Progressive Modeling of Macroeconomic Time Series The LSE Methodology
Cite this chapter.
- Grayham E. Mizon
Part of the book series: Recent Economic Thought Series ((RETH,volume 46))
260 Accesses
54 Citations
Econometric models, large and small, have played an increasingly important role in macroeconomic forecasting and policy analysis. However, there is a wide range of model types used for this purpose, including simultaneous-equation models in either reduced or structural form, vector autoregressive models (VAR), autoregressive distributed-lag models, autoregressive integrated moving-average models, leading-indicator models, and error-correction models (ECM). Hendry, Pagan, and Sargan (1984) discuss a typology for dynamic single-equation models for time-series variables, and Hendry (1994) presents a typology for the various types of dynamic model used in the analysis of systems of equations. There is also a wide range of views about the appropriate way to develop and evaluate models. Sims (1980, 1992) advocates the use of VAR models, which can accurately represent the time-series properties of data, while eschewing the reliance on “incredible dentifying restrictions” that characterizes the use of simultaneous equation models of the structural or Cowles Commission type. The potential value of structure (loosely defined) within the context of VAR models has led to the development of structural VAR models, and Canova (1995) provides a recent review of this literature. Leamer (1978, 1983), on the other hand, has been critical of the use of non-Bayesian models that do not analyze formally the role and value of a priori information, especially when there is no checking of model sensitivity. Summers (1991), though aware of the important developments made in theoretical statistics and econometrics in this century, argues that too much emphasis is placed on the technical aspects of modeling and not enough on the real issues that are concerned with the analysis of well-established and fundamental relationships between economic variables. One approach to modeling that does not overemphasize the role of model evaluation and statistical technique is that associated with real business cycle analysis and the calibration of economic theory, rather than its evaluation. Kydland and Prescott (1982, 1995) have been pioneers in this field, and Canova, Finn, and Pagan (1994) provide a critique.
This is a preview of subscription content, log in via an institution to check access.

Access this chapter
Subscribe and save.
- Get 10 units per month
- Download Article/Chapter or eBook
- 1 Unit = 1 Article or 1 Chapter
- Cancel anytime
- Available as PDF
- Read on any device
- Instant download
- Own it forever
- Compact, lightweight edition
- Dispatched in 3 to 5 business days
- Free shipping worldwide - see info
- Durable hardcover edition
Tax calculation will be finalised at checkout
Purchases are for personal use only
Institutional subscriptions
Unable to display preview. Download preview PDF.
Similar content being viewed by others
An Introduction to Time Series Modeling and Forecasting
Nonlinear Time Series Models and Model Selection
Multivariate Time Series Models
Akaike, H. (1974). “A New Look at the Statistics Model Identification.” IEEE Transactions on Automatic Control AC-19, 716–723.
Article Google Scholar
Baillie, R.T. (1987). “Inference in Dynamic Models Containing Surprise Variables.” Journal of Econometrics 35, 101–117.
Bernanke, B. (1986). “Alternative Explorations of the Money Income Correlation.” Carnegie Rochester Conference in Public Policy 25, 49–100.
Beveridge, S., and C. Nelson. (1981). “A New Approach to Decomposition of Economic Time Series into Permanent and Transitory Components with Particular Attention to Measurement of Business Cycle.” Journal of Monetary Economics 7 ,151–174.
Blanchard, O. (1987). “Comment to Runkle’s Vector Autoregression and Reality. ”Journal of Bussiness and Economic Statistics 5, 449–451.
Google Scholar
Blanchard, O. (1989). “A Traditional Interpretation of Macroeconomic Fluctuations.” American Economic Review 79, 1146–1164.
Blanchard, O., and D. Quah. (1989). “The Dynamic Effects of Aggregate Demand and Supply Disturbances.” American Economic Review 79, 655–673.
Blanchard, O., and D. Quah. (1993). “Fundamentalness and the Interpretation of Time Series Evidence: A replay to Lippi and Reichlin.” American Economic Review 83(3), 653–658.
Blanchard, O., and M. Watson. (1986). “Are Business Cycles all Alike?” In Gordon, R. (ed.), The American Business Cycle. Chicago: University of Chicago Press.
Brock, W., and C. Sayers. (1988). “Is the Business Cycle Characterized by Deterministic Chaos?” Journal of Monetary Economics 22, 71–90.
Campbell, J., and A. Deaton. (1989). “Why Is Consumption so Smooth?” Review of Economic Studies 56, 357–373.
Canova, F. (1991). “The Sources of Financial Crises: Pre and Post Fed Evidence.” International Economic Review 32, 689–713.
Canova, F. (1993). “Modelling and Forecasting Exchange Rates with Bayesian Time Vary-ing Coefficient Model.” Journal of Economics Dynamic and Control 17, 233–261.
Canova, F. (1994). “VAR Models: Specification, Estimation, Inference and Forecasting.” In H. Pesaran and M. Wickens (eds.), Handbook of Applied Econometrics. Oxford: Basil Blackwell.
Canova, F., M. Finn, and A. Pagan. (1993). “Evaluating a Real Business Cycle Model.” In C. Hargreaves (ed.), Nonstationary Time Series Analysis and Cointegration. Oxford: Oxford University Press.
Clements, M.P., and G.E. Mizon. (1991). “Empirical Analysis of Macroeconomic Time Series: VAR and Structural Models.” European Economic Review 35, 887–917.
Cooley, T., and S. Leroy. (1985). “Atheoretical Macroeconometrics: A Critique.” Journal of Monetary Economics 16, 283–308.
Dickey, D., and W. Fuller. (1979). “Distribution of the Estimators for a Autoregressive Time Series with a Unit Root.” Journal of the American Statistical Association 74, 427–431.
Danthine, J.P. (1992). “Calibrated Macro Models: What and What For?” Manuscript, University of Lousanne.
DeJong, D., and Whiteman, C. (1991). “Reconsidering Trends and Random Walks in Macroeconomic Time Series.” Journal of Monetary Economics 28(2), 221–254.
Doan, T. (1994). RATS Manual , Version 4.10. Evanston, II .
Doan, T., R. Litterman, and C. Sims. (1984). “Forecasting and Conditional Projections Using Realist Prior Distributions.” Econometric Reviews 3, 1–100.
Engle, R. (1982). “Autoregressive Conditional Heteroskedasticity with Estimates of the Variance of the UK Inflation.” Econometrica 50, 987–1006.
Engle, R., and C. Granger. (1987). “Co-Integration and Error Correction: Representation, Estimation and Testing.” Econometrica 55, 251–276.
Engle, R., D. Lilien, and R. Robbins. (1987). “Estimating Time Varying Risk Premia in the Term Structure: The ARCH-M Model.” Econometrica 55 ,391–408.
Evans, G. (1989). “Output and Unemployment Dynamics in the United States: 19501985.” Journal of Applied Econometrics 4, 213–237.
Fair, R. (1988). “VAR Models as Structural Approximations.” Discussion Paper 856, Cowles Foundation.
Friedman, M., and A. Schwartz. (1963). A Monetary History of the United States, 1867–1960 . Princeton, NJ: Princeton University Press.
Gali, J. (1992). “How Well Does the ISLM Model Fit Postwar U.S. Data?” Quarterly Journal of Economics 107, 709–735.
Gali, J., and M. Hammour. (1991). “Long Run Effects of Business Cycles.” Manuscript, Columbia University.
Gallant, R., D. Hsieh, and G. Tauchen. (1991). “On Fitting Recalcitrant Series: The Pound/ Dollar Exchange Rate, 1974–1983.” In W. Barnett, J. Powell and G. Tauchen, (eds.), Nonparametric and Semiparametric Methods in Econometrics and Statistics . Proceedings of the fifth International Symposium in Economic Theory and Econometrics. Cambridge: Cambridge University Press.
Geweke, J. (1982). “The Measurement of Linear Dependence and Feedback Between Multiple Time Series.” Journal of the American Statistical Association 77, 304–313.
Giannini, C. (1992). Topics in Structural VARs. Berlin: Springer and Verlag.
Granger, C., and Anderson. (1978). Introduction to Bilinear Time Series. Gottingen, Germany: Vandenhoeck and Ruprecht.
Griffiths, W., and H. Lutkepohl. (1990). “Confidence Interval for Impulse Responses from VAR Models: A Comparison of Asymptotic Theory and Simulation Approaches.” Manuscript, University of New England.
Hamilton, J. (1989). “A New Approach to Economic Analysis of Nonstationary Time Series and the Business Cycles.” Econometrica 57, 357–384.
Hamilton, J. (1994). Applied Time Series Analysis . Princeton, NJ: Princeton University Press.
Hansen. L. (1982). “Large Sample Properties of Generalized Methods of Moment Estimators.” Econometrica 50, 1029–1054.
Hansen, L., W. Roberds, and T. Sargent. (1991). “Time Series Implications of Present Value Budget Balance and of Martingale Models of Consumption and Taxes.” In L. Hansen and T. Sargent (eds.), Rational Expectations Econometrics. Boulder, Co: Westview Press.
Hansen, L., and T. Sargent. (1980). “Formulating and Estimating Linear Rational Expectations Models.” Journal of Economic Dynamics and Control 2, 1–46.
Hansen, L., and T. Sargent. (1991). “Two Difficulties in Interpreting Vector Autoregressions.” In L. Hansen and T. Sargent (eds.), Rational Expectations Econometrics. Boulder, Co: Westview Press.
Hansen, L., and K. Singleton. (1983). “Stochastic Consumption, Risk Aversion and the Temporal Behavior of Asset Returns.” Journal of Political Economy 91, 249–265.
Harvey, A.C. (1985). “Trends and Cycles in Macroeconomics.” Journal of Business and Economic Statistics 3, 216–227.
Hendry, D., and G. Mizon. (1990). “Evaluating Dynamic Econometric Models by Encompassing the VAR.” In P.C.B. Phillips and V.B. Hall (eds.), Models , Methods and Applications of Econometrics: Essays in Honor of Rex Bergstrom. Oxford: Oxford University Press.
Hsiao, C. (1981). “Autoregressive Modelling and Money-Income Causality Detection.” Journal of Monetary Economics 7, 85–106.
Johansen, S. (1988). “Statistical Analysis of Cointegrating Vectors.” Journal of Economic Dynamics and Control 12, 231–254.
Johansen, S., and K. Juselius. (1990). “Maximum Likelihood Estimation and Inference on Cointegration-with Application to the Demand for Money.” Oxford Bulletin of Economics and Statistics 52, 169–210.
Judge, G., W. Griffith, R. Hill, H. Lutkepohl, and T. Lee. (1980). The Theory and Practice of Econometrics (2nd ed.). New York: Wiley.
Keating, J. (1990). “Identifying VAR Models under Rational Expectations.” Journal of Monetary Economics 25, 453–476.
Kirchgassner, G. (1991). “Comments on M.P. Clements and G.E. Mizon: Empirical Analysis of Macroeconomic Time Series: VAR and Structural Models.” European Economic Review 35, 918–922.
King, R., C. Plosser, and S. Rebelo. (1988). “Production, Growth and Business Cycles, 1.” Journal of Monetary Economics 21, 309–342.
King, R., C. Plosser, J. Stock, and M. Watson. (1991). “Stochastic Trends and Economic Fluctuations.” American Economic Review 81, 819–840.
Kocherlakota, N. (1990). “On the Discount Factor in Growing Economies.” Journal of Monetary Economics 25, 43–48.
Koopmans, T. (1963). “Identification Problems in Economic Model Construction.” In W. Hood and T.J. Koopmans (eds.), Studies in Econometric Methods. New Haven, CT: Yale University Press.
Kydland, F., and E. Prescott. (1982). “Time to Build and Aggregate Fluctuations.” Econometrica 50, 1345–1370.
Kydland, F., and E. Prescott. (1991). “The Econometrics of the General Equilibrium Approach to Business Cycles.” Scandinavian Journal of Economics 93, 161–178.
Leamer, E. (1978). Specification Searches and ah-Hoc Inference with Nonexperimental Data. New York: Wiley.
Leamer, E. (1985). “Vector Autoregression for Causal Inference?” Carnegie Rochester Conference Series on Public Policy 22, 255–304.
Leeper, E. (1989). “Policy Rules, Information and Fiscal Effects in a ‘Ricardian’ Model.” International Finance Discussion Paper 360, Board of Governors of the Federal Reserve System.
Leeper E., and R. Gordon. (1992). “In Search for the Liquidity Effects.” Journal of Monetary Economics 29, 341–371.
Lippi, M., and L. Reichlin. (1992). “VAR Analysis, Non-Fundamental Representation, Blaschke Matrices.” Manuscript, OCFE.
Lippi, M., and L. Reichlin. (1993). “A Note on Measuring the Dynamic Effects of Aggregate Demand and Supply Disturbances.” American Economic Review 83(3), 644–652.
Litterman, R. (1980). “Techniques for Forecasting Vector Autoregressions.” Ph.D. thesis, University of Minnesota.
Litterman, R. (1984). “Forecasting and Policy Analysis with Bayesian Vector Autoregression Models.” Quarterly Review (Federal Reserve Bank of Minneapolis) 8 (Fall), 30–41.
Litterman, R. (1986). “Specifying Vector Autoregressions for Macroeconomic Forecasting.” In P. Goel and A. Zellner (eds.), Bayesian Inference and Decision Techniques. Amsterdam: North Holland.
Litterman, R., and L. Weiss. (1985). “Money, Real Interest Rates and Output: A Reinterpretation of Postwar U.S. Data.” Econometrica 52, 129–156.
Liu, T. (1960). “Underidentification, Structural Estimation and Forecasting.” Econometrica 28, 855–865.
Long, J., and C. Plosser. (1983). “Real Business Cycles.” Journal of Political Economy 91, 1345–1370.
Luppoletti, W., and R. Webb. (1986). “Defining and Improving the Accuracy of Macroeconomic Forecasts: Contributions from a VAR Model.” Journal of Business 59, 263–285.
Lutkepohl, H. (1986). “Comparison of Criteria for Estimating the Order of a Vector Auto-regressive Process.” Journal of Time Series Analysis 6, 35–52; and “Correction,” 8, 373.
Lutkepohl, H. (1991). Introduction to Multiple Time Series Analysis. New York: Springer and Verlag.
Maravall, A. (1993). “Stochastic Linear Trends: Models and Estimators.” Journal of Econometrics 56, 5–37.
McNees, S. (1986). “Forecasting Accuracy of Alternative Techniques: A Comparison of U.S. Macroeconomic Forecasts” (with comments). Journal of Business and Economic Statistics 4, 23–28.
McNees, S. (1990). “The Role of Judgement in Macroeconomic Forecasting Accuracy.” International Journal of Forecasting 6, 287–299.
Nelson, and C. Plosser. (1982). “Trends and Random Walks in Macroeconomic Time Series.” Journal of Monetary Economics 10, 139–162.
Nickelsburg, G. (1985). “Small Sample Properties of Dimensionality Statistics for Fitting VAR Models to Aggregate Economic Data.” Journal of Econometrics 28, 183–192.
Nicholls, D.F., and B.G. Quinn. (1982). Random Coefficient Autoregressive Models: An Introduction. New York: Springer and Verlag.
Book Google Scholar
Ohanian, L. (1988). “The Spurious Effect of Unit Roots on Vector Autoregressions: A Monte Carlo Study.” Journal of Econometrics 39, 251–266.
Perron, P. (1989). “The Great Crash, the Oil Price Shock and the Unit Root Hypothesis.” Econometrica 57, 1361–1401.
Phillips, P.C. (1988). “Optimal Inference in Cointegrated Systems.” Econometrica 55, 277–301.
Phillips, P.C., and P. Perron. (1986). “Testing for Unit Roots in Time Series Regressions.” Biometrika 70, 335–346.
Quah, D. (1990). “Permanent and Transitory Movements in Labor Income: An Explanation Based on Excess Smoothness in Consumption.” Journal of Political Economy 98, 449–475.
Quah, D. (1991). “Identifying Vector Autoregressions: A Discussion of P. Englund, A. Vredin and A. Warne: Macroeconomic Shocks in Sweden 1925–1986.” Manuscript, London School of Economics.
Quah, D. (1992). “Lecture Notes in Macroeconometrics.” Manuscript, London School of Economics.
Quah, D., and T. Sargent. (1993). “A Dynamic Index Model for a Large Cross Section.” In J. Stock and M. Watson (eds.), New Research on Business Cycles , Indicators and Forecasting. Chicago: University of Chicago Press.
Reinsel, G. (1983). “Some Results on Multivariate Autoregressive Index Models.” Biometrika 70, 145–156.
Robertson, D., and M. Wickens. (1992). “Measuring Real and Nominal Macroeconomic Shocks and Their International Transmission Under Different Monetary Systems.” Working paper, London Business School.
Rozanov, Y. (1967). Stationary Random Processes. San Francisco: Holden Day.
Runkle, D. (1987). “Vector Autoregression and Reality.” Journal of Business and Economic Statistics 5, 437–454.
Sargent, T. (1979). “Estimating Vector Autoregressions Using Methods Not Based on Explicit Economic Theory.” Quarterly Review (Federal Reserve Bank of Minneapolis), 3 (Summer), 8–15.
Sargent, T. (1984). “Autoregression, Expectations and Advice.” American Economic Review , Papers and Proceedings 74, 408–415.
Sargent, T. (1986). Macroeconomic Theory (2nd ed.). New York: Academic Press.
Sargent, T., and R. Lucas. (1980). Rational Expectations and Econometric Practice. Minneapolis, MN: University of Minnesota Press.
Sargent, T., and C. Sims. (1977). “Business Cycle Modelling Without Pretending to Have Too Much A Priori Economic Theory.” In C. Sims (ed.), New Methods in Business Cycle Research. Minneapolis, MN: Federal Reserve Bank of Minneapolis.
Schwarz, G. (1978). “Estimating the Dimension of a Model.” Annals of Statistics 6, 461–464.
Shapiro, M., and M. Watson. (1989). “Sources of Business Cycle Fluctuations” NBER Macroeconomic Annual (pp. 111–148). Cambridge, MA: MIT Press.
Sims, C. (1972). “Money, Income and Causality.” American Economic Review 62, 540–553.
Sims, C. (1980a). “Macroeconomics and Reality.” Econometrica 48, 1–48.
Sims, C. (1980b). “A Comparison of Interwar and Postwar Business Cycles: Monetarism Reconsidered.” American Economic Review Papers and Proceedings 70, 250–257.
Sims, C. (1982). “Policy Analysis with Econometric Models.” Brookings Papers of Economic Activity 2, 107–152.
Sims, C. (1986). “Are Forecasting Models Usable for Policy Analysis.” Quarterly Review (Federal Reserve Bank of Minneapolis), 10 (Winter), 2–16.
Sims, C. (1987). “Comment to Runkle’s Vector Autoregression and Reality.” Journal of Business and Economic Statistics 5, 443–449.
Sims, C. (1988a). “Identifying Policy Effects.” In R. Bryant, D. Henderson, G. Holtman, P. Hooper, and A. Symansky (eds.), Empirical Macroeconomics for Interdependent Economies (pp. 305–321). Washington DC: Brookings Institution.
Sims, C. (1988b). “Bayesian Prospective on Unit Roots Econometrics.” Journal of Economic Dynamic and Control 12, 436–474.
Sims, C. (1989b). “A Nine Variable Probabilistic Model of the U.S. Economy.” Discussion Paper 14, Institute for Empirical Macroeconomics, Federal Reserve of Minneapolis.
Sims, C., J. Stock, and M. Watson. (1990). “Inference in Linear Time Series Models with Some Unit Roots.” Econometrica 58, 113–144.
Sims, C., and H. Ulhig. (1991). “Unit Rooters: An Elicopter Tour.” Econometrica 59(6), 1591–1600.
Spencer, D. (1989). “Does Money Matter? The Robustness of the Evidence from Vector Autoregressions.” Journal of Money, Banking and Credit 21, 442–454.
Stock, J., and M. Watson. (1988). “Testing for Common Trends.” Journal of the American Statistical Association 83, 1097–1107.
Stock, J., and M. Watson. (1989). “Interpreting the Evidence on Money-Income Causality.” Journal of Econometrics 40, 161–181.
Taylor, J. (1980). “Aggregate Dynamics and Staggered Contracts.” Journal of Political Economy 88, 1–24.
Theil, H. (1971). Principles of Econometrics. New York: Wiley.
Todd, R. (1984). “Improving Economic Forecasting with Bayesian Vector Autoregression.” Quarterly Review (Federal Reserve Bank of Minneapolis), 8 (Fall), 18–29.
Todd, R. (1990). “Vector Autoregression Evidence on Monetarism: Another Look at the Robustness Debate.” Quarterly Review (Federal Reserve Bank of Minneapolis), 14 (Spring), 17–37.
Watson, M. (1986). “Univariate Detrending Methods with Stochastic Trends.” Journal of Monetary Economics 18, 49–75.
Watson, M. (1987). “Comment to Runkle’s Vector Autoregression and Reality.” Journal of Business and Economic Statistics 5, 451–453.
Watson, M. (1994). “VAR and Cointegration.” In R. Engle and D. McFadden (eds.), Handbook of Econometrics (Vol. 4).
Wickens, M. (1992). “VAR Analysis in the Presence of Cointegration.” Working paper, London Business School.
Zellner, A. (1962). “An Efficient Methods for Estimating Seemingly Unrelated Regressions and Tests for Aggregation Bias.” Journal of the American. Statistical Association 57, 348–368.
Download references
You can also search for this author in PubMed Google Scholar
Editor information
Editors and affiliations.
University of California, Davis, California, USA
Kevin D. Hoover
Rights and permissions
Reprints and permissions
Copyright information
© 1995 Springer Science+Business Media New York
About this chapter
Mizon, G.E. (1995). Progressive Modeling of Macroeconomic Time Series The LSE Methodology . In: Hoover, K.D. (eds) Macroeconometrics. Recent Economic Thought Series, vol 46. Springer, Dordrecht. https://doi.org/10.1007/978-94-011-0669-6_4
Download citation
DOI : https://doi.org/10.1007/978-94-011-0669-6_4
Publisher Name : Springer, Dordrecht
Print ISBN : 978-94-010-4293-2
Online ISBN : 978-94-011-0669-6
eBook Packages : Springer Book Archive
Share this chapter
Anyone you share the following link with will be able to read this content:
Sorry, a shareable link is not currently available for this article.
Provided by the Springer Nature SharedIt content-sharing initiative
- Publish with us
Policies and ethics
- Find a journal
- Track your research
Navigation Menu
Search code, repositories, users, issues, pull requests..., provide feedback.
We read every piece of feedback, and take your input very seriously.
Saved searches
Use saved searches to filter your results more quickly.
To see all available qualifiers, see our documentation .
LSE Department of Methodology
- 5 followers
- Columbia House, Houghton Street, London WC2A 2AE
- http://www.lse.ac.uk/methodology
- Methodology.Admin@lse.ac.uk
Popular repositories Loading
Coursepack for MY464
Coursepack for MY451
Repositories
Top languages
Most used topics.
Browser does not support script.
- 30 Year Anniversary
- Methods surgery
- Methods Short Courses
- Seminar series
- Software tutorials
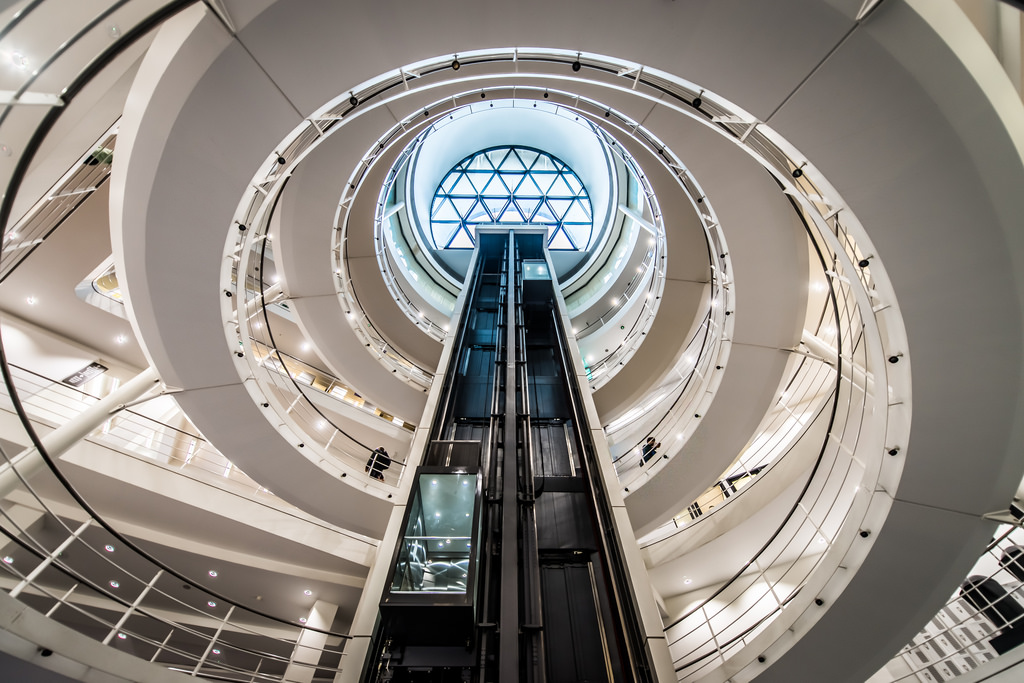
Auditing in the Department of Methodology
Application for auditing 2024/25 courses will open in september 2024. .
Auditing in the Department of Methodology allows someone to attend lectures and seminars of certain courses if space allows but without submitting assessed coursework or sitting a final exam. The course is not taken for credit, and details of audited courses do not appear on transcripts.
To attend a seminar group as an auditor, you must apply for a place. Auditing places will only be allocated if space permits. We expect auditors to attend all seminars where possible to avoid disruption to teaching.
This page only applies to the auditing of Methodology courses.
How can I apply to audit a course?
Registered LSE MSc students and staff should apply by filling out an online form. After submitting the form, please save a copy of your response.
What is the application timeline?
There are two application periods across the year.
Autumn Term
You can apply from Monday 23 September 2024 until Friday 4 October 2024 . You can apply to audit any Autumn Term and/or Winter Term courses.
Winter Term
You can apply from Monday 13 January 2025 until Friday 24 January 2025 . You can apply to audit any Winter Term courses.
The results of the Autumn Term courses will be released via email during the week of 14 October 2024, and those of the Winter Term courses during the week of 3 February 2025.

I have submitted the application form.
We allocate auditing places after the course selection period and appreciate your patience.
In the meantime, feel free to attend the lecture and seminar, but bear in mind that you will need to give up the seminar seat to any students taking the course for credit or if the group is full.
How will the auditing places be allocated?
Our priority will always be students taking a course for credit. If we have spaces available in the seminars after the course selection period, we will offer places to auditors.
Auditing places are allocated on a first-come, first-served basis, and we cannot guarantee auditing places.
Frequently Asked Questions
I only intend to attend the lecture, but not the seminar.
You do not need to seek permission to audit lectures only for Methodology courses.
Students are welcome to attend lectures in person or access the passive parts of our courses, i.e., recorded lectures on Moodle and any self-directed exercises. All Methodology Moodle pages are open-access, and lecture recordings will be posted there.
I am an LSE MRes/Research student; how should I apply to audit?
Registered LSE MRes/Research students can apply to audit Methodology courses via LSE For You by marking them as 'audit' in the final step when composing your 'Student Statement'. There is no need to fill out the online form.
I am not an LSE student; can I still apply to audit?
Non-LSE students are permitted to audit courses only when they register as an Intercollegiate Student , if eligible.
The course(s) that I am interested in auditing are not included in the application form.
Unfortunately, some course(s) do not allow auditing due to course design. You are welcome to attend the lecture or visit the Moodle page.
When and where are the seminars?
Please refer to the timetable information page for the lecture and seminar details. If you are an auditor, the lecture and seminar will not appear on your personal timetable.
Can I indicate my preference for the seminar group?
As we cannot guarantee places in any seminar group, there is no need to indicate a preference. Please also bear in mind that there may be limited flexibility in changing the seminar groups after allocation.
I have changed my mind and want to stop auditing.
Please email [email protected] , as we usually have a waiting list. If you have already attended some seminars, please also inform the seminar teacher.
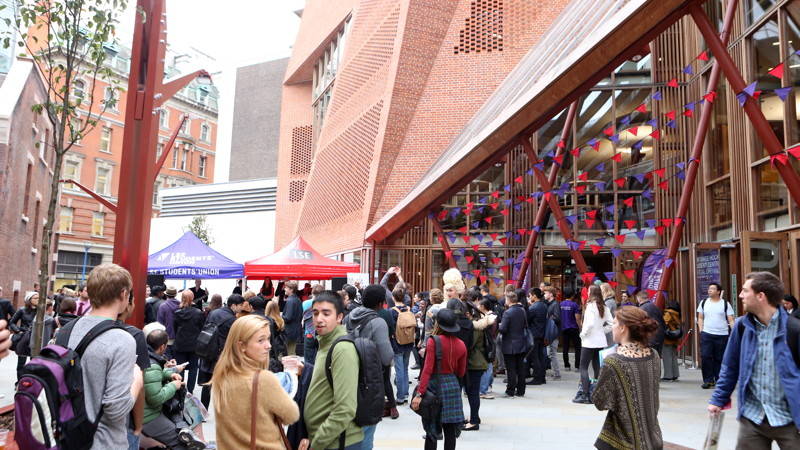
Timetable viewed by course code Lectures and seminars
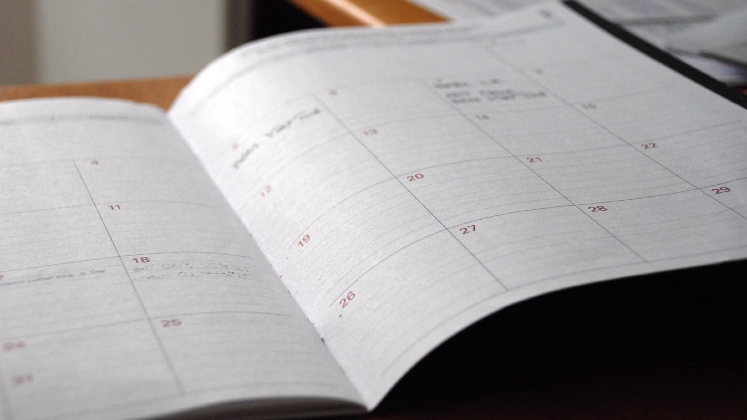
Moodle Learn more about our courses
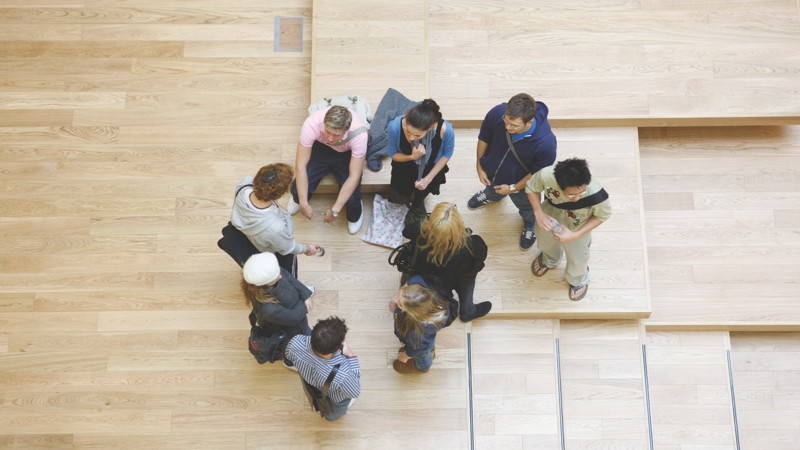
LSE Calendar Information on Methodology courses and MSc programmes
- Skip to Content
- Skip to Search
August 27 2024
For media and investors only
Issued: 27 August 2024, London UK
Statement: Zantac (ranitidine) litigation – Delaware Supreme Court to review Superior Court’s Daubert decision
GSK plc (LSE/NYSE: GSK) welcomes today’s decision by the Delaware Supreme Court that it will review the Delaware Superior Court’s decision allowing the introduction of plaintiffs’ expert evidence at trial. Interlocutory reviews are granted in exceptional circumstances, and GSK is pleased that the Supreme Court is of the view that such circumstances are present here.
The scientific consensus remains that there is no consistent or reliable evidence that ranitidine increases the risk of any cancer. Since 2019, there are 16 epidemiological studies looking at human data regarding the use of ranitidine, including outcomes for more than 1 million patients using ranitidine, supporting this consensus.
GSK is committed to vigorously defending itself and managing this litigation in the best interests of the Company and its shareholders. The Delaware litigation will progress in parallel with the Delaware Supreme Court review. Alongside review by the Delaware Supreme Court, the Company will press additional defenses in the litigation, including failure to provide proof of use and proof of diagnosis requirements recently ordered by the Court.
Notes to Editors
* The Daubert standard, established in the U.S. Supreme Court case Daubert v. Merrell Dow Pharmaceuticals, Inc. 509 US 579 (1993) provides criteria for evaluating whether expert testimony is admissible under Federal Rule of Evidence 702. Under Rule 702 and Daubert, an expert may offer testimony if he or she is qualified by knowledge, education, training or experience in a given area and the testimony offered is reliable, relevant and helpful to the jury. In applying the Daubert standard, the Court acts as a gatekeeper, ensuring that expert opinions meet certain standards for reliability and that speculative or unreliable opinions are not presented to the jury. In Daubert, the Supreme Court identified four factors to guide assessment of an expert’s methodology: (1) whether the expert’s methodology has been tested or is capable of being tested; (2) whether the theory or technique used by the expert has been subjected to peer review and publication; (3) whether there is a known or potential error rate of the methodology; and (4) whether the technique has been generally accepted in the relevant scientific community. The Daubert standard is applicable to expert testimony in all federal cases. Many states also have adopted standards identical to the federal Daubert standard.
The term “Daubert Standard” comes from the United States Supreme Court case: Daubert v Merrell Dow Pharmaceuticals Inc 509 US 579 (1993).
GSK is a global biopharma company with a purpose to unite science, technology, and talent to get ahead of disease together. Find out more at gsk.com.
Cautionary statement regarding forward-looking statements
GSK cautions investors that any forward-looking statements or projections made by GSK, including those made in this announcement, are subject to risks and uncertainties that may cause actual results to differ materially from those projected. Such factors include, but are not limited to, those described under Item 3.D “Risk factors” in GSK’s Annual Report on Form 20-F for 2023, and GSK’s Q2 Results for 2024.
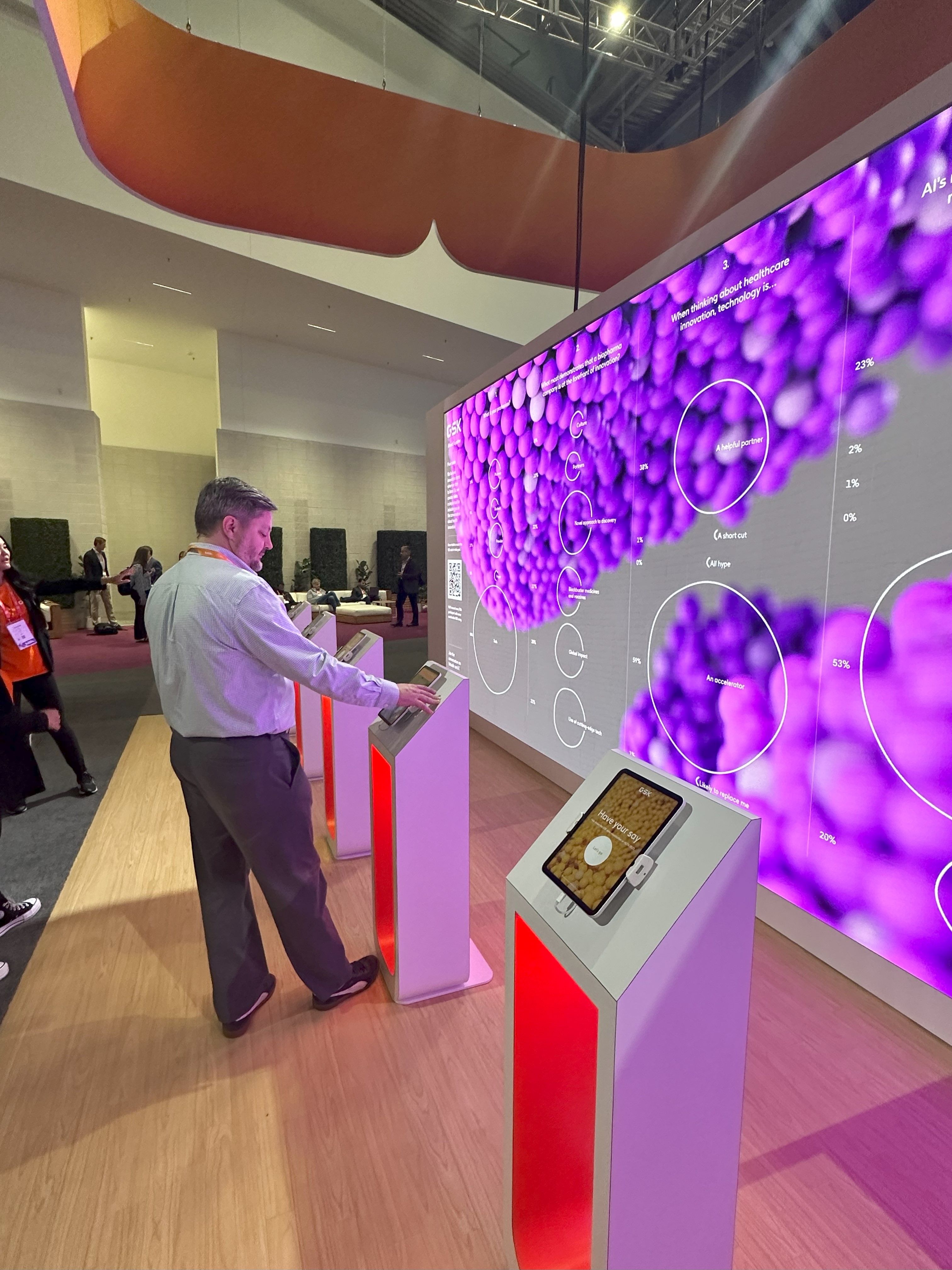
Media contacts
Contact our GSK US Media Team. We are committed to responding to the information needs of the media as quickly as possible
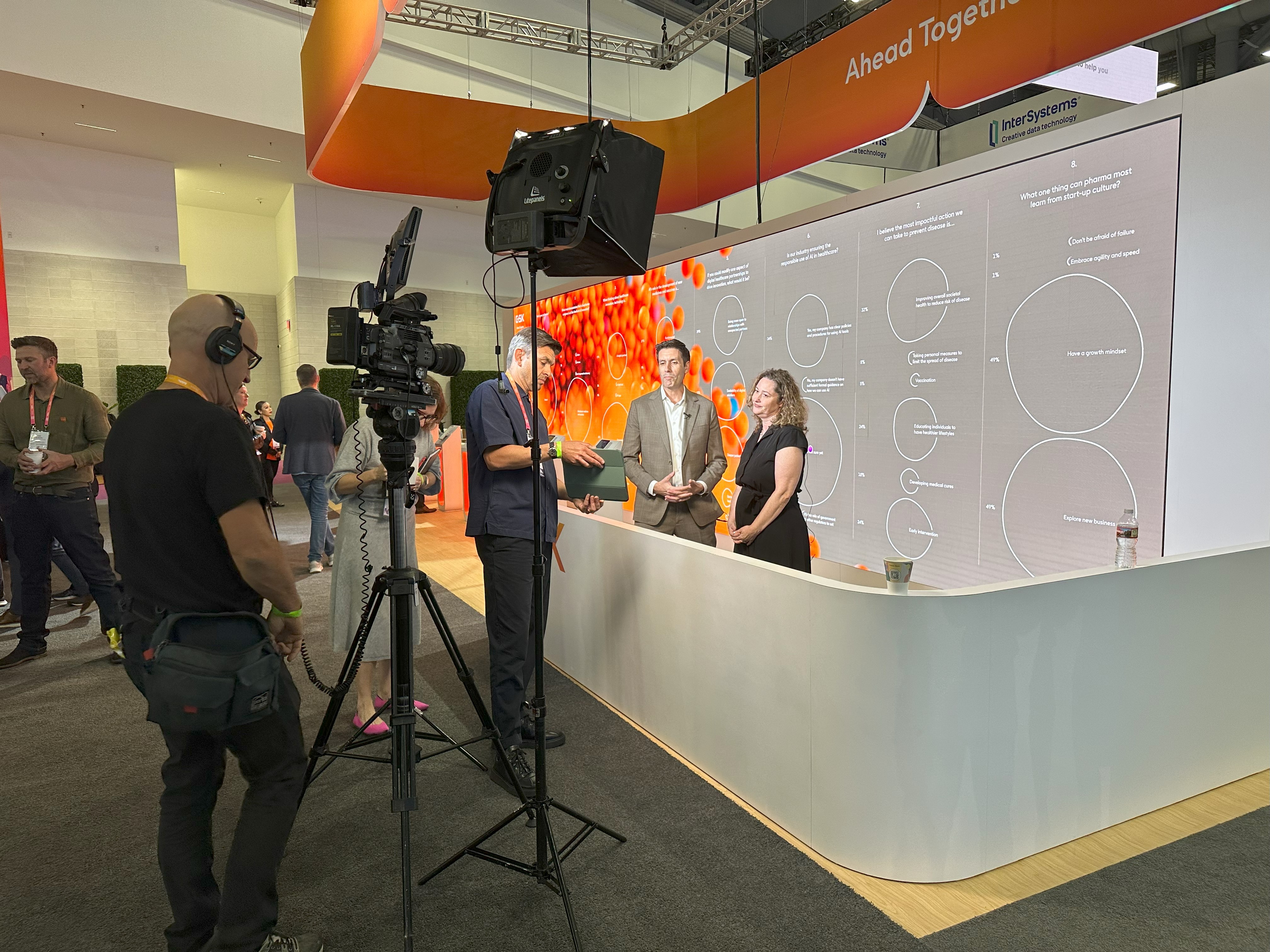
US resources, press releases, information on our social media channels, and our photo and video library
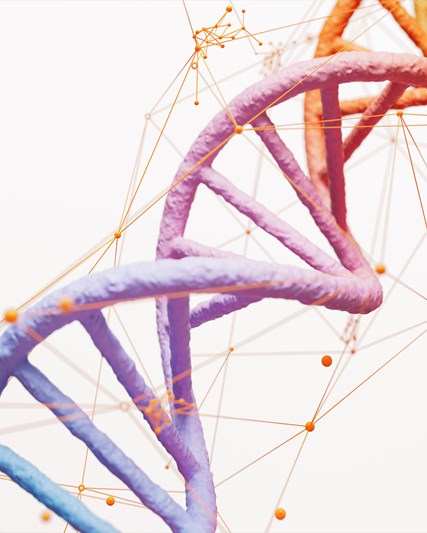
We unite science, technology and talent to get ahead of disease together
You are leaving our website
The information you are about to be referred to may not comply with the local regulatory requirements. Further information relevant to the local environment is available from the company or via the Product Information.
Browser does not support script.
- Autumn Term events schedule
- Student Voice
- You've got this
- LSE Volunteer Centre
- Key information
- My Skills and Opportunities
- Digital Skills Lab
- Student Wellbeing Service
- PhD Academy
- LSE Careers
- Student Services Centre
- Timetable publication information
- Students living in halls
- Faith Centre
SPSS is an easy-to-use and powerful data management and analysis software package that performs a wide variety of statistical procedures. The original acronym stands for ‘Statistical Package for the Social Sciences’. SPSS runs on Windows, Macintosh and UNIX platforms.
SPSS provides a comprehensive set of flexible tools that can be used to accomplish various data analysis tasks and has the option of using drop-down menus or scripting. It is well-suited to accommodate different exploration strategies such as surveys and experiments in diverse fields of enquiry. SPSS is among the used for statistical analysis in business, government, health, education, research and academic organisations.
SPSS vs Stata: Which is right for you?
SPSS is similar to Stata in that you can either use drop-down menus or use coding to do analysis. SPSS deals better with very large datasets but is more expensive than Stata. Stata generally has better support available from various sources such as the statalist forum, documentation online and built-in help. You can request a license and instructions on how to download and install SPSS by emailing [email protected] .
SPSS vs R or Python
SPSS is easier to learn than R and Python, providing easier access to running some types of analysis. It is still a popular choice in the social sciences, but R and Python are catching up. R and Python are open-source and freely available, whereas SPSS needs a licence, which can be expensive. R and Python are better options for data visualisation and machine learning but you will have to learn to code in order to fully utilise them.
We also have workshops and self-study courses in Stata , Python and R . See below if you're not sure which is right for you.
SPSS fundamentals workshop series
The Digital Skills Lab is currently running a 4-part SPSS fundamentals workshop series. Workshops will run throughout the year. Click on the link below to book your place or express an interest so that you are notified as soon as workshops are available to book.
Workshops will take place on campus in LRB.R.08 on the lower ground floor of the Library.
The SPSS fundamentals workshop series teaches you the basics of using SPSS for statistical analysis. At the end of this SPSS series, you will be able to import, manage, and explore data in SPSS, as well as perform various statistical tests. Our SPSS Fundamentals workshop series is ideal for those with no or very little prior experience of using SPSS, or those looking for a refresher.
Click on the link below to check availability and book your place:
SPSS Fundamentals Workshop Series
SPSS 1: Foundations of Data Management
Get started with the SPSS interface and how to use it as a data management tool for your analysis. We will be loading in datasets, making and recoding variables and other pre-analysis steps.
You will learn how to:
- Work with the SPSS interface
- Import data from csv and Excel files
- Process imported datasets
- Recode variables
- Compute new variables
SPSS 2: Data Exploration
Learn how to explore your data pre-analysis in SPSS. We will be running descriptive statistics and making data visualisations to review and explore our data.
- Explore categorical variables with frequencies and bar charts
- Explore continuous variables with descriptive statistics and box plots
- Explore two continuous variables with correlations and scatterplots
SPSS 3: Introduction to inferential statistics
Learn how to get started with inferential statistics in SPSS. We will be performing some fundamental statistical tests including chi-square, t-tests, and ANOVAs. You will also try and form some of your own ideas and hypothesis from data, and use that to understand the statistical outputs.
- How to perform cross-tabulation and chi-squared tests
- How to perform different types of t-tests
- How to perform different types of ANOVAs
SPSS 4: Regressions in SPSS
Learn how to run linear regressions in SPSS and how to interpret the results. You will also try and form some of your own ideas and hypothesis from data, and use that to understand the statistical outputs.
- How to perform and interpret simple linear regression
- How to perform and interpret multiple linear regression
Other support
The Department of Methodology also has some online tutorials in SPSS that were produced in 2011 that students have found useful along with a YouTube training channel . The Department of Methodology also provides training for PhD and MSc students as well as staff in the design of social research and in qualitative and quantitative analysis. Information on this can be found on their Methods training page .
- Skip to Content
- Skip to Search
27 August 2024
For media and investors only
Issued: 27 August 2024, London UK
Statement: Zantac (ranitidine) litigation – Delaware Supreme Court to review Superior Court’s Daubert decision
GSK plc (LSE/NYSE: GSK) welcomes today’s decision by the Delaware Supreme Court that it will review the Delaware Superior Court’s decision allowing the introduction of plaintiffs’ expert evidence at trial. Interlocutory reviews are granted in exceptional circumstances, and GSK is pleased that the Supreme Court is of the view that such circumstances are present here.
The scientific consensus remains that there is no consistent or reliable evidence that ranitidine increases the risk of any cancer. Since 2019, there are 16 epidemiological studies looking at human data regarding the use of ranitidine, including outcomes for more than 1 million patients using ranitidine, supporting this consensus.
GSK is committed to vigorously defending itself and managing this litigation in the best interests of the Company and its shareholders. The Delaware litigation will progress in parallel with the Delaware Supreme Court review. Alongside review by the Delaware Supreme Court, the Company will press additional defenses in the litigation, including failure to provide proof of use and proof of diagnosis requirements recently ordered by the Court.
Notes to Editors
* The Daubert standard, established in the U.S. Supreme Court case Daubert v. Merrell Dow Pharmaceuticals, Inc. 509 US 579 (1993) provides criteria for evaluating whether expert testimony is admissible under Federal Rule of Evidence 702. Under Rule 702 and Daubert, an expert may offer testimony if he or she is qualified by knowledge, education, training or experience in a given area and the testimony offered is reliable, relevant and helpful to the jury. In applying the Daubert standard, the Court acts as a gatekeeper, ensuring that expert opinions meet certain standards for reliability and that speculative or unreliable opinions are not presented to the jury. In Daubert, the Supreme Court identified four factors to guide assessment of an expert’s methodology: (1) whether the expert’s methodology has been tested or is capable of being tested; (2) whether the theory or technique used by the expert has been subjected to peer review and publication; (3) whether there is a known or potential error rate of the methodology; and (4) whether the technique has been generally accepted in the relevant scientific community. The Daubert standard is applicable to expert testimony in all federal cases. Many states also have adopted standards identical to the federal Daubert standard.
The term “Daubert Standard” comes from the United States Supreme Court case: Daubert v Merrell Dow Pharmaceuticals Inc 509 US 579 (1993).
GSK is a global biopharma company with a purpose to unite science, technology, and talent to get ahead of disease together. Find out more at gsk.com.
Cautionary statement regarding forward-looking statements
GSK cautions investors that any forward-looking statements or projections made by GSK, including those made in this announcement, are subject to risks and uncertainties that may cause actual results to differ materially from those projected. Such factors include, but are not limited to, those described under Item 3.D “Risk factors” in GSK’s Annual Report on Form 20-F for 2023, and GSK’s Q2 Results for 2024.
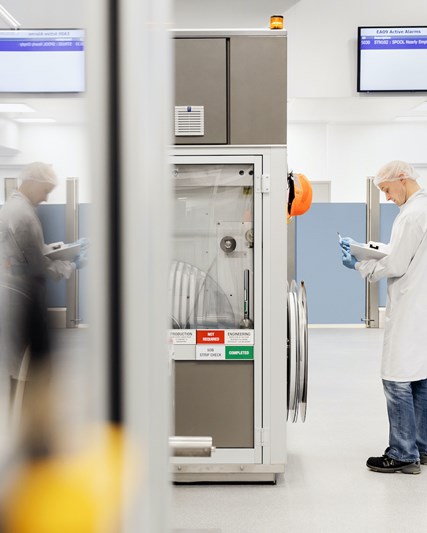
Media contacts
Access the contact details of our media relations teams at GSK
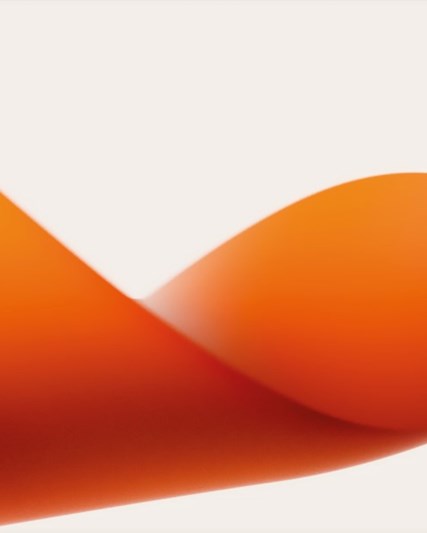
Media library
Search and download images and B-roll video for use in the media.
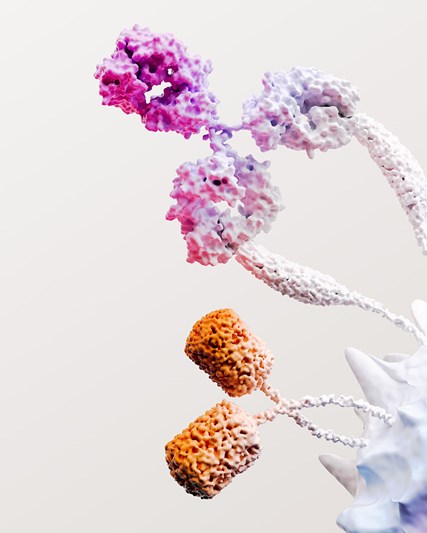
We are a global biopharma company with a purpose to unite science, technology and talent to get ahead of disease together
Event Categories: BSPS Choice Group Conjectures and Refutations Popper Seminar Sigma Club
« All Events
AI in public policy: opportunities and challenges
5 november, 6:30 pm – 8:00 pm, event navigation.
- Lucy McDonald (King’s College London): ‘Power and Romantic Relationships’ »
THIS EVENT IS PART OF THE LSE PUBLIC LECTURE SERIES.
In a world increasingly shaped by digital transformation, AI and data science present new opportunities to change policymaking in nearly all areas of policy. Yet the capabilities of these emerging technologies are still unfolding and need to be better understood, both in terms of their benefits and their limitations.
This events launch the publication of the most recent issue of the LSE Public Policy Review , which brings together contributions from a range of disciplines – from philosophy to statistics, government and law – to reflect together on future directions, applications, and consequences of the use of AI in public policies. Join our panellists as they discuss how emerging technologies can transform evidence-based policy development through their analytical capabilities, predictive powers, and real-time monitoring, while also bringing questions around regulation, transparency, accountability and ethics to the fore.
Meet our speakers and chair
Helen Margetts ( @HelenMargetts ) is Professor of Society and the Internet at the University of Oxford and the Programme Director for Public Policy at The Alan Turing Institute for Data Science and Artificial Intelligence.
Andrew Murray is Professor of Law at LSE and a Fellow of the Royal Society of Arts (FRSA). He is the Academic Director of LSE’s Law, Technology and Society research group and is Academic Director of LSE Online. He has been since 2014 a visiting Professor at the Amsterdam Law and Technology Institute and was in Spring 2015 and Spring 2017 a visiting Professor at the Paris Institute of Political Science (Sciences Po).
Kate Vredenburgh is Assistant Professor in the Department of Philosophy, Logic and Scientific Method at LSE. She works in the philosophy of social science, political philosophy, and the philosophy of technology. Much of her research and teaching interact with other disciplines, such as economics, sociology, and computer science.
Ken Benoit ( @kenbenoit ) is Director of the Data Science Institute at LSE and Professor of Computational Social Science in the Department of Methodology.
More about this event
This event will be available to watch on LSE Live. LSE Live is the new home for our live streams, allowing you to tune in and join the global debate at LSE, wherever you are in the world. If you can’t attend live, a video will be made available shortly afterwards on LSE’s YouTube channel .
The LSE School of Public Policy ( @LSEPublicPolicy ) equips you with the skills and ideas to transform people and societies. It is an international community where ideas and practice meet. Their approach creates professionals with the ability to analyse, understand and resolve the challenges of contemporary governance.
The LSE Public Policy Review is a journal published by LSE Press ( @LSEPress ) and hosted by the School of Public Policy.
The Data Science Institute ( @LSEDataScience ) is an interdisciplinary institute established to foster the study of data science and new forms of data with a focus on their social, economic and political aspects.
LINK TO THE EVENT PAGE.

IMAGES
COMMENTS
Department of Methodology. The Department is an international centre of excellence in social science methodology. We offer postgraduate programmes in social research methods, applied social data science and demography. We also run courses for students across LSE covering research design, qualitative, quantitative and computational methods.
Study in the Department of Methodology. Our students study specialised master's degrees and globally competitive PhD programmes. We take around 80 postgraduate students each year from a range of backgrounds and provide teaching to thousands of LSE students who take our courses and workshops. The Department of Methodology ensures that LSE's ...
The Department of Methodology runs two master's programmes and two Doctoral programmes: The Department also runs a Visiting Research Student in Social Research Methods scheme. Our students join the Department of Methodology from all over the world and at varying stages in their careers. The Department provides an extensive range of courses ...
The Departmental of Methodology's key function is to provide training for PhD and MSc students and LSE staff in the design of social research and in qualitative and quantitative analysis For more ...
Methodology training. The Department of Methodology at LSE provides specialist training in advanced qualitative and quantitative methods. Much of what they do is geared towards doctoral students and will take place in the teaching room in the PhD Academy on the 4th floor of the LSE Lionel Robbins building (see maps and directions ).
The focus of the Department of Methodology's MSc in Applied Social Data Science contrasts to the MSc in Data Science offered by the Department of Statistics in the following ways. Firstly, the MSc in Applied Social Data Science offers a more solid training on social science research design, which we believe is a fundamental part of what data science means.
Hendry, Pagan, and Sargan (1984) discuss a typology for dynamic single-equation models for time-series variables, and Hendry (1994) presents a typology for the various types of dynamic model used in the analysis of systems of equations. There is also a wide range of views about the appropriate way to develop and evaluate models.
Department of Methodology. The Department is an international centre of excellence in social science methodology. We offer postgraduate programmes in social research methods, applied social data science and demography. We also run courses for students across LSE covering research design, qualitative, quantitative and computational methods.
GitHub organization for the Dept. of Methodology, London School of Economics - LSE Department of Methodology
Email: [email protected] Tel: +44 (0)20 7955 7639. Department Operations, Research Activities, PhD Programmes. Camilya Maleh Department and Research Operations Manager. Ask me about: Day-to-day running of the MPhil/PhD programmes; department operations; research activities, including communications and events.
Language Centre. LSE Law School. Department of Management. Marshall Institute. Department of Mathematics. Department of Media and Communications. Department of Methodology. Department of Philosophy, Logic and Scientific Method. Department of Psychological and Behavioural Science.
The latest posts from @methodologylse
For queries about our short courses, please email [email protected]. Please note that Methods Short Courses run exclusively during Winter and Spring Term. The new schedule will be published in January 2025. To browse our past short courses and keep up to date with upcoming ones, ...
573 Followers, 1,149 Following, 20 Posts - LSE Department of Methodology (@lsemethodology) on Instagram: " Welcome to our IG account! We're an international centre of excellence in social science methodology #lsemethodology #partoflse"
All Methodology Moodle pages are open-access, and lecture recordings will be posted there. I am an LSE MRes/Research student; how should I apply to audit? Registered LSE MRes/Research students can apply to audit Methodology courses via LSE For You by marking them as 'audit' in the final step when composing your 'Student Statement'. There is no ...
The Department of Methodology is an internationally recognised centre of excellence in research and teaching in the area of social science research methodology. The disciplinary backgrounds of the staff include political science, statistics, sociology, social psychology, anthropology and criminology. The Department coordinates and provides a ...
The Digital Skills Lab offers support in a range of specialist research tools. See below to find out what's on offer. If you need help with a specific tool not listed, please email [email protected] to see how we can help. If you need help with NVivo, SPSS, Stata or Qualtrics, check out the Digital Skills Lab drop-in sessions via Teams .
The LSE Calendar is the School's central source of Programme Regulations and Course Guides, as well as School and Academic Regulations. All courses in the LSE Calendar with the prefix 'MY' are Department of Methodology courses. Explore what we have to offer! Undergraduate courses | Taught master's courses | Research courses. Most of our courses are available to students from across LSE, not ...
The methodology is often referred to as general-to-specific modelling, "Gets modeling" or "Hendry's methodology". The software package OxMetrics implements this process via the PcGive module Autometrics. In the 1970s, when the LSE approach was in its infancy, Edward E. Leamer was an early critic of model discovery methodologies. [citation needed]
Registered LSE MRes/Research students can apply to audit Methodology courses via LSE For You by marking them as 'audit' in the final step when composing your 'Student Statement'. Non-LSE students are not permitted to audit courses unless they register as an Intercollegiate Student, if eligible.
GSK plc (LSE/NYSE: GSK) welcomes today's decision by the Delaware Supreme Court that it will review the Delaware Superior Court's decision allowing the introduction of plaintiffs' expert evidence at trial. ... In Daubert, the Supreme Court identified four factors to guide assessment of an expert's methodology: (1) whether the expert's ...
The Department of Methodology also has some online tutorials in SPSS that were produced in 2011 that students have found useful along with a YouTube training channel. The Department of Methodology also provides training for PhD and MSc students as well as staff in the design of social research and in qualitative and quantitative analysis.
Research. The Department of Methodology is a national centre of excellence in methodology. Our faculty pursue research in a number of different disciplines; their work can be found in journals covering a variety of different domains of enquiry. The Department is also home to a number of funded research projects. Past research projects. POP at LSE.
The Department of Methodology at LSE supports both standalone qualitative and quantitative research, as well as interesting ways of combining them. We encourage applications from candidates who demonstrate an interest in a substantive area of research and particular methodological approach, aiming at a methodological development.
GSK plc (LSE/NYSE: GSK) welcomes today's decision by the Delaware Supreme Court that it will review the Delaware Superior Court's decision allowing the introduction of plaintiffs' expert evidence at trial. ... In Daubert, the Supreme Court identified four factors to guide assessment of an expert's methodology: (1) whether the expert's ...
THIS EVENT IS PART OF THE LSE PUBLIC LECTURE SERIES. In a world increasingly shaped by digital transformation, AI and data science present new opportunities to change policymaking in nearly all areas of policy. ... is Director of the Data Science Institute at LSE and Professor of Computational Social Science in the Department of Methodology ...