Press Releases EMERGING Fund – Press Releases
Emerging in the news in the news, restaurant industry insights on the restaurant industry., technology insights on technology., staffing / hr insights on staffing and hr., operations insights on operations., data intelligence insights on data intelligence., real estate insights on real estate., beverage insights on beverage..
- Acceleration Platform
- Investor Login

The State of the Restaurant Industry & Customer Satisfaction
Marisa upson.
Marisa began her career in hospitality as the owner of Breath of Life, a spa and retreat located on the Central Coast of California. From there she he... continue
July 10, 2024
Customer satisfaction is essential to any business’s success. As guests become even more sensitive to price increases, it becomes mission-critical, particularly in the restaurant industry.
Today’s restaurant guests spend up to 10% more than they did two years ago. They’re responding to this increase by going out less or expecting more. Knowing what added value you can give your guests without reducing your profit margins is one of the keys. Unfortunately, the challenges many face across the country are more widespread, with increasing minimum wages and other regulations taking a toll on already reduced profits.
Let’s take a look at what some operators are experiencing and the actions they’re taking to help them succeed.
Plummeting Profit Margins
In a recent interview with the Los Angeles Times, Francesco Zimone said his profit margins from four L’Antica Pizzeria da Michele restaurants plummeted from 20% to 2% in 2023. In early 2024, the pressure decreased as the supply chain issues lessened, and he could buy in bulk. This year, he expects up to 5% to 7% profit margins.
Tip : Restaurant operators can often benefit from Group Purchasing Organizations (GPOs). One of the industry leaders in this field is Buyers Edge Platform . Tapping into their 100,000 foodservice members suddenly aligns you with the organizations with hundreds of locations. Leveraging this power reduces your everyday costs, boosting profitability.
Additional Challenges in the Industry
Other concerns affecting the industry include increasing minimum wages and legislation dictating what restaurants can charge. For instance, in California, as of July 1, restaurants can no longer add service charges or other surcharges to their guests’ bills. This includes surcharges that go towards health insurance, employee tips, and other benefits. Restaurants wanting to provide these benefits will have to increase prices amidst an environment and customers with lower tolerance.
Improving the Dining Experience
So, the question becomes, what can restaurants give their customers to improve the experience and add value, making it, in their eyes, worthwhile?
According to the recent American Customer Satisfaction Index (ACSI) Restaurant and Food Delivery Study , the customer satisfaction of full-service restaurants is up 4%. They also found that households with less than $75,000 yearly earnings are reducing their restaurant visits.
Forrest Morgeson, Associate Professor of Marketing at Michigan State University and Director of Research Emeritus at the ACSI shared this in a recent press release, “Customers are being forced to make decisions between groceries and restaurants, with full-service restaurant inflation about two times that of groceries in the past year and fast food and fast casual restaurant prices up three times the rate of groceries. With customers seemingly viewing dining out a luxury, restaurants that can differentiate themselves in terms of quality and value will have a competitive advantage.”
The top two restaurants for consumer preferences were LongHorn Steakhouse and Texas Roadhouse. They also noted better performance across the full-service spectrum, particularly in food order accuracy and servers’ courtesy and helpfulness.
Improving the dining experience can be as easy as making sure guests are greeted promptly and authentically when they walk in the front door. All interactions following the initial touch point should align with that level of service.
A person in the hospitality industry for many years recently shared an important lesson she’d learned along the way. “If a customer comes within 10 feet of you, you “own” them. It’s up to you to make sure they feel welcome and taken care of and answer any questions they may have.” All of us in the industry would do well to remember that bit of advice.
How can the restaurant customer experience be improved?
Suffice it to say food and beverages that are remembered long after your customers leave are critical to the overall experience. Your guests’ experience, however, is centered predominantly around their interactions with your staff. It’s about clear, timely, and caring communications.
Other essentials are ambiance and music that align with your brand, minimizing wait times, and making your guests feel at home.
Can guests decline a service charge at a restaurant?
That depends on the restaurant and where it’s located. Some restaurants do not make service charges mandatory. Additionally, a growing number of states are initiating bills addressing these charges. In many states, restaurants are required to disclose the fees or mandatory tips to guests before they place their orders. If performed as required, guests must pay the service charge.
Marisa began her career in hospitality as the owner of Breath of Life, a spa and retreat located on the Central Coast of California. From there she headed east, managing several resorts and restaurants from venues as varied as guest ranches in Colorado to remote resorts surrounded by the mesas and beautiful canyons of the Southwest. Her varied care... continue
Categories:
Subscribe to our latest insights.

Get a FREE Menu Price Comparison
Will ai revolutionize the restaurant drive-thru as we’ve come to know it, restaurants’ different approaches to a changing economic climate, analyzing wingstop’s ultra-successful model, restaurants boost sales with a value menu, family dining habits revealed in national study, charge up your restaurant, the restaurant menu redefined, a mid-year 2024 restaurant industry update as fall approaches, are you capital raise ready.
Information
- Author Services
Initiatives
You are accessing a machine-readable page. In order to be human-readable, please install an RSS reader.
All articles published by MDPI are made immediately available worldwide under an open access license. No special permission is required to reuse all or part of the article published by MDPI, including figures and tables. For articles published under an open access Creative Common CC BY license, any part of the article may be reused without permission provided that the original article is clearly cited. For more information, please refer to https://www.mdpi.com/openaccess .
Feature papers represent the most advanced research with significant potential for high impact in the field. A Feature Paper should be a substantial original Article that involves several techniques or approaches, provides an outlook for future research directions and describes possible research applications.
Feature papers are submitted upon individual invitation or recommendation by the scientific editors and must receive positive feedback from the reviewers.
Editor’s Choice articles are based on recommendations by the scientific editors of MDPI journals from around the world. Editors select a small number of articles recently published in the journal that they believe will be particularly interesting to readers, or important in the respective research area. The aim is to provide a snapshot of some of the most exciting work published in the various research areas of the journal.
Original Submission Date Received: .
- Active Journals
- Find a Journal
- Proceedings Series
- For Authors
- For Reviewers
- For Editors
- For Librarians
- For Publishers
- For Societies
- For Conference Organizers
- Open Access Policy
- Institutional Open Access Program
- Special Issues Guidelines
- Editorial Process
- Research and Publication Ethics
- Article Processing Charges
- Testimonials
- Preprints.org
- SciProfiles
- Encyclopedia
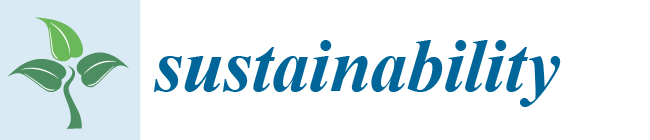
Article Menu

- Subscribe SciFeed
- Recommended Articles
- Google Scholar
- on Google Scholar
- Table of Contents
Find support for a specific problem in the support section of our website.
Please let us know what you think of our products and services.
Visit our dedicated information section to learn more about MDPI.
JSmol Viewer
Factors affecting customer satisfaction in fast food restaurant “jollibee” during the covid-19 pandemic.
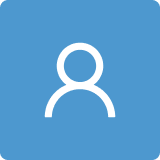
1. Introduction
2. theoretical framework, 3. methodology, 3.1. participants, 3.2. questionnaire, 3.3. statistical analysis: structural equation modeling, 5. discussion, limitations and future research, 6. conclusions, author contributions, institutional review board statement, informed consent statement, data availability statement, acknowledgments, conflicts of interest, appendix a. instruments.
___15 to 20 years old ___21 to 26 years old | ||||||
___27 to 33 years old ___34 to 40 years old | ||||||
___41 to 46 years old ___47 to 53 years old | ||||||
___54 and above. | ||||||
___once a week ___twice a week ___thrice a week | ||||||
___4 times a week and above | ||||||
___once a month | ||||||
___Student ___Employed ___Unemployed ___Other | ||||||
___₱11,999 and below ___₱12,000 to ₱20,999 | ||||||
___₱21,000 to ₱40,999 ___₱41,000 to ₱60,999 | ||||||
___₱61,000 to ₱80,999 ___₱81,000 and above | ||||||
___Yes ___No ___Sometimes | ||||||
___Yes ___No ___Sometimes | ||||||
Answer the following items by marking the column that corresponds to your answer. | ||||||
5—Very satisfied | ||||||
4—Somewhat satisfied | ||||||
3—Neither satisfied nor dissatisfied | ||||||
2—Somewhat dissatisfied | ||||||
1—Very dissatisfied | ||||||
T1. Virtual signs and messages for customers. | ||||||
T2. Staffs’ uniform. | ||||||
T3. Store appearance. | ||||||
T4. Reception Appearance (counters and waiting areas). | ||||||
T5. Accessibility to locations. | [ ] | |||||
T6. Store hygiene. | [ ] | |||||
REL1. Accommodation on customers. | [ ] | |||||
REL2. Speed in serving the food orders of the customers. | [ ] | |||||
REL3. Accuracy in responding to the food orders of the customers. | [ ] | |||||
REL4. Staff returns personal belongings and other valuable items. | ||||||
RES1. Assistance provided by guards or other staffs upon entry. | [ ] | |||||
RES2. Queue waiting time. | ||||||
RES3. Staffs promptly serve all customers. | [ ] | |||||
RES4. Staff courteousness. | [ ] | |||||
A1. Product knowledge of the staff. | [ ] | |||||
A2. Product quality assurance. | [ ] | |||||
A3. Staff communication skill. | [ ] | |||||
A4. All customer concerns and requests were done. | [ ] | |||||
E1. Staffs understand customer needs. | [ ] | |||||
E2. Staffs apologize when committing mistakes. | [ ] | |||||
E3. Staffs apologize when customer requests were not done. | [ ] | |||||
E4. Staffs willingness to help. | [ ] | |||||
E5. Staffs’ courtesy. | [ ] | |||||
SQ1. Overall facility appearance. | [ ] | |||||
SQ2. All the discussed services were done accurately. | [ ] | |||||
SQ3. Overall staff responsiveness to customers. | [ ] | |||||
SQ4. All services and requests done were explained. | ||||||
SQ5. Staff is competent in dealing with customer concerns. | ||||||
FQ1. Quality of fried chicken among competitors. | ||||||
FQ2. Quality of yum burger among competitors. | ||||||
FQ3. Quality of the fries among competitors. | ||||||
FQ4. Quality of the jolly spaghetti among competitors. | ||||||
FQ5. Quality of the sundae among competitors. | ||||||
FQ6. Overall food quality | ||||||
5—Very cheap | ||||||
4—Somewhat cheap | ||||||
3—Neither costly nor cheap | ||||||
2—Somewhat costly | ||||||
1—Very Costly | ||||||
P1. Compatibility of the price to the food quality. | ||||||
P2. Pricing compared to other fast food restaurants. | ||||||
P3. Affordability (5—Very affordable and 1—Very expensive). | [ ] | |||||
P4. Implementation of discount and buying package (5—Very satisfied and 1—Very dissatisfied). | [ ] | |||||
P5. Satisfaction based on overall pricing (5—Very satisfied and 1—Very dissatisfied). | ||||||
5—Strongly agree | ||||||
4—Somewhat agree | ||||||
3—Neither agree nor disagree | ||||||
2—Somewhat disagree | ||||||
1—Strongly disagree | ||||||
CP1. The social distancing has not affected my satisfaction when ordering and queuing in Jollibee. | ||||||
CP2. It is better to eat my orders from Jollibee inside their restaurants than to take it at home. | ||||||
CP3. Quarantines do not stop me from buying foods to Jollibee (ordering through online transactions). | ||||||
CP4. The use of face mask and face shield didn’t stop me from queuing and ordering to Jollibee. | ||||||
CP5. I still prefer to eat in Jollibee even if there are restaurants nearer in my location. | ||||||
CP6. The COVID-19 pandemic didn’t affect the quality of their foods. | ||||||
CP7. The COVID-19 pandemic didn’t affect their customer service quality. | ||||||
CP8. The COVID-19 pandemic didn’t affect their food pricing. | ||||||
CP9. The total number of COVID-19 cases do not affect my habit from dining inside the Jollibee. | ||||||
CI1.I like eating Jollibee because of their good TV commercials. | ||||||
CI2. I like eating to Jollibee because it has been with me since childhood. | ||||||
CI3. I like eating to Jollibee because I have good memories and experiences with it. | ||||||
CI4. I love going to Jollibee because it reminds me of the good Filipino tradition, through their influence in the commercial ads. | ||||||
CI5. I like eating to Jollibee because it reminds me of someone. | ||||||
C1. Satisfaction regarding the price given. | ||||||
C2. Satisfaction regarding the overall service quality given. | ||||||
C3. Recommend Jollibee to a friend or peer. | ||||||
C4. Continue patronizing Jollibee’s foods and beverages. | ||||||
C5. Overall satisfaction. |
- Mwangi, C.W. Strategic Responses to Competition among Large Fast Food Restaurants in Nairobi Central Business District. Ph.D. Thesis, School of Business, University of Nairobi, Nairobi, Kenya, 2010. [ Google Scholar ]
- Banterle, A.; Cavaliere, A.; Carraresi, L.; Stranieri, S. Food smes face increasing competition in the EU market: Marketing Management Capability is a tool for becoming a price maker. Agribusiness 2013 , 30 , 113–131. [ Google Scholar ] [ CrossRef ]
- Bowen, J.T.; Chen McCain, S.-L. Transitioning loyalty programs. Int. J. Contemp. Hosp. Manag. 2015 , 27 , 415–430. [ Google Scholar ] [ CrossRef ]
- Oliva, T.A.; Oliver, R.L.; MacMillan, I.C. A catastrophe model for Developing Service Satisfaction Strategies. J. Mark. 1992 , 56 , 83. [ Google Scholar ] [ CrossRef ]
- Zineldin, M. Exploring the common ground of total relationship management (TRM) and Total Quality Management (TQM). Manag. Decis. 1999 , 37 , 719–730. [ Google Scholar ] [ CrossRef ]
- Gerpott, T.J.; Rams, W.; Schindler, A. Customer retention, loyalty, and satisfaction in the German Mobile Cellular Telecommunications Market. Telecommun. Policy 2001 , 25 , 249–269. [ Google Scholar ] [ CrossRef ]
- Hansemark, O.C.; Albinsson, M. Customer satisfaction and retention: The experiences of individual employees. Manag. Serv. Qual. Int. J. 2004 , 14 , 40–57. [ Google Scholar ] [ CrossRef ]
- Nunkoo, R.; Teeroovengadum, V.; Ringle, C.M.; Sunnassee, V. Service quality and customer satisfaction: The moderating effects of Hotel Star Rating. Int. J. Hosp. Manag. 2020 , 91 , 102414. [ Google Scholar ] [ CrossRef ]
- Shim, S.; Eastlick, M.A.; Lotz, S.L.; Warrington, P. An online prepurchase intentions model. J. Retail. 2001 , 77 , 397–416. [ Google Scholar ] [ CrossRef ]
- Sheth, J. Impact of COVID-19 on consumer behavior: Will the old habits return or die? J. Bus. Res. 2020 , 117 , 280–283. [ Google Scholar ] [ CrossRef ]
- Limakrisna, N.; Ali, H. Model of customer satisfaction: Empirical study at fast food restaurants in bandung. Int. J. Bus. Commer. 2016 , 5 , 132–146. [ Google Scholar ]
- Balinado, J.R.; Prasetyo, Y.T.; Young, M.N.; Persada, S.F.; Miraja, B.A.; Perwira Redi, A.A. The effect of service quality on customer satisfaction in an automotive after-sales service. J. Open Innov. Technol. Mark. Complex. 2021 , 7 , 116. [ Google Scholar ] [ CrossRef ]
- Ong, A.K.; Cleofas, M.A.; Prasetyo, Y.T.; Chuenyindee, T.; Young, M.N.; Diaz, J.F.; Nadlifatin, R.; Redi, A.A. Consumer behavior in clothing industry and its relationship with open innovation dynamics during the COVID-19 pandemic. J. Open Innov. Technol. Mark. Complex. 2021 , 7 , 211. [ Google Scholar ] [ CrossRef ]
- Prasetyo, Y.T.; Tanto, H.; Mariyanto, M.; Hanjaya, C.; Young, M.N.; Persada, S.F.; Miraja, B.A.; Redi, A.A. Factors affecting customer satisfaction and loyalty in online food delivery service during the COVID-19 pandemic: Its relation with open innovation. J. Open Innov. Technol. Mark. Complex. 2021 , 7 , 76. [ Google Scholar ] [ CrossRef ]
- Alano, C.M. Top 10 Fast-Food Restaurants. Available online: https://www.philstar.com/lifestyle/food-and-leisure/2008/07/17/73334/top-10-fast-food-restaurants (accessed on 16 October 2021).
- Statista Research Department. Largest Foodservice Companies Philippines 2017, by Market Share. 2022. Available online: https://www.statista.com/statistics/1049413/philippines-largest-foodservice-companies-by-market-share/ (accessed on 16 October 2021).
- Altejar, L.; Dizon, C. Study of the Effects of Customer Service Quality and Product Quality on Customer Satisfaction and Customer Loyalty. Coll. Bus. Adm. Major Mark. Manag. Access Address 2019 , 10 , m9. [ Google Scholar ]
- A Jolly Toast to a New Era for Jollibee BusinessMirror. Available online: https://businessmirror.com.ph/2019/11/05/a-jolly-toast-to-a-new-era-for-jollibee/ (accessed on 16 October 2021).
- Beneschan, M. Analyzing Jollibee Restaurant Data. Available online: https://mikebeneschan.medium.com/analyzing-jollibee-restaurant-data-f57a24c6d942 (accessed on 20 March 2022).
- Venzon, C. Can Jollibee Take a Bite Out of the Global Fast-Food Market? Available online: https://asia.nikkei.com/Spotlight/The-Big-Story/Can-Jollibee-take-a-bite-out-of-the-global-fast-food-market (accessed on 20 March 2022).
- Venzon, C. Jollibee’s Quest for Global Dominance Derailed by Virus. Available online: https://asia.nikkei.com/Business/Business-Spotlight/Jollibee-s-quest-for-global-dominance-derailed-by-virus (accessed on 20 March 2022).
- Naik, C.K.; Gantasala, S.B.; Prabhakar, G.V. Service quality (SERVQUAL) and its effect on customer satisfaction in retailing. Eur. J. Soc. Sci. 2010 , 16 , 231–243. [ Google Scholar ]
- Nguyen, Q.; Nisar, T.M.; Knox, D.; Prabhakar, G.P. Understanding customer satisfaction in the UK Quick Service Restaurant Industry. Br. Food J. 2018 , 120 , 1207–1222. [ Google Scholar ] [ CrossRef ] [ Green Version ]
- Lee, Y.L.; Hing, N. Measuring Quality in Restaurant Operations: An application of the SERVQUAL instrument. Int. J. Hosp. Manag. 1995 , 14 , 293–310. [ Google Scholar ] [ CrossRef ]
- Ong, A.K.; Prasetyo, Y.T.; Picazo, K.L.; Salvador, K.A.; Miraja, B.A.; Kurata, Y.B.; Chuenyindee, T.; Nadlifatin, R.; Redi, A.A.; Young, M.N. Gym-goers preference analysis of fitness centers during the COVID-19 pandemic: A conjoint analysis approach for business sustainability. Sustainability 2021 , 13 , 10481. [ Google Scholar ] [ CrossRef ]
- Gimeno-Arias, F.; Santos-Jaén, J.M.; Palacios-Manzano, M.; Garza-Sánchez, H.H. Using PLS-SEM to analyze the effect of CSR on corporate performance: The mediating role of human resources management and customer satisfaction. an empirical study in the Spanish food and beverage manufacturing sector. Mathematics 2021 , 9 , 2973. [ Google Scholar ] [ CrossRef ]
- Bahta, D.; Yun, J.; Islam, M.R.; Bikanyi, K.J. How does CSR enhance the financial performance of smes? the mediating role of firm reputation. Econ. Res.-Ekon. Istraž. 2020 , 34 , 1428–1451. [ Google Scholar ] [ CrossRef ]
- Islam, T.; Islam, R.; Pitafi, A.H.; Xiaobei, L.; Rehmani, M.; Irfan, M.; Mubarak, M.S. The impact of corporate social responsibility on customer loyalty: The mediating role of corporate reputation, customer satisfaction, and Trust. Sustain. Prod. Consum. 2021 , 25 , 123–135. [ Google Scholar ] [ CrossRef ]
- Zeithaml, V.A.; Bitner, M.J.; Gremler, D.D.; Mende, M. Services Marketing: Integrating Customer Focus across the Firm ; McGraw Hill LLC: Dubuque, IA, USA, 2023. [ Google Scholar ]
- Omar, M.S.; Ariffin, H.F.; Ahmad, R. Service quality, customers’ satisfaction and the moderating effects of gender: A study of Arabic restaurants. Procedia Soc. Behav. Sci. 2016 , 224 , 384–392. [ Google Scholar ] [ CrossRef ] [ Green Version ]
- Vatolkina, N.; Gorbashko, E.; Kamynina, N.; Fedotkina, O. E-service quality from attributes to outcomes: The similarity and difference between digital and hybrid services. J. Open Innov. Technol. Mark. Complex. 2020 , 6 , 143. [ Google Scholar ] [ CrossRef ]
- Parasuraman, A.; Zeithaml, V.A.; Berry, L.L. A conceptual model of service quality and its implications for future research. J. Mark. 1985 , 49 , 41. [ Google Scholar ] [ CrossRef ]
- Iberahim, H.; Mohd Taufik, N.K.; Mohd Adzmir, A.S.; Saharuddin, H. Customer satisfaction on reliability and responsiveness of self service technology for retail banking services. Procedia Econ. Financ. 2016 , 37 , 13–20. [ Google Scholar ] [ CrossRef ] [ Green Version ]
- Grandey, A.A.; Goldberg, L.; Pugh, S.D. Employee satisfaction, responsiveness, and customer satisfaction: Linkages and boundary conditions. Acad. Manag. Proc. 2011 , 2011 , 1–6. [ Google Scholar ] [ CrossRef ]
- Chuenyindee, T.; Ong, A.K.; Ramos, J.P.; Prasetyo, Y.T.; Nadlifatin, R.; Kurata, Y.B.; Sittiwatethanasiri, T. Public Utility Vehicle Service Quality and customer satisfaction in the Philippines during the COVID-19 pandemic. Util. Policy 2022 , 75 , 101336. [ Google Scholar ] [ CrossRef ]
- Joon Choi, B.; Sik Kim, H. The impact of outcome quality, Interaction Quality, and peer-to-peer quality on customer satisfaction with a Hospital Service. Manag. Serv. Qual. Int. J. 2013 , 23 , 188–204. [ Google Scholar ] [ CrossRef ]
- Kalaja, R.; Myshketa, R.; Scalera, F. Service Quality Assessment in health care sector: The case of durres public hospital. Procedia-Soc. Behav. Sci. 2016 , 235 , 557–565. [ Google Scholar ] [ CrossRef ]
- Sahari, N.; Basir, N.M.; Jangga, R. Factors of food dimension affecting customer satisfaction in family restaurants. In Proceedings of the 3rd International Conference on Business and Economic Research, Bandung, Indonesia, 12–13 March 2012; pp. 2831–2846. [ Google Scholar ]
- Shen, X.X.; Tan, K.C.; Xie, M. An integrated approach to innovative product development using Kano’s model and QFD. Eur. J. Innov. Manag. 2000 , 3 , 91–99. [ Google Scholar ] [ CrossRef ]
- Kivela, J.; Inbakaran, R.; Reece, J. Consumer research in the restaurant environment, part 1: A conceptual model of dining satisfaction and return patronage. Int. J. Contemp. Hosp. Manag. 1999 , 11 , 205–222. [ Google Scholar ] [ CrossRef ]
- Yüksel, A.; Yüksel, F. Measurement of Tourist Satisfaction with Restaurant Services: A segment-based approach. J. Vacat. Mark. 2003 , 9 , 52–68. [ Google Scholar ] [ CrossRef ]
- Saad Andaleeb, S.; Conway, C. Customer satisfaction in the restaurant industry: An examination of the transaction-specific model. J. Serv. Mark. 2006 , 20 , 3–11. [ Google Scholar ] [ CrossRef ]
- Dai, B. The Impact of Perceived Price Fairness of Dynamic Pricing on Customer Satisfaction and Behavioral Intentions: The Moderating Role of Customer Loyalty ; Auburn University: Auburn, AL, USA, 2010. [ Google Scholar ]
- Sabir, R.I.; Ghafoor, O.; Hafeez, I.; Akhtar, N.; Rehman, A.U. Factors affecting customers satisfaction in restaurants industry in Pakistan. Int. Rev. Manag. Bus. Res. 2014 , 3 , 869. [ Google Scholar ]
- Gustafsson, A.; Johnson, M.D.; Roos, I. The effects of customer satisfaction, Relationship Commitment Dimensions, and triggers on customer retention. J. Mark. 2005 , 69 , 210–218. [ Google Scholar ] [ CrossRef ] [ Green Version ]
- Sulek, J.M.; Hensley, R.L. The relative importance of food, atmosphere, and fairness of wait. Cornell Hotel. Restaur. Adm. Q. 2004 , 45 , 235–247. [ Google Scholar ] [ CrossRef ] [ Green Version ]
- Rozekhi, N.A.; Hussin, S.; Siddiqe, A.S.K.A.R.; Rashid, P.D.A.; Salmi, N.S. The influence of food quality on customer satisfaction in fine dining restaurant: Case in Penang. Int. Acad. Res. J. Bus. Technol. 2016 , 2 , 45–50. [ Google Scholar ]
- Peri, C. The Universe of Food Quality. Food Qual. Prefer. 2006 , 17 , 3–8. [ Google Scholar ] [ CrossRef ]
- Al-Tit, A.A. The effect of service and food quality on customer satisfaction and hence customer retention. Asian Soc. Sci. 2015 , 11 , 129. [ Google Scholar ] [ CrossRef ]
- Intelligence, N. Impact of Coronavirus (COVID-19) on Consumer Behavior in 2020. Available online: https://www.numerator.com/resources/blog/impact-covid-19-consumer-behavior (accessed on 16 October 2021).
- The Impact of COVID-19 on Restaurant Consumers. Available online: https://www.revenuemanage.com/en/insights/the-impact-of-covid-19-on-restaurant-consumers/ (accessed on 16 October 2021).
- Nayeem, T. Cultural influences on consumer behaviour. Int. J. Bus. Manag. 2012 , 7 , 78. [ Google Scholar ] [ CrossRef ] [ Green Version ]
- German, J.D.; Redi, A.A.; Prasetyo, Y.T.; Persada, S.F.; Ong, A.K.; Young, M.N.; Nadlifatin, R. Choosing a package carrier during COVID-19 pandemic: An integration of pro-environmental planned behavior (PEPB) theory and Service Quality (SERVQUAL). J. Clean. Prod. 2022 , 346 , 131123. [ Google Scholar ] [ CrossRef ]
- Gumasing, M.J.; Prasetyo, Y.T.; Ong, A.K.; Nadlifatin, R. Determination of factors affecting the response efficacy of Filipinos under Typhoon Conson 2021 (jolina): An extended protection motivation theory approach. Int. J. Disaster Risk Reduct. 2022 , 70 , 102759. [ Google Scholar ] [ CrossRef ]
- De Mooij, M. Global Marketing and Advertising: Understanding Cultural Paradoxes , 3rd ed.; Sage Publications Asia-Pacific: Singapore, 2010. [ Google Scholar ]
- Main Page. Available online: https://is.theorizeit.org/wiki/Main_Page (accessed on 2 February 2022).
- Mueller, R.O.; Hancock, G.R. Structural Equation Modeling ; Routledge/Taylor & Francis Group: London, UK, 2019. [ Google Scholar ]
- Hair, J.F.; Black, W.C.; Babin, B.J.; Anderson, R.E.B. Multivariate Data Analysis , 8th ed.; Cengage: Hampshire, UK, 2019; pp. 146–157, 675–680. [ Google Scholar ]
- Dash, G.; Paul, J. CB-SEM vs. PLS-SEM methods for research in Social Sciences and Technology forecasting. Technol. Forecast. Soc. Change 2021 , 173 , 121092. [ Google Scholar ] [ CrossRef ]
- Taber, K.S. The use of Cronbach’s alpha when developing and Reporting Research Instruments in science education. Res. Sci. Educ. 2017 , 48 , 1273–1296. [ Google Scholar ] [ CrossRef ] [ Green Version ]
- Lin, H.-F. A stage model of Knowledge Management: An empirical investigation of process and effectiveness. J. Inf. Sci. 2007 , 33 , 643–659. [ Google Scholar ] [ CrossRef ]
- Verhoef, P.C.; Franses, P.H.; Hoekstra, J.C. The effect of relational constructs on customer referrals and number of services purchased from a multiservice provider: Does age of relationship matter? J. Acad. Mark. Sci. 2002 , 30 , 202–216. [ Google Scholar ] [ CrossRef ] [ Green Version ]
- Yuduang, N.; Ong, A.K.; Prasetyo, Y.T.; Chuenyindee, T.; Kusonwattana, P.; Limpasart, W.; Sittiwatethanasiri, T.; Gumasing, M.J.; German, J.D.; Nadlifatin, R. Factors influencing the perceived effectiveness of COVID-19 risk assessment mobile application “Morchana” in Thailand: Utaut2 approach. Int. J. Environ. Res. Public Health 2022 , 19 , 5643. [ Google Scholar ] [ CrossRef ]
- Gefen, D.; Straub, D.; Boudreau, M. Structural Equation Modeling and Regression: Guidelines for Research Practice. Commun. Assoc. Inf. Syst. 2000 , 4 , 7. [ Google Scholar ] [ CrossRef ] [ Green Version ]
- Almohaimmeed, B.M. Restaurant quality and customer satisfaction. Int. Rev. Manag. Mark. 2017 , 7 , 42–49. [ Google Scholar ]
- Tat, H.H.; Sook-Min, S.; Ai-Chin, T.; Rasli, A.; Hamid, A.B.A. Consumers’ purchase intentions in fast food restaurants: An empirical study on undergraduate students. Int. J. Bus. Soc. Sci. 2011 , 2 , 214–221. [ Google Scholar ]
- Qin, H.; Prybutok, V.R. Service quality, customer satisfaction, and behavioral intentions in fast-food restaurants. Int. J. Qual. Serv. Sci. 2009 , 1 , 78–95. [ Google Scholar ] [ CrossRef ]
- Namkung, Y.; Jang, S.C. Are highly satisfied restaurant customers really different? A quality perception perspective. Int. J. Contemp. Hosp. Manag. 2008 , 20 , 142–155. [ Google Scholar ] [ CrossRef ]
- Lau, T.; Cheung, M.L.; Pires, G.D.; Chan, C. Customer satisfaction with sommelier services of upscale Chinese restaurants in Hong Kong. Int. J. Wine Bus. Res. 2019 , 31 , 532–554. [ Google Scholar ] [ CrossRef ]
- Kincaid, C.; Baloglu, S.; Mao, Z.; Busser, J. What really brings them back? Int. J. Contemp. Hosp. Manag. 2010 , 22 , 209–220. [ Google Scholar ] [ CrossRef ]
- Khan, N.R.; Shaikh, U. Impact of service quality on customer satisfaction: Evidences from the restaurant industry in Pakistan. Manag. Mark. 2011 , 9 , 343–355. [ Google Scholar ]
- Lee, Y.-K.; Park, K.-H.; Park, D.-H.; Lee, K.A.; Kwon, Y.-J. The relative impact of service quality on service value, customer satisfaction, and customer loyalty in Korean family restaurant context. Int. J. Hosp. Tour. Adm. 2005 , 6 , 27–51. [ Google Scholar ] [ CrossRef ]
- Tan, Q.; Oriade, A.; Fallon, P. Service quality and customer satisfaction in Chinese fast food sector: A proposal for CFFRSERV. Adv. Hosp. Tour. Res. (AHTR) 2014 , 2 , 30–53. [ Google Scholar ]
- Moorthy, K.; En Chee, L.; Chuan Yi, O.; Soo Ying, O.; Yee Woen, O.; Mun Wei, T. Customer loyalty to newly opened cafés and restaurants in Malaysia. J. Foodserv. Bus. Res. 2016 , 20 , 525–541. [ Google Scholar ] [ CrossRef ]
- Mensah, I.; Mensah, R.D. Effects of service quality and customer satisfaction on repurchase intention in restaurants on University of Cape Coast campus. J. Tour. Herit. Serv. Mark. 2018 , 4 , 27–36. [ Google Scholar ]
- Jawabreh, O.; Al Jaffal, T.; Abdelrazaq, H.; Mahmoud, R. The impact of menus on the customer satisfaction in restaurants classified in Aqaba special economic zone authority (ASEZA). J. Tour. Hosp. Sport. 2018 , 33 , 29–39. [ Google Scholar ]
- Ryu, K.; Han, H. Influence of the quality of food, service, and physical environment on customer satisfaction and behavioral intention in quick-casual restaurants: Moderating role of perceived price. J. Hosp. Tour. Res. 2009 , 34 , 310–329. [ Google Scholar ] [ CrossRef ]
- Law, A.K.Y.; Hui, Y.V.; Zhao, X. Modeling repurchase frequency and customer satisfaction for fast food outlets. Int. J. Qual. Reliab. Manag. 2004 , 21 , 545–563. [ Google Scholar ] [ CrossRef ]
- Jang, S.C.; Ha, J. The influence of cultural experience: Emotions in relation to authenticity at ethnic restaurants. J. Foodserv. Bus. Res. 2015 , 18 , 287–306. [ Google Scholar ] [ CrossRef ]
- Almendral, A. Jollibee: A Taste of Home for Filipinos. Available online: https://theworld.org/stories/2012-10-26/jollibee-taste-home-filipinos (accessed on 20 March 2022).
Click here to enlarge figure
Characteristics | Category | Frequency | Percentage (%) |
---|---|---|---|
Age | 15 to 20 years old | 31 | 10.20 |
21 to 26 years old | 173 | 57.09 | |
27 to 33 years old | 51 | 16.83 | |
34 to 40 years old | 25 | 8.250 | |
41 to 46 years old | 10 | 3.30 | |
47 to 53 years old | 10 | 3.30 | |
54 years old and above | 3 | 1.030 | |
Frequency of Eating in Jollibee | Once a week | 87 | 28.71 |
Twice a week | 67 | 22.11 | |
Thrice a week | 18 | 5.940 | |
4 times a week | 13 | 4.290 | |
Once a month | 118 | 38.95 | |
Occupation | Student | 64 | 21.12 |
Unemployed | 34 | 11.22 | |
Employed | 185 | 61.06 | |
Other | 20 | 6.60 | |
Number of Children | 0 | 233 | 76.90 |
1 | 38 | 12.54 | |
2 | 15 | 4.950 | |
3 | 11 | 3.630 | |
4 and above | 6 | 1.980 | |
Monthly Income | ₱11,999 and below | 87 | 28.71 |
₱12,000 to ₱20,999 | 78 | 25.74 | |
₱21,000 to ₱40,999 | 93 | 30.69 | |
₱41,000 to ₱60,999 | 21 | 6.930 | |
₱61,000 to ₱80,999 | 11 | 3.630 | |
₱81,000 and above | 13 | 4.290 | |
Customers Who Eat because of Discount | Yes | 57 | 18.81 |
No | 146 | 48.18 | |
Sometimes | 100 | 33.00 | |
Customers Who Eat because of permanent discount | Yes | 31 | 10.23 |
No | 272 | 89.77 |
Hypothesis | Preliminary Model | Final Model | |||
---|---|---|---|---|---|
Effect (β) | p-Value | Effect (β) | p-Value | ||
1 | A → SQ | 0.173 | 0.153 | - | - |
2 | T → SQ | 0.355 | 0.000 | 0.359 | 0.002 |
3 | REL → SQ | 0.155 | 0.186 | - | - |
4 | RES → SQ | 0.151 | 0.195 | - | - |
5 | E → SQ | 0.552 | 0.000 | 0.676 | 0.002 |
6 | SQ → C | 0.281 | 0.001 | 0.319 | 0.001 |
7 | CP → C | 0.327 | 0.002 | 0.245 | 0.019 |
8 | FQ → C | 0.371 | 0.000 | 0.265 | 0.004 |
9 | P → C | 0.221 | 0.019 | 0.209 | 0.018 |
10 | CI → C | 0.389 | 0.001 | 0.282 | 0.001 |
Latent Variables | Items | Cronbach’s α | Factor Loadings | Average Variance Extracted (AVE) | Composite Reliability (Re) | Variance Inflation Factor (VIF) |
---|---|---|---|---|---|---|
A | A1 | 0.876 | 0.76 | 0.65 | 0.879 | 4.990 |
A2 | 0.74 | |||||
A3 | 0.84 | |||||
A4 | 0.84 | |||||
T | T1 | 0.864 | 0.67 | 0.57 | 0.869 | 3.480 |
T2 | 0.76 | |||||
T3 | 0.79 | |||||
T4 | 0.78 | |||||
T6 | 0.84 | |||||
REL | REL1 | 0.825 | 0.79 | 0.55 | 0.827 | 3.829 |
REL2 | 0.74 | |||||
REL3 | 0.68 | |||||
REL4 | 0.73 | |||||
E | E1 | 0.935 | 0.81 | 0.73 | 0.930 | 4.209 |
E2 | 0.84 | |||||
E3 | 0.89 | |||||
E4 | 0.85 | |||||
E5 | 0.86 | |||||
CP | CP1 | 0.875 | 0.65 | 0.48 | 0.878 | 2.673 |
CP3 | 0.56 | |||||
CP4 | 0.62 | |||||
CP5 | 0.69 | |||||
CP6 | 0.78 | |||||
CP7 | 0.82 | |||||
CP8 | 0.81 | |||||
CP9 | 0.51 | |||||
FQ | FQ1 | 0.853 | 0.75 | 0.46 | 0.834 | 2.318 |
FQ2 | 0.72 | |||||
FQ3 | 0.58 | |||||
FQ4 | 0.68 | |||||
FQ5 | 0.66 | |||||
FQ6 | 0.84 | |||||
CI | CI1 | 0.834 | 0.53 | 0.53 | 0.842 | 1.582 |
CI2 | 0.82 | |||||
CI3 | 0.90 | |||||
CI4 | 0.78 | |||||
CI5 | 0.51 | |||||
P | P1 | 0.900 | 0.77 | 0.63 | 0.897 | 1.995 |
P2 | 0.80 | |||||
P3 | 0.79 | |||||
P4 | 0.80 | |||||
P5 | 0.84 | |||||
SQ | SQ1 | 0.927 | 0.77 | 0.56 | 0.864 | 4.467 |
SQ2 | 0.90 | |||||
SQ3 | 0.82 | |||||
SQ4 | 0.88 | |||||
SQ5 | 0.90 | |||||
C | C1 | 0.914 | 0.78 | 0.50 | 0.831 | - |
C2 | 0.87 | |||||
C3 | 0.72 | |||||
C4 | 0.71 | |||||
C5 | 0.80 |
Goodness of Fit Measures of the SEM | Parameter Estimates | Minimum Cut-Off | Recommended By |
---|---|---|---|
Goodness of Fit Index (GFI) | 0.802 | >0.80 | [ ] |
Adjusted Goodness of Fit Index (AGFI) | 0.811 | >0.80 | [ ] |
Root Mean Square Error of Approximation (RMSEA) | 0.065 | <0.07 | [ ] |
Incremental Fit Index (IFI) | 0.886 | >0.80 | [ ] |
Tucker Lewis Index (TLI) | 0.873 | >0.80 | [ ] |
Comparative Fit Index (CFI) | 0.885 | >0.80 | [ ] |
MDPI stays neutral with regard to jurisdictional claims in published maps and institutional affiliations. |
Share and Cite
Ong, A.K.S.; Prasetyo, Y.T.; Mariñas, K.A.; Perez, J.P.A.; Persada, S.F.; Nadlifatin, R.; Chuenyindee, T.; Buaphiban, T. Factors Affecting Customer Satisfaction in Fast Food Restaurant “Jollibee” during the COVID-19 Pandemic. Sustainability 2022 , 14 , 15477. https://doi.org/10.3390/su142215477
Ong AKS, Prasetyo YT, Mariñas KA, Perez JPA, Persada SF, Nadlifatin R, Chuenyindee T, Buaphiban T. Factors Affecting Customer Satisfaction in Fast Food Restaurant “Jollibee” during the COVID-19 Pandemic. Sustainability . 2022; 14(22):15477. https://doi.org/10.3390/su142215477
Ong, Ardvin Kester S., Yogi Tri Prasetyo, Klint Allen Mariñas, Jehorom Px Alegre Perez, Satria Fadil Persada, Reny Nadlifatin, Thanatorn Chuenyindee, and Thapanat Buaphiban. 2022. "Factors Affecting Customer Satisfaction in Fast Food Restaurant “Jollibee” during the COVID-19 Pandemic" Sustainability 14, no. 22: 15477. https://doi.org/10.3390/su142215477
Article Metrics
Article access statistics, further information, mdpi initiatives, follow mdpi.
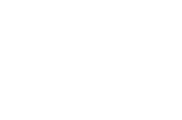
Subscribe to receive issue release notifications and newsletters from MDPI journals
- Open access
- Published: 04 June 2020
Satisfaction and revisit intentions at fast food restaurants
- Amer Rajput 1 &
- Raja Zohaib Gahfoor 2
Future Business Journal volume 6 , Article number: 13 ( 2020 ) Cite this article
186k Accesses
70 Citations
10 Altmetric
Metrics details
This study is to identify the positive association of food quality, restaurant service quality, physical environment quality, and customer satisfaction with revisit intention of customers at fast food restaurants. Additionally, word of mouth is investigated as moderator on the relationship of customer satisfaction with revisit intentions of customers at fast food restaurants. Data were collected through a questionnaire survey from 433 customers of fast food restaurants through convenience sampling. Hypotheses of proposed model were tested using structural equation modeling with partial least squares SEM-PLS in SMART PLS 3. The results confirmed the positive association of food quality, restaurant service quality, physical environment quality, and customer satisfaction with revisit intentions of customers at fast food restaurants. However, word of mouth does not positively moderate the relationship of customer satisfaction with revisit intentions of customers at fast food restaurants. This study emphasizes the importance of revisit intention as a vital behavioral reaction in fast food restaurants. This study reveals revisit intention’s positive association with food quality, restaurant service quality, physical environment quality, and customer satisfaction based on stimulus-organism-response (S-O-R) theory. Furthermore, it is identified that social conformity theory does not hold its assumption when consumers experience quality and they are satisfied because word of mouth does not moderate the relationship of customer satisfaction with revisit intention of customer.
Introduction
Background of the study.
Hospitality industry is observing diversified changes in highly competitive environment for restaurants [ 1 ]. Consumers are becoming conscious of food quality (FQ), restaurant service quality (RSQ), and physical environment quality (PEQ) of the fast food restaurants. Consumers switch easily in case of just one evasive experience [ 2 , 3 ]. Fast food restaurants must attract new customers and retain the existing customers. There is a growing trend in Pakistani culture to dine out at fast food restaurants with family, friends, and colleagues [ 4 ]. Restaurants focus to provide a dining experience by combining tangible and intangible essentials [ 5 ]. Decisive objective is to achieve customer satisfaction (CS), word of mouth (WOM), and future revisit intention (RVI) at fast food restaurant.
Restaurants differ in offerings, appearance, service models, and cuisines; this classifies restaurants as downscale and upscale [ 6 , 7 ]. Revisit intention is the willingness of a consumer to revisit a place due to satisfactory experience. Customer satisfaction generates a probability to revisit in presence or absence of an affirmative attitude toward the restaurant [ 8 ]. Revisit intention is a substantial topic in hospitality research [ 8 , 9 , 10 ]. To date there has been little agreement on that word of mouth can affect revisit intention after experience of customer satisfaction. For instance, when a customer is satisfied at a fast food restaurant experience, however, the customer’s family and friends do not share the same satisfying experience. Will this word of mouth affect the customer’s revisit intention? Food quality is acknowledged as a basic component of the restaurant’s overall experience to affect consumer revisit intention. Fast food quality is substantially associated with customer satisfaction and it is an important predictor of behavioral intention [ 11 ]. Service quality is an essential factor to produce consumers’ revisit intentions [ 12 ]. Furthermore, physical environment quality affects behavior of consumers at restaurants, hotels, hospitals, retail stores, and banks [ 13 ]. Physical environment quality is a precursor of customer satisfaction [ 9 ]. This suggests that customer satisfaction is associated with fast food quality, restaurant service quality, physical environment quality, and revisit intention.
Aims of the study
This study is to investigate the association of fast food quality, restaurant service quality, physical environment quality with customer’s revisit intention through mediation of customer satisfaction using S-O-R theory and moderation of word of mouth on the relationship of customer satisfaction with revisit intention based on social conformity theory. This study empirically tests a conceptual research framework based on S-O-R and social conformity theory adding value to the knowledge. Objectives of the study are given below.
To investigate the association of fast food quality, restaurant service quality, and physical environment quality with revisit intention through customer satisfaction based on S-O-R theory in the context of Pakistani fast food restaurants.
To investigate moderation of WOM on relationship of customer satisfaction with revisit intention based on social conformity theory in the context of Pakistani fast food restaurants.
Furthermore, little empirical evidence is present about customer satisfaction with respect to fast food restaurant service quality [ 14 ]. Customer satisfaction is a post-consumption assessment in service industry. Customer satisfaction acts as the feedback mechanism to boost consumer experience [ 15 ]. Customer satisfaction brings competitive advantage to the firm and produces positive behavioral revisit intention [ 16 ]. Marketing literature emphasizes customer satisfaction in anticipation of positive word of mouth, revisit intention, and revisit behavior [ 5 ]. Behavioral intention is assessed through positive WOM, and it is important in service industry [ 15 ], whereas social influence in shape of WOM affects the behavior of individuals toward conformity leading to a driving effect based on social conformity theory [ 17 ].
- Food quality
Food quality plays a central role in the restaurant industry. Food quality is essential to satisfy consumer needs. Food quality is a substantial condition to fulfill the needs and expectations of the consumer [ 18 ]. Food quality is acknowledged as a basic component of the restaurant’s overall experience. Food quality is a restaurant selection’s most important factor, and it is considerably related to customer satisfaction [ 11 ]. Food quality affects customer loyalty, and customer assesses the restaurant on the basis of food quality [ 19 ]. Food quality entails food taste, presentation, temperature, freshness, nutrition, and menu variety. Food quality influences customers’ decisions to revisit the restaurant [ 20 ]. Academic curiosity is increasing in the restaurant’s menus, as variety of menu items is considered the critical characteristic of food quality [ 11 ]. Taste is sensual characteristic of food. Taste is assessed after consumption. Nonetheless, customers foresee taste before consumption through price, quality, food labels, and brand name. Taste of food is important to accomplish customer satisfaction. Presentation of food enhances dining customer satisfaction [ 21 , 22 ]. Customer’s concerns of healthy food substantially affect customer’s expectations and choice of a restaurant [ 23 ]. Freshness is assessed with the aroma, juiciness, crispness, and fresh posture of the food. Food quality enhances customer satisfaction [ 24 ].
- Restaurant service quality
Quality as a construct is projected by Juran and Deming [ 25 , 26 ]. Service quality is comparatively a contemporary concept. Service quality assesses the excellence of brands in industry of travel, retail, hotel, airline, and restaurant [ 27 ]. Restaurant service quality affects dining experiences of customers. Service quality creates first impression on consumers and affects consumers’ perception of quality [ 28 ]. Service industry provides good service quality to the customers to attain sustainable competitive advantage. Customer satisfaction depends on quality of service at the restaurant [ 29 ]. Service quality entails price, friendliness, cleanliness, care, diversity, speed of service, and food consistency according to menu. Customer satisfaction also depends on communication between restaurant’s personnel and the customers [ 30 ]. Consumer’s evaluation of service quality is affected by level of friendliness and care. Service quality leads to positive word of mouth, customer satisfaction, better corporate image, attraction for the new customers, increase revisits, and amplified business performance. Service quality increases revisits and behavioral intentions of customers in hospitality industry [ 12 ].
- Physical environment quality
PEQ is a setting to provide products and services in a restaurant. Physical environment quality contains artifacts, decor, spatial layout, and ambient conditions in a restaurant. Customers desire dining experience to be pleasing; thus, they look for a physical environment quality [ 31 ]. Physical environment quality satisfies and attracts new customers. PEQ increases financial performance, and it creates memorable experience for the customers [ 9 ]. Consumers perceive the quality of a restaurant based on cleanliness, quirky, comfortable welcoming, physical environment quality, and other amenities that create the ambiance [ 32 ]. Effect of physical environment quality on behaviors is visible in service businesses such as restaurants, hotels, hospitals, retail stores, and banks [ 33 ]. Physical environment quality is an antecedent of customer satisfaction [ 34 ]. Thus, restaurants need to create attractive and distinctive physical environment quality.
- Customer satisfaction
Customer satisfaction contains the feelings of pleasure and well-being. Customer satisfaction develops from gaining what customer expects from the service. Customer satisfaction is broadly investigated in consumer behavior and social psychology. Customer satisfaction is described “as the customer’s subjective assessment of the consumption experience, grounded on certain associations between the perceptions of customer and objective characteristics of the product” [ 35 ]. Customer satisfaction is the extent to which an experience of consumption brings good feelings. Customer satisfaction is stated as “a comparison of the level of product or service performance, quality, or other outcomes perceived by the consumer with an evaluative standard” [ 36 ]. Customer satisfaction constructs as a customer’s wholesome evaluation of an experience. Customer satisfaction is a reaction of fulfilling customer’s needs.
Customer satisfaction brings escalated repeat purchase behavior and intention to refer [ 37 ]. Dissatisfied consumers are uncertain to return to the place [ 38 ]. Satisfactory restaurant experience can enhance revisit intention of the consumer. Positive WOM is generated when customers are not only satisfied with the brand but they demand superior core offering and high level of service [ 15 ].
- Word of mouth
Word of mouth is described as “person-to-person, oral communication between a communicator and receiver which is perceived as a non-commercial message” [ 39 ]. WOM is also defined as “the informal positive or negative communication by customers on the objectively existing and/or subjectively perceived characteristics of the products or services” [ 40 ]. Moreover, [ 41 ] defines it as “an informal person to person communication between a perceived non-commercial communicator and a receiver regarding a brand, a product, an organization or a service”. WOM is described as a positive or negative statement made by probable, actual or former customers about a product or a company, which is made available through offline or online channels [ 42 , 43 ]. WOM is an important and frequent sensation; it is known for long time that people habitually exchange their experiences of consumptions with others. Consumers complain about bad hotel stays, talk about new shoes, share info about the finest way of getting out tough stains, spread word about experience of products, services, companies, restaurants, and stores. Social talks made more than 3.3 billion of brand impressions per day [ 44 ].
WOM has substantial impact on consumer’s purchasing decision; therefore, a vital marketing strategy is to initiate positive WOM [ 45 ]. However, negative WOM is more informative and diagnostic where customers express their dissatisfaction [ 38 ]. Word of mouth communications are more informative than traditional marketing communications in service sector. WOM is more credible than advertisement when it is from friends and family [ 46 ]. WOM is a vital influencer in purchase intention. WOM escalates affection that enhances commitment of consumer purchase intention. WOM is generated before or after the purchase. WOM helps the consumers to acquire more knowledge for the product and to reduce the perceived risk [ 47 ]. WOM in the dining experience is very important. People tend to follow their peers’ opinions when they are to dine out.
- Revisit intention
To predicting and to explain human behavior is the key determination of consumer behavior research. Consumer needs differ and emerge frequently with diverse outlooks. Revisit intention is to endorse “visitors being willing to revisit the similar place, for satisfactory experiences, and suggest the place to friends to develop the loyalty” [ 48 ]. Consumer forms an attitude toward the service provider based on the experience of service. This attitude can be steady dislike or like of the service. This is linked to the consumer’s intention to re-patronize the service and to start WOM. Repurchase intention is at the core of customer loyalty and commitment. Repurchase intention is a significant part of behavioral and attitudinal constructs. Revisit intention is described as optimistic probability to revisit the restaurant. Revisit intention is the willingness of a consumer to visit the restaurant again. Furthermore, the ease of visitors, transportation in destination, entertainment, hospitability, and service satisfaction influence visitor’s revisit intention.
Consumer behavior encircles the upcoming behavioral intention and post-visit evaluation. Post-visit evaluation covers perceived quality, experience, value, and the satisfaction. Restaurant managers are interested to understand the factors of consumer revisit intention, as it is cost effective to retain the existing customers in comparison with attract new customers [ 49 ]. Substantial consideration is prevailing in literature for the relationship among quality attributes, customer satisfaction, and revisit intention. There is a positive association between customer satisfaction and revisit intention. Indifferent consumer, accessibility of competitive alternatives and low switching cost can end up in a state where satisfied consumers defect to other options [ 2 ]. Consumer behavior varies for choice of place to visit, assessments, and behavioral intentions [ 50 ]. The assessments are about the significance perceived by regular customers’ satisfactions. Whereas, future behavioral intentions point to the consumer’s willingness to revisit the similar place and suggest it to the others [ 51 ].
S-O-R model is primarily established on the traditional stimulus–response theory. This theory explicates individual’s behavior as learned response to external stimuli. The theory is questioned for oversimplifying ancestries of the behaviors and ignoring one’s mental state. [ 52 ] extended the S-O-R model through integrating the notion of organism between stimulus and response. S-O-R concept is embraced to reveal individual’s affective and cognitive conditions before the response behavior [ 53 ]. S-O-R framework considers that environment comprises stimuli (S) leading changes to the individual’s internal conditions called organism (O), further leading to responses (R) [ 52 ]. In S-O-R model, the stimuli comprise of various components of physical environment quality, organism indicates to internal structures and processes bridging between stimuli and final responses or actions of a consumer [ 9 ]. Behavioral responses of an individual in a physical environment quality are directly influenced by the physical environment quality stimulus [ 54 ]. S-O-R framework is implemented in diverse service contexts to examine how physical environment quality affects customer’s emotion and behavior [ 55 ]. The effect of stimulation in an online shopping environment on impulsive purchase is investigated through S-O-R framework [ 56 ]. The effects of background music, on consumers’ affect and cognition, and psychological responses influence behavioral intentions [ 57 ]. Perceived flow and website quality toward customer satisfaction affect purchase intention in hotel website based on S-O-R framework [ 58 ]. Therefore, this study conceptualizes food quality, restaurant service quality, and physical environment quality as stimuli; customer satisfaction as organism; and revisit intention as response.
Moreover, social conformity theory (SCT) is to support the logical presence of WOM in the conceptual framework as a moderator on the relationship of customer satisfaction and revisit intention. Social conformity influences individual’s attitudes, beliefs and behaviors leading to a herding effect [ 17 , 59 ]. Thus, social influence (WOM) moderates the relationship of customer satisfaction and revisit intention. Following hypotheses are postulated, see Fig. 1 .
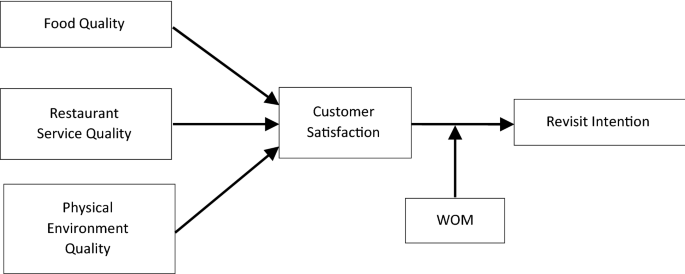
Conceptual research framework
Food quality is positively associated with customer satisfaction in fast food restaurant.
Restaurant service quality is positively associated with customer satisfaction in fast food restaurant.
Physical environment quality is positively associated with customer satisfaction in fast food restaurant.
Customer satisfaction is positively associated with revisit intention of customer in fast food restaurant.
Customer satisfaction mediates between food quality and revisit intention of customer in fast food restaurant.
Customer satisfaction mediates between restaurant service quality and revisit intention of customer in fast food restaurant.
Customer satisfaction mediates between physical environment quality and revisit intention of customer in fast food restaurant.
WOM positively moderates the relationship between customer satisfaction and revisit intention of customer in fast food restaurant.
There are two research approaches such as deductive (quantitative) and inductive (qualitative). This study utilized the quantitative research approach as it aligns with the research design and philosophy. Quantitative research approach mostly relies on deductive logic. Researcher begins with hypotheses development and then collects data. Data are used to determine whether empirical evidence supports the hypotheses [ 60 ]. The questionnaires survey is used. This study chose the mono-method with cross-sectional time horizon of 6 months. Deductive approach is utilized in this study. Cross-sectional time horizon also known as “snapshot” is used when investigation is related with the study of a specific phenomenon at a particular time [ 61 ]. Questionnaire survey is mostly used technique for data collection in marketing research due to its effectiveness and low cost [ 62 ]. Data are collected through self-administered questionnaires. Following the footsteps of Lai and Chen [ 63 ] and Widianti et al. [ 64 ] convenience sampling is applied. Famous fast food restaurants in twin cities (Rawalpindi and Islamabad) of Pakistan were chosen randomly. Furthermore, 650 questionnaires (with consideration of low response rate) were distributed to the customers at famous fast food restaurants. Moreover, researchers faced difficulty in obtaining fast food restaurant’s consumers data.
It yielded a response rate of 68.92% with 448 returned questionnaires. Fifteen incomplete questionnaires are not included; thus, 433 responses are employed for data analysis from fast food restaurant customers. The obtained number of usable responses was suitable to apply structural equation modeling [ 65 , 66 , 67 , 68 ].
Sample characteristics describe that there are 39.7% females and 60.3% males. There are 31.4% respondents of age group 15–25 years, 48.3% of age group 26–35, 12.2% of age ranges between 36 and 45, 6.7% of age ranges between 46 and 55, and 1.4% of age group is above 56 years. The educational level of the respondents indicates that mostly respondents are undergraduate and graduate. Occupation of respondents reflects that 28.6% work in private organizations and 24.9% belong to student category. Monthly income of 29.3% respondents ranges between Rupees 20,000 and 30,000 and 25.6% have monthly income of Rupees 41,000–50,000. Average monthly spending in fast food restaurants is about Rupees 3000–6000, see Table 1 .
Measures of the constructs
Food quality is adopted from measures developed by [ 69 ]. Food quality contains six items such as: food presentation is visually attractive, the restaurant offers a variety of menu items, and the restaurant offers healthy options. Restaurant service quality is adopted with six items [ 70 ]. This construct contains items such as: efficient and effective process in the welcoming and ushering of the customers, efficient and effective explanation of the menu, efficient and effective process in delivery of food. Physical environment quality is adopted with four items [ 71 ], and one item is adopted from measures developed by [ 70 ]. The items are such as: the restaurant has visually striking building exteriors and parking space, the restaurant has visually eye-catching dining space that is comfortable and easy to move around and within, and the restaurant has suitable music and/or illumination in accordance with its ambience. Revisit intention is measured through four adapted items [ 8 ]; such as: I would visit again in the near future and I am interested in revisiting again. Customer satisfaction is measured by three adopted items [ 29 ]; such as: I am satisfied with the service at this restaurant, and the restaurant always comes up to my expectations. Word of mouth is measured with four adopted items such as: my family/friends mentioned positive things I had not considered about this restaurant, my family/friends provided me with positive ideas about this restaurant [ 72 ]. Each item is measured on 5-point Likert scale, where 1 = strongly disagree, 3 = uncertain, and 5 = strongly agree.
Results and discussion
Validity and reliability.
Validity taps the ability of the scale to measure the construct; in other words, it means that the representative items measure the concept adequately [ 73 ]. The content validity is executed in two steps; firstly, the items are presented to the experts for further modifications; secondly, the constructive feedback about understanding of it was acquired by few respondents who filled the questionnaires. Each set of items is a valid indicator of the construct as within-scale factor analysis is conducted.
The factor analyses allotted the items to their respective factor. Fornell and Lacker’s [ 74 ] composite reliability p is calculated for each construct using partial least squares (PLS) structural equation modeling and Cronbach’s coefficient α [ 75 ]. Cronbach’s α is used to evaluate the reliability of all items that indicates how well the items in a set are positively related to one another. Each Cronbach’s α of the instrument is higher than .7 (ranging from .74 to .91); see Table 2 .
Common method bias
Same measures are used to collect data for all respondents; thus, there can be common method bias [ 76 ]. Firstly, questionnaire is systematically constructed with consideration of study design. Secondly, respondents were assured for the responses to be kept anonymous [ 77 ]. Common method bias possibility is assessed through Harman’s single factor test [ 78 , 79 , 80 , 81 , 82 , 83 ]. Principal axis factor analysis on measurement items is exercised. The single factor did not account for most of the bias and it accounted for 43.82% variance that is less than 50%. Thus, common method bias is not an issue [ 80 , 81 ].
SEM-PLS model assessment
Survey research faces a challenge to select an appropriate statistical model to analyze data. Partial least squares grounded structural equation modeling (SEM-PLS) and covariance-based structural equation modeling (CB-SEM) are generally used multivariate data analysis methods. CB-SEM is based on factor analysis that uses maximum likelihood estimation. PLS-SEM is based on the principal component concept; it uses the partial least squares estimator [ 84 ]. PLS-SEM is considered appropriate to examine complex cause–effect relationship models. PLS-SEM is a nonparametric approach with low reservations on data distribution and sample size [ 84 ].
Measurement model assessment
To evaluate convergent validity measurement model (outer model) is assessed that includes composite reliability (CR) to evaluate internal consistency, individual indicator reliability, and average variance extracted (AVE) [ 85 ]. Indicator reliability explains the variation in the items by a variable. Outer loadings assess indicator reliability; a higher value (an item with a loading of .70) on a variable indicates that the associated measure has considerable mutual commonality [ 85 ]. Two items RSQ 14 and PEQ 24 are dropped due to lower value less than .60 [ 86 ]. Composite reliability is assessed through internal consistency reliability. CR values of all the latent variables have higher values than .80 to establish internal consistency [ 85 ]; see Table 2 .
Convergent validity is the extent to which a measure correlates positively with alternative measures of the same variable. Convergent validity is ensured through higher values than .50 of AVE [ 74 ], see Table 2 . Discriminant validity is the degree to which a variable is truly distinct from other variables. Square root of AVE is higher than the inter-construct correlations except customer satisfaction to hold discriminant validity [ 74 ]. Additional evidence for discriminant validity is that indicators’ individual loadings are found to be higher than the respective cross-loadings, see Table 3 .
Structural model assessment
Structural model is assessed after establishing the validity and reliability of the variables. Structural model assessment includes path coefficients to calculate the importance and relevance of structural model associations. Model’s predictive accuracy is calculated through R 2 value. Model’s predictive relevance is assessed with Q 2 , and value of f 2 indicates substantial impact of the exogenous variable on an endogenous variable in PLS-SEM [ 85 ]. SEM is rigueur in validating instruments and testing linkages between constructs [ 87 ]. SMART-PLS produces reports of latent constructs correlations, path coefficients with t test values. The relationships between six constructs of food quality, restaurant service quality, physical environment quality, customer satisfaction, word-of-mouth, and revisit intention are displayed in Fig. 2 after bootstrapping. Bootstrapping is a re-sampling approach that draws random samples (with replacements) from the data and uses these samples to estimate the path model multiple times under slightly changed data constellations [ 88 ]. Purpose of bootstrapping is to compute the standard error of coefficient estimates in order to examine the coefficient’s statistical significance [ 89 ].
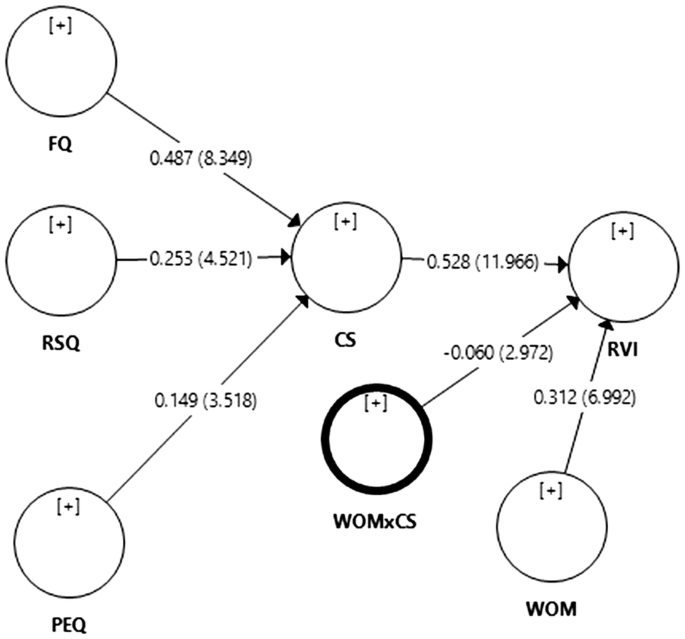
Bootstrapping and path coefficients
Food quality is positively associated to customer satisfaction in fast food restaurant; H 1 is supported as path coefficient = .487, T value = 8.349, P value = .000. Restaurant service quality is positively associated with customer satisfaction; H 2 is supported as path coefficient = .253, T value = 4.521, P value = .000. Physical environment quality is positively associated with customer satisfaction in fast food restaurant; H 3 is supported as path coefficient = .149, T value = 3.518, P value = .000. Customer satisfaction is positively associated with revisit intention of customer in fast food restaurant; H 4 is supported as path coefficient = .528, T value = 11.966, P value = .000. WOM positively moderates the relationship between customer satisfaction and revisit intention of customer in fast food restaurant; H 8 is not supported as path coefficient = − .060, T value = 2.972, P value = .003; see Table 4 .
Assessing R 2 and Q 2
Coefficient of determination R 2 value is used to evaluate the structural model. This coefficient estimates the predictive precision of the model and is deliberated as the squared correlation between actual and predictive values of the endogenous construct. R 2 values represent the exogenous variables’ mutual effects on the endogenous variables. This signifies the amount of variance in endogenous constructs explained by total number of exogenous constructs associated to it [ 88 ]. The endogenous variables customer satisfaction and revisit intention have R 2 = .645 and .671, respectively, that assures the predictive relevance of structural model. Further the examination of the endogenous variables’ predictive power has good R 2 values.
Blindfolding is to cross-validate the model’s predictive relevance for each of the individual endogenous variables with value of Stone–Geisser Q 2 [ 90 , 91 ]. By performing the blindfolding test with an omission distance of 7 yielded cross-validated redundancy Q 2 values of all the endogenous variables [ 88 ]. Customer satisfaction’s Q 2 = .457 and RVI’s Q 2 = .501; this indicates large effect sizes. PLS structural model has predictive relevance because values of Q 2 are greater than 0, see Table 5 .
Assessing f 2
Effect size f 2 is the measure to estimate the change in R 2 value when an exogenous variable is omitted from the model. f 2 size effect illustrates the influence of a specific predictor latent variable on an endogenous variable. Effect size f 2 varies from small to medium for all the exogenous variables in explaining CS and RVI as shown Table 6 .
Additionally, H 5 : CS mediates between food quality and RVI is supported as CS partially mediates between FQ and RVI. Variation accounted for (VAF) value indicates that 70% of the total effect of an exogenous variable FQ on RVI is explained by indirect effect. Therefore, the effect of FQ on RVI is partially mediated through CS. Similarly, the VAF value indicates that 70% of the total effect of an exogenous variable RSQ and 35% VAF of PEQ on RVI is explained by indirect effect. Therefore, the effects of RSQ and PEQ on RVI are also partially mediated through CS. H 6 is supported as the effect of CS is partially mediated between RSQ and RVI of customer in fast food restaurant. H 7 is supported as the effect of CS is partially mediated between PEQ and RVI of customer in fast food restaurant, see Table 7 . This clearly indicates that customer satisfaction mediates between all of our exogenous variables (food quality, restaurant service quality and physical environment quality) and dependent variable revisit intention of customer in fast food restaurant [ 88 , 92 ] (Additional files 1 , 2 and 3 ).
This is interesting to note that food quality, restaurant service quality, physical environment quality, and customer satisfaction are important triggers of revisit intention at fast food restaurants. However, surprisingly, word of mouth does not moderate the relationship of customer satisfaction with revisit intention of customer at fast food restaurant. The results of the study correspond with some previous findings [ 15 , 29 , 32 , 69 , 93 ]. Positive relationship between customer satisfaction and revisit intention is consistent with the findings of the previous studies [ 5 , 8 , 94 , 95 , 96 ]. Food quality is positively associated with revisit intention; this result as well corresponds to a previous study [ 24 ]. Furthermore, interior and amusing physical environment is an important antecedent of revisit intention at a fast food restaurant; this finding is congruent with previous findings [ 29 , 70 , 97 , 98 ] and contrary to some previous studies [ 9 , 15 ].
Intensified competition, industry’s volatile nature, and maturity of the business are some challenges that fast food restaurants face [ 5 ]. Amid economic crunch, competition becomes even more evident, driving fast food restaurants to look for unconventional ways to appeal the customers. In fact, these findings somehow show that significance of physical environment quality in creating revisit intention is probably lower in comparison with food quality and restaurant service quality. Nonetheless, fast food restaurant’s management should not underrate the fact that physical environment quality considerably affects the revisit intention. Due to this, the importance of physical environment quality must not be overlooked when formulating strategies for improving customer satisfaction, revisit intention and creating long-term relationships with customers.
Managerial implications
The results imply that restaurant management should pay attention to customer satisfaction because it directly affects revisit intention. Assessing customer satisfaction has become vital to successfully contest in the modern fast food restaurant business. From a managerial point of view, the results of this study will help restaurant managers to better understand the important role of food quality, restaurant service quality and physical environment quality as marketing tool to retain and satisfy customers.
Limitations
There are certain limitations with this study. This study is cross sectional, and it can be generalized to only two cities of Pakistan. Scope of research was limited as the data were collected from two cities of Pakistan (Islamabad and Rawalpindi) using convenience sampling.
Future research
A longitudinal study with probability sampling will help the researchers to comprehensively investigate the relationships among the constructs. Moreover, it would be useful for future research models to add information overload as an explanatory variable and brand image as moderating variable in the research framework. Additionally, moderation of WOM can be investigated in other relationships of conceptual model.
The study encircles the key triggers of customer satisfaction and revisit intention in fast food restaurants. It also offers a model that defines relationships between three factors of restaurant offer (food quality, restaurant service quality, and physical environment quality), customer satisfaction, word of mouth, and revisit intention at fast food restaurants. The model specially focuses the revisit intention as dependent variable of conceptual model despite behavior intentions. The findings suggest the revisit intention is positively associated with customer satisfaction, food quality, restaurant service quality, and physical environment quality in a fast food restaurant.
However, contrary to the findings of a previous study [ 99 ], WOM do not positively moderate between the relationship of customer satisfaction and revisit intention. The empirical findings confirm the significant impact of food quality, restaurant service quality, physical environment quality, and customer satisfaction which are important antecedents of revisit intention at fast food restaurant through mediation of customer satisfaction. Moreover, findings of the research support the assumptions of SOR theory strengthening our conceptual model which states the external stimuli (FQ, RSQ, PEQ) produced internal organism (CS) which led to the response (RVI). However; assumption of social conformity theory failed to influence the satisfied customer. In other words, customer satisfaction plays dominating role over social influence (i.e. WOM) in making revisit intention. Therefore, WOM was not able to influence the strength of relationship of CS and RVI.
Availability of data and materials
The datasets used and/or analyzed during the current study are available from the corresponding author on reasonable request.
Abbreviations
Social conformity theory
Stimulus-organism-response
Structural equation modeling with partial least squares
Rhou Y, Singal M (2020) A review of the business case for CSR in the hospitality industry. Int J Hosp Manag 84:102330
Google Scholar
Berezina K, Cobanoglu C, Miller BL, Kwansa FA (2012) The impact of information security breach on hotel guest perception of service quality, satisfaction, revisit intentions and word-of-mouth. Int J Hosp Manag 24(7):991–1010
Shariff SNFBA, Omar MB, Sulong SNB, Majid HABMA, Ibrahim HBM, Jaafar ZB, Ideris MSKB (2015) The influence of service quality and food quality towards customer fulfillment and revisit intention. Can Soc Sci 11(8):138–144
Rana M, Lodhi R, Butt G, Dar W (2017) How determinants of customer satisfaction are affecting the brand image and behavioral intention in fast food industry of Pakistan. J Tour Hospit 6(316):2167-0269
Marinkovic V, Senic V, Ivkov D, Dimitrovski D, Bjelic M (2014) The antecedents of satisfaction and revisit intentions for full-service restaurants. Mark Intell Plan 32(3):311–327
Harun A, Prybutok G, Prybutok VR (2018) Insights into the antecedents of fast-food purchase intention and the relative positioning of quality. Qual Manag J 25(2):83–100
Qin H, Prybutok VR (2009) Service quality, customer satisfaction, and behavioral intentions in fast-food restaurants. Int J Qual Serv Sci 1(1):78–95
Chen JV, Htaik S, Hiele TM, Chen C (2017) Investigating international tourists’ intention to revisit Myanmar based on need gratification, flow experience and perceived risk. J Qual Assur Hosp Tour 18(1):25–44
Ali F, Amin M, Ryu K (2016) The role of physical environment, price perceptions, and consumption emotions in developing customer satisfaction in Chinese resort hotels. J Qual Assur Hosp Tour 17(1):45–70
Pareigis J, Edvardsson B, Enquist B (2011) Exploring the role of the service environment in forming customer’s service experience. Int J Qual Serv Sci 3(1):110–124
Ozdemir B, Caliskan O (2015) Menu design: a review of literature. J Foodserv Bus Res 18(3):189–206
Sadeghi M, Zandieh D, Mohammadi M, Yaghoubibijarboneh B, Nasrolahi Vosta S (2017) Investigating the impact of service climate on intention to revisit a hotel: the mediating role of perceived service quality and relationship quality. Int J Manag Sci Eng Manag 12(1):12–20
Blackston M, Lebar E (2015) Constructing consumer-brand relationships to better market and build businesses. In: Fournier S, Breazeale M, Avery J (eds) Strong brands, strong relationships. Routledge, Abingdon, p 376
Malik SA, Jaswal LH, Malik SA, Awan TM (2013) Measuring service quality perceptions of the customers of restaurant in Pakistan. Int J Qual Res 7(2):187–200
Sivadas E, Jindal RP (2017) Alternative measures of satisfaction and word of mouth. J Serv Mark 31(2):119–130
Ha Y, Im H (2012) Role of web site design quality in satisfaction and word of mouth generation. J Serv Manag 23(1):79–96
Zhang W, Yang J, Ding X-Y, Zou X-M, Han H-Y, Zhao Q-C (2019) Groups make nodes powerful: Identifying influential nodes in social networks based on social conformity theory and community features. Expert Syst Appl 125:249–258
Peri C (2006) The universe of food quality. Food Qual Prefer 17(1):3–8
Susskind AM, Chan EK (2000) How restaurant features affect check averages: a study of the Toronto restaurant market. Cornell Hotel Restaurant Adm Q 41(6):56–63
Jin N, Lee S, Huffman L (2012) Impact of restaurant experience on brand image and customer loyalty: moderating role of dining motivation. J Travel Tour Mark 29(6):532–551
Carins JE, Rundle-Thiele S, Ong DL (2020) Keep them coming back: the role of variety and aesthetics in institutional food satisfaction. Food Qual Prefer 80:103832
Josiam BM, Monteiro PA (2004) Tandoori tastes: perceptions of Indian restaurants in America. Int J Contemp Hosp Manag 16(1):18–26
Choi J, Zhao J (2010) Factors influencing restaurant selection in south florida: Is health issue one of the factors influencing consumers’ behavior when selecting a restaurant? J Foodserv Bus Res 13(3):237–251
Ryu K, Han H (2010) Influence of the quality of food, service, and physical environment on customer satisfaction and behavioral intention in quick-casual restaurants: moderating role of perceived price. J Hosp Tour Res 34(3):310–329
Deming WE (1982) Quality, productivity, and competitive position. Massachusetts Institute of Technology, Center for Advanced Engineering Study, Cambridge
Juran JM, Gryna FM, Bingham RS (1974) Quality control handbook. McGraw-Hill, Michigan
Dabholkar PA (2015) How to improve perceived service quality by increasing customer participation. Paper presented at the Proceedings of the 1990 Academy of Marketing Science (AMS) annual conference
Lai IK (2015) The roles of value, satisfaction, and commitment in the effect of service quality on customer loyalty in Hong Kong–style tea restaurants. Cornell Hosp Q 56(1):118–138
Jalilvand MR, Salimipour S, Elyasi M, Mohammadi M (2017) Factors influencing word of mouth behaviour in the restaurant industry. Mark Intell Plan 35(1):81–110
Wall EA, Berry LL (2007) The combined effects of the physical environment and employee behavior on customer perception of restaurant service quality. Cornell Hotel Restaurant Adm Q 48(1):59–69
Yuksel A, Yuksel F, Bilim Y (2010) Destination attachment: effects on customer satisfaction and cognitive, affective and conative loyalty. Tour Manag 31(2):274–284
Adam I, Adongo CA, Dayour F (2015) International tourists’ satisfaction with Ghanaian upscale restaurant services and revisit intentions. J Q Assur Hosp Tour 16(2):181–201
Baek E, Choo HJ, Yoon S-Y, Jung H, Kim G, Shin H, Kim H (2015) An exploratory study on visual merchandising of an apparel store utilizing 3D technology. J Global Fashion Mark 6(1):33–46
Wu H-C, Ko YJ (2013) Assessment of service quality in the hotel industry. J Qual Assur Hosp Tour 14(3):218–244
Pizam A, Shapoval V, Ellis T (2016) Customer satisfaction and its measurement in hospitality enterprises: a revisit and update. Int J Contemp Hosp Manag 28(1):2–35
Westbrook RA, Oliver RL (1991) The dimensionality of consumption emotion patterns and consumer satisfaction. J Consum Res 18(1):84–91
Prayag G, Hosany S, Muskat B, Del Chiappa G (2017) Understanding the relationships between tourists’ emotional experiences, perceived overall image, satisfaction, and intention to recommend. J Travel Res 56(1):41–54
Alegre J, Garau J (2010) Tourist satisfaction and dissatisfaction. Ann TTour Res 37(1):52–73
Arndt J (1967) Word of mouth advertising: a review of the literature. Advertising Research Foundation, New York
Bayus BL (1985) Word of mouth-the indirect effects of marketing efforts. J Advert Res 25(3):31–39
Harrison-Walker LJ (2001) The measurement of word-of-mouth communication and an investigation of service quality and customer commitment as potential antecedents. J Serv Res 4(1):60–75
Curina I, Francioni B, Hegner SM, Cioppi M (2020) Brand hate and non-repurchase intention: a service context perspective in a cross-channel setting. J Retail Consum Serv 54:102031
Hennig-Thurau T, Gwinner KP, Walsh G, Gremler DD (2004) Electronic word-of-mouth via consumer-opinion platforms: What motivates consumers to articulate themselves on the internet? J Interact Mark 18(1):38–52
Berger J, Schwartz EM (2011) What drives immediate and ongoing word of mouth? J Mark Res 48(5):869–880
Moliner-Velázquez B, Ruiz-Molina M-E, Fayos-Gardó T (2015) Satisfaction with service recovery: moderating effect of age in word-of-mouth. J Consum Mark 32(6):470–484
Royo-Vela M, Casamassima P (2011) The influence of belonging to virtual brand communities on consumers’ affective commitment, satisfaction and word-of-mouth advertising: the ZARA case. Online Inf Rev 35(4):517–542
Dhillon J (2013) Understanding word-of-mouth communication: a case study of banking sector in India. J Bus Manag 9(3):64–72
Chien M (2017) An empirical study on the effect of attractiveness of ecotourism destination on experiential value and revisit intention. Appl Ecol Environ Res 15(2):43–53
Abubakar AM, Ilkan M, Al-Tal RM, Eluwole KK (2017) eWOM, revisit intention, destination trust and gender. J Hosp Tour Manag 31:220–227
Chen C-F, Tsai D (2007) How destination image and evaluative factors affect behavioral intentions? Tour Manag 28(4):1115–1122
Allameh SM, Khazaei Pool J, Jaberi A, Salehzadeh R, Asadi H (2015) Factors influencing sport tourists’ revisit intentions: the role and effect of destination image, perceived quality, perceived value and satisfaction. Asia Pac J Mark Logist 27(2):191–207
Mehrabian A, Russell JA (1974) The basic emotional impact of environments. Percept Motor Skills 38(1):283–301
Zhang KZK, Benyoucef M (2016) Consumer behavior in social commerce: a literature review. Decision Support Syst 86:95–108. https://doi.org/10.1016/j.dss.2016.04.001
Article Google Scholar
Lee H-J, Yun Z-S (2015) Consumers’ perceptions of organic food attributes and cognitive and affective attitudes as determinants of their purchase intentions toward organic food. Food Qual Prefer 39:259–267
Yeh C-H, Wang Y-S, Li H-T, Lin S-Y (2017) The effect of information presentation modes on tourists’ responses in Internet marketing: the moderating role of emotions. J Travel Tour Mark 34(8):1018–1032
Lim SH, Lee S, Kim DJ (2017) Is online consumers’ impulsive buying beneficial for e-commerce companies? An empirical investigation of online consumers’ past impulsive buying behaviors. Inf Syst Manag 34:85–100
Wang L, Baker J, Wakefield K, Wakefield R (2017) Is background music effective on retail websites? J Promot Manag 23(1):1–23
Ali F (2016) Hotel website quality, perceived flow, customer satisfaction and purchase intention. J Hosp Tour Technol 7(2):213–228
Wang Z, Du C, Fan J, Xing Y (2017) Ranking influential nodes in social networks based on node position and neighborhood. Neurocomputing 260:466–477
Saunders MN (2011) Research methods for business students, 5/e. Pearson Education India, Bengaluru
Flick U (2015) Introducing research methodology: a beginner’s guide to doing a research project. Sage, Thousand Oaks
Zikmund WG, Babin BJ, Carr JC, Griffin M (2013) Business research methods. Cengage Learning, Boston
Lai W-T, Chen C-F (2011) Behavioral intentions of public transit passengers—the roles of service quality, perceived value, satisfaction and involvement. Transp Policy 18(2):318–325
Widianti T, Sumaedi S, Bakti IGMY, Rakhmawati T, Astrini NJ, Yarmen M (2015) Factors influencing the behavioral intention of public transport passengers. Int J Qual Reliab Manag 32(7):666–692
Hair JF Jr, Sarstedt M, Hopkins L, Kuppelwieser VG (2014) Partial least squares structural equation modeling (PLS-SEM) An emerging tool in business research. Eur Bus Rev 26(2):106–121
Krejcie RV, Morgan DW (1970) Determining sample size for research activities. Educ Psychol Measur 30(3):607–610
Rahi S, Alnaser FM, Ghani MA (2019) Designing survey research: recommendation for questionnaire development, calculating sample size and selecting research paradigms. In: Economic and Social Development: Book of Proceedings, pp 1157–1169
Wahab S, bin Mohamad Shah MF, Faisalmein SN (2019) The relationship between management competencies and internal marketing knowledge towards internal marketing performance. Paper presented at the Proceedings of the Regional Conference on Science, Technology and Social Sciences (RCSTSS 2016)
Namkung Y, Jang S (2007) Does food quality really matter in restaurants? Its impact on customer satisfaction and behavioral intentions. J Hosp Tour Res 31(3):387–409
Liu C-H, Chou S-F, Gan B, Tu J-H (2015) How “quality” determines customer satisfaction: evidence from the mystery shoppers’ evaluation. TQM J 27(5):576–590
Meng JG, Elliott KM (2008) Predictors of relationship quality for luxury restaurants. J Retail Consum Serv 15(6):509–515
Cham TH, Lim YM, Aik NC, Tay AGM (2016) Antecedents of hospital brand image and the relationships with medical tourists’ behavioral intention. Int J Pharm Healthc Mark 10(4):412–431
Sekaran U (2006) Research methods for business: a skill building approach. Wiley, New York
Fornell C, Larcker DF (1981) Evaluating structural equation models with unobservable variables and measurement error. J Mark Res 18(1):39–50. https://doi.org/10.2307/3151312
Cronbach LJ (1951) Coefficient alpha and the internal structure of tests. Psychometrika 16(3):297–334
Simonin BL (1999) Ambiguity and the process of knowledge transfer in strategic alliances. Strateg Manag J 20(7):595–623. https://doi.org/10.1002/(sici)1097-0266(199907)20:7%3c595:aid-smj47%3e3.0.co;2-5
Robson MJ, Katsikeas CS, Bello DC (2008) Drivers and performance outcomes of trust in international strategic alliances: the role of organizational complexity. Organ Sci 19(4):647–665. https://doi.org/10.1287/orsc.1070.0329
Greene CN, Organ DW (1973) An evaluation of causal models linking the received role with job satisfaction. Adm Sci Q 18(1):95–103
Konrad AM, Linnehan F (1995) Formalized HRM structures: Coordinating equal employment opportunity or concealing organizational practices? Acad Manag J 38(3):787–820
Podsakoff PM, MacKenzie SB, Lee J-Y, Podsakoff NP (2003) Common method biases in behavioral research: a critical review of the literature and recommended remedies. J Appl Psychol 88(5):879–903. https://doi.org/10.1037/0021-9010.88.5.879
Podsakoff PM, Organ DW (1986) Self-reports in organizational research: problems and prospects. J Manag 12(4):531–544. https://doi.org/10.1177/014920638601200408
Scott SG, Bruce RA (1994) Determinants of innovative behavior: a path model of individual innovation in the workplace. Acad Manag J 37(3):580–607
Simonin BL (2004) An empirical investigation of the process of knowledge transfer in international strategic alliances. J Int Bus Stud 35(5):407–427
Lowry PB, Gaskin J (2014) Partial least squares (PLS) structural equation modeling (SEM) for building and testing behavioral causal theory: When to choose it and how to use it. IEEE Trans Prof Commun 57(2):123–146
Hair JF Jr, Hult GTM, Ringle C, Sarstedt M (2016) A primer on partial least squares structural equation modeling (PLS-SEM). Sage Publications, Thousand Oaks
Nunnally JC (1978) Psychometric theory. McGraw-Hill, New York
Gefen D, Straub D, Boudreau M-C (2000) Structural equation modeling and regression: guidelines for research practice. Commun Assoc Inf Syst 4(1):7
Hair J (2013) Using the SmartPLS software. Kennesaw State University. Powerpoint presentation/lecture
Vinzi VE, Chin WW, Henseler J, Wang H (2010) Handbook of partial least squares: concepts, methods and applications. Springer, Berlin
Geisser S (1974) A predictive approach to the random effect model. Biometrika 61(1):101–107
Stone M (1974) Cross-validatory choice and assessment of statistical predictions. J R Stat Soc Ser B (Methodol) 36:111–147
Carrión GC, Nitzl C, Roldán JL (2017) Mediation analyses in partial least squares structural equation modeling: guidelines and empirical examples. In: Latan H, Noonan R (eds) Partial least squares path modeling. Springer, Berlin, pp 173–195
Lockyer T (2003) Hotel cleanliness—How do guests view it? Let us get specific. A New Zealand study. Int J Hosp Manag 22(3):297–305
Ha J, Jang SS (2010) Perceived values, satisfaction, and behavioral intentions: the role of familiarity in Korean restaurants. Int J Hosp Manag 29(1):2–13
Ryu K, Han H, Jang S (2010) Relationships among hedonic and utilitarian values, satisfaction and behavioral intentions in the fast-casual restaurant industry. Int J Contemp Hosp Manag 22(3):416–432
Ryu K, Lee H-R, Gon Kim W (2012) The influence of the quality of the physical environment, food, and service on restaurant image, customer perceived value, customer satisfaction, and behavioral intentions. Int J Contemp Hosp Manag 24(2):200–223
Jang S, Liu Y, Namkung Y (2011) Effects of authentic atmospherics in ethnic restaurants: investigating Chinese restaurants. Int J Contemp Hosp Manag 23(5):662–680
Martín-Ruiz D, Barroso-Castro C, Rosa-Díaz IM (2012) Creating customer value through service experiences: an empirical study in the hotel industry. Tour Hosp Manag 18(1):37–53
Kuo Y-F, Hu T-L, Yang S-C (2013) Effects of inertia and satisfaction in female online shoppers on repeat-purchase intention: the moderating roles of word-of-mouth and alternative attraction. Manag Serv Qual Int J 23(3):168–187
Download references
Acknowledgements
The authors gratefully acknowledge the conducive research environment support provided by Department of Management Sciences at COMSATS University Islamabad, Wah Campus and Higher Education Commission Pakistan for provision of free access to digital library.
The authors declare that there was no source of funding for this research.
Author information
Authors and affiliations.
Department of Management Sciences, COMSATS University Islamabad, Wah Campus, G. T. Road, Wah Cantt., 47040, Pakistan
Amer Rajput
Management Sciences, Riphah International University, Al-Mizan IIMCT Complex, 274-Peshawar Road, Rawalpindi, Pakistan
Raja Zohaib Gahfoor
You can also search for this author in PubMed Google Scholar
Contributions
RG conceptualized the study while corresponding author AR furnished the data analysis and finalized the manuscript for the submission. Both authors read and approved the final manuscript..
Corresponding author
Correspondence to Amer Rajput .
Ethics declarations
Competing interests.
The authors declare that they have no competing interests.
Additional information
Publisher's note.
Springer Nature remains neutral with regard to jurisdictional claims in published maps and institutional affiliations.
Supplementary information
Additional file 1..
PLS Algorithm.
Additional file 2.
Bootstrapping.
Additional file 3.
Blindfolding.
Rights and permissions
Open Access This article is licensed under a Creative Commons Attribution 4.0 International License, which permits use, sharing, adaptation, distribution and reproduction in any medium or format, as long as you give appropriate credit to the original author(s) and the source, provide a link to the Creative Commons licence, and indicate if changes were made. The images or other third party material in this article are included in the article's Creative Commons licence, unless indicated otherwise in a credit line to the material. If material is not included in the article's Creative Commons licence and your intended use is not permitted by statutory regulation or exceeds the permitted use, you will need to obtain permission directly from the copyright holder. To view a copy of this licence, visit http://creativecommons.org/licenses/by/4.0/ .
Reprints and permissions
About this article
Cite this article.
Rajput, A., Gahfoor, R.Z. Satisfaction and revisit intentions at fast food restaurants. Futur Bus J 6 , 13 (2020). https://doi.org/10.1186/s43093-020-00021-0
Download citation
Received : 18 October 2019
Accepted : 26 February 2020
Published : 04 June 2020
DOI : https://doi.org/10.1186/s43093-020-00021-0
Share this article
Anyone you share the following link with will be able to read this content:
Sorry, a shareable link is not currently available for this article.
Provided by the Springer Nature SharedIt content-sharing initiative
Customer satisfaction: A meta-analysis of the empirical evidence
- Published: December 2001
- Volume 29 , pages 16–35, ( 2001 )
Cite this article
- David M. Szymanski 1 &
- David H. Henard 2
8762 Accesses
1053 Citations
3 Altmetric
Explore all metrics
The growing number of academic studies on customer satisfaction and the mixed findings they report complicate efforts among managers and academics to identify the antecedents to, and outcomes of, businesses having more-versus less-satisfied customers. These mixed findings and the growing emphasis by managers on having satisfied customers point to the value of empirically synthesizing the evidence on customer satisfaction to assess current knowledge. To this end, the authors conduct a meta-analysis of the reported findings on customer satisfaction. They document that equity and disconfirmation are most strongly related to customer satisfaction on average. They also find that measurement and method factors that characterize the research often moderate relationship strength between satisfaction and its antecedents and outcomes. The authors discuss the implications surrounding these effects and offer several directions for future research.
This is a preview of subscription content, log in via an institution to check access.
Access this article
Subscribe and save.
- Get 10 units per month
- Download Article/Chapter or eBook
- 1 Unit = 1 Article or 1 Chapter
- Cancel anytime
Price excludes VAT (USA) Tax calculation will be finalised during checkout.
Instant access to the full article PDF.
Rent this article via DeepDyve
Institutional subscriptions
Similar content being viewed by others
Choosing Appropriate Customer Satisfaction Measures - First Steps towards a Normative Framework
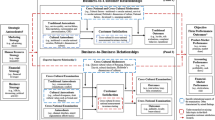
Customer satisfaction and international business: A multidisciplinary review and avenues for research
Do managers know what their customers think and why.
Adams, J. Stacy. 1963. “Towards an Understanding of Inequity.” Journal of Abnormal and Social Psychology 67 (November): 422–436.
Google Scholar
Studies whose findings are included in the meta-analysis. Not all of these studies are cited in the text Aiello, Albert, Jr, John A. Czepiel, and Larry J. Rosenberg. 1977. “Scaling the Heights of Consumer Satisfaction: An Evaluation of Alternative Measures.” In Consumer Satisfaction. Dissatisfaction and Complaining Behavior . Ed. Ralph L. Day. Bloomington: Indiana University School of Business, 43–50.
Anderson, Eugene W., Claes Fornell, and Donald R. Lehmann. 1994. “Customer Satisfaction, Market Share, and Profitability: Findings From Sweden.” Journal of Marketing 58 (July): 53–66.
Article Google Scholar
——, and Roland T. Rust. 1997. “Customer Satisfaction, Productivity, and Profitability: Differences Between Goods and Services.” Marketing Science 16 (2): 129–145.
— and Mary W. Sullivan. 1993. “The Antecedents and Consequences of Customer Satisfaction for Firms.” Marketing Science 12 (2): 125–143.
Studies whose findings are included in the meta-analysis. Not all of these studies are cited in the text Andreasen, Alan R. 1984. “Life Status Changes and Changes in Consumer Preferences and Satisfaction.” Journal of Consumer Research 11 (December): 784–795.
— 1988. “Consumer Complaints and Redress: What We Know and What We Don’t Know.” In The Frontier of Research in the Consumer Interest . Ed. E. Scott Maynes. Columbia, MO: American Council on Consumer Interest, 675–722.
Studies whose findings are included in the meta-analysis. Not all of these studies are cited in the text Baer, Robert and Donna J. Hill. 1994. “The Impact of Satisfaction on Brand Loyalty: Urging on Classifying Satisfaction and Brand Loyalty.” Journal of Consumer Satisfaction, Dissatisfaction, and Complaining Behavior 7: 152–160.
Studies whose findings are included in the meta-analysis. Not all of these studies are cited in the text Bearden, William O. and Jesse E. Teel. 1983. “Selected Determinants of Consumer Satisfaction and Complaint Report.” Journal of Marketing Research 20 (February): 21–28.
Studies whose findings are included in the meta-analysis. Not all of these studies are cited in the text Bitner, Mary Jo. 1987. “Contextual Cues and Customer Satisfaction: The Role of Physical Surroundings and Employee Behaviors in Service Settings.” Ph.D. dissertation. University of Washington, Seattle.
—. 1990. “Evaluating Service Encounters: The Effects of Physical Surroundings and Employee Responses.” Journal of Marketing 54 (April): 69–82.
Studies whose findings are included in the meta-analysis. Not all of these studies are cited in the text Bloemer, Jose and Ko de Ruyter. 1995. “Integrating Service Quality and Satisfaction: Pain in the Neck or Marketing Opportunity?” Journal of Consumer Satisfaction, Dissatisfaction and Complaining Behavior 8: 44–52.
Bolton, Ruth N. and James H. Drew. 1991. “A Longitudinal Analysis of the Impact of Service Changes on Customer Attitudes.” Journal of Marketing 55 (January): 1–9.
Burnett, John J. and Patrick M. Dunne. 1986. “An Appraisal of the Use of Student Subjects in Marketing Research.” Journal of Business Research 14: 329–343.
Studies whose findings are included in the meta-analysis. Not all of these studies are cited in the text Cadotte, Ernest R., Robert B. Woodruff, and Roger L. Jenkins. 1987. “Expectations and Norms in Models of Consumer Satisfaction.” Journal of Marketing Research 14 (August): 305–314.
Studies whose findings are included in the meta-analysis. Not all of these studies are cited in the text Churchill, Gilbert A. and Carol Surprenant. 1982. “An Investigation Into The Determinants of Customer Satisfaction.” Journal of Marketing Research 19 (November): 491–504.
Studies whose findings are included in the meta-analysis. Not all of these studies are cited in the text Clemmer, Elizabeth C.. 1988. “The Role of Fairness in Customer Satisfaction With Services.” Ph.D. dissertation. University of Maryland College Park.
Cohen, Jacob and Patricia Cohen. 1983. Applied Multiple Regression/ Correlation Analysis for the Behavioral Sciences Hillsdale, NJ: Lawrence Erlbaum.
Studies whose findings are included in the meta-analysis. Not all of these studies are cited in the text Crosby, Lawrence A. and James R. Taylor. 1982. “Consumer Satisfaction With Michigan’s Container Deposit Law—An Ecological Perspective.” Journal of Marketing 46 (Winter): 47–60.
Day, George. 1984. “Modeling Choices Among Alternative Responses to Dissatisfaction.” In Advances in Consumer Research . Ed. Thomas Kinnear. Provo, UT: Association for Consumer Research, 496–499.
Studies whose findings are included in the meta-analysis. Not all of these studies are cited in the text Deeter-Schmelz, Dawn R. 1996. “Customer Contact Teams: The Impact, Structure and Process on Team Effectiveness and Customer Satisfaction.” Ph.D. dissertation. University of South Florida.
Studies whose findings are included in the meta-analysis. Not all of these studies are cited in the text Djupvik, Harald and Dag Eilertsen. 1994. “Customer Satisfaction Monitoring to Understand the Market—Norwegian Telecom.” Marketing and Research Today 22 (February): 4–18.
Studies whose findings are included in the meta-analysis. Not all of these studies are cited in the text Duhaine, Carole P.. 1988. “Customer Satisfaction With the Distribution System for Durable Products.” Journal of Consumer Satisfaction, Dissatisfaction and Complaining Behavior 1: 53–59.
Duncan, Otis D.. 1975. Introduction to Structural Equation Models . New York: Academic Press.
Fisk, Raymond P. and Clifford E. Young. 1985. “Disconfirmation of Equity Expectations: Effects on Consumer Satisfaction With Services.” In Advances in Consumer Research . Vol. 12. Eds. Elizabeth C. Hirschman and Morris B. Holbrook. Provo, UT: Association for Consumer Research, 340–345.
Folkes, Valerie S.. 1984. “Consumer Reactions to Product Failure: An Attribution Approach.” Journal of Consumer Research 14 (March): 398–409.
Studies whose findings are included in the meta-analysis. Not all of these studies are cited in the text Fu, Shenzhao and Debra S. Perkins. 1995. “Determinants of Technology Licensee’s Satisfaction: An Empirical Investigation.” Journal of Consumer Satisfaction, Dissatisfaction, and Complaining Behavior 8: 223–233.
Garland, Barbara and Robert A. Westbrook. 1989. “An Exploration of Client Satisfaction in a Nonprofit Context.” Journal of the Academy of Marketing Science 17 (Fall): 297–303.
Studies whose findings are included in the meta-analysis. Not all of these studies are cited in the text Gibbon, Cheryl. 1994. “Supplier Quality, Customer Commitment and Satisfaction, and Marketplace Performance.”, Ph.D. dissertation, York University, North York, Ontario.
Studies whose findings are included in the meta-analysis. Not all of these studies are cited in the text Gilly, Mary C. and Betsy D. Gelb. 1982. “Post-Purchase Consumer Processes and the Complaining Consumer.” Journal of Consumer Research 9 (December): 323–328.
Glass, Gene V., Barry McGaw, and Mary Lee Smith. 1981. Meta-Analysis in Social Research . Beverly Hills, CA: Sage.
Goodwin, Cathy and Ivan Ross. 1992. “Consumer Responses to Service Failure: Influence of Procedural and Interactional Fairness Perceptions.” Journal of Business Research 25 (September): 149–163.
Gronroos, Christian. 1982. Strategic Management and Marketing in the Service Sector . Helsinfors, Sweden: Swedish School of Economics and Business Administration.
Hair, Joseph F., Jr., Rolph E. Anderson, Ronald L. Tatham, and William C Black. 1995. Multivariate Data Analysis . Upper Saddle River, NJ: Prentice Hall.
Halstead, Diane, David Hartman, and Sandra L. Schmidt 1994. “Multisource Effects on the Satisfaction Formation Process.”, Journal of the Academy of Marketing Science 22 (2): 114–129.
Hedges, Larry V. and Ingram Olkin. 1985. Statistical Methods for Meta-Analysis . San Diego, CA: Academic Press.
Studies whose findings are included in the meta-analysis. Not all of these studies are cited in the text Hiltz, Starr Roxanne and Kenneth Johnson. 1990. “User Satisfaction With Computer-Mediated Communication Systems.”, Management Science 36 (6): 739–764.
Hirschman, Albert O., 1970. Exit, Voice, and Loyalty . Cambridge, MA: Harvard University Press.
Hocutt, Mary Ann, Goutam Chakraborty, and John C. Mowen. 1997. “The Impact of Perceived Justice on Customer Satisfaction and Intention to Complain in a Service Recovery.” In Advances in Consumer Research , Vol. 24. Eds. Merrie Brucks and Deborah J. Macinnis, 457–463.
Hunter, John E. and Frank L. Schmidt. 1990. Methods of Meta Analysis . Newbury Park, CA: Sage.
Johnson, Michael D.. 1998. Customer Orientation and Market Action . Upper Saddle River, NJ: Prentice Hall.
Studies whose findings are included in the meta-analysis. Not all of these studies are cited in the text Kennedy John R. and Peter C. Thirkell. 1988. “An Extended Perspective on the Antecedents of Satisfaction.” Journal of Consumer Satisfaction, Dissatisfaction and Complaining Behavior 1: 2–9.
LaBarbera, Priscilla A. and David Mazursky. 1983. “A Longitudinal Assessment of Consumer Satisfaction/Dissatisfaction: The Dynamic Aspect of the Cognitive Process.”, Journal of Marketing Research 20 (November): 393–404.
Studies whose findings are included in the meta-analysis. Not all of these studies are cited in the text Lai, Mengkuan and Richard Widdows. 1993. “Determinants of Consumer’s Satisfaction With Service: A Preliminary Study.”, Journal of Consumer Satisfaction. Dissatisfaction and Complaining Behavior 6: 166–174.
Studies whose findings are included in the meta-analysis. Not all of these studies are cited in the text LaTour, Stephen A. and Nancy C. Peat. 1979. “The Role of Situationally Produced Expectations, Others’ Experiences, and Prior Experiences in Determining Consumer Satisfaction.” In Advances in Consumer Research , Vol. 7. Ed. Jerry C. Olson. Ann Arbor, MI: Association for Consumer Research, 588–592.
Studies whose findings are included in the meta-analysis. Not all of these studies are cited in the text Liljander, Veronica. 1994. “Modeling Perceived Service Quality Using Different Comparison Standards.” Journal of Consumer Satisfaction, Dissatisfaction, and Complaining Behavior 7: 126–142.
Studies whose findings are included in the meta-analysis. Not all of these studies are cited in the text Mano, Haim and Richard L. Oliver. 1993. “Assessing the Dimensionality and Structure of the Consumption Experience: Evaluation, Feeling, and Satisfaction.” Journal of Consumer Research 20 (December): 451–466.
Matt, George E. and Thomas D. Cook. 1994. “Threats to the Validity of Research Synthesis.” In The Handbook of Research Synthesis . Eds. Harris Cooper and Larry V. Hedges New York: Rusell Sage Foundation.
Mittal, Vikas, William T. Ross, Jr., and Patrick M. Baldasare. 1998. “The Asymmetric Impact of Negative and Positive Attribute-Level Performance on Overall Satisfaction and Repurchase Intentions.”, Journal of Marketing 62 (January): 33–47.
Studies whose findings are included in the meta-analysis. Not all of these studies are cited in the text Mullan, Dennis J. 1996. “An Investigation of a Residential Customer Satisfaction Model at an Electric Utility.” Ph.D. dissertation. Wayne State University, Detroit, MI.
Neter, John, William Wasserman, and Michael H. Kutner. 1989. Applied Linear Regression Models . Homewood, IL: Irwin.
Nyer, Prashanth. 1999 “Cathartic Complaining as a Means of Reducing Consumer Dissatisfaction.” Journal of Consumer Satisfaction, Dissatisfaction, and complaining Behavior . 12: 15–25.
Oliva, Terence A., Richard L. Oliver, and Ian C. MacMillan. 1992. “A Catastrophe Model for Developing Service Satisfaction Strategies.” Journal of Marketing 56 (July): 83–95.
Oliver, Richard L. 1980. “A Cognitive Model of the Antecedents and Consequences of Satisfaction Decisions.” Journal of Marketing Research 17 (November): 460–469.
— 1981. “Measurement and Evaluation of Satisfaction Processes in Retail Settings.” Journal of Retailing 57 (Fall): 25–48.
— 1987. “An Investigation of the Interrelationship Between Consumer (Dis) Satisfaction and Complaining Reports.” In Advances in Consumer Research Vol. 14. Eds. Melanie Wallendorf and Paul Anderson. Provo, UT: Association for Consumer Research, 218–222.
Studies whose findings are included in the meta-analysis. Not all of these studies are cited in the text — 1993. “Cognitive, Affective, and Attribute Bases of the Satisfaction Response.” Journal of Consumer Research 20 (December): 418–430.
— 1997. Satisfaction: A Behavioral Perspective on the Consumer . New York: McGraw-Hill.
— and Wayne S. DeSarbo. 1988. “Response Determinants in Satisfaction Judgments.” Journal of Consumer Research 14 (March): 495–507.
— and G. Linda. 1981. “Effects of Satisfaction and Its Antecedents on Consumer Preference and Intention.” In Advances in Consumer Research . Ed. Kent B. Monroe. Ann Arbor, MI: Association for Consumer Research, 88–93.
Studies whose findings are included in the meta-analysis. Not all of these studies are cited in the text —, Roland T. Rust, and Sajeev Varki. 1997. “Customer Delight: Foundations, Findings, and Managerial Insight.” Journal of Retailing 73 (Fall): 311–336.
Studies whose findings are included in the meta-analysis. Not all of these studies are cited in the text — and John E. Swan. 1989a “Consumer Perceptions of Interpersonal Equity and Satisfaction in Transactions: A Field Survey Approach.” Journal of Marketing 53 (April): 21–35.
Studies whose findings are included in the meta-analysis. Not all of these studies are cited in the text — and —. 1989b. “Equity and Disconfirmation Perceptions as Influences on Merchant and Product Satisfaction.” Journal of Consumer Research 16 (December): 372–383.
Studies whose findings are included in the meta-analysis. Not all of these studies are cited in the text Paul, Kenneth. 1995. “Market Orientation, Quality and Customer Satisfaction in an Industrial Services Market.” Ph.D. dissertation, University of Memphis, TN.
Parasuraman, A., Valerie A. Zeithaml, and Leonard L. Berry. 1985. “A Conceptual Model of Service Quality and Its Implications for Future Research.” Journal of Marketing 49 (Fall): 41–50.
Park, C. Whan and V. Parker Lessig. 1977. “Students and Housewives: Differences in Susceptibility to Reference Group Influence.” Journal of Consumer Research 4 (September): 102–110.
Studies whose findings are included in the meta-analysis. Not all of these studies are cited in the text Prakesh, Ved. 1984. “Validity and Reliability of the Confirmation of Expectations Paradigm as a Determinant of Consumer Satisfaction.” Journal of the Academy of Marketing Science 12 (4): 63–76.
Studies whose findings are included in the meta-analysis. Not all of these studies are cited in the text — and John W. Lounsbury. 1984. “The Role of Expectations in the Determination of Consumer Satisfaction.” Journal of the Academy of Marketing Science 12 (3): 1–17.
Richins, Marsha L.. 1983. “Negative Word-of-Mouth by Dissatisfied Customers: A Pilot Study.” Journal of Marketing 47 (Winter): 68–78.
Rust, Roland T. and Anthony J. Zahorik. 1993. “Customer Satisfaction, Customer Retention, and Market Share.” Journal of Retailing 69 (2): 193–215.
Studies whose findings are included in the meta-analysis. Not all of these studies are cited in the text Singh, Jagdip. 1991. “Understanding the Structure of Consumers’ Satisfaction Evaluations of Service Delivery.” Journal of the Academy of Marketing Science 19 (3): 223–244.
Studies whose findings are included in the meta-analysis. Not all of these studies are cited in the text Sirgy, M. Joseph. 1984. “A Social Cognition Model of Consumer Satisfaction/Dissatisfaction: An Experiment.” Psychology and Marketing 1 (2): 27–44.
Studies whose findings are included in the meta-analysis. Not all of these studies are cited in the text Spreng, Richard A., Gilbert D. Harrell, and Robert D. Mackoy. 1995. “Service Recovery: Impact on Satisfaction and Intentions.” Journal of Services Marketing 9 (1): 15–22.
Studies whose findings are included in the meta-analysis. Not all of these studies are cited in the text —, Scott B. McKenzie, and Richard W. Olshavsky. 1996. “A Reexamination of the Determinants of Consumer Satisfaction.” Journal of Marketing 60 (July): 15–32.
— and Richard W. Olshavsky. 1993. “A Desires Congruency Model of Consumer Satisfaction.” Journal of the Academy of Marketing Science 21 (3): 169–177.
Sulek, Joanne M., Mary R. Lind, and Ann S. Marucheck. 1995. “The Impact of Customer Service Intervention and Facility Design on Firm Performance.” Management Science 41 (November): 1763–1773.
Studies whose findings are included in the meta-analysis. Not all of these studies are cited in the text Swan, John E. 1977. “Consumer Satisfaction With a Retail Store Related to the Fulfillment of Expectations on an Initial Shopping Trip.” In Consumer Satisfaction, Dissatisfaction and Complaining Behavior . Ed. Ralph L. Day. Bloomington: Indiana University School of Business, 10–17.
Studies whose findings are included in the meta-analysis. Not all of these studies are cited in the text —, 1988. “Consumer Satisfaction Related to Disconfirmation of Expectations and Product Performance.” Journal of Consumer Satisfaction, Dissatisfaction and Complaining Behavior 1: 40–47.
— and Alice A. Mercer. 1982. “Consumer Satisfaction as a Function of Equity and Disconfirmation.” In Conceptual and Empirical Contributions to Consumer Satisfaction and Complaining Behavior . Eds. H. Keith Hunt and Ralph L. Day. Bloomington: Indiana University Press, 2–8.
— and Richard L. Oliver. 1985. “Automobile Buyer Satisfaction With the Salesperson Related to Equity and Disconfirmation.” In Consumer Satisfaction, Dissatisfaction and Complaining Behavior . Eds. H. K. Hunt and R. L. Day. Bloomington: Indiana University Press.
Studies whose findings are included in the meta-analysis. Not all of these studies are cited in the text — and —. 1991. “An Applied Analysis of Buyer Equity Perceptions and Satisfaction With Automobile Salespeople.” Journal of Personal Selling and Sales Management 11 (2): 15–26.
Studies whose findings are included in the meta-analysis. Not all of these studies are cited in the text — and I. Frederick Trawick. 1981. “Disconfirmation of Expectations and Satisfaction With a Retail Service.” Journal of Retailing 57 (Fall): 49–67.
Tabachnick, Barbara G. and Linda S. Fidell. 1996. Using Multivariate Statistics . 3d ed. New York: HarperCollins.
Tavassoli, Nader T. 1998. “Language in Multimedia: Interaction of Spoken and Written Information.” Journal of Consumer Research 25 (March): 26–43.
Tax, Stephen S. and Murali Chandrashekaran. 1992. “Consumer Decision Making Following a Failed Service Encounter: A Pilot Study.” Journal of Consumer Satisfaction, Dissatisfaction and Complaining Behavior 5: 55–68.
Studies whose findings are included in the meta-analysis. Not all of these studies are cited in the text Taylor, Steven A. 1996. “Consumer Satisfaction With Marketing Education: Extending Services Theory to Academic Practice.” Journal of Consumer Satisfaction, Dissatisfaction, and Complaining Behavior 9: 207–220.
Studies whose findings are included in the meta-analysis. Not all of these studies are cited in the text — and Thomas L. Baker. 1994. “An Assessment of the Relationship Between Service Quality and Customer Satisfaction in the Formation of Consumers’ Purchase Intentions.” Journal of Retailing 70 (2): 163–178.
Studies whose findings are included in the meta-analysis. Not all of these studies are cited in the text Tessler, Richard and David Mechanic. 1975. “Consumer Satisfaction With Prepaid Group Practice: A Comparative Study.” Journal of Health and Social Behavior 16 (March): 95–113.
Thibaut, J. W. and H. H. Kelley. 1959. The Social Psychology of Groups . New York: John Wiley.
Studies whose findings are included in the meta-analysis. Not all of these studies are cited in the text Tse, David K. and Peter C. Wilton. 1988. “Models of Consumer Satisfaction Formation: An Extension.” Journal of Marketing Research 25 (May): 204–212.
Ursic, Michael L. 1985. “A Model of the Consumer Decision to Seek Legal Redress.” Journal of Consumer Affairs 19 (Summer): 20–36.
Studies whose findings are included in the meta-analysis. Not all of these studies are cited in the text Webb, D. and Abiodun Jagun. 1997. “Customer Care, Customer Satisfaction, Value, Loyalty and Complaining Behavior: Validation in a UK University Setting.” Journal of Consumer Satisfaction, Dissatisfaction, and Complaining Behavior 10: 139–151.
Weiner, Bernard. 1986. An Attributional Theory of Motivation and Emotion . New York: Springer.
Studies whose findings are included in the meta-analysis. Not all of these studies are cited in the text Westbrook, Robert A. 1980a. “Consumer Satisfaction as a Function of Personal Competence/Efficacy.” Journal of the Academy of Marketing Science 8 (4): 427–437.
Studies whose findings are included in the meta-analysis. Not all of these studies are cited in the text — 1980b. “Intrapersonal Affective Influences on Consumer Satisfaction With Products.” Journal of Consumer Research 7 (June): 49–54.
Studies whose findings are included in the meta-analysis. Not all of these studies are cited in the text — 1980c. “A Rating Scale for Measuring Product/Service Satisfaction.” Journal of Marketing 44 (Fall): 68–72.
Studies whose findings are included in the meta-analysis. Not all of these studies are cited in the text — 1981. “Sources of Consumer Satisfaction With Retail Outlets.” Journal of Retailing 57 (3): 68–85.
— 1987. “Product/Consumption-Based Affective Responses and Postpurchase Processes.” Journal of Marketing Research 24 (August): 258–270.
Studies whose findings are included in the meta-analysis. Not all of these studies are cited in the text — and Joseph A. Cote, Jr. 1979. “An Exploratory Study of Non-Product-Related Influences Upon Consumer Satisfaction.” In Advances in Consumer Research , Vol. 7. Ed. Jerry C. Olson. Ann Arbor, MI: Association for Consumer Research, 577–581.
Studies whose findings are included in the meta-analysis. Not all of these studies are cited in the text — and Richard L. Oliver. 1991. “The Dimensionality of Consumption Emotion Patterns and Consumer Satisfaction.” Journal of Consumer Research 18 (June): 84–91.
Yi, Youjae. 1990. “A Critical Review of Consumer Satisfaction.” In Review of Marketing . Ed. Valerie A. Zeithaml. Chicago: American Marketing Association.
Zeithaml, Valerie, Leonard Berry, and A. Parasuraman. 1993. “The Nature and Determinants of Customer Expectations of Service.” Journal of the Academy of Marketing Science 21 (1): 1–12.
Download references
Author information
Authors and affiliations.
Texas A&M University, Texas, USA
David M. Szymanski
North Carolina State University, North Carolina, USA
David H. Henard
You can also search for this author in PubMed Google Scholar
Additional information
David M. Szymanski (Ph.D., University of Wisconsin-Madison) is the Al and Marion Withers Research Fellow and Director, Center for Retailing Studies in the Lowry Mays College and Graduate School of Business, Texas A&M University. His research interests include applied meta-analysis, marketing strategy, personal selling and sales management, product innovation, and retail strategy. Representative research has appeared in the Journal of Marketing , the Journal of Marketing Research , and the Journal of Personal Selling and Sales Management .
David H. Henard (Ph.D., Texas A&M University) is an assistant professor of marketing at North Carolina State University. His research interests include product innovation, new product development, and corporate reputation.
Rights and permissions
Reprints and permissions
About this article
Szymanski, D.M., Henard, D.H. Customer satisfaction: A meta-analysis of the empirical evidence. JAMS 29 , 16–35 (2001). https://doi.org/10.1177/0092070301291002
Download citation
Issue Date : December 2001
DOI : https://doi.org/10.1177/0092070301291002
Share this article
Anyone you share the following link with will be able to read this content:
Sorry, a shareable link is not currently available for this article.
Provided by the Springer Nature SharedIt content-sharing initiative
- Customer Satisfaction
- Consumer Research
- Service Recovery
- Consumer Satisfaction
- Repurchase Intention
- Find a journal
- Publish with us
- Track your research
- Subscribe to Newsletter
- Connect with ACSI
- Restaurants
Fast Food Restaurants
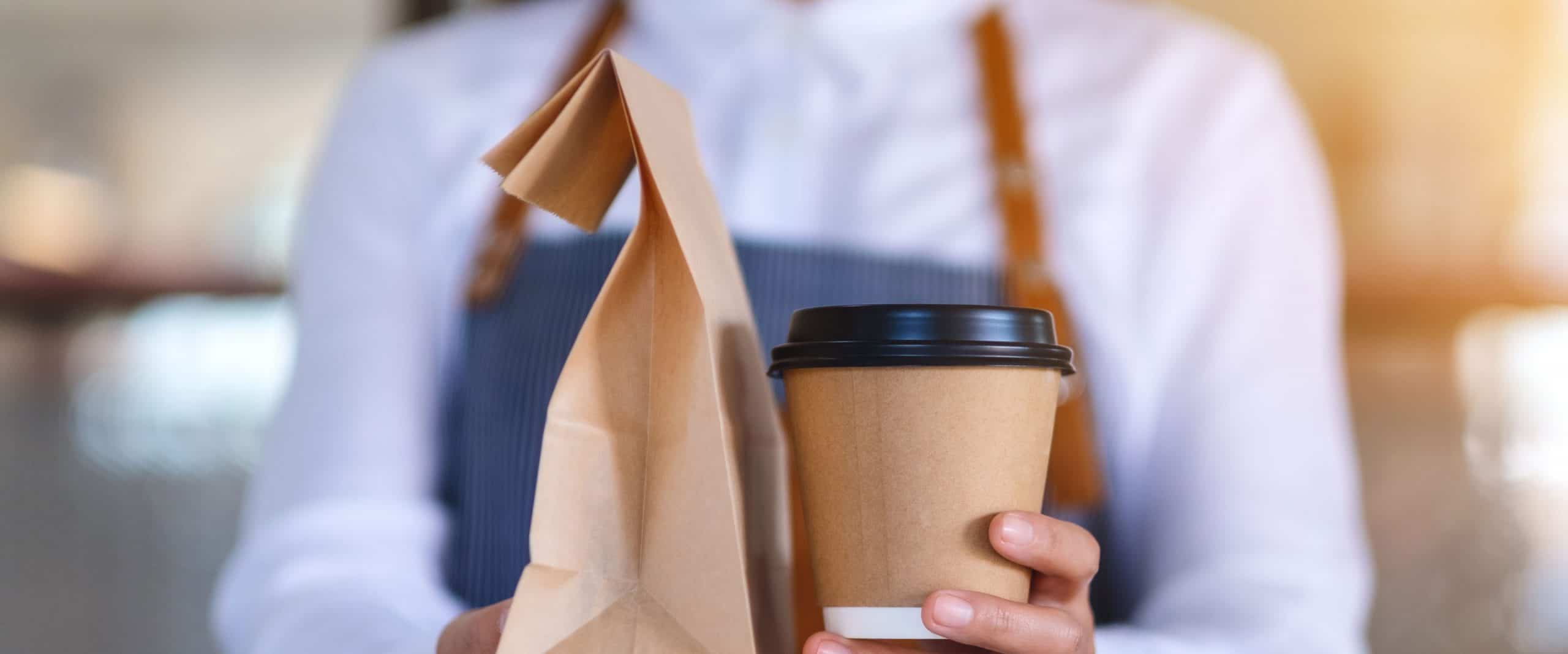
Customer satisfaction benchmarks and strategic insights for fast food restaurants
Understand the key drivers of satisfaction to build profitable customer loyalty by becoming an ACSI client today.
FAST FOOD RESTAURANTS
Customers are not starved for options when it comes to choosing a fast food business to visit. Stand out against the competition with ACSI benchmarking. Discover which elements of the customer experience keep your customers coming back and what you can do to attract more.
At a glance:
23 major chains, plus aggregate of smaller fast food outlets
Satisfaction Benchmarks by Company
Company | 2023 | 2024 | % CHANGE |
---|---|---|---|
Fast Food Restaurants | 78 | 79 | 1% |
All Others | 82 | 83 | 1% |
Chick-fil-A | 85 | 83 | -2% |
KFC (Yum! Brands) | 81 | 81 | 0% |
Arby’s (Inspire Brands) | 77 | 80 | 4% |
Culver’s | NM | 80 | NA |
Panera Bread | 76 | 80 | 5% |
Starbucks | 78 | 80 | 3% |
Domino’s | 78 | 79 | 1% |
Papa Johns | 80 | 79 | -1% |
Pizza Hut (Yum! Brands) | 78 | 79 | 1% |
Five Guys | 78 | 78 | 0% |
Burger King (RBI) | 76 | 77 | 1% |
Chipotle | 75 | 77 | 3% |
Dunkin’ (Inspire Brands) | 77 | 77 | 0% |
Panda Express | 77 | 77 | 0% |
Sonic (Inspire Brands) | 72 | 76 | 6% |
Wendy’s | 74 | 76 | 3% |
Little Caesars | 74 | 75 | 1% |
Dairy Queen | 75 | 74 | -1% |
Subway | 75 | 74 | -1% |
Taco Bell (Yum! Brands) | 71 | 73 | 3% |
Jack in the Box | 73 | 72 | -1% |
Popeyes (RBI) | 74 | 72 | -3% |
McDonald’s | 69 | 71 | 3% |
NM = Not Measured NA = Not Available
Fast Food Restaurant Benchmarks
Benchmarks | 2023 | 2024 |
---|---|---|
Accuracy of food order | 85 | 86 |
Quality of mobile app | 84 | 86 |
Reliability of mobile app (minimal down time, crashes, lags) | 84 | 85 |
Beverage quality (taste, temperature) | 83 | 84 |
Courtesy and helpfulness of staff | 83 | 84 |
Food quality (taste, temperature, freshness of ingredients) | 83 | 84 |
Restaurant layout and cleanliness | 82 | 83 |
Speed of check-out or delivery | 82 | 83 |
Website satisfaction | 83 | 83 |
Variety of beverages on menu | 80 | 82 |
Variety of food on menu | 80 | 82 |
Industry averages are weighted by companies’ market shares. 2024 results based on data collected April 2023 – March 2024.
Connect With ACSI
Want to get in touch with one of our experts? We’d love to hear from you. Reach out to us using the form below.
Academia.edu no longer supports Internet Explorer.
To browse Academia.edu and the wider internet faster and more securely, please take a few seconds to upgrade your browser .
Enter the email address you signed up with and we'll email you a reset link.
- We're Hiring!
- Help Center
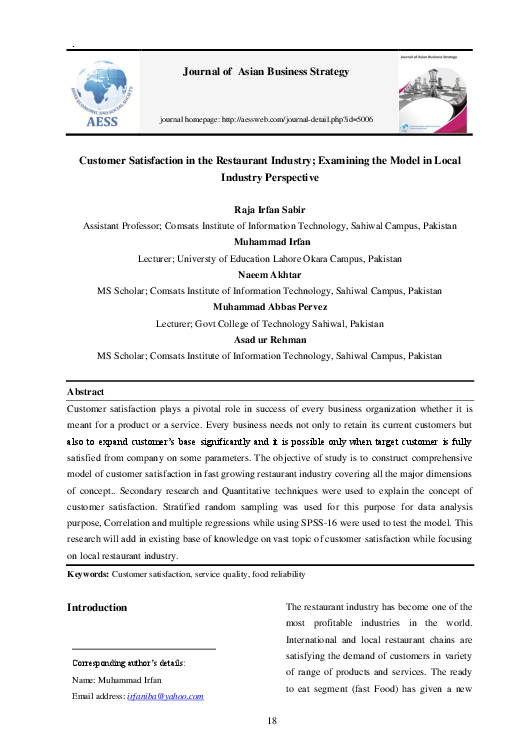
Customer Satisfaction in the Restaurant Industry; Examining the Model in Local Industry Perspective

Customer satisfaction plays a pivotal role in success of every business organization whether it is meant for a product or a service. Every business needs not only to retain its current customers but also to expand customer’s base significantly and it is possible only when target customer is fully satisfied from company on some parameters. The objective of study is to construct comprehensive model of customer satisfaction in fast growing restaurant industry covering all the major dimensions of concept.. Secondary research and Quantitative techniques were used to explain the concept of customer satisfaction. Stratified random sampling was used for this purpose for data analysis purpose, Correlation and multiple regressions while using SPSS-16 were used to test the model. This research will add in existing base of knowledge on vast topic of customer satisfaction while focusing on local restaurant industry.
Related Papers
Restaurant service industry is becoming more important in generating income in many countries at present. In this paper we use different factors that have a greater influence on customer satisfaction are employees performance, price, food quality and physical environment and we select fast food restaurant named FRIED CHICKS. Through simple random sampling a sample of 200 questionnaires is taken from youth in the Lahore city of Pakistan. The correlation and regression method is used to measure the relationship of customer satisfaction with these factors. The results show that customer satisfaction has a positive relationship with employee performance, food quality and physical environment and a negative relationship with price. So these factors play an important role in customer satisfaction.
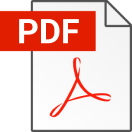
International Journal of Management and Accounting
Md. Rubel Hossain
At present, the service industry makes an important contribution to World's GDP, especially in Bangladesh. Customer satisfaction is one of the Catchwords of every service industry. The study is conducted to identify some key factors of customer satisfaction in the restaurant industry. In the Rangpur city area, 20 restaurants were selected to conduct the study. This research is based on an empirical study. Simple random sampling methods were used to select 120 customers. 5 points Likert scale and open-ended questions were used to prepare a questionnaire. This study tried to adjust customer’s perceptions with service quality for the customers’ satisfaction with the restaurant industry. Undesirable perceptions of customers were found concerning product and service quality, pricing policy, the value of the restaurant, and loyalty of those restaurants side by side concerning waiters' service and space convenience customers' perceptions were encouraging. Based on findings it w...
European Journal of Business and Management
Muhammad Murtaza
Structured questionnaire technique was used to determine the customer satisfaction. Data was gathered through snowball sampling and the model is tested. SPSS software analysis is used to determine the means and results are interpreted.
Danielle Mantho , Syed Andaleeb
International Review of Management and Business Research
Asad Rehman
The purpose this study is to investigate, compare and evaluate the determinants of the customers satisfaction in fast food industry. Quality service, price, environment are used as an independent variables and customers satisfaction use as dependent variable. The research is quantitative in nature and random sampling technique has been used for data collection through a questionnaire survey and the sample size for this study was 100. Data have been analyzed through correlation and, multiple regressions.
Customer retention is an important element of Fast Food Industry in today's increasingly competitive environment. FFRs management must identify and improve upon factors that can limit customer defection. These include employee performance and professionalism, willingness to solve problems, friendliness, level of knowledge, communication skills, and selling skills, among others. In Pakistan due to the increasing competition among the FFRs (Fast Food Restaurants) customer satisfaction has become a major ingredient to fast food companies for their success and growth. The present study provides the level of customer satisfaction of FFRs operating in Pakistan. To measure the level of customer satisfaction SERVQUAL Model was being adopted and descriptive statistics was used to analyze the data. The results of the study indicate that the customers of FFRs in Pakistan are not much more satisfied with the services provided by these restaurants.
Lima Nasrin Eni
To explore the determinants of customer satisfaction at Kishoreganj based fast-food restaurants in Bangladesh is the core objective of this research. The research design is descriptive and quantitative in nature where data was collected from 280 (sample size) frequent fast food experiencing customers comprising of students, job holders and others residing in Kishoreganj districts of Bangladesh, applying nonprobability convenience sampling technique through structured online survey questionnaire consisting of demographic data namely age, gender, occupation and income etc. and determinants of consumer satisfaction on five point Likert rating ranging from strongly disagree to strongly agree. Collected data was analyzed through multiple linear regressions by using statistical software SPSS version 20. Research findings show that at P < 0.05, four independent variables like price fairness (β =0.213), ambience (β =0.344), service quality (β =0.224), food quality (β =0.231), etc. are po...
Studia commercialia Bratislavensia
Shahzad Ahmad Khan
International Journal of Applied Sciences in Tourism and Events
Rafael M O D E S T U S Ziku
This study presents literature review relating to antecedents of customer satisfaction in food service business. The review is based on literatures about customer satisfaction in food service related business that have been published in peer reviewed journals in the period from 2012 to 2016. A total of 17 articles were reviewed. Through a systematic review process, the result of this study shows there are three major antecedents of customer satisfaction in food service related business, namely quality of service, food attributes, and atmospheric factors.
Muhammad Yasir Rafiq
Lahore City is famous for its food and culture in all over the world. The objective of this study is to determine how the quality of restaurants effect on customer satisfaction in Lahore. Survey method has been adopted and information and data was collected through structured questionnaire. There were 539 customers selected randomly from small, large and full service restaurants. Restaurant Quality was measure on the base of six dimensions: Price, Menu, Food Quality, Seating arrangement, Cleanliness and Service Quality. The demographic profile of customers are presented using frequency distribution. Moreover, considering six restaurant's quality dimensions as independent variables and customer's satisfaction as dependent variable, regression analysis has been performed. It has been reveled from results that of restaurant's quality dimension has positive and significant impact on customer's satisfaction. , regression
Loading Preview
Sorry, preview is currently unavailable. You can download the paper by clicking the button above.

RELATED PAPERS
Shikha Bhagat
Management Science Letters
ahmad torfi
Junaid Aftab , Entrepreneurship and Innovation Management Journal ISSN: 2310-0079
Noonamsom Narumon
Faaria Hassan
Binus Business Review
Budi Suharjo
International Journal of Economics, Business and Accounting Research (IJEBAR)
Sum Sochenda
IOER International Multidisciplinary Research Journal
IOER International Multidisciplinary Research Journal ( IIMRJ) , walter juera
Winston Asiedu Inkumsah
Dr Omkar Dastane
CHALLENGES OF TOURISM AND BUSINESS LOGISTICS IN THE 21ST CENTURY »ISCTBL 2022
Natasha Miteva
GRADIVA REVIEW JOURNAL
Dr. Bhavani Shree A R E P A L L I MBA
- We're Hiring!
- Help Center
- Find new research papers in:
- Health Sciences
- Earth Sciences
- Cognitive Science
- Mathematics
- Computer Science
- Academia ©2024

An official website of the United States government
The .gov means it’s official. Federal government websites often end in .gov or .mil. Before sharing sensitive information, make sure you’re on a federal government site.
The site is secure. The https:// ensures that you are connecting to the official website and that any information you provide is encrypted and transmitted securely.
- Publications
- Account settings
Preview improvements coming to the PMC website in October 2024. Learn More or Try it out now .
- Advanced Search
- Journal List
- Elsevier - PMC COVID-19 Collection

Customer satisfaction with Restaurants Service Quality during COVID-19 outbreak: A two-stage methodology
Masoumeh zibarzani.
a Department of Management, Faculty of Social Sciences and Economics, Alzahra University, Tehran, Iran
Rabab Ali Abumalloh
b Computer Department, Community College, Imam Abdulrahman Bin Faisal University, P.O. Box. 1982, Dammam, Saudi Arabia
Mehrbakhsh Nilashi
c Centre for Global Sustainability Studies (CGSS), Universiti Sains Malaysia, 11800, Penang, Malaysia
d UCSI Graduate Business School, UCSI University, No. 1 Jalan Menara Gading, UCSI Heights, 56000, Cheras, Kuala Lumpur, Malaysia
Sarminah Samad
e Department of Business Administration, College of Business and Administration, Princess Nourah Bint Abdulrahman University, Riyadh, Saudi Arabia
O.A. Alghamdi
f Business Administration Dept., Applied College, Najran University, Najran, Saudi Arabia
Fatima Khan Nayer
g Artificial Intelligence and Data Analytics (AIDA) Research Lab, College of Computer and Information Sciences, Prince Sultan University, Saudi Arabia
Muhammed Yousoof Ismail
h Department of MIS, Dhofar University, Oman
Saidatulakmal Mohd
i School of Social Sciences, Universiti Sains Malaysia, 11800, Penang, Malaysia
Noor Adelyna Mohammed Akib
Online reviews have been used effectively to understand customers' satisfaction and preferences. COVID-19 crisis has significantly impacted customers' satisfaction in several sectors such as tourism and hospitality. Although several research studies have been carried out to analyze consumers' satisfaction using survey-based methodologies, consumers' satisfaction has not been well explored in the event of the COVID-19 crisis, especially using available data in social network sites. In this research, we aim to explore consumers' satisfaction and preferences of restaurants' services during the COVID-19 crisis. Furthermore, we investigate the moderating impact of COVID-19 safety precautions on restaurants' quality dimensions and satisfaction. We applied a new approach to achieve the objectives of this research. We first developed a hybrid approach using clustering, supervised learning, and text mining techniques. Learning Vector Quantization (LVQ) was used to cluster customers' preferences. To predict travelers' preferences, decision trees were applied to each segment of LVQ. We used a text mining technique; Latent Dirichlet Allocation (LDA), for textual data analysis to discover the satisfaction criteria from online customers' reviews. After analyzing the data using machine learning techniques, a theoretical model was developed to inspect the relationships between the restaurants’ quality factors and customers' satisfaction. In this stage, Partial Least Squares (PLS) technique was employed. We evaluated the proposed approach using a dataset collected from the TripAdvisor platform. The outcomes of the two-stage methodology were discussed and future research directions were suggested according to the limitations of this study.
Credit author statement
Masoumeh Zibarzani: Conceptualization, Methodology, Investigation, Software, Data Curation, Formal analysis, Writing - Original Draft, Writing - Review & Editing, Validation. Rabab Ali Abumalloh: Conceptualization, Methodology, Investigation, Software, Data Curation, Formal analysis, Writing - Original Draft, Writing - Review & Editing, Validation. Mehrbakhsh Nilashi: Supervision, Conceptualization, Methodology, Investigation, Software, Data Curation, Formal analysis, Writing - Original Draft, Writing - Review & Editing, Validation. Sarminah Samad: Methodology, Writing - Original Draft, Writing - Review & Editing, Validation. OA Alghamdi: Writing - Review & Editing, Validation. Fatima Khan Nayer: Investigation, Writing - Review & Editing, Visualization. Muhammed Yousoof Ismail: Investigation, Writing - Review & Editing, Visualization. Saidatulakmal Mohd: Investigation, Writing - Review & Editing, Visualization. Noor Adelyna Mohammed Akib: Investigation, Writing - Review & Editing, Visualization.
1. Introduction
The COVID-19 pandemic developed an almost overnight world distribution and brought the global economy to its knees [ [1] , [2] , [3] , [4] ]. The hospitality industry, in particular, faced an unprecedented challenge. The lockdowns, social distancing, and travel and mobility restrictions stripped many hospitality businesses of their customers [ 5 ]. The pandemic has hit the restaurant industry the hardest. Restaurants had to limit their operations just to take-outs [ 6 ]. With every 1% increase in new daily COVID-19 cases, restaurants lost 0.06% of their daily orders [ 7 ].
While authorities have started to ease restrictions [ 2 , 3 ], the hospitality industry, including the restaurant sector, is beginning to recover from the outbreak's damage. But the profound impact of the COVID-19 crisis continues to play a role in hospitality businesses [ 8 , 9 ]. Customers are less willing to dine in restaurants [ 10 ]. Recent studies suggest that outbreak information cues, such as the number of new cases or deaths, encourage customers to seek safety and hence avoid particular services that increase their risk [ 11 , 12 ]. Consequently, the operations and business environment in the hospitality industry face substantial changes. There is a need for strategies to respond to threats and open new opportunities for the sustainability of the restaurant industry [ 6 ], by which businesses have to adapt to meet customers' expectations and enhance their willingness to patronize their businesses [ 13 ].
Unlike physical products, restaurant services are evaluated by the customer in a more complex way. Restaurants offer services that are largely experiential products [ 14 ] and, in that sense, they are evaluated based on a collection of cognitive and affective attributes [ 15 ]. Such motivations can reshape individuals' decision-making processes [ 2 , 3 ]. The survival of many hospitality businesses, including restaurants, which heavily rely on human-to-human contact, depends on increasing the demand for their services. Such businesses have a higher breakeven point, and it is of utmost importance to figure out what will make customers return. Customers' needs and preferences have to be re-evaluated. The main question is: What are the determinants of restaurant customers' satisfaction in a time of pandemic and what are the proper means of investigating customers’ behaviours and preferences?
Online customer reviews provide business owners with insights into the thoughts and feedback of customers during the outbreak and hence can be considered as good sources of information [ 16 ]. Online reviews could reveal the main concerns of customers [ 11 ], identify the factors that can lead to a customer being satisfied or dissatisfied with a service [ 17 ], and could be used to estimate customers’ satisfaction levels [ 18 ].
Data analysis tools and approaches need to be upgraded constantly to meet the new requirements of data collection and analysis of online reviews. Researchers have focused on developing new approaches to capture customers' sentiments during the outbreak. Few studies showed a shift in customers' review patterns due to the outbreak [ 6 , 14 , 19 ], while others reported only slight changes in the evaluation of restaurant features [ 20 , 21 ]. However, only a limited number of studies are available, and there is a research gap that offers new ways to observe and interpret customers’ evaluations of restaurants.
This study aims to interpret customers’ evaluations of restaurants' services and satisfaction levels during the COVID-19 outbreak. A new method based on machine learning techniques was developed using social data, which is represented in the forms of textual comments and ratings. The online textual reviews and ratings were collected from TripAdvisor. The main contributions of this work to the body of knowledge are as follows:
- i. Although several studies have been carried out to analyze consumers' satisfaction using survey-based methodologies, consumers' satisfaction has not been well explored during the COVID-19 outbreak, especially through the use of social data that are posted on social networking sites. In this research, we explore consumers' satisfaction and their preferences of restaurants' services during the COVID-19 crisis. The moderating effect of COVID-19 safety precautions on restaurants' quality dimensions and satisfaction is investigated as well.
- ii. The first stage consisted of the analysis of social big data. The use of Latent Dirichlet Allocation (LDA) for text mining was applied to discover the satisfaction dimensions. The model has been used to analyze online textual reviews effectively in this study. During the outbreak and after loosening the confinement situation, it is of utmost importance to extract the indicators of customers' satisfaction and to improve the service quality based on customers' opinions that are reflected in online reviews. In the context of restaurants, just a few studies have explored customers' satisfaction during the pandemic, and the issue is not fully explored. More importantly, there is a need to develop new methods to investigate the reviews accordingly.
- iii. We investigate big data consisting of the reviews that customers have generated using a hybrid approach of text mining, clustering, and prediction learning techniques. We used Learning Vector Quantization (LVQ), a supervised classification algorithm, to segment and groups travelers with similar preferences. Later, based on a set of performance criteria for restaurants, customers' satisfaction was predicted using Classification and Regression Trees (CART).
- iv. In the second stage, based on the Stimulus-Organism-Response (SOR) model a set of hypotheses were developed to investigate the relationships between the performance criteria and satisfaction, considering the moderating effect of the COVID-19 safety measures. We used Partial Least Squares Structural Equation Modeling (PLS-SEM) and analyzed data from customers who had previous experience with the TripAdvisor platform. We also performed a subgroup analysis to examine the proposed research model based on the type of travel.
- v. The results of the two-stage methodology were combined to provide a rich discussion and future guidelines to contribute to the decision-making in complex situations like the current pandemic.
The remainder of this paper is organized as follows: In Section 2 and Section 3 , we present the related work and theoretical background. Section 4 discusses the analysis of online reviews using machine learning. In Section 5 and Section 6 , we present the research hypotheses and PLS-SEM results. In Section 7 , a discussion of the results is provided. In Section 8 , we conclude our work. The list of abbreviations used in this study is presented in Table 1 .
List of acronyms used in this study.
Acronyms | Description |
---|---|
CART | Classification and Regression Tree |
LDA | Latent Dirichlet Allocation |
LVQ | Learning Vector Quantization |
NLP | Natural Language Processing |
PCA | Principal Component Analysis |
PLS-SEM | Partial Least Squares Structural Equation Modeling |
SOM | Self-Organizing Map |
SVM | Support Vector Machine |
CV | Convergent Validity |
IC | Internal Consistency |
DV | Discriminant Validity |
CR | Composite Reliability |
AVE | Average Variance Extracted |
CA | Cronbach's Alpha |
CL | Cross-Loadings |
FL | Fornell-Larcker |
Q | Stone-Geisser's Q Square |
R | Coefficients of Determination |
SOR | Stimulus-Organism-Response |
2. Related works
2.1. covid-19 and hospitality industry.
The COVID-19 pandemic posed unprecedented challenges to businesses around the world, and the hospitality industry was no exception [ 2 , 3 ]. The industry relies heavily on customer demand to remain viable. This has created a need for intensive research efforts to help the industry adjust its operations in the time of the pandemic. The hospitality industry is recovering, albeit slowly. Hospitality businesses, due to their reliance on the physical human-to-human interaction, had to implement drastic changes to their operations to win back customers [ 5 , 13 ] and to ensure employees' and customers’ safety [ 2 , 3 ]. However, studies show that customers are still reluctant to stay in hotels or dine in restaurants [ 10 ]. Google Trends data shows a noticeable decline in the trend in the use of hospitality services [ 22 ]. OpenTable has created an online data showcase that monitors the state of restaurants worldwide [ 23 ]. This ongoing study shows that the industry has not been able to reach the number of customers they had before the pandemic. Even though the decline in customer numbers has recovered from 99% in March 2020 to 40% in March 2021, data indicate a significant drop in seated customers at a global level.
Despite all the industry efforts, consumers' behaviour is still impacting the restaurant industry. The pandemic has had effects on people's decision-making, emotions, and perceptions [ 24 ]. It has shifted customers' emotions toward negativity [ 25 ], partly due to the excessive mental load that people experience to ensure the health of their families and loved ones [ 24 ] and their loss of freedom [ 25 ]. These, in conjunction with external factors, have posed a challenge to the previous findings on sentiment analysis in the field of marketing [ 19 , 26 , 27 ].
With changing consumer behaviour, scholars have responded to emerging needs. There has been a shift in the hospitality marketing and management field. Questions about what factors encourage customers to return, their sentiment about restaurants, and their perception of the ‘new normal’ in the industry remain unanswered. The argument on the future of hospitality industries in the pandemic is still ongoing, and research is required to pinpoint how the industry might continue to remain viable.
2.2. Online review of hospitality
Online reviews are one of the most typical forms of information-sharing concerning customers' behaviour [ 6 , 28 ]. There is a distinction between two types of online reviews: consumer-generated reviews and professional reviews, where the former are provided by customers and the latter are written by professional editors [ 29 ]. However, prior research has shown that consumer-generated reviews are positively associated with the online popularity of reviewed businesses. This effect is not present with editor reviews. Reviews offer a description of the customer's experiences in a textual format, and hence a qualitative style [ 6 ] and have an impact on the behaviour of other consumers [ 30 , 31 ]. Reviews are written from a customer's perspective [ 32 , 33 ], and potential clients perceive them as reliable and relevant information [ 34 , 35 ]. Their effect is of a real-time nature [ 36 ], which can address the changing demands of customers [ 37 ].
Hospitality research has been paying attention to customer reviews for more than a decade [ 29 ]. Previous studies have shown that tourists prefer recommendations over advertisements when they choose a restaurant [ 9 , 38 ]. Customers tend to share their reviews aiming to inform other customers [ 39 ]. To understand what leads customers to share their reviews, three motivations are identified; self-focused, other-focused, and company-focused reasons [ 40 ]. Konuk [ 39 ] showed that customers' tendencies to share their feedback online increase when they are satisfied with the product or service. However, when a product or service disappoints the customer, he/she tends to share even more, to taint the company's image or to inform other customers [ 39 , 41 ].
With the growth in the volume of the information shared by customers on the internet, thousands of consumers can discuss and evaluate products and services [ 42 ] and, as a result, online reviews have become a critical source of information [ 43 ]. Compared to surveys, the traditional sources of information about customers, online reviews are less costly and more easily collected [ 44 , 45 ]. Consequently, companies consider them as alternative data sources that are easier to manage [ 37 ]. On the other hand, the use of text-mining technology has enabled researchers to discover new information and analyze online reviews automatically [ 6 ].
2.3. Evaluating customer satisfaction through online reviews in the hospitality industry
Researchers have used online reviews to explain customer satisfaction or dissatisfaction [ 31 , 46 ] from the marketing and hospitality research field, as well as in other disciplines [ 6 , 37 , 47 , 48 ]. In addition, previous works have shown that the volume of reviews (the number of comments or ratings) could be used as an indicator of satisfaction [ 49 , 50 ].
In the hospitality context, both reviews and ratings have been used to assess the degree of satisfaction. Ratings of hotels, restaurants and the like could shed light directly on the levels of customer satisfaction and dissatisfaction, as well as their causes [ 51 ], providing restaurant quality-improvement hints [ 52 ]. There are earlier works that determine customer satisfaction that rely on ratings (e.g. Refs. [ 53 , 54 ]). However, the majority of research has focused on reviews or on a combination of reviews and ratings.
Previous studies on online reviews have extended the traditional approach to understanding customer satisfaction, taking into account the real opinions of travellers reflected in those reviews. Rajaguru and Hassanli [ 55 ] used online reviews coupled with ratings to investigate customer satisfaction with hotels. They confirmed that customers’ perceptions of value for money are affected by hotel star ratings. Bi et al. [ 32 ] combined neural networks and an effect-based Kano model to study customer satisfaction via online reviews. Huifeng et al. [ 33 ]; using both reviews and ratings, investigated the relationship between online customer reviews and restaurant revisits and showed that the effect declines over time. Padma and Ahn [ 17 ] performed a content analysis of online reviews and ratings available on TripAdvisor to examine the satisfaction and dissatisfaction of luxury hotel customers. Studies have explored the sentiment of reviews as an indicator of customer satisfaction [ [56] , [57] , [58] ]. Tao and Kim [ 59 ] used online comments and ratings of cruise-ship customers to gauge their experiences of the service and predict their levels of satisfaction. A fuzzy evaluation method has been designed to calculate customer satisfaction based on online reviews [ 18 ].
Using text-mining approaches, various factors that lead to satisfaction or dissatisfaction have been identified in previous studies. Xu [ 60 ] stressed that the performance of the drivers and the cost of the order affect both the satisfaction and the volume of takeaway restaurants' reviews. Bilgihan et al. [ 61 ] detected three distinct types of customer perceptions that are reflected in their reviews: functional, mechanic, and humanistic. In another study, six emotions expressed in reviews were identified: joy, sadness, anger, fear, trust, and disgust, which could be indicators of their levels of (dis) satisfaction [ 62 ]. In another example of text-mining technology, Yan et al. [ 63 ] analyzed online reviews from a local online community in China. They found that, on the topic of restaurants, variables such as price, value, and atmosphere discussed in reviews predicted customers' revisit intentions. Their results confirmed the findings of a similar study that used sentiment analysis of Google Maps reviews [ 64 ]. Li, H. et al. [ 65 ] did not find the price to be a key indicator based on Airbnb users’ experience. Other features that customers value include service, food, place, performance, excitement factors, amenities, waiting time, location, brand, and experience [ 14 , 21 , [63] , [64] , [65] , [66] , [67] , [68] ].
2.4. Evaluating service quality through online reviews in the hospitality industry
Service quality could be defined as the contrast between what the customer expects and what they receive [ 69 ]. Businesses assess their service quality, based on customers’ perceptions, to create new opportunities [ 70 ]. The quality of services could temper customer satisfaction, loyalty [ 71 ] and company profitability [ 72 ] and hence the survival of the business [ 73 ]; this includes the hospitality industry [ 74 ]. Businesses improve their services, trying to reach higher levels of satisfaction, hoping to benefit from a larger share of the market [ 75 ].
For years, service management scholars and practitioners have been trying to improve the means of measuring service quality. The indicators of good service quality differ depending on the context [ 76 ]. The same is valid for the level of service quality, which depends on the product category [ 77 ]. In addition, many models and their subsequent results lack tangibility and do not properly shed light on customers' service experiences in reality. In order to compensate for the shortcomings of previous studies, many approaches have been developed to assess ‘perceived’ service quality based on information on customer experiences [ 78 , 79 ].
Customers' reviews could serve as valuable sources of such information [ 67 ]. To draw inferences from customers’ reviews, an accurate systematic analysis, which allows the identification of relevant service quality dimensions reflected in online reviews, helps to assess the service quality in a proper manner [ 72 ]. Service quality has been framed as a multidimensional and hierarchical construct [ 78 , 80 ]:
- i. Interaction: Interaction is the perception of customers of their interactions with employees when they receive a service. Yan et al. [ 63 ] conducted a content analysis on 10,136 restaurant reviews in an online life community in China and found two indicators of service quality: employee appearance and employee attitude. Clemes et al. [ 81 ] discussed that the quality of interaction could be measured via the three dimensions of interpersonal, professional and problem-solving skills. Some authors portrayed interaction quality in terms of employee characteristics, such as their reliability [ 15 ], responsiveness [ 82 ], assurances [ 15 ], inclusiveness [ 83 ], performance and empathy of service professionals and courteous attitude [ 15 , 74 ]. Other attributes expected of service employees include the amount of help they are willing to offer, their extent of being friendly or knowledgeable about what they do, attention to specific needs of customers, and the accuracy, reliability, and promptness of the service provided [ [84] , [85] , [86] ].
- ii. Physical environment: Physical environment consists of the surrounding built environment, whether manmade, natural, or social [ 81 ], which influences the perceptions of overall quality [ 14 , 87 ] and might eventually influence customers' satisfaction [ 88 ]. Moreover, it plays a critical role in shaping customers' experiences [ 89 , 90 ], and it could provoke customers' positive emotions. Previous works assert that the arousal of positive emotion has a strong impact [ 91 ]. The physical environment has been divided into sub-dimensions, including ambient conditions, facility aesthetics, the interior, exterior and other important tangible factors [ 92 ], spatial layout, seating comfort, view, location, occasions and noise level [ 93 ], elements such as service facilities, equipment, cleanliness and transportation [ 94 ] and physical appearance [ 15 , 75 , 85 , 91 , [95] , [96] , [97] , [98] , [99] ].
- iii. Outcome quality: Outcome quality is the technical quality as evaluated during service delivery [ 78 ], which determines customers' perception of service quality [ 100 ]. Service attributes determine a customer's overall experience [ 101 ] and, considering the restaurant context, have been measured via factors regarding the food and the menu [ 84 , 102 ]. Attributes such as service responsiveness, reliability, serviceability, cleanliness, safety, maintenance of the facility and price, together with elements such as service facilities, equipment, conformance levels and product/destination image, have also been investigated [ 72 , 82 , 92 , 93 , 96 ].
2.5. Customer online reviews before and during the COVID-19 outbreak
In the previous sections, we established that researchers have been studying online information sharing and customer reviews in the hospitality industry long before the COVID-19 outbreak (e.g. Refs. [ 9 , 51 , 103 , 104 ]). This pandemic has hit the restaurant industry hard, such that for every 1% increase in daily new COVID-19 cases, restaurants reported a 0.06% decrease in their orders [ 7 ], which harmed their revenue [ 6 ]. The outbreak has changed customers' needs and preferences. While their preferences concerning food, environment, and service remain salient and important in the long run, efforts have been made to observe and interpret new changes in restaurant customers' preferences due to the pandemic [ 21 ]. Findings suggest a shift in customers' review patterns. They have been assigning lower ratings [ 6 ] and have been evaluating the same restaurant features differently. For example, Jia [ 6 ] suggested that customers were less annoyed by queuing, a feature that raised negative feedback in normal times. The features considered important by customers have changed in some cases. Luo and Xu [ 21 ] reported more frequent use of terms such as ‘delivery’ and ‘online ordering’ in the area of takeaways and ‘hygiene practices’ and ‘outdoor seating’ regarding dine-in experiences in Yelp reviews compared to previous reviews. Yang et al.‘s [ 14 ] analysis of reviews showed a more salient focus on packaging and delivery quality, in addition to hygiene, due to the COVID-19 pandemic. Another study reported an increase in the values customers placed on delivery and customer service provided by meal-kit companies after the outbreak, while the freshness and type of food served had lost some of their precedence [ 20 ]. The value placed on features such as safety, social distancing and mask policies are considered as important as previously dominant features such as service, overall experience and food quality [ 16 ]. Kutlubay et al. [ 105 ] examined the differences in comments and ratings provided by customers before and after the COVID-19 outbreak. They showed that the customer ratings had dropped during the pandemic. Their results also indicated a higher number of negative emotions in reviews in the early period of the outbreak. In contrast, Sun et al. [ 106 ] found that customers were more prone to post positive reviews and higher ratings after the COVID-19 outbreak if the service provider had implemented safety measures strictly. Another study reported a difference in customer reactions to services before and during the pandemic [ 107 ]. There have also been several reports about the changes in customer preferences for services [ [108] , [109] , [110] ].
3. Theoretical background
The second phase of the study is designed to investigate the role of the extracted performance and satisfaction criteria. Appraisal theory points out that customers evaluate a service's performance upon getting exposed to an environmental stimulus [ 111 ], but it is not clear how they would react to that stimulus. The stimulus can provoke positive and negative sentiments that impact customers' evaluation process. This is explained in the expectancy disconfirmation theory [ 112 ]. The theory explains that customer satisfaction is related to the prior expectation of the service. Although previous research investigated the relationship between customers' evaluation and their satisfaction, their expectations depend on several dimensions of the product or service. Customers' expectations vary as they have different perceptions of the same event [ 19 ]. Expectations depend on multiple dimensions that have been investigated focusing on various aspects in previous studies. The Stimulus-Organism-Response (SOR) model [ 113 ] has been used in the literature to study the stimuli and the behavioral responses. The model has been used to explain customers' expectations, based on their evaluation of the service and their overall satisfaction [ 114 , 115 ]. Additionally, the SOR model differentiates between the stimulus or environmental stimulus (a set of sensory variables) and the response, calling the former as the independent variable and the latter as the dependent variable. The stimulus influences the customers' organism, which is the internal process that intermediate the relationship between the stimulus and response. Customers' organism is reflected by the emotional reactions (in the forms of arousal and pleasure) [ 116 , 117 ]. These variables have been investigated in the hospitality industry literature [ 118 ], and there is a wide range of variables that directly influence customers' responses. Multiple studies have suggested various variables as moderators as well [ [119] , [120] , [121] ].
Hence, the SOR paradigm will be adopted in this study to explain what factors that have an impact on customer satisfaction for several reasons. First, the SOR model has been broadly applied to explain consumer behaviour [ [122] , [123] , [124] ]. This model has been used in the Information System literature and has been proven to be highly effective. It has been utilized in the hospitality context in explaining the relationships among the service features (stimuli), customers' emotions (organism), and eWOM (response) [ [125] , [126] , [127] ]. Its effectiveness has been also proven in explaining consumers' responses during the recent pandemic [ 128 ]. For example, Liu et al. [ 123 ]) adopted the SOR model to investigate the influence of task-related signs and mood-related signs on perceived enjoyment and perceived usefulness, and accordingly the intention to purchase. Zhang et al. [ 129 ] inspected the impacts of three variables (sociability, personalization, and interactivity) on the virtual experience of customers. Second, the SOR model presents a precise and structured method to explore the influence of interpersonal interaction variables, as surroundings stimuli, on customers’ overall experiences and their future intention and behaviour.
4. Machine learning methodology
The proposed method, which is based on machine learning techniques, is presented in Fig. 1 . The main goal of the study is to examine the available information on restaurant websites to assist travelers in making decisions. Numerical ratings of quality aspects and textual reviews of services are two important types of information that are available on restaurant websites. The numerical ratings are based on the level of quality (e.g., food, service, value, and atmosphere). We used LDA to discover satisfaction dimensions from the data that was collected from restaurants' websites on TripAdvisor. LDA is a foundational scheme in the field of topic modeling, and because of its flexibility, it allows complex analyses of textual data. LDA allows the extraction of latent topics from large amounts of unstructured review data. To determine the aspects of consumer satisfaction, we use LDA. For the numerical reviews of travelers, our method included clustering analysis. It is crucial to look for groups of travelers with similar tastes based on the data posted on restaurants’ websites. Customers' reviews on services can be clustered to produce more accurate predictions of travelers' preferences for restaurants services. LVQ is the basic method that we used for clustering analysis. Finally, we used the CART technique to predict the preferences based on quality factors.

The proposed method.
4.1. Data collection and analysis
TripAdvisor was used to obtain data for this study. The information was collected from the restaurants' websites, which are provided on the TripAdvisor platform. The data was acquired using a crawler that crawled restaurants' information through their URLs. The crawler was built to collect key data such as restaurants' information, traveler information (Travelled with family, Travelled solo, Travelled with friends, and Travelled as a couple), trip information, and users' ratings of Food, Service, Value and Atmosphere. Totally, We gathered 2158 records from 50 restaurants using the crawler. The data was preprocessed, and the database's useless records were deleted. Additionally, non-English reviews were removed from the collected data at this point. Moreover, records that do not include ratings for restaurant features (such as food, service, value, and atmosphere), or do not contain information about restaurants or travel were excluded.
We applied LDA on the textual data to generate the satisfaction dimensions. Then, the data were divided into two main groups, restaurants with COVID-19 safety precautions, and restaurants with no COVID-19 safety precautions. Then, we applied LVQ clustering to the whole dataset. The learning rate for LVQ was set to 0.05. For restaurants with COVID-19 safety precautions and restaurants with no COVID-19 safety precautions, data were clustered in 3 segments. Segment 1, Segment 2, and Segment 3 including, 828 (38.4%), 352 (16.3%) and 978 (45.3%) records, respectively. The coefficient of determination (R 2 ) values for clusters was 0.871, indicating that LVQ has generated high-quality segments. In Table 2 , the cluster centroids are presented. In Table 3 , we present LVQ segments, COVID-19 safety precautions, and level of satisfaction. In Table 1 of Appendix A , we present LVQ segments, COVID-19 safety precautions, and level of satisfaction in four groups. In Fig. 2 , we present a part of the generated trees. Users can rate restaurants by giving them a rate from 1 to 5 stars on TripAdvisor with respect to four criteria, namely food, service, value, and atmosphere [ 130 ]. Previous studies have successfully used the restaurant attributes to explain the customers’ decision-making process in the context of restaurants [ 131 , 132 ]. We also added COVID-19 safety precautions to the list in light of recent changes with the emerging COVID-19 crisis [ 2 , 3 , 133 ]. The CART technique was used to predict the level of satisfaction in 3 segments according to the aforementioned variables as the inputs.
Cluster centroids.
Attribute | Segment 1 (LVQ1 × 1: 828) | Segment 2 (LVQ2 × 1: 352) | Segment 3 (LVQ3 × 1: 978) |
---|---|---|---|
Food | 3.624396 | 4.275568 | 3.505112 |
Service | 2.553140 | 2.579545 | 4.566462 |
Value | 3.160628 | 4.573864 | 3.992843 |
Atmosphere | 2.786232 | 4.477273 | 2.617587 |
LVQ segments, COVID-19 safety precautions and level of satisfaction.
Customer Satisfaction Level | LVQ Segments | |||||
---|---|---|---|---|---|---|
Segment 1 | Segment 2 | Segment 3 | ||||
COVID-19 Safety Precautions | No | Customer Satisfaction Level | High | 0 | 33 | 47 |
Low | 350 | 39 | 192 | |||
Moderate | 83 | 102 | 243 | |||
Yes | Customer Satisfaction Level | High | 210 | 167 | 414 | |
Low | 24 | 1 | 3 | |||
Moderate | 161 | 10 | 79 |

CART analysis results.
5. Research model and hypotheses
Based on the literature, the current study develops a research model referring to the SOR model and analyzes the prominent emotion-inducing factors investigated in the first stage of the study to predict the potential customers' responses during the current pandemic. Therefore, the stimuli are four criteria, namely food, service, value, and atmosphere [ 131 , 132 ], whereas, the organism is the positive feelings toward the restaurant services which are presented in the form of satisfaction. In this study, the responses are the consumer's intention to choose a restaurant as reflected in their reviews and ratings. Based on that, the following hypotheses are provided:
The quality of food has an impact on customers' satisfaction with the restaurant.
The provided service has an impact on customers' satisfaction with the restaurant.
The perceived value has an impact on customers' satisfaction with the restaurant.
The atmosphere has an impact on customers' satisfaction with the restaurant.
We argue that the ambiguity the customers face during the COVID-19 pandemic has a moderating effect on the relationship between stimulus and organism. As the SOR framework suggests, customers might need to consider the safety precautions in their decision-making process. Previous studies have reported that consumers are sensitive to restaurants’ safety measures during the pandemic [ 134 ] with more focus concerning the COVID-19 safety guidelines in restaurants [ 16 ] as well as other sectors [ 106 , 135 ]. The atmosphere, on the other hand, could be affected by the safety measures as well. Based on this, we present the following hypotheses:
COVID-19 safety precautions have a moderating impact on the relationship between the provided service and customers' satisfaction with the restaurant.
COVID-19 safety precautions have a moderating impact on the relationship between the atmosphere and customers' satisfaction with the restaurant.
We present the research hypotheses and the initial research model in Fig. 3 .

Initial research model.
6. PLS-SEM methodology
6.1. structural equation modeling.
To assess the research model, PLS-SEM was used, in which both the outer model and the inner model were examined. The survey was answered by 1358 participants who had previous experience with the TripAdvisor portal. The questionnaire entailed three main parts to allow the participants to read a preface about the goal of the research, followed by simple demographic questions, and finally the main questions of the survey. To gather the data, the researchers distributed a large-scale survey for six months, starting from January 2021. Demographic data are displayed in Table 4 . Survey indicators with supporting previous literature are displayed in Table 1 of Appendix B .
Demographic results of the participants (N = 1358).
Feature | Item | Frequency | Percentage |
---|---|---|---|
Gender | Female | 670 | 49.34 |
Male | 688 | 50.66 | |
Age | 18–20 | 148 | 10.89 |
21–30 | 547 | 40.28 | |
>30 | 663 | 48.82 | |
Marital status | Married | 610 | 44.92 |
Single | 748 | 55.08 | |
Occupation | Employee | 525 | 38.66 |
Employer | 350 | 25.77 | |
Student | 165 | 12.15 | |
Retired | 208 | 15.31 | |
Others | 110 | 8.1 | |
Usage of TripAdvisor for Booking Restaurants in the Last Six Months | 1-3 times | 450 | 33.14 |
4-6 times | 375 | 27.61 | |
Over 6 times | 533 | 39.25 | |
Mode of Travel | Family | 373 | 27.48 |
Solo | 294 | 21.65 | |
Friends | 337 | 24.81 | |
Couples | 354 | 26.07 |
The outer model and the inner model should be inspected in terms of several tests to check the reliability and validity of the model of the study. SmartPLS software ( www.SmartPLS.com ) was used to perform the analysis tests. The factors of the model and the relationships among these factors should be examined (Hair et al., 2016). The reason for the choice of the SmartPLS is that it enables the evaluation of small and large-sized samples. As we aimed to analyze the groups of the participants based on their mode of travel, the choice of SmartPLS was suitable.
- i. Assessment of the Outer Model
SmartPLS was used to inspect the outer model in terms of reliability and validity by conducting three main tests: Convergent Validity, Internal Consistency, and Discriminant Validity (we referred to them as CV, IC, and DV, respectively). CV measure inspects the items of the survey considering their outer loadings, as the least acceptable value for each indicator should be 0.7 [ 136 ], otherwise, it could be removed based on the results of Composite Reliability (CR) or Average Variance Extracted (AVE) tests. Based on this rule, and referring to the test result, all the items in the survey were kept in the research model. The second assessment of CV evaluation is the AVE test, which checks the degree of interrelation among the items of a particular factor. The result of the test should surpass 0.5 for all factors, which was met for all factors. Following that, the IC needs to be checked based on Cronbach's Alpha (CA) and Composite Reliability (CR) tests. CA result should surpass the value of 0.7 for all the factors. On the other hand, the CR test should have values above 0.7 for each factor. CA and CR tests were confirmed in this study based on the result, as presented in Table 5 .
Constructs’ reliability and validity.
Construct | CA | CR | AVE |
---|---|---|---|
Atmosphere | 0.762 | 0.811 | 0.518 |
COVID-19 Safety Precautions | 0.743 | 0.836 | 0.56 |
Customer Satisfaction | 0.766 | 0.863 | 0.679 |
Food | 0.767 | 0.838 | 0.512 |
Service | 0.816 | 0.873 | 0.579 |
Value | 0.868 | 0.902 | 0.648 |
Cross-Loadings (CL) and Fornell-Larcker (FL) measures were performed to inspect the Discriminant Validity (DV) of the research model. FL compares the level of divergence among variables with the AVE value of the individual variables. Finally, the outer loadings of all the items for a particular variable need to be higher than their cross-loadings (in the CL test). Each of the FL and CL tests achieved the required conditions, indicating the validity of the research variables. Table 6 presents the FL test, while the result of the CL test is presented in Table 1 of Appendix C .
- ii. Assessment of the Inner Model
Fornell-larcker criterion.
Construct | Atmosphere | COVID-19 Safety Precautions | Customer Satisfaction | Food Quality | Services | Value |
---|---|---|---|---|---|---|
Atmosphere | ||||||
COVID-19 Safety Precautions | 0.541 | |||||
Customer Satisfaction | 0.72 | 0.423 | ||||
Food Quality | 0.595 | 0.68 | 0.618 | |||
Services | 0.545 | 0.748 | 0.486 | 0.694 | ||
Value | 0.618 | 0.633 | 0.437 | 0.648 | 0.767 |
Three main measures should be inspected to examine the structural model. First, for the Path Coefficient (PC) test, bootstrapping technique was conducted using the SmartPLS tool. The significance threshold that should be met for research hypotheses is p < 0.01 (Hair et al., 2015). The paths between food and travelers' satisfaction, service and travelers' satisfaction, value and travelers' satisfaction, atmosphere and travelers’ satisfaction were proved to be valid and significant. Additionally, the path between COVID-19 safety precautions and travelers' satisfaction was confirmed (p < 0.01). Path coefficient results are presented in Table 7 .
Path coefficient result (N = 1358).
Hypotheses | Link | β | t-value | p-value | Supported |
---|---|---|---|---|---|
Food - > Customer Satisfaction | 0.336 | 11.252 | 0 | Yes | |
Service - > Customer Satisfaction | 0.224 | 6.979 | 0 | Yes | |
Value - > Customer Satisfaction | 0.259 | 7.466 | 0 | Yes | |
Atmosphere - > Customer Satisfaction | 0.734 | 31.012 | 0 | Yes | |
Moderating Effect 1 - > Customer Satisfaction | 0.098 | 4.802 | 0 | Yes | |
Moderating Effect 2 - > Customer Satisfaction | 0.092 | 3.893 | 0 | Yes | |
Additional | COVID-19 Safety Precautions - > Customer Satisfaction | 0.218 | 7.22 | 0 | Yes |
Next, the Coefficients of Determination (R 2 ) measure is used to inspect the predictive accuracy of the model by investigating the ratio of the change of the endogenous construct through evaluating its exogenous constructs [ 136 ]. The value of R 2 should fall in the interval of 0–1, indicating more predictive accuracy with higher outcomes [ 136 ]. Based on the result, customers’ satisfaction factor has R 2 value of 0.679, which is considered high. This result indicates that the model has high predictive accuracy.
Finally, the predictive relevance (Q 2 value) measure was performed. The predictive relevance has to be more than zero for the endogenous construct. The blindfolding technique was used to calculate the Q 2 measure using the SmartPLS package. Based on the test's result, customers' satisfaction achieved Q 2 values more than zero (0.445), indicating the predictive validity of the hypothesized research model. Referring to the outcome of these three measures, the final research model was given in Fig. 4 .

Final research model.
- iii. The Moderating Impact
In this research, the moderation effect of COVID-19 safety precautions on two relationships was inspected. The moderation impact indicates the external influence of a factor on a specific path in the research model, in which this link becomes stronger or weaker based on this influence [ 136 ]. The first moderating effect was significant and the results indicated that the COVID-19 safety precautions strengthened the positive relationship between service and customer satisfaction ( Fig. 5 a). The moderating effect of COVID-19 safety precautions on the positive relationship between the atmosphere and customers’ satisfaction was also found to be significant ( Fig. 5 b).

The moderating impact of COVID-19 safety precautions.
- iv. The Subgroups Analysis
In this research, we aimed to explore the research model based on the travel mode. Hence, referring to Table 4 , the distribution of respondents in this research based on the mode of travel is as follows: families: 373, solo: 294, friends: 337, couples: 354. Following that, the inner model was examined for each subgroup and the research paths were inspected. As Table 8 displays, the research paths were supported in all four groups. The difference appeared in the moderating impact, as in group 1 the two moderating impacts were not supported, while in the third and fourth groups, only the second moderating impact was rejected. On the other hand, the analysis of the paths based on the second group presented the support to both moderating impacts.
Path coefficient result of subgroups.
Group | Hypotheses | Link | β | t-value | p-value | Supported |
---|---|---|---|---|---|---|
Group 1 (N = 294) Solo | Food - > Customer Satisfaction | 0.308 | 4.523 | 0 | Yes | |
Service - > Customer Satisfaction | 0.217 | 2.69 | 0.007 | Yes | ||
Value - > Customer Satisfaction | 0.276 | 3.675 | 0 | Yes | ||
Atmosphere- > Customer Satisfaction | 0.757 | 15.621 | 0 | Yes | ||
Moderating Effect 1 - > Customer Satisfaction | 0.101 | 1.906 | 0.057 | No | ||
Moderating Effect 2 - > Customer Satisfaction | 0.076 | 1.304 | 0.193 | No | ||
Additional | COVID-19 Safety Precautions - > Customer Satisfaction | 0.198 | 2.834 | 0.005 | Yes | |
Group 2 (N = 354) Couples | Food - > Customer Satisfaction | 0.33 | 5.635 | 0 | Yes | |
Service - > Customer Satisfaction | 0.201 | 3.03 | 0.003 | Yes | ||
Value - > Customer Satisfaction | 0.213 | 2.999 | 0.003 | Yes | ||
Atmosphere - > Customer Satisfaction | 0.706 | 14.401 | 0 | Yes | ||
Moderating Effect 1 - > Customer Satisfaction | 0.109 | 2.578 | 0.01 | Yes | ||
Moderating Effect 2 - > Customer Satisfaction | 0.123 | 2.7 | 0.007 | Yes | ||
Additional | COVID-19 Safety Precautions - > Customer Satisfaction | 0.208 | 3.482 | 0.001 | Yes | |
Group 3 (N = 373) Families | Food - > Customer Satisfaction | 0.351 | 6.03 | 0 | Yes | |
Service - > Customer Satisfaction | 0.286 | 4.35 | 0 | Yes | ||
Value - > Customer Satisfaction | 0.346 | 5.571 | 0 | Yes | ||
Atmosphere - > Customer Satisfaction | 0.755 | 17.616 | 0 | Yes | ||
Moderating Effect 1 - > Customer Satisfaction | 0.086 | 2.252 | 0.025 | Yes | ||
Moderating Effect 2 - > Customer Satisfaction | 0.07 | 1.608 | 0.108 | No | ||
Additional | COVID-19 Safety Precautions - > Customer Satisfaction | 0.232 | 3.948 | 0 | Yes | |
Group 4 (N = 337) Friends | Food - > Customer Satisfaction | 0.334 | 5.563 | 0 | Yes | |
Service - > Customer Satisfaction | 0.184 | 2.462 | 0.014 | Yes | ||
Value - > Customer Satisfaction | 0.193 | 2.621 | 0.009 | Yes | ||
Atmosphere - > Customer Satisfaction | 0.721 | 15.569 | 0 | Yes | ||
Moderating Effect 1 - > Customer Satisfaction | 0.099 | 2.341 | 0.02 | Yes | ||
Moderating Effect 2 - > Customer Satisfaction | 0.093 | 1.877 | 0.061 | No | ||
Additional | COVID-19 Safety Precautions - > Customer Satisfaction | 0.221 | 3.297 | 0.001 | Yes |
7. Discussion
Online consumer reviews are playing a significant role in the image of online businesses. Consumers discuss and evaluate products and services online and their reviews provide a critical source of information for potential customers as well as business owners [ 42 ]. Customers reach their purchase decision based on these reviews [ 43 ], and business owners consider the information extracted from the reviews to make managerial decisions [ 45 ].
The use of text-mining technology enables researchers and practitioners to discover new insights about the preferences of the customers [ 6 ]. Customers' satisfaction with restaurants has been investigated via text-mining based on the online reviews in previous literature [ 14 , 137 ]. However, the impact of the COVID-19 outbreak has changed the business landscape, and eventually, the customer's choices and preferences have to be reinvestigated, as businesses managers need to adapt their operations and management policies. Hence, this research aims to investigate the determinants of the customers' sentiments with a restaurant within the context of the current pandemic. As the outcomes of the research presented, customers are concerned about the COVID-19 safety precautions. The results indicated that while the investigated features are important contributors to customer satisfaction, the COVID-19 safety measures observed in the restaurants could impact the link between these factors and satisfaction.
Our findings showed that atmosphere is one of the essential factors to customer satisfaction during the outbreak. The results are consistent with proceeding studies (e.g. Ref. [ 63 ]). Moreover, our findings showed that the impact of the COVID-19 safety precautions on the relationship between atmosphere and satisfaction is significant. The effect, however, varied between the investigated subgroups. Results indicated that the effect was only present for couples. Similarly, the results showed that service is significant in determining customer satisfaction and the effect is moderated by the COVID-19 safety precautions. Although service quality is a multidimensional construct, it has been investigated both as a compound [ 72 ] and as a separate dimension [ 80 ]. Conversely, the effect was not confirmed for the solo customers of restaurants, while it was confirmed for couples, friends, and families. We also found that food and value play a significant role in the satisfaction of the customers during the outbreak, which is in line with the studies before the outbreak and provides additional support for previous studies [ 55 , 63 , 84 , 102 ]. Safety precautions were also indicated as an influential factor to customers’ satisfaction. This implies that to adapt to the changes imposed by the current pandemic, restaurants must consider aspects related to the COVID-19 safety precautions regarding their services.
8. Conclusion and implications
This paper examines customers' satisfaction with restaurants and the most important factors that impact customers’ satisfaction during the COVID-19 pandemic. We used a two-step method based on machine learning and survey-based approaches. In the first step, the information provided on restaurant websites was examined using a newly proposed method based on machine learning techniques (text mining, clustering, and prediction learning techniques). We used both the numerical ratings of quality aspects and textual reviews of service in restaurants. The big social data was extracted from TripAdvisor consisting of 2158 records from 50 restaurants. LDA was used to discover satisfaction dimensions and the numerical reviews were analyzed using LVQ cluster analysis. Finally, the CART technique was used to predict the level of satisfaction in the generated segments. The tree showed that the lowest satisfaction was reported in restaurants that did not follow the COVID-19 safety measures. Besides, customers who rated food higher were the largest satisfied group (24.6%).
In doing so, we provided insights into the use of machine learning techniques in the field, by exploring the use of LDA for text mining to discover the satisfaction dimensions. Text mining techniques have been used actively in the management and hospitality literature. However, the use of LDA remains rare. There is also a need to develop new methods to investigate the reviews according to the latest trends in the review patterns, which could help researchers to learn and predict the context of the reviews during the COVID-19 pandemic.
In the second step, a set of hypotheses were examined using PLS-SEM methodology, by analyzing 1358 survey responses gathered in six months. The results indicated that four items of atmosphere, food, value, and service infer customer satisfaction with restaurants significantly, among which the most prominent effect was imposed by the atmosphere. We were able to confirm that the COVID-19 safety precautions observed by the restaurants amplify the effect that atmosphere and service have on customer satisfaction.
Another significant finding is the different levels of importance of the COVID-19 safety precautions in the defined market segments. We developed our hypothesis based on the SOR model and examined the moderating effect of the COVID-19 safety precautions across four subgroups namely solo, family, couple, and friends who visit the restaurants and reviewed them. Customers who were couples, with friends, or family members while visiting restaurants showed more sensitivity toward the COVID-19 safety precautions, while solo travelers did not. The magnitude of the effect of the COVID-19 safety measures on customer satisfaction was the highest for families, followed by couples, friends, and solo travelers, respectively. Whereas, the moderating effect of the COVID-19 safety measures on the relationship between each of the atmosphere and service and satisfaction was not present for solo travelers. The effect on the satisfaction was more eminent in the other three groups regarding the service, rather than the atmosphere. Solo travelers who value individual habits and seek self-satisfaction are typically less demanding than customers in groups (couple, family, friends) [ 138 ], and hence they might be aware of the COVID-19 safety precautions but complain less about them concerning service and atmosphere.
This is in line with the theory expectation disconfirmation theory, which explains the differences in customers' satisfaction by their expectations. Customers from different groups naturally have different expectations of the services and therefore, the same stimulus could provoke different sentiments. According to the construal level theory [ 139 ], people interpret the same event differently. Customers’ preferences might vary according to their goals of visiting. For example, family and couple travelers tend to be more willing to pay in their travel experience, depicting a lower construal level, while solo travelers present a higher construal level which makes them more likely to be satisfied. [ 140 ], explaining their attitude toward the safety precautions. This effect has been observed in drivers of ratings and satisfaction in studies of other sectors [ 141 ].
The study contributes to the existing knowledge by proposing a new approach for predicting satisfaction based on online reviews, in the forms of text and ratings, and travelers' behavioral preferences during the pandemic. It adds to the previous studies that have used a broad range of methods to identify indicators of customers’ satisfaction [ 6 , 9 , 48 ]. The findings explored the role of factors with a more extensive description. Moreover, the presented insights about the market segmentation could address the research gap by identifying the shared views and key factors during the pandemic for four different groups of customers. In doing so, this study broadens the scope of the analysis of online reviews during the pandemic by improving our understanding of customer preferences in the post-COVID-19 period.
In terms of managerial implications, the findings can help restaurant managers in their decision-making process during and after the COVID-19 crisis. This research highlights the important aspects of business that are significant to the customers during the pandemic. The restaurants could provide differentiated service for each group of customers according to the variations in the impacts of the driving factors, of each group, on the level of satisfaction. The findings could help managers to optimally allocate resources to prioritize the needs and expectations of each group focusing on the provided services. Utilizing the outcomes of the study, the business owners in this sector might be able to mitigate easier the changes imposed by the COVID-19 crisis. The ability to differentiate service quality according to the preferences of each subgroup of restaurant customers enables managers to adjust their services promptly, leading to higher levels of satisfaction which is a key driver of revenue growth [ 2 , 3 ].
The pandemic has influenced the consumer's perceived level of importance of the features, hence, it is of essential value for restaurants to comprehend the changes and adapt accordingly, especially because it is more likely that the effects might persist a long time after the pandemic is over. That, of course, requires the presence of the restaurants in the platforms that enable customers to express their views and make their feedback heard. Restaurants and dining businesses have played an important role in the development of the tourism field, which accordingly has an important impact on the growth of the community [ 142 , 143 ]. Hence, it is vital to investigate customers' perceptions using new approaches that integrate up-to-date analysis methods. The novel method of this study that integrates two approaches to capture customers' assessment of restaurants can help to meet this goal.
Our work, however, had limitations that should be addressed. We did not investigate any causal relationship. Our findings were based on predictive outcomes. Further experiential studies are required to confirm the causal relationships. We relied only on reviews posted during the pandemic, which limits the number of reviews in this study. Future study could be conducted focusing on differences between the reviews and the ratings in three time periods; before the COVID-19 outbreak, the early months of the pandemic, and the subsequent pandemic period to examine how the state of the pandemic have changed customers' preferences and levels of satisfaction over the time. In addition, our findings should be carefully generalized to other business sectors in the hospitality industry. Moreover, we did not take into account the context or different cultural features in our study. This limits our findings from being generalized to other cultures, specifically in the countries where English is not used by native people. A multi-language analysis can be conducted to address this limitation. Future studies are encouraged to enrich the data with more predictors and more variables that could impact customers’ satisfaction.
Acknowledgments
The authors are thankful to the Deanship of Scientific Research at Najran University for funding this work under the Research Collaboration Funding program grant code (NU/RC/SERC/11/13).
Appendix A.
LVQ segments, COVID-19 safety precautions and level of satisfaction in four groups
Customer Satisfaction Level | Travel Type | ||||||||||||||
---|---|---|---|---|---|---|---|---|---|---|---|---|---|---|---|
Travelled as a couple | Travelled solo | Travelled with family | Travelled with friends | ||||||||||||
LVQ Segments | |||||||||||||||
Segment 1 | Segment 2 | Segment 3 | Segment 1 | Segment 2 | Segment 3 | Segment 1 | Segment 2 | Segment 3 | Segment 1 | Segment 2 | Segment 3 | ||||
COVID-19 Safety Precautions | No | Customer Satisfaction Level | High | 0 | 5 | 9 | 0 | 7 | 6 | 0 | 1 | 9 | 0 | 20 | 23 |
Low | 62 | 6 | 41 | 65 | 8 | 26 | 47 | 5 | 26 | 176 | 20 | 99 | |||
Moderate | 12 | 11 | 48 | 12 | 18 | 29 | 17 | 16 | 44 | 42 | 57 | 122 | |||
Yes | Customer Satisfaction Level | High | 0 | 0 | 0 | 140 | 117 | 305 | 40 | 34 | 71 | 30 | 16 | 38 | |
Low | 0 | 0 | 0 | 18 | 0 | 1 | 5 | 1 | 1 | 1 | 0 | 1 | |||
Moderate | 0 | 0 | 0 | 121 | 8 | 60 | 28 | 2 | 9 | 12 | 0 | 10 |
Appendix B.
Survey items
Construct | Indicators | Research Items | References |
---|---|---|---|
Atmosphere | AT1 | The appearance of the employees is neat. | [ ] |
AT2 | The interior design of the restaurant is attractive. | ||
AT3 | The temperature of the restaurant is acceptable. | ||
AT4 | The layout allows easy movement around. | ||
Food Quality | FO1 | The restaurant served good quality food. | [ ] |
FO2 | The restaurant served food that meets the hygiene measures. | ||
FO3 | The restaurant served food based on social distancing measures. | ||
FO4 | The restaurant served tasty food. | ||
FO5 | Dining at this restaurant looks safe to me. | ||
COVID-19 Safety Precautions | CSP1 | Only immunized people are allowed to enter the restaurant. | From the Online Reviews |
CSP2 | Workers wear masks all the time. | ||
CSP3 | Workers sanitize surfaces frequently. | ||
CSP4 | Hand sanitizers are available. | ||
Satisfaction | SAT1 | I am satisfied with this restaurant. | [ ] |
SAT2 | My satisfaction with this restaurant is high. | ||
SAT3 | I am glad that I selected this restaurant. | ||
Service | SE1 | The restaurant presents comfortable utilities. | [ ] |
SE2 | Service is provided at an acceptable time. | ||
SE3 | The services are presented quickly. | ||
SE4 | The provided services met my expectations | ||
SE5 | The presented services are of high quality. | ||
Value | VA1 | The price meets the provided services. | [ ] |
VA2 | The food deserves the price I paid. | ||
VA3 | The restaurant provides a worthy value of money. | ||
VA4 | Overall, the restaurant deserves the price I paid. | ||
VA5 | The served food worth's the presented prices |
Appendix C.
Cross-loadings Test
Atmosphere | COVID-19 Safety Precautions | Customer Satisfaction | Food Quality | Services | Value | |
---|---|---|---|---|---|---|
AT1 | 0.674 | 0.445 | 0.289 | 0.437 | 0.43 | 0.513 |
AT2 | 0.682 | 0.479 | 0.316 | 0.404 | 0.455 | 0.578 |
AT3 | 0.71 | 0.571 | 0.332 | 0.462 | 0.496 | 0.587 |
AT4 | 0.857 | 0.296 | 0.806 | 0.452 | 0.346 | 0.35 |
CSP1 | 0.3 | 0.761 | 0.267 | 0.474 | 0.632 | 0.381 |
CSP2 | 0.258 | 0.791 | 0.265 | 0.481 | 0.602 | 0.374 |
CSP3 | 0.322 | 0.724 | 0.293 | 0.546 | 0.488 | 0.44 |
CSP4 | 0.626 | 0.715 | 0.396 | 0.512 | 0.585 | 0.618 |
FO1 | 0.471 | 0.568 | 0.383 | 0.727 | 0.699 | 0.65 |
FO2 | 0.389 | 0.585 | 0.351 | 0.723 | 0.493 | 0.393 |
FO3 | 0.428 | 0.476 | 0.458 | 0.797 | 0.463 | 0.431 |
FO4 | 0.281 | 0.467 | 0.265 | 0.606 | 0.372 | 0.341 |
FO5 | 0.504 | 0.426 | 0.621 | 0.781 | 0.482 | 0.447 |
SAT1 | 0.806 | 0.296 | 0.857 | 0.452 | 0.346 | 0.35 |
SAT2 | 0.555 | 0.376 | 0.854 | 0.527 | 0.444 | 0.376 |
SAT3 | 0.483 | 0.4 | 0.758 | 0.578 | 0.436 | 0.364 |
SE1 | 0.44 | 0.5 | 0.346 | 0.546 | 0.784 | 0.694 |
SE2 | 0.485 | 0.556 | 0.355 | 0.55 | 0.815 | 0.741 |
SE3 | 0.347 | 0.672 | 0.374 | 0.51 | 0.728 | 0.381 |
SE4 | 0.309 | 0.664 | 0.373 | 0.486 | 0.688 | 0.367 |
SE5 | 0.489 | 0.516 | 0.39 | 0.541 | 0.805 | 0.735 |
VA1 | 0.545 | 0.54 | 0.447 | 0.617 | 0.739 | 0.863 |
VA2 | 0.456 | 0.524 | 0.261 | 0.469 | 0.509 | 0.764 |
VA3 | 0.443 | 0.473 | 0.307 | 0.49 | 0.513 | 0.783 |
VA4 | 0.5 | 0.503 | 0.259 | 0.436 | 0.49 | 0.749 |
VA5 | 0.534 | 0.522 | 0.416 | 0.554 | 0.742 | 0.858 |
- Restaurant Customer Data Platform
- Restaurant Reputation Management
- Marketing Automation
- Schedule Free Demo
- What is a Restaurant Customer Data Platform?
What is Restaurant Reputation Management?
- What is Restaurant Marketing Automation?
Restaurant Marketing
- Restaurant Operations
- Restaurant Reputation Mgmt
- Restaurant Analytics
- Coffee Shop Marketing Ideas and Trends
- Retail Marketing
- Retail WiFi Marketing
- Restaurant Marketing Blog
- Help Center
- Restaurant Marketing Videos
- Restaurant Marketing White Papers and Case Studies
- Press/Articles
- Enterprise Integrations
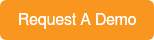
Game-Changing Restaurant Customer Satisfaction Tips and Trends
Restaurant customer satisfaction.
In a world where customers are always looking for the best deal, it is more important than ever for restaurants to focus on customer satisfaction.
Satisfied customers are more likely to return and recommend your restaurant to others, while unhappy customers can damage your reputation with just one review.
But what exactly is restaurant customer satisfaction? And how can you make sure your customers are happy?
Here’s a look at what you need to know about customer satisfaction and how to achieve it.
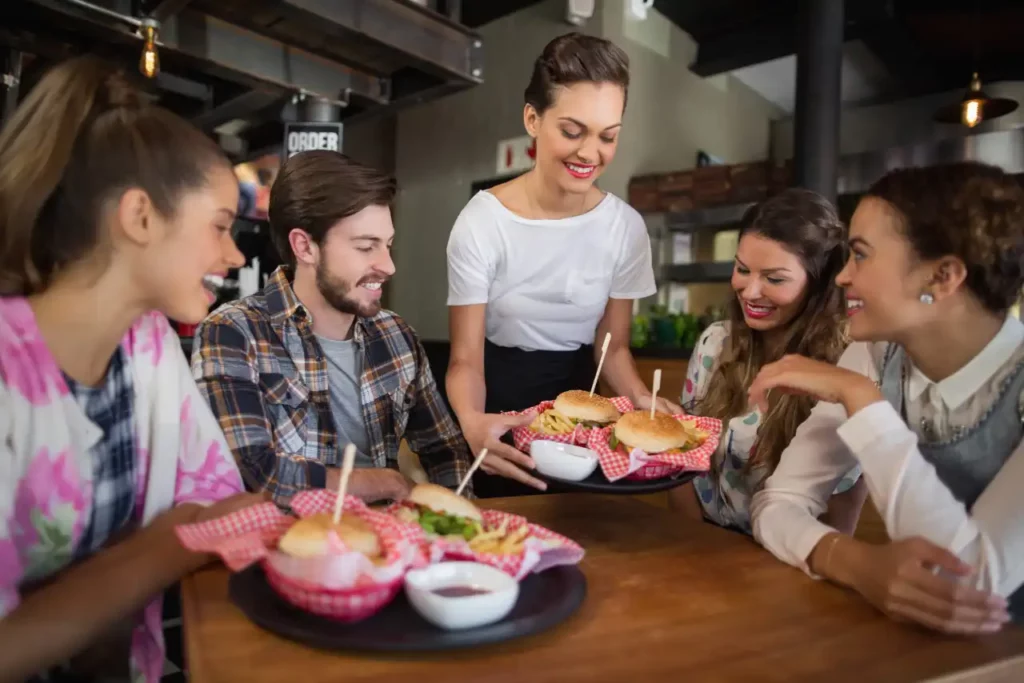
What is Customer Satisfaction?
Customer satisfaction is the degree to which a guest is happy with a product or service. In the restaurant industry, customer satisfaction can be affected by everything from the quality of the food to the speed of service.
Creating a culture of guest satisfaction starts with your employees. Train your staff to provide exceptional customer service and make sure they understand the importance of customer satisfaction.
In addition, regularly collect feedback from guests to get a sense of what they’re thinking and how they feel about your restaurant.
Measuring Restaurant Customer Satisfaction
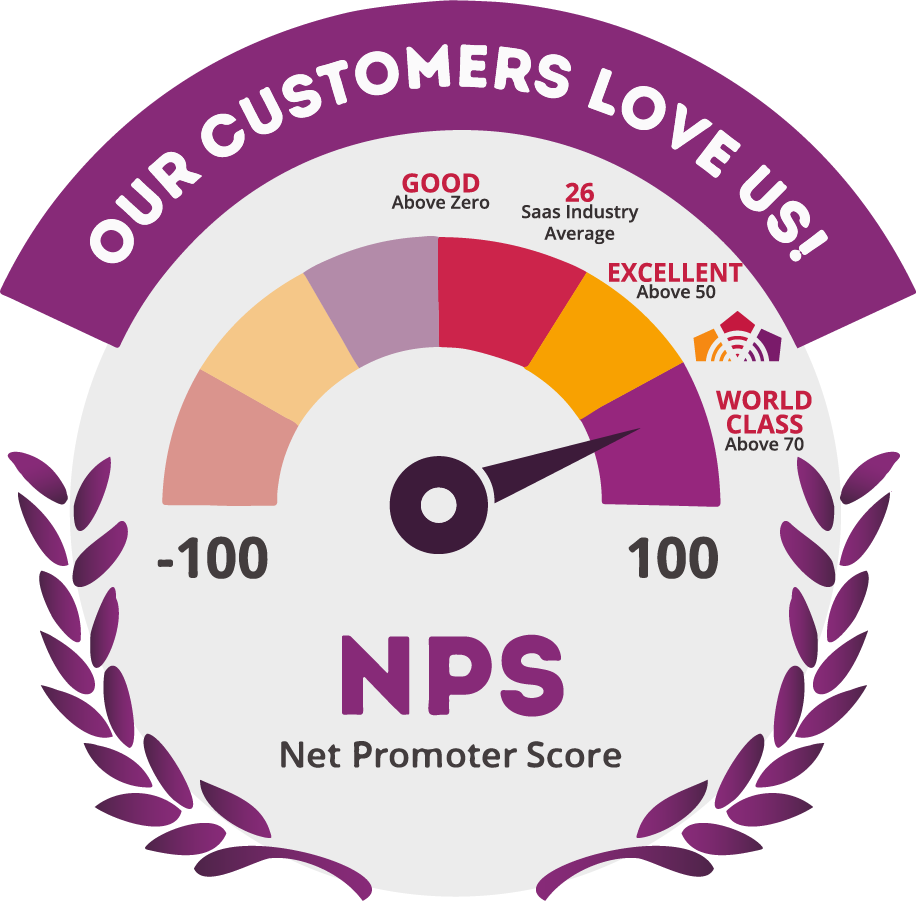
Based on their answer, guests are placed into one of three categories:
- Detractors (0-6): These are the guests who are unhappy with your restaurant and are unlikely to recommend it to others.
- Passives (7-8): These guests are satisfied with your restaurant but may be swayed by a competitor.
- Promoters (9-10): These guests are your biggest fans and are likely to recommend your restaurant to others.
To calculate your NPS, you take the percentage of promoters and subtract the percentage of detractors. For example, if 80% of guests are promoters and 20% are detractors, your NPS would be 60.
While the NPS is a good way to get a general sense of customer satisfaction, it’s also important to collect more detailed feedback from guests. This can be done through surveys, focus groups, or even simply talking to them in person.
Why is Customer Satisfaction So Important for Restaurants?
The importance of customer satisfaction cannot be overstated. Happy guests are more likely to return and spend more money at your restaurant, while unhappy guests can quickly damage your reputation.
In addition, satisfied guests are more likely to recommend your restaurant to others. In fact, word-of-mouth is one of the most effective forms of marketing. According to Nielsen, 92% of consumers believe suggestions from friends and family over all other forms of advertising.
Finally, happy guests can also help you attract new customers. In today’s world, online reviews are more important than ever. Sites like Yelp and Google can make or break a restaurant, so it’s crucial to make sure your guests are satisfied.
Remember that the importance of customer satisfaction does not discriminate. It can make or break the success of any restaurant, regardless of its size, location, demographics, or even the quality of the cuisine.
However, restaurant owners can find it increasingly difficult to identify the major contributing factors to customer satisfaction, especially when they disagree with what guests feel that they need.
It’s not about what restaurants need to feel satisfied, it’s about what guests feel restaurants need to do to satisfy them. It’s important not to inconvenience guests at the restaurant’s convenience.
The more satisfied guests are, the more likely they are to spend more, visit repetitively, share their positive experiences, and recommend restaurants to friends, families, and strangers online.
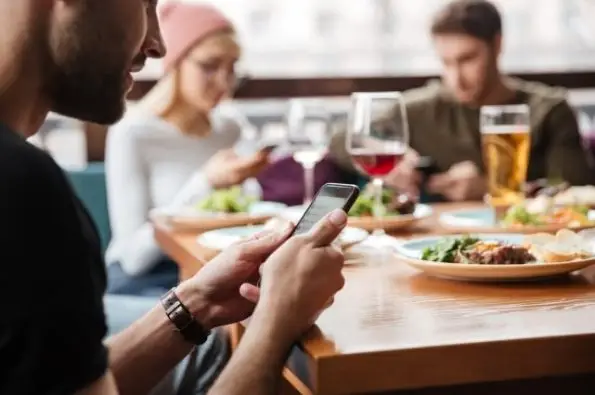
Telling Statistics of Guest Satisfaction
There are a variety of reasons that restaurant guest satisfaction is important, but let’s sum it up to these powerful statistics:
- 7 out of 10 U.S. consumers say they’ve spent more money to do business with a company that delivers great service. (American Express)
- A moderate increase in customer experience generates an average revenue increase of $823 million over three years for a company with $1 billion in annual revenues. (Temkin Group)
- A Harvard Business School researcher found that a one-star increase in a restaurant’s Yelp rating correlated with a 5-9% increase in revenue.
- 25 percent more people turn to consumer reviews on sites like OpenTable, Yelp, and TripAdvisor than those who rely on reviews by professional food critics. 60 percent read reviews before going out for a meal, a habit that takes precedence over getting directions to a restaurant or looking at food photos. (OpenTable)
- Diners say that complimentary extras (69 percent) and seating preferences (65 percent) would go far in increasing customer loyalty. (OpenTable)
- 75 percent of consumers will not visit or patronize a restaurant with negative reviews about its cleanliness. (Harris Poll for Cintas Corporation)
- 38% of all customer complaints are on social media and review sites. Restaurants get only 14% of all complaints. (Jay Baer, Food Service Magazine)
- After one negative experience, 51% of customers will never do business with that company again. (New Voice Media)
These statistics underline the importance of satisfying every guest who comes to your place of business or orders online. Here are some powerful ways to keep them satisfied and coming back for more.
Ways to Improve Restaurant Customer Satisfaction
When it comes to the restaurant industry, keeping your guests happy is key to success. In fact, a recent study found that a 1-point increase in guest satisfaction scores can translate into a 3-5% increase in profits.
So what can you do to ensure your guests are satisfied? Here are a few tips:
1) Make sure your restaurant menu is easy to navigate and offers something for everyone.
If you want to keep your guests happy, it’s important to make sure your restaurant menu is easy to navigate and offers something for everyone.
This means ensuring that there are plenty of options for different dietary needs, as well as varying tastes and budgets. It also means keeping the menu layout simple and organized, so guests can quickly find what they’re looking for.
One way to make sure your menu is customer-friendly is to offer a variety of choices. This means having options for different dietary needs, as well as different taste preferences.
For example, if you’re a vegan restaurant, it’s important to make sure you have plenty of vegan-friendly options. If you’re a pizzeria, you should offer a variety of pizza toppings so that everyone can find something they like.
It’s also important to keep your menu layout simple and organized. Focus on making it easy for guests to find what they’re looking for.
By offering a customer-friendly menu, you can help ensure that your guests have a positive dining experience and keep coming back for more. Oracle’s GloriaFood offers an online restaurant menu that is free of charge.
2) Keep your dining area clean and clutter-free.
No one wants to eat in a dirty restaurant. Not only is it unappetizing, but it can also be dangerous.
A cluttered dining area can also be a hazard, as people can easily trip over wires or cables.
Make sure your dining area is clean and clutter-free so that your guests will feel comfortable and safe while they’re eating.
3) Train your staff to be friendly and attentive.
No matter how good your food is, if your staff members are unfriendly and unhelpful, you’re going to lose customers.
It’s important to train your staff on the basics of customer service so that they can provide a positive experience for every patron who walks through your door.
This includes being friendly and attentive, as well as helping guests navigate your menu and answering any questions they may have.
4) Offer prompt and consistent service.
To provide prompt and consistent service, it is important that your waitstaff be well-trained and knowledgeable about the menu.
They should also be familiar with the restaurant’s policies and procedures so that they can effectively deal with any situation that may arise.
In addition, it is essential to have a system in place for taking orders, delivering food, and collecting payments so that everything runs smoothly.
By following these tips, you can ensure that your customers are happy with the service they receive.
5) Use quality ingredients in your recipes.
Cooking with quality ingredients is important for a few reasons. First, it ensures that your food will taste great.
Second, it shows your guests that you care about their experience and are willing to put in the extra effort to make sure they have a good meal.
Finally, it sends the message that you take your cooking seriously and are committed to providing a high-quality dining experience for your guests.
6) Price your items fairly.
When pricing your menu items, you want to make sure that you are fair to both your customers and your business. You don’t want to charge too much, lest you scare customers away, but you also need to make a profit.
7) Reward loyal guests with discounts or special offers.
Loyalty programs are a great way to show your customers that you appreciate their business.
By offering special rewards and discounts, you can encourage customers to keep coming back and make them feel appreciated.
This can lead to increased customer loyalty and better word-of-mouth advertising for your business.
Other areas to improve for better guest satisfaction.
- Updated interior design : A restaurant’s physical environment will immediately evoke positive or negative feelings about a restaurant’s brand.
- Ambient light : While rarely mentioned, ambient lighting that is too bright or too dark has a high likelihood of becoming a deterrent and a silent brand detractor
- Colors : The colors of a restaurant will immediately and subconsciously showcase the quality of a brand. They also evoke specific feelings. For example, red and yellow stimulate appetites, and blue decreases appetites but increases feelings of calmness and comfort. Blue creates the appearance that time passes quickly. Red restaurants create the appearance that time passes by slower for guests.
- Music : Multiple studies show that music has a direct impact on the amount the guests spend when visiting restaurants. Music should match a brand’s image. Slow music increases a guest’s willingness to spend more money, while faster music can contribute to more alcoholic beverage sales and create a more casual environment.
- Background noises : Restaurants should have a medium sound level. Guests in quiet restaurants spend more money and eat more of their food. But they can also contribute the low sound levels to a low-quality restaurant with high-cost food. Guests in noisy restaurants both spend and eat less. So, if traffic is high it’s important to have interior soundproofing present.
- Aesthetics : Not only do guests prefer a positive overall brand interior appearance, but attractive restaurants are also an additional social media marketing tool.
By following these tips, you can create a customer-centric restaurant that is sure to please.
And remember, satisfied guests equals increased profits. So it’s definitely worth your while to invest in customer satisfaction!
What Contributes to Poor Customer Satisfaction?
We recently put together a great article on the contributing factors of poor customer service and satisfaction. You can find it here .
To summarize, they include:
- Neglecting the fundamentals of running a business
- Misunderstanding what your guests want and need
- Lack of cleanliness
- Non-versatile menu options
- Underestimating the power of unhappy guests
- Not creating enough reasons for guests to come back (loyalty programs)
- Poor food quality
- Lack of brand consistency
- No Incentives/Poor Incentives
- Poor customer service
- Bad environment
- If a location is outdated, amplifies sound too much, has music that is too loud, or has no music at all, consumers classify it as a bad environment.
- These are the top factors to improve a restaurant’s environment:
- Unreasonable pricing
- Lack of future planning
- No competitive differentiation
- Poor location
Analyzing Guest Behavior for Satisfaction
Knowing how your guests are behaving is key to engaging them and keeping them satisfied. But how do you collect this data?
The secret to capturing this data is through a captive portal that works through your existing WiFi service. If you’ve already provided free WiFi to your guests, you can turn this into a symbiotic process.
A captive portal is simply the page that a guest is directed to when logging into your WiFi. There are many things you can do when developing a captive portal. You’ll find several examples here. You can build customer profiles, onboard guest data onto third-party applications, and automate your marketing campaigns quickly and easily.
Once you begin collecting guest data, you might find some surprises about how your loyal patrons typically behave. Then you can adjust marketing and operations procedures to optimize your business over time.
You will see things like guest dwell times, popular days and times, age and gender distribution, guest repeat rates, postal codes, birthdates, and more.
Then, you create specific guest lists to send targeted marketing messages based on behavior and demographic data. This is known as customer segmentation and can provide a much higher ROI on your marketing efforts.
Customer Data Platform Insights Can Help Improve Satisfaction
Another great way to improve restaurant customer satisfaction is to keep your guests engaged with your marketing. Customer segmentation and personalization of your marketing messages is crucial in today’s competitive marketplace.
Segmentation refers to using guest data to create much more effective email content because it can be tailored for each individual or each customer persona.
Using personalized messaging has been proven to improve open rates and generate more revenue because it allows restaurant marketing professionals to create more relevant content that will more effectively pique the interest of the consumer. This leads to better overall trust and satisfaction.
By collecting guest data and grouping those guests into separate lists, marketers can focus on sending highly targeted messages to each customer segment, increasing open rates, and encouraging more engagement.
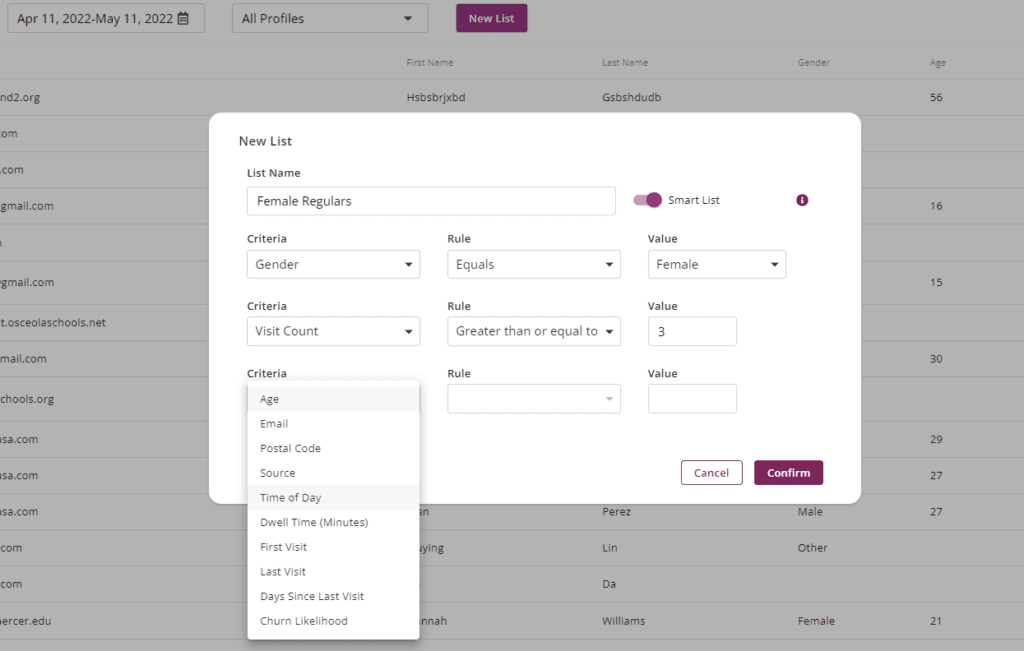
How to Collect Individual Customer Data
To execute an effective and engaging marketing campaign, you’ll need to have comprehensive data about your individual guests.
There are various ways in which to collect guest data, but most are either expensive or take a great deal of time and energy. The only efficient way to gather enough comprehensive, verified data to effectively create a personalized campaign is to use a quality customer data platform .
It will allow you to passively gather the necessary individual guest information through your website, your on-site WiFi, your online ordering system, and other means. Then you can use the data to create targeted, segmented marketing messages.
The platform also tracks the physical behavior of your guests, logging things such as dwell times, repeat visits, coupon redemption, days of the week, and hours of the day they have visited.
With this kind of thorough and reliable data, marketers can begin using it to build powerful, data-driven targeted restaurant marketing campaigns to engage and further solidify guest satisfaction.
Using Data to Build Engaging Marketing Messages
According to research from Salesforce, the world’s #1 customer relationship management platform, data targeting and segmenting were used 51% more by over-performing businesses than those who were underperforming.
This makes perfect sense when thinking about today’s consumers. They expect personalized messaging and they quickly sniff out when they’re receiving a simple generic mass-marketing message.
The simplest way to get started creating segmented and personalized campaigns is to make your customer profile data filterable.
This will allow you to start building segments of specific guests based on their demographics and behavior data.
Using these tools, marketers will have the guest information necessary to create targeted and personalized marketing campaigns. This will give you the upper hand over your competition when rebuilding your revenue stream before, during, and after challenging times.
Likewise, you will be able to see the results of online and offline campaigns to measure ROI and optimize over time.
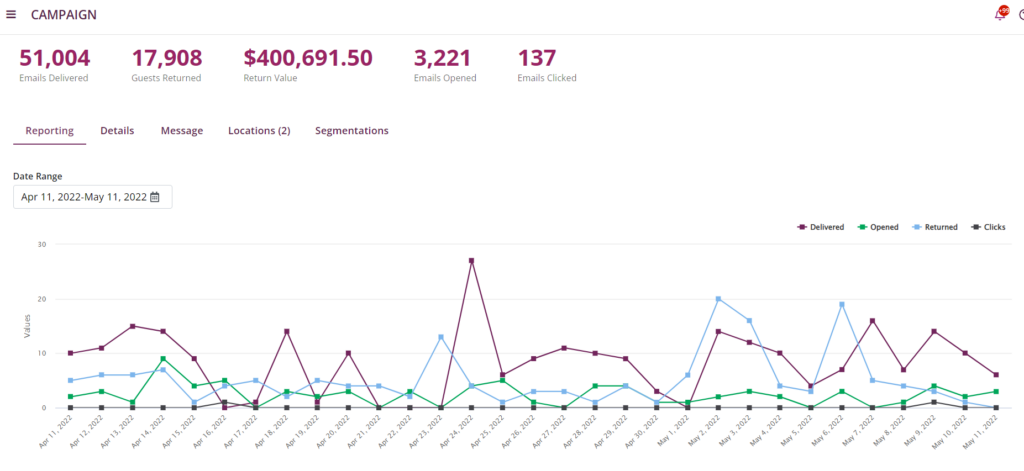
Marketing Attribution and Examples
Accurate marketing attribution for restaurants, or any brick-and-mortar business, has always been a thorn in the side of marketing professionals. However, in today’s competitive environment, it is absolutely necessary.
In fact, adding attribution to your restaurant marketing strategy can improve your results tremendously. According to Gartner, the typical outcome of implementing attribution is a 20-30% gain in media efficiency and corresponding increases in ROI.
What is Marketing Attribution?
Marketing attribution is the marketing term used for applying credit to individual marketing tactics and messaging and attributing ROI to them. When done correctly, it allows marketers to apply more focus on tactics and messaging that are working well, and less focus on those that aren’t.
The main ingredient for effective, proper marketing attribution is accurate, comprehensive data.
Traditionally, this type of data was difficult and expensive to acquire. And it was simply a snapshot in time. In other words, if you wanted updated data, you would have to pay for it over and over.
Fortunately, today’s technology has eliminated this difficult challenge.
Using a restaurant customer data platform like Bloom Intelligence, marketers will have the ability to see the most accurate and reliable guest data available today to measure customer sentiment and satisfaction.
As soon as you install the platform, you will begin seeing data updated in real time. You’ll see things like daily/hourly visitors, repeat visitors, dwell times, popular visit times and so much more.
If a guest logs in to your WiFi, they are taken to a WiFi landing page, or captive portal . This page requires the user to enter information such as their name and email address before accessing the internet. At this point, the platform will create a customer profile for the patron and all of their previous and future data will be added to the profile.
How Does WiFi Marketing Attribution Work?
Now that you have the guest’s contact information, you can begin marketing to them. And you don’t have to send mass-marketing messages to your entire database. As discussed above, you can segment your database based on customer demographics and their online and offline behavior.
For instance, you can create a marketing campaign for women under 40 who visit your store on Sundays. Or, you can send a targeted message to men between 21 and 30 years old who have ordered online more than 3 times.
And since the platform will recognize when a guest comes back or orders online, marketing attribution on these campaigns becomes extremely easy.
With Bloom, you’ll be able to see who received a marketing message, who opened it, who came back to your location or ordered online, and whether or not they redeemed any offer that you attached to the message.
This is data that restaurant owners and operators haven’t had access to before. And its cost is pennies on the dollar compared to traditional market research solutions.
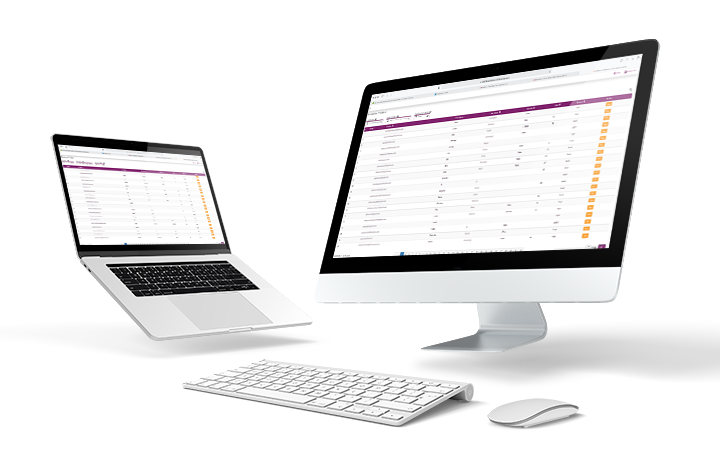
Let’s take a look at a couple of examples of WiFi marketing attribution.
Attribution Example 1
In this example, we will assume that you are finding your guest dwell times and online ordering are decreasing and you want them to increase. You create an email that you will send to your entire database. The email will contain an offer for a free dessert or 50% off an appetizer.
You send out the email and wait for the results.
A few days later, patrons begin redeeming the offer in your store or online. With Bloom Intelligence, you are not simply counting how many guests redeemed the offer. You will be able to see an accurate picture of how many people returned, even if they did not redeem the offer. Plus, you’ll be able to see accurate dwell time and online ordering measurements.
If the KPIs increase, then you can attribute this to your marketing campaign. You can then make an intelligent, data-driven decision around the ROI of the campaign and decide if you would like to continue, make changes to, or eliminate the campaign.
Attribution Example 2
With Bloom Intelligence, you have the ability to ask your guests their birthday. So you and your marketing team decide that you’d like to help increase guest loyalty by executing a “free meal on your birthday” campaign.
You create an email that you will send to anyone in your customer database who has entered their birthday. The email will be sent a few days prior so they can make plans to come to your place of business or order online. The email will also contain a redemption code.
From that moment on, you will be able to see how many people received the offer, how many viewed the offer, how many returned on their birthday or ordered online, and how many redeemed the offer. Over time you can attribute ROI to the campaign and decide whether to keep it running, make changes to it, or eliminate it altogether.
Ongoing Testing and Optimization
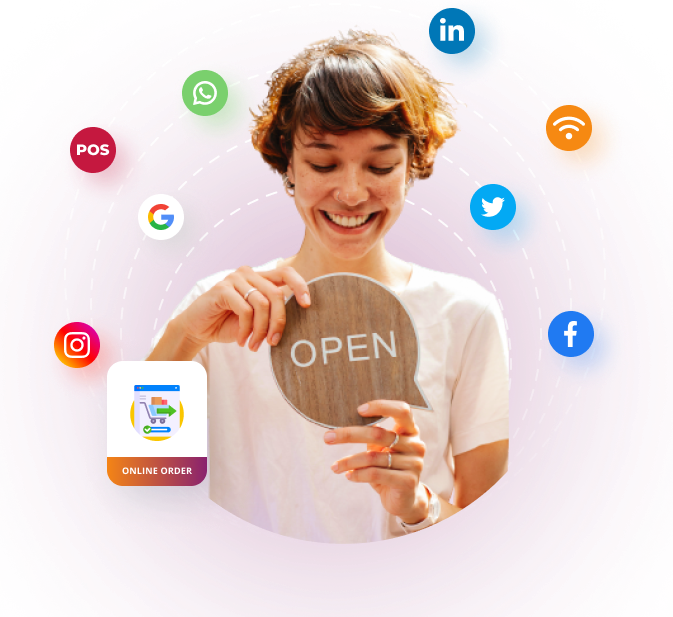
When you create and send your marketing message to each list, it is vital that you monitor the ROI of each campaign and measure sentiment to confirm more restaurant customer satisfaction.
As initial results come in, analyze the results and keep them documented in your platform.
The next time you send a message to each group, you’ll want to make a small change to the message.
You should only make a single change to the message while testing. Send the message and compare its results to the original message.
If the results are not as good as the original, go back to your original message and test a different aspect of the message.
If the results are better, keep the change and continue testing and optimizing over time. This is known as A/B testing.
Side note: don’t forget that you’re not just targeting current guests. You should also be seeking out new lookalike prospects online and targeting them in an effective manner using the data you’ve collected. These prospects should have a higher probability of becoming new guests, providing a more cost-effective means of acquiring new customers.
Of course, none of this will be as effective if you have no way to accurately measure the results of your efforts.
You need to make sure that the solution you’re using provides detailed and comprehensive reporting features, and the ability to track testing campaigns, as well as overall and individual guest satisfaction.
It can be tempting to use your own intuition to predict what will improve your restaurant’s customer satisfaction, but it’s vital to understand that guest perceptions, experiences, and likes/dislikes are limitless.
Basing marketing decisions on one person’s “feelings” can be detrimental to your business.
All of this will allow you to optimize your marketing messages based on accurate, tangible data, and strive for continuous improvement of guest engagement and satisfaction. If you’re not measuring your marketing campaigns and ROI at this level of detail, you will not know for sure if what you are doing is helping or hurting your business.
You want to continuously test your marketing messages. Without testing, you’re always leaving potential revenue on the table. Just make sure that the tools you are using can give you the accurate reports and KPIs required to determine which messages are engaging your guests better than others.
And always allow plenty of time to give yourself a large enough sample size to confidently determine your winners – the more, the better.
Bloom Intelligence Restaurant Guest Data Platform
Bloom Intelligence gives you the ability to track the results of every campaign you create – all the way down to a guest returning to your place of business and/or redeeming an offer. Bloom’s WiFi analytics detect the device of any consumer who walks back into your location that received one of your offers, messages, or surveys.
To learn more about the ways the Bloom Intelligence growth tools can help you improve your restaurant marketing to drive more sales, improve overall customer experience, measure customer sentiment, and grow your business, schedule a free demo today.
To see more of what Bloom can do for your restaurant or coffee shop marketing, schedule a free demo today , or call us at 727-877-8181 .
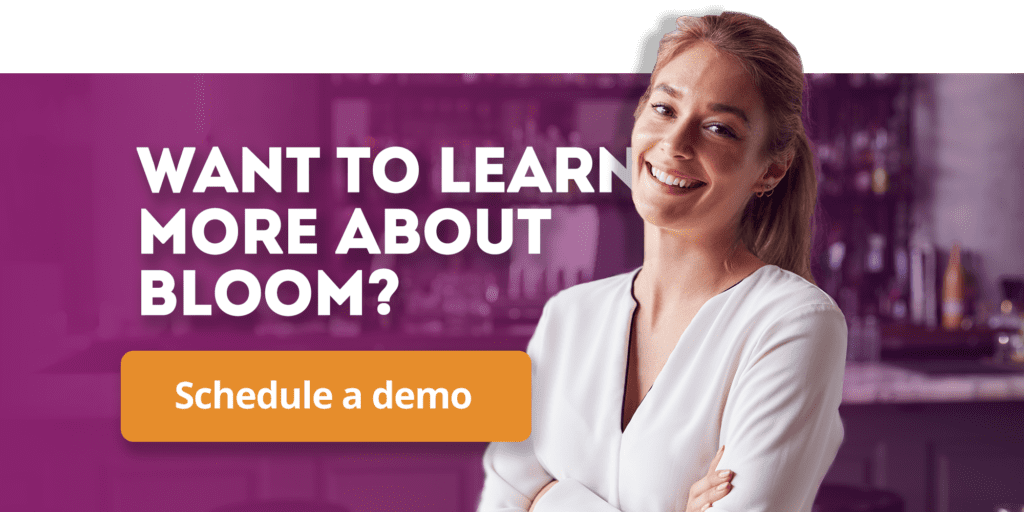
Related Posts
How to respond efficiently to online reviews to boost your bottom line.
In today’s competitive food service industry, monitoring restaurant ratings and reviews has become an essential strategy for those seeking to thrive and grow. With the proliferation of online platforms such…
Set It and Forget It: Marketing Automation Tricks to Fill Empty Tables
When it comes to running an efficient restaurant business, every minute counts. Restaurant operators and marketers juggle multiple responsibilities, from staff management and purchasing to finances and guest satisfaction. Amid…
The Power of Using a Restaurant Marketing Platform: Boosting Efficiency and ROI
Now more than ever, restaurateurs need every advantage to attract and retain their guests. Restaurant marketing software offers a powerful solution, streamlining operations and maximizing return on investment (ROI) while…
Restaurant Marketing FAQs
What is Restaurant Marketing?
Restaurant marketing is the process of getting people to visit your restaurants. Restaurant marketing creates loyalty, provides data to research, analytics, and allows restaurants to gain a better understanding of their ideal customer profile. It utilizes all customer channels: guest WiFi, website, social, rating sites, mobile apps, email, text, and advertising.
What is WiFi Marketing?
WiFi marketing is a marketing technique that uses guest WiFi to collect & clean customer data such as names, emails, phone numbers, customer behavior, and demographics. This data is used to personalize marketing campaigns to increase customer loyalty, build online reviews, and save at-risk customers. The performance of every campaign can be tracked down to the tangible ROI of a customer walking back in your door.
Restaurant reputation management is the process for restaurants to manage customer feedback and creating systems to improve customer experiences, passively build positive online reviews, and save at-risk customers. It is a very important aspect of running a successful restaurant business.
How Does Bloom Identify and Bring Back Lost Customers?
Bloom Intelligence uses machine learning to identify at-risk customers. When one is recognized, the system will send them a message with an incentive to get them to return and re-establish their visit pattern. Bloom users are seeing up to 37% of churning customers return.
SAVE TIME, INCREASE CUSTOMER LIFETIME VALUES, CREATE NEW CUSTOMERS
What our happy customers are saying
“SaaS that covered so many bases for us instead of having to use multiple software products. Bloom Intelligence has simplified our responses to reviews, customer feedback, and more. I highly recommend Bloom Intelligence.”
Robert Sanderson
“Bloom Intelligence really is a step ahead in terms of marketing software and metrics. Their product is reliable, fast and innovative and has helped the company I work for really grow.”
John Marchetti
“Working with Bloom Intelligence has been amazing. They assist you every step of the way and work with you hand in hand to make sure you are optimizing your advertising potential. We are excited to use this tool to help learn more about our customers so that we can personally engage with them and understand our strengths/weaknesses.”
Ariel Ramirez
“In these challenging times, it has been a pleasure working with Bloom Intelligence to help facilitate our service offering to our clients. They were extremely responsive and provided support to mitigate risk and minimize revenue loss. Great partner!”
“We’re extremely pleased with the wealth of customer data that we’re able to gather, at a very attractive price. In addition, we’re able to communicate our new product promotions by using the landing page as a digital billboard. A “no-brainer” for anyone working with limited Marketing $$.”
Bob Cross, Vice President of Operations
Subscribe Here for the Latest Updates
Never miss a post! We'll keep you up-to-date on the latest restaurant and retail WiFi marketing information.
Drop us a line!
We would love to hear from you! If you have any questions, comments or ideas about our restaurant marketing blog, drop us a line and let us know.
Or call us at 727-877-8181 .
The Influence of Service Quality and Price on Customer Satisfaction at Kopi Nako Bandung
- August 2024
- Sinergi International Journal of Management and Business 2(3):167-176
- 2(3):167-176

- This person is not on ResearchGate, or hasn't claimed this research yet.

Abstract and Figures

Discover the world's research
- 25+ million members
- 160+ million publication pages
- 2.3+ billion citations

- Anindya Gaine

- Megan Otsuka

- Yulia Larasati Putri
- Hardi Utomo
- J EUR SOC POLICY

- Assma Hajji
- Judith Kieninger

- Lisa Leinert
- GOV INFORM Q

- HEALTH AFFAIR
- Aditi P. Sen
- Jessica Y Chang
- John Hargraves
- RESOUR POLICY

- COMPUT ELECTR ENG
- Zhiting Song
- Yanming Sun
- Peipei Liang
- Recruit researchers
- Join for free
- Login Email Tip: Most researchers use their institutional email address as their ResearchGate login Password Forgot password? Keep me logged in Log in or Continue with Google Welcome back! Please log in. Email · Hint Tip: Most researchers use their institutional email address as their ResearchGate login Password Forgot password? Keep me logged in Log in or Continue with Google No account? Sign up

IMAGES
VIDEO
COMMENTS
Customer satisfaction is essential to any business's success. As guests become even more sensitive to price increases, it becomes mission-critical, particularly in the restaurant industry. Today's restaurant guests spend up to 10% more than they did two years ago. They're responding to this increase by going out less or expecting more.
This research was undertaken to evaluate the levels of service quality and clients' satisfaction on the services offered by two leading fast-casual dining restaurants in the Province of Nueva ...
Purpose To determine the factors that explain customer satisfaction in the full service restaurant industry. Design/methodology/approach Secondary research and qualitative interviews were used to ...
Ratings of hotels, restaurants and the like could shed light directly on the levels of customer satisfaction and dissatisfaction, as well as their causes [51], providing restaurant quality-improvement hints [52]. There are earlier works that determine customer satisfaction that rely on ratings (e.g. Refs. [53, 54]). However, the majority of ...
Factors Affecting Customer Satisfaction in Fast Food ...
Revisit intention is the willingness of a consumer to revisit a place due to satisfactory experience. Customer satisfaction generates a probability to revisit in presence or absence of an affirmative attitude toward the restaurant [8]. Revisit intention is a substantial topic in hospitality research [8, 9, 10].
customer satisfaction in the restaurant business in general. 1.2 Structure of thesis The study consists of five chapters. The literature review, presented in chapter 2, includes scientific data on customer satisfaction, its' importance and measurement, and customer satisfaction in the restaurant business.
This research is prepared based on researches relating to customer experience at restaurants. The research applied three databases: Science Direct, Emerald, and EBSCO. The literature reviewed is customer experience at restaurants in the last 10 years, specifically 2012 to 2022. 4. Data Collection.
Food quality seems to be accepted as a fundamental component to satisfy restaurant customers; however, it has been often overlooked in restaurant service quality and satisfaction studies. The primary objective of this study was to investigate how food quality is perceived in relation to satisfaction and behavioral intentions in mid- to upscale ...
Return patronage intention is defined as "the likelihood that a current customer of a restaurant expects to return in the future for a dining experience" (Young, Clark, & McIntyre, Citation 2007, p.92). As one of the most crucial antecedents of return patronage intentions, customer satisfaction has received a great deal of attention.
Abstract. This research examined how multi-sensory dimensions like sight, sound, and smell would influence customer attitudes toward quick-service restaurants, restaurant patronage intentions, food purchasing decisions, food consumption satisfaction, restaurant attachment, repurchase intention, and positive word of mouth in South African quick-service restaurants.
1. The conceptual framework for the meta-analysis is grounded in the available empirical evidence and the work of Oliver (1997). Oliver rightfully takes the opportunity in his book to embellish and advance thought on customer satisfaction so as to "infuse `new wine into old bottles' so that new work... will be forthcoming" (p. 261).
ABSTRACT. This study aimed to explore the impact of restaurant quality on customer satisfaction. Restaurant quality was measured using 11 dimensions related to. halal, food, hygiene, menu and ...
Full article: Customer satisfaction, loyalty, knowledge and ...
Number of pages and appendix pages 37+ 4 pages. The thesis is based upon the way of inspecting about how the customer satisfaction is im-proved in restaurant service. To collect the information's about it the research was focused in a restaurant which is situated in Helsinki.
The growing number of academic studies on customer satisfaction and the mixed findings they report complicate efforts among managers and academics to identify the antecedents to, and outcomes of, businesses having more-versus less-satisfied customers. These mixed findings and the growing emphasis by managers on having satisfied customers point to the value of empirically synthesizing the ...
The National Cross-Industry Measure of Customer Satisfaction ACSI LLC | 3916 Ranchero Drive | Ann Arbor, MI 48108 Phone: (734) 913-0788 | E-mail: [email protected]
This research will add in existing base of knowledge on vast topic of customer satisfaction while focusing on local restaurant industry. Keywords: Customer satisfaction, service quality, food reliability Introduction1 The restaurant industry has become one of the most profitable industries in the world.
A moderate increase in customer experience generates an average revenue increase of $823 million over three years for a company with $1 billion in annual revenues. (Temkin Group) A Harvard Business School researcher found that a one-star increase in a restaurant's Yelp rating correlated with a 5-9% increase in revenue.
Ratings of hotels, restaurants and the like could shed light directly on the levels of customer satisfaction and dissatisfaction, as well as their causes , providing restaurant quality-improvement hints . There are earlier works that determine customer satisfaction that rely on ratings (e.g. Refs. [53,54]). However, the majority of research has ...
Customer satisfaction is a critical concept for operations to survive. While traditionally considered a cognitive concept, recent research has highlighted the link between satisfaction, emotional factors, and cognitive aspects (Bigné, Andreu, and Gnoth 2005 ; Tang et al. 2018 ).
Promoters (9-10): These guests are your biggest fans and are likely to recommend your restaurant to others. To calculate your NPS, you take the percentage of promoters and subtract the percentage of detractors. For example, if 80% of guests are promoters and 20% are detractors, your NPS would be 60. While the NPS is a good way to get a general ...
The primary objectives of the paper include exploration of determinants of poor customer satisfaction, reasons why customers dine at restaurants in spite of knowing that some restaurants do not ...
It clearly specifies that customer satisfaction partially mediates information usefulness - customer loyalty link. Table 6 illustrates the structured model's indirect effects between the exogenous and endogenous constructs (with information usefulness and/or customer satisfaction as mediating factors). It clarifies that there are ...
not included in the research, then Customer Satisfaction (Variable Y) in Kopi Nako Ban dung will still increase by 6,629%. Service Quality (X1) has a positive value of 0.27 0.