Have a language expert improve your writing
Run a free plagiarism check in 10 minutes, automatically generate references for free.
- Knowledge Base
- Methodology
- A Quick Guide to Experimental Design | 5 Steps & Examples

A Quick Guide to Experimental Design | 5 Steps & Examples
Published on 11 April 2022 by Rebecca Bevans . Revised on 5 December 2022.
Experiments are used to study causal relationships . You manipulate one or more independent variables and measure their effect on one or more dependent variables.
Experimental design means creating a set of procedures to systematically test a hypothesis . A good experimental design requires a strong understanding of the system you are studying.
There are five key steps in designing an experiment:
- Consider your variables and how they are related
- Write a specific, testable hypothesis
- Design experimental treatments to manipulate your independent variable
- Assign subjects to groups, either between-subjects or within-subjects
- Plan how you will measure your dependent variable
For valid conclusions, you also need to select a representative sample and control any extraneous variables that might influence your results. If if random assignment of participants to control and treatment groups is impossible, unethical, or highly difficult, consider an observational study instead.
Table of contents
Step 1: define your variables, step 2: write your hypothesis, step 3: design your experimental treatments, step 4: assign your subjects to treatment groups, step 5: measure your dependent variable, frequently asked questions about experimental design.
You should begin with a specific research question . We will work with two research question examples, one from health sciences and one from ecology:
To translate your research question into an experimental hypothesis, you need to define the main variables and make predictions about how they are related.
Start by simply listing the independent and dependent variables .
Then you need to think about possible extraneous and confounding variables and consider how you might control them in your experiment.
Finally, you can put these variables together into a diagram. Use arrows to show the possible relationships between variables and include signs to show the expected direction of the relationships.
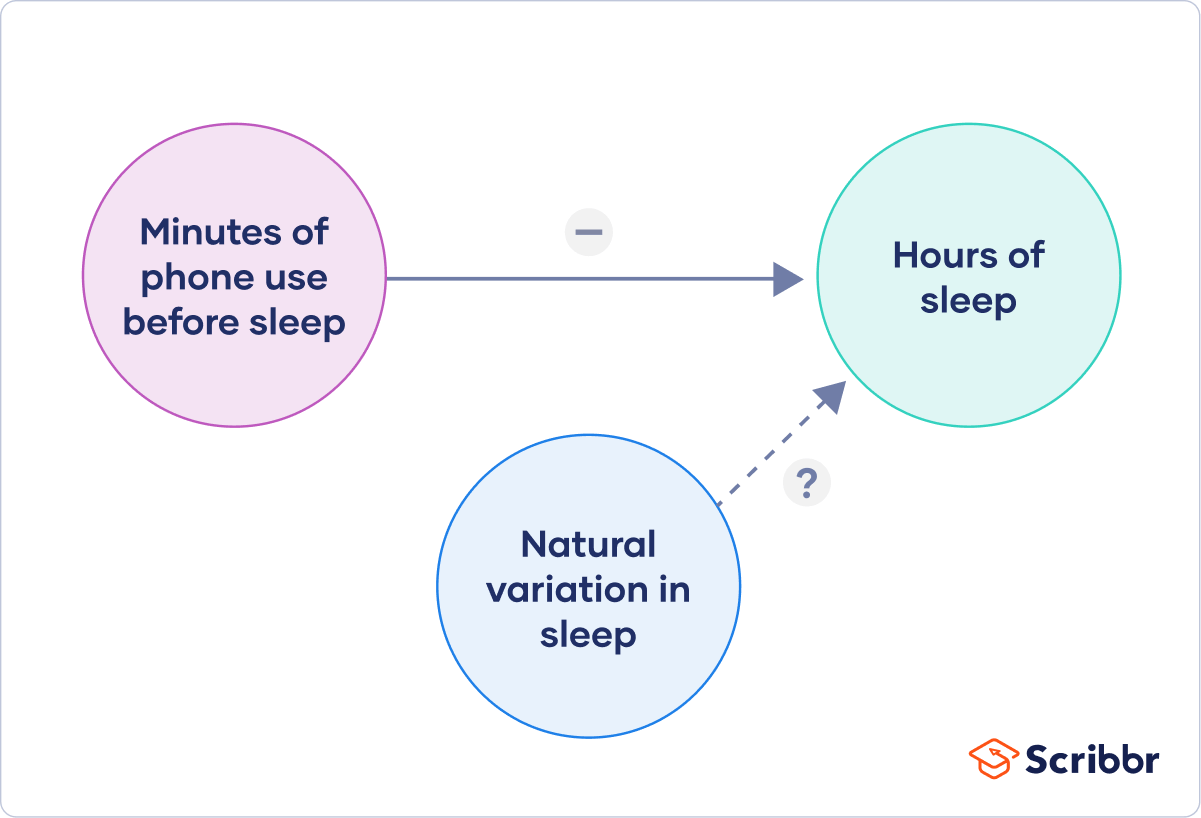
Here we predict that increasing temperature will increase soil respiration and decrease soil moisture, while decreasing soil moisture will lead to decreased soil respiration.
Prevent plagiarism, run a free check.
Now that you have a strong conceptual understanding of the system you are studying, you should be able to write a specific, testable hypothesis that addresses your research question.
The next steps will describe how to design a controlled experiment . In a controlled experiment, you must be able to:
- Systematically and precisely manipulate the independent variable(s).
- Precisely measure the dependent variable(s).
- Control any potential confounding variables.
If your study system doesn’t match these criteria, there are other types of research you can use to answer your research question.
How you manipulate the independent variable can affect the experiment’s external validity – that is, the extent to which the results can be generalised and applied to the broader world.
First, you may need to decide how widely to vary your independent variable.
- just slightly above the natural range for your study region.
- over a wider range of temperatures to mimic future warming.
- over an extreme range that is beyond any possible natural variation.
Second, you may need to choose how finely to vary your independent variable. Sometimes this choice is made for you by your experimental system, but often you will need to decide, and this will affect how much you can infer from your results.
- a categorical variable : either as binary (yes/no) or as levels of a factor (no phone use, low phone use, high phone use).
- a continuous variable (minutes of phone use measured every night).
How you apply your experimental treatments to your test subjects is crucial for obtaining valid and reliable results.
First, you need to consider the study size : how many individuals will be included in the experiment? In general, the more subjects you include, the greater your experiment’s statistical power , which determines how much confidence you can have in your results.
Then you need to randomly assign your subjects to treatment groups . Each group receives a different level of the treatment (e.g. no phone use, low phone use, high phone use).
You should also include a control group , which receives no treatment. The control group tells us what would have happened to your test subjects without any experimental intervention.
When assigning your subjects to groups, there are two main choices you need to make:
- A completely randomised design vs a randomised block design .
- A between-subjects design vs a within-subjects design .
Randomisation
An experiment can be completely randomised or randomised within blocks (aka strata):
- In a completely randomised design , every subject is assigned to a treatment group at random.
- In a randomised block design (aka stratified random design), subjects are first grouped according to a characteristic they share, and then randomly assigned to treatments within those groups.
Sometimes randomisation isn’t practical or ethical , so researchers create partially-random or even non-random designs. An experimental design where treatments aren’t randomly assigned is called a quasi-experimental design .
Between-subjects vs within-subjects
In a between-subjects design (also known as an independent measures design or classic ANOVA design), individuals receive only one of the possible levels of an experimental treatment.
In medical or social research, you might also use matched pairs within your between-subjects design to make sure that each treatment group contains the same variety of test subjects in the same proportions.
In a within-subjects design (also known as a repeated measures design), every individual receives each of the experimental treatments consecutively, and their responses to each treatment are measured.
Within-subjects or repeated measures can also refer to an experimental design where an effect emerges over time, and individual responses are measured over time in order to measure this effect as it emerges.
Counterbalancing (randomising or reversing the order of treatments among subjects) is often used in within-subjects designs to ensure that the order of treatment application doesn’t influence the results of the experiment.
Finally, you need to decide how you’ll collect data on your dependent variable outcomes. You should aim for reliable and valid measurements that minimise bias or error.
Some variables, like temperature, can be objectively measured with scientific instruments. Others may need to be operationalised to turn them into measurable observations.
- Ask participants to record what time they go to sleep and get up each day.
- Ask participants to wear a sleep tracker.
How precisely you measure your dependent variable also affects the kinds of statistical analysis you can use on your data.
Experiments are always context-dependent, and a good experimental design will take into account all of the unique considerations of your study system to produce information that is both valid and relevant to your research question.
Experimental designs are a set of procedures that you plan in order to examine the relationship between variables that interest you.
To design a successful experiment, first identify:
- A testable hypothesis
- One or more independent variables that you will manipulate
- One or more dependent variables that you will measure
When designing the experiment, first decide:
- How your variable(s) will be manipulated
- How you will control for any potential confounding or lurking variables
- How many subjects you will include
- How you will assign treatments to your subjects
The key difference between observational studies and experiments is that, done correctly, an observational study will never influence the responses or behaviours of participants. Experimental designs will have a treatment condition applied to at least a portion of participants.
A confounding variable , also called a confounder or confounding factor, is a third variable in a study examining a potential cause-and-effect relationship.
A confounding variable is related to both the supposed cause and the supposed effect of the study. It can be difficult to separate the true effect of the independent variable from the effect of the confounding variable.
In your research design , it’s important to identify potential confounding variables and plan how you will reduce their impact.
In a between-subjects design , every participant experiences only one condition, and researchers assess group differences between participants in various conditions.
In a within-subjects design , each participant experiences all conditions, and researchers test the same participants repeatedly for differences between conditions.
The word ‘between’ means that you’re comparing different conditions between groups, while the word ‘within’ means you’re comparing different conditions within the same group.
Cite this Scribbr article
If you want to cite this source, you can copy and paste the citation or click the ‘Cite this Scribbr article’ button to automatically add the citation to our free Reference Generator.
Bevans, R. (2022, December 05). A Quick Guide to Experimental Design | 5 Steps & Examples. Scribbr. Retrieved 6 May 2024, from https://www.scribbr.co.uk/research-methods/guide-to-experimental-design/
Is this article helpful?
Rebecca Bevans
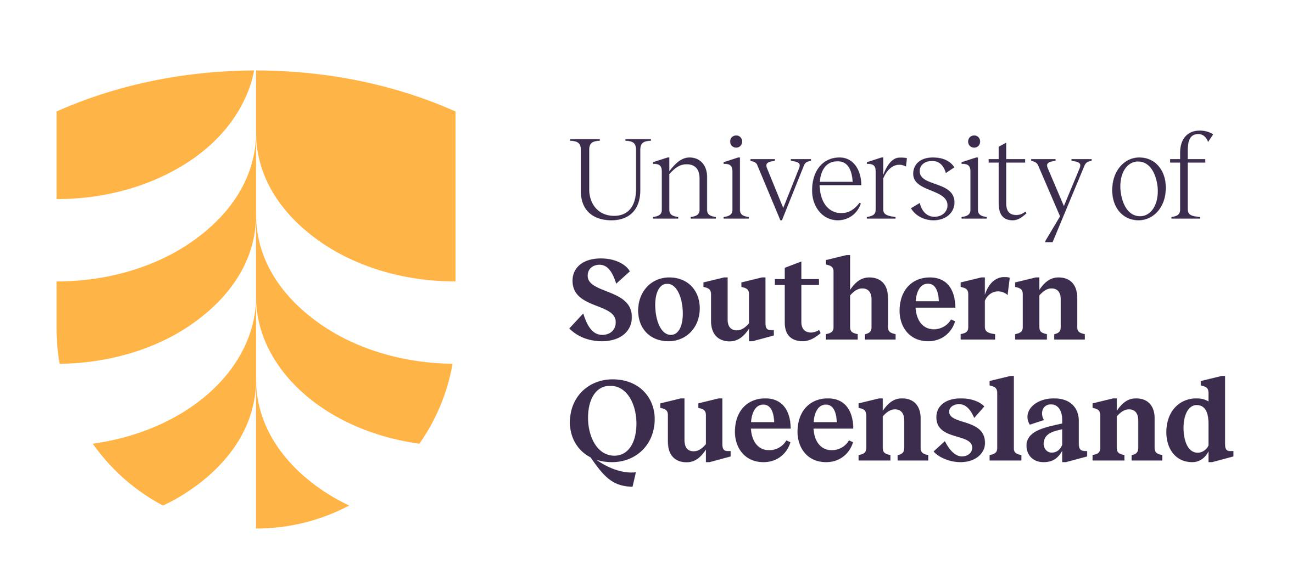
Want to create or adapt books like this? Learn more about how Pressbooks supports open publishing practices.
10 Experimental research
Experimental research—often considered to be the ‘gold standard’ in research designs—is one of the most rigorous of all research designs. In this design, one or more independent variables are manipulated by the researcher (as treatments), subjects are randomly assigned to different treatment levels (random assignment), and the results of the treatments on outcomes (dependent variables) are observed. The unique strength of experimental research is its internal validity (causality) due to its ability to link cause and effect through treatment manipulation, while controlling for the spurious effect of extraneous variable.
Experimental research is best suited for explanatory research—rather than for descriptive or exploratory research—where the goal of the study is to examine cause-effect relationships. It also works well for research that involves a relatively limited and well-defined set of independent variables that can either be manipulated or controlled. Experimental research can be conducted in laboratory or field settings. Laboratory experiments , conducted in laboratory (artificial) settings, tend to be high in internal validity, but this comes at the cost of low external validity (generalisability), because the artificial (laboratory) setting in which the study is conducted may not reflect the real world. Field experiments are conducted in field settings such as in a real organisation, and are high in both internal and external validity. But such experiments are relatively rare, because of the difficulties associated with manipulating treatments and controlling for extraneous effects in a field setting.
Experimental research can be grouped into two broad categories: true experimental designs and quasi-experimental designs. Both designs require treatment manipulation, but while true experiments also require random assignment, quasi-experiments do not. Sometimes, we also refer to non-experimental research, which is not really a research design, but an all-inclusive term that includes all types of research that do not employ treatment manipulation or random assignment, such as survey research, observational research, and correlational studies.
Basic concepts
Treatment and control groups. In experimental research, some subjects are administered one or more experimental stimulus called a treatment (the treatment group ) while other subjects are not given such a stimulus (the control group ). The treatment may be considered successful if subjects in the treatment group rate more favourably on outcome variables than control group subjects. Multiple levels of experimental stimulus may be administered, in which case, there may be more than one treatment group. For example, in order to test the effects of a new drug intended to treat a certain medical condition like dementia, if a sample of dementia patients is randomly divided into three groups, with the first group receiving a high dosage of the drug, the second group receiving a low dosage, and the third group receiving a placebo such as a sugar pill (control group), then the first two groups are experimental groups and the third group is a control group. After administering the drug for a period of time, if the condition of the experimental group subjects improved significantly more than the control group subjects, we can say that the drug is effective. We can also compare the conditions of the high and low dosage experimental groups to determine if the high dose is more effective than the low dose.
Treatment manipulation. Treatments are the unique feature of experimental research that sets this design apart from all other research methods. Treatment manipulation helps control for the ‘cause’ in cause-effect relationships. Naturally, the validity of experimental research depends on how well the treatment was manipulated. Treatment manipulation must be checked using pretests and pilot tests prior to the experimental study. Any measurements conducted before the treatment is administered are called pretest measures , while those conducted after the treatment are posttest measures .
Random selection and assignment. Random selection is the process of randomly drawing a sample from a population or a sampling frame. This approach is typically employed in survey research, and ensures that each unit in the population has a positive chance of being selected into the sample. Random assignment, however, is a process of randomly assigning subjects to experimental or control groups. This is a standard practice in true experimental research to ensure that treatment groups are similar (equivalent) to each other and to the control group prior to treatment administration. Random selection is related to sampling, and is therefore more closely related to the external validity (generalisability) of findings. However, random assignment is related to design, and is therefore most related to internal validity. It is possible to have both random selection and random assignment in well-designed experimental research, but quasi-experimental research involves neither random selection nor random assignment.
Threats to internal validity. Although experimental designs are considered more rigorous than other research methods in terms of the internal validity of their inferences (by virtue of their ability to control causes through treatment manipulation), they are not immune to internal validity threats. Some of these threats to internal validity are described below, within the context of a study of the impact of a special remedial math tutoring program for improving the math abilities of high school students.
History threat is the possibility that the observed effects (dependent variables) are caused by extraneous or historical events rather than by the experimental treatment. For instance, students’ post-remedial math score improvement may have been caused by their preparation for a math exam at their school, rather than the remedial math program.
Maturation threat refers to the possibility that observed effects are caused by natural maturation of subjects (e.g., a general improvement in their intellectual ability to understand complex concepts) rather than the experimental treatment.
Testing threat is a threat in pre-post designs where subjects’ posttest responses are conditioned by their pretest responses. For instance, if students remember their answers from the pretest evaluation, they may tend to repeat them in the posttest exam.
Not conducting a pretest can help avoid this threat.
Instrumentation threat , which also occurs in pre-post designs, refers to the possibility that the difference between pretest and posttest scores is not due to the remedial math program, but due to changes in the administered test, such as the posttest having a higher or lower degree of difficulty than the pretest.
Mortality threat refers to the possibility that subjects may be dropping out of the study at differential rates between the treatment and control groups due to a systematic reason, such that the dropouts were mostly students who scored low on the pretest. If the low-performing students drop out, the results of the posttest will be artificially inflated by the preponderance of high-performing students.
Regression threat —also called a regression to the mean—refers to the statistical tendency of a group’s overall performance to regress toward the mean during a posttest rather than in the anticipated direction. For instance, if subjects scored high on a pretest, they will have a tendency to score lower on the posttest (closer to the mean) because their high scores (away from the mean) during the pretest were possibly a statistical aberration. This problem tends to be more prevalent in non-random samples and when the two measures are imperfectly correlated.
Two-group experimental designs

Pretest-posttest control group design . In this design, subjects are randomly assigned to treatment and control groups, subjected to an initial (pretest) measurement of the dependent variables of interest, the treatment group is administered a treatment (representing the independent variable of interest), and the dependent variables measured again (posttest). The notation of this design is shown in Figure 10.1.

Statistical analysis of this design involves a simple analysis of variance (ANOVA) between the treatment and control groups. The pretest-posttest design handles several threats to internal validity, such as maturation, testing, and regression, since these threats can be expected to influence both treatment and control groups in a similar (random) manner. The selection threat is controlled via random assignment. However, additional threats to internal validity may exist. For instance, mortality can be a problem if there are differential dropout rates between the two groups, and the pretest measurement may bias the posttest measurement—especially if the pretest introduces unusual topics or content.
Posttest -only control group design . This design is a simpler version of the pretest-posttest design where pretest measurements are omitted. The design notation is shown in Figure 10.2.

The treatment effect is measured simply as the difference in the posttest scores between the two groups:
![Rendered by QuickLaTeX.com \[E = (O_{1} - O_{2})\,.\]](https://usq.pressbooks.pub/app/uploads/quicklatex/quicklatex.com-90f2ce47275e7b35def5c5b52b7d7d45_l3.png)
The appropriate statistical analysis of this design is also a two-group analysis of variance (ANOVA). The simplicity of this design makes it more attractive than the pretest-posttest design in terms of internal validity. This design controls for maturation, testing, regression, selection, and pretest-posttest interaction, though the mortality threat may continue to exist.

Because the pretest measure is not a measurement of the dependent variable, but rather a covariate, the treatment effect is measured as the difference in the posttest scores between the treatment and control groups as:
Due to the presence of covariates, the right statistical analysis of this design is a two-group analysis of covariance (ANCOVA). This design has all the advantages of posttest-only design, but with internal validity due to the controlling of covariates. Covariance designs can also be extended to pretest-posttest control group design.
Factorial designs
Two-group designs are inadequate if your research requires manipulation of two or more independent variables (treatments). In such cases, you would need four or higher-group designs. Such designs, quite popular in experimental research, are commonly called factorial designs. Each independent variable in this design is called a factor , and each subdivision of a factor is called a level . Factorial designs enable the researcher to examine not only the individual effect of each treatment on the dependent variables (called main effects), but also their joint effect (called interaction effects).

In a factorial design, a main effect is said to exist if the dependent variable shows a significant difference between multiple levels of one factor, at all levels of other factors. No change in the dependent variable across factor levels is the null case (baseline), from which main effects are evaluated. In the above example, you may see a main effect of instructional type, instructional time, or both on learning outcomes. An interaction effect exists when the effect of differences in one factor depends upon the level of a second factor. In our example, if the effect of instructional type on learning outcomes is greater for three hours/week of instructional time than for one and a half hours/week, then we can say that there is an interaction effect between instructional type and instructional time on learning outcomes. Note that the presence of interaction effects dominate and make main effects irrelevant, and it is not meaningful to interpret main effects if interaction effects are significant.
Hybrid experimental designs
Hybrid designs are those that are formed by combining features of more established designs. Three such hybrid designs are randomised bocks design, Solomon four-group design, and switched replications design.
Randomised block design. This is a variation of the posttest-only or pretest-posttest control group design where the subject population can be grouped into relatively homogeneous subgroups (called blocks ) within which the experiment is replicated. For instance, if you want to replicate the same posttest-only design among university students and full-time working professionals (two homogeneous blocks), subjects in both blocks are randomly split between the treatment group (receiving the same treatment) and the control group (see Figure 10.5). The purpose of this design is to reduce the ‘noise’ or variance in data that may be attributable to differences between the blocks so that the actual effect of interest can be detected more accurately.
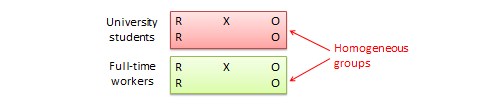
Solomon four-group design . In this design, the sample is divided into two treatment groups and two control groups. One treatment group and one control group receive the pretest, and the other two groups do not. This design represents a combination of posttest-only and pretest-posttest control group design, and is intended to test for the potential biasing effect of pretest measurement on posttest measures that tends to occur in pretest-posttest designs, but not in posttest-only designs. The design notation is shown in Figure 10.6.
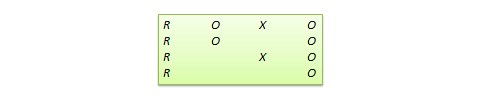
Switched replication design . This is a two-group design implemented in two phases with three waves of measurement. The treatment group in the first phase serves as the control group in the second phase, and the control group in the first phase becomes the treatment group in the second phase, as illustrated in Figure 10.7. In other words, the original design is repeated or replicated temporally with treatment/control roles switched between the two groups. By the end of the study, all participants will have received the treatment either during the first or the second phase. This design is most feasible in organisational contexts where organisational programs (e.g., employee training) are implemented in a phased manner or are repeated at regular intervals.

Quasi-experimental designs
Quasi-experimental designs are almost identical to true experimental designs, but lacking one key ingredient: random assignment. For instance, one entire class section or one organisation is used as the treatment group, while another section of the same class or a different organisation in the same industry is used as the control group. This lack of random assignment potentially results in groups that are non-equivalent, such as one group possessing greater mastery of certain content than the other group, say by virtue of having a better teacher in a previous semester, which introduces the possibility of selection bias . Quasi-experimental designs are therefore inferior to true experimental designs in interval validity due to the presence of a variety of selection related threats such as selection-maturation threat (the treatment and control groups maturing at different rates), selection-history threat (the treatment and control groups being differentially impacted by extraneous or historical events), selection-regression threat (the treatment and control groups regressing toward the mean between pretest and posttest at different rates), selection-instrumentation threat (the treatment and control groups responding differently to the measurement), selection-testing (the treatment and control groups responding differently to the pretest), and selection-mortality (the treatment and control groups demonstrating differential dropout rates). Given these selection threats, it is generally preferable to avoid quasi-experimental designs to the greatest extent possible.

In addition, there are quite a few unique non-equivalent designs without corresponding true experimental design cousins. Some of the more useful of these designs are discussed next.
Regression discontinuity (RD) design . This is a non-equivalent pretest-posttest design where subjects are assigned to the treatment or control group based on a cut-off score on a preprogram measure. For instance, patients who are severely ill may be assigned to a treatment group to test the efficacy of a new drug or treatment protocol and those who are mildly ill are assigned to the control group. In another example, students who are lagging behind on standardised test scores may be selected for a remedial curriculum program intended to improve their performance, while those who score high on such tests are not selected from the remedial program.

Because of the use of a cut-off score, it is possible that the observed results may be a function of the cut-off score rather than the treatment, which introduces a new threat to internal validity. However, using the cut-off score also ensures that limited or costly resources are distributed to people who need them the most, rather than randomly across a population, while simultaneously allowing a quasi-experimental treatment. The control group scores in the RD design do not serve as a benchmark for comparing treatment group scores, given the systematic non-equivalence between the two groups. Rather, if there is no discontinuity between pretest and posttest scores in the control group, but such a discontinuity persists in the treatment group, then this discontinuity is viewed as evidence of the treatment effect.
Proxy pretest design . This design, shown in Figure 10.11, looks very similar to the standard NEGD (pretest-posttest) design, with one critical difference: the pretest score is collected after the treatment is administered. A typical application of this design is when a researcher is brought in to test the efficacy of a program (e.g., an educational program) after the program has already started and pretest data is not available. Under such circumstances, the best option for the researcher is often to use a different prerecorded measure, such as students’ grade point average before the start of the program, as a proxy for pretest data. A variation of the proxy pretest design is to use subjects’ posttest recollection of pretest data, which may be subject to recall bias, but nevertheless may provide a measure of perceived gain or change in the dependent variable.

Separate pretest-posttest samples design . This design is useful if it is not possible to collect pretest and posttest data from the same subjects for some reason. As shown in Figure 10.12, there are four groups in this design, but two groups come from a single non-equivalent group, while the other two groups come from a different non-equivalent group. For instance, say you want to test customer satisfaction with a new online service that is implemented in one city but not in another. In this case, customers in the first city serve as the treatment group and those in the second city constitute the control group. If it is not possible to obtain pretest and posttest measures from the same customers, you can measure customer satisfaction at one point in time, implement the new service program, and measure customer satisfaction (with a different set of customers) after the program is implemented. Customer satisfaction is also measured in the control group at the same times as in the treatment group, but without the new program implementation. The design is not particularly strong, because you cannot examine the changes in any specific customer’s satisfaction score before and after the implementation, but you can only examine average customer satisfaction scores. Despite the lower internal validity, this design may still be a useful way of collecting quasi-experimental data when pretest and posttest data is not available from the same subjects.
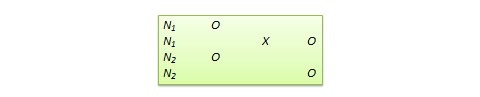
An interesting variation of the NEDV design is a pattern-matching NEDV design , which employs multiple outcome variables and a theory that explains how much each variable will be affected by the treatment. The researcher can then examine if the theoretical prediction is matched in actual observations. This pattern-matching technique—based on the degree of correspondence between theoretical and observed patterns—is a powerful way of alleviating internal validity concerns in the original NEDV design.

Perils of experimental research
Experimental research is one of the most difficult of research designs, and should not be taken lightly. This type of research is often best with a multitude of methodological problems. First, though experimental research requires theories for framing hypotheses for testing, much of current experimental research is atheoretical. Without theories, the hypotheses being tested tend to be ad hoc, possibly illogical, and meaningless. Second, many of the measurement instruments used in experimental research are not tested for reliability and validity, and are incomparable across studies. Consequently, results generated using such instruments are also incomparable. Third, often experimental research uses inappropriate research designs, such as irrelevant dependent variables, no interaction effects, no experimental controls, and non-equivalent stimulus across treatment groups. Findings from such studies tend to lack internal validity and are highly suspect. Fourth, the treatments (tasks) used in experimental research may be diverse, incomparable, and inconsistent across studies, and sometimes inappropriate for the subject population. For instance, undergraduate student subjects are often asked to pretend that they are marketing managers and asked to perform a complex budget allocation task in which they have no experience or expertise. The use of such inappropriate tasks, introduces new threats to internal validity (i.e., subject’s performance may be an artefact of the content or difficulty of the task setting), generates findings that are non-interpretable and meaningless, and makes integration of findings across studies impossible.
The design of proper experimental treatments is a very important task in experimental design, because the treatment is the raison d’etre of the experimental method, and must never be rushed or neglected. To design an adequate and appropriate task, researchers should use prevalidated tasks if available, conduct treatment manipulation checks to check for the adequacy of such tasks (by debriefing subjects after performing the assigned task), conduct pilot tests (repeatedly, if necessary), and if in doubt, use tasks that are simple and familiar for the respondent sample rather than tasks that are complex or unfamiliar.
In summary, this chapter introduced key concepts in the experimental design research method and introduced a variety of true experimental and quasi-experimental designs. Although these designs vary widely in internal validity, designs with less internal validity should not be overlooked and may sometimes be useful under specific circumstances and empirical contingencies.
Social Science Research: Principles, Methods and Practices (Revised edition) Copyright © 2019 by Anol Bhattacherjee is licensed under a Creative Commons Attribution-NonCommercial-ShareAlike 4.0 International License , except where otherwise noted.
Share This Book
- Experimental Research Designs: Types, Examples & Methods

Experimental research is the most familiar type of research design for individuals in the physical sciences and a host of other fields. This is mainly because experimental research is a classical scientific experiment, similar to those performed in high school science classes.
Imagine taking 2 samples of the same plant and exposing one of them to sunlight, while the other is kept away from sunlight. Let the plant exposed to sunlight be called sample A, while the latter is called sample B.
If after the duration of the research, we find out that sample A grows and sample B dies, even though they are both regularly wetted and given the same treatment. Therefore, we can conclude that sunlight will aid growth in all similar plants.
What is Experimental Research?
Experimental research is a scientific approach to research, where one or more independent variables are manipulated and applied to one or more dependent variables to measure their effect on the latter. The effect of the independent variables on the dependent variables is usually observed and recorded over some time, to aid researchers in drawing a reasonable conclusion regarding the relationship between these 2 variable types.
The experimental research method is widely used in physical and social sciences, psychology, and education. It is based on the comparison between two or more groups with a straightforward logic, which may, however, be difficult to execute.
Mostly related to a laboratory test procedure, experimental research designs involve collecting quantitative data and performing statistical analysis on them during research. Therefore, making it an example of quantitative research method .
What are The Types of Experimental Research Design?
The types of experimental research design are determined by the way the researcher assigns subjects to different conditions and groups. They are of 3 types, namely; pre-experimental, quasi-experimental, and true experimental research.
Pre-experimental Research Design
In pre-experimental research design, either a group or various dependent groups are observed for the effect of the application of an independent variable which is presumed to cause change. It is the simplest form of experimental research design and is treated with no control group.
Although very practical, experimental research is lacking in several areas of the true-experimental criteria. The pre-experimental research design is further divided into three types
- One-shot Case Study Research Design
In this type of experimental study, only one dependent group or variable is considered. The study is carried out after some treatment which was presumed to cause change, making it a posttest study.
- One-group Pretest-posttest Research Design:
This research design combines both posttest and pretest study by carrying out a test on a single group before the treatment is administered and after the treatment is administered. With the former being administered at the beginning of treatment and later at the end.
- Static-group Comparison:
In a static-group comparison study, 2 or more groups are placed under observation, where only one of the groups is subjected to some treatment while the other groups are held static. All the groups are post-tested, and the observed differences between the groups are assumed to be a result of the treatment.
Quasi-experimental Research Design
The word “quasi” means partial, half, or pseudo. Therefore, the quasi-experimental research bearing a resemblance to the true experimental research, but not the same. In quasi-experiments, the participants are not randomly assigned, and as such, they are used in settings where randomization is difficult or impossible.
This is very common in educational research, where administrators are unwilling to allow the random selection of students for experimental samples.
Some examples of quasi-experimental research design include; the time series, no equivalent control group design, and the counterbalanced design.
True Experimental Research Design
The true experimental research design relies on statistical analysis to approve or disprove a hypothesis. It is the most accurate type of experimental design and may be carried out with or without a pretest on at least 2 randomly assigned dependent subjects.
The true experimental research design must contain a control group, a variable that can be manipulated by the researcher, and the distribution must be random. The classification of true experimental design include:
- The posttest-only Control Group Design: In this design, subjects are randomly selected and assigned to the 2 groups (control and experimental), and only the experimental group is treated. After close observation, both groups are post-tested, and a conclusion is drawn from the difference between these groups.
- The pretest-posttest Control Group Design: For this control group design, subjects are randomly assigned to the 2 groups, both are presented, but only the experimental group is treated. After close observation, both groups are post-tested to measure the degree of change in each group.
- Solomon four-group Design: This is the combination of the pretest-only and the pretest-posttest control groups. In this case, the randomly selected subjects are placed into 4 groups.
The first two of these groups are tested using the posttest-only method, while the other two are tested using the pretest-posttest method.
Examples of Experimental Research
Experimental research examples are different, depending on the type of experimental research design that is being considered. The most basic example of experimental research is laboratory experiments, which may differ in nature depending on the subject of research.
Administering Exams After The End of Semester
During the semester, students in a class are lectured on particular courses and an exam is administered at the end of the semester. In this case, the students are the subjects or dependent variables while the lectures are the independent variables treated on the subjects.
Only one group of carefully selected subjects are considered in this research, making it a pre-experimental research design example. We will also notice that tests are only carried out at the end of the semester, and not at the beginning.
Further making it easy for us to conclude that it is a one-shot case study research.
Employee Skill Evaluation
Before employing a job seeker, organizations conduct tests that are used to screen out less qualified candidates from the pool of qualified applicants. This way, organizations can determine an employee’s skill set at the point of employment.
In the course of employment, organizations also carry out employee training to improve employee productivity and generally grow the organization. Further evaluation is carried out at the end of each training to test the impact of the training on employee skills, and test for improvement.
Here, the subject is the employee, while the treatment is the training conducted. This is a pretest-posttest control group experimental research example.
Evaluation of Teaching Method
Let us consider an academic institution that wants to evaluate the teaching method of 2 teachers to determine which is best. Imagine a case whereby the students assigned to each teacher is carefully selected probably due to personal request by parents or due to stubbornness and smartness.
This is a no equivalent group design example because the samples are not equal. By evaluating the effectiveness of each teacher’s teaching method this way, we may conclude after a post-test has been carried out.
However, this may be influenced by factors like the natural sweetness of a student. For example, a very smart student will grab more easily than his or her peers irrespective of the method of teaching.
What are the Characteristics of Experimental Research?
Experimental research contains dependent, independent and extraneous variables. The dependent variables are the variables being treated or manipulated and are sometimes called the subject of the research.
The independent variables are the experimental treatment being exerted on the dependent variables. Extraneous variables, on the other hand, are other factors affecting the experiment that may also contribute to the change.
The setting is where the experiment is carried out. Many experiments are carried out in the laboratory, where control can be exerted on the extraneous variables, thereby eliminating them.
Other experiments are carried out in a less controllable setting. The choice of setting used in research depends on the nature of the experiment being carried out.
- Multivariable
Experimental research may include multiple independent variables, e.g. time, skills, test scores, etc.
Why Use Experimental Research Design?
Experimental research design can be majorly used in physical sciences, social sciences, education, and psychology. It is used to make predictions and draw conclusions on a subject matter.
Some uses of experimental research design are highlighted below.
- Medicine: Experimental research is used to provide the proper treatment for diseases. In most cases, rather than directly using patients as the research subject, researchers take a sample of the bacteria from the patient’s body and are treated with the developed antibacterial
The changes observed during this period are recorded and evaluated to determine its effectiveness. This process can be carried out using different experimental research methods.
- Education: Asides from science subjects like Chemistry and Physics which involves teaching students how to perform experimental research, it can also be used in improving the standard of an academic institution. This includes testing students’ knowledge on different topics, coming up with better teaching methods, and the implementation of other programs that will aid student learning.
- Human Behavior: Social scientists are the ones who mostly use experimental research to test human behaviour. For example, consider 2 people randomly chosen to be the subject of the social interaction research where one person is placed in a room without human interaction for 1 year.
The other person is placed in a room with a few other people, enjoying human interaction. There will be a difference in their behaviour at the end of the experiment.
- UI/UX: During the product development phase, one of the major aims of the product team is to create a great user experience with the product. Therefore, before launching the final product design, potential are brought in to interact with the product.
For example, when finding it difficult to choose how to position a button or feature on the app interface, a random sample of product testers are allowed to test the 2 samples and how the button positioning influences the user interaction is recorded.
What are the Disadvantages of Experimental Research?
- It is highly prone to human error due to its dependency on variable control which may not be properly implemented. These errors could eliminate the validity of the experiment and the research being conducted.
- Exerting control of extraneous variables may create unrealistic situations. Eliminating real-life variables will result in inaccurate conclusions. This may also result in researchers controlling the variables to suit his or her personal preferences.
- It is a time-consuming process. So much time is spent on testing dependent variables and waiting for the effect of the manipulation of dependent variables to manifest.
- It is expensive.
- It is very risky and may have ethical complications that cannot be ignored. This is common in medical research, where failed trials may lead to a patient’s death or a deteriorating health condition.
- Experimental research results are not descriptive.
- Response bias can also be supplied by the subject of the conversation.
- Human responses in experimental research can be difficult to measure.
What are the Data Collection Methods in Experimental Research?
Data collection methods in experimental research are the different ways in which data can be collected for experimental research. They are used in different cases, depending on the type of research being carried out.
1. Observational Study
This type of study is carried out over a long period. It measures and observes the variables of interest without changing existing conditions.
When researching the effect of social interaction on human behavior, the subjects who are placed in 2 different environments are observed throughout the research. No matter the kind of absurd behavior that is exhibited by the subject during this period, its condition will not be changed.
This may be a very risky thing to do in medical cases because it may lead to death or worse medical conditions.
2. Simulations
This procedure uses mathematical, physical, or computer models to replicate a real-life process or situation. It is frequently used when the actual situation is too expensive, dangerous, or impractical to replicate in real life.
This method is commonly used in engineering and operational research for learning purposes and sometimes as a tool to estimate possible outcomes of real research. Some common situation software are Simulink, MATLAB, and Simul8.
Not all kinds of experimental research can be carried out using simulation as a data collection tool . It is very impractical for a lot of laboratory-based research that involves chemical processes.
A survey is a tool used to gather relevant data about the characteristics of a population and is one of the most common data collection tools. A survey consists of a group of questions prepared by the researcher, to be answered by the research subject.
Surveys can be shared with the respondents both physically and electronically. When collecting data through surveys, the kind of data collected depends on the respondent, and researchers have limited control over it.
Formplus is the best tool for collecting experimental data using survey s. It has relevant features that will aid the data collection process and can also be used in other aspects of experimental research.
Differences between Experimental and Non-Experimental Research
1. In experimental research, the researcher can control and manipulate the environment of the research, including the predictor variable which can be changed. On the other hand, non-experimental research cannot be controlled or manipulated by the researcher at will.
This is because it takes place in a real-life setting, where extraneous variables cannot be eliminated. Therefore, it is more difficult to conclude non-experimental studies, even though they are much more flexible and allow for a greater range of study fields.
2. The relationship between cause and effect cannot be established in non-experimental research, while it can be established in experimental research. This may be because many extraneous variables also influence the changes in the research subject, making it difficult to point at a particular variable as the cause of a particular change
3. Independent variables are not introduced, withdrawn, or manipulated in non-experimental designs, but the same may not be said about experimental research.
Conclusion
Experimental research designs are often considered to be the standard in research designs. This is partly due to the common misconception that research is equivalent to scientific experiments—a component of experimental research design.
In this research design, one or more subjects or dependent variables are randomly assigned to different treatments (i.e. independent variables manipulated by the researcher) and the results are observed to conclude. One of the uniqueness of experimental research is in its ability to control the effect of extraneous variables.
Experimental research is suitable for research whose goal is to examine cause-effect relationships, e.g. explanatory research. It can be conducted in the laboratory or field settings, depending on the aim of the research that is being carried out.
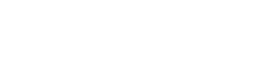
Connect to Formplus, Get Started Now - It's Free!
- examples of experimental research
- experimental research methods
- types of experimental research
- busayo.longe
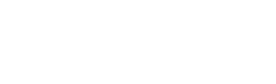
You may also like:
Response vs Explanatory Variables: Definition & Examples
In this article, we’ll be comparing the two types of variables, what they both mean and see some of their real-life applications in research

Experimental Vs Non-Experimental Research: 15 Key Differences
Differences between experimental and non experimental research on definitions, types, examples, data collection tools, uses, advantages etc.
Simpson’s Paradox & How to Avoid it in Experimental Research
In this article, we are going to look at Simpson’s Paradox from its historical point and later, we’ll consider its effect in...
What is Experimenter Bias? Definition, Types & Mitigation
In this article, we will look into the concept of experimental bias and how it can be identified in your research
Formplus - For Seamless Data Collection
Collect data the right way with a versatile data collection tool. try formplus and transform your work productivity today..
Experimental design: Guide, steps, examples
Last updated
27 April 2023
Reviewed by
Miroslav Damyanov
Experimental research design is a scientific framework that allows you to manipulate one or more variables while controlling the test environment.
When testing a theory or new product, it can be helpful to have a certain level of control and manipulate variables to discover different outcomes. You can use these experiments to determine cause and effect or study variable associations.
This guide explores the types of experimental design, the steps in designing an experiment, and the advantages and limitations of experimental design.
Make research less tedious
Dovetail streamlines research to help you uncover and share actionable insights
- What is experimental research design?
You can determine the relationship between each of the variables by:
Manipulating one or more independent variables (i.e., stimuli or treatments)
Applying the changes to one or more dependent variables (i.e., test groups or outcomes)
With the ability to analyze the relationship between variables and using measurable data, you can increase the accuracy of the result.
What is a good experimental design?
A good experimental design requires:
Significant planning to ensure control over the testing environment
Sound experimental treatments
Properly assigning subjects to treatment groups
Without proper planning, unexpected external variables can alter an experiment's outcome.
To meet your research goals, your experimental design should include these characteristics:
Provide unbiased estimates of inputs and associated uncertainties
Enable the researcher to detect differences caused by independent variables
Include a plan for analysis and reporting of the results
Provide easily interpretable results with specific conclusions
What's the difference between experimental and quasi-experimental design?
The major difference between experimental and quasi-experimental design is the random assignment of subjects to groups.
A true experiment relies on certain controls. Typically, the researcher designs the treatment and randomly assigns subjects to control and treatment groups.
However, these conditions are unethical or impossible to achieve in some situations.
When it's unethical or impractical to assign participants randomly, that’s when a quasi-experimental design comes in.
This design allows researchers to conduct a similar experiment by assigning subjects to groups based on non-random criteria.
Another type of quasi-experimental design might occur when the researcher doesn't have control over the treatment but studies pre-existing groups after they receive different treatments.
When can a researcher conduct experimental research?
Various settings and professions can use experimental research to gather information and observe behavior in controlled settings.
Basically, a researcher can conduct experimental research any time they want to test a theory with variable and dependent controls.
Experimental research is an option when the project includes an independent variable and a desire to understand the relationship between cause and effect.
- The importance of experimental research design
Experimental research enables researchers to conduct studies that provide specific, definitive answers to questions and hypotheses.
Researchers can test Independent variables in controlled settings to:
Test the effectiveness of a new medication
Design better products for consumers
Answer questions about human health and behavior
Developing a quality research plan means a researcher can accurately answer vital research questions with minimal error. As a result, definitive conclusions can influence the future of the independent variable.
Types of experimental research designs
There are three main types of experimental research design. The research type you use will depend on the criteria of your experiment, your research budget, and environmental limitations.
Pre-experimental research design
A pre-experimental research study is a basic observational study that monitors independent variables’ effects.
During research, you observe one or more groups after applying a treatment to test whether the treatment causes any change.
The three subtypes of pre-experimental research design are:
One-shot case study research design
This research method introduces a single test group to a single stimulus to study the results at the end of the application.
After researchers presume the stimulus or treatment has caused changes, they gather results to determine how it affects the test subjects.
One-group pretest-posttest design
This method uses a single test group but includes a pretest study as a benchmark. The researcher applies a test before and after the group’s exposure to a specific stimulus.
Static group comparison design
This method includes two or more groups, enabling the researcher to use one group as a control. They apply a stimulus to one group and leave the other group static.
A posttest study compares the results among groups.
True experimental research design
A true experiment is the most common research method. It involves statistical analysis to prove or disprove a specific hypothesis .
Under completely experimental conditions, researchers expose participants in two or more randomized groups to different stimuli.
Random selection removes any potential for bias, providing more reliable results.
These are the three main sub-groups of true experimental research design:
Posttest-only control group design
This structure requires the researcher to divide participants into two random groups. One group receives no stimuli and acts as a control while the other group experiences stimuli.
Researchers perform a test at the end of the experiment to observe the stimuli exposure results.
Pretest-posttest control group design
This test also requires two groups. It includes a pretest as a benchmark before introducing the stimulus.
The pretest introduces multiple ways to test subjects. For instance, if the control group also experiences a change, it reveals that taking the test twice changes the results.
Solomon four-group design
This structure divides subjects into two groups, with two as control groups. Researchers assign the first control group a posttest only and the second control group a pretest and a posttest.
The two variable groups mirror the control groups, but researchers expose them to stimuli. The ability to differentiate between groups in multiple ways provides researchers with more testing approaches for data-based conclusions.
Quasi-experimental research design
Although closely related to a true experiment, quasi-experimental research design differs in approach and scope.
Quasi-experimental research design doesn’t have randomly selected participants. Researchers typically divide the groups in this research by pre-existing differences.
Quasi-experimental research is more common in educational studies, nursing, or other research projects where it's not ethical or practical to use randomized subject groups.
- 5 steps for designing an experiment
Experimental research requires a clearly defined plan to outline the research parameters and expected goals.
Here are five key steps in designing a successful experiment:
Step 1: Define variables and their relationship
Your experiment should begin with a question: What are you hoping to learn through your experiment?
The relationship between variables in your study will determine your answer.
Define the independent variable (the intended stimuli) and the dependent variable (the expected effect of the stimuli). After identifying these groups, consider how you might control them in your experiment.
Could natural variations affect your research? If so, your experiment should include a pretest and posttest.
Step 2: Develop a specific, testable hypothesis
With a firm understanding of the system you intend to study, you can write a specific, testable hypothesis.
What is the expected outcome of your study?
Develop a prediction about how the independent variable will affect the dependent variable.
How will the stimuli in your experiment affect your test subjects?
Your hypothesis should provide a prediction of the answer to your research question .
Step 3: Design experimental treatments to manipulate your independent variable
Depending on your experiment, your variable may be a fixed stimulus (like a medical treatment) or a variable stimulus (like a period during which an activity occurs).
Determine which type of stimulus meets your experiment’s needs and how widely or finely to vary your stimuli.
Step 4: Assign subjects to groups
When you have a clear idea of how to carry out your experiment, you can determine how to assemble test groups for an accurate study.
When choosing your study groups, consider:
The size of your experiment
Whether you can select groups randomly
Your target audience for the outcome of the study
You should be able to create groups with an equal number of subjects and include subjects that match your target audience. Remember, you should assign one group as a control and use one or more groups to study the effects of variables.
Step 5: Plan how to measure your dependent variable
This step determines how you'll collect data to determine the study's outcome. You should seek reliable and valid measurements that minimize research bias or error.
You can measure some data with scientific tools, while you’ll need to operationalize other forms to turn them into measurable observations.
- Advantages of experimental research
Experimental research is an integral part of our world. It allows researchers to conduct experiments that answer specific questions.
While researchers use many methods to conduct different experiments, experimental research offers these distinct benefits:
Researchers can determine cause and effect by manipulating variables.
It gives researchers a high level of control.
Researchers can test multiple variables within a single experiment.
All industries and fields of knowledge can use it.
Researchers can duplicate results to promote the validity of the study .
Replicating natural settings rapidly means immediate research.
Researchers can combine it with other research methods.
It provides specific conclusions about the validity of a product, theory, or idea.
- Disadvantages (or limitations) of experimental research
Unfortunately, no research type yields ideal conditions or perfect results.
While experimental research might be the right choice for some studies, certain conditions could render experiments useless or even dangerous.
Before conducting experimental research, consider these disadvantages and limitations:
Required professional qualification
Only competent professionals with an academic degree and specific training are qualified to conduct rigorous experimental research. This ensures results are unbiased and valid.
Limited scope
Experimental research may not capture the complexity of some phenomena, such as social interactions or cultural norms. These are difficult to control in a laboratory setting.
Resource-intensive
Experimental research can be expensive, time-consuming, and require significant resources, such as specialized equipment or trained personnel.
Limited generalizability
The controlled nature means the research findings may not fully apply to real-world situations or people outside the experimental setting.
Practical or ethical concerns
Some experiments may involve manipulating variables that could harm participants or violate ethical guidelines .
Researchers must ensure their experiments do not cause harm or discomfort to participants.
Sometimes, recruiting a sample of people to randomly assign may be difficult.
- Experimental research design example
Experiments across all industries and research realms provide scientists, developers, and other researchers with definitive answers. These experiments can solve problems, create inventions, and heal illnesses.
Product design testing is an excellent example of experimental research.
A company in the product development phase creates multiple prototypes for testing. With a randomized selection, researchers introduce each test group to a different prototype.
When groups experience different product designs , the company can assess which option most appeals to potential customers.
Experimental research design provides researchers with a controlled environment to conduct experiments that evaluate cause and effect.
Using the five steps to develop a research plan ensures you anticipate and eliminate external variables while answering life’s crucial questions.
Editor’s picks
Last updated: 11 January 2024
Last updated: 15 January 2024
Last updated: 25 November 2023
Last updated: 12 May 2023
Last updated: 30 April 2024
Last updated: 18 May 2023
Last updated: 10 April 2023
Latest articles
Related topics, .css-je19u9{-webkit-align-items:flex-end;-webkit-box-align:flex-end;-ms-flex-align:flex-end;align-items:flex-end;display:-webkit-box;display:-webkit-flex;display:-ms-flexbox;display:flex;-webkit-flex-direction:row;-ms-flex-direction:row;flex-direction:row;-webkit-box-flex-wrap:wrap;-webkit-flex-wrap:wrap;-ms-flex-wrap:wrap;flex-wrap:wrap;-webkit-box-pack:center;-ms-flex-pack:center;-webkit-justify-content:center;justify-content:center;row-gap:0;text-align:center;max-width:671px;}@media (max-width: 1079px){.css-je19u9{max-width:400px;}.css-je19u9>span{white-space:pre;}}@media (max-width: 799px){.css-je19u9{max-width:400px;}.css-je19u9>span{white-space:pre;}} decide what to .css-1kiodld{max-height:56px;display:-webkit-box;display:-webkit-flex;display:-ms-flexbox;display:flex;-webkit-align-items:center;-webkit-box-align:center;-ms-flex-align:center;align-items:center;}@media (max-width: 1079px){.css-1kiodld{display:none;}} build next, decide what to build next.

Users report unexpectedly high data usage, especially during streaming sessions.

Users find it hard to navigate from the home page to relevant playlists in the app.

It would be great to have a sleep timer feature, especially for bedtime listening.

I need better filters to find the songs or artists I’m looking for.
- Types of experimental
Log in or sign up
Get started for free

Experimental Research Design — 6 mistakes you should never make!
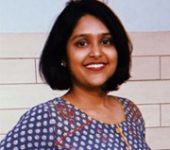
Since school days’ students perform scientific experiments that provide results that define and prove the laws and theorems in science. These experiments are laid on a strong foundation of experimental research designs.
An experimental research design helps researchers execute their research objectives with more clarity and transparency.
In this article, we will not only discuss the key aspects of experimental research designs but also the issues to avoid and problems to resolve while designing your research study.
Table of Contents
What Is Experimental Research Design?
Experimental research design is a framework of protocols and procedures created to conduct experimental research with a scientific approach using two sets of variables. Herein, the first set of variables acts as a constant, used to measure the differences of the second set. The best example of experimental research methods is quantitative research .
Experimental research helps a researcher gather the necessary data for making better research decisions and determining the facts of a research study.
When Can a Researcher Conduct Experimental Research?
A researcher can conduct experimental research in the following situations —
- When time is an important factor in establishing a relationship between the cause and effect.
- When there is an invariable or never-changing behavior between the cause and effect.
- Finally, when the researcher wishes to understand the importance of the cause and effect.
Importance of Experimental Research Design
To publish significant results, choosing a quality research design forms the foundation to build the research study. Moreover, effective research design helps establish quality decision-making procedures, structures the research to lead to easier data analysis, and addresses the main research question. Therefore, it is essential to cater undivided attention and time to create an experimental research design before beginning the practical experiment.
By creating a research design, a researcher is also giving oneself time to organize the research, set up relevant boundaries for the study, and increase the reliability of the results. Through all these efforts, one could also avoid inconclusive results. If any part of the research design is flawed, it will reflect on the quality of the results derived.
Types of Experimental Research Designs
Based on the methods used to collect data in experimental studies, the experimental research designs are of three primary types:
1. Pre-experimental Research Design
A research study could conduct pre-experimental research design when a group or many groups are under observation after implementing factors of cause and effect of the research. The pre-experimental design will help researchers understand whether further investigation is necessary for the groups under observation.
Pre-experimental research is of three types —
- One-shot Case Study Research Design
- One-group Pretest-posttest Research Design
- Static-group Comparison
2. True Experimental Research Design
A true experimental research design relies on statistical analysis to prove or disprove a researcher’s hypothesis. It is one of the most accurate forms of research because it provides specific scientific evidence. Furthermore, out of all the types of experimental designs, only a true experimental design can establish a cause-effect relationship within a group. However, in a true experiment, a researcher must satisfy these three factors —
- There is a control group that is not subjected to changes and an experimental group that will experience the changed variables
- A variable that can be manipulated by the researcher
- Random distribution of the variables
This type of experimental research is commonly observed in the physical sciences.
3. Quasi-experimental Research Design
The word “Quasi” means similarity. A quasi-experimental design is similar to a true experimental design. However, the difference between the two is the assignment of the control group. In this research design, an independent variable is manipulated, but the participants of a group are not randomly assigned. This type of research design is used in field settings where random assignment is either irrelevant or not required.
The classification of the research subjects, conditions, or groups determines the type of research design to be used.
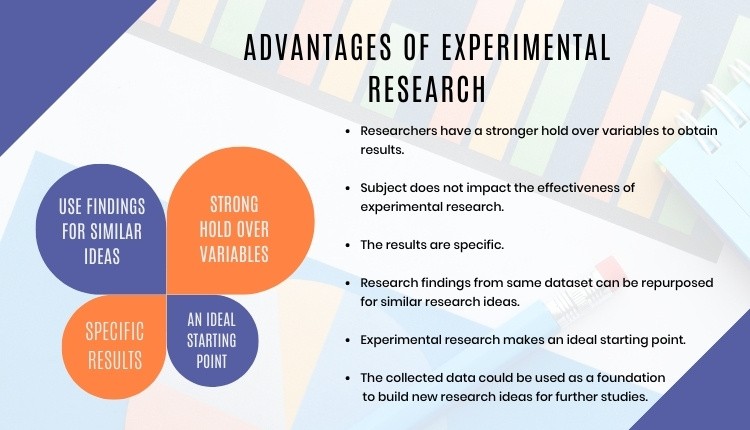
Advantages of Experimental Research
Experimental research allows you to test your idea in a controlled environment before taking the research to clinical trials. Moreover, it provides the best method to test your theory because of the following advantages:
- Researchers have firm control over variables to obtain results.
- The subject does not impact the effectiveness of experimental research. Anyone can implement it for research purposes.
- The results are specific.
- Post results analysis, research findings from the same dataset can be repurposed for similar research ideas.
- Researchers can identify the cause and effect of the hypothesis and further analyze this relationship to determine in-depth ideas.
- Experimental research makes an ideal starting point. The collected data could be used as a foundation to build new research ideas for further studies.
6 Mistakes to Avoid While Designing Your Research
There is no order to this list, and any one of these issues can seriously compromise the quality of your research. You could refer to the list as a checklist of what to avoid while designing your research.
1. Invalid Theoretical Framework
Usually, researchers miss out on checking if their hypothesis is logical to be tested. If your research design does not have basic assumptions or postulates, then it is fundamentally flawed and you need to rework on your research framework.
2. Inadequate Literature Study
Without a comprehensive research literature review , it is difficult to identify and fill the knowledge and information gaps. Furthermore, you need to clearly state how your research will contribute to the research field, either by adding value to the pertinent literature or challenging previous findings and assumptions.
3. Insufficient or Incorrect Statistical Analysis
Statistical results are one of the most trusted scientific evidence. The ultimate goal of a research experiment is to gain valid and sustainable evidence. Therefore, incorrect statistical analysis could affect the quality of any quantitative research.
4. Undefined Research Problem
This is one of the most basic aspects of research design. The research problem statement must be clear and to do that, you must set the framework for the development of research questions that address the core problems.
5. Research Limitations
Every study has some type of limitations . You should anticipate and incorporate those limitations into your conclusion, as well as the basic research design. Include a statement in your manuscript about any perceived limitations, and how you considered them while designing your experiment and drawing the conclusion.
6. Ethical Implications
The most important yet less talked about topic is the ethical issue. Your research design must include ways to minimize any risk for your participants and also address the research problem or question at hand. If you cannot manage the ethical norms along with your research study, your research objectives and validity could be questioned.
Experimental Research Design Example
In an experimental design, a researcher gathers plant samples and then randomly assigns half the samples to photosynthesize in sunlight and the other half to be kept in a dark box without sunlight, while controlling all the other variables (nutrients, water, soil, etc.)
By comparing their outcomes in biochemical tests, the researcher can confirm that the changes in the plants were due to the sunlight and not the other variables.
Experimental research is often the final form of a study conducted in the research process which is considered to provide conclusive and specific results. But it is not meant for every research. It involves a lot of resources, time, and money and is not easy to conduct, unless a foundation of research is built. Yet it is widely used in research institutes and commercial industries, for its most conclusive results in the scientific approach.
Have you worked on research designs? How was your experience creating an experimental design? What difficulties did you face? Do write to us or comment below and share your insights on experimental research designs!
Frequently Asked Questions
Randomization is important in an experimental research because it ensures unbiased results of the experiment. It also measures the cause-effect relationship on a particular group of interest.
Experimental research design lay the foundation of a research and structures the research to establish quality decision making process.
There are 3 types of experimental research designs. These are pre-experimental research design, true experimental research design, and quasi experimental research design.
The difference between an experimental and a quasi-experimental design are: 1. The assignment of the control group in quasi experimental research is non-random, unlike true experimental design, which is randomly assigned. 2. Experimental research group always has a control group; on the other hand, it may not be always present in quasi experimental research.
Experimental research establishes a cause-effect relationship by testing a theory or hypothesis using experimental groups or control variables. In contrast, descriptive research describes a study or a topic by defining the variables under it and answering the questions related to the same.

good and valuable
Very very good
Good presentation.
Rate this article Cancel Reply
Your email address will not be published.
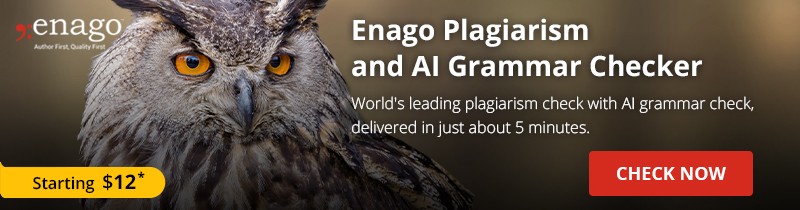
Enago Academy's Most Popular Articles
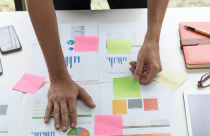
- Publishing Research
- Reporting Research
How to Optimize Your Research Process: A step-by-step guide
For researchers across disciplines, the path to uncovering novel findings and insights is often filled…
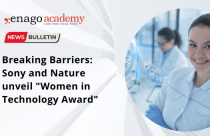
- Industry News
- Trending Now
Breaking Barriers: Sony and Nature unveil “Women in Technology Award”
Sony Group Corporation and the prestigious scientific journal Nature have collaborated to launch the inaugural…
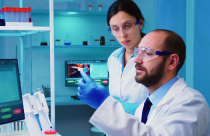
Achieving Research Excellence: Checklist for good research practices
Academia is built on the foundation of trustworthy and high-quality research, supported by the pillars…
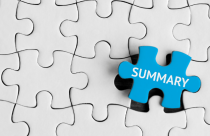
- Promoting Research
Plain Language Summary — Communicating your research to bridge the academic-lay gap
Science can be complex, but does that mean it should not be accessible to the…
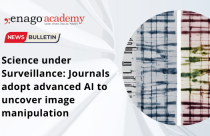
Science under Surveillance: Journals adopt advanced AI to uncover image manipulation
Journals are increasingly turning to cutting-edge AI tools to uncover deceitful images published in manuscripts.…
Choosing the Right Analytical Approach: Thematic analysis vs. content analysis for…
Comparing Cross Sectional and Longitudinal Studies: 5 steps for choosing the right…
Research Recommendations – Guiding policy-makers for evidence-based decision making

Sign-up to read more
Subscribe for free to get unrestricted access to all our resources on research writing and academic publishing including:
- 2000+ blog articles
- 50+ Webinars
- 10+ Expert podcasts
- 50+ Infographics
- 10+ Checklists
- Research Guides
We hate spam too. We promise to protect your privacy and never spam you.
I am looking for Editing/ Proofreading services for my manuscript Tentative date of next journal submission:
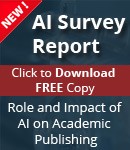
As a researcher, what do you consider most when choosing an image manipulation detector?
- What is New
- Download Your Software
- Behavioral Research
- Software for Consumer Research
- Software for Human Factors R&D
- Request Live Demo
- Contact Sales
Sensor Hardware
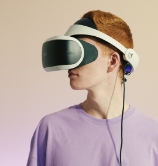
We carry a range of biosensors from the top hardware producers. All compatible with iMotions
iMotions for Higher Education
Imotions for business.
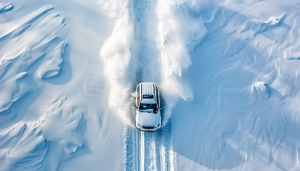
New OSM Reference System from iMotions
Laila Mowla
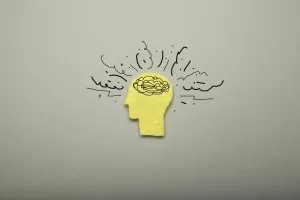
How to Measure Stress
Measure Stress
News & Events
- iMotions Lab
- iMotions Online
Eye Tracking
- Eye Tracking Screen Based
- Eye Tracking VR
- Eye Tracking Glasses
- Eye Tracking Webcam
- FEA (Facial Expression Analysis)
- Voice Analysis
- EDA/GSR (Electrodermal Activity)
- EEG (Electroencephalography)
- ECG (Electrocardiography)
- EMG (Electromyography)
- Respiration
- iMotions Lab: New features
- iMotions Lab: Developers
- EEG sensors
- Sensory and Perceptual
- Consumer Inights
- Human Factors R&D
- Work Environments, Training and Safety
- Customer Stories
- Published Research Papers
- Document Library
- Customer Support Program
- Help Center
- Release Notes
- Contact Support
- Partnerships
- Mission Statement
- Ownership and Structure
- Executive Management
- Job Opportunities
Publications
- Newsletter Sign Up
Experimental Design: The Complete Pocket Guide
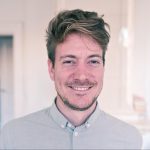
Bryn Farnsworth
Our comprehensive manual on experimental design provides guidance on avoiding common mistakes and pitfalls when establishing the optimal experiment for your research.
Table of Contents
- Introduction to experimental methods
Humans are a quite curious species. We explore new grounds, improve products and services, find faster and safer ways to produce or transport goods, and we solve the mysteries of global diseases. All of these activities are guided by asking the right questions, by searching for answers in the right spots and taking appropriate decisions. Academic and commercial research have professionalized this quest for knowledge and insights into ourselves and the world surrounding us.
Every day, research institutions across the globe investigate the inner workings of our universe – from cellular levels of our synapses and neurons to macroscopic levels of planets and solar systems – by means of experimentation. Simply put: Experiments are the professional way to answer questions, identify cause and effect or determine predictors and outcomes. These insights help us understand how and why things are what they are and can ultimately be used to change the world by improving the good and overcoming the bad.
N.B. this post is an excerpt from our Experimental Design Guide. You can download your free copy below and get even more insights into the world of Experimental Design.
Free 44-page Experimental Design Guide
For Beginners and Intermediates
- Respondent management with groups and populations
- How to set up stimulus selection and arrangement
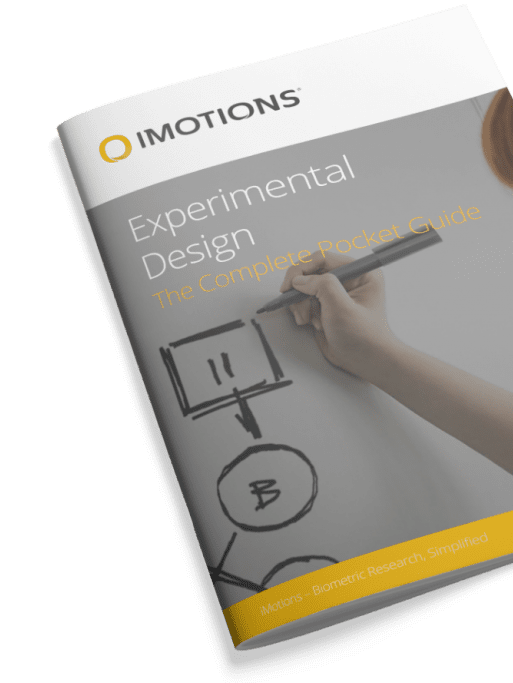
In contrast to the early years of scientific research, modern-age experiments are not merely results of scientists randomly probing assumptions combined with the pure luck to be at the right place at the right time and observe outcomes.
Today’s scientific insights are the result of careful thinking and experimental planning, proper collecting of data, and drawing of appropriate conclusions.
Experimental Design Example
Researchers use experiments to learn something new about the world, to answer questions or to probe theoretical assumptions.
Typical examples for research questions in human cognitive-behavioral research are:
• How does sensory stimulation affect human attention? How do, for example, moving dot patterns, sounds or electrical stimulation alter our perception of the world?
• What are the changes in human physiology during information uptake? How do heart rate and galvanic skin response, for example, change as we recall correct or incorrect information?
• How does virtual reality compared to real physical environments affect human behavior? Do humans learn faster in the real world compared to VR?
• How does stress affect the interaction with other colleagues or machines in the workplace?
• How does packaging of a product affect shoppers’ frustration levels? Is the new package intuitive to open, and if not, how does it affect the behavior of the person?
• How does the new TV commercial impact on emotional expressions and brand memory? Does gender have an influence on purchase decisions after watching the ad?
• How does a website affect users’ stress levels in terms of galvanic skin response, ECG and facial expressions?
• Which intersections in town cause most frustration in bicyclists?
• What are the aspects in a presidential campaign speech that drive voters’ decisions?
As you can see, research questions can be somewhat generic. Experiments are supposed to clarify these questions in a more standardized framework. In order to do so, several steps are necessary to fine-tune the research question into a more testable form:
Step 1: Phrase a hypothesis
First, the general research question is broken down into a testable hypothesis or several hypotheses. Hypotheses are explicit statements about cause and effect and address what outcomes occur when specific factors are manipulated:

Hypotheses phrase a relationship between one or more independent variables and one or more dependent variables:
•Independent variable
The independent variable (IV) is strategically changed, or manipulated, by the experimenter. IVs are also referred to as factors.
• Dependent variable (DV)
The dependent variable (DV) is measured by the experimenter. Experiments with one DV are called univariate, experiments with two or more DV are called multivariate.
The general research question “How does stress affect the interaction with others? ” might lead to the following hypotheses about how stress (independent variable) affects interaction with others (dependent variable):
1) “Having to reply to 100 or more incoming emails per hour results in reduced verbal interaction with colleagues.”
Independent variable: Number of emails per hour Dependent variable: Number of verbal interactions with colleagues per hour
2) “Sleeping 8 hours or more per night results in increased informal sport activities with colleagues.”
Independent variable : Duration of sleep per night Dependent variable : Number of sport meetups with colleagues per week
3) “Regular physical exercise in the evening results in increased occurrences of smiles when talking to others in business meetings.”
Independent variable : Number of evening sport activities per week Dependent variable : Smile occurrences when talking with others
Hypotheses make the research question more explicit by stating an observable relationship between cause and effect. Hypotheses also determine which stimuli are used and what respondents are exposed to.
A stimulus doesn’t have to be just pictures or tones, much more constitutes a stimulus, for example, questionnaires, websites, videos, speech and conversations with others, visual and proprioceptive input while driving and much more. We will address stimuli in more detail below.
Step 2: Sample Groups
Define sample groups.
After specifying the hypothesis, you need to clarify the respondent group characteristics for your experiment. This step is necessary to exclude side effects that could alter the outcomes of your experimental data collection. Make sure that demographic characteristics such as age, gender, education level, income, marital status, occupation etc. are consistent across the respondent pool. Individual characteristics such as state of health or exposure to certain life events should be considered as they might affect experimental outcomes. For example, mothers might respond differently to a TV ad for baby toys than women without kids. Soldiers suffering from PTSD might respond differently to stress-provoking stimuli than software developers.
Step 3: Assign subjects to groups
In this step, you randomly distribute subjects to the different experimental conditions. For example, for your stress in the workplace study you could create two experimental groups, where group one receives 10 emails per hour, and group two receives 100 emails per hour. You could now analyze how the two groups differ in their social interaction with others within the next 6 hours. Ideally, the assignment to experimental groups is done in a randomized fashion, such that all respondents have the same probability for ending up in the available experimental groups. There should not be any bias to assign specific respondents to one group or the other.
Step 4: Determine sampling frequency.
How often do you want to measure from respondents? Clinical trials typically measure patients’ state of health once per month over the course of several months or years. In usability studies you might ask respondents once at the end of the session several questions, either verbally or via surveys and questionnaires.
However, when you collect cognitive-behavioral data from EEG, EMG, ECG, GSR or other biosensors while respondents are doing a specific task, you are collecting tens to hundreds of data points per second – even though all of these sub-second samples might be used to compute an overall score reflecting a certain cognitive or affective state. We will address later in this guide which sensors are ideal to collect specific cognitive-behavioral metrics.
Step 5: Conduct the experiment and collect data.
In this step, you execute the experimental paradigm according to the selected methods. Make sure to observe, monitor, and report any important moments during data collection. Prior to conducting the experiment, run a pilot test to rule out any issues that might arise during data collection (stimulus was wrong length/non-randomized/not optimal, etc.)
Check out : 7 Tips & Tricks For a Smooth Lab Experience
Step 6: Pre-process data and analyze metrics.
In human cognitive-behavioral research, raw data can consist of self-reports or data from biosensors. Of course, video footage of experimental sessions such as focus groups and interviews also constitute raw data and have to be analyzed using coding schemes. Due to the wide range of statistical methods to analyze raw data and metrics, we will not address this step in the current guide. However, one crucial aspect should be mentioned here: The selection of a specific statistical method for data analysis should always be driven by the original hypothesis and the collected data.
Of course, not all experiments require the precise specification of all of these steps. Sometimes you as a researcher don’t have control of certain factors, or you are lacking access to specific respondent populations. Dependent on the amount of control that you have over the relationship between cause and effect, the following types of experiments can be distinguished:
Types of Experimental design
1. laboratory experiments.
Whenever we speak informally of experiments, lab experiments might come to mind where researchers in white lab coats observe others from behind one-side mirrors, taking minute notes on the performance and behavior of human participants executing key-press tasks in front of somewhat unpredictable machines. In fact, this is how human cognitive-behavioral research started (see the Milgram experiment ).
Gladfully, the days of sterile lab environments are long gone, and you can run your study wearing your favorite sweater. However, a core aspect still holds: Being able to control all factors and conditions that could have an effect. For example, in lab experiments you can select specific respondent groups and assign them to different experimental conditions, determine the precise timing and configuration of all stimuli, and exclude any problematic side-effects.
What you should know about laboratory experiments…
- Precise control of all external and internal factors that could affect experimental outcomes.
- Random assignment of respondents to experimental groups, ideally by means of randomization.
- Allows identification of cause-effect relationships with highest accuracy.
- Since everything is standardized, others can replicate your study, which makes your study more “credible” compared to non-standardized scenarios.
Limitations.
- Controlled experiments do not reflect the real world. Respondents might not respond naturally because the lab doesn’t reflect the natural environment. In technical terms, lab experiments are lacking ecological validity.
- Observer effects might change respondents’ behavior. An experimenter sitting right next to a respondent or observing them via webcam might bias experimental outcomes (read up on the Hawthorne Effect ).
2. Field experiments
In contrast to lab experiments, field experiments are done in the natural surroundings of respondents. While the experimenter manipulates the “cause”-aspect, there’s no control of what else could potentially affect the effects and outcomes (such as the Hofling’s Hospital Experiment based on Milgram‘s work).
Quite often, engineers also conduct field tests of prototypes of soft- and hardware to validate earlier lab tests and to obtain broader feedback from respondents in real life.
What you should know about field experiments…
>> strengths..
- Field experiments reflect real-life scenarios more than lab experiments. They have higher ecological validity
- When experiments are covert and respondents don’t feel observed, the observed behavior is much closer to real life compared to lab settings.
>> Limitations.
- No control over external factors that could potentially affect outcomes. The outcomes are therefore much more varied. More respondents are therefore needed to compensate the variation.
- Difficult to replicate by others.
- Limited ability to obtain informed consent from respondents.
3. Natural experiments.
Natural experiments are pure observation studies in the sense that the experimenter doesn’t have any control. Respondent groups are observed as-is and not strategically assigned to different experimental conditions.
You might want to compare existing iPhone and Android users, people living close to Chernobyl and people living somewhere else, or patients suffering from cancer and healthy populations. In this case, the groups that you’d like to compare already exist by nature – you don’t have to create them.
What you should know about natural experiments…
- Behavior in natural experiments more likely reflects real life.
- Ideal in situations where it would be ethically unacceptable to manipulate the group assignment (e.g., expose respondents to radiation).
- More expensive and time-consuming than lab experiments.
- No control over any factors implies that replication by others is almost impossible.
How can I measure human behavior?
Laboratory, field and natural experiments all have one aspect in common: Insights are accomplished empirically. “Empirical” means that research questions and hypotheses are not answered by mere reflection or thought experiments.
Instead of leaning back in a chair and pondering over the potential outcomes of a thought experiment, researchers in human cognitive-behavioral science accomplish their work by means of active observation and probing of the environment in order to identify the underlying processes as well as the ultimate “driving forces” of human behavior.
Within the last decades, researchers have developed intricate experimental techniques and procedures that have found their way also into commercial testing of emotional, cognitive and attentional effects of new products and services, or how personality traits and problem-solving strategies have an impact on brand likeability and consumer preferences.
Two ways to study Human Behavior
Qualitative studies on human behavior.
Qualitative studies gather observational insights. Examples include the investigation of diary entries, open questionnaires, unstructured interviews or observations. Because nothing is counted or quantified and every observation is described as-is, qualitative data is also referred to as descriptive.
In qualitative field studies or usability studies, for example, researchers directly observe how respondents are using the technology, allowing them to directly ask questions, probe on behavior or potentially even adjust the experimental protocol to incorporate the individual’s behavior. The focus of qualitative studies is primarily on understanding how respondents see the world and why they react in a specific way.
What you should know about qualitative studies…
- Ideal to answer “why” and “how to fix a problem?” questions.
- Focus on individual experience of the respondent.
- Small respondent samples required.
- Knowledge gained in the specific study might not be transferrable to other groups.
- Data collection might take longer per respondent.
- Risk that results are affected by researcher’s biases and preferences.
Typical use cases.
- UX, web and software usability tests (description of user journeys).
- Open-ended interviews and surveys on biographical events.
- Focus groups with / without experimenter present.
Check out: How to Deliver better UX with Emotion Detection
Quantitative studies
Quantitative studies by contrast, quantitative studies characterize the systematic empirical investigation of observable phenomena via statistical, mathematical or computational techniques. In other words, quantitative studies use numbers to describe and characterize human behavior.
Examples for quantitative techniques include structured surveys and interviews, observations with dedicated coding schemes (e.g., counting the number of cigarettes smoked within a day), or physiological measurements from EEG, EMG, ECG, GSR and other sensors producing numerical output. Whenever researchers are using quantitative methods, they translate behavioral observations into countable numbers and statistical outputs. All of this is done to guarantee maximum experimental control.
What you should know about quantitative studies…
- Ideal for answering “how many” and “how much” questions.
- Useful to analyze large respondent groups, focus on entire populations.
- High amount of standardization requires less time than qualitative studies.
- Provides numerical values that can be analyzed statistically.
- Experimenter might miss out phenomena because the measurement tool is too narrow.
- Contextual factors are often ignored or missing.
- Studies are expensive and time-consuming.
- Behavioral observation using coding schemes (e.g., on facial expressions or action occurrences within a certain time frame)
- Structured interviews and surveys containing single- or multiple-choice questions as well as scales.
- Physiological measurements of bodily processes (EEG, EMG, GSR etc.)
Psychology Research with iMotions
The world’s leading human behavior software
Check out: Qualitative vs Quantitative Research
Which numbers could human cognitive-behavioral research potentially use to describe our complex inner workings, our intelligence, personality traits or skill levels? What are measurable indicators of a person being a shopaholic, for example?
Indicators that can be counted might be the average time spent in department stores during a week, the cumulative amount of money laid out for certain lifestyle products, or the number of shoe boxes filling up the closet under the stairs (have a look at our reading tip on measurement and the assignment of numbers or events).
The basic principle is that hidden factors of our personality can be made visible (and therefore measurable) by breaking them into feasible and tangible, graspable and observable units which can be counted numerically. This “making visible” of latent constructs of our personality and identity is referred to as operationalization.
While some measures are more suitable to capture an underlying latent characteristic, others might fail. So the question is, what actually constitutes an appropriate measure?
Measurements to avoid bias
This is generally described with respect to the following criteria:
Objectivity
Objectivity is the most general requirement and reflects the fact that measures should come to the same result no matter who is using them. Also, they should generate the same outcomes independent of the outside influences. For example, a multiple-choice personality questionnaire or survey is objective if it returns the same score irrelevant of whether the participant responds verbally or in written form. Further, the result should be independent of the knowledge or attitude of the experimenter, so that the results are purely driven by the performance of the respondent.
Reliability
A measure is said to have high reliability if it returns the same value under consistent conditions. There are several sub-categories of reliability. For example, “retest reliability” describes the stability of a measure over time, “inter-rater reliability” reflects the amount to which different experimenters give consistent estimates of the same behavior, while “split-half reliability” breaks a test into two and examines to what extent the two halves generate identical results.
This is the final and most crucial criterion. It reflects the extent to which a measure collects what it is supposed to collect. Imagine an experiment where body size is collected to measure its relationship with happiness. Obviously, the measure is both objective and reliable (body size measures are quite consistent irrespective of the person taking the measurement) but it is truly a poor measure with respect to its construct validity (i.e., its capability to truly capture the underlying variable) for happiness.
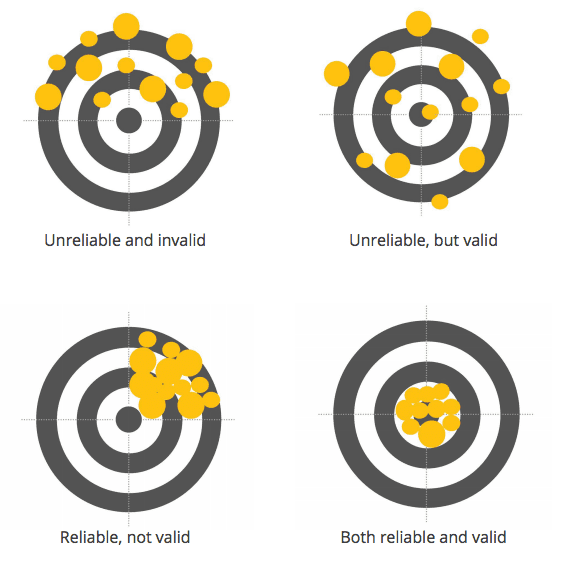
Once you have identified measures that fulfill objectivity, reliability and validity criteria at the same time, you are on the right track to generate experimental outcomes that will push beyond the frontiers of our existing knowledge.
Respondent Management
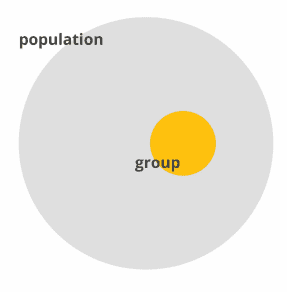
While Iceland has research programs where experiments are applied to the entire nation, other countries and situations do not allow testing everybody. Of course, it would grant maximum insights into your research question, but due to time and resource constraints studies and experiments are generally carried out on respondent groups rather than entire populations.
The most challenging part is to find respondents that truly represent the larger target population allowing you to generalize, or infer, from your study group findings to the population. You might have heard the phrase “representative sample” before. This describes respondent groups where each and every member of the population has an equal chance of being selected for your experiment. Populations don’t necessarily have to be entire countries – the term simply reflects “all people that share certain characteristics” (height, weight, BMI, hemoglobin levels, experience, income, nationality etc.) which are considered relevant for your experiment.
Exemplary populations are:
- Female academics between 30 and 40 years in the US with an average annual income of $50k
- Software developers with more than 5 years of experience in C#
- Patients suffering from secondary progressive Multiple Sclerosis
- After-work shoppers of any age and gender
- Danish mothers up to 50 years
- People wearing glasses
A sample now can be a group of 100 Multiple Sclerosis patients, or 20 dog owners. Finding “representative samples” is not that easy as there is some bias in almost all studies. Samples can be found as following:
Non-random respondent sampling
Non-random sampling can be done during initial pre-screening phases, where generalization is not important. In that case, the experimental outcomes only apply to the tested respondent group. Sampling is done as following:
- Volunteers . You ask people on the street, and whoever agrees to participate is tested.
- Snowball sample . One case identifies others of his kind (e.g., HSE shoppers).
- Convenience sample . You test your co-workers and colleagues or other readily available groups.
- Quota sample . At-will selection of a fixed number from several groups (e.g., 30 male and 30 female respondents).
Random respondent sampling .
Random sampling is actually giving everyone in the population the same chance of being included in your experiment. The benefit of being able to conclude from your research findings obtained from several respondents to the general public comes, however, with high demands on time and resources. The following random sampling strategies exist:
Simple random sampling
In random samples chances for everyone are identical to being included in your test. This means that you had to identify, for example, every female academic between 30 and 40 years in the US with an average annual income of $50k, or every dog owner. Subsequently, you draw random samples and only contact those. Random sampling disallows any selection bias based on volunteering or cooperation.
Systematic sampling
Instead of a completely random selection, you systematically select every nth person from an existing list, for example ordered by respondent age, disease duration, membership, distance etc.
Multistage sampling
Sampling can be done in multiple steps. For example, to find representative students for testing, you can first draw a random selection of counties, then proceed with random drawing of cities, schools, and classes. Finally, you randomly draw students for observation and recording.
Cluster sampling
Particularly for self-reports, studies are carried out on large and geographically dispersed populations. In order to obtain the required number of respondents for testing, clusters may be identified and randomly drawn. Subsuequently, all members of the drawn samples are tested. For example, clustering might be done using households – in this case, all household members are tested, reducing the time and resources for testing massively.
Which sampling method you use is generally determined by feasibility in terms of time and resources. It might often be difficult to obtain truly random samples, particularly in field research. You can find more details on suggested procedures for representative sampling in Banerjee and colleagues (2007; 2010).
How many respondents do I need?
Sampling strategies are closely linked to the sample size of your experiment. If you would like to do a single case study, of course only one respondent is needed. In this case, however, you cannot generalize any findings to the larger population. On the other hand, sampling from the entire population is not possible. The question is, how many respondents are suitable for your experiment? What is the ideal sample size?
Martinez and colleagues (2014) as well as Niles (2011) provide recommendations. Without delving too deep into statistics, the main message is about this: Always collect as many respondents as necessary. For quantitative usability testing 20 respondents might be sufficient, but more respondents should be tested whenever the expected effects are smaller, for example, if there’s only subtle differences between the different stimulus conditions.
This is why academic researchers run studies with dozens to hundreds or thousands of respondents. With more respondents, you reduce the ambiguity of individual variation that could have affected experimental outcomes.Top of Page
The amount of security about your findings is typically expressed with respect to confidence, which is roughly expressed with the following formula:
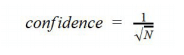
N is the sample size. As you can see, higher respondent samples cause confidence to become smaller (which is the desired outcome). In other words, testing more people gives you more accurate results.
For example, if you tested the preference for a new product with 10 out of 10,000 respondents, then the confidence is at 32%. If 7 out of 10 respondents (70%) liked the new product, the actual proportion in the population could be as low as 48% (70-32) and as high as 100% (70+32, you can’t be above 100). With a variation from 48% to 100%, your test might not be that helpful.
If you increase the sample size to 100 respondents out of 10,000, the confidence is at 10%. With 70 out of 100 respondents liking the product, the actual value in the population is somewhere between 60% and 80%. You’re getting much closer!
If you would like to further reduce the confidence to 5%, you have to test at least 500 randomly-selected respondents. The bottom line is, you have to test lots of respondents before being able to get to conclusions. For more information visit the Creative Research Systems website , where you can find a more exact formula as well as a sample size calculator tool.
Cross-sectional vs. longitudinal designs
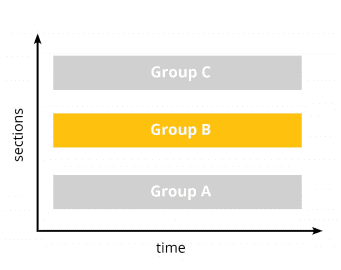
Experimental design and the way your study is carried out depends on the nature of your research question. If you’re interested in how a new TV advertisement is perceived by the general public in terms of attention, cognition and affect, there’s several ways to design your study. Do you want to compare cognitive-behavioral outcomes of the ad among different populations of low and high-income households at the same point in time? Or, do you want to measure the TV ad effects in a single population (say, male high-income shoppers with specific demographic characteristics) over an extended period of time? The former approach is generally referred to as cross-sectional design. The latter is called longitudinal design. The two can further be combined (mixed design)
Cross-sectional design
In cross-sectional studies two or more groups are compared at a single point in time. Similar to taking a snapshot, every respondent is invited and tested just once. In our example, you would show the new TV ad to respondents from low- and high-income households. You would not, however, invite them and show them the TV ad again a week later.
Other examples of cross-sectional studies are:
- Gaming. Compare effects of video games on emotional responsiveness of healthy children and children suffering from ADHS.
- Web testing. Compare website usability evaluation of young, middle-aged and senior shoppers.
- Psychology. Compare evaluation of parenting style of mothers and fathers.
The primary benefit of a cross-sectional experimental design is that it allows you to compare many different variables at the same time. You could, for example, investigate the impact of age, gender, experience or educational levels on respondents’ cognitive-emotional evaluation of the TV ad with little or no additional cost. The only thing you have to do is collect the data (for example, by means of interviews or surveys).
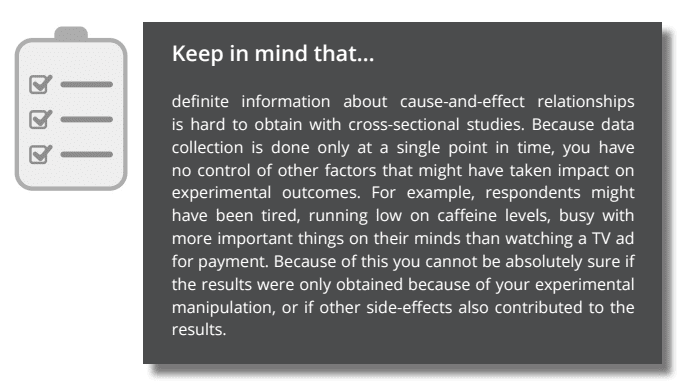
Longitudinal design
In a longitudinal study you conduct several observations of the same respondent group over time, lasting from hours to days, months and many years. By doing this, you establish a sequence of events and minimize the noise that could potentially affect each of the single measurements. In other words, you simply make the outcomes more robust against potential side effects.
For example, you could show a TV ad several times to your group of interest (male high-income shoppers) and see how their preference for the ad changes over time.
Other examples for longitudinal designs are:
- Media / package testing. Two or more media trailers or packages are shown in sequence to a group of respondents who evaluate how much they like each of the presented items.
- Food and flavor testing. Respondents are exposed to two or more flavors presented in sequence and asked for their feedback.
- UI and UX testing. Respondents navigate two or more websites and are interviewed with respect to usability questions.
- Psychology and Training. A group of respondents attending a professional training session answers a questionnaire on emotional well-being before, during and after training.
- Physiology. You monitor EEG, GSR, EMG, facial expressions, etc. while respondents are exposed to pictures, sounds or video stimuli.
The primary benefit of longitudinal designs is that you obtain a time-course of values within one group of respondents. Even if you only obtain cognitive-affective test scores before and after the experimental intervention, you are more likely to understand the impact of the intervention on already existing levels of attention, cognition or affect. Therefore, longitudinal studies are more likely to suggest cause-and-effect relationships than cross-sectional studies.
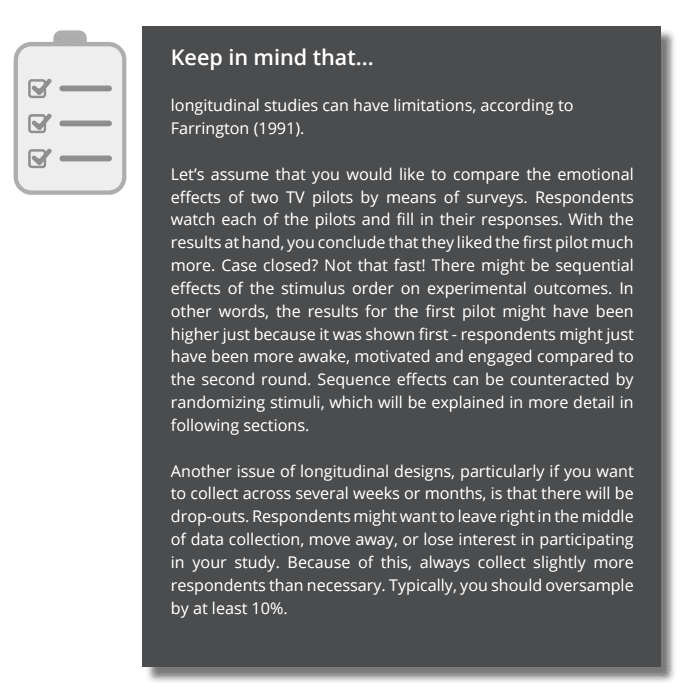
Mixed design
Mixed designs combine the best of two worlds as they allow you to collect longitudinal data across several groups. Strictly spoken, whenever you collect physiological data (like EEG, GSR, EMG, ECG, facial expressions, etc.) from several respondent groups in order to compare different populations, you have a mixed study design. The data itself is longitudinal (several samples over time), while the group comparison has cross-sectional aspects.
Typical examples for mixed designs are:
- Product / media testing. Two or more versions of a product or service are compared with respect to cognitive-behavioral outcomes of two or more groups (e.g., novices and experts, male and female, young and old).
- A-B testing. Two versions of a website or app are compared with respect to cognitive-behavioral outcomes of two or more groups.
Mixed design experiments are ideal for collecting time-courses across several groups of interest, allowing you to investigate the driving forces of human behavior in more detail than cross-sectional or longitudinal designs alone.
Ultimately, which design you choose is driven primarily by your research question. Of course, you can run a cross-sectional study first to get an idea of the potential factors affecting outcomes, and then do a more fine-grained longitudinal study to investigate cause and effect in more detail.
In the next section we will explain in more detail how stimuli should be arranged and which sensors are relevant.
Selecting and arranging stimuli
Experiments in human cognitive-behavior research typically involve some kind of stimulation used to evoke a reaction from respondents. The two most crucial stimulus-related questions are: Which stimuli do I need? In which sequence shall I present the stimuli?
Types of stimuli
Stimuli come in a range of modalities including audio, visual, haptic, olfactory etc. Multimodal stimuli combine several modalities. The following stimuli are used in academic and commercial research studies on human behavior:
- Images / pictures
- Software interfaces
- Devices (car interieur, aircraft cockpit, milkshake machine etc.)
- Communication with others via phone, web or face-to-face
- Complex scenes (VR, real environments)
- Sound (sine waves, complex sound, spoken language, music)
- Olfaction (flavors, smells)
- Haptic stimuli (object exploration by touch, pressure plates, vibrating sensors, haptic robots)
- Questionnaires and surveys (web- or software-based, paper and pencil)
Stimulus sequence
Stimuli are generally presented to respondents in a specific sequence. What are typical sequences used in human cognitive-behavioral research?
Fixed stimulus sequence
Fixed sequences are necessary whenever randomized sequences do not make sense or cannot be employed. For example, when combining a website test with a website-related interview it doesn’t make sense to ask website-related questions first and then tell the respondent to actually use the website.
Here, the only meaningful sequence is to do the website exploration first and the questionnaire second. When it comes to comparing different versions of a stimulus, for example, websites A and B, fixed sequences can also be used.
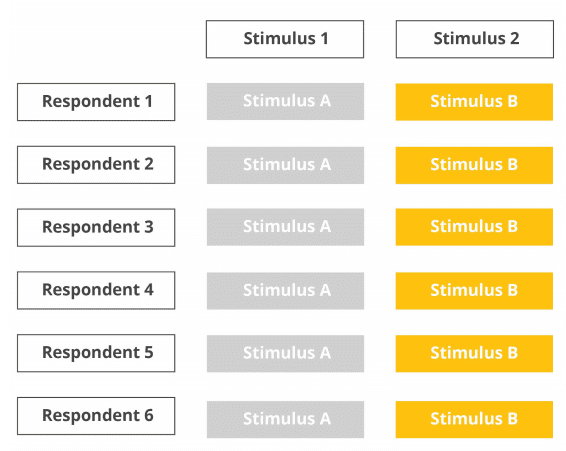
Random stimulus sequence
As you have learned before, presenting stimuli in the same sequence to all respondents bears the risk of sequential effects. Respondents might rate the first stimulus always higher because they are still motivated, engaged and curious.
After two long hours at the lab, exhaustion might take over, so ratings might be low even if the tested product or service exceeds all previous expectations. This can be avoided by presenting stimuli in random order.
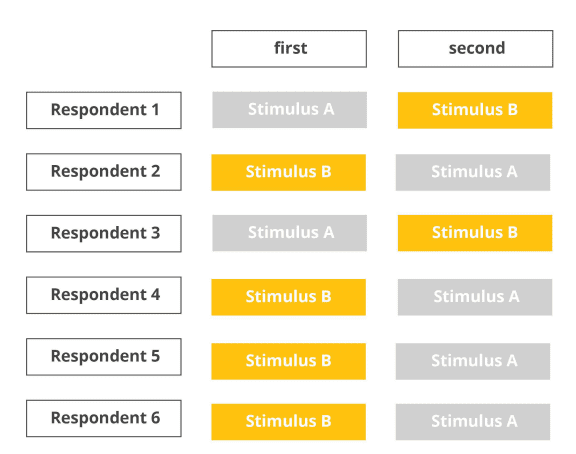
Counterbalanced sequence
To avoid the issues of complete randomization, counterbalanced designs try to achieve an even distribution of conditions across the stimulus slots of the experiment. In the example below, two stimulus conditions A and B are counterbalanced across six respondents, so that three respondents are exposed to stimulus A first, and the other three respondents are exposed to stimulus B first.
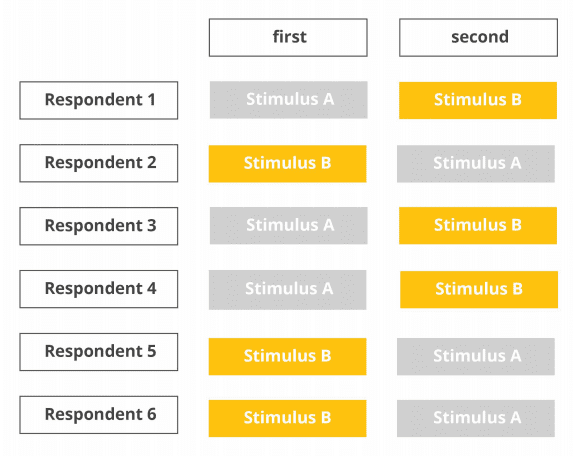
Block design
Sometimes it doesn’t make sense to randomize the entire stimulus list as there might be some internal logic and sequence. Let’s assume you would like to evaluate respondents’ behavior when unpacking several food packages.
For each package, there’s a fixed evaluation protocol where (a) the package is unveiled and (b) respondents are asked to describe their associations verbally. Then, (c) they should pick up the package and open it and (d) describe their experience. This sequence from step (a) to (d) can also be characterized as an experimental “block”, which is supposed to be repeated for all tested packages.
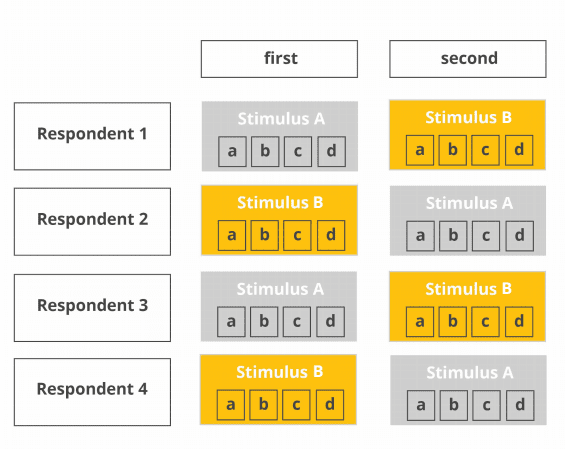
While the package presentation sequence is randomized, the content of each of the blocks stays the same.
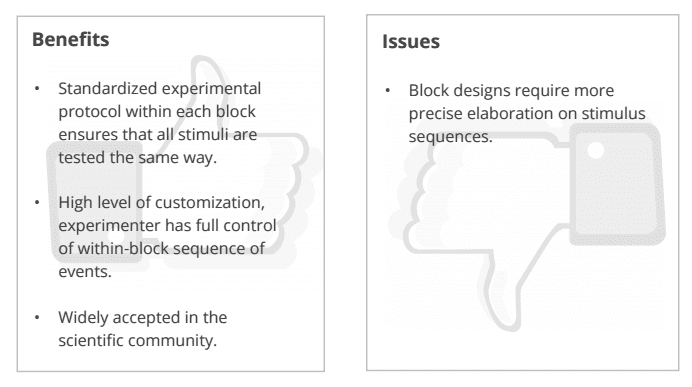
Repeated design
EEG and other physiological recordings sometimes require repeated presentations of the same stimulus. This is necessary because the stimulus-driven changes in brain activity are much smaller compared to the ongoing activity. Presenting the same stimulus several times makes sure that enough data is present to get to valid conclusions.
However, stimulus repetition can also be done for eye tracking studies. In this case, the randomization procedures listed above apply as well.
You might be interested in the number of repetitions necessary to get to results. Unfortunately, this cannot be answered globally, as it depends on several factors such as magnitude of the expected effect/difference between two conditions, stimulus modality, physiological effect of interest, and other factors that take impact on experimental outcomes.
Also, there are strong statistical considerations which are beyond the scope of this general introduction.
Modalities and sensors
Whenever you design experiments for human cognitive-behavior research, you certainly want to consider which biosensors you collect data from. Human behavior is a complex interplay of a variety of different processes, ranging from completely unconscious modulations of emotional reactions to decision-making based on conscious thoughts and cognition. In fact, each of our emotional and cognitive responses is driven by factors such as arousal, workload, and environmental conditions that impact our well-being in that very moment.
All of these aspects of human behavior can be captured by self-reports (via interviews or surveys), specific devices (such as eye trackers, EEG systems, GSR and ECG sensors ) or camera-based facial expression analysis.
TV ads, video games, movies, websites, devices as well as social interaction partners in private life and in the workplace – we could process none of these without our vision. The human brain is fine-tuned for visual input and controlling eye movements. Therefore, it makes immediate sense to collect information on gaze position and pupil dilation from eye tracking. If you present visual stimuli on screen, you should always collect eye tracking data to be absolutely sure where respondents are directing their gaze to and how this is affecting cognitive processing. Second, monitoring pupil dilation can give valuable insights into arousal and stress levels of a respondent. As pupil dilation is an autonomic process, it cannot be controlled consciously. Eye tracking recordings allow you to monitor both respondents’ engagement and motivation as well as arousal levels during the encounter with emotional or cognitively challenging stimuli.
Galvanic skin response (GSR) or electrodermal activity (EDA) reflects the amount of sweat secretion from sweat glands in our skin. Increased sweating results in higher skin conductivity. When exposed to emotional content, we sweat emotionally. GSR recordings in conjunction with EEG are extremely powerful as skin conductance is controlled subconsciously, that is, by deeper and older brain structures than the cognitive processes that are monitored by EEG. Therefore, adding GSR offers tremendous insights into the unfiltered, unbiased emotional arousal of a respondent.
Facial Expression Analysis
With facial expression analysis you can assess if respondents are truly expressing their positive attitude in observable behavior. Facial expression analysis is a non-intrusive method to assess head position and orientation (so you always know where your respondents are positioned relative to the stimulus), expressions (such as lifting of the eyebrows or opening of the mouth) and global facial expressions of basic emotions (joy, anger, surprise etc.) using a webcam placed in front of the respondent. Facial data is extremely helpful to monitor engagement, frustration or drowsiness.
(facial) EMG
Electromyographic sensors monitor the electric energy generated by body movements. EMG sensors can be used to monitor muscular responses of the face, hands or fingers in response to any type of stimulus material. Even subtle activation patterns associated with consciously controlled hand/finger movements (startle reflex) can be assessed with EMG. Collecting synchronized EMG data is relevant for anyone interested in how movements of the eyes and limbs are prepared and executed, but also how movements are prevented and actions are inhibited.
Monitoring heart activity with ECG electrodes attached to the chest or optical heart rate sensors attached to finger tips allows you to track respondents’ physical state, their anxiety and stress levels (arousal), and how changes in physiological state relate to their actions and decisions. Tracking respondents’ physical exhaustion with ECG sensors can provide helpful insights into cognitive-affective processes under bodily straining activity.
Electroencephalography (EEG) is a neuroimaging technique measuring electrical activity generated by the brain from the scalp surface using portable sensors and amplifier systems. It undoubtedly is your means of choice when it comes to assess brain activity associated with perception, cognitive behavior, and emotional processes. EEG reveals substantial insights into sub-second brain dynamics of engagement, motivation, frustration, cognitive workload, and further metrics associated with stimulus processing, action preparation, and execution. Simply put: EEG impressively tells which parts of the brain are active while we perform a task or are exposed to certain stimulus material.
Self-reports
Any experiment should contain self-reported data collection stages, for example at the beginning of the session, during data collection , and at the very end. Gathering demographic data (gender, age, socio-economical status, etc.) helps describing the respondent group in more detail. Also, self-reported data from interviews and surveys helps tremendously to gain insights into the subjective world of the respondents – their self-perceived levels of attention, motivation and engagement – beyond quantitative values reported by biosensors. Of course, survey results can be utilized to segment your respondents into specific groups for analysis (e.g., young vs. old; male vs. female; novice vs. experienced users).
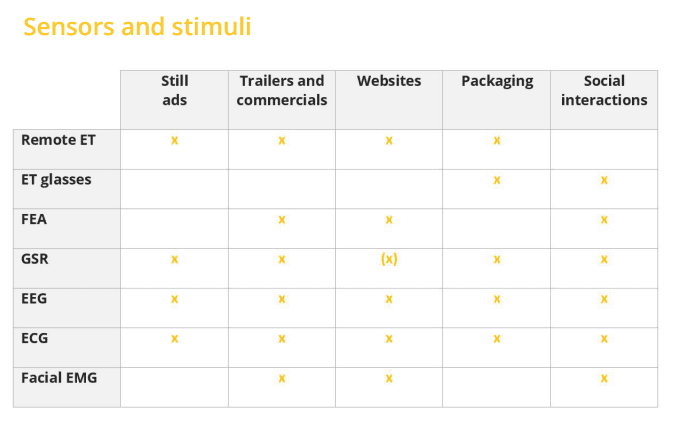
Experimental design done right with iMotions
Properly designed experiments allow you deep insights into attention, cognition and emotional processing of your desired target population when confronted with physical objects or stimuli. Experimental research has come up with dedicated recommendations on how to prevent experimenter or segmentation bias – randomization strategies for respondent and stimulus selection are an excellent starting point.
Before you get started designing your next human cognitive-behavioral experiment, you certainly want to think about how to arrange stimuli, how to select respondents and which biosensors to use in order to gain maximum insights.
What if there was a multimodal software solution that allows for loading and arranging any type of stimuli, for example, in fixed or randomized sequences, while recording data from EEG, eye tracking, facial expression analysis and other biosensors (such as GSR, ECG, EMG) without having to manually piece everything together?
The iMotions Platform
The iMotions Platform is one easy-to-use software solution for study design, multi-sensor calibration, data collection, and analysis.
Out of the box, iMotions supports over 50 leading biosensors including facial expression analysis, GSR, eye tracking, EEG, ECG, and EMG, as well as surveys for multimodal human behavior research.
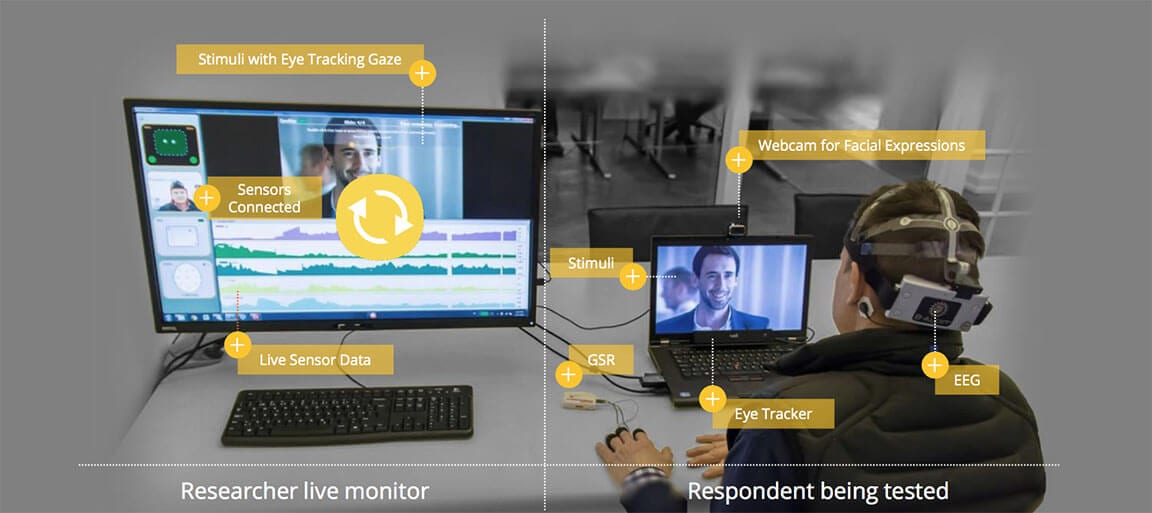
- Banerjee, Chaudhury, et al. (2007). Statistics without tears – inputs for sample size calculations. Indian Psychiatry Journal, 16, 150–152.
- Banerjee & Chaudhury (2010). Statistics without tears: Populations and samples. Industrial Psychiatry Journal, 19(1), 60–65.
- Creative Research Systems (2003). Sample Size Calculator. Retrieved from http://www.surveysystem.com/sscalc.htm on 2016-08-06.
- Cooper, Camic et al. (2012). APA handbook of research methods in psychology, Vol 1: Foundations, planning, measures, and psychometrics.
- Cooper, Camic et al. (2012). APA handbook of research methods in psychology, Vol 2: Research designs: Quantitative, qualitative, neuropsychological, and biological.
- Farrington (1991). Longitudinal research strategies: advantages, problems, and prospects. Journal of the American Academy of Child and Adolescent Psychiatry, 30(3), 369–374.
- Hofling et al. (1966). An experimental study of nurse-physician relationships“. Journal of Nervous and Mental Disease, 143, pp. 171-180.
- McLeod (2007). The Milgram Experiment. Retrieved from www.simplypsychology.org/milgram.html on 2016-07-31.
- Martinez-Mesa, Gonzalez-Chica et al. (2014). Sample size: How many participants do need in my research? Anais Brasileiros de Dermatologia, 89(4), 609–615.
- Monahan & Fisher (2010). Benefits of observer effects: Lessons from the field Qualitative Research, 10(1), pp. 357-376.
- Niles (2014). Sample size: How many survey participants do I need ? Retrieved from http://www.sciencebuddies.org/science-fair-projects/project_ideas/Soc_participants.shtml on 2016-08-06
- Ryan (2006). Modern Experimental Design (2nd edition). New York: Wiley Interscience.
Last edited
About the author
See what is next in human behavior research
Follow our newsletter to get the latest insights and events send to your inbox.
Related Posts
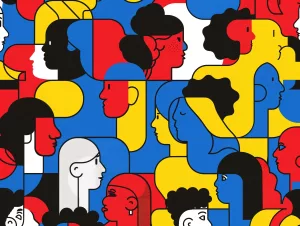
What is Social Behavior?
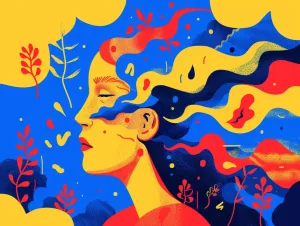
What is Covert Behavior?
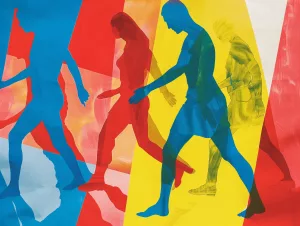
What is Overt Behavior?
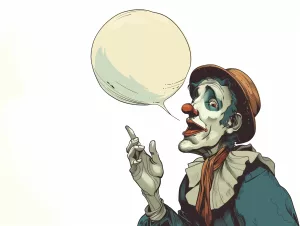
Beyond Words: Behavioral Expressions in Communication
You might also like these.
Human Factors and UX
Work and Safety
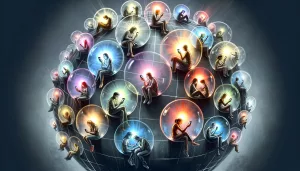
Technological Influences on Behavior: Insights and Implications
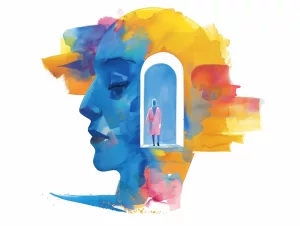
Understanding Human Behavior: An Essential Guide
Case Stories
Explore Blog Categories
Best Practice
Collaboration, product guides, product news, research fundamentals, research insights, 🍪 use of cookies.
We are committed to protecting your privacy and only use cookies to improve the user experience.
Chose which third-party services that you will allow to drop cookies. You can always change your cookie settings via the Cookie Settings link in the footer of the website. For more information read our Privacy Policy.
- gtag This tag is from Google and is used to associate user actions with Google Ad campaigns to measure their effectiveness. Enabling this will load the gtag and allow for the website to share information with Google. This service is essential and can not be disabled.
- Livechat Livechat provides you with direct access to the experts in our office. The service tracks visitors to the website but does not store any information unless consent is given. This service is essential and can not be disabled.
- Pardot Collects information such as the IP address, browser type, and referring URL. This information is used to create reports on website traffic and track the effectiveness of marketing campaigns.
- Third-party iFrames Allows you to see thirdparty iFrames.
Experimental Design: Types, Examples & Methods
Saul Mcleod, PhD
Editor-in-Chief for Simply Psychology
BSc (Hons) Psychology, MRes, PhD, University of Manchester
Saul Mcleod, PhD., is a qualified psychology teacher with over 18 years of experience in further and higher education. He has been published in peer-reviewed journals, including the Journal of Clinical Psychology.
Learn about our Editorial Process
Olivia Guy-Evans, MSc
Associate Editor for Simply Psychology
BSc (Hons) Psychology, MSc Psychology of Education
Olivia Guy-Evans is a writer and associate editor for Simply Psychology. She has previously worked in healthcare and educational sectors.
On This Page:
Experimental design refers to how participants are allocated to different groups in an experiment. Types of design include repeated measures, independent groups, and matched pairs designs.
Probably the most common way to design an experiment in psychology is to divide the participants into two groups, the experimental group and the control group, and then introduce a change to the experimental group, not the control group.
The researcher must decide how he/she will allocate their sample to the different experimental groups. For example, if there are 10 participants, will all 10 participants participate in both groups (e.g., repeated measures), or will the participants be split in half and take part in only one group each?
Three types of experimental designs are commonly used:
1. Independent Measures
Independent measures design, also known as between-groups , is an experimental design where different participants are used in each condition of the independent variable. This means that each condition of the experiment includes a different group of participants.
This should be done by random allocation, ensuring that each participant has an equal chance of being assigned to one group.
Independent measures involve using two separate groups of participants, one in each condition. For example:
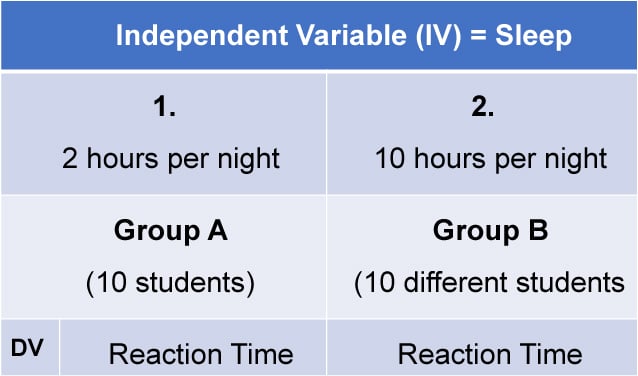
- Con : More people are needed than with the repeated measures design (i.e., more time-consuming).
- Pro : Avoids order effects (such as practice or fatigue) as people participate in one condition only. If a person is involved in several conditions, they may become bored, tired, and fed up by the time they come to the second condition or become wise to the requirements of the experiment!
- Con : Differences between participants in the groups may affect results, for example, variations in age, gender, or social background. These differences are known as participant variables (i.e., a type of extraneous variable ).
- Control : After the participants have been recruited, they should be randomly assigned to their groups. This should ensure the groups are similar, on average (reducing participant variables).
2. Repeated Measures Design
Repeated Measures design is an experimental design where the same participants participate in each independent variable condition. This means that each experiment condition includes the same group of participants.
Repeated Measures design is also known as within-groups or within-subjects design .
- Pro : As the same participants are used in each condition, participant variables (i.e., individual differences) are reduced.
- Con : There may be order effects. Order effects refer to the order of the conditions affecting the participants’ behavior. Performance in the second condition may be better because the participants know what to do (i.e., practice effect). Or their performance might be worse in the second condition because they are tired (i.e., fatigue effect). This limitation can be controlled using counterbalancing.
- Pro : Fewer people are needed as they participate in all conditions (i.e., saves time).
- Control : To combat order effects, the researcher counter-balances the order of the conditions for the participants. Alternating the order in which participants perform in different conditions of an experiment.
Counterbalancing
Suppose we used a repeated measures design in which all of the participants first learned words in “loud noise” and then learned them in “no noise.”
We expect the participants to learn better in “no noise” because of order effects, such as practice. However, a researcher can control for order effects using counterbalancing.
The sample would be split into two groups: experimental (A) and control (B). For example, group 1 does ‘A’ then ‘B,’ and group 2 does ‘B’ then ‘A.’ This is to eliminate order effects.
Although order effects occur for each participant, they balance each other out in the results because they occur equally in both groups.
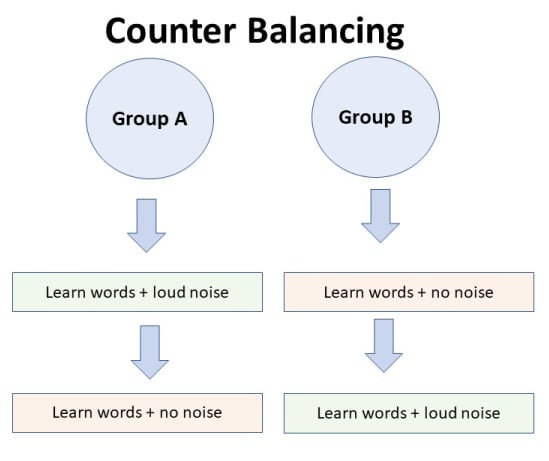
3. Matched Pairs Design
A matched pairs design is an experimental design where pairs of participants are matched in terms of key variables, such as age or socioeconomic status. One member of each pair is then placed into the experimental group and the other member into the control group .
One member of each matched pair must be randomly assigned to the experimental group and the other to the control group.
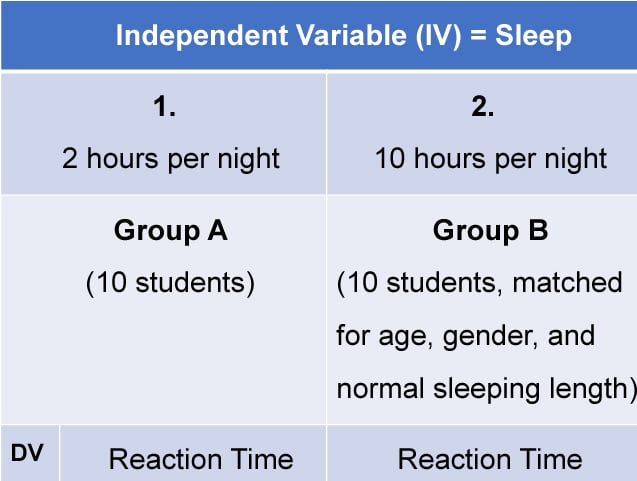
- Con : If one participant drops out, you lose 2 PPs’ data.
- Pro : Reduces participant variables because the researcher has tried to pair up the participants so that each condition has people with similar abilities and characteristics.
- Con : Very time-consuming trying to find closely matched pairs.
- Pro : It avoids order effects, so counterbalancing is not necessary.
- Con : Impossible to match people exactly unless they are identical twins!
- Control : Members of each pair should be randomly assigned to conditions. However, this does not solve all these problems.
Experimental design refers to how participants are allocated to an experiment’s different conditions (or IV levels). There are three types:
1. Independent measures / between-groups : Different participants are used in each condition of the independent variable.
2. Repeated measures /within groups : The same participants take part in each condition of the independent variable.
3. Matched pairs : Each condition uses different participants, but they are matched in terms of important characteristics, e.g., gender, age, intelligence, etc.
Learning Check
Read about each of the experiments below. For each experiment, identify (1) which experimental design was used; and (2) why the researcher might have used that design.
1 . To compare the effectiveness of two different types of therapy for depression, depressed patients were assigned to receive either cognitive therapy or behavior therapy for a 12-week period.
The researchers attempted to ensure that the patients in the two groups had similar severity of depressed symptoms by administering a standardized test of depression to each participant, then pairing them according to the severity of their symptoms.
2 . To assess the difference in reading comprehension between 7 and 9-year-olds, a researcher recruited each group from a local primary school. They were given the same passage of text to read and then asked a series of questions to assess their understanding.
3 . To assess the effectiveness of two different ways of teaching reading, a group of 5-year-olds was recruited from a primary school. Their level of reading ability was assessed, and then they were taught using scheme one for 20 weeks.
At the end of this period, their reading was reassessed, and a reading improvement score was calculated. They were then taught using scheme two for a further 20 weeks, and another reading improvement score for this period was calculated. The reading improvement scores for each child were then compared.
4 . To assess the effect of the organization on recall, a researcher randomly assigned student volunteers to two conditions.
Condition one attempted to recall a list of words that were organized into meaningful categories; condition two attempted to recall the same words, randomly grouped on the page.
Experiment Terminology
Ecological validity.
The degree to which an investigation represents real-life experiences.
Experimenter effects
These are the ways that the experimenter can accidentally influence the participant through their appearance or behavior.
Demand characteristics
The clues in an experiment lead the participants to think they know what the researcher is looking for (e.g., the experimenter’s body language).
Independent variable (IV)
The variable the experimenter manipulates (i.e., changes) is assumed to have a direct effect on the dependent variable.
Dependent variable (DV)
Variable the experimenter measures. This is the outcome (i.e., the result) of a study.
Extraneous variables (EV)
All variables which are not independent variables but could affect the results (DV) of the experiment. Extraneous variables should be controlled where possible.
Confounding variables
Variable(s) that have affected the results (DV), apart from the IV. A confounding variable could be an extraneous variable that has not been controlled.
Random Allocation
Randomly allocating participants to independent variable conditions means that all participants should have an equal chance of taking part in each condition.
The principle of random allocation is to avoid bias in how the experiment is carried out and limit the effects of participant variables.

Order effects
Changes in participants’ performance due to their repeating the same or similar test more than once. Examples of order effects include:
(i) practice effect: an improvement in performance on a task due to repetition, for example, because of familiarity with the task;
(ii) fatigue effect: a decrease in performance of a task due to repetition, for example, because of boredom or tiredness.

- Skip to main content
- Skip to primary sidebar
- Skip to footer
- QuestionPro

- Solutions Industries Gaming Automotive Sports and events Education Government Travel & Hospitality Financial Services Healthcare Cannabis Technology Use Case NPS+ Communities Audience Contactless surveys Mobile LivePolls Member Experience GDPR Positive People Science 360 Feedback Surveys
- Resources Blog eBooks Survey Templates Case Studies Training Help center

Home Market Research
Experimental Research: What it is + Types of designs
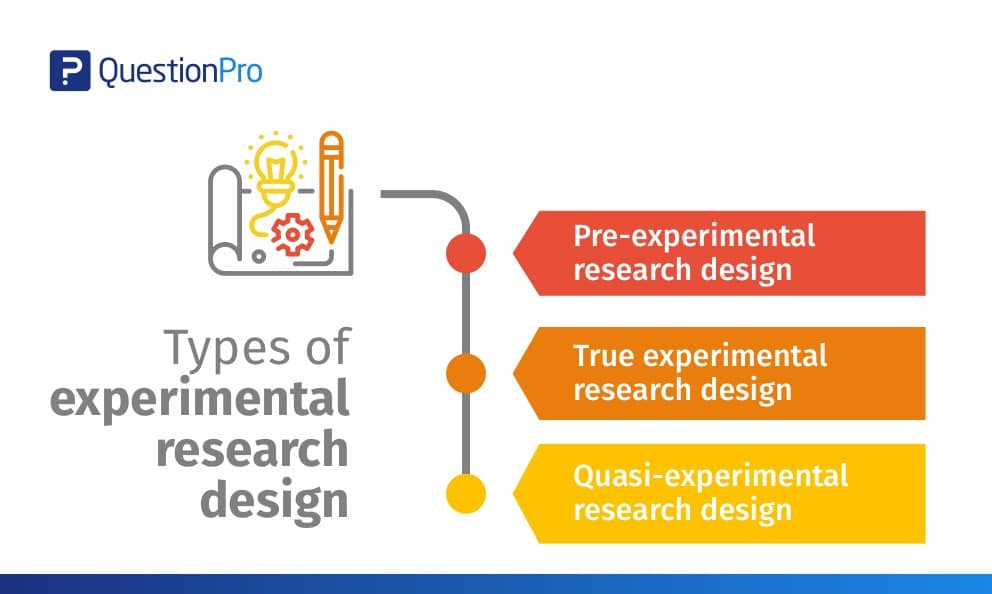
Any research conducted under scientifically acceptable conditions uses experimental methods. The success of experimental studies hinges on researchers confirming the change of a variable is based solely on the manipulation of the constant variable. The research should establish a notable cause and effect.
What is Experimental Research?
Experimental research is a study conducted with a scientific approach using two sets of variables. The first set acts as a constant, which you use to measure the differences of the second set. Quantitative research methods , for example, are experimental.
If you don’t have enough data to support your decisions, you must first determine the facts. This research gathers the data necessary to help you make better decisions.
You can conduct experimental research in the following situations:
- Time is a vital factor in establishing a relationship between cause and effect.
- Invariable behavior between cause and effect.
- You wish to understand the importance of cause and effect.
Experimental Research Design Types
The classic experimental design definition is: “The methods used to collect data in experimental studies.”
There are three primary types of experimental design:
- Pre-experimental research design
- True experimental research design
- Quasi-experimental research design
The way you classify research subjects based on conditions or groups determines the type of research design you should use.
0 1. Pre-Experimental Design
A group, or various groups, are kept under observation after implementing cause and effect factors. You’ll conduct this research to understand whether further investigation is necessary for these particular groups.
You can break down pre-experimental research further into three types:
- One-shot Case Study Research Design
- One-group Pretest-posttest Research Design
- Static-group Comparison
0 2. True Experimental Design
It relies on statistical analysis to prove or disprove a hypothesis, making it the most accurate form of research. Of the types of experimental design, only true design can establish a cause-effect relationship within a group. In a true experiment, three factors need to be satisfied:
- There is a Control Group, which won’t be subject to changes, and an Experimental Group, which will experience the changed variables.
- A variable that can be manipulated by the researcher
- Random distribution
This experimental research method commonly occurs in the physical sciences.
0 3. Quasi-Experimental Design
The word “Quasi” indicates similarity. A quasi-experimental design is similar to an experimental one, but it is not the same. The difference between the two is the assignment of a control group. In this research, an independent variable is manipulated, but the participants of a group are not randomly assigned. Quasi-research is used in field settings where random assignment is either irrelevant or not required.
Importance of Experimental Design
Experimental research is a powerful tool for understanding cause-and-effect relationships. It allows us to manipulate variables and observe the effects, which is crucial for understanding how different factors influence the outcome of a study.
But the importance of experimental research goes beyond that. It’s a critical method for many scientific and academic studies. It allows us to test theories, develop new products, and make groundbreaking discoveries.
For example, this research is essential for developing new drugs and medical treatments. Researchers can understand how a new drug works by manipulating dosage and administration variables and identifying potential side effects.
Similarly, experimental research is used in the field of psychology to test theories and understand human behavior. By manipulating variables such as stimuli, researchers can gain insights into how the brain works and identify new treatment options for mental health disorders.
It is also widely used in the field of education. It allows educators to test new teaching methods and identify what works best. By manipulating variables such as class size, teaching style, and curriculum, researchers can understand how students learn and identify new ways to improve educational outcomes.
In addition, experimental research is a powerful tool for businesses and organizations. By manipulating variables such as marketing strategies, product design, and customer service, companies can understand what works best and identify new opportunities for growth.
Advantages of Experimental Research
When talking about this research, we can think of human life. Babies do their own rudimentary experiments (such as putting objects in their mouths) to learn about the world around them, while older children and teens do experiments at school to learn more about science.
Ancient scientists used this research to prove that their hypotheses were correct. For example, Galileo Galilei and Antoine Lavoisier conducted various experiments to discover key concepts in physics and chemistry. The same is true of modern experts, who use this scientific method to see if new drugs are effective, discover treatments for diseases, and create new electronic devices (among others).
It’s vital to test new ideas or theories. Why put time, effort, and funding into something that may not work?
This research allows you to test your idea in a controlled environment before marketing. It also provides the best method to test your theory thanks to the following advantages:
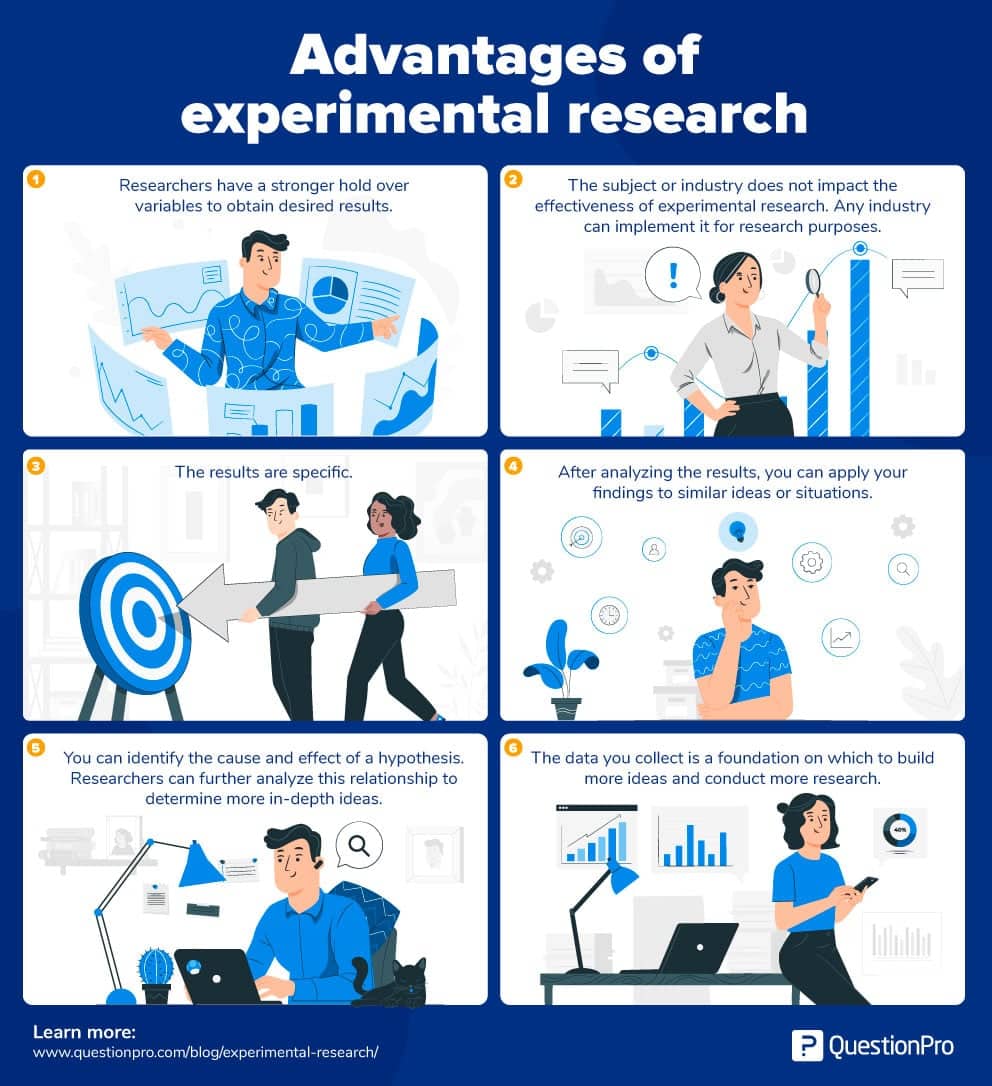
- Researchers have a stronger hold over variables to obtain desired results.
- The subject or industry does not impact the effectiveness of experimental research. Any industry can implement it for research purposes.
- The results are specific.
- After analyzing the results, you can apply your findings to similar ideas or situations.
- You can identify the cause and effect of a hypothesis. Researchers can further analyze this relationship to determine more in-depth ideas.
- Experimental research makes an ideal starting point. The data you collect is a foundation for building more ideas and conducting more action research .
Whether you want to know how the public will react to a new product or if a certain food increases the chance of disease, experimental research is the best place to start. Begin your research by finding subjects using QuestionPro Audience and other tools today.
LEARN MORE FREE TRIAL
MORE LIKE THIS
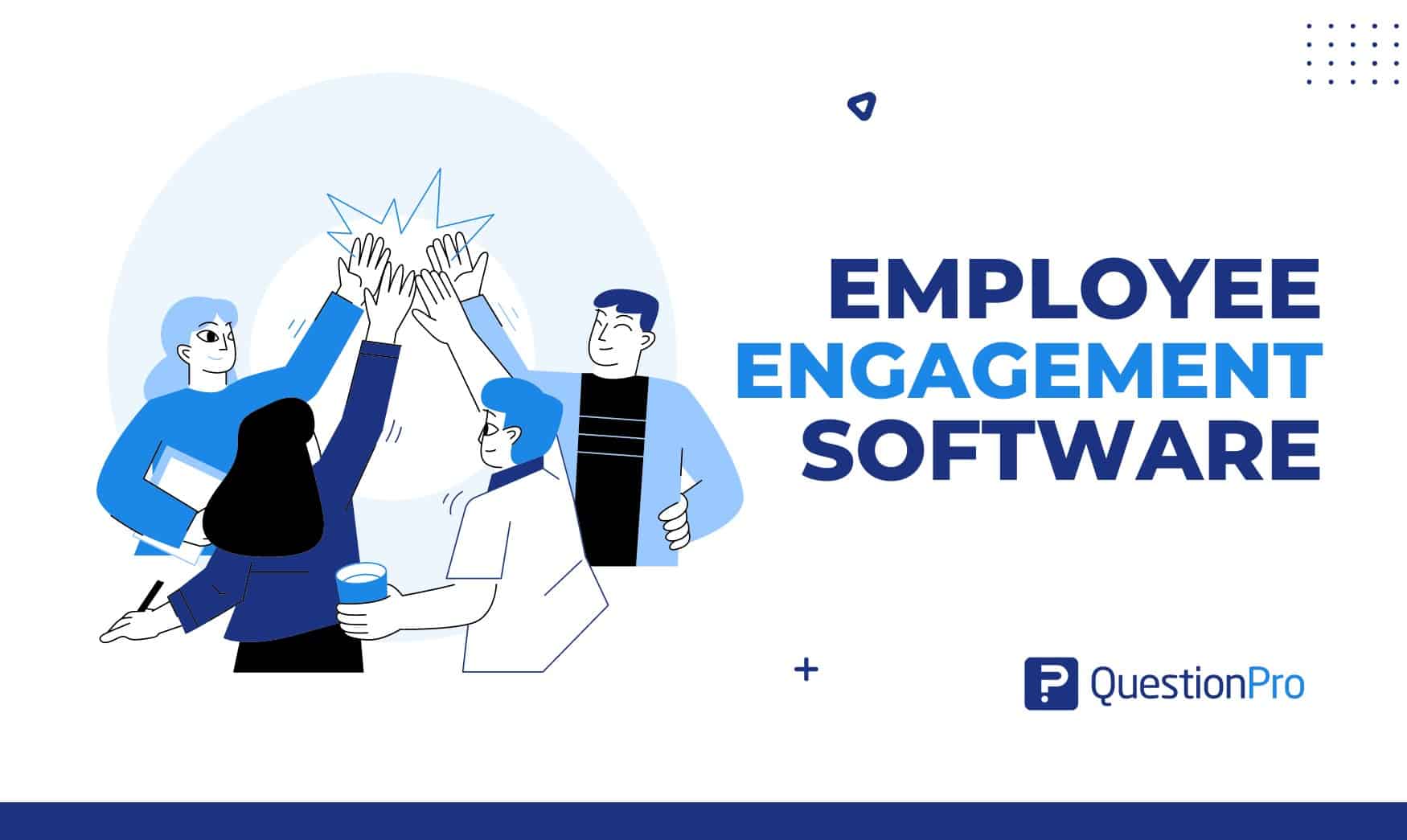
Top 20 Employee Engagement Software Solutions
May 3, 2024
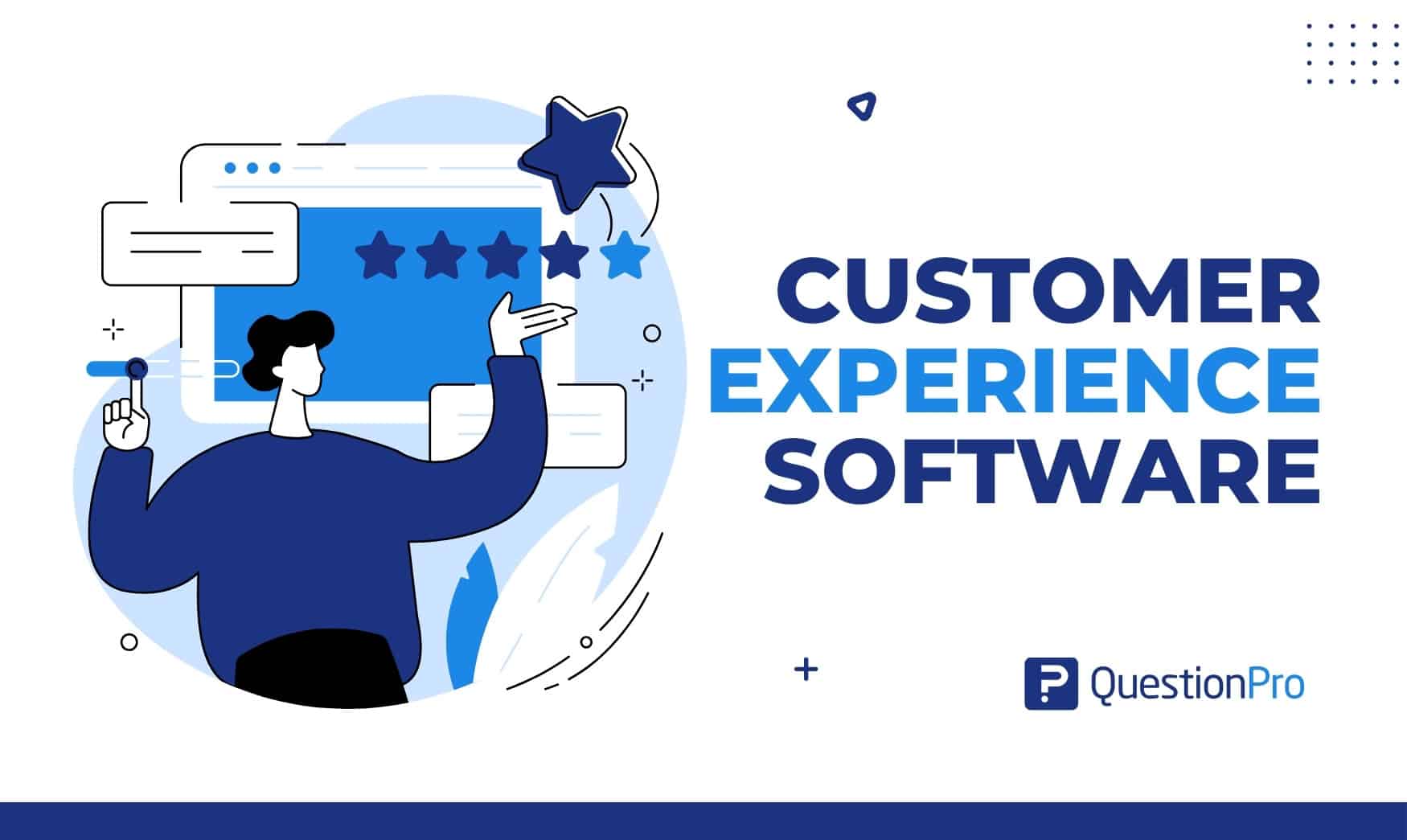
15 Best Customer Experience Software of 2024
May 2, 2024
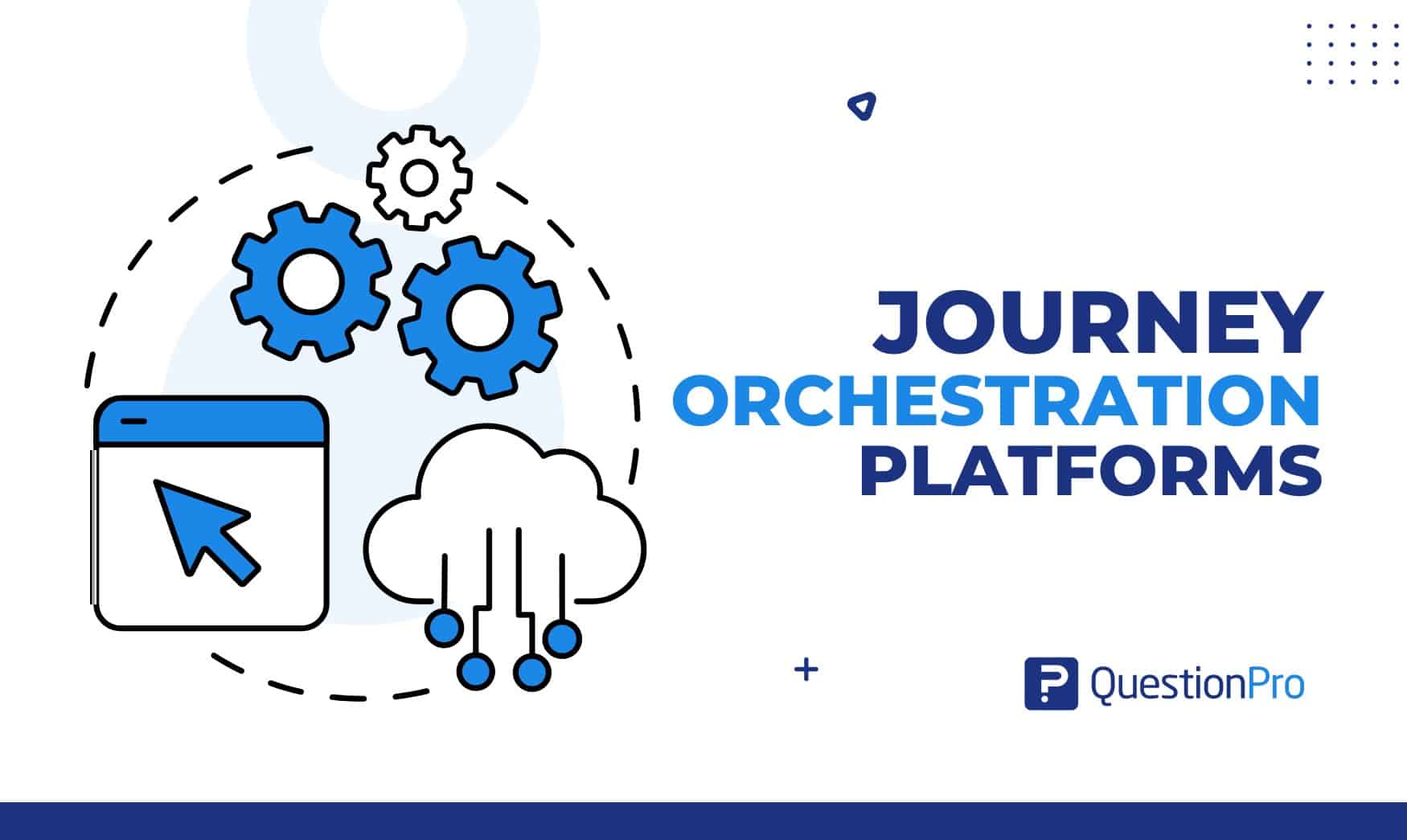
Journey Orchestration Platforms: Top 11 Platforms in 2024
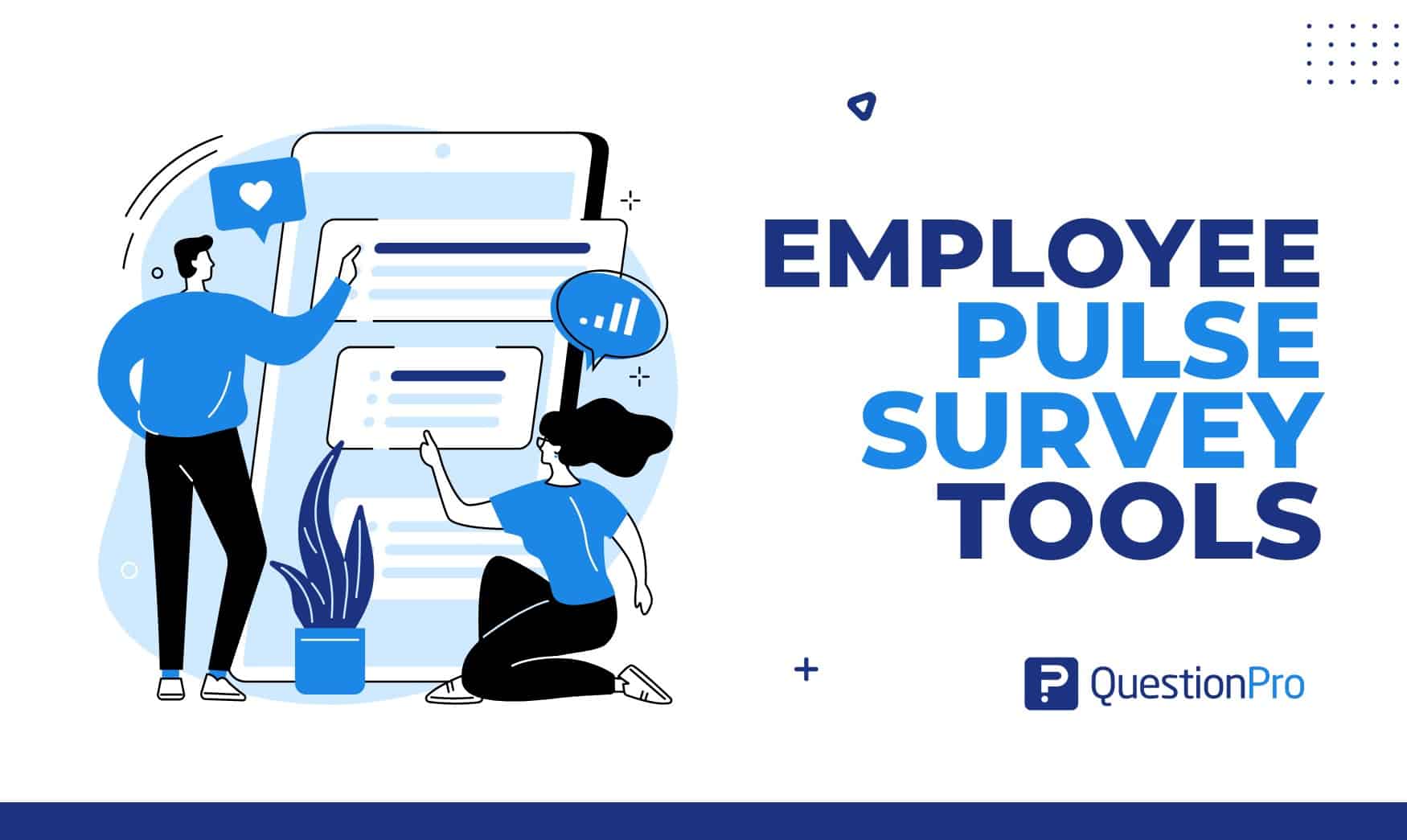
Top 12 Employee Pulse Survey Tools Unlocking Insights in 2024
May 1, 2024
Other categories
- Academic Research
- Artificial Intelligence
- Assessments
- Brand Awareness
- Case Studies
- Communities
- Consumer Insights
- Customer effort score
- Customer Engagement
- Customer Experience
- Customer Loyalty
- Customer Research
- Customer Satisfaction
- Employee Benefits
- Employee Engagement
- Employee Retention
- Friday Five
- General Data Protection Regulation
- Insights Hub
- Life@QuestionPro
- Market Research
- Mobile diaries
- Mobile Surveys
- New Features
- Online Communities
- Question Types
- Questionnaire
- QuestionPro Products
- Release Notes
- Research Tools and Apps
- Revenue at Risk
- Survey Templates
- Training Tips
- Uncategorized
- Video Learning Series
- What’s Coming Up
- Workforce Intelligence
- How it works
A Complete Guide to Experimental Research
Published by Carmen Troy at August 14th, 2021 , Revised On August 25, 2023
A Quick Guide to Experimental Research
Experimental research refers to the experiments conducted in the laboratory or observation under controlled conditions. Researchers try to find out the cause-and-effect relationship between two or more variables.
The subjects/participants in the experiment are selected and observed. They receive treatments such as changes in room temperature, diet, atmosphere, or given a new drug to observe the changes. Experiments can vary from personal and informal natural comparisons. It includes three types of variables ;
- Independent variable
- Dependent variable
- Controlled variable
Before conducting experimental research, you need to have a clear understanding of the experimental design. A true experimental design includes identifying a problem , formulating a hypothesis , determining the number of variables, selecting and assigning the participants, types of research designs , meeting ethical values, etc.
There are many types of research methods that can be classified based on:
- The nature of the problem to be studied
- Number of participants (individual or groups)
- Number of groups involved (Single group or multiple groups)
- Types of data collection methods (Qualitative/Quantitative/Mixed methods)
- Number of variables (single independent variable/ factorial two independent variables)
- The experimental design
Types of Experimental Research
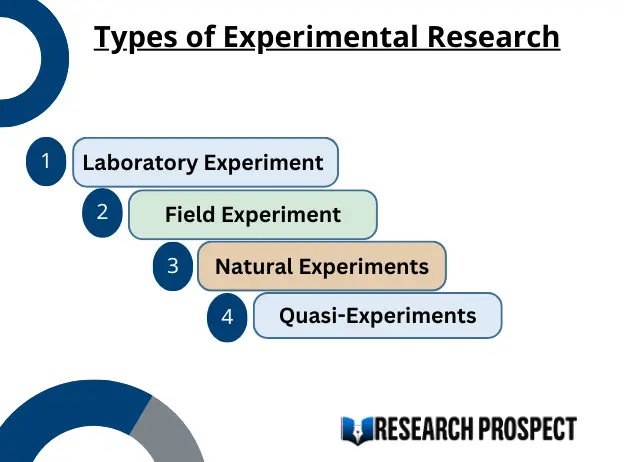
Laboratory Experiment
It is also called experimental research. This type of research is conducted in the laboratory. A researcher can manipulate and control the variables of the experiment.
Example: Milgram’s experiment on obedience.
Field Experiment
Field experiments are conducted in the participants’ open field and the environment by incorporating a few artificial changes. Researchers do not have control over variables under measurement. Participants know that they are taking part in the experiment.
Natural Experiments
The experiment is conducted in the natural environment of the participants. The participants are generally not informed about the experiment being conducted on them.
Examples: Estimating the health condition of the population. Did the increase in tobacco prices decrease the sale of tobacco? Did the usage of helmets decrease the number of head injuries of the bikers?
Quasi-Experiments
A quasi-experiment is an experiment that takes advantage of natural occurrences. Researchers cannot assign random participants to groups.
Example: Comparing the academic performance of the two schools.
Does your Research Methodology Have the Following?
- Great Research/Sources
- Perfect Language
- Accurate Sources
If not, we can help. Our panel of experts makes sure to keep the 3 pillars of Research Methodology strong.
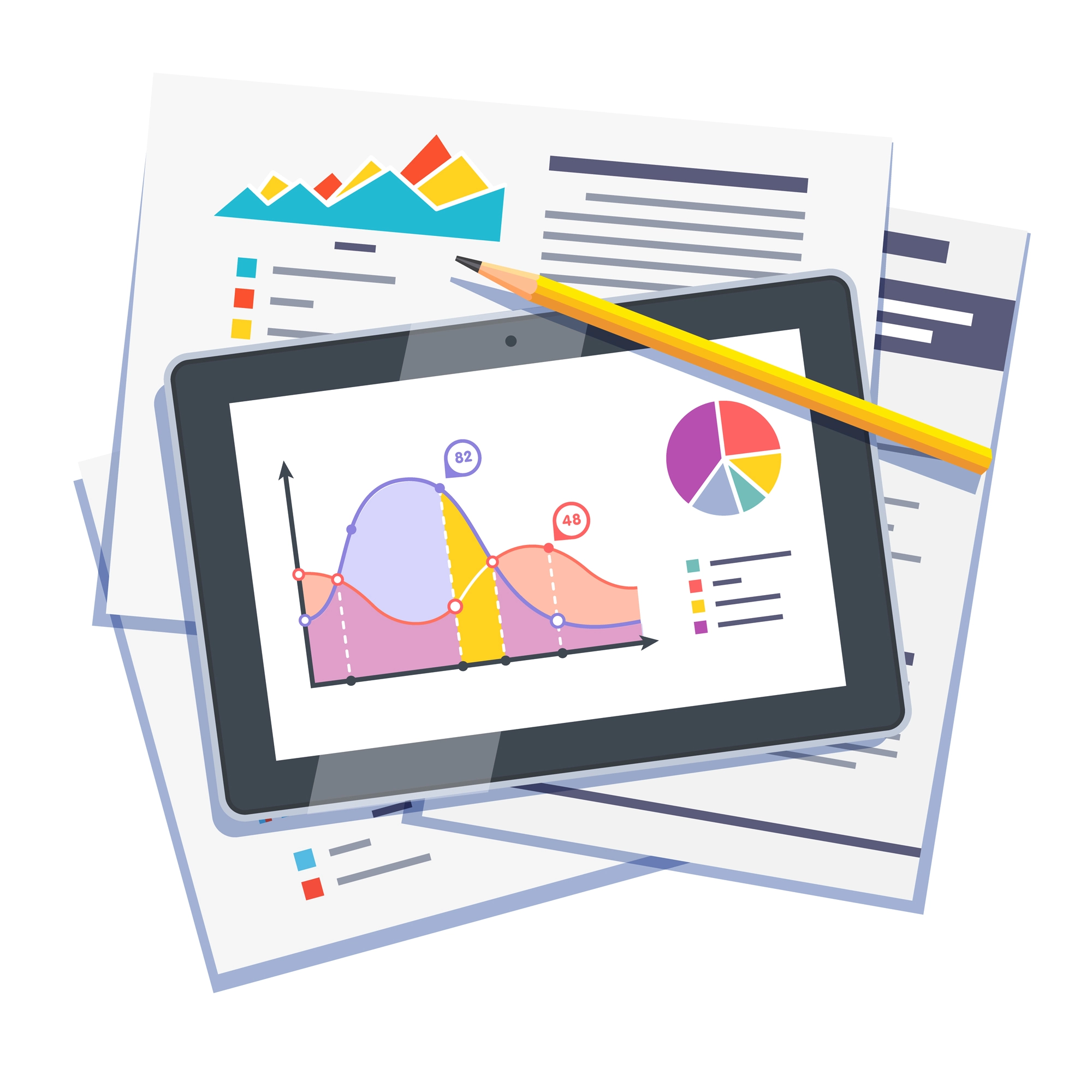
How to Conduct Experimental Research?
Step 1. identify and define the problem.
You need to identify a problem as per your field of study and describe your research question .
Example: You want to know about the effects of social media on the behavior of youngsters. It would help if you found out how much time students spend on the internet daily.
Example: You want to find out the adverse effects of junk food on human health. It would help if you found out how junk food frequent consumption can affect an individual’s health.
Step 2. Determine the Number of Levels of Variables
You need to determine the number of variables . The independent variable is the predictor and manipulated by the researcher. At the same time, the dependent variable is the result of the independent variable.
In the first example, we predicted that increased social media usage negatively correlates with youngsters’ negative behaviour.
In the second example, we predicted the positive correlation between a balanced diet and a good healthy and negative relationship between junk food consumption and multiple health issues.
Step 3. Formulate the Hypothesis
One of the essential aspects of experimental research is formulating a hypothesis . A researcher studies the cause and effect between the independent and dependent variables and eliminates the confounding variables. A null hypothesis is when there is no significant relationship between the dependent variable and the participants’ independent variables. A researcher aims to disprove the theory. H0 denotes it. The Alternative hypothesis is the theory that a researcher seeks to prove. H1or HA denotes it.
Why should you use a Plagiarism Detector for your Paper?
It ensures:
- Original work
- Structure and Clarity
- Zero Spelling Errors
- No Punctuation Faults
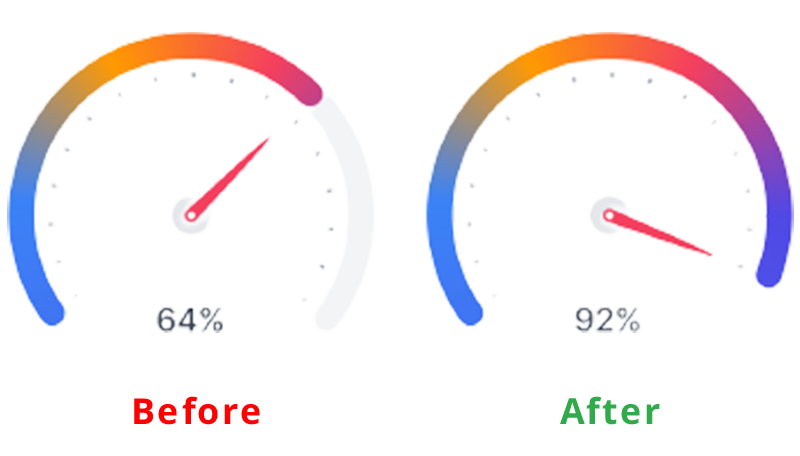
Step 4. Selection and Assignment of the Subjects
It’s an essential feature that differentiates the experimental design from other research designs . You need to select the number of participants based on the requirements of your experiment. Then the participants are assigned to the treatment group. There should be a control group without any treatment to study the outcomes without applying any changes compared to the experimental group.
Randomisation: The participants are selected randomly and assigned to the experimental group. It is known as probability sampling. If the selection is not random, it’s considered non-probability sampling.
Stratified sampling : It’s a type of random selection of the participants by dividing them into strata and randomly selecting them from each level.
Matching: Even though participants are selected randomly, they can be assigned to the various comparison groups. Another procedure for selecting the participants is ‘matching.’ The participants are selected from the controlled group to match the experimental groups’ participants in all aspects based on the dependent variables.
What is Replicability?
When a researcher uses the same methodology and subject groups to carry out the experiments, it’s called ‘replicability.’ The results will be similar each time. Researchers usually replicate their own work to strengthen external validity.
Step 5. Select a Research Design
You need to select a research design according to the requirements of your experiment. There are many types of experimental designs as follows.
Step 6. Meet Ethical and Legal Requirements
- Participants of the research should not be harmed.
- The dignity and confidentiality of the research should be maintained.
- The consent of the participants should be taken before experimenting.
- The privacy of the participants should be ensured.
- Research data should remain confidential.
- The anonymity of the participants should be ensured.
- The rules and objectives of the experiments should be followed strictly.
- Any wrong information or data should be avoided.
Tips for Meeting the Ethical Considerations
To meet the ethical considerations, you need to ensure that.
- Participants have the right to withdraw from the experiment.
- They should be aware of the required information about the experiment.
- It would help if you avoided offensive or unacceptable language while framing the questions of interviews, questionnaires, or Focus groups.
- You should ensure the privacy and anonymity of the participants.
- You should acknowledge the sources and authors in your dissertation using any referencing styles such as APA/MLA/Harvard referencing style.
Step 7. Collect and Analyse Data.
Collect the data by using suitable data collection according to your experiment’s requirement, such as observations, case studies , surveys , interviews , questionnaires, etc. Analyse the obtained information.
Step 8. Present and Conclude the Findings of the Study.
Write the report of your research. Present, conclude, and explain the outcomes of your study .
Frequently Asked Questions
What is the first step in conducting an experimental research.
The first step in conducting experimental research is to define your research question or hypothesis. Clearly outline the purpose and expectations of your experiment to guide the entire research process.
You May Also Like
The technique of ANOVA helps in identifying how independent variables affect dependent variables. By carrying out this process, you can figure out whether you should reject a null hypothesis or accept the alternate hypothesis.
Interval data is a type of discrete data that can be calculated along a scale where every point is placed at an equal interval from another, just as the name explains itself.
Action research for my dissertation?, A brief overview of action research as a responsive, action-oriented, participative and reflective research technique.
USEFUL LINKS
LEARNING RESOURCES
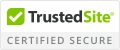
COMPANY DETAILS
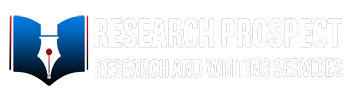
- How It Works
- Privacy Policy

Home » Experimental Design – Types, Methods, Guide
Experimental Design – Types, Methods, Guide
Table of Contents

Experimental Design
Experimental design is a process of planning and conducting scientific experiments to investigate a hypothesis or research question. It involves carefully designing an experiment that can test the hypothesis, and controlling for other variables that may influence the results.
Experimental design typically includes identifying the variables that will be manipulated or measured, defining the sample or population to be studied, selecting an appropriate method of sampling, choosing a method for data collection and analysis, and determining the appropriate statistical tests to use.
Types of Experimental Design
Here are the different types of experimental design:
Completely Randomized Design
In this design, participants are randomly assigned to one of two or more groups, and each group is exposed to a different treatment or condition.
Randomized Block Design
This design involves dividing participants into blocks based on a specific characteristic, such as age or gender, and then randomly assigning participants within each block to one of two or more treatment groups.
Factorial Design
In a factorial design, participants are randomly assigned to one of several groups, each of which receives a different combination of two or more independent variables.
Repeated Measures Design
In this design, each participant is exposed to all of the different treatments or conditions, either in a random order or in a predetermined order.
Crossover Design
This design involves randomly assigning participants to one of two or more treatment groups, with each group receiving one treatment during the first phase of the study and then switching to a different treatment during the second phase.
Split-plot Design
In this design, the researcher manipulates one or more variables at different levels and uses a randomized block design to control for other variables.
Nested Design
This design involves grouping participants within larger units, such as schools or households, and then randomly assigning these units to different treatment groups.
Laboratory Experiment
Laboratory experiments are conducted under controlled conditions, which allows for greater precision and accuracy. However, because laboratory conditions are not always representative of real-world conditions, the results of these experiments may not be generalizable to the population at large.
Field Experiment
Field experiments are conducted in naturalistic settings and allow for more realistic observations. However, because field experiments are not as controlled as laboratory experiments, they may be subject to more sources of error.
Experimental Design Methods
Experimental design methods refer to the techniques and procedures used to design and conduct experiments in scientific research. Here are some common experimental design methods:
Randomization
This involves randomly assigning participants to different groups or treatments to ensure that any observed differences between groups are due to the treatment and not to other factors.
Control Group
The use of a control group is an important experimental design method that involves having a group of participants that do not receive the treatment or intervention being studied. The control group is used as a baseline to compare the effects of the treatment group.
Blinding involves keeping participants, researchers, or both unaware of which treatment group participants are in, in order to reduce the risk of bias in the results.
Counterbalancing
This involves systematically varying the order in which participants receive treatments or interventions in order to control for order effects.
Replication
Replication involves conducting the same experiment with different samples or under different conditions to increase the reliability and validity of the results.
This experimental design method involves manipulating multiple independent variables simultaneously to investigate their combined effects on the dependent variable.
This involves dividing participants into subgroups or blocks based on specific characteristics, such as age or gender, in order to reduce the risk of confounding variables.
Data Collection Method
Experimental design data collection methods are techniques and procedures used to collect data in experimental research. Here are some common experimental design data collection methods:
Direct Observation
This method involves observing and recording the behavior or phenomenon of interest in real time. It may involve the use of structured or unstructured observation, and may be conducted in a laboratory or naturalistic setting.
Self-report Measures
Self-report measures involve asking participants to report their thoughts, feelings, or behaviors using questionnaires, surveys, or interviews. These measures may be administered in person or online.
Behavioral Measures
Behavioral measures involve measuring participants’ behavior directly, such as through reaction time tasks or performance tests. These measures may be administered using specialized equipment or software.
Physiological Measures
Physiological measures involve measuring participants’ physiological responses, such as heart rate, blood pressure, or brain activity, using specialized equipment. These measures may be invasive or non-invasive, and may be administered in a laboratory or clinical setting.
Archival Data
Archival data involves using existing records or data, such as medical records, administrative records, or historical documents, as a source of information. These data may be collected from public or private sources.
Computerized Measures
Computerized measures involve using software or computer programs to collect data on participants’ behavior or responses. These measures may include reaction time tasks, cognitive tests, or other types of computer-based assessments.
Video Recording
Video recording involves recording participants’ behavior or interactions using cameras or other recording equipment. This method can be used to capture detailed information about participants’ behavior or to analyze social interactions.
Data Analysis Method
Experimental design data analysis methods refer to the statistical techniques and procedures used to analyze data collected in experimental research. Here are some common experimental design data analysis methods:
Descriptive Statistics
Descriptive statistics are used to summarize and describe the data collected in the study. This includes measures such as mean, median, mode, range, and standard deviation.
Inferential Statistics
Inferential statistics are used to make inferences or generalizations about a larger population based on the data collected in the study. This includes hypothesis testing and estimation.
Analysis of Variance (ANOVA)
ANOVA is a statistical technique used to compare means across two or more groups in order to determine whether there are significant differences between the groups. There are several types of ANOVA, including one-way ANOVA, two-way ANOVA, and repeated measures ANOVA.
Regression Analysis
Regression analysis is used to model the relationship between two or more variables in order to determine the strength and direction of the relationship. There are several types of regression analysis, including linear regression, logistic regression, and multiple regression.
Factor Analysis
Factor analysis is used to identify underlying factors or dimensions in a set of variables. This can be used to reduce the complexity of the data and identify patterns in the data.
Structural Equation Modeling (SEM)
SEM is a statistical technique used to model complex relationships between variables. It can be used to test complex theories and models of causality.
Cluster Analysis
Cluster analysis is used to group similar cases or observations together based on similarities or differences in their characteristics.
Time Series Analysis
Time series analysis is used to analyze data collected over time in order to identify trends, patterns, or changes in the data.
Multilevel Modeling
Multilevel modeling is used to analyze data that is nested within multiple levels, such as students nested within schools or employees nested within companies.
Applications of Experimental Design
Experimental design is a versatile research methodology that can be applied in many fields. Here are some applications of experimental design:
- Medical Research: Experimental design is commonly used to test new treatments or medications for various medical conditions. This includes clinical trials to evaluate the safety and effectiveness of new drugs or medical devices.
- Agriculture : Experimental design is used to test new crop varieties, fertilizers, and other agricultural practices. This includes randomized field trials to evaluate the effects of different treatments on crop yield, quality, and pest resistance.
- Environmental science: Experimental design is used to study the effects of environmental factors, such as pollution or climate change, on ecosystems and wildlife. This includes controlled experiments to study the effects of pollutants on plant growth or animal behavior.
- Psychology : Experimental design is used to study human behavior and cognitive processes. This includes experiments to test the effects of different interventions, such as therapy or medication, on mental health outcomes.
- Engineering : Experimental design is used to test new materials, designs, and manufacturing processes in engineering applications. This includes laboratory experiments to test the strength and durability of new materials, or field experiments to test the performance of new technologies.
- Education : Experimental design is used to evaluate the effectiveness of teaching methods, educational interventions, and programs. This includes randomized controlled trials to compare different teaching methods or evaluate the impact of educational programs on student outcomes.
- Marketing : Experimental design is used to test the effectiveness of marketing campaigns, pricing strategies, and product designs. This includes experiments to test the impact of different marketing messages or pricing schemes on consumer behavior.
Examples of Experimental Design
Here are some examples of experimental design in different fields:
- Example in Medical research : A study that investigates the effectiveness of a new drug treatment for a particular condition. Patients are randomly assigned to either a treatment group or a control group, with the treatment group receiving the new drug and the control group receiving a placebo. The outcomes, such as improvement in symptoms or side effects, are measured and compared between the two groups.
- Example in Education research: A study that examines the impact of a new teaching method on student learning outcomes. Students are randomly assigned to either a group that receives the new teaching method or a group that receives the traditional teaching method. Student achievement is measured before and after the intervention, and the results are compared between the two groups.
- Example in Environmental science: A study that tests the effectiveness of a new method for reducing pollution in a river. Two sections of the river are selected, with one section treated with the new method and the other section left untreated. The water quality is measured before and after the intervention, and the results are compared between the two sections.
- Example in Marketing research: A study that investigates the impact of a new advertising campaign on consumer behavior. Participants are randomly assigned to either a group that is exposed to the new campaign or a group that is not. Their behavior, such as purchasing or product awareness, is measured and compared between the two groups.
- Example in Social psychology: A study that examines the effect of a new social intervention on reducing prejudice towards a marginalized group. Participants are randomly assigned to either a group that receives the intervention or a control group that does not. Their attitudes and behavior towards the marginalized group are measured before and after the intervention, and the results are compared between the two groups.
When to use Experimental Research Design
Experimental research design should be used when a researcher wants to establish a cause-and-effect relationship between variables. It is particularly useful when studying the impact of an intervention or treatment on a particular outcome.
Here are some situations where experimental research design may be appropriate:
- When studying the effects of a new drug or medical treatment: Experimental research design is commonly used in medical research to test the effectiveness and safety of new drugs or medical treatments. By randomly assigning patients to treatment and control groups, researchers can determine whether the treatment is effective in improving health outcomes.
- When evaluating the effectiveness of an educational intervention: An experimental research design can be used to evaluate the impact of a new teaching method or educational program on student learning outcomes. By randomly assigning students to treatment and control groups, researchers can determine whether the intervention is effective in improving academic performance.
- When testing the effectiveness of a marketing campaign: An experimental research design can be used to test the effectiveness of different marketing messages or strategies. By randomly assigning participants to treatment and control groups, researchers can determine whether the marketing campaign is effective in changing consumer behavior.
- When studying the effects of an environmental intervention: Experimental research design can be used to study the impact of environmental interventions, such as pollution reduction programs or conservation efforts. By randomly assigning locations or areas to treatment and control groups, researchers can determine whether the intervention is effective in improving environmental outcomes.
- When testing the effects of a new technology: An experimental research design can be used to test the effectiveness and safety of new technologies or engineering designs. By randomly assigning participants or locations to treatment and control groups, researchers can determine whether the new technology is effective in achieving its intended purpose.
How to Conduct Experimental Research
Here are the steps to conduct Experimental Research:
- Identify a Research Question : Start by identifying a research question that you want to answer through the experiment. The question should be clear, specific, and testable.
- Develop a Hypothesis: Based on your research question, develop a hypothesis that predicts the relationship between the independent and dependent variables. The hypothesis should be clear and testable.
- Design the Experiment : Determine the type of experimental design you will use, such as a between-subjects design or a within-subjects design. Also, decide on the experimental conditions, such as the number of independent variables, the levels of the independent variable, and the dependent variable to be measured.
- Select Participants: Select the participants who will take part in the experiment. They should be representative of the population you are interested in studying.
- Randomly Assign Participants to Groups: If you are using a between-subjects design, randomly assign participants to groups to control for individual differences.
- Conduct the Experiment : Conduct the experiment by manipulating the independent variable(s) and measuring the dependent variable(s) across the different conditions.
- Analyze the Data: Analyze the data using appropriate statistical methods to determine if there is a significant effect of the independent variable(s) on the dependent variable(s).
- Draw Conclusions: Based on the data analysis, draw conclusions about the relationship between the independent and dependent variables. If the results support the hypothesis, then it is accepted. If the results do not support the hypothesis, then it is rejected.
- Communicate the Results: Finally, communicate the results of the experiment through a research report or presentation. Include the purpose of the study, the methods used, the results obtained, and the conclusions drawn.
Purpose of Experimental Design
The purpose of experimental design is to control and manipulate one or more independent variables to determine their effect on a dependent variable. Experimental design allows researchers to systematically investigate causal relationships between variables, and to establish cause-and-effect relationships between the independent and dependent variables. Through experimental design, researchers can test hypotheses and make inferences about the population from which the sample was drawn.
Experimental design provides a structured approach to designing and conducting experiments, ensuring that the results are reliable and valid. By carefully controlling for extraneous variables that may affect the outcome of the study, experimental design allows researchers to isolate the effect of the independent variable(s) on the dependent variable(s), and to minimize the influence of other factors that may confound the results.
Experimental design also allows researchers to generalize their findings to the larger population from which the sample was drawn. By randomly selecting participants and using statistical techniques to analyze the data, researchers can make inferences about the larger population with a high degree of confidence.
Overall, the purpose of experimental design is to provide a rigorous, systematic, and scientific method for testing hypotheses and establishing cause-and-effect relationships between variables. Experimental design is a powerful tool for advancing scientific knowledge and informing evidence-based practice in various fields, including psychology, biology, medicine, engineering, and social sciences.
Advantages of Experimental Design
Experimental design offers several advantages in research. Here are some of the main advantages:
- Control over extraneous variables: Experimental design allows researchers to control for extraneous variables that may affect the outcome of the study. By manipulating the independent variable and holding all other variables constant, researchers can isolate the effect of the independent variable on the dependent variable.
- Establishing causality: Experimental design allows researchers to establish causality by manipulating the independent variable and observing its effect on the dependent variable. This allows researchers to determine whether changes in the independent variable cause changes in the dependent variable.
- Replication : Experimental design allows researchers to replicate their experiments to ensure that the findings are consistent and reliable. Replication is important for establishing the validity and generalizability of the findings.
- Random assignment: Experimental design often involves randomly assigning participants to conditions. This helps to ensure that individual differences between participants are evenly distributed across conditions, which increases the internal validity of the study.
- Precision : Experimental design allows researchers to measure variables with precision, which can increase the accuracy and reliability of the data.
- Generalizability : If the study is well-designed, experimental design can increase the generalizability of the findings. By controlling for extraneous variables and using random assignment, researchers can increase the likelihood that the findings will apply to other populations and contexts.
Limitations of Experimental Design
Experimental design has some limitations that researchers should be aware of. Here are some of the main limitations:
- Artificiality : Experimental design often involves creating artificial situations that may not reflect real-world situations. This can limit the external validity of the findings, or the extent to which the findings can be generalized to real-world settings.
- Ethical concerns: Some experimental designs may raise ethical concerns, particularly if they involve manipulating variables that could cause harm to participants or if they involve deception.
- Participant bias : Participants in experimental studies may modify their behavior in response to the experiment, which can lead to participant bias.
- Limited generalizability: The conditions of the experiment may not reflect the complexities of real-world situations. As a result, the findings may not be applicable to all populations and contexts.
- Cost and time : Experimental design can be expensive and time-consuming, particularly if the experiment requires specialized equipment or if the sample size is large.
- Researcher bias : Researchers may unintentionally bias the results of the experiment if they have expectations or preferences for certain outcomes.
- Lack of feasibility : Experimental design may not be feasible in some cases, particularly if the research question involves variables that cannot be manipulated or controlled.
About the author
Muhammad Hassan
Researcher, Academic Writer, Web developer
You may also like

Questionnaire – Definition, Types, and Examples

Case Study – Methods, Examples and Guide

Observational Research – Methods and Guide

Quantitative Research – Methods, Types and...

Qualitative Research Methods

Explanatory Research – Types, Methods, Guide
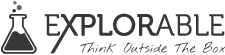
- Foundations
- Write Paper
Search form
- Experiments
- Anthropology
- Self-Esteem
- Social Anxiety

Experimental Research
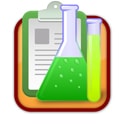
Experimental research is commonly used in sciences such as sociology and psychology, physics, chemistry, biology and medicine etc.
This article is a part of the guide:
- Pretest-Posttest
- Third Variable
- Research Bias
- Independent Variable
- Between Subjects
Browse Full Outline
- 1 Experimental Research
- 2.1 Independent Variable
- 2.2 Dependent Variable
- 2.3 Controlled Variables
- 2.4 Third Variable
- 3.1 Control Group
- 3.2 Research Bias
- 3.3.1 Placebo Effect
- 3.3.2 Double Blind Method
- 4.1 Randomized Controlled Trials
- 4.2 Pretest-Posttest
- 4.3 Solomon Four Group
- 4.4 Between Subjects
- 4.5 Within Subject
- 4.6 Repeated Measures
- 4.7 Counterbalanced Measures
- 4.8 Matched Subjects
It is a collection of research designs which use manipulation and controlled testing to understand causal processes. Generally, one or more variables are manipulated to determine their effect on a dependent variable.
The experimental method is a systematic and scientific approach to research in which the researcher manipulates one or more variables, and controls and measures any change in other variables.
Experimental Research is often used where:
- There is time priority in a causal relationship ( cause precedes effect )
- There is consistency in a causal relationship (a cause will always lead to the same effect)
- The magnitude of the correlation is great.
(Reference: en.wikipedia.org)
The word experimental research has a range of definitions. In the strict sense, experimental research is what we call a true experiment .
This is an experiment where the researcher manipulates one variable, and control/randomizes the rest of the variables. It has a control group , the subjects have been randomly assigned between the groups, and the researcher only tests one effect at a time. It is also important to know what variable(s) you want to test and measure.
A very wide definition of experimental research, or a quasi experiment , is research where the scientist actively influences something to observe the consequences. Most experiments tend to fall in between the strict and the wide definition.
A rule of thumb is that physical sciences, such as physics, chemistry and geology tend to define experiments more narrowly than social sciences, such as sociology and psychology, which conduct experiments closer to the wider definition.
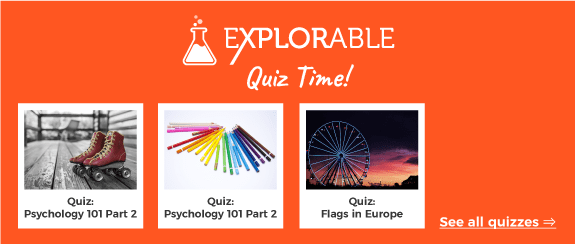
Aims of Experimental Research
Experiments are conducted to be able to predict phenomenons. Typically, an experiment is constructed to be able to explain some kind of causation . Experimental research is important to society - it helps us to improve our everyday lives.
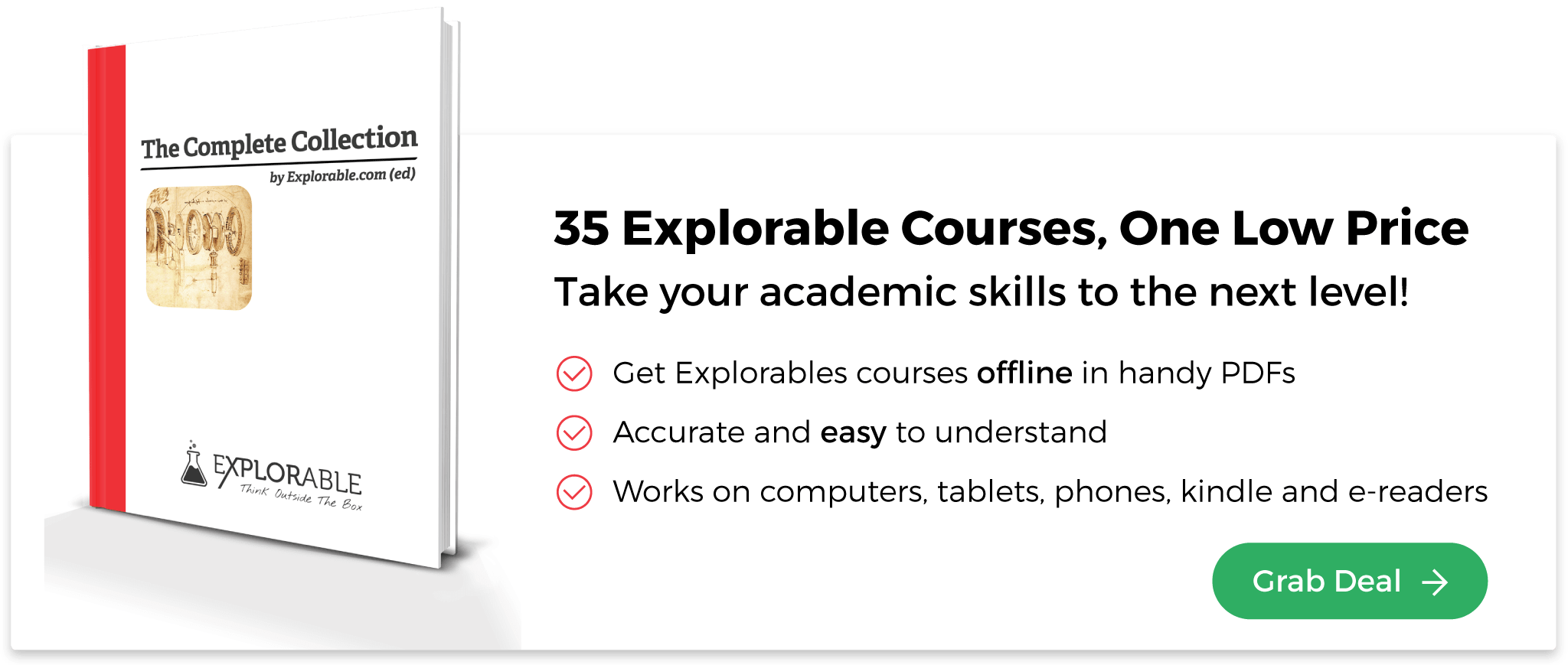
Identifying the Research Problem
After deciding the topic of interest, the researcher tries to define the research problem . This helps the researcher to focus on a more narrow research area to be able to study it appropriately. Defining the research problem helps you to formulate a research hypothesis , which is tested against the null hypothesis .
The research problem is often operationalizationed , to define how to measure the research problem. The results will depend on the exact measurements that the researcher chooses and may be operationalized differently in another study to test the main conclusions of the study.
An ad hoc analysis is a hypothesis invented after testing is done, to try to explain why the contrary evidence. A poor ad hoc analysis may be seen as the researcher's inability to accept that his/her hypothesis is wrong, while a great ad hoc analysis may lead to more testing and possibly a significant discovery.
Constructing the Experiment
There are various aspects to remember when constructing an experiment. Planning ahead ensures that the experiment is carried out properly and that the results reflect the real world, in the best possible way.
Sampling Groups to Study
Sampling groups correctly is especially important when we have more than one condition in the experiment. One sample group often serves as a control group , whilst others are tested under the experimental conditions.
Deciding the sample groups can be done in using many different sampling techniques. Population sampling may chosen by a number of methods, such as randomization , "quasi-randomization" and pairing.
Reducing sampling errors is vital for getting valid results from experiments. Researchers often adjust the sample size to minimize chances of random errors .
Here are some common sampling techniques :
- probability sampling
- non-probability sampling
- simple random sampling
- convenience sampling
- stratified sampling
- systematic sampling
- cluster sampling
- sequential sampling
- disproportional sampling
- judgmental sampling
- snowball sampling
- quota sampling
Creating the Design
The research design is chosen based on a range of factors. Important factors when choosing the design are feasibility, time, cost, ethics, measurement problems and what you would like to test. The design of the experiment is critical for the validity of the results.
Typical Designs and Features in Experimental Design
- Pretest-Posttest Design Check whether the groups are different before the manipulation starts and the effect of the manipulation. Pretests sometimes influence the effect.
- Control Group Control groups are designed to measure research bias and measurement effects, such as the Hawthorne Effect or the Placebo Effect . A control group is a group not receiving the same manipulation as the experimental group. Experiments frequently have 2 conditions, but rarely more than 3 conditions at the same time.
- Randomized Controlled Trials Randomized Sampling, comparison between an Experimental Group and a Control Group and strict control/randomization of all other variables
- Solomon Four-Group Design With two control groups and two experimental groups. Half the groups have a pretest and half do not have a pretest. This to test both the effect itself and the effect of the pretest.
- Between Subjects Design Grouping Participants to Different Conditions
- Within Subject Design Participants Take Part in the Different Conditions - See also: Repeated Measures Design
- Counterbalanced Measures Design Testing the effect of the order of treatments when no control group is available/ethical
- Matched Subjects Design Matching Participants to Create Similar Experimental- and Control-Groups
- Double-Blind Experiment Neither the researcher, nor the participants, know which is the control group. The results can be affected if the researcher or participants know this.
- Bayesian Probability Using bayesian probability to "interact" with participants is a more "advanced" experimental design. It can be used for settings were there are many variables which are hard to isolate. The researcher starts with a set of initial beliefs, and tries to adjust them to how participants have responded
Pilot Study
It may be wise to first conduct a pilot-study or two before you do the real experiment. This ensures that the experiment measures what it should, and that everything is set up right.
Minor errors, which could potentially destroy the experiment, are often found during this process. With a pilot study, you can get information about errors and problems, and improve the design, before putting a lot of effort into the real experiment.
If the experiments involve humans, a common strategy is to first have a pilot study with someone involved in the research, but not too closely, and then arrange a pilot with a person who resembles the subject(s) . Those two different pilots are likely to give the researcher good information about any problems in the experiment.
Conducting the Experiment
An experiment is typically carried out by manipulating a variable, called the independent variable , affecting the experimental group. The effect that the researcher is interested in, the dependent variable(s) , is measured.
Identifying and controlling non-experimental factors which the researcher does not want to influence the effects, is crucial to drawing a valid conclusion. This is often done by controlling variables , if possible, or randomizing variables to minimize effects that can be traced back to third variables . Researchers only want to measure the effect of the independent variable(s) when conducting an experiment , allowing them to conclude that this was the reason for the effect.
Analysis and Conclusions
In quantitative research , the amount of data measured can be enormous. Data not prepared to be analyzed is called "raw data". The raw data is often summarized as something called "output data", which typically consists of one line per subject (or item). A cell of the output data is, for example, an average of an effect in many trials for a subject. The output data is used for statistical analysis, e.g. significance tests, to see if there really is an effect.
The aim of an analysis is to draw a conclusion , together with other observations. The researcher might generalize the results to a wider phenomenon, if there is no indication of confounding variables "polluting" the results.
If the researcher suspects that the effect stems from a different variable than the independent variable, further investigation is needed to gauge the validity of the results. An experiment is often conducted because the scientist wants to know if the independent variable is having any effect upon the dependent variable. Variables correlating are not proof that there is causation .
Experiments are more often of quantitative nature than qualitative nature, although it happens.
Examples of Experiments
This website contains many examples of experiments. Some are not true experiments , but involve some kind of manipulation to investigate a phenomenon. Others fulfill most or all criteria of true experiments.
Here are some examples of scientific experiments:
Social Psychology
- Stanley Milgram Experiment - Will people obey orders, even if clearly dangerous?
- Asch Experiment - Will people conform to group behavior?
- Stanford Prison Experiment - How do people react to roles? Will you behave differently?
- Good Samaritan Experiment - Would You Help a Stranger? - Explaining Helping Behavior
- Law Of Segregation - The Mendel Pea Plant Experiment
- Transforming Principle - Griffith's Experiment about Genetics
- Ben Franklin Kite Experiment - Struck by Lightning
- J J Thomson Cathode Ray Experiment
- Psychology 101
- Flags and Countries
- Capitals and Countries
Oskar Blakstad (Jul 10, 2008). Experimental Research. Retrieved May 08, 2024 from Explorable.com: https://explorable.com/experimental-research
You Are Allowed To Copy The Text
The text in this article is licensed under the Creative Commons-License Attribution 4.0 International (CC BY 4.0) .
This means you're free to copy, share and adapt any parts (or all) of the text in the article, as long as you give appropriate credit and provide a link/reference to this page.
That is it. You don't need our permission to copy the article; just include a link/reference back to this page. You can use it freely (with some kind of link), and we're also okay with people reprinting in publications like books, blogs, newsletters, course-material, papers, wikipedia and presentations (with clear attribution).
Want to stay up to date? Follow us!
Get all these articles in 1 guide.
Want the full version to study at home, take to school or just scribble on?
Whether you are an academic novice, or you simply want to brush up your skills, this book will take your academic writing skills to the next level.
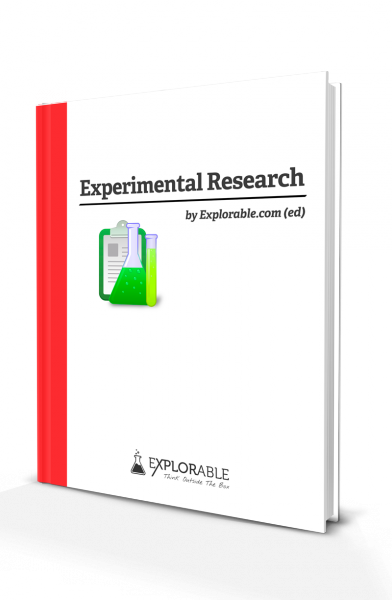
Download electronic versions: - Epub for mobiles and tablets - For Kindle here - For iBooks here - PDF version here
Save this course for later
Don't have time for it all now? No problem, save it as a course and come back to it later.
Footer bottom
- Privacy Policy
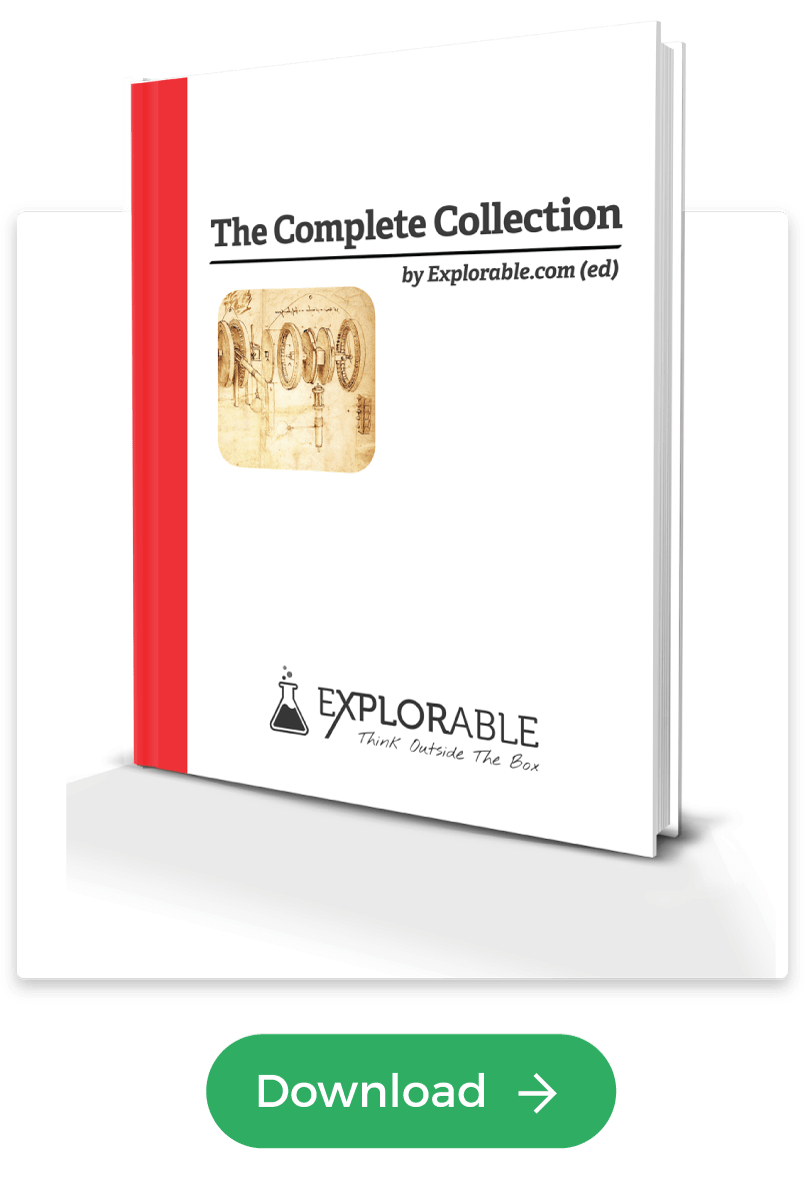
- Subscribe to our RSS Feed
- Like us on Facebook
- Follow us on Twitter

Experimental Research: Meaning And Examples Of Experimental Research
Ever wondered why scientists across the world are being lauded for discovering the Covid-19 vaccine so early? It’s because every…

Ever wondered why scientists across the world are being lauded for discovering the Covid-19 vaccine so early? It’s because every government knows that vaccines are a result of experimental research design and it takes years of collected data to make one. It takes a lot of time to compare formulas and combinations with an array of possibilities across different age groups, genders and physical conditions. With their efficiency and meticulousness, scientists redefined the meaning of experimental research when they discovered a vaccine in less than a year.
What Is Experimental Research?
Characteristics of experimental research design, types of experimental research design, advantages and disadvantages of experimental research, examples of experimental research.
Experimental research is a scientific method of conducting research using two variables: independent and dependent. Independent variables can be manipulated to apply to dependent variables and the effect is measured. This measurement usually happens over a significant period of time to establish conditions and conclusions about the relationship between these two variables.
Experimental research is widely implemented in education, psychology, social sciences and physical sciences. Experimental research is based on observation, calculation, comparison and logic. Researchers collect quantitative data and perform statistical analyses of two sets of variables. This method collects necessary data to focus on facts and support sound decisions. It’s a helpful approach when time is a factor in establishing cause-and-effect relationships or when an invariable behavior is seen between the two.
Now that we know the meaning of experimental research, let’s look at its characteristics, types and advantages.
The hypothesis is at the core of an experimental research design. Researchers propose a tentative answer after defining the problem and then test the hypothesis to either confirm or disregard it. Here are a few characteristics of experimental research:
- Dependent variables are manipulated or treated while independent variables are exerted on dependent variables as an experimental treatment. Extraneous variables are variables generated from other factors that can affect the experiment and contribute to change. Researchers have to exercise control to reduce the influence of these variables by randomization, making homogeneous groups and applying statistical analysis techniques.
- Researchers deliberately operate independent variables on the subject of the experiment. This is known as manipulation.
- Once a variable is manipulated, researchers observe the effect an independent variable has on a dependent variable. This is key for interpreting results.
- A researcher may want multiple comparisons between different groups with equivalent subjects. They may replicate the process by conducting sub-experiments within the framework of the experimental design.
Experimental research is equally effective in non-laboratory settings as it is in labs. It helps in predicting events in an experimental setting. It generalizes variable relationships so that they can be implemented outside the experiment and applied to a wider interest group.
The way a researcher assigns subjects to different groups determines the types of experimental research design .
Pre-experimental Research Design
In a pre-experimental research design, researchers observe a group or various groups to see the effect an independent variable has on the dependent variable to cause change. There is no control group as it is a simple form of experimental research . It’s further divided into three categories:
- A one-shot case study research design is a study where one dependent variable is considered. It’s a posttest study as it’s carried out after treating what presumably caused the change.
- One-group pretest-posttest design is a study that combines both pretest and posttest studies by testing a single group before and after administering the treatment.
- Static-group comparison involves studying two groups by subjecting one to treatment while the other remains static. After post-testing all groups the differences are observed.
This design is practical but lacks in certain areas of true experimental criteria.
True Experimental Research Design
This design depends on statistical analysis to approve or disregard a hypothesis. It’s an accurate design that can be conducted with or without a pretest on a minimum of two dependent variables assigned randomly. It is further classified into three types:
- The posttest-only control group design involves randomly selecting and assigning subjects to two groups: experimental and control. Only the experimental group is treated, while both groups are observed and post-tested to draw a conclusion from the difference between the groups.
- In a pretest-posttest control group design, two groups are randomly assigned subjects. Both groups are presented, the experimental group is treated and both groups are post-tested to measure how much change happened in each group.
- Solomon four-group design is a combination of the previous two methods. Subjects are randomly selected and assigned to four groups. Two groups are tested using each of the previous methods.
True experimental research design should have a variable to manipulate, a control group and random distribution.
With experimental research, we can test ideas in a controlled environment before marketing. It acts as the best method to test a theory as it can help in making predictions about a subject and drawing conclusions. Let’s look at some of the advantages that make experimental research useful:
- It allows researchers to have a stronghold over variables and collect desired results.
- Results are usually specific.
- The effectiveness of the research isn’t affected by the subject.
- Findings from the results usually apply to similar situations and ideas.
- Cause and effect of a hypothesis can be identified, which can be further analyzed for in-depth ideas.
- It’s the ideal starting point to collect data and lay a foundation for conducting further research and building more ideas.
- Medical researchers can develop medicines and vaccines to treat diseases by collecting samples from patients and testing them under multiple conditions.
- It can be used to improve the standard of academics across institutions by testing student knowledge and teaching methods before analyzing the result to implement programs.
- Social scientists often use experimental research design to study and test behavior in humans and animals.
- Software development and testing heavily depend on experimental research to test programs by letting subjects use a beta version and analyzing their feedback.
Even though it’s a scientific method, it has a few drawbacks. Here are a few disadvantages of this research method:
- Human error is a concern because the method depends on controlling variables. Improper implementation nullifies the validity of the research and conclusion.
- Eliminating extraneous variables (real-life scenarios) produces inaccurate conclusions.
- The process is time-consuming and expensive
- In medical research, it can have ethical implications by affecting patients’ well-being.
- Results are not descriptive and subjects can contribute to response bias.
Experimental research design is a sophisticated method that investigates relationships or occurrences among people or phenomena under a controlled environment and identifies the conditions responsible for such relationships or occurrences
Experimental research can be used in any industry to anticipate responses, changes, causes and effects. Here are some examples of experimental research :
- This research method can be used to evaluate employees’ skills. Organizations ask candidates to take tests before filling a post. It is used to screen qualified candidates from a pool of applicants. This allows organizations to identify skills at the time of employment. After training employees on the job, organizations further evaluate them to test impact and improvement. This is a pretest-posttest control group research example where employees are ‘subjects’ and the training is ‘treatment’.
- Educational institutions follow the pre-experimental research design to administer exams and evaluate students at the end of a semester. Students are the dependent variables and lectures are independent. Since exams are conducted at the end and not the beginning of a semester, it’s easy to conclude that it’s a one-shot case study research.
- To evaluate the teaching methods of two teachers, they can be assigned two student groups. After teaching their respective groups on the same topic, a posttest can determine which group scored better and who is better at teaching. This method can have its drawbacks as certain human factors, such as attitudes of students and effectiveness to grasp a subject, may negatively influence results.
Experimental research is considered a standard method that uses observations, simulations and surveys to collect data. One of its unique features is the ability to control extraneous variables and their effects. It’s a suitable method for those looking to examine the relationship between cause and effect in a field setting or in a laboratory. Although experimental research design is a scientific approach, research is not entirely a scientific process. As much as managers need to know what is experimental research , they have to apply the correct research method, depending on the aim of the study.
Harappa’s Thinking Critically program makes you more decisive and lets you think like a leader. It’s a growth-driven course for managers who want to devise and implement sound strategies, freshers looking to build a career and entrepreneurs who want to grow their business. Identify and avoid arguments, communicate decisions and rely on effective decision-making processes in uncertain times. This course teaches critical and clear thinking. It’s packed with problem-solving tools, highly impactful concepts and relatable content. Build an analytical mindset, develop your skills and reap the benefits of critical thinking with Harappa!
Explore Harappa Diaries to learn more about topics such as Main Objective Of Research , Definition Of Qualitative Research , Examples Of Experiential Learning and Collaborative Learning Strategies to upgrade your knowledge and skills.
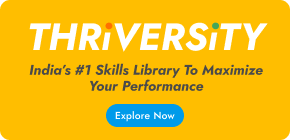
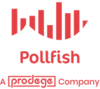
- Pollfish School
- Market Research
- Survey Guides
- Get started
- What is Experimental Research & How is it Significant for Your Business
What is Experimental Research & How is it Significant for Your Business
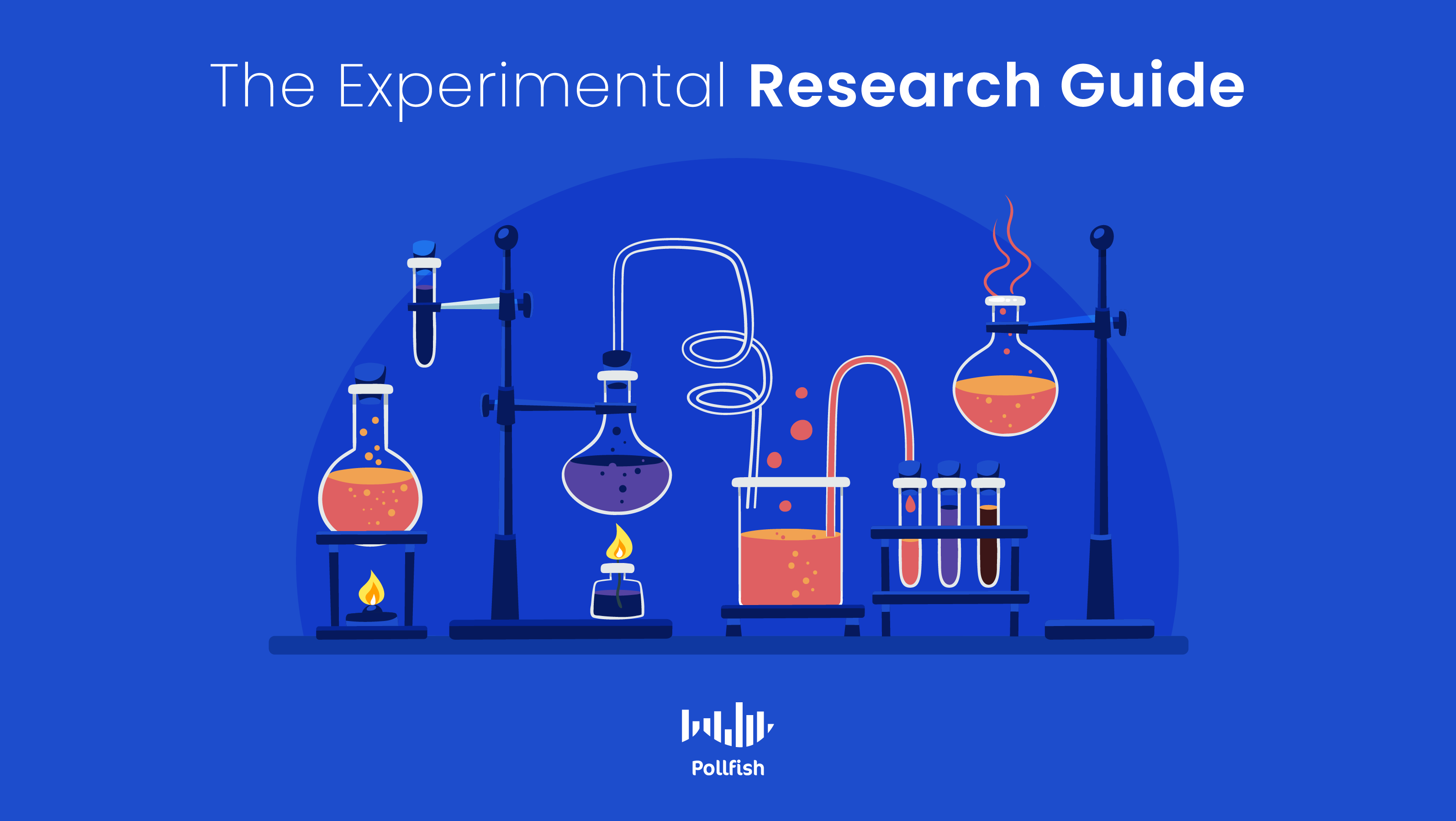
Experimental research uses a scientific method for conducting research, employing the most methodical research design. Known as the gold standard, it involves performing experiments to reach conclusions and can be conducted based on some of the findings from previous forms of research.
Logically, it would follow correlational research, which studies the relationships between variables. It can also follow causal research , a kind of experimental research in itself, as it establishes cause and effect relationships between previously studied variables.
Experimental research is typically used in psychology, physical and social sciences, along with education. However, it too can be applied to business.
This article expounds on experimental research, how it is conducted, how it differs from other forms of research, its key aspects and how survey studies can complement it.
Defining Experimental Research
Experimental research is a kind of study that rigidly follows a scientific research design. It involves testing or attempting to prove a hypothesis by way of experimentation . As such, it uses one or more independent variables, manipulating them and then using them on one or more dependent variables .
In this process, the researchers can measure the effect of the independent variable(s) on the dependent variable(s). This kind of study is performed over some time, so that researchers can form a corroborated conclusion about the two variables.
The experimental research design must be carried out in a controlled environment .
Throughout the experiment, the researcher collects data that can support or refute a hypothesis, thus, this research is also referred to as hypothesis testing or a deductive research method.
The Key Aspects of Experimental Research
There are various attributes that are formative of and unique to experimental research in addition to its main purpose. Understanding these is key to understanding this kind of research in-depth and what to expect when performing it.
The following enumerates the defining characteristics of this kind of research:
- It includes a hypothesis, a variable that will be manipulated by the researcher along with the variable that will be measured and compared .
- The data in this research must be able to be quantified.
- The observation of the subjects, however, must be executed qualitatively.
- The latter is rarer, as it is difficult to manipulate treatments and to control external occurrences in a live setting.
- It relies on making comparisons between two or more groups (the variables).
- Some variables are given an experimental stimulus called a treatment; this is the treatment group.
- The variables that do not receive a stimulus are known as the control group.
- First, researchers must consider how the variables are related and only afterward can they move on to making predictions that can be tested.
- Time is a crucial component when putting forth a cause-and-effect relationship.
- Pre-experimental research design
- True experimental research design
- Quasi-experimental research design
The Three Types of Experimental Research
Experimental research encompasses three subtypes that researchers can implement. They all fall under experimental research, differing in how the subjects are classified. They can be classified based on their conditions or groups.
Pre-experimental research design:
This entails a group or several groups to be observed after factors of cause and effect are implemented.
- Researchers implement this research design when they need to learn whether further investigation is required for these particular groups.
- One-shot Case Study Research Design
- One-group Pretest-posttest Research Design
- Static-group Comparison
Quasi-experimental Research Design
Representing half or pseudo, the moniker “quasi” is used to allude to resembling true experimental research, but not entirely.
- The participants are not randomly assigned, rather they are used when randomization is impossible or impractical.
- Quasi-experimental research is typically used in the education field.
- Examples include: the time series, no equivalent control group design, and the counterbalanced design.
True Experimental Research Design
This kind of experimental research design studies statistical analysis to confirm or debunk a hypothesis.
- It is regarded as the most accurate form of research.
- True experimental research can produce a cause-effect relationship within a group.
- A control group (unaltered) and an experimental group (to undergo changes in variables)
- Random distribution
- Variables can be manipulated
Why Your Business Needs Experimental Research
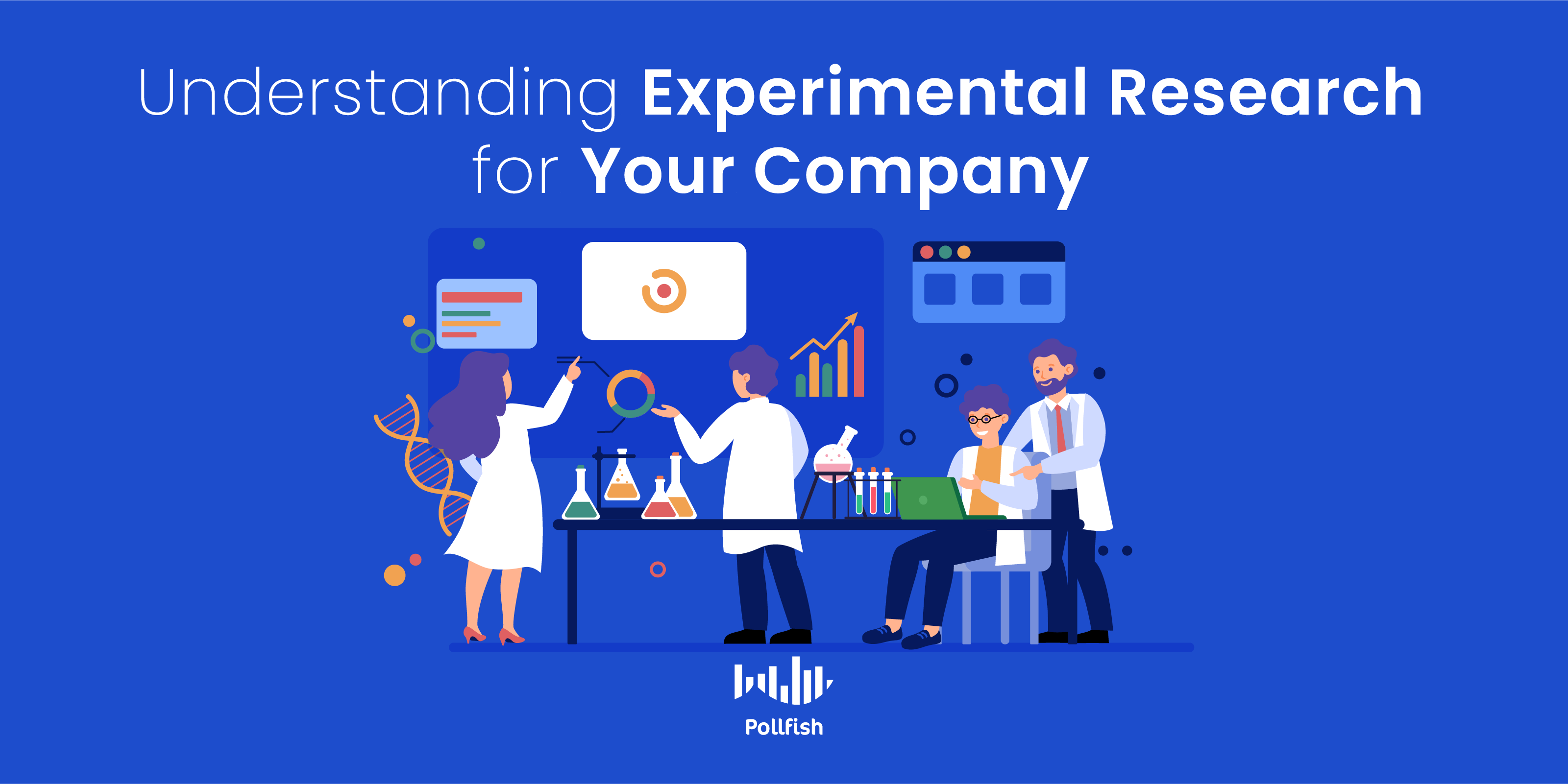
There are various benefits to conducting experimental research for businesses. Firstly, this form of research can help businesses test a new strategy before fully engaging in/ launching it.
The strategy can involve anything from content marketing strategy, to a new product launch. This is especially useful for technology companies, which conduct experimentation frequently. In fact, this kind of research is essential to an R & D (research and development) department.
This makes experimental research a much-needed effort when it comes to spurring innovation. Whether it involves a slight rebranding or an upgrade of products, experimental research guides these campaigns in a science-backed manner.
Secondly, a business must excel in meeting customer needs. Customer experience is an overwhelmingly important side of any business, as customers are willing to make on-the-stop purchases and pay more for a good CX .
As such, each product addition and change in a customer journey must be carried out wisely. Businesses ought to avoid creating unwanted services, or those that cause any aversion within customers. Instead, they should only invest in the most profitable services, products and experiences, a feat that cannot be accomplished solely on guesswork.
Experimenting allows brands to understand customer preferences and changes in their behaviors , as the experiments create stimuli and changes in independent variables.
Additionally, experimental research grants companions an understanding of their business environment. In turn, this helps them predict outcomes, or create hypotheses about outcomes to guide them in further research, if need be. For example, a business may consider testing the reactions of its competitors should it raise its costs on various offers.
Aside from discovering if this yields a profitable change, it can discover how companies in the same niche respond and if those responses drive more sales, etc.
Key Independent Variables
- Digital user experience (DX) such as new site features
- Advertisements
- Marketing activity (SEO, SEM, social media announcements, retargeting, etc.)
- Inventory (new products or upgrades)
- Interactions with sales agents
Key Dependent variables
- VoC feedback (whether positive or negative)
- Site traffic
- In-store visits
- Time spent on a website, bounce rates, etc.
An Example of Experimental Research for Business
Market researchers can apply experimental research to a wide breadth of testing needs. Virtually anything that requires proof, confirmation, or is clouded by uncertainty can put experimentation into practice.
The following is an example of how a business can use this research:
A product manager needs to convince the higher-ups in a denim company to launch a new product line at a particular department store. The objective of this launch is to increase sales, expand the company’s floor presence and widen the offerings.
The manager has to prove that this line is needed in order for the company to pitch the idea to the department store. The product manager can then conduct experimental research to provide a strong case for their theory, that a new line can raise sales.
The product manager performs experimental research by executing a test in a few stores, in which the new line of denim is sold. These stores are varied in location to signify the target market sales before and after the launch. The test runs for a month to determine if the hypothesis (the new line resulting in increased attention and sales) can be proven.
This represents a field experiment. The product manager must heed the sales and foot traffic of the new product line, paying attention to spikes in revenue and overall sales to justify the new line.
Experimental Research Survey Examples
Survey research runs contrary to experimental research, unlike the other main forms of research such as exploratory, descriptive and correlational research. This is because the nature of surveys is observational, while experimental research, as its name signifies, relies on experimentations, that is testing out changes and studying the reactions to the changes.
Despite the contrast of survey research to experimental research, they are not completely at odds. In fact, surveys are a potent method to gain further insight into an existing experiment or understand variables before conducting an experiment in the first place.
As such, businesses can adopt a wide variety of surveys to complement their experimental research. Here are some of the key forms of surveys that work in tandem with experimentation:
- Discovers the aspects of statistical significance within variables.
- Helpful in that causal research is quantitative in essence.
- Delves into past events, occurrences and attitudes in regards to the variables.
- Shows whether the variables changed and how so.
- Can find causative elements between variables over a period of time.
- Useful for formulating hypotheses.
- Helps businesses zero in on variables that contribute to or result from certain kinds of customer experiences.
- Allows businesses to test CX in relation to the responses from this survey.
- Measures various matters critical in a business or organization; surveys employees.
- Deployed more frequently, so variables can always be continually tracked.
- Helps answer the what, why and how with open-ended questions.
- Extracts key high-level information in depth.
How Experimental Research Differs from Correlational, Exploratory, Descriptive and Causal Research
Experimental research differs from exploratory, descriptive and correlational research in self-evident ways. It is, however, often conflated with causal research. However, they too have notable differences.
Causal research involves finding the cause-and-effect relationships between variables. Thus, it too employs experimentation. However, this means that causal research is a form of experimental research, not the other way around.
Experimental research, on the other hand, is fully science and experiment-based, as it chiefly seeks to prove or disprove a hypothesis. While this largely involves studying independent and dependent variables, as it does in causal research, it is not solely based on these aspects. Instead, it can introduce a new variable without knowing the dependent variable or experiment on an entirely new idea (as in the example used in the previous selection).
Causal research looks into the comparison of variable relationships to find a cause and effect, while experimental research states an expected relationship between variables and is bent on testing a hypothesis.
As far as comparisons to correlational research go, while experimental research also studies the relationships between variables, it functions far beyond this by manipulating the variables and virtually all subjects involved in experiments .
On the contrary, correlational research does not apply any alterations or conditioning to variables. Instead, it is a purely observational research method. As such, it merely detects whether there is a correlation between only 2 variables. In contrast, experimental research studies and experiments with several at a time.
Exploratory research is vastly different from experimental research, as it forms the very foundation of a research problem and establishes a hypothesis for further research. As such, it is conducted as the very first kind of research around a new topic and does not fixate on variables.
Descriptive research , like exploratory research and unlike experimental research, is conducted early in the full research process, following exploratory research. Like exploratory research, it seeks to paint a picture of a problem or phenomenon , as it zeros in an already-established issue and delves further, in pursuit of all the details and conditions surrounding it.
Thus, unlike experimental research, it only observes; it does not manipulate variables in any capacity or setting.
The Advantages and Disadvantages of Experimental Research
Experimental research offers several benefits for researchers and businesses. However, as with all other research methods, it too carries a few disadvantages that researchers should be aware of.
The Advantages
- Researchers have a full level of control in an experiment.
- It can be used in a wide variety of fields and verticals.
- The results are specific and conclusive.
- The results allow researchers to apply their findings to similar phenomena or contexts.
- It can determine the validity of a hypothesis, or disprove one.
- Researchers can manipulate variables and use them in as many variations as they desire without tarnishing the validity of the research.
- It discovers the cause and effect among variables.
- Researchers can further analyze relationships through testing.
- It helps researchers understand a specific environment fully.
- The studies can be replicated so that the researchers can repeat their experiments to test other variables or confirm the results again.
The Disadvantages
- It involves a lot of resources, time and money, as such, it is not easy to conduct.
- It can form artificial environments when researchers unwittingly over-manipulate variables as a means of duplicating real-world instances.
- It is vulnerable to flaws in the methodology, along with other mistakes that can’t always be predicted.
- Flawed experiments may require researchers to start their experiments anew to avoid false calculations, measuring results from artificial scenarios or other mistakes.
- Some variables cannot be manipulated and some forms of research experiments are too impractical to conduct.
How to Conduct Experimental Research
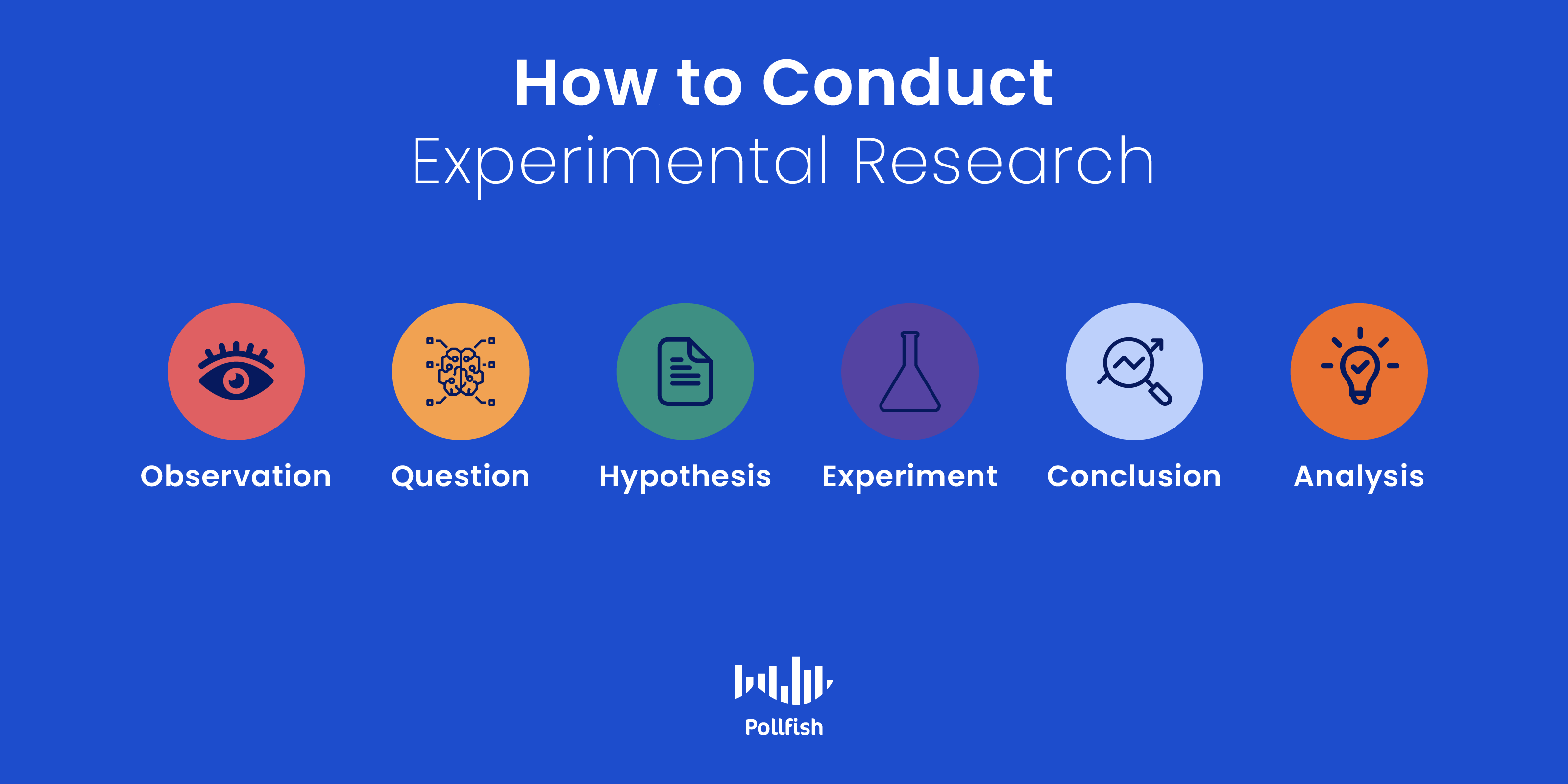
Experimental research is often the final form of research conducted in the research process and is considered conclusive research. The following explains the general steps required to successfully complete experimental research.
- Form a specific research question.
- Gather all available literature and other resources around the subject.
- Conduct secondary research around the subject and primary research via surveys .
- Consider how they relate to your question and how they line up with the secondary research you conducted.
- After your initial studies, form a hypothesis.
- First, decide which variable(s) is dependent/ independent (if it doesn’t involve experimenting).
- Decide how far to vary the independent variable.
- In the experiment, manipulate the independent variable(s).
- Measure the dependent variable(s) while you study the independent variable(s) alongside.
- Make sure to control potential confounding variables.
- Keep the study size in mind; a larger study pool creates statistical findings.
- Assign your subjects to “treatment” groups randomly, with each to receive a different level of “treatment.”
- Use a control group, which receives no manipulation. This shows you the test subjects as they appear/behave without any experimental intervention.
- Completely randomized design: every subject gets randomly assigned to a treatment.
- Randomized block design : aka stratified random design, subjects get first grouped based on a shared characteristic, then assigned to treatments within their groups at random.
- Independent measure : subjects receive only one of the possible levels of an experimental treatment.
- Repeated measures design : every subject gets each of the experimental treatments consecutively, as their responses are measured. It also refers to measuring the effect of an emerging effect over time.
- Continue experimenting on variables as needed, take measurements and take notes.
- Based on your experiment(s), put together a logical conclusion. It is possible that it may need testing over time.
Using Experimental Research and Going Further
Although experimental research can be very complex, this research method is the most conclusive. Using a scientific approach, it can help you form tests on various business matters. While it is critical for understanding your target market’s and customers’ existing behaviors, it can also be used to experiment on a wide variety of other matters.
Before launching a new product, or an updated one, for example, you can conduct an experiment to understand the product in action. This helps you avoid any glitches or undesirable qualities that will incur problems for your customs and a bad reputation for your brand.
Experimental research is not for every business, yet if you decide to implement this form of research, consider using surveys in tandem. An online survey platform can help you establish and distribute your surveys to a wide network via organic sampling to avoid biases.
Although it isn’t a requirement, in today’s age of excelling in customer experience (CX), it is of the essence to have as much data on your target market as possible. An online survey tool makes this possible.
Do you want to distribute your survey? Pollfish offers you access to millions of targeted consumers to get survey responses from $0.95 per complete. Launch your survey today.
Privacy Preference Center
Privacy preferences.
Chapter 10 Experimental Research
Experimental research, often considered to be the “gold standard” in research designs, is one of the most rigorous of all research designs. In this design, one or more independent variables are manipulated by the researcher (as treatments), subjects are randomly assigned to different treatment levels (random assignment), and the results of the treatments on outcomes (dependent variables) are observed. The unique strength of experimental research is its internal validity (causality) due to its ability to link cause and effect through treatment manipulation, while controlling for the spurious effect of extraneous variable.
Experimental research is best suited for explanatory research (rather than for descriptive or exploratory research), where the goal of the study is to examine cause-effect relationships. It also works well for research that involves a relatively limited and well-defined set of independent variables that can either be manipulated or controlled. Experimental research can be conducted in laboratory or field settings. Laboratory experiments , conducted in laboratory (artificial) settings, tend to be high in internal validity, but this comes at the cost of low external validity (generalizability), because the artificial (laboratory) setting in which the study is conducted may not reflect the real world. Field experiments , conducted in field settings such as in a real organization, and high in both internal and external validity. But such experiments are relatively rare, because of the difficulties associated with manipulating treatments and controlling for extraneous effects in a field setting.
Experimental research can be grouped into two broad categories: true experimental designs and quasi-experimental designs. Both designs require treatment manipulation, but while true experiments also require random assignment, quasi-experiments do not. Sometimes, we also refer to non-experimental research, which is not really a research design, but an all-inclusive term that includes all types of research that do not employ treatment manipulation or random assignment, such as survey research, observational research, and correlational studies.
Basic Concepts
Treatment and control groups. In experimental research, some subjects are administered one or more experimental stimulus called a treatment (the treatment group ) while other subjects are not given such a stimulus (the control group ). The treatment may be considered successful if subjects in the treatment group rate more favorably on outcome variables than control group subjects. Multiple levels of experimental stimulus may be administered, in which case, there may be more than one treatment group. For example, in order to test the effects of a new drug intended to treat a certain medical condition like dementia, if a sample of dementia patients is randomly divided into three groups, with the first group receiving a high dosage of the drug, the second group receiving a low dosage, and the third group receives a placebo such as a sugar pill (control group), then the first two groups are experimental groups and the third group is a control group. After administering the drug for a period of time, if the condition of the experimental group subjects improved significantly more than the control group subjects, we can say that the drug is effective. We can also compare the conditions of the high and low dosage experimental groups to determine if the high dose is more effective than the low dose.
Treatment manipulation. Treatments are the unique feature of experimental research that sets this design apart from all other research methods. Treatment manipulation helps control for the “cause” in cause-effect relationships. Naturally, the validity of experimental research depends on how well the treatment was manipulated. Treatment manipulation must be checked using pretests and pilot tests prior to the experimental study. Any measurements conducted before the treatment is administered are called pretest measures , while those conducted after the treatment are posttest measures .
Random selection and assignment. Random selection is the process of randomly drawing a sample from a population or a sampling frame. This approach is typically employed in survey research, and assures that each unit in the population has a positive chance of being selected into the sample. Random assignment is however a process of randomly assigning subjects to experimental or control groups. This is a standard practice in true experimental research to ensure that treatment groups are similar (equivalent) to each other and to the control group, prior to treatment administration. Random selection is related to sampling, and is therefore, more closely related to the external validity (generalizability) of findings. However, random assignment is related to design, and is therefore most related to internal validity. It is possible to have both random selection and random assignment in well-designed experimental research, but quasi-experimental research involves neither random selection nor random assignment.
Threats to internal validity. Although experimental designs are considered more rigorous than other research methods in terms of the internal validity of their inferences (by virtue of their ability to control causes through treatment manipulation), they are not immune to internal validity threats. Some of these threats to internal validity are described below, within the context of a study of the impact of a special remedial math tutoring program for improving the math abilities of high school students.
- History threat is the possibility that the observed effects (dependent variables) are caused by extraneous or historical events rather than by the experimental treatment. For instance, students’ post-remedial math score improvement may have been caused by their preparation for a math exam at their school, rather than the remedial math program.
- Maturation threat refers to the possibility that observed effects are caused by natural maturation of subjects (e.g., a general improvement in their intellectual ability to understand complex concepts) rather than the experimental treatment.
- Testing threat is a threat in pre-post designs where subjects’ posttest responses are conditioned by their pretest responses. For instance, if students remember their answers from the pretest evaluation, they may tend to repeat them in the posttest exam. Not conducting a pretest can help avoid this threat.
- Instrumentation threat , which also occurs in pre-post designs, refers to the possibility that the difference between pretest and posttest scores is not due to the remedial math program, but due to changes in the administered test, such as the posttest having a higher or lower degree of difficulty than the pretest.
- Mortality threat refers to the possibility that subjects may be dropping out of the study at differential rates between the treatment and control groups due to a systematic reason, such that the dropouts were mostly students who scored low on the pretest. If the low-performing students drop out, the results of the posttest will be artificially inflated by the preponderance of high-performing students.
- Regression threat , also called a regression to the mean, refers to the statistical tendency of a group’s overall performance on a measure during a posttest to regress toward the mean of that measure rather than in the anticipated direction. For instance, if subjects scored high on a pretest, they will have a tendency to score lower on the posttest (closer to the mean) because their high scores (away from the mean) during the pretest was possibly a statistical aberration. This problem tends to be more prevalent in non-random samples and when the two measures are imperfectly correlated.
Two-Group Experimental Designs
The simplest true experimental designs are two group designs involving one treatment group and one control group, and are ideally suited for testing the effects of a single independent variable that can be manipulated as a treatment. The two basic two-group designs are the pretest-posttest control group design and the posttest-only control group design, while variations may include covariance designs. These designs are often depicted using a standardized design notation, where R represents random assignment of subjects to groups, X represents the treatment administered to the treatment group, and O represents pretest or posttest observations of the dependent variable (with different subscripts to distinguish between pretest and posttest observations of treatment and control groups).
Pretest-posttest control group design . In this design, subjects are randomly assigned to treatment and control groups, subjected to an initial (pretest) measurement of the dependent variables of interest, the treatment group is administered a treatment (representing the independent variable of interest), and the dependent variables measured again (posttest). The notation of this design is shown in Figure 10.1.

Figure 10.1. Pretest-posttest control group design
The effect E of the experimental treatment in the pretest posttest design is measured as the difference in the posttest and pretest scores between the treatment and control groups:
E = (O 2 – O 1 ) – (O 4 – O 3 )
Statistical analysis of this design involves a simple analysis of variance (ANOVA) between the treatment and control groups. The pretest posttest design handles several threats to internal validity, such as maturation, testing, and regression, since these threats can be expected to influence both treatment and control groups in a similar (random) manner. The selection threat is controlled via random assignment. However, additional threats to internal validity may exist. For instance, mortality can be a problem if there are differential dropout rates between the two groups, and the pretest measurement may bias the posttest measurement (especially if the pretest introduces unusual topics or content).
Posttest-only control group design . This design is a simpler version of the pretest-posttest design where pretest measurements are omitted. The design notation is shown in Figure 10.2.

Figure 10.2. Posttest only control group design.
The treatment effect is measured simply as the difference in the posttest scores between the two groups:
E = (O 1 – O 2 )
The appropriate statistical analysis of this design is also a two- group analysis of variance (ANOVA). The simplicity of this design makes it more attractive than the pretest-posttest design in terms of internal validity. This design controls for maturation, testing, regression, selection, and pretest-posttest interaction, though the mortality threat may continue to exist.
Covariance designs . Sometimes, measures of dependent variables may be influenced by extraneous variables called covariates . Covariates are those variables that are not of central interest to an experimental study, but should nevertheless be controlled in an experimental design in order to eliminate their potential effect on the dependent variable and therefore allow for a more accurate detection of the effects of the independent variables of interest. The experimental designs discussed earlier did not control for such covariates. A covariance design (also called a concomitant variable design) is a special type of pretest posttest control group design where the pretest measure is essentially a measurement of the covariates of interest rather than that of the dependent variables. The design notation is shown in Figure 10.3, where C represents the covariates:

Figure 10.3. Covariance design
Because the pretest measure is not a measurement of the dependent variable, but rather a covariate, the treatment effect is measured as the difference in the posttest scores between the treatment and control groups as:

Figure 10.4. 2 x 2 factorial design
Factorial designs can also be depicted using a design notation, such as that shown on the right panel of Figure 10.4. R represents random assignment of subjects to treatment groups, X represents the treatment groups themselves (the subscripts of X represents the level of each factor), and O represent observations of the dependent variable. Notice that the 2 x 2 factorial design will have four treatment groups, corresponding to the four combinations of the two levels of each factor. Correspondingly, the 2 x 3 design will have six treatment groups, and the 2 x 2 x 2 design will have eight treatment groups. As a rule of thumb, each cell in a factorial design should have a minimum sample size of 20 (this estimate is derived from Cohen’s power calculations based on medium effect sizes). So a 2 x 2 x 2 factorial design requires a minimum total sample size of 160 subjects, with at least 20 subjects in each cell. As you can see, the cost of data collection can increase substantially with more levels or factors in your factorial design. Sometimes, due to resource constraints, some cells in such factorial designs may not receive any treatment at all, which are called incomplete factorial designs . Such incomplete designs hurt our ability to draw inferences about the incomplete factors.
In a factorial design, a main effect is said to exist if the dependent variable shows a significant difference between multiple levels of one factor, at all levels of other factors. No change in the dependent variable across factor levels is the null case (baseline), from which main effects are evaluated. In the above example, you may see a main effect of instructional type, instructional time, or both on learning outcomes. An interaction effect exists when the effect of differences in one factor depends upon the level of a second factor. In our example, if the effect of instructional type on learning outcomes is greater for 3 hours/week of instructional time than for 1.5 hours/week, then we can say that there is an interaction effect between instructional type and instructional time on learning outcomes. Note that the presence of interaction effects dominate and make main effects irrelevant, and it is not meaningful to interpret main effects if interaction effects are significant.
Hybrid Experimental Designs
Hybrid designs are those that are formed by combining features of more established designs. Three such hybrid designs are randomized bocks design, Solomon four-group design, and switched replications design.
Randomized block design. This is a variation of the posttest-only or pretest-posttest control group design where the subject population can be grouped into relatively homogeneous subgroups (called blocks ) within which the experiment is replicated. For instance, if you want to replicate the same posttest-only design among university students and full -time working professionals (two homogeneous blocks), subjects in both blocks are randomly split between treatment group (receiving the same treatment) or control group (see Figure 10.5). The purpose of this design is to reduce the “noise” or variance in data that may be attributable to differences between the blocks so that the actual effect of interest can be detected more accurately.
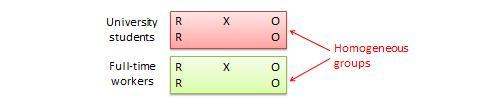
Figure 10.5. Randomized blocks design.
Solomon four-group design . In this design, the sample is divided into two treatment groups and two control groups. One treatment group and one control group receive the pretest, and the other two groups do not. This design represents a combination of posttest-only and pretest-posttest control group design, and is intended to test for the potential biasing effect of pretest measurement on posttest measures that tends to occur in pretest-posttest designs but not in posttest only designs. The design notation is shown in Figure 10.6.
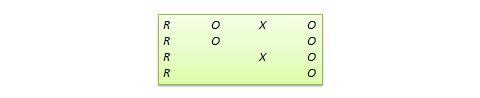
Figure 10.6. Solomon four-group design
Switched replication design . This is a two-group design implemented in two phases with three waves of measurement. The treatment group in the first phase serves as the control group in the second phase, and the control group in the first phase becomes the treatment group in the second phase, as illustrated in Figure 10.7. In other words, the original design is repeated or replicated temporally with treatment/control roles switched between the two groups. By the end of the study, all participants will have received the treatment either during the first or the second phase. This design is most feasible in organizational contexts where organizational programs (e.g., employee training) are implemented in a phased manner or are repeated at regular intervals.

Figure 10.7. Switched replication design.
Quasi-Experimental Designs
Quasi-experimental designs are almost identical to true experimental designs, but lacking one key ingredient: random assignment. For instance, one entire class section or one organization is used as the treatment group, while another section of the same class or a different organization in the same industry is used as the control group. This lack of random assignment potentially results in groups that are non-equivalent, such as one group possessing greater mastery of a certain content than the other group, say by virtue of having a better teacher in a previous semester, which introduces the possibility of selection bias . Quasi-experimental designs are therefore inferior to true experimental designs in interval validity due to the presence of a variety of selection related threats such as selection-maturation threat (the treatment and control groups maturing at different rates), selection-history threat (the treatment and control groups being differentially impact by extraneous or historical events), selection-regression threat (the treatment and control groups regressing toward the mean between pretest and posttest at different rates), selection-instrumentation threat (the treatment and control groups responding differently to the measurement), selection-testing (the treatment and control groups responding differently to the pretest), and selection-mortality (the treatment and control groups demonstrating differential dropout rates). Given these selection threats, it is generally preferable to avoid quasi-experimental designs to the greatest extent possible.
Many true experimental designs can be converted to quasi-experimental designs by omitting random assignment. For instance, the quasi-equivalent version of pretest-posttest control group design is called nonequivalent groups design (NEGD), as shown in Figure 10.8, with random assignment R replaced by non-equivalent (non-random) assignment N . Likewise, the quasi -experimental version of switched replication design is called non-equivalent switched replication design (see Figure 10.9).

Figure 10.8. NEGD design.

Figure 10.9. Non-equivalent switched replication design.
In addition, there are quite a few unique non -equivalent designs without corresponding true experimental design cousins. Some of the more useful of these designs are discussed next.
Regression-discontinuity (RD) design . This is a non-equivalent pretest-posttest design where subjects are assigned to treatment or control group based on a cutoff score on a preprogram measure. For instance, patients who are severely ill may be assigned to a treatment group to test the efficacy of a new drug or treatment protocol and those who are mildly ill are assigned to the control group. In another example, students who are lagging behind on standardized test scores may be selected for a remedial curriculum program intended to improve their performance, while those who score high on such tests are not selected from the remedial program. The design notation can be represented as follows, where C represents the cutoff score:

Figure 10.10. RD design.
Because of the use of a cutoff score, it is possible that the observed results may be a function of the cutoff score rather than the treatment, which introduces a new threat to internal validity. However, using the cutoff score also ensures that limited or costly resources are distributed to people who need them the most rather than randomly across a population, while simultaneously allowing a quasi-experimental treatment. The control group scores in the RD design does not serve as a benchmark for comparing treatment group scores, given the systematic non-equivalence between the two groups. Rather, if there is no discontinuity between pretest and posttest scores in the control group, but such a discontinuity persists in the treatment group, then this discontinuity is viewed as evidence of the treatment effect.
Proxy pretest design . This design, shown in Figure 10.11, looks very similar to the standard NEGD (pretest-posttest) design, with one critical difference: the pretest score is collected after the treatment is administered. A typical application of this design is when a researcher is brought in to test the efficacy of a program (e.g., an educational program) after the program has already started and pretest data is not available. Under such circumstances, the best option for the researcher is often to use a different prerecorded measure, such as students’ grade point average before the start of the program, as a proxy for pretest data. A variation of the proxy pretest design is to use subjects’ posttest recollection of pretest data, which may be subject to recall bias, but nevertheless may provide a measure of perceived gain or change in the dependent variable.

Figure 10.11. Proxy pretest design.
Separate pretest-posttest samples design . This design is useful if it is not possible to collect pretest and posttest data from the same subjects for some reason. As shown in Figure 10.12, there are four groups in this design, but two groups come from a single non-equivalent group, while the other two groups come from a different non-equivalent group. For instance, you want to test customer satisfaction with a new online service that is implemented in one city but not in another. In this case, customers in the first city serve as the treatment group and those in the second city constitute the control group. If it is not possible to obtain pretest and posttest measures from the same customers, you can measure customer satisfaction at one point in time, implement the new service program, and measure customer satisfaction (with a different set of customers) after the program is implemented. Customer satisfaction is also measured in the control group at the same times as in the treatment group, but without the new program implementation. The design is not particularly strong, because you cannot examine the changes in any specific customer’s satisfaction score before and after the implementation, but you can only examine average customer satisfaction scores. Despite the lower internal validity, this design may still be a useful way of collecting quasi-experimental data when pretest and posttest data are not available from the same subjects.
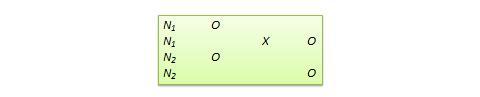
Figure 10.12. Separate pretest-posttest samples design.
Nonequivalent dependent variable (NEDV) design . This is a single-group pre-post quasi-experimental design with two outcome measures, where one measure is theoretically expected to be influenced by the treatment and the other measure is not. For instance, if you are designing a new calculus curriculum for high school students, this curriculum is likely to influence students’ posttest calculus scores but not algebra scores. However, the posttest algebra scores may still vary due to extraneous factors such as history or maturation. Hence, the pre-post algebra scores can be used as a control measure, while that of pre-post calculus can be treated as the treatment measure. The design notation, shown in Figure 10.13, indicates the single group by a single N , followed by pretest O 1 and posttest O 2 for calculus and algebra for the same group of students. This design is weak in internal validity, but its advantage lies in not having to use a separate control group.
An interesting variation of the NEDV design is a pattern matching NEDV design , which employs multiple outcome variables and a theory that explains how much each variable will be affected by the treatment. The researcher can then examine if the theoretical prediction is matched in actual observations. This pattern-matching technique, based on the degree of correspondence between theoretical and observed patterns is a powerful way of alleviating internal validity concerns in the original NEDV design.

Figure 10.13. NEDV design.
Perils of Experimental Research
Experimental research is one of the most difficult of research designs, and should not be taken lightly. This type of research is often best with a multitude of methodological problems. First, though experimental research requires theories for framing hypotheses for testing, much of current experimental research is atheoretical. Without theories, the hypotheses being tested tend to be ad hoc, possibly illogical, and meaningless. Second, many of the measurement instruments used in experimental research are not tested for reliability and validity, and are incomparable across studies. Consequently, results generated using such instruments are also incomparable. Third, many experimental research use inappropriate research designs, such as irrelevant dependent variables, no interaction effects, no experimental controls, and non-equivalent stimulus across treatment groups. Findings from such studies tend to lack internal validity and are highly suspect. Fourth, the treatments (tasks) used in experimental research may be diverse, incomparable, and inconsistent across studies and sometimes inappropriate for the subject population. For instance, undergraduate student subjects are often asked to pretend that they are marketing managers and asked to perform a complex budget allocation task in which they have no experience or expertise. The use of such inappropriate tasks, introduces new threats to internal validity (i.e., subject’s performance may be an artifact of the content or difficulty of the task setting), generates findings that are non-interpretable and meaningless, and makes integration of findings across studies impossible.
The design of proper experimental treatments is a very important task in experimental design, because the treatment is the raison d’etre of the experimental method, and must never be rushed or neglected. To design an adequate and appropriate task, researchers should use prevalidated tasks if available, conduct treatment manipulation checks to check for the adequacy of such tasks (by debriefing subjects after performing the assigned task), conduct pilot tests (repeatedly, if necessary), and if doubt, using tasks that are simpler and familiar for the respondent sample than tasks that are complex or unfamiliar.
In summary, this chapter introduced key concepts in the experimental design research method and introduced a variety of true experimental and quasi-experimental designs. Although these designs vary widely in internal validity, designs with less internal validity should not be overlooked and may sometimes be useful under specific circumstances and empirical contingencies.
- Social Science Research: Principles, Methods, and Practices. Authored by : Anol Bhattacherjee. Provided by : University of South Florida. Located at : http://scholarcommons.usf.edu/oa_textbooks/3/ . License : CC BY-NC-SA: Attribution-NonCommercial-ShareAlike
Experimental Study on Strength Enhancement and Porosity Variation of 3D-Printed Gypsum Rocks: Insights on Vacuum Infiltration Post-Processing
- Original Paper
- Open access
- Published: 07 May 2024
Cite this article
You have full access to this open access article
- Yulong Shao 1 ,
- Jineon Kim 1 ,
- Jingwei Yang 2 ,
- Jae-Joon Song ORCID: orcid.org/0000-0002-0308-7696 1 &
- Juhyuk Moon 2
Three-dimensional printing (3DP) technology has shown great potential in rock mechanics and mining engineering due to its ability to create complex and customized objects with high precision and accuracy. At present, an emerging research focus is improving the mechanical properties of 3D-printed samples, which originally has low strength and stiffness, to match those of natural rocks. The objective of this study was to investigate the effectiveness of different post-treatments on the strength enhancement of 3D-printed gypsum samples. To achieve this goal, 3D-printed gypsum samples were subjected to different post-treatments including dipped infiltration treatment and vacuum infiltration treatment using different infiltrants: water, saltwater, ColorBond, and StrengthMax. Subsequently, each sample was subjected to ultrasonic wave velocity testing and uniaxial compression experiments to characterize their mechanical properties, CT scans to investigate their microstructural characteristics. Additionally, X-ray Diffraction (XRD) and Scanning Electron Microscope (SEM) tests were conducted to explore the underlying reasons for changes in macroscopic strength. Finally, the physical characteristics and mechanical properties of untreated and post-processed 3D-printed gypsum samples were compared with natural rocks. The results showed that the strength of samples treated with water and saltwater was much lower than that of those treated with ColorBond and StrengthMax, while the porosity was the opposite. In water-treated and saltwater-treated samples, water or saltwater treatment can alter particle characteristics, but weak adhesive bonding and numerous pores result in low mechanical strength. Samples treated with Colorbond or StrengthMax exhibit improved strength due to effective gap filling and cohesive structure formation, with StrengthMax-treated samples showing higher strength despite having more pores than Colorbond-treated ones. Moreover, the physical and mechanical properties of these treated samples matched a wider range of natural rock types compared to the untreated samples.
This study investigates the impact of different post-treatments and infiltrants on the physical and mechanical properties of 3D-printed gypsum samples.
A novel method is proposed in this study to quantitatively evaluate the degree of infiltration by comparing the changes in porosity before and after vacuum infiltration of 3D-printed samples based on 2D CT images.
The study provides an in-depth analysis of the mechanisms responsible for the macroscopic strength enhancement in 3D-printed samples after vacuum infiltration treatment.
Vacuum infiltration treatment using StrengthMax significantly improves the physical and mechanical properties of 3D-printed gypsum rocks, allowing them to replicate a wider variety of natural rocks compared to untreated samples.
Avoid common mistakes on your manuscript.
1 Introduction
The application of three-dimensional printing (3DP) technology in rock mechanics research has become popular due to its capability of producing rock-like models of various shapes and sizes that closely resemble actual rocks. These models can be quickly, accurately, and inexpensively produced in large batches, enabling researchers to gain deeper insights into the physical and mechanical properties of natural rocks (Gao et al. 2021 ). 3D printed samples offer advantages over molded samples, including the ability to create intricate designs, rapid prototyping, reduced material waste, and lower tooling costs, while providing greater design flexibility and improved mechanical properties in certain cases. With the development of 3DP technology, there are now various types of printing materials available. In the field of rock mechanics research, the main printing materials used are as follows: gypsum powder (Fereshtenejad et al. 2021 ; Fereshtenejad and Song 2016 ; Jiang et al. 2016a , b , c ; Jiang and Song 2018 ; Kong et al. 2018 ; Sharafisafa et al. 2018a , b ; Wu et al. 2020 ), photosensitive resin (Li et al. 2021 ; Suzuki et al. 2019 ; Zhou et al. 2019 , 2020 ), sandstone powder (Osinga et al. 2015, Wang et al. 2022 ; Yu et al. 2021 ; Zhang and Li 2022 ), polylactic acid (PLA) (Jiang and Zhao 2015 ; Jiang et al. 2016a , b , c ; Li et al. 2022a , b ; Zhou and Zhu 2018 ), and cement-based materials (Feng et al. 2019 , 2022 ; Mei et al. 2022 ). A series of mechanical experiments have been conducted on the 3D-printed rocks using the aforementioned printing materials, which include mechanical properties testing (Fereshtenejad and Song 2016 , Jiang et al. 2016a , b , c , Kong et al. 2018 , Osinga et al. 2015, Sharafisafa et al. 2018a , b , Wang et al. 2022 , Wu et al. 2020 , Zhou and Zhu 2018 ), crack propagation and failure mechanisms (Jiang et al. 2016a , b , c ; Sharafisafa et al. 2018a , b ; Sharafisafa et al. 2019 ; Yu et al. 2021 ; Zhou et al. 2019 , 2020 ), shear and permeability characteristics (Fereshtenejad et al. 2021 ; Li et al. 2021 ; Suzuki et al. 2019 ; Wang et al. 2020 ), and physical modeling (Feng et al. 2019 ; Jiang and Song 2018 ; Li et al. 2022a , b ; Song et al. 2018 ). The relative merits and applications of printing materials are tabulated in Table 1 . Compared to other printing materials, gypsum powder is widely regarded as an effective material for producing rock-like samples (Kong et al. 2018 ; Wu et al. 2020 ), since it has composition and structure that closely resemble those of natural rocks. It also has the advantage of producing samples with a high level of precision at low cost. However, a significant limitation of 3D-printed gypsum rocks is that they have comparatively low strength and high ductility, which differs from the typical properties of natural rocks. Consequently, enhancing the mechanical properties of 3D-printed samples to match the strength and stiffness of natural rocks has emerged as a key research focus for 3DP technology applications in rock mechanics.
To improve the strength and stiffness of 3D-printed gypsum samples, two approaches can be considered: the optimization of 3D printing parameters and the post-processing of printed samples. The selection of optimal 3D printing parameters involves setting different printing parameters including printing directions (Fereshtenejad and Song 2016 ; Hamano et al. 2021 ; He et al. 2020 ), layer thicknesses (Farzadi et al. 2014 ; Fereshtenejad and Song 2016 ), binder saturation levels (Fereshtenejad and Song 2016 ; Liu et al. 2019 ), and printing delay times (Farzadi et al. 2015 ), to develop the strength and stiffness of 3D-printed gypsum samples. The mechanical properties of 3D-printed gypsum samples can vary significantly depending on the printing direction which is primarily determined by the bedding direction and movement of the printer head. This can have a significant impact on not only the mechanical anisotropy but also the strength and stiffness of the 3D-printed gypsum samples, as demonstrated in our previous research (Shao et al. 2023 ). While some studies (Farzadi et al. 2014 ; Fereshtenejad and Song 2016 ) have reported that changing the layer thickness can also affect the strength of 3D-printed gypsum samples, its impact is relatively small compared to other printing parameters. The level of binder saturation is a crucial factor affecting the strength and stiffness of 3D-printed gypsum samples, and increasing the level of binder saturation has been reported to enhance the strength of 3D-printed samples (Fereshtenejad and Song 2016 ; Liu et al. 2019 ). The observed enhancement can be attributed to a chemical reaction between the water present in the binder and the hemihydrate calcium sulfate (CaSO 4 ·1/2H 2 O) in the raw powder, resulting in the formation of high-strength dihydrate calcium sulfate (CaSO 4 ·2H 2 O) and bonding the particles together to create a highly strong interlocking structure (Hamano et al. 2021 ). In addition, print delay time is also one of the factors affecting the mechanical properties of 3D-printed gypsum samples. Farzadi et al. ( 2015 ) investigated the effects of four different print delay times (50,100,300 and 500 ms) on the physical and mechanical properties of 3D-printed gypsum samples. The results showed that the compressive strength, toughness and elastic modulus of samples printed with a delay of 300 ms were higher than those of other samples. However, it should be noted that even with optimal printing parameters, the strength and stiffness of the 3D-printed gypsum samples remain relatively low due to limitations and defects in both the printing material and the 3D printer itself, which makes these samples difficult to use as accurate replications of natural rock.
Compared with optimal printing parameter settings, the post-processing of 3D-printed samples has a more significant impact on the strength enhancement of 3D-printed gypsum samples. The common post-treatments for strength enhancement include heat treatment (Fereshtenejad and Song 2016 ; Liu et al. 2019 ), dipped infiltration treatment (Shah et al. 2020 ; Shakor et al. 2022 ), and vacuum infiltration treatment ( Kong et al. 2019 , Wang et al. 2021). While heat treatment has been shown to improve mechanical properties in some studies (Liu et al. 2019 ), excessively high temperature or prolonged heating times can have a negative impact on the mechanical properties of samples. Therefore, optimal heating conditions may vary between researchers. For example, Fereshtenejad and Song ( 2016 ) recommended heating at 150 °C for 60 min, while Zhou et al. (2013) suggested a temperature of 200 °C for 30 min as optimal. Placing 3D-printed gypsum samples in a fast-curing infiltrant for a certain time is also an effective method to improve mechanical properties. There are many types of infiltrants used to enhance the strength of 3D-printed gypsum samples, including water (Mandal and Basu 2018 ), salt water (Rodríguez-González et al. 2022 ), wax (Ishutov and Hasiuk 2019 ; Shah et al. 2020 ), and resin materials (Kong et al. 2019 , Wang et al. 2021, Wu et al. 2020 ). Wang et al. (2021) demonstrated that dipping 3D-printed gypsum samples in ColorBond (manufactured by 3D Systems, Inc.) results in excellent mechanical properties. This treatment reduced surface roughness, mitigated the step effect, and enhanced the strength of powder-based models to a level comparable to that of functional models. In fact, the degree of infiltration is a crucial factor in this treatment that directly affects the mechanical properties of 3D-printed gypsum samples. Compared to dip infiltration treatment, vacuum infiltration treatment can maximize the degree of infiltration, resulting in a significant improvement in the strength of the post-processed samples (Ayres et al. 2019 ). Wu et al. ( 2020 ) utilized the vacuum infiltration technology, with ColorBond as the infiltrant, to increase the strength of 3D-printed gypsum samples to 56.7 MPa. Through this approach, high-stress soft rocks could be replicated using 3D-printed gypsum samples.
Previous studies have consistently demonstrated that vacuum infiltration treatment can significantly enhance the physical and mechanical properties of 3D-printed gypsum samples. However, further research is necessary to determine the ideal infiltrant material, evaluate the impact of vacuum infiltration on the pore structure and porosity of 3D-printed gypsum samples, quantitatively measure the degree of infiltration, and uncover the underlying reasons for the macroscopic strength improvement of post-treated samples. To address these research gaps, this study investigates the effects of various infiltration methods and infiltrant materials on the mechanical and microstructural properties of 3D-printed gypsum samples. This investigation includes ultrasonic wave velocity tests, uniaxial compression tests, X-ray diffraction (XRD) tests, scanning electron microscopy (SEM) tests, and Micro-CT scanning. We analyze the ultrasonic wave velocity characteristics, mechanical properties, compositional changes, and microstructural properties of 3D-printed samples before and after vacuum infiltration treatment. The results help us identify the most optimal infiltrant material and effective post-processing method, while also revealing the underlying reasons for the macroscopic strength enhancement in 3D-printed samples after vacuum infiltration treatment. Furthermore, we compare the enhanced mechanical properties of the post-processed samples with those found in natural rocks.
2 Experiments and Methods
2.1 experimental scheme and equipment.
The experimental process consists of 3D-printed samples preparation (Fig. 1 b), post-processing (Fig. 1 c-e), ultrasonic wave velocity and mechanical properties measurement (Fig. 1 f and g), SEM tests (Fig. 1 h), XRD tests (Fig. 1 i), and CT scanning (Fig. 1 j). Two different sizes of 3D-printed gypsum samples, Φ 25 mm × 50 mm and Φ 15 mm × 30 mm, were manufactured using a gypsum powder-based 3D printer. Layer thickness and binder saturation level were set to 0.1 mm and 100%, respectively, and all samples were fabricated in conjunction with the movement direction of the printer head. The large-sized 3D-printed gypsum samples were subjected to uniaxial compression tests and small-sized samples were subjected to CT scanning tests. The experiment processes for each sample are illustrated in red and blue arrows in Fig. 1 , respectively. All 3D-printed samples were put into a drying oven at a constant temperature of 40 °C for 4 days to ensure consistent sample quality and physical behavior. After the first stage of heat treatment, the samples were treated by different infiltration treatments, including dipped infiltration and vacuum infiltration. For the vacuum infiltration, the vacuum infiltration equipment shown in Fig. 1 d was used. Liu et al. ( 2019 ) have reported that the mechanical properties and physical characteristics of 3D-printed gypsum samples remain stable after being placed in an oven at 40 ℃ for 14 days. Therefore, the infiltration-treated samples were then kept in the drying oven again for 10 days, for the second stage of heat treatment, before conducting the uniaxial compressive tests and CT scanning tests. It should be noted that the small-sized samples were scanned by Micro-CT after both the first and second heat treatment to compare the microstructural variation before and after the vacuum infiltration treatment, as shown in Fig. 1 j. After undergoing uniaxial compression testing, large-sized samples were subject to SEM and XRD tests, aiming to detect alterations in sample composition and internal microstructure, as depicted in Fig. 1 h and i.
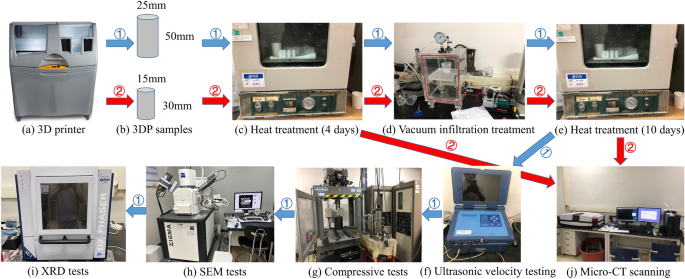
Overview of the experimental scheme in this study. Two different sizes of 3DP samples, 50 mm × 25 mm and 30 mm × 15 mm, are utilized to obtain mechanical properties (experiment 1 in blue arrows) and microstructural variation (experiment 2 in red arrows), respectively
2.2 Vacuum Infiltration Equipment and Post-Processing
In this study, a specially designed vacuum infiltration equipment was utilized to ensure pump, a vacuum chamber and four valves (valves 1 to 4), as shown in Fig. 2 . The vacuum chamber is connected to the infiltrant material, vacuum pump, and atmospheric pressure through valve 1, valve 2, and valve 3, respectively. The vacuum infiltration treatment is conducted through the following steps: First, a plastic cup containing the 3D-printed samples is placed in the closed vacuum chamber of which all valves are closed. Then, valve 2 is opened and the vacuum pump is operated to create a low-pressure vacuum environment (− 20 kPa) inside the vacuum chamber, which is maintained for 24 h. Afterward, valve 1 is opened to allow the infiltrant to flow into the plastic cup within the vacuum chamber. Once the infiltrant fully covers the sample, valve 1 is immediately closed and valve 2 is opened again, with the vacuum pump operating, to maintain a vacuum pressure of − 20 kPa for 5 min. Finally, after 5 min, valve 2 is closed, the vacuum pump is turned off, and valve 3 is opened to make the infiltrant permeate into the internal pores of the samples by backward atmospheric pressure. After bubbling ceases on the surface of the samples, excess infiltrant is wiped off, and the 3D-printed samples are dried in a drying oven at 40 °C for 10 days before undergoing uniaxial compression tests or CT scanning experiments.
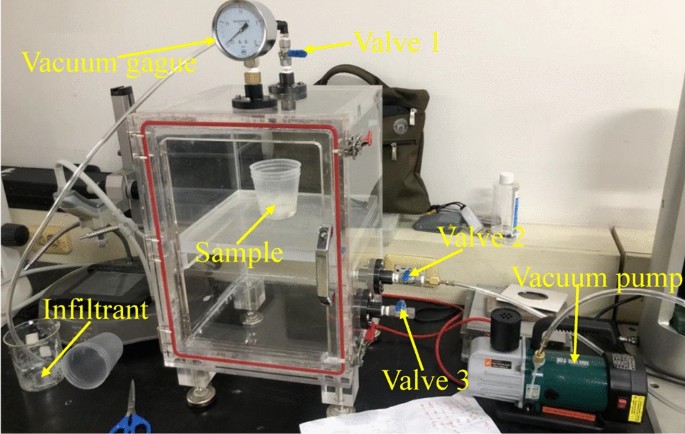
Vacuum infiltration equipment
Four different infiltrants including water, saltwater, ColorBond and StrengthMax (manufactured by 3D Systems, Inc.) were adopted in this study, as shown in Table 2 . The viscosity of infiltrants in ascending order is water, saltwater, ColorBond and StrengthMax. To investigate the effects of different post-treatments and infiltrants on the mechanical properties of 3D-printed samples, nine groups with the size of Φ 25 mm × 50 mm were manufactured to subject different post-treatments using different infiltrants. To enhance the reliability of the data, we utilized two samples for each group, presenting their average values as the final data. The detailed post-processing schemes for different sizes of 3D-printed gypsum samples using different infiltrants are shown in Table 3 , where signs “V” and “D” represent vacuum infiltration treatment and dipped infiltration treatment, respectively. The numbers 1, 2, 3 and 4 denote different infiltrants, which are water, saltwater, ColorBond and StrengthMax, respectively. For example, 1-V and 3-D each indicate 3D-printed samples treated by water using vacuum infiltration and ColorBond using dipped infiltration, respectively. The number 0 denotes the blank group, which is the sample that is not treated by any infiltrants. Alongside the larger samples, four 3D-printed samples with smaller size of Φ 15 mm × 30 mm are prepared to study the effect of infiltration on the porosity variation of 3D-printed samples after vacuum infiltration treatment.
2.3 Image Processing and 3D Reconstruction
The Skyscan1272 X-ray CT scanner with a resolution of 8 μm, operating at a maximum voltage of 100 kV and a maximum current of 100 μA, was used to analyze the microstructure of the 3D-printed gypsum samples, as shown in Fig. 1 h. The 3D-printed sample was placed on a brass stage that was rotated 360°, with an exposure time of 4500 ms for each rotation and an imaging rotation step of 0.3°. The pixel size of a CT image is inversely proportional to the maximum scanning range of Micro-CT equipment (Wehr and Lohr 1999 ). And 3D-printed samples with the same printing orientation exhibit uniform pore distribution, owing to the distinctive layer-by-layer additive manufacturing technique and consistent size of raw printing powder (Shao et al. 2023 ). Therefore, to acquire CT images with pixel size of 8 μm in this study, only a partial section of the 3D-printed sample with a height of 12 mm from the top was selected as the scanning objective, as depicted in Fig. 3 . The duration of each scanning process for the 3D-printed samples was approximately 3 h.
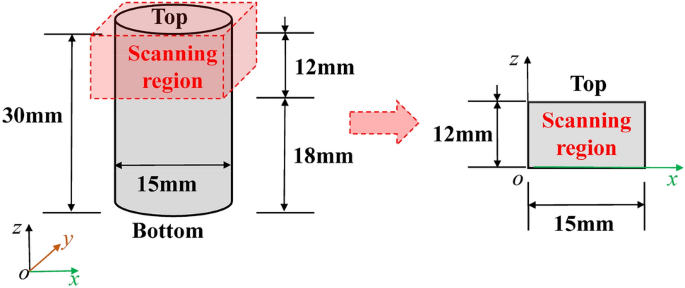
Selection of the scanning region of the 3D-printed sample
The raw CT images were post-processed by an image processing software—Avizo (Scientific 2018 ). The schematic of 3D reconstruction and microstructure analysis for Micro-CT scanning images are shown in Fig. 4 . The results of CT scans can be affected by various factors, including the different positioning and orientation of the sample at different scanning sessions (Kalender and Polacin 1991 ; Nakashima et al. 2011 ). This difference in sample posture can cause image displacement or distortion, which can result in errors during subsequent image analysis and comparison if left uncorrected. Therefore, to ensure the accuracy and comparability of the results, it is necessary to register the CT images to identical sample postures before any further analysis. The registration of CT images of 3D-printed samples obtained before and after post-treatment is first conducted by aligning the CT images based on the common geometric features with distinct characteristics (Studholme et al. 1999 ), as shown in Fig. 4 b. Then, grey value registration, which is a commonly used image registration method that aligns two images based on their grey values (Collignon et al. 1995), was utilized to eliminate or minimize position, rotation, scaling, distortion and other variations in the CT images of 3D-printed samples, as shown in Fig. 4 c. The CT images of 3D-printed samples scanned before vacuum infiltration treatment were used as the reference for geometric and grey value alignment. Median filtering was then applied to the registered image to smooth noise, while preserving the contours of images (Huang et al. 1979 ). Finally, the pore structure was extracted and analyzed by 3D reconstruction of 2D CT images after thresholding, as shown in Fig. 4 e and f. Figure 5 depicts the results of the alignment and 3D reconstruction of CT images of 3D-printed samples before and after vacuum infiltration treatment.
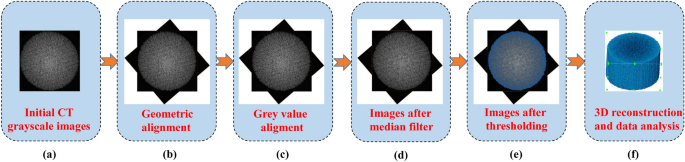
Schematic of image processing and 3D reconstruction for Micro-CT scanning images
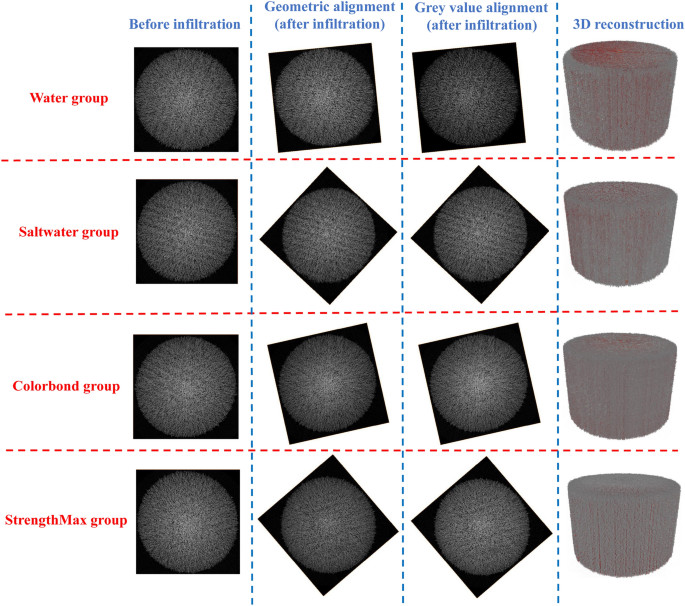
Alignment process and 3D reconstruction based on 2D CT images of 3D-printed gypsum samples subjected to vacuum infiltration treatment using four different infiltrants. Grey color and red color denote 3D reconstruction model before and after vacuum infiltration treatment, respectively
3 Results and Analysis
3.1 physical change.
The surface morphology of 3D-printed samples after infiltration using different infiltrants is shown in Fig. 6 . Compared with the surface of the 3D-printed sample before infiltration, the 3D-printed samples infiltrated by water and saltwater developed rough surfaces after infiltration. The surface of the saltwater was especially rough which can be attributed to the fact that gypsum particles on the surface of the 3D-printed samples are gradually dissolved by water erosion, resulting in small pits and unevenness on the surface. Additionally, salt ions increase the dissolution rate of gypsum particles, and salt residues may attach to the surface of 3D-printed samples and form white crystals or precipitates after drying. The samples infiltrated by ColorBond and StrengthMax, on the other hand, had smooth surfaces since the gypsum particle does not react with ColorBond and StrengthMax. The ColorBond or StrengthMax infiltrants can provide a protective layer over the surface of the sample that helps prevent scratches and wear, thus increasing the lifespan and durability of the samples (Kyriakou 2013 ).
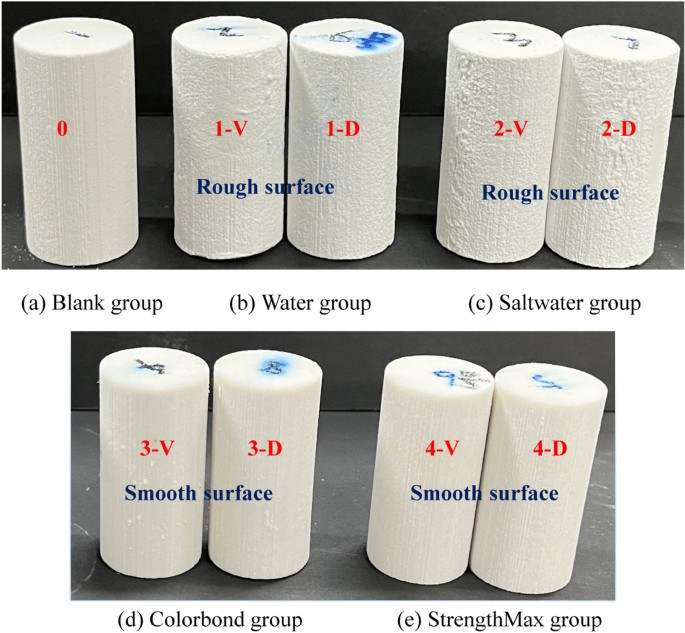
Surface morphology of 3D-printed samples after infiltration of different infiltrants
The weight of 3D-printed samples before and after application of different infiltration treatments are shown in Fig. 7 . The weight increment of 3D-printed samples treated by vacuum infiltration is higher than that by dipped infiltration. This is mainly because more infiltrants can be injected into the sample under high pressure difference using the vacuum infiltration treatment. It is notable that the weight increment of the 3D-printed sample (6.08 g) subjected to vacuum infiltration treatment using ColorBond is the highest. When considering the amount of injected infiltrants for the water and saltwater group, the actual injected amount is likely to be larger than the observed weight increments since the gypsum particle can dissolve into water which induces mass reduction of the 3D-printed sample.
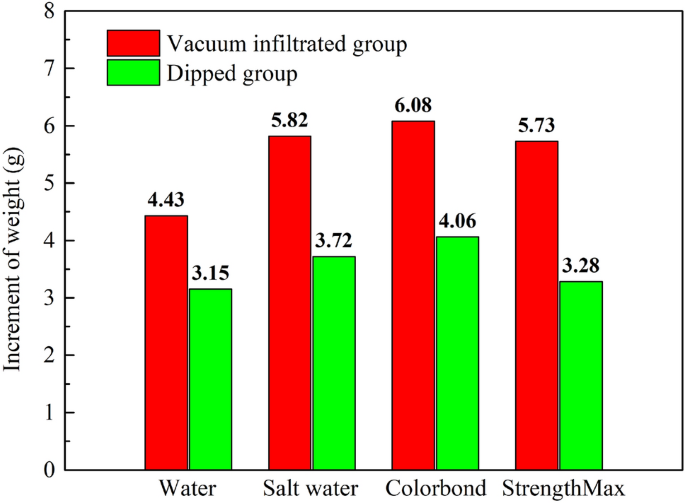
Weight increment of 3D-printed samples measured after infiltration
3.2 Ultrasonic Wave Velocity
Wave velocity is an important physical property of rock that can reflect the mechanical behavior of rock under different loading and environmental conditions (Zhang and Zhao 2014 ). The ultrasonic wave velocity of 3D-printed samples after infiltration is shown in Fig. 8 . For the 3D-printed sample without infiltration, P-wave velocity and S-wave velocity are 2.58 km/s and 1.78 km/s, respectively. The P-wave velocity of the 3D-printed samples is observed to be higher after vacuum infiltration compared to 3D-printed rocks that underwent dipped infiltration treatment and also those without infiltration. However, the impact of vacuum infiltration and dipped infiltration on the S-wave velocity is uncertain. The observed increase in P-wave velocity can be attributed to the infiltration resulting in the filling of internal pores, reducing porosity, increasing density, and subsequently enhancing sample strength, as demonstrated in Sects. 3.3 and 3.4 . In case of infiltration using water and saltwater, the P-wave velocity of 3D-printed samples does not show significant improvement. However, the S-wave velocity does show a comparatively greater improvement, which may be due to the solubility of gypsum particles in water.
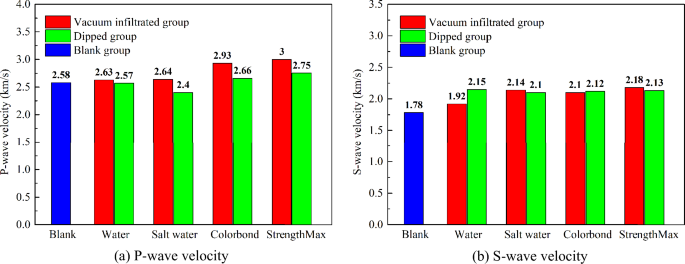
Ultrasonic wave velocity of 3D-printed samples after infiltration
3.3 Mechanical Property Change
3.3.1 stress–strain curves.
To investigate the effectiveness of the vacuum infiltration treatment in enhancing the strength of 3D-printed samples and determine the optimal infiltrant, the stress–strain curves obtained from uniaxial compressive experiments of 3D-printed samples subjected to different infiltration and natural rocks were compared, as shown in Fig. 9 . The results revealed that the infiltrants ColorBond and StrengthMax were the most effective in improving the strength and stiffness of the 3D-printed gypsum samples, particularly when infiltration was performed by vacuum infiltration using StrengthMax. On the other hand, water and saltwater treatments had minimal effects on the strength of 3D-printed samples.
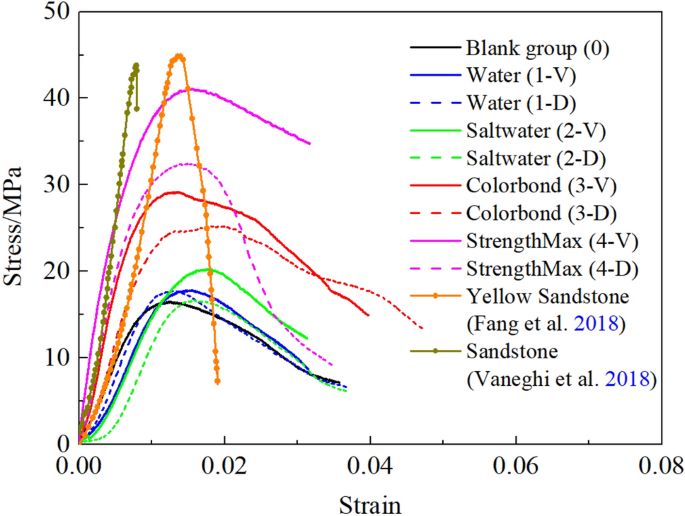
Stress–strain curves of 3D-printed samples after infiltration
Comparing the full stress–strain curve of natural sandstone (Vaneghi et al. 2018 ; Zhao et al. 2022 ) with that of 3D-printed samples, it was observed that the stress–strain curve of 3D-printed samples treated by vacuum infiltration using StengthMax was the most similar, indicating the potential of such samples as a substitute for natural sandstone. However, notable drawbacks were also identified, as the post-peak failure curve showed significant differences due to the high ductility of the 3D-printed gypsum samples, which has been reported in previous studies (Fereshtenejad and Song 2016 ; Liu et al. 2019 ).
3.3.2 Mechanical Properties
As shown in Fig. 10 , a comparison was made between the mechanical properties of 3D-printed samples after infiltration, including UCS, elastic modulus, and peak strain. The results showed that the effect of different infiltrants on the strength of 3D-printed samples increased in the order of water, saltwater, ColorBond, and StrengthMax, with StrengthMax exhibiting the most significant effect (41.11 MPa). Moreover, the strength of 3D-printed samples treated with vacuum infiltration was higher than those of the dipped group under the same infiltrant, demonstrating the effectiveness of vacuum infiltration treatment in enhancing the strength of 3D-printed samples. ColorBond and StrengthMax greatly enhanced the elastic modulus of the samples, with the elastic modulus of 3D-printed samples treated with vacuum infiltration being more than twice that of the untreated samples. To the contrary, the elastic modulus of 3D-printed samples treated with water and saltwater decreased, even though their strength increased. This can be explained by the fact that water can have an effect on the crystal structure of gypsum and increment of porosity, which in turn affects the physical and mechanical properties of 3D-printed gypsum samples. The peak strain of infiltrated 3D-printed samples was slightly larger compared to the untreated samples, mainly due to the effect of strength enhancement after infiltration. Among the four infiltrants tested in this study, StrengthMax was the most effective in improving the strength and stiffness of 3D-printed gypsum samples. The vacuum infiltration technique, combined with StrengthMax, significantly increased the strength of 3D-printed gypsum samples, achieving the objective of replicating high-strength natural rocks with 3D-printed gypsum samples.
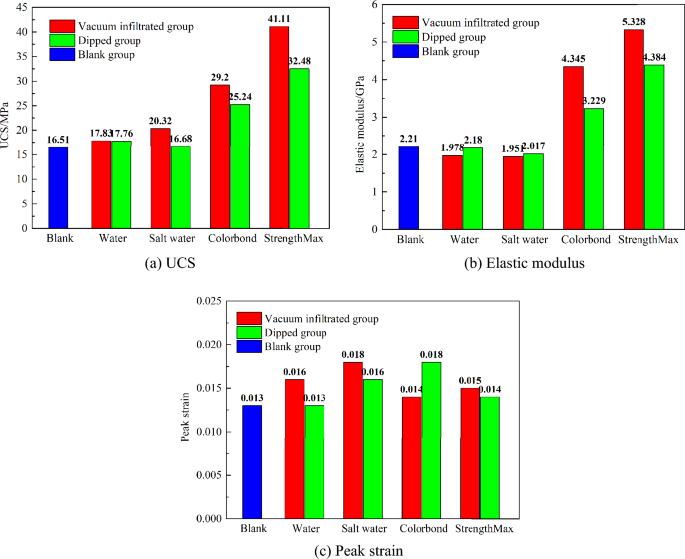
Mechanical properties of 3D-printed samples subjected to different infiltration methods
3.3.3 Failure Modes
The failure mode of 3D-printed samples under uniaxial compression is also crucial for evaluating whether 3D-printed samples can mimic natural rocks. As shown in Fig. 11 , the failure modes of the 3D-printed samples of different infiltration methods were analyzed. The blank group’s failure was primarily due to tensile cracks that were oriented parallel to the bedding planes. These fracture originate from the mechanical anisotropy of 3D printed samples, which is mainly determined by the orientation of the bedding plane, as reported in previous studies (Fereshtenejad and Song 2016 ; Liu et al. 2019 ). Similarly, for the samples that underwent water and saltwater treatment, tensile cracks were observed at failure. However, for the samples treated with ColorBond and StrengthMax, a significantly different failure mode characterized by a combined tensile-shear failure was observed. The main reason for the different failure modes is that, for water and saltwater, the water reacts chemically with the unreacted hemihydrate gypsum to form dihydrate gypsum. However, upon heating the 3D-printed sample in the oven, the excess water evaporates, and the sample maintains its anisotropic characteristics. ColorBond and StrengthMax, on the other hand, do not react with the gypsum components. They act as a binder for gypsum particles and fill in the pores even after heating. As a result, they eliminate or weaken the anisotropic characteristics of the samples, altering their failure mode from tensile into shear failure. This is currently the only known method to eliminate the anisotropic characteristics of 3D-printed gypsum samples and achieve the replication of natural rocks with isotropic mechanical characteristics.
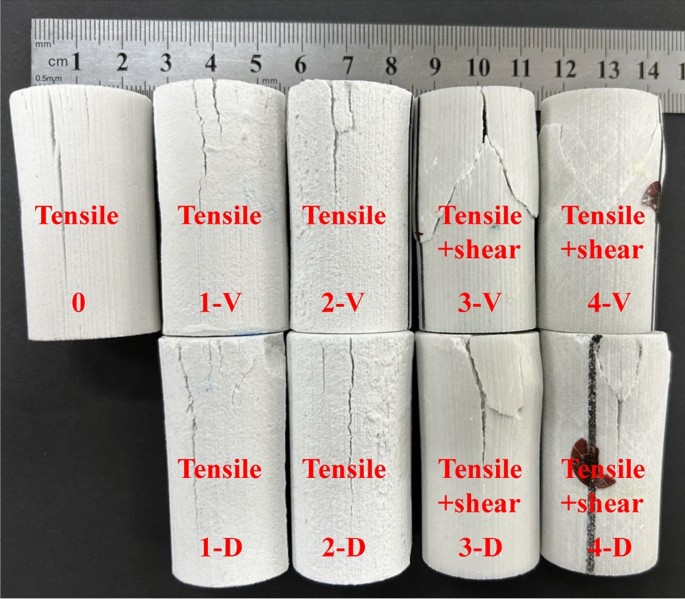
Failure modes of 3D-printed samples after uniaxial compression tests
3.4 Microstructural Change
3.4.1 3d pore network model and porosity change.
After performing geometric and grayscale alignments, threshold segmentation, and 3D reconstruction, the 3D pore network models of the 3D-printed samples before and after vacuum infiltration treatment were successfully obtained, as shown in Fig. 12 . The gray cylindrical model represents the 3D reconstructed model of the sample, and the blue cubic model represents the pore network model. The multi-colored arrow indicates the porosity, where yellow and red colors denote low and high porosity. The porosity of the 3D-printed sample can be defined as the ratio of the total pore volume to the whole sample volume, as shown in the following formula:
where P is the porosity in 3D. V pores denotes the total volume of pores, and V matrix denotes the whole volume of the sample.
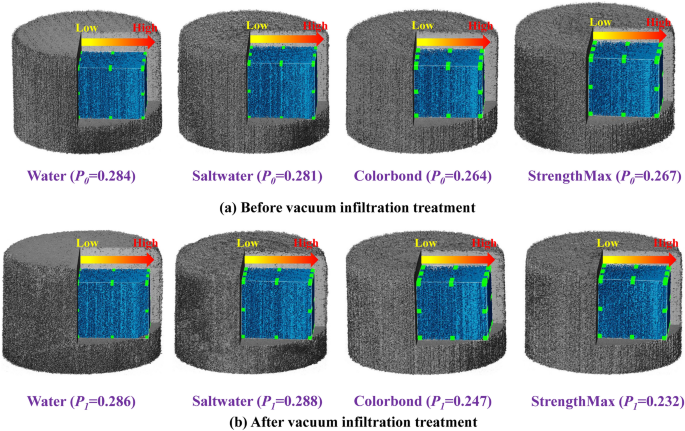
3D reconstruction and pore structure model. The arrow denotes the porosity for which yellow and red indicate low and high porosity, respectively. P 0 is the porosity measured before vacuum infiltration treatment, and P 1 is the porosity measured after vacuum infiltration treatment
The initial porosity ( P 0 ) of 3D-printed samples before vacuum infiltration treatment using water, saltwater, ColorBond, and StrengthMax were 0.284, 0.281, 0.264, and 0.267, respectively. The final porosity ( P 1 ) of these samples were 0.286, 0.288, 0.247, and 0.232, respectively. After vacuum infiltration treatment, the porosity of samples that used ColorBond and StrengthMax decreased by 0.017 and 0.035, while of those that used water and saltwater increased by 0.002 and 0.007. According to the 3D-reconstructed pore network model, the porosity inside the 3D-printed samples gradually increased from the center to the sample boundary. This trend attributes to the fact that moisture evaporates faster near the sample surface during the heating process of the 3D-printed samples, resulting in higher porosity in these regions.
3.4.2 Porosity Change at Different Elevations
The porosity of 3D-printed samples at different elevations can be defined by the ratio of the pore area to the sample area in 2D CT images, as in the following formula:
where P′ is the porosity in 2D. A pores denotes the total area of pores, and A matrix denotes the whole area of the sample.
Figure 13 shows the porosity of 3D-printed samples at different elevations before and after vacuum infiltration treatment using different infiltrants. Figure 13 a, b, c, and d represent the porosity change of 3D-printed samples at different elevations when using water, saltwater, ColorBond, and StrengthMax, respectively. Overall, the results indicate that the porosity of 3D-printed samples treated with the same infiltrant does not vary significantly by elevation, due to the highly uniform pore distribution of 3D printing. At the same elevation, the porosity ( \({P}_{1}{\prime}\) ) of 3D-printed samples treated with water and saltwater is observed to be larger than their initial porosity ( \({P}_{0}{\prime}\) ), while the samples treated with ColorBond and StrengthMax show smaller porosity after treatment.
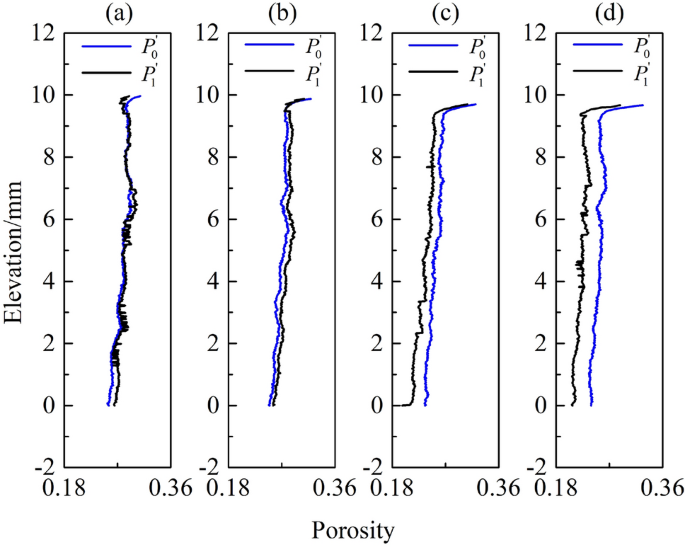
Relationship between elevations and porosity of 3DP samples with different infiltrated materials, where Fig. a, b, c and d denote water, saltwater, ColorBond and StrengthMax groups, respectively. \({P}_{0}^{\mathrm{^{\prime}}}\) is the porosity measured before vacuum infiltration treatment. \({P}_{1}^{\mathrm{^{\prime}}}\) is the porosity measured before vacuum infiltration treatment
To highlight the changes in porosity of the 3D-printed samples before and after vacuum infiltration treatment at different elevations, the porosity difference ( \(\Delta {P}\) ) was calculated using Eq. 3 .
Figure 14 shows the porosity differences of the 3D printed-samples before and after vacuum infiltration treatment when using different infiltrants. The results indicate that the porosity of the 3D-printed samples treated with ColorBond and StrengthMax were decreased ( \(\Delta {P}<0\) ), while the porosity of the 3D printed-samples treated with water and saltwater were increased ( \(\Delta {P}>0\) ). Specifically, the porosity of 3D-printed samples treated with StrengthMax decreased most significantly, with porosity differences in the range of -0.02 to -0.04. The porosity difference of the 3D-printed samples treated with ColorBond mainly distributed around −0.015. The porosity difference after saltwater treatment was slightly greater than that after water treatment, mainly due to the greater solubility of gypsum in saltwater. It is worth noting that for elevations higher than 9.5 mm, which is in the vicinity of the sample upper boundary, the porosity differences of the 3D-printed samples treated with water and saltwater were both less than 0. This is because the sample surface was severely damaged due to direct contact with water and saltwater, as shown in Fig. 6 b and c.
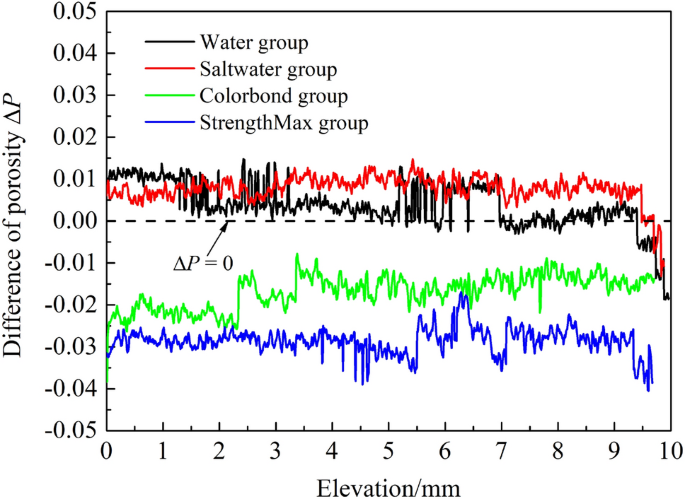
Porosity change of 3D-printed samples at different elevations after infiltration
3.4.3 Degree of Infiltration
The mechanical properties of 3D-printed samples after vacuum infiltration are directly related to the degree of which the infiltrants have penetrated into the sample. A higher degree of infiltration implies that more infiltrants have filled the pores, resulting in increased mechanical strength. Conversely, a lower degree of infiltration implies less pores are filled and lower mechanical strength. To investigate the degree of infiltration, the sample was sawed along an elevation 8 mm from the top of the sample after vacuum infiltration treatment. The observed cross sections are shown in Fig. 15 . d and ID represent the boundary distance, which is the distance from the sample center to infiltration boundary, and the depth of infiltration, respectively.
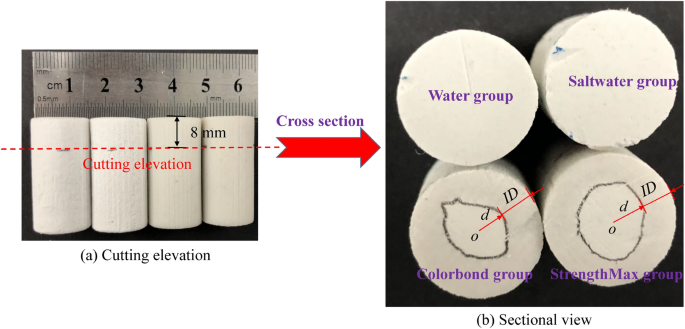
Cross section of 3D-printed samples treated by different infiltrants
As shown in Fig. 15 , it is apparent that the 3D-printed samples treated with ColorBond and StrengthMax have clear boundaries between the infiltrated region and non-infiltrated region, and that the shape of the boundary is elliptical. However, the cross-sections of the 3D-printed samples treated with water and saltwater do not show such clear boundaries, mainly because the moisture inside the 3D printed samples evaporates during the heat treatment. The ellipse in the 3D-printed sample treated with StrengthMax has a larger area than that of ColorBond, indicating that the degree of infiltration for StrengthMax is smaller. To quantitatively characterize the degree of infiltration, a new indicator δ is proposed as described by Eq. 4 .
where δ represents the degree of infiltration, ranging from 0 to 1. A 1 represents the area of the ellipse formed by the infiltration boundary, and A 0 represents the cross-sectional area of the sample. Table 4 presents the infiltration depth ( ID ), distance ( d ) and degree of infiltration ( δ ) for 3D-printed samples treated with ColorBond and StrengthMax. The infiltration depth of ColorBond-treated samples ranged from 1.99 to 5 mm, while the infiltration depth of StrengthMax-treated samples ranged from 2.2 to 2.7 mm. This reveals that the anisotropy of infiltration is stronger in the ColorBond-treated samples. In terms of the degree of infiltration, the ColorBond-treated samples showed a higher degree of infiltration (0.77) than the StrengthMax-treated samples (0.66). This reflects the fact that ColorBond has a lower viscosity than StrengthMax, making it easier to infiltrate into the pore channels of the 3D-printed sample.
A novel method is proposed in this study to quantify the degree of infiltration using 2D CT images. The degree of infiltration is derived by comparing the changes in porosity within different regions of interest (ROIs) of 2D CT images before and after vacuum infiltration treatment. 2D CT images of 3D-printed samples before and after vacuum infiltration treatment were first selected at the same elevation as the cross sections of Fig. 15 . Then, as depicted in Fig. 16 , circular ROIs with radius ranging from 1 to 7.5 mm were defined. The porosity values derived from each ROI were then plotted against their corresponding ROI radius to visualize the spatial variation of porosity within the sample. Figure 17 shows the observed spatial variation of porosity before and after vacuum infiltration treatment using different infiltrants. The plots illustrate a gradual increase in porosity for regions closer to the sample boundary, which is in line with the results presented in Fig. 12 . Comparing the effect of different infiltrants, the porosity increased in all ranges of ROI radiuses for the samples treated with water and saltwater. However, for samples treated with ColorBond and StrengthMax, the porosity increased within a certain radius and decreased beyond this radius. This shift in porosity is closely related to the degree of infiltration, as the pores filled with infiltrants exhibit a decrease of porosity, and the pores without infiltrants exhibit an increase of porosity due to the evaporation of water after heating treatment. Therefore, the critical radius of this porosity shift can be used as an indicator to assess the infiltration depth and the degree of infiltration.
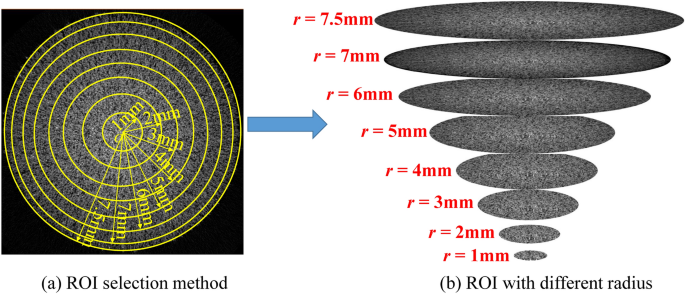
Selection scheme of circular ROIs of CT image of 3D-printed rocks
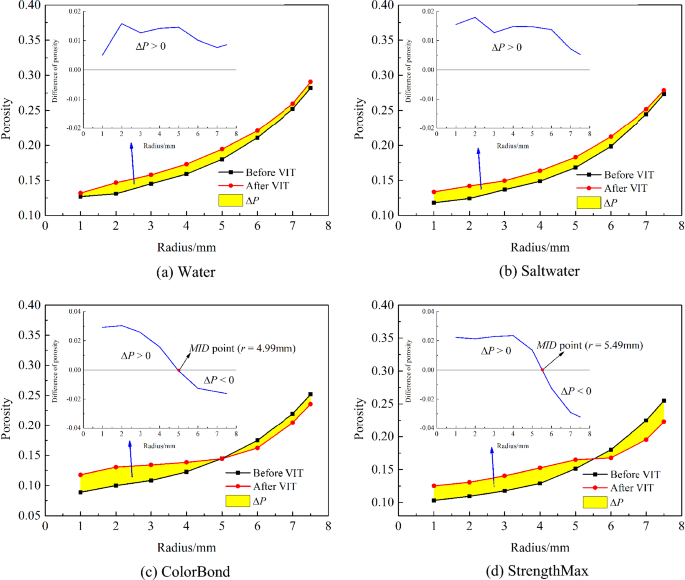
Porosity distribution of 3D-printed gypsum rocks treated with different infiltrants
The degree of infiltration can be derived using Eq. 4 based on the critical radius. To validate the effectiveness of this derivation, the degree of infiltration derived from 2D CT images ( δ 1 ) was compared with that observed from the sample cross sections ( δ 0 ). Table 5 presents the infiltration depth and degree of infiltration derived from the 2D CT images of the samples treated with ColorBond and StrengthMax. The degree of infiltration for ColorBond and StrengthMax were derived to be 0.56 and 0.462, respectively. It is worth noting that the degree of infiltration derived from the 2D CT images is lower than that observed from the cross sections. Several reasons can be listed to why δ 1 is lower than δ 0 . Firstly, the ROI has circular shape while the actual non-infiltrated area is irregular and elliptical. Secondly, the critical radius is calculated as an intersection of two curves, rather than obtaining the exact value.
Method one’s accuracy in determining δ 0 hinges on the clarity of boundaries between infiltrated and non-infiltrated regions, making it a straightforward and time-efficient approach. However, its effectiveness is compromised when infiltrants fail to create distinct boundaries, as demonstrated in the water and saltwater groups in this study. On the other hand, method two, calculating δ 1 , offers greater flexibility in infiltrant selection. It is less constrained by the choice of infiltrants. However, its precision is contingent on several factors, including Micro-CT scanning resolution, accurate alignment of scanned regions, proper calibration of CT images in both scans, and the choice of an appropriate threshold for segmentation. When deciding which method to use for evaluating the degree of infiltration, it’s imperative to carefully consider the specific experimental conditions and requirements to choose the one that best suits your needs.
3.4.4 Pore Characteristics
Infiltrants are considered to improve the strength of gypsum samples by filling the sample pores, enhancing the binding force between the particles, and forming chemical bonds between the matrix material (Fan and Khodadadi 2011 ). To explicitly observe such effects, the pore changes of the 3D-printed samples before and after vacuum infiltration treatment were compared using 2D CT images. As shown in Fig. 18 , there was no significant change in the pores of the 3D-printed samples when treated with water or saltwater. On the other hand, a noticeable reduction in the number of pores was observed in 3D-printed samples treated with ColorBond and StrengthMax. As depicted by the yellow dashed areas in Fig. 18 , the CT images illustrate strong evidence that ColorBond and StrengthMax have pore-filling effects on 3D-printed samples. This observation also indicates the possibility of using vacuum infiltration technique for eliminating or reducing the anisotropic characteristics of 3D-printed gypsum samples for modeling isotropic rocks. However, it should be noted that only a certain portion of connected pores can be filled with infiltrants. In order to improve the strength and isotropy of 3D-printed samples, it is necessary to increase the degree of infiltration, i.e., the infiltration depth of vacuum infiltration treatment, which is also the focus of further research.
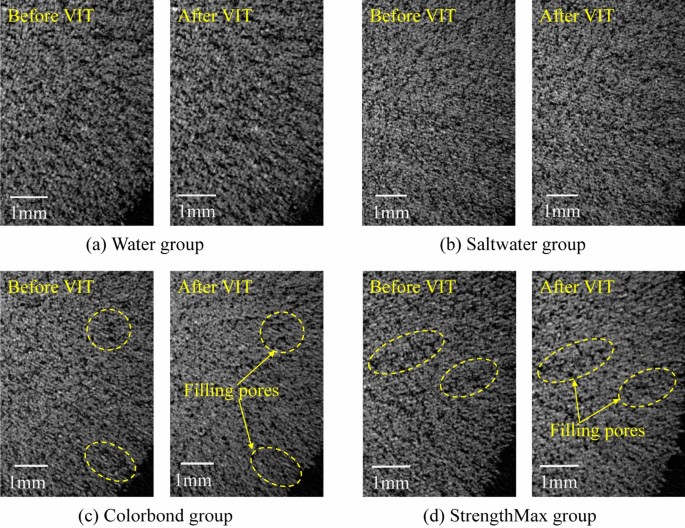
Pore changes observed in 2D CT images of 3D-printed samples before and after vacuum infiltration treatment (VIT)
3.5 Strength Enhancement Mechanism
3.5.1 xrd analysis.
The XRD patterns were measured with an X-ray diffractometer equipped with Cu-Kα radiation (λ = 1.5418 Å) in the range of 2θ between 5° and 60°, and with setting conditions of 40 kV of voltage, 40 mA of current, 0.02° of step size, and 1°/min of scanning speed. In our previous study (Shao et al. 2023 ), the printing principle for the formation of 3D-printed gypsum samples involved a chemical reaction between CaSO 4 ·1/2H 2 O in the raw printing material and the water in the binder, resulting in the generation of CaSO 4 ·2H 2 O, as represented by Eq. 5 .
As shown in Fig. 19 , it was evident that XRD patterns imply that all groups of 3D-printed samples primarily consist of CaSO 4 ·1/2H 2 O, CaSO 4 ·2H 2 O, and to a lesser extent CaSO 4 . This suggests that post-processing treatments used in this study did not introduce another new crystalline substance to the 3D-printed samples. It was worth noting that the peak intensity (when 2θ = 11.55° and 14.69°) of 3D-printed samples subjected to water and saltwater treatments showed significant changes relative to the peak intensity of the other groups. Specifically, the peak intensity of CaSO 4 ·1/2H 2 O decreased while the peak intensity of CaSO 4 ·2H 2 O increased. This was primarily due to water reacting with the residual CaSO 4 ·1/2H 2 O in the 3D-printed samples, resulting in the generation of new CaSO 4 ·2H 2 O. In contrast, the XRD pattern for 3D-printed samples subjected to Colorbond and StrengthMax treatments closely resembled that of untreated 3D-printed samples. This indicates that Colorbond and StrengthMax do not undergo a chemical reaction with the 3D-printed samples but rather serve to physically fill voids and bond particles, a phenomenon also confirmed in the SEM images in Fig. 20 .
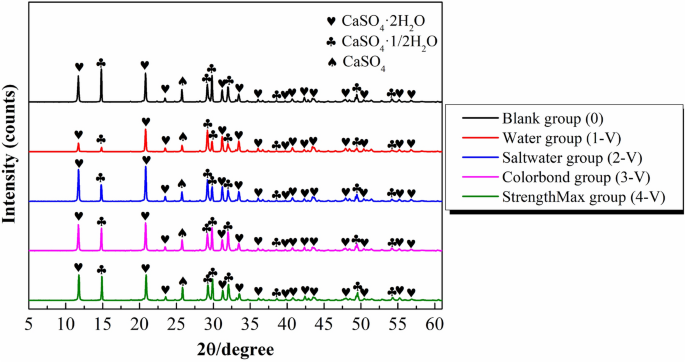
XRD results of 3D-printed samples after vacuum infiltration treatment with different infiltrants
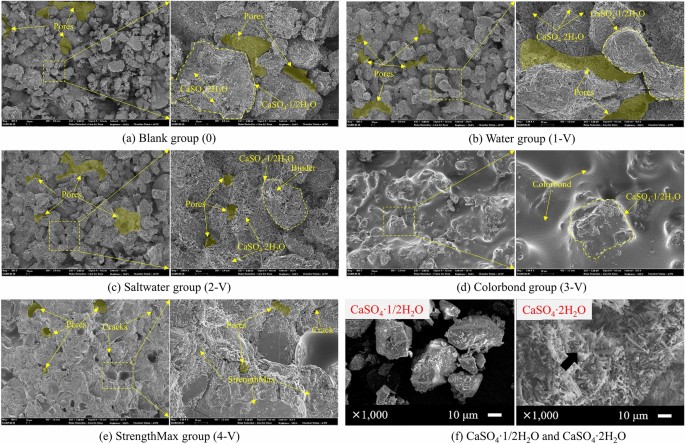
SEM images of 3D-printed samples after vacuum infiltration treatment with different infiltrations
3.5.2 SEM Analysis
For analyzing the microstructure of 3D-printed samples after various post-treatment methods, SEM tests were conducted on a ZEISS SIGMA FE-SEM with a magnification range of 12–10 6 and a scan voltage range of 0.1–30 kV. 3D-printed samples subjected to various post-processing methods were examined using FE-SEM at magnifications of 200 and 2000 times to observe pore distribution, particle shapes, the morphology of cementitious materials, and inter-particle bonding within the 3D-printed samples. Figure 20 a presents an SEM image of the 3D-printed sample without undergoing any post-processing. Figure 20 b–e display SEM images of 3D-printed samples subjected to vacuum infiltration treatment with water, saltwater, Colorbond, and StrengthMax, respectively. Hamano et al. ( 2021 ) conducted SEM tests on extracted CaSO 4 ·1/2H 2 O and CaSO 4 ·2H 2 O from 3D-printed gypsum samples in order to comprehensively reveal the microscopic compositional changes, as depicted in Fig. 20 f. In comparison to CaSO 4 ·1/2H 2 O in the raw printing materials, the newly formed CaSO 4 ·2H 2 O after printing exhibited smaller dimensions, a more elongated, needle-like shape, and interconnected attachments to larger CaSO 4 ·1/2H 2 O, thus forming particles with a degree of strength. When 3D-printed samples underwent no treatment, particles were distinctly visible, and inter-particle pores were prominent, with connections between particles appearing very loose and fragile. This factor contributes to the low macroscopic strength of 3D-printed samples. Similarly, in the water and saltwater treatment groups, a significant number of pores were observed. Notably, more CaSO 4 ·2H 2 O particles were attached around the CaSO 4 ·1/2H 2 O particles, mainly due to a more thorough hydration reaction within the samples, in accordance with the XRD results. However, for 3D-printed samples underwent Colorbond and StrengthMax treatment, particles were challenging to visually identify. Pores between particles were either fully or partially covered, forming a cohesive structure that significantly improved the macroscopic strength of the 3D-printed samples. Since Colorbond has higher fluidity compared to StrengthMax, it demonstrated better pore-filling efficiency between particles. It was worth noting that while it could infiltrate more Colorbond into 3D-printed samples under the same vacuum pressure, its enhancement of macroscopic strength was lower than that achieved by StrengthMax. This is primarily due to Colorbond’s lower strength after curing compared to the cured strength of StrengthMax.
To intuitively illustrate the impact of different infiltrants on the microstructure of 3D-printed samples, Fig. 21 provides a schematic representation of the effect of these infiltrants on sample strength. In the untreated 3D-printed samples, the bonding between particles (primarily composed of CaSO 4 ·1/2H 2 O and CaSO 4 ·2H 2 O) relies on the adhesive properties of binder. However, due to the relatively weak adhesive and the presence of numerous pores, the samples exhibit weaker mechanical characteristics on a macroscopic scale. 3D-printed samples subjected to water or saltwater treatment undergo changes in particle shape and size. This transformation primarily occurs because water reacts chemically with CaSO 4 ·1/2H 2 O within the sample. Nonetheless, the presence of numerous pores and the primary particle bonding via the adhesive still result in low sample strength. In contrast, samples treated with Colorbond or StrengthMax treatment demonstrate a distinct behavior. These infiltrants effectively fill the gaps between particles and form a continuous covering that envelops the connected particles, creating a unified structure that exhibits high-strength mechanical properties on a macroscopic scale. It is worth noting that, compared to Colorbond-treated samples, even though StrengthMax-treated samples have more pores, they can still display higher macroscopic strength.
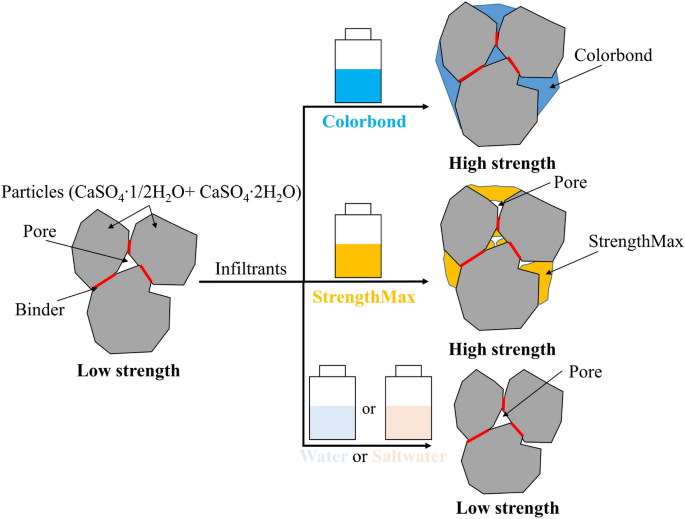
Schematic of strength enhancement mechanism of 3D-printed samples treated with different infiltrants
4 Discussion
4.1 comparison of physical and mechanical properties between 3d-printed rocks after vacuum infiltration treatment and natural rocks, 4.1.1 comparison of wave properties between 3d-printed gypsum rocks and natural rocks.
The ultrasonic wave velocity of natural rocks is an important property that holds significant implications for various fields, including the study of Earth’s interior, mineral resource exploration and mining, and the design of rock engineering projects (Wyllie et al. 1958 ). In order to assess the feasibility of using 3DP technology to replicate natural rocks, it is essential to compare the ultrasonic wave velocity properties of 3D-printed rocks with those of natural rocks. As shown in Fig. 22 , the ultrasonic wave velocity of 3D-printed rocks were compared with the ultrasonic wave velocity of natural rocks reported in literature (Mavko et al. 2020, Tendürüs et al. 2010 , Wightman et al. 2003, Wyllie et al. 1956 ). The solid line represents the ultrasonic wave velocity of untreated 3D-printed rocks, and the dashed line represents the ultrasonic wave velocity of 3D-printed rocks subjected to vacuum infiltration treatment using StrengthMax. The comparison shows that the P-wave and S-wave velocities of untreated 3D-printed rocks only match those of low-strength sandstones. However, the 3D-printed rocks treated with StrengthMax show significant improvements in P-wave and S-wave velocities, with velocities similar to those of sandstones, shales, and mudstones. Nevertheless, there still remains a notable disparity between the ultrasonic wave velocity of 3D-printed rocks and rocks such as granite, marble, basalt, and gneiss, indicating that 3D-printed gypsum samples are unable to effectively replicate rocks with high wave velocities ultrasonic wave velocities.
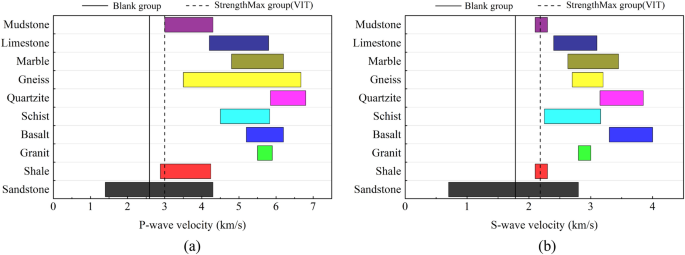
Comparison of wave velocity between natural rocks and 3-D printed gypsum rock
4.1.2 Comparison of Mechanical Properties between 3D-Printed Gypsum Rocks and Natural Rocks
In addition to ultrasonic wave velocity, a comparison was made between the uniaxial compressive strength and elastic modulus of natural rocks and 3D-printed rocks. The strength and elastic modulus values of natural rocks were obtained from literature sources and used for comparison. (Attewell and Farmer 2012, Dinçer et al. 2004 , Dyke and Dobereiner 1991, Josh et al. 2012 , Kong et al. 2018 , Moradian and Behnia 2009 , Nasseri et al. 2003 , Rybacki et al. 2015 , Santi et al. 2000 , Schön 2015, Shalabi et al. 2007 , Shirlaw et al. 2018 , Yang et al. 2009 , Yılmaz and Sendır 2002 ). Similar to Fig. 22 , the solid line in Fig. 23 represents the properties of untreated 3D-printed rocks, and the dashed line represents that of 3D-printed rocks subjected to vacuum infiltration treatment using StrengthMax. The comparison shows that while the strength of untreated 3D-printed rocks may be comparable to certain low-strength natural rocks, such as sandstones, shales, and mudstones, their high plasticity imposes limitations on replicating a broader range of natural rocks. In contrast, 3D-printed gypsum rocks treated with StrengthMax exhibit higher strength that is comparable to that of sandstones, shales, mudstones, limestones, and some slates, while also having higher elastic modulus that can match those of sandstones, slates, and mudstones. According to the engineering rock classification by Deere and Miller (1966), the untreated 3D-printed rocks can be classified as class E, very low in strength, and 3D-printed rocks after vacuum infiltration treatment can be classified as class D, low in strength. This indicates that 3D-printed rocks treated with StrengthMax can replicate a wider range of natural rocks. Nevertheless, it should be noted that 3D-printed gypsum rocks are still not suitable for replicating high-strength natural rocks such as granite, marble, basalt, gneiss and quartzite.
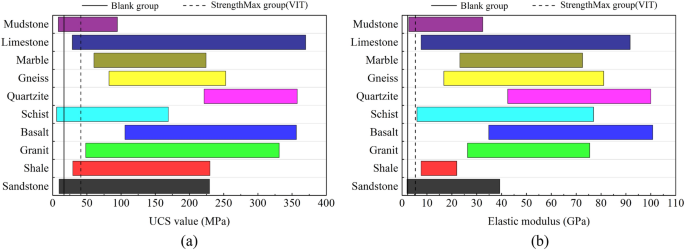
Comparison of mechanical properties between natural rocks and 3-D printed gypsum rock
4.2 Comparison of Post-Processing Methods for Strength Enhancement of 3D-Printed Gypsum Rocks
Table 6 provides a summary of common strategies employed to enhance the strength of 3D-printed gypsum samples. Four methods have been identified for strength enhancement: 1. Optimization of printing parameters, 2. Heat treatment, 3. Dipped infiltration treatment and 4. Vacuum infiltration treatment. Optimizing the printing parameters, including printing direction, binder saturation level, layer thickness and printing delay time, can yield relatively strong samples. However, there still exists a substantial gap in strength and stiffness between printed rocks and natural rocks. Compared to the optimization of printing parameters, heating treatment proves to be a more effective method for strength enhancement. It is crucial to emphasize that the selection of heating temperature and duration is critical during the heat treatment process. Excessive temperature or prolonged heating time may damage the bonding strength of the binder and lead to the decomposition of gypsum particles. Conversely, inadequate temperature or insufficient heating time may result in insufficient moisture evaporation.
Compared with the other methods, infiltration treatment stands out as the most promising method for enhancing the strength of 3D-printed samples. However, it is important to note that the degree of infiltration directly determines the strength and stiffness of the sample. Additionally, careful selection of infiltrants is crucial as different infiltrants show notable variations in strength. Based on the findings of this study, StrengthMax demonstrates the most promising outcomes for strength enhancement. Comparing the application methods of infiltration, vacuum infiltration is proved to be more effective than dipped infiltration because it achieves a higher degree of infiltration, yielding a higher improvement in strength. It is notable that the strength of 3D-printed samples that have undergone ColorBond treatment using vacuum pressure of −120 kPa is reported to be 56.7 MPa (Zhang et al., 2020), which is greater than that of 3D-printed samples that have undergone StrengthMax treatment using vacuum pressure of − 20 kPa (41.11 MPa). This indicates that the magnitude of vacuum pressure also plays a key role in strength enhancement.
The following ranking can be made based on the comparison of common strategies of strength enhancement for 3D-printed gypsum samples: vacuum infiltration treatments > dipped infiltration treatments > heat treatments > printing parameters. Overall, by combining different strength enhancement methods, it is believed that the replication of high-strength rocks using 3D-printed gypsum rocks can be achieved. This advancement holds great potential for the widespread application of 3DP technology in rock mechanics in the future.
5 Conclusion
In this study, we investigated the effects of dipped infiltration treatment and vacuum infiltration treatment on the physical and mechanical properties of 3D-printed gypsum samples. Through a comparison of the mechanical properties of samples treated with water, saltwater, ColorBond and StrengthMax, StrengthMax was revealed to be the optimal infiltrant for strength enhancement. Additionally, the microstructural changes of 3D-printed gypsum samples before and after vacuum infiltration treatment were analyzed using CT scanning technology. Finally, the microscopic mechanisms and reasons behind the macroscopic strength changes in 3D-printed samples were explored by conducting XRD tests and SEM tests. The key findings from this study can be summarized as follows:
Vacuum infiltration treatment was found to be more effective than dipped infiltration treatment in enhancing the strength and stiffness of 3D-printed gypsum samples. The strength enhancement effect of different infiltrants increased in the order of water, saltwater, ColorBond, and StrengthMax, with StrengthMax exhibiting the most significant effect.
When 3D-printed gypsums were treated with water or saltwater, only tensile cracks were observed at failure, similar to untreated samples. However, samples treated with ColorBond and StrengthMax showed a significant change in failure mode, transitioning from a purely tensile failure to a combined tensile-shear failure. This transformation can be attributed to the adhesive properties of ColorBond or StrengthMax, which act as a bonding agent for gypsum particles, effectively eliminating or reducing the anisotropic characteristics of 3D-printed gypsum rocks.
After vacuum infiltration treatment, the porosity of ColorBond and StrengthMax-treated samples decreased by 0.017 and 0.035, respectively, while the porosity of water and saltwater-treated samples increased by 0.002 and 0.007, respectively. This phenomenon is mainly due to moisture evaporation during the heating process. In terms of the degree of infiltration, the ColorBond-treated samples showed a higher degree of infiltration ( δ = 0.77) than the StrengthMax-treated samples ( δ = 0.66), due to the fact that ColorBond has lower viscosity and can more easily infiltrate into the 3D-printed samples.
Water and saltwater treatments alter particle characteristics through chemical reactions, but numerous pores and primary adhesive bonding still yield low sample strength. In contrast, Colorbond and StrengthMax-treated samples exhibit superior strength, with effective gap filling and cohesive structures. Compared to Colorbond-treated samples, StrengthMax-treated samples still display higher macroscopic strength, despite them having more pores than Colorbond-treated ones.
Data availability
The data that support the findings of this study are available from the corresponding author, upon reasonable request.
Ayres TJ, Sama SR, Joshi SB, Manogharan GP (2019) Influence of resin infiltrants on mechanical and thermal performance in plaster binder jetting additive manufacturing. Addit Manuf 30:100885
CAS Google Scholar
Di Buduo CA, Soprano PM, Tozzi L, Marconi S, Auricchio F, Kaplan DL, Balduini A (2017) Modular flow chamber for engineering bone marrow architecture and function. Biomaterials 146:60–71
Article Google Scholar
Dinçer I, Acar A, Çobanoğlu I, Uras Y, Environment (2004) Correlation between schmidt hardness, uniaxial compressive strength and young’s modulus for andesites, basalts and tuffs. Bulletin of Engineering Geology 63:141–148
Fan L, Khodadadi JM (2011) Thermal conductivity enhancement of phase change materials for thermal energy storage: a review. Renewable Sustainable Energy Reviews 151:24–46
Farzadi A, Solati-Hashjin M, Asadi-Eydivand M, Abu Osman NA (2014) Effect of layer thickness and printing orientation on mechanical properties and dimensional accuracy of 3D printed porous samples for bone tissue engineering. PLoS ONE 99:e108252
Farzadi A, Waran V, Solati-Hashjin M, Rahman ZAA, Asadi M, Osman NAA (2015) Effect of layer printing delay on mechanical properties and dimensional accuracy of 3D printed porous prototypes in bone tissue engineering. Ceram Int 417:8320–8330
Feng X-T, Gong Y-H, Zhou Y-Y, Li Z-W, Liu X-F (2019) The 3D-printing technology of geological models using rock-like materials. Rock Mechanics Rock Engineering 52:2261–2277
Feng X, Xue F, Carvelli V, Zhu Y, Zhao T (2022) Mechanical performance of a 3D printed scaled mine roadway model. International Journal of Rock Mechanics Mining Sciences 158:105173
Fereshtenejad S, Kim J, Song J-J (2021) The effect of joint roughness on shear behavior of 3D-printed samples containing a non-persistent joint. Petrophysics 6205:553–563
Google Scholar
Fereshtenejad S, Song JJ (2016) Fundamental study on applicability of powder-based 3D printer for physical modeling in rock mechanics. Rock Mechanics Rock Engineering 49:2065–2074
Gao Y-t, T-h Wu, Zhou Y (2021) Application and prospective of 3D printing in rock mechanics: a review. International Journal of Minerals, Metallurgy Materials 28:1–17
Article CAS Google Scholar
Hamano R, Nakagawa Y, Irawan V, Ikoma T (2021) Mechanical anisotropy and fracture mode of binder jetting 3D printed calcium sulfate moldings. Appl Mater Today 25:101160
He F, Liu Q, Deng P (2020) Investigation of the anisotropic characteristics of layered rocks under uniaxial compression based on the 3D printing technology and the combined finite-discrete element method. Advances in Materials Science Engineering 2020:1–10
Huang T, Yang G, Tang G (1979) A fast two-dimensional median filtering algorithm. IEEE Transactions on Acoustics, Speech, Signal Processing 271:13–18
Ishutov S, Hasiuk F (2019) Editorial for special issue on 3D printing. Transp Porous Media 129:427–430
Jiang C, Zhao G-F (2015) A preliminary study of 3D printing on rock mechanics. Rock Mechanics Rock Engineering 48:1041–1050
Jiang C, Zhao G-F, Zhu J, Zhao Y-X, Shen L (2016a) Investigation of dynamic crack coalescence using a gypsum-like 3D printing material. Rock Mechanics Rock Engineering 49:3983–3998
Jiang Q, Feng X, Song L, Gong Y, Zheng H (2016b) Modeling rock specimens through 3D printing: tentative experiments and prospects. Acta Mech Sin 32:101–111
Jiang Q, Feng X, Song L, Gong Y, Zheng H, Cui J (2016c) Modeling rock specimens through 3D printing: tentative experiments and prospects. Acta Mech Sin 32:101–111
Jiang Q, Song L (2018) Application and prospect of 3D printing technology to physical modeling in rock mechanics. Chinese Journal of Rock Mechanics Engineering 371:23–37
Josh M, Esteban L, Delle Piane C, Sarout J, Dewhurst D, Clennell M (2012) Laboratory characterisation of shale properties. Journal of Petroleum Science Engineering 88:107–124
Kalender WA, Polacin A (1991) Physical performance characteristics of spiral CT scanning. Med Phys 185:910–915
Kong L, Ostadhassan M, Li C, Tamimi N (2018) Can 3-D printed gypsum samples replicate natural rocks? an experimental study. Rock Mechanics Rock Engineering 51:3061–3074
Kong L, Ostadhassan M, Liu B, Li C, Liu K (2019) Multifractal characteristics of MIP-based pore size distribution of 3D-printed powder-based rocks: a study of post-processing effect. Transp Porous Media 129:599–618
Kyriakou D (2013) Keeping up with best practice. Plumbing Connection Summer 2013:48–51
Li B, Wang J, Liu R, Jiang Y (2021) Nonlinear fluid flow through three-dimensional rough fracture networks: insights from 3D-printing, CT-scanning, and high-resolution numerical simulations. Journal of Rock Mechanics Geotechnical Engineering 135:1020–1032
Li L, Yang J, Fu J, Wang S, Zhang C, Xiang M (2022a) Experimental investigation on the invert stability of operating railway tunnels with different drainage systems using 3D printing technology. Journal of Rock Mechanics Geotechnical Engineering 145:1470–1485
Li L, Yang J, Fu J, Wang S, Zhang C, Xiang M, Engineering G (2022b) Experimental investigation on the invert stability of operating railway tunnels with different drainage systems using 3D printing technology. Journal of Rock Mechanics Geotechnical Engineering 145:1470–1485
Liu Q, He F, Deng P, Tian Y (2019) Application of 3D printing technology in physical modelling in rock mechanics. Rock Soil Mechanics 4009:3397–3404
Mandal S, Basu B (2018) Probing the influence of post-processing on microstructure and in situ compression failure with in silico modeling of 3D-printed scaffolds. J Mater Res 3314:2062–2076
Mei S, Feng X-T, Li Z, Yang C, Gao J (2022) A novel 3D printing technology for synthetic hard rock and the fabrication of jinping marble. Rock Mechanics Rock Engineering 5512:7695–7714
Moradian Z, Behnia M (2009) Predicting the uniaxial compressive strength and static young’s modulus of intact sedimentary rocks using the ultrasonic test. Int J Geomech 91:14–19
Nakashima H, Yukawa Y, Ito K, Horie Y, Machino M, Kanbara S, Morita D, Imagama S, Ishiguro N, Kato F (2011) Extension CT scan: its suitability for assessing fusion after posterior lumbar interbody fusion. Eur Spine J 20:1496–1502
Nasseri M, Rao K, Ramamurthy T (2003) Anisotropic strength and deformational behavior of himalayan schists. International Journal of Rock Mechanics Mining Sciences 401:3–23
Rodríguez-González P, Zapico P, Robles-Valero P, Barreiro J (2022) Novel post-processing procedure to enhance casting molds manufactured by binder jetting AM. Addit Manuf 59:103142
Rybacki E, Reinicke A, Meier T, Makasi M, Dresen G (2015) What controls the mechanical properties of shale rocks?–part I: strength and young’s modulus. Journal of Petroleum Science Engineering 135:702–722
Santi PM, Holschen JE, Stephenson RW (2000) Improving elastic modulus measurements for rock based on geology. Environmental Engineering Geoscience 64:333–346
TF Scientific 2018 Amira-avizo software Thermo Scientific™ Amira‐Avizo Software
Shah RJ, Lagdive SB, Machchhar AH, Periyanan K, Cherian S (2020) Technique to fabricate hollow maxillary complete denture using 3D printed template. J Prosthodont 294:360–363
Shakor P, Chu S, Puzatova A, Dini E (2022) Review of binder jetting 3D printing in the construction industry. Progress in Additive Manufacturing 74:643–669
Shalabi FI, Cording EJ, Al-Hattamleh OH (2007) Estimation of rock engineering properties using hardness tests. Eng Geol 903–4:138–147
Shao YL, Yang JW, Kim J et al (2023) A comprehensive experimental study on mechanical anisotropy and failure mode of 3D printed gypsum rocks: from composition and microstructure to macroscopic mechanical properties response. Rock Mech Rock Eng 56:1–26
Sharafisafa M, Shen L, Xu Q (2018a) Characterisation of mechanical behaviour of 3D printed rock-like material with digital image correlation. International Journal of Rock Mechanics Mining Sciences 112:122–138
Sharafisafa M, Shen L, Xu Q, Sciences M (2018b) Characterisation of mechanical behaviour of 3D printed rock-like material with digital image correlation. International Journal of Rock Mechanics Mining Sciences 112:122–138
Sharafisafa M, Shen L, Zheng Y, Xiao J (2019) The effect of flaw filling material on the compressive behaviour of 3D printed rock-like discs. International Journal of Rock Mechanics Mining Sciences 117:105–117
J Shirlaw L Pakianathan and J Kumarasamy 2018 A review of the variability of the strength and abrasivity of the rocks of the jurong group and tebak formation. Underground Singapore:
Song L, Jiang Q, Shi Y-E, Feng X-T, Li Y, Su F, Liu C (2018) Feasibility investigation of 3D printing technology for geotechnical physical models: study of tunnels. Rock Mechanics Rock Engineering 51:2617–2637
Studholme C, Hill DL, Hawkes D (1999) An overlap invariant entropy measure of 3D medical image alignment. Pattern Recogn 321:71–86
Suzuki A, Minto JM, Watanabe N, Li K, Horne RN (2019) Contributions of 3D printed fracture networks to development of flow and transport models. Transp Porous Media 129:485–500
Tendürüs M, van Wijngaarden GJ, Kars H, Sintubin M, Stewart I, Niemi T, Altunel E (2010) Long-term effect of seismic activities on archaeological remains: a test study from zakynthos, greece. Ancient Earthquakes 471:145–156
Vaneghi RG, Ferdosi B, Okoth AD, Kuek B (2018) Strength degradation of sandstone and granodiorite under uniaxial cyclic loading. Journal of Rock Mechanics Geotechnical Engineering 101:117–126
Wang P, Huang Z, Ren F, Zhang L, Cai M-f (2020) Research on direct shear behaviour and fracture patterns of 3D-printed complex jointed rock models. Rock Solid Mechanics 411:46–56
Wang W, Zhao Y, Jiang L, Zuo J, Liu G, Mitri HS (2022) Preliminary study on size effect of fractured rock mass with sand powder 3D printing. Processes 1010:1974
Wehr A, Lohr U (1999) Airborne laser scanning—an introduction and overview. Journal of Photogrammetry Remote Sensing 542–3:68–82
Wu Z, Zhang B, Weng L, Liu Q, Wong LNY (2020) A new way to replicate the highly stressed soft rock: 3D printing exploration. Rock Mechanics Rock Engineering 53:467–476
Wyllie M, Gregory A, Gardner G (1958) An experimental investigation of factors affecting elastic wave velocities in porous media. Geophysics 233:459–493
Wyllie MRJ, Gregory AR, Gardner LW (1956) Elastic wave velocities in heterogeneous and porous media. Geophysics 211:41–70
Yang S, Dai Y, Han L, Jin Z (2009) Experimental study on mechanical behavior of brittle marble samples containing different flaws under uniaxial compression. Eng Fract Mech 7612:1833–1845
Yılmaz I, Sendır H (2002) Correlation of schmidt hardness with unconfined compressive strength and young’s modulus in gypsum from sivas (Turkey). Eng Geol 663–4:211–219
Yu C, Tian W, Zhang C, Chai S, Cheng X, Wang X (2021) Temperature-dependent mechanical properties and crack propagation modes of 3D printed sandstones. International Journal of Rock Mechanics Mining Sciences 146:104868
Zhang K, Li N (2022) A new method to replicate high-porosity weak rocks subjected to cyclic freezing-thawing: Sand 3D printing and digital image correlation explorations. International Journal of Rock Mechanics Mining Sciences 157:105174
Zhang QB, Zhao J (2014) A review of dynamic experimental techniques and mechanical behaviour of rock materials. Rock Mechanics Rock Engineering 47:1411–1478
Zhao Y, Fu X, Shi Y, Zhao B, Fu X, Zhang X, Chen Y (2022) Research on mechanical behavior and energy evolution of coal and rocks with different thin spray-on liners thickness under uniaxial compressive loading. Sustainability 1410:5909
Zhou T, Zhu J (2018) Identification of a suitable 3D printing material for mimicking brittle and hard rocks and its brittleness enhancements. Rock Mechanics Rock Engineering 51:765–777
Zhou T, Zhu J, Ju Y, Xie H (2019) Volumetric fracturing behavior of 3D printed artificial rocks containing single and double 3D internal flaws under static uniaxial compression. Eng Fract Mech 205:190–204
Zhou T, Zhu J, Xie H (2020) Mechanical and volumetric fracturing behaviour of three-dimensional printing rock-like samples under dynamic loading. Rock Mechanics Rock Engineering 53:2855–2864
Download references
Acknowledgements
This work was supported by grants from the Human Resources Development program (No. 20204010600250) and the Training Program of CCUS for the Green Growth (No. 20214000000500) by the Korea Institute of Energy Technology Evaluation and Planning (KETEP), funded by the Ministry of Trade, Industry, and Energy of the Korean Government (MOTIE).
Open Access funding enabled and organized by Seoul National University. Korea Institute of Energy Technology Evaluation and Planning, No. 20204010600250, Jae-Joon Song, Ministry of Trade, Industry, and Energy of the Korean Government, No. 20214000000500, Jae-Joon Song.
Author information
Authors and affiliations.
Department of Energy Resources Engineering, Research Institute of Energy and Resources, Seoul National University, Seoul, 08826, Republic of Korea
Yulong Shao, Jineon Kim & Jae-Joon Song
Department of Civil & Environmental Engineering, Seoul National University, 1 Gwanak-ro, Gwanak-gu, Seoul, 08826, Republic of Korea
Jingwei Yang & Juhyuk Moon
You can also search for this author in PubMed Google Scholar
Contributions
Yulong Shao: Experiments, analysis and writing original draft preparation. Jineon Kim: Experiments and writing reviewing. Jingwei Yang: CT scanning experiments. Jae-Joon Song: Supervising, funding and writing reviewing. Juhyuk Moon: CT scanning experiments.
Corresponding author
Correspondence to Jae-Joon Song .
Ethics declarations
Conflict of interest.
The authors declare that they have no conflict of interest.
Additional information
Publisher's note.
Springer Nature remains neutral with regard to jurisdictional claims in published maps and institutional affiliations.
Rights and permissions
Open Access This article is licensed under a Creative Commons Attribution 4.0 International License, which permits use, sharing, adaptation, distribution and reproduction in any medium or format, as long as you give appropriate credit to the original author(s) and the source, provide a link to the Creative Commons licence, and indicate if changes were made. The images or other third party material in this article are included in the article's Creative Commons licence, unless indicated otherwise in a credit line to the material. If material is not included in the article's Creative Commons licence and your intended use is not permitted by statutory regulation or exceeds the permitted use, you will need to obtain permission directly from the copyright holder. To view a copy of this licence, visit http://creativecommons.org/licenses/by/4.0/ .
Reprints and permissions
About this article
Shao, Y., Kim, J., Yang, J. et al. Experimental Study on Strength Enhancement and Porosity Variation of 3D-Printed Gypsum Rocks: Insights on Vacuum Infiltration Post-Processing. Rock Mech Rock Eng (2024). https://doi.org/10.1007/s00603-024-03913-7
Download citation
Received : 18 June 2023
Accepted : 11 April 2024
Published : 07 May 2024
DOI : https://doi.org/10.1007/s00603-024-03913-7
Share this article
Anyone you share the following link with will be able to read this content:
Sorry, a shareable link is not currently available for this article.
Provided by the Springer Nature SharedIt content-sharing initiative
- 3D-printed gypsum rocks
- Degree of infiltration
- Microstructural characteristics
- Mechanical properties
- Strength enhancement
- Vacuum infiltration treatment
- Find a journal
- Publish with us
- Track your research
Help | Advanced Search
Computer Science > Artificial Intelligence
Title: crispr-gpt: an llm agent for automated design of gene-editing experiments.
Abstract: The introduction of genome engineering technology has transformed biomedical research, making it possible to make precise changes to genetic information. However, creating an efficient gene-editing system requires a deep understanding of CRISPR technology, and the complex experimental systems under investigation. While Large Language Models (LLMs) have shown promise in various tasks, they often lack specific knowledge and struggle to accurately solve biological design problems. In this work, we introduce CRISPR-GPT, an LLM agent augmented with domain knowledge and external tools to automate and enhance the design process of CRISPR-based gene-editing experiments. CRISPR-GPT leverages the reasoning ability of LLMs to facilitate the process of selecting CRISPR systems, designing guide RNAs, recommending cellular delivery methods, drafting protocols, and designing validation experiments to confirm editing outcomes. We showcase the potential of CRISPR-GPT for assisting non-expert researchers with gene-editing experiments from scratch and validate the agent's effectiveness in a real-world use case. Furthermore, we explore the ethical and regulatory considerations associated with automated gene-editing design, highlighting the need for responsible and transparent use of these tools. Our work aims to bridge the gap between beginner biological researchers and CRISPR genome engineering techniques, and demonstrate the potential of LLM agents in facilitating complex biological discovery tasks.
Submission history
Access paper:.
- HTML (experimental)
- Other Formats
References & Citations
- Google Scholar
- Semantic Scholar
BibTeX formatted citation

Bibliographic and Citation Tools
Code, data and media associated with this article, recommenders and search tools.
- Institution
arXivLabs: experimental projects with community collaborators
arXivLabs is a framework that allows collaborators to develop and share new arXiv features directly on our website.
Both individuals and organizations that work with arXivLabs have embraced and accepted our values of openness, community, excellence, and user data privacy. arXiv is committed to these values and only works with partners that adhere to them.
Have an idea for a project that will add value for arXiv's community? Learn more about arXivLabs .

IMAGES
VIDEO
COMMENTS
Step 1: Define your variables. You should begin with a specific research question. We will work with two research question examples, one from health sciences and one from ecology: Example question 1: Phone use and sleep. You want to know how phone use before bedtime affects sleep patterns.
Step 1: Define your variables. You should begin with a specific research question. We will work with two research question examples, one from health sciences and one from ecology: Example question 1: Phone use and sleep. You want to know how phone use before bedtime affects sleep patterns.
10 Experimental research. 10. Experimental research. Experimental research—often considered to be the 'gold standard' in research designs—is one of the most rigorous of all research designs. In this design, one or more independent variables are manipulated by the researcher (as treatments), subjects are randomly assigned to different ...
Experimental research is scientifically-driven, quantitative research involving two sets of variables. The first set of variables, known as the independent variables, are manipulated by the researcher, in order to determine the impact on the second set of variables—the dependent variables. Using the experimental method, you can test whether ...
The pre-experimental research design is further divided into three types. One-shot Case Study Research Design. In this type of experimental study, only one dependent group or variable is considered. The study is carried out after some treatment which was presumed to cause change, making it a posttest study.
Experimental design is a research method that enables researchers to assess the effect of multiple factors on an outcome.. You can determine the relationship between each of the variables by: Manipulating one or more independent variables (i.e., stimuli or treatments). Applying the changes to one or more dependent variables (i.e., test groups or outcomes)
There are 3 types of experimental research designs. These are pre-experimental research design, true experimental research design, and quasi experimental research design. 1. The assignment of the control group in quasi experimental research is non-random, unlike true experimental design, which is randomly assigned. 2.
A research design is a strategy for answering your research question using empirical data. Creating a research design means making decisions about: Your overall research objectives and approach. Whether you'll rely on primary research or secondary research. Your sampling methods or criteria for selecting subjects. Your data collection methods.
Step 3: Assign subjects to groups. In this step, you randomly distribute subjects to the different experimental conditions. For example, for your stress in the workplace study you could create two experimental groups, where group one receives 10 emails per hour, and group two receives 100 emails per hour.
Three types of experimental designs are commonly used: 1. Independent Measures. Independent measures design, also known as between-groups, is an experimental design where different participants are used in each condition of the independent variable. This means that each condition of the experiment includes a different group of participants.
Create an exemplary data set for a completely randomized design with two treatments, four experimental units per treatment (replicates), and four observational units per experimental unit. Compute the noncentrality parameter of the F distribution with the appropriate degrees of freedom under the alternative hypothesis that the two treatments ...
Experimental research makes an ideal starting point. The data you collect is a foundation for building more ideas and conducting more action research. Whether you want to know how the public will react to a new product or if a certain food increases the chance of disease, experimental research is the best place to start. ...
Collect the data by using suitable data collection according to your experiment's requirement, such as observations, case studies , surveys , interviews, questionnaires, etc. Analyse the obtained information. Step 8. Present and Conclude the Findings of the Study. Write the report of your research.
Researchers within the field of applied linguistics have long used experiments to investigate cause-effect relationships regarding the use and learning of second languages (L2s).
Experimental Design. Experimental design is a process of planning and conducting scientific experiments to investigate a hypothesis or research question. It involves carefully designing an experiment that can test the hypothesis, and controlling for other variables that may influence the results. Experimental design typically includes ...
Experimental research is commonly used in sciences such as sociology and psychology, physics, chemistry, biology and medicine etc. It is a collection of research designs which use manipulation and controlled testing to understand causal processes. Generally, one or more variables are manipulated to determine their effect on a dependent variable.
Methods and procedures. Our study is a mixed factorial design, with one between-groups factor (i.e., frequency level of coffee drinking) and two within-subjects factor (i.e., coffee brands and ...
This article aims to present general guidelines to one of the many roles of a neurosurgeon: Writing an experimental research paper. Every research report must use the "IMRAD formula: introduction, methods, results and discussion". After the IMRAD is finished, abstract should be written and the title should be "created".
Here are some examples of experimental research: This research method can be used to evaluate employees' skills. Organizations ask candidates to take tests before filling a post. It is used to screen qualified candidates from a pool of applicants. This allows organizations to identify skills at the time of employment.
Step 2: Evaluate the Literature. A thorough examination of the relevant studies is essential to the research process. It enables the researcher to identify the precise aspects of the problem. Once ...
About Press Copyright Contact us Creators Advertise Developers Terms Privacy Policy & Safety How YouTube works Test new features NFL Sunday Ticket Press Copyright ...
Experimental research is a kind of study that rigidly follows a scientific research design. It involves testing or attempting to prove a hypothesis by way of experimentation. As such, it uses one or more independent variables, manipulating them and then using them on one or more dependent variables.
Chapter 10 Experimental Research. Experimental research, often considered to be the "gold standard" in research designs, is one of the most rigorous of all research designs. In this design, one or more independent variables are manipulated by the researcher (as treatments), subjects are randomly assigned to different treatment levels ...
In his book Experimental Research Designs in Social Work: Theory and Applications, Bruce A. Thyer presents a compelling argument for integrating experimental studies into social work practice and e...
Three-dimensional printing (3DP) technology has shown great potential in rock mechanics and mining engineering due to its ability to create complex and customized objects with high precision and accuracy. At present, an emerging research focus is improving the mechanical properties of 3D-printed samples, which originally has low strength and stiffness, to match those of natural rocks. The ...
The therapy remains experimental and the results need to be replicated in a larger group of people. Months following the treatment, Cook was sitting with friends on a balcony that had Christmas ...
The introduction of genome engineering technology has transformed biomedical research, making it possible to make precise changes to genetic information. However, creating an efficient gene-editing system requires a deep understanding of CRISPR technology, and the complex experimental systems under investigation. While Large Language Models (LLMs) have shown promise in various tasks, they ...