
An official website of the United States government
The .gov means it’s official. Federal government websites often end in .gov or .mil. Before sharing sensitive information, make sure you’re on a federal government site.
The site is secure. The https:// ensures that you are connecting to the official website and that any information you provide is encrypted and transmitted securely.
- Publications
- Account settings
- My Bibliography
- Collections
- Citation manager

Save citation to file
Email citation, add to collections.
- Create a new collection
- Add to an existing collection
Add to My Bibliography
Your saved search, create a file for external citation management software, your rss feed.
- Search in PubMed
- Search in NLM Catalog
- Add to Search
Statistics review 9: one-way analysis of variance
Affiliation.
- 1 School of Computing, Mathematical and Information Sciences, University of Brighton, Brighton, UK. [email protected]
- PMID: 15025774
- PMCID: PMC420045
- DOI: 10.1186/cc2836
This review introduces one-way analysis of variance, which is a method of testing differences between more than two groups or treatments. Multiple comparison procedures and orthogonal contrasts are described as methods for identifying specific differences between pairs of treatments.
PubMed Disclaimer
Box plots of the Simplified…
Box plots of the Simplified Acute Physiology Score (SAPS) scores according to infection.…
Box plots of plasma colloid…
Box plots of plasma colloid osmotic pressure (COP) for each age group. Means…
Plot of residuals versus fits…
Plot of residuals versus fits for the data in Table 4. Response is…
Normal probability plot of residuals…
Normal probability plot of residuals for the data in 4. Response is Simplified…
Similar articles
- Evaluation of the statistical power for multiple tests: a case study. Yeo A, Qu Y. Yeo A, et al. Pharm Stat. 2009 Jan-Mar;8(1):5-11. doi: 10.1002/pst.319. Pharm Stat. 2009. PMID: 18381588
- Evaluating statistics in clinical trials: making the unintelligible intelligible. Gilmore SJ. Gilmore SJ. Australas J Dermatol. 2008 Nov;49(4):177-84; quiz 185-6. doi: 10.1111/j.1440-0960.2008.00465_1.x. Australas J Dermatol. 2008. PMID: 18855776 Review.
- Sample size calculation for the Power Model for dose proportionality studies. Sethuraman VS, Leonov S, Squassante L, Mitchell TR, Hale MD. Sethuraman VS, et al. Pharm Stat. 2007 Jan-Mar;6(1):35-41. doi: 10.1002/pst.241. Pharm Stat. 2007. PMID: 17323313
- Variance estimation in clinical studies with interim sample size re-estimation. Miller F. Miller F. Biometrics. 2005 Jun;61(2):355-61. doi: 10.1111/j.1541-0420.2005.00315.x. Biometrics. 2005. PMID: 16011681
- How to deal with multiple endpoints in clinical trials. Neuhäuser M. Neuhäuser M. Fundam Clin Pharmacol. 2006 Dec;20(6):515-23. doi: 10.1111/j.1472-8206.2006.00437.x. Fundam Clin Pharmacol. 2006. PMID: 17109645 Review.
- Biohybrids for Combined Therapies of Skin Wounds: Agglomerates of Mesenchymal Stem Cells with Gelatin Hydrogel Beads Delivering Phages and Basic Fibroblast Growth Factor. Moghtader F, Tabata Y, Karaöz E. Moghtader F, et al. Gels. 2024 Jul 25;10(8):493. doi: 10.3390/gels10080493. Gels. 2024. PMID: 39195022 Free PMC article.
- Nanomechanical resilience and thermal stability of RSJ2 phage. Sae-Ueng U, Bunsuwansakul C, Showpanish K, Phironrit N, Thadajarassiri J, Nehls C. Sae-Ueng U, et al. Sci Rep. 2024 Aug 20;14(1):19389. doi: 10.1038/s41598-024-70056-8. Sci Rep. 2024. PMID: 39169068 Free PMC article.
- Applications of Machine Learning in Periodontology and Implantology: A Comprehensive Review. Șalgău CA, Morar A, Zgarta AD, Ancuța DL, Rădulescu A, Mitrea IL, Tănase AO. Șalgău CA, et al. Ann Biomed Eng. 2024 Sep;52(9):2348-2371. doi: 10.1007/s10439-024-03559-0. Epub 2024 Jun 17. Ann Biomed Eng. 2024. PMID: 38884831 Free PMC article. Review.
- Multimodality quantitative ultrasound envelope statistics imaging based support vector machines for characterizing tissue scatterer distribution patterns: Methods and application in detecting microwave-induced thermal lesions. Li S, Tsui PH, Wu W, Zhou Z, Wu S. Li S, et al. Ultrason Sonochem. 2024 Jul;107:106910. doi: 10.1016/j.ultsonch.2024.106910. Epub 2024 May 17. Ultrason Sonochem. 2024. PMID: 38772312 Free PMC article.
- Polymorphisms and expressions of ADSL, MC4R and CAPN1 genes and their effects on economic traits in Egyptian chicken breeds. Aboelhassan DM, Darwish HR, Mansour H, Abozaid H, Ghaly IS, Radwan HA, Hassan ER, Farag IM. Aboelhassan DM, et al. Mol Biol Rep. 2023 Dec 10;51(1):4. doi: 10.1007/s11033-023-08999-w. Mol Biol Rep. 2023. PMID: 38071695 Free PMC article.
- Whitely E, Ball J. Statistics review 5: Comparison of means. Crit Care. 2002;6:424–428. doi: 10.1186/cc1548. - DOI - PMC - PubMed
- Bland M. An Introduction to Medical Statistics. 3. Oxford, UK: Oxford University Press; 2001.
- Bewick V, Cheek L, Ball J. Statistics review 7: Correlation and Regression. Crit Care. 2003;7:451–459. doi: 10.1186/cc2401. - DOI - PMC - PubMed
- Le Gall JR, Lemeshow S, Saulnier F. A new simplified acute physiology score (SAPS II) based on a European/North American multicenter study. JAMA. 1993;270:2957–2963. doi: 10.1001/jama.270.24.2957. - DOI - PubMed
- Montgomery DC. Design and Analysis of Experiments. 4. New York, USA: Wiley; 1997.
Publication types
- Search in MeSH
Related information
- PubChem Compound
- PubChem Substance
LinkOut - more resources
Full text sources.
- BioMed Central
- Europe PubMed Central
- PubMed Central
- MedlinePlus Health Information
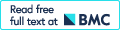
- Citation Manager
NCBI Literature Resources
MeSH PMC Bookshelf Disclaimer
The PubMed wordmark and PubMed logo are registered trademarks of the U.S. Department of Health and Human Services (HHS). Unauthorized use of these marks is strictly prohibited.

An official website of the United States government
The .gov means it’s official. Federal government websites often end in .gov or .mil. Before sharing sensitive information, make sure you’re on a federal government site.
The site is secure. The https:// ensures that you are connecting to the official website and that any information you provide is encrypted and transmitted securely.
- Publications
- Account settings
Preview improvements coming to the PMC website in October 2024. Learn More or Try it out now .
- Advanced Search
- Journal List

The multivariate analysis of variance as a powerful approach for circular data
Lukas landler.
1 Institute of Zoology, University of Natural Resources and Life Sciences (BOKU), Gregor-Mendel-Straße 33, 1180 Vienna, Austria
Graeme D. Ruxton
2 School of Biology, University of St Andrews, St Andrews, KY16 9TH UK
E. Pascal Malkemper
3 Research Group Neurobiology of Magnetoreception, Max Planck Institute for Neurobiology of Behavior – caesar, Ludwig-Erhard-Allee 2, 53175 Bonn, Germany
4 Department of Game Management and Wildlife Biology, Faculty of Forestry and Wood Sciences, Czech University of Life Sciences, 16521 Prague 6, Czech Republic
Associated Data
Data and code are available in the Additional file 1 .
A broad range of scientific studies involve taking measurements on a circular, rather than linear, scale (often variables related to times or orientations). For linear measures there is a well-established statistical toolkit based on linear modelling to explore the associations between this focal variable and potentially several explanatory factors and covariates. In contrast, statistical testing of circular data is much simpler, often involving either testing whether variation in the focal measurements departs from circular uniformity, or whether a single explanatory factor with two levels is supported.
We use simulations and example data sets to investigate the usefulness of a MANOVA approach for circular data in comparison to commonly used statistical tests.
Here we demonstrate that a MANOVA approach based on the sines and cosines of the circular data is as powerful as the most-commonly used tests when testing deviation from a uniform distribution, while additionally offering extension to multi-factorial modelling that these conventional circular statistical tests do not.
Conclusions
The herein presented MANOVA approach offers a substantial broadening of the scientific questions that can be addressed statistically using circular data.
Supplementary Information
The online version contains supplementary material available at 10.1186/s40462-022-00323-8.
Introduction
Some scales of measurement in science are inherently periodic rather than linear. Data on compass orientations, times of day and times of year are obvious examples of this. Such data are often called circular, since it is easy to imagine the data being mapped onto the circumference of a circle. As soon as one considers that 355° is closer to 5° than it is to 340°, it is clear that such circular data needs different statistical treatment from linear variables (such as mass and age), and a body of statistical theory has developed to allow investigation of circular data (for example: Batschelet [ 1 ], Fisher [ 2 ], Pewsey et al. [ 3 ] and Landler et al. [ 4 ]).
Rayleigh’s test on the null hypothesis that the population mean vector length is zero is often cited as the first statistical testing procedure designed for circular data. Since Lord Rayleigh [ 5 ] formulated his seminal Rayleigh test in the late nineteenth century, circular statistics as an academic discipline has steadily developed and expanded the range of possible approaches, nowadays including a myriad of test alternatives and software [ 6 – 8 ], second order analyses [ 9 ], as well as Akaike and Bayesian Information criterion methods [ 10 – 12 ]. All have the goal of helping researchers understand and correctly interpret data distributed on a circle. However, recently we confirmed that, despite all the developments, the Rayleigh test is still one of the most powerful tests for using a single sample to test the null hypothesis that the underlying population is uniformly distributed with no preferred direction [ 4 ]. In contrast to some alternative tests, the Rayleigh test also controls type I error rates for aggregated data (e.g. if directions are only recorded to the nearest degree, or even ten degrees) and can be used to detect a variety of deviations from uniformity, with the exception of multimodal symmetrical distributions [ 13 , 14 ].
One feature all commonly-used tests for circular statistics based on null-hypothesis testing have in common is the inability to use multiple covariates and/or multiple explanatory factors if the circular variable is the response. That is, investigation is limited to a single independent variable (although this variable can be discrete, continuous and circular, or continuous and linear). This is in stark contrast to analyses of linear dependent variables, where multiple independent variables are analyzed routinely within one testing procedure.
In a recent analysis we compared a suite of available tests designed to compare two samples to test the null hypothesis that they come from the same underlying population and found that also in this testing situation a very common test, the Watson U 2 test [ 15 ], showed superior power to many other standard options [ 16 ]. Within this study, we also experimented with a new approach, which exploits a well-known procedure to linearize circular data by using sines and cosines of the angles (see for example Pewsey et al. [ 3 ]). In order to use two linear factors (the sines and cosines of the recorded angles) as dependent variables, we needed to switch from univariate linear models to a multivariate analysis of variance. This approach allows the use of multiple response variables [ 17 ]. In our study we used MANOVA to test for a difference of two circular distributions by using the sample id as a factor with two levels (see Pail et al. [ 18 ] for one earlier study that used a MANOVA to analyze effects of a linear variable on a directional response, and Sect. 7.4 of Mardia & Jupp [ 19 ] for methods to deal with more than two distributions). Perhaps surprisingly, this novel approach offered very similar performance to the best-established tests in this simple testing situation, for instance, the Watson U 2 test [ 16 ]. It is important to add that the sine and cosine of an angle (theta), while derived from the same number, are orthogonal to each other by definition. This fact might add to the power of the MANOVA approach.
Although our previous investigation was restricted to a single two-level explanatory factor, it is clear that the MANOVA approach allows for the use of more than two levels per factor, multiple grouping factors, as well as linear covariates [ 20 – 22 ]. However, it is less clear how this would relate to circular distributions, or in other words, how p values obtained in such more-complex analyses would relate to specific null hypotheses.
While we have already shown that differences between two populations can be reliably tested, we wanted to also use this approach to test for significantly clustered (i.e., non-random) unimodal distributions based on just a single sample of data. Testing the null-hypothesis that a single sample of data comes from an underlying uniform distribution is very likely the most commonly applied procedure in circular statistics. We hypothesized that the intercept of the null model without any explanatory variables could be used to derive p values related to significant clustering. As such approach would be novel to circular statistics, we tested this hypothesis and compared this MANOVA intercept approach with the two most powerful tests for unimodal deviations from uniformity in a single sample, the Rayleigh and Hermans–Rasson tests [ 4 , 23 ]. We then simulated some example situations with known underlying distributions to further explore the MANOVA approach’s general usefulness in situations including grouping and linear factors.
This paper explores the use of MANOVA applied to circular response variables, and provides advice for people interested in using this for their own research. We show that the intercept of the MANOVA models is a reliable proxy of unimodal clustering and that grouping factors as well as linear covariates can be accommodated in the very flexible approach, which considerably enhances model performance and interpretation of circular data.
Statistical tests used
All analyses were performed in the statistical software R [ 24 ], R code used for simulations and R output can be found in the Additional file 1 (the R code and example data can also be found on github: https://github.com/Malkemperlab/Circular-MANOVA ). For all analyses we performed the MANOVA approaches using the summary function for the “manova” function in base R. We exploited a common transformation of circular variables into two orthogonal linear variables for the response matrix. This was achieved by using sine and cosine of the angle (in radians). This approach has previously been used to perform linear models with angles as independent variables (see [ 3 ]). If the angles are viewed as vectors on a unit circle, the sine and cosine of the angle represent the x and y component of such a vector. However, this view might only be valid for the case of a MANOVA model without any independent variables (intercept only). In such a special case, a significant p value is expected to indicate that the (vector) distribution differs significantly from the center of such unit circle (0,0). Following this logic, independent factors can then change the weight (length) of the vectors (linear variables) or group the vectors and allow testing for differences between the groups (grouping factors).
In our power analysis the independent variables were either absent (intercept-only approach) or different for each of the analyses. The intercept-only approach used no response variables, therefore, only a single p value was reported. In the linear MANOVA approach, a linear variable was added, therefore reporting the p value of the intercept and the covariate. In the grouped MANOVA approach a grouping factor was added, therefore, generating p values for both the intercept and the grouping factor. In the last variant of MANOVA model explored, linear and grouping factors were combined in one MANOVA analysis, allowing the analysis of significant intercept, linear and grouping effects. Throughout, we compared two MANOVA approaches, using both conventional theory and numerical simulation to evaluate p values. For the simulation-based MANOVA approaches, the obtained p values were adjusted by comparing them to 9999 iterations of the same analysis using samples from a uniform random distribution. The Rayleigh test was calculated using the function rayleigh.test of the package circular [ 8 ]. The Hermans-Rasson (HR) test was performed using the R package CircMLE [ 10 ] and function HermansRasson2T [ 23 ].
Simulation tests
All tests described above were applied to the following samples, which were drawn from the given distribution (9999 iterations), power was defined as the proportion of significant test results (p< 0.05). For all simulations circular distributions were generated using the function rcircmix from the package NPcirc [ 25 ].
First, in order to test type I error rate, we simulated samples from circular uniform distributions (type “unif” in rcircmix, sample sizes: 5, 10, 15, 25, 50 and 100), from a continuous as well as binned distribution (with data aggregated into 10° bins around the circle, for all binned data the minimum sample size used was 10). The binning was done to test for sensitivity to such rounding of measured data, which can cause issues with some circular tests [ 14 ].
In a next step, we tested samples drawn from a unimodal von Mises (type “vm”, kappa = 1, mean direction: 0, sample sizes: 5, 10, 15, 25, 50 and 100) and wrapped skew normal (type “wsn”, dispersion parameter = 2, skewness: 30, location parameter: 0, sample sizes: 5, 10, 15, 25, 50 and 100) distribution. We then simulated samples from both a symmetrical (mean directions: 0° and 180°) and an asymmetrical (mean directions: 0° and 240°) bimodal von Mises distribution (type “vm”, kappa = 1, sample sizes: 10,20,30,50,100,200). To further extend this power comparison of basic distributions, we used samples from symmetrical (mean directions: 0°, 120° and 240°) and asymmetrical (mean directions: 0°, 120° and 270°) trimodal von Mises distributions (type “vm”, kappa = 1, sample sizes: 15, 30, 45, 75, 150, 300).
In order to gain insights into the usefulness of the MANOVA approach for comparisons we simulated data for a hypothetical scenario. In this scenario, we work on an animal population where we know the age and sex (called group 1 and group 2 herein) of the tested individuals. The assumption is that the homeward orientation after displacement becomes more clustered with age (which in this example ranges from 1 year to 5 years) and that group 1 is either less clustered or directionally differently oriented than group 2. To keep the presentation consistent, data were plotted according to the sample size of the potential treatment combination. Two groups and five ages resulted in 10 treatment combinations, hence the minimum sample size was 10.
Real data examples
In order to test the presented approach on real data, we used three publicly available data sets from studies on animal behavior. The first one is a data set from Gagliardo et al. [ 26 ] embedded in the R package circular . In this study a translocation experiment was performed on pigeons, with three groups: control, sectioned olfactory nerve and section of the ophthalmic branch of the trigeminal nerve. The expectations were a difference between groups, an overall orientation towards the home direction, and no directional clustering in the group with the sectioned olfactory nerve.
The second data set was from Lindecke et al. [ 27 ] involving migratory bats ( Pipistrella pygmaeus ). The expectation was a difference between two treatment groups (with a 180° switch in preferred direction) and an effect of age on the treatment effect (interaction between age and treatment). For our analysis we started with a full model including the factors age, sex, treatment, temperature and wind speed. Then we used our self-written AIC based model selection function (see Additional file 1 ). This function first orders the independent variables based on their Eta-squared values from the full model (using the function eta_squared from the package effectsize [ 28 ] on both underlying ANOVAs, i.e., for each response variable). The first n -variables are then used in an AIC based model comparison ( n can be specified in the function input - in the case of the bat examples no variable was discarded at this step). For the AIC based model comparison all possible variable combinations are used in a MANOVA model and ranked according to their AIC values (mean AIC from both underlying ANOVAs, i.e., for each of the two response variables).
The third data set is from Obleser et al. [ 29 ], where the flight direction of deer was investigated. This data set included numerous potential explanatory variables, i.e. hour of the day, temperature, light intensity, wind speed, wind direction, sun direction, hide direction, previous alignment, distance of the hide, group size, sex, age, observer direction and vegetation height, which were included in a full model. In a further step we used our AIC based model selection function to reduce the model (the first 10 independent variables were used in the first selection step to allow fast calculation, see above for explanation). The original study observed a north-south flight direction of roe deer; thus the dependent variable was hypothesized to be axial. We, therefore, doubled the angles and reduced to modulo 360° (= 2*pi in radians) for our analysis [ 1 ]. All independent variables were added as axial as well as non-transformed directions. Hence this data set represented a complex system of multiple variables. In the published paper this was overcome by performing several different graphical and statistical analyses, in our approach we tested all the underlying hypotheses in one single model.
Type I error
Type I error was slightly above 0.05 at very low sample sizes for the MANOVA approach but kept expected alpha values at sample sizes of at least 10–15 (Fig. 1 ). The simulation MANOVA approach controlled type I error throughout all sample sizes. The HR test showed slightly increasing type I error rates with increasing sample sizes for binned data (10° bins), as demonstrated earlier [ 14 ].

Type I error rates at different sample sizes of the four statistical tests used for continuous data ( a ) and binned data ( b )
Power analysis—basic distributions
For unimodal distributions the MANOVA approach and the Rayleigh test performed equally well, with the HR test showing just slightly lower power (Fig. 2 a, b). The standard MANOVA approach showed artificially increased power levels at a sample size of 5, due to increased type I error rate at such small sample sizes. This was controlled for by the simulation MANOVA approach. When evaluating symmetrical bimodal distributions (Fig. 2 c), the HR test was the only test with useful power to detect clustering, however, for asymmetrical bimodal distributions (Fig. 2 d), the results were similar to the unimodal distribution, with the HR test performing slightly worse than both the other tests. None of the tests were able to detect clustering in symmetrical trimodal distributions (Fig. 2 e) over the range of sample size used (15–300). All of the tests had very similar (low) power to detect clustering of asymmetrical trimodal distributions (Fig. 2 f). Taken all the analyses together, for the standard distributions, the power of the MANOVA approach was almost identical to the very powerful Rayleigh test, and only shared the same weakness of low power with symmetrical distributions, which traditionally can be overcome by simple data transformation [ 1 ].

Power of the four statistical tests in situations with different underlying distributions: unimodal von Mises ( a ), unimodal wrapped skew normal ( b ), symmetrical bimodal von Mises ( c ), asymmetrical bimodal von Mises ( d ), symmetrical trimodal von Mises (E), asymmetrical trimodal von Mises ( f )
Power analysis—hypothetical examples
All tests controlled type I error when all treatment combinations were sampled from the same distributions (Fig. 3 a). The overall power to detect orientation non-uniformity was similar between tests when the mean direction was the same for all distributions, however, the MANOVA approach in addition appropriately identified a linear and/or grouping effect (Fig. 3 b–d). In the case of differing mean directions between groups, the MANOVA approaches performed very well in detecting deviations from uniformity (Fig. 4 ). The exception was the case of two groups with mean orientations 180° apart, in this special case only the HR test showed moderate power to detect non-random orientation (Fig. 4 c). However, the MANOVA approach showed good power to detect differences between groups, and hence an effect on orientation. The MANOVA approach performed almost as well as the HR test when there was a linear effect (different between groups) added to the two groups with opposite orientations (Fig. 4 d).

Power of several hypothetical examples for the proposed approach. In a hypothetical 5-year study (linear variable) of a population composed of two groups (grouping variable) the orientation performance of subjects is measured. P values for the MANOVA intercepts as well as Rayleigh and HR test are shown in the left panel, p values for the linear variables in the center and grouping variables in the right panel. In the case of randomized data ( a ), all p values of all measured tests remained at nominal levels. In all following distributions the clustering of orientations continuously increased each year, however, mean orientation was identical between all groups. First, we only changed the yearly increase from 0–2 ( b ) to 2–4 ( c ). In a second step, we used different yearly clustering increases for group 1 and group 2 ( d )

Continuation of our hypothetical example, analyzing the usefulness of the MANOVA approach for biological data. In addition to the yearly increase of clustering, we now manipulated the orientation direction for each group, using either a 90° ( a , b ) or a 180° ( c , d ) difference. This was then either combined with a group difference in yearly clustering increase ( b , d ), or not ( a , c ). The left panel shows the power for the intercept of MANOVA approaches as well as Rayleigh and HR test, the center panel shows the power of detecting the linear effect, the right panel shows power for detecting group differences
The MANOVA performed on the pigeon data showed a highly significant treatment effect and an overall significant non-uniform orientation (intercept) (Table 1 ), which is in line with the conclusions made in the original study [ 26 ].
MANOVA table for the pigeon example, showing the significant overall orientation (intercept) and treatment effect
Factor | Pillai | approx. F | p |
---|---|---|---|
Intercept | 0.63 | 89.50 | < 0.01 |
Treatment | 0.30 | 9.31 | < 0.01 |
For the bat example, the MANOVA analysis elegantly combined all possible separate analyses in one model and showed that the treatment as well as age-by-treatment interaction were both significant (Table 2 ). Hence, the treatment effect changes with age of the bat. This corroborates (in a much more compact way) the results already presented in the original study by Lindecke et al. [ 27 ]. The overall orientation (intercept) was not significant, which is expected as the treatment groups showed opposite (overall axial) orientation.
MANOVA table for the bat example, showing the significant treatment effect, as well as interaction with age
Factor | Pillai | approx. F | p |
---|---|---|---|
Intercept | 0.02 | 0.52 | 0.598 |
Age | 0.05 | 1.23 | 0.302 |
Treatment | 0.21 | 5.82 | < 0.01 |
Age by treatment | 0.15 | 3.82 | 0.029 |
For the deer example, our approach showed the potential of the MANOVA approach to handle multiple potential explanatory variables. While in the original study numerous separate analyses were made and effect strengths compared [ 28 ], we can show in our rudimentary model selection approach that the axis of the hide as well as the previous alignment of the deer axis influenced the flight direction the most, i.e. were retained in the final model and showed a highly significant p value (Table 3 ). This supports the idea brought forward in the paper by Obleser et al. [ 29 ], that animals tend to align along the magnetic north-south axis, and (even more so) use the same axis as their preferred flight direction.
MANOVA table of the selected model, after eliminating non-significant factors, for the deer example, showing significant effects of the previous axial alignment as well as the hide axis—expressed as cosine and sine of the axial directions in radians. In addition, sun axis, wind speed, wind direction and temperature showed marginal significant/trending effects
Row | Pillai | approx. F | p |
---|---|---|---|
Intercept | 0.07 | 6.56 | < 0.01 |
Cosine of previous alignment | 0.09 | 8.03 | < 0.01 |
Cosine of hide axis | 0.08 | 7.42 | < 0.01 |
Sine of hide axis | 0.08 | 7.11 | < 0.01 |
Sine of sun axis | 0.03 | 2.87 | 0.06 |
Wind speed | 0.03 | 2.67 | 0.072 |
Cosine of wind direction | 0.03 | 2.32 | 0.101 |
Temperature | 0.04 | 3.17 | 0.044 |
Our previous published work demonstrated that MANOVA is as powerful as the available conventional tests to investigate whether two samples come from the same underlying distribution. Here we have demonstrated that the effectiveness of this approach extends much more widely. Firstly, our analysis shows that the MANOVA approach for circular data is as powerful to determine significant departure from uniformity in a single sample of data as the popular and effective Rayleigh test. In addition, adding linear and grouping variables can vastly improve the power of the MANOVA, but also enhance the range of hypothesis testing. If one suspected that a certain linear variable or a grouping factor might be responsible for a change in orientation this can be tested explicitly without applying multiple tests (i.e., avoiding the issue of multiple hypothesis testing and inflating the type I error). There are no restrictions on the type of distributions to be used for this approach.
A potential weakness of the conventional MANOVA approach (where the p values are obtained by theoretical approaches) is the increased type I error rate for very low sample sizes (i.e., over all of n < 15). However, in such cases the p value can be calculated by Monte Carlo simulations, in this case it retains power levels and controls alpha inflation. We provide R code to perform this test in Additional file 1 . If symmetrical multimodal orientations can be expected in a diversity of real-world situations generating circular data, the data can be transformed prior to analysis by MANOVA (see our deer example for axial data). However, if cofactors (or groupings) are not needed, and the type of modality is not known, one might prefer to use the HR test, which is more powerful than most other available options in such a situation.
In contrast to other more sophisticated circular models, e.g. the Bayesian GLM [ 11 ], our approach provides insight into the circular response variable and its deviation from uniformity for single samples, in addition to testing for the significant contributions of groups and linear factors.
In principle, MANOVAs might be used to incorporate random effects as well, e.g., in a repeated-measures MANOVA. However, such approach will require validation for the intercept approach described here, this potentially opens the possibility to perform experiments and accompanying analyses without any of the limitations previously experienced in circular statistics. Thus, the MANOVA approach demonstrated here, in combination with recent developments in Bayesian approaches, offers a substantial broadening of the scientific questions that can be addressed statistically with circular data. The fact that it also performs well when testing very simple hypotheses that are currently evaluated by a collection of specialist tests, should make its wider adoption all the more attractive.
We show that the herein presented MANOVA approach has the potential to extend the range of scientific questions substantially that can be tested statistically using circular data. In addition, using such approach would lead to more powerful analyses than currently possible and paves the way to make the linear statistical toolbox available for circular data.
Acknowledgements
We thank two anonymous reviewers for their valuable comments on an earlier version of this manuscript.
Author contributions
LL, GDR and EPM conceptualized the problem and discussed the analytic approaches. LL prepared the code. LL, GDR and EPM interpreted the results. LL, GDR and EPM wrote the manuscript. All authors read and approved the final manuscript.
LL is supported by the Austrian Science Fund (FWF, Grant Number: P32586). EPM receives funding from the European Research Council (ERC) under the European Union’s Horizon 2020 research and innovation program (Grant Agreement No. 948728).
Availability of data and materials
Declarations.
Not applicable.
All authors gave their consent.
The authors declare that they have no competing interests.
Publisher’s note
Springer Nature remains neutral with regard to jurisdictional claims in published maps and institutional affiliations.
- Search Menu
Sign in through your institution
- Browse content in Arts and Humanities
- Browse content in Archaeology
- Anglo-Saxon and Medieval Archaeology
- Archaeological Methodology and Techniques
- Archaeology by Region
- Archaeology of Religion
- Archaeology of Trade and Exchange
- Biblical Archaeology
- Contemporary and Public Archaeology
- Environmental Archaeology
- Historical Archaeology
- History and Theory of Archaeology
- Industrial Archaeology
- Landscape Archaeology
- Mortuary Archaeology
- Prehistoric Archaeology
- Underwater Archaeology
- Zooarchaeology
- Browse content in Architecture
- Architectural Structure and Design
- History of Architecture
- Residential and Domestic Buildings
- Theory of Architecture
- Browse content in Art
- Art Subjects and Themes
- History of Art
- Industrial and Commercial Art
- Theory of Art
- Biographical Studies
- Byzantine Studies
- Browse content in Classical Studies
- Classical History
- Classical Philosophy
- Classical Mythology
- Classical Numismatics
- Classical Literature
- Classical Reception
- Classical Art and Architecture
- Classical Oratory and Rhetoric
- Greek and Roman Papyrology
- Greek and Roman Epigraphy
- Greek and Roman Law
- Greek and Roman Archaeology
- Late Antiquity
- Religion in the Ancient World
- Social History
- Digital Humanities
- Browse content in History
- Colonialism and Imperialism
- Diplomatic History
- Environmental History
- Genealogy, Heraldry, Names, and Honours
- Genocide and Ethnic Cleansing
- Historical Geography
- History by Period
- History of Emotions
- History of Agriculture
- History of Education
- History of Gender and Sexuality
- Industrial History
- Intellectual History
- International History
- Labour History
- Legal and Constitutional History
- Local and Family History
- Maritime History
- Military History
- National Liberation and Post-Colonialism
- Oral History
- Political History
- Public History
- Regional and National History
- Revolutions and Rebellions
- Slavery and Abolition of Slavery
- Social and Cultural History
- Theory, Methods, and Historiography
- Urban History
- World History
- Browse content in Language Teaching and Learning
- Language Learning (Specific Skills)
- Language Teaching Theory and Methods
- Browse content in Linguistics
- Applied Linguistics
- Cognitive Linguistics
- Computational Linguistics
- Forensic Linguistics
- Grammar, Syntax and Morphology
- Historical and Diachronic Linguistics
- History of English
- Language Evolution
- Language Reference
- Language Acquisition
- Language Variation
- Language Families
- Lexicography
- Linguistic Anthropology
- Linguistic Theories
- Linguistic Typology
- Phonetics and Phonology
- Psycholinguistics
- Sociolinguistics
- Translation and Interpretation
- Writing Systems
- Browse content in Literature
- Bibliography
- Children's Literature Studies
- Literary Studies (Romanticism)
- Literary Studies (American)
- Literary Studies (Asian)
- Literary Studies (European)
- Literary Studies (Eco-criticism)
- Literary Studies (Modernism)
- Literary Studies - World
- Literary Studies (1500 to 1800)
- Literary Studies (19th Century)
- Literary Studies (20th Century onwards)
- Literary Studies (African American Literature)
- Literary Studies (British and Irish)
- Literary Studies (Early and Medieval)
- Literary Studies (Fiction, Novelists, and Prose Writers)
- Literary Studies (Gender Studies)
- Literary Studies (Graphic Novels)
- Literary Studies (History of the Book)
- Literary Studies (Plays and Playwrights)
- Literary Studies (Poetry and Poets)
- Literary Studies (Postcolonial Literature)
- Literary Studies (Queer Studies)
- Literary Studies (Science Fiction)
- Literary Studies (Travel Literature)
- Literary Studies (War Literature)
- Literary Studies (Women's Writing)
- Literary Theory and Cultural Studies
- Mythology and Folklore
- Shakespeare Studies and Criticism
- Browse content in Media Studies
- Browse content in Music
- Applied Music
- Dance and Music
- Ethics in Music
- Ethnomusicology
- Gender and Sexuality in Music
- Medicine and Music
- Music Cultures
- Music and Media
- Music and Religion
- Music and Culture
- Music Education and Pedagogy
- Music Theory and Analysis
- Musical Scores, Lyrics, and Libretti
- Musical Structures, Styles, and Techniques
- Musicology and Music History
- Performance Practice and Studies
- Race and Ethnicity in Music
- Sound Studies
- Browse content in Performing Arts
- Browse content in Philosophy
- Aesthetics and Philosophy of Art
- Epistemology
- Feminist Philosophy
- History of Western Philosophy
- Meta-Philosophy
- Metaphysics
- Moral Philosophy
- Non-Western Philosophy
- Philosophy of Language
- Philosophy of Mind
- Philosophy of Perception
- Philosophy of Science
- Philosophy of Action
- Philosophy of Law
- Philosophy of Religion
- Philosophy of Mathematics and Logic
- Practical Ethics
- Social and Political Philosophy
- Browse content in Religion
- Biblical Studies
- Christianity
- East Asian Religions
- History of Religion
- Judaism and Jewish Studies
- Qumran Studies
- Religion and Education
- Religion and Health
- Religion and Politics
- Religion and Science
- Religion and Law
- Religion and Art, Literature, and Music
- Religious Studies
- Browse content in Society and Culture
- Cookery, Food, and Drink
- Cultural Studies
- Customs and Traditions
- Ethical Issues and Debates
- Hobbies, Games, Arts and Crafts
- Natural world, Country Life, and Pets
- Popular Beliefs and Controversial Knowledge
- Sports and Outdoor Recreation
- Technology and Society
- Travel and Holiday
- Visual Culture
- Browse content in Law
- Arbitration
- Browse content in Company and Commercial Law
- Commercial Law
- Company Law
- Browse content in Comparative Law
- Systems of Law
- Competition Law
- Browse content in Constitutional and Administrative Law
- Government Powers
- Judicial Review
- Local Government Law
- Military and Defence Law
- Parliamentary and Legislative Practice
- Construction Law
- Contract Law
- Browse content in Criminal Law
- Criminal Procedure
- Criminal Evidence Law
- Sentencing and Punishment
- Employment and Labour Law
- Environment and Energy Law
- Browse content in Financial Law
- Banking Law
- Insolvency Law
- History of Law
- Human Rights and Immigration
- Intellectual Property Law
- Browse content in International Law
- Private International Law and Conflict of Laws
- Public International Law
- IT and Communications Law
- Jurisprudence and Philosophy of Law
- Law and Politics
- Law and Society
- Browse content in Legal System and Practice
- Courts and Procedure
- Legal Skills and Practice
- Legal System - Costs and Funding
- Primary Sources of Law
- Regulation of Legal Profession
- Medical and Healthcare Law
- Browse content in Policing
- Criminal Investigation and Detection
- Police and Security Services
- Police Procedure and Law
- Police Regional Planning
- Browse content in Property Law
- Personal Property Law
- Restitution
- Study and Revision
- Terrorism and National Security Law
- Browse content in Trusts Law
- Wills and Probate or Succession
- Browse content in Medicine and Health
- Browse content in Allied Health Professions
- Arts Therapies
- Clinical Science
- Dietetics and Nutrition
- Occupational Therapy
- Operating Department Practice
- Physiotherapy
- Radiography
- Speech and Language Therapy
- Browse content in Anaesthetics
- General Anaesthesia
- Clinical Neuroscience
- Browse content in Clinical Medicine
- Acute Medicine
- Cardiovascular Medicine
- Clinical Genetics
- Clinical Pharmacology and Therapeutics
- Dermatology
- Endocrinology and Diabetes
- Gastroenterology
- Genito-urinary Medicine
- Geriatric Medicine
- Infectious Diseases
- Medical Toxicology
- Medical Oncology
- Pain Medicine
- Palliative Medicine
- Rehabilitation Medicine
- Respiratory Medicine and Pulmonology
- Rheumatology
- Sleep Medicine
- Sports and Exercise Medicine
- Community Medical Services
- Critical Care
- Emergency Medicine
- Forensic Medicine
- Haematology
- History of Medicine
- Browse content in Medical Skills
- Clinical Skills
- Communication Skills
- Nursing Skills
- Surgical Skills
- Browse content in Medical Dentistry
- Oral and Maxillofacial Surgery
- Paediatric Dentistry
- Restorative Dentistry and Orthodontics
- Surgical Dentistry
- Medical Ethics
- Medical Statistics and Methodology
- Browse content in Neurology
- Clinical Neurophysiology
- Neuropathology
- Nursing Studies
- Browse content in Obstetrics and Gynaecology
- Gynaecology
- Occupational Medicine
- Ophthalmology
- Otolaryngology (ENT)
- Browse content in Paediatrics
- Neonatology
- Browse content in Pathology
- Chemical Pathology
- Clinical Cytogenetics and Molecular Genetics
- Histopathology
- Medical Microbiology and Virology
- Patient Education and Information
- Browse content in Pharmacology
- Psychopharmacology
- Browse content in Popular Health
- Caring for Others
- Complementary and Alternative Medicine
- Self-help and Personal Development
- Browse content in Preclinical Medicine
- Cell Biology
- Molecular Biology and Genetics
- Reproduction, Growth and Development
- Primary Care
- Professional Development in Medicine
- Browse content in Psychiatry
- Addiction Medicine
- Child and Adolescent Psychiatry
- Forensic Psychiatry
- Learning Disabilities
- Old Age Psychiatry
- Psychotherapy
- Browse content in Public Health and Epidemiology
- Epidemiology
- Public Health
- Browse content in Radiology
- Clinical Radiology
- Interventional Radiology
- Nuclear Medicine
- Radiation Oncology
- Reproductive Medicine
- Browse content in Surgery
- Cardiothoracic Surgery
- Gastro-intestinal and Colorectal Surgery
- General Surgery
- Neurosurgery
- Paediatric Surgery
- Peri-operative Care
- Plastic and Reconstructive Surgery
- Surgical Oncology
- Transplant Surgery
- Trauma and Orthopaedic Surgery
- Vascular Surgery
- Browse content in Science and Mathematics
- Browse content in Biological Sciences
- Aquatic Biology
- Biochemistry
- Bioinformatics and Computational Biology
- Developmental Biology
- Ecology and Conservation
- Evolutionary Biology
- Genetics and Genomics
- Microbiology
- Molecular and Cell Biology
- Natural History
- Plant Sciences and Forestry
- Research Methods in Life Sciences
- Structural Biology
- Systems Biology
- Zoology and Animal Sciences
- Browse content in Chemistry
- Analytical Chemistry
- Computational Chemistry
- Crystallography
- Environmental Chemistry
- Industrial Chemistry
- Inorganic Chemistry
- Materials Chemistry
- Medicinal Chemistry
- Mineralogy and Gems
- Organic Chemistry
- Physical Chemistry
- Polymer Chemistry
- Study and Communication Skills in Chemistry
- Theoretical Chemistry
- Browse content in Computer Science
- Artificial Intelligence
- Computer Architecture and Logic Design
- Game Studies
- Human-Computer Interaction
- Mathematical Theory of Computation
- Programming Languages
- Software Engineering
- Systems Analysis and Design
- Virtual Reality
- Browse content in Computing
- Business Applications
- Computer Security
- Computer Games
- Computer Networking and Communications
- Digital Lifestyle
- Graphical and Digital Media Applications
- Operating Systems
- Browse content in Earth Sciences and Geography
- Atmospheric Sciences
- Environmental Geography
- Geology and the Lithosphere
- Maps and Map-making
- Meteorology and Climatology
- Oceanography and Hydrology
- Palaeontology
- Physical Geography and Topography
- Regional Geography
- Soil Science
- Urban Geography
- Browse content in Engineering and Technology
- Agriculture and Farming
- Biological Engineering
- Civil Engineering, Surveying, and Building
- Electronics and Communications Engineering
- Energy Technology
- Engineering (General)
- Environmental Science, Engineering, and Technology
- History of Engineering and Technology
- Mechanical Engineering and Materials
- Technology of Industrial Chemistry
- Transport Technology and Trades
- Browse content in Environmental Science
- Applied Ecology (Environmental Science)
- Conservation of the Environment (Environmental Science)
- Environmental Sustainability
- Environmentalist Thought and Ideology (Environmental Science)
- Management of Land and Natural Resources (Environmental Science)
- Natural Disasters (Environmental Science)
- Nuclear Issues (Environmental Science)
- Pollution and Threats to the Environment (Environmental Science)
- Social Impact of Environmental Issues (Environmental Science)
- History of Science and Technology
- Browse content in Materials Science
- Ceramics and Glasses
- Composite Materials
- Metals, Alloying, and Corrosion
- Nanotechnology
- Browse content in Mathematics
- Applied Mathematics
- Biomathematics and Statistics
- History of Mathematics
- Mathematical Education
- Mathematical Finance
- Mathematical Analysis
- Numerical and Computational Mathematics
- Probability and Statistics
- Pure Mathematics
- Browse content in Neuroscience
- Cognition and Behavioural Neuroscience
- Development of the Nervous System
- Disorders of the Nervous System
- History of Neuroscience
- Invertebrate Neurobiology
- Molecular and Cellular Systems
- Neuroendocrinology and Autonomic Nervous System
- Neuroscientific Techniques
- Sensory and Motor Systems
- Browse content in Physics
- Astronomy and Astrophysics
- Atomic, Molecular, and Optical Physics
- Biological and Medical Physics
- Classical Mechanics
- Computational Physics
- Condensed Matter Physics
- Electromagnetism, Optics, and Acoustics
- History of Physics
- Mathematical and Statistical Physics
- Measurement Science
- Nuclear Physics
- Particles and Fields
- Plasma Physics
- Quantum Physics
- Relativity and Gravitation
- Semiconductor and Mesoscopic Physics
- Browse content in Psychology
- Affective Sciences
- Clinical Psychology
- Cognitive Psychology
- Cognitive Neuroscience
- Criminal and Forensic Psychology
- Developmental Psychology
- Educational Psychology
- Evolutionary Psychology
- Health Psychology
- History and Systems in Psychology
- Music Psychology
- Neuropsychology
- Organizational Psychology
- Psychological Assessment and Testing
- Psychology of Human-Technology Interaction
- Psychology Professional Development and Training
- Research Methods in Psychology
- Social Psychology
- Browse content in Social Sciences
- Browse content in Anthropology
- Anthropology of Religion
- Human Evolution
- Medical Anthropology
- Physical Anthropology
- Regional Anthropology
- Social and Cultural Anthropology
- Theory and Practice of Anthropology
- Browse content in Business and Management
- Business Ethics
- Business Strategy
- Business History
- Business and Technology
- Business and Government
- Business and the Environment
- Comparative Management
- Corporate Governance
- Corporate Social Responsibility
- Entrepreneurship
- Health Management
- Human Resource Management
- Industrial and Employment Relations
- Industry Studies
- Information and Communication Technologies
- International Business
- Knowledge Management
- Management and Management Techniques
- Operations Management
- Organizational Theory and Behaviour
- Pensions and Pension Management
- Public and Nonprofit Management
- Social Issues in Business and Management
- Strategic Management
- Supply Chain Management
- Browse content in Criminology and Criminal Justice
- Criminal Justice
- Criminology
- Forms of Crime
- International and Comparative Criminology
- Youth Violence and Juvenile Justice
- Development Studies
- Browse content in Economics
- Agricultural, Environmental, and Natural Resource Economics
- Asian Economics
- Behavioural Finance
- Behavioural Economics and Neuroeconomics
- Econometrics and Mathematical Economics
- Economic History
- Economic Systems
- Economic Methodology
- Economic Development and Growth
- Financial Markets
- Financial Institutions and Services
- General Economics and Teaching
- Health, Education, and Welfare
- History of Economic Thought
- International Economics
- Labour and Demographic Economics
- Law and Economics
- Macroeconomics and Monetary Economics
- Microeconomics
- Public Economics
- Urban, Rural, and Regional Economics
- Welfare Economics
- Browse content in Education
- Adult Education and Continuous Learning
- Care and Counselling of Students
- Early Childhood and Elementary Education
- Educational Equipment and Technology
- Educational Strategies and Policy
- Higher and Further Education
- Organization and Management of Education
- Philosophy and Theory of Education
- Schools Studies
- Secondary Education
- Teaching of a Specific Subject
- Teaching of Specific Groups and Special Educational Needs
- Teaching Skills and Techniques
- Browse content in Environment
- Applied Ecology (Social Science)
- Climate Change
- Conservation of the Environment (Social Science)
- Environmentalist Thought and Ideology (Social Science)
- Management of Land and Natural Resources (Social Science)
- Natural Disasters (Environment)
- Pollution and Threats to the Environment (Social Science)
- Social Impact of Environmental Issues (Social Science)
- Sustainability
- Browse content in Human Geography
- Cultural Geography
- Economic Geography
- Political Geography
- Browse content in Interdisciplinary Studies
- Communication Studies
- Museums, Libraries, and Information Sciences
- Browse content in Politics
- African Politics
- Asian Politics
- Chinese Politics
- Comparative Politics
- Conflict Politics
- Elections and Electoral Studies
- Environmental Politics
- Ethnic Politics
- European Union
- Foreign Policy
- Gender and Politics
- Human Rights and Politics
- Indian Politics
- International Relations
- International Organization (Politics)
- Irish Politics
- Latin American Politics
- Middle Eastern Politics
- Political Behaviour
- Political Economy
- Political Institutions
- Political Methodology
- Political Communication
- Political Philosophy
- Political Sociology
- Political Theory
- Politics and Law
- Politics of Development
- Public Policy
- Public Administration
- Qualitative Political Methodology
- Quantitative Political Methodology
- Regional Political Studies
- Russian Politics
- Security Studies
- State and Local Government
- UK Politics
- US Politics
- Browse content in Regional and Area Studies
- African Studies
- Asian Studies
- East Asian Studies
- Japanese Studies
- Latin American Studies
- Middle Eastern Studies
- Native American Studies
- Scottish Studies
- Browse content in Research and Information
- Research Methods
- Browse content in Social Work
- Addictions and Substance Misuse
- Adoption and Fostering
- Care of the Elderly
- Child and Adolescent Social Work
- Couple and Family Social Work
- Direct Practice and Clinical Social Work
- Emergency Services
- Human Behaviour and the Social Environment
- International and Global Issues in Social Work
- Mental and Behavioural Health
- Social Justice and Human Rights
- Social Policy and Advocacy
- Social Work and Crime and Justice
- Social Work Macro Practice
- Social Work Practice Settings
- Social Work Research and Evidence-based Practice
- Welfare and Benefit Systems
- Browse content in Sociology
- Childhood Studies
- Community Development
- Comparative and Historical Sociology
- Disability Studies
- Economic Sociology
- Gender and Sexuality
- Gerontology and Ageing
- Health, Illness, and Medicine
- Marriage and the Family
- Migration Studies
- Occupations, Professions, and Work
- Organizations
- Population and Demography
- Race and Ethnicity
- Social Theory
- Social Movements and Social Change
- Social Research and Statistics
- Social Stratification, Inequality, and Mobility
- Sociology of Religion
- Sociology of Education
- Sport and Leisure
- Urban and Rural Studies
- Browse content in Warfare and Defence
- Defence Strategy, Planning, and Research
- Land Forces and Warfare
- Military Administration
- Military Life and Institutions
- Naval Forces and Warfare
- Other Warfare and Defence Issues
- Peace Studies and Conflict Resolution
- Weapons and Equipment

- < Previous chapter
- Next chapter >
2 Multivariate Analysis of Variance: Overview and Key Concepts
- Published: February 2013
- Cite Icon Cite
- Permissions Icon Permissions
This chapter presents multivariate analysis of variance (MANOVA) as a generalization of the t -test and analysis of variance (ANOVA). The t -test is a strategy to test hypotheses about differences between two groups on a single mean. When there are more than two means, it is possible to use a series of t -tests to evaluate hypotheses about the difference between each pair of group means (e.g., for three groups, there are three unique pairs of means). However, conducting multiple t -tests can lead to inflation of the type I error rate. Consequently, ANOVA is used to test hypotheses about differences between three or more groups on a single mean; and MANOVA is a strategy to test hypotheses about differences between two or more groups on two or more means (i.e., a vector of means). As a generalization of the t -test and ANOVA, MANOVA may be understood as the ratio between two measures of multivariate variance.
Personal account
- Sign in with email/username & password
- Get email alerts
- Save searches
- Purchase content
- Activate your purchase/trial code
- Add your ORCID iD
Institutional access
Sign in with a library card.
- Sign in with username/password
- Recommend to your librarian
- Institutional account management
- Get help with access
Access to content on Oxford Academic is often provided through institutional subscriptions and purchases. If you are a member of an institution with an active account, you may be able to access content in one of the following ways:
IP based access
Typically, access is provided across an institutional network to a range of IP addresses. This authentication occurs automatically, and it is not possible to sign out of an IP authenticated account.
Choose this option to get remote access when outside your institution. Shibboleth/Open Athens technology is used to provide single sign-on between your institution’s website and Oxford Academic.
- Click Sign in through your institution.
- Select your institution from the list provided, which will take you to your institution's website to sign in.
- When on the institution site, please use the credentials provided by your institution. Do not use an Oxford Academic personal account.
- Following successful sign in, you will be returned to Oxford Academic.
If your institution is not listed or you cannot sign in to your institution’s website, please contact your librarian or administrator.
Enter your library card number to sign in. If you cannot sign in, please contact your librarian.
Society Members
Society member access to a journal is achieved in one of the following ways:
Sign in through society site
Many societies offer single sign-on between the society website and Oxford Academic. If you see ‘Sign in through society site’ in the sign in pane within a journal:
- Click Sign in through society site.
- When on the society site, please use the credentials provided by that society. Do not use an Oxford Academic personal account.
If you do not have a society account or have forgotten your username or password, please contact your society.
Sign in using a personal account
Some societies use Oxford Academic personal accounts to provide access to their members. See below.
A personal account can be used to get email alerts, save searches, purchase content, and activate subscriptions.
Some societies use Oxford Academic personal accounts to provide access to their members.
Viewing your signed in accounts
Click the account icon in the top right to:
- View your signed in personal account and access account management features.
- View the institutional accounts that are providing access.
Signed in but can't access content
Oxford Academic is home to a wide variety of products. The institutional subscription may not cover the content that you are trying to access. If you believe you should have access to that content, please contact your librarian.
For librarians and administrators, your personal account also provides access to institutional account management. Here you will find options to view and activate subscriptions, manage institutional settings and access options, access usage statistics, and more.
Our books are available by subscription or purchase to libraries and institutions.
Month: | Total Views: |
---|---|
October 2022 | 5 |
November 2022 | 13 |
December 2022 | 3 |
January 2023 | 5 |
February 2023 | 4 |
March 2023 | 16 |
April 2023 | 8 |
May 2023 | 7 |
June 2023 | 5 |
July 2023 | 10 |
August 2023 | 6 |
September 2023 | 6 |
October 2023 | 8 |
November 2023 | 9 |
December 2023 | 12 |
January 2024 | 2 |
February 2024 | 8 |
March 2024 | 5 |
April 2024 | 8 |
May 2024 | 4 |
June 2024 | 6 |
July 2024 | 7 |
August 2024 | 8 |
September 2024 | 7 |
- About Oxford Academic
- Publish journals with us
- University press partners
- What we publish
- New features
- Open access
- Rights and permissions
- Accessibility
- Advertising
- Media enquiries
- Oxford University Press
- Oxford Languages
- University of Oxford
Oxford University Press is a department of the University of Oxford. It furthers the University's objective of excellence in research, scholarship, and education by publishing worldwide
- Copyright © 2024 Oxford University Press
- Cookie settings
- Cookie policy
- Privacy policy
- Legal notice
This Feature Is Available To Subscribers Only
Sign In or Create an Account
This PDF is available to Subscribers Only
For full access to this pdf, sign in to an existing account, or purchase an annual subscription.
- Published: 01 March 2004
Statistics review 9: One-way analysis of variance
- Viv Bewick 1 ,
- Liz Cheek 1 &
- Jonathan Ball 2
Critical Care volume 8 , Article number: 130 ( 2004 ) Cite this article
52k Accesses
235 Citations
2 Altmetric
Metrics details
This review introduces one-way analysis of variance, which is a method of testing differences between more than two groups or treatments. Multiple comparison procedures and orthogonal contrasts are described as methods for identifying specific differences between pairs of treatments.
Introduction
Analysis of variance (often referred to as ANOVA) is a technique for analyzing the way in which the mean of a variable is affected by different types and combinations of factors. One-way analysis of variance is the simplest form. It is an extension of the independent samples t-test (see statistics review 5 [ 1 ]) and can be used to compare any number of groups or treatments. This method could be used, for example, in the analysis of the effect of three different diets on total serum cholesterol or in the investigation into the extent to which severity of illness is related to the occurrence of infection.
Analysis of variance gives a single overall test of whether there are differences between groups or treatments. Why is it not appropriate to use independent sample t-tests to test all possible pairs of treatments and to identify differences between treatments? To answer this it is necessary to look more closely at the meaning of a P value.
When interpreting a P value, it can be concluded that there is a significant difference between groups if the P value is small enough, and less than 0.05 (5%) is a commonly used cutoff value. In this case 5% is the significance level, or the probability of a type I error. This is the chance of incorrectly rejecting the null hypothesis (i.e. incorrectly concluding that an observed difference did not occur just by chance [ 2 ]), or more simply the chance of wrongly concluding that there is a difference between two groups when in reality there no such difference.
If multiple t-tests are carried out, then the type I error rate will increase with the number of comparisons made. For example, in a study involving four treatments, there are six possible pairwise comparisons. (The number of pairwise comparisons is given by 4 C 2 and is equal to 4!/ [2!2!], where 4! = 4 × 3 × 2 × 1.) If the chance of a type I error in one such comparison is 0.05, then the chance of not committing a type I error is 1 - 0.05 = 0.95. If the six comparisons can be assumed to be independent (can we make a comment or reference about when this assumption cannot be made?), then the chance of not committing a type I error in any one of them is 0.95 6 = 0.74. Hence, the chance of committing a type I error in at least one of the comparisons is 1 - 0.74 = 0.26, which is the overall type I error rate for the analysis. Therefore, there is a 26% overall type I error rate, even though for each individual test the type I error rate is 5%. Analysis of variance is used to avoid this problem.
One-way analysis of variance
In an independent samples t-test, the test statistic is computed by dividing the difference between the sample means by the standard error of the difference. The standard error of the difference is an estimate of the variability within each group (assumed to be the same). In other words, the difference (or variability) between the samples is compared with the variability within the samples.
In one-way analysis of variance, the same principle is used, with variances rather than standard deviations being used to measure variability. The variance of a set of n values (x 1 , x 2 ... x n ) is given by the following (i.e. sum of squares divided by the degrees of freedom):
Where the sum of squares =
and the degrees of freedom = n - 1
Analysis of variance would almost always be carried out using a statistical package, but an example using the simple data set shown in Table 1 will be used to illustrate the principles involved.
The grand mean of the total set of observations is the sum of all observations divided by the total number of observations. For the data given in Table 1 , the grand mean is 16. For a particular observation x, the difference between x and the grand mean can be split into two parts as follows:
x - grand mean = (treatment mean - grand mean) + (x - treatment mean)
Total deviation = deviation explained by treatment + unexplained deviation (residual)
This is analogous to the regression situation (see statistics review 7 [ 3 ]) with the treatment mean forming the fitted value. This is shown in Table 2 .
The total sum of squares for the data is similarly partitioned into a 'between treatments' sum of squares and a 'within treatments' sum of squares. The within treatments sum of squares is also referred to as the error or residual sum of squares.
The degrees of freedom (df) for these sums of squares are as follows:
Total df = n - 1 (where n is the total number of observations) = 9 - 1 = 8
Between treatments df = number of treatments - 1 = 3 - 1 = 2
Within treatments df = total df - between treatments df = 8 - 2 = 6
This partitioning of the total sum of squares is presented in an analysis of variance table (Table 3 ). The mean squares (MS), which correspond to variance estimates, are obtained by dividing the sums of squares (SS) by their degrees of freedom.
The test statistic F is equal to the 'between treatments' mean square divided by the error mean square. The P value may be obtained by comparison of the test statistic with the F distribution with 2 and 6 degrees of freedom (where 2 is the number of degrees of freedom for the numerator and 6 for the denominator). In this case it was obtained from a statistical package. The P value of 0.0039 indicates that at least two of the treatments are different.
As a published example we shall use the results of an observational study into the prevalence of infection among intensive care unit (ICU) patients. One aspect of the study was to investigate the extent to which severity of illness was related to the occurrence of infection. Patients were categorized according to the presence of infection. The categories used were no infection, infection on admission, ICU-acquired infection, and both infection on admission and ICU-acquired infection. (These are referred to as infection states 1–4.) To assess the severity of illness, the Simplified Acute Physiology Score (SAPS) II system was used [ 4 ]. Findings in 400 patients (100 in each category) were analyzed. (It is not necessary to have equal sample sizes.) Table 4 shows some of the scores together with the sample means and standard deviations for each category of infection. The whole data set is illustrated in Fig. 1 using box plots.
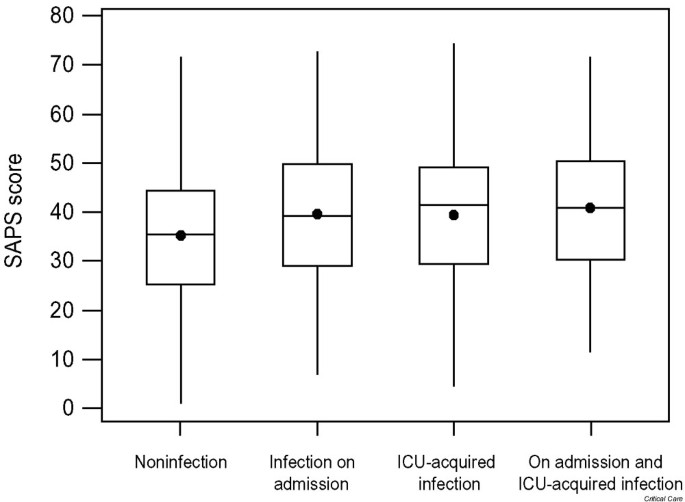
Box plots of the Simplified Acute Physiology Score (SAPS) scores according to infection. Means are shown by dots, the boxes represent the median and the interquartile range with the vertical lines showing the range. ICU, intensive care unit.
The analysis of variance output using a statistical package is shown in Table 5 .
Multiple comparison procedures
When a significant effect has been found using analysis of variance, we still do not know which means differ significantly. It is therefore necessary to conduct post hoc comparisons between pairs of treatments. As explained above, when repeated t-tests are used, the overall type I error rate increases with the number of pairwise comparisons. One method of keeping the overall type I error rate to 0.05 would be to use a much lower pairwise type I error rate. To calculate the pairwise type I error rate α needed to maintain a 0.05 overall type I error rate in our four observational group example, we use 1 - (1 - α) N = 0.05, where N is the number of possible pairwise comparisons. In this example there were four means, giving rise to six possible comparisons. Rearranging this gives α = 1 - (0.95) 1/6 = 0.0085. A method of approximating this calculated value is attributed to Bonferoni. In this method the overall type I error rate is divided by the number of comparisons made, to give a type I error rate for the pairwise comparison. In our four treatment example, this would be 0.05/6 = 0.0083, indicating that a difference would only be considered significant if the P value were below 0.0083. The Bonferoni method is often regarded as too conservative (i.e. it fails to detect real differences).
There are a number of specialist multiple comparison tests that maintain a low overall type I error. Tukey's test and Duncan's multiple-range test are two of the procedures that can be used and are found in most statistical packages.
Duncan's multiple-range test
We use the data given in Table 4 to illustrate Duncan's multiple-range test. This procedure is based on the comparison of the range of a subset of the sample means with a calculated least significant range. This least significant range increases with the number of sample means in the subset. If the range of the subset exceeds the least significant range, then the population means can be considered significantly different. It is a sequential test and so the subset with the largest range is compared first, followed by smaller subsets. Once a range is found not to be significant, no further subsets of this group are tested.
The least significant range, R p , for subsets of p sample means is given by:
Where r p is called the least significant studentized range and depends upon the error degrees of freedom and the numbers of means in the subset. Tables of these values can be found in many statistics books [ 5 ]; s 2 is the error mean square from the analysis of variance table, and n is the sample size for each treatment. For the data in Table 4 , s 2 = 208.9, n = 100 (if the sample sizes are not equal, then n is replaced with the harmonic mean of the sample sizes [ 5 ]) and the error degrees of freedom = 396. So, from the table of studentized ranges [ 5 ], r 2 = 2.77, r 3 = 2.92 and r 4 = 3.02. The least significant range (R p ) for subsets of 2, 3 and 4 means are therefore calculated as R 2 = 4.00, R 3 = 4.22 and R 4 = 4.37.
To conduct pairwise comparisons, the sample means must be ordered by size:
The subset with the largest range includes all four infections, and this will compare infection 4 with infection 1. The range of that subset is the difference between the sample means
Sequentially, we now need to compare subsets of three groups (i.e. infection state 2 with infection state 1, and infection state 4 with infection state 3):
As the range of infection states 4 to 3 was not significant, no smaller subsets within that range can be compared. This leaves a single two-group subset to be compared, namely that of infection 3 with infection 1:
Table 6 gives the output from a statistical package showing the results of Duncan's multiple-range test on the data from Table 4 .
In some investigations, specific comparisons between sets of means may be suggested before the data are collected. These are called planned or a priori comparisons. Orthogonal contrasts may be used to partition the treatment sum of squares into separate components according to the number of degrees of freedom. The analysis of variance for the SAPS II data shown in Table 5 gives a between infection state, sum of squares of 1780.2 with three degrees of freedom. Suppose that, in advance of carrying out the study, it was required to compare the SAPS II scores of patients with no infection with the other three infection categories collectively. We denote the true population mean SAPS II scores for the four infection categories by μ 1 , μ 2 , μ 3 and μ 4 , with μ 1 being the mean for the no infection group. The null hypothesis states that the mean for the no infection group is equal to the average of the other three means. This can be written as follows:
μ 1 = (μ 2 + μ 3 + μ 4)/3 (i.e. 3μ 1 - μ 2 - μ 3 - μ 4 = 0)
The coefficients of μ 1 , μ 2 , μ 3 and μ 4 (3, -1, -1 and -1) are called the contrast coefficients and must be specified in a statistical package in order to conduct the hypothesis test. Each contrast of this type (where differences between means are being tested) has one degree of freedom. For the SAPS II data, two further contrasts, which are orthogonal (i.e. independent), are therefore possible. These could be, for example, a contrast between infection states 3 and 4, and a contrast between infection state 2 and infection states 3 and 4 combined. The coefficients for these three contrasts are given in Table 7 .
The calculation of the contrast sum of squares has been conducted using a statistical package and the results are shown in Table 8 . The sums of squares for the contrasts add up to the infection sum of squares. Contrast 1 has a P value of 0.006, indicating a significant difference between the no infection group and the other three infection groups collectively. The other two contrasts are not significant.
Polynomial contrasts
Where the treatment levels have a natural order and are equally spaced, it may be of interest to test for a trend in the treatment means. Again, this can be carried out using appropriate orthogonal contrasts. For example, in an investigation to determine whether the plasma colloid osmotic pressure (COP) of healthy infants was related to age, the plasma COP of 10 infants from each of three age groups, 1–4 months, 5–8 months and 9–12 months, was measured. The data are given in Table 9 and illustrated in Fig. 2 .
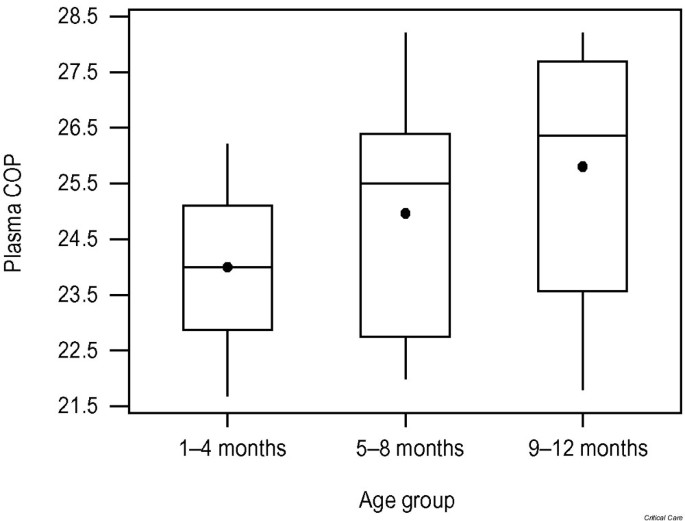
Box plots of plasma colloid osmotic pressure (COP) for each age group. Means are shown by dots, boxes indicate median and interquartile range, with vertical lines depicting the range.
With three age groups we can test for a linear and a quadratic trend. The orthogonal contrasts for these trends are set up as shown in Table 10 . The linear contrast compares the lowest with the highest age group, and the quadratic contrast compares the middle age group with the lowest and highest age groups together.
The analysis of variance with the tests for the trends is given in Table 11 . The P value of 0.138 indicates that there is no overall difference between the mean plasma COP levels at each age group. However, the linear contrast with a P value of 0.049 indicates that there is a significant linear trend, suggesting that plasma COP does increase with age in infants. The quadratic contrast is not significant.
Assumptions and limitations
The underlying assumptions for one-way analysis of variance are that the observations are independent and randomly selected from Normal populations with equal variances. It is not necessary to have equal sample sizes.
The assumptions can be assessed by looking at plots of the residuals. The residuals are the differences between the observed and fitted values, where the fitted values are the treatment means. Commonly, a plot of the residuals against the fitted values and a Normal plot of residuals are produced. If the variances are equal then the residuals should be evenly scattered around zero along the range of fitted values, and if the residuals are Normally distributed then the Normal plot will show a straight line. The same methods of assessing the assumptions are used in regression and are discussed in statistics review 7 [ 3 ].
If the assumptions are not met then it may be possible to transform the data. Alternatively the Kruskal-Wallis nonparametric test could be used. This test will be covered in a future review.
Figs 3 and 4 show the residual plots for the data given in Table 4 . The plot of fitted values against residuals suggests that the assumption of equal variance is reasonable. The Normal plot suggests that the distribution of the residuals is approximately Normal.
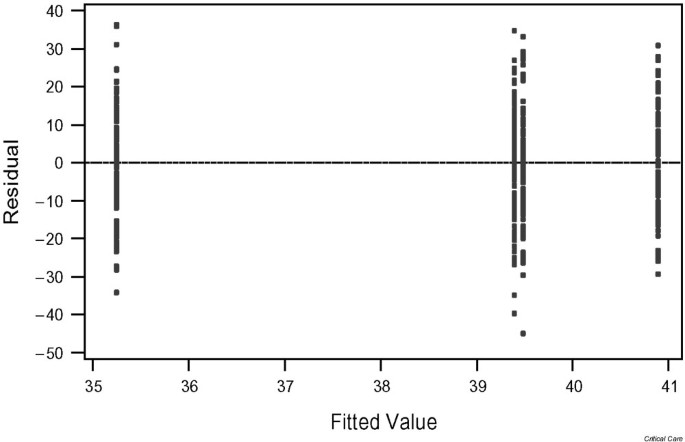
Plot of residuals versus fits for the data in Table 4 . Response is Simplified Acute Physiology Score.
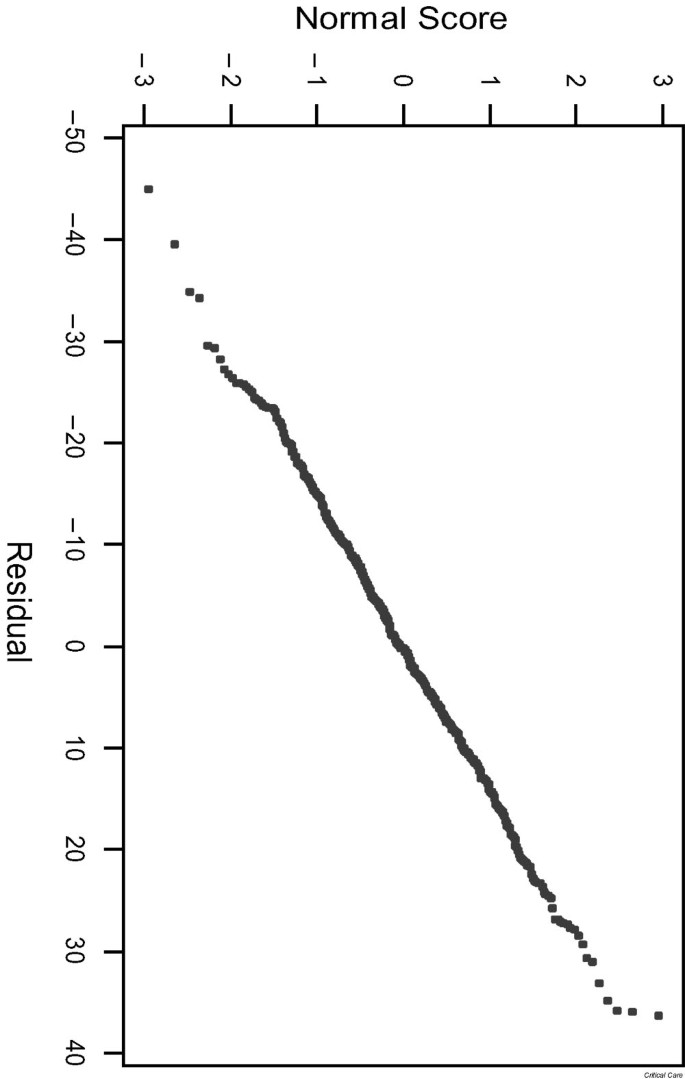
Normal probability plot of residuals for the data in 4 . Response is Simplified Acute Physiology Score.
One-way analysis of variance is used to test for differences between more than two groups or treatments. Further investigation of the differences can be carried out using multiple comparison procedures or orthogonal contrasts.
Data from studies with more complex designs can also be analyzed using analysis of variance (e.g. see Armitage and coworkers [ 6 ] or Montgomery [ 5 ]).
Abbreviations
colloid osmotic pressure
degrees of freedom
intensive care unit
Simplified Acute Physiology Score.
Whitely E, Ball J: Statistics review 5: Comparison of means. Crit Care 2002, 6: 424-428. 10.1186/cc1548
Article Google Scholar
Bland M: An Introduction to Medical Statistics 3 Edition Oxford, UK: Oxford University Press 2001.
Google Scholar
Bewick V, Cheek L, Ball J: Statistics review 7: Correlation and Regression. Crit Care 2003, 7: 451-459. 10.1186/cc2401
Article PubMed Central PubMed Google Scholar
Le Gall JR, Lemeshow S, Saulnier F: A new simplified acute physiology score (SAPS II) based on a European/North American multicenter study. JAMA 1993, 270: 2957-2963. 10.1001/jama.270.24.2957
Article CAS PubMed Google Scholar
Montgomery DC: Design and Analysis of Experiments 4 Edition New York, USA: Wiley 1997.
Armitage P, Berry G, Matthews JNS: Statistical Methods in Medical Research 4 Edition Oxford, UK: Blackwell Science 2002.
Book Google Scholar
Download references
Author information
Authors and affiliations.
Senior Lecturer, School of Computing, Mathematical and Information Sciences, University of Brighton, Brighton, UK
Viv Bewick & Liz Cheek
Lecturer in Intensive Care Medicine, St George's Hospital Medical School, London, UK
Jonathan Ball
You can also search for this author in PubMed Google Scholar
Corresponding author
Correspondence to Viv Bewick .
Additional information
Competing interests.
None declared.
Rights and permissions
Reprints and permissions
About this article
Cite this article.
Bewick, V., Cheek, L. & Ball, J. Statistics review 9: One-way analysis of variance. Crit Care 8 , 130 (2004). https://doi.org/10.1186/cc2836
Download citation
Published : 01 March 2004
DOI : https://doi.org/10.1186/cc2836
Share this article
Anyone you share the following link with will be able to read this content:
Sorry, a shareable link is not currently available for this article.
Provided by the Springer Nature SharedIt content-sharing initiative
- analysis of variance
- multiple comparisons
- orthogonal contrasts
- type I error
Critical Care
ISSN: 1364-8535
- Submission enquiries: [email protected]
Analyses of Variance - Science topic

- Recruit researchers
- Join for free
- Login Email Tip: Most researchers use their institutional email address as their ResearchGate login Password Forgot password? Keep me logged in Log in or Continue with Google Welcome back! Please log in. Email · Hint Tip: Most researchers use their institutional email address as their ResearchGate login Password Forgot password? Keep me logged in Log in or Continue with Google No account? Sign up
- Open access
- Published: 14 September 2024
Development of an infertility perception scale for women (IPS-W)
- Miok Kim 1 &
- Minkyung Ban 2
BMC Women's Health volume 24 , Article number: 513 ( 2024 ) Cite this article
Metrics details
The purpose of this study was to develop an Infertility Perception Scale for Women (IPS-W).
Initial items were based on an extensive literature review and in-depth interviews with five infertile women and fifteen women not diagnosed with infertility. Forty-one items were derived from a pilot survey. Data were collected from 203 women who had experienced intrauterine insemination (IUI) and in-vitro fertilization (IVF) more than once. The data were analyzed to verify the reliability and validity of the scale.
Four factors containing 21 items were extracted from the exploratory factor analysis (EFA) to verify the construct validity. The four factors of infertility perception scale were perceived feelings, personal stigma, social stigma, and acceptance. These factors explained 59.3% of the total variance. The confirmatory factor analysis (CFA) confirmed a four-factor structure of the 21-item IPS-W. All fit indices were satisfactory (χ 2 /df ≤ 3, RMSEA < 0.08). These items were verified through convergent, discriminant, known group validity, concurrent validity testing. The internal consistency reliability was acceptable (Cronbach’s α = 0.90).
The scale reflects the perception of infertility within the cultural context of Korea. The findings can help nurses provide support that is appropriate for individual circumstances by examining how women experiencing infertility perceive infertility.
Peer Review reports
Introduction
Infertility, defined as the failure to achieve pregnancy within one year of regular unprotected sexual intercourse for couples of reproductive age, is perceived in the socio-cultural context of Korea as an impediment to an essential social rite of passage and a health problem that threatens family continuity [ 1 ]. In fact, Koreans struggling with infertility believe that giving birth to a child is a prerequisite for a stable marital relationship and that having children allows the development of family relationships [ 2 ].
Infertility is perceived negatively due to the uncertainty, loss of control, and stressful situations [ 3 ] it causes, having a negative impact on quality of life [ 4 ]. Accordingly, decisions about treatment procedures should be made by each individual and as a couple after exploring various options and with an appropriate social support system. However, individuals with infertility often decide on infertility treatment without an appropriate decision-making process, due to the fear of experiencing social prejudice and negative perceptions when revealing infertility problems [ 5 ].
The psychological difficulties faced by individuals with infertility are partly caused by negative social perceptions [ 5 , 6 ]. Approximately half of all infertile couples tend to hide their infertility problem out of fear of social stigma [ 7 ]. Such stigmatizing views of infertility create a vicious cycle leading infertile individuals to have a negative perception of their problem, avoid or prematurely discontinue infertility treatment, and feel isolated from society [ 5 ].
Harzif, Santawi, and Wijaya [ 8 ] compared differences in perceptions towards infertility treatment between urban and rural areas by examining the level of knowledge on the risk factors for infertility, attitudes towards infertility, the social impact of infertility, and other options for infertile couples. However, as yet, the perceptions of the individuals receiving infertility treatment have not been specifically measured [ 9 ]. Despite the limited tools available for measuring infertility perceptions, some studies have assessed the stigmatizing characteristics of infertility; however, in most cases, the term “infertility” does not appear in the generic tools for measuring stigma [ 7 , 10 , 11 ]. Taebe et al. [ 5 ] developed the female infertility stigma instrument (ISI-F) based on the premise that infertility represents one of the biggest challenges in female reproductive and sexual health in most societies. However, the development of ISI-F relied on females attempting natural pregnancy, including ovulation induction. Consequently, while it shares some of the stigmatizing characteristics of infertile individuals, this tool may not adequately reflect the psycho-emotional difficulties of individuals receiving infertility treatments. The Infertility Stigma Scale (ISS) developed by Fu et al. [ 12 ] is designed to measure the perceived self-stigmas that a female receiving infertility treatment places on herself. However, the scale only encompasses the stigmatizing characteristics of infertility, therefore it does not allow for the assessement of the general perception that females undergoing infertility treatment have about their infertility, especially in a Korean context. The concept of stigma refers to a psychological attitude linked to a series of negative outcomes [ 12 ]. In contrast, perception refers to the process of recognizing and interpreting the nature and meaning of all types of stimuli and may vary depending on how individuals interpret the situation and the society they live in. Perception can be used to explore problems and make decisions on what should be changed and the strategy ahead [ 13 ]. Self-perceived stigma acts as a stress factor that leads to negative social stigma about infertility and interferes with life adaptation [ 14 ]; hence, it is all the more important to improve the perceptions of infertility.
Therefore, this study aims to develop a sensitive tool to measure how women who experience infertility treatment perceive their infertility, positioning them as active agents in coping with infertility. The goal is to assess their personal perceptions of infertility and facilitate a positive shift in those perceptions.
The development and validation of the infertility perception scale was performed in accordance with the method proposed by Devellis [ 15 ]. This study adheres to the Strengthening the Reporting of Observational Studies in Epidemiology (STROBE) guidelines.
Development of the infertility perception scale
Development of preliminary items.
To identify the components of infertility perception, previous literature published in RISS, PUBMED, EMBASE, and NDSL were searched for relevant studies and existing tools in English or Korean. Only studies with full-text availability were included. The search keywords included “infertility,” “fertility,” “infertile women,” “infertility for women,” “infertility experiences,” “experiences of infertility,” “assisted reproductive technology,” “intrauterine insemination,” “in vitro fertilization,” “perception,” and “awareness.” Among articles from PubMed, EMBase, and RISS, a total 42 articles were selected and reviewed, excluding duplicate articles ( n = 102), studies with no women subjects ( n = 48), studies not relevant to perception ( n = 205), systematic reviews ( n = 6), and case studies ( n = 21).
In-depth interviews were then conducted to confirm the initially identified items of infertility perception. The interviews were conducted separately for women who had not experienced infertility and women who were receiving infertility treatment to avoid the possible influence of social perception on the perception of infertility among the women under treatment [ 16 ]. Moreover, the emotional ups and downs experienced by individuals receiving infertility treatment may vary significantly depending on the number of follicles, the number and quality of the collected eggs, the need to undergo repetitive infertility treatments, and added negative emotions from previous failed treatments.
The women without experience of infertility were married women aged 20–69 years (three women per age group) selected by purposive sampling with consideration of their education level, economic status, and type of residence. The interviews, which lasted between 20 and 40 min, were conducted between April 7 and April 20, 2020.
Subsequently, interviews were conducted on six women undergoing IVF procedures to identify the central concept of infertility perception. Announcements were posted inside treatment centers to recruit potential participants. The interviews, which lasted between 50 and 70 min, were conducted between May 10 and May 18, 2020.
The central question in the interviews was “How do you feel about infertility?” The supplementary question for women not diagnosed with infertility was “What did you think when you found out someone who was diagnosed with infertility or has experienced receiving infertility treatment?” The supplementary question for women receiving infertility treatment was “What feelings or thoughts did you have as you were diagnosed with infertility and undergoing infertility treatment?” The in-depth interviews were recorded with the consent of the participants, transcribed immediately, and analyzed according to the content analysis procedure proposed by Krippendorff [ 17 ].
After defining the conceptual framework through content analysis based on literature review and in-depth interviews, a total of 103 items were derived. Subsequently, duplicate items and those with unclear content were deleted, revised, or supplemented through a meeting with an expert with infertility nursing and research experience. As a result, a total of 66 preliminary items were derived, including 29, 18, and 19 items in personal, relational, and social dimensions, respectively.
To avoid central bias, during the instrument development process, the 4-point scale proposed by Lynn [ 18 ] was used to grade each item based on the level of agreement (1: “Strongly disagree” to 4: “Strongly agree”). The items in sections 1, 2, and 3 of the scale are reverse-scored, so that a higher total score indicates a more positive perception of infertility.
Content validity testing
In this study, content validity was tested twice by calculating the content validity index (CVI) based on expert opinion. The appropriateness of each item was assessed using a 4-point Likert scale (4: “Highly relevant”; 3: “Quite relevant”; 2: “Somewhat relevant”; and 1: “Not relevant”). Items with an Item-Content Validity Index (I-CVI) ≥ 0.78 were selected. The first content validity testing was conducted in September 2020 by a 10-member expert panel [ 18 ].
The second content validity testing was conducted by five members from the first 10-member expert panel. Items with I-CVI ≥ 0.78 were selected, leading to a total of 41 preliminary items.

Pilot study
A pilot study was conducted with 20 women living in city B who had been diagnosed with infertility and received at least one round of assisted reproductive therapy to assess the level of understanding about the instrument and the time required to complete the questionnaire. The number of participants was based on the sample size of 20–40 participants for pilot studies proposed by Devellis [ 15 ]. Item appropriateness was assessed through questions such as “Are there any items that are difficult to understand?”, “Are there any items with ambiguous expressions?”, and “Are there any items that you believe lack relevance to the perception of infertility?”
Instrument evaluation
The instrument was assessed through item analysis, exploratory factor analysis (EFA), confirmatory factor analysis (CFA), convergent validity, discriminant validity, criterion validity, and reliability testing.
The subjects were married women aged 20 and above diagnosed with infertility; had received at least one round of IUI or IVF; currently receiving infertility treatment; understood the study’s purpose; and signed an informed consent. Those who had difficulties in understanding and responding to the self-reported questionnaire; had problems with cognitive comprehension; or had other physical disorders in addition to infertility were excluded. Based on the criteria that a sample size more than 200 or approximately five times the number of items would be appropriate for factor analysis [ 15 , 19 ] and considering a dropout rate of 10%, a total of 212 subjects were recruited.
Data was collected from eligible, voluntary participants from two hospitals between November 30, 2020 and February 5, 2021. In our study, a high valid response rate of 94.9% (203 valid responses out of 214 recruited participants) was achieved. This was primarily attributed to the structured approach in participant recruitment and data collection. Participants were initially approached by a researcher or trained assistant at the infertility treatment hospital, who provided a detailed explanation of the study’s purpose, the voluntary nature of participation, and assurances of confidentiality. Only those who expressed voluntary willingness to participate were provided with a QR code to access an online survey, which took approximately 15 to 20 min to complete. Conducting the survey during patients’ waiting times further facilitated participation. These factors collectively ensured that participants felt informed and comfortable in participating in the study.
For the concurrent validity, ISS developed by Fu et al. [ 12 ] was used. After obtaining permission, ISS was translated into Korean and was reviewed. Subsequently, the translated version was translated back into the original language and compared with the original items. The final version consisted of 27 items in Korean. The self-reported instrument consisted of four domains (self-devaluation, social withdrawal, public stigma, and family stigma) in 5-point scale (1: “Do not agree at all” to 5: “Strongly agree”). At the time of development, the reliability of the scale was indicated by Cronbach’s alpha = 0.94. Cronbach’s alpha was 0.86, 0.77, 0.92, and 0.84 for self-devaluation, social withdrawal, public stigma, and family stigma, respectively. In this study, the reliability of the scale was indicated by Cronbach’s alpha = 0.97. Cronbach’s alpha was 0.94, 0.86, 0.95, and 0.91 for self-devaluation, social withdrawal, public stigma, and family stigma, respectively.
Based on the evidence that a stigmatizing perception of infertility is associated with greater experience of negative emotions such as depression and anxiety [ 20 ], the Korean version of the Center for Epidemiologic Studies Depression Scale-Revised (K-CESD-R) [ 21 ] was used for known-group validity. The scale measures the severity of depressive symptoms according to four levels based on the frequency of the symptoms experienced during the past week. Each item receives 0 point for occurring never or rarely (during less than 1 day), 1 point for occurring some or a little of the time (1–2 days), 2 points for occurring occasionally or a moderate amount of time (3–4 days), and 3 points for occurring most or all of the time (5–7 days). The total scores for all the items of 0–15, 16–24, and 25–60 points were defined as normal, probable depression, and definite depression, respectively. According to Cho & Kim [ 22 ], the reliability of the scale was indicated by Cronbach’s alpha = 0.90 in the normal group ( N = 540), 0.93 in the clinical patient group ( N = 164), and 0.89 in the major depression group ( N = 46). In this study, the reliability of the scale was indicated by Cronbach’s alpha = 0.94.
Collected data were analyzed using SPSS version 25.0 program (IBM Corp., Armonk, NY, USA). The general and infertility-related characteristics of the subjects were analyzed by descriptive statistics using the frequency, percentage, mean, and standard deviation (SD). Item analysis was performed using mean, SD, skewness, and kurtosis. Moreover, item-total correlation analysis was performed, and items with an item-total correlation coefficient inferior to 0.30 were reviewed and deleted [ 23 ]. The construct validity was tested by EFA and the appropriateness of factor analysis was identified using the Kaiser-Meyer-Olkin (KMO) test and Bartlett’s test of sphericity. Furthermore, principal component analysis was used for factor extraction, while varimax rotation was used for factor rotation. For determination of the number of factors, eigenvalue ≥ 1, factor loading ≥ 0.50, commonality ≥ 0.40, and scree plot were considered [ 24 ].
The concurrent validity was tested using the Pearson’s correlation between the developed instrument and ISS [ 12 ], while the known-groups validity was tested using K-CESD-R [ 26 ]. Meanwhile, the differences in the infertility perception scores among the normal, probable depression, and definite depression groups were analyzed using one-way ANOVA, while a post-hoc test was performed using Scheffe’s test. Cronbach’s alpha was calculated to test the reliability.
General characteristics
The subjects’ age averaged 37.03 ± 4.56. The most response to cause of infertility was “unexplained” (57.1%). Most subjects (83.7%) had no children. The current treatments were IVF (57.1%) and IUI (42.9%). The 31.5% considered the treatment as moderately affordable, 15.8% viewed it as hardly affordable. The 11.3% had experience counseling for infertility. The 68.5% answered that their spousal support to infertility treatment was passive (Table 1 ).
Item analysis
Analysis of the items in the scale showed that the mean value was 1.27-3.00 and the SD was 0.48–0.97. The absolute skew and kurtosis values were 0.03–1.93 and 0.07–4.32, respectively, satisfying the criteria of an absolute skew value ≤ 2.0 and absolute kurtosis value ≤ 7.0 [ 25 ]. Accordingly, since multivariate normality was confirmed, all items were used in the analysis. The item-total correlation coefficient was 0.04–0.77, with 12 out of 41 items (items #6, 8, 9, 15, 22, 25, 28, 33, 36, 37, 38, and 39) showing an item-total correlation coefficient ≤ 0.30. Since these items were assessed as offering little contribution to the scale, they were deleted, leaving remaining items in the scale.
Validity testing
Construct validity testing.
A KMO value of 0.91 for 29 items and a Bartlett’s test of sphericity χ² value of 3137.40 ( p < .001) wear measured, confirming that the data were appropriate for factor analysis. After the first EFA, two items that were double-loaded on two factors (items #30 and 31) were deleted. After the second EFA on the remaining 27 items, three items that were double-loaded on two factors (items #12, 29, and 35) and three items with factor loading < 0.50 (items #1, 23, and 34) were deleted. Subsequently, the third EFA was performed using 21 items. The results showed a KMO value of 0.89 and a Bartlett’s test of sphericity χ² value of 1997.07 ( p < .001). Moreover, the commonality was 0.40–0.81, factor loading was 0.51–0.89, and there were four factors with eigenvalue > 1, which had a cumulative explanatory power of 59.3% (Table 2 ).
Four factors extracted according to infertility perception explained 59.3% of the total variance. In social science, an explanation of 40–60% of the variance for multifactor patterns is considered to be sufficient [ 26 ]. Factor-1 was named “perceived feelings,” consisting of six items explaining 34.6% of the total variance. Factor-2 was named “personal stigma,” consisting of eight items explaining 12.0% of the total variance. Factor-3 was named “social stigma,” consisting of three items explaining 6.6% of the total variance. Factor-4 was named “acceptance,” consisting of four items explaining 6.1% of the total variance.
According to the results of the exploratory factor analysis, the 21-item IPS-W with 4 factors underwent confirmatory factor analysis. The model fit was evaluated against predefined cutoff values for each fit index (χ 2 /df ≤ 3, AGFI ≥ 0.90, GFI ≥ 0.90, CFI ≥ 0.90, RMSEA < 0.08) [ 27 ]. The model achieved the following fit indices: χ 2 /df = 2.09, AGFI = 0.81, GFI = 0.85, CFI = 0.90, RMSEA = 0.07 (Fig. 1 ).
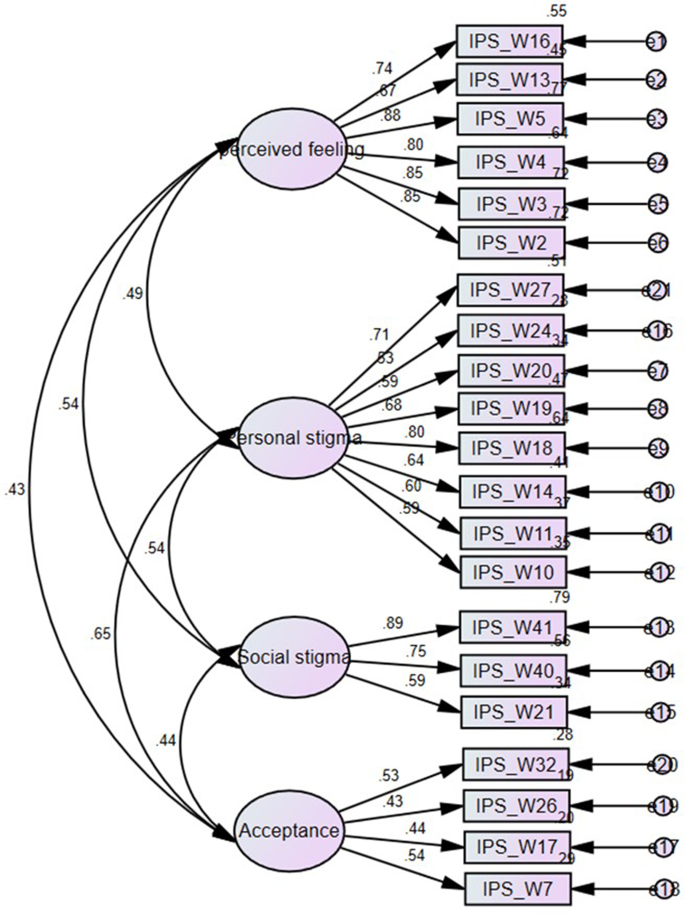
Measurement model of an infertility perception scale for women (IPS-W). χ 2 /df = 2.09, AGFI = 0.81, GFI = 0.85, CFI = 0.90, RMSEA = 0.07 χ 2 /df = Chi-square divided by degrees of freedom; AGFI = Adjustedm Goodness of Fit Index; GFI = Goodness of Fit Index; CFI = Comparative Fit Index; RMSEA = Root Mean Square Error of Approximation
Multi-trait/multi-item matrix analysis was performed. Convergent validity was validated since the correlation between each item and the total score in the corresponding subscale was 0.59–0.89, which satisfied the cut-off value of 0.40. Moreover, discriminant validity was also validated since the value derived by subtracting twice the standard error from the correlation coefficient between the item and the corresponding subscale was larger than the correlation coefficient of other sub-components.
Criterion validity testing
Concurrent validity was first tested based on the correlation of the scale with ISS. The results showed a positive correlation with a correlation coefficient of 0.63 ( p < .001), while also showing positive correlations between sub-factors with correlation coefficients ranging between 0.30 and 0.54. Accordingly, the concurrent validity of the scale was validated (Table 3 ).
Secondly, the known-groups validity was tested by dividing the subjects into groups by the level of depression based on CES-D cut-off points and analyzing the differences in infertility perception among the groups. Since the results showed significant differences in infertility perception according to the level of depression, the known-groups validity was validated (F = 18.84, p < .001; Table 4 ).
Reliability testing
The Cronbach’s alpha value of 21 infertility perception items was 0.90, and for each sub-domain, the Cronbach’s alpha value was 0.91, 0.85, 0.77, and 0.60 for Factor-1, 2, 3, and 4, respectively. According to the rationale by Hair et al. [ 19 ], a reliability ≥ 0.70 is considered acceptable for any new instrument (Table 2 ).
Factor-1 (perceived feelings) represents overall feelings about infertility, having a total explanatory power of 34.6%. Infertility not only causes psychological distress, such as sadness and loss of hope for the future [ 4 ], but it is also defined as an infertility crisis accompanied by physical, economic, and social stress [ 28 ]. The 50% of women considered this process as the most stressful experience in their life [ 29 ], while 84.2% of Korean women receiving infertility treatment experienced depression [ 4 ]. In Factor-1, the overall feeling about infertility was reflected by negative emotions including a sense of loss, anxiety, despair, depression, sadness, and guilt. Therefore, efforts are needed to understand the negative emotions of people who experience infertility and to alleviate these emotions.
Factor-2 refers to “personal stigma.” Infertility can be seen as the fault of the individual, while individuals who experience infertility perceive it as a void and a problem that is difficult to reveal. Personal stigma refers to the extent to which a person believes that negative stereotypes associated with a group they belong to will also be applied to them, while experiencing more personal stigma results in increased self-stigma, which is the feeling that the stigma is applicable to themselves [ 30 ]. Factor-2 reflects previous reports that women who marry but have not given birth are stigmatized as not fulfilling the role of a married woman and denying their own femininity [ 31 ]. Moreover, infertility diagnosis and treatment endlessly give women existential angst and hoping for a child is perceived as the attempt of women to understand their own existence even if they realize that such hope is in vain [ 32 ].
Infertility is understood differently depending on the socio-cultural context. A significant number of women diagnosed with infertility in Korea experience embarrassment and despair from their unexpected difficulties with fertility. Originally, they thought that having a child would be a natural process after getting married, and thus experience confusion about their sense of identity as a woman when this does not happen [ 33 , 34 ]. The perceptions of the Korean society surrounding infertility has been emphasized as an important factor influencing the sense of identity and emotions of individuals with infertility [ 35 ]. In other words, individuals currently undergoing infertility treatment have always perceived infertility negatively, rather than positively, as a member of the society before being diagnosed with infertility themselves. Such perception becomes palpable in their own lives once they are diagnosed with infertility, which adds stigmatizing characteristics to their perception of infertility.
Factor-3 (“social stigma”) includes stigmatizing characteristics about infertility at the societal level, believing that childbirth are an essential social rite of passage. But as they face difficulties with fertility, the views of other people become uncomfortable to women, leading them to withdraw from relationships. This could be interpreted as a factor similar to the “social withdrawal” mentioned by Fu et al. [ 12 ]. This process is well explained by studies reporting that, under the ideology of the Korean society, which regards motherhood as the main definer of a woman’s identity and has favorable views on having children, having friends or relatives that perpetrate a negative stigma around infertility and take a cruel view of women regardless of whether they are directly responsible for the infertility [ 36 ] can cause women with infertility to withdraw from relationships [ 33 ]. In fact, over 50% of Korean women who received infertility treatment experienced prejudice due to infertility and 43% reported serious withdrawal from relationships for this reason [ 34 ].
As previously described, infertility is not well understood and not adequately perceived within many societies [ 37 , 38 ]. Negative social perception about infertility can cause women who experience infertility to internalize social stigma, withdraw from interpersonal relationships, lose self-esteem, and experience decline in quality of life [ 14 ]. All items included in Factors 2 and 3 are close to problems stemming from perceived stigma, meaning one’s own belief that the society views them as a member of a stigmatized group [ 39 ].
Factor-4 reflects “acceptance,” whereby infertility is perceived as an acceptable problem that can be overcome together as a couple, rather than a problem that causes a negative outcome in life. Having no child due to infertility and the surrounding stigma can place a serious burden on the couple’s relationship and the difficulties may destroy the marriage or strengthen their bond [ 40 ]. The perception of infertility is deeply associated with the socio-cultural context due to the longstanding family norms in Korea. Recognizing infertility as a problem that can be overcome and understanding that individuals and the society must work together to resolve the problem.
Meanwhile, Factor-4 had a somewhat low reliability score of 0.60. This could be due to large differences in how positively individuals receiving infertility treatment think about infertility within their socio-cultural and familial context and how significant are their psycho-emotional changes, which may vary according to treatment stage, frequency of treatment, and outcomes during treatment (number of follicles, quality of eggs, number of embryos, quality of embryos, etc.). In the future, it may be necessary to repeat the measurements after unifying the infertility treatment on IUI or IVF or using the same measurement point like the start of treatment rather than during treatment to reduce the factors possibly acting as variables.
Validity and reliability
Concurrent validity test of the developed scale and ISS suggested that a more negative perception of infertility led to a higher perceived stigma about infertility [ 16 , 41 ]. Moreover, known-groups validity for comparing the level of infertility perception between groups showed that the depression groups perceived infertility more negatively than the normal group, consistent with a previous study [ 20 ], which validated the known-groups validity of the scale developed in the present study. The reliability of our scale corresponded to a Cronbach’s alpha of 0.90, indicating that the reliability was at an acceptable level and that all items were easily understood by women receiving infertility treatment.
Up to now, while there have been efforts to measure infertility perception, such efforts have focused mostly on the individual’s knowledge about infertility diagnosis and treatment or the stigmatizing characteristics of infertility within various socio-cultural contexts. In Korea, in particular, infertility has usually been perceived as having stigmatizing characteristics such as insufficiency and difficulty, but it is important to view infertility as a problem that can be overcome by working together, rather than a negative life event that can destroy the sense of identity of women and cause relationship problems among couples. The newly developed infertility perception scale also includes a significant number of the stigmatizing characteristics of infertility. Hence, efforts are needed to solve the issues surrounding inadequate beliefs through the understanding of the stigmatizing characteristics of infertility in order to transition towards a positive perception of infertility. Infertility not only causes individuals to experience various negative emotions but also impacts their life cycle, potentially increasing the risk of conditions such as coronary heart disease (CHD) [ 42 ] and elevating the likelihood of early menopause [ 43 ]. Therefore, a positive perception of infertility by those affected directly influences the individual, playing a crucial role in maintaining and promoting overall health.
Strengths, limitations, and future research
This study convenience sampled women receiving infertility treatment at two Korean hospitals specializing in infertility treatment, limiting the generalization of the findings. In addition, although we selected two institutions with similar treatment processes and patient education, there is a limitation in that we were unable to systematically analyze the similarities and differences. Prior to data collection, the authors informed the study participants that the survey was anonymous and that their responses would be kept confidential. However, there is a limitation that, due to social stigma, participants might have underreported or misreported their true opinions. Moreover, Cultural differences across countries can significantly influence perceptions of infertility, where what may be considered stigma or acceptable responses in one culture may not be the same in another. Therefore, it is essential to assess the validity and reliability across diverse populations, including different ethnic groups, and adjust the questionnaire accordingly to ensure cultural relevance and applicability. In future research, it is recommended that the validity and reliability of the scale be tested only among women who are at the IVF stage, as IVF is in the final stage of assisted reproductive therapy. Additionally, follow-up studies should aim to identify the level of infertility perception and to develop and test the impact of nursing interventions.
The infertility perception scale developed in the present study could predict the psycho-emotional state of women receiving infertility treatment by examining their infertility perception. These findings could be used for the development of nursing interventions that could help women experiencing infertility to approach positively the problem of infertility.
Conclusions
We developed and tested an infertility perception scale consisting in a 4-point Likert scale with 21 items under four factors. The scale has a score range of 21–84 points, with higher scores indicating more negative perceptions of infertility. The scale can be used to measure infertility perception among women who are experiencing infertility and their spouse, family, and friends. The findings can help nurses provide support that is appropriate for individual circumstances by examining how women experiencing infertility perceive infertility.
Data availability
Datasets used/or analyzed during the current study are available from the corresponding author on reasonable request.
Abbreviations
Infertility Perception Scale for Women
Intrauterine insemination
in-Vitro Fertilization
Exploratory Factor Analysis
Confirmatory Factor Analysis
Female Infertility Stigma Instrument
Infertility Stigma Scale
Strengthening the Reporting of Observational Studies in Epidemiology
Content Validity Index
Item-Content Validity Index
Korean version of the Center for Epidemiologic Studies Depression Scale-Revised
Kaiser-Meyer-Olkin
Root-mean-square error of approximation
Adjusted Goodness-of-Fit Index
Goodness-of-Fit Index
Comparative Fit Index
Lee YH, Park JS. Factors affecting the infertility-related quality of life among the infertility women. J Korean Soc Maternal Child Health. 2019;23(3):191–201. https://doi.org/10.21896/jksmch.2019.23.3.191 .
Article Google Scholar
Podolska M, Bidzan M. Infertility as a psychological problem. Ginekologia Polska. 2011;82(1):44–9.
PubMed Google Scholar
Covington SN, Burns LH, editors. Infertility counseling: a comprehensive handbook for clinicians. Cambridge University Press; 2006.
Hwang NM. Factors related to the depression of infertile women. Health Social Welf Rev. 2013;33(3):161–87.
Taebi M, Kariman N, Montazeri A, et al. Development and psychometric evaluation of the female infertility stigma instrument (ISI-F): protocol for a mixed method study. Reproductive Health. 2020;17:70. https://doi.org/10.1186/s12978-020-0904-5 .
Article PubMed PubMed Central Google Scholar
Amiri M, Khosravi A, Chaman R, Sadeghi Z, Raei M, Jahanitiji MA, Mehrabian F. Social consequences of infertility on families in Iran. Global J Health Sci. 2016;8(5):89–94. https://doi.org/10.5539/gjhs.v8n5p89 .
Ergin RN, Polat A, Kars B, Öztekin D, Sofuoğlu K, Çalışkan E. Social stigma and familial attitudes related to infertility. Turkish J Obstet Gynecol. 2018;15(1):46–9. https://doi.org/10.4274/tjod.04307 .
Harzif AK, Santawi VPA, Wijaya S. Discrepancy in perception of infertility and attitude towards treatment options: Indonesian urban and rural area. Reproductive Health. 2019;16:126. https://doi.org/10.1186/s12978-019-0792-8 .
Meissner C, Schippert C, von Versen-Höynck F. Awareness, knowledge, and perceptions of infertility, fertility assessment, and assisted reproductive technologies in the era of oocyte freezing among female and male university students. J Assist Reprod Genet. 2016;33:719–29. https://doi.org/10.1007/s10815-016-0717-1 .
Article CAS PubMed PubMed Central Google Scholar
Jansen NA, Saint Onge JM. An internet forum analysis of stigma power perceptions among women seeking fertility treatment in the United States. Soc Sci Med. 2015;147:184–9. https://doi.org/10.1016/j.socscimed.2015.11.002 .
Article PubMed Google Scholar
Naab F, Brown R, Heidrich S. Psychosocial health of infertile Ghanaian women and their infertility beliefs. J Nurs Scholarsh. 2013;45(2):132–40. https://doi.org/10.1111/jnu.12013 .
Fu B, Qin N, Cheng L, Tang G, Cao Y, Yan C, Huang X, Yan P, Zhu S, Lei J. Development and validation of an infertility stigma scale for Chinese women. J Psychosom Res. 2015;79(1):69–75. https://doi.org/10.1016/j.jpsychores.2014.11.014 .
Introduction to perception. Retrieved from https://courses.lumenlearning.com/boundless-psychology/chapter/introduction-to-perception/
Gong JS. The effect of ex-prisoner’s perception of stigma on trust in international relationship. Korean Secur J. 2018;57:57–84.
Google Scholar
DeVellis RF. Scale development: theory and applications. 4th ed. Sage; 2016. pp. 39–199.
Seifer DB, Petok WD, Agrawal A, Glenn TL, Bayer AH, Witt BR, Burgin BD, Lieman HJ. Psychological experience and coping strategies of patients in the Northeast US delaying care for infertility during the COVID-19 pandemic. Reproductive Biology Endocrinol. 2021;19(1):28. https://doi.org/10.1186/s12958-021-00721-4 .
Article CAS Google Scholar
Krippendorff K. Content analysis: an introduction to its methodology. 2nd ed. Sage; 2004. pp. 1–413.
Lynn MR. Determination and quantification of content validity. Nurs Res. 1986;35(6):382–5.
Article CAS PubMed Google Scholar
Hair JF, Black WC, Babin BJ, Anderson RE. Multivariate data analysis: a global perspective. 7th ed. Pearson Education; 2010. pp. 111–3.
Davis M, Ventura JL, Wieners M, Covington SN, Vanderhoof VH, Ryan ME, et al. The psychosocial transition associated with spontaneous 46, XX primary ovarian insufficiency: illness uncertainty, stigma, goal flexibility, and purpose in life as factors in emotional health. Fertil Steril. 2010;93(7):2321–9. https://doi.org/10.1016/j.fertnstert.2008.12.122 .
Lee S, Oh ST, Ryu SY, Jun JY, Lee K, Lee E, et al. Validation of the Korean version of Center for epidemiologic studies Depression Scale-revised (K-CESD-R). Korean J Psychosom Med. 2016;24(1):83–93.
Cho MJ, Kim KH. Diagnostic validity of the CES-D (Korean version) in the assessment of DSM-III-R major depression. J Korean Neuropsychiatric Association. 1993;32(3):381–99.
Lee CY. (2016). Advanced nursing statistics (pp. 231–264). Soomunsa.
Noh GS. (2019). The proper methods of statistical analysis for dissertation: SPSS & AMOS 21 (pp. 141–193). Hanbit Academy.
Curran PJ, West SG, Finch JF. The robustness of test statistics to non-normality and specification error in confirmatory factor analysis. Psychol Methods. 1996;1(1):16–29. https://doi.org/10.1037/1082-989X.1.1.16 .
Sternke EA, Abrahamson K. Perceptions of women with infertility on stigma and disability. Sex Disabil. 2015;33:3–17. https://doi.org/10.1007/s11195-014-9348-6 .
Moon SB. Understanding and application of structural equation modeling. Seoul: Hakjisa; 2009.
Faramarzi M, Pasha H, Esmailzadeh S, Kheirkhah F, Heidary S, Afshar Z. The effect of cognitive behavioral therapy and pharmacotherapy on infertility stress: a randomized controlled trial. Int J Fertility Steril. 2013;7(3):199–206.
Herrmann D, Scherg H, Verres R, Von Hagens C, Strowitzki T, Wischmann T. Resilience in infertile couples acts as a protective factor against infertility-specific distress and impaired quality of life. J Assist Reprod Genet. 2011;28(11):1111–7. https://doi.org/10.1007/s10815-011-9637-2 .
Muñoz M, Finnegan K, Zeladita J, Caldas A, Sanchez E, Callacna M, et al. Community-based DOT-HAART accompaniment in an urban resource-poor setting. AIDS Behav. 2010;14(3):721–30. https://doi.org/10.1007/s10461-009-9559-5 .
Jeong KI. A study on hermeneutic grounded theory of women who experience infertility suffering and relief from pain. Korean J Social Welf Stud. 2014;45(4):5–35. https://doi.org/10.16999/KASWS.2014.45.4.5 .
Boz I, Okumus H. The everything about the existence experiences of Turkish women with infertility: solicited diaries in qualitative research. J Nurs Res. 2017;25(4):268–75. https://doi.org/10.1097/jnr.0000000000000166 .
Yang SN. The experience of infertility treatments among working women without children. Korean J Social Welf Educ. 2019;48:93–120. https://doi.org/10.1111/j.1467-9566.2009.01213.x .
Jeong YM, Kang SK. A phenomenological study on infertile experiences of women: Waiting for Meeting. Stud Life Cult. 2017;44:271–319.
Ryu SJ. A phenomenological study on infertility as the missing piece in socialization process: focusing on university students’ perception of infertility. Family Cult. 2021;33(2):38–65. https://doi.org/10.21478/family.33.2.202106.002 .
Ryu SJ. Infertility, the social context of deferred motherhood: a qualitative inquiry based on an online infertility community. J Asian Women. 2019;58(2):5–38.
Bunting L, Boivin J. Knowledge about infertility risk factors, fertility myths and illusory benefits of healthy habits in young people. Hum Reprod. 2008;23(8):1858–64. https://doi.org/10.1093/humrep/den168 .
Fido A. Emotional distress in infertile women in Kuwait. Int J Fertil Women’s Med. 2004;49(1):24–8.
LeBel TP. Perceptions of and responses to stigma. Sociol Compass. 2008;2(2):409–32. https://doi.org/10.1111/j.1751-9020.2007.00081.x .
Valsangkar S, Bodhare T, Bele S, Sai S. An evaluation of the effect of infertility on marital, sexual satisfaction indices and health-related quality of life in women. J Hum Reproductive Sci. 2011;4(2):80–5. https://doi.org/10.4103/0974-1208.86088 .
Sobral MP, Costa ME, Schmidt L, Martins MV. COMPI fertility problem stress scales is a brief, valid and reliable tool for assessing stress in patients seeking treatment. Hum Reprod. 2017;32(2):375–82. https://doi.org/10.1093/humrep/dew315 .
Farland LV, Wang YX, Gaskins AJ, Rich-Edwards JW, Wang S, Magnus MC, et al. Infertility and risk of cardiovascular disease: a prospective cohort study. J Am Heart Association. 2023;12(5):e027755. https://doi.org/10.1161/JAHA.122.027755 .
Liang C, Chung HF, Dobson AJ, Cade JE, Greenwood DC, Hayashi K, et al. Is there a link between infertility, miscarriage, stillbirth, and premature or early menopause? Results from pooled analyses of 9 cohort studies. Am J Obstet Gynecol. 2023;229(1):47e1. 47.e9.
Download references
Acknowledgements
This work was supported by the National Research Foundation of Korea (NRF) grant funded by the Korea government (MSIT) (No. 2019R1F1A106291912).
Author information
Authors and affiliations.
Department of Nursing, College of Nursing, Dankook University, Cheonan, South Korea
Department of Nursing, College of Nursing, Catholic University of Pusan, Busan, South Korea
Minkyung Ban
You can also search for this author in PubMed Google Scholar
Contributions
Conceptualization or/and Methodology: Kim Miok. Data curation or/and Analysis: Ban Minkyung. Funding acquisition: Kim MiokInvestigation: Ban Minkyung. Project administration or/and Supervision: Kim Miok. Resources or/and Software: Kim Miok, Ban Minkyung. Validation: Kim Miok. Visualization: Kim Miok, Ban Minkyung. Writing: original draft or/and review & editing: Kim Miok, Ban Minkyung.
Corresponding author
Correspondence to Minkyung Ban .
Ethics declarations
Ethics approval.
This study obtained an approval from the Institutional Review Board (IRB) of Dankook University (IRB approval no.: DKU 2021-01-022). Consent was obtained from the subjects after informing them about the purpose and procedures of the study and the protection of personal information. Only those who voluntarily consented to participate in the study were included. The subjects were given a small token of appreciation for their participation.
Consent to participate
Informed consent was obtained from all individual participants included in the study.
Consent for publication
Not applicable.
Competing interests
The authors declare no competing interests.
Additional information
Publisher’s note.
Springer Nature remains neutral with regard to jurisdictional claims in published maps and institutional affiliations.
Electronic supplementary material
Below is the link to the electronic supplementary material.
Supplementary Material 1
Rights and permissions.
Open Access This article is licensed under a Creative Commons Attribution-NonCommercial-NoDerivatives 4.0 International License, which permits any non-commercial use, sharing, distribution and reproduction in any medium or format, as long as you give appropriate credit to the original author(s) and the source, provide a link to the Creative Commons licence, and indicate if you modified the licensed material. You do not have permission under this licence to share adapted material derived from this article or parts of it. The images or other third party material in this article are included in the article’s Creative Commons licence, unless indicated otherwise in a credit line to the material. If material is not included in the article’s Creative Commons licence and your intended use is not permitted by statutory regulation or exceeds the permitted use, you will need to obtain permission directly from the copyright holder. To view a copy of this licence, visit http://creativecommons.org/licenses/by-nc-nd/4.0/ .
Reprints and permissions
About this article
Cite this article.
Kim, M., Ban, M. Development of an infertility perception scale for women (IPS-W). BMC Women's Health 24 , 513 (2024). https://doi.org/10.1186/s12905-024-03336-0
Download citation
Received : 21 September 2023
Accepted : 27 August 2024
Published : 14 September 2024
DOI : https://doi.org/10.1186/s12905-024-03336-0
Share this article
Anyone you share the following link with will be able to read this content:
Sorry, a shareable link is not currently available for this article.
Provided by the Springer Nature SharedIt content-sharing initiative
- Infertility, female
- Social stigma
- Factor analysis, statistical
BMC Women's Health
ISSN: 1472-6874
- General enquiries: [email protected]
Analysis of variance in uncertain environments
- Original Article
- Open access
- Published: 10 June 2017
- Volume 3 , pages 189–196, ( 2017 )
Cite this article
You have full access to this open access article
- A. Parchami 1 ,
- M. Nourbakhsh 2 &
- M. Mashinchi 1
2541 Accesses
28 Citations
Explore all metrics
Testing one-way analysis of variance (ANOVA) is used for experimental data analysis in which there is a continuous response variable and a single independent classification variable. In this paper, we extend one-way ANOVA to a case where observed data are imprecise numbers rather than real numbers. Several fast computable formulas are calculated for symmetric triangular and normal fuzzy data. Similar to the classical testing ANOVA, the total observed variation in the response variable is explained as the sum of observed variation due to the effects of the classification variable and the observed variation due to random error. A real case is given to clarify the proposed method.
Similar content being viewed by others
Two-Way ANOVA for Fuzzy Observations and an Implication
The Fuzzy Approach to Assessment of ANOVA Results
Testing Independence with Fuzzy Data
Avoid common mistakes on your manuscript.
Introduction and literature review
Analysis of variance (ANOVA) is concerned with analyzing variation in the means of several independent populations which developed by Ronald Fisher. The most important point in traditional ANOVA is a test about the significance of the difference among population means. This test permits the user to conclude whether the differences among the means of several populations are too deviated to be attributed to the sampling error or not [ 7 ]. The basic formulas of classical ANOVA model can be referred to any statistical book [ 1 , 10 , 19 ], but the classical ANOVA has been briefly reviewed in “Classical ANOVA” of this manuscript from [ 21 ].
In many applied sciences like geology, astrology, economics, medical sciences, engineering and environmental sciences, there exist actual cases where imprecise quantities can be assigned to experimental results. In such practical cases, non-precise numbers are suitable models to formalize and handle such imprecise quantities, which is the justification for require to fuzzy sets in ANOVA. The previous related works on fuzzy ANOVA are listed in the next subsection.
Fuzzy ANOVA
During the past three decades, several scientific works have been published in different fields of ANOVA using fuzzy set theory. These fields are as follow: one-way ANOVA testing procedure based on normal fuzzy random variables (FRVs) [ 18 ], asymptotic bootstrap ANOVA test for FRVs by one-sample [ 17 ], providing ANOVA method for the functional data in Hilbert space [ 2 ], asymptotic bootstrap ANOVA test for simple FRVs on the basis of multi-sample [ 7 , 8 ], an optimization method for one-way ANOVA problem by the cuts of FRVs when observations are imprecise [ 29 ], investigating on one-way fuzzy ANOVA and comparing it with a regression model [ 4 ], processing method for ANOVA for the vague data using the moment correction [ 15 ], providing one-way ANOVA for triangular fuzzy numbers on the basis of the extension principle [ 21 ], developing one-way ANOVA for the closed interval observations [ 9 ], providing R package ‘SAFD’ for ANOVA test based on defuzzification of the FRVs [ 16 ], extending the classical ANOVA test statistics to investigate on fuzzy means of imprecise observation by bootstrap approach [ 25 ], providing ANOVA test based on least squares approach for FRVs [ 12 ], and proposing a decision-rule approach to test fuzzy hypotheses in ANOVA considering triangular imprecise observation and vague hypotheses [ 13 ].
Unlike these studies, another simple method for one-way ANOVA, as a meaningful extension on the classical ANOVA, is presented in this paper where the observations are symmetric triangular or normal fuzzy numbers. The proposed ANOVA method is on the basis of a distance between symmetric fuzzy numbers which is easy to be used by professional clients.
The organization of this paper is as follows. After introducing symmetric triangular and normal fuzzy numbers, some arithmetic operations for imprecise numbers are reviewed in “Preliminaries”. In “Classical ANOVA”, classical ANOVA is briefly explained from [ 21 ]. In “Analysis of variance based on fuzzy observations”, a new method for ANOVA test for fuzzy observations is provided and discussed. In “Fast computation formulas”, several fast computable formulas are presented for symmetric triangular and normal fuzzy data. A case study on several brands of soap is presented in “A case study on the process of soap production” which is revealing the ideas of this paper. Conclusion part is given in the final section.
Preliminaries
In this section, we review briefly some definitions which will be referred through this paper. Let X be a universal set and \(F(X)=\left\{ {A|A:X\rightarrow [0,1]} \right\} \) . Any \(A\in F(X)\) is called a fuzzy set on X . In particular, let F ( R ) be the set of fuzzy numbers on R (the set of real numbers). The \(\alpha \) -cut of A is the crisp set given by \(A_\alpha =\{x\in X| A(x)\ge \alpha \}\) , for any \(\alpha \in (0,1]\) .
Definition 2.1
An especial case of fuzzy numbers called symmetric triangular fuzzy number (STFN) is defined by
and it is denoted with \(T(a,s_a )\) .
Another especial case of fuzzy numbers called normal fuzzy number (NFN) is defined by
and it is shown with \(N(a,s_a )\) . Here, \(a\in R\) and \(s_a \in R^{+}\) are named the core/mean and the spread of STFNs and NFNs, respectively. Moreover, \(F_T (R)\) and \(F_N (R)\) denote the sets of all STFNs and NFNs on R [ 23 ].
The following distance will be used through Sect. 4 to solve the problem of testing ANOVA based on fuzzy observations.
Definition 2.2
[ 23 , 29 ] For any \(\tilde{A}, \tilde{B}\in F(R)\) ,
is called the distance of \(\tilde{A}\) and \(\tilde{B}\) where
measured the distance between \(\tilde{A}_\alpha =\left[ {a_1 (\alpha ),a_2 (\alpha )} \right] \) and \(\tilde{B}_\alpha =\left[ {b_1 (\alpha ), b_2 (\alpha )} \right] \) , and g is a non-decreasing function on [0,1] with properties \(g(0)=0\) and \(\int _{ 0}^{ 1} {g(\alpha ) d\alpha } =\frac{1}{2}\) (for instance \(g(\alpha )=\frac{m+1}{2}\alpha ^{m}\) where \(m=1,2,3,\ldots )\) .
Definition 2.2 is an extended version of the absolute deviation between two real numbers. Since, for two real numbers a and b by indicator functions \(I_{\{a\}} \) and \(I_{\{b\}} \) we have
More details of Definition 2.2 is presented in [ 23 ].
Theorem 2.1
The distance between two STFNs \(\tilde{A}=T(a,s_a )\in F_T (R)\) and \(\tilde{B}=T(b,s_b ) \quad \in F_T (R)\) is
where the weighted function is \(g(\alpha )=\frac{m+1}{2}\alpha ^{m}\) , for \(m=1,2,3,\ldots \) .
For any \(\alpha \in (0,1]\) ,
\(\tilde{A}_\alpha =\left[ {a-s_a (1-\alpha ) , a+s_a (1-\alpha )} \right] \) and \(\tilde{B}_\alpha =[ b-s_b (1-\alpha ) , b+s_b (1-\alpha ) ]\) .
Hence, according to Definition 2.2 ,
and so, using \(g(\alpha )=\frac{m+1}{2}\alpha ^{m}\) in Definition 2.2 , it can be calculated that
for \(m=1,2,3,\ldots .\) \(\square \)
Theorem 2.2
The distance between two NFNs \(\tilde{A}=N(a,s_a )\in F_N (R)\) and \(\tilde{B}=N(b,s_b ) \in F_N (R)\) is
One can obtain \(\tilde{A}_\alpha =[ a-s_a \sqrt{-\ln \alpha },a+s_a \sqrt{-\ln \alpha } ]\) and \(\tilde{B}_\alpha =\left[ {b-s_b \sqrt{-\ln \alpha },} \right. \quad \left. {b+s_b \sqrt{-\ln \alpha }} \right] \) , for any \(\alpha \in (0,1]\) . Therefore, considering Definition 2.2 ,
Hence, using \(g(\alpha )=\frac{m+1}{2}\alpha ^{m}\) , \(m=1,2,3,\ldots \) in Definition 2.2 , it can be concluded that
\( =(a-b)^{2}+\frac{1}{(m+1)}(s_a -s_b )^{2}.\) \(\square \)
Note that in some cases the integral used in Definition 2.2 leads to an improper integral as appeared in the proof of Theorem 2.2 .
Classical ANOVA
Although the classical ANOVA model can be referred to many statistical books as well as well-known references, e.g., see [ 1 , 10 , 19 ], but for a short introduction and review the methodology of ANOVA we propose “Classical ANOVA” from [ 21 ] since the considered notations are same here. So, we only present some classical formulas in Table 1 known as ANOVA table. To test whether the factor level means \(\mu _i \) ’s are the same in quantity or not, the classical ANOVA has been contemplate to accept/reject hypothesis “ H \(_{0 }\) : \(\mu _1 =\mu _2 ={\cdots }=\mu _r \) ”, against “ \(H_{1 }\) : not all \(\mu _i \) ’s are equal”, based on observed random samples [ 11 ].
Analysis of variance based on fuzzy observations
Quantities of continuous variables are not precise numbers and a more appropriate way to describing them is using imprecise/fuzzy numbers [ 24 , 26 , 27 ]. To explain such situation, one can see several applied examples from references [ 11 , 14 , 28 ]. It must be noted that in this section, only the observed amounts of precise random variables can be regarded as imprecise numbers while the model for observed values is still precise as follows
Note that a similar idea is used in [ 6 , 11 , 27 ] and in a such situation, observations and recorded data can be looked as STFNs \(\tilde{y}_{ij} =T(y_{ij} ,s_{y_{ij} } )\) or NFNs \(\tilde{y}_{ij} =N(y_{ij} ,s_{y_{ij} } )\) , where \(\tilde{y}_{ij} \) is interpreted as “approximately \(y_{ij} \) ”, for \(i=1,\ldots ,r\) and \(j=1,\ldots ,n_i .\)
Considering above discussion, in this section and hereafter, it is assumed that we are concerned with a classical analysis of variance, where the whole components of the problem such as random variables, hypothesis and parameters of populations are crisp as are introduced in “Classical ANOVA”. Therefore, the considered ANOVA model is \(Y_{ij} =\mu _i +\varepsilon _{ij} \) , where \(\varepsilon _{ij} \sim N(0,\sigma ^{2})\) and so \(Y_{ij}^{\prime }\hbox {s}\) are ordinary random variables. But, just one point that will departed from classical ANOVA assumptions in the model ( 7 ) is that the sampled observations are fuzzy numbers rather than being real numbers and nothing else is altered in the prior testing ANOVA.
Regarding the introduced distance between two fuzzy numbers in Definition 2.2 , the observed values of the statistics SST, SSTR, SSE, MSTR, MSE and F can be calculated by the following extended formulas based on fuzzy observations:
In testing ANOVA based on fuzzy numbers, the observed amount of the test statistic is precisely computable by
in which \(\widetilde{\text {mstr}}=\frac{\widetilde{\text {sstr}}}{r-1}\) and \(\widetilde{\text {mse}}=\frac{\widetilde{\text {sse}}}{n_t -r}\) are respectively treatment mean square and the mean of squares.
The major problem in testing ANOVA is calculating the sum of squares of fuzzy observations. In this paper, we have used a logical defuzzification in Eqs. ( 8 – 10 ), since:
Although the square of a LR fuzzy number can be computed by extension principle, the result is not necessarily in the class of LR fuzzy numbers too [ 23 ]. Hence, one is faced with calculating the membership function of the addition of fuzzy numbers, with not necessarily the same shape functions, based on the Zadeh’s extension principle.
Calculation of \(\widetilde{\text {sst}}\) , \(\widetilde{\text {sstr}}\) and \(\widetilde{\text {sse}}\) by Zadeh’s extension principle, will lead us to a very vague observed statistic at the end of computations, since usually the experimenter is faced to \(\sum _{i=1}^r {n_i } \) fuzzy numbers in any testing ANOVA. In other words, as \(\sum _{i=1}^r {n_i } \) increases, then the support of \(\widetilde{\text {sst}}\) , \(\widetilde{\text {sstr}}\) and \(\widetilde{\text {sse}}\) become larger and this means the vagueness of the observed fisher statistic. For instance, see the observed fisher statistic in Sect. 8.2 of [ 21 ] which is obtained via Zadeh’s extension principle approach.
Following (ii), the truth level of decision making can be low in many applied situations. See definition “the fuzziness of the decision” from [ 11 ].
The decision rule Let \(\tilde{f}\) be the observed value of the test statistic and \(F_{1-\alpha ;r-1,n_t -r} \) be the \(\alpha \) th quantile of the fisher distribution with \(r-1\) and \(n_t -r\) degrees of freedom. At the given significance level \(\alpha \) , \(H_{0}\) is accepted if \(\tilde{f}\le F_{1-\alpha ;r-1,n_t -r} \) ; otherwise \(H_{1}\) is accepted.
Considering Remark 2.1 , if the observed data for test are real numbers \(y_{ij} \) which can be formulated by indicator functions \(I_{\{y_{ij} \}} \) for \(i = 1,\ldots ,r\) and \(j = 1,\ldots ,n_i \) , then all the introduced extended statistics in Eqs. ( 8 )–( 11 ) coincide to statistics of classical ANOVA.
The rejection region in testing ANOVA is \(F>F_{1-\alpha ; r-1,n_t -r} \) and so under hypothesis \(H_0 \) the error probability is
where \(F_{1-\alpha ;r-1,n_t -r} \) is \(\alpha \) th quantile of F distribution. Therefore, in testing ANOVA based on fuzzy numbers, the p -value can be calculated by
\(p{\text {-value}}=P\left( {F>\tilde{f}} \right) \)
in which \(\tilde{f}\) is introduced by ( 11 ) as the observed amount of test statistic on the basis of fuzzy observations.
Fast computation formulas
Anova for symmetric triangular fuzzy observations.
In this subsection, we assume all observations are STFNs. Then the observed values of statistics are obtained by the following theorems in testing ANOVA on the basis of STFNs.
Theorem 5.1
Based on symmetric triangular fuzzy observations \(\tilde{y}_{ij} =T(y_{ij} ,s_{y_{ij} } ) \quad \in F_T (R)\) , \(i=1,2,\ldots ,r, j=1,2,\ldots ,n_i \) , the observed values of \(\widetilde{\text {sst}}\) , \(\widetilde{sstr}\) and \(\widetilde{sse}\) in testing ANOVA are the following real numbers:
where \({\text {sst}}_y =\sum \nolimits _{i=1}^r {\sum \nolimits _{j=1}^{n_i } {(y_{ij} -\overline{y_{..} } )^{2}} } \) , \({\text {sstr}}_y =\sum \nolimits _{i=1}^r {n_i (\overline{y_{i.} } -\overline{y_{..} } )^{2}} \) and \({\text {sse}}_y =\sum \nolimits _{i=1}^r {\sum \nolimits _{j=1}^{n_i } {(y_{ij} -\overline{y_{i.} } )^{2}} } \) are the observed sum of squares for the mean values of \(\tilde{y}_{ij} {\prime }\hbox {s}\) , and also \({\text {sst}}_{s_y } =\sum _{i=1}^r {\sum \nolimits _{j=1}^{n_i } {(s_{y_{ij} } -\overline{s_{y_{..} } } )^{2}} } \) , \({\text {sstr}}_{s_y } =\sum \nolimits _{i=1}^r {n_i (\overline{s_{y_{i.} } } -\overline{s_{y_{..} } } )^{2}} \) and \({\text {sse}}_{s_y } =\sum \nolimits _{i=1}^r {\sum \nolimits _{j=1}^{n_i } {(s_{y_{ij} } -\overline{s_{y_{i.} } } )^{2}} } \) are the observed sum of squares for spreads of \(\tilde{y}_{ij} {\prime }\hbox {s}\) .
From Eq. ( 8 ), Definition 2.2 and Theorem 2.1 , we have
where \(\overline{y_{..} } =\frac{1}{n_t }\sum \nolimits _{i=1}^r {\sum \nolimits _{j=1}^{n_i } {y_{ij} } } \) , \(\overline{s_{y_{..} } } =\frac{1}{n_t }\sum \nolimits _{i=1}^r {\sum \nolimits _{j=1}^{n_i } {s_{y_{ij} } } } \) and \(g(\alpha )=\frac{m+1}{2}\alpha ^{m}.\) One can similarly prove Eqs. ( 13 ) and ( 14 ), by considering \(\overline{y_{i.} } =\frac{1}{n_i }\sum \nolimits _{j=1}^{n_i } {y_{ij} } \) and \(\overline{s_{y_{i.} } } =\frac{1}{n_i }\sum _{j=1}^{n_i } {s_{y_{ij} } } \) for \(i=1,\ldots ,r\) . \(\square \)
Theorem 5.2
Under the same assumption of Theorem 5.1 , the observed amount of ANOVA test statistic is
in which \({\text {mstr}}_y =\frac{{\text {sstr}}_y }{r-1}\) and \({\text {mse}}_y =\frac{{\text {sse}}_y }{n_t -r}\) are the observed mean squares of the mean values of \(\tilde{y}_{ij} \) ’s, and also, \({\text {mstr}}_{s_y } =\frac{{\text {sstr}}_{s_y } }{r-1}\) and \({\text {mse}}_{s_y } =\frac{{\text {sse}}_{s_y } }{n_t -r}\) are the observed mean squares of spreads of \(\tilde{y}_{ij} \) ’s.
By Eqs. ( 13 and 14 ), mean squares can be obtained as
Therefore, Eq. ( 15 ) can be easily followed from \(\widetilde{f}=\frac{\widetilde{{\text {mstr}}}}{\widetilde{{\text {mse}}}}\) . \(\square \)
ANOVA for normal fuzzy observations
In this subsection, we assume all observations are NFNs in testing ANOVA. Then the observed values of statistics in ANOVA model can be easily obtained by the following theorems.
Theorem 5.3
Based on normal fuzzy observations \(\tilde{y}_{ij} =N(y_{ij} ,s_{y_{ij} } )\in F_N (R)\) , \(i = 1,2,\ldots ,r, \quad j = 1,2,\ldots ,n_i \) , the observed values of \(\widetilde{sst}\) , \(\widetilde{sstr}\) and \(\widetilde{\text {sse}}\) in testing ANOVA are the following real numbers:
where \({\text {sst}}_y \) , \({\text {sstr}}_y \) and \({\text {sse}}_y \) are the observed sum of squares for the mean values of \(\tilde{y}_{ij} {\prime }\hbox {s}\) , and also \({\text {sst}}_{s_y } \) , \({\text {sstr}}_{s_y } \) and \({\text {sse}}_{s_y } \) are the observed sum of squares for spreads of \(\tilde{y}_{ij} {\prime }\hbox {s}\) .
From Eq. ( 8 ) and Theorem 2.2 , one can conclude that
by considering \(g(\alpha )=\frac{m+1}{2}\alpha ^{m}\) , \(m=1,2,3,\ldots \) in Definition 2.2 . Similarly, one can prove Eqs. ( 20 ) and ( 21 ). \(\square \)
As a result of Eq. ( 19 ) for ANOVA based on normal fuzzy observations, not only increasing \(sst_y \) can be caused the increase of \(\widetilde{{\text {sst}}}\) , but also increasing \({\text {sst}}_{s_y } \) can be caused the increase of \(\widetilde{{\text {sst}}}\) . Similar results can be presented on the basis of formulas ( 19 ) and ( 20 ) in ANOVA based on normal fuzzy numbers, and also on the basis of formulas ( 12 )–( 14 ) for ANOVA based on symmetric triangular fuzzy numbers.
Theorem 5.4
Under the same assumption of Theorem 5.3 , the observed value of ANOVA test statistic is
in which \({\text {mstr}}_y \) and \({\text {mse}}_y \) are the observed mean squares of the mean values of \(\tilde{y}_{ij} {\prime }\hbox {s}\) , and also, \(mstr_{s_y } \) and \({\text {mse}}_{s_y } \) are the observed mean squares of spreads of \(\tilde{y}_{ij} \) ’s.
By Eqs. ( 11 ), ( 19 ) and ( 20 ), mean squares can be obtained as
Therefore, Eq. ( 22 ) can be easily followed from \(\widetilde{f}=\frac{\widetilde{\text {mstr}}}{\widetilde{\text {mse}}}\) . \(\square \)
In ANOVA test based on STFNs and NFNs, all the introduced extended statistics in Eqs. ( 12 )–( 24 ) reduce to presented statistics in Sect. 3 of [ 21 ] for classical ANOVA, where spreads of \(\tilde{y}_{ij} {\prime }\hbox {s}\) are a fixed number for \(i = 1,\ldots ,r\) and \(j = 1,\ldots ,n_i \) .
Testing ANOVA discussed here is different from Wu’s approach [ 28 ]. His ANOVA method constructed on the basis of the cuts of FRVs, optimistic and pessimistic degrees by optimization. Also, the presented work in [ 21 ] is based on extension principle which can lead user to a very fuzzy decision by a very vague fisher statistic in practice. The major advantages of the presented method in this paper are efficiency for large sampling data and its simplicity in use by clients in the real situations.
In the next section, the presented ANOVA method will be exemplified for fuzzy observations by a relevant study.
A case study on the process of soap production
The aim of this study is to investigate on the amount of solubility for three different kinds of soaps in tepid water. The treatment factor, soap, has been selected to have three levels: regular, deodorant, and moisturizing brands, from the same manufactory. This example is extracted from an experiment done by Suyapa Silvia [ 3 ] where the data are fuzzified. It must be stressed that the condition of the presented ANOVA test/problem in this paper is exactly same as the presented ANOVA test in [ 21 ], but their solutions are different. Therefore, we do not present the story of experiment process to shorten the length of paper and we refer the readers to Sect. 8.2 of [ 21 ] for more details about gathering data. Now, we are going to respond to this question: Is there a significant difference in the weight loss (between three kinds of soaps) because of dissolution in water when allowed to soak for the same time?
There are some unavoidable elements in the experiment reported by Suyapa Silvia [ 3 ], such as: (1) inability of the experimenter to cut exactly the same cubes with same weights, (2) limitation of digital laboratory scales of precision of 10 mg, and etc. Hence, a better plan to record the observations is to use STFNs where some of them are definitely non-positive. Therefore, in this real case, we rewrite the data by STFNs as in Table 2 , to protect the unavoidable elements described in above. The spread of each observed symmetric triangular fuzzy number is considered as a function of its mean in Table 2 . In other words fuzzy observations have been considered by STFNs \(\tilde{y}_{ij} =T(y_{ij} , s_{y_{ij} } )\) , such that \(s_{y_{ij} } ={\left| {y_{ij} } \right| }/{10}\) , for \(i = 1,2,\ldots ,r\) and \(j = 1,2,\ldots ,n_i \) . Note that in this study some observations are not reported by positive STFNs.
For the presented triangular fuzzy observations in Table 2 , we wish to test whether the weight loss of three soaps are the same or not. In other words, we are going to test “ \(H_0 :\mu _1 =\mu _2 =\mu _3 \) ”, versus “ \(H_1 :\) not all \(_{ }\mu _i \) ’s are equal, for \( i=\) 1, 2, 3”.
Considering Theorems 5.1 and 5.2 , one can calculate the observed values of ANOVA statistics which are reported in Table 3 , based on the given STFNs. For instance, the total sum of squares is calculated for \(m=1\) by Theorem 5.1 as follows
And, also by Theorem 5.2 the observed value of ANOVA test statistic for \(m=1\) is equal to
By comparing the computed ANOVA test statistic, one can accept the alternative hypothesis \(H_1 \) at significance level 0.05. The critical value of ANOVA test is \(F_{1-\alpha ;r-1,n_t -r} =F_{0.95;2,9} =4.256\) and also the computed p -value \( =18\times 10^{-5}\) shows accuracy of \(H_1 \) strongly. Therefore, we conclude that there is a relation between soaps and weight loss based on recorded vague data in Table 2 . One can see the solution of this applied case study, but by extension principle, in Sect. 8.2 of [ 21 ], which leads the decision maker to a very vague observed fisher statistic, and as presented in Remark 4.1 , this matter can be caused lowering the truth level of decision.
Conclusions and future works
In most applied sciences, there are several situations for which non-precise values can be assigned to their experiments. Fuzzy/non-precise numbers are suitable models to formalize the observed values in such situations and the observed data could be presented by the notion of fuzzy sets to analyze the experiment. The main contribution of this article is extending ANOVA test for fuzzy observations based on a distance between symmetric fuzzy numbers. Several fast computing formulas obtained where the observations are STFNs or NFNs. When all observations are real numbers, the presented ANOVA method reduces to the classical ANOVA approach, since the vagueness of the observed statistics is removed and what remained are only the central points of the statistics, as one can see in Theorems 5.1 , 5.3 and Remark 4.2 . The proposed ANOVA approach is a meaningful generalization for the classical ANOVA. Efficiency in large sampling is the major advantage of this method, and also this method is easy to be used by professional clients who are familiar with ANOVA, which are some of contributions of the presented manuscript.
For future research work, one can try to use the same approach of this paper to extend other experimental designs such as random block design, Latin square design, etc., where the observations are fuzzy numbers rather than being real numbers. Another interesting topic for future works is to extend the results of this paper for trapezoidal fuzzy numbers and non-symmetric fuzzy numbers, or in general for LR fuzzy numbers.
Cochran WG, Cox GM (1957) Experimental designs, 2nd edn. Wiley, New York
MATH Google Scholar
Cuevas A, Febrero M, Fraiman R (2004) An ANOVA test for functional data. Comput Stat Data Anal 47:111–122
Article MathSciNet MATH Google Scholar
Dean A, Voss D (1999) Design and analysis of experiments. Springer, New York
Book MATH Google Scholar
De Garibay VG (1987) Behaviour of Fuzzy ANOVA. Kybernetes 16(2):107–112
Article Google Scholar
Dubois D, Prade H (1980) Fuzzy sets and systems: theory and application. Academic, New York
Filzmoser P, Viertl R (2004) Testing hypotheses with fuzzy data: the fuzzy \(p\) -value. Metrika 59:21–29
Gil MA, Montenegro M, González-Rodríguez G, Colubi A, Casals MR (2006) Bootstrap approach to the multi-sample test of means with imprecise data. Comput Stat Data Anal 51(1):148–162
González-Rodríguez G, Colubi A, Gil MA (2011) Fuzzy data treated as functional data: a one-way ANOVA test approach. Comput Stat Data Anal 56:943–955
Hesamian G (2016) One-way ANOVA based on interval information. Int J Syst Sci 47(11):2682–2690
Hocking RR (1996) Methods and applications of linear models: regression and the analysis of variance. Wiley, New York
Google Scholar
Ivani R, Sanaei Nejad SH, Ghahraman B, Astaraei AR, Feizi H (2016) Fuzzy analysis of variance and its practical application in agriculture. In: Kahraman C, Kabak O (eds) Fuzzy statistical decision-making: theory and applications. Studies in fuzziness and soft computing. Springer, Switzerland, pp 315–327
Jiryaei A, Parchami A, Mashinchi M (2013) One-way ANOVA and least squares method based on fuzzy random variables. Turk J Fuzzy Syst 4(1):18–33
Kalpanapriya D, Pandian P (2012) Fuzzy hypothesis testing of ANOVA model with fuzzy data. Int J Mod Eng Res 2:2951–2956
Kaya I, Kahraman C (2011) Process capability analyses with fuzzy parameters. Expert Syst Appl 38(9):11918–11927
Konishi M, Okuda T, Asai K (2006) Analysis of variance based on fuzzy interval data using moment correction method. Int J Innov Comput Inf Control 2(1):83–99
Lubiano MA, Trutschnig W (2010) ANOVA for fuzzy random variables using the R-package SAFD. Comb Soft Comput Stat Methods Data Anal Adv Intell Soft Comput 77:449–456
Montenegro M, Colubi A, Casals MR, Gil MA (2004) Asymptotic and bootstrap techniques for testing the expected value of a fuzzy random variable. Metrika 59:31–49
Montenegro M, Gonzalez-Rodriguez G, Gil MA, Colubi A, Casals MR (2004) Introduction to ANOVA with fuzzy random variables. In: López-Díaz MC, Angeles Gil M, Grzegorzewski P, Hryniewicz O, Lawry J (eds) Soft Methodol Random Inf Syst. Springer, Berlin, pp 487–494
Chapter Google Scholar
Montgomery DC (1991) Design and analysis of experiments, 3rd edn. Wiley, New York
Nguyen HT, Walker EA (2005) A first course in fuzzy logic, 3rd edn. Chapman Hall/CRC, Paris
Nourbakhsh M, Parchami A, Mashinchi M (2013) Analysis of variance based on fuzzy observations. Int J Syst Sci 44(4):714–726
Parchami A, Ivani R, Mashinchi M, Kaya İ (2017) An implication of fuzzy ANOVA: metal uptake and transport by corn grown on a contaminated soil. Chemom Intell Lab Syst 164:56–63
Parchami A, Sadeghpour-Gildeh B, Nourbakhsh M, Mashinchi M (2014) A new generation of process capability indices based on fuzzy measurements. J Appl Stat 41(5):1122–1136
Parchami A, Taheri SM, Mashinchi M (2012) Testing fuzzy hypotheses based on vague observations: a \(p\) -value approach. Stat Pap 53(2):469–484
Rodriguez G, Colubi A, Gil MA (2012) Fuzzy data treated as functional data: a one-way ANOVA test approach. Comput Stat Data Anal 56(4):943–955
Taheri SM, Arefi M (2009) Testing hypotheses based on fuzzy test statistic. Soft Comput 13:617–625
Article MATH Google Scholar
Viertl R (2011) Statistical methods for fuzzy data. Wiley, New York
Wu HC (2007) Analysis of variance for fuzzy data. Int J Syst Sci 38:235–246
Xu R, Li C (2001) Multidimensional least-squares fitting with a fuzzy model. Fuzzy Sets Syst 119:215–223
Zadeh LA (1975) The concept of a linguistic variable and its application to approximate reasoning—I. Inf Sci 8:199–249
Zadeh LA (1965) Fuzzy sets. Inf Control 8:338–359
Download references
Author information
Authors and affiliations.
Department of Statistics, Faculty of Mathematics and Computer, Shahid Bahonar University of Kerman, Kerman, Iran
A. Parchami & M. Mashinchi
School of Mathematics, Iran University of Science and Technology, Narmak, Tehran, Iran
M. Nourbakhsh
You can also search for this author in PubMed Google Scholar
Corresponding author
Correspondence to A. Parchami .
Rights and permissions
Open Access This article is distributed under the terms of the Creative Commons Attribution 4.0 International License ( http://creativecommons.org/licenses/by/4.0 /), which permits unrestricted use, distribution, and reproduction in any medium, provided you give appropriate credit to the original author(s) and the source, provide a link to the Creative Commons license, and indicate if changes were made.
Reprints and permissions
About this article
Parchami, A., Nourbakhsh, M. & Mashinchi, M. Analysis of variance in uncertain environments. Complex Intell. Syst. 3 , 189–196 (2017). https://doi.org/10.1007/s40747-017-0046-8
Download citation
Received : 21 February 2017
Accepted : 31 May 2017
Published : 10 June 2017
Issue Date : October 2017
DOI : https://doi.org/10.1007/s40747-017-0046-8
Share this article
Anyone you share the following link with will be able to read this content:
Sorry, a shareable link is not currently available for this article.
Provided by the Springer Nature SharedIt content-sharing initiative
- Symmetric triangular fuzzy number
- Symmetric normal fuzzy number
- Arithmetic operations
- Testing hypotheses
- Analysis of variance
- Find a journal
- Publish with us
- Track your research

COMMENTS
In one-way analysis of variance, the same principle is used, with variances rather than standard deviations being used to measure variability. The variance of a set of n values (x 1, x 2 ... x n) is given by the following (i.e. sum of squares divided by the degrees of freedom): Where the sum of squares = and the degrees of freedom = n - 1.
Most readers are already aware of the fact that the most common analytical method for this is the one-way analysis of variance (ANOVA). The present article aims to examine the necessity of using a one-way ANOVA instead of simply repeating the comparisons using Student's t-test. ANOVA literally means analysis of variance, and the present article ...
Analysis of variance is a procedure that examines the effect of one (or more) independent variable(s) on one (or more) dependent variable(s). ... In the literature, many variants of post-hoc tests are discussed, which differ, ... A review of the descriptive results reveals differences in the mean sales volumes for the combinations of factor ...
Analysis of variance (often referred to as ANOVA) is a. technique for analyzing the way in which the mean of a. variable is affected by different types and combinations of. factors. One-way ...
The methods introduced so far test difference hypotheses for a maximum of two groups or conditions with a t- test. Many research designs will actually include more than two groups or conditions, however, and these situations require a different statistical approach. One particularly widespread test is the so-called Analysis of variance (ANOVA).
This chapter introduces the statistical test, analysis of variance, also known as the ANOVA. The ANOVA is used to determine if there is a difference in population means between three or more groups without using multiple t-tests or determining an independent variable's effects.This makes it a bivariate statistical model for multiple groups as well as an omnibus test.
The development of analysis of variance (ANOVA) methodology has in turn had an influenced on the types of experimental research being carried out in many fields. ANOVA is one of the most commonly used statistical techniques, with applications across the full spectrum of experiments in agriculture, biology, chemistry, toxicology, pharmaceutical ...
Analysis of variance (ANOVA) [57] is a statistical test used to detect the difference in the mean of the test group when there is a parameter-dependent variable and one or more independent ...
ANOVA (analysis of variance) is the mainstay of most general textbooks and courses on applied statistics. Open any book aimed at advanced undergraduate level to intermediate graduates, and often half the chapters are illustrated using ANOVA. Statistically oriented packages such as SAS employ ANOVA throughout their basic routines.
Abstract. This review introduces one-way analysis of variance, which is a method of testing differences between more than two groups or treatments. Multiple comparison procedures and orthogonal contrasts are described as methods for identifying specific differences between pairs of treatments.
Introduction. Analysis of variance (ANOVA) is the most efficient parametric method available for the analysis of data from experiments.It was devised originally to test the differences between several different groups of treatments thus circumventing the problem of making multiple comparisons between the group means using t-tests (Snedecor and Cochran, 1980).
In order to use two linear factors (the sines and cosines of the recorded angles) as dependent variables, we needed to switch from univariate linear models to a multivariate analysis of variance. This approach allows the use of multiple response variables [17].
One-way analysis of variance (ANOVA) A parametric test of statistical significance that assesses whether differences in the means of several samples (groups) can lead the researcher to reject the null hypothesis that the means of the populations from which the samples are drawn are the same. Q-Q plot.
The t-distribution is similar to the normal distribution when the estimate of variance is based on many degrees of freedom (df) (i.e., larger samples), but has relatively more scores in its tails when there are fewer degrees of freedom (i.e., smaller samples). The t-distribution has n− 1 degrees of freedom, where n is the sample size. Figure 2.1 shows the t distribution with four df and the ...
In one-way analysis of variance, the same principle is used, with variances rather than standard deviations being used to measure variability. The variance of a set of n values (x 1, x 2 ... x n) is given by the following (i.e. sum of squares divided by the degrees of freedom): Where the sum of squares =. and the degrees of freedom = n - 1.
Explore the latest full-text research PDFs, articles, conference papers, preprints and more on ANALYSES OF VARIANCE. Find methods information, sources, references or conduct a literature review on ...
Microsoft Word - 2 - Manova 4.3.05. Multivariate Analysis of Variance. What Multivariate Analysis of Variance is. The general purpose of multivariate analysis of variance (MANOVA) is to determine. whether multiple levels of independent variables on their own or in combination with one. another have an effect on the dependent variables.
Analysis of variance (often referred to as ANOVA) is a technique for analyzing the way in which the mean of a variable is affected by different types and combinations of factors. One-way analysis of variance is the simplest form. It is an extension of the independent samples t-test (see statistics review 5 [1]) and can be used to compare any ...
2.3 Statistical analysis. Analysis of variance (ANOVA) was used to determine differences in plasma lipid and lipoprotein fatty acids between the two groups of the formula-fed infants, and between each group of formula-fed infants and the breast-fed infants. Differences were considered significant at P <0.05.
Analysis of variance (Anova ) is a collection of statistical models and their associated estimation procedures (such as the "variation" among and between groups), as used to analyze the differences among group means in a sample. Anova was developed by the statistician and evolutionary biologist Ronald Fisher 1890-1962.
Conducted a historical analysis of the period from 1925 to 1950 to investigate the incorporation of ANOVA techniques in psychological research. In addition to attempts to identify the earliest uses of ANOVA in psychology, the gradual incorporation of the technique was examined by counting its appearance in 6,457 articles appearing in major American psychological journals from 1935 through 1952 ...
The purpose of this study was to develop an Infertility Perception Scale for Women (IPS-W). Initial items were based on an extensive literature review and in-depth interviews with five infertile women and fifteen women not diagnosed with infertility. Forty-one items were derived from a pilot survey. Data were collected from 203 women who had experienced intrauterine insemination (IUI) and in ...
Testing one-way analysis of variance (ANOVA) is used for experimental data analysis in which there is a continuous response variable and a single independent classification variable. In this paper, we extend one-way ANOVA to a case where observed data are imprecise numbers rather than real numbers. Several fast computable formulas are calculated for symmetric triangular and normal fuzzy data ...