P-Value And Statistical Significance: What It Is & Why It Matters
Saul Mcleod, PhD
Editor-in-Chief for Simply Psychology
BSc (Hons) Psychology, MRes, PhD, University of Manchester
Saul Mcleod, PhD., is a qualified psychology teacher with over 18 years of experience in further and higher education. He has been published in peer-reviewed journals, including the Journal of Clinical Psychology.
Learn about our Editorial Process
Olivia Guy-Evans, MSc
Associate Editor for Simply Psychology
BSc (Hons) Psychology, MSc Psychology of Education
Olivia Guy-Evans is a writer and associate editor for Simply Psychology. She has previously worked in healthcare and educational sectors.
On This Page:
The p-value in statistics quantifies the evidence against a null hypothesis. A low p-value suggests data is inconsistent with the null, potentially favoring an alternative hypothesis. Common significance thresholds are 0.05 or 0.01.
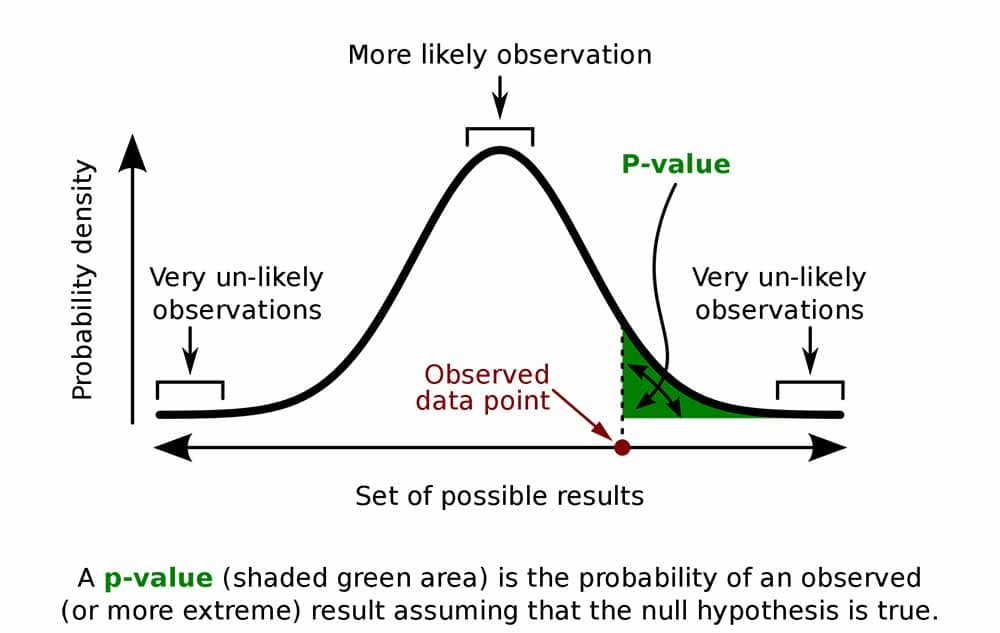

Hypothesis testing
When you perform a statistical test, a p-value helps you determine the significance of your results in relation to the null hypothesis.
The null hypothesis (H0) states no relationship exists between the two variables being studied (one variable does not affect the other). It states the results are due to chance and are not significant in supporting the idea being investigated. Thus, the null hypothesis assumes that whatever you try to prove did not happen.
The alternative hypothesis (Ha or H1) is the one you would believe if the null hypothesis is concluded to be untrue.
The alternative hypothesis states that the independent variable affected the dependent variable, and the results are significant in supporting the theory being investigated (i.e., the results are not due to random chance).
What a p-value tells you
A p-value, or probability value, is a number describing how likely it is that your data would have occurred by random chance (i.e., that the null hypothesis is true).
The level of statistical significance is often expressed as a p-value between 0 and 1.
The smaller the p -value, the less likely the results occurred by random chance, and the stronger the evidence that you should reject the null hypothesis.
Remember, a p-value doesn’t tell you if the null hypothesis is true or false. It just tells you how likely you’d see the data you observed (or more extreme data) if the null hypothesis was true. It’s a piece of evidence, not a definitive proof.
Example: Test Statistic and p-Value
Suppose you’re conducting a study to determine whether a new drug has an effect on pain relief compared to a placebo. If the new drug has no impact, your test statistic will be close to the one predicted by the null hypothesis (no difference between the drug and placebo groups), and the resulting p-value will be close to 1. It may not be precisely 1 because real-world variations may exist. Conversely, if the new drug indeed reduces pain significantly, your test statistic will diverge further from what’s expected under the null hypothesis, and the p-value will decrease. The p-value will never reach zero because there’s always a slim possibility, though highly improbable, that the observed results occurred by random chance.
P-value interpretation
The significance level (alpha) is a set probability threshold (often 0.05), while the p-value is the probability you calculate based on your study or analysis.
A p-value less than or equal to your significance level (typically ≤ 0.05) is statistically significant.
A p-value less than or equal to a predetermined significance level (often 0.05 or 0.01) indicates a statistically significant result, meaning the observed data provide strong evidence against the null hypothesis.
This suggests the effect under study likely represents a real relationship rather than just random chance.
For instance, if you set α = 0.05, you would reject the null hypothesis if your p -value ≤ 0.05.
It indicates strong evidence against the null hypothesis, as there is less than a 5% probability the null is correct (and the results are random).
Therefore, we reject the null hypothesis and accept the alternative hypothesis.
Example: Statistical Significance
Upon analyzing the pain relief effects of the new drug compared to the placebo, the computed p-value is less than 0.01, which falls well below the predetermined alpha value of 0.05. Consequently, you conclude that there is a statistically significant difference in pain relief between the new drug and the placebo.
What does a p-value of 0.001 mean?
A p-value of 0.001 is highly statistically significant beyond the commonly used 0.05 threshold. It indicates strong evidence of a real effect or difference, rather than just random variation.
Specifically, a p-value of 0.001 means there is only a 0.1% chance of obtaining a result at least as extreme as the one observed, assuming the null hypothesis is correct.
Such a small p-value provides strong evidence against the null hypothesis, leading to rejecting the null in favor of the alternative hypothesis.
A p-value more than the significance level (typically p > 0.05) is not statistically significant and indicates strong evidence for the null hypothesis.
This means we retain the null hypothesis and reject the alternative hypothesis. You should note that you cannot accept the null hypothesis; we can only reject it or fail to reject it.
Note : when the p-value is above your threshold of significance, it does not mean that there is a 95% probability that the alternative hypothesis is true.
One-Tailed Test
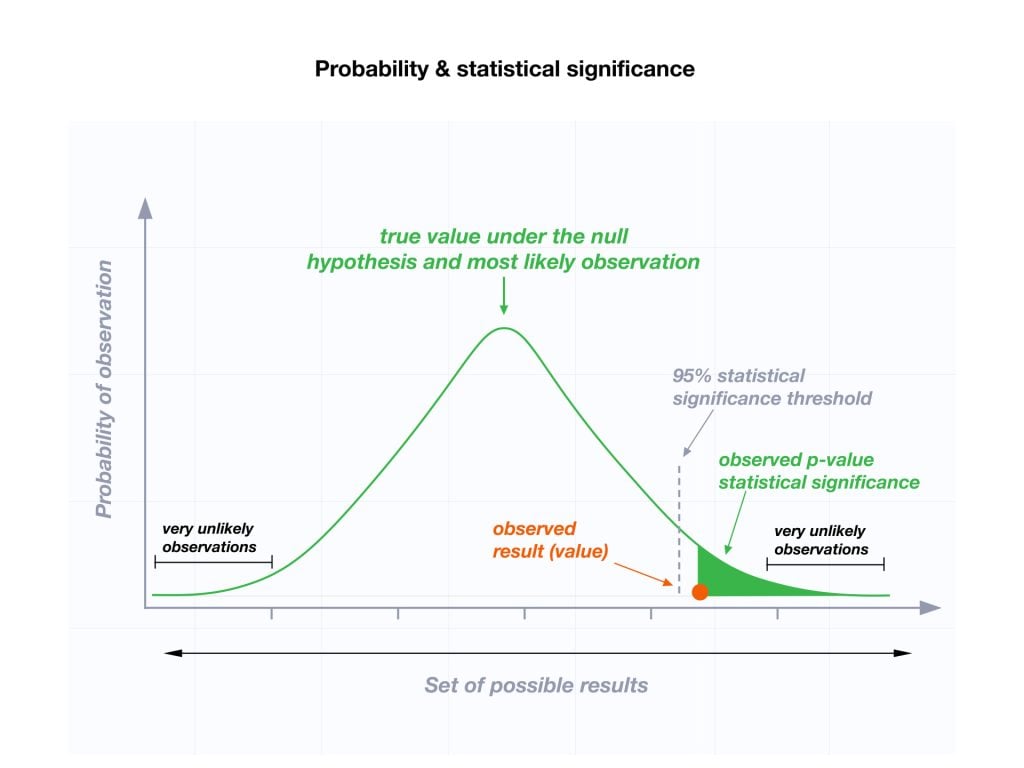
Two-Tailed Test
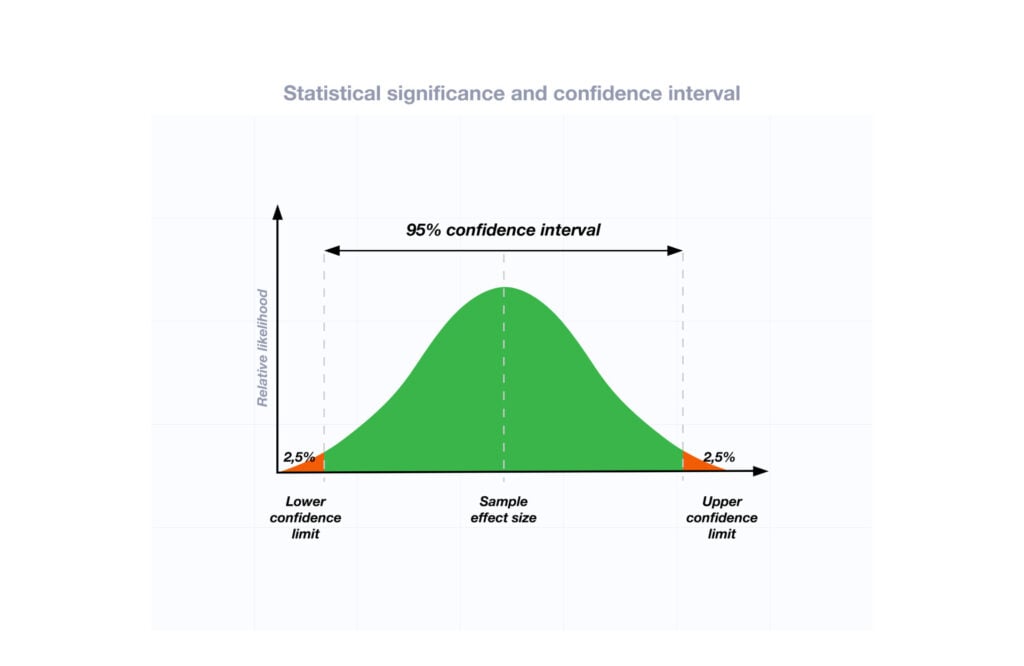
How do you calculate the p-value ?
Most statistical software packages like R, SPSS, and others automatically calculate your p-value. This is the easiest and most common way.
Online resources and tables are available to estimate the p-value based on your test statistic and degrees of freedom.
These tables help you understand how often you would expect to see your test statistic under the null hypothesis.
Understanding the Statistical Test:
Different statistical tests are designed to answer specific research questions or hypotheses. Each test has its own underlying assumptions and characteristics.
For example, you might use a t-test to compare means, a chi-squared test for categorical data, or a correlation test to measure the strength of a relationship between variables.
Be aware that the number of independent variables you include in your analysis can influence the magnitude of the test statistic needed to produce the same p-value.
This factor is particularly important to consider when comparing results across different analyses.
Example: Choosing a Statistical Test
If you’re comparing the effectiveness of just two different drugs in pain relief, a two-sample t-test is a suitable choice for comparing these two groups. However, when you’re examining the impact of three or more drugs, it’s more appropriate to employ an Analysis of Variance ( ANOVA) . Utilizing multiple pairwise comparisons in such cases can lead to artificially low p-values and an overestimation of the significance of differences between the drug groups.
How to report
A statistically significant result cannot prove that a research hypothesis is correct (which implies 100% certainty).
Instead, we may state our results “provide support for” or “give evidence for” our research hypothesis (as there is still a slight probability that the results occurred by chance and the null hypothesis was correct – e.g., less than 5%).
Example: Reporting the results
In our comparison of the pain relief effects of the new drug and the placebo, we observed that participants in the drug group experienced a significant reduction in pain ( M = 3.5; SD = 0.8) compared to those in the placebo group ( M = 5.2; SD = 0.7), resulting in an average difference of 1.7 points on the pain scale (t(98) = -9.36; p < 0.001).
The 6th edition of the APA style manual (American Psychological Association, 2010) states the following on the topic of reporting p-values:
“When reporting p values, report exact p values (e.g., p = .031) to two or three decimal places. However, report p values less than .001 as p < .001.
The tradition of reporting p values in the form p < .10, p < .05, p < .01, and so forth, was appropriate in a time when only limited tables of critical values were available.” (p. 114)
- Do not use 0 before the decimal point for the statistical value p as it cannot equal 1. In other words, write p = .001 instead of p = 0.001.
- Please pay attention to issues of italics ( p is always italicized) and spacing (either side of the = sign).
- p = .000 (as outputted by some statistical packages such as SPSS) is impossible and should be written as p < .001.
- The opposite of significant is “nonsignificant,” not “insignificant.”
Why is the p -value not enough?
A lower p-value is sometimes interpreted as meaning there is a stronger relationship between two variables.
However, statistical significance means that it is unlikely that the null hypothesis is true (less than 5%).
To understand the strength of the difference between the two groups (control vs. experimental) a researcher needs to calculate the effect size .
When do you reject the null hypothesis?
In statistical hypothesis testing, you reject the null hypothesis when the p-value is less than or equal to the significance level (α) you set before conducting your test. The significance level is the probability of rejecting the null hypothesis when it is true. Commonly used significance levels are 0.01, 0.05, and 0.10.
Remember, rejecting the null hypothesis doesn’t prove the alternative hypothesis; it just suggests that the alternative hypothesis may be plausible given the observed data.
The p -value is conditional upon the null hypothesis being true but is unrelated to the truth or falsity of the alternative hypothesis.
What does p-value of 0.05 mean?
If your p-value is less than or equal to 0.05 (the significance level), you would conclude that your result is statistically significant. This means the evidence is strong enough to reject the null hypothesis in favor of the alternative hypothesis.
Are all p-values below 0.05 considered statistically significant?
No, not all p-values below 0.05 are considered statistically significant. The threshold of 0.05 is commonly used, but it’s just a convention. Statistical significance depends on factors like the study design, sample size, and the magnitude of the observed effect.
A p-value below 0.05 means there is evidence against the null hypothesis, suggesting a real effect. However, it’s essential to consider the context and other factors when interpreting results.
Researchers also look at effect size and confidence intervals to determine the practical significance and reliability of findings.
How does sample size affect the interpretation of p-values?
Sample size can impact the interpretation of p-values. A larger sample size provides more reliable and precise estimates of the population, leading to narrower confidence intervals.
With a larger sample, even small differences between groups or effects can become statistically significant, yielding lower p-values. In contrast, smaller sample sizes may not have enough statistical power to detect smaller effects, resulting in higher p-values.
Therefore, a larger sample size increases the chances of finding statistically significant results when there is a genuine effect, making the findings more trustworthy and robust.
Can a non-significant p-value indicate that there is no effect or difference in the data?
No, a non-significant p-value does not necessarily indicate that there is no effect or difference in the data. It means that the observed data do not provide strong enough evidence to reject the null hypothesis.
There could still be a real effect or difference, but it might be smaller or more variable than the study was able to detect.
Other factors like sample size, study design, and measurement precision can influence the p-value. It’s important to consider the entire body of evidence and not rely solely on p-values when interpreting research findings.
Can P values be exactly zero?
While a p-value can be extremely small, it cannot technically be absolute zero. When a p-value is reported as p = 0.000, the actual p-value is too small for the software to display. This is often interpreted as strong evidence against the null hypothesis. For p values less than 0.001, report as p < .001
Further Information
- P-values and significance tests (Kahn Academy)
- Hypothesis testing and p-values (Kahn Academy)
- Wasserstein, R. L., Schirm, A. L., & Lazar, N. A. (2019). Moving to a world beyond “ p “< 0.05”.
- Criticism of using the “ p “< 0.05”.
- Publication manual of the American Psychological Association
- Statistics for Psychology Book Download
Bland, J. M., & Altman, D. G. (1994). One and two sided tests of significance: Authors’ reply. BMJ: British Medical Journal , 309 (6958), 874.
Goodman, S. N., & Royall, R. (1988). Evidence and scientific research. American Journal of Public Health , 78 (12), 1568-1574.
Goodman, S. (2008, July). A dirty dozen: twelve p-value misconceptions . In Seminars in hematology (Vol. 45, No. 3, pp. 135-140). WB Saunders.
Lang, J. M., Rothman, K. J., & Cann, C. I. (1998). That confounded P-value. Epidemiology (Cambridge, Mass.) , 9 (1), 7-8.

Have a language expert improve your writing
Run a free plagiarism check in 10 minutes, generate accurate citations for free.
- Knowledge Base
- Understanding P values | Definition and Examples
Understanding P-values | Definition and Examples
Published on July 16, 2020 by Rebecca Bevans . Revised on June 22, 2023.
The p value is a number, calculated from a statistical test, that describes how likely you are to have found a particular set of observations if the null hypothesis were true.
P values are used in hypothesis testing to help decide whether to reject the null hypothesis. The smaller the p value, the more likely you are to reject the null hypothesis.
Table of contents
What is a null hypothesis, what exactly is a p value, how do you calculate the p value, p values and statistical significance, reporting p values, caution when using p values, other interesting articles, frequently asked questions about p-values.
All statistical tests have a null hypothesis. For most tests, the null hypothesis is that there is no relationship between your variables of interest or that there is no difference among groups.
For example, in a two-tailed t test , the null hypothesis is that the difference between two groups is zero.
- Null hypothesis ( H 0 ): there is no difference in longevity between the two groups.
- Alternative hypothesis ( H A or H 1 ): there is a difference in longevity between the two groups.
Prevent plagiarism. Run a free check.
The p value , or probability value, tells you how likely it is that your data could have occurred under the null hypothesis. It does this by calculating the likelihood of your test statistic , which is the number calculated by a statistical test using your data.
The p value tells you how often you would expect to see a test statistic as extreme or more extreme than the one calculated by your statistical test if the null hypothesis of that test was true. The p value gets smaller as the test statistic calculated from your data gets further away from the range of test statistics predicted by the null hypothesis.
The p value is a proportion: if your p value is 0.05, that means that 5% of the time you would see a test statistic at least as extreme as the one you found if the null hypothesis was true.
P values are usually automatically calculated by your statistical program (R, SPSS, etc.).
You can also find tables for estimating the p value of your test statistic online. These tables show, based on the test statistic and degrees of freedom (number of observations minus number of independent variables) of your test, how frequently you would expect to see that test statistic under the null hypothesis.
The calculation of the p value depends on the statistical test you are using to test your hypothesis :
- Different statistical tests have different assumptions and generate different test statistics. You should choose the statistical test that best fits your data and matches the effect or relationship you want to test.
- The number of independent variables you include in your test changes how large or small the test statistic needs to be to generate the same p value.
No matter what test you use, the p value always describes the same thing: how often you can expect to see a test statistic as extreme or more extreme than the one calculated from your test.
P values are most often used by researchers to say whether a certain pattern they have measured is statistically significant.
Statistical significance is another way of saying that the p value of a statistical test is small enough to reject the null hypothesis of the test.
How small is small enough? The most common threshold is p < 0.05; that is, when you would expect to find a test statistic as extreme as the one calculated by your test only 5% of the time. But the threshold depends on your field of study – some fields prefer thresholds of 0.01, or even 0.001.
The threshold value for determining statistical significance is also known as the alpha value.
P values of statistical tests are usually reported in the results section of a research paper , along with the key information needed for readers to put the p values in context – for example, correlation coefficient in a linear regression , or the average difference between treatment groups in a t -test.
P values are often interpreted as your risk of rejecting the null hypothesis of your test when the null hypothesis is actually true.
In reality, the risk of rejecting the null hypothesis is often higher than the p value, especially when looking at a single study or when using small sample sizes. This is because the smaller your frame of reference, the greater the chance that you stumble across a statistically significant pattern completely by accident.
P values are also often interpreted as supporting or refuting the alternative hypothesis. This is not the case. The p value can only tell you whether or not the null hypothesis is supported. It cannot tell you whether your alternative hypothesis is true, or why.
If you want to know more about statistics , methodology , or research bias , make sure to check out some of our other articles with explanations and examples.
- Normal distribution
- Descriptive statistics
- Measures of central tendency
- Correlation coefficient
- Null hypothesis
Methodology
- Cluster sampling
- Stratified sampling
- Types of interviews
- Cohort study
- Thematic analysis
Research bias
- Implicit bias
- Cognitive bias
- Survivorship bias
- Availability heuristic
- Nonresponse bias
- Regression to the mean
A p -value , or probability value, is a number describing how likely it is that your data would have occurred under the null hypothesis of your statistical test .
P -values are usually automatically calculated by the program you use to perform your statistical test. They can also be estimated using p -value tables for the relevant test statistic .
P -values are calculated from the null distribution of the test statistic. They tell you how often a test statistic is expected to occur under the null hypothesis of the statistical test, based on where it falls in the null distribution.
If the test statistic is far from the mean of the null distribution, then the p -value will be small, showing that the test statistic is not likely to have occurred under the null hypothesis.
Statistical significance is a term used by researchers to state that it is unlikely their observations could have occurred under the null hypothesis of a statistical test . Significance is usually denoted by a p -value , or probability value.
Statistical significance is arbitrary – it depends on the threshold, or alpha value, chosen by the researcher. The most common threshold is p < 0.05, which means that the data is likely to occur less than 5% of the time under the null hypothesis .
When the p -value falls below the chosen alpha value, then we say the result of the test is statistically significant.
No. The p -value only tells you how likely the data you have observed is to have occurred under the null hypothesis .
If the p -value is below your threshold of significance (typically p < 0.05), then you can reject the null hypothesis, but this does not necessarily mean that your alternative hypothesis is true.
Cite this Scribbr article
If you want to cite this source, you can copy and paste the citation or click the “Cite this Scribbr article” button to automatically add the citation to our free Citation Generator.
Bevans, R. (2023, June 22). Understanding P-values | Definition and Examples. Scribbr. Retrieved April 15, 2024, from https://www.scribbr.com/statistics/p-value/
Is this article helpful?
Rebecca Bevans
Other students also liked, an easy introduction to statistical significance (with examples), test statistics | definition, interpretation, and examples, what is effect size and why does it matter (examples), what is your plagiarism score.
Thank you for visiting nature.com. You are using a browser version with limited support for CSS. To obtain the best experience, we recommend you use a more up to date browser (or turn off compatibility mode in Internet Explorer). In the meantime, to ensure continued support, we are displaying the site without styles and JavaScript.
- View all journals
- Explore content
- About the journal
- Publish with us
- Sign up for alerts
- Published: 26 November 2021
The clinician’s guide to p values, confidence intervals, and magnitude of effects
- Mark R. Phillips ORCID: orcid.org/0000-0003-0923-261X 1 na1 ,
- Charles C. Wykoff 2 , 3 ,
- Lehana Thabane ORCID: orcid.org/0000-0003-0355-9734 1 , 4 ,
- Mohit Bhandari ORCID: orcid.org/0000-0001-9608-4808 1 , 5 &
- Varun Chaudhary ORCID: orcid.org/0000-0002-9988-4146 1 , 5
for the Retina Evidence Trials InterNational Alliance (R.E.T.I.N.A.) Study Group
Eye volume 36 , pages 341–342 ( 2022 ) Cite this article
16k Accesses
5 Citations
14 Altmetric
Metrics details
- Outcomes research
A Correction to this article was published on 19 January 2022
This article has been updated
Introduction
There are numerous statistical and methodological considerations within every published study, and the ability of clinicians to appreciate the implications and limitations associated with these key concepts is critically important. These implications often have a direct impact on the applicability of study findings – which, in turn, often determine the appropriateness for the results to lead to modification of practice patterns. Because it can be challenging and time-consuming for busy clinicians to break down the nuances of each study, herein we provide a brief summary of 3 important topics that every ophthalmologist should consider when interpreting evidence.
p -values: what they tell us and what they don’t
Perhaps the most universally recognized statistic is the p-value. Most individuals understand the notion that (usually) a p -value <0.05 signifies a statistically significant difference between the two groups being compared. While this understanding is shared amongst most, it is far more important to understand what a p -value does not tell us. Attempting to inform clinical practice patterns through interpretation of p -values is overly simplistic, and is fraught with potential for misleading conclusions. A p -value represents the probability that the observed result (difference between the groups being compared)—or one that is more extreme—would occur by random chance, assuming that the null hypothesis (the alternative scenario to the study’s hypothesis) is that there are no differences between the groups being compared. For example, a p -value of 0.04 would indicate that the difference between the groups compared would have a 4% chance of occurring by random chance. When this probability is small, it becomes less likely that the null hypothesis is accurate—or, alternatively, that the probability of a difference between groups is high [ 1 ]. Studies use a predefined threshold to determine when a p -value is sufficiently small enough to support the study hypothesis. This threshold is conventionally a p -value of 0.05; however, there are reasons and justifications for studies to use a different threshold if appropriate.
What a p -value cannot tell us, is the clinical relevance or importance of the observed treatment effects. [ 1 ]. Specifically, a p -value does not provide details about the magnitude of effect [ 2 , 3 , 4 ]. Despite a significant p -value, it is quite possible for the difference between the groups to be small. This phenomenon is especially common with larger sample sizes in which comparisons may result in statistically significant differences that are actually not clinically meaningful. For example, a study may find a statistically significant difference ( p < 0.05) between the visual acuity outcomes between two groups, while the difference between the groups may only amount to a 1 or less letter difference. While this may be in fact a statistically significant difference, the difference is likely not large enough to make a meaningful difference for patients. Thus, p -values lack vital information on the magnitude of effects for the assessed outcomes [ 2 , 3 , 4 ].
Overcoming the limitations of interpreting p -values: magnitude of effect
To overcome this limitation, it is important to consider both (1) whether or not the p -value of a comparison is significant according to the pre-defined statistical plan, and (2) the magnitude of the treatment effects (commonly reported as an effect estimate with 95% confidence intervals) [ 5 ]. The magnitude of effect is most often represented as the mean difference between groups for continuous outcomes, such as visual acuity on the logMAR scale, and the risk or odds ratio for dichotomous/binary outcomes, such as occurrence of adverse events. These measures indicate the observed effect that was quantified by the study comparison. As suggested in the previous section, understanding the actual magnitude of the difference in the study comparison provides an understanding of the results that an isolated p -value does not provide [ 4 , 5 ]. Understanding the results of a study should shift from a binary interpretation of significant vs not significant, and instead, focus on a more critical judgement of the clinical relevance of the observed effect [ 1 ].
There are a number of important metrics, such as the Minimally Important Difference (MID), which helps to determine if a difference between groups is large enough to be clinically meaningful [ 6 , 7 ]. When a clinician is able to identify (1) the magnitude of effect within a study, and (2) the MID (smallest change in the outcome that a patient would deem meaningful), they are far more capable of understanding the effects of a treatment, and further articulate the pros and cons of a treatment option to patients with reference to treatment effects that can be considered clinically valuable.
The role of confidence intervals
Confidence intervals are estimates that provide a lower and upper threshold to the estimate of the magnitude of effect. By convention, 95% confidence intervals are most typically reported. These intervals represent the range in which we can, with 95% confidence, assume the treatment effect to fall within. For example, a mean difference in visual acuity of 8 (95% confidence interval: 6 to 10) suggests that the best estimate of the difference between the two study groups is 8 letters, and we have 95% certainty that the true value is between 6 and 10 letters. When interpreting this clinically, one can consider the different clinical scenarios at each end of the confidence interval; if the patient’s outcome was to be the most conservative, in this case an improvement of 6 letters, would the importance to the patient be different than if the patient’s outcome was to be the most optimistic, or 10 letters in this example? When the clinical value of the treatment effect does not change when considering the lower versus upper confidence intervals, there is enhanced certainty that the treatment effect will be meaningful to the patient [ 4 , 5 ]. In contrast, if the clinical merits of a treatment appear different when considering the possibility of the lower versus the upper confidence intervals, one may be more cautious about the expected benefits to be anticipated with treatment [ 4 , 5 ].
There are a number of important details for clinicians to consider when interpreting evidence. Through this editorial, we hope to provide practical insights into fundamental methodological principals that can help guide clinical decision making. P -values are one small component to consider when interpreting study results, with much deeper appreciation of results being available when the treatment effects and associated confidence intervals are also taken into consideration.
Change history
19 january 2022.
A Correction to this paper has been published: https://doi.org/10.1038/s41433-021-01914-2
Li G, Walter SD, Thabane L. Shifting the focus away from binary thinking of statistical significance and towards education for key stakeholders: revisiting the debate on whether it’s time to de-emphasize or get rid of statistical significance. J Clin Epidemiol. 2021;137:104–12. https://doi.org/10.1016/j.jclinepi.2021.03.033
Article PubMed Google Scholar
Gagnier JJ, Morgenstern H. Misconceptions, misuses, and misinterpretations of p values and significance testing. J Bone Joint Surg Am. 2017;99:1598–603. https://doi.org/10.2106/JBJS.16.01314
Goodman SN. Toward evidence-based medical statistics. 1: the p value fallacy. Ann Intern Med. 1999;130:995–1004. https://doi.org/10.7326/0003-4819-130-12-199906150-00008
Article CAS PubMed Google Scholar
Greenland S, Senn SJ, Rothman KJ, Carlin JB, Poole C, Goodman SN, et al. Statistical tests, p values, confidence intervals, and power: a guide to misinterpretations. Eur J Epidemiol. 2016;31:337–50. https://doi.org/10.1007/s10654-016-0149-3
Article PubMed PubMed Central Google Scholar
Phillips M. Letter to the editor: editorial: threshold p values in orthopaedic research-we know the problem. What is the solution? Clin Orthop. 2019;477:1756–8. https://doi.org/10.1097/CORR.0000000000000827
Devji T, Carrasco-Labra A, Qasim A, Phillips MR, Johnston BC, Devasenapathy N, et al. Evaluating the credibility of anchor based estimates of minimal important differences for patient reported outcomes: instrument development and reliability study. BMJ. 2020;369:m1714. https://doi.org/10.1136/bmj.m1714
Carrasco-Labra A, Devji T, Qasim A, Phillips MR, Wang Y, Johnston BC, et al. Minimal important difference estimates for patient-reported outcomes: a systematic survey. J Clin Epidemiol. 2020;0. https://doi.org/10.1016/j.jclinepi.2020.11.024
Download references
Author information
Authors and affiliations.
Department of Health Research Methods, Evidence, and Impact, McMaster University, Hamilton, ON, Canada
Mark R. Phillips, Lehana Thabane, Mohit Bhandari & Varun Chaudhary
Retina Consultants of Texas (Retina Consultants of America), Houston, TX, USA
Charles C. Wykoff
Blanton Eye Institute, Houston Methodist Hospital, Houston, TX, USA
Biostatistics Unit, St. Joseph’s Healthcare-Hamilton, Hamilton, ON, Canada
Lehana Thabane
Department of Surgery, McMaster University, Hamilton, ON, Canada
Mohit Bhandari & Varun Chaudhary
NIHR Moorfields Biomedical Research Centre, Moorfields Eye Hospital, London, UK
Sobha Sivaprasad
Cole Eye Institute, Cleveland Clinic, Cleveland, OH, USA
Peter Kaiser
Retinal Disorders and Ophthalmic Genetics, Stein Eye Institute, University of California, Los Angeles, CA, USA
David Sarraf
Department of Ophthalmology, Mayo Clinic, Rochester, MN, USA
Sophie J. Bakri
The Retina Service at Wills Eye Hospital, Philadelphia, PA, USA
Sunir J. Garg
Center for Ophthalmic Bioinformatics, Cole Eye Institute, Cleveland Clinic, Cleveland, OH, USA
Rishi P. Singh
Cleveland Clinic Lerner College of Medicine, Cleveland, OH, USA
Department of Ophthalmology, University of Bonn, Boon, Germany
Frank G. Holz
Singapore Eye Research Institute, Singapore, Singapore
Tien Y. Wong
Singapore National Eye Centre, Duke-NUD Medical School, Singapore, Singapore
Centre for Eye Research Australia, Royal Victorian Eye and Ear Hospital, East Melbourne, VIC, Australia
Robyn H. Guymer
Department of Surgery (Ophthalmology), The University of Melbourne, Melbourne, VIC, Australia
You can also search for this author in PubMed Google Scholar
- Varun Chaudhary
- , Mohit Bhandari
- , Charles C. Wykoff
- , Sobha Sivaprasad
- , Lehana Thabane
- , Peter Kaiser
- , David Sarraf
- , Sophie J. Bakri
- , Sunir J. Garg
- , Rishi P. Singh
- , Frank G. Holz
- , Tien Y. Wong
- & Robyn H. Guymer
Contributions
MRP was responsible for conception of idea, writing of manuscript and review of manuscript. VC was responsible for conception of idea, writing of manuscript and review of manuscript. MB was responsible for conception of idea, writing of manuscript and review of manuscript. CCW was responsible for critical review and feedback on manuscript. LT was responsible for critical review and feedback on manuscript.
Corresponding author
Correspondence to Varun Chaudhary .
Ethics declarations
Competing interests.
MRP: Nothing to disclose. CCW: Consultant: Acuela, Adverum Biotechnologies, Inc, Aerpio, Alimera Sciences, Allegro Ophthalmics, LLC, Allergan, Apellis Pharmaceuticals, Bayer AG, Chengdu Kanghong Pharmaceuticals Group Co, Ltd, Clearside Biomedical, DORC (Dutch Ophthalmic Research Center), EyePoint Pharmaceuticals, Gentech/Roche, GyroscopeTx, IVERIC bio, Kodiak Sciences Inc, Novartis AG, ONL Therapeutics, Oxurion NV, PolyPhotonix, Recens Medical, Regeron Pharmaceuticals, Inc, REGENXBIO Inc, Santen Pharmaceutical Co, Ltd, and Takeda Pharmaceutical Company Limited; Research funds: Adverum Biotechnologies, Inc, Aerie Pharmaceuticals, Inc, Aerpio, Alimera Sciences, Allergan, Apellis Pharmaceuticals, Chengdu Kanghong Pharmaceutical Group Co, Ltd, Clearside Biomedical, Gemini Therapeutics, Genentech/Roche, Graybug Vision, Inc, GyroscopeTx, Ionis Pharmaceuticals, IVERIC bio, Kodiak Sciences Inc, Neurotech LLC, Novartis AG, Opthea, Outlook Therapeutics, Inc, Recens Medical, Regeneron Pharmaceuticals, Inc, REGENXBIO Inc, Samsung Pharm Co, Ltd, Santen Pharmaceutical Co, Ltd, and Xbrane Biopharma AB—unrelated to this study. LT: Nothing to disclose. MB: Research funds: Pendopharm, Bioventus, Acumed – unrelated to this study. VC: Advisory Board Member: Alcon, Roche, Bayer, Novartis; Grants: Bayer, Novartis – unrelated to this study.
Additional information
Publisher’s note Springer Nature remains neutral with regard to jurisdictional claims in published maps and institutional affiliations.
The original version of this article was revised: In this article the middle initial in author name Sophie J. Bakri was missing.
Rights and permissions
Reprints and permissions
About this article
Cite this article.
Phillips, M.R., Wykoff, C.C., Thabane, L. et al. The clinician’s guide to p values, confidence intervals, and magnitude of effects. Eye 36 , 341–342 (2022). https://doi.org/10.1038/s41433-021-01863-w
Download citation
Received : 11 November 2021
Revised : 12 November 2021
Accepted : 15 November 2021
Published : 26 November 2021
Issue Date : February 2022
DOI : https://doi.org/10.1038/s41433-021-01863-w
Share this article
Anyone you share the following link with will be able to read this content:
Sorry, a shareable link is not currently available for this article.
Provided by the Springer Nature SharedIt content-sharing initiative
Quick links
- Explore articles by subject
- Guide to authors
- Editorial policies

- Search Search Please fill out this field.
What Is P-Value?
Understanding p-value.
- P-Value in Hypothesis Testing
The Bottom Line
- Corporate Finance
- Financial Analysis
P-Value: What It Is, How to Calculate It, and Why It Matters
:max_bytes(150000):strip_icc():format(webp)/100378251brianbeersheadshot__brian_beers-5bfc26274cedfd0026c00ebd.jpg)
Yarilet Perez is an experienced multimedia journalist and fact-checker with a Master of Science in Journalism. She has worked in multiple cities covering breaking news, politics, education, and more. Her expertise is in personal finance and investing, and real estate.
:max_bytes(150000):strip_icc():format(webp)/YariletPerez-d2289cb01c3c4f2aabf79ce6057e5078.jpg)
In statistics, a p-value is a number that indicates how likely you are to obtain a value that is at least equal to or more than the actual observation if the null hypothesis is correct.
The p-value serves as an alternative to rejection points to provide the smallest level of significance at which the null hypothesis would be rejected. A smaller p-value means stronger evidence in favor of the alternative hypothesis.
P-value is often used to promote credibility for studies or reports by government agencies. For example, the U.S. Census Bureau stipulates that any analysis with a p-value greater than 0.10 must be accompanied by a statement that the difference is not statistically different from zero. The Census Bureau also has standards in place stipulating which p-values are acceptable for various publications.
Key Takeaways
- A p-value is a statistical measurement used to validate a hypothesis against observed data.
- A p-value measures the probability of obtaining the observed results, assuming that the null hypothesis is true.
- The lower the p-value, the greater the statistical significance of the observed difference.
- A p-value of 0.05 or lower is generally considered statistically significant.
- P-value can serve as an alternative to—or in addition to—preselected confidence levels for hypothesis testing.
Jessica Olah / Investopedia
P-values are usually found using p-value tables or spreadsheets/statistical software. These calculations are based on the assumed or known probability distribution of the specific statistic tested. P-values are calculated from the deviation between the observed value and a chosen reference value, given the probability distribution of the statistic, with a greater difference between the two values corresponding to a lower p-value.
Mathematically, the p-value is calculated using integral calculus from the area under the probability distribution curve for all values of statistics that are at least as far from the reference value as the observed value is, relative to the total area under the probability distribution curve.
The calculation for a p-value varies based on the type of test performed. The three test types describe the location on the probability distribution curve: lower-tailed test, upper-tailed test, or two-tailed test .
In a nutshell, the greater the difference between two observed values, the less likely it is that the difference is due to simple random chance, and this is reflected by a lower p-value.
The P-Value Approach to Hypothesis Testing
The p-value approach to hypothesis testing uses the calculated probability to determine whether there is evidence to reject the null hypothesis. The null hypothesis, also known as the conjecture, is the initial claim about a population (or data-generating process). The alternative hypothesis states whether the population parameter differs from the value of the population parameter stated in the conjecture.
In practice, the significance level is stated in advance to determine how small the p-value must be to reject the null hypothesis. Because different researchers use different levels of significance when examining a question, a reader may sometimes have difficulty comparing results from two different tests. P-values provide a solution to this problem.
Even a low p-value is not necessarily proof of statistical significance, since there is still a possibility that the observed data are the result of chance. Only repeated experiments or studies can confirm if a relationship is statistically significant.
For example, suppose a study comparing returns from two particular assets was undertaken by different researchers who used the same data but different significance levels. The researchers might come to opposite conclusions regarding whether the assets differ.
If one researcher used a confidence level of 90% and the other required a confidence level of 95% to reject the null hypothesis, and if the p-value of the observed difference between the two returns was 0.08 (corresponding to a confidence level of 92%), then the first researcher would find that the two assets have a difference that is statistically significant , while the second would find no statistically significant difference between the returns.
To avoid this problem, the researchers could report the p-value of the hypothesis test and allow readers to interpret the statistical significance themselves. This is called a p-value approach to hypothesis testing. Independent observers could note the p-value and decide for themselves whether that represents a statistically significant difference or not.
Example of P-Value
An investor claims that their investment portfolio’s performance is equivalent to that of the Standard & Poor’s (S&P) 500 Index . To determine this, the investor conducts a two-tailed test.
The null hypothesis states that the portfolio’s returns are equivalent to the S&P 500’s returns over a specified period, while the alternative hypothesis states that the portfolio’s returns and the S&P 500’s returns are not equivalent—if the investor conducted a one-tailed test , the alternative hypothesis would state that the portfolio’s returns are either less than or greater than the S&P 500’s returns.
The p-value hypothesis test does not necessarily make use of a preselected confidence level at which the investor should reset the null hypothesis that the returns are equivalent. Instead, it provides a measure of how much evidence there is to reject the null hypothesis. The smaller the p-value, the greater the evidence against the null hypothesis.
Thus, if the investor finds that the p-value is 0.001, there is strong evidence against the null hypothesis, and the investor can confidently conclude that the portfolio’s returns and the S&P 500’s returns are not equivalent.
Although this does not provide an exact threshold as to when the investor should accept or reject the null hypothesis, it does have another very practical advantage. P-value hypothesis testing offers a direct way to compare the relative confidence that the investor can have when choosing among multiple different types of investments or portfolios relative to a benchmark such as the S&P 500.
For example, for two portfolios, A and B, whose performance differs from the S&P 500 with p-values of 0.10 and 0.01, respectively, the investor can be much more confident that portfolio B, with a lower p-value, will actually show consistently different results.

Is a 0.05 P-Value Significant?
A p-value less than 0.05 is typically considered to be statistically significant, in which case the null hypothesis should be rejected. A p-value greater than 0.05 means that deviation from the null hypothesis is not statistically significant, and the null hypothesis is not rejected.
What Does a P-Value of 0.001 Mean?
A p-value of 0.001 indicates that if the null hypothesis tested were indeed true, then there would be a one-in-1,000 chance of observing results at least as extreme. This leads the observer to reject the null hypothesis because either a highly rare data result has been observed or the null hypothesis is incorrect.
How Can You Use P-Value to Compare 2 Different Results of a Hypothesis Test?
If you have two different results, one with a p-value of 0.04 and one with a p-value of 0.06, the result with a p-value of 0.04 will be considered more statistically significant than the p-value of 0.06. Beyond this simplified example, you could compare a 0.04 p-value to a 0.001 p-value. Both are statistically significant, but the 0.001 example provides an even stronger case against the null hypothesis than the 0.04.
The p-value is used to measure the significance of observational data. When researchers identify an apparent relationship between two variables, there is always a possibility that this correlation might be a coincidence. A p-value calculation helps determine if the observed relationship could arise as a result of chance.
U.S. Census Bureau. “ Statistical Quality Standard E1: Analyzing Data .”
:max_bytes(150000):strip_icc():format(webp)/investing7-5bfc2b8d46e0fb0051bddff8.jpg)
- Terms of Service
- Editorial Policy
- Privacy Policy
- Your Privacy Choices
Log in using your username and password
- Search More Search for this keyword Advanced search
- Latest content
- Current issue
- Write for Us
- BMJ Journals More You are viewing from: Google Indexer
You are here
- Volume 15, Issue 2
- What is a p value and what does it mean?
- Article Text
- Article info
- Citation Tools
- Rapid Responses
- Article metrics

- Dorothy Anne Forbes
- Correspondence to Dorothy Anne Forbes Faculty of Nursing, University of Alberta, Level 3, Edmonton Clinic Health Academy, Edmonton, Alberta, T6G 1C9, Canada; dorothy.forbes{at}ualberta.ca
https://doi.org/10.1136/ebnurs-2012-100524
Statistics from Altmetric.com
Request permissions.
If you wish to reuse any or all of this article please use the link below which will take you to the Copyright Clearance Center’s RightsLink service. You will be able to get a quick price and instant permission to reuse the content in many different ways.
Researchers aim to make the strongest possible conclusions from limited amounts of data. To do this, they need to overcome two problems. First, important differences in the findings can be obscured by natural variability and experimental imprecision. Thus, it is difficult to distinguish real differences from random variability. Second, researchers' natural inclination is to conclude that differences are real, and to minimise the contribution of random variability. Statistical probability minimises this from happening. 1
Statistical probability or p values reveal whether the findings in a research study are statistically significant, meaning that the findings are unlikely to have occurred by chance. To understand the p value concept, it is important to understand its relationship with the α level. Before conducting a study, researchers specify the α level which is most often set at 0.05 (5%). This conventional level was based on the writings of Sir Ronald Fisher, an influential statistician, who in 1926 reported that he preferred the 0.05 cut-off for separating the probable from the improbable. 2 Researchers who set α at 0.05 are willing to accept that there is a 5% chance that their findings are wrong. However, researchers may adopt probability cut-offs that are more generous (eg, an α set at 0.10 means there is a 10% chance that the conclusions are wrong) or more stringent (eg, an α set at 0.01 means there is a 1% chance that the conclusions are wrong). The design of the study, purpose or intuition may influence the researcher's setting of the α level. 2
To illustrate how setting the α level may affect the conclusions of a study, let us examine a research study that compared the annual incomes of hospital based nurses and community based nurses. The mean annual income for hospital based nurses was reported to be $70 000 and for community based nurses to be $60 000. The p value of this study was 0.08. If the researchers set the α level at 0.05, they would conclude that there was no significant difference between the annual incomes of hospital and community-based nurses, since the p value of 0.08 exceeded the α level of 0.05. However, if the α level had been set at 0.10, the p value of 0.08 would be less than the α level and the researchers would conclude that there was a significant difference between the annual incomes of hospital and community based nurses. Two very different conclusions. 3
It is easy to read far too much into the word significant because the statistical use of the word has a meaning entirely distinct from its usual meaning. Just because a difference is statistically significant does not mean that it is important or interesting. In the example above, at the 0.10 α level, although the findings are statistically significant, results due to chance occur 1 out of 10 times. Thus, chance of conclusion error is higher than when the α level is set at 0.05 and results due to chance occur 5 out of 100 times or 1 in 20 times. In the end, the reader must decide if the researchers selected the appropriate α level and whether the conclusions are meaningful or not.
- ↵ Graphpad . What is a p value ? 2011 . http://www.graphpad.com/articles/pvalue.htm (accessed 10 Dec 2011) .
- Munroe BH ,
- Jacobsen BS
- El-Masri MM
Competing interests None.
Read the full text or download the PDF:

Statistics Made Easy
An Explanation of P-Values and Statistical Significance
In statistics, p-values are commonly used in hypothesis testing for t-tests, chi-square tests, regression analysis, ANOVAs, and a variety of other statistical methods.
Despite being so common, people often interpret p-values incorrectly, which can lead to errors when interpreting the findings from an analysis or a study.
This post explains how to understand and interpret p-values in a clear, practical way.
Hypothesis Testing
To understand p-values, we first need to understand the concept of hypothesis testing .
A hypothesis test is a formal statistical test we use to reject or fail to reject some hypothesis. For example, we may hypothesize that a new drug, method, or procedure provides some benefit over a current drug, method, or procedure.
To test this, we can conduct a hypothesis test where we use a null and alternative hypothesis:
Null hypothesis – There is no effect or difference between the new method and the old method.
Alternative hypothesis – There does exist some effect or difference between the new method and the old method.
A p-value indicates how believable the null hypothesis is, given the sample data. Specifically, assuming the null hypothesis is true, the p-value tells us the probability of obtaining an effect at least as large as the one we actually observed in the sample data.
If the p-value of a hypothesis test is sufficiently low, we can reject the null hypothesis. Specifically, when we conduct a hypothesis test, we must choose a significance level at the outset. Common choices for significance levels are 0.01, 0.05, and 0.10.
If the p-values is less than our significance level, then we can reject the null hypothesis.
Otherwise, if the p-value is equal to or greater than our significance level, then we fail to reject the null hypothesis.
How to Interpret a P-Value
The textbook definition of a p-value is:
A p-value is the probability of observing a sample statistic that is at least as extreme as your sample statistic, given that the null hypothesis is true.
For example, suppose a factory claims that they produce tires that have a mean weight of 200 pounds. An auditor hypothesizes that the true mean weight of tires produced at this factory is different from 200 pounds so he runs a hypothesis test and finds that the p-value of the test is 0.04. Here is how to interpret this p-value:
If the factory does indeed produce tires that have a mean weight of 200 pounds, then 4% of all audits will obtain the effect observed in the sample, or larger, because of random sample error. This tells us that obtaining the sample data that the auditor did would be pretty rare if indeed the factory produced tires that have a mean weight of 200 pounds.
Depending on the significance level used in this hypothesis test, the auditor would likely reject the null hypothesis that the true mean weight of tires produced at this factory is indeed 200 pounds. The sample data that he obtained from the audit is not very consistent with the null hypothesis.
How Not to Interpret a P-Value
The biggest misconception about p-values is that they are equivalent to the probability of making a mistake by rejecting a true null hypothesis (known as a Type I error).
There are two primary reasons that p-values can’t be the error rate:
1. P-values are calculated based on the assumption that the null hypothesis is true and that the difference between the sample data and the null hypothesis is simple caused by random chance. Thus, p-values can’t tell you the probability that the null is true or false since it is 100% true based on the perspective of the calculations.
2. Although a low p-value indicates that your sample data are unlikely assuming the null is true, a p-value still can’t tell you which of the following cases is more likely:
- The null is false
- The null is true but you obtained an odd sample
In regards to the previous example, here is a correct and incorrect way to interpret the p-value:
- Correct Interpretation: Assuming the factory does produce tires with a mean weight of 200 pounds, you would obtain the observed difference that you did obtain in your sample or a more extreme difference in 4% of audits due to random sampling error.
- Incorrect Interpretation: If you reject the null hypothesis, there is a 4% chance that you are making a mistake.
Examples of Interpreting P-Values
The following examples illustrate correct ways to interpret p-values in the context of hypothesis testing.
A phone company claims that 90% of its customers are satisfied with their service. To test this claim, an independent researcher gathered a simple random sample of 200 customers and asked them if they are satisfied with their service, to which 85% responded yes. The p-value associated with this sample data turned out to be 0.018.
Correct interpretation of p-value: Assuming that 90% of the customers actually are satisfied with their service, the researcher would obtain the observed difference that he did obtain in his sample or a more extreme difference in 1.8% of audits due to random sampling error.
A company invents a new battery for phones. The company claims that this new battery will work for at least 10 minutes longer than the old battery. To test this claim, a researcher takes a simple random sample of 80 new batteries and 80 old batteries. The new batteries run for an average of 120 minutes with a standard deviation of 12 minutes and the old batteries run for an average of 115 minutes with a standard deviation of 15 minutes. The p-value that results from the test for a difference in population means is 0.011.
Correct interpretation of p-value: Assuming that the new battery works for the same amount of time or less than the old battery, the researcher would obtain the observed difference or a more extreme difference in 1.1% of studies due to random sampling error.

Published by Zach
Leave a reply cancel reply.
Your email address will not be published. Required fields are marked *
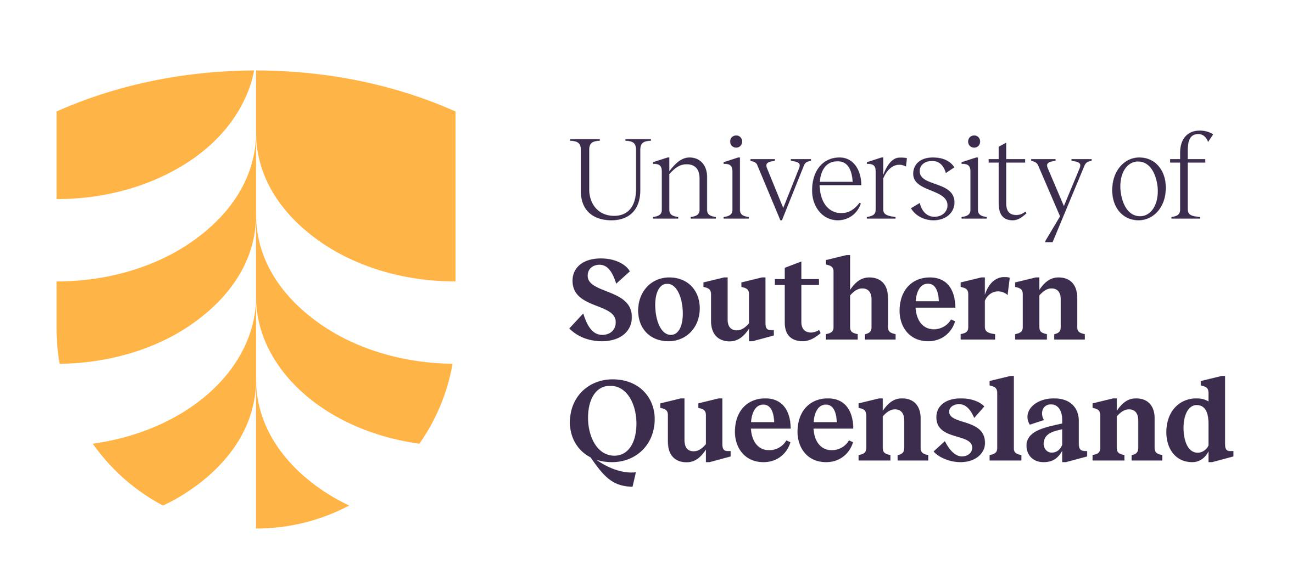
Want to create or adapt books like this? Learn more about how Pressbooks supports open publishing practices.
Section 2.1: p Values
Learning Objectives
At the end of this section you should be able to answer the following questions:
- What is a p value?
- How can you interpret a p value?
- What question can p value answer?
An important area of statistics is probability, and it is the basis for all of the tests we will be reviewing in this textbook. One important kind of probability is a conditional probability. For example, given the weather forecast for today, what is the likelihood that it will rain?
The p value itself is a figure or numeral – typically represented by a number between 0 and 1.00 – that provides the probability of a result (for a particular test statistic) being due to a true effect rather than chance. The p value is a conditional probability and relies on a number of assumptions about the test statistics used.
Here is an example from psychology that provides an illustration of the p value:
Psychological scientists at your university are evaluating a clinical therapy that is believed to reduce anxiety in young adults. In a field study, these scientists use two groups to test the therapy – one group receives the clinical therapy and a second group that does not receive the clinical therapy – which are respectively known as the experimental and control groups. Anxiety in participants is then measured in both groups after the therapy takes place (or not). Using a T-test statistic – which examines the different means for anxiety between two groups – the result of the test statistic is t(18)= 2.7, p = .01.
The p value is indicated by the statement of p = .01 that appears after the 2.7, which is the value of the T-test statistic. You interpret the significance of a p value based on a critical value for p values, which is often designated as .05. You also note that p values less than .05 are considered significant in most research. In our example p = .01 which is below .05. This means that the test statistic of t(18)= 2.7 provides evidence for a difference between the control and experimental groups.
When concluding there is a difference between the control and experimental groups, a researcher is really referring back to the populations from which the two groups are assumed to be drawn. Hence, there is an inference from the samples back to the populations.
It is critical to remember that a p value does NOT answer “What is the probability that the difference is due to chance?” A p value does answer: ‘Assuming that there is no real difference in the populations (that correspond to the two groups), what is the probability that the difference between the means of randomly selected subjects will be as large as or larger than actually observed?’ This distinction might sound academic, but it is very important.
Statistics for Research Students Copyright © 2022 by University of Southern Queensland is licensed under a Creative Commons Attribution 4.0 International License , except where otherwise noted.
Share This Book
- How it works
P-Value: A Complete Guide
Published by Owen Ingram at August 31st, 2021 , Revised On August 3, 2023
You might have come across this term many times in hypothesis testing . Can you tell me what p-value is and how to calculate it? For those who are new to this term, sit back and read this guide to find out all the answers. Those already familiar with it, continue reading because you might get a chance to dig deeper about the p-value and its significance in statistics .
Before we start with what a p-value is, there are a few other terms you must be clear of. And these are the null hypothesis and alternative hypothesis .
What are the Null Hypothesis and Alternative Hypothesis?
The alternative hypothesis is your first hypothesis predicting a relationship between different variables . On the contrary, the null hypothesis predicts that there is no relationship between the variables you are playing with.
For instance, if you want to check the impact of two fertilizers on the growth of two sets of plants. Group A of plants is given fertilizer A, while B is given fertilizer B. Now by using a two-tailed t-test , you can find out the difference between the two fertilizers.
Null Hypothesis : There is no difference in growth between the two sets of plants.
Alternative Hypothesis: There is a difference in growth between the two groups.
What is the P-value?
The p-value in statistics is the probability of getting outcomes at least as extreme as the outcomes of a statistical hypothesis test, assuming the null hypothesis to be correct. To put it in simpler words, it is a calculated number from a statistical test that shows how likely you are to have found a set of observations if the null hypothesis were plausible.
This means that p-values are used as alternatives to rejection points for providing the smallest level of significance at which the null hypothesis can be rejected . If the p-value is small, it implies that the evidence in favour of the alternative hypothesis is bigger. Similarly, if the value is big, the evidence in favour of the alternative hypothesis would be small.
How is the P-value Calculated?
You can either use the p-value tables or statistical software to calculate the p-value. The calculated numbers are based on the known probability distribution of the statistic being tested.
The online p-value tables depict how frequently you can expect to see test statistics under the null hypothesis. P-value depends on the statistical test one uses to test a hypothesis.
- Different statistical tests can have different predictions, hence developing different test statistics. Researchers can choose a statistical test depending on what best suits their data and the effect they want to test
- The number of independent variables in your test determines how large or small the test statistic must be to produce the same p-value
Get statistical analysis help at an affordable price
- An expert statistician will complete your work
- Rigorous quality checks
- Confidentiality and reliability
- Any statistical software of your choice
- Free Plagiarism Report

When is a P-value Statistically Significant?
Before we talk about when a p-value is statistically significant, let’s first find out what does it mean to be statistically significant.
Any guesses?
To be statistically significant is another way of saying that a p-value is so small that it might reject a null hypothesis.
Now the question is how small?
If a p-value is smaller than 0.05 then it is statistically significant. This means that the evidence against the null hypothesis is strong. The fact that there is less than a 5 per cent chance of the null hypothesis being correct and plausible, we can accept the alternative hypothesis and reject the null hypothesis.
Nevertheless , if the p-value is less than the threshold of significance , the null hypothesis can be rejected, but that does not mean there would be a 95 percent probability of the alternative hypothesis being true. Note that the p-value is dependent or conditioned upon the null hypothesis is plausible, but it is not related to the correctness and falsity of the alternative hypothesis.
When the p-value is greater than 0.05, it is not statistically significant. It also indicates that the evidence for the null hypothesis is strong. So, the alternative hypothesis, in this case, is rejected, and the null hypothesis is retained. An important thing to keep in mind here is that you still cannot accept the null hypothesis. You can only fail to reject it or reject it.
Here is a table showing hypothesis interpretations:
Is it clear now? We thought so! Let’s move on to the next heading, then.
How to Use P-value in Hypothesis Testing?
Follow these three simple steps to use p-value in hypothesis testing .
Step 1: Find the level of significance. Make sure to choose the significance level during the initial steps of the design of a hypothesis test. It is usually 0.10, 0.05, and 0.01.
Step 2: Now calculate the p-value. As we discussed earlier, there are two ways of calculating it. A simple way out would be using Microsoft Excel, which allows p-value calculation with Data Analysis ToolPak .
Step 3: Start comparing the p-value with the significance level and deduce conclusions accordingly. Following the general rule, if the value is less than the level of significance, there is enough evidence to reject the null hypothesis of an experiment.
FAQs About P-Value
What is a null hypothesis.
It is a statistical theory suggesting that there is no relationship between a set of variables .
What is an alternative hypothesis?
The alternative hypothesis is your first hypothesis predicting a relationship between different variables .
What is the p-value?
The p-value in statistics is the probability of getting outcomes at least as extreme as the outcomes of a statistical hypothesis test, assuming the null hypothesis to be correct. It is a calculated number from a statistical test that shows how likely you are to have found a set of observations if the null hypothesis were plausible.
What is the level of significance?
To be statistically significant is another way of saying that a p-value is so small that it might reject a null hypothesis. This table shows when the p-value is significant.
You May Also Like
One way ANOVA test is a kind of ANOVA that aims to find if there is a significant statistical difference among the means of two or more independent groups.
Statistical power is a decision by a researcher/statistician that results of a study/experiment can be explained by factors other than chance alone. The statistical power of a study is also referred to as its sensitivity in some cases.
Descriptive statistics is the summarising and organising of the characteristics of a dataset. Here is a definitive guide to descriptive statistics.
USEFUL LINKS
LEARNING RESOURCES
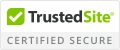
COMPANY DETAILS
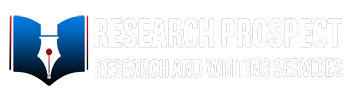
- How It Works
p-value Calculator
What is p-value, how do i calculate p-value from test statistic, how to interpret p-value, how to use the p-value calculator to find p-value from test statistic, how do i find p-value from z-score, how do i find p-value from t, p-value from chi-square score (χ² score), p-value from f-score.
Welcome to our p-value calculator! You will never again have to wonder how to find the p-value, as here you can determine the one-sided and two-sided p-values from test statistics, following all the most popular distributions: normal, t-Student, chi-squared, and Snedecor's F.
P-values appear all over science, yet many people find the concept a bit intimidating. Don't worry – in this article, we will explain not only what the p-value is but also how to interpret p-values correctly . Have you ever been curious about how to calculate the p-value by hand? We provide you with all the necessary formulae as well!
🙋 If you want to revise some basics from statistics, our normal distribution calculator is an excellent place to start.
Formally, the p-value is the probability that the test statistic will produce values at least as extreme as the value it produced for your sample . It is crucial to remember that this probability is calculated under the assumption that the null hypothesis H 0 is true !
More intuitively, p-value answers the question:
Assuming that I live in a world where the null hypothesis holds, how probable is it that, for another sample, the test I'm performing will generate a value at least as extreme as the one I observed for the sample I already have?
It is the alternative hypothesis that determines what "extreme" actually means , so the p-value depends on the alternative hypothesis that you state: left-tailed, right-tailed, or two-tailed. In the formulas below, S stands for a test statistic, x for the value it produced for a given sample, and Pr(event | H 0 ) is the probability of an event, calculated under the assumption that H 0 is true:
Left-tailed test: p-value = Pr(S ≤ x | H 0 )
Right-tailed test: p-value = Pr(S ≥ x | H 0 )
Two-tailed test:
p-value = 2 × min{Pr(S ≤ x | H 0 ), Pr(S ≥ x | H 0 )}
(By min{a,b} , we denote the smaller number out of a and b .)
If the distribution of the test statistic under H 0 is symmetric about 0 , then: p-value = 2 × Pr(S ≥ |x| | H 0 )
or, equivalently: p-value = 2 × Pr(S ≤ -|x| | H 0 )
As a picture is worth a thousand words, let us illustrate these definitions. Here, we use the fact that the probability can be neatly depicted as the area under the density curve for a given distribution. We give two sets of pictures: one for a symmetric distribution and the other for a skewed (non-symmetric) distribution.
- Symmetric case: normal distribution:
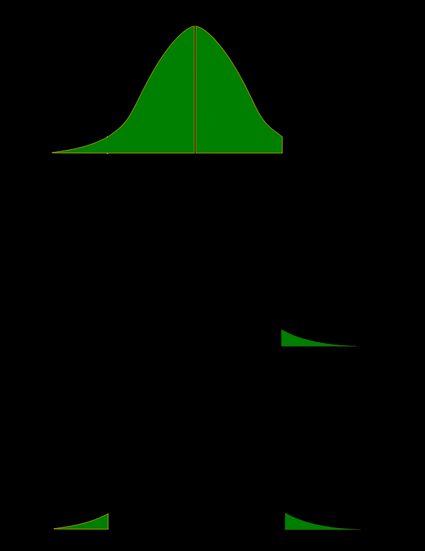
- Non-symmetric case: chi-squared distribution:
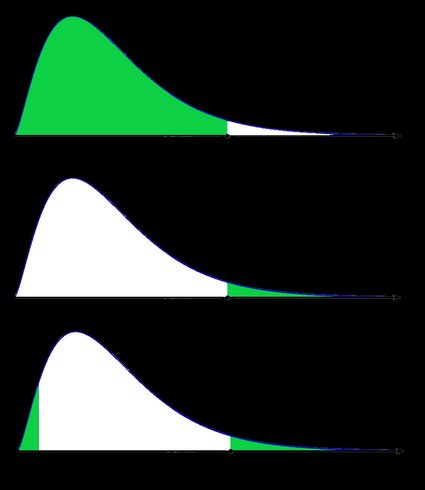
In the last picture (two-tailed p-value for skewed distribution), the area of the left-hand side is equal to the area of the right-hand side.
To determine the p-value, you need to know the distribution of your test statistic under the assumption that the null hypothesis is true . Then, with the help of the cumulative distribution function ( cdf ) of this distribution, we can express the probability of the test statistics being at least as extreme as its value x for the sample:
Left-tailed test:
p-value = cdf(x) .
Right-tailed test:
p-value = 1 - cdf(x) .
p-value = 2 × min{cdf(x) , 1 - cdf(x)} .
If the distribution of the test statistic under H 0 is symmetric about 0 , then a two-sided p-value can be simplified to p-value = 2 × cdf(-|x|) , or, equivalently, as p-value = 2 - 2 × cdf(|x|) .
The probability distributions that are most widespread in hypothesis testing tend to have complicated cdf formulae, and finding the p-value by hand may not be possible. You'll likely need to resort to a computer or to a statistical table, where people have gathered approximate cdf values.
Well, you now know how to calculate the p-value, but… why do you need to calculate this number in the first place? In hypothesis testing, the p-value approach is an alternative to the critical value approach . Recall that the latter requires researchers to pre-set the significance level, α, which is the probability of rejecting the null hypothesis when it is true (so of type I error ). Once you have your p-value, you just need to compare it with any given α to quickly decide whether or not to reject the null hypothesis at that significance level, α. For details, check the next section, where we explain how to interpret p-values.
As we have mentioned above, the p-value is the answer to the following question:
What does that mean for you? Well, you've got two options:
- A high p-value means that your data is highly compatible with the null hypothesis; and
- A small p-value provides evidence against the null hypothesis , as it means that your result would be very improbable if the null hypothesis were true.
However, it may happen that the null hypothesis is true, but your sample is highly unusual! For example, imagine we studied the effect of a new drug and got a p-value of 0.03 . This means that in 3% of similar studies, random chance alone would still be able to produce the value of the test statistic that we obtained, or a value even more extreme, even if the drug had no effect at all!
The question "what is p-value" can also be answered as follows: p-value is the smallest level of significance at which the null hypothesis would be rejected. So, if you now want to make a decision on the null hypothesis at some significance level α , just compare your p-value with α :
- If p-value ≤ α , then you reject the null hypothesis and accept the alternative hypothesis; and
- If p-value ≥ α , then you don't have enough evidence to reject the null hypothesis.
Obviously, the fate of the null hypothesis depends on α . For instance, if the p-value was 0.03 , we would reject the null hypothesis at a significance level of 0.05 , but not at a level of 0.01 . That's why the significance level should be stated in advance and not adapted conveniently after the p-value has been established! A significance level of 0.05 is the most common value, but there's nothing magical about it. Here, you can see what too strong a faith in the 0.05 threshold can lead to. It's always best to report the p-value, and allow the reader to make their own conclusions.
Also, bear in mind that subject area expertise (and common reason) is crucial. Otherwise, mindlessly applying statistical principles, you can easily arrive at statistically significant, despite the conclusion being 100% untrue.
As our p-value calculator is here at your service, you no longer need to wonder how to find p-value from all those complicated test statistics! Here are the steps you need to follow:
Pick the alternative hypothesis : two-tailed, right-tailed, or left-tailed.
Tell us the distribution of your test statistic under the null hypothesis: is it N(0,1), t-Student, chi-squared, or Snedecor's F? If you are unsure, check the sections below, as they are devoted to these distributions.
If needed, specify the degrees of freedom of the test statistic's distribution.
Enter the value of test statistic computed for your data sample.
Our calculator determines the p-value from the test statistic and provides the decision to be made about the null hypothesis. The standard significance level is 0.05 by default.
Go to the advanced mode if you need to increase the precision with which the calculations are performed or change the significance level .
In terms of the cumulative distribution function (cdf) of the standard normal distribution, which is traditionally denoted by Φ , the p-value is given by the following formulae:
Left-tailed z-test:
p-value = Φ(Z score )
Right-tailed z-test:
p-value = 1 - Φ(Z score )
Two-tailed z-test:
p-value = 2 × Φ(−|Z score |)
p-value = 2 - 2 × Φ(|Z score |)
🙋 To learn more about Z-tests, head to Omni's Z-test calculator .
We use the Z-score if the test statistic approximately follows the standard normal distribution N(0,1) . Thanks to the central limit theorem, you can count on the approximation if you have a large sample (say at least 50 data points) and treat your distribution as normal.
A Z-test most often refers to testing the population mean , or the difference between two population means, in particular between two proportions. You can also find Z-tests in maximum likelihood estimations.
The p-value from the t-score is given by the following formulae, in which cdf t,d stands for the cumulative distribution function of the t-Student distribution with d degrees of freedom:
Left-tailed t-test:
p-value = cdf t,d (t score )
Right-tailed t-test:
p-value = 1 - cdf t,d (t score )
Two-tailed t-test:
p-value = 2 × cdf t,d (−|t score |)
p-value = 2 - 2 × cdf t,d (|t score |)
Use the t-score option if your test statistic follows the t-Student distribution . This distribution has a shape similar to N(0,1) (bell-shaped and symmetric) but has heavier tails – the exact shape depends on the parameter called the degrees of freedom . If the number of degrees of freedom is large (>30), which generically happens for large samples, the t-Student distribution is practically indistinguishable from the normal distribution N(0,1).
The most common t-tests are those for population means with an unknown population standard deviation, or for the difference between means of two populations , with either equal or unequal yet unknown population standard deviations. There's also a t-test for paired (dependent) samples .
🙋 To get more insights into t-statistics, we recommend using our t-test calculator .
Use the χ²-score option when performing a test in which the test statistic follows the χ²-distribution .
This distribution arises if, for example, you take the sum of squared variables, each following the normal distribution N(0,1). Remember to check the number of degrees of freedom of the χ²-distribution of your test statistic!
How to find the p-value from chi-square-score ? You can do it with the help of the following formulae, in which cdf χ²,d denotes the cumulative distribution function of the χ²-distribution with d degrees of freedom:
Left-tailed χ²-test:
p-value = cdf χ²,d (χ² score )
Right-tailed χ²-test:
p-value = 1 - cdf χ²,d (χ² score )
Remember that χ²-tests for goodness-of-fit and independence are right-tailed tests! (see below)
Two-tailed χ²-test:
p-value = 2 × min{cdf χ²,d (χ² score ), 1 - cdf χ²,d (χ² score )}
(By min{a,b} , we denote the smaller of the numbers a and b .)
The most popular tests which lead to a χ²-score are the following:
Testing whether the variance of normally distributed data has some pre-determined value. In this case, the test statistic has the χ²-distribution with n - 1 degrees of freedom, where n is the sample size. This can be a one-tailed or two-tailed test .
Goodness-of-fit test checks whether the empirical (sample) distribution agrees with some expected probability distribution. In this case, the test statistic follows the χ²-distribution with k - 1 degrees of freedom, where k is the number of classes into which the sample is divided. This is a right-tailed test .
Independence test is used to determine if there is a statistically significant relationship between two variables. In this case, its test statistic is based on the contingency table and follows the χ²-distribution with (r - 1)(c - 1) degrees of freedom, where r is the number of rows, and c is the number of columns in this contingency table. This also is a right-tailed test .
Finally, the F-score option should be used when you perform a test in which the test statistic follows the F-distribution , also known as the Fisher–Snedecor distribution. The exact shape of an F-distribution depends on two degrees of freedom .
To see where those degrees of freedom come from, consider the independent random variables X and Y , which both follow the χ²-distributions with d 1 and d 2 degrees of freedom, respectively. In that case, the ratio (X/d 1 )/(Y/d 2 ) follows the F-distribution, with (d 1 , d 2 ) -degrees of freedom. For this reason, the two parameters d 1 and d 2 are also called the numerator and denominator degrees of freedom .
The p-value from F-score is given by the following formulae, where we let cdf F,d1,d2 denote the cumulative distribution function of the F-distribution, with (d 1 , d 2 ) -degrees of freedom:
Left-tailed F-test:
p-value = cdf F,d1,d2 (F score )
Right-tailed F-test:
p-value = 1 - cdf F,d1,d2 (F score )
Two-tailed F-test:
p-value = 2 × min{cdf F,d1,d2 (F score ), 1 - cdf F,d1,d2 (F score )}
Below we list the most important tests that produce F-scores. All of them are right-tailed tests .
A test for the equality of variances in two normally distributed populations . Its test statistic follows the F-distribution with (n - 1, m - 1) -degrees of freedom, where n and m are the respective sample sizes.
ANOVA is used to test the equality of means in three or more groups that come from normally distributed populations with equal variances. We arrive at the F-distribution with (k - 1, n - k) -degrees of freedom, where k is the number of groups, and n is the total sample size (in all groups together).
A test for overall significance of regression analysis . The test statistic has an F-distribution with (k - 1, n - k) -degrees of freedom, where n is the sample size, and k is the number of variables (including the intercept).
With the presence of the linear relationship having been established in your data sample with the above test, you can calculate the coefficient of determination, R 2 , which indicates the strength of this relationship . You can do it by hand or use our coefficient of determination calculator .
A test to compare two nested regression models . The test statistic follows the F-distribution with (k 2 - k 1 , n - k 2 ) -degrees of freedom, where k 1 and k 2 are the numbers of variables in the smaller and bigger models, respectively, and n is the sample size.
You may notice that the F-test of an overall significance is a particular form of the F-test for comparing two nested models: it tests whether our model does significantly better than the model with no predictors (i.e., the intercept-only model).
Can p-value be negative?
No, the p-value cannot be negative. This is because probabilities cannot be negative, and the p-value is the probability of the test statistic satisfying certain conditions.
What does a high p-value mean?
A high p-value means that under the null hypothesis, there's a high probability that for another sample, the test statistic will generate a value at least as extreme as the one observed in the sample you already have. A high p-value doesn't allow you to reject the null hypothesis.
What does a low p-value mean?
A low p-value means that under the null hypothesis, there's little probability that for another sample, the test statistic will generate a value at least as extreme as the one observed for the sample you already have. A low p-value is evidence in favor of the alternative hypothesis – it allows you to reject the null hypothesis.
Implied probability
Plastic footprint, probability.
- Biology (100)
- Chemistry (100)
- Construction (144)
- Conversion (295)
- Ecology (30)
- Everyday life (262)
- Finance (570)
- Health (440)
- Physics (510)
- Sports (104)
- Statistics (182)
- Other (182)
- Discover Omni (40)
- Open access
- Published: 24 June 2020
P-values – a chronic conundrum
- Jian Gao ORCID: orcid.org/0000-0001-8101-740X 1
BMC Medical Research Methodology volume 20 , Article number: 167 ( 2020 ) Cite this article
21k Accesses
19 Citations
27 Altmetric
Metrics details
In medical research and practice, the p -value is arguably the most often used statistic and yet it is widely misconstrued as the probability of the type I error, which comes with serious consequences. This misunderstanding can greatly affect the reproducibility in research, treatment selection in medical practice, and model specification in empirical analyses. By using plain language and concrete examples, this paper is intended to elucidate the p -value confusion from its root, to explicate the difference between significance and hypothesis testing, to illuminate the consequences of the confusion, and to present a viable alternative to the conventional p -value.
The confusion with p -values has plagued the research community and medical practitioners for decades. However, efforts to clarify it have been largely futile, in part, because intuitive yet mathematically rigorous educational materials are scarce. Additionally, the lack of a practical alternative to the p -value for guarding against randomness also plays a role. The p -value confusion is rooted in the misconception of significance and hypothesis testing. Most, including many statisticians, are unaware that p -values and significance testing formed by Fisher are incomparable to the hypothesis testing paradigm created by Neyman and Pearson. And most otherwise great statistics textbooks tend to cobble the two paradigms together and make no effort to elucidate the subtle but fundamental differences between them. The p -value is a practical tool gauging the “strength of evidence” against the null hypothesis. It informs investigators that a p -value of 0.001, for example, is stronger than 0.05. However, p -values produced in significance testing are not the probabilities of type I errors as commonly misconceived. For a p -value of 0.05, the chance a treatment does not work is not 5%; rather, it is at least 28.9%.
Conclusions
A long-overdue effort to understand p -values correctly is much needed. However, in medical research and practice, just banning significance testing and accepting uncertainty are not enough. Researchers, clinicians, and patients alike need to know the probability a treatment will or will not work. Thus, the calibrated p -values (the probability that a treatment does not work) should be reported in research papers.
Peer Review reports
Without any exaggeration, humankind’s wellbeing is profoundly affected by p -values: Health depends on prevention and intervention, ascertaining their efficacies relies on research, and research findings hinge on p -values. The p-value is a sine qua non for deciding if a research finding is real or by chance, a treatment is effective or even harmful, a paper will be accepted or rejected, a grant will be funded or declined, or if a drug will be approved or denied by U.S. Food & Drug Administration (FDA).
Yet, the misconception of p -values is pervasive and virtually universal. “The P value is probably the most ubiquitous and at the same time, misunderstood, misinterpreted, and occasionally miscalculated index in all of biomedical research [ 1 ].” Even “among statisticians there is a near ubiquitous misinterpretation of p values as frequentist error probabilities [ 2 ].”
The extent of the p -value confusion is well illustrated by a survey of medical residents published in the Journal of the American Medical Association ( JAMA) . In the article, 88% of the residents expressed fair to complete confidence in understanding p -values, but 100% of them had the p-value interpretation wrong [ 1 , 3 ]. Make no mistake, they are the future experts and leaders in clinical research that will affect public health policies, treatment options, and ultimately people’s health.
The survey published in JAMA used multiple-choice format with four potential answers for a correct interpretation of p > 0.05 [ 3 ]:
The chances are greater than 1 in 20 that a difference would be found again if the study were repeated.
The probability is less than 1 in 20 that a difference this large could occur by chance alone.
The probability is greater than 1 in 20 that a difference this large could occur by chance alone.
The chance is 95% that the study is correct.
How could it be possible that 100% of the residents selected incorrect answers when one of the possible choices was supposed to be correct? As reported in the paper [ 3 ], 58.8% of the residents selected choice c which was designated by the authors as the correct answer. The irony is that choice c is not correct either. In fact, none of the four choices are correct. So, not only were the residents who picked choice c wrong but also the authors as well. Keep in mind, the paper was peer-reviewed and published by one of the most prestigious medical journals in the world.
This is no coincidence -- most otherwise great statistics textbooks make no effort or fail to clarify the massive confusion about p -values, and even provide outright wrong interpretations. The confusion is near-universal among medical researchers and clinicians [ 4 , 5 , 6 ].
Unfortunately, the misunderstanding of p -values is not inconsequential. For a p-value of 0.05, the chance a treatment doesn’t work is not 5%; rather, it is at least 28.9% [ 7 ].
After decades of misunderstanding and inaction, the pendulum of p -values finally started to swing in 2014 when the American Statistical Association (ASA) was taunted by two pairs of questions and answers on its discussion forum:
Q: Why do so many colleges and grad schools teach p = 0.05?
A: Because that’s still what the scientific community and journal editors use.
Q: Why do so many people still use p = 0.05?
A: Because that’s what they were taught in college or grad school.
The questions and answers, posted by George Cobb, Professor Emeritus of Mathematics & Statistics from Mount Holyoke College, spurred the ASA Board into action. In 2015, for the first time, the ASA board decided to take on the challenge of developing a policy statement on p -values, much like the American Heart Association (AHA) policy statement on dietary fat and heart disease. After months of preparation, in October 2015, a group of 20 experts gathered at the ASA Office in Alexandria, Virginia and laid out the roadmap during a two-day meeting. Over the next three months, multiple drafts of the p -value statement were produced. On January 29, 2016, the ASA Executive Committee approved the p -value statement with six principles listed on what p -values are or are not [ 8 ].
Although the statement hardly made any ripples in medical journals, it grabbed many statisticians’ attention and ignited a rebellion against p -values among some scientists. In March 2019, Nature published a comment with over 800 signatories calling for an end of significance testing with p < 0.05 [ 9 ]. At the same time, the American Statistician that carried the ASA’s p -value statement published a special issue with 43 articles exploring ways to report results without statistical significance testing. Unfortunately, no consensus was reached for a better alternative in gauging the reliability of studies, and the authors even disagreed on whether the p -value should continue to be used or abandoned. The only agreement reached was the abolishment of significance testing as summarized in the special issue’s editorial: “statistically significant” – don’t say it and don’t use it [ 10 ].
So, for researchers, practitioners, and journals in the medical field, what will replace significance testing? And what is significance testing anyway? Is it different from hypothesis testing? Should p -values be banned too? If not, how should p-values be used and interpreted? In healthcare or medicine, we must accept uncertainty as the editorial of the special issue urged, but do we need to know how likely a given treatment will work or not?
To answer these questions, we must get to the bottom of the misconception and confusion, and we must identify a practical alternative(s). However, despite numerous publications on this topic, few studies aimed for these goals are understandable to non-statisticians and retain mathematical rigor at the same time. This paper is intended to fill this gap by using plain language and concrete examples to elucidate the p -value confusion from its root, to intuitively describe the true meaning of p -values, to illuminate the consequences of the confusion, and to present a viable alternative to the conventional p -value.
The root of confusion
The p-value confusion began 100 years ago when the father of modern statistics, Ronald Aylmer Fisher, formed the paradigm of significance testing. But it should be noted Fisher bears no blame for the misconception; it is the users who tend to muddle Fisher’s significance testing with hypothesis testing developed by Jerzy Neyman and Egon Pearson. To clarify the confusion, this section uses concrete examples and plain language to illustrate the essence of significance and hypothesis testing and to explicate the difference between the p -value and the type I error.
- Significance testing
Suppose a painkiller has a proven track record of lasting for 24 h and now another drug manufacturer claims its new over-the-counter painkiller lasts longer. An investigator wants to test if the claim is true. Instead of collecting data from all the patients who took the new medication, which is often infeasible, the investigator decided to randomly survey 50 patients to gather data on how long (hours) the new painkiller lasts. Thus, the investigator now has a random variable \( \overline{X} \) , the average hours from a sample of 50 patients. This is a random variable because the 50 patients are randomly selected, and nobody knows what value this variable will take before conducting the survey and calculating the average. Nevertheless, each survey does produce a fixed number, \( \overline{x} \) , which itself is not a random variable, rather it is a realization or observation of the random variable \( \overline{X} \) (hereafter, let \( \overline{X} \) denote a random variable and \( \overline{x} \) denote a fixed value, an observation of \( \overline{X} \) ).
Intuitively, if the survey yielded a value (average hours the painkiller lasts) very close to 24, say, 23 or 25, the investigator would not believe the new painkiller is worse or better. If the survey came to an average of 32 h the investigator would believe it indeed lasts longer. However, it would be hard to form an opinion if the survey showed an average of 22 or 26 h. Does the new painkiller really last shorter, longer, or it is due to random chance (after all, only 50 patients were surveyed)?
This is where the significance test formulated by Fisher in the 1920s comes in. Note that although modern significance testing began with the Student’s t -test in 1908, it was Fisher who extended the test to the testing of two samples, regression coefficients, as well as analysis of variance, and created the paradigm of significance testing.
In Fisher’s significance testing, the Central Limit Theorem (CLT) plays a vital role, which states that given a population with a mean of μ and a variance of σ 2 , regardless of the shape of its distribution, the sample mean statistic \( \overline{X} \) has a normal distribution with the same mean μ and variance σ 2 /n, or \( \frac{\left(\overline{X}-\upmu \right)}{\sigma /\sqrt{n}} \) has a standard normal distribution with a mean of 0 and a variance of 1, as long as the sample size n is large enough. In practice, the distribution of the study population is often unknown and n ≥ 30 is considered sufficient for the sample mean statistic to have an approximately normal distribution.
In conducting the significance test, a null hypothesis is first formed, i.e., there is no difference between the new and old painkillers, or the new painkiller also lasts for 24 h (the mean of \( \overline{X} \) = μ =24). Under this assumption and based on CLT, \( \overline{X} \) is normally distributed with a mean of 24 and a variance of σ 2 /50. Assume σ 2 = 200 (typically σ 2 is unknown but can be estimated), then \( \overline{X} \) has a normal distribution N (24, 2) , or \( Z=\left(\overline{X}-24\right)/2 \) has a standard normal distribution with a mean of 0 and standard deviation of 1 (Z is a standardized random variable). The next step is to calculate z = \( \mid \overline{x}-24\mid /2 \) based on the survey data and then find the p -value or the probability of |Z| > z from a normal distribution table (z is a fixed value or an observation of Z). Fisher suggested if the p -value is smaller than 0.05 then the hypothesis is rejected. He argued that the farther the sample mean \( \overline{x} \) from the population mean μ, the smaller the p -value, the less likely the null hypothesis is true. Just as Fisher stated, “Either an exceptionally rare chance has occurred or the theory [H 0 ] is not true [ 11 ].”
Based on this paradigm, if the survey came back with an average of 26 h, i.e., \( \overline{x}=26, \) then z = 1 and p = 0.3173, as a result, the investigator accepts the null hypothesis (orthodoxically, fails to reject the null hypothesis), i.e., the new painkiller does not last longer and the difference between 24 and 26 h is due to chance or random factors. On the other hand, if the survey revealed an average of 28 h, i.e., \( \overline{x}=28, \) then z = 2, and p = 0.0455, thus the null hypothesis is rejected. In other words, the new painkiller is deemed lasting longer.
Now, can the p -value, 0.0455, be interpreted as the probability of the type I error, or only 4.55% chance the new painkiller does not last longer (no difference), or the probability that the difference between 24 and 28 h is due to chance, or the investigator could make a mistake by rejecting the null hypothesis but only wrong about 4.55% of the time? The answer is No.
So, what is a p -value? Precisely, a p-value tells us how often we would see a difference as extreme as or more extreme than what is observed if there really were no difference . Drawing a bell curve with the p -value on it will readily delineate this definition or concept.
In the example above, if the new painkiller also lasts for 24 h, the p-value of 0.0455 means there is a 4.55% chance that the investigator would observe \( \overline{x}\le 20 \) or \( \overline{x}\ge 28 \) ; it is not 4.55% chance the new painkiller also lasts for 24 h. It is categorically wrong to believe the p -value is the probability of the null hypothesis being true (there is no difference), or 1 – p is the probability of the null hypothesis being false (there is a difference) because the p -value is deduced based on the premise that the null hypothesis is true. The p-value, a conditional probability given H 0 is true, is totally invalidated if the null hypothesis is deemed not true.
In addition, p -values are data-dependent: each test (survey) produces a different p-value; for the same analysis, it is illogical to say the error rate or the type I error is 31.73% based on one sample (survey) and 4.55% based on another. There is no theoretical or empirical basis for such frequency interpretations. In fact, Fisher himself was fully aware that his p -value, a relative measure of evidence against the null hypothesis, does not bear any interpretation of the long-term error rate. When the p -value was misinterpreted, he protested the p-value was not the type I error rate, had no long-run frequentist characteristics, and should not be explained as a frequency of error if the test was repeated [ 12 ].
Interestingly, Fisher was an abstract thinker at the highest level, but often developed solutions and tests without solid theoretical foundation. He was an obstinate proponent of inductive inference, i.e., reasoning from specific to general, or from sample to population, which is reflected by his significance testing.
- Hypothesis testing
On the contrary, mathematicians Jerzy Neyman and Egon Pearson dismissed the idea of inductive inference all together and insisted reasoning should be deductive, i.e., from general to specific. In 1928, they published the landmark paper on the theoretical foundation for a statistical inference method that they called “hypothesis test [ 12 ].” In the paper, they introduced the concepts of alternative hypothesis H 1, type I and type II errors, which were groundbreaking. The Neyman and Pearson’s hypothesis test is deductive in nature, i.e., reasoning from general to particular. The type I and type II errors, which must be set ahead, formulate a “rule of behavior” such that “in the long run of experience, we shall not be too often wrong,” as stated by Neyman and Pearson [ 13 ].
The hypothesis test can be illustrated by a four-step process with the painkiller example.
The first step is to lay out what the investigator seeks to test, i.e. to establish a null hypothesis, H 0, and an alternative hypothesis, H 1 :
The second step is to set the criteria for the decision, or to specify an acceptable rate of mistake if the test is conducted many times. Specifically, that is to set the probability of the type I error, α, and the probability of the type II error, β.
A type I error refers to the mistake of rejecting the null hypothesis when it is true (claiming the treatment works or the new drug lasts longer but actually it does not). Conventionally and almost universally, the probability of the type I error or α is set to 0.05, which means 5% of the time one will be wrong if carrying out the test many times. A type II error is the failure to reject the null hypothesis that is not true; the probability of the type II error, β, is conventionally set as 0.2, which is equivalent to a power of 0.8, the probability of detecting the difference if it exists. Table 1 summarizes the type I and type II errors.
The third step is to select a statistic and the associated distribution for the test. For the painkiller example, the statistic is Z = ( \( \overline{X}-24 \) )/2, and the distribution is the standard normal. Because the type I error has been set to 0.05 and Z has a standard normal distribution under the null hypothesis, as shown in Fig. 1 , 1.96 becomes the critical value, − 1.96 ≤ z ≤ 1.96 becomes the acceptance region, and z < − 1.96 or z > 1.96 becomes the rejection regions.
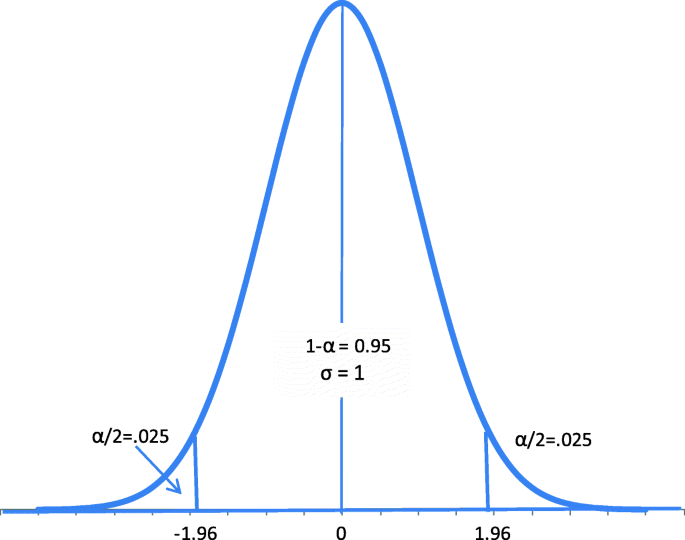
Standard Normal Distribution with Critical Value 1.96
The final step is to calculate the z value and make a decision. Similar to significance testing, if the survey resulted in \( \overline{x}=26, \) then z = 1 < 1.96 and the investigator accepts the null hypothesis; if the survey revealed \( \overline{x}=28, \) then z = 2 > 1.96 and the investigator rejects the null hypothesis and accepts the alternative hypothesis. It is interesting to note, in significance testing, “one can never accept the null hypothesis, only failed to reject it,” while that is not the case in hypothesis testing.
Unlike Fisher’s significance test, the hypothesis test possesses a nice frequency explanation: one can be wrong by rejecting the null hypothesis but cannot be wrong more than 5% of the time in the long run if the test is performed many times. Quite intuitively, every time the null hypothesis is rejected (when z < − 1.96 or z > 1. 96) there is a chance that the null hypothesis is true, and a mistake is made. When the null hypothesis is true, Z = ( \( \overline{X}-24 \) )/2 is a random variable with a standard normal distribution as shown in Fig. 1 , thus 5% of the time z = ( \( \overline{x}-24 \) )/2 would fall outside (− 1.96, 1.96) and the decision will be wrong 5% of the time. Of course, when the null hypothesis is not true, rejecting it is not a mistake.
Noticeably, the p -value plays no role in hypothesis testing under the framework of the Neyman-Pearson paradigm [ 12 , 14 ]. However, most, including many statisticians, are unaware that p -values and significance testing created by Fisher are incomparable to the hypothesis testing paradigm created by Neyman and Pearson [ 14 , 15 ], and many statistics textbooks tend to cobble them together [ 2 , 14 ]. The near-universal confusion is, at least in part, caused by the subtle similarities and differences between the two tests:
Both the significance and hypothesis tests use the same statistic and distribution, for example, Z = ( \( \overline{X}-24 \) )/2 and N (0, 1).
The hypothesis test compares the observed z with the critical value 1.96, while the significance test compares the p -value (based on z) to 0.05, which are linked by P (| Z | > 1.96) = 0.05.
The hypothesis test sets the type I error α at 0.05, while the significance test also uses 0.05 as the significance level.
One of the key differences is, for the p -value to be meaningful in significance testing, the null hypothesis must be true, while this is not the case for the critical value in hypothesis testing. Although the critical value is derived from α based on the null hypothesis, rejecting the null hypothesis is not a mistake when it is not true; when it is true, there is a 5% chance that z = ( \( \overline{x}-24 \) )/2 will fall outside (− 1.96, 1.96), and the investigator will be wrong 5% of the time (bear in mind, the null hypothesis is either true or false when a decision is made). In addition, the type I error and the resultant critical value is set ahead and fixed, while the p -value is a moving “target” varying from sample to sample even for the same test.
As if it was not confusing enough, the understanding and interpretation of p -values are also complicated by non-experimental studies where model misspecifications and even p-hacking are common, which often misleads the audience to believe the model and the findings are valid for its small p -values [ 16 ]. In fact, p-values have little value in assessing if the relationship between an outcome and exposure(s) is causal or just an artifact of confounding – one cannot claim the use of smartphones causes gun violence even if the p -value for their correlation is close to zero. To see the p-value problem at its core and to elucidate the confusion, the discussion of p-values should be in the context of experimental designs such as randomized controlled trials where the model or the outcome and exposure(s) are correctly specified.
The Link between P -values and Type I Errors
The p-value fallacy can be readily quantified under a Bayesian framework [ 7 , 17 , 18 ]. However, “those ominous words [Bayes theorem], with their associations of hazy prior probabilities and abstruse mathematical formulas, strike fear into the hearts of most us, clinician, researcher, and editor alike [ 19 ],” as Frank Davidoff, former Editor of the Annals of Internal Medicine , wrote. It is understandable but still unfortunate that Bayesian methods such as Bayes factors, despite their merit, are still considered exotic by the medical research community.
Thanks to Sellke, Bayarri, and Berger, the difference between the p -value and the type I error is quantified [ 7 ]. Based on the conditional frequentist approach, which was formalized by Kiefer and further developed by others [ 20 , 21 , 22 , 23 ], Berger and colleagues established the lower bound of the error rate P(H 0 │| Z| >z 0 ) or the type I error given the p-value [ 7 ]:
As shown, the lower bound equation is mathematically straightforward. Noteworthy is that the derivation of the lower bound is also ingeniously elegant (a simplified proof is provided in the Supplementary File for those who are interested in it). The relationship between p -values and type I errors (lower bound) can be readily seen from Table 2 showing some of the commonly reported results [ 7 ].
As seen in Table 2 , the difference between p -values and the error probabilities (lower bound) is quite striking. A p-value of 0.05, commonly misinterpreted as only 5% chance the treatment does not work, seems to offer strong evidence against the null hypothesis; however, the true probability of the treatment not working is at least 0.289. Keep in mind, the relationship between the p-value and the type I error is the lower bound; in fact, many prefer to report the upper bound [ 6 , 7 ].
The discrepancy between the p-value and the lower-bound error rate explains the big puzzle of why so many wonder drugs and treatments worldwide lose their amazing power outside clinical trials [ 24 , 25 , 26 ]. This discrepancy likely also contributes to the frequently reported contradictory findings on risk factors and health outcomes in observational studies. For example, an early study published in the New England Journal of Medicine found drinking coffee was associated with a high risk of pancreatic cancer [ 27 ]. The finding became a big headline in The New York Times [ 28 ] and the leading author and probably many frightened readers stopped drinking coffee. Later studies, however, concluded the finding was a fluke [ 29 , 30 ]. Likewise, the p -value fallacy may also contribute to the ongoing confusion of dietary fat intake and heart disease. On the one hand, a meta-analysis published in Annals of Internal Medicine in 2014 concluded “Current evidence does not clearly support cardiovascular guidelines that encourage high consumption of polyunsaturated fatty acids and low consumption of total saturated fats [ 31 ].” On the other hand, in the 2017 recommendation, the American Heart Association (AHA) stated “Taking into consideration the totality of the scientific evidence, satisfying rigorous criteria for causality, we conclude strongly that lowering intake of saturated fat and replacing it with unsaturated fats, especially polyunsaturated fats, will lower the incidence of CVD [ 32 ].”
In short, the misunderstanding and misinterpretation of the relationship between the p -value and the type I error all too often exaggerate the true effects of treatments and risk factors, which in turn leads to conflicting findings with real public health consequences.
The future of P -values
It is readily apparent that the p-value conundrum poses a serious challenge to researchers and practitioners alike in healthcare with real-life consequences. To address the p-value complications, some believe the use of p -values should be banned or discouraged [ 33 , 34 ]. In fact, since 2015, Basic and Applied Social Psychology has officially banned significance tests and p-values [ 35 ], and Epidemiology has a longstanding policy discouraging the use of significance testing and p -values [ 36 , 37 ]. On the other hand, many are against a total ban [ 38 , 39 ]. P -values do possess practical utility -- they offer insight into what is observed and are the first line of defense against being fooled by randomness. You would be more suspicious of a coin being fair if nine heads turned up after ten flips versus, for example, if seven heads did. Similarly, you would like to see how strong the evidence is against the null hypothesis: say, a p-value of 0.0499 or 0.0001.
“It is hopeless to expect users to change their reliance on p -values unless they are offered an alternative way of judging the reliability of their conclusions [ 40 ].” Rather than banning the use of p-values, many believe the conventional significance level of 0.05 should be lowered for better research reproducibility [ 41 ]. In 2018, 72 statisticians and scientists made the case for changing p < 0.05 to p < 0.005 [ 42 ]. Inevitably, like most medical treatments, the proposed change is accompanied by some side effects: For instance, to achieve the same power of 80%, α = 0.005 requires a 70% larger sample size compared to α = 0.05, which could lead to fewer studies due to limited resources [ 43 ].
Other alternatives (e.g., second-generation p -values [ 44 ], and analysis of credibility [ 45 ]) have been proposed in the special issue of the American Statistician ; however, no consensus was reached. As a result, instead of recommending a ban of p -values, the accompanying editorial of the special issue called for an end of statistical significance testing [ 46 ]: “‘statistically significant’ – don’t say it and don’t use it [ 10 ].”
Will researchers and medical journals heed the “mandate” banning significance testing? It does not seem to be likely, at least thus far. Even if they do, it is no more than just a quibble – a significance test is done as long as the p -value is produced or reported – anyone seeing the result would know the p-value is greater or less than 0.05; the only difference is “Don’t ask, don’t tell.”
In any event, it is the right call to end dichotomizing the p-value and using it as the sole criterion to judge the results [ 47 ]. There is no practical difference between p = 0.049 and p = 0.051, and “God loves the .06 nearly as much as the .05 [ 48 ].” Furthermore, not all the results with a p -value close to 0.05 are valueless. Doctors and patients need to put p -values into context when making treatment choices, which can be well illustrated by a hypothetical but not unrealistic example. Suppose a study finds a new procedure (a kind of spine surgery) is effective in relieving debilitating neck and back pain with a p -value of 0.05, but when the procedure fails, it cripples the patient. If the patient believes there is only a 5% chance the procedure does not work or fails, he or she would probably take the chance. However, after learning the actual chance of failure is nearly 30% or higher based on the calibrated p-value, one would probably think twice. On the other hand, even if the p-value is 0.1 and the real chance of failure is nearly 40% or higher, if it does not cause serious side effects when the procedure fails, one would probably like to give it a try.
Taken together, in medicine or healthcare, the use of p -values needs more context (the balance of harms and benefits) than thresholds. However, banning significance testing and accepting uncertainty as called for by the editorial of the special issue are not enough [ 10 ]. When making treatment decisions, what researchers, practitioners, and patients alike need to know is the probability that a treatment does or does not work (the type I error). In this regard, the calibrated p -value, compared to other proposals [ 44 , 45 ], offers several advantages: (1) It provides a lower-bound, (2) it is fully frequentist although it can have a Bayesian interpretation, (3) it is easy to understand, and (4) it is easy to implement. Of course, other recommendations for improving the use of p -values may work well under different circumstances such as improving research reproducibility [ 49 , 50 ].
In medical research and practice, the p-value produced from significance testing has been widely misconstrued as the probability of the type I error, or the probability a treatment does not work. This misunderstanding comes with serious consequences: poor research reproducibility and inflated medical treatment effects. For a p -value of 0.05, the type I error or the chance a treatment does not work is not 5%; rather, it is at least 28.9%. Nevertheless, banning significance testing and accepting uncertainty, albeit well justified in many circumstances, offer little to apprise clinicians and patients of the probability a treatment will or will not work. In this respect, the calibrated p-value, a link between the p-value and the type I error, is practical and instructive.
In short, a long-overdue effort to understand p -values correctly is urgently needed and better education on statistical reasoning including Bayesian methods is desired [ 15 ]. Meanwhile, a rational action that medical journals can take is to require authors to report both conventional p-values and calibrated ones in research papers.
Availability of data and materials
Not applicable.
Abbreviations
U.S. Food & Drug Administration
Journal of the American Medical Association
American Statistical Association
Central Limit Theorem
American Heart Association
Goodman S. A dirty dozen: twelve p-value misconceptions. Semin Hematol. 2008;45(3):135–40.
Article PubMed Google Scholar
Hubbard R, Bayarri MR. Confusion over measures of evidence (p's) versus errors (α's) in classical statistical testing. Am Stat. 2003;57(3):171–82.
Article Google Scholar
Windish DM, Huot SJ, Green ML. Medicine residents’ understanding of the biostatistics and results in the medical literature. JAMA. 2007;298:1010–22.
Article CAS PubMed Google Scholar
Berger JO, Sellke T. Testing a point null hypothesis: the irreconcilability of p-values and evidence (with discussions). J Am Stat Assoc. 1987;82(397):112–39.
Google Scholar
Schervish MJ. P values: what they are and what they are not. Am Stat. 1996;50(3):203–6.
Berger JO. Could Fisher, Jeffreys and Neyman have agreed on testing? Stat Sci. 2003;18:1–32.
Sellke T, Bayarri MJ, Berger JO. Calibration of p value for testing precise null hypothesis. Am Stat. 2001;55(1):62–71.
Wassersteinm RL, Lazar NA. The ASA's statement on p-values: context, process, and purpose. Am Stat. 2016;70(2):129–33.
Amrhein V, Greenland S, McShane B. Scientists rise up against statistical significance. Nature. 2019;567(7748):305–7.
Wasserstein RL, Schirm AL, Lazar AN. Moving to a world beyond “p < 0.05”. Am Stat. 2019;73(S1):1–19.
Fisher RA. Statistical methods and scientific inference (2nd edition). Edinburgh: Oliver and Boyd; 1959.
Lehmann EL. Neyman's statistical philosophy. Probab Math Stat. 1995;15:29–36.
Neyman J, Pearson E. On the use and interpretation of certain test criteria for purpose of statistical inference. Biometrika. 1928;20:175–240.
Lehmann EL. The Fisher, Neyman-Pearson theories of testing hypotheses: one theory or two? J Am Stat Assoc. 1993;88(424):1242–9.
Gigerenzer G. Mindless statistics. J Socio-Econ. 2004;33:587–606.
Greenland S, Senn SJ, Rothman KJ, et al. Statistical tests, P values, confidence intervals, and power: a guide to misinterpretations. Eur J Epidemiol. 2016;31(4):337–50.
Article PubMed PubMed Central Google Scholar
Goodman SN. Toward evidence-based medical statistics. 1: the P value fallacy. Ann Intern Med. 1999;130(12):995–1004.
Goodman SN. Toward evidence-based medical statistics. 2: the Bayes factor. Ann Intern Med. 1999;130(12):1005–13.
Davidoff F. Standing statistics right up. Ann Intern Med. 1999;130(12):1019–21.
Kiefer J. Admissibility off conditional confidence procedures. Ann Math Statist. 1976;4:836–65.
Kiefer J. Conditional confidence statements and confidence estimators (with discussion). J Am Stat Assoc. 1977;72:789–827.
Berger JO, Brown LD, Wolper RL. A unified conditional frequentist and Bayesian test for fixed and sequential simple hypothesis testing. Ann Stat. 1994;22:1787–807.
Berger JO, Boukai B, Wang Y. Unified frequentist and Bayesian testing of a precise hypothesis (with discussion). Stat Sci. 1997;12:133–60.
Matthews R. The great health hoax. The Sunday Telegraph; 1998. Archived: http://junksciencearchive.com/sep98/matthews.html . Accessed 6 June 2020.
Ioannidis JP. Contradicted and initially stronger effects in highly cited clinical research. JAMA. 2005;294(2):218–28.
Ioannidis JP. Why most discovered true associations are inflated. Epidemiology. 2008;19(5):640–8.
MacMahon B, Yen S, Trichopoulos D, Warren K, Nardi G. Coffee and cancer of the pancreas. N Engl J Med. 1981;304(11):630–3.
http://www.nytimes.com/1981/03/12/us/study-links-coffee-use-to-pancreas-cancer.html . Accessed 2 May 2020.
Hsieh CC, MacMahon B, Yen S, Trichopoulos D, Warren K, Nardi G. Coffee and pancreatic cancer (chapter 2). N Engl J Med. 1986;315(9):587–9.
CAS PubMed Google Scholar
Turati F, Galeone C, Edefonti V. A meta-analysis of coffee consumption and pancreatic cancer. Ann Oncol. 2012;23(2):311–8.
Chowdhury R, Warnakula S, Kunutsor S, et al. Association of dietary, circulating, and supplement fatty acids with coronary risk: a systematic review and meta-analysis. Ann Intern Med. 2014;160:398–406.
Sacks FM, Lichtenstein AH, Wu JHY, et al. Dietary fats and cardiovascular disease: a presidential advisory from the American Heart Association. Circulation. 2017;136(3):e1–e23.
Goodman SN. Why is getting rid of p-values so hard? Musings on science and statistics. Am Stat. 2019;73(S1):26–30.
Tong C. Statistical inference enables bad science; statistical thinking enables good science. Am Stat. 2019;73(S1):246–26.
Trafimow D, Marks M. Editorial. Basic Appl Soc Psychol. 2015;37:1–2.
Lang JM, Rothman KJ, Cann CI. That confounded P-value. Epidemiology. 1998;9(1):7–8.
http://journals.lww.com/epidem/Pages/informationforauthors.aspx . Accessed 2 May 2020.
Kruege JI, Heck PR. Putting the p-value in its place. Am Stat. 2019;73(S1):122–8.
Greenland S. Valid p-values behave exactly as they should: some misleading criticisms of p-values and their resolution with s-values. Am Stat. 2019;73(S1):106–14.
Colquhoun D. The false positive risk: a proposal concerning what to do about p-values. Am Stat. 2019;73(S1):192–201.
Johnson VE. Revised standards for statistical evidence. Proc Natl Acad Sci U S A. 2013;110(48):19313–7.
Article CAS PubMed PubMed Central Google Scholar
Benjamin DJ, Berger JO, Johannesson M, et al. Redefine statistical significance. Nat Hum Behav. 2018;2:6–10.
Lakens D, Adolfi FG, Albers CJ, et al. Justify your alpha. Nat Hum Behav. 2018;2:168–71.
Blume JD, Greevy RA, Welty VF, et al. An introduction to second-generation p-values. Am Stat. 2019;73(S1):157–67.
Matthews RAJ. Moving towards the post p < 0.05 era via the analysis of credibility. Am Stat. 2019;73(S1):202–12.
McShane BB, Gal D, Gelman A, et al. Abandon statistical significance. Am Stat. 2019;73(S1):235–45.
Liao JM, Stack CB, Goodman S. Annals understanding clinical research: interpreting results with large p values. Ann Intern Med. 2018;169(7):485–6.
Rosnow RL, Rosenthal R. Statistical procedures and the justification of knowledge in psychological science. Am Psychol. 1989;44:1276–84.
Benjamin D, Berger JO. Three recommendations for improving the use of p-values. Am Stat. 2019;73(S1):186–91.
Held L, Ott M. On p-values and Bayes factors. Annual Review of Statistics and Its Application. 2018;5:393–419.
Download references
Acknowledgements
This material is based upon work supported (or supported in part) by the Department of Veterans Affairs, Veterans Health Administration, Office of Research and Development. The author is indebted to Mr. Fred Malphurs, a retired senior healthcare executive, a visionary leader, who devoted his entire 38-year career to Veterans healthcare, for his unwavering support of research to improve healthcare efficiency and effectiveness. The author is also grateful to the Reviewers and Editorial Board members for their insightful and constructive comments and advice. The author would also like to thank Andrew Toporek and an anonymous reviewer for their helpful suggestions and assistance.
Author information
Authors and affiliations.
Department of Veterans Affairs, Office of Productivity, Efficiency and Staffing (OPES, RAPID), Albany, USA
You can also search for this author in PubMed Google Scholar
Contributions
JG conceived/designed the study and wrote the manuscript. The author read and approved the final manuscript.
Author’s information
Director of Analytical Methodologies, Office of Productivity, Efficiency and Staffing, RAPID, U.S. Department of Veterans Affairs.
Corresponding author
Correspondence to Jian Gao .
Ethics declarations
Ethics approval and consent to participate, consent for publication, competing interests, additional information, publisher’s note.
Springer Nature remains neutral with regard to jurisdictional claims in published maps and institutional affiliations.
Supplementary information
Additional file 1., rights and permissions.
Open Access This article is licensed under a Creative Commons Attribution 4.0 International License, which permits use, sharing, adaptation, distribution and reproduction in any medium or format, as long as you give appropriate credit to the original author(s) and the source, provide a link to the Creative Commons licence, and indicate if changes were made. The images or other third party material in this article are included in the article's Creative Commons licence, unless indicated otherwise in a credit line to the material. If material is not included in the article's Creative Commons licence and your intended use is not permitted by statutory regulation or exceeds the permitted use, you will need to obtain permission directly from the copyright holder. To view a copy of this licence, visit http://creativecommons.org/licenses/by/4.0/ . The Creative Commons Public Domain Dedication waiver ( http://creativecommons.org/publicdomain/zero/1.0/ ) applies to the data made available in this article, unless otherwise stated in a credit line to the data.
Reprints and permissions
About this article
Cite this article.
Gao, J. P-values – a chronic conundrum. BMC Med Res Methodol 20 , 167 (2020). https://doi.org/10.1186/s12874-020-01051-6
Download citation
Received : 21 February 2020
Accepted : 12 June 2020
Published : 24 June 2020
DOI : https://doi.org/10.1186/s12874-020-01051-6
Share this article
Anyone you share the following link with will be able to read this content:
Sorry, a shareable link is not currently available for this article.
Provided by the Springer Nature SharedIt content-sharing initiative
- Type I error
- Research reproducibility
- Calibrated P -values
BMC Medical Research Methodology
ISSN: 1471-2288
- General enquiries: [email protected]
Thanks for visiting! GoodRx is not available outside of the United States. If you are trying to access this site from the United States and believe you have received this message in error, please reach out to [email protected] and let us know.

An official website of the United States government
The .gov means it’s official. Federal government websites often end in .gov or .mil. Before sharing sensitive information, make sure you’re on a federal government site.
The site is secure. The https:// ensures that you are connecting to the official website and that any information you provide is encrypted and transmitted securely.
- Publications
- Account settings
Preview improvements coming to the PMC website in October 2024. Learn More or Try it out now .
- Advanced Search
- Journal List
- Clin Orthop Relat Res
- v.468(8); 2010 Aug

In Brief: The P Value: What Is It and What Does It Tell You?
Frederick dorey.
Department of Pediatrics, Children’s Hospital Los Angeles, 4650 Sunset Boulevard, Mailstop 54, Los Angeles, CA 90027 USA
In medical papers today there usually are several statements based on the result of hypothesis tests presented, along with the associated p values. For example, a recent article by van Raaij et al. [ 1 ] compared the use of laterally wedged insoles with valgus braces for reduction of pain or improving function in selected patients with osteoarthritis. One of the statements made in that randomized study was that “At 6 months, 71% of patients in the insole group complied with the treatment, which was greater (p = 0.015) than 45% for the brace group” [ 1 ].
How does this hypothesis test address the issue of compliance between these two approaches, what information is supplied by the associated p value, and how should it be interpreted?
The primary purpose of an hypothesis test is to decide if the results of a study, based on a small sample, provide enough evidence against the null hypothesis (denoted by H 0 ), so that it is reasonable to believe that in a larger target population, H 0 is false, thus accepting the associated alternative hypothesis (denoted by H 1 ) as being true. The null hypothesis for this situation states that there is no meaningful clinical difference between the two treatment approaches in terms of the percent compliance in the target population [ 1 ]; formally stated, the expected difference between the percent compliance in the two samples should be zero. The alternative hypothesis is that there is a meaningful difference in percent compliance between the two treatments in the target population. van Raaij et al. reported a large difference of 26% between the two treatments [ 1 ]. The hypothesis test is designed to help determine if a 26% difference is so large and the resulting p value of 0.015 so small that we should reject H 0 .
First and foremost, a p value is simply a probability. However, it is a conditional probability, in that its calculation is based on an assumption (condition) that H 0 is true. This is the most critical concept to keep in mind as it means that one cannot infer from the p value whether H 0 is true or false. More specifically, after we assume H 0 is true, the p value only gives us the probability that, simply owing to the chance selection of patients from the larger (target) population, the clinical experiment resulted in a difference in the samples, as large or larger, than the actual 26% observed [ 1 ]. If a resulting small p value suggests that chance was not responsible for the observed difference of 26% and the randomization of patients, as in this case [ 1 ], makes the presence of bias unlikely, then the most likely conclusion is that in the target population the treatments must produce different compliance results.
Thus a p value is simply a measure of the strength of evidence against H 0 . A study with a p = 0.531 has much less evidence against H 0 than a study with a p = 0.058. However, a study with a p = 0.058 provides similar evidence as a study with a p = 0.049 and a study with a p = 0.049 also has much less evidence than one with a p = 0.015. Although a very small p value does provide strong evidence that H 0 is not true, a very large p value, even as large as 0.88, does not provide real evidence that H 0 is true. For example, the alternative hypothesis might in fact still be true but owing to a small sample size, the study did not have enough power to detect that H 0 was likely to be false. This notion, referred to as the power of the test, will be discussed later.
Authors sometimes take a formal approach in evaluating the results of an hypothesis test. An artificial cut point is chosen, called the significance level, and the result is called statistically significant if the p value is less than the significance level leading to the rejection of the null hypothesis. Although 5% usually is taken as the significance level, there is no real scientific reason for choosing that versus any other small value. Always rejecting H 0 when p is less than 5% results in an incorrect rejection of the null hypothesis 5% of the time. However, as there is no real practical difference between a p value of 0.06 and 0.045 from a probability point of view, it is difficult to understand why this rigorous interpretation has become the standard today. In the study by van Raaij et al. [ 1 ], the result is statistically significant at the 5% level as p = 0.015. However, if a similar difference of 26% had been found in a study with only 24 patients with insoles and 22 patients with braces, the associated p value (chi square test) would have been 0.081, a result that would be called not statistically significant. That would not have meant that there was no difference between the two treatments, but only that, with the given small sample size there is not enough evidence to reject H 0 .
Myths and Misconceptions
There are several misconceptions associated with the interpretation of a p value. One of the most common ones is that the p value gives the probability that H 0 is true. As mentioned earlier, as the p value is calculated based on an assumption that H 0 is true it cannot provide information regarding whether H 0 is in fact true. This argument also shows that first, p cannot be the probability that the alternative hypothesis is true. Second, the p value is very dependent on the sample size. Third, it is not true that the p value is the probability that any observed difference is simply attributable to the chance selection of subjects from the target population. The p value is calculated based on an assumption that chance is the only reason for observing any difference. Thus it cannot provide evidence for the truth of that statement. The concept of a p value is not simple and any statements associated with it must be considered cautiously. A wealth of information and references concerning these and other misinterpretations of p values can be found on the WEB. Finally, it is important to reemphasize that if the result of an hypothesis test is that a difference was not statistically significant, it does not mean that there is no difference between the treatment groups in the target population.
The only question that the p value addresses is, does the experiment provide enough evidence to reasonably reject H 0 . The actual p value always should be indicated when presenting the results of a clinical study, as the p value as a probability, provides a continuous measure of the evidence against H 0 . In the study by van Raaij et al. [ 1 ], randomization of the patients, the observed difference of 26% between the treatments, and the very small p value of 0.015 suggest that rejection of the null hypothesis is reasonable. Finally, the question of just how much difference might exist between the treatments in the target population is not directly addressed by the p value. Although 26% is a reasonable estimate of that difference, a confidence interval is more appropriate to address that question.

IMAGES
VIDEO
COMMENTS
A p-value, or probability value, is a number describing how likely it is that your data would have occurred by random chance (i.e., that the null hypothesis is true). The level of statistical significance is often expressed as a p-value between 0 and 1. The smaller the p -value, the less likely the results occurred by random chance, and the ...
The p value is a number, calculated from a statistical test, that describes how likely you are to have found a particular set of observations if the null hypothesis were true. P values are used in hypothesis testing to help decide whether to reject the null hypothesis. The smaller the p value, the more likely you are to reject the null hypothesis.
P Values. P values are used in research to determine whether the sample estimate is significantly different from a hypothesized value. The p-value is the probability that the observed effect within the study would have occurred by chance if, in reality, there was no true effect. Conventionally, data yielding a p<0.05 or p<0.01 is considered ...
The calculation of a P value in research and especially the use of a threshold to declare the statistical significance of the P value have both been challenged in recent years. There are at least two important reasons for this challenge: research data contain much more meaning than is summarized in a P value and its statistical significance, and these two concepts are frequently misunderstood ...
What a p-value cannot tell us, ... Phillips M. Letter to the editor: editorial: threshold p values in orthopaedic research-we know the problem. What is the solution? Clin Orthop. 2019;477:1756-8.
The P value means the probability, for a given statistical model that, when the null hypothesis is true, the statistical summary would be equal to or more extreme than the actual observed results [ 2 ]. Therefore, P values only indicate how incompatible the data are with a specific statistical model (usually with a null-hypothesis).
Here is the technical definition of P values: P values are the probability of observing a sample statistic that is at least as extreme as your sample statistic when you assume that the null hypothesis is true. Let's go back to our hypothetical medication study. Suppose the hypothesis test generates a P value of 0.03.
In null-hypothesis significance testing, the -value is the probability of obtaining test results at least as extreme as the result actually observed, under the assumption that the null hypothesis is correct. A very small p-value means that such an extreme observed outcome would be very unlikely under the null hypothesis. Even though reporting p-values of statistical tests is common practice in ...
P-Value: The p-value is the level of marginal significance within a statistical hypothesis test representing the probability of the occurrence of a given event. The p-value is used as an ...
What is a p-value. The p-value, or probability value, is the probability that your results occurred randomly given that the null hypothesis is true. P-values are used in hypothesis testing to find evidence that differences in values or groups exist. P-values are determined through the calculation of the test statistic for the test you are using ...
Statistical probability or p values reveal whether the findings in a research study are statistically significant, meaning that the findings are unlikely to have occurred by chance. To understand the p value concept, it is important to understand its relationship with the α level. Before conducting a study, researchers specify the α level ...
The textbook definition of a p-value is: A p-value is the probability of observing a sample statistic that is at least as extreme as your sample statistic, given that the null hypothesis is true. For example, suppose a factory claims that they produce tires that have a mean weight of 200 pounds. An auditor hypothesizes that the true mean weight ...
The p value itself is a figure or numeral - typically represented by a number between 0 and 1.00 - that provides the probability of a result (for a particular test statistic) being due to a true effect rather than chance. The p value is a conditional probability and relies on a number of assumptions about the test statistics used.
The p-value is the probability of the observed data given that the null hypothesis is true, which is a probability that measures the consistency between the data and the hypothesis being tested if, and only if, the statistical model used to compute the p-value is correct ( 9 ). The smaller the p-value the greater the discrepancy: "If p is ...
The p-value in statistics is the probability of getting outcomes at least as extreme as the outcomes of a statistical hypothesis test, assuming the null hypothesis to be correct. To put it in simpler words, it is a calculated number from a statistical test that shows how likely you are to have found a set of observations if the null hypothesis ...
To determine the p-value, you need to know the distribution of your test statistic under the assumption that the null hypothesis is true.Then, with the help of the cumulative distribution function (cdf) of this distribution, we can express the probability of the test statistics being at least as extreme as its value x for the sample:Left-tailed test:
Without any exaggeration, humankind's wellbeing is profoundly affected by p-values: Health depends on prevention and intervention, ascertaining their efficacies relies on research, and research findings hinge on p-values.The p-value is a sine qua non for deciding if a research finding is real or by chance, a treatment is effective or even harmful, a paper will be accepted or rejected, a ...
The p-value is the probability of observing such an extreme value if the null hypothesis, the hypothesis that there is no difference between populations, is true. The lower the p-value, the stronger the evidence that the null hypothesis is false and that there is a real difference between the populations. Collecting a representative sample: At ...
Hypothesis tests are used to test the validity of a claim that is made about a population. This claim that's on trial, in essence, is called the null hypothesis (H 0).The alternative hypothesis (H a) is the one you would believe if the null hypothesis is concluded to be untrue.Learning how to find the p-value in statistics is a fundamental skill in testing, helping you weigh the evidence ...
A p-value, or statistical significance, does not measure the size of an effect or the importance of a result. By itself, a p-value does not provide a good measure of evidence regarding a model or hypothesis. There has been much reaction worldwide to the ASA's statement. I would like to elaborate on point #5, on the role of p-values and ...
The GoodRx Research team notes that a common misinterpretation is that a p-value is the probability that the null hypothesis is true. However, a p-value is actually a probability calculated based on the assumption that the null hypothesis is true. This difference is important because of concerns with reproducibility of trial results.
Successful publication of a research study usually requires a small p value, typically p < 0.05. Many clinicians believe that a p value represents the probability that the null hypothesis is true, so that a small p value means the null hypothesis must be false. In fact, the p value provides very weak evidence against the null hypothesis, and the probability that the null hypothesis is true is ...
The p -value is one of the most widely used statistical terms in decision making in biomedical research, which assists the investigators to conclude about the significance of a research consideration. Up today, most researchers base their decision on the value of the probability p. However, the term p -value is often miss- or over- interpreted ...
Stock market data coverage from CNN. View US markets, world markets, after hours trading, quotes, and other important stock market activity.
Conclusion. The only question that the p value addresses is, does the experiment provide enough evidence to reasonably reject H 0.The actual p value always should be indicated when presenting the results of a clinical study, as the p value as a probability, provides a continuous measure of the evidence against H 0.In the study by van Raaij et al. [], randomization of the patients, the observed ...