determined by time/scope constraints. | No formal quality assessment. | May be graphical and tabular. | Characterizes quantity and quality of literature, perhaps by study design and other key features. May identify need for primary or secondary research. | |
Technique that statistically combines the results of quantitative studies to provide a more precise effect of the results. | Aims for exhaustive, comprehensive searching. May use funnel plot to assess completeness. | Quality assessment may determine inclusion/exclusion and/or sensitivity analyses. | Graphical and tabular with narrative commentary. | Numerical analysis of measures of effect assuming absence of heterogeneity. | |
Refers to any combination of methods where one significant component is a literature review (usually systematic). Within a review context it refers to a combination of review approaches for example combining quantitative with qualitative research or outcome with process studies. | Requires either very sensitive search to retrieve all studies or separately conceived quantitative and qualitative strategies. | Requires either a generic appraisal instrument or separate appraisal processes with corresponding checklist. | Typically both components will be presented as narrative and in tables. May also employ graphical means of integrating quantitative and qualitative studies. | Analysis may characterise both literatures and look for correlations between characteristics or use gap analysis to identify aspects absent in one literature but missing in the other. | |
Generic term: summary of the [medical] literature that attempts to survey the literature and describe its characteristics. | May or may not include comprehensive searching (depends whether systematic overview or not) | May or may not include quality assessment (depends whether systematic overview or not) | Synthesis depends on whether systematic or not. Typically narrative but may include tabular features. | Analysis may be chronological, conceptual, thematic, etc. | |
Method for integrating or comparing the findings from qualitative studies. It looks for ‘themes’ or ‘constructs’ that lie in or across individual qualitative studies. | May employ selective or purposive sampling. | Quality assessment typically used to mediate messages not for inclusion/exclusion. | Qualitative, narrative synthesis. | Thematic analysis, may include conceptual models. | |
Assessment of what is already known about a policy or practice issue, by using systematic review methods to search and critically appraise existing research. | Completeness of searching determined by time constraints. | Time-limited formal quality assessment. | Typically narrative and tabular. | Quantities of literature and overall quality/direction of effect of literature. | |
Preliminary assessment of potential size and scope of available research literature. Aims to identify nature and extent of research evidence (usually including ongoing research). | Completeness of searching determined by time/scope constraints. May include research in progress. | No formal quality assessment. | Typically tabular with some narrative commentary. | Characterizes quantity and quality of literature, perhaps by study design and other key features. Attempts to specify a viable review. | |
Tend to address more current matters in contrast to other combined retrospective and current approaches. May offer new perspectives on issue or point out area for further research. | Aims for comprehensive searching of current literature. | No formal quality assessment. | Typically narrative, may have tabular accompaniment. | Current state of knowledge and priorities for future investigation and research. | |
Seeks to systematically search for, appraise and synthesis research evidence, often adhering to guidelines on the conduct of a review. | Aims for exhaustive, comprehensive searching. | Quality assessment may determine inclusion/exclusion. | Typically narrative with tabular accompaniment. | What is known; recommendations for practice. What remains unknown; uncertainty around findings, recommendations for future research. | |
Combines strengths of critical review with a comprehensive search process. Typically addresses broad questions to produce ‘best evidence synthesis’. | Aims for exhaustive, comprehensive searching. | May or may not include quality assessment. | Minimal narrative, tabular summary of studies. | What is known; recommendations for practice. Limitations. | |
Attempt to include elements of systematic review process while stopping short of systematic review. Typically conducted as postgraduate student assignment. | May or may not include comprehensive searching. | May or may not include quality assessment. | Typically narrative with tabular accompaniment. | What is known; uncertainty around findings; limitations of methodology. | |
Specifically refers to review compiling evidence from multiple reviews into one accessible and usable document. Focuses on broad condition or problem for which there are competing interventions and highlights reviews that address these interventions and their results. | Identification of component reviews, but no search for primary studies. | Quality assessment of studies within component reviews and/or of reviews themselves. | Graphical and tabular with narrative commentary. | What is known; recommendations for practice. What remains unknown; recommendations for future research. |
Grant MJ, Booth A. [ Table 1 - Main review types characterized by methods used]. A typology of reviews: an analysis of 14 review types and associated methodologies. Health Info Libr J. 2009 Jun;26(2):91-108. doi: 10.1111/j.1471-1842.2009.00848.x. PMID: 19490148. .: https://pubmed.ncbi.nlm.nih.gov/19490148/
Further Reading :
Munn, Z., Stern, C., Aromataris, E., Lockwood, C., & Jordan, Z. (2018). What kind of systematic review should I conduct? A proposed typology and guidance for systematic reviewers in the medical and health sciences. BMC Medical Research Methodology, 18 (1)5. (Open Access) https://bmcmedresmethodol.biomedcentral.com/articles/10.1186/s12874-017-0468-4
Higgins JPT, Thomas J, Chandler J, Cumpston M, Li T, Page MJ, Welch VA (editors). Cochrane Handbook for Systematic Reviews of Interventions version 6.3 (updated February 2022). Cochrane, 2022. Available from www.training.cochrane.org/handbook.
The Campbell Collaboration. Campbell systematic reviews: Policies and guidelines https://doi.org/10.4073/cpg.2016.1.
- << Previous: Home
- Next: Systematic Search Techniques >>
- Last Updated: Jun 28, 2024 12:51 PM
- URL: https://libguides.ntu.edu.sg/systematic-reviews
You are expected to comply with University policies and guidelines namely, Appropriate Use of Information Resources Policy , IT Usage Policy and Social Media Policy . Users will be personally liable for any infringement of Copyright and Licensing laws. Unless otherwise stated, all guide content is licensed by CC BY-NC 4.0 .
info This is a space for the teal alert bar.
notifications This is a space for the yellow alert bar.

- National University
Ask Us! National University Library
- NCU Office of the Registrar
- NU Library (formerly NCU)
- 1 ADA Accomodations and Services
- 1 AMA Style
- 1 APA Style
- 4 Brightspace Help
- 33 Business Research
- 2 Citation Styles
- 1 Consultations
- 14 Database Help
- 20 Database Problems
- 30 Database Questions
- 4 Database Subscriptions
- 2 Delivery Services
- 1 Dictionaries &
- 5 Dissertation Center Documents
- 34 Dissertation Research
- 6 Dissertations and Theses
- 5 Ebook Central Questions
- 22 Education Research
- 1 Encyclopedias
- 4 Evaluating Information
- 1 Faculty Textbooks
- 1 Institutional Review Board (IRB)
- 13 Interlibrary Loan
- 11 Journal Articles
- 11 Legal Research
- 20 Library Access
- 2 Library Collection Development
- 1 Library Contact Details
- 1 Library Guides
- 1 Library Hours and Holidays
- 1 Library Location
- 1 Library Membership
- 2 Library Policies
- 1 Library Services
- 1 Library Workshops
- 1 Literary Research
- 2 Literature Review Help
- 36 Locate items in the Library
- 21 Locate Items Outside the Library
- 7 Login Help
- 12 Marriage & Family Therapy Research
- 1 Music Research
- 1 Newspaper Articles
- 7 Nursing and Healthcare Research
- 2 Off-Site Access
- 22 OpenAthens
- 9 Organizing Research and Citations
- 3 Plagiarism and Academic Integrity
- 2 Psychology and Counseling Research
- 14 Psychology Research
- 1 Publications
- 25 Reference Management
- 24 RefWorks
- 1 Research Assistance
- 2 Research Consultations
- 6 Research Methods
- 66 Research Techniques
- 4 Service Desk
- 6 Software and Apps
- 1 Statistics
- 4 Technical Assistance
- 13 Technical Issues
- 5 Test Preparation
- 2 Testing Services
- 4 Tests & Measurements
- 7 Textbooks
- 3 Tutoring Services
- 1 Writing Assistance
- 1 Writing Center
NU Library Chat
Can't find what you need? Submit a question and we'll get back to you as soon as possible.
What's the difference between a meta-analysis, systematic review, and literature review?
The difference between literature review and systematic review comes back to the initial research question. Whereas the systematic review is very specific and focused , the standard literature review is much more general . The components of a literature review, for example, are similar to any other research paper. Meanwhile, whereas a systematic review can include several research studies to answer a specific question, typically a meta analysis includes a comparison of different studies to suss out any inconsistencies or discrepancies.
- Elsevier
Learn more:
- NU Library Guide to Systematic Reviews & Meta-Analyses
- Systematic Literature Review or Literature Review | Elsevier. (2022, March 18). Elsevier Author Services - Articles .
- Systematic Review VS Meta-Analysis | Elsevier Blog. (2020, April 8). Elsevier Author Services - Articles.
- SAGE Research Methods has several ebooks and video tutorials on systematic or literature reviews.
- Cochrane Library includes a large collection of systematic reviews on physical and mental health topics.
- Many health research databases, including CINAHL or PubMed , have the option to restrict your results to systematic reviews only. Look for a "methodology" or "article type" option to the left of your results list.
Still need help?
Didn't get the answer you needed? Contact a librarian through email, phone, SMS, or chat for personalized assistance!

© Copyright 2024 National University. All Rights Reserved.
Privacy Policy | Consumer Information
Principles of Meta-Analysis
- First Online: 11 August 2022
Cite this chapter
- Rob Dekkers 4 ,
- Lindsey Carey 5 &
- Peter Langhorne 6
1969 Accesses
Meta-analysis is a common feature of quantitative synthesis for systematic reviews, one of the four archetypes in this book.
This is a preview of subscription content, log in via an institution to check access.
Access this chapter
Subscribe and save.
- Get 10 units per month
- Download Article/Chapter or eBook
- 1 Unit = 1 Article or 1 Chapter
- Cancel anytime
- Available as PDF
- Read on any device
- Instant download
- Own it forever
- Available as EPUB and PDF
- Compact, lightweight edition
- Dispatched in 3 to 5 business days
- Free shipping worldwide - see info
- Durable hardcover edition
Tax calculation will be finalised at checkout
Purchases are for personal use only
Institutional subscriptions
By some the replication continuum is attributed to the work of Lipsey and Wilson ( 1993 ). However, there is no mention of it. Also, the statement ‘the closer to pure replications your collection of studies, the easier it is to argue comparability’ does not appear in the text of Lipsey and Wilson nor can it be interpreted as a paraphrased statement. This means caution is required when looking for the origins of the replication continuum.
Hendrick ( 1990 ) refers to a working paper written by him in 1974 about the dichotomy ‘strict replication’ and ‘conceptual replication.’
The term Simpson’s paradox was introduced by Blyth ( 1972 ), inspired by Simpson ( 1951 ). However, notions by Pearson et al. ( 1899 , p. 278) and Yule ( 1903 , pp. 132–4) about combining data seem to predate Simpson ( 1951 ).
The other two of the three preceding systematic reviews with meta-analysis were dated fifteen years before this systematic review using the odds ratio for conducting the meta-analysis.
The authors do not use the term ‘grey literature’, which is introduced here for consistency of terminology in the book.
See Section 3.3 for more detail on ontology in the context of research paradigms.
See Dickersin ( 1990 , pp. 1385–1386) for some historical notes with regard to publication bias.
Aguinis H, Pierce CA, Bosco FA, Dalton DR, Dalton CM (2011) Debunking myths and urban legends about meta-analysis. Organ Res Methods 14(2):306–331. https://doi.org/10.1177/1094428110375720
Article Google Scholar
Allen M, Preiss R (1993) Replication and meta-analysis: a necessary connection. J Soc Behav Pers 8(6):9–20
Google Scholar
Animasaun IL, Ibraheem RO, Mahanthesh B, Babatunde HA (2019) A meta-analysis on the effects of haphazard motion of tiny/nano-sized particles on the dynamics and other physical properties of some fluids. Chin J Phys 60:676–687. https://doi.org/10.1016/j.cjph.2019.06.007
Anzures-Cabrera J, Higgins JPT (2010) Graphical displays for meta-analysis: an overview with suggestions for practice. Res Synth Methods 1(1):66–80. https://doi.org/10.1002/jrsm.6
Bakbergenuly I, Hoaglin DC, Kulinskaya E (2019) Pitfalls of using the risk ratio in meta-analysis. Res Synth Methods 10(3):398–419. https://doi.org/10.1002/jrsm.1347
Bakbergenuly I, Kulinskaya E (2017) Beta-binomial model for meta-analysis of odds ratios. Stat Med 36(11):1715–1734. https://doi.org/10.1002/sim.7233
Baker WL, Michael White C,Cappelleri JC, Kluger J, Coleman CI, From the Health Outcomes P, Group EC (2009) Understanding heterogeneity in meta‐analysis: the role of meta‐regression. Int J Clin Pract 63(10):1426–1434. https://doi.org/10.1111/j.1742-1241.2009.02168.x
Bax L, Ikeda N, Fukui N, Yaju Y, Tsuruta H, Moons KGM (2008) More than numbers: the power of graphs in meta-analysis. Am J Epidemiol 169(2):249–255. https://doi.org/10.1093/aje/kwn340
Bax L, Yu L-M, Ikeda N, Moons KGM (2007) A systematic comparison of software dedicated to meta-analysis of causal studies. BMC Med Res Methodol 7(1):40. https://doi.org/10.1186/1471-2288-7-40
Bender R, Bunce C, Clarke M, Gates S, Lange S, Pace NL, Thorlund K (2008) Attention should be given to multiplicity issues in systematic reviews. J Clin Epidemiol 61(9):857–865. https://doi.org/10.1016/j.jclinepi.2008.03.004
Bennett DA, Latham NK, Stretton C, Anderson CS (2004) Capture-recapture is a potentially useful method for assessing publication bias. J Clin Epidemiol 57(4):349–357. https://doi.org/10.1016/j.jclinepi.2003.09.015
Biggerstaff BJ, Tweedie RL (1997) Incorporating variability in estimates of heterogeneity in the random effects model in meta-analysis. Stat Med 16(7):753–768. https://doi.org/10.1002/(SICI)1097-0258(19970415)16:7<753::AID-SIM494>3.0.CO;2-G
Blyth CR (1972) On Simpson’s paradox and the sure-thing principle. J Am Stat Assoc 67(338):364–366. https://doi.org/10.1080/01621459.1972.10482387
Borenstein M, Hedges LV, Higgins JPT, Rothstein HR (2010) A basic introduction to fixed-effect and random-effects models for meta-analysis. Res Synth Methods 1(2):97–111. https://doi.org/10.1002/jrsm.12
Bravata DM, Olkin I (2001) Simple pooling versus combining in meta-analysis. Eval Health Prof 24(2):218–230. https://doi.org/10.1177/01632780122034885
Brown SA, Upchurch SL, Acton GJ (2003) A framework for developing a coding scheme for meta-analysis. West J Nurs Res 25(2):205–222. https://doi.org/10.1177/0193945902250038
Cheung MW-L, Ho RCM, Lim Y, Mak A (2012) Conducting a meta-analysis: basics and good practices. Int J Rheum Dis 15(2):129–135. https://doi.org/10.1111/j.1756-185X.2012.01712.x
Chiolero A, Santschi V, Burnand B, Platt RW, Paradis G (2012) Meta-analyses: with confidence or prediction intervals? Eur J Epidemiol 27(10):823–825. https://doi.org/10.1007/s10654-012-9738-y
Chootrakool H, Shi JQ, Yue R (2011) Meta-analysis and sensitivity analysis for multi-arm trials with selection bias. Stat Med 30(11):1183–1198. https://doi.org/10.1002/sim.4143
Chow SL (1987) Meta-analysis of pragmatic and theoretical research: a critique. J Psychol 121(3):259–271. https://doi.org/10.1080/00223980.1987.9712666
Copas JB (2013) A likelihood-based sensitivity analysis for publication bias in meta-analysis. J Roy Stat Soc Ser C (Appl Stat) 62(1):47–66. https://doi.org/10.1111/j.1467-9876.2012.01049.x
Copas J, Shi JQ (2000) Meta-analysis, funnel plots and sensitivity analysis. Biostatistics 1(3):247–262. https://doi.org/10.1093/biostatistics/1.3.247
Copas JB, Shi JQ (2001) A sensitivity analysis for publication bias in systematic reviews. Stat Methods Med Res 10(4):251–265. https://doi.org/10.1177/096228020101000402
Cortoni F, Babchishin KM, Rat C (2017) The proportion of sexual offenders who are female is higher than thought: a meta-analysis. Crim Justice Behav 44(2):145–162. https://doi.org/10.1177/0093854816658923
Dalton JE, Bolen SD, Mascha EJ (2016) Publication bias: the elephant in the review. Anesth Analg 123(4):812–813. https://doi.org/10.1213/ane.0000000000001596
De Wolff MS, van Ijzendoorn MH (1997) Sensitivity and attachment: a meta-analysis on parental antecedents of infant attachment. Child Dev 68(4):571–591. https://doi.org/10.1111/j.1467-8624.1997.tb04218.x
Deeks JJ, Higgins JPT, Altman DG (2021) Analysing data and undertaking meta-analyses. In: Higgins JP, Thomas J, Chandler J, Cumpston M, Li T, Page MJ, Welch VA (eds) Cochrane handbook for systematic reviews of interventions (Version 6.2 ed): cochrane. https://training.cochrane.org/handbook/current/chapter-10
DerSimonian R, Laird N (1986) Meta-analysis in clinical trials. Control Clin Trials 7(3):177–188. https://doi.org/10.1016/0197-2456(86)90046-2
Dickersin K (1990) The existence of publication bias and risk factors for its occurrence. JAMA 263(10):1385–1389. https://doi.org/10.1001/jama.1990.03440100097014
Doucouliagos H, Ulubaşoğlu MA (2008) Democracy and economic growth: a meta-analysis. Am J Polit Sci 52(1):61–83. https://doi.org/10.1111/j.1540-5907.2007.00299.x
Duval S, Tweedie R (2000) Trim and fill: a simple funnel-plot–based method of testing and adjusting for publication bias in meta-analysis. Biometrics 56(2):455–463. https://doi.org/10.1111/j.0006-341X.2000.00455.x
Egger M, Smith GD, Phillips AN (1997) Meta-analysis: principles and procedures. BMJ 315(7121):1533–1537. https://doi.org/10.1136/bmj.315.7121.1533
Egger M, Smith GD, Schneider M, Minder C (1997) Bias in meta-analysis detected by a simple, graphical test. BMJ 315(7109):629–634. https://doi.org/10.1136/bmj.315.7109.629
Elvik R (2005) Can we trust the results of meta-analyses?: a systematic approach to sensitivity analysis in meta-analyses. Transp Res Rec 1908(1):221–229. https://doi.org/10.1177/0361198105190800127
Ewing R, Cervero R (2010) Travel and the built environment. J Am Plan Assoc 76(3):265–294. https://doi.org/10.1080/01944361003766766
Franco A, Malhotra N, Simonovits G (2014) Publication bias in the social sciences: unlocking the file drawer. Science 345(6203):1502–1505. https://doi.org/10.1126/science.1255484
Galbraith RF (1988) A note on graphical presentation of estimated odds ratios from several clinical trials. Stat Med 7(8):889–894. https://doi.org/10.1002/sim.4780070807
Glass GV (1976) Primary, secondary, and meta-analysis of research. Educ Res 5(10):3–8. https://doi.org/10.3102/0013189X005010003
Göritz AS (2006) Incentives in web studies: methodological issues and a review. Int J Internet Sci 1(1):58–70
Gøtzsche PC, Hróbjartsson A, Marić K, Tendal B (2007) Data extraction errors in meta-analyses that use standardized mean differences. JAMA 298(4):430–437. https://doi.org/10.1001/jama.298.4.430
Govindan K, Rajeev A, Padhi SS, Pati RK (2020) Supply chain sustainability and performance of firms: a meta-analysis of the literature. Transp Res Part E Logist Transp Rev 137:101923. https://doi.org/10.1016/j.tre.2020.101923
Guzzo RA, Jackson SE, Katzell RA (1987) Meta-analysis analysis. Res Organ Behav 9:407–442
Hartung J, Knapp G (2001) A refined method for the meta-analysis of controlled clinical trials with binary outcome. Stat Med 20(24):3875–3889. https://doi.org/10.1002/sim.1009
Hendrick C (1990) Replications, strict replications, and conceptual replications: are they important? J Soc Behav Personal 5(4):41–49
Higgins JPT, Thompson SG (2002) Quantifying heterogeneity in a meta-analysis. Stat Med 21(11):1539–1558. https://doi.org/10.1002/sim.1186
Higgins JPT, Thompson SG, Deeks JJ, Altman DG (2003) Measuring inconsistency in meta-analyses. BMJ 327(7414):557–560. https://doi.org/10.1136/bmj.327.7414.557
Hoobler JM, Masterson CR, Nkomo SM, Michel EJ (2018) The business case for women leaders: meta-analysis, research critique, and path forward. J Manag 44(6):2473–2499. https://doi.org/10.1177/0149206316628643
Hook EB, Regal RR (1995) Capture-recapture methods in epidemiology: methods and limitations. Epidemiol Rev 17(2):243–264. https://doi.org/10.1093/oxfordjournals.epirev.a036192
Howard GS, Maxwell SE (1980) Correlation between student satisfaction and grades: a case of mistaken causation? J Educ Psychol 72(6):810–820. https://doi.org/10.1037/0022-0663.72.6.810
IntHout J, Ioannidis JPA, Borm GF (2014) The Hartung-Knapp-Sidik-Jonkman method for random effects meta-analysis is straightforward and considerably outperforms the standard DerSimonian-Laird method. BMC Med Res Methodol 14(1):25. https://doi.org/10.1186/1471-2288-14-25
Itani O, Jike M, Watanabe N, Kaneita Y (2017) Short sleep duration and health outcomes: a systematic review, meta-analysis, and meta-regression. Sleep Med 32:246–256. https://doi.org/10.1016/j.sleep.2016.08.006
Kaufmann E, Reips U-D, Maag Merki K (2016) Avoiding methodological biases in meta-analysis. Zeitschrift Für Psychologie 224(3):157–167. https://doi.org/10.1027/2151-2604/a000251
Kim KH (2005) Can only intelligent people be creative? A meta-analysis. J Second Gift Educ 16(2–3):57–66. https://doi.org/10.4219/jsge-2005-473
Kontopantelis E, Reeves D (2012) Performance of statistical methods for meta-analysis when true study effects are non-normally distributed: a simulation study. Stat Methods Med Res 21(4):409–426. https://doi.org/10.1177/0962280210392008
Kontopantelis E, Reeves D (2012) Performance of statistical methods for meta-analysis when true study effects are non-normally distributed: a comparison between DerSimonian–Laird and restricted maximum likelihood. Stat Methods Med Res 21(6):657–659. https://doi.org/10.1177/0962280211413451
L’Abbé KA, Detsky AS, O’Rourke K (1987) Meta-analysis in clinical research. Ann Intern Med 107(2):224–233. https://doi.org/10.7326/0003-4819-107-2-224
Lajeunesse MJ (2016) Facilitating systematic reviews, data extraction and meta-analysis with the metagear package for R. Methods Ecol Evol 7(3):323–330. https://doi.org/10.1111/2041-210X.12472
Lakens D (2013) Calculating and reporting effect sizes to facilitate cumulative science: a practical primer for t-tests and ANOVAs. Front Psychol 4(863). https://doi.org/10.3389/fpsyg.2013.00863
Lipsey MW, Wilson DB (1993) The efficacy of psychological, educational, and behavioral treatment: confirmation from meta-analysis. Am Psychol 48(12):1181–1209. https://doi.org/10.1037/0003-066X.48.12.1181
Lloyd S, Schmidt U, Khondoker M, Tchanturia K (2015) Can psychological interventions reduce perfectionism? A systematic review and meta-analysis. Behav Cogn Psychother 43(6):705–731. https://doi.org/10.1017/S1352465814000162
Lopes JSS, Machado AF, Cavina AP, Kirsch Michelletti J, Castilho de Almeida A, Pastre CM (2019) Specific interventions for prevention of muscle injury in lower limbs: systematic review and meta-analysis. Fisioterapia Movimento 32:e003224. https://doi.org/10.1590/1980-5918.032.AO24
López-López JA, Page MJ, Lipsey MW, Higgins JPT (2018) Dealing with effect size multiplicity in systematic reviews and meta-analyses. Res Synth Methods 9(3):336–351. https://doi.org/10.1002/jrsm.1310
Macaskill P, Walter SD, Irwig L (2001) A comparison of methods to detect publication bias in meta-analysis. Stat Med 20(4):641–654. https://doi.org/10.1002/sim.698
Mathes T, Kuss O (2018) A comparison of methods for meta-analysis of a small number of studies with binary outcomes. Res Synth Methods 9(3):366–381. https://doi.org/10.1002/jrsm.1296
Mantel N, Haenszel W (1959) Statistical aspects of the analysis of data from retrospective studies of disease. JNCI J Nat Cancer Inst 22(4):719–748. https://doi.org/10.1093/jnci/22.4.719
Mavros MN, Alexiou VG, Vardakas KZ, Falagas ME (2013) Understanding of statistical terms routinely used in meta-analyses: an international survey among researchers. PLoS One 8(1):e47229. https://doi.org/10.1371/journal.pone.0047229
McDaniel MA, Rothstein HR, Whetzel DL (2006) Publication bias: a case study of four test vendors. Pers Psychol 59(4):927–953. https://doi.org/10.1111/j.1744-6570.2006.00059.x
McKenzie JE, Beller EM, Forbes AB (2016) Introduction to systematic reviews and meta-analysis. Respirology 21(4):626–637. https://doi.org/10.1111/resp.12783
McShane BB, Böckenholt U (2017) Single-paper meta-analysis: benefits for study summary, theory testing, and replicability. J Consum Res 43(6):1048–1063. https://doi.org/10.1093/jcr/ucw085
Munn Z, Tufanaru C, Aromataris E (2014) JBI’s systematic reviews: data extraction and synthesis. AJN Am J Nurs 114(7):49–54. https://doi.org/10.1097/01.Naj.0000451683.66447.89
Nakagawa S, Noble DWA, Senior AM, Lagisz M (2017) Meta-evaluation of meta-analysis: ten appraisal questions for biologists. BMC Biol 15(1):18. https://doi.org/10.1186/s12915-017-0357-7
Neyeloff JL, Fuchs SC, Moreira LB (2012) Meta-analyses and forest plots using a microsoft excel spreadsheet: step-by-step guide focusing on descriptive data analysis. BMC Res Notes 5(1):52. https://doi.org/10.1186/1756-0500-5-52
O’Keefe DJ, Hale SL (2001) An odds-ratio-based meta-analysis of research on the door-in-the-face influence strategy. Commun Rep 14(1):31–38. https://doi.org/10.1080/08934210109367734
Pastor DA, Lazowski RA (2018) On the multilevel nature of meta-analysis: a tutorial, comparison of software programs, and discussion of analytic choices. Multivar Behav Res 53(1):74–89. https://doi.org/10.1080/00273171.2017.1365684
Pearson K, Lee A, Bramley-Moore L (1899) VI. Mathematical contributions to the theory of evolution–VI. Genetic (Reproductive) selection: inheritance of fertility in man, and of fecundity in thoroughbred racehorses. Philos Trans R Soc Lond 192:257–330. https://doi.org/10.1098/rsta.1899.0006
Pedder H, Sarri G, Keeney E, Nunes V, Dias S (2016) Data extraction for complex meta-analysis (DECiMAL) guide. Syst Rev 5(1):212. https://doi.org/10.1186/s13643-016-0368-4
Philibert A, Loyce C, Makowski D (2012) Assessment of the quality of meta-analysis in agronomy. Agr Ecosyst Environ 148:72–82. https://doi.org/10.1016/j.agee.2011.12.003
Pigott TD, Polanin JR (2020) Methodological guidance paper: high-quality meta-analysis in a systematic review. Rev Educ Res 90(1):24–46. https://doi.org/10.3102/0034654319877153
Polák P (2017) The productivity paradox: a meta-analysis. Inf Econ Policy 38:38–54. https://doi.org/10.1016/j.infoecopol.2016.11.003
Poorolajal J, Haghdoost AA, Mahmoodi M, Majdzadeh R, Nasseri-Moghaddam S, Fotouhi A (2010) Capture-recapture method for assessing publication bias. J Res Med Sci 15(2):107–115
Rice K, Higgins JPT, Lumley T (2018) A re-evaluation of fixed effect(s) meta-analysis. J R Stat Soc A Stat Soc 181(1):205–227. https://doi.org/10.1111/rssa.12275
Rosenthal R (1979) The “File Drawer Problem” and tolerance for null results. Psychol Bull 86(3):638–641. https://doi.org/10.1037/0033-2909.86.3.638
Russo MW (2007) How to review a meta-analysis. Gastroenterol Hepatol 3(8):637–642
Schmid EJ, Koch GG, LaVange LM (1991) An overview of statistical issues and methods of meta-analysis. J Biopharm Stat 1(1):103–120. https://doi.org/10.1080/10543409108835008
Schmidt FL (2017) Statistical and measurement pitfalls in the use of meta-regression in meta-analysis. Career Dev Int 22(5):469–476. https://doi.org/10.1108/CDI-08-2017-0136
Schmidt FL, Oh I-S, Hayes TL (2009) Fixed-versus random-effects models in meta-analysis: model properties and an empirical comparison of differences in results. Br J Math Stat Psychol 62(1):97–128. https://doi.org/10.1348/000711007X255327
Sera F, Armstrong B, Blangiardo M, Gasparrini A (2019) An extended mixed-effects framework for meta-analysis. Stat Med 38(29):5429–5444. https://doi.org/10.1002/sim.8362
Shah SA, Sander S, White CM, Rinaldi M, Coleman CI (2007) Evaluation of echinacea for the prevention and treatment of the common cold: a meta-analysis. Lancet Infect Dis 7(7):473–480. https://doi.org/10.1016/S1473-3099(07)70160-3
Sidik K, Jonkman JN (2006) Robust variance estimation for random effects meta-analysis. Comput Stat Data Anal 50(12):3681–3701. https://doi.org/10.1016/j.csda.2005.07.019
Song F, Sheldon TA, Sutton AJ, Abrams KR, Jones DR (2001) Methods for exploring heterogeneity in meta-analysis. Eval Health Prof 24(2):126–151. https://doi.org/10.1177/016327870102400203
Simpson EH (1951) The interpretation of interaction in contingency tables. J Roy Stat Soc Ser B (Methodol) 13(2):238–241. https://doi.org/10.1111/j.2517-6161.1951.tb00088.x
Stanley TD (2001) Wheat from chaff: meta-analysis as quantitative literature review. J Econ Perspect 15(3):131–150. https://doi.org/10.1257/jep.15.3.131
Stanley TD, Doucouliagos H (2015) Neither fixed nor random: weighted least squares meta-analysis. Stat Med 34(13):2116–2127. https://doi.org/10.1002/sim.6481
Stanley TD, Doucouliagos H, Giles M, Heckemeyer JH, Johnston RJ, Laroche P, Nelson JP, Paldam M, Poot J, Pugh G, Rosenberger RS, Rost K (2013) Meta-analysis of economics research reporting guidelines. J Econ Surv 27(2):390–394. https://doi.org/10.1111/joes.12008
Stanley TD, Jarrell SB (2005) Meta-regression analysis: a quantitative method of literature surveys. J Econ Surv 19(3):299–308. https://doi.org/10.1111/j.0950-0804.2005.00249.x
Sutton AJ, Abrams KR (2001) Bayesian methods in meta-analysis and evidence synthesis. Stat Methods Med Res 10(4):277–303. https://doi.org/10.1177/096228020101000404
Sutton AJ, Higgins JPT (2008) Recent developments in meta-analysis. Stat Med 27(5):625–650. https://doi.org/10.1002/sim.2934
Suurmond R, van Rhee H, Hak T (2017) Introduction, comparison, and validation of Meta-Essentials: a free and simple tool for meta-analysis. Res Synth Methods 8(4):537–553. https://doi.org/10.1002/jrsm.1260
Takeshima N, Sozu T, Tajika A, Ogawa Y, Hayasaka Y, Furukawa TA (2014) Which is more generalizable, powerful and interpretable in meta-analyses, mean difference or standardized mean difference? BMC Med Res Methodol 14(1):30. https://doi.org/10.1186/1471-2288-14-30
Tang S-H, Hall VC (1995) The overjustification effect: a meta-analysis. Appl Cogn Psychol 9(5):365–404. https://doi.org/10.1002/acp.2350090502
Tendal B, Nüesch E, Higgins JPT, Jüni P, Gøtzsche PC (2011) Multiplicity of data in trial reports and the reliability of meta-analyses: empirical study. BMJ 343:d4829. https://doi.org/10.1136/bmj.d4829
Terrin N, Schmid CH, Lau J, Olkin I (2003) Adjusting for publication bias in the presence of heterogeneity. Stat Med 22(13):2113–2126. https://doi.org/10.1002/sim.1461
Thompson SG, Higgins JPT (2002) How should meta-regression analyses be undertaken and interpreted? Stat Med 21(11):1559–1573. https://doi.org/10.1002/sim.1187
Tipton E, Pustejovsky JE, Ahmadi H (2019) A history of meta-regression: technical, conceptual, and practical developments between 1974 and 2018. Res Synth Methods 10(2):161–179. https://doi.org/10.1002/jrsm.1338
Uttl B, White CA, Gonzalez DW (2017) Meta-analysis of faculty’s teaching effectiveness: student evaluation of teaching ratings and student learning are not related. Stud Educ Eval 54:22–42. https://doi.org/10.1016/j.stueduc.2016.08.007
van Houwelingen HC, Arends LR, Stijnen T (2002) Advanced methods in meta-analysis: multivariate approach and meta-regression. Stat Med 21(4):589–624. https://doi.org/10.1002/sim.1040
Verhaeghen P (2003) Aging and vocabulary score: a meta-analysis. Psychol Aging 18(2):332–339. https://doi.org/10.1037/0882-7974.18.2.332
Veroniki AA, Jackson D, Bender R, Kuss O, Langan D, Higgins JPT, Knapp G, Salanti G (2019) Methods to calculate uncertainty in the estimated overall effect size from a random-effects meta-analysis. Res Synth Methods 10(1):23–43. https://doi.org/10.1002/jrsm.1319
Viechtbauer W (2007) Confidence intervals for the amount of heterogeneity in meta-analysis. Stat Med 26(1):37–52. https://doi.org/10.1002/sim.2514
Walker HM (1940) Degrees of freedom. J Educ Psychol 31(4):253–269. https://doi.org/10.1037/h0054588
Wanous JP, Sullivan SE, Malinak J (1989) The role of judgment calls in meta-analysis. J Appl Psychol 74(2):259–264. https://doi.org/10.1037/0021-9010.74.2.259
Woodward ND, Purdon SE, Meltzer HY, Zald DH (2005) A meta-analysis of neuropsychological change to clozapine, olanzapine, quetiapine, and risperidone in schizophrenia. Int J Neuropsychopharmacol 8(3):457–472. https://doi.org/10.1017/s146114570500516x
Yule GU (1903) Notes on the Theory of Association of Attributes in Statistics. Biometrika 2(2):121–134. https://doi.org/10.2307/2331677
Yusuf S, Peto R, Lewis J, Collins R, Sleight P (1985) Beta blockade during and after myocardial infarction: an overview of the randomized trials. Prog Cardiovasc Dis 27(5):335–371. https://doi.org/10.1016/S0033-0620(85)80003-7
Zeng Y, Luo T, Xie H, Huang M, Cheng ASK (2014) Health benefits of qigong or tai chi for cancer patients: a systematic review and meta-analyses. Complement Ther Med 22(1):173–186. https://doi.org/10.1016/j.ctim.2013.11.010
Download references
Author information
Authors and affiliations.
University of Glasgow, Glasgow, UK
Rob Dekkers
Glasgow Caledonian University, Glasgow, UK
Lindsey Carey
Peter Langhorne
You can also search for this author in PubMed Google Scholar
Corresponding author
Correspondence to Rob Dekkers .
Rights and permissions
Reprints and permissions
Copyright information
© 2022 Springer Nature Switzerland AG
About this chapter
Dekkers, R., Carey, L., Langhorne, P. (2022). Principles of Meta-Analysis. In: Making Literature Reviews Work: A Multidisciplinary Guide to Systematic Approaches. Springer, Cham. https://doi.org/10.1007/978-3-030-90025-0_7
Download citation
DOI : https://doi.org/10.1007/978-3-030-90025-0_7
Published : 11 August 2022
Publisher Name : Springer, Cham
Print ISBN : 978-3-030-90024-3
Online ISBN : 978-3-030-90025-0
eBook Packages : Education Education (R0)
Share this chapter
Anyone you share the following link with will be able to read this content:
Sorry, a shareable link is not currently available for this article.
Provided by the Springer Nature SharedIt content-sharing initiative
- Publish with us
Policies and ethics
- Find a journal
- Track your research

Systematic Reviews
- The Research Question
- Inclusion and Exclusion Criteria
- Original Studies
- Translating
- Deduplication
- Project Management Tools
- Useful Resources
- What is not a systematic review?
Typology of Reviews
There are other types of reviews, and some are often mistaken for systematic reviews. Some may even call themselves 'systematic reviews.' However, understanding the scope of other reviews and methods can help one distinguish between them and a systematic review proper. Here are some common review types:
- Technique that statistically combines the results of quantitative studies to provide a more precise effect of the results. May be a component of a systematic review.
- Generic term: published materials that provide examination of recent or current literature. Can cover a wide range of subjects at various levels of completeness and comprehensiveness. May include research findings.
- Preliminary assessment of potential size and scope of available research literature. Aims to identify nature and extent of research evidence (usually including ongoing research).
- Assessment of what is already known about a policy or practice issue, by using systematic review methods to search and critically appraise existing research.
- Specifically refers to reviews compiling evidence from multiple reviews into one accessible and usable document. Focuses on broad condition or problem for which there are competing interventions and highlights reviews that address these interventions and their results.
- Attempts to include elements of the systematic review process while stopping short of a systematic review. Typically conducted as a postgraduate student assignment.
The above definitions are taken from A typology of reviews: an analysis of 14 review types and associated methodologies. The document is listed below.
- A typology of reviews: an analysis of 14 review types and associated methodologies
- Additional Resource: "Meeting the review family: exploring review types and associated information retrieval requirements" more... less... Sutton A, Clowes M, Preston L, Booth A. Meeting the review family: exploring review types and associated information retrieval requirements. Health Info Libr J. 2019 Sep;36(3):202-222. doi: 10.1111/hir.12276. PMID: 31541534.
Meta-Analysis
Meta-analysis is the use of statistical methods to summarise the results of independent studies. By combining information from all relevant studies, meta-analyses can provide more precise estimates of the effects of health care than those derived from the individual studies included within a review. Meta-analyses also facilitate investigations of the consistency of evidence across studies, and the exploration of differences across studies ( Cochrane Handbook, 1.2.2 ). More information on meta-analyses can be found in Cochrane Handbook, Chapter 9 .
A meta-analysis goes beyond critique and integration and conducts secondary statistical analyses on the outcomes of similar studies. Systematic reviews may use quantitative methods to synthesize and summarize the results.
An advantage of a meta-analysis is the ability to be completely objective in evaluating research findings. Not all topics, however, have sufficient research evidence to allow a meta-analysis to be conducted. In that case, an integrative review is an appropriate strategy.
Literature Reviews
Literatures reviews focus on the existing literature of a subject. They lack the rigorous systematic methodology of systematic reviews. They rarely conduct exhaustive search strategies and do not publish the search strategy (although there are exceptions due to the general nature of literature reviews.) Literature reviews may examine the literature that is the most commonly cited within a certain time frame. Synthesis according to some criteria is typically employed. Literature reviews can take many forms: theses, dissertations, a component within a research paper, or lab report. Please see the University of North Carolina at Chapel Hill's information on literature reviews here.
Scoping Review or (Mapping Review)
In general, scoping reviews are commonly used for ‘reconnaissance’ – to clarify working definitions and conceptual boundaries of a topic or field. Scoping reviews are useful for when a body of literature has not yet been comprehensively reviewed, or exhibits a complex or heterogeneous nature not amenable to a more precise systematic review of the evidence. While scoping reviews may be conducted to determine the value and probable scope of a full systematic review, they may also be undertaken as exercises in and of themselves to summarize and disseminate research findings, to identify research gaps, and to make recommendations for future research.
From Peters, MD, Godfrey, CM, Khalil , H, McInerney, P, Parker, D & Soares , CB 2015, ' Guidance for conducting systematic scoping reviews', International Journal of Evidence-Based Healthcare, vol. 13, no. 3, pp. 141-146 :
- Guidance for conducting systematic scoping reviews
- PRISMA for Scoping Reviews The PRISMA extension for scoping reviews was published in 2018. The checklist contains 20 essential reporting items and 2 optional items to include when completing a scoping review. Scoping reviews serve to synthesize evidence and assess the scope of literature on a topic. Among other objectives, scoping reviews help determine whether a systematic review of the literature is warranted. more... less... Check out the Statement/Explanatory paper by Tricco et al. (2018) and the additional Tip Sheets for Items 1-22 in the PRISMA checklist for Scoping Reviews
Rapid reviews
Rapid reviews utilize systematic review methodology, but they have a more streamlined process for possible time constraints. Defining the limitations and the drawbacks of implementing a streamlined process (and a process that may not incorporate all the components of a systematic review for transparency and systematization) must be described. To learn more about rapid reviews, check out the link below.
- A scoping review of rapid review methods
Umbrella Review
An Umbrella review is a synthesis of existing reviews, only including the highest level of evidence such as systematic reviews and meta-analyes. It specifically refers to a review that compiles evidence from multiple reviews into one accessible and usable document. Umbrella reviews focus on either a broad condition or problem for which there are competing interventions. These reviews can highlight the different interventions and their results.
Methodology paper : Aromataris , E, Fernandez, R, Godfrey, CM, Holly, C, Khalil , H & Tungpunkom , P 2015, 'Summarizing systematic reviews: Methodological development, conduct and reporting of an umbrella review approach', Int J Evid Based Healthc , vol. 13, no. 3, pp. 132-140.
- Summarizing systematic reviews
Systematized reviews
A systematized review attempts to include elements of the systematic review process while stopping short of the systematic review. Systematized reviews are typically conducted as a postgraduate student assignment, in recognition that they are not able to draw upon the resources required for a full systematic review (such as having two reviewers for extensive literature screening).
- << Previous: Useful Resources
- Last Updated: Jul 29, 2024 2:41 PM
- URL: https://libguides.sph.uth.tmc.edu/SystematicReviews
- Research article
- Open access
- Published: 22 August 2024
A systematic review and meta-analysis of randomized trials of substituting soymilk for cow’s milk and intermediate cardiometabolic outcomes: understanding the impact of dairy alternatives in the transition to plant-based diets on cardiometabolic health
- M. N. Erlich 1 , 2 ,
- D. Ghidanac 1 , 2 ,
- S. Blanco Mejia 1 , 2 ,
- T. A. Khan 1 , 2 ,
- L. Chiavaroli 1 , 2 , 3 ,
- A. Zurbau 1 , 2 ,
- S. Ayoub-Charette 1 , 2 ,
- A. Almneni 4 ,
- M. Messina 5 ,
- L. A. Leiter 1 , 2 , 3 , 6 , 7 ,
- R. P. Bazinet 1 ,
- D. J. A. Jenkins 1 , 2 , 3 , 6 , 7 ,
- C. W. C. Kendall 1 , 2 , 8 &
- J. L. Sievenpiper 1 , 2 , 3 , 6 , 7
BMC Medicine volume 22 , Article number: 336 ( 2024 ) Cite this article
1561 Accesses
82 Altmetric
Metrics details
Dietary guidelines recommend a shift to plant-based diets. Fortified soymilk, a prototypical plant protein food used in the transition to plant-based diets, usually contains added sugars to match the sweetness of cow’s milk and is classified as an ultra-processed food. Whether soymilk can replace minimally processed cow’s milk without the adverse cardiometabolic effects attributed to added sugars and ultra-processed foods remains unclear. We conducted a systematic review and meta-analysis of randomized controlled trials, to assess the effect of substituting soymilk for cow’s milk and its modification by added sugars (sweetened versus unsweetened) on intermediate cardiometabolic outcomes.
MEDLINE, Embase, and the Cochrane Central Register of Controlled Trials were searched (through June 2024) for randomized controlled trials of ≥ 3 weeks in adults. Outcomes included established markers of blood lipids, glycemic control, blood pressure, inflammation, adiposity, renal disease, uric acid, and non-alcoholic fatty liver disease. Two independent reviewers extracted data and assessed risk of bias. The certainty of evidence was assessed using GRADE (Grading of Recommendations, Assessment, Development, and Evaluation). A sub-study of lactose versus sucrose outside of a dairy-like matrix was conducted to explore the role of sweetened soymilk which followed the same methodology.
Eligibility criteria were met by 17 trials ( n = 504 adults with a range of health statuses), assessing the effect of a median daily dose of 500 mL of soymilk (22 g soy protein and 17.2 g or 6.9 g/250 mL added sugars) in substitution for 500 mL of cow’s milk (24 g milk protein and 24 g or 12 g/250 mL total sugars as lactose) on 19 intermediate outcomes. The substitution of soymilk for cow’s milk resulted in moderate reductions in non-HDL-C (mean difference, − 0.26 mmol/L [95% confidence interval, − 0.43 to − 0.10]), systolic blood pressure (− 8.00 mmHg [− 14.89 to − 1.11]), and diastolic blood pressure (− 4.74 mmHg [− 9.17 to − 0.31]); small important reductions in LDL-C (− 0.19 mmol/L [− 0.29 to − 0.09]) and c-reactive protein (CRP) (− 0.82 mg/L [− 1.26 to − 0.37]); and trivial increases in HDL-C (0.05 mmol/L [0.00 to 0.09]). No other outcomes showed differences. There was no meaningful effect modification by added sugars across outcomes. The certainty of evidence was high for LDL-C and non-HDL-C; moderate for systolic blood pressure, diastolic blood pressure, CRP, and HDL-C; and generally moderate-to-low for all other outcomes. We could not conduct the sub-study of the effect of lactose versus added sugars, as no eligible trials could be identified.
Conclusions
Current evidence provides a good indication that replacing cow’s milk with soymilk (including sweetened soymilk) does not adversely affect established cardiometabolic risk factors and may result in advantages for blood lipids, blood pressure, and inflammation in adults with a mix of health statuses. The classification of plant-based dairy alternatives such as soymilk as ultra-processed may be misleading as it relates to their cardiometabolic effects and may need to be reconsidered in the transition to plant-based diets.
Trial registration
ClinicalTrials.gov identifier, NCT05637866.
Peer Review reports
Major dietary guidelines recommend a shift to plant-based diets for public and planetary health [ 1 , 2 , 3 , 4 , 5 , 6 , 7 , 8 ] , while recommending simultaneous reductions in ultra-processed foods [ 2 , 3 , 4 , 5 , 6 , 7 , 8 ]. The shift to plant-based diets has resulted in an explosion of dairy, meat, and egg alternatives with plant protein foods projected to reach almost 10% of the global protein market by 2030 [ 9 ]. Although these foods can aid in the transition to plant-based diets, food classification systems such as the World Health Organization (WHO)-endorsed NOVA classification system classify them as ultra-processed foods to be avoided [ 10 ].
Dairy alternatives are an important example of a food category at the crossroads of these competing recommendations. School milk programs provide > 150 million servings of cow’s milk to children worldwide [ 11 ]. These programs are in addition to the food service and procurement policies of public institutions such as schools, universities, hospitals, long-term care homes, and prisons. Many of these programs and policies do not allow for the free replacement of cow’s milk with nutrient-dense plant milks [ 12 , 13 ]. Although the Dietary Guidelines for Americans [ 1 ], Canada’s Food Guide [ 3 ], and several European food-based dietary guidelines [ 14 ] recognize fortified soymilk [ 1 ] as nutritionally equivalent to cow’s milk, school nutrition programs in the United States (US) [ 12 ] and Europe [ 13 ] only provide funding for cow’s milk. There is a bipartisan bill before the US congress to change this policy and provide funding for fortified soymilk [ 15 ]. A major barrier to the use of fortified soymilk is that it contains added sugars to match the sweetness of cow’s milk at a level which would disqualify it from meeting the Food and Drug Administration’s proposed definition of “healthy” [ 16 ] (although its total sugar content is usually ~ 60% less than that of cow’s milk given the higher sweetness intensity of sucrose vs lactose) [ 17 ] and is classified (irrespective of its sugar content) as an ultra-processed food to be avoided [ 10 , 18 ]. Cow’s milk, on the other hand, enjoys classification as a “healthy,” minimally processed food to be encouraged [ 10 , 18 ].
As industry innovates in response to the growing demand and policy makers develop public health nutrition policies and programs in response to the evolving dietary guidance for more plant-based diets, it is important to understand whether nutrient-dense ultra-processed plant protein foods can replace minimally processed dairy foods without the adverse cardiometabolic effects attributed to added sugars and ultra-processed foods. We conducted a systematic review and meta-analysis of randomized controlled trials of the effect of substituting soymilk for minimally processed cow’s milk and its modification by added sugars (sweetened versus unsweetened) on intermediate cardiometabolic outcomes as a basis for understanding the role of nutrient-dense ultra-processed plant protein foods in the transition to plant-based diets.
We followed the Cochrane Handbook for Systematic Reviews of Interventions to conduct this systematic review and meta-analysis and reported our results by the Preferred Reporting Items for Systematic Reviews and Meta-Analysis (PRISMA) guidelines [ 19 , 20 ] (Additional file 1 : Table 1). To explore whether added sugars mediate any effects observed in sweetened soymilk studies, we conducted an additional systematic review and meta-analysis sub-study. This separate investigation followed the same protocol and methodology as our main study. It focused on controlled trials examining the impact of lactose in isocaloric comparisons with fructose-containing sugars (such as sucrose, high-fructose corn syrup [HFCS], or fructose) when not included in a dairy-like matrix, on all outcomes in the main study. The protocol is registered at ClinicalTrials.gov (NCT05637866).
Data sources and search strategy
We searched MEDLINE, Embase, and the Cochrane Central Register of Controlled Trials databases through June 2024. The detailed search strategies for the main study and sub-study were based on validated search terms [ 21 ] (Additional file 1 : Tables 2 and 4). Manual searches of the reference lists of included studies supplemented the systematic search.
Study selection
The main study included randomized controlled trials in human adults with any health status. Included trials had a study duration of ≥ 3 weeks and investigated the effects of soymilk compared with cow’s milk in energy matched conditions on intermediate cardiometabolic outcomes (Additional file 1 : Table 3). Trials that included other comparators that were not cow’s milk or had no viable outcome data were excluded. No restrictions were placed on language. For the sub-study, we included controlled trials involving adults of all health statuses that had a study duration of ≥ 3 weeks and investigated the effects of added sugars compared with lactose on the same intermediate cardiometabolic outcomes (Additional file 1 : Table 5).
Data extraction
A minimum of two investigators (ME, DG, SBM, AA) independently extracted relevant data from eligible studies. Extracted data included study design, sample size, sample characteristics (age, body mass index [BMI], sex, health status), intervention characteristics (soymilk volume, total sugars content, soy protein dose), control characteristics (cow’s milk volume, total sugars content, milk protein dose, milk fat content), baseline outcome levels, background diet, follow-up duration, setting, funding sources, and outcome data. The authors were contacted for missing outcome data when it was indicated that a relevant outcome was measured but not reported. Graphically presented data were extracted from figures using Plot Digitizer [ 22 ].
Outcomes for the main study and sub-study included blood lipids (low-density lipoprotein cholesterol [LDL-C], high-density lipoprotein cholesterol [HDL-C], non-high-density lipoprotein cholesterol [non-HDL-C], triglycerides, and apolipoprotein B [ApoB]), glycemic control (hemoglobin A1c [HbA1c], fasting plasma glucose, 2-h postprandial glucose, fasting insulin, and plasma glucose area under the curve [PG-AUC]), blood pressure (systolic blood pressure and diastolic blood pressure), inflammation (c-reactive protein [CRP]), adiposity (body weight, BMI, body fat, and waist circumference), kidney function and structure (creatinine, creatinine clearance, glomerular filtration rate [GFR], estimated glomerular filtration rate [eGFR], albuminuria, and albumin-creatinine ratio [ACR]), uric acid, and non-alcoholic fatty liver disease (NAFLD) (intrahepatocellular lipid [IHCL], alanine transaminase [ALT], aspartate aminotransferase [AST], and fatty liver index).
Mean differences (MDs) between the intervention and control arm and respective standard errors were extracted for each trial. If these were not provided, they were derived from available data using published formulas [ 19 ]. Mean pairwise difference in change-from-baseline values were preferred over end values. When median data was provided, they were converted to mean data with corresponding variances using methods developed by McGrath et al. [ 23 ]. When no variance data was available, the standard deviation of the MDs was borrowed from a trial similar in size, participants, and nature of intervention. All disagreements were reconciled by consensus or with a senior reviewer (JLS).
Risk of bias assessment
Included studies were assessed for the risk of bias independently and in duplicate by at least two investigators (ME, DG, SBM, AA) using the Cochrane Risk of Bias (ROB) 2 Tool [ 24 ]. The assessment was performed across six domains of bias (randomization process, deviations from intended interventions, missing outcome data, measurement of the outcome, selection of the reported result, and overall bias). Crossover studies were assessed for an additional domain of bias (risk of bias arising from period or carryover effects). The ROB for each domain was assessed as “low” (plausible bias unlikely to seriously alter the results), “high” (plausible bias that seriously weakens confidence in results), or “some concern” (plausible bias that raises some doubt about the results). Reviewer discrepancies were resolved by consensus or arbitration by a senior investigator (JLS).
Statistical analysis
STATA (version 17; StataCorp LP, College Station, TX) was used for all analyses for the main study and sub-study. The principal effect measures were the mean pair-wise differences in change from baseline (or alternatively, end differences) between the intervention arm providing the soymilk and the cow’s milk comparator/control arm in each trial (significance at P MD < 0.05). Results are reported as MDs with 95% confidence intervals (95% CI). As one of our primary research questions relates to the role of added sugars as a mediator in any observed differences between soymilk and cow’s milk, we stratified results by the presence of added sugars in the soymilk (sweetened versus unsweetened) and assessed effect modification by this variable on pooled estimates. Data were pooled using the generic inverse variance method with DerSimonian and Laird random effect models [ 25 ]. Fixed effects were used when less than five trials were available for an outcome [ 26 ]. A paired analysis was applied for crossover designs and for within-individual correlation coefficient between treatment of 0.5 as described by Elbourne et al. [ 27 , 28 ].
Heterogeneity was assessed using the Cochran’s Q statistic and quantified using the I 2 statistic, where I 2 ≥ 50% and P Q < 0.10 were used as evidence of substantial heterogeneity [ 19 ]. Potential sources of heterogeneity were explored using sensitivity analyses. Sensitivity analyses were done via two methods. We conducted an influence analysis by systematically removing one trial at a time and recalculating the overall effect estimate and heterogeneity. A trial was considered influential if its removal explained the substantial heterogeneity or altered the direction, magnitude, or significance of the summary estimate. To determine whether the overall summary estimates were robust to the use of an assumed correlation coefficient for crossover trials, we conducted a second sensitivity analysis by using correlation coefficients of 0.25 and 0.75. If ≥ 10 trials were available, meta-regression analyses were used to assess the significance of each subgroup categorically and when possible, continuously (significance at P < 0.05). A priori subgroup analyses included soy protein dose, follow-up duration, baseline outcome levels, comparator, design, age, health status, funding, and risk of bias.
If ≥ 6 trials are available [ 29 ], dose–response analyses were performed using meta-regression to assess linear (by generalized least squares trend (GLST) estimation models) and non-linear spline curve modeling (by MKSPLINE procedure) dose–response gradients (significance at P < 0.05).
If ≥ 10 studies were available, publication bias was assessed by inspection of contour-enhanced funnel plots and formal testing with Egger’s and Begg’s tests (significance at P < 0.10) [ 30 , 31 , 32 ]. If evidence of publication bias was suspected, the Duval and Tweedie trim-and-fill method was performed to adjust for funnel plot asymmetry by imputing missing study data and assess for small-study effects [ 33 ].
Certainty of evidence
The Grading of Recommendations Assessment, Development, and Evaluation (GRADE) approach was used to assess the certainty of evidence. The GRADE Handbook and GRADEpro V.3.2 software were used [ 34 , 35 ]. A minimum of two investigators (ME, DG, SBM) independently performed GRADE assessments for each outcome [ 36 ]. Discrepancies were resolved by consensus or arbitration by the senior author (JLS). The overall certainty of evidence was graded as either high, moderate, low, or very low. Randomized trials are initially graded as high by default and then downgraded or upgraded based on prespecified criteria. Reasons for downgrading the evidence included study limitations (risk of bias assessed by the Cochrane ROB Tool), inconsistency of results (substantial unexplained interstudy heterogeneity, I 2 > 50% and P Q < 0.10), indirectness of evidence (presence of factors that limit the generalizability of the results), imprecision (the 95% CI for effect estimates overlap with the MID for benefit or harm), and publication bias (evidence of small-study effects). The evidence was upgraded if a significant dose–response gradient was detected. We defined the importance of the magnitude of the pooled effect estimates using prespecified MIDs (Additional file 1 : Table 6) with GRADE guidance [ 36 , 37 , 38 , 39 , 40 , 41 , 42 , 43 , 44 , 45 , 46 , 47 , 48 , 49 , 50 ] according to five levels: very large (≥ 10 MID); large (≥ 5 MID); moderate (≥ 2 MID); small important (≥ 1 MID); and trivial/unimportant (< 1 MID) effects.
Search results
Figure 1 in Appendix shows the flow of the literature for the main analysis. We identified 522 reports through database and manual searches. A total of 17 reports [ 51 , 52 , 53 , 54 , 55 , 56 , 57 , 58 , 59 , 60 , 61 , 62 , 63 , 64 , 65 , 66 , 67 ] met the inclusion criteria and contained data for LDL (10 trials, n = 312), HDL-C (8 trials, n = 271), non-HDL-C (7 trials, n = 243), triglycerides (9 trials, n = 278), HbA1c (1 trial, n = 25), fasting plasma glucose (5 trials, n = 147), 2-h plasma glucose (1 trial, n = 28), fasting insulin (4 trials, n = 119), systolic blood pressure (5 trials, n = 158), diastolic blood pressure (5 trials, n = 158), CRP (5 trials, n = 147), body weight (6 trials, n = 163), BMI (6 trials, n = 173), body fat (1 trial, n = 43), waist circumference (3 trials, n = 90), creatinine (1 trial, n = 25), eGFR (1 trial, n = 25), ALT (1 trial, n = 24), and AST (1 trial, n = 24) involving 504 participants. No trials were available for ApoB, PG-AUC, creatinine clearance, eGFR, albuminuria, ACR, uric acid, IHCL, or fatty liver index.
Additional file 1 : Fig. 1 shows the flow of literature for the sub-study. We identified 1010 reports through database and manual searches. After excluding 305 duplicates, a total of 705 reports were reviewed by title and abstract. No reports met the inclusion criteria and therefore no data was available for analysis.
Trial characteristics
Table 1 shows the characteristics of the included trials. The trials were conducted in a variety of locations, with most conducted in Iran (7/17 trials, 41%), followed by the US (3/17 trials, 18%), Italy (2/17 trials, 12%), Brazil (1/17 trials, 6%), Scotland (1/17 trials, 6%), Sweden (1/17 trials, 6%), Spain (1/17 trials, 6%), and Australia (1/17 trials, 6%). All trials took place in outpatient settings (17/17, 100%). The median trial size was 25 participants (range, 7–60 participants). The median age of the participants was 48.5 years (range, 20–70 years) and the median BMI was 27.9 kg/m 2 (range, 20–31.1 kg/m 2 ). The trials included participants with hypercholesterolemia (4/17 trials, 25%), overweight or obesity (4/17 trials, 25%), type 2 diabetes (2/17 trials, 12%), hypertension (1/17 trials, 6%), rheumatoid arthritis (1/17 trials, 6%), or were healthy (3/17 trials, 18%) or post-menopausal (2/17 trials, 12%). Both trials with crossover design (10/17 trials, 59%) and parallel design (7/17 trials, 41%) were included. The intervention included sweetened (11/17 trials, 65%) and unsweetened (6/17 trials, 35%) soymilk.
The median soymilk dose was 500 mL/day (range, 240–1000 mL/day) with a median soy protein of 22 g/day (range, 2.5–70 g/day) or 6.6 g/250 mL (range, 2.6–35 g/250 mL) and median total (added) sugars of 17.2 g/day (range, 4.0–32 g/day) or 6.9 g/250 mL (range, 1–16 g/250 mL) in the sweetened soymilk. The comparators included skim (0% milk fat) (2/17 trials, 12%), low-fat (1% milk fat) (4/17 trials, 24%), reduced fat (1.5–2.5% milk fat) (7/17 trials, 41%), and whole (3% milk fat) (1/17 trials, 6%) cow’s milk. Three trials did not report the milk fat content of cow’s milk used. The median cow’s milk dose was 500 mL/day (range, 236–1000 mL/day) with a median milk protein of 24 g/day (range, 3.3–70 g/day) or 8.3 g/250 mL (range, 3.4–35 g/250 mL) and median total (lactose) sugars of 24 g/day (range, 11.5–49.2 g/day) or 12 g/250 mL (range, 10.8–12.8 g/250 mL). The median study duration was 4 weeks (range, 4–16 weeks). The trials received funding from industry (1/17 trials, 6%), agency (8/17 trials, 47%), both industry and agency (4/16 trials, 25%), or they did not report the funding source (4/17 trials, 24%).
Additional file 1 : Fig. 2 shows the ROB assessments of the included trials. Two trials were assessed as having some concerns from period or carryover effects: Bricarello et al. [ 53 ] and Steele [ 67 ]. All other trials were judged as having an overall low risk of bias. There was no evidence of serious risk of bias across the included trials.
Markers of blood lipids
Figure 2 and Additional file 1 : Figs. 3–6 show the effect of substituting soymilk for cow’s milk on markers of blood lipids. The substitution resulted in a small important reduction in LDL-C (10 trials; MD: − 0.19 mmol/L; 95% CI: − 0.29 to − 0.09 mmol/L; P MD < 0.001; no heterogeneity: I 2 = 0.0%; P Q = 0.823), a trivial increase in HDL-C (8 trials; MD: 0.05 mmol/L; 95% CI: 0.00 to 0.09 mmol/L; P MD = 0.036; no heterogeneity: I 2 = 0.0%; P Q = 0.053), a moderate reduction in non-HDL-C (7 trials; MD: − 0.26 mmol/L; 95% CI: − 0.43 to − 0.10 mmol/L; P MD = 0.002; no heterogeneity: I 2 = 0.0%; P Q = 0.977), and no effect on triglycerides. There were no interactions by added sugars in soymilk for any blood lipid markers ( P = 0.49–0.821).
Markers of glycemic control
Figure 2 and Additional file 1 : Figs. 7–10 show the effect of substituting soymilk for cow’s milk on markers of glycemic control. The substitution had no effect on HbA1c, fasting plasma glucose, 2-h plasma glucose, or fasting insulin. There was no interaction by added sugars in soymilk for fasting plasma glucose ( P = 0.747) but there was an interaction for fasting insulin ( P = 0.026), where a lack of effect remained in both groups with neither the sweetened soymilk (non-significant increasing effect) nor the unsweetened soymilk (non-significant decreasing effect) showing an effect on fasting insulin. We could not assess this interaction for HbA1c or 2-h plasma glucose, as there was only one trial available for each outcome.
Blood pressure
Figure 2 and Additional file 1 : Figs. 11 and 12 show the effect of substituting soymilk for cow’s milk on blood pressure. The substitution resulted in a moderate reduction in both systolic blood pressure (5 trials; MD: − 8.00 mmHg; 95% CI: − 14.89 to − 1.11 mmHg; P MD = 0.023; substantial heterogeneity: I 2 = 86.89%; P Q ≤ 0.001) and diastolic blood pressure (5 trials; MD: − 4.74 mmHg; 95% CI: − 9.17 to − 0.31 mmHg; P MD = 0.036; substantial heterogeneity: I 2 = 77.3%; P Q = 0.001). There were no interactions by added sugars in soymilk for blood pressure ( P = 0.747 and 0.964).
Markers of inflammation
Figure 2 and Additional file 1 : Fig. 13 show the effect of substituting soymilk for cow’s milk on markers of inflammation. The substitution resulted in a small important reduction in CRP (5 trials; MD: − 0.81 mg/dL; 95% CI: − 1.26 to − 0.37 mg/dL; P MD = < 0.001; no heterogeneity: I 2 = 0.0%; P Q = 0.814). There was no interaction by added sugars in soymilk for CRP ( P = 0.275).
Markers of adiposity
Figure 2 and Additional file 1 : Figs. 14–17 show the effect of substituting soymilk for cow’s milk on markers of adiposity. The substitution had no effect on body weight, BMI, body fat, or waist circumference. There were no interactions by added sugars in soymilk for any adiposity outcome ( P = 0.664–0.733).
Markers of kidney function
Figure 2 and Additional file 1 : Figs. 18 and 19 show the effect of substituting soymilk for cow’s milk on markers of kidney function. The substitution had no effect on creatinine or eGFR. We could not assess the interaction by added sugars in soymilk for creatinine or eGFR, as there was only one trial available for each outcome which included soymilk without added sugars.
Markers of NAFLD
Figure 2 and Additional file 1 : Figs. 20 and 21 show the effect of substituting soymilk for cow’s milk on markers of NAFLD. The substitution had no effect on ALT or AST. We could not assess heterogeneity or the interaction by added sugars in soymilk for ALT or AST, as there was only one trial available for each outcome which included soymilk without added sugars.
Sensitivity analysis
Additional file 1 : Figs. 22–33 present the influence analyses across all outcomes. The removal of Bricarello et al. [ 53 ] or Steele [ 67 ] each resulted in loss of significant effect for HDL-C. The removal of Onning et al. [ 62 ] or Steele [ 67 ] each resulted in a partial explanation of heterogeneity for triglycerides. The removal of Hasanpour et al. [ 56 ] explained the heterogeneity for fasting insulin. The removal of Keshavarz et al. [ 57 ] or Miraghajani et al. [ 59 ] each resulted in a loss of significant effect for systolic blood pressure and the removal of Rivas et al. [ 63 ] resulted in a partial explanation of the heterogeneity for systolic blood pressure. The removal of Hasanpour et al. [ 56 ], Keshavarz et al. [ 57 ], Miraghajani et al. [ 59 ], or Rivas et al. [ 63 ] each resulted in a loss of significant effect for diastolic blood pressure and the removal of Rivas et al. [ 63 ] resulted in a partial explanation of heterogeneity for diastolic blood pressure. The removal of Mohammad-Shahi et al. [ 58 ] resulted in loss of significant effect for CRP.
Additional file 1 : Table 8 shows the sensitivity analyses for the different correlation coefficients (0.25 and 0.75) used in paired analyses of crossover trials for all outcomes. The different correlation coefficients did not alter the direction, magnitude, or significance of the effect or evidence for heterogeneity, with the following exceptions: loss of significance for the effect of the substitution on HDL-C (8 trials; MD: 0.04 mmol/L; 95% CI: − 0.10 to 0.01 mmol/L; P MD = 0.107; I 2 = 0.0%; P Q = 0.670) with the use of 0.25 and (8 trials; MD: 0.05 mmol/L; 95% CI: − 0.10 to 0.01 mmol/L; P MD = 0.089; I 2 = 0.0%; P Q = 0.640) with the use of 0.75.
Subgroup analyses
Additional file 1 : Figs. 34–36 present the subgroup analyses and continuous meta-regression analyses for LDL-C. Subgroup analysis was not conducted for any other outcome as there were < 10 trials included. There was no significant effect modification by health status, BMI, age, comparator, baseline LDL-C, study design, follow-up duration, funding source, dose of soy protein, or risk of bias for LDL-C. However, there were tendencies towards a greater reduction in LDL-C by point estimates in groups with certain health statuses (hypercholesterolemic and overweight/obesity), a higher baseline LDL-C, and a higher soy protein dose (> 25 g/day).
Dose–response analyses
Additional file 1 : Figs. 37–42 present linear and non-linear dose–response analyses for LDL-C, HDL-C, non-HDL-C, triglycerides, body weight, and BMI. There was no dose–response seen for the effect of substituting soymilk for cow’s milk, with the exception of a positive linear dose–response for triglycerides ( P linear = 0.038). We did not downgrade the certainty of evidence as the greater reduction in triglycerides seen at lower doses of soy protein was lost at higher doses. There were no dose–response analyses performed for the remaining outcomes because there were < 6 trials available for each.
Publication bias assessment
Additional file 1 : Fig. 43 presents the contour-enhanced funnel plot for assessment of publication bias for LDL-C. There was no asymmetry at the visual inspection and no evidence (Begg’s test = 0.721, Egger’s test = 0.856) of funnel plot asymmetry for LDL-C. No other publication bias analyses could be performed as there were < 10 trials available for each.
Adverse events and acceptability
Additional file 1 : Table 9 shows the reported adverse events and acceptability of study beverages. Adverse events were reported in nine trials. In one trial by Gardner et al. [ 55 ], one participant experienced a recurrence of a cancer; however, it was considered to be unrelated to the short-term consumption of the study milks. Three trials (Miraghajani et al., Hasanpour et al., and Mohammad-Shahi, et al.) [ 56 , 58 , 59 ] reported one to two withdrawals due to digestive difficulties related to soymilk consumption. Two trials (Sirtori et al. 1999 and 2002) [ 65 , 66 ] reported one or more participants with digestive difficulties related to cow’s milk consumption. Two trials (Nourieh et al. and Keshavarz et al.) [ 57 , 61 ] each reported two participant withdrawals related to digestive problems that were not specific to either study beverage. Of these, four trials indicated that most participants found the soymilk and cow’s milk acceptable and tolerable. One trial, by Onning et al. [ 62 ], incorporated a sensory evaluation of appearance, consistency, flavor, and overall impression, which showed declining scores for both types of milk over the 3-week test period.
GRADE assessment
Additional file 1 : Table 10 presents the GRADE assessment. The certainty of evidence for the effect of substituting soymilk for cow’s milk was high for LDL-C, non-HDL-C, fasting plasma glucose, and waist circumference. The certainty of evidence was moderate for HDL-C, triglycerides, fasting insulin, systolic blood pressure, diastolic blood pressure, CRP, body weight, and BMI owing to a downgrade for imprecision of the pooled effect estimates and was moderate for body fat owing to a downgrade for indirectness. The certainty of evidence was low for HbA1c, 2-h plasma glucose, creatinine, eGFR, ALT, and AST owing to downgrades for indirectness and imprecision.
We conducted a systematic review and meta-analysis of 17 trials that examined the effect of substituting soymilk (median dose of 22 g/day or 6.6 g/250 mL serving of soy protein per day and 17.2 g/day or 6.9 g/250 mL of total [added] sugars in the sweetened soymilk) for cow’s milk (median dose of 24 g/day or 8.3 g/250 mL of milk protein and 24 g/day or 12 g/250 mL of total sugars [lactose]) and its modification by added sugars (sweetened versus unsweetened soymilk) on 19 intermediate cardiometabolic outcomes over a median follow-up period of 4 weeks in adults of varying health status. The substitution of soymilk for cows’ milk led to moderate reductions in non-HDL-C (− 0.26 mmol/L or ~ − 7%) and systolic blood pressure (− 8.00 mmHg) and diastolic blood pressure (− 4.74 mmHg); small important reductions in LDL-C (− 0.19 mmol/L or ~ − 6%) and CRP (− 0.81 mg/L or ~ 22%); and a trivial increase in HDL-C (0.05 mmol/L or ~ 4%), with no adverse effects on other intermediate cardiometabolic outcomes. There was no meaningful interaction by added sugars in soymilk, with sweetened and unsweetened soymilk showing similar effects across outcomes. There was no dose–response relationship seen across the outcomes for which dose–response analyses were performed.
Findings in relation to the literature
Our findings agree with previous evidence syntheses of soy. Regulatory authorities such as the United States Food and Drug Administration (FDA) and Health Canada have conducted comprehensive evaluations of the randomized controlled trials of the effect of soy protein from different sources on total-C and LDL-C, resulting in approved health claims for soy protein (based on an intake of 25 g/day of soy protein irrespective of source) for cholesterol reduction [ 68 ] and coronary heart disease risk reduction [ 69 ]. Updated systematic reviews and meta-analyses of the 46 randomized controlled trials included in the re-evaluation of the FDA health claim [ 70 ] showed reductions in LDL-C of − 3.2% [ 71 ]. This reduction has been stable since the health claim was first approved in 1999 [ 72 ] and is smaller but consistent with our findings specifically for soymilk. No increase in HDL-C, however, was detected. Previous systematic reviews and meta-analyses of randomized controlled trials of soy protein and soy isoflavones have also shown significant but smaller reductions in systolic blood pressure (1.70 mmHg) and diastolic blood pressure (− 1.27 mmHg) [ 73 ] than was found in the current analysis. These reductions in LDL-C and blood pressure are further supported by reductions in clinical events with updated pooled analyses of prospective cohort studies showing that legumes including soy are associated with reduced incidence of total cardiovascular disease and coronary heart disease [ 74 ].
Systematic reviews and meta-analyses that specifically isolated the effect of soymilk (as a single food matrix) in its intended substitution for cow’s milk are lacking. Sohouli and coworkers [ 75 ] conducted a systematic review and meta-analysis of 18 randomized controlled trials in 665 individuals of varying health status that assessed the effect of soymilk in comparison with a mix of comparators on intermediate cardiometabolic outcomes but did not isolate its substitution with cow’s milk. This synthesis showed similar improvements in LDL-C (− 0.24 mmol/L), systolic blood pressure (− 7.38 mmHg), diastolic blood pressure (− 4.36 mmHg), and CRP (− 1.07, mg/L), while also showing reductions in waist circumference and TNF-α [ 75 ]. The substitution of legumes that includes soy for various animal protein sources and more specifically legumes/nuts (the only exposure available) for dairy in syntheses of prospective cohort studies has also shown reductions in incident total cardiovascular disease and all-cause mortality [ 76 ].
Indirect evidence from dietary patterns that contain soy foods including soymilk in substitution for different animal sources of protein including cow’s milk further supports our findings. Systematic reviews and meta-analyses of randomized trials of the Portfolio diet and vegetarian and vegan dietary patterns have shown additive reductions in LDL-C, non-HDL-C, blood pressure, and CRP when soy foods including soymilk are combined with other foods that target these same intermediate risk factors with displacement of different animal sources of protein including cow’s milk [ 77 , 78 ]. These reductions have also been shown to translate to reductions in clinical events with systematic reviews and meta-analyses of prospective cohort studies showing that adherence to these dietary patterns is associated with reductions in incident coronary heart disease, total cardiovascular disease, and all-cause mortality [ 79 , 80 , 81 ].
Potential mechanisms of action
The potential mechanism mediating the effects of soy remains unclear. Specific components within the soy food matrix, including soy protein and phytochemicals like isoflavones [ 82 ], have been implicated. The well-established lipid-lowering effect of soy [ 72 ] may be attributed to the 7S globulin fraction of soy protein, which exerts its primary action by upregulating LDL-C receptors predominantly within the liver, thereby augmenting the clearance of LDL-C from circulation [ 82 ]. The isoflavone, fiber, fatty acids, and anti-nutrient components may also exert some mediation [ 83 ]. The reduction in blood pressure has been most linked to the soy isoflavones [ 83 ]. There is evidence that soy isoflavones may modulate the renin–angiotensin–aldosterone system (RAAS), with the capacity to inhibit the production of angiotensin II and aldosterone, thereby contributing to the regulation of blood pressure [ 73 ]. Another blood pressure lowering mechanism may involve the ability of soy isoflavones to enhance endothelial function by mitigating oxidative stress and inflammation, consequently promoting the release of the relaxing factor nitric oxide (NO) [ 73 ]. This potential mechanism of isoflavones may also explain the reductions seen in inflammation.
Strengths and limitations
Our evidence synthesis had several strengths. First, we completed a comprehensive and reproducible systematic search and selection process of the available literature examining the effect of substituting soymilk for cow’s milk on intermediate cardiometabolic outcomes. Second, we synthesized the totality of available evidence from a large body of randomized controlled trials, which gives the greatest protection against systematic error. Third, we included an extensive and comprehensive list of outcomes to fully capture the impact of soymilk on cardiometabolic health. Fourth, we only included randomized controlled trials that compared soymilk to cow’s milk directly, to increase the specificity of our conclusion. Finally, we included a GRADE assessment to explore the certainty of available evidence.
There were also several limitations. First, we could not conduct the sub-study of the effect of lactose versus added sugars outside of a dairy-like matrix, as no eligible trials could be identified. Although this analysis is important for isolating the effect of added sugars as a mediator of any adverse effects, we did not observe any meaningful interaction by added sugars in soymilk. Second, there was serious imprecision in the pooled estimates across many of the outcomes with the 95% confidence intervals overlapping the MID in each case, with the exception of LDL-C, non-HDL-C, fasting plasma glucose, and waist circumference. The certainty of evidence for HDL-C, triglycerides, HbA1c, fasting plasma glucose, 2-h plasma glucose, fasting insulin, systolic blood pressure, diastolic blood pressure, CRP, body weight, BMI, body fat, creatinine, eGFR, ALT, and AST was downgraded for this reason. Third, there was evidence of indirectness related to insufficient trials for HbA1c, 2-h plasma glucose, creatinine, eGFR, ALT, and AST, which limits generalizability. Each outcome with data from only 1 trial was downgraded for this reason. Another source of indirectness could be the median follow-up duration of 4 weeks (range, 4–16 weeks). This time frame may be sufficient for observing certain effects, but other outcomes may require a longer period to manifest changes. Despite acknowledging this variation in response time among different outcomes, we did not further downgrade for this aspect of indirectness. Instead, we tailored our conclusions to reflect short-to-moderate term effects. Finally, although publication bias was not suspected, we were only able to make this assessment for LDL-C, as there were < 10 trials for all other outcomes.
Considering these strengths and limitations, we assessed the certainty of evidence as high for LDL-C and non-HDL-C; moderate for systolic blood pressure, diastolic blood pressure, CRP, and HDL-C; and moderate-to-low for all outcomes where significant effects were not observed.
Implications
This work has important implications for plant protein foods in the recommended shift to more plant-based diets. Major international dietary guidelines in the US [ 1 ], Canada [ 3 ], and Europe [ 4 , 5 , 6 ] recommend fortified soymilk as the only suitable replacement for cow’s milk. Our findings support this recommendation showing soymilk including sweetened soymilk (up to 7 g added sugars per 250 mL) does not have any adverse effects compared with cow’s milk across 19 intermediate cardiometabolic outcomes with benefits for lipids, blood pressure, and inflammation. This evidence suggests that it may be misleading as it relates to their cardiometabolic effects to classify fortified soymilk as an ultra-processed food to be avoided while classifying cow’s milk as a minimally processed food to be encouraged (based on the WHO-endorsed NOVA classification system [ 10 ]). It also suggests that it may be misleading not to allow fortified soymilk that is sweetened with small amounts of sugars to be classified as “healthy” (based on the FDA’s new proposed definition that only permits this claim on products with added sugars ≤ 2.5 g or 5% daily value (DV) per 250 mL serving [ 16 ]). The proposed FDA criteria would prevent this claim on soymilk products designed to be iso-sweet analogs of cow’s milk (in which 5 g or 10% daily value [DV] of added sugars from sucrose in soymilk is equivalent to the 12 g of lactose in cow’s milk per 250 mL serving, as sucrose is 1.4 sweeter than lactose [ 17 ]). To prevent confusion, policy makers may want to exempt fortified soymilk from classification as an ultra-processed food and allow added sugars up to 10% DV for the definition of “healthy,” as has been proposed by the FDA for sodium and saturated fat in dairy products (including soy-based dairy alternatives) to account for accepted processing and preservation methods [ 16 ]. These policy considerations would balance the need to limit nutrient-poor energy-dense foods with the need to promote nutrient-dense foods like fortified soymilk in the shift to healthy plant-based diets.
In conclusion, the evidence provides a good indication that substituting either sweetened or unsweetened soymilk for cow’s milk in adults with varying health statuses does not have the adverse effects on intermediate cardiometabolic outcomes attributed to added sugars and ultra-processed foods in the short-to-moderate term. There appear even to be advantages with small to moderate reductions in established markers of blood lipids (LDL-C, non-HDL-C) that are in line with approved health claims for cholesterol and coronary heart disease risk reduction, as well as small to moderate reductions in blood pressure and inflammation (CRP). Sources of uncertainty include imprecision and indirectness in several of the estimates. There remains a need for more well-powered randomized controlled trials of the effect of substituting soymilk for cow’s milk on less studied intermediate cardiometabolic outcomes, especially established markers of glycemic control, kidney structure and function, and NAFLD. There is also a need for trials comparing lactose versus added sugars outside of a dairy-like matrix to understand better the role of added sugars at different levels in substitution for lactose across outcomes. In the meantime, our findings support the use of fortified soymilk with up to 7 g added sugars per 250 mL as a suitable replacement for cow’s milk and suggest that its classification as ultra-processed and/or not healthy based on small amounts of added sugars may be misleading and need to be reconsidered to facilitate the recommended transition to plant-based diets.
Availability of data and materials
All data generated or analyzed during this study are included in this published article and its Additional file 1 : information files.
Abbreviations
Grading of Recommendations, Assessment, Development, and Evaluation
Non-high-density lipoprotein cholesterol
Low-density lipoprotein cholesterol
C-reactive protein
High-density lipoprotein cholesterol
World Health Organization
United States
Preferred Reporting Items for Systematic Reviews and Meta-Analysis
High-fructose corn syrup
Body mass index
Apolipoprotein B
Hemoglobin A1c
Plasma glucose area under the curve
Glomerular filtration rate
Estimated glomerular filtration rate
Albumin-creatinine ratio
Non-alcoholic fatty liver disease
Intrahepatocellular lipid
Alanine transaminase
Aspartate aminotransferase
Mean difference
Risk of bias
95% Confidence interval
Generalized least squares trend
Food and Drug Administration
Tumor necrosis factor alpha
Renin-angiotensin-aldosterone system
Nitric oxide
Daily value
Dietary guidelines for Americans, 2020–2025. 2020 [9:[Available from: www.dietaryguidelines.gov .
Canada, Health. Canada’s Food Guide. Ottawa; 2019. https://food-guide.canada.ca/en/ .
Canada’s food guide Ottawa 2019 [Available from: https://food-guide.canada.ca/en/ .
Blomhoff R, Andersen R, Arnesen EK, Christensen JJ, Eneroth H, Erkkola M, Gudanaviciene I, Halldórsson ÞI, Höyer-Lund A, Lemming EW. Nordic nutrition recommendations 2023: integrating environmental aspects. Nordisk Ministerråd; 2023.
García EL, Lesmes IB, Perales AD, Arribas VM, del Puy Portillo Baquedano M, Velasco AMR, Salvo UF, Romero LT, Porcel FBO, Laín SA. Report of the Scientific Committee of the Spanish Agency for Food Safety and Nutrition (AESAN) on sustainable dietary and physical activity recommendations for the Spanish population. Wiley Online Library; 2023. Report No.: 2940–1399.
Brink E, van Rossum C, Postma-Smeets A, Stafleu A, Wolvers D, van Dooren C, et al. Development of healthy and sustainable food-based dietary guidelines for the Netherlands. Public Health Nutr. 2019;22(13):2419–35.
Article PubMed PubMed Central Google Scholar
Lichtenstein AH, Appel LJ, Vadiveloo M, Hu FB, Kris-Etherton PM, Rebholz CM, et al. 2021 dietary guidance to improve cardiovascular health: a scientific statement from the American Heart Association. Circulation. 2021;144(23):e472–87.
Article PubMed Google Scholar
Willett W, Rockström J, Loken B, Springmann M, Lang T, Vermeulen S, et al. Food in the Anthropocene: the EAT–Lancet Commission on healthy diets from sustainable food systems. The lancet. 2019;393(10170):447–92.
Article Google Scholar
Bartashus J, Srinivasan G. Plant-based foods poised for explosive growth. Bloomberg Intelligence. 2021.
Monteiro CA, Cannon G, Lawrence M, Costa Louzada Md, Pereira Machado P. Ultra-processed foods, diet quality, and health using the NOVA classification system. Rome: FAO; 2019. p. 48.
International Dairy Federation. The contribution of school milk programmes to the nutrition of children worldwide. Brussels: Belgium; 2020.
Google Scholar
USDA Food and Nutrition Service. Special Milk Program [Available from: https://www.fns.usda.gov/smp/special-milk-program .
The European Parliament. European Parliament resolution of 9 May 2023 on the implementation of the school scheme [Available from: https://www.europarl.europa.eu/doceo/document/TA-9-2023-0135_EN.html .
European Commission. Summary of FBDG recommendations for milk and dairy products for the EU, Iceland, Norway, Switzerland and the United Kingdom. [Available from: https://knowledge4policy.ec.europa.eu/health-promotion-knowledge-gateway/food-based-dietary-guidelines-europe-table-7_en .
Addressing Digestive Distress in Stomachs of Our Youth (ADD SOY) Act, House of Representatives, 1st Sess.; 2023. https://troycarter.house.gov/sites/evo-subsites/troycarter.house.gov/files/evo-media-document/add-soy-act.pdf .
Food and Drug Administration. Food labeling: nutrient content claims; definition of term “healthy”. In: Department of Health and Human Services (HHS); 2022. https://www.federalregister.gov/documents/2022/09/29/2022-20975/food-labeling-nutrient-content-claims-definition-of-term-healthy .
Helstad S. Chapter 20 - corn sweeteners. In: Serna-Saldivar SO, editor. Corn. 3rd ed. Oxford: AACC International Press; 2019. p. 551–91.
Chapter Google Scholar
Messina M, Sievenpiper JL, Williamson P, Kiel J, Erdman JW. Perspective: soy-based meat and dairy alternatives, despite classification as ultra-processed foods, deliver high-quality nutrition on par with unprocessed or minimally processed animal-based counterparts. Adv Nutr. 2022;13(3):726–38.
Article PubMed PubMed Central CAS Google Scholar
Higgins J, Thomas J, Chandler J. Cochrane handbook for systematic reviews of interventions version 6.2. 2021.
Moher D, Liberati A, Tetzlaff J, Altman DG, Group* P. Preferred reporting items for systematic reviews and meta-analyses: the PRISMA statement. Ann Intern Med. 2009;151(4):264–9.
BMJ Best Practice. Search strategies [Available from: https://bestpractice.bmj.com/info/toolkit/learn-ebm/study-design-search-filters/ .
Rohatgi A. WebPlotDigitizer 4.6; 2022. https://automeris.io/WebPlotDigitizer/ .
McGrath S, Zhao X, Steele R, Thombs BD, Benedetti A, Collaboration DESD. Estimating the sample mean and standard deviation from commonly reported quantiles in meta-analysis. Stat Methods Med Res. 2020;29(9):2520–37.
Sterne JAC, Savovic J, Page MJ, Elbers RG, Blencowe NS, Boutron I, et al. RoB 2: a revised tool for assessing risk of bias in randomised trials. BMJ. 2019;366: l4898.
DerSimonian R, Laird N. Meta-analysis in clinical trials. Control Clin Trials. 1986;7(3):177–88.
Article PubMed CAS Google Scholar
Tufanaru C, Munn Z, Stephenson M, Aromataris E. Fixed or random effects meta-analysis? Common methodological issues in systematic reviews of effectiveness. Int J Evid Based Healthc. 2015;13(3):196–207.
Elbourne DR, Altman DG, Higgins JP, Curtin F, Worthington HV, Vail A. Meta-analyses involving cross-over trials: methodological issues. Int J Epidemiol. 2002;31(1):140–9.
Balk EM, Earley A, Patel K, Trikalinos TA, Dahabreh IJ. Empirical assessment of within-arm correlation imputation in trials of continuous outcomes. 2013.
Fu R, Gartlehner G, Grant M, Shamliyan T, Sedrakyan A, Wilt TJ, et al. Conducting quantitative synthesis when comparing medical interventions: AHRQ and the Effective Health Care Program. J Clin Epidemiol. 2011;64(11):1187–97.
Peters JL, Sutton AJ, Jones DR, Abrams KR, Rushton L. Contour-enhanced meta-analysis funnel plots help distinguish publication bias from other causes of asymmetry. J Clin Epidemiol. 2008;61(10):991–6.
Egger M, Smith GD, Schneider M, Minder C. Bias in meta-analysis detected by a simple, graphical test. BMJ. 1997;315(7109):629–34.
Begg CB, Mazumdar M. Operating characteristics of a rank correlation test for publication bias. Biometrics. 1994;50(4):1088–101.
Duval S, Tweedie R. Trim and fill: a simple funnel-plot-based method of testing and adjusting for publication bias in meta-analysis. Biometrics. 2000;56(2):455–63.
Schünemann H, Brożek J, Guyatt G, Oxman A. GRADE handbook. Grading of Recommendations Assessment, Development and Evaluation, Grade Working Group. 2013.
McMaster University and Evidence Prime. GRADEpro GDT: GRADEpro Guideline Development Tool [Software]. gradepro.org .
Brunetti M, Shemilt I, Pregno S, Vale L, Oxman AD, Lord J, et al. GRADE guidelines: 10. Considering resource use and rating the quality of economic evidence. J Clin Epidemiol. 2013;66(2):140–50.
Guyatt GH, Oxman AD, Kunz R, Brozek J, Alonso-Coello P, Rind D, et al. GRADE guidelines 6. Rating the quality of evidence—imprecision. J Clin Epidemiol. 2011;64(12):1283–93.
Guyatt GH, Oxman AD, Kunz R, Woodcock J, Brozek J, Helfand M, et al. GRADE guidelines: 8. Rating the quality of evidence—indirectness. J Clin Epidemiol. 2011;64(12):1303–10.
Guyatt GH, Oxman AD, Kunz R, Woodcock J, Brozek J, Helfand M, et al. GRADE guidelines: 7. Rating the quality of evidence—inconsistency. J Clin Epidemiol. 2011;64(12):1294–302.
Guyatt GH, Oxman AD, Montori V, Vist G, Kunz R, Brozek J, et al. GRADE guidelines: 5. Rating the quality of evidence—publication bias. J Clin Epidemiol. 2011;64(12):1277–82.
Guyatt GH, Oxman AD, Santesso N, Helfand M, Vist G, Kunz R, et al. GRADE guidelines: 12. Preparing summary of findings tables-binary outcomes. J Clin Epidemiol. 2013;66(2):158–72.
Guyatt GH, Oxman AD, Sultan S, Glasziou P, Akl EA, Alonso-Coello P, et al. GRADE guidelines: 9. Rating up the quality of evidence. J Clin Epidemiol. 2011;64(12):1311–6.
Guyatt GH, Oxman AD, Vist G, Kunz R, Brozek J, Alonso-Coello P, et al. GRADE guidelines: 4. Rating the quality of evidence—study limitations (risk of bias). J Clin Epidemiol. 2011;64(4):407–15.
Guyatt GH, Thorlund K, Oxman AD, Walter SD, Patrick D, Furukawa TA, et al. GRADE guidelines: 13. Preparing summary of findings tables and evidence profiles-continuous outcomes. J Clin Epidemiol. 2013;66(2):173–83.
Kaminski-Hartenthaler A, Gartlehner G, Kien C, Meerpohl JJ, Langer G, Perleth M, et al. GRADE-Leitlinien: 11. Gesamtbeurteilung des Vertrauens in Effektschätzer für einen einzelnen Studienendpunkt und für alle Endpunkte. Zeitschrift für Evidenz, Fortbildung und Qualität im Gesundheitswesen. 2013;107(9):638–45.
Langendam M, Carrasco-Labra A, Santesso N, Mustafa RA, Brignardello-Petersen R, Ventresca M, et al. Improving GRADE evidence tables part 2: a systematic survey of explanatory notes shows more guidance is needed. J Clin Epidemiol. 2016;74:19–27.
Santesso N, Carrasco-Labra A, Langendam M, Brignardello-Petersen R, Mustafa RA, Heus P, et al. Improving GRADE evidence tables part 3: detailed guidance for explanatory footnotes supports creating and understanding GRADE certainty in the evidence judgments. J Clin Epidemiol. 2016;74:28–39.
Santesso N, Glenton C, Dahm P, Garner P, Akl EA, Alper B, et al. GRADE guidelines 26: informative statements to communicate the findings of systematic reviews of interventions. J Clin Epidemiol. 2020;119:126–35.
Balshem H, Helfand M, Schünemann HJ, Oxman AD, Kunz R, Brozek J, et al. GRADE guidelines: 3. Rating the quality of evidence. J Clin Epidemiol. 2011;64(4):401–6.
Schünemann HJ, Higgins JPT, Vist GE, Glasziou P, Akl EA, Skoetz N, Guyatt GH, Group, Cochrane GRADEing Methods and Group, the Cochrane Statistical Methods. Chapter 14: completing ‘summary of findings’ tables and grading the certainty of the evidence. Cochrane handbook for systematic reviews of interventions. 2019. p. 375–402.
Azadbakht L, Nurbakhsh S. Effect of soy drink replacement in a weight reducing diet on anthropometric values and blood pressure among overweight and obese female youths. Asia Pac J Clin Nutr. 2011;20(3):383–9.
PubMed CAS Google Scholar
Beavers KM, Serra MC, Beavers DP, Cooke MB, Willoughby DS. Soymilk supplementation does not alter plasma markers of inflammation and oxidative stress in postmenopausal women. Nutr Res. 2009;29(9):616–22.
Bricarello LP, Kasinski N, Bertolami MC, Faludi A, Pinto LA, Relvas WG, et al. Comparison between the effects of soy milk and non-fat cow milk on lipid profile and lipid peroxidation in patients with primary hypercholesterolemia. Nutrition. 2004;20(2):200–4.
Faghih S, Hedayati M, Abadi A, Kimiagar M. Comparison of the effects of cow’s milk, fortified soy milk, and calcium supplement on plasma adipocytokines in overweight and obese women. Iranian Journal of Endocrinology and Metabolism. 2009;11(6):692–8.
Gardner CD, Messina M, Kiazand A, Morris JL, Franke AA. Effect of two types of soy milk and dairy milk on plasma lipids in hypercholesterolemic adults: a randomized trial. J Am Coll Nutr. 2007;26(6):669–77.
Hasanpour A, Babajafari S, Mazloomi SM, Shams M. The effects of soymilk plus probiotics supplementation on cardiovascular risk factors in patients with type 2 diabetes mellitus: a randomized clinical trial. BMC Endocr Disord. 2023;23(1):36.
Keshavarz SA, Nourieh Z, Attar MJ, Azadbakht L. Effect of soymilk consumption on waist circumference and cardiovascular risks among overweight and obese female adults. Int J Prev Med. 2012;3(11):798–805.
PubMed PubMed Central Google Scholar
Mohammad-Shahi M, Mowla K, Haidari F, Zarei M, Choghakhori R. Soy milk consumption, markers of inflammation and oxidative stress in women with rheumatoid arthritis: a randomised cross-over clinical trial. Nutr Diet. 2016;73(2):139–45.
Miraghajani MS, Esmaillzadeh A, Najafabadi MM, Mirlohi M, Azadbakht L. Soy milk consumption, inflammation, coagulation, and oxidative stress among type 2 diabetic patients with nephropathy. Diabetes Care. 2012;35(10):1981–5.
Mitchell JH, Collins AR. Effects of a soy milk supplement on plasma cholesterol levels and oxidative DNA damage in men—a pilot study. Eur J Nutr. 1999;38(3):143–8.
Nourieh Z, Keshavarz SA, Attar MJH, Azadbakht L. Effects of soy milk consumption on inflammatory markers and lipid profiles among non-menopausal overweight and obese female adults. Int J Prev Med. 2012;3:798.
Onning G, Akesson B, Oste R, Lundquist I. Effects of consumption of oat milk, soya milk, or cow’s milk on plasma lipids and antioxidative capacity in healthy subjects. Ann Nutr Metab. 1998;42(4):211–20.
Rivas M, Garay RP, Escanero JF, Cia P Jr, Cia P, Alda JO. Soy milk lowers blood pressure in men and women with mild to moderate essential hypertension. J Nutr. 2002;132(7):1900–2.
Ryan-Borchers TA, Park JS, Chew BP, McGuire MK, Fournier LR, Beerman KA. Soy isoflavones modulate immune function in healthy postmenopausal women. Am J Clin Nutr. 2006;83(5):1118–25.
Sirtori CR, Pazzucconi F, Colombo L, Battistin P, Bondioli A, Descheemaeker K. Double-blind study of the addition of high-protein soya milk v. cows’ milk to the diet of patients with severe hypercholesterolaemia and resistance to or intolerance of statins. Br J Nutr. 1999;82(2):91–6.
Sirtori CR, Bosisio R, Pazzucconi F, Bondioli A, Gatti E, Lovati MR, et al. Soy milk with a high glycitein content does not reduce low-density lipoprotein cholesterolemia in type II hypercholesterolemic patients. Ann Nutr Metab. 2002;46(2):88–92.
Steele M. Effect on serum cholesterol levels of substituting milk with a soya beverage. Aust J Nutr Diet. 1992;49(1):24–8.
Summary of Health Canada’s assessment of a health claim about soy protein and cholesterol lowering Ottawa: Health Canada; 2015 [Available from: https://www.canada.ca/en/health-canada/services/food-nutrition/food-labelling/health-claims/assessments/summary-assessment-health-claim-about-protein-cholesterol-lowering.html .
Food and Drug Administration. Food labeling health claims; soy protein and coronary heart disease. Fed Regist. 1999;64:57699–733.
Food and Drug Administration. Food labeling health claims; soy protein and coronary heart disease. Fed Regist. 2017;82:50324–46.
Blanco Mejia S, Messina M, Li SS, Viguiliouk E, Chiavaroli L, Khan TA, et al. A meta-analysis of 46 studies identified by the FDA demonstrates that soy protein decreases circulating LDL and total cholesterol concentrations in adults. J Nutr. 2019;149(6):968–81.
Jenkins DJA, Blanco Mejia S, Chiavaroli L, Viguiliouk E, Li SS, Kendall CWC, et al. Cumulative meta-analysis of the soy effect over time. J Am Heart Assoc. 2019;8(13):e012458.
Mosallanezhad Z, Mahmoodi M, Ranjbar S, Hosseini R, Clark CCT, Carson-Chahhoud K, et al. Soy intake is associated with lowering blood pressure in adults: a systematic review and meta-analysis of randomized double-blind placebo-controlled trials. Complement Ther Med. 2021;59:102692.
Viguiliouk E, Glenn AJ, Nishi SK, Chiavaroli L, Seider M, Khan T, et al. Associations between dietary pulses alone or with other legumes and cardiometabolic disease outcomes: an umbrella review and updated systematic review and meta-analysis of prospective cohort studies. Adv Nutr. 2019;10(Suppl_4):S308–19.
Sohouli MH, Lari A, Fatahi S, Shidfar F, Găman M-A, Guimaraes NS, et al. Impact of soy milk consumption on cardiometabolic risk factors: a systematic review and meta-analysis of randomized controlled trials. Journal of Functional Foods. 2021;83:104499.
Neuenschwander M, Stadelmaier J, Eble J, Grummich K, Szczerba E, Kiesswetter E, et al. Substitution of animal-based with plant-based foods on cardiometabolic health and all-cause mortality: a systematic review and meta-analysis of prospective studies. BMC Med. 2023;21(1):404.
Chiavaroli L, Nishi SK, Khan TA, Braunstein CR, Glenn AJ, Mejia SB, et al. Portfolio dietary pattern and cardiovascular disease: a systematic review and meta-analysis of controlled trials. Prog Cardiovasc Dis. 2018;61(1):43–53.
Viguiliouk E, Kendall CW, Kahleova H, Rahelic D, Salas-Salvado J, Choo VL, et al. Effect of vegetarian dietary patterns on cardiometabolic risk factors in diabetes: a systematic review and meta-analysis of randomized controlled trials. Clin Nutr. 2019;38(3):1133–45.
Glenn AJ, Guasch-Ferre M, Malik VS, Kendall CWC, Manson JE, Rimm EB, et al. Portfolio diet score and risk of cardiovascular disease: findings from 3 prospective cohort studies. Circulation. 2023;148(22):1750–63.
Glenn AJ, Lo K, Jenkins DJA, Boucher BA, Hanley AJ, Kendall CWC, et al. Relationship between a plant-based dietary portfolio and risk of cardiovascular disease: findings from the Women’s Health Initiative prospective cohort study. J Am Heart Assoc. 2021;10(16): e021515.
Lo K, Glenn AJ, Yeung S, Kendall CWC, Sievenpiper JL, Jenkins DJA, Woo J. Prospective association of the portfolio diet with all-cause and cause-specific mortality risk in the Mr. OS and Ms. OS study. Nutrients. 2021;13(12):4360. https://doi.org/10.3390/nu13124360 .
Jenkins DJ, Mirrahimi A, Srichaikul K, Berryman CE, Wang L, Carleton A, et al. Soy protein reduces serum cholesterol by both intrinsic and food displacement mechanisms. J Nutr. 2010;140(12):2302S-S2311.
Ramdath DD, Padhi EM, Sarfaraz S, Renwick S, Duncan AM. Beyond the cholesterol-lowering effect of soy protein: a review of the effects of dietary soy and its constituents on risk factors for cardiovascular disease. Nutrients. 2017;9(4):324. https://doi.org/10.3390/nu9040324 .
Download references
Acknowledgements
Aspects of this work were presented at the following conferences: Canadian Nutrition Society (CNS), Quebec City, Canada, May 4–6, 2023; 40th International Symposium on Diabetes and Nutrition, Pula, Croatia, June 15–18, 2023; and Nutrition 2023—American Society for Nutrition (ASN), Boston, USA, July 22–25, 2023.
Authors’ Twitter handles
@Toronto_3D_Unit.
This work was supported by the United Soybean Board (the United States Department of Agriculture Soybean Checkoff Program [funding reference number, 2411–108-0101]) and the Canadian Institutes of Health Research (funding reference number, 129920) through the Canada-wide Human Nutrition Trialists’ Network (NTN). The Diet, Digestive tract, and Disease (3D) Centre, funded through the Canada Foundation for Innovation and the Ministry of Research and Innovation’s Ontario Research Fund, provided the infrastructure for the conduct of this work. ME was funded by a CIHR Canada Graduate Scholarship and Toronto 3D PhD Scholarship award. DG was funded by an Ontario Graduate Scholarship. TAK and AZ were funded by a Toronto 3D Postdoctoral Fellowship Award. LC was funded by a Toronto 3D New Investigator Award. SA-C was funded by a CIHR Canadian Graduate Scholarship. DJAJ was funded by the Government of Canada through the Canada Research Chair Endowment. None of the sponsors had any role in study design; in the collection, analysis, and interpretation of data; in the writing of the report; and in the decision to submit the article for publication. But one of the co-authors, Mark Messina, who was involved in all aspects of the study except data collection or analysis, is the Director of Nutrition Science and Research at the Soy Nutrition Institute Global, an organization that receives partial funding from the principal funder, the United Soybean Board (USB).
Author information
Authors and affiliations.
Department of Nutritional Sciences, Temerty Faculty of Medicine, University of Toronto, Toronto, ON, Canada
M. N. Erlich, D. Ghidanac, S. Blanco Mejia, T. A. Khan, L. Chiavaroli, A. Zurbau, S. Ayoub-Charette, L. A. Leiter, R. P. Bazinet, D. J. A. Jenkins, C. W. C. Kendall & J. L. Sievenpiper
Toronto 3D Knowledge Synthesis and Clinical Trials Unit, Clinical Nutrition and Risk Factor Modification Centre, St. Michael’s Hospital, Toronto, ON, Canada
M. N. Erlich, D. Ghidanac, S. Blanco Mejia, T. A. Khan, L. Chiavaroli, A. Zurbau, S. Ayoub-Charette, L. A. Leiter, D. J. A. Jenkins, C. W. C. Kendall & J. L. Sievenpiper
Li Ka Shing Knowledge Institute, St. Michael’s Hospital, Toronto, ON, Canada
L. Chiavaroli, L. A. Leiter, D. J. A. Jenkins & J. L. Sievenpiper
Royal College of Surgeons in Ireland, Dublin, Ireland
Soy Nutrition Institute Global, Washington, DC, USA
Department of Medicine, Temerty Faculty of Medicine, University of Toronto, Toronto, ON, Canada
L. A. Leiter, D. J. A. Jenkins & J. L. Sievenpiper
Division of Endocrinology and Metabolism, Department of Medicine, St. Michael’s Hospital, Toronto, ON, Canada
College of Pharmacy and Nutrition, University of Saskatchewan, Saskatoon, SK, Canada
C. W. C. Kendall
You can also search for this author in PubMed Google Scholar
Contributions
The authors’ responsibilities were as follows: JLS designed the research (conception, development of overall research plan, and study oversight); ME and DG acquired the data; ME, SBM, TAK, and SAC performed the data analysis; JLS, ME, DG, SBM, AA, TAK, and LC interpreted the data; JLS and ME drafted the manuscript, have primary responsibility for the final content, and take responsibility for the integrity of the data and accuracy of the data analysis; JLS, MNE, DG, SBM, TAK, LC, AZ, SAC, AA, MM, LAL, RPB, CWCK, and DJD contributed to the project conception and critical revision of the manuscript for important intellectual content and read and approved the final version of the manuscript. The corresponding author attests that all listed authors meet the authorship criteria and that no others meeting the criteria have been omitted. All authors read and approved the final manuscript.
Corresponding author
Correspondence to J. L. Sievenpiper .
Ethics declarations
Ethics approval and consent to participate.
Not applicable.
Consent for publication
Competing interests.
TAK reports receiving grants from Institute for the Advancement of Food and Nutrition Sciences (IAFNS, formerly ILSI North America) and National Honey Board (USDA Checkoff program). He has received honorariums from Advancement of Food and Nutrition Sciences (IAFNS), the International Food Information Council (IFIC), the Calorie Control Council (CCC), the International Sweeteners Association (ISA), and AmCham Dubai. He has received funding from the Toronto 3D Knowledge Synthesis and Clinical Trials foundation. LC has received research support from the Canadian Institutes of health Research (CIHR), Protein Industries Canada (a Government of Canada Global Innovation Clusters), The United Soybean Board (USDA soy “Checkoff” program), and the Alberta Pulse Growers Association. AZ is a part-time research associate at INQUIS Clinical Research, Ltd., a contract research organization. She has received consulting fees from Glycemic Index Foundation Inc. SA-C has received an honorarium from the International Food Information Council (IFIC) for a talk on artificial sweeteners, the gut microbiome, and the risk for diabetes. MM was employed by the Soy Nutrition Institute Global, an organization that receives funding from the United Soybean Board (USB) and from members involved in the soy industry. RPB has received industrial grants, including those matched by the Canadian government, and/or travel support or consulting fees largely related to work on brain fatty acid metabolism or nutrition from Arctic Nutrition, Bunge Ltd., Dairy Farmers of Canada, DSM, Fonterra Inc, Mead Johnson, Natures Crops International, Nestec Inc. Pharmavite, Sancero Inc., and Spore Wellness Inc. Moreover, Dr. Bazinet is on the executive of the International Society for the Study of Fatty Acids and Lipids and held a meeting on behalf of Fatty Acids and Cell Signaling, both of which rely on corporate sponsorship. Dr. Bazinet has given expert testimony in relation to supplements and the brain. DJAJ has received research grants from Saskatchewan & Alberta Pulse Growers Associations, the Agricultural Bioproducts Innovation Program through the Pulse Research Network, the Advanced Foods and Material Network, Loblaw Companies Ltd., Unilever Canada and Netherlands, Barilla, the Almond Board of California, Agriculture and Agri-food Canada, Pulse Canada, Kellogg’s Company, Canada, Quaker Oats, Canada, Procter & Gamble Technical Centre Ltd., Bayer Consumer Care, Springfield, NJ, Pepsi/Quaker, International Nut & Dried Fruit Council (INC), Soy Foods Association of North America, the Coca-Cola Company (investigator initiated, unrestricted grant), Solae, Haine Celestial, the Sanitarium Company, Orafti, the International Tree Nut Council Nutrition Research and Education Foundation, the Peanut Institute, Soy Nutrition Institute (SNI), the Canola and Flax Councils of Canada, the Calorie Control Council, the Canadian Institutes of Health Research (CIHR), the Canada Foundation for Innovation (CFI), and the Ontario Research Fund (ORF). He has received in-kind supplies for trials as a research support from the Almond board of California, Walnut Council of California, the Peanut Institute, Barilla, Unilever, Unico, Primo, Loblaw Companies, Quaker (Pepsico), Pristine Gourmet, Bunge Limited, Kellogg Canada, and WhiteWave Foods. He has been on the speaker’s panel, served on the scientific advisory board and/or received travel support and/or honoraria from Lawson Centre Nutrition Digital Series, Nutritional Fundamentals for Health (NFH)-Nutramedica, Saint Barnabas Medical Center, The University of Chicago, 2020 China Glycemic Index (GI) International Conference, Atlantic Pain Conference, Academy of Life Long Learning, the Almond Board of California, Canadian Agriculture Policy Institute, Loblaw Companies Ltd, the Griffin Hospital (for the development of the NuVal scoring system), the Coca-Cola Company, Epicure, Danone, Diet Quality Photo Navigation (DQPN), Better Therapeutics (FareWell), Verywell, True Health Initiative (THI), Heali AI Corp, Institute of Food Technologists (IFT), Soy Nutrition Institute (SNI), Herbalife Nutrition Institute (HNI), Saskatchewan & Alberta Pulse Growers Associations, Sanitarium Company, Orafti, the International Tree Nut Council Nutrition Research and Education Foundation, the Peanut Institute, Herbalife International, Pacific Health Laboratories, Barilla, Metagenics, Bayer Consumer Care, Unilever Canada and Netherlands, Solae, Kellogg, Quaker Oats, Procter & Gamble, Abbott Laboratories, Dean Foods, the California Strawberry Commission, Haine Celestial, PepsiCo, the Alpro Foundation, Pioneer Hi-Bred International, DuPont Nutrition and Health, Spherix Consulting and WhiteWave Foods, the Advanced Foods and Material Network, the Canola and Flax Councils of Canada, Agri-Culture and Agri-Food Canada, the Canadian Agri-Food Policy Institute, Pulse Canada, the Soy Foods Association of North America, the Nutrition Foundation of Italy (NFI), Nutra-Source Diagnostics, the McDougall Program, the Toronto Knowledge Translation Group (St. Michael’s Hospital), the Canadian College of Naturopathic Medicine, The Hospital for Sick Children, the Canadian Nutrition Society (CNS), the American Society of Nutrition (ASN), Arizona State University, Paolo Sorbini Foundation, and the Institute of Nutrition, Metabolism and Diabetes. He received an honorarium from the United States Department of Agriculture to present the 2013 W.O. Atwater Memorial Lecture. He received the 2013 Award for Excellence in Research from the International Nut and Dried Fruit Council. He received funding and travel support from the Canadian Society of Endocrinology and Metabolism to produce mini cases for the Canadian Diabetes Association (CDA). He is a member of the International Carbohydrate Quality Consortium (ICQC). His wife, Alexandra L Jenkins, is a director and partner of INQUIS Clinical Research for the Food Industry, his 2 daughters, Wendy Jenkins and Amy Jenkins, have published a vegetarian book that promotes the use of the foods described here, The Portfolio Diet for Cardiovascular Risk Reduction (Academic Press/Elsevier 2020 ISBN:978–0-12–810510-8), and his sister, Caroline Brydson, received funding through a grant from the St. Michael’s Hospital Foundation to develop a cookbook for one of his studies. He is also a vegan. CWCK has received grants or research support from the Advanced Food Materials Network, Agriculture and Agri-Foods Canada (AAFC), Almond Board of California, Barilla, Canadian Institutes of Health Research (CIHR), Canola Council of Canada, International Nut and Dried Fruit Council, International Tree Nut Council Research and Education Foundation, Loblaw Brands Ltd, the Peanut Institute, Pulse Canada, and Unilever. He has received in-kind research support from the Almond Board of California, Barilla, California Walnut Commission, Kellogg Canada, Loblaw Companies, Nutrartis, Quaker (PepsiCo), the Peanut Institute, Primo, Unico, Unilever, and WhiteWave Foods/Danone. He has received travel support and/or honoraria from the Barilla, California Walnut Commission, Canola Council of Canada, General Mills, International Nut and Dried Fruit Council, International Pasta Organization, Lantmannen, Loblaw Brands Ltd, Nutrition Foundation of Italy, Oldways Preservation Trust, Paramount Farms, the Peanut Institute, Pulse Canada, Sun-Maid, Tate & Lyle, Unilever, and White Wave Foods/Danone. He has served on the scientific advisory board for the International Tree Nut Council, International Pasta Organization, McCormick Science Institute, and Oldways Preservation Trust. He is a founding member of the International Carbohydrate Quality Consortium (ICQC), Executive Board Member of the Diabetes and Nutrition Study Group (DNSG) of the European Association for the Study of Diabetes (EASD), is on the Clinical Practice Guidelines Expert Committee for Nutrition Therapy of the EASD, and is a Director of the Toronto 3D Knowledge Synthesis and Clinical Trials foundation. JLS has received research support from the Canadian Foundation for Innovation, Ontario Research Fund, Province of Ontario Ministry of Research and Innovation and Science, Canadian Institutes of health Research (CIHR), Diabetes Canada, American Society for Nutrition (ASN), National Honey Board (U.S. Department of Agriculture [USDA] honey “Checkoff” program), Institute for the Advancement of Food and Nutrition Sciences (IAFNS), Pulse Canada, Quaker Oats Center of Excellence, INC International Nut and Dried Fruit Council Foundation, The United Soybean Board (USDA soy “Checkoff” program), Protein Industries Canada (a Government of Canada Global Innovation Cluster), Almond Board of California, European Fruit Juice Association, The Tate and Lyle Nutritional Research Fund at the University of Toronto, The Glycemic Control and Cardiovascular Disease in Type 2 Diabetes Fund at the University of Toronto (a fund established by the Alberta Pulse Growers), The Plant Protein Fund at the University of Toronto (a fund which has received contributions from IFF among other donors), The Plant Milk Fund at the University of Toronto (a fund established by the Karuna Foundation through Vegan Grants), and The Nutrition Trialists Network Fund at the University of Toronto (a fund established by donations from the Calorie Control Council and Physicians Committee for Responsible Medicine). He has received food donations to support randomized controlled trials from the Almond Board of California, California Walnut Commission, Danone, Nutrartis, Soylent, and Dairy Farmers of Canada. He has received travel support, speaker fees and/or honoraria from Danone, FoodMinds LLC, Nestlé, Abbott, General Mills, Nutrition Communications, International Food Information Council (IFIC), Arab Beverages, International Sweeteners Association, Association Calorie Control Council, and Phynova. He has or has had ad hoc consulting arrangements with Perkins Coie LLP, Tate & Lyle, Ingredion, and Brightseed. He is on the Clinical Practice Guidelines Expert Committees of Diabetes Canada, European Association for the study of Diabetes (EASD), Canadian Cardiovascular Society (CCS), and Obesity Canada/Canadian Association of Bariatric Physicians and Surgeons. He serves as an unpaid member of the Board of Trustees of IAFNS. He is a Director at Large of the Canadian Nutrition Society (CNS), founding member of the International Carbohydrate Quality Consortium (ICQC), Executive Board Member of the Diabetes and Nutrition Study Group (DNSG) of the EASD, and Director of the Toronto 3D Knowledge Synthesis and Clinical Trials foundation. His spouse is an employee of AB InBev. All other authors declare that they have no competing interests.
Additional information
Publisher’s note.
Springer Nature remains neutral with regard to jurisdictional claims in published maps and institutional affiliations.
Supplementary Information
12916_2024_3524_moesm1_esm.docx.
Additional file 1: This file contains Additional file 1 material, including the PRISMA checklist, further details on the search process, and additional results.
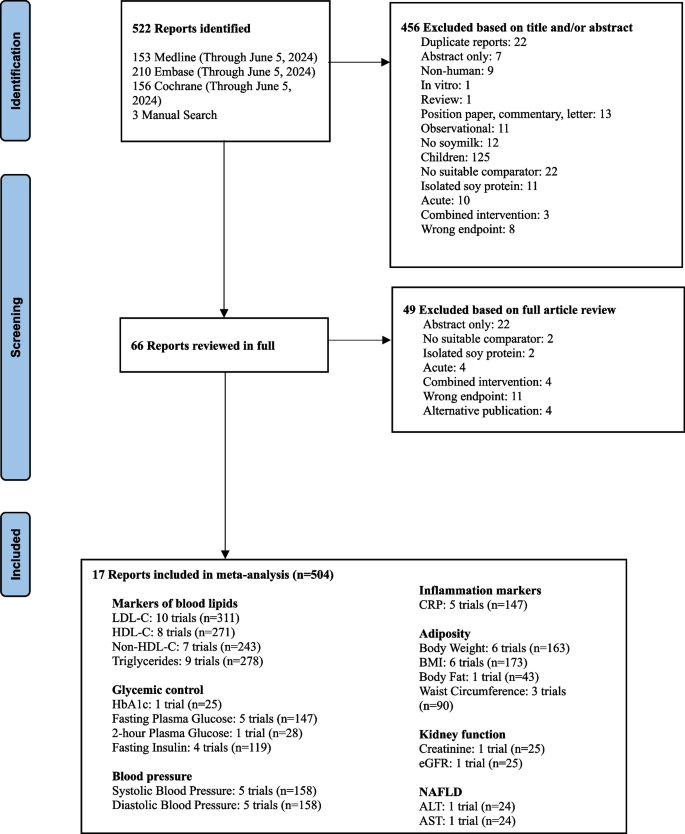
Flow of literature on the effect of substituting soymilk for cow’s milk on intermediate cardiometabolic outcomes. Exclusion criteria: duplicate, abstract only (conference abstract), non-human (animal study), in vitro, review/position paper/commentary/letter, observational (observational study), no soymilk (intervention was not soymilk), children (participants < 18 years of age), no suitable comparator (comparator was not cow’s milk), isolated soy protein (an ISP powder was given to participants), acute (follow-up of < 3 weeks), combined intervention (effects of intervention and comparator could not be isolated), wrong endpoint (no data for outcomes of interest), alternative publication (repeated data from original publication)

A summary plot for the effect of substituting soymilk for cow’s milk on intermediate cardiometabolic outcomes. Analyses were conducted using generic, inverse variance random-effects models (at least 5 trials available), or fixed-effects models (fewer than 5 trials available). Between-study heterogeneity was assessed by the Cochrane Q statistic, where P Q < 0.100 was considered statistically significant, and quantified by the I 2 statistic, where I 2 ≥ 50% was considered evidence of substantial heterogeneity. The GRADE of randomized controlled trials are rated as “high” certainty of evidence and can be downgraded by 5 domains and upgraded by 1 domain. The white squares represent no downgrades, the filled black squares indicate a single downgrade or upgrades for each outcome, and the black square with a white “2” indicates a double downgrade for each outcome. Because all included trials were randomized or nonrandomized controlled trials, the certainty of the evidence was graded as high for all outcomes by default and then downgraded or upgraded based on prespecified criteria. Criteria for downgrades included risk of bias (downgraded if most trials were considered to be at high ROB); inconsistency (downgraded if there was substantial unexplained heterogeneity: I 2 ≥ 50%; P Q < 0.10); indirectness (downgraded if there were factors absent or present relating to the participants, interventions, or outcomes that limited the generalizability of the results); imprecision (downgraded if the 95% CI crossed the minimally important difference (MID) for harm or benefit); and publication bias (downgraded if there was evidence of publication bias based on the funnel plot asymmetry and/or significant Egger or Begg test ( P < 0.10)), with confirmation by adjustment using the trim-and-fill analysis of Duval and Tweedie. The criteria for upgrades included a significant dose–response gradient. For the interpretation of the magnitude, we used the MIDs to assess the importance of magnitude of our point estimate using the effect size categories according to the new GRADE guidance. Then, we used the MIDs to assess the importance of the magnitude of our point estimates using the effect size categories according to the GRADE guidance as follows: a large effect (≥ 5 × MID); moderate effect (≥ 2 × MID); small important effect (≥ 1 × MID); and trivial/unimportant effect (< 1 MID). *HDL-C values reversed to show benefit. **LDL-C was not downgraded for imprecision, as the degree to which the upper 95% CI crosses the MID is not clinically meaningful. Additionally, the moderate change in non-HDL-C, with high certainty of evidence, substantiates the high certainty of the LDL-C results.
Rights and permissions
Open Access This article is licensed under a Creative Commons Attribution 4.0 International License, which permits use, sharing, adaptation, distribution and reproduction in any medium or format, as long as you give appropriate credit to the original author(s) and the source, provide a link to the Creative Commons licence, and indicate if changes were made. The images or other third party material in this article are included in the article's Creative Commons licence, unless indicated otherwise in a credit line to the material. If material is not included in the article's Creative Commons licence and your intended use is not permitted by statutory regulation or exceeds the permitted use, you will need to obtain permission directly from the copyright holder. To view a copy of this licence, visit http://creativecommons.org/licenses/by/4.0/ . The Creative Commons Public Domain Dedication waiver ( http://creativecommons.org/publicdomain/zero/1.0/ ) applies to the data made available in this article, unless otherwise stated in a credit line to the data.
Reprints and permissions
About this article
Cite this article.
Erlich, M.N., Ghidanac, D., Blanco Mejia, S. et al. A systematic review and meta-analysis of randomized trials of substituting soymilk for cow’s milk and intermediate cardiometabolic outcomes: understanding the impact of dairy alternatives in the transition to plant-based diets on cardiometabolic health. BMC Med 22 , 336 (2024). https://doi.org/10.1186/s12916-024-03524-7
Download citation
Received : 20 December 2023
Accepted : 09 July 2024
Published : 22 August 2024
DOI : https://doi.org/10.1186/s12916-024-03524-7
Share this article
Anyone you share the following link with will be able to read this content:
Sorry, a shareable link is not currently available for this article.
Provided by the Springer Nature SharedIt content-sharing initiative
- Soy protein
- Cardiovascular disease
- Systematic review
- Meta-analysis
- Randomized controlled feeding trials
BMC Medicine
ISSN: 1741-7015
- General enquiries: [email protected]
- Introduction
- Conclusions
- Article Information
IHCA indicates in-hospital cardiac arrest; OHCA, out-of-hospital cardiac arrest.
OR indicates odds ratio. Different size markers account for weight.
eMethods. Detailed Search Strategy
eTable. Summary of Included Studies
eFigure 1. Subgroup Analysis of Studies With Cutoff ≥40 vs <40 Cases of OHCA per Year
eFigure 2. Sensitivity Analysis With a Dose-Response Meta-analysis of Logarithmic Odds Ratios Against Mean Hospital Volume
eReferences
See More About
Sign up for emails based on your interests, select your interests.
Customize your JAMA Network experience by selecting one or more topics from the list below.
- Academic Medicine
- Acid Base, Electrolytes, Fluids
- Allergy and Clinical Immunology
- American Indian or Alaska Natives
- Anesthesiology
- Anticoagulation
- Art and Images in Psychiatry
- Artificial Intelligence
- Assisted Reproduction
- Bleeding and Transfusion
- Caring for the Critically Ill Patient
- Challenges in Clinical Electrocardiography
- Climate and Health
- Climate Change
- Clinical Challenge
- Clinical Decision Support
- Clinical Implications of Basic Neuroscience
- Clinical Pharmacy and Pharmacology
- Complementary and Alternative Medicine
- Consensus Statements
- Coronavirus (COVID-19)
- Critical Care Medicine
- Cultural Competency
- Dental Medicine
- Dermatology
- Diabetes and Endocrinology
- Diagnostic Test Interpretation
- Drug Development
- Electronic Health Records
- Emergency Medicine
- End of Life, Hospice, Palliative Care
- Environmental Health
- Equity, Diversity, and Inclusion
- Facial Plastic Surgery
- Gastroenterology and Hepatology
- Genetics and Genomics
- Genomics and Precision Health
- Global Health
- Guide to Statistics and Methods
- Hair Disorders
- Health Care Delivery Models
- Health Care Economics, Insurance, Payment
- Health Care Quality
- Health Care Reform
- Health Care Safety
- Health Care Workforce
- Health Disparities
- Health Inequities
- Health Policy
- Health Systems Science
- History of Medicine
- Hypertension
- Images in Neurology
- Implementation Science
- Infectious Diseases
- Innovations in Health Care Delivery
- JAMA Infographic
- Law and Medicine
- Leading Change
- Less is More
- LGBTQIA Medicine
- Lifestyle Behaviors
- Medical Coding
- Medical Devices and Equipment
- Medical Education
- Medical Education and Training
- Medical Journals and Publishing
- Mobile Health and Telemedicine
- Narrative Medicine
- Neuroscience and Psychiatry
- Notable Notes
- Nutrition, Obesity, Exercise
- Obstetrics and Gynecology
- Occupational Health
- Ophthalmology
- Orthopedics
- Otolaryngology
- Pain Medicine
- Palliative Care
- Pathology and Laboratory Medicine
- Patient Care
- Patient Information
- Performance Improvement
- Performance Measures
- Perioperative Care and Consultation
- Pharmacoeconomics
- Pharmacoepidemiology
- Pharmacogenetics
- Pharmacy and Clinical Pharmacology
- Physical Medicine and Rehabilitation
- Physical Therapy
- Physician Leadership
- Population Health
- Primary Care
- Professional Well-being
- Professionalism
- Psychiatry and Behavioral Health
- Public Health
- Pulmonary Medicine
- Regulatory Agencies
- Reproductive Health
- Research, Methods, Statistics
- Resuscitation
- Rheumatology
- Risk Management
- Scientific Discovery and the Future of Medicine
- Shared Decision Making and Communication
- Sleep Medicine
- Sports Medicine
- Stem Cell Transplantation
- Substance Use and Addiction Medicine
- Surgical Innovation
- Surgical Pearls
- Teachable Moment
- Technology and Finance
- The Art of JAMA
- The Arts and Medicine
- The Rational Clinical Examination
- Tobacco and e-Cigarettes
- Translational Medicine
- Trauma and Injury
- Treatment Adherence
- Ultrasonography
- Users' Guide to the Medical Literature
- Vaccination
- Venous Thromboembolism
- Veterans Health
- Women's Health
- Workflow and Process
- Wound Care, Infection, Healing
Get the latest research based on your areas of interest.
Others also liked.
- Download PDF
- X Facebook More LinkedIn
Goh AXC , Seow JC , Lai MYH, et al. Association of High-Volume Centers With Survival Outcomes Among Patients With Nontraumatic Out-of-Hospital Cardiac Arrest : A Systematic Review and Meta-Analysis . JAMA Netw Open. 2022;5(5):e2214639. doi:10.1001/jamanetworkopen.2022.14639
Manage citations:
© 2024
- Permissions
Association of High-Volume Centers With Survival Outcomes Among Patients With Nontraumatic Out-of-Hospital Cardiac Arrest : A Systematic Review and Meta-Analysis
- 1 Yong Loo Lin School of Medicine, National University of Singapore, Singapore
- 2 Center for Quantitative Medicine, Duke-NUS (National University of Singapore) Medical School, Singapore
- 3 Health Services and Systems Research, Duke-NUS Medical School, Singapore
- 4 Department of Emergency Medicine, Singapore General Hospital, Singapore
- 5 Department of Cardiology, National University Heart Center, Singapore
- 6 Academic Foundation Programme, Royal Free London NHS (National Health Service) Foundation Trust, London, United Kingdom
- 7 Prehospital and Emergency Research Center, Duke-NUS Medical School, Singapore
Question Is treatment at a high-volume center associated with improved survival and neurological outcomes among adult patients with nontraumatic out-of-hospital cardiac arrest (OHCA)?
Findings In this systematic review and meta-analysis of 16 articles involving 82 769 patients with OHCA, survival to discharge or 30 days improved with treatment at a high-volume center; there was no association between center volume and good neurological outcomes at 30 days or at hospital discharge.
Meaning These findings suggest that treatment at a high-volume center may improve survival but not neurological outcomes in patients with OHCA; more studies evaluating the relative importance of center volume compared with other variables associated with survival outcomes in these patients are required.
Importance Although high volume of cases of out-of-hospital cardiac arrest (OHCA) is a key feature of cardiac arrest centers, which have proven survival benefit, the role of center volume as an independent variable associated with improved outcomes is unclear.
Objective To assess the association of high-volume centers with survival and neurological outcomes in nontraumatic OHCA.
Data Sources Medline, Embase, and the Cochrane Central Register of Controlled Trials were searched from inception to October 11, 2021, for studies including adult patients with nontraumatic OHCA who were treated at high-volume vs non–high-volume centers.
Study Selection Randomized clinical trials, nonrandomized studies of interventions, prospective cohort studies, and retrospective cohort studies were selected that met the following criteria: (1) adult patients with OHCA of nontraumatic etiology, (2) comparison of high-volume with low-volume centers, (3) report of a volume-outcome association, and (4) report of outcomes of interest. At least 2 authors independently reviewed each article, blinded to each other’s decision.
Data Extraction and Synthesis Data abstraction and quality assessment were independently conducted by 2 authors. Meta-analyses were performed for adjusted odds ratios (aORs) and crude ORs using a random-effects model. This study followed the Preferred Reporting Items for Systematic Reviews and Meta-analyses (PRISMA) reporting guideline.
Main Outcomes and Measures Survival and good neurological outcomes according to the Cerebral Performance Categories Scale at hospital discharge or 30 days.
Results A total of 16 studies involving 82 769 patients were included. Five studies defined high volume as 40 or more cases of OHCA per year; 3 studies defined high volume as greater than 100 cases of OHCA per year. All other studies differed in definitions. Survival to discharge or 30 days improved with treatment at high-volume centers, regardless of whether aORs (1.28 [95% CI, 1.00-1.64]) or crude ORs (1.43 [95% CI, 1.09-1.87]) were pooled. There was no association between center volume and good neurological outcomes at 30 days or hospital discharge in patients with OHCA (aOR, 0.96 [95% CI, 0.77-1.20]).
Conclusions and Relevance In this meta-analysis and systematic review, care at high-volume centers was associated with improved survival outcomes, even after adjustment for potential confounders, but was not associated with improved neurological outcomes for patients with nontraumatic OHCA. More studies evaluating the relative importance of center volume compared with other variables (eg, the availability of treatment modalities) associated with survival outcomes in patients with OHCA are required.
Out-of-hospital cardiac arrest (OHCA) is a time-critical medical emergency that results in substantial disease burden. 1 , 2 Outcomes in OHCA can be poor despite the return of spontaneous circulation 3 because post–cardiac arrest syndrome, a systemic ischemia-reperfusion injury, is a major contributor to mortality and morbidity in patients with OHCA. 4 Consequently, post–cardiac arrest care has been advocated as the fifth link in the chain of survival. 5 Considering the advanced treatment required, such as targeted temperature management (TTM) and percutaneous coronary intervention (PCI), specialized tertiary centers with access to such facilities are recommended to manage cases of OHCA. 6 - 8
The association of regionalization of care to high-volume hospitals and improved outcomes has been observed in cardiological diseases and procedures, including cardiogenic shock and extracorporeal membrane oxygenation. 9 , 10 Although there have been suggestions of such benefits in OHCA management, they have not been consistently observed. A refined understanding of the volume-outcome association in patients with OHCA aids policy recommendations on emergency transportation to improve the care of these patients. 11 Furthermore, although high volume of cases of OHCA has been deemed a key feature of cardiac arrest centers (CACs), 7 it is unclear whether case volume is independently associated with improved outcomes for patients with OHCA who are treated at CACs. 12
We hypothesized that a high-volume center is associated with better clinical outcomes, namely survival to hospital discharge or 30 days and neurological outcomes at hospital discharge or 30 days among patients with OHCA. We performed a systematic review and meta-analysis to test this hypothesis.
This systematic review and meta-analysis adhered to the Preferred Reporting Items for Systematic Reviews and Meta-analyses ( PRISMA ) reporting guideline. The study protocol has been published on the International Prospective Register of Systematic Reviews (PROSPERO identifier: CRD42022300967 ).
We performed a systematic literature search in Medline, Embase, and the Cochrane Central Register of Controlled Trials using a search strategy developed in consultation with a medical information specialist on October 11, 2021. To retrieve relevant articles, we used keywords and MeSH terms such as hospital volume , patient volume , regionalisation , out-of-hospital cardiac arrest , and other synonyms in the search strategy. We consulted content experts (M.E.H.O. and A.F.W.H.) for additional references and hand-searched bibliographies of relevant sources to identify additional relevant studies. We used EndNote, version X9 (Clarivate Analytics), 13 to view and sieve articles. The search was repeated on January 1, 2022, which found no additional eligible articles. The detailed search strategy is available in the eMethods in the Supplement .
Three authors (A.X.C.G., J.C.S., and M.Y.H.L.) sorted the retrieved articles using predefined criteria. At least 2 authors independently reviewed each article, blinded to each other’s decision. Disputes were resolved through consensus with a senior author (A.F.W.H.). The following inclusion criteria were used: (1) studies of adult patients with OHCA of nontraumatic etiology, (2) studies comparing high-volume centers with low-volume centers, (3) studies reporting a volume-outcome association, and (4) studies reporting outcomes of interest such as survival to hospital discharge or 30 days and good neurological outcomes at hospital discharge or 30 days. Cerebral Performance Categories Scale scores of 1 or 2 were considered a good neurological outcome, as defined by the studies included. The outcomes evaluated were at discharge and 30 days, because long-term outcomes were not reported in the literature. We included randomized clinical trials, nonrandomized studies of interventions, prospective cohort studies, and retrospective cohort studies. We excluded conference abstracts and reports without primary data such as reviews, meta-analyses, protocols, letters, commentaries, and editorials. We excluded studies with no control group or with only pediatric patients (<18 years of age) and non-English language studies without an English translation.
Data on general article information (author, year, and country), baseline demographic characteristics of patients (age, sex, and OHCA etiology), definition of high volume (annual volume of cases of OHCA), study location (emergency department, intensive care unit, or hospital), and outcomes of interest (survival and good neurological outcomes at hospital discharge or to 30 days) were abstracted by 3 authors (A.X.C.G., J.C.S., and M.Y.H.L.). The process of data abstraction was blinded among the authors, using a predesigned data abstraction form. Disputes were resolved through consensus with a senior author (A.F.W.H.). We also abstracted adjusted odds ratios (aORs) and crude ORs for binary outcomes from each article. For ORs adjusted using incremental or hierarchical statistical models, we abstracted the aOR for the final model presented. Where multiple statistical approaches were presented (eg, regression modeling and propensity score matching) in the same study, we considered the approach used in the primary analysis. When summary effect size estimates were unavailable, we calculated ORs and 95% CIs using summary data within 2 × 2 contingency tables if reported in the study.
We performed conventional pairwise meta-analyses comparing high-volume and low-volume centers. We preferentially analyzed aORs over ORs because aORs are less likely to be influenced by confounders. Both aORs and ORs were pooled and presented because they provide different insight on direct and indirect associations, respectively. We analyzed the estimate for the highest vs lowest volume (eg, quartile 1 vs quartile 4 for studies that split volume into quartiles; high vs low volume for studies that split volume into low, medium, and high) to identify the possible association of volume with outcomes. We applied a DerSimonian-Laird random-effects model with inverse variance weights owing to expected between-study variations in population and interventions. Heterogeneity was assessed using the I 2 statistic with thresholds of 25% for low levels, 50% for moderate levels, and 75% for high levels. To account for heterogeneity, subgroup analyses were performed to compare studies defining high volume as 40 or more vs less than 40 cases of OHCA annually and also among all included studies for predefined, clinically important Utstein Formula of Survival variables 14 : initial shockable rhythm and presence of prehospital return of spontaneous circulation whenever possible. The cutoff of at least 40 cases of OHCA per year for high-volume centers was based on recommendations from the published 2020 Acute CardioVascular Care of the European Society of Cardiology (ACVC) position paper. 7 To further explore any possible volume-outcome association, we performed a dose-response meta-analysis (DRMA) as a sensitivity analysis according to the method described by Berlin et al, 15 using mean or median center volumes that were assigned to the corresponding natural logs of ORs or 95% CIs for each respective study arm. All analyses were performed using RevMan, version 5.4 (Cochrane Collaboration), 16 and R, version 4.1.0 (R Core Team). 17 Two-tailed statistical significance was set at P < .05. Publication bias was assessed through visually inspecting funnel plots when 10 or more studies reported an outcome. The quality of observational studies was evaluated on the Newcastle-Ottawa scale.
The database search yielded 2335 articles. A total of 618 duplicated articles were removed; 1679 articles were excluded based on their titles and abstracts; and a further 22 articles were excluded on full-text review. Sixteen studies 18 - 33 qualified for analysis. The study selection process and reasons for excluding the 22 studies are detailed in the flowchart in Figure 1 . Interrater agreement was excellent (κ = 0.978).
A total of 82 769 patients were included in the 16 studies. One study was conducted in Austria, 29 1 in Australia, 33 1 in Canada, 33 1 in France, 21 2 in Japan, 24 , 26 4 in South Korea, 20 , 25 , 28 , 30 2 in the United Kingdom, 22 , 32 and 4 in the US. 18 , 19 , 23 , 27 Two studies 18 , 23 reported data from the Cardiac Arrest Registry to Enhance Survival. Two studies 23 , 29 included prospective cohorts and 14 studies 18 - 28 , 30 - 33 included retrospective cohorts.
The characteristics and quality assessment of the included studies are presented in the eTable in the Supplement . The summary of meta-analysis results is presented in the Table .
The defining cutoff values of high-volume centers varied across studies. High volume was defined as 40 or more cases of OHCA per year in 5 studies 19 , 23 , 26 , 27 , 31 ; more than 100 cases of OHCA per year in 3 studies 28 , 29 , 32 ; more than 84 cases of OHCA within 5 years in 1 study 18 ; more than 79 cases of OHCA within 15 months in 1 study 24 ; more than 33 cases of OHCA per year in 1 study 20 ; more than 25 cases of OHCA per year in 1 study 22 ; more than 15 cases of OHCA per year in 1 study 21 ; more than 25 cases of TTM per year in 1 study 33 ; more than 15.5 cases of TTM per year in 1 study 25 ; and more than 69 cases of cardiopulmonary resuscitation within 2 years in 1 study. 30
The studies varied in how the defining cutoff was derived. Among the 5 studies that defined high volume as 40 or more cases of OHCA per year, 2 studies 23 , 26 adapted the cutoff values from recent studies that evaluated the impact of volume on patients with OHCA, 1 study 27 adopted the recommended annual volume of cases of OHCA proposed by the American Heart Association for CACs, 1 study 19 plotted survival against the annual number of cases of OHCA in the database for all hospitals and found the highest survival rate in the group with 40 or more cases of OHCA per year, and 1 study 31 provided no details on the derivation. Among the 3 studies that defined high volume as more than 100 cases of OHCA per year, 1 study 28 adapted the definition from previous studies, whereas the remaining 2 studies 29 , 32 did not describe how the definition was derived. The lone study that defined high volume as more than 84 cases of OHCA within 5 years derived this definition by patient data aggregation at the hospital level and calculation of the number of post–cardiac arrest episodes within each hospital. 18 The lone study that defined high volume as more than 79 cases of OHCA within 15 months 24 derived the definition by trisecting the total number of annual cases of OHCA equally into low-, medium-, and high-volume groups. The lone study that defined high volume as more than 33 cases of OHCA per year 20 derived the definition from previous research in South Korea. The lone study that defined high volume as more than 25 cases of TTM per year 33 based the definition on consensus among the investigators. The studies that defined high volume as more than 15 and more than 25 cases of OHCA per year 21 , 22 did not explain the derivation. The studies that defined high volume as more than 15.5 cases of TTM per year 25 and more than 69 cases of cardiopulmonary resuscitation within 2 years 30 conducted sensitivity analysis using the area under the receiver operating characteristic curve.
Ten studies, 18 - 24 , 30 - 32 which included 54 531 patients, reported aORs for survival to 30 days or at hospital discharge. Pooled analysis revealed an increase in survival among patients treated at high-volume centers (aOR, 1.28 [95% CI, 1.00-1.64]) ( Figure 2 ). There was high between-study heterogeneity ( I 2 = 85%).
Eleven studies, 20 - 25 , 28 - 32 which included 55 477 patients, reported crude ORs for survival to 30 days or at hospital discharge. Pooled analysis revealed a significant increase in survival among patients treated at high-volume centers (OR, 1.43 [95% CI, 1.09-1.87]). There was high between-study heterogeneity ( I 2 = 94%).
Nine studies, 18 , 22 - 27 , 29 , 33 which included 32 944 patients, reported aORs for good neurological outcomes at 30 days or at hospital discharge. Pooled analysis showed no significant difference in neurological outcomes among patients treated at high-volume centers (aOR, 0.96 [95% CI, 0.77-1.20]) ( Figure 3 ). There was high between-study heterogeneity ( I 2 = 79%).
Six studies 18 , 24 - 26 , 28 , 33 including 26 220 patients reported crude ORs for good neurological outcomes at 30 days or at hospital discharge. Pooled analysis revealed no significant difference in neurological outcomes among patients treated at high-volume centers (OR, 1.09 [95% CI, 0.88-1.35]). There was high between-study heterogeneity ( I 2 = 81%).
There was no significant difference in survival to hospital discharge or 30 days between patients treated at centers with a cutoff value for high volume of 40 or more cases of OHCA per year 19 , 23 , 24 , 28 , 31 , 32 and those treated at centers with a cutoff value for high volume of less than 40 cases of OHCA per year 18 , 20 - 22 , 25 , 30 (χ 2 1 = 2.35; P = .13) (eFigure 1 in the Supplement ). A DRMA found no significant association between center volume and survival to discharge or 30 days ( P = .84) (eFigure 2 in the Supplement ).
There was no significant difference in neurological outcomes between patients treated at centers with a cutoff value for high volume of 40 or more cases of OHCA per year 23 , 24 , 26 - 29 and those treated at centers with a cutoff value for high volume of less than 40 cases of OHCA per year 18 , 22 , 25 (χ 2 1 = 0.06; P = .81) (eFigure 1 in the Supplement ). A DRMA found no significant association between center volume and neurological outcomes at discharge or 30 days ( P = .78) (eFigure 2 in the Supplement ).
To our knowledge, this is the first systematic review and meta-analysis on the association of treatment at high-volume centers with the outcomes of patients with OHCA. The main results suggest that patients with OHCA treated at high-volume centers have improved survival compared with patients treated at low-volume centers. This survival benefit was attenuated, but remained resilient, after aORs were pooled. However, there was no association between center volume and neurological outcomes in patients with OHCA. A DRMA did not detect a dose-response association between survival or neurological outcomes at discharge or 30 days.
Regionalization of care is a proven approach in areas such as major trauma care and coronary artery disease. 7 The success of this approach has been attributed to increased familiarity of procedures, 34 experienced personnel, and well-established protocols. 35 However, evidence for regionalization in cardiac arrest care emerged more recently and has inconclusive benefits. 12 The recent 2020 ACVC position paper recommended the regionalization of patients with OHCA in CACs if local facilities are unable to deliver comprehensive post–cardiac arrest care. 7 Although the definition of CACs varies widely, it is often understood as having high annual OHCA volume and the capability to deliver a bundle of interventions. 8 Our finding of improved survival in high-volume centers supports this recommendation. However, closer inspection of the studies that reported aORs revealed that PCI, extracorporeal membrane oxygenation, and TTM capabilities were not adjusted for by most studies. Hence, the survival benefit of high annual OHCA volume may have been confounded by the aforementioned factors. For example, Callaway et al 19 found that PCI capability and center volume of 40 or more annual cases of OHCA resulted in higher survival, but none were independent factors in determining survival.
Interestingly, we did not find evidence of better neurological outcomes in patients with OHCA treated at high-volume centers. The narrow final pooled 95% CIs further suggest that even if an association was found, as in the case of survival to discharge and survival to 30 days, it would unlikely be a large one. Given that a previous meta-analysis by Yeo et al 8 found better survival and neurological outcomes in patients with OHCA treated at CACs, volume may not be directly associated with survival, and other components in the CAC bundle of interventions such as the availability of 24/7 access to PCI, TTM, and protocolized care in the intensive care unit contribute more to the benefit of CACs. This may be supported by the finding by Yeo et al 8 that the effect of CACs, while significant, was attenuated when a sensitivity analysis with high-volume centers only was conducted. Instead of volume-based regionalization, it may be prudent for the regionalization of cardiac arrest care to focus on other aspects of postresuscitation care such as the availability of advanced treatment modalities, structured algorithms of care, and rehabilitation. Future studies investigating the effect of high-volume centers on patients with OHCA may consider adjusting for other components of post–cardiac arrest care.
Contrary to the statement made by the ACVC in their 2020 position paper that treatment of at least 40 patients with OHCA per year was associated with improved outcomes, we did not find significant subgroup differences across both survival and neurological outcomes between studies that defined high volume as 40 or more vs less than 40 cases of OHCA per year. This may be because the cutoff value was based on a 2010 study by Callaway et al, 19 who derived the value by plotting survival against the annual number of cases of OHCA treated by each hospital, although their study was not intended or designed to study threshold effects and to recommend a threshold. Therefore, caution should be exercised when adopting the cutoff of 40 or more annual cases of OHCA. Future studies may consider using standardized methods to determine individualized cutoff volumes, which can then be meta-analyzed to derive a universal cutoff value.
The DRMA found a lack of dose-response association between center volume and survival or neurological outcomes in patients with OHCA. This may be due to the inclusion of studies in which participating centers had access to the aforementioned bundle of interventions, regardless of center volume, because low volume does not mean limited resources. 24 It is also possible that insufficient statistical power had prevented the detection of a dose-response association, even if one was present. Finally, our DRMA can only be interpreted for the range of dosage represented, and findings cannot be extrapolated beyond this range.
Overall, we found that treatment at high-volume centers was associated with better survival outcomes but not neurological outcomes, which is becoming relatively important in cardiac arrest care compared with survival alone. 36 Although our findings certainly do not support depriving patients with OHCA of care at CACs, it is important to highlight the potential pitfalls of using high volume as a key factor in deciding where patients with OHCA should be transported, as well as adopting 40 or more annual cases of OHCA as a cutoff for high volume.
To our knowledge, this is the first systematic review and meta-analysis to assess the benefits of high-volume centers in the treatment of OHCA involving OHCA registries and databases from various nations and a large sample of 82 769 patients. Although the inclusion of studies from various geographical locations may increase the generalizability of our findings, it may have led to the high statistical heterogeneity. The inclusion of studies with varying definitions of high volume and approaches to analyzing volume may also have contributed to the high clinical heterogeneity. All included studies were nonrandomized studies of intervention, which are inherently susceptible to selection and observation biases. High-quality randomized clinical trials are needed to confirm the present findings, although this may be ethically challenging. Although we reduced the effect of confounding through pooling estimates from adjusted analysis, there remains the possibility of residual confounding arising from individual studies. Insufficient studies performed subgroup analysis according to prehospital Utstein Formula of Survival variables 37 , 38 such as initial shockable rhythm or prehospital return of spontaneous circulation, which precluded subgroup analyses to determine differences in OHCA outcomes in different subpopulations treated at high-volume centers. Long-term neurological and functional outcomes were not reported in the literature and therefore could not be assessed.
In this meta-analysis and systematic review, treatment of patients with OHCA at a high-volume center was associated with improved survival but not improved neurological outcomes at hospital discharge or 30 days. More high-quality studies are needed to evaluate the relative importance of center volume compared with other variables in post–cardiac arrest care such as PCI and TTM as an independent variable associated with survival outcomes in patients with OHCA. Future studies should also determine the volume range at which a measurable effect on survival or neurological outcomes can be observed.
Accepted for Publication: April 13, 2022.
Published: May 31, 2022. doi:10.1001/jamanetworkopen.2022.14639
Open Access: This is an open access article distributed under the terms of the CC-BY License . © 2022 Goh AXC et al. JAMA Network Open .
Corresponding Author: Andrew Fu Wah Ho, MBBS, MMed, MPH, Department of Emergency Medicine, Singapore General Hospital, Outram Road, Singapore 169608 ( [email protected] ).
Author Contributions: Ms Goh had full access to all the data in the study and takes responsibility for the integrity of the data and the accuracy of the data analysis. Ms Goh, Msrrs Seow and Yeo, and Dr Andrew Fu Wah Ho contributed equally to this study.
Concept and design: A.X.C. Goh, Ong, Lim, Yeo, A.F.W. Ho.
Acquisition, analysis, or interpretation of data: A.X.C. Goh, Seow, Lai, Liu, Y.M. Goh, Ong, J.S.Y. Ho, A.F.W. Ho.
Drafting of the manuscript: A.X.C. Goh, Seow, Lai, Y.M. Goh, Yeo, A.F.W. Ho.
Critical revision of the manuscript for important intellectual content: A.X.C. Goh, Seow, Lai, Liu, Ong, Lim, J.S.Y. Ho, Yeo, A. Ho.
Statistical analysis: A. Goh, Seow, Yeo, A.F.W. Ho.
Obtained funding: Ong, A.F.W. Ho.
Administrative, technical, or material support: A.X.C. Goh, Seow, Lai, Liu, Ong, J.S.Y. Ho, A.F.W. Ho.
Supervision: A.X.C. Goh, Ong, A.F.W. Ho.
Conflict of Interest Disclosures: None reported.
- Register for email alerts with links to free full-text articles
- Access PDFs of free articles
- Manage your interests
- Save searches and receive search alerts
Information
- Author Services
Initiatives
You are accessing a machine-readable page. In order to be human-readable, please install an RSS reader.
All articles published by MDPI are made immediately available worldwide under an open access license. No special permission is required to reuse all or part of the article published by MDPI, including figures and tables. For articles published under an open access Creative Common CC BY license, any part of the article may be reused without permission provided that the original article is clearly cited. For more information, please refer to https://www.mdpi.com/openaccess .
Feature papers represent the most advanced research with significant potential for high impact in the field. A Feature Paper should be a substantial original Article that involves several techniques or approaches, provides an outlook for future research directions and describes possible research applications.
Feature papers are submitted upon individual invitation or recommendation by the scientific editors and must receive positive feedback from the reviewers.
Editor’s Choice articles are based on recommendations by the scientific editors of MDPI journals from around the world. Editors select a small number of articles recently published in the journal that they believe will be particularly interesting to readers, or important in the respective research area. The aim is to provide a snapshot of some of the most exciting work published in the various research areas of the journal.
Original Submission Date Received: .
- Active Journals
- Find a Journal
- Proceedings Series
- For Authors
- For Reviewers
- For Editors
- For Librarians
- For Publishers
- For Societies
- For Conference Organizers
- Open Access Policy
- Institutional Open Access Program
- Special Issues Guidelines
- Editorial Process
- Research and Publication Ethics
- Article Processing Charges
- Testimonials
- Preprints.org
- SciProfiles
- Encyclopedia
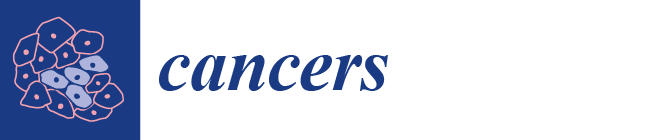
Article Menu
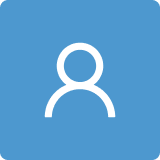
- Subscribe SciFeed
- Google Scholar
- on Google Scholar
- Table of Contents
Find support for a specific problem in the support section of our website.
Please let us know what you think of our products and services.
Visit our dedicated information section to learn more about MDPI.
JSmol Viewer
Limited evidence for the benefits of exercise in older adults with hematological malignancies: a systematic review and meta-analysis.
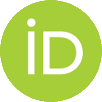
Simple Summary
1. introduction, 2. materials and methods, 2.1. information sources and search strategy, 2.2. eligibility criteria, 2.3. data collection process, 2.4. outcomes, 2.5. risk of bias assessment, 2.6. certainty of evidence, 2.7. data synthesis and analysis, 3.1. study characteristics, 3.2. exercise interventions, 3.3. effects of exercise interventions on primary outcomes, 3.4. effects of exercise interventions on secondary outcomes, 3.5. feasibility, adverse events, adherence and exclusion criteria, 3.6. risk of bias in individual studies and across studies, 3.7. quality of evidence (grade), 3.8. ongoing studies registered in clinical trials, 4. discussion, strengths and limitations, 5. conclusions, supplementary materials, author contributions, institutional review board statement, informed consent statement, acknowledgments, conflicts of interest.
- Fitzmaurice, C.; Akinyemiju, T.F.; Al Lami, F.H.; Alam, T.; Alizadeh-Navaei, R.; Allen, C.; Alsharif, U.; Alvis-Guzman, N.; Amini, E.; Anderson, B.O.; et al. Global, Regional, and National Cancer Incidence, Mortality, Years of Life Lost, Years Lived With Disability, and Disability-Adjusted Life-Years for 29 Cancer Groups, 1990 to 2016: A Systematic Analysis for the Global Burden of Disease Study. JAMA Oncol. 2019 , 5 , 1749–1768. [ Google Scholar ]
- Zhang, N.; Wu, J.; Wang, Q.; Liang, Y.; Li, X.; Chen, G.; Ma, L.; Liu, X.; Zhou, F. Global burden of hematologic malignancies and evolution patterns over the past 30 years. Blood Cancer J. 2023 , 13 , 82. [ Google Scholar ] [ CrossRef ] [ PubMed ]
- Howlader, N.A.; Krapcho, M.; Miller, D.; Brest, A.; Yu, M.; Ruhl, J.; Tatalovich, Z.; Mariotto, A.; Lewis, D.R.; Chen, H.S.; et al. (Eds.) SEER Cancer Statistics Review, 1975–2018, National Cancer Institute. Bethesda, MD. Based on November 2020 SEER Data Submission, Posted to the SEER Web Site. Available online: https://seer.cancer.gov/csr/1975_2018/ (accessed on 21 April 2021).
- Krok-Schoen, J.L.; Fisher, J.L.; Stephens, J.A.; Mims, A.; Ayyappan, S.; Woyach, J.A.; Rosko, A.E. Incidence and survival of hematological cancers among adults ages ≥75 years. Cancer Med. 2018 , 7 , 3425–3433. [ Google Scholar ] [ CrossRef ]
- Cordoba, R.; A Eyre, T.; Klepin, H.D.; Wildes, T.M.; Goede, V. Haematological Malignancies in Older People 1 A comprehensive approach to therapy of haematological malignancies in older patients. Lancet Haematol. 2021 , 8 , E840–E852. [ Google Scholar ] [ CrossRef ]
- Handforth, C.; Clegg, A.; Young, C.; Simpkins, S.; Seymour, M.T.; Selby, P.J.; Young, J. The prevalence and outcomes of frailty in older cancer patients: A systematic review. Ann. Oncol. 2015 , 26 , 1091–1101. [ Google Scholar ] [ CrossRef ] [ PubMed ]
- Cheng, K.K.; Lee, D.T. Effects of pain, fatigue, insomnia, and mood disturbance on functional status and quality of life of elderly patients with cancer. Crit. Rev. Oncol. Hematol. 2011 , 78 , 127–137. [ Google Scholar ] [ CrossRef ]
- Su, W.Y.E.; Chen, H.; Wu, M.; Lai, Y. Fatigue among older advanced cancer patients. Int. J. Gerontol. 2011 , 5 , 84–88. [ Google Scholar ] [ CrossRef ]
- Soones, T.; Ombres, R.; Escalante, C. An update on cancer-related fatigue in older adults: A narrative review. J. Geriatr. Oncol. 2022 , 13 , 125–131. [ Google Scholar ] [ CrossRef ] [ PubMed ]
- Wall, S.A.; Stevens, E.; Vaughn, J.; Bumma, N.; Rosko, A.E.; Borate, U. Multidisciplinary Approach to Older Adults with Hematologic Malignancies—A Paradigm Shift. Curr. Hematol. Malign- Rep. 2022 , 17 , 31–38. [ Google Scholar ] [ CrossRef ]
- Coelho, A.; Parola, V.; Cardoso, D.; Bravo, M.E.; Apóstolo, J. Use of non-pharmacological interventions for comforting patients in palliative care: A scoping review. JBI Database Syst. Rev. Implement Rep. 2017 , 15 , 1867–1904. [ Google Scholar ] [ CrossRef ]
- Abdelbasset, W.K.; Nambi, G.; Elsayed, S.H.; Osailan, A.M.; Eid, M.M. Falls and potential therapeutic interventions among elderly and older adult patients with cancer: A systematic review. Afr. Health Sci. 2021 , 21 , 1776–1783. [ Google Scholar ] [ CrossRef ] [ PubMed ]
- Brick, R.; Turner, R.; Bender, C.; Douglas, M.; Eilers, R.; Ferguson, R.; Leland, N.; Lyons, K.D.; Toto, P.; Skidmore, E. Impact of non-pharmacological interventions on activity limitations and participation restrictions in older breast cancer survivors: A scoping review. J. Geriatr. Oncol. 2022 , 13 , 132–142. [ Google Scholar ] [ CrossRef ] [ PubMed ]
- Cardoso, C.S.; Matos, J.R.; Prazeres, F.; Gomes, B. Non-pharmacological interventions in primary care to improve the quality of life of older patients with palliative care needs: A systematic review of randomised controlled trials. BMJ Open 2023 , 13 , e073950. [ Google Scholar ] [ CrossRef ]
- Pedersen, M.; Engedal, M.S.; Tolver, A.; Larsen, M.T.; Kornblit, B.T.; Lomborg, K.; Jarden, M. Effect of non-pharmacological interventions on symptoms and quality of life in patients with hematological malignancies—A systematic review. Crit. Rev. Oncol. 2024 , 196 , 104327. [ Google Scholar ] [ CrossRef ]
- Yang, Y.-P.; Pan, S.-J.; Qiu, S.-L.; Tung, T.-H. Effects of physical exercise on the quality-of-life of patients with haematological malignancies and thrombocytopenia: A systematic review and meta-analysis. World J. Clin. Cases 2022 , 10 , 3143–3155. [ Google Scholar ] [ CrossRef ]
- Großek, A.; Großek, K.; Bloch, W. Safety and feasibility of exercise interventions in patients with hematological cancer undergoing chemotherapy: A systematic review. Support. Care Cancer 2023 , 31 , 335. [ Google Scholar ] [ CrossRef ]
- Knips, L.; Bergenthal, N.; Streckmann, F.; Monsef, I.; Elter, T.; Skoetz, N. Aerobic physical exercise for adult patients with haematological malignancies. Cochrane Database Syst. Rev. 2019 , 2019 , CD009075. [ Google Scholar ] [ CrossRef ]
- Xu, W.; Yang, L.; Wang, Y.; Wu, X.; Wu, Y.; Hu, R. Effects of exercise interventions for physical fitness, fatigue, and quality of life in adult hematologic malignancy patients without receiving hematopoietic stem cell transplantation: A systematic review and meta-analysis. Support. Care Cancer 2022 , 30 , 7099–7118. [ Google Scholar ] [ CrossRef ] [ PubMed ]
- Abo, S.; Denehy, L.; Ritchie, D.; Lin, K.-Y.; Edbrooke, L.; McDonald, C.; Granger, C.L. People With Hematological Malignancies Treated With Bone Marrow Transplantation Have Improved Function, Quality of Life, and Fatigue Following Exercise Intervention: A Systematic Review and Meta-Analysis. Phys. Ther. 2021 , 101 , pzab130. [ Google Scholar ] [ CrossRef ]
- Moore, M.; Northey, J.M.; Crispin, P.; Semple, S.; Toohey, K. Effects of Exercise Rehabilitation on Physical Function in Adults With Hematological Cancer Receiving Active Treatment: A Systematic Review and Meta-Analysis. Semin. Oncol. Nurs. 2023 , 39 , 151504. [ Google Scholar ] [ CrossRef ]
- Izquierdo, M.; Merchant, R.A.; Morley, J.E.; Anker, S.D.; Aprahamian, I.; Arai, H.; Aubertin-Leheudre, M.; Bernabei, R.; Cadore, E.L.; Cesari, M.; et al. International Exercise Recommendations in Older Adults (ICFSR): Expert Consensus Guidelines. J. Nutr. Health Aging 2021 , 25 , 824–853. [ Google Scholar ] [ CrossRef ]
- Chodzko-Zajko, W.J.; Proctor, D.N.; Fiatarone Singh, M.A.; Minson, C.T.; Nigg, C.R.; Salem, G.J.; Skinner, J.S. Exercise and Physical Activity for Older Adults. Med. Sci. Sports Exerc. 2009 , 41 , 1510–1530. [ Google Scholar ] [ CrossRef ] [ PubMed ]
- Kilari, D.; Soto-Perez-De-Celis, E.; Mohile, S.G.; Alibhai, S.M.; Presley, C.J.; Wildes, T.M.; Klepin, H.D.; Demark-Wahnefried, W.; Jatoi, A.; Harrison, R.; et al. Designing exercise clinical trials for older adults with cancer: Recommendations from 2015 Cancer and Aging Research Group NCI U13 Meeting. J. Geriatr. Oncol. 2016 , 7 , 293–304. [ Google Scholar ] [ CrossRef ] [ PubMed ]
- Campbell, K.L.; Winters-Stone, K.M.; Wiskemann, J.; May, A.M.; Schwartz, A.L.; Courneya, K.S.; Zucker, D.S.; Matthews, C.E.; Ligibel, J.A.; Gerber, L.H.; et al. Exercise Guidelines for Cancer Survivors: Consensus Statement from International Multidisciplinary Roundtable. Med. Sci. Sports Exerc. 2019 , 51 , 2375–2390. [ Google Scholar ] [ CrossRef ] [ PubMed ]
- Klepin, H.D.; Mohile, S.G.; Exterman, S.M.M.; Karger, S. (Eds.) Cancer and Aging: From Bench to Clinics. Exercise for Older Cancer Patients: Feasible and Helpful? 2012; Available online: https://karger.com/books/book/241/chapter-abstract/5166165/Exercise-for-Older-Cancer-Patients-Feasible-and?redirectedFrom=PDF (accessed on 11 July 2024).
- Higgins, J.P.T.; Chandler, J.; Cumpston, M.; Li, T.; Page, M.J.; Welch, V.A.; Cochrane (Eds.) Cochrane Handbook for Systematic Reviews of Interventions Version 6.4 ; 2023; Available online: https://training.cochrane.org/handbook (accessed on 11 July 2024).
- Page, M.J.; McKenzie, J.E.; Bossuyt, P.M.; Boutron, I.; Hoffmann, T.C.; Mulrow, C.D.; Shamseer, L.; Tetz-laff, J.M.; Akl, E.A.; Brennan, S.E.; et al. The PRISMA 2020 statement: An updated guideline for reporting systematic reviews. BMJ 2021 , 372 , 71. [ Google Scholar ] [ CrossRef ]
- Rethlefsen, M.L.; Kirtley, S.; Waffenschmidt, S.; Ayala, A.P.; Moher, D.; Page, M.J.; Koffel, J.B.; PRISMA-S Group. PRISMA-S: An extension to the PRISMA Statement for Reporting Literature Searches in Systematic Reviews. Syst. Rev. 2021 , 10 , 39. [ Google Scholar ] [ CrossRef ]
- Schardt, C.; Adams, M.B.; Owens, T.; Keitz, S.; Fontelo, P. Utilization of the PICO framework to improve searching PubMed for clinical questions. BMC Med. Inform. Decis. Mak. 2007 , 7 , 16. [ Google Scholar ] [ CrossRef ] [ PubMed ]
- Sterne, J.A.C.; Savović, J.; Page, M.J.; Elbers, R.G.; Blencowe, N.S.; Boutron, I.; Cates, C.J.; Cheng, H.Y.; Corbett, M.S.; Eldridge, S.M.; et al. RoB 2: A revised tool for assessing risk of bias in randomised trials. BMJ 2019 , 366 , l4898. [ Google Scholar ] [ CrossRef ]
- Ryan, R.H.S. How to GRADE the Quality of the Evidence. Cochrane Consumers and Communication Group Version 3.0. Available online: http://cccrg.cochrane.org/author-resources (accessed on 1 January 2020).
- Garber, C.E.; Blissmer, B.; Deschenes, M.R.; Franklin, B.A.; LaMonte, M.J.; Lee, I.-M.; Nieman, D.C.; Swain, D.P. American College of Sports Medicine position stand. Quantity and quality of exercise for developing and maintaining cardiorespiratory, musculoskeletal, and neuromotor fitness in apparently healthy adults: Guidance for prescribing exercise. Med. Sci. Sport. Exerc. 2011 , 43 , 1334–1359. [ Google Scholar ] [ CrossRef ]
- Andersen, H.H.; Vinther, A.; Lund, C.M.; Paludan, C.; Jørgensen, C.T.; Nielsen, D.; Juhl, C.B. Effectiveness of different types, delivery modes and extensiveness of exercise in patients with breast cancer receiving systemic treatment—A systematic review and meta-analysis. Crit. Rev. Oncol. 2022 , 178 , 103802. [ Google Scholar ] [ CrossRef ]
- Ramírez-Vélez, R.; Zambom-Ferraresi, F.; García-Hermoso, A.; Kievisiene, J.; Rauckiene-Michealsson, A.; Agostinis-Sobrinho, C. Evidence-Based Exercise Recommendations to Improve Mental Wellbeing in Women with Breast Cancer during Active Treatment: A Systematic Review and Meta-Analysis. Cancers 2021 , 13 , 264. [ Google Scholar ] [ CrossRef ] [ PubMed ]
- Accogli, M.A.; Denti, M.; Costi, S.; Fugazzaro, S. Therapeutic education and physical activity are feasible and safe in hematologic cancer patients referred to chemotherapy: Results of a randomized controlled trial. Support. Care Cancer 2022 , 31 , 61. [ Google Scholar ] [ CrossRef ]
- Alibhai, S.M.H.; O’neill, S.; Fisher-Schlombs, K.; Breunis, H.; Timilshina, N.; Brandwein, J.M.; Minden, M.D.; Tomlinson, G.A.; Culos-Reed, S.N. A pilot phase II RCT of a home-based exercise intervention for survivors of AML. Support. Care Cancer 2014 , 22 , 881–889. [ Google Scholar ] [ CrossRef ]
- Alibhai, S.; Durbano, S.; Breunis, H.; Brandwein, J.; Timilshina, N.; Tomlinson, G.; Oh, P.; Culos-Reed, S. A phase II exercise randomized controlled trial for patients with acute myeloid leukemia undergoing induction chemotherapy. Leuk. Res. 2015 , 39 , 1178–1186. [ Google Scholar ] [ CrossRef ] [ PubMed ]
- Baumann, F.T.; Kraut, L.; Schüle, K.; Bloch, W.; A Fauser, A. A controlled randomized study examining the effects of exercise therapy on patients undergoing haematopoietic stem cell transplantation. Bone Marrow Transplant. 2010 , 45 , 355–362. [ Google Scholar ] [ CrossRef ]
- Baumann, F.T.; Zopf, E.M.; Nykamp, E.; Kraut, L.; Schüle, K.; Elter, T.; Fauser, A.A.; Bloch, W. Physical activity for patients undergoing an allogeneic hematopoietic stem cell transplantation: Benefits of a moderate exercise intervention. Eur. J. Haematol. 2011 , 87 , 148–156. [ Google Scholar ] [ CrossRef ] [ PubMed ]
- Bayram, S.; Barğı, G.; Çelik, Z.; Güçlü, M.B. Effects of pulmonary rehabilitation in hematopoietic stem cell transplantation recipients: A randomized controlled study. Support. Care Cancer 2024 , 32 , 72. [ Google Scholar ] [ CrossRef ]
- Bird, L.; Arthur, A.; Niblock, T.; Stone, R.; Watson, L.; Cox, K. Rehabilitation programme after stem cell transplantation: Randomized controlled trial. J. Adv. Nurs. 2010 , 66 , 607–615. [ Google Scholar ] [ CrossRef ]
- Bryant, A.L.; Deal, A.M.; Battaglini, C.L.; Phillips, B.; Pergolotti, M.; Coffman, E.; Foster, M.C.; Wood, W.A.; Bailey, C.; Hackney, A.C.; et al. The Effects of Exercise on Patient-Reported Outcomes and Performance-Based Physical Function in Adults With Acute Leukemia Undergoing Induction Therapy: Exercise and Quality of Life in Acute Leukemia (EQUAL). Integr. Cancer Ther. 2018 , 17 , 263–270. [ Google Scholar ] [ CrossRef ]
- Chang, P.-H.; Lai, Y.-H.; Shun, S.-C.; Lin, L.-Y.; Chen, M.-L.; Yang, Y.; Tsai, J.-C.; Huang, G.-S.; Cheng, S.-Y. Effects of a Walking Intervention on Fatigue-Related Experiences of Hospitalized Acute Myelogenous Leukemia Patients Undergoing Chemotherapy: A Randomized Controlled Trial. J. Pain Symptom Manag. 2008 , 35 , 524–534. [ Google Scholar ] [ CrossRef ]
- Chen, F.; Mao, L.; Wang, Y.; Xu, J.; Li, J.; Zheng, Y. The Feasibility and Efficacy of Self-help Relaxation Exercise in Symptom Distress in Patients With Adult Acute Leukemia: A Pilot Randomized Controlled Trial. Pain Manag. Nurs. 2021 , 22 , 791–797. [ Google Scholar ] [ CrossRef ] [ PubMed ]
- Chow, E.J.; Doody, D.R.; Di, C.; Armenian, S.H.; Baker, K.S.; Bricker, J.B.; Gopal, A.K.; Hagen, A.M.; Ketterl, T.G.; Lee, S.J.; et al. Feasibility of a behavioral intervention using mobile health applications to reduce cardiovascular risk factors in cancer survivors: A pilot randomized controlled trial. J. Cancer Surviv. 2021 , 15 , 554–563. [ Google Scholar ] [ CrossRef ] [ PubMed ]
- Chuang, T.-Y.; Yeh, M.-L.; Chung, Y.-C. A nurse facilitated mind-body interactive exercise (Chan-Chuang qigong) improves the health status of non-Hodgkin lymphoma patients receiving chemotherapy: Randomised controlled trial. Int. J. Nurs. Stud. 2017 , 69 , 25–33. [ Google Scholar ] [ CrossRef ] [ PubMed ]
- Cohen, L.; Warneke, C.; Fouladi, R.T.; Rodriguez, M.A.; Chaoul-Reich, A. Psychological adjustment and sleep quality in a randomized trial of the effects of a Tibetan yoga intervention in patients with lymphoma. Cancer 2004 , 100 , 2253–2260. [ Google Scholar ] [ CrossRef ] [ PubMed ]
- Coleman, E.A.; Coon, S.; Hall-Barrow, J.; Richards, K.; Gaylor, D.; Stewart, B. Feasibility of Exercise During Treatment for Multiple Myeloma. Cancer Nurs. 2003 , 26 , 410–419. [ Google Scholar ] [ CrossRef ]
- Coleman, E.A.; Goodwin, J.A.; Kennedy, R.; Coon, S.K.; Richards, K.; Enderlin, C.; Stewart, C.B.; McNatt, P.; Lockhart, K.; Anaissie, E.J. Effects of Exercise on Fatigue, Sleep, and Performance: A Randomized Trial. Oncol. Nurs. Forum 2012 , 39 , 468–477. [ Google Scholar ] [ CrossRef ]
- Courneya, K.S.; Sellar, C.M.; Stevinson, C.; McNeely, M.L.; Peddle, C.J.; Friedenreich, C.M.; Tankel, K.; Basi, S.; Chua, N.; Mazurek, A.; et al. Randomized Controlled Trial of the Effects of Aerobic Exercise on Physical Functioning and Quality of Life in Lymphoma Patients. J. Clin. Oncol. 2009 , 27 , 4605–4612. [ Google Scholar ] [ CrossRef ]
- DeFor, T.E.; Burns, L.J.; Gold, E.-M.A.; Weisdorf, D.J. A Randomized Trial of the Effect of a Walking Regimen on the Functional Status of 100 Adult Allogeneic Donor Hematopoietic Cell Transplant Patients. Biol. Blood Marrow Transplant. 2007 , 13 , 948–955. [ Google Scholar ] [ CrossRef ]
- Eckert, R.; Huberty, J.; Kurka, J.; Laird, B.; Mesa, R.; Palmer, J. A Randomized Pilot Study of Online Hatha Yoga for Physical and Psychological Symptoms Among Survivors of Allogenic Bone Marrow Transplant. Int. J. Yoga Ther. 2022 , 32 , 12. [ Google Scholar ] [ CrossRef ]
- Furzer, B.J.; Ackland, T.R.; Wallman, K.E.; Petterson, A.S.; Gordon, S.M.; Wright, K.E.; Joske, D.J.L. A randomised controlled trial comparing the effects of a 12-week supervised exercise versus usual care on outcomes in haematological cancer patients. Support. Care Cancer 2016 , 24 , 1697–1707. [ Google Scholar ] [ CrossRef ]
- Gallardo-Rodríguez, A.G.; Fuchs-Tarlovsky, V.; Ocharán-Hernández, M.E.; Ramos-Peñafiel, C.O. Cross-Training and Resistance Training in Adults with Type B Acute Lymphoblastic Leukemia during the Induction Phase: A Randomized Blind Pilot Study. J. Clin. Med. 2023 , 12 , 5008. [ Google Scholar ] [ CrossRef ] [ PubMed ]
- Hacker, E.D.; Collins, E.; Park, C.; Peters, T.; Rondelli, D. Strength training to enhance early recovery after hematopoietic stem cell transplantation. J. Clin. Oncol. 2016 , 34 , 190. [ Google Scholar ] [ CrossRef ]
- Hacker, E.D.P.; Richards, R.L.; Abu Zaid, M.; Chung, S.-Y.; Perkins, S.; Farag, S.S. STEPS to Enhance Physical Activity After Hematopoietic Cell Transplantation for Multiple Myeloma. Cancer Nurs. 2022 , 45 , 211–223. [ Google Scholar ] [ CrossRef ]
- Hathiramani, S.; Pettengell, R.; Moir, H.; Younis, A. Relaxation versus exercise for improved quality of life in lymphoma survivors—A randomised controlled trial. J. Cancer Surviv. 2021 , 15 , 470–480. [ Google Scholar ] [ CrossRef ]
- Huberty, J.; Eckert, R.; Dueck, A.; Kosiorek, H.; Larkey, L.; Gowin, K.; Mesa, R. Online yoga in myeloproliferative neoplasm patients: Results of a randomized pilot trial to inform future research. BMC Complement. Altern. Med. 2019 , 19 , 121. [ Google Scholar ] [ CrossRef ]
- Hung, Y.-C.; Bauer, J.D.; Horsely, P.; Coll, J.; Bashford, J.; A Isenring, E. Telephone-delivered nutrition and exercise counselling after auto-SCT: A pilot, randomised controlled trial. Bone Marrow Transplant. 2014 , 49 , 786–792. [ Google Scholar ] [ CrossRef ] [ PubMed ]
- Jacobsen, P.B.; Le-Rademacher, J.; Jim, H.; Syrjala, K.; Wingard, J.R.; Logan, B.; Wu, J.; Majhail, N.S.; Wood, W.; Rizzo, J.D.; et al. Exercise and Stress Management Training Prior to Hematopoietic Cell Transplantation: Blood and Marrow Transplant Clinical Trials Network (BMT CTN) 0902. Biol. Blood Marrow Transplant. 2014 , 20 , 1530–1536. [ Google Scholar ] [ CrossRef ]
- Jarden, M.; Baadsgaard, M.T.; Hovgaard, D.J.; Boesen, E.; Adamsen, L. A randomized trial on the effect of a multimodal intervention on physical capacity, functional performance and quality of life in adult patients undergoing allogeneic SCT. Bone Marrow Transplant. 2009 , 43 , 725–737. [ Google Scholar ] [ CrossRef ]
- Jarden, M.; Møller, T.; Christensen, K.B.; Kjeldsen, L.; Birgens, H.S.; Adamsen, L. Multimodal intervention integrated into the clinical management of acute leukemia improves physical function and quality of life during consolidation chemotherapy: A randomized trial ‘PACE-AL. Haematologica 2016 , 101 , e316–e319. [ Google Scholar ] [ CrossRef ]
- Kim, S.-D.; Kim, H.-S. Effects of a Relaxation Breathing Exercise on Anxiety, Depression, and Leukocyte in Hemopoietic Stem Cell Transplantation Patients. Cancer Nurs. 2005 , 28 , 79–83. [ Google Scholar ] [ CrossRef ]
- Knols, R.H.; de Bruin, E.D.; Uebelhart, D.; Aufdemkampe, G.; Schanz, U.; Stenner-Liewen, F.; Hitz, F.; Taverna, C.; Aaronson, N.K. Effects of an outpatient physical exercise program on hematopoietic stem-cell transplantation recipients: A randomized clinical trial. Bone Marrow Transplant. 2011 , 46 , 1245–1255. [ Google Scholar ] [ CrossRef ] [ PubMed ]
- Kobayashi, D.; Watanabe, R.; Yamamoto, M.; Kizaki, M. Efficacy of physical exercise using the balance board game on physical and psychological function in patients with hematological malignancies confined to a bioclean room. Phys. Ther. Res. 2020 , 23 , 172–179. [ Google Scholar ] [ CrossRef ] [ PubMed ]
- Koutoukidis, D.A.; Land, J.; Hackshaw, A.; Heinrich, M.; McCourt, O.; Beeken, R.J.; Philpott, S.; DeSilva, D.; Rismani, A.; Rabin, N.; et al. Fatigue, quality of life and physical fitness following an exercise intervention in multiple myeloma survivors (MASCOT): An exploratory randomised Phase 2 trial utilising a modified Zelen design. Br. J. Cancer 2020 , 123 , 187–195. [ Google Scholar ] [ CrossRef ]
- McCourt, O.; Fisher, A.; Ramdharry, G.; Land, J.; Roberts, A.L.; Rabin, N.; Yong, K. Exercise prehabilitation for people with myeloma undergoing autologous stem cell transplantation: Results from PERCEPT pilot randomised controlled trial. Acta Oncol. 2023 , 62 , 696–705. [ Google Scholar ] [ CrossRef ]
- Mello, M.; Tanaka, C.; Dulley, F.L. Effects of an exercise program on muscle performance in patients undergoing allogeneic bone marrow transplantation. Bone Marrow Transplant. 2003 , 32 , 723–728. [ Google Scholar ] [ CrossRef ]
- Oechsle, K.; Aslan, Z.; Suesse, Y.; Jensen, W.; Bokemeyer, C.; de Wit, M. Multimodal exercise training during myeloablative chemotherapy: A prospective randomized pilot trial. Support. Care Cancer 2014 , 22 , 63–69. [ Google Scholar ] [ CrossRef ]
- Pahl, A.; Wehrle, A.; Kneis, S.; Gollhofer, A.; Bertz, H. Feasibility of whole body vibration during intensive chemotherapy in patients with hematological malignancies—A randomized controlled pilot study. BMC Cancer 2018 , 18 , 920. [ Google Scholar ] [ CrossRef ]
- Pahl, A.; Wehrle, A.; Kneis, S.; Gollhofer, A.; Bertz, H. Whole body vibration training during allogeneic hematopoietic cell transplantation—The effects on patients’ physical capacity. Ann. Hematol. 2020 , 99 , 635–648. [ Google Scholar ] [ CrossRef ] [ PubMed ]
- Persoon, S.; ChinAPaw, M.J.M.; Buffart, L.M.; Liu, R.D.K.; Wijermans, P.; Koene, H.R.; Minnema, M.C.; Lugtenburg, P.J.; Marijt, E.W.A.; Brug, J.; et al. Randomized controlled trial on the effects of a supervised high intensity exercise program in patients with a hematologic malignancy treated with autologous stem cell transplantation: Results from the EXIST study. PLoS ONE 2017 , 12 , e0181313. [ Google Scholar ] [ CrossRef ]
- Potiaumpai, M.; Cutrono, S.; Medina, T.; Koeppel, M.; Pereira, D.L.; Pirl, W.F.; Jacobs, K.A.; Eltoukhy, M.; Signorile, J.F. Multidirectional Walking in Hematopoietic Stem Cell Transplant Patients. Med. Sci. Sports Exerc. 2021 , 53 , 258–266. [ Google Scholar ] [ CrossRef ]
- Safran, E.E.; Mutluay, F.; Uzay, A. Effects of neuromuscular electrical stimulation combined with resistance exercises on muscle strength in adult hematological cancer patients: A randomized controlled study. Leuk. Res. 2022 , 121 , 106932. [ Google Scholar ] [ CrossRef ] [ PubMed ]
- Schumacher, H.; Stüwe, S.; Kropp, P.; Diedrich, D.; Freitag, S.; Greger, N.; Junghanss, C.; Freund, M.; Hilgendorf, I. A prospective, randomized evaluation of the feasibility of exergaming on patients undergoing hematopoietic stem cell transplantation. Bone Marrow Transplant. 2018 , 53 , 584–590. [ Google Scholar ] [ CrossRef ] [ PubMed ]
- Shelton, M.L.; Lee, J.Q.; Morris, G.S.; Massey, P.R.; Kendall, D.G.; Munsell, M.F.; Anderson, K.O.; Simmonds, M.J.; Giralt, S.A. A randomized control trial of a supervised versus a self-directed exercise program for allogeneic stem cell transplant patients. Psycho-Oncol. 2009 , 18 , 353–359. [ Google Scholar ] [ CrossRef ]
- Streckmann, F.; Kneis, S.; Leifert, J.A.; Baumann, F.T.; Kleber, M.; Ihorst, G.; Herich, L.; Grüssinger, V.; Gollhofer, A.; Bertz, H. Exercise program improves therapy-related side-effects and quality of life in lymphoma patients undergoing therapy. Ann. Oncol. 2014 , 25 , 493–499. [ Google Scholar ] [ CrossRef ] [ PubMed ]
- Vallerand, J.R.; Rhodes, R.E.; Walker, G.J.; Courneya, K.S. Feasibility and preliminary efficacy of an exercise telephone counseling intervention for hematologic cancer survivors: A phase II randomized controlled trial. J. Cancer Surviv. 2018 , 12 , 357–370. [ Google Scholar ] [ CrossRef ]
- Waked, I.S. A Randomized Controlled Trial of the Effects of Supervised Aerobic Training Program on Anthropometry, Lipid Profile and Body Composition in Obese Adult Leukemic Patients. Iran. J. Blood Cancer 2019 , 11 , 26–32. [ Google Scholar ]
- Wehrle, A.; Kneis, S.; Dickhuth, H.-H.; Gollhofer, A.; Bertz, H. Endurance and resistance training in patients with acute leukemia undergoing induction chemotherapy—A randomized pilot study. Support. Care Cancer 2019 , 27 , 1071–1079. [ Google Scholar ] [ CrossRef ]
- Wiskemann, J.; Dreger, P.; Schwerdtfeger, R.; Bondong, A.; Huber, G.; Kleindienst, N.; Ulrich, C.M.; Bohus, M. Effects of a partly self-administered exercise program before, during, and after allogeneic stem cell transplantation. Blood 2011 , 117 , 2604–2613. [ Google Scholar ] [ CrossRef ]
- Wood, W.A.; Weaver, M.; Smith-Ryan, A.E.; Hanson, E.D.; Shea, T.C.; Battaglini, C.L. Lessons learned from a pilot randomized clinical trial of home-based exercise prescription before allogeneic hematopoietic cell transplantation. Support. Care Cancer 2020 , 28 , 5291–5298. [ Google Scholar ] [ CrossRef ]
- Yeh, M.-L.; Chung, Y.-C. A randomized controlled trial of qigong on fatigue and sleep quality for non-Hodgkin’s lymphoma patients undergoing chemotherapy. Eur. J. Oncol. Nurs. 2016 , 23 , 81–86. [ Google Scholar ] [ CrossRef ]
- Jarden, M.; Møller, T.; Christensen, K.B.; Buchardt, A.; Kjeldsen, L.; Adamsen, L. Longitudinal symptom burden in adult patients with acute leukaemia participating in the PACE-AL randomised controlled exercise trial—An explorative analysis. Eur. J. Cancer Care 2021 , 30 , e13462. [ Google Scholar ] [ CrossRef ]
- Wiskemann, J.; Kuehl, R.; Dreger, P.; Schwerdtfeger, R.; Huber, G.; Ulrich, C.M.; Jaeger, D.; Bohus, M. Efficacy of exercise training in SCT patients-who benefits most? Bone Marrow Transplant. 2014 , 49 , 443–448. [ Google Scholar ] [ CrossRef ] [ PubMed ]
- Knowles, R.; Kemp, E.; Miller, M.; Davison, K.; Koczwara, B. Physical activity interventions in older people with cancer: A review of systematic reviews. Eur. J. Cancer Care 2022 , 31 , e13637. [ Google Scholar ] [ CrossRef ]
- Mikkelsen, M.K.; Juhl, C.B.; Lund, C.M.; Jarden, M.; Vinther, A.; Nielsen, D.L. The effect of exercise-based interventions on health-related quality of life and physical function in older patients with cancer receiving medical antineoplastic treatments: A systematic review. Eur. Rev. Aging Phys. Act. 2020 , 17 , 18. [ Google Scholar ] [ CrossRef ] [ PubMed ]
- Sheill, G.; Guinan, E.; Brady, L.; Hevey, D.; Hussey, J. Exercise interventions for patients with advanced cancer: A systematic review of recruitment, attrition, and exercise adherence rates. Palliat. Support. Care 2019 , 17 , 686–696. [ Google Scholar ] [ CrossRef ] [ PubMed ]
- Allen, N.E.; Sherrington, C.; Suriyarachchi, G.D.; Paul, S.S.; Song, J.; Canning, C.G. Exercise and motor training in people with Parkinson’s disease: A systematic review of participant characteristics, intervention delivery, retention rates, adherence, and adverse events in clinical trials. Park. Dis. 2011 , 2012 , 854328. [ Google Scholar ] [ CrossRef ] [ PubMed ]
- Jansons, P.S.; Haines, T.P.; O’brien, L. Interventions to achieve ongoing exercise adherence for adults with chronic health conditions who have completed a supervised exercise program: Systematic review and meta-analysis. Clin. Rehabil. 2017 , 31 , 465–477. [ Google Scholar ] [ CrossRef ]
- Larsen, R.F.; Jarden, M.; Minet, L.R.; Frølund, U.C.; Abildgaard, N. Supervised and home-based physical exercise in patients newly diagnosed with multiple myeloma-a randomized controlled feasibility study. Pilot Feasibility Stud. 2019 , 5 , 130. [ Google Scholar ] [ CrossRef ]
- Rosko, A.; Huang, Y.; Jones, D.; Presley, C.J.; Jaggers, J.; Owens, R.; Naughton, M.; Krok-Schoen, J.L. Feasibility of implementing an exercise intervention in older adults with hematologic malignancy. J. Geriatr. Oncol. 2022 , 13 , 234–240. [ Google Scholar ] [ CrossRef ]
- Mazzoni, A.-S.; Brooke, H.L.; Berntsen, S.; Nordin, K.; Demmelmaier, I. Exercise Adherence and Effect of Self-Regulatory Behavior Change Techniques in Patients Undergoing Curative Cancer Treatment: Secondary Analysis from the Phys-Can Randomized Controlled Trial. Integr. Cancer Ther. 2020 , 19 , 1534735420946834. [ Google Scholar ] [ CrossRef ]
- Collado-Mateo, D.; Lavín-Pérez, A.; Peñacoba, C.; Del Coso, J.; Leyton-Román, M.; Luque-Casado, A.; Gasque, P.; Fernández-Del-Olmo, M.; Amado-Alonso, D. Key Factors Associated with Adherence to Physical Exercise in Patients with Chronic Diseases and Older Adults: An Umbrella Review. Int. J. Environ. Res. Public Health 2021 , 18 , 2023. [ Google Scholar ] [ CrossRef ] [ PubMed ]
- Colton, A.; Smith, M.A.; Broadbent, S.; Rune, K.T.; Wright, H.H. Perceptions of Older Adults with Hematological Cancer on Diet and Exercise Behavior and Its Role in Navigating Daily Tasks. Int. J. Environ. Res. Public Health 2022 , 19 , 15044. [ Google Scholar ] [ CrossRef ] [ PubMed ]
Click here to enlarge figure
Author, Year, Country | Diagnose | Study Design | Sample Size, n IG/CG Female (%) | Age Mean, (Range) Median, (Range) | Exclusion Criteria | Timing of Intervention | Intervention Group | Control Group | |
---|---|---|---|---|---|---|---|---|---|
Type: T Intensity: I Length: L Duration: D | Extensiveness | Type: T Intensity: I Length: L Duration: D | |||||||
Accogli 2022 Italy [ ] | Lymph, Leuk, MM | RCT | 46 (23/23) (47.8) | Mean 59.9 Median IG: 66.7 (51.3–72.1) CG: 60.4 (49.9–67.5) | Poor prognosis (<12 months) and clinical conditions hindering participation (e.g., dementia, psychiatric pathology, blindness) | Before, during and after chemotherapy | Supervised, hospital-based: T: Therapeutic education I: Individual intensity L: 2 × 60 min (group) and face-to-face (individual) 6 × 20 min 1x/week or every 2 weeks Unsupervised, homebased: T: Individual physical exercise D: 8 weeks | Less | T: Educational therapeutic group sessions L: 2x in total |
Alibhai 2014 Canada [ ] | AML | RCT Feasibility | 38 (21/17) (55.3) | Mean 56.1 IG: 53.9 CG: 58.8 | Another active malignancy, life expectancy < 3 months, severe or unstable cardiorespiratory or musculoskeletal disease, awaiting HSCT and regular participation in a moderate-vigorous PA program | After HSCT or chemotherapy | Supervised, hospital-based: T: Workout and education (group) L: 1.5 h/week Unsupervised, home-based: T: Aerobic, resistance and flexibility components I: Moderate intensity L: 30 min, 3–5x/week D: 12 weeks | Moderate | T: UC, Usual level of PA |
Alibhai 2015 Canada [ ] | AML | RCT | 81 (57/24) (45.7) | Mean 57 (23–80) IG: 58 CG: 52 Median 59 >60: IG, n = 32 CG, n = 7 | Another active malignancy, life expectancy < 1-month, significant medical comorbidity that would preclude exercise, uncontrolled pain, hemo-dynamic instability | During chemotherapy | , hospital-based: T: Individualized aerobic (treadmill, hall walking, stationary cycling), resistance (body weight, bands, free weights) and flexibility training I: RPE of 3–6, equivalent to 50–75% of HRR L: 30–60 min, 4–5x/week | Moderate | T: UC, Suggestions to walk regularly and weekly document on tracking sheets |
Baumann 2010 Germany [ ] | AML, ALL, CML, MM, NHL/CLL, MDS/MPS, Solid tumor, Immuno-deficiency | RCT Pilot | 64 32/32 (45.4) | Mean IG: 44.9 CG: 44.1 | Severe orthopaedic illness of legs, severe heart failure (NYHA III-IV), metastatic bone disease, thrombocytopenia (≤30 × 10 /L) and/or acute somatic complaint (e.g., infection, fever, acute bleeding) | During ASCT, allo-HSCT or chemotherapy | hospital-based: T: Aerobic (ergometer) and ADL training (walking, stepping, stretching) I: Aerobic: 80% of achieved watt load in WHO-test, ADL-training: Borg scale: “slighty strenuous”-“strenuous” L: Aerobic 10–20 min + ADL 20 min/2x daily, ADL 5x/week D: Mean: 26.6 days | Moderate | T: UC, Standard mobilization program |
Baumann 2011 Germany [ ] | AML ALL, CML, CLL, MPS, MDS, CMML, MM, PID | RCT | 47 (24/23) (51.5) | Mean IG: 41.4 CG: 42.8 | Severe cardiac disease (NYHA III-IV) or orthopaedic illness of the legs, bone metastases, thrombocytopenia (≤10 × 10 /L) or acute bleeding, respectively, and/or acute health or somatic complaints (e.g., infection, fever) | During allo-HSCT | hospital-based: T: Aerobic (ergometer) and ADL training (walking, stepping, stretching) I: Aerobic: 80% of achieved watt load in WHO-test, ADL-training: Borg scale: “slighty strenuous”-”strenuous L: Aerobic: 10–20 min + ADL 20 min, 1–2x/day D: Mean: 56.1 days | Moderate | T: UC, Standard PT |
Bayram 2024 Turkey [ ] | ALL, AML, Biphenotypic Leuk, MDS, NHL, Burkitt Lymph, CNS Lymph, Myelofibrosis, Thalassemia major, MM | RCT | 30 (15/15) (26.7) | Mean IG: 45.67 CG: 52.07 | Orthopaedic, neurological, or cognitive disease affecting functional capacity, psychiatric disorders, pneumonia, acute infections, sepsis, and pulmonary diseases | During HSCT | hospital-based: T: Aerobic (arm ergometer), resistance (free weights) and inspiratory muscle (inspiratory pressure device) exercises I: Aerobic: 50–80% of HR. Resistance: 4–6 on modified Borg scale, 3 sets of 10 reps. Inspiratory: 30% of max inspiratory pressure L: Aerobic: 10–30 min, 1x/day, 5 days/week. Resistance: 10–15 min, 5 days/week. Inspiratory: 15 min, 2x/day, 5 days/week D: During inpatient period. Mean: 25.2 days | Extensive | T: Aerobic and resistance exercises I: As IG L: As IG D: During inpatient period. Mean: 21.33 days |
Bird 2010 UK [ ] | Leuk, Lymph, MM, other | RCT | 58 (29/29) (34.5) | Median 55 IG: 57 CG:52 | NR | After ASCT or allo-HSCT | hospital-based: T: Circuit training exercise, relaxation, and information (group) I: NR L: 1x/week D: 10 weeks | Less | T: UC, Self-managed program: information leaflets and home-based exercise program |
Bryant 2018 USA [ ] | AML, ALL | RCT Pilot | 18 (9/9) (29.4) | Mean IG: 52 (34–67) CG: 49 (28–69) Median IG: 58 (34–67) CG: 48 (28–69) | Cardiovasc. disease, acute or chronic respiratory disease, acute or chronic bone, muscle, or joint abnormalities, altered mental state, dementia or any other psychological condition, another active malignancy, active bleeding, acute thrombosis, ischemia, hemodynamic instability, or uncontrolled pain | During chemotherapy | hospital-based: T: Aerobic (walking or stationary bike) and resistance training (resistance band) I: Aerobic: 50–70% of HRR. Resistance: Increased from lighter to heavier resistance, 10 RM L: 20–40 min, 2x/day, 4x/week D: 4 weeks | Moderate | T: UC |
Chang 2008 Taiwan [ ] | AML | RCT | 24 (12/12) (45.5) | Mean IG: 49.4 CG: 53.3 | NR | During chemotherapy | hospital-based: T: Walking exercise program I: A speed to reach target HR (resting heart rate plus 30) L: 12 min, 5x/week D: 3 weeks | Less | T: Nurse-led control L/D: 1x/day, 5 days/week, 3 weeks |
Chen 2021 China [ ] | AML, ALL | RCT Pilot | 30 (15/15) (58.6) | Mean IG: 40.2 CG: 37.6 | Medical conditions in arms, legs, or abdomen, paralysis, or disability and intended to receive HSCT in the next 3 months | During chemotherapy | , hospital-based T: Individualized self-help relaxation exercises I: NR L: 30 min, 2x daily D: 4 weeks | Less | T: UC |
Chow 2020 USA [ ] | Leuk, Lymph, other | RCT Pilot | 41 (24/17) (48.8) | Median 45.1 (20.2–54.8) IG: 44.0 (20.9–54.0) CG: 46.0 (20.2–54.8) | Pre-existing ischemic heart disease or ongoing symptomatic cardiomyopathy, active cGvHD, pregnant | After ASCT, allo-HSCT | , home-based: T: Individualized, multiple mHealth app-based lifestyle counselling and goal-setting intervention, step count goals based on the past week’s daily average steps I: NR L: 16 weeks | Less | T: Fitbit tracker and Healthwatch360 app, no goal setting or peer support |
Chuang 2017 Taiwan [ ] | NHL | RCT | 100 (50/50) (45.0) | Mean IG: 55.9 CG: 64.5 | Major medical disease, MM, or bone metastasis with medical contra-indications for exercise and already practicing qigong or other exercise regular | During chemotherapy | , home-based: T: Chan-Chuang qigong program with weekly telephone calls I: NR L: 25 min, 2–3x/day D: 21 consecutive days | Less | T: UC, Nursing on side effects of chemotherapy and care |
Cohen 2004 USA [ ] | Lymph, HL, NHL | RCT | 39 (20/19) (30.8) | Mean 51 | Major psychotic illness, <18 years | During and after chemotherapy | , hospital-based: T: Group-based Tibetan yoga program I: NR L: 7x/week D: 7 weeks | Less | T: UC |
Coleman 2003 USA [ ] | MM | RCT Pilot Feasibility | 24 (14/10) (41.7) | Mean 55 (42–74) | NR | During chemotherapy and ASCT | , home-based: T: Aerobic (walking, running, or cycling) and strength training (exercise bands), exercise log I: Borg Scale 12–15 L: Approx. 50 min, individual frequency D: 26 weeks | Moderate | T: UC, Encouragement to remain active and walk |
Coleman 2012 USA [ ] | MM | RCT | 187 (95/92) (41.7) | Mean IG: 56.0 (25–76) CG: 56.4 (35–76) | Unable to understand intent of the study, major psychiatric illness, or presence of microcytic or macrocytic anaemia, uncontrolled hypertension, RBC transfusions within two weeks of study enrolment, or recombinant epoetin alfa within eight weeks of study enrolment | During unspecified intensive treatment (PBSCT) | , home-based: T: Individualized combination of stretching, aerobic exercise (walk, jog on treadmills) and strength resistance training (exercise bands), exercise log I: Aerobic:65–80% max HR, Borg Scale 11–13. Strength: 60–80% of 1 RM L: Individual length and frequency D: 15 weeks | Moderate | T: UC, Recommendation to walk L: 20 min, 3x/week |
Courneya 2009 Canada [ ] | Lymph, NHL indolent, NHL aggressive, HL | RCT | 122 (60/62) (41) | Mean 53.2 (18–80) >60: n = 49 | Uncontrolled hypertension, cardiac illness, resides >80 km from facility, not approved by oncologist | Before, during and after chemotherapy | , hospital-based: T: Aerobic (ergometer) I: Initial 60% of VO peak, progressing 75% L: 15–45 min, 3x/week D: 12 weeks | Moderate | T: UC, Supervised exercise L: 12 sessions, 1 month, after postintervention assessments |
Defor 2007 USA [ ] | AA, ALL, AML, MDS, CML, NHL/HL, other malignancies | RCT | 100 (51/49) (39.0) | Median 47 (18–68) IG: 46 (18–68) CG: 49 (22–64) | Unavailable treadmills at hospital admission (n = 21 excluded) | During and after allo-HSCT From transplant admission to day 100 posttransplant | Supervised, hospital-based: T: Individualized treadmill I: Comfortable speed L: 15 min, 2x/day , homebased: T: Walking I: Comfortable speed L: 30 min, 1x/day | Moderate | T: UC, Not asked to perform any formal exercise |
Eckert 2022 USA [ ] | BMT patients | RCT Feasibility | 72 (33/39) (55.6) | NR | Engaged in yoga in past year, history of recurrent falls (>two falls in 2 months), residency outside USA, participation in a previous study with the research team, ECOG 3 questionnaire score > 3, pregnant | After ASCT | , home-based: T: Online Hatha yoga program I: NR L: Min. 60 min/week D: 12 weeks | Less | T: Online cancer health education podcasts L: 60 min/week D: 12 weeks |
Furzer 2016 Australia [ ] | NHL, HL, MM | RCT | 37 (18/19) (NR) | Mean 48.9 (22–68) IG: 48.2 (22–64) CG: 49.6 (25–68) | Hematologist did not approve exercise due to identified risks | After chemotherapy or radiation or HSCT | , in local gyms and clinics: T: Aerobic (individual) and resistance training (machines and dumbbells) I: Cardio: 50–85% of HRmax, RPE of 10–16. Resistance: Initial 3 sets of 10–15 rep at 50% of 1 RM to 2–3 sets of 6–8 rep at 80% of 1 RM L: Max. 30 min, 3x/week D: 12 weeks | Moderate | T: Diary and general healthy lifestyle advice |
Gallardo-Rodriquez 2023 Mexico [ ] | ALL | RCT Pilot 3-arm | 33 (11/11/11) (66.7) | Mean 23.7 (18–45) CEG: 20.5 (18–36) REG: 22.5 (18–36) CG: 28.0 (18–45) | Neutropenia, infections, bleeding at admission, were nonmotile or unable to carry out exercise; with a CNS disease preventing movement, alterations of heart function, with bone marrow or CNS relapse, with a referral from another hospital | During chemotherapy treatment | , hospital- and home-based: T: Cross-training or resistance (weights) exercises I: RPE of 3–6 (50–75% of HRR), 3–5 sets of 8–15 reps L: 30–50 min, 3–5x/week D: During inpatient period | Moderate | T: Mobilization I: Low L: 30 min, daily |
Hacker 2017 USA [ ] | ALL, AML, CLL, CML, HL, NHL, MM, MDS | RCT | 67 (33/34) (38.8) | Mean 53.3 IG: 51.9 CG: 54.6 | Significant comorbidity, like impending pathological fracture, making exercise potentially unsafe | During and after ASCT or allo-HSCT | Supervised, hospital-based: T: Progressive resistance training (elastic resistance bands and body weights) I: Moderate intensity, Borg scale 13 L: 2–3x/week, D: During inpatient period Unsupervised, home-based: T: Progressive resistance training (elastic resistance bands and body weights) I: Moderate intensity, Borg scale 13 L: 2–3x/week D: 6 weeks after discharge | Moderate | T: UC, Attention control with health education |
Hacker 2022 USA [ ] | MM | RCT Pilot | 32 (17/15) (34.4) | Mean 62.78 IG: 62.21 CG: 63.44 | NR | After ASCT and after discharge | Supervised, hospital-based: T: Weekly goal setting, daily step tracking, and individualized coaching I: NR L: Daily Unsupervised, home-based: T: Free-living PA, step trackers I: NR L: Daily D: 6 weeks | Moderate | T: UC, Recommendations regarding rest, PA, and exercise |
Hathiramani 2020 UK [ ] | Lymph | RCT | 46 (23/23) (63) | Mean 61 IG: 61.5 CG: 60.4 | Active disease, unstable angina or unexplained electrocardiogram, poor PS (ECOG 3 or more), pregnancy, difficulty breathing at rest, persistent cough, fever or illness, or any cognitive impairment limiting the ability to give informed consent or complete questionnaires | During and after chemotherapy | , home-based: T: Individual elements of aerobic (walking), resistance training (resistance bands, body weight), core stability and stretches I: Aerobic: Moderate intensity Resistance: ACSM guidelines with 3 sets for 8–12 rep L: 50 min, 3x/week D: 12 weeks | Moderate | T: Bed or chair-based program, mindfulness-based. CD audio guidance to relaxation techniques: mindfulness meditation, breathing exercises, guided visualization and progressive muscle relaxation. I: No advice to exercise outside of normal habits, nor asked to avoid activity L: 50 min, 3x/week |
Huberty 2019 USA [ ] | MPN: Polycythaemia Vera, Essential, Thrombocythemia, Myelofibrosis | RCT Pilot | 62 (34/28) (93.7) | Mean 56.9 IG: 58.3 CG: 55.0 | Reported performing tai chi, qi gong, or yoga for ≥60 min/week, reported engaging in ≥150 min/week of PA, utilized the study’s online yoga site: Udaya.com, (accessed on 21 August 2024) syncope in the last two months, recurrent falls: ≥2 in past two months, score of ≥15 on the PHQ-9, score of >3 on the ECOG-3, pregnant, residency outside USA | During or after chemotherapy | , home-based: T: Online homebased Hatha/Vinyasa yoga I: NR L: 5–30 min, 60 min/week D: 12 weeks | Less | T: UC, Maintain usual activity |
Hung 2014 Australia [ ] | Lymph, ML | RCT Pilot | 37 (18/19) (46) | Mean IG: 57.5 CG: 59.9 | Undergoing allo-HSCT, deemed unsuitable for study participation by physicians | After ASCT | , home-based: T: Individual telephone-delivered nutrition and exercise counselling, unsupervised aerobic (walking or cycling) and resistance (sit-to-stand or free weight) I: Recommendations based on ACSM guidelines for cancer survivors L: Various length, 3–7x/week D: 12 weeks | Moderate | T: UC |
Jacobsen 2014 USA [ ] | ALL, CML, CLL, MDS, MM, Lymph | RCT 4-arm | 711 (180/178/178/175) (43) | Median IG E: 58 (20–76) IG SM: 58 (20–75) IG E/SM: 57 (18–75) CG: 55 (19–76) >65, n = 154 (21.6%) | Orthopaedic, neurological, or other problems that prevented safe ambulation or protocol adherence, participation in another clinical trial with QoL or functional status as a primary endpoint, planned anticancer therapies other than tyrosine kinase inhibitor or rituximab within 100 days after HSCT, planned donor lymphocyte infusion within 100 days after HSCT, planned tandem transplantation | Before, during and after allo-HSCT or ASCT | , home-based: T: Self-directed exercise program, a DVD reinforcing the program, tracking of participation in exercise and/or stress management. Exercise component: Calculation of target HR and pedometer. The stress management component also included provision of a relaxation CD I: 50–75% of estimated HRR L: 20–30 min, 3–5x/week D: 180 dayS | Moderate | T: DVD with general instruction about HSCT L: 45 min |
Jarden 2009 Denmark [ ] | CML, AML, ALL, AA, MDS, WM, PNH, MF | RCT | 42 (21/21) (38.1) | Mean 39.2 (18–60) IG: 40.9 (18–60) CG: 37.4 (18–55) Median 40.5 IG: 45.0 CG: 38.0 | Prior HSCT, recent cardiovascular, or pulmonary disease, abnormal EKG, psychiatric disorder, and motor, musculoskeletal or neurological dysfunction requiring walking aids and bony metastasis. Prior to testing: Signs of infection, anaemia, neutropenia, or thrombocytopenia, disqualified or testing postponed | During allo-HSCT | , hospital-based: T: Multimodal program of aerobic (ergometer), resistance (machines and weights) and active exercises, progressive relaxation, and psychoeducation I: Aerobic: Low to moderate, 50–75% HR max. RPE: 10–13. Dynamic and stretching 1–2 sets, 10–12 reps. Static: 1 set, hold for 15–30 s. Resistance: Low to moderate, 1–2 sets of 10–12 reps. Progressive relaxation: low L: 60–70 min, 3–5x/week D: 4–6 weeks | Moderate | T: UC, Conventional treatment and care, standard care for PA, PT is individualized, not providing a stationary cycle unless requested L: PT < 1½ hour/week, after allogeneic HSCT (day +1) |
Jarden 2016 Denmark [ ] Jarden 2021 Denmark [ ] | Acute Leuk, AML de novo, AML following MDS, APL, ALL | RCT | 70 (34/36) (41.4) | Mean 53.1 (19.8–73.7) IG: 51.1 (19.8–70.0) CG: 55.0 (20.3–73.7) | Severe or unstable psychological, cardio-respiratory, neurological, or musculoskeletal disease, secondary active malignancy, abnormal EKG | During chemotherapy | , hospital-based: T: Multimodal intervention of aerobic (ergometer), strength (weights) and relaxation exercise, nutrition support, pedometer, and health counselling I: Aerobic: 75–80% of HRmax. Dynamic resistance: Moderate to hard, 2 sets, 12 reps L: 60 min, 3x/week D: 12 weeks | Extensive | T: UC |
Kim 2005 South Korea [ ] | AML, ALL, SAA | RCT | 35 (18/17) (51.4) | Mean IG: 32.9 CG: 34.3 (20–48) | Medicated for anxiety or depression | After allo-HSCT | , hospital-based: T: Individual physical exercises combined with relaxation breathing I: NR L: 30 min/daily D: 6 weeks | Less | T: UC, Routine care |
Knols 2011 Switzerland [ ] | AML, CLL, ALL, HL, NHL, MM, Osteo-myelofibrosis, Leuk, Amyloidosis, Testicular C. | RCT | 131 64/67 (41.2) | Mean 46.7 IG: 46.6 (18–75) CG: 46.6 (20–67) | GvHD except for grade I not requiring treatment, painful joints, unstable osteolysis, chronic pain, lesions of the central or peripheral nervous system, uncontrolled cardiovascular disease, thyroid disease, or diabetes | After allo-HSCT or ASCT | , physiotherapy practice or fitness centre: T: Individual, physical exercises with both endurance aerobic (ergometer or walking tread mill) and resistance strength (machines and dumbbells) exercises I: Individual HR (from 50–60%, increasing to 70–80% of estimated HR max) L: 30 min, 2x/week D: 12 weeks | Moderate | T: UC |
Kobayashi 2020 Japan [ ] | AML, DLBCL, ALL | RCT Crossover | 33 (13/20) (18.2) | Mean Wii PT/Therapist PT: 44.9 Therapist PT/Wii PT: 44.6 | Grade 2 or worse CTC for Adverse Events version 4.0 | During chemotherapy | , hospital-based: T: Individual aerobic and resistance exercises using the Wii Fit balance board I: NR L: 30 min, 5x/week D: 1 week (and then crossover) | Less | T: Individual aerobic and resistance exercises. I: Aerobic: 40–60%. Resistance: Borg 11–13 L: 30 min, 5x/week D: 1 week and then crossover |
Koutoukidis 2020 UK [ ] | MM, Myeloma IgG, Myeloma IgA, Myeloma Light chain, non-sec/oligo-sec. | RCT | 131 (89/42) (45) | Median IG: 64 (35–86) CG: 63 (40–80) | Spinal instability. Recent spinal or other surgery for pathological fractures within 4 weeks. Abnormal EKG with unexplained clinical indication after cardiological work-up. At risk of pathological fracture based on Mirel’s score. Currently enrolled in research exercise study. Unstable angina. Musculoskeletal mobility limitations. Cognitive impairment hindering completion of questionnaire | After auto-HSCT, radiotherapy or chemotherapy | Supervised, hospital-based: T: Individual aerobic (treadmill walking, ergometer, cross-trainer or stepping) and resistance (weightlifting, body weight or resistance bands) training, exercise diaries, goal setting with physiotherapist I: Aerobic: 50–75% of predicted HR max-Resistance: 10 RM L: 1x/week D: 6 months Unsupervised, home-based: T: Individual aerobic training and resistance training, exercise diaries, goal setting with PT I: Aerobic: 50–75% of predicted HR max. Resistance: 10 RM L: Max 30 min, 2–3x/week D: 6 months | Moderate | T: UC |
McCourt 2023 UK [ ] | MM | RCT Pilot | 50 (23/27) (38) | Mean 60.4 (37–72) IG: 59.3 (37–72) CG: 61.3 (40–72) | Declined or not suitable for auto-HSCT or too close to transplantation, restricted mobility, non-English language | Before, during and after ASCT | Supervised, hospital-based: T: Aerobic (treadmill walking or ergometer) and resistance (machines and resistance bands) exercise and behaviour change support I: Aerobic: 60–80% of HRR. Resistance: 10 RM and individually tailored to progress and/or adapt to bone disease. L: 1x/week Unsupervised, home-based: T: Aerobic (walking) and resistance (resistance bands) exercise and behaviour change support, virtual I: Aerobic: 60–80% of HRR. Resistance: 10 RM and individually tailored to progress and/or adapt to bone disease L: Aerobic exercise (Phase 1 and 3): 15–40 min, 3x/week. Resistance exercise (Phase 1 and 3): 3x/week. During phase 2 (transplant admission) | Extensive | T: UC |
Mello 2003 Brazil [ ] | CML, AML, SAA, NHL, MDS | RCT | 18 (9/9) (55.6) | Mean IG: 27.9 (18–39) CG: 30.2 (18–44) | NR | During and after allo-HSCT | , hospital-based: T: Individualized exercise program with active exercise, muscle stretching and treadmill walking I: Progressing, no higher than 70% of HR max L: 40 min, 5x/week D: 6 weeks | Moderate | NR |
Oechsle 2014 Germany [ ] | AML, NHL, MM, Germ cell | RCT Pilot | 58 (29/29) (29.2) | Mean IG: 51.7 CG: 52.9 | Symptomatic cardiovascular diseases, tumor infiltration of the skeletal system with risk of pathologic fractures or compression of spinal cord, epilepsy, rheumatologic diseases, BMI < 18, BMI > 30, insufficient cognitive function, inadequate knowledge of German language for questionnaire analysis | During chemotherapy | , hospital-based: T: Aerobic (ergometer) and strength (body weights and resistance bands) training I: Ergometer individually adjusted Strength training: Up to 20 min at 40–60% of estimated 1 RM, sets of 16–25 repetitions L: 30–40 min, 5x/week D: Median: 21 days | Moderate | T: UC, Standard PT |
Pahl 2018 Germany [ ] | Leuk, AML, ALL, APL, NHL, HL, T-cell lymph, WM, MM, PMF | RCT Pilot | 17 (10/7) (30) | Median 55 (47–63) IG: 47 (19–62) CG: 56 (32–63) | Unstable bone metastasis, knee or hip endoprosthesis, epilepsy, pacemaker, severe cardiovascular disease and threshold blood-count values below safety criteria, stents, or former joint injuries | During chemotherapy | , hospital-based: T: Whole body vibration (Galileo Sport vibration platform), including three sets of two to four different exercises (body weight) I: Borg scale 14–16 L: 20 min, 3x/week D: Median: 27 days | Moderate | T: Aerobic exercise; ergometer I: Borg scale 14–16 L: 20 min. |
Pahl 2020 Germany [ ] | AML, ALL, CLL, CMML, MDS, Lymph, MM, MF, Septic granulomatosis Immuno-deficiency, SAA | RCT | 44 (18/26) (31.8) | Median IG: 55 (50–63) CG: 56 (32–63) | Unstable bone metastasis, endoprosthesis of knee or hip, epilepsy, pacemaker, and severe cardiovascular disease | During allo-HSCT | , hospital-based: T: Whole body vibration (Galileo Sport vibration platform) I: NR L: 20 min, 5x/week D: 35–44 days | Moderate | T: Mobilization and stretching L: 5x/week |
Persoon 2017 Netherlands [ ] | MM, (N)HL, Lymph | RCT | 109 (54/55) (36.7) | Median 55 (19–67) IG: 53.5 (20–67) CG: 56 (19–67) | NR | After ASCT | , at local physiotherapy practices: T: Aerobic interval (cycling) and resistance (machines) training, counselling sessions (5x) I: Resistance: High intensity. Week 1–12 2 × 10 rep at 65–80% of 1 RM, week 12–18 2 × 20 rep at 35–40% of 1 RM L: 60 min, 1–2x/week D: 18 weeks | Extensive | T: UC, Not encouraged to exercise, participate in sports, PT, or rehabilitation programs |
Potiaumpai 2021 USA [ ] | AML, ALL, CML, MDS, MM, other Lymph | RCT | 35 (19/16) (45.7) | Mean 58.8 IG: 59.3 CG: 58.2 | Dementia, altered mental status, severe psychiatric conditions, pre-existing comorbid conditions that would contraindicate exercise testing, concurrent non-transplant-related chemotherapy, or radiation | Before and after allo-HSCT or ASCT | , hospital-based: T: Multidirectional drills and walking program I: Exertion level of moderate intensity during the multidirectional drills and a high intensity during the walking portion L: 5–30 min, 3x/weeks D: Varied | Moderate | T: UC |
Safran 2022 Turkey [ ] | AML, B-cell ALL, T-cell ALL, MDS, NHL, MF | RCT | 43 (21/22) (51.6) | Mean IG: 38 (23–63) CG: 40.5 (24–58) | <18 years, ECOG > 3, comorbidities causing fatigue (e.g., multiple sclerosis, Parkinson’s disease, heart failure), rapid deterioration of general condition (sudden uncontrolled weight loss, confused consciousness, high CRP values), brain metastases or metastases to the femur, DVT within last 6 months, neuropathy, and rejecting NMES intervention or exercise therapy | During chemotherapy, after allo-HSCT | , hospital-based: T: Resistance exercise (body weights and resistance bands) combined with neuromuscular electrical stimulation I: Borg scale: Initial recommended RPE is 12–13 and is increased to about 15–16. The intensity was adjusted to a target score of 12–14 (moderate level) using the RPE scale. Intensity (~RPE 15–16) and resistance were gradually increased L: 60–90 min, 2–3x/week D: 4 weeks | Moderate | T: Resistance exercise L: 40–60 min, 2–3 days/week |
Schumacher 2018 Germany [ ] | MM, AML/MDS, NHL Teratoma, CML, CLL | RCT feasibility | 42 (19/23) (40.5) | Median IG: 56.0 (21–65) CG: 56.5 (21–65) | Lack of compliance. Intercurrent diseases, like pulmonary and cardiac insufficiency or uncontrolled infections | During and after allo-HSCT or ASCT | , hospital-based: T: Exergaming on Nintendo Wii for exercising ping pong, tennis, boxing, frisbee, or aerobics and balance I: NR L: 30 min, 5x/week D: During and 30 days post HSCT | Moderate | T: PT program, eccentric and concentric movements, from supine to standing, walking, stepping or treadmill walking, stretching, strength exercise i.e., elastic bands and body weight |
Shelton 2009 USA [ ] | Lymph, Leuk | RCT | 53 (26/27) (37.7) | Mean IG: 43.7 (22–68) CG: 48.9 (29–70) | <18 years, psychiatric disorder, significant cardiovascular disease, paraplegic or hemiplegic, unable to speak or understand English | After allo-HSCT | , hospital-based: T: Aerobic (treadmill and ergometer) and resistance (weights and machines) exercises I: Aerobic: 60–75% of age-predicted HR max. Strength: 1–3 sets of 10 reps L: 20–30 min aerobic, resistance individual, 3x/week D: 4 weeks | Moderate | T: multidisciplinary, inpatient, educational session incl. focus on staying active, information to exercise safely |
Streckmann 2014 Germany [ ] | HL, B-NHL, T-NHL, MM | RCT | 56 (28/28) (25) | Mean IG: 44 (20–67) CG: 48 (19–73) | Unstable osteolysis, severe acute infections, severe cardiac and pulmonary impairments, restrictions for PA | During chemotherapy | , hospital-based: T: Aerobic (treadmill and ergometer), sensorimotor and strength (resistance bands) training I: Initial 60–70% HR max. At the end of session 70–80%. Sensorimotor training: Progressively increasing task difficulty. Strength training: 1 min at max force L: 60 min, 2x/week D: 36 weeks | Extensive | T: UC, Standard clinical care, incl. PT |
Vallerand 2018 Canada [ ] | Leuk., HL, NHL | RCT | 51 (26/25) (60.8) | Mean 52.6 <60: n = 33 >60: n = 18 | Chronic medical condition precluding from aerobic exercise, plan of being away from home > 2 weeks, baseline exercise levels of ≥240 min. weekly | During or after chemotherapy, radiation, HSCT | , home-based: T: Tele counselling with PA guidance with a goal of increasing aerobic exercise (walking, group fitness) levels by at least 60 min/week up to 300 min/week of moderate-vigorous aerobic exercise I: Aerobic exercise: Moderate-vigorous L: Tele-health calls: Mean: 17 min, 1x/week. Aerobic: 60–300 min/week. D: 12 weeks | Moderate | T: UC, PT guidelines, goal setting of increasing aerobic exercise levels I: Aerobic exercise: moderate-vigorous L: 60–300 min/week |
Waked 2019 Egypt [ ] | ALL | RCT | 54 (27/27) (34) | Mean IG: 33.4 CG: 32.4 | Antecedent neurological, developmental, or genetic disorder. Relapsed or secondary ALL. Received testicular, mediastinal, or craniospinal irradiation. Growth hormone insufficiency, hormone therapy. Medications that interfere with lipid metabolism. Diseases affecting cholesterol metabolism such as diabetes mellitus, thyroid dysfunction, or nephrotic syndrome | After treatment | , hospital-based: T: Aerobic training (ergometer) I: 60% of predictive age HR max L: 30–40 min, 3x/week D: 12 weeks | Moderate | T: UC, Normal daily activities |
Wehrle 2019 Germany [ ] | AML, ALL | RCT Pilot 3-arm | 29 (9/10/10) (41) | Median EG: 47.7 (21.9–63.4) RG: 47.4 (41.2–62.2) CG: 50.6 (35.0–58.1) | Karnofsky score < 60, uncontrolled hypertension, cardiac illness (NYHA III-IV), instable bone metastases, lack of informed consent after screening | During chemotherapy | , hospital-based: T: Either aerobic (ergometer or treadmill) or resistance (body weight) training I: Endurance: 60–70% of HRmax, RPE of 12–14 Resistance: RPE 12–14 L: 30–45 min, 3x/week D: 5 weeks (median) | Moderate | T: Mobilization and stretching program, I: low intensity |
Wiskemann 2011 Germany [ ] Wiskemann 2014 Germany [ ] | AML, ALL, CML, CLL, MDS, Sec. AML, MPS, MM, other Lymph, AA | RCT | 105 (52/53) (32.4) | Mean 48.8 (18–71) IG: 47.6 (18–70) CG: 50 (20–71) | NR | Before, during and after allo-HSCT | , hospital-based and home-based: T: Aerobic (ergometer/treadmill or walking) and resistance (resistance bands) exercises I: Tailored intensity. Endurance: Borg scale: 12–14. Resistance: Borg scale: 14–16 w/8–20 rep × 2–3 sets L: 20–40 min, 2–5x/week. Endurance: 3–5x/week. Resistance: 2x/week | Moderate | T/I: Recommend moderate PA, received step counters L: Same frequency of social contact as in IG. PT up to 3x/week |
Wood 2020 USA [ ] | AML, MDS, ALL, CML, HL, MM, MF, AA, MCL, HLH | RCT Pilot | 34 (17/17) (43) | Median 52 (28–73) | Transplant ineligibility, uncertain transplant candidacy, comorbid illness that would preclude maximal effort during exercise testing or participation in regular exercise determined by the treating physician or study exercise physiologist | Before allo-HSCT | , home-based: T: Aerobic exercise (walking, jogging, running, cycling, cross trainer or stair climbing) I: 80% HR max. From week 2 Interval, 2 min 80%, 3 min low recovery L: 30 min, 3–4x/week D: Mean: 11 weeks | Moderate | T: Fitbit Surge, no further instructions and information |
Yeh 2016 Taiwan [ ] | NHL | RCT | 108 (54/54) (44.1) | Mean 59.8 (23–90) | Major medical disease, as uncontrolled arrhythmia, hypertension, unstable angina, severe respiratory disease, acute infection, multiple myeloma, bone metastasis, psychiatric disorders. Medical contraindications for exercise, e.g., orthopaedic problems and neurologic or musculoskeletal disturbances, or already practicing qigong or other exercise training programs | During chemotherapy | , home-based: T: Chan-Chuang qigong exercise, guidance booklet and weekly phone call I: NR L: 20–60 min, 2–3x/day (max. 5 times). D: 3 weeks | Less | T: UC |
Outcomes | SMD (95% CI) | Participants Completed Outcome Measures, n Studies, (n) | Quality of Evidence GRADE | Comments |
---|---|---|---|---|
Physical function * 12MWT; 2MSC; 2MWT; 6MWT; Accelerometer; KPS; SWT; TUG | 0.29 (0.12–0.45) | 1219 (25) | ⨁◯◯◯ Very low | Downgraded, due to RoB (majority of trials), Inconsistency (moderate heterogeneity: 48.17%), and risk of Publication bias (Egger’s test p = 0.0516) |
Aerobic capacity Aerobic Power Index; Modified Balke; Modified endurance test; Power Max, Timed Stair Climb; VO2 Max; VO2 Max Relative; VO2 Peak; VO2 Peak modified | 0.53 (0.27–0.79) | 853 (17) | ⨁⨁◯◯ Low | Downgraded, due to Inconsistency (substantial heterogeneity: 69.21%) and risk of Publication bias (Egger’s test p = 0.0443) |
Muscle strength GRIP; Max test; Isometric Knee Extension test; STS | 0.47 (0.17–0.78) | 1091 (25) | ⨁⨁⨁◯ Moderate | Downgraded, due to Inconsistency (substantial/considerable heterogeneity: 82.59%) |
Body composition BMI; BodPod; DEXA; SECA bioimpedance, Tanita Bioelectrical impedance | 0.20 (0.03–0.37) | 654 (12) | ⨁⨁⨁⨁ High | No change |
Physical activity GLTEQ; IPAQ; PASE | 0.32 (−0.00–0.65) | 358 (5) | ⨁◯◯◯ Very low | Downgraded, due to RoB (majority of trials), Inconsistency (moderate heterogeneity: 56.97%), Imprecision (95% CI does not exclude 0), and risk of Publication bias (Egger’s test p = 0.0132) |
QoL Global * CMSAS; EORTC QLQ-C30; FACT; FACT-An; FACT-BMT; FACT-Leu; GLQOL; POMS; PROMIS | 0.34 (0.04–0.64) | 1447 (29) | ⨁⨁◯◯ Low | Downgraded, due to RoB (majority of trials) and Inconsistency (substantial/considerable heterogeneity: 87.39%) |
QoL Emotional CMSAS; EORTC QLQ-C30; FACT-General, FACT-Leu; Happiness Scale; NCCN Distress Thermometer; POMS; PROMIS; SF-12; SF-36 | 0.33 (0.05–0.60) | 1764 (28) | ⨁⨁◯◯ Low | Downgraded, due to Inconsistency (substantial/considerable heterogeneity: 86.93%) |
QoL Functional EORTC QLQ-C30; FACT; FACT-An; FACT-BMT; FACT-Leu; FACT-TOI | 0.33 (0.09–0.57) | 455 (10) | ⨁⨁◯◯ Low | Downgraded, due to RoB (majority of trials), and Inconsistency (moderate heterogeneity: 37.54%) |
QoL Physical CMSAS; EORTC QLQ-C30; FACT-An; FACT-BMT; FACT-Leu; FACT-TOI; PROMIS; SF-12; SF-36 | 0.32 (0.03–0.60) | 1731 (28) | ⨁⨁◯◯ Low | Downgraded, due to RoB (majority of trials) and Inconsistency (substantial/considerable heterogeneity: 87.72%) |
Anxiety HADS; POMS; PROMIS; STAI | 0.21 (0.13–0.55) | 917 (17) | ⨁◯◯◯ Very low | Downgraded, due to RoB (majority of trials), Inconsistency (Substantial/considerable heterogeneity: 84.23%) and Imprecision (95% CI does not exclude 0) |
Depression CES-D; HADS; POMS; PROMIS | 0.37 (0.09–0.64) | 919 (17) | ⨁◯◯◯ Very low | Downgraded, due to RoB (majority of trials), Inconsistency (substantial/considerable heterogeneity: 76.33%), and risk of Publication bias (Egger’s test p = 0.0184) |
Fatigue BFI; EORTC QLQ-C30; FACT-An; FACIT-F; FACT-F; MFI; MPN-SAF; 11-point rating scale; POMS; PROMIS; SCFS | 0.44 (0.16–0.71) | 1860 (31) | ⨁⨁◯◯ Low | Downgraded, due to RoB (majority of trials) and Inconsistency (substantial/considerable heterogeneity: 87.89%) |
Pain EORTC QLQ-C30; PROMIS; SF-36 | 0.43 (0.13–0.73) | 811 (14) | ⨁⨁◯◯ Low | Downgraded, due to RoB (majority of trials) and Inconsistency (substantial/considerable heterogeneity: 77.82%) |
Recruitment IG and CG | Retention IG and CG | Participation | Adverse Events | |||
---|---|---|---|---|---|---|
IG | IG | |||||
Author Year/Country | Sample Size Estimated, n | Eligibility Assessed, n | Included, n | Completed Post-Test, n | Adherence to Exercise (%) | AE Type, n |
Accogli [ ] 2022, Italy | 40 | 193 | 46 | 42 | 90 | No AE |
Alibhai [ ] 2014, Canada | 40 | 232 | 38 | 36 | 28 | NR |
Alibhai [ ] 2015, Canada | 72 | 264 | 81 | 70 | 54 | AE: 4 grade II musculoskeletal events |
Baumann [ ] 2010, Germany | 60 | NR | 64 | 49 | NR | NR |
Baumann [ ] 2011, Germany | 45 | NR | 47 | 33 | NR | No AE |
Bayram [ ] 2024, Turkey | 28 | 39 | 30 | 26 | 20 (IMT) | No AE |
Bird [ ] 2010, UK | 132 | 158 | 58 | 46 | NR | No AE |
Bryant [ ] 2018, USA | 30 | 82 | 18 | 17 | 80 | No AE |
Chang [ ] 2008, Taiwan | NR | 28 | 24 | 22 | NR | No AE |
Chen [ ] 2021, China | 30 | 46 | 30 | 29 | 98 | NR |
Chow [ ] 2020, USA | 41 | 420 | 41 | 37 | 75 | NR |
Chuang [ ] 2017, Taiwan | 100 | 105 | 100 | 96 | 96 | No AE |
Cohen [ ] 2004, USA | 38 | NR | 39 | 30 | 32 | NR |
Coleman [ ] 2003, USA | NR | NR | 24 | 13 | NR | No AE |
Coleman [ ] 2012, USA | 200 | NR | 187 | 166 | NR | NR |
Courneya [ ] 2009, Canada | 120 | 1306 | 122 | 117 | 92 | No SAE. AE: 3 back, hip, and knee pain |
Defor [ ] 2007, USA | NR | 122 | 100 | 85 | 24 | NR |
Eckert [ ] 2022, USA | NR | 326 | 72 | 43 | NR | No AE |
Furzer [ ] 2016, Australia | NR | 89 | 44 | 37 | 91 | No SAE. AE: 2 minor exercise modifications due to pre-existing knee and back injuries |
Gallardo-Rodriquez [ ] 2023, Mexico | 114 | 50 | 33 | 18 | NR | No (significant) AE |
Hacker [ ] 2017, USA | NR | 118 | 67 | 67 | 83 | NR |
Hacker [ ] 2022, USA | NR | 45 | 32 | 30 | NR | NR |
Hathiramani [ ] 2020, UK | 46 | 62 | 46 | 38 | NR | No AE |
Huberty [ ] 2019, USA | NR | 260 | 62 | 48 | 15 | No AE |
Hung [ ] 2014, Australia | NR | 55 | 37 | 33 | NR | No AE |
Jacobsen [ ] 2014, USA | 700 | NR | 711 | 560 | NR | No AE |
Jarden [ ] 2009, Denmark | 40 | 82 | 42 | 34 | NR | No AE |
Jarden [ ] 2016, Denmark Jarden [ ] 2021, Denmark | 70 | 170 | 70 | 62 | 71 | No SAE. AE: 8: sport-related (n = 5), cardioresp (n = 5), dizziness (n = 3), gastrointestinal (n = 3), pain/discomfort (n = 2) and bruising (n = 1) |
Kim [ ] 2005, S. Korea | 42 | NR | 42 | 35 | NR | NR |
Knols [ ] 2011, Switzerland | 128 | 310 | 131 | 114 | 85 | No AE |
Kobayashi [ ] 2020, Japan | 32 | 33 | 33 | 22 | 67 | No AE |
Koutoukidis [ ] 2020, UK | 140 | 313 | 131 | 99 | 75 | No AE |
McCourt [ ] 2023, UK | NR | 123 | 50 | 33 | NR | No SAE. AE: 1 mild episode of dizziness |
Mello [ ] 2003, Brazil | NR | 32 | 18 | 18 | NR | NR |
Oechsle [ ] 2014, Germany | 48 | NR | 58 | 48 | NR | No AE |
Pahl [ ] 2018, Germany | NR | 121 | 20 | 11 | 62 | No AE |
Pahl [ ] 2020, Germany | NR | 112 | 71 | 44 | 59 | No SAE. AE: 2 sessions stopped prematurely due to knee pain and discomfort |
Persoon [ ] 2017, The Netherlands | 120 | 469 | 109 | 97 | 86 | AE: 1 strained calf muscle |
Potiaumpai [ ] 2021, USA | NR | 57 | 36 | 32 | 79 | NR |
Safran [ ] 2022, Turkey | 32 | 77 | 43 | 31 | NR | No AE |
Schumacher [ ] 2018, Germany | NR | 49 | 42 | 31 | NR | No AE |
Shelton [ ] 2009, USA | 164 | 250 | 61 | 53 | 75 | NR |
Streckmann [ ] 2014, Germany | 184 | 186 | 61 | 51 | 65 | No AE |
Vallerand [ ] 2018, Canada | 50 | 407 | 51 | 51 | 93 | No AE |
Waked [ ] 2019, Egypt | 54 | 60 | 54 | 50 | NR | NR |
Wehrle [ ] 2019, Germany | 36 | 39 | 29 | 22 | 68 | No AE |
Wiskemann [ ] 2011, Germany Wiskemann [ ] 2014, Germany | NR | 141 | 105 | 80 | 87 | NR |
Wood [ ] 2020, USA | 60 | 113 | 34 | 16 | NR | NR |
Yeh [ ] 2016, Taiwan | 64 | 118 | 108 | 102 | 100 | No AE |
Total | NR (n = 16) | 7262 NR (n = 8) | 3552 | 2924 (82.3%) | Mean: 70% (15–100) NR (n = 21) | No AE (n = 26) AE (n = 7) SAE (n = 1) NR (n = 15) |
Trial Identifier Design | Investigator Country | Title | Diagnosis | Sample Size, n | Age | Intervention Type and Duration | Treatment Trajectory | Primary Outcome | Study Status |
---|---|---|---|---|---|---|---|---|---|
NCT05642884 RCT | Smith Giri USA | Prehabilitation Feasibility Among Older Adults Undergoing Transplantation | MM | 30 | >60 years | Home-based prehabilitation multimodal exercise program delivered using a telehealth format 8 weeks | Before ASCT | Feasibility | Recruiting 2023-07-10 Estimated completion 2025-12-31 |
NCT04898790 RCT | Thuy Koll USA | Improving Cognitive Function in Older Adults Undergoing Stem Cell Transplant (PROACTIVE) | Leukemia Lymphoma MM MDS MPN | 88 | >60 years | Partially supervised PA in the Community Health Activities Model Program for Seniors 12 weeks | Undergoing HSCT | Change in executive function and working memory | Recruiting 2021-11-18 Estimated completion 2025-04 |
NCT04670029 RCT | Magali Bavaloine France | Impact of an APA Program on EFS in Patients with Diffuse Large-cell B Lymphoma Treated in 1st Line (PHARAOM) | Diffuse Large B Cell Lymphoma | 186 | >65 years | Partially supervised adapted physical activity with aerobic and anaerobic sessions on site and at home | During treatment | To detect an absolute difference of 15% in event-free survival between groups | Recruiting 2021-09-08 Estimated completion 2029-02 |
NCT04057443 RCT | Maite Antonio Spain | Nutritional and Physical Exercise Intervention in Older Patients with Malignant Hemopathies | MDS LPS MM | 80 | >70 years | Nutritional support according to nutritional body composition parameters (Nutritional assessment and sarcopenia evaluation). Diet counselling, oral supplemented nutrition, enteral or parenteral nutrition. Exercise program with a mixed structure, designed individually with group sessions. 24 weeks, 3 days a week | During treatment | Adherence to oncological treatment from baseline to post treatment or 6 months. Difference between dose administered and prescribed. | Unknown status Start 2019-04-11 Estimated completion 2023-06-01 |
The statements, opinions and data contained in all publications are solely those of the individual author(s) and contributor(s) and not of MDPI and/or the editor(s). MDPI and/or the editor(s) disclaim responsibility for any injury to people or property resulting from any ideas, methods, instructions or products referred to in the content. |
Share and Cite
Jarden, M.; Tscherning Lindholm, S.; Kaldan, G.; Grønset, C.; Faebo Larsen, R.; Larsen, A.T.S.; Schaufuss Engedal, M.; Kramer Mikkelsen, M.; Nielsen, D.; Vinther, A.; et al. Limited Evidence for the Benefits of Exercise in Older Adults with Hematological Malignancies: A Systematic Review and Meta-Analysis. Cancers 2024 , 16 , 2962. https://doi.org/10.3390/cancers16172962
Jarden M, Tscherning Lindholm S, Kaldan G, Grønset C, Faebo Larsen R, Larsen ATS, Schaufuss Engedal M, Kramer Mikkelsen M, Nielsen D, Vinther A, et al. Limited Evidence for the Benefits of Exercise in Older Adults with Hematological Malignancies: A Systematic Review and Meta-Analysis. Cancers . 2024; 16(17):2962. https://doi.org/10.3390/cancers16172962
Jarden, Mary, Sofie Tscherning Lindholm, Gudrun Kaldan, Charlotte Grønset, Rikke Faebo Larsen, Anders Thyge Steen Larsen, Mette Schaufuss Engedal, Marta Kramer Mikkelsen, Dorte Nielsen, Anders Vinther, and et al. 2024. "Limited Evidence for the Benefits of Exercise in Older Adults with Hematological Malignancies: A Systematic Review and Meta-Analysis" Cancers 16, no. 17: 2962. https://doi.org/10.3390/cancers16172962
Article Metrics
Article access statistics, supplementary material.
ZIP-Document (ZIP, 12748 KiB)
Further Information
Mdpi initiatives, follow mdpi.
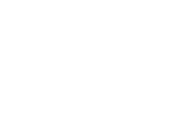
Subscribe to receive issue release notifications and newsletters from MDPI journals

An official website of the United States government
The .gov means it’s official. Federal government websites often end in .gov or .mil. Before sharing sensitive information, make sure you’re on a federal government site.
The site is secure. The https:// ensures that you are connecting to the official website and that any information you provide is encrypted and transmitted securely.
- Publications
- Account settings
Preview improvements coming to the PMC website in October 2024. Learn More or Try it out now .
- Advanced Search
- Journal List
- Dtsch Arztebl Int
- v.106(27); 2009 Jul
Systematic Literature Reviews and Meta-Analyses
Meike ressing.
1 Institut für Medizinische Biometrie, Epidemiologie und Informatik, Universitätsmedizin der Johannes Gutenberg-Universität Mainz
Maria Blettner
Stefanie j. klug.
Because of the rising number of scientific publications, it is important to have a means of jointly summarizing and assessing different studies on a single topic. Systematic literature reviews, meta-analyses of published data, and meta-analyses of individual data (pooled reanalyses) are now being published with increasing frequency. We here describe the essential features of these methods and discuss their strengths and weaknesses.
This article is based on a selective literature search. The different types of review and meta-analysis are described, the methods used in each are outlined so that they can be evaluated, and a checklist is given for the assessment of reviews and meta-analyses of scientific articles.
Systematic literature reviews provide an overview of the state of research on a given topic and enable an assessment of the quality of individual studies. They also allow the results of different studies to be evaluated together when these are inconsistent. Meta-analyses additionally allow calculation of pooled estimates of an effect. The different types of review and meta-analysis are discussed with examples from the literature on one particular topic.
Conclusions
Systematic literature reviews and meta-analyses enable the research findings and treatment effects obtained in different individual studies to be summed up and evaluated.
Every year, there is a great increase in the number of scientific publications. For example, the literature database PubMed registered 361 000 new publications in 1987, with 448 000 in 1997 and 766 000 in 2007 (research in Medline, last updated in January 2009). These figures make it clear how increasingly difficult it is for physicians in private practice, clinicians and scientists to obtain comprehensive current information on any given medical topic. This is why it is necessary to summarize and critically analyze individual studies on the same theme.
Summaries of individual studies are mostly prepared when the results of individual studies are unclear or inconsistent. They are also used to study relationships for which the individual studies do not have adequate statistical power, as the number of cases is too low ( 1 ).
The Cochrane Collaboration undertakes systematic processing and summary of the primary literature for many therapeutic topics, particularly randomized clinical studies ( www.cochrane.org ). They have published a handbook for the performance of systematic reviews and meta-analyses of randomized clinical studies ( 2 ). Cook et al. have published methodological guidelines for this process ( 3 ). Instructions of this sort help to lay down standards for the summary of individual studies. Guidelines have also been drawn up for the publication of meta-analyses on randomized clinical studies ( 4 ) and on observational studies ( 5 ).
Publications on individual studies may be summarized in various forms ( 1 , 6 – 10 ):
- Narrative reviews
- Systematic review articles
- Meta-analyses of published data
- Pooled reanalyses (meta-analyses with individual data).
These terms are often not clearly allocated in the literature. The aim of the present article is to describe and distinguish these forms and to allow the reader to perform a critical analysis of the results of individual studies and the quality of the systematic review or meta-analysis.
The various types of systematic reviews and meta-analyses of scientific articles will be defined and the procedure will be explained. A selective literature search was performed for this purpose.
A "review" is the qualitative summary of the results of individual studies ( 1 ). A distinction is made between narrative reviews and systematic reviews ( table 1 ). Narrative reviews (A) mostly provide a broad overview of a specific topic ( 1 , 11 ). They are therefore a good way of rapidly obtaining current information on research on a given topic. However, the articles to be included are selected subjectively and unsystematically ( 1 , 11 ). For some time, the Deutsches Ärzteblatt has been using the term "selective literature review" for this type of review. Narrative reviews will not be further discussed in this article.
Preparation of a detailed study protocol and analysis plan | – | + | + | + | + |
Literature search for suitable studies in accordance with prospectively defined inclusion and exclusion criteria | – | + | + | + | + |
Quantitative summary of the results (calculation of pooled estimates, examination of heterogeneity, sensitivity analyses) | – | – | + | + | + |
Analysis of individual data | – | – | – | + | + |
Common study protocol for the individual studies and prospectively planned analysis | – | – | – | – | + |
In contrast, systematic review articles (B) claim that, if possible, they consider all published studies on a specific theme—after the application of previously defined inclusion and exclusion criteria ( 11 ). The aim is to extract relevant information systematically from the publications. What is important is to analyze the methodological quality of the included publications and to investigate the reasons for any differences between the results in the different studies. The results of each study are presented and analyzed according to defined criteria, such as study design and mode of recruitment.
The same applies to the meta-analysis of published data (C). In addition, the results are quantitatively summarized using statistical methods and pooled effect estimates ( glossary ) are calculated ( 1 ).
The summary of individual data
Distortion of study results from systematic errors
The confidence interval is the range within which the true value lies with a specified probability, usually 95%.
A confounder is a factor which is linked to both the studied disease and the studied exposure. For this reason, it can either enhance or weaken the true association between the disease and the target parameter.
An effect estimate, such as the odd ratio or relative risk, estimates the extent of the change in the frequency of a disease caused by a specific exposure.
Contact with a specific risk factor
A forest plot is a graphical representation of the individual studies, as well as the pooled estimate. The effect estimate of each individual study is generally represented on the horizontal or vertical axis, with a confidence interval. The larger the area of the effect estimate of the individual study, the greater is the weight of the study, as a result of the study size and other factors. The pooled effect estimate is mostly represented in the form of a diamond.
In a funnel plot, the study size is plotted against the effect estimates of the individual studies. The variances or the standard error of the effect estimate of the individual studies is given, rather than the study size. Smaller studies give larger variances and standard errors. The effect estimates from large studies are less scattered around the pooled effect estimate than are the effect estimates of small studies. This gives the shape of a funnel. A publication bias can be visualized with the help of funnel plots.
Statistical heterogeneity describes the differences between the studies with respect to the effect estimates. These may be caused by methodological differences between the studies, such as differences in study population or study size, or differences in the methods of measurement.
In individual data, all data (e.g. age, gender, diagnosis) are at the level of the individual.
In medicine and epidemiology, the odds is the ratio of the probability of exposure and the probability of not being exposed. The quotient of the odds of the cases and the odds of the controls gives the odds ratio. For rare diseases, the odds ratio is an approximation to the relative risk.
See individual data
Publication bias means that studies which failed to find any influence of exposure on the target disease ("negative studies") are more rarely published than studies which showed a positive or statistically significant association. Publication bias can be visualized with funnel plots.
A risk factor modifies the probability of the development of a specific disease. This can, for example, be an external environmental effect or an individual predisposition.
To calculate the relative risk, the probability that an exposed individual falls ill is divided by the probability that a non-exposed person falls ill. The relative risk is calculated on the basis of incident diseases.
Using sensitivity analyses, it is examined whether excluding individual studies from the analysis influences the pooled estimate. This tests the stability of the pooled effect estimate.
In subgroup analysis, separate groups in the study population, such as a homogenous ethnic group, are analyzed separately.
A pooled reanalysis (D) is a quantitative compilation of original data ( glossary ) from individual studies for combined analysis ( 1 ). The authors of each study included in the analysis then provide individual data ( glossary ). These are then compiled in a combined database and analyzed according to standard criteria fixed in advance. This form of pooled reanalysis is also referred to as "meta-analysis of individual data".
In a prospectively planned meta-analysis (E), the summary of the individual studies and the combined analysis is included in the planning of the individual studies. For this reason, the individual studies are performed in a standard manner. Prospectively planned meta-analyses will not be further discussed in this article.
It is essential for all forms of summary—except the narrative review—that they should include a prospectively prepared study protocol, with descriptions of the questions to be answered, the hypotheses, the inclusion and exclusion criteria, the selection of studies, and, where applicable, the combination of the data and the recoding of the individual data (only for pooled reanalysis).
Types of study summaries
The procedure for the summary of the studies will now be presented (modified from [7, 10, 12, 13]). This is intended to enable the reader to assess whether a given summary fulfils specific criteria ( Box ).
Checklist for the analysis of a systematic summary
- Was there an a priori study protocol?
- Was there an a priori hypothesis?
- Was there a detailed description of the literature search used?
- Were prospectively specified inclusion and exclusion criteria clearly described and applied?
- Was the possible heterogeneity between the studies considered?
- Was there a clear description of the statistical methods used?
- Were the limitations of the summary discussed?
1. Was the question to be answered specified in advance?
The question to be answered in the review or meta-analysis and the hypotheses must be clearly defined and laid down in writing prospectively in a study protocol.
2. Were the inclusion and exclusion criteria specified in advance?
On the basis of the inclusion and exclusion criteria, it is decided whether the studies found in the literature search (see point 3) are included in the review/meta-analysis.
3. Were precautions taken to find all studies performed with reference to the specific question to be answered?
An extensive literature search must be performed for studies on the topic. If at all possible, this should be in several literature databases. To avoid bias, all relevant articles should be considered, whatever their language. Moreover, a search should be performed in the literature lists of the articles found and for unpublished studies in congress volumes, as well as with search machines on the Internet.
4. Was the relevant information extracted from the published articles or were the original data combined?
For a systematic review article (B) and for a meta-analysis of published data (C), relevant information should be extracted from the publications.
For a pooled reanalysis (D), authors of all identified studies must be contacted and requested to provide individual data. The individual data must then be coded according to standard specifications, compiled in a combined database and analyzed.
5. Was a descriptive analysis of the data performed?
In all forms of summary, it is usual for the most important characteristics of the individual studies to be presented in overview tables. Table 2 shows an example of such a table, taken from a meta-analysis with published data (C) ( 14 ). This helps to make the differences between the studies clear with respect to the data examined.
Brinton/Jones, 1986 (USA) ( – ) | pop | Invasive/in situ | None | 772/801 | 1982–1984 | 51 (18) | NK |
Peters, 1986 (USA) ( ) | pop | Invasive | None | 200/200 | 1980–1981 | 26 (NK) | NK |
Ebeling, 1987 (Germany) ( ) | hosp | Invasive | None | 129/275 | 1983–1985 | 66 (46) | NK |
Brinton, 1990 (4 countries ) ( , ) | pop/hosp | Invasive | FISH | 759/1429 | 1986–1987 | 25 (11) | 6 (1) |
WHO, 1993 (9 countries ) ( – ) | hosp | Invasive/in situ | None | 3848/13 644 | 1979–1988 | 41 (8) | 15 (4) |
Ursin, 1994 (USA) ( ) | pop | Invasive | None | 195/386 | 1977–1991 | 81 (36) | NK |
Cuzick, 1996 (GB) ( ) | pop | Invasive | None | 121/241 | 1985–1991 | 92 (62) | NK |
Madeleine, 2001 (USA) ( ) | pop | In situ | PCR/ serology | 132/478 | 1990–1996 | 84 (29) | NK |
Berrington, 2002 (GB) ( ) | pop | Invasive | Serology | 221/393 | 1984–1988 | 88 (47) | NK |
Moreno, 2002 (8 studies ) ( ) | pop/hosp | Invasive/in situ | PCR | 2171/2299 | 1985–1997 | 36 (11) | NK |
NK, not known; FISH, fluorescent in situ hybridation; *1 squamous cell carcinoma only; *2 ever use → 2 years’ use;
*3 relative risks for injectable contrceptives adjusted for oral contraceptive use; *4 Costa Rica, Colombia, Mexico, Panama;
*5 Australia, Chile, Colombia, Israel, Kenya, Mexico, Nigeria, Philippines, Thailand; *6 adenocarcinoma of the cervix only;
*7 Brasil, Colombia, Morocco, Paraguay, Peru, Philippines, Spain, Thailand (Shortened from: Smith J, Green J, Berrington de Gonzalez A et al.: Cervical cancer and use of hormonal contraceptives: a systematic review. Lancet 2003; 361: 1159–67. With the kind permission of Elsevier)
6. Were the calculations of the effect estimates of the individual studies and of the pooled effect estimate presented?
How were the effect estimates of the individual studies calculated?—Systematic review articles (B) usually contain tables with the effect estimates of the individual studies. In a meta-analysis of published data (C), the effect estimates of individual studies (for example, odds ratio or relative risk, see Glossary ) are either directly extracted from the publications or recalculated in a standard manner from the data in each publication ( figure 1 ). Depending on the nature of the factors and target parameters (binary, categorical or continuous variables), a logistic or a linear regression model is used to calculate the effect estimates of the individual studies in the meta-analyses of published data (C) and pooled reanalyses (D).

The results of the individual studies and the pooled estimate, presented as forest plots on the association between oral contraceptives and cervical carcinoma, as an example of the meta-analysis of published data ( 14 ); N.A. = not available; * never use means <2 years use. CI = confidence interval
(Shortened from: Smith J, Green J, Berrington de Gonzalez A et al.: Cervical cancer and use of hormonal contraceptives: a systematic review. Lancet 2003; 361: 1159–67. With the kind permission of Elsevier).
How was the pooled effect estimate calculated?— The effect estimates of the individual studies are combined by statistical procedures to give a common pooled effect estimate ( 9 ) ( figure 1 ). In meta-analyses with published data (C), two methods are mostly used to calculate a pooled effect estimate: either the fixed effect model or the random effect model (15, 16). They differ with respect to assumptions about the heterogeneity of the estimate between individual studies (see point 7). The method used should be given in the publication and justified. The effect estimates of the individual studies and the pooled effect estimates can be graphically presented in the form of so-called forest plots ( Glossary ; Figure 1 ; [14]).
In pooled reanalyses (D), the pooled effect estimates are mostly calculated by logistic or linear regression. However, the statistical analysis must adequately allow for the origin of the data sets from different studies. The results of the pooled reanalyses can be presented like the results of a single combined study ( table 3 ).
Never | 7356/21682 | – | 1.00 | – | – | |
5+ years | Current user | 880/1466 | 11.1 | 1.90 | 1.69–2.13 | s. |
2–9 years | 747/1510 | 9.3 | 1.28 | N. A. | s. | |
10+ years | 412/1654 | 8.1 | 0.94 | N. A. | n. s. |
Trend test: χ 2 = 66.2; p < 0.0001
RR, relative risk, adjusted for age, study or study center, age at first sexual intercourse,
number of sex partners, number of full-term pregnancies, smoking and screening status;
* Information taken from the publication; CI, confidence interval; N.A., not available;
s., significance at the level α = 5%; n.s., not significant at the level α = 5%
(Shortened and modified from: International Collaboration of Epidemiological Studies of Cervical Cancer: Cervical cancer and hormonal contraceptives: collaborative reanalysis of individual data for 16,573 women with cervical cancer and 35,509 women without cervical cancer from 24 epidemiological studies. Lancet 2007; 370: 1609–21. With the kind permission of Elsevier)
7. Were problems considered in the interpretation of pooled estimates?
Was the heterogeneity between the estimates considered?—There may be marked differences between the estimates in the individual studies. This statistical heterogeneity ( glossary ) between the studies may be caused by differences in study design, study populations (age, gender, ethnic group), methods of recruitment, diagnosis, or methods of measurement ( 17 , 18 ). The methodological heterogeneity between the studies can be visualized in an overview table, in which the most important characteristics of the individual studies are presented ( table 2 ). The heterogeneity can be formally investigated with the help of statistical tests. If there is statistical heterogeneity between the studies, the random effect model, rather than the fixed effect model, should be used for the calculation of the pooled estimate ( 7 , 15 , 16 ). There is, however, no clear definition as to when the statistical heterogeneity between the studies is so large that the pooled effect estimate should not be calculated ( 1 , 19 ). In addition, the heterogeneity between the studies should be examined by subgroup analysis ( glossary ). For example, this might involve combined analysis of only studies with the same characteristics in the study population, such as homogenous age groups, the same ethnic groups or the same histological findings. Moreover, studies with the same characteristics—such as study quality or study size—may be considered separately in subgroup analyses. This may indicate whether the effect of the corresponding risk factors ( glossary ) is different in the different subgroups.
Were sensitivity analyses performed?— Like subgroup analyses, sensitivity analyses ( glossary ) serve to test the stability of the pooled estimate. It is, for example, possible that the pooled effect estimate is mainly determined by one large study. If this study is excluded from the analysis, the pooled effect estimate may change. This must be borne in mind in the discussion and interpretation of the results.
Was a possible publication bias considered?— A publication bias ( glossary ) can be visualized with a so-called funnel plot ( glossary ) ( 7 , 20 – 22 ). Figure 2 shows an example with simulated data. In the upper funnel plot ( Figure 2a ), there is a roughly funnel shaped distribution of the effect estimates of the individual studies around the pooled effect estimates (middle broken line). There is no publication bias here. In the lower funnel plot ( Figure 2b ), the small studies are missing, which in this example show no increased risk. For this reason, there is probably a publication bias, because these studies had not been published.

Visualization of publication bias with funnel plots of simulated data a) No publication bias; b) Publication bias; SE = standard error; OR = odds ratio
8. How were the results interpreted?
In the interpretation of the results, possible limitations should be discussed and considered. For example, the reliability of the results of individual studies can be limited by the inadequate quality of the individual studies—for example, by selection of the study population or from aggregated data ( glossary ).
The method section describes the individual steps for the extraction of the relevant points which must be considered in the systematic summary of scientific articles ( Box ). This checklist can also be used to analyze the quality of systematic review articles or meta-analysis.
Publications on the association between the administration of oral contraceptives and the development of cervical carcinoma were used as examples of the performance of a systematic literature review (B), a meta-analysis of published data (C), and a pooled reanalysis (D). This association has been scientifically studied for a long period.
In 1996, La Vecchia et al. published a systematic review article (B) on this topic, including six studies ( 23 ). Their overview table contained a variety of information on the individual studies. No pooled effect estimate was calculated.
In 2003, Smith et al. ( 14 ) presented a meta-analysis of published data (C) of 28 studies on the same topic. The included studies were first summarized in a descriptive overview, as is common in systematic review articles ( table 2 ). This table shows that the study methods were heterogenous ( glossary ); for example, HPV was detected in different ways ( table 2 ). The heterogeneity was also formally investigated with statistical tests and various subgroup analyses were performed. In contrast to the systematic review article (B) of LaVecchia et al., pooled effect estimates were calculated with the published data ( figure 1 ). The effect estimates for the individual studies and the pooled effect estimates with their confidence intervals ( glossary ) were presented as a forest plot ( figure 1 ).
In 2007, a pooled reanalysis (D) was published for 24 studies on the same topic for which the original data were available ( 24 ). In contrast to the meta-analysis of published data, the pooled effect estimates were calculated from the original data and only the combined results were presented ( table 3 ). This kind of analysis is only possible in a pooled reanalysis, as the original data with precise information on all parameters for each participant are then available. Nevertheless, here too it is necessary to consider that the individual data ( glossary ) are derived from different studies.
Systematic review articles (B) can provide a comprehensive overview of the current state of research ( 1 ). They are also necessary for the development of S2 and S3 guidelines for formal evidence-based research ( 25 ). Meta-analyses of published data (C) are performed to calculate additional pooled effect estimates from the individual studies ( 1 ). Like systematic review articles, they are feasible whether the authors of the original articles are prepared to cooperate or not.
The calculated pooled effect estimates may be of limited validity for various reasons. Firstly, it has not been clearly defined what is the maximum order of heterogeneity between the studies which is negligible and which then allows a meaningful calculation of a pooled effect estimate (1, 19). If the individual studies are too heterogenous, a pooled effect estimate should not be calculated. Secondly, the pooled effect estimate is mostly calculated from aggregated data. Subgroup analyses and the consideration of potential confounders ( glossary ) are often impossible, or only possible to a limited extent ( 1 , 19 ). Thirdly, publication bias is also a problem for the meta-analysis of published data.
In a pooled reanalysis (D), potential confounders and risk factors can be more easily considered ( 7 ), as they are usually only published in an aggregated form. With the individual data, the outcome parameters, risk factors, and confounders used in the analysis can be categorized in a standard manner and properly incorporated in the analysis. Individual data can be removed in accordance with the prospective specifications in the study protocol, without it being necessary to exclude the whole study. The disadvantages of pooled reanalysis are that it demands a great deal of time and money and that it is dependent on the willingness of the authors of the individual studies to cooperate. If not all authors send their individual data, this may result in biased results.
The level of evidence of the type of summary increases from the systematic review to the meta-analysis of published data to the pooled reanalysis. It is important that all three forms of summary should be performed with high quality.
Key messages
- The various forms of summary can be categorized as systematic review articles, meta-analyses of published data, and pooled reanalyses.
- Systematic review articles can provide a rapid overview of the status of research on a specific topic.
- Meta-analyses of published data and pooled reanalyses additionally permit the calculation of pooled effect estimates.
- Pooled reanalyses allow a detailed evaluation on the basis of individual data.
- Like any original study, all these types of summary must have an a priori study protocol, laying down in detail the research questions, the hypothesis, the literature search, the inclusion and exclusion criteria, and the analysis strategies.
Acknowledgments
Translated from the original German by Rodney A. Yeates, M.A., Ph.D.
Conflict of interest statement
The authors declare that there is no conflict of interest in the sense of the guidelines of the International Committee of Medical Journal Editors.

IMAGES
COMMENTS
Meta-analysis is a statistical tool that provides pooled estimates of effect from the data extracted from individual studies in the systematic review. The graphical output of meta-analysis is a forest plot which provides information on individual studies and the pooled effect. Systematic reviews of literature can be undertaken for all types of ...
A systematic review is an article that synthesizes available evidence on a certain topic utilizing a specific research question, pre-specified eligibility criteria for including articles, and a systematic method for its production. Whereas a meta-analysis is a quantitative, epidemiological study design used to assess the results of articles ...
A systematic review collects all possible studies related to a given topic and design, and reviews and analyzes their results [ 1 ]. During the systematic review process, the quality of studies is evaluated, and a statistical meta-analysis of the study results is conducted on the basis of their quality. A meta-analysis is a valid, objective ...
Systematic reviews involve the application of scientific methods to reduce bias in review of literature. The key components of a systematic review are a well-defined research question, comprehensive literature search to identify all studies that potentially address the question, systematic assembly of the studies that answer the question, critical appraisal of the methodological quality of the ...
A meta-analysis is the statistical extension of a systematic review. In a meta-analysis, statistical techniques are used to combine the results of included studies and present a single pooled estimate for the result. Therefore, along with providing the results for each included study, the meta-analysis possibly increases the strength of ...
Review Manager (RevMan) is a web-based software that manages the entire literature review process and meta-analysis. The meta-analyst uploads all studies to RevMan library, where they can be managed and exanimated for inclusion. Like CMA, RevMan enables authors to conduct overall analysis and moderator analysis. 4.4.6.3 Stata
A meta-analysis (Clinical Vignette 2), much like a systematic review and often an extension of one, also hinges on a systematic and exhaustive search of the literature. A meta-analysis differs from a systematic review in that instead of simply collecting and analysing the data, it employs statistical methods to quantitatively synthesize the ...
Meta-analysis is a statistical tool that is used to mathematically pool data derived from a systematic review, Published online: June 28, 2021; PII: S097475591600350. and generate a summary conclusion [4]. Meta-analysis of data is inappro-priate if not derived from a systematic review.
In this chapter, we summarize the key principles involved in designing and conducting a rigorous systematic review focused on an intervention question. We provide key definitions on what systematic reviews and meta-analysis are and how they differ from other types of reviews. We cover the principles for designing a good systematic review ...
Meta-analysis is a specialised type of systematic review which is quantitative and rigorous, often comparing data and results across multiple similar studies. This is a common approach in medical research where several papers might report the results of trials of a particular treatment, for instance. The meta-analysis then statistical ...
Systematic reviews combine study data in a number of ways to reach an overall understanding of the evidence. Meta-analysis is a type of statistical synthesis. Narrative synthesis combines the findings of multiple studies using words. All systematic reviews, including those that use meta-analysis, are likely to contain an element of narrative ...
Systematic reviews with meta-analysis represent the gold standard for conducting reliable and transparent reviews of the literature. The purpose of this article is threefold: (a) to address why and when it is worthwhile to conduct a systematic review with meta-analysis, covering advantages of this approach in the context of the statistics reform in the behavioral sciences; (b) to explain how ...
There are many potential sources of missing data in a systematic review or meta-analysis (see Table 10.12.a). For example, a whole study may be missing from the review, an outcome may be missing from a study, summary data may be missing for an outcome, and individual participants may be missing from the summary data.
A meta-analysis and literature reviews differ in purpose, methodology, and outcomes. The primary purpose of a meta-analysis is to provide a quantitative analysis of data from multiple studies, producing a precise estimate of the effect size through statistical methods. A literature review synthesizes findings to offer an overview of current ...
Chapter 19: Systematic Reviews: Meta-Analysis and Metasynthesis. - The systematic and rigorous integration and synthesis of evidence is a cornerstone of EBP. - Systematic review is a review that methodically integrates research evidence about a specific research question using careful sampling and data collection procedures that are spelled out ...
Systematic reviews and meta-analysis are the highest quality evidence (level 1) on a research topic because their study design reduce bias and produce more reliable findings. It is a misconception that systematic reviews and meta-analysis are the same and the terms are used interchangeably. A systematic review is a detailed, systematic and ...
Meta-analysis: Technique that statistically combines the results of quantitative studies to provide a more precise effect of the results. ... Refers to any combination of methods where one significant component is a literature review (usually systematic). Within a review context it refers to a combination of review approaches for example ...
Whereas the systematic review is very specific and focused, the standard literature review is much more general. The components of a literature review, for example, are similar to any other research paper. Meanwhile, whereas a systematic review can include several research studies to answer a specific question, typically a meta analysis ...
The term meta-analysis is sometimes confused with systematic reviews. However, meta-analysis is a non-essential component of a systematic review. The archetype of systematic reviews follows protocols for finding and retrieving relevant studies, and a structured approach to analysis and synthesis. One of these approaches is meta-analysis as ...
Here are some common review types: Meta-analysis. Technique that statistically combines the results of quantitative studies to provide a more precise effect of the results. May be a component of a systematic review. Literature review. Generic term: published materials that provide examination of recent or current literature.
However, an integrative review includes qualitative data. The text of the article was divided up into two sections; one heading was integrative review and the other was meta-analysis. Yet, the integrative review included statistical data. This was more like a systematic review with a meta-analysis. No review method was actually followed.
Design. We followed the Cochrane Handbook for Systematic Reviews of Interventions to conduct this systematic review and meta-analysis and reported our results by the Preferred Reporting Items for Systematic Reviews and Meta-Analysis (PRISMA) guidelines [19, 20] (Additional file 1: Table 1).To explore whether added sugars mediate any effects observed in sweetened soymilk studies, we conducted ...
Findings In this systematic review and meta-analysis of 16 articles involving 82 769 patients with OHCA, survival to discharge or 30 days improved with treatment at a high-volume center; there was no association between center volume and good neurological outcomes at 30 days or at hospital discharge.
Older patients receiving antineoplastic treatment face challenges such as frailty and reduced physical capacity and function. This systematic review and meta-analysis aimed to evaluate the effects of exercise interventions on physical function outcomes, health-related quality of life (QoL), and symptom burden in older patients above 65 years with hematological malignancies undergoing ...
Conclusions. Systematic literature reviews and meta-analyses enable the research findings and treatment effects obtained in different individual studies to be summed up and evaluated. Keywords: literature search, systematic review, meta-analysis, clinical research, epidemiology. Every year, there is a great increase in the number of scientific ...
Systematic review: We reviewed the literature pertaining to Alzheimer's disease (AD), biomarkers for AD and clinical development for AD, proteomics analyses and mass spectrometry (MS) techniques, and mechanistic findings related to proteins of interest that we have identified in our biomarker research. The use of recently improved proteomics ...
Methods. The review protocol followed the PRISMA checklist for systematic reviews (Moher, Liberati, Tetzlaff, and Altman, Citation 2009) and was registered in the PROSPERO database for systematic review [CRD42023452079].A systematic literature search was conducted using the PubMed and Embase databases, and the last search was performed on April 29, 2024 (see supplementary materials for full ...
The protocol for this systematic review was registered in the International Prospective Register of Systematic Reviews (PROSPERO) with a registration number CRD42021261805. This review was designed and performed in accordance with the guidelines of Preferred Reporting Items for Systematic Review and Meta-Analysis Protocols (PRISMA-P) 2015 . All ...