- USC Libraries
- Research Guides

Organizing Your Social Sciences Research Paper
- Independent and Dependent Variables
- Purpose of Guide
- Design Flaws to Avoid
- Glossary of Research Terms
- Reading Research Effectively
- Narrowing a Topic Idea
- Broadening a Topic Idea
- Extending the Timeliness of a Topic Idea
- Academic Writing Style
- Applying Critical Thinking
- Choosing a Title
- Making an Outline
- Paragraph Development
- Research Process Video Series
- Executive Summary
- The C.A.R.S. Model
- Background Information
- The Research Problem/Question
- Theoretical Framework
- Citation Tracking
- Content Alert Services
- Evaluating Sources
- Primary Sources
- Secondary Sources
- Tiertiary Sources
- Scholarly vs. Popular Publications
- Qualitative Methods
- Quantitative Methods
- Insiderness
- Using Non-Textual Elements
- Limitations of the Study
- Common Grammar Mistakes
- Writing Concisely
- Avoiding Plagiarism
- Footnotes or Endnotes?
- Further Readings
- Generative AI and Writing
- USC Libraries Tutorials and Other Guides
- Bibliography
Definitions
Dependent Variable The variable that depends on other factors that are measured. These variables are expected to change as a result of an experimental manipulation of the independent variable or variables. It is the presumed effect.
Independent Variable The variable that is stable and unaffected by the other variables you are trying to measure. It refers to the condition of an experiment that is systematically manipulated by the investigator. It is the presumed cause.
Cramer, Duncan and Dennis Howitt. The SAGE Dictionary of Statistics . London: SAGE, 2004; Penslar, Robin Levin and Joan P. Porter. Institutional Review Board Guidebook: Introduction . Washington, DC: United States Department of Health and Human Services, 2010; "What are Dependent and Independent Variables?" Graphic Tutorial.
Identifying Dependent and Independent Variables
Don't feel bad if you are confused about what is the dependent variable and what is the independent variable in social and behavioral sciences research . However, it's important that you learn the difference because framing a study using these variables is a common approach to organizing the elements of a social sciences research study in order to discover relevant and meaningful results. Specifically, it is important for these two reasons:
- You need to understand and be able to evaluate their application in other people's research.
- You need to apply them correctly in your own research.
A variable in research simply refers to a person, place, thing, or phenomenon that you are trying to measure in some way. The best way to understand the difference between a dependent and independent variable is that the meaning of each is implied by what the words tell us about the variable you are using. You can do this with a simple exercise from the website, Graphic Tutorial. Take the sentence, "The [independent variable] causes a change in [dependent variable] and it is not possible that [dependent variable] could cause a change in [independent variable]." Insert the names of variables you are using in the sentence in the way that makes the most sense. This will help you identify each type of variable. If you're still not sure, consult with your professor before you begin to write.
Fan, Shihe. "Independent Variable." In Encyclopedia of Research Design. Neil J. Salkind, editor. (Thousand Oaks, CA: SAGE, 2010), pp. 592-594; "What are Dependent and Independent Variables?" Graphic Tutorial; Salkind, Neil J. "Dependent Variable." In Encyclopedia of Research Design , Neil J. Salkind, editor. (Thousand Oaks, CA: SAGE, 2010), pp. 348-349;
Structure and Writing Style
The process of examining a research problem in the social and behavioral sciences is often framed around methods of analysis that compare, contrast, correlate, average, or integrate relationships between or among variables . Techniques include associations, sampling, random selection, and blind selection. Designation of the dependent and independent variable involves unpacking the research problem in a way that identifies a general cause and effect and classifying these variables as either independent or dependent.
The variables should be outlined in the introduction of your paper and explained in more detail in the methods section . There are no rules about the structure and style for writing about independent or dependent variables but, as with any academic writing, clarity and being succinct is most important.
After you have described the research problem and its significance in relation to prior research, explain why you have chosen to examine the problem using a method of analysis that investigates the relationships between or among independent and dependent variables . State what it is about the research problem that lends itself to this type of analysis. For example, if you are investigating the relationship between corporate environmental sustainability efforts [the independent variable] and dependent variables associated with measuring employee satisfaction at work using a survey instrument, you would first identify each variable and then provide background information about the variables. What is meant by "environmental sustainability"? Are you looking at a particular company [e.g., General Motors] or are you investigating an industry [e.g., the meat packing industry]? Why is employee satisfaction in the workplace important? How does a company make their employees aware of sustainability efforts and why would a company even care that its employees know about these efforts?
Identify each variable for the reader and define each . In the introduction, this information can be presented in a paragraph or two when you describe how you are going to study the research problem. In the methods section, you build on the literature review of prior studies about the research problem to describe in detail background about each variable, breaking each down for measurement and analysis. For example, what activities do you examine that reflect a company's commitment to environmental sustainability? Levels of employee satisfaction can be measured by a survey that asks about things like volunteerism or a desire to stay at the company for a long time.
The structure and writing style of describing the variables and their application to analyzing the research problem should be stated and unpacked in such a way that the reader obtains a clear understanding of the relationships between the variables and why they are important. This is also important so that the study can be replicated in the future using the same variables but applied in a different way.
Fan, Shihe. "Independent Variable." In Encyclopedia of Research Design. Neil J. Salkind, editor. (Thousand Oaks, CA: SAGE, 2010), pp. 592-594; "What are Dependent and Independent Variables?" Graphic Tutorial; “Case Example for Independent and Dependent Variables.” ORI Curriculum Examples. U.S. Department of Health and Human Services, Office of Research Integrity; Salkind, Neil J. "Dependent Variable." In Encyclopedia of Research Design , Neil J. Salkind, editor. (Thousand Oaks, CA: SAGE, 2010), pp. 348-349; “Independent Variables and Dependent Variables.” Karl L. Wuensch, Department of Psychology, East Carolina University [posted email exchange]; “Variables.” Elements of Research. Dr. Camille Nebeker, San Diego State University.
- << Previous: Design Flaws to Avoid
- Next: Glossary of Research Terms >>
- Last Updated: Apr 24, 2024 10:51 AM
- URL: https://libguides.usc.edu/writingguide
Have a language expert improve your writing
Run a free plagiarism check in 10 minutes, automatically generate references for free.
- Knowledge Base
- Methodology
- Independent vs Dependent Variables | Definition & Examples
Independent vs Dependent Variables | Definition & Examples
Published on 4 May 2022 by Pritha Bhandari . Revised on 17 October 2022.
In research, variables are any characteristics that can take on different values, such as height, age, temperature, or test scores.
Researchers often manipulate or measure independent and dependent variables in studies to test cause-and-effect relationships.
- The independent variable is the cause. Its value is independent of other variables in your study.
- The dependent variable is the effect. Its value depends on changes in the independent variable.
Your independent variable is the temperature of the room. You vary the room temperature by making it cooler for half the participants, and warmer for the other half.
Table of contents
What is an independent variable, types of independent variables, what is a dependent variable, identifying independent vs dependent variables, independent and dependent variables in research, visualising independent and dependent variables, frequently asked questions about independent and dependent variables.
An independent variable is the variable you manipulate or vary in an experimental study to explore its effects. It’s called ‘independent’ because it’s not influenced by any other variables in the study.
Independent variables are also called:
- Explanatory variables (they explain an event or outcome)
- Predictor variables (they can be used to predict the value of a dependent variable)
- Right-hand-side variables (they appear on the right-hand side of a regression equation).
These terms are especially used in statistics , where you estimate the extent to which an independent variable change can explain or predict changes in the dependent variable.
Prevent plagiarism, run a free check.
There are two main types of independent variables.
- Experimental independent variables can be directly manipulated by researchers.
- Subject variables cannot be manipulated by researchers, but they can be used to group research subjects categorically.
Experimental variables
In experiments, you manipulate independent variables directly to see how they affect your dependent variable. The independent variable is usually applied at different levels to see how the outcomes differ.
You can apply just two levels in order to find out if an independent variable has an effect at all.
You can also apply multiple levels to find out how the independent variable affects the dependent variable.
You have three independent variable levels, and each group gets a different level of treatment.
You randomly assign your patients to one of the three groups:
- A low-dose experimental group
- A high-dose experimental group
- A placebo group
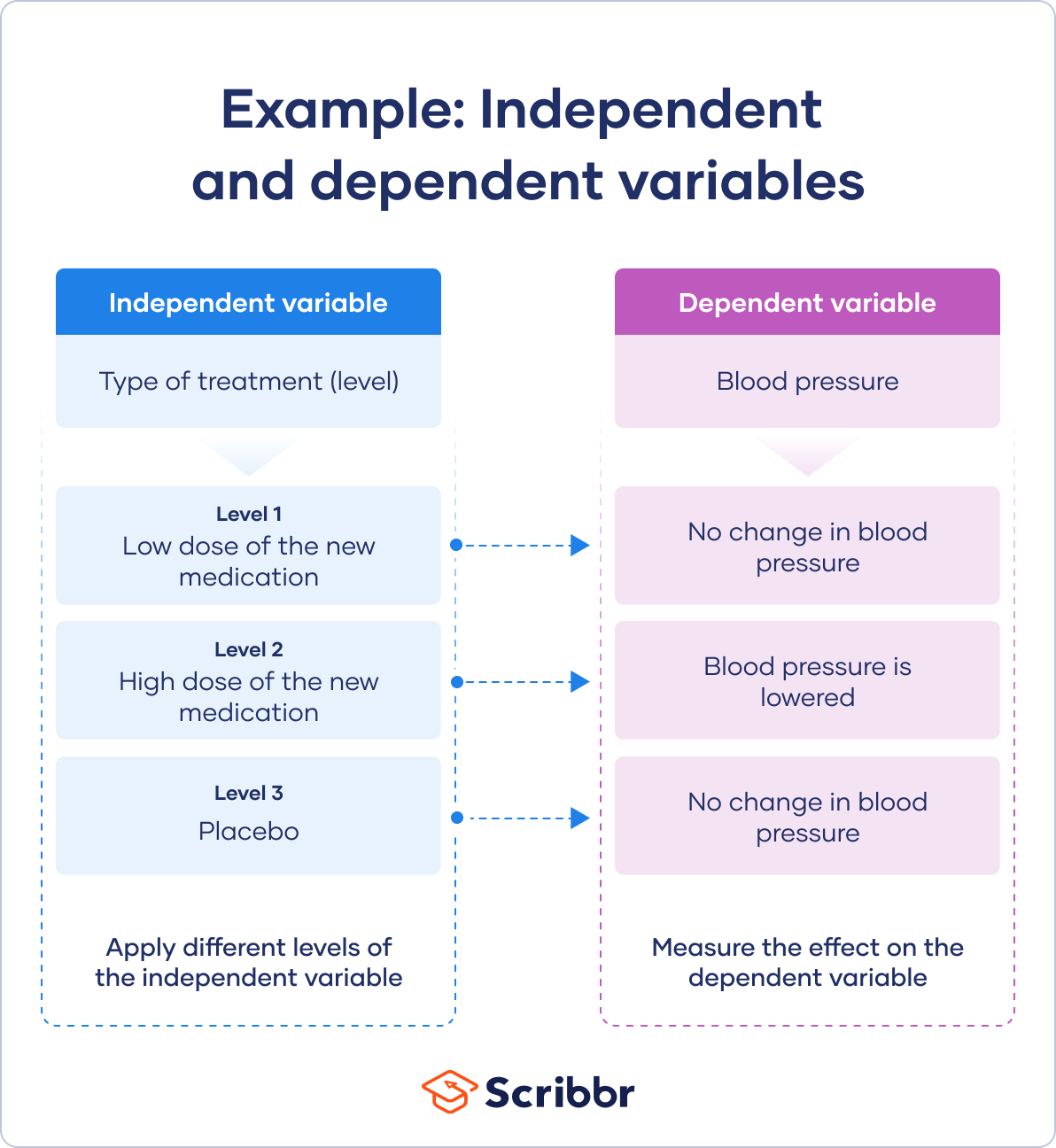
A true experiment requires you to randomly assign different levels of an independent variable to your participants.
Random assignment helps you control participant characteristics, so that they don’t affect your experimental results. This helps you to have confidence that your dependent variable results come solely from the independent variable manipulation.
Subject variables
Subject variables are characteristics that vary across participants, and they can’t be manipulated by researchers. For example, gender identity, ethnicity, race, income, and education are all important subject variables that social researchers treat as independent variables.
It’s not possible to randomly assign these to participants, since these are characteristics of already existing groups. Instead, you can create a research design where you compare the outcomes of groups of participants with characteristics. This is a quasi-experimental design because there’s no random assignment.
Your independent variable is a subject variable, namely the gender identity of the participants. You have three groups: men, women, and other.
Your dependent variable is the brain activity response to hearing infant cries. You record brain activity with fMRI scans when participants hear infant cries without their awareness.
A dependent variable is the variable that changes as a result of the independent variable manipulation. It’s the outcome you’re interested in measuring, and it ‘depends’ on your independent variable.
In statistics , dependent variables are also called:
- Response variables (they respond to a change in another variable)
- Outcome variables (they represent the outcome you want to measure)
- Left-hand-side variables (they appear on the left-hand side of a regression equation)
The dependent variable is what you record after you’ve manipulated the independent variable. You use this measurement data to check whether and to what extent your independent variable influences the dependent variable by conducting statistical analyses.
Based on your findings, you can estimate the degree to which your independent variable variation drives changes in your dependent variable. You can also predict how much your dependent variable will change as a result of variation in the independent variable.
Distinguishing between independent and dependent variables can be tricky when designing a complex study or reading an academic paper.
A dependent variable from one study can be the independent variable in another study, so it’s important to pay attention to research design.
Here are some tips for identifying each variable type.
Recognising independent variables
Use this list of questions to check whether you’re dealing with an independent variable:
- Is the variable manipulated, controlled, or used as a subject grouping method by the researcher?
- Does this variable come before the other variable in time?
- Is the researcher trying to understand whether or how this variable affects another variable?
Recognising dependent variables
Check whether you’re dealing with a dependent variable:
- Is this variable measured as an outcome of the study?
- Is this variable dependent on another variable in the study?
- Does this variable get measured only after other variables are altered?
Independent and dependent variables are generally used in experimental and quasi-experimental research.
Here are some examples of research questions and corresponding independent and dependent variables.
For experimental data, you analyse your results by generating descriptive statistics and visualising your findings. Then, you select an appropriate statistical test to test your hypothesis .
The type of test is determined by:
- Your variable types
- Level of measurement
- Number of independent variable levels
You’ll often use t tests or ANOVAs to analyse your data and answer your research questions.
In quantitative research , it’s good practice to use charts or graphs to visualise the results of studies. Generally, the independent variable goes on the x -axis (horizontal) and the dependent variable on the y -axis (vertical).
The type of visualisation you use depends on the variable types in your research questions:
- A bar chart is ideal when you have a categorical independent variable.
- A scatterplot or line graph is best when your independent and dependent variables are both quantitative.
To inspect your data, you place your independent variable of treatment level on the x -axis and the dependent variable of blood pressure on the y -axis.
You plot bars for each treatment group before and after the treatment to show the difference in blood pressure.
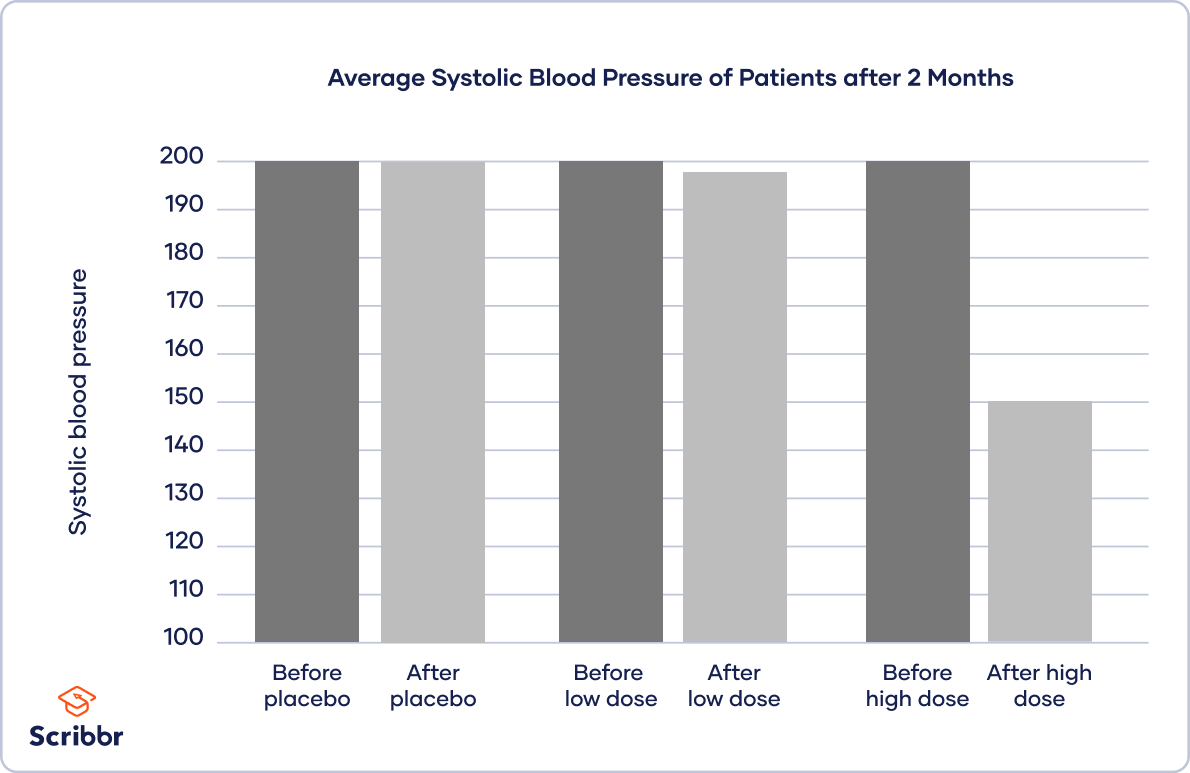
An independent variable is the variable you manipulate, control, or vary in an experimental study to explore its effects. It’s called ‘independent’ because it’s not influenced by any other variables in the study.
- Right-hand-side variables (they appear on the right-hand side of a regression equation)
A dependent variable is what changes as a result of the independent variable manipulation in experiments . It’s what you’re interested in measuring, and it ‘depends’ on your independent variable.
In statistics, dependent variables are also called:
Determining cause and effect is one of the most important parts of scientific research. It’s essential to know which is the cause – the independent variable – and which is the effect – the dependent variable.
You want to find out how blood sugar levels are affected by drinking diet cola and regular cola, so you conduct an experiment .
- The type of cola – diet or regular – is the independent variable .
- The level of blood sugar that you measure is the dependent variable – it changes depending on the type of cola.
Yes, but including more than one of either type requires multiple research questions .
For example, if you are interested in the effect of a diet on health, you can use multiple measures of health: blood sugar, blood pressure, weight, pulse, and many more. Each of these is its own dependent variable with its own research question.
You could also choose to look at the effect of exercise levels as well as diet, or even the additional effect of the two combined. Each of these is a separate independent variable .
To ensure the internal validity of an experiment , you should only change one independent variable at a time.
No. The value of a dependent variable depends on an independent variable, so a variable cannot be both independent and dependent at the same time. It must be either the cause or the effect, not both.
Cite this Scribbr article
If you want to cite this source, you can copy and paste the citation or click the ‘Cite this Scribbr article’ button to automatically add the citation to our free Reference Generator.
Bhandari, P. (2022, October 17). Independent vs Dependent Variables | Definition & Examples. Scribbr. Retrieved 22 April 2024, from https://www.scribbr.co.uk/research-methods/independent-vs-dependent-variables/
Is this article helpful?
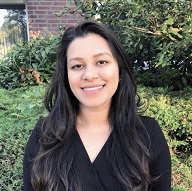
Pritha Bhandari
Other students also liked, a quick guide to experimental design | 5 steps & examples, quasi-experimental design | definition, types & examples, types of variables in research | definitions & examples.
Variables: Definition, Examples, Types of Variables in Research

What is a Variable?
Within the context of a research investigation, concepts are generally referred to as variables. A variable is, as the name applies, something that varies.
Examples of Variable
These are all examples of variables because each of these properties varies or differs from one individual to another.
- income and expenses,
- family size,
- country of birth,
- capital expenditure,
- class grades,
- blood pressure readings,
- preoperative anxiety levels,
- eye color, and
- vehicle type.
What is Variable in Research?
A variable is any property, characteristic, number, or quantity that increases or decreases over time or can take on different values (as opposed to constants, such as n , that do not vary) in different situations.
When conducting research, experiments often manipulate variables. For example, an experimenter might compare the effectiveness of four types of fertilizers.
In this case, the variable is the ‘type of fertilizers.’ A social scientist may examine the possible effect of early marriage on divorce. Her early marriage is variable.
A business researcher may find it useful to include the dividend in determining the share prices . Here, the dividend is the variable.
Effectiveness, divorce, and share prices are variables because they also vary due to manipulating fertilizers, early marriage, and dividends.
11 Types of Variables in Research
Qualitative variables.
An important distinction between variables is the qualitative and quantitative variables.
Qualitative variables are those that express a qualitative attribute, such as hair color, religion, race, gender, social status, method of payment, and so on. The values of a qualitative variable do not imply a meaningful numerical ordering.
The value of the variable ‘religion’ (Muslim, Hindu.., etc..) differs qualitatively; no ordering of religion is implied. Qualitative variables are sometimes referred to as categorical variables.
For example, the variable sex has two distinct categories: ‘male’ and ‘female.’ Since the values of this variable are expressed in categories, we refer to this as a categorical variable.
Similarly, the place of residence may be categorized as urban and rural and thus is a categorical variable.
Categorical variables may again be described as nominal and ordinal.
Ordinal variables can be logically ordered or ranked higher or lower than another but do not necessarily establish a numeric difference between each category, such as examination grades (A+, A, B+, etc., and clothing size (Extra large, large, medium, small).
Nominal variables are those that can neither be ranked nor logically ordered, such as religion, sex, etc.
A qualitative variable is a characteristic that is not capable of being measured but can be categorized as possessing or not possessing some characteristics.
Quantitative Variables
Quantitative variables, also called numeric variables, are those variables that are measured in terms of numbers. A simple example of a quantitative variable is a person’s age.
Age can take on different values because a person can be 20 years old, 35 years old, and so on. Likewise, family size is a quantitative variable because a family might be comprised of one, two, or three members, and so on.
Each of these properties or characteristics referred to above varies or differs from one individual to another. Note that these variables are expressed in numbers, for which we call quantitative or sometimes numeric variables.
A quantitative variable is one for which the resulting observations are numeric and thus possess a natural ordering or ranking.
Discrete and Continuous Variables
Quantitative variables are again of two types: discrete and continuous.
Variables such as some children in a household or the number of defective items in a box are discrete variables since the possible scores are discrete on the scale.
For example, a household could have three or five children, but not 4.52 children.
Other variables, such as ‘time required to complete an MCQ test’ and ‘waiting time in a queue in front of a bank counter,’ are continuous variables.
The time required in the above examples is a continuous variable, which could be, for example, 1.65 minutes or 1.6584795214 minutes.
Of course, the practicalities of measurement preclude most measured variables from being continuous.
Discrete Variable
A discrete variable, restricted to certain values, usually (but not necessarily) consists of whole numbers, such as the family size and a number of defective items in a box. They are often the results of enumeration or counting.
A few more examples are;
- The number of accidents in the twelve months.
- The number of mobile cards sold in a store within seven days.
- The number of patients admitted to a hospital over a specified period.
- The number of new branches of a bank opened annually during 2001- 2007.
- The number of weekly visits made by health personnel in the last 12 months.
Continuous Variable
A continuous variable may take on an infinite number of intermediate values along a specified interval. Examples are:
- The sugar level in the human body;
- Blood pressure reading;
- Temperature;
- Height or weight of the human body;
- Rate of bank interest;
- Internal rate of return (IRR),
- Earning ratio (ER);
- Current ratio (CR)
No matter how close two observations might be, if the instrument of measurement is precise enough, a third observation can be found, falling between the first two.
A continuous variable generally results from measurement and can assume countless values in the specified range.
Dependent Variables and Independent Variable
In many research settings, two specific classes of variables need to be distinguished from one another: independent variable and dependent variable.
Many research studies aim to reveal and understand the causes of underlying phenomena or problems with the ultimate goal of establishing a causal relationship between them.
Look at the following statements:
- Low intake of food causes underweight.
- Smoking enhances the risk of lung cancer.
- Level of education influences job satisfaction.
- Advertisement helps in sales promotion.
- The drug causes improvement of health problems.
- Nursing intervention causes more rapid recovery.
- Previous job experiences determine the initial salary.
- Blueberries slow down aging.
- The dividend per share determines share prices.
In each of the above queries, we have two independent and dependent variables. In the first example, ‘low intake of food’ is believed to have caused the ‘problem of being underweight.’
It is thus the so-called independent variable. Underweight is the dependent variable because we believe this ‘problem’ (the problem of being underweight) has been caused by ‘the low intake of food’ (the factor).
Similarly, smoking, dividend, and advertisement are all independent variables, and lung cancer, job satisfaction, and sales are dependent variables.
In general, an independent variable is manipulated by the experimenter or researcher, and its effects on the dependent variable are measured.
Independent Variable
The variable that is used to describe or measure the factor that is assumed to cause or at least to influence the problem or outcome is called an independent variable.
The definition implies that the experimenter uses the independent variable to describe or explain its influence or effect of it on the dependent variable.
Variability in the dependent variable is presumed to depend on variability in the independent variable.
Depending on the context, an independent variable is sometimes called a predictor variable, regressor, controlled variable, manipulated variable, explanatory variable, exposure variable (as used in reliability theory), risk factor (as used in medical statistics), feature (as used in machine learning and pattern recognition) or input variable.
The explanatory variable is preferred by some authors over the independent variable when the quantities treated as independent variables may not be statistically independent or independently manipulable by the researcher.
If the independent variable is referred to as an explanatory variable, then the term response variable is preferred by some authors for the dependent variable.
Dependent Variable
The variable used to describe or measure the problem or outcome under study is called a dependent variable.
In a causal relationship, the cause is the independent variable, and the effect is the dependent variable. If we hypothesize that smoking causes lung cancer, ‘smoking’ is the independent variable and cancer the dependent variable.
A business researcher may find it useful to include the dividend in determining the share prices. Here dividend is the independent variable, while the share price is the dependent variable.
The dependent variable usually is the variable the researcher is interested in understanding, explaining, or predicting.
In lung cancer research, the carcinoma is of real interest to the researcher, not smoking behavior per se. The independent variable is the presumed cause of, antecedent to, or influence on the dependent variable.
Depending on the context, a dependent variable is sometimes called a response variable, regressand, predicted variable, measured variable, explained variable, experimental variable, responding variable, outcome variable, output variable, or label.
An explained variable is preferred by some authors over the dependent variable when the quantities treated as dependent variables may not be statistically dependent.
If the dependent variable is referred to as an explained variable, then the term predictor variable is preferred by some authors for the independent variable.
Levels of an Independent Variable
If an experimenter compares an experimental treatment with a control treatment, then the independent variable (a type of treatment) has two levels: experimental and control.
If an experiment were to compare five types of diets, then the independent variables (types of diet) would have five levels.
In general, the number of levels of an independent variable is the number of experimental conditions.
Background Variable
In almost every study, we collect information such as age, sex, educational attainment, socioeconomic status, marital status, religion, place of birth, and the like. These variables are referred to as background variables.
These variables are often related to many independent variables, so they indirectly influence the problem. Hence they are called background variables.
The background variables should be measured if they are important to the study. However, we should try to keep the number of background variables as few as possible in the interest of the economy.
Moderating Variable
In any statement of relationships of variables, it is normally hypothesized that in some way, the independent variable ’causes’ the dependent variable to occur.
In simple relationships, all other variables are extraneous and are ignored.
In actual study situations, such a simple one-to-one relationship needs to be revised to take other variables into account to explain the relationship better.
This emphasizes the need to consider a second independent variable that is expected to have a significant contributory or contingent effect on the originally stated dependent-independent relationship.
Such a variable is termed a moderating variable.
Suppose you are studying the impact of field-based and classroom-based training on the work performance of health and family planning workers. You consider the type of training as the independent variable.
If you are focusing on the relationship between the age of the trainees and work performance, you might use ‘type of training’ as a moderating variable.
Extraneous Variable
Most studies concern the identification of a single independent variable and measuring its effect on the dependent variable.
But still, several variables might conceivably affect our hypothesized independent-dependent variable relationship, thereby distorting the study. These variables are referred to as extraneous variables.
Extraneous variables are not necessarily part of the study. They exert a confounding effect on the dependent-independent relationship and thus need to be eliminated or controlled for.
An example may illustrate the concept of extraneous variables. Suppose we are interested in examining the relationship between the work status of mothers and breastfeeding duration.
It is not unreasonable in this instance to presume that the level of education of mothers as it influences work status might have an impact on breastfeeding duration too.
Education is treated here as an extraneous variable. In any attempt to eliminate or control the effect of this variable, we may consider this variable a confounding variable.
An appropriate way of dealing with confounding variables is to follow the stratification procedure, which involves a separate analysis of the different levels of lies in confounding variables.
For this purpose, one can construct two crosstables for illiterate mothers and the other for literate mothers.
Suppose we find a similar association between work status and duration of breastfeeding in both the groups of mothers. In that case, we conclude that mothers’ educational level is not a confounding variable.
Intervening Variable
Often an apparent relationship between two variables is caused by a third variable.
For example, variables X and Y may be highly correlated, but only because X causes the third variable, Z, which in turn causes Y. In this case, Z is the intervening variable.
An intervening variable theoretically affects the observed phenomena but cannot be seen, measured, or manipulated directly; its effects can only be inferred from the effects of the independent and moderating variables on the observed phenomena.
We might view motivation or counseling as the intervening variable in the work-status and breastfeeding relationship.
Thus, motive, job satisfaction, responsibility, behavior, and justice are some of the examples of intervening variables.
Suppressor Variable
In many cases, we have good reasons to believe that the variables of interest have a relationship, but our data fail to establish any such relationship. Some hidden factors may suppress the true relationship between the two original variables.
Such a factor is referred to as a suppressor variable because it suppresses the relationship between the other two variables.
The suppressor variable suppresses the relationship by being positively correlated with one of the variables in the relationship and negatively correlated with the other. The true relationship between the two variables will reappear when the suppressor variable is controlled for.
Thus, for example, low age may pull education up but income down. In contrast, a high age may pull income up but education down, effectively canceling the relationship between education and income unless age is controlled for.
4 Relationships Between Variables

In dealing with relationships between variables in research, we observe a variety of dimensions in these relationships.
Positive and Negative Relationship
Symmetrical relationship, causal relationship, linear and non-linear relationship.
Two or more variables may have a positive, negative, or no relationship. In the case of two variables, a positive relationship is one in which both variables vary in the same direction.
However, they are said to have a negative relationship when they vary in opposite directions.
When a change in the other variable does not accompany the change or movement of one variable, we say that the variables in question are unrelated.
For example, if an increase in wage rate accompanies one’s job experience, the relationship between job experience and the wage rate is positive.
If an increase in an individual’s education level decreases his desire for additional children, the relationship is negative or inverse.
If the level of education does not have any bearing on the desire, we say that the variables’ desire for additional children and ‘education’ are unrelated.
Strength of Relationship
Once it has been established that two variables are related, we want to ascertain how strongly they are related.
A common statistic to measure the strength of a relationship is the so-called correlation coefficient symbolized by r. r is a unit-free measure, lying between -1 and +1 inclusive, with zero signifying no linear relationship.
As far as the prediction of one variable from the knowledge of the other variable is concerned, a value of r= +1 means a 100% accuracy in predicting a positive relationship between the two variables, and a value of r = -1 means a 100% accuracy in predicting a negative relationship between the two variables.
So far, we have discussed only symmetrical relationships in which a change in the other variable accompanies a change in either variable.
This relationship does not indicate which variable is the independent variable and which variable is the dependent variable.
In other words, you can label either of the variables as the independent variable.
Such a relationship is a symmetrical relationship. In an asymmetrical relationship, a change in variable X (say) is accompanied by a change in variable Y, but not vice versa.
The amount of rainfall, for example, will increase productivity, but productivity will not affect the rainfall. This is an asymmetrical relationship.
Similarly, the relationship between smoking and lung cancer would be asymmetrical because smoking could cause cancer, but lung cancer could not cause smoking.
Indicating a relationship between two variables does not automatically ensure that changes in one variable cause changes in another.
It is, however, very difficult to establish the existence of causality between variables. While no one can ever be certain that variable A causes variable B , one can gather some evidence that increases our belief that A leads to B.
In an attempt to do so, we seek the following evidence:
- Is there a relationship between A and B? When such evidence exists, it indicates a possible causal link between the variables.
- Is the relationship asymmetrical so that a change in A results in B but not vice-versa? In other words, does A occur before B? If we find that B occurs before A, we can have little confidence that A causes.
- Does a change in A result in a change in B regardless of the actions of other factors? Or, is it possible to eliminate other possible causes of B? Can one determine that C, D, and E (say) do not co-vary with B in a way that suggests possible causal connections?
A linear relationship is a straight-line relationship between two variables, where the variables vary at the same rate regardless of whether the values are low, high, or intermediate.
This is in contrast with the non-linear (or curvilinear) relationships, where the rate at which one variable changes in value may differ for different values of the second variable.
Whether a variable is linearly related to the other variable or not can simply be ascertained by plotting the K values against X values.
If the values, when plotted, appear to lie on a straight line, the existence of a linear relationship between X and Y is suggested.
Height and weight almost always have an approximately linear relationship, while age and fertility rates have a non-linear relationship.
Frequently Asked Questions about Variable
What is a variable within the context of a research investigation.
A variable, within the context of a research investigation, refers to concepts that vary. It can be any property, characteristic, number, or quantity that can increase or decrease over time or take on different values.
How is a variable used in research?
In research, a variable is any property or characteristic that can take on different values. Experiments often manipulate variables to compare outcomes. For instance, an experimenter might compare the effectiveness of different types of fertilizers, where the variable is the ‘type of fertilizers.’
What distinguishes qualitative variables from quantitative variables?
Qualitative variables express a qualitative attribute, such as hair color or religion, and do not imply a meaningful numerical ordering. Quantitative variables, on the other hand, are measured in terms of numbers, like a person’s age or family size.
How do discrete and continuous variables differ in terms of quantitative variables?
Discrete variables are restricted to certain values, often whole numbers, resulting from enumeration or counting, like the number of children in a household. Continuous variables can take on an infinite number of intermediate values along a specified interval, such as the time required to complete a test.
What are the roles of independent and dependent variables in research?
In research, the independent variable is manipulated by the researcher to observe its effects on the dependent variable. The independent variable is the presumed cause or influence, while the dependent variable is the outcome or effect that is being measured.
What is a background variable in a study?
Background variables are information collected in a study, such as age, sex, or educational attainment. These variables are often related to many independent variables and indirectly influence the main problem or outcome, hence they are termed background variables.
How does a suppressor variable affect the relationship between two other variables?
A suppressor variable can suppress or hide the true relationship between two other variables. It does this by being positively correlated with one of the variables and negatively correlated with the other. When the suppressor variable is controlled for, the true relationship between the two original variables can be observed.

Your email address will not be published. Required fields are marked *

An official website of the United States government
The .gov means it’s official. Federal government websites often end in .gov or .mil. Before sharing sensitive information, make sure you’re on a federal government site.
The site is secure. The https:// ensures that you are connecting to the official website and that any information you provide is encrypted and transmitted securely.
- Publications
- Account settings
Preview improvements coming to the PMC website in October 2024. Learn More or Try it out now .
- Advanced Search
- Journal List
- J Korean Med Sci
- v.37(16); 2022 Apr 25

A Practical Guide to Writing Quantitative and Qualitative Research Questions and Hypotheses in Scholarly Articles
Edward barroga.
1 Department of General Education, Graduate School of Nursing Science, St. Luke’s International University, Tokyo, Japan.
Glafera Janet Matanguihan
2 Department of Biological Sciences, Messiah University, Mechanicsburg, PA, USA.
The development of research questions and the subsequent hypotheses are prerequisites to defining the main research purpose and specific objectives of a study. Consequently, these objectives determine the study design and research outcome. The development of research questions is a process based on knowledge of current trends, cutting-edge studies, and technological advances in the research field. Excellent research questions are focused and require a comprehensive literature search and in-depth understanding of the problem being investigated. Initially, research questions may be written as descriptive questions which could be developed into inferential questions. These questions must be specific and concise to provide a clear foundation for developing hypotheses. Hypotheses are more formal predictions about the research outcomes. These specify the possible results that may or may not be expected regarding the relationship between groups. Thus, research questions and hypotheses clarify the main purpose and specific objectives of the study, which in turn dictate the design of the study, its direction, and outcome. Studies developed from good research questions and hypotheses will have trustworthy outcomes with wide-ranging social and health implications.
INTRODUCTION
Scientific research is usually initiated by posing evidenced-based research questions which are then explicitly restated as hypotheses. 1 , 2 The hypotheses provide directions to guide the study, solutions, explanations, and expected results. 3 , 4 Both research questions and hypotheses are essentially formulated based on conventional theories and real-world processes, which allow the inception of novel studies and the ethical testing of ideas. 5 , 6
It is crucial to have knowledge of both quantitative and qualitative research 2 as both types of research involve writing research questions and hypotheses. 7 However, these crucial elements of research are sometimes overlooked; if not overlooked, then framed without the forethought and meticulous attention it needs. Planning and careful consideration are needed when developing quantitative or qualitative research, particularly when conceptualizing research questions and hypotheses. 4
There is a continuing need to support researchers in the creation of innovative research questions and hypotheses, as well as for journal articles that carefully review these elements. 1 When research questions and hypotheses are not carefully thought of, unethical studies and poor outcomes usually ensue. Carefully formulated research questions and hypotheses define well-founded objectives, which in turn determine the appropriate design, course, and outcome of the study. This article then aims to discuss in detail the various aspects of crafting research questions and hypotheses, with the goal of guiding researchers as they develop their own. Examples from the authors and peer-reviewed scientific articles in the healthcare field are provided to illustrate key points.
DEFINITIONS AND RELATIONSHIP OF RESEARCH QUESTIONS AND HYPOTHESES
A research question is what a study aims to answer after data analysis and interpretation. The answer is written in length in the discussion section of the paper. Thus, the research question gives a preview of the different parts and variables of the study meant to address the problem posed in the research question. 1 An excellent research question clarifies the research writing while facilitating understanding of the research topic, objective, scope, and limitations of the study. 5
On the other hand, a research hypothesis is an educated statement of an expected outcome. This statement is based on background research and current knowledge. 8 , 9 The research hypothesis makes a specific prediction about a new phenomenon 10 or a formal statement on the expected relationship between an independent variable and a dependent variable. 3 , 11 It provides a tentative answer to the research question to be tested or explored. 4
Hypotheses employ reasoning to predict a theory-based outcome. 10 These can also be developed from theories by focusing on components of theories that have not yet been observed. 10 The validity of hypotheses is often based on the testability of the prediction made in a reproducible experiment. 8
Conversely, hypotheses can also be rephrased as research questions. Several hypotheses based on existing theories and knowledge may be needed to answer a research question. Developing ethical research questions and hypotheses creates a research design that has logical relationships among variables. These relationships serve as a solid foundation for the conduct of the study. 4 , 11 Haphazardly constructed research questions can result in poorly formulated hypotheses and improper study designs, leading to unreliable results. Thus, the formulations of relevant research questions and verifiable hypotheses are crucial when beginning research. 12
CHARACTERISTICS OF GOOD RESEARCH QUESTIONS AND HYPOTHESES
Excellent research questions are specific and focused. These integrate collective data and observations to confirm or refute the subsequent hypotheses. Well-constructed hypotheses are based on previous reports and verify the research context. These are realistic, in-depth, sufficiently complex, and reproducible. More importantly, these hypotheses can be addressed and tested. 13
There are several characteristics of well-developed hypotheses. Good hypotheses are 1) empirically testable 7 , 10 , 11 , 13 ; 2) backed by preliminary evidence 9 ; 3) testable by ethical research 7 , 9 ; 4) based on original ideas 9 ; 5) have evidenced-based logical reasoning 10 ; and 6) can be predicted. 11 Good hypotheses can infer ethical and positive implications, indicating the presence of a relationship or effect relevant to the research theme. 7 , 11 These are initially developed from a general theory and branch into specific hypotheses by deductive reasoning. In the absence of a theory to base the hypotheses, inductive reasoning based on specific observations or findings form more general hypotheses. 10
TYPES OF RESEARCH QUESTIONS AND HYPOTHESES
Research questions and hypotheses are developed according to the type of research, which can be broadly classified into quantitative and qualitative research. We provide a summary of the types of research questions and hypotheses under quantitative and qualitative research categories in Table 1 .
Research questions in quantitative research
In quantitative research, research questions inquire about the relationships among variables being investigated and are usually framed at the start of the study. These are precise and typically linked to the subject population, dependent and independent variables, and research design. 1 Research questions may also attempt to describe the behavior of a population in relation to one or more variables, or describe the characteristics of variables to be measured ( descriptive research questions ). 1 , 5 , 14 These questions may also aim to discover differences between groups within the context of an outcome variable ( comparative research questions ), 1 , 5 , 14 or elucidate trends and interactions among variables ( relationship research questions ). 1 , 5 We provide examples of descriptive, comparative, and relationship research questions in quantitative research in Table 2 .
Hypotheses in quantitative research
In quantitative research, hypotheses predict the expected relationships among variables. 15 Relationships among variables that can be predicted include 1) between a single dependent variable and a single independent variable ( simple hypothesis ) or 2) between two or more independent and dependent variables ( complex hypothesis ). 4 , 11 Hypotheses may also specify the expected direction to be followed and imply an intellectual commitment to a particular outcome ( directional hypothesis ) 4 . On the other hand, hypotheses may not predict the exact direction and are used in the absence of a theory, or when findings contradict previous studies ( non-directional hypothesis ). 4 In addition, hypotheses can 1) define interdependency between variables ( associative hypothesis ), 4 2) propose an effect on the dependent variable from manipulation of the independent variable ( causal hypothesis ), 4 3) state a negative relationship between two variables ( null hypothesis ), 4 , 11 , 15 4) replace the working hypothesis if rejected ( alternative hypothesis ), 15 explain the relationship of phenomena to possibly generate a theory ( working hypothesis ), 11 5) involve quantifiable variables that can be tested statistically ( statistical hypothesis ), 11 6) or express a relationship whose interlinks can be verified logically ( logical hypothesis ). 11 We provide examples of simple, complex, directional, non-directional, associative, causal, null, alternative, working, statistical, and logical hypotheses in quantitative research, as well as the definition of quantitative hypothesis-testing research in Table 3 .
Research questions in qualitative research
Unlike research questions in quantitative research, research questions in qualitative research are usually continuously reviewed and reformulated. The central question and associated subquestions are stated more than the hypotheses. 15 The central question broadly explores a complex set of factors surrounding the central phenomenon, aiming to present the varied perspectives of participants. 15
There are varied goals for which qualitative research questions are developed. These questions can function in several ways, such as to 1) identify and describe existing conditions ( contextual research question s); 2) describe a phenomenon ( descriptive research questions ); 3) assess the effectiveness of existing methods, protocols, theories, or procedures ( evaluation research questions ); 4) examine a phenomenon or analyze the reasons or relationships between subjects or phenomena ( explanatory research questions ); or 5) focus on unknown aspects of a particular topic ( exploratory research questions ). 5 In addition, some qualitative research questions provide new ideas for the development of theories and actions ( generative research questions ) or advance specific ideologies of a position ( ideological research questions ). 1 Other qualitative research questions may build on a body of existing literature and become working guidelines ( ethnographic research questions ). Research questions may also be broadly stated without specific reference to the existing literature or a typology of questions ( phenomenological research questions ), may be directed towards generating a theory of some process ( grounded theory questions ), or may address a description of the case and the emerging themes ( qualitative case study questions ). 15 We provide examples of contextual, descriptive, evaluation, explanatory, exploratory, generative, ideological, ethnographic, phenomenological, grounded theory, and qualitative case study research questions in qualitative research in Table 4 , and the definition of qualitative hypothesis-generating research in Table 5 .
Qualitative studies usually pose at least one central research question and several subquestions starting with How or What . These research questions use exploratory verbs such as explore or describe . These also focus on one central phenomenon of interest, and may mention the participants and research site. 15
Hypotheses in qualitative research
Hypotheses in qualitative research are stated in the form of a clear statement concerning the problem to be investigated. Unlike in quantitative research where hypotheses are usually developed to be tested, qualitative research can lead to both hypothesis-testing and hypothesis-generating outcomes. 2 When studies require both quantitative and qualitative research questions, this suggests an integrative process between both research methods wherein a single mixed-methods research question can be developed. 1
FRAMEWORKS FOR DEVELOPING RESEARCH QUESTIONS AND HYPOTHESES
Research questions followed by hypotheses should be developed before the start of the study. 1 , 12 , 14 It is crucial to develop feasible research questions on a topic that is interesting to both the researcher and the scientific community. This can be achieved by a meticulous review of previous and current studies to establish a novel topic. Specific areas are subsequently focused on to generate ethical research questions. The relevance of the research questions is evaluated in terms of clarity of the resulting data, specificity of the methodology, objectivity of the outcome, depth of the research, and impact of the study. 1 , 5 These aspects constitute the FINER criteria (i.e., Feasible, Interesting, Novel, Ethical, and Relevant). 1 Clarity and effectiveness are achieved if research questions meet the FINER criteria. In addition to the FINER criteria, Ratan et al. described focus, complexity, novelty, feasibility, and measurability for evaluating the effectiveness of research questions. 14
The PICOT and PEO frameworks are also used when developing research questions. 1 The following elements are addressed in these frameworks, PICOT: P-population/patients/problem, I-intervention or indicator being studied, C-comparison group, O-outcome of interest, and T-timeframe of the study; PEO: P-population being studied, E-exposure to preexisting conditions, and O-outcome of interest. 1 Research questions are also considered good if these meet the “FINERMAPS” framework: Feasible, Interesting, Novel, Ethical, Relevant, Manageable, Appropriate, Potential value/publishable, and Systematic. 14
As we indicated earlier, research questions and hypotheses that are not carefully formulated result in unethical studies or poor outcomes. To illustrate this, we provide some examples of ambiguous research question and hypotheses that result in unclear and weak research objectives in quantitative research ( Table 6 ) 16 and qualitative research ( Table 7 ) 17 , and how to transform these ambiguous research question(s) and hypothesis(es) into clear and good statements.
a These statements were composed for comparison and illustrative purposes only.
b These statements are direct quotes from Higashihara and Horiuchi. 16
a This statement is a direct quote from Shimoda et al. 17
The other statements were composed for comparison and illustrative purposes only.
CONSTRUCTING RESEARCH QUESTIONS AND HYPOTHESES
To construct effective research questions and hypotheses, it is very important to 1) clarify the background and 2) identify the research problem at the outset of the research, within a specific timeframe. 9 Then, 3) review or conduct preliminary research to collect all available knowledge about the possible research questions by studying theories and previous studies. 18 Afterwards, 4) construct research questions to investigate the research problem. Identify variables to be accessed from the research questions 4 and make operational definitions of constructs from the research problem and questions. Thereafter, 5) construct specific deductive or inductive predictions in the form of hypotheses. 4 Finally, 6) state the study aims . This general flow for constructing effective research questions and hypotheses prior to conducting research is shown in Fig. 1 .

Research questions are used more frequently in qualitative research than objectives or hypotheses. 3 These questions seek to discover, understand, explore or describe experiences by asking “What” or “How.” The questions are open-ended to elicit a description rather than to relate variables or compare groups. The questions are continually reviewed, reformulated, and changed during the qualitative study. 3 Research questions are also used more frequently in survey projects than hypotheses in experiments in quantitative research to compare variables and their relationships.
Hypotheses are constructed based on the variables identified and as an if-then statement, following the template, ‘If a specific action is taken, then a certain outcome is expected.’ At this stage, some ideas regarding expectations from the research to be conducted must be drawn. 18 Then, the variables to be manipulated (independent) and influenced (dependent) are defined. 4 Thereafter, the hypothesis is stated and refined, and reproducible data tailored to the hypothesis are identified, collected, and analyzed. 4 The hypotheses must be testable and specific, 18 and should describe the variables and their relationships, the specific group being studied, and the predicted research outcome. 18 Hypotheses construction involves a testable proposition to be deduced from theory, and independent and dependent variables to be separated and measured separately. 3 Therefore, good hypotheses must be based on good research questions constructed at the start of a study or trial. 12
In summary, research questions are constructed after establishing the background of the study. Hypotheses are then developed based on the research questions. Thus, it is crucial to have excellent research questions to generate superior hypotheses. In turn, these would determine the research objectives and the design of the study, and ultimately, the outcome of the research. 12 Algorithms for building research questions and hypotheses are shown in Fig. 2 for quantitative research and in Fig. 3 for qualitative research.

EXAMPLES OF RESEARCH QUESTIONS FROM PUBLISHED ARTICLES
- EXAMPLE 1. Descriptive research question (quantitative research)
- - Presents research variables to be assessed (distinct phenotypes and subphenotypes)
- “BACKGROUND: Since COVID-19 was identified, its clinical and biological heterogeneity has been recognized. Identifying COVID-19 phenotypes might help guide basic, clinical, and translational research efforts.
- RESEARCH QUESTION: Does the clinical spectrum of patients with COVID-19 contain distinct phenotypes and subphenotypes? ” 19
- EXAMPLE 2. Relationship research question (quantitative research)
- - Shows interactions between dependent variable (static postural control) and independent variable (peripheral visual field loss)
- “Background: Integration of visual, vestibular, and proprioceptive sensations contributes to postural control. People with peripheral visual field loss have serious postural instability. However, the directional specificity of postural stability and sensory reweighting caused by gradual peripheral visual field loss remain unclear.
- Research question: What are the effects of peripheral visual field loss on static postural control ?” 20
- EXAMPLE 3. Comparative research question (quantitative research)
- - Clarifies the difference among groups with an outcome variable (patients enrolled in COMPERA with moderate PH or severe PH in COPD) and another group without the outcome variable (patients with idiopathic pulmonary arterial hypertension (IPAH))
- “BACKGROUND: Pulmonary hypertension (PH) in COPD is a poorly investigated clinical condition.
- RESEARCH QUESTION: Which factors determine the outcome of PH in COPD?
- STUDY DESIGN AND METHODS: We analyzed the characteristics and outcome of patients enrolled in the Comparative, Prospective Registry of Newly Initiated Therapies for Pulmonary Hypertension (COMPERA) with moderate or severe PH in COPD as defined during the 6th PH World Symposium who received medical therapy for PH and compared them with patients with idiopathic pulmonary arterial hypertension (IPAH) .” 21
- EXAMPLE 4. Exploratory research question (qualitative research)
- - Explores areas that have not been fully investigated (perspectives of families and children who receive care in clinic-based child obesity treatment) to have a deeper understanding of the research problem
- “Problem: Interventions for children with obesity lead to only modest improvements in BMI and long-term outcomes, and data are limited on the perspectives of families of children with obesity in clinic-based treatment. This scoping review seeks to answer the question: What is known about the perspectives of families and children who receive care in clinic-based child obesity treatment? This review aims to explore the scope of perspectives reported by families of children with obesity who have received individualized outpatient clinic-based obesity treatment.” 22
- EXAMPLE 5. Relationship research question (quantitative research)
- - Defines interactions between dependent variable (use of ankle strategies) and independent variable (changes in muscle tone)
- “Background: To maintain an upright standing posture against external disturbances, the human body mainly employs two types of postural control strategies: “ankle strategy” and “hip strategy.” While it has been reported that the magnitude of the disturbance alters the use of postural control strategies, it has not been elucidated how the level of muscle tone, one of the crucial parameters of bodily function, determines the use of each strategy. We have previously confirmed using forward dynamics simulations of human musculoskeletal models that an increased muscle tone promotes the use of ankle strategies. The objective of the present study was to experimentally evaluate a hypothesis: an increased muscle tone promotes the use of ankle strategies. Research question: Do changes in the muscle tone affect the use of ankle strategies ?” 23
EXAMPLES OF HYPOTHESES IN PUBLISHED ARTICLES
- EXAMPLE 1. Working hypothesis (quantitative research)
- - A hypothesis that is initially accepted for further research to produce a feasible theory
- “As fever may have benefit in shortening the duration of viral illness, it is plausible to hypothesize that the antipyretic efficacy of ibuprofen may be hindering the benefits of a fever response when taken during the early stages of COVID-19 illness .” 24
- “In conclusion, it is plausible to hypothesize that the antipyretic efficacy of ibuprofen may be hindering the benefits of a fever response . The difference in perceived safety of these agents in COVID-19 illness could be related to the more potent efficacy to reduce fever with ibuprofen compared to acetaminophen. Compelling data on the benefit of fever warrant further research and review to determine when to treat or withhold ibuprofen for early stage fever for COVID-19 and other related viral illnesses .” 24
- EXAMPLE 2. Exploratory hypothesis (qualitative research)
- - Explores particular areas deeper to clarify subjective experience and develop a formal hypothesis potentially testable in a future quantitative approach
- “We hypothesized that when thinking about a past experience of help-seeking, a self distancing prompt would cause increased help-seeking intentions and more favorable help-seeking outcome expectations .” 25
- “Conclusion
- Although a priori hypotheses were not supported, further research is warranted as results indicate the potential for using self-distancing approaches to increasing help-seeking among some people with depressive symptomatology.” 25
- EXAMPLE 3. Hypothesis-generating research to establish a framework for hypothesis testing (qualitative research)
- “We hypothesize that compassionate care is beneficial for patients (better outcomes), healthcare systems and payers (lower costs), and healthcare providers (lower burnout). ” 26
- Compassionomics is the branch of knowledge and scientific study of the effects of compassionate healthcare. Our main hypotheses are that compassionate healthcare is beneficial for (1) patients, by improving clinical outcomes, (2) healthcare systems and payers, by supporting financial sustainability, and (3) HCPs, by lowering burnout and promoting resilience and well-being. The purpose of this paper is to establish a scientific framework for testing the hypotheses above . If these hypotheses are confirmed through rigorous research, compassionomics will belong in the science of evidence-based medicine, with major implications for all healthcare domains.” 26
- EXAMPLE 4. Statistical hypothesis (quantitative research)
- - An assumption is made about the relationship among several population characteristics ( gender differences in sociodemographic and clinical characteristics of adults with ADHD ). Validity is tested by statistical experiment or analysis ( chi-square test, Students t-test, and logistic regression analysis)
- “Our research investigated gender differences in sociodemographic and clinical characteristics of adults with ADHD in a Japanese clinical sample. Due to unique Japanese cultural ideals and expectations of women's behavior that are in opposition to ADHD symptoms, we hypothesized that women with ADHD experience more difficulties and present more dysfunctions than men . We tested the following hypotheses: first, women with ADHD have more comorbidities than men with ADHD; second, women with ADHD experience more social hardships than men, such as having less full-time employment and being more likely to be divorced.” 27
- “Statistical Analysis
- ( text omitted ) Between-gender comparisons were made using the chi-squared test for categorical variables and Students t-test for continuous variables…( text omitted ). A logistic regression analysis was performed for employment status, marital status, and comorbidity to evaluate the independent effects of gender on these dependent variables.” 27
EXAMPLES OF HYPOTHESIS AS WRITTEN IN PUBLISHED ARTICLES IN RELATION TO OTHER PARTS
- EXAMPLE 1. Background, hypotheses, and aims are provided
- “Pregnant women need skilled care during pregnancy and childbirth, but that skilled care is often delayed in some countries …( text omitted ). The focused antenatal care (FANC) model of WHO recommends that nurses provide information or counseling to all pregnant women …( text omitted ). Job aids are visual support materials that provide the right kind of information using graphics and words in a simple and yet effective manner. When nurses are not highly trained or have many work details to attend to, these job aids can serve as a content reminder for the nurses and can be used for educating their patients (Jennings, Yebadokpo, Affo, & Agbogbe, 2010) ( text omitted ). Importantly, additional evidence is needed to confirm how job aids can further improve the quality of ANC counseling by health workers in maternal care …( text omitted )” 28
- “ This has led us to hypothesize that the quality of ANC counseling would be better if supported by job aids. Consequently, a better quality of ANC counseling is expected to produce higher levels of awareness concerning the danger signs of pregnancy and a more favorable impression of the caring behavior of nurses .” 28
- “This study aimed to examine the differences in the responses of pregnant women to a job aid-supported intervention during ANC visit in terms of 1) their understanding of the danger signs of pregnancy and 2) their impression of the caring behaviors of nurses to pregnant women in rural Tanzania.” 28
- EXAMPLE 2. Background, hypotheses, and aims are provided
- “We conducted a two-arm randomized controlled trial (RCT) to evaluate and compare changes in salivary cortisol and oxytocin levels of first-time pregnant women between experimental and control groups. The women in the experimental group touched and held an infant for 30 min (experimental intervention protocol), whereas those in the control group watched a DVD movie of an infant (control intervention protocol). The primary outcome was salivary cortisol level and the secondary outcome was salivary oxytocin level.” 29
- “ We hypothesize that at 30 min after touching and holding an infant, the salivary cortisol level will significantly decrease and the salivary oxytocin level will increase in the experimental group compared with the control group .” 29
- EXAMPLE 3. Background, aim, and hypothesis are provided
- “In countries where the maternal mortality ratio remains high, antenatal education to increase Birth Preparedness and Complication Readiness (BPCR) is considered one of the top priorities [1]. BPCR includes birth plans during the antenatal period, such as the birthplace, birth attendant, transportation, health facility for complications, expenses, and birth materials, as well as family coordination to achieve such birth plans. In Tanzania, although increasing, only about half of all pregnant women attend an antenatal clinic more than four times [4]. Moreover, the information provided during antenatal care (ANC) is insufficient. In the resource-poor settings, antenatal group education is a potential approach because of the limited time for individual counseling at antenatal clinics.” 30
- “This study aimed to evaluate an antenatal group education program among pregnant women and their families with respect to birth-preparedness and maternal and infant outcomes in rural villages of Tanzania.” 30
- “ The study hypothesis was if Tanzanian pregnant women and their families received a family-oriented antenatal group education, they would (1) have a higher level of BPCR, (2) attend antenatal clinic four or more times, (3) give birth in a health facility, (4) have less complications of women at birth, and (5) have less complications and deaths of infants than those who did not receive the education .” 30
Research questions and hypotheses are crucial components to any type of research, whether quantitative or qualitative. These questions should be developed at the very beginning of the study. Excellent research questions lead to superior hypotheses, which, like a compass, set the direction of research, and can often determine the successful conduct of the study. Many research studies have floundered because the development of research questions and subsequent hypotheses was not given the thought and meticulous attention needed. The development of research questions and hypotheses is an iterative process based on extensive knowledge of the literature and insightful grasp of the knowledge gap. Focused, concise, and specific research questions provide a strong foundation for constructing hypotheses which serve as formal predictions about the research outcomes. Research questions and hypotheses are crucial elements of research that should not be overlooked. They should be carefully thought of and constructed when planning research. This avoids unethical studies and poor outcomes by defining well-founded objectives that determine the design, course, and outcome of the study.
Disclosure: The authors have no potential conflicts of interest to disclose.
Author Contributions:
- Conceptualization: Barroga E, Matanguihan GJ.
- Methodology: Barroga E, Matanguihan GJ.
- Writing - original draft: Barroga E, Matanguihan GJ.
- Writing - review & editing: Barroga E, Matanguihan GJ.
Get science-backed answers as you write with Paperpal's Research feature
What is Research Methodology? Definition, Types, and Examples
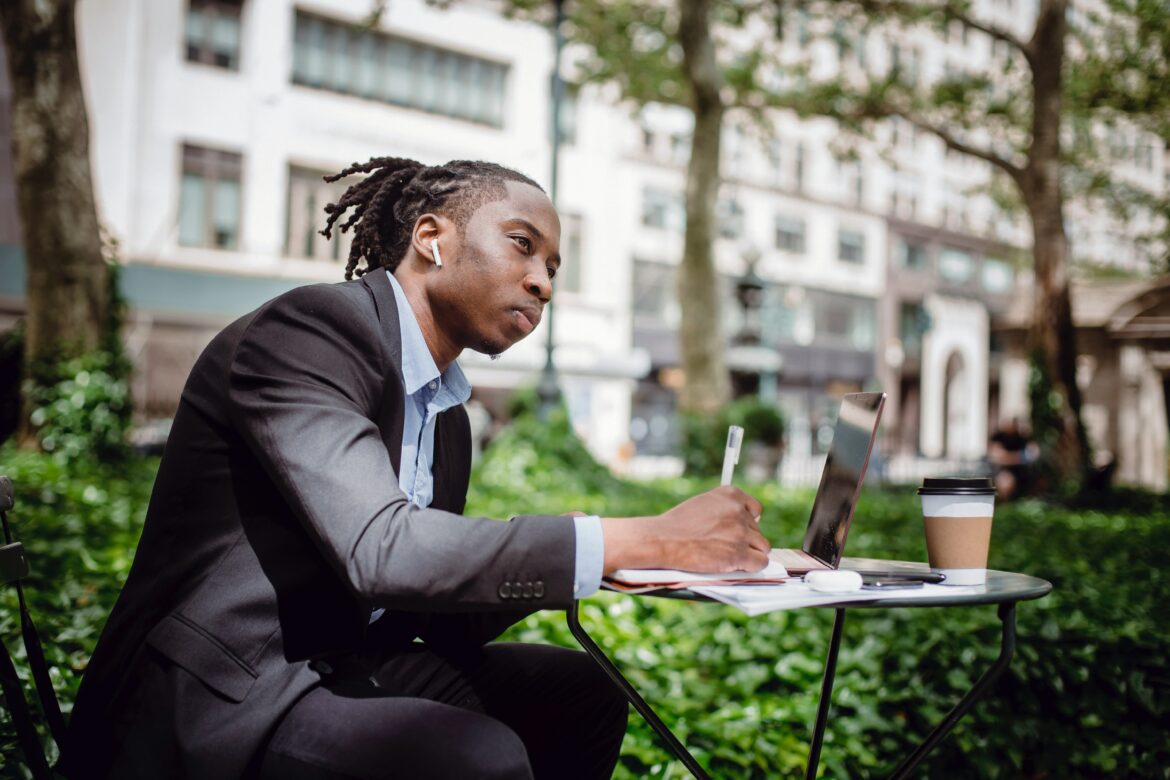
Research methodology 1,2 is a structured and scientific approach used to collect, analyze, and interpret quantitative or qualitative data to answer research questions or test hypotheses. A research methodology is like a plan for carrying out research and helps keep researchers on track by limiting the scope of the research. Several aspects must be considered before selecting an appropriate research methodology, such as research limitations and ethical concerns that may affect your research.
The research methodology section in a scientific paper describes the different methodological choices made, such as the data collection and analysis methods, and why these choices were selected. The reasons should explain why the methods chosen are the most appropriate to answer the research question. A good research methodology also helps ensure the reliability and validity of the research findings. There are three types of research methodology—quantitative, qualitative, and mixed-method, which can be chosen based on the research objectives.
What is research methodology ?
A research methodology describes the techniques and procedures used to identify and analyze information regarding a specific research topic. It is a process by which researchers design their study so that they can achieve their objectives using the selected research instruments. It includes all the important aspects of research, including research design, data collection methods, data analysis methods, and the overall framework within which the research is conducted. While these points can help you understand what is research methodology, you also need to know why it is important to pick the right methodology.
Why is research methodology important?
Having a good research methodology in place has the following advantages: 3
- Helps other researchers who may want to replicate your research; the explanations will be of benefit to them.
- You can easily answer any questions about your research if they arise at a later stage.
- A research methodology provides a framework and guidelines for researchers to clearly define research questions, hypotheses, and objectives.
- It helps researchers identify the most appropriate research design, sampling technique, and data collection and analysis methods.
- A sound research methodology helps researchers ensure that their findings are valid and reliable and free from biases and errors.
- It also helps ensure that ethical guidelines are followed while conducting research.
- A good research methodology helps researchers in planning their research efficiently, by ensuring optimum usage of their time and resources.
Writing the methods section of a research paper? Let Paperpal help you achieve perfection
Types of research methodology.
There are three types of research methodology based on the type of research and the data required. 1
- Quantitative research methodology focuses on measuring and testing numerical data. This approach is good for reaching a large number of people in a short amount of time. This type of research helps in testing the causal relationships between variables, making predictions, and generalizing results to wider populations.
- Qualitative research methodology examines the opinions, behaviors, and experiences of people. It collects and analyzes words and textual data. This research methodology requires fewer participants but is still more time consuming because the time spent per participant is quite large. This method is used in exploratory research where the research problem being investigated is not clearly defined.
- Mixed-method research methodology uses the characteristics of both quantitative and qualitative research methodologies in the same study. This method allows researchers to validate their findings, verify if the results observed using both methods are complementary, and explain any unexpected results obtained from one method by using the other method.
What are the types of sampling designs in research methodology?
Sampling 4 is an important part of a research methodology and involves selecting a representative sample of the population to conduct the study, making statistical inferences about them, and estimating the characteristics of the whole population based on these inferences. There are two types of sampling designs in research methodology—probability and nonprobability.
- Probability sampling
In this type of sampling design, a sample is chosen from a larger population using some form of random selection, that is, every member of the population has an equal chance of being selected. The different types of probability sampling are:
- Systematic —sample members are chosen at regular intervals. It requires selecting a starting point for the sample and sample size determination that can be repeated at regular intervals. This type of sampling method has a predefined range; hence, it is the least time consuming.
- Stratified —researchers divide the population into smaller groups that don’t overlap but represent the entire population. While sampling, these groups can be organized, and then a sample can be drawn from each group separately.
- Cluster —the population is divided into clusters based on demographic parameters like age, sex, location, etc.
- Convenience —selects participants who are most easily accessible to researchers due to geographical proximity, availability at a particular time, etc.
- Purposive —participants are selected at the researcher’s discretion. Researchers consider the purpose of the study and the understanding of the target audience.
- Snowball —already selected participants use their social networks to refer the researcher to other potential participants.
- Quota —while designing the study, the researchers decide how many people with which characteristics to include as participants. The characteristics help in choosing people most likely to provide insights into the subject.
What are data collection methods?
During research, data are collected using various methods depending on the research methodology being followed and the research methods being undertaken. Both qualitative and quantitative research have different data collection methods, as listed below.
Qualitative research 5
- One-on-one interviews: Helps the interviewers understand a respondent’s subjective opinion and experience pertaining to a specific topic or event
- Document study/literature review/record keeping: Researchers’ review of already existing written materials such as archives, annual reports, research articles, guidelines, policy documents, etc.
- Focus groups: Constructive discussions that usually include a small sample of about 6-10 people and a moderator, to understand the participants’ opinion on a given topic.
- Qualitative observation : Researchers collect data using their five senses (sight, smell, touch, taste, and hearing).
Quantitative research 6
- Sampling: The most common type is probability sampling.
- Interviews: Commonly telephonic or done in-person.
- Observations: Structured observations are most commonly used in quantitative research. In this method, researchers make observations about specific behaviors of individuals in a structured setting.
- Document review: Reviewing existing research or documents to collect evidence for supporting the research.
- Surveys and questionnaires. Surveys can be administered both online and offline depending on the requirement and sample size.
Let Paperpal help you write the perfect research methods section. Start now!
What are data analysis methods.
The data collected using the various methods for qualitative and quantitative research need to be analyzed to generate meaningful conclusions. These data analysis methods 7 also differ between quantitative and qualitative research.
Quantitative research involves a deductive method for data analysis where hypotheses are developed at the beginning of the research and precise measurement is required. The methods include statistical analysis applications to analyze numerical data and are grouped into two categories—descriptive and inferential.
Descriptive analysis is used to describe the basic features of different types of data to present it in a way that ensures the patterns become meaningful. The different types of descriptive analysis methods are:
- Measures of frequency (count, percent, frequency)
- Measures of central tendency (mean, median, mode)
- Measures of dispersion or variation (range, variance, standard deviation)
- Measure of position (percentile ranks, quartile ranks)
Inferential analysis is used to make predictions about a larger population based on the analysis of the data collected from a smaller population. This analysis is used to study the relationships between different variables. Some commonly used inferential data analysis methods are:
- Correlation: To understand the relationship between two or more variables.
- Cross-tabulation: Analyze the relationship between multiple variables.
- Regression analysis: Study the impact of independent variables on the dependent variable.
- Frequency tables: To understand the frequency of data.
- Analysis of variance: To test the degree to which two or more variables differ in an experiment.
Qualitative research involves an inductive method for data analysis where hypotheses are developed after data collection. The methods include:
- Content analysis: For analyzing documented information from text and images by determining the presence of certain words or concepts in texts.
- Narrative analysis: For analyzing content obtained from sources such as interviews, field observations, and surveys. The stories and opinions shared by people are used to answer research questions.
- Discourse analysis: For analyzing interactions with people considering the social context, that is, the lifestyle and environment, under which the interaction occurs.
- Grounded theory: Involves hypothesis creation by data collection and analysis to explain why a phenomenon occurred.
- Thematic analysis: To identify important themes or patterns in data and use these to address an issue.
How to choose a research methodology?
Here are some important factors to consider when choosing a research methodology: 8
- Research objectives, aims, and questions —these would help structure the research design.
- Review existing literature to identify any gaps in knowledge.
- Check the statistical requirements —if data-driven or statistical results are needed then quantitative research is the best. If the research questions can be answered based on people’s opinions and perceptions, then qualitative research is most suitable.
- Sample size —sample size can often determine the feasibility of a research methodology. For a large sample, less effort- and time-intensive methods are appropriate.
- Constraints —constraints of time, geography, and resources can help define the appropriate methodology.

Got writer’s block? Kickstart your research paper writing with Paperpal now!
How to write a research methodology .
A research methodology should include the following components: 3,9
- Research design —should be selected based on the research question and the data required. Common research designs include experimental, quasi-experimental, correlational, descriptive, and exploratory.
- Research method —this can be quantitative, qualitative, or mixed-method.
- Reason for selecting a specific methodology —explain why this methodology is the most suitable to answer your research problem.
- Research instruments —explain the research instruments you plan to use, mainly referring to the data collection methods such as interviews, surveys, etc. Here as well, a reason should be mentioned for selecting the particular instrument.
- Sampling —this involves selecting a representative subset of the population being studied.
- Data collection —involves gathering data using several data collection methods, such as surveys, interviews, etc.
- Data analysis —describe the data analysis methods you will use once you’ve collected the data.
- Research limitations —mention any limitations you foresee while conducting your research.
- Validity and reliability —validity helps identify the accuracy and truthfulness of the findings; reliability refers to the consistency and stability of the results over time and across different conditions.
- Ethical considerations —research should be conducted ethically. The considerations include obtaining consent from participants, maintaining confidentiality, and addressing conflicts of interest.
Streamline Your Research Paper Writing Process with Paperpal
The methods section is a critical part of the research papers, allowing researchers to use this to understand your findings and replicate your work when pursuing their own research. However, it is usually also the most difficult section to write. This is where Paperpal can help you overcome the writer’s block and create the first draft in minutes with Paperpal Copilot, its secure generative AI feature suite.
With Paperpal you can get research advice, write and refine your work, rephrase and verify the writing, and ensure submission readiness, all in one place. Here’s how you can use Paperpal to develop the first draft of your methods section.
- Generate an outline: Input some details about your research to instantly generate an outline for your methods section
- Develop the section: Use the outline and suggested sentence templates to expand your ideas and develop the first draft.
- P araph ras e and trim : Get clear, concise academic text with paraphrasing that conveys your work effectively and word reduction to fix redundancies.
- Choose the right words: Enhance text by choosing contextual synonyms based on how the words have been used in previously published work.
- Check and verify text : Make sure the generated text showcases your methods correctly, has all the right citations, and is original and authentic. .
You can repeat this process to develop each section of your research manuscript, including the title, abstract and keywords. Ready to write your research papers faster, better, and without the stress? Sign up for Paperpal and start writing today!
Frequently Asked Questions
Q1. What are the key components of research methodology?
A1. A good research methodology has the following key components:
- Research design
- Data collection procedures
- Data analysis methods
- Ethical considerations
Q2. Why is ethical consideration important in research methodology?
A2. Ethical consideration is important in research methodology to ensure the readers of the reliability and validity of the study. Researchers must clearly mention the ethical norms and standards followed during the conduct of the research and also mention if the research has been cleared by any institutional board. The following 10 points are the important principles related to ethical considerations: 10
- Participants should not be subjected to harm.
- Respect for the dignity of participants should be prioritized.
- Full consent should be obtained from participants before the study.
- Participants’ privacy should be ensured.
- Confidentiality of the research data should be ensured.
- Anonymity of individuals and organizations participating in the research should be maintained.
- The aims and objectives of the research should not be exaggerated.
- Affiliations, sources of funding, and any possible conflicts of interest should be declared.
- Communication in relation to the research should be honest and transparent.
- Misleading information and biased representation of primary data findings should be avoided.
Q3. What is the difference between methodology and method?
A3. Research methodology is different from a research method, although both terms are often confused. Research methods are the tools used to gather data, while the research methodology provides a framework for how research is planned, conducted, and analyzed. The latter guides researchers in making decisions about the most appropriate methods for their research. Research methods refer to the specific techniques, procedures, and tools used by researchers to collect, analyze, and interpret data, for instance surveys, questionnaires, interviews, etc.
Research methodology is, thus, an integral part of a research study. It helps ensure that you stay on track to meet your research objectives and answer your research questions using the most appropriate data collection and analysis tools based on your research design.
Accelerate your research paper writing with Paperpal. Try for free now!
- Research methodologies. Pfeiffer Library website. Accessed August 15, 2023. https://library.tiffin.edu/researchmethodologies/whatareresearchmethodologies
- Types of research methodology. Eduvoice website. Accessed August 16, 2023. https://eduvoice.in/types-research-methodology/
- The basics of research methodology: A key to quality research. Voxco. Accessed August 16, 2023. https://www.voxco.com/blog/what-is-research-methodology/
- Sampling methods: Types with examples. QuestionPro website. Accessed August 16, 2023. https://www.questionpro.com/blog/types-of-sampling-for-social-research/
- What is qualitative research? Methods, types, approaches, examples. Researcher.Life blog. Accessed August 15, 2023. https://researcher.life/blog/article/what-is-qualitative-research-methods-types-examples/
- What is quantitative research? Definition, methods, types, and examples. Researcher.Life blog. Accessed August 15, 2023. https://researcher.life/blog/article/what-is-quantitative-research-types-and-examples/
- Data analysis in research: Types & methods. QuestionPro website. Accessed August 16, 2023. https://www.questionpro.com/blog/data-analysis-in-research/#Data_analysis_in_qualitative_research
- Factors to consider while choosing the right research methodology. PhD Monster website. Accessed August 17, 2023. https://www.phdmonster.com/factors-to-consider-while-choosing-the-right-research-methodology/
- What is research methodology? Research and writing guides. Accessed August 14, 2023. https://paperpile.com/g/what-is-research-methodology/
- Ethical considerations. Business research methodology website. Accessed August 17, 2023. https://research-methodology.net/research-methodology/ethical-considerations/
Paperpal is a comprehensive AI writing toolkit that helps students and researchers achieve 2x the writing in half the time. It leverages 21+ years of STM experience and insights from millions of research articles to provide in-depth academic writing, language editing, and submission readiness support to help you write better, faster.
Get accurate academic translations, rewriting support, grammar checks, vocabulary suggestions, and generative AI assistance that delivers human precision at machine speed. Try for free or upgrade to Paperpal Prime starting at US$19 a month to access premium features, including consistency, plagiarism, and 30+ submission readiness checks to help you succeed.
Experience the future of academic writing – Sign up to Paperpal and start writing for free!
Related Reads:
- Dangling Modifiers and How to Avoid Them in Your Writing
- Webinar: How to Use Generative AI Tools Ethically in Your Academic Writing
- Research Outlines: How to Write An Introduction Section in Minutes with Paperpal Copilot
- How to Paraphrase Research Papers Effectively
Language and Grammar Rules for Academic Writing
Climatic vs. climactic: difference and examples, you may also like, what is academic writing: tips for students, what is hedging in academic writing , how to use ai to enhance your college..., how to use paperpal to generate emails &..., ai in education: it’s time to change the..., is it ethical to use ai-generated abstracts without..., do plagiarism checkers detect ai content, word choice problems: how to use the right..., how to avoid plagiarism when using generative ai..., what are journal guidelines on using generative ai....
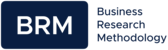
Regression Analysis
Regression analysis is a quantitative research method which is used when the study involves modelling and analysing several variables, where the relationship includes a dependent variable and one or more independent variables. In simple terms, regression analysis is a quantitative method used to test the nature of relationships between a dependent variable and one or more independent variables.
The basic form of regression models includes unknown parameters (β), independent variables (X), and the dependent variable (Y).
Regression model, basically, specifies the relation of dependent variable (Y) to a function combination of independent variables (X) and unknown parameters (β)
Y ≈ f (X, β)
Regression equation can be used to predict the values of ‘y’, if the value of ‘x’ is given, and both ‘y’ and ‘x’ are the two sets of measures of a sample size of ‘n’. The formulae for regression equation would be
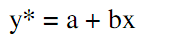
Do not be intimidated by visual complexity of correlation and regression formulae above. You don’t have to apply the formula manually, and correlation and regression analyses can be run with the application of popular analytical software such as Microsoft Excel, Microsoft Access, SPSS and others.
Linear regression analysis is based on the following set of assumptions:
1. Assumption of linearity . There is a linear relationship between dependent and independent variables.
2. Assumption of homoscedasticity . Data values for dependent and independent variables have equal variances.
3. Assumption of absence of collinearity or multicollinearity . There is no correlation between two or more independent variables.
4. Assumption of normal distribution . The data for the independent variables and dependent variable are normally distributed
My e-book, The Ultimate Guide to Writing a Dissertation in Business Studies: a step by step assistance offers practical assistance to complete a dissertation with minimum or no stress. The e-book covers all stages of writing a dissertation starting from the selection to the research area to submitting the completed version of the work within the deadline. John Dudovskiy
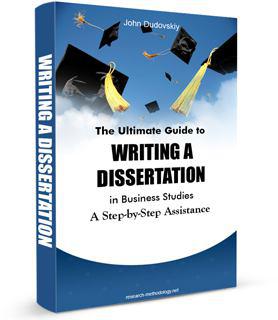
- Privacy Policy

Home » Quantitative Research – Methods, Types and Analysis
Quantitative Research – Methods, Types and Analysis
Table of Contents

Quantitative Research
Quantitative research is a type of research that collects and analyzes numerical data to test hypotheses and answer research questions . This research typically involves a large sample size and uses statistical analysis to make inferences about a population based on the data collected. It often involves the use of surveys, experiments, or other structured data collection methods to gather quantitative data.
Quantitative Research Methods

Quantitative Research Methods are as follows:
Descriptive Research Design
Descriptive research design is used to describe the characteristics of a population or phenomenon being studied. This research method is used to answer the questions of what, where, when, and how. Descriptive research designs use a variety of methods such as observation, case studies, and surveys to collect data. The data is then analyzed using statistical tools to identify patterns and relationships.
Correlational Research Design
Correlational research design is used to investigate the relationship between two or more variables. Researchers use correlational research to determine whether a relationship exists between variables and to what extent they are related. This research method involves collecting data from a sample and analyzing it using statistical tools such as correlation coefficients.
Quasi-experimental Research Design
Quasi-experimental research design is used to investigate cause-and-effect relationships between variables. This research method is similar to experimental research design, but it lacks full control over the independent variable. Researchers use quasi-experimental research designs when it is not feasible or ethical to manipulate the independent variable.
Experimental Research Design
Experimental research design is used to investigate cause-and-effect relationships between variables. This research method involves manipulating the independent variable and observing the effects on the dependent variable. Researchers use experimental research designs to test hypotheses and establish cause-and-effect relationships.
Survey Research
Survey research involves collecting data from a sample of individuals using a standardized questionnaire. This research method is used to gather information on attitudes, beliefs, and behaviors of individuals. Researchers use survey research to collect data quickly and efficiently from a large sample size. Survey research can be conducted through various methods such as online, phone, mail, or in-person interviews.
Quantitative Research Analysis Methods
Here are some commonly used quantitative research analysis methods:
Statistical Analysis
Statistical analysis is the most common quantitative research analysis method. It involves using statistical tools and techniques to analyze the numerical data collected during the research process. Statistical analysis can be used to identify patterns, trends, and relationships between variables, and to test hypotheses and theories.
Regression Analysis
Regression analysis is a statistical technique used to analyze the relationship between one dependent variable and one or more independent variables. Researchers use regression analysis to identify and quantify the impact of independent variables on the dependent variable.
Factor Analysis
Factor analysis is a statistical technique used to identify underlying factors that explain the correlations among a set of variables. Researchers use factor analysis to reduce a large number of variables to a smaller set of factors that capture the most important information.
Structural Equation Modeling
Structural equation modeling is a statistical technique used to test complex relationships between variables. It involves specifying a model that includes both observed and unobserved variables, and then using statistical methods to test the fit of the model to the data.
Time Series Analysis
Time series analysis is a statistical technique used to analyze data that is collected over time. It involves identifying patterns and trends in the data, as well as any seasonal or cyclical variations.
Multilevel Modeling
Multilevel modeling is a statistical technique used to analyze data that is nested within multiple levels. For example, researchers might use multilevel modeling to analyze data that is collected from individuals who are nested within groups, such as students nested within schools.
Applications of Quantitative Research
Quantitative research has many applications across a wide range of fields. Here are some common examples:
- Market Research : Quantitative research is used extensively in market research to understand consumer behavior, preferences, and trends. Researchers use surveys, experiments, and other quantitative methods to collect data that can inform marketing strategies, product development, and pricing decisions.
- Health Research: Quantitative research is used in health research to study the effectiveness of medical treatments, identify risk factors for diseases, and track health outcomes over time. Researchers use statistical methods to analyze data from clinical trials, surveys, and other sources to inform medical practice and policy.
- Social Science Research: Quantitative research is used in social science research to study human behavior, attitudes, and social structures. Researchers use surveys, experiments, and other quantitative methods to collect data that can inform social policies, educational programs, and community interventions.
- Education Research: Quantitative research is used in education research to study the effectiveness of teaching methods, assess student learning outcomes, and identify factors that influence student success. Researchers use experimental and quasi-experimental designs, as well as surveys and other quantitative methods, to collect and analyze data.
- Environmental Research: Quantitative research is used in environmental research to study the impact of human activities on the environment, assess the effectiveness of conservation strategies, and identify ways to reduce environmental risks. Researchers use statistical methods to analyze data from field studies, experiments, and other sources.
Characteristics of Quantitative Research
Here are some key characteristics of quantitative research:
- Numerical data : Quantitative research involves collecting numerical data through standardized methods such as surveys, experiments, and observational studies. This data is analyzed using statistical methods to identify patterns and relationships.
- Large sample size: Quantitative research often involves collecting data from a large sample of individuals or groups in order to increase the reliability and generalizability of the findings.
- Objective approach: Quantitative research aims to be objective and impartial in its approach, focusing on the collection and analysis of data rather than personal beliefs, opinions, or experiences.
- Control over variables: Quantitative research often involves manipulating variables to test hypotheses and establish cause-and-effect relationships. Researchers aim to control for extraneous variables that may impact the results.
- Replicable : Quantitative research aims to be replicable, meaning that other researchers should be able to conduct similar studies and obtain similar results using the same methods.
- Statistical analysis: Quantitative research involves using statistical tools and techniques to analyze the numerical data collected during the research process. Statistical analysis allows researchers to identify patterns, trends, and relationships between variables, and to test hypotheses and theories.
- Generalizability: Quantitative research aims to produce findings that can be generalized to larger populations beyond the specific sample studied. This is achieved through the use of random sampling methods and statistical inference.
Examples of Quantitative Research
Here are some examples of quantitative research in different fields:
- Market Research: A company conducts a survey of 1000 consumers to determine their brand awareness and preferences. The data is analyzed using statistical methods to identify trends and patterns that can inform marketing strategies.
- Health Research : A researcher conducts a randomized controlled trial to test the effectiveness of a new drug for treating a particular medical condition. The study involves collecting data from a large sample of patients and analyzing the results using statistical methods.
- Social Science Research : A sociologist conducts a survey of 500 people to study attitudes toward immigration in a particular country. The data is analyzed using statistical methods to identify factors that influence these attitudes.
- Education Research: A researcher conducts an experiment to compare the effectiveness of two different teaching methods for improving student learning outcomes. The study involves randomly assigning students to different groups and collecting data on their performance on standardized tests.
- Environmental Research : A team of researchers conduct a study to investigate the impact of climate change on the distribution and abundance of a particular species of plant or animal. The study involves collecting data on environmental factors and population sizes over time and analyzing the results using statistical methods.
- Psychology : A researcher conducts a survey of 500 college students to investigate the relationship between social media use and mental health. The data is analyzed using statistical methods to identify correlations and potential causal relationships.
- Political Science: A team of researchers conducts a study to investigate voter behavior during an election. They use survey methods to collect data on voting patterns, demographics, and political attitudes, and analyze the results using statistical methods.
How to Conduct Quantitative Research
Here is a general overview of how to conduct quantitative research:
- Develop a research question: The first step in conducting quantitative research is to develop a clear and specific research question. This question should be based on a gap in existing knowledge, and should be answerable using quantitative methods.
- Develop a research design: Once you have a research question, you will need to develop a research design. This involves deciding on the appropriate methods to collect data, such as surveys, experiments, or observational studies. You will also need to determine the appropriate sample size, data collection instruments, and data analysis techniques.
- Collect data: The next step is to collect data. This may involve administering surveys or questionnaires, conducting experiments, or gathering data from existing sources. It is important to use standardized methods to ensure that the data is reliable and valid.
- Analyze data : Once the data has been collected, it is time to analyze it. This involves using statistical methods to identify patterns, trends, and relationships between variables. Common statistical techniques include correlation analysis, regression analysis, and hypothesis testing.
- Interpret results: After analyzing the data, you will need to interpret the results. This involves identifying the key findings, determining their significance, and drawing conclusions based on the data.
- Communicate findings: Finally, you will need to communicate your findings. This may involve writing a research report, presenting at a conference, or publishing in a peer-reviewed journal. It is important to clearly communicate the research question, methods, results, and conclusions to ensure that others can understand and replicate your research.
When to use Quantitative Research
Here are some situations when quantitative research can be appropriate:
- To test a hypothesis: Quantitative research is often used to test a hypothesis or a theory. It involves collecting numerical data and using statistical analysis to determine if the data supports or refutes the hypothesis.
- To generalize findings: If you want to generalize the findings of your study to a larger population, quantitative research can be useful. This is because it allows you to collect numerical data from a representative sample of the population and use statistical analysis to make inferences about the population as a whole.
- To measure relationships between variables: If you want to measure the relationship between two or more variables, such as the relationship between age and income, or between education level and job satisfaction, quantitative research can be useful. It allows you to collect numerical data on both variables and use statistical analysis to determine the strength and direction of the relationship.
- To identify patterns or trends: Quantitative research can be useful for identifying patterns or trends in data. For example, you can use quantitative research to identify trends in consumer behavior or to identify patterns in stock market data.
- To quantify attitudes or opinions : If you want to measure attitudes or opinions on a particular topic, quantitative research can be useful. It allows you to collect numerical data using surveys or questionnaires and analyze the data using statistical methods to determine the prevalence of certain attitudes or opinions.
Purpose of Quantitative Research
The purpose of quantitative research is to systematically investigate and measure the relationships between variables or phenomena using numerical data and statistical analysis. The main objectives of quantitative research include:
- Description : To provide a detailed and accurate description of a particular phenomenon or population.
- Explanation : To explain the reasons for the occurrence of a particular phenomenon, such as identifying the factors that influence a behavior or attitude.
- Prediction : To predict future trends or behaviors based on past patterns and relationships between variables.
- Control : To identify the best strategies for controlling or influencing a particular outcome or behavior.
Quantitative research is used in many different fields, including social sciences, business, engineering, and health sciences. It can be used to investigate a wide range of phenomena, from human behavior and attitudes to physical and biological processes. The purpose of quantitative research is to provide reliable and valid data that can be used to inform decision-making and improve understanding of the world around us.
Advantages of Quantitative Research
There are several advantages of quantitative research, including:
- Objectivity : Quantitative research is based on objective data and statistical analysis, which reduces the potential for bias or subjectivity in the research process.
- Reproducibility : Because quantitative research involves standardized methods and measurements, it is more likely to be reproducible and reliable.
- Generalizability : Quantitative research allows for generalizations to be made about a population based on a representative sample, which can inform decision-making and policy development.
- Precision : Quantitative research allows for precise measurement and analysis of data, which can provide a more accurate understanding of phenomena and relationships between variables.
- Efficiency : Quantitative research can be conducted relatively quickly and efficiently, especially when compared to qualitative research, which may involve lengthy data collection and analysis.
- Large sample sizes : Quantitative research can accommodate large sample sizes, which can increase the representativeness and generalizability of the results.
Limitations of Quantitative Research
There are several limitations of quantitative research, including:
- Limited understanding of context: Quantitative research typically focuses on numerical data and statistical analysis, which may not provide a comprehensive understanding of the context or underlying factors that influence a phenomenon.
- Simplification of complex phenomena: Quantitative research often involves simplifying complex phenomena into measurable variables, which may not capture the full complexity of the phenomenon being studied.
- Potential for researcher bias: Although quantitative research aims to be objective, there is still the potential for researcher bias in areas such as sampling, data collection, and data analysis.
- Limited ability to explore new ideas: Quantitative research is often based on pre-determined research questions and hypotheses, which may limit the ability to explore new ideas or unexpected findings.
- Limited ability to capture subjective experiences : Quantitative research is typically focused on objective data and may not capture the subjective experiences of individuals or groups being studied.
- Ethical concerns : Quantitative research may raise ethical concerns, such as invasion of privacy or the potential for harm to participants.
About the author
Muhammad Hassan
Researcher, Academic Writer, Web developer
You may also like

Questionnaire – Definition, Types, and Examples

Case Study – Methods, Examples and Guide

Observational Research – Methods and Guide

Qualitative Research Methods

Explanatory Research – Types, Methods, Guide

Survey Research – Types, Methods, Examples
Numbers, Facts and Trends Shaping Your World
Read our research on:
Full Topic List
Regions & Countries
- Publications
- Our Methods
- Short Reads
- Tools & Resources
Read Our Research On:
U.S. Survey Methodology
A typical Pew Research Center national survey – regardless of mode – is designed and implemented with a total survey error approach in mind, aimed at minimizing coverage error (deviations of frame population from target population), sampling error (deviation of sample from sampling frame), nonresponse error (respondents’ deviation from sample), measurement error (deviations of responses from underlying measurements/attributes), and processing and adjustment error (error introduced post data collection but prior to data analysis).
Since 2014, Pew Research Center has conducted surveys online in the United States using our American Trends Panel (ATP), a randomly selected, probability-based sample of U.S. adults ages 18 and older. The panel was initially built to supplement the prevalent mode of data collection at the Center during that time: random-digit-dial (RDD) telephone surveys. However, at this point, the Center has switched almost completely to conducting its U.S. surveys online using the ATP.
Panel members are recruited offline, and survey questionnaires are taken via self-administered online surveys. Those who don’t have internet access can take our surveys on internet-enabled tablets we provide to them. Panelists typically take one to three surveys each month.
Most of our surveys are representative of the entire noninstitutionalized adult population of the United States. We do not exclude anyone from our analyses based on demographic characteristics. Some surveys are conducted among subgroups in the panel, such as Black Americans or young people, and may include a supplement sample (called “ oversamples ”) from another panel such as Ipsos’ KnowledgePanel. Pew Research Center also conducts international surveys that involve sampling and interviewing people in multiple countries.
On occasion, Center researchers conduct surveys with special populations, such as topic experts (e.g., technologists ) or members of a certain profession (e.g., journalists ). The principles are the same whether the sample is of the general population or some other group. Decisions must be made about the size of the sample and the level of precision desired so that the survey can provide accurate estimates for the population of interest and any subgroups within the population that will be analyzed. Some special challenges arise when sampling these populations. In particular, it may be difficult to find a sampling frame or list for the population of interest, and this may influence how the population is defined. In addition, information may be available for only some methods of contacting potential respondents (e.g., email addresses but not phone numbers) and may vary for people within the sample. If most members in the population of interest have internet access, and email addresses are available for contacting them, the web often provides a convenient and inexpensive way to survey experts or other industry-based populations.
Creating good measures requires asking unambiguous and unbiased questions . There are several steps involved in developing a survey questionnaire . The first is identifying what topics will be covered in the survey. For Pew Research Center surveys, this involves thinking about what is happening in our nation and the world and what will be relevant to the public, policymakers and the media. We also track opinion on a variety of issues over time, so we often ensure that we update these trends on a regular basis so we can understand whether people’s opinions are changing.
Each Pew Research Center survey report includes a “topline questionnaire” with all of the questions from that survey with the exact question wording and response options as they were read to respondents. This topline provides the results from the current survey for each question, as well as results from previous surveys in which the same questions were asked. For most studies, it is our policy to release ATP datasets within twelve months of data collection. Please visit our datasets page for further information.
U.S. Surveys
Other research methods, sign up for our weekly newsletter.
Fresh data delivered Saturday mornings
1615 L St. NW, Suite 800 Washington, DC 20036 USA (+1) 202-419-4300 | Main (+1) 202-857-8562 | Fax (+1) 202-419-4372 | Media Inquiries
Research Topics
- Age & Generations
- Coronavirus (COVID-19)
- Economy & Work
- Family & Relationships
- Gender & LGBTQ
- Immigration & Migration
- International Affairs
- Internet & Technology
- Methodological Research
- News Habits & Media
- Non-U.S. Governments
- Other Topics
- Politics & Policy
- Race & Ethnicity
- Email Newsletters
ABOUT PEW RESEARCH CENTER Pew Research Center is a nonpartisan fact tank that informs the public about the issues, attitudes and trends shaping the world. It conducts public opinion polling, demographic research, media content analysis and other empirical social science research. Pew Research Center does not take policy positions. It is a subsidiary of The Pew Charitable Trusts .
Copyright 2024 Pew Research Center
Terms & Conditions
Privacy Policy
Cookie Settings
Reprints, Permissions & Use Policy
- Open access
- Published: 27 April 2024
Ascertaining the Francophone population in Ontario: validating the language variable in health data
- Ricardo Batista 1 , 2 , 3 , 10 ,
- Amy T. Hsu 2 , 3 , 5 ,
- Louise Bouchard 1 , 6 ,
- Michael Reaume 7 ,
- Emily Rhodes 3 ,
- Ewa Sucha 2 ,
- Eva Guerin 1 ,
- Denis Prud’homme 1 , 4 ,
- Douglas G. Manuel 2 , 3 , 8 , 9 &
- Peter Tanuseputro 2 , 3 , 8
BMC Medical Research Methodology volume 24 , Article number: 98 ( 2024 ) Cite this article
Metrics details
Language barriers can impact health care and outcomes. Valid and reliable language data is central to studying health inequalities in linguistic minorities. In Canada, language variables are available in administrative health databases; however, the validity of these variables has not been studied. This study assessed concordance between language variables from administrative health databases and language variables from the Canadian Community Health Survey (CCHS) to identify Francophones in Ontario.
An Ontario combined sample of CCHS cycles from 2000 to 2012 (from participants who consented to link their data) was individually linked to three administrative databases (home care, long-term care [LTC], and mental health admissions). In total, 27,111 respondents had at least one encounter in one of the three databases. Language spoken at home (LOSH) and first official language spoken (FOLS) from CCHS were used as reference standards to assess their concordance with the language variables in administrative health databases, using the Cohen kappa, sensitivity, specificity, positive predictive value (PPV), and negative predictive values (NPV).
Language variables from home care and LTC databases had the highest agreement with LOSH (kappa = 0.76 [95%CI, 0.735–0.793] and 0.75 [95%CI, 0.70–0.80], respectively) and FOLS (kappa = 0.66 for both). Sensitivity was higher with LOSH as the reference standard (75.5% [95%CI, 71.6–79.0] and 74.2% [95%CI, 67.3–80.1] for home care and LTC, respectively). With FOLS as the reference standard, the language variables in both data sources had modest sensitivity (53.1% [95%CI, 49.8–56.4] and 54.1% [95%CI, 48.3–59.7] in home care and LTC, respectively) but very high specificity (99.8% [95%CI, 99.7–99.9] and 99.6% [95%CI, 99.4–99.8]) and predictive values. The language variable from mental health admissions had poor agreement with all language variables in the CCHS.
Conclusions
Language variables in home care and LTC health databases were most consistent with the language often spoken at home. Studies using language variables from administrative data can use the sensitivity and specificity reported from this study to gauge the level of mis-ascertainment error and the resulting bias.
Peer Review reports
Introduction
In recent years, studies have provided evidence for the existence of health disparities across linguistic groups in Canada [ 1 , 2 ]. However, most studies relied on census and survey data to examine the disparities by language characteristics [ 3 , 4 , 5 ]. Administrative health databases are widely used to assess health and health care disparities; but the availability and quality of the language information is a barrier to performing health research on linguistic groups in Canada [ 6 , 7 ]. Methodological challenges have hindered further research on the relationship between linguistic factors and health outcomes. Quality issues derived from collection methods, type of language recorded, and access to data prevent researchers from further exploring how linguistic factors are impacting health care and outcomes [ 8 , 9 , 10 ]. Some studies have used language variables collected in healthcare databases; however, since their validity has never been formally assessed, the use of these variables has been limited and has generated conflicting results [ 8 , 9 , 11 ].
Language variables and linguistic groups
Linguistic groups are usually defined through language variables, either by a single variable that represents a simple linguistic concept (e.g., mother tongue, language most often spoken at home [LOSH], language of preference, etc.) or a combination of multiple variables (e.g., First Official Language Spoken [FOLS], which is derived from the Mother Tongue, Knowledge of Canadian Official Languages and LOSH) [ 12 ]. Many of these language variables are routinely collected in the census and. Canadian Community Health Survey [CCHS]) in Canada and less often in administrative health databases.
Mother tongue, LOSH and more increasingly FOLS, are the language variables most commonly used in Canada to define and describe the characteristics of linguistic groups and to conduct comparative analyses in many studies, including those focusing on healthcare [ 9 , 12 , 13 , 14 , 15 ]. FOLS, which is defined within the framework of the Official Languages Act and represents a combination of several language variables, is increasingly being used in analyses and reports by Statistics Canada [ 14 , 16 , 17 ]. FOLS is valuable for research purposes because it establishes linguistic groups denoting Canada’s two official languages (English and French) while also including persons whose mother tongue is neither English nor French but who use one or both of these languages on a regular basis. Francophones are a linguistic minority outside Quebec. In Ontario, francophones make up about 4% of the population and research shows that francophone Ontarians face important health inequalities [ 5 , 18 , 19 ], but most of the analyses use survey data and only a few studies have used health data to identify the linguistic groups [ 11 , 20 , 21 , 22 ]. However, no previous study has examined the validity of the language information in administrative health data. Thus, we used several health databases from Ontario to assess its validity to identify francophones in health research.
This study sought to determine the ability to ascertain Francophones in Ontario using administrative health databases. Specifically, we assessed measures validity derived from language variables in administrative health databases to identify francophones, against a national survey standard, the CCHS, and determined the language concept captured by these variables.
The study used a data linkage of Ontario combined samples of the CCHS cycles 1.1 (2000–2001) to 2012 that were securely linked to three administrative health databases using anonymized and unique encoded identifiers and analyzed in a secure environment at ICES ( https://www.ices.on.ca/ ; formerly Institute for Clinical Evaluative Sciences).
Data sources
The study population included Ontario respondents to the CCHS cycle 1.1 (2000–2001) to 2012 cycle, 20 years and older who: (1) agreed to have their survey responses shared with the provinces and linked to their health care data (approximately 85% of participants) and (2) were eligible for Ontario’s universal health insurance plan (OHIP). The CCHS is a cross-sectional national representative survey that collects information related to health status, health care utilization and health determinants of the Canadian population aged 12 years or older living in private dwellings in all provinces and territories. To the best of our knowledge, there are no systematic differences between participants in CCHS who provided consent to link their data and those who did not.
Thus, for creating the study dataset, the CCHS samples for Ontario (cycle 1.1 [2000–2001], cycle 2.1 [2003], cycle 3.1 [2005], cycle 4.1 [2007], 2009–2010 and 2011–2012) were combined. Then, the CCHS combined dataset was linked to three health databases that contain language information: the Continuing Care Reporting System (CCRS), which collects population-based resident information of patients receiving 24-hour nursing care in publicly funded residential long-term care; the Home Care Reporting System (HCRS), which comprises data using the Resident Assessment Instrument-Home Care (RAI-HC), which collects information on adults expected to receive home care services for at least six months; and the Ontario Mental Health Reporting System (OMHRS), which collects data on patients admitted to inpatient mental health services. Eligible participants were identified using OHIP and Registered Persons Database (RPDB) and were linked over the same period covered by the survey. Each dataset used in the study is described in Appendix 1 .
Reference standard
Although there is no consensus regarding a reference standard for evaluating the quality of administrative data [ 23 ], numerous studies have used data from national representative surveys that provide accurate estimates of population characteristics, such as the CCHS, to validate administrative data in ascertaining chronic conditions (e.g., diabetes, hypertension, osteoporosis) [ 24 , 25 , 26 , 27 , 28 , 29 , 30 , 31 ]. Language variables collected in self-report surveys (e.g., Census, CCHS) are more explicitly defined than administrative databases. The CCHS includes original language variables (e.g., mother tongue, LOSH, and knowledge of official languages) and derived variables, such as FOLS, which are based on two or more language variables. Despite minor modifications to variable definitions since the inception of the CCHS, these variables provide accurate estimates of the linguistic characteristics of the Canadian population [ 19 , 32 , 33 ].
Given the validity of national representative surveys conducted by Statistics Canada, we used the language variables from the CCHS, LOSH, an original variable collected in the survey and FOLS, which is a derived variable from the knowledge of official languages, mother tongue, and LOSH [ 34 ] as the reference standard measures to assess the capacity of health data to ascertain the French-speaking population. The levels of non-response for the language variables in CCHS was low across cycles (< 5%), ranging from 0.2 to 2.7%. The levels of missing values in health data were also lower than 5%. We did not exclude the records with missing values for these variables and made no imputations.
- CCRS: Continuing Care Reporting System, HCRS: Home Care Reporting System, OMHRS: Ontario Mental Health Reporting System, CCHS: Canadian Community Health Survey
Administrative data and language information
The three administrative health databases (CCRS, HCRS and OMHRS) containing language information were used to identify Francophones. Without a clear and specific language definition, administrative health databases may be subject to interviewer bias (i.e., the interviewer may assume the respondent’s language without explicitly asking for this information). Thus, the language variables from CCHS were used as the reference standard to validate the language variables in the health data. There are several language variables included in the survey (see Appendix 2 ), but LOSH and FOLS were used for the validity analysis.
The language variables Mother tongue, LOSH and language of conversation in CCHS allowed to derive the Knowledge of official languages and FOLS, following Statistics Canada’s definition [ 34 ]. Details on the collection of language variables are provided in Appendix 2 .
Although it is possible to make population estimates using CCHS survey weights, in this study we reported unweighted values, which were used to perform the individual data linkage and the analyses.
Descriptive analyses of the language variables in all databases were performed. First, a frequency analysis of all language variables was conducted, and the proportion of participants in each linguistic group was reported. We provide a covariate description of the sample stratified by language group (i.e. francophones) and by age group, sex, rural/urban area of residence, marital and immigrant status, education and income levels. Second, the linked data set was used to evaluate the concordance of the language variables in identifying francophones by performing an agreement analysis using Cohen’s kappa coefficient, which is a widely used measure of concordance between assessors and indicates the proportion of agreement beyond that expected by chance [ 35 ]. The levels of agreement for kappa were considered poor (κ < 0.20), fair (κ = 0.20 to 0.39), moderate (κ = 0.40 to 0.59), good (κ = 0.60 to 0.79), or very good (κ = 0.80 to 1.00) [ 25 , 36 ]. Next, validity analyses were performed to determine the language concept captured by the language variables in administrative data. The validity of the language variables in administrative health data for identifying francophones was assessed by calculating the sensitivity, specificity, positive predictive value (PPV), and negative predictive value (NPV) [ 36 , 37 ] using FOLS and LOSH as reference standards. All analyses were conducted using SAS 9.4 (SAS Institute, Inc., Cary, NC).
This project was approved by ICES’ Privacy and Compliance Office. ICES is a prescribed entity under Sect. 45 of Ontario’s Personal Health Information Protection Act, which does not require review by a Research Ethics Board.
The combined CCHS sample consisted of 198,509 respondents, which were individually linked to their provincial health card number that allowed individual linkage to the administrative databases, resulting in Ontarians within CCRS (including 212,954 individuals)), HCRS ( n = 716,698 individuals) and OMHRS ( n = 233,408 individuals). The linked dataset consisted of individuals who participated in at least one cycle of the CCHS cycle and who were captured in at least one of the three administrative health databases for the timespan of the CCHS cycles (2000–2012). The final study sample consisted of 27,111 CCHS respondents who received home care services (HCRS) or long-term care services (CCRS) or were admitted to an inpatient mental health service (OMHRS) (Fig. 1 ). A summary of the characteristics of these databases by language group is provided in Table S1 in supplementary material.
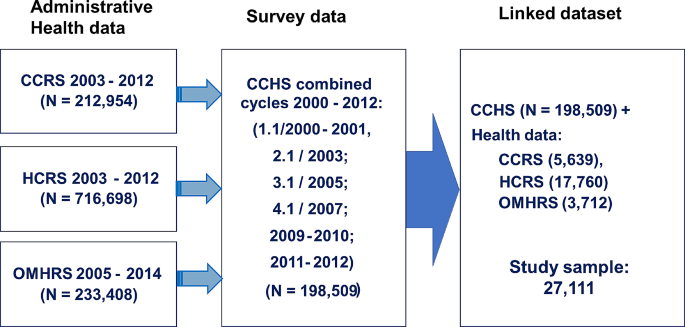
Sample size from each data source and linked sample. CCRS: Continuing Care Reporting System. HCRS: Home Care Reporting System. OMHRS: Ontario Mental Health Reporting System. CCHS: Canadian Community Health Survey
Table 1 presents the unweighted frequencies for the characteristics of the 198,509 respondents from the combined CCHS cycles, and the characteristics of francophones identified by FOLS and LOSH (a weighed sample is presented in Table S2 , Appendix 3 ).
Within the study sample, 6.3% were French speakers by mother tongue, 6.0% were identified as Francophone by FOLS, and 3.6% reported using French as the language often spoken at home (Table 2 and Table S3 , Appendix 3 ). Less than 2% of respondents conducted the interview in French or indicated French as their preferred language for the interview. Even fewer respondents (1.8%) reported speaking French with their doctor. Based on the language variables in administrative health databases, long-term care data (CCRS) identified the largest proportion of French speakers (3.2%), followed by home care data using the HCRS (2.8%).
The analysis of the levels of concordance between the two data sources (self-report surveys and administrative health databases) showed that the language variables in the health data from home care and long-term care had the highest agreement with LOSH (kappa = 0.76 [0.73–0.79] and 0.75 [0.70–0.80], respectively) (Table 3 ). The language variables from these two databases (HCRS and CCRS) also held a high level of agreement with FOLS (kappa = 0.66 [0.61–0.71] for both). The language variable in OMHRS (mental health) had poor agreement with the language variables from survey data.
When comparing language variables from administrative health databases to self-reported data, we found that the language variables from home care and long-term care databases (HCRS and CCRS) were modestly sensitive (53.1% [49.8–56.4] and 54.1% [48.3–59.7], respectively) but highly specific (99.8% [99.7–99.9] and 99.6% [99.4–99.8], respectively) when FOLS was used as the reference standard. Furthermore, these variables also had very high PPVs (94.4% [92.0-96.2] and 91.2% [85.9–94.7], respectively) and NPVs (96.9% [96.3–97.3] for both data sources) (see Fig. 2 and Table S3 in supplementary material). The sensitivity was even higher when LOSH was used as the reference standard (75.5% [71.6–79.0] and 74.2% [67.3–80.1] for HCRS and CCRS, respectively). The predictive values were also very high with this reference standard for both the HCRS and CCRS databases (PPV 79.6% [75.2–82.4] and 78.0% [71.0-83.6], respectively; and NPV of 99.1% [98.9–99.2] and 98.8% [98.5–99.2], respectively) (Fig. 2 ).
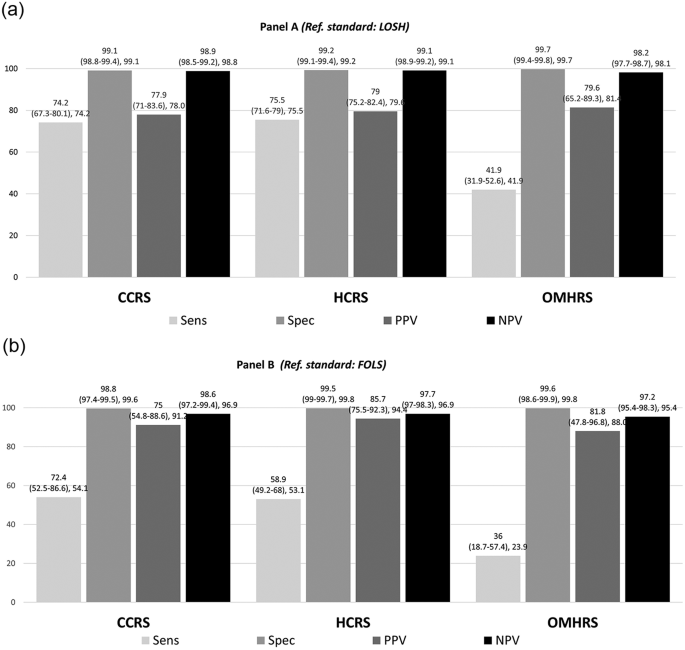
Sensitivity, specificity and predictive values of language variables in administrative health data ( n = 27,111). Sens: sensitivity; Spec: specificity; PPV: positive predictive value; NPV: negative predictive value, CCRS: Continuing Care Reporting System, HCRS: Home Care Reporting System, OMHRS: Ontario Mental Health Reporting System
Consistent with the agreement analysis, the language variable from OMHRS had very low sensitivity (23.9% [18.1–30.9]) against LOSH but very high specificity (99.8% [99.5–99.9]) and high predictive values (PPV and NPV). Details of all estimations are provided in Table S4 , Appendix 3 .
This study sought to assess the validity of language variables in administrative health databases by comparing language variables recorded in these databases to language data from the CCHS (i.e., LOSH and FOLS), which was taken to be the reference standard. Agreement and validity analyses were carried out, with the objective of identifying Francophones in Ontario in administrative data. Language variables from home care and long-term care data had the highest level of agreement with LOSH and FOLS, while the language variable from mental health admissions had poor agreement with the language variables in the CCHS.
While “primary language” is the language variable most commonly used to collect information in healthcare settings [ 8 , 38 , 39 ], the definition varies across databases and across the healthcare literature; some studies define primary language to be analogous to the language most commonly used (e.g., at home, at school, at work) [ 8 , 40 ], while others consider the respondent’s first language learned (or mother tongue) to be their primary language [ 41 , 42 , 43 ]. The results of this study suggest that the linguistic concept captured by the language variables in both home care and long-term care databases is most similar to LOSH, which showed the highest level of agreement of the language information from home care and LTC settings (kappa = 0.764 and 0.75, respectively). Health care professionals who perform the interviews for home care using the HCRS are encouraged to “observe and listen” to the patient and their family to identify the patient’s primary language and to determine the need for an interpreter [ 44 ]. Thus, it is not surprising that the language variable in home care and long-term care databases (HCRS and CCRS) corresponds to the language that the patient most commonly uses to communicate in their own home. This definition of primary language (i.e., language most commonly used either at home or on a day-to-day basis) is similar to that used in previous healthcare studies performed with administrative data [ 45 , 46 , 47 ].
This study found a high level of concordance between language variables in administrative databases (HCRS and CCRS) when using FOLS as the reference standard. There was very high specificity for ascertaining the Francophones comparing administrative health databases to both LOSH and FOLS, but sensitivity was higher when compared against LOSH. These findings suggest that some home care and long-term care recipients who were identified as Francophones in administrative health databases captured those whose LOSH was French but often missed those whose FOLS was French, which is consistent with the finding of a higher proportion of French speakers with FOLS. Furthermore, given that mother tongue, a component of FOLS, captured the greatest number of Francophones, it is likely that FOLS identified Francophones by mother tongue who no longer speak French on a regular basis at home. In addition, given the higher level of bilingualism among francophones, might influence the decision of many of them to report English as the main language when seeking and receiving care in Ontario. This offer francophones some advantage in a linguistic minority context, when services in French are not available or experience of discrimination or lower quality of care.
The very high predictive values for both CCRS and HCRS implied that participants identified as Francophones in the administrative health databases are very likely to have self-identified as Francophones in the CCHS. Coding errors in administrative health databases may partly account for low sensitivity. It is also possible that administrative health data captured individuals who are fully bilingual and comfortable seeking care from English providers (and thus more likely to be coded as Anglophone), whereas survey data may have included more unilingual Francophones (or Francophones with low English proficiency), who are less likely to seek healthcare services in Ontario [ 48 , 49 ], which are generally provided in English.
Interestingly, the rate of bilingualism is higher among Francophones than Anglophones [ 50 ], which is consistent with the finding of very high specificity and very high negative predictive value. In other words, some Francophones were identified as Anglophones, but very few Anglophones were identified as Francophones. Overall, these results highlight the importance of individual language preference for multilingual patients when seeking care, which may depend on the context (e.g., interpreter use, bilingual provider), as shown in other studies [ 41 , 51 ].
Concordance and sensitivity for identifying Francophones were very low for the OMHRS database. The poor concordance for the language variable in the database related to mental health hospitalizations (i.e., OMHRS) may be related to data entry errors and underreporting. Unlike home care and long-term care assessments, which are performed in the outpatient setting, data for OMHRS are collected in acute care settings. As such, it is likely that interviewers spend less time performing assessments for OMHRS because of competing tasks (e.g., admission documents, clinical care) that must also be performed simultaneously. Furthermore, since the OMHRS captures patients admitted to inpatient mental health hospitals, patients may not be able to provide accurate information due to an underlying mental health disorder (e.g., depression, mania, psychosis). In these situations, reported answers may be influenced by an accompanying person or may be assumed by the interviewer. These factors could bias the interviewers to report the patient’s language as English since it is the most common language at most hospitals in Ontario.
Despite the high concordance of primary language captured in administrative health databases with the language reported in survey data, these results do not imply that there is a single approach to identifying linguistic groups. The approach to selecting the most appropriate language variable for a study should be guided by the design and research question of the study [ 7 , 12 ], since these elements can impact the language concept of interest. For example, researchers examining the impacts of language barriers may choose a variable that identifies people who can and cannot speak a given language, while researchers studying disparities across ethnolinguistic groups may select a variable such as mother tongue to identify all members of the group in question.
The study design should also be taken into account when performing validation studies of language variables in other administrative databases. Researchers should carefully consider the linguistic concept in the context of the proposed research question while also examining the quality of the administrative data to determine the optimal reference standard for validation. For example, FOLS, which creates linguistic groups denoting Canada’s two official languages (English and French), may not be relevant when studying minority groups other than Francophones, which consist of a higher proportion of individuals who speak neither English nor French. In such instances, language variables such as LOSH or mother tongue may be more suitable.
Strengths and limitations
For this study, two language variables from a self-report survey (CCHS) were used as the reference standard for respondents’ language. This reference standard, which has not been validated to our knowledge, is subject to self-reporting bias since respondents may overestimate or underestimate their language proficiency. However, self-reported data have previously been used in validation studies of other administrative databases [ 24 , 25 , 26 ]. Moreover, the CCHS is a nationally representative survey that provides robust cross-sectional estimates of sociodemographic and health characteristics of the Canadian population [ 52 ]. Finally, the proportions of Francophones and other linguistic groups by mother tongue, LOSH and FOLS from the CCHS are consistent with those obtained from census data [ 14 ].
Nevertheless, there may remain response bias in the CCHS, as some bilingual participants may have reported English or French as the language often spoken at home despite speaking both languages on a regular basis. Contextual factors may also influence an individual’s decision to report his or her primary language in administrative health databases. Since English is the most common language in Ontario, Francophones who also speak English may have reported their primary language as English because they perceived this answer to be more favorable (social desirability bias). This factor may have led to an underestimation of the number of Francophones identified by administrative health databases.
Conclusions and implications
To our knowledge, no previous study has examined the agreement between language variables in survey data and administrative health databases. This study revealed that language variables in administrative health databases of home care and long-term care have a high level of concordance with LOSH and FOLS and, thus, can be used to reliably identify linguistic groups for the purpose of performing research to assess the impact of language factors on health outcomes. However, caution must be exercised when using language variables collected from acute care settings (such as OMHRS), as these variables may be less reliable. These results suggest that the language concept captured by administrative health databases, particularly from home care and long-term care data, is most similar to language spoken at home. Reporting guidelines recommend studies that use routinely collected data report potential measurement error and how measurement error potentially biases the study’s findings [ 37 ]. Hence, the findings from this study can be used for this purpose.
Data availability
The data set from this study is held securely in coded form at ICES. While data sharing agreements prohibit ICES from making the data set publicly available, access may be granted to those who meet prespecified criteria for confidential access, available at www.ices.on.ca/DAS . The full data set creation plan and underlying analytic code are available from the authors upon request, understanding that the computer programs may rely upon coding templates or macros that are unique to ICES and are therefore either inaccessible or may require modification.
Abbreviations
Canadian Community Health Survey
Continuing Care Reporting System
First official language spoken
Home Care Reporting System
Knowledge of Official Languages
Language often spoken at home
Long-term care
Negative predictive value
Ontario’s universal health insurance plan
Ontario Mental Health Reporting System
Positive predictive value
Resident Assessment Instrument-Home Care
Bouchard L, Gaboury I, Chomienne M-H, Gilbert A, Dubois L. La santé en situation linguistique minoritaire. Healthc Policy. 2009;4:36–42.
PubMed PubMed Central Google Scholar
Bouchard L, Desmeules M. Linguistic minorities in Canada and Health. Healthc Policy. 2013;9:38–47.
Leis A, Bouchard L, Editorial. The Health of Official Language Minority populations. Can J Public Health. 2013;104:2.
Google Scholar
Gagnon-Arpin I, Bouchard L, Leis A, Bélanger M. Access to and use of Health Care Services in the Minority Language. In: Landry R, editor. Life in an Official Minority Language in Canada. Moncton, New Brunswick: Canadian Institute for Research on Linguistic Minorities; 2014. pp. 177–98.
Bouchard L, Batista R, Reaume M. Résultats de l’enquête sur la santé dans les collectivités canadiennes. Ottawa, ON: Université d’Ottawa et Institut du savoir Montfort; 2021. p. 32. Évolution de principaux indicateurs de la santé de la population francophone et anglophone de l’Ontario: 2001–2014.
Gaboury I, Noël JG, Forgues É, Bouchard L. Les données administratives et d’enquêtes sur l’état de santé et l’accès aux services des communautés francophones en situation minoritaire: Potentiel D’analyse et état de situation. Ottawa, ON: Consortium national de formation en santé; 2009. p. 44.
Bouchard L, Lizotte M. Les données linguistiques pour la recherche et la planification en santé: possibilités et limites pour l’étude des francophones en situation minoritaire. Minorité Linguistique et Société 2024; sous presse.
Hasnain-Wynia R, Baker DW. Obtaining data on patient race, ethnicity, and primary language in health care organizations: current challenges and proposed solutions. Health Serv Res. 2006;41:1501–18.
Article PubMed PubMed Central Google Scholar
Chin MH. Using patient race, ethnicity, and language data to achieve health equity. J Gen Intern Med. 2015;30:703–5.
Makvandi E, Bouchard L, Bergeron PJ, Sedigh G. Methodological issues in analyzing small populations using CCHS cycles based on the Official Language Minority studies. Can J Public Health-Revue Canadienne De Sante Publique. 2013;104:S55–9.
Article Google Scholar
Tempier R, Bouattane EM, Hirdes JP. Access to psychiatrists by french-speaking patients in Ontario hospitals:2005 to 2013. Healthc Manage Forum. 2015;28:167–71.
Article PubMed Google Scholar
Noël JG, Forgues E, Landry R. Qui sont les francophones ? Analyse de définitions selon les variables du recensement. Moncton, NB: Institut canadien de recherche sur les minorités linguistiques, 2014, p. 64.
Statistics Canada. Linguistic characteristics of canadians. 2011 Census of Population. Ottawa, ON: Statistics Canada; 2012. p. 24.
Statistics Canada. English, French and official language minorities in Canada Census in brief . Ottawa, ON: Statistics Canada; 2017. p. 12.
Institute of Medicine. Race, ethnicity, and Language data: standardization for Health Care Quality Improvement. Washington, DC: The National Academy; 2009. p. 287.
Statistics Canada. French and the Francophonie in Canada. Census in brief. Ottawa, ON: Statistics Canada; 2012. p. 13.
Corbeil J-P, Grenier C, Lafrenière S. Minorities speak up: results of the Survey of the vitality of Official-Language minorities. Ottawa, ON: Statistics Canada; 2006. p. 176.
Bourbonnais V. La santé des aînés francophones en situation linguistique minoritaire: État Des lieux en Ontario. Departement De Sociologie, Facultes De Sciences Sociales. Ottawa, ON: University of Ottawa; 2007. p. 126.
Bouchard L, Batal M, Imbeault P, Gagnon-Arpin I, Makandi E, Sedigh G. La santé Des francophones de l’Ontario Un portrait régional tiré des Enquêtes sur la santé dans les collectivités canadiennes (ESCC). Rapports sur la santé des francophones de l’Ontario. Ottawa, ON: Réseau de recherche appliquée sur la santé des francophones de l’Ontario; 2012. p. 75.
Sucha E, SIlva E, Batista R, Bouchard L. Mortality in francophone minority in Canada – A 16-year follow-up study. Mortality by socioeconomic status among Canadian francophones and anglophones living outside Québec. Ottawa, ON: Réseau de recherche appliquée sur la santé des francophones de l’Ontario, University of Ottawa; 2014. p. 16.
Auger N, Harper S, Barry AD, Trempe N, Daniel M. Life expectancy gap between the Francophone majority and anglophone minority of a Canadian population. Eur J Epidemiol. 2012;27:27–38.
Lo E, Tu MT, Trempe N, Auger N. Linguistic mortality gradients in Quebec and the role of migrant composition. Can J Public Health. 2018;109:15–26.
Iron K, Manuel D. Quality assessment of administrative data (QuAAD): an opportunity for enhancing Ontario’s health data. Atlases and reports. Ottawa, ON: Institute for Clinical Evaluative Sciences; 2007. p. 35.
Tu K, Wang M, Jaakkimainen RL, et al. Assessing the validity of using administrative data to identify patients with epilepsy. Epilepsia. 2014;55:335–43.
Lix LM, Yogendran MS, Shaw SY, Targownick LE, Jones J, Bataineh O. Comparing administrative and survey data for ascertaining cases of irritable bowel syndrome: a population-based investigation. BMC Health Serv Res. 2010;10:31.
Muggah E, Graves E, Bennett C, Manuel DG. Ascertainment of chronic diseases using population health data: a comparison of health administrative data and patient self-report. BMC Public Health. 2013;13:16.
Muhajarine N, Mustard C, Roos LL, Young TK, Gelskey DE. Comparison of survey and physician claims data for detecting hypertension. J Clin Epidemiol. 1997;50:711–8.
Article CAS PubMed Google Scholar
Hux JE, Ivis F, Flintoft V, Bica A. Diabetes in Ontario. Determ Preval Incidence Using Validated Administrative data Algorithm. 2002;25:512–6.
Huzel L, Roos LL, Anthonisen NR, Manfreda J. Diagnosing asthma: the fit between survey and administrative database. Can Respir J. 2002;9:407–12.
Lix LM, Yogendran MS, Leslie WD, et al. Using multiple data features improved the validity of osteoporosis case ascertainment from administrative databases. J Clin Epidemiol. 2008;61:1250–60.
Tu K, Campbell NRC, Chen Z-L, Cauch-Dudek KJ, McAlister FA. Accuracy of administrative databases in identifying patients with hypertension. Open Med. 2007;1:e18–26.
Bouchard L, Makvandi E, Sedigh G, Van Kemenade S. The Health of the Francophone Population Aged 65 and over in Ontario. A region-by-region portrait based on the Canadian Community Health Survey (CCHS). Ottawa2014, p. 48.
Belanger M, Bouchard L, Gaboury I, et al. Perceived health status of francophones and anglophones in an officially bilingual Canadian province. Can J Public Health = Revue canadienne de sante Publique. 2011;102:122–6.
Statistics Canada. First Official Language Spoken Statistics Canada. 2018. (accessed April 26, 2019). http://www23.statcan.gc.ca/imdb/p3Var.pl?Function=DEC&Id=34004
Watson PF, Petrie A. Method agreement analysis: a review of correct methodology. Theriogenology. 2010;73:1167–79.
Cunningham M. More than just the Kappa Coefficient: a program to fully characterize inter-rater reliability between two raters. SAS Global Forum 2009. Pittsburgh, PA: University of Pittsburgh; 2009. p. 7.
Benchimol EI, Smeeth L, Guttmann A, et al. The REporting of studies conducted using Observational routinely-collected health data (RECORD) Statement. PLoS Med. 2015;12:e1001885.
Human Rights & Health Equity Office. Guide to Demographic Data Collection in Health-care settings. Toronto, ON: Human Rights & Health Equity Office, Sinai Health System; 2017. p. 30.
Hedges Greising C. Collecting race, ethnicity, and primary Language Data: Tools to Improve Quality of Care and Reduce Health Care disparities. Issue brief. Chicago, IL: Health Reseach and Educational Trust; 2012. p. 6.
Hasnain-Wynia R, Pierce D, Pittman MA. Who, when, and how: the current state of race, ethnicity, and primary Language Data Collection in hospitals. New York, NY: The Commonwealth Fund; 2004. p. 42.
Duong LM, Singh SD, Buchanan N, Phillips JL, Gerlach K. Evaluation of primary/preferred language data collection. J Registry Manag. 2012;39:121–32.
PubMed Google Scholar
Ulmer C, McFadden B, Nerenz D. Defining Language need and categories for Collection. In: Ulmer CMB, Nerenz DR, editors. Race, ethnicity, and Language Data: standardization for Health Care Quality Improvement. Washington DC: Institute of Medicine, National Academies; 2009. pp. 93–125.
John-Baptiste A, Naglie G, Tomlinson G, et al. The effect of English language proficiency on length of stay and in-hospital mortality. J Gen Intern Med. 2004;19:221–8.
Morris J, Fries B, Bernabei R, et al. Resident Assessment Instrument-Home Care (RAI-HC) user’s Manual, Canadian Version. Washington DC: Canadian Institute for Health Information; 2010. p. 176.
Cheng EM, Chen A, Cunningham W. Primary Language and Receipt of Recommended Health Care among hispanics in the United States. J Gen Intern Med. 2007;22:283–8.
Hines AL, Andrews RM, Moy E, Barrett ML, Coffey RM. Disparities in Rates of Inpatient Mortality and adverse events: Race/Ethnicity and Language as Independent contributors. Int J Environ Res Public Health. 2014;11:13017–34.
Karliner LS, Kim SE, Meltzer DO, Auerbach AD, Auerbach AD. Influence of language barriers on outcomes of hospital care for general medicine inpatients. J Hosp Med. 2010;5:276–82.
Bélanger R, Mayer-Crittenden C, Mainguy J, Coutu A. Enquête sur l’offre active pour les services auxiliaires de santé du Nord-Est De l’Ontario. Reflets. 2018;24:212–47.
Forgues É, Landry R. L’accès aux services de santé en français et leur utilisation en contexte francophone minoritaire . Moncton, NB: Société Santé en français et Institut canadien de recherche sur les minorités linguistiques, 2014, p.158.
Statistics Canada. L’évolution Du Bilinguisme français-anglais Au Canada De 1901 à 2011. Ottawa, ON: Statistics Canada; 2012. p. 5.
Klinger EV, Carlini SV, Gonzalez I, et al. Accuracy of race, ethnicity, and language preference in an electronic health record. J Gen Intern Med. 2015;30:719–23.
Statistics Canada. Canadian Community Health Survey - Annual Component (CCHS). Statistics Canada. 2018.). http://www23.statcan.gc.ca/imdb/p2SV.pl?Function=getSurvey&SDDS=3226
Download references
Acknowledgements
Parts or all of this material are based on data and/or information compiled and provided by Immigration, Refugees and Citizenship Canada (IRCC) as of May 31, 2017. However, the analyses, conclusions, opinions and statements expressed in the material are those of the author(s) and not necessarily those of IRCC. Parts of this material are based on data and/or information compiled and provided by CIHI. However, the analyses, conclusions, opinions and statements expressed in the material are those of the author(s) and not necessarily those of CIHI.
This study was primarily supported by the Institut du Savoir Montfort and the Programme de subventions Savoir Montfort, concours 2018–2019 funded by Fondation Monfort. This study was also supported by ICES, which is funded by an annual grant from the Ontario MOHLTC. ICES has been approved by Ontario’s Information and Privacy Commissioner since 2005. The opinions, results, and conclusions reported in this article are those of the authors and are independent from the funding sources. No endorsement by ICES or the Ontario MOHLTC is intended or should be inferred. ICES collects information most notably for purposes of Sect. 45 of Ontario’s Personal Health Information Protection Act (PHIPA). RB is supported by the Institut du Savoir Montfort. Tanuseputro is supported by a PSI Graham Farquharson Knowledge Translation Fellowship.
Author information
Authors and affiliations.
Institut du Savoir Montfort, Ottawa, ON, Canada
Ricardo Batista, Louise Bouchard, Eva Guerin & Denis Prud’homme
ICES uOttawa, Ottawa, ON, Canada
Ricardo Batista, Amy T. Hsu, Ewa Sucha, Douglas G. Manuel & Peter Tanuseputro
Clinical Epidemiology Program, Ottawa Hospital Research Institute, Ottawa, ON, Canada
Ricardo Batista, Amy T. Hsu, Emily Rhodes, Douglas G. Manuel & Peter Tanuseputro
Université de Moncton, Moncton, New Brunswick, Canada
Denis Prud’homme
Elizabeth Bruyère Research Institute, Ottawa, ON, Canada
School of Social and Anthropological Studies, University of Ottawa, Ottawa, ON, Canada
Louise Bouchard
University of Manitoba, Winnipeg, MB, Canada
Michael Reaume
Faculty of Medicine, University of Ottawa, Ottawa, ON, Canada
Douglas G. Manuel & Peter Tanuseputro
Statistics Canada, Ottawa, ON, Canada
Douglas G. Manuel
Institut du Savoir Montfort, ICES and Ottawa Hospital Research Institute, 1053 Carling Ave Box 693, 2-006 Admin Services Building, Ottawa, ON, K1Y 4E9, Canada
Ricardo Batista
You can also search for this author in PubMed Google Scholar
Contributions
RB, AH, ES, DP, DM, PT, conceived the study. ES conducted the statistical analysis. RB drafted the manuscript. All other authors (DP, AH, LB, MR, ER, EG, ES, DM, and PT) were involved in the analysis and interpretation of the results, revised the manuscript, and gave final approval for publication.
Corresponding author
Correspondence to Ricardo Batista .
Ethics declarations
Ethics approval and consent to participate.
All methods used in this study were carried out in accordance with relevant ethics guidelines and regulations to conduct health research projects. This project was conducted under Sect. 45 and approved by the ICES Privacy and Compliance Office. ICES is a prescribed entity under Sect. 45 of Ontario’s Personal Health Information Protection Act. Section 45 authorizes ICES to collect personal health information, without consent, for the purpose of analysis or compiling statistical information with respect to the management of, evaluation or monitoring of allocation of resources to or planning for all or part of the health system.
Consent for publication
Not applicable.
Competing interests
The authors declare that they have no competing interests.
Additional information
Publisher’s note.
Springer Nature remains neutral with regard to jurisdictional claims in published maps and institutional affiliations.
Electronic supplementary material
Below is the link to the electronic supplementary material.
Supplementary Material 1
Rights and permissions.
Open Access This article is licensed under a Creative Commons Attribution 4.0 International License, which permits use, sharing, adaptation, distribution and reproduction in any medium or format, as long as you give appropriate credit to the original author(s) and the source, provide a link to the Creative Commons licence, and indicate if changes were made. The images or other third party material in this article are included in the article’s Creative Commons licence, unless indicated otherwise in a credit line to the material. If material is not included in the article’s Creative Commons licence and your intended use is not permitted by statutory regulation or exceeds the permitted use, you will need to obtain permission directly from the copyright holder. To view a copy of this licence, visit http://creativecommons.org/licenses/by/4.0/ . The Creative Commons Public Domain Dedication waiver ( http://creativecommons.org/publicdomain/zero/1.0/ ) applies to the data made available in this article, unless otherwise stated in a credit line to the data.
Reprints and permissions
About this article
Cite this article.
Batista, R., Hsu, A., Bouchard, L. et al. Ascertaining the Francophone population in Ontario: validating the language variable in health data. BMC Med Res Methodol 24 , 98 (2024). https://doi.org/10.1186/s12874-024-02220-7
Download citation
Received : 11 July 2023
Accepted : 15 April 2024
Published : 27 April 2024
DOI : https://doi.org/10.1186/s12874-024-02220-7
Share this article
Anyone you share the following link with will be able to read this content:
Sorry, a shareable link is not currently available for this article.
Provided by the Springer Nature SharedIt content-sharing initiative
- Linguistic variables
- Administrative health data
- Case ascertainment
- Francophones
BMC Medical Research Methodology
ISSN: 1471-2288
- General enquiries: [email protected]

COMMENTS
Types of Variables in Research. Types of Variables in Research are as follows: Independent Variable. This is the variable that is manipulated by the researcher. It is also known as the predictor variable, as it is used to predict changes in the dependent variable. Examples of independent variables include age, gender, dosage, and treatment type ...
Examples. Discrete variables (aka integer variables) Counts of individual items or values. Number of students in a class. Number of different tree species in a forest. Continuous variables (aka ratio variables) Measurements of continuous or non-finite values. Distance.
The independent variable is the cause. Its value is independent of other variables in your study. The dependent variable is the effect. Its value depends on changes in the independent variable. Example: Independent and dependent variables. You design a study to test whether changes in room temperature have an effect on math test scores.
Examples. Discrete variables (aka integer variables) Counts of individual items or values. Number of students in a class. Number of different tree species in a forest. Continuous variables (aka ratio variables) Measurements of continuous or non-finite values. Distance.
Designation of the dependent and independent variable involves unpacking the research problem in a way that identifies a general cause and effect and classifying these variables as either independent or dependent. The variables should be outlined in the introduction of your paper and explained in more detail in the methods section. There are no ...
The Role of Variables in Research. In scientific research, variables serve several key functions: Define Relationships: Variables allow researchers to investigate the relationships between different factors and characteristics, providing insights into the underlying mechanisms that drive phenomena and outcomes. Establish Comparisons: By manipulating and comparing variables, scientists can ...
While the independent variable is the " cause ", the dependent variable is the " effect " - or rather, the affected variable. In other words, the dependent variable is the variable that is assumed to change as a result of a change in the independent variable. Keeping with the previous example, let's look at some dependent variables ...
The independent variable is the cause. Its value is independent of other variables in your study. The dependent variable is the effect. Its value depends on changes in the independent variable. Example: Independent and dependent variables. You design a study to test whether changes in room temperature have an effect on maths test scores.
Variables in Research. The definition of a variable in the context of a research study is some feature with the potential to change, typically one that may influence or reflect a relationship or ...
As we mentioned, research methodology refers to the collection of practical decisions regarding what data you'll collect, from who, how you'll collect it and how you'll analyse it. Research design, on the other hand, is more about the overall strategy you'll adopt in your study. For example, whether you'll use an experimental design ...
Research methods are specific procedures for collecting and analyzing data. Developing your research methods is an integral part of your research design. When planning your methods, there are two key decisions you will make. First, decide how you will collect data. Your methods depend on what type of data you need to answer your research question:
Qualitative Variables. An important distinction between variables is the qualitative and quantitative variables. Qualitative variables are those that express a qualitative attribute, such as hair color, religion, race, gender, social status, method of payment, and so on.The values of a qualitative variable do not imply a meaningful numerical ordering.
Definition: Independent variable is a variable that is manipulated or changed by the researcher to observe its effect on the dependent variable. It is also known as the predictor variable or explanatory variable. The independent variable is the presumed cause in an experiment or study, while the dependent variable is the presumed effect or outcome.
The purpose of research is to describe and explain variance in the world, that is, variance that. occurs naturally in the world or chang e that we create due to manipulation. Variables are ...
The methods used in many methodological studies have been borrowed from systematic and scoping reviews. This practice has influenced the direction of the field, with many methodological studies including searches of electronic databases, screening of records, duplicate data extraction and assessments of risk of bias in the included studies.
INTRODUCTION. Scientific research is usually initiated by posing evidenced-based research questions which are then explicitly restated as hypotheses.1,2 The hypotheses provide directions to guide the study, solutions, explanations, and expected results.3,4 Both research questions and hypotheses are essentially formulated based on conventional theories and real-world processes, which allow the ...
Definition, Types, and Examples. Research methodology 1,2 is a structured and scientific approach used to collect, analyze, and interpret quantitative or qualitative data to answer research questions or test hypotheses. A research methodology is like a plan for carrying out research and helps keep researchers on track by limiting the scope of ...
research variables could affect the outcome. There are many types of variable but the most important, for the vast majority of research methods, are the independent and dependent variables. A researcher must determine which variable needs to be manipulated to generate quantifiable results. The independent variable is the core of the experiment and
Regression analysis is a quantitative research method which is used when the study involves modelling and analysing several variables, where the relationship includes a dependent variable and one or more independent variables. In simple terms, regression analysis is a quantitative method used to test the nature of relationships between a dependent variable and one or more independent variables.
Quantitative research methods. You can use quantitative research methods for descriptive, correlational or experimental research. In descriptive research, you simply seek an overall summary of your study variables.; In correlational research, you investigate relationships between your study variables.; In experimental research, you systematically examine whether there is a cause-and-effect ...
Experimental research design is used to investigate cause-and-effect relationships between variables. This research method involves manipulating the independent variable and observing the effects on the dependent variable. Researchers use experimental research designs to test hypotheses and establish cause-and-effect relationships.
There are five types of variable in terms of research methodology as follows. Independent Variable The variable, value of which affects the value of another variable is known as independent variable.
ABOUT PEW RESEARCH CENTER Pew Research Center is a nonpartisan fact tank that informs the public about the issues, attitudes and trends shaping the world. It conducts public opinion polling, demographic research, media content analysis and other empirical social science research. Pew Research Center does not take policy positions.
Background Language barriers can impact health care and outcomes. Valid and reliable language data is central to studying health inequalities in linguistic minorities. In Canada, language variables are available in administrative health databases; however, the validity of these variables has not been studied. This study assessed concordance between language variables from administrative health ...
Existing studies have focused mainly on the environmental quality of scenic spots, such as sufficient oxygen content in the air and a high concentration of negative oxygen ions. The perceptions of soundscape in scenic areas are generally good, but there are few reports on the quantitative evaluation of soundscape quality in scenic areas. In this study, we analysed existing methods for ...
Qualitative research involves collecting and analyzing non-numerical data (e.g., text, video, or audio) to understand concepts, opinions, or experiences. It can be used to gather in-depth insights into a problem or generate new ideas for research. Qualitative research is the opposite of quantitative research, which involves collecting and ...
Mathematical Methods in the Applied Sciences is an interdisciplinary applied mathematics journal that connects mathematicians and scientists worldwide. In this paper, the direct similarity reduction method is used to reduce the modified variable coefficient Zakharove-Kusnetsov equation (vcmZK), which represents the propagation of quantum dust ...