- Resources Home 🏠
- Try SciSpace Copilot
- Search research papers
- Add Copilot Extension
- Try AI Detector
- Try Paraphraser
- Try Citation Generator
- April Papers
- June Papers
- July Papers


AI for thesis writing — Unveiling 7 best AI tools

Table of Contents
Writing a thesis is akin to piecing together a complex puzzle. Each research paper, every data point, and all the hours spent reading and analyzing contribute to this monumental task.
For many students, this journey is a relentless pursuit of knowledge, often marked by sleepless nights and tight deadlines.
Here, the potential of AI for writing a thesis or research papers becomes clear: artificial intelligence can step in, not to take over but to assist and guide.
Far from being just a trendy term, AI is revolutionizing academic research, offering tools that can make the task of thesis writing more manageable, more precise, and a little less overwhelming.
In this article, we’ll discuss the impact of AI on academic writing process, and articulate the best AI tools for thesis writing to enhance your thesis writing process.
The Impact of AI on Thesis Writing
Artificial Intelligence offers a supportive hand in thesis writing, adeptly navigating vast datasets, suggesting enhancements in writing, and refining the narrative.
With the integration of AI writing assistant, instead of requiring you to manually sift through endless articles, AI tools can spotlight the most pertinent pieces in mere moments. Need clarity or the right phrasing? AI-driven writing assistants are there, offering real-time feedback, ensuring your work is both articulative and academically sound.
AI tools for thesis writing harness Natural Language Processing (NLP) to generate content, check grammar, and assist in literature reviews. Simultaneously, Machine Learning (ML) techniques enable data analysis, provide personalized research recommendations, and aid in proper citation.
And for the detailed tasks of academic formatting and referencing? AI streamlines it all, ensuring your thesis meets the highest academic standards.
However, understanding AI's role is pivotal. It's a supportive tool, not the primary author. Your thesis remains a testament to your unique perspective and voice.
AI for writing thesis is there to amplify that voice, ensuring it's heard clearly and effectively.
How AI tools supplement your thesis writing
AI tools have emerged as invaluable allies for scholars. With just a few clicks, these advanced platforms can streamline various aspects of thesis writing, from data analysis to literature review.
Let's explore how an AI tool can supplement and transform your thesis writing style and process.
Efficient literature review : AI tools can quickly scan and summarize vast amounts of literature, making the process of literature review more efficient. Instead of spending countless hours reading through papers, researchers can get concise summaries and insights, allowing them to focus on relevant content.
Enhanced data analysis : AI algorithms can process and analyze large datasets with ease, identifying patterns, trends, and correlations that might be difficult or time-consuming for humans to detect. This capability is especially valuable in fields with massive datasets, like genomics or social sciences.
Improved writing quality : AI-powered writing assistants can provide real-time feedback on grammar, style, and coherence. They can suggest improvements, ensuring that the final draft of a research paper or thesis is of high quality.
Plagiarism detection : AI tools can scan vast databases of academic content to ensure that a researcher's work is original and free from unintentional plagiarism .
Automated citations : Managing and formatting citations is a tedious aspect of academic writing. AI citation generators can automatically format citations according to specific journal or conference standards, reducing the chances of errors.
Personalized research recommendations : AI tools can analyze a researcher's past work and reading habits to recommend relevant papers and articles, ensuring that they stay updated with the latest in their field.
Interactive data visualization : AI can assist in creating dynamic and interactive visualizations, making it easier for researchers to present their findings in a more engaging manner.
Top 7 AI Tools for Thesis Writing
The academic field is brimming with AI tools tailored for academic paper writing. Here's a glimpse into some of the most popular and effective ones.
Here we'll talk about some of the best ai writing tools, expanding on their major uses, benefits, and reasons to consider them.
If you've ever been bogged down by the minutiae of formatting or are unsure about specific academic standards, Typeset is a lifesaver.
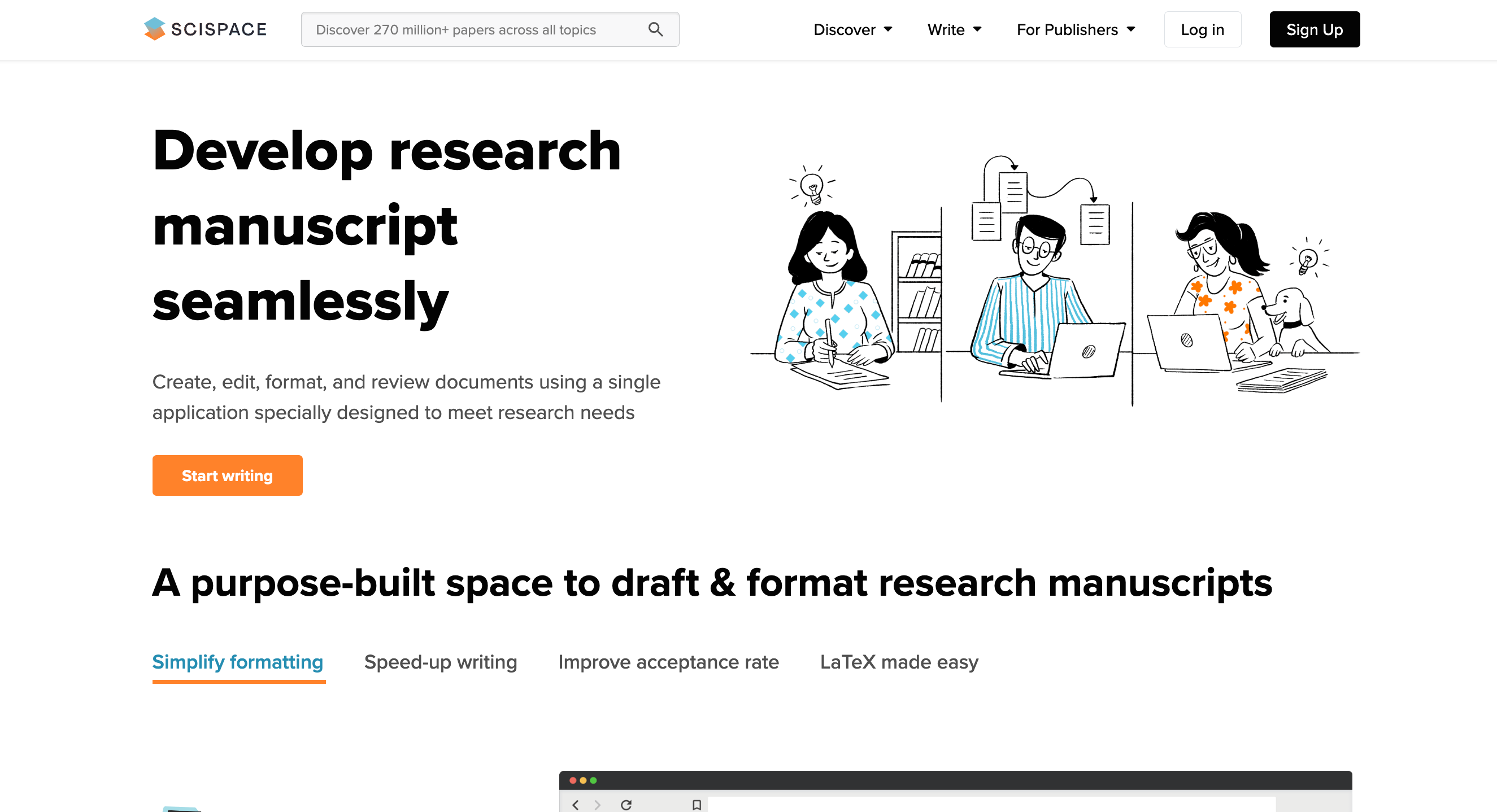
Typeset specializes in formatting, ensuring academic papers align with various journal and conference standards.
It automates the intricate process of academic formatting, saving you from the manual hassle and potential errors, inflating your writing experience.
An AI-driven writing assistant, Wisio elevates the quality of your thesis content. It goes beyond grammar checks, offering style suggestions tailored to academic writing.
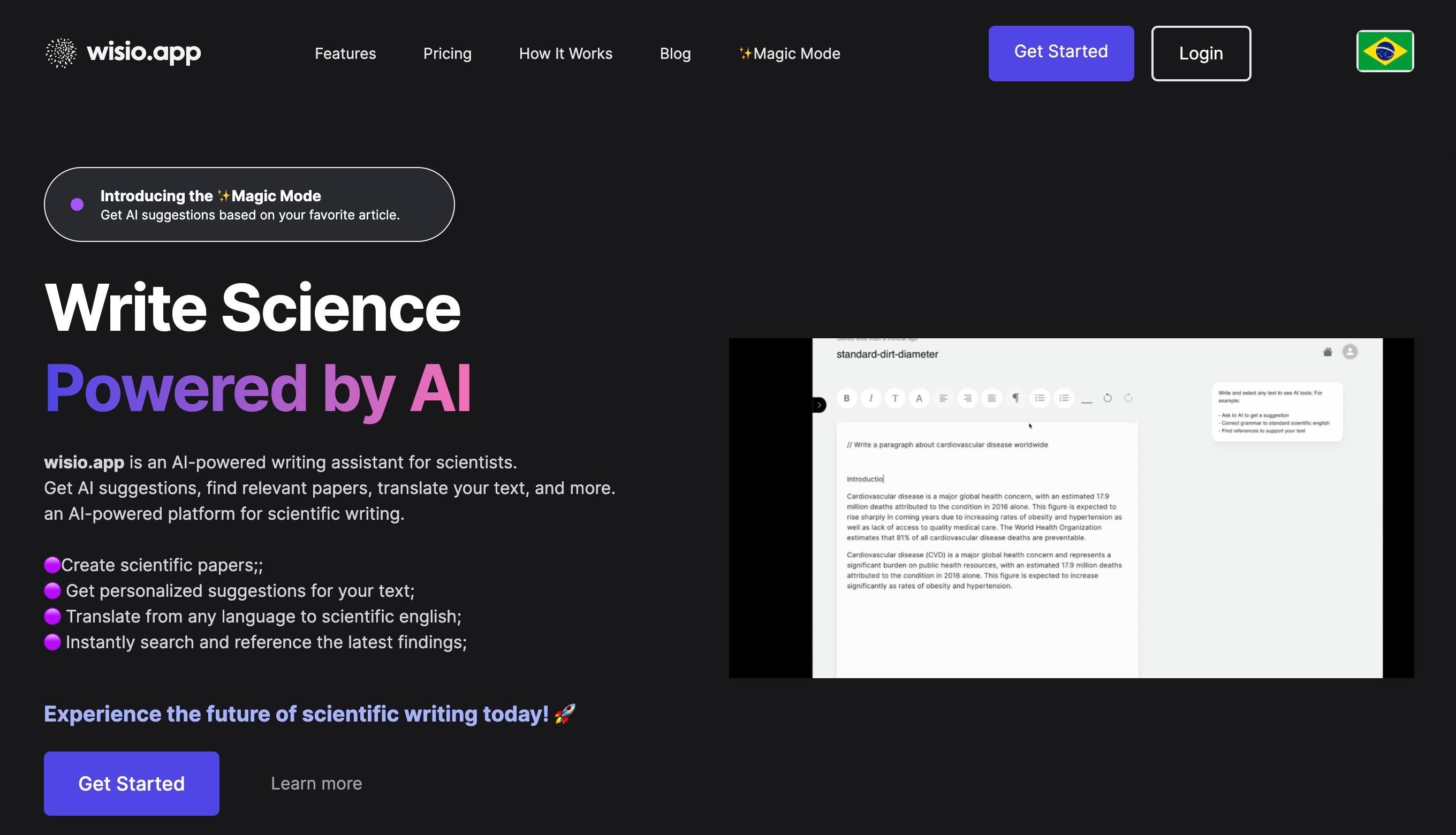
This ensures your thesis is both grammatically correct and maintains a scholarly tone. For moments of doubt or when maintaining a consistent style becomes challenging, Wisio acts as your personal editor, providing real-time feedback.
Known for its ability to generate and refine thesis content using AI algorithms, Texti ensures logical and coherent content flow according to the academic guidelines.
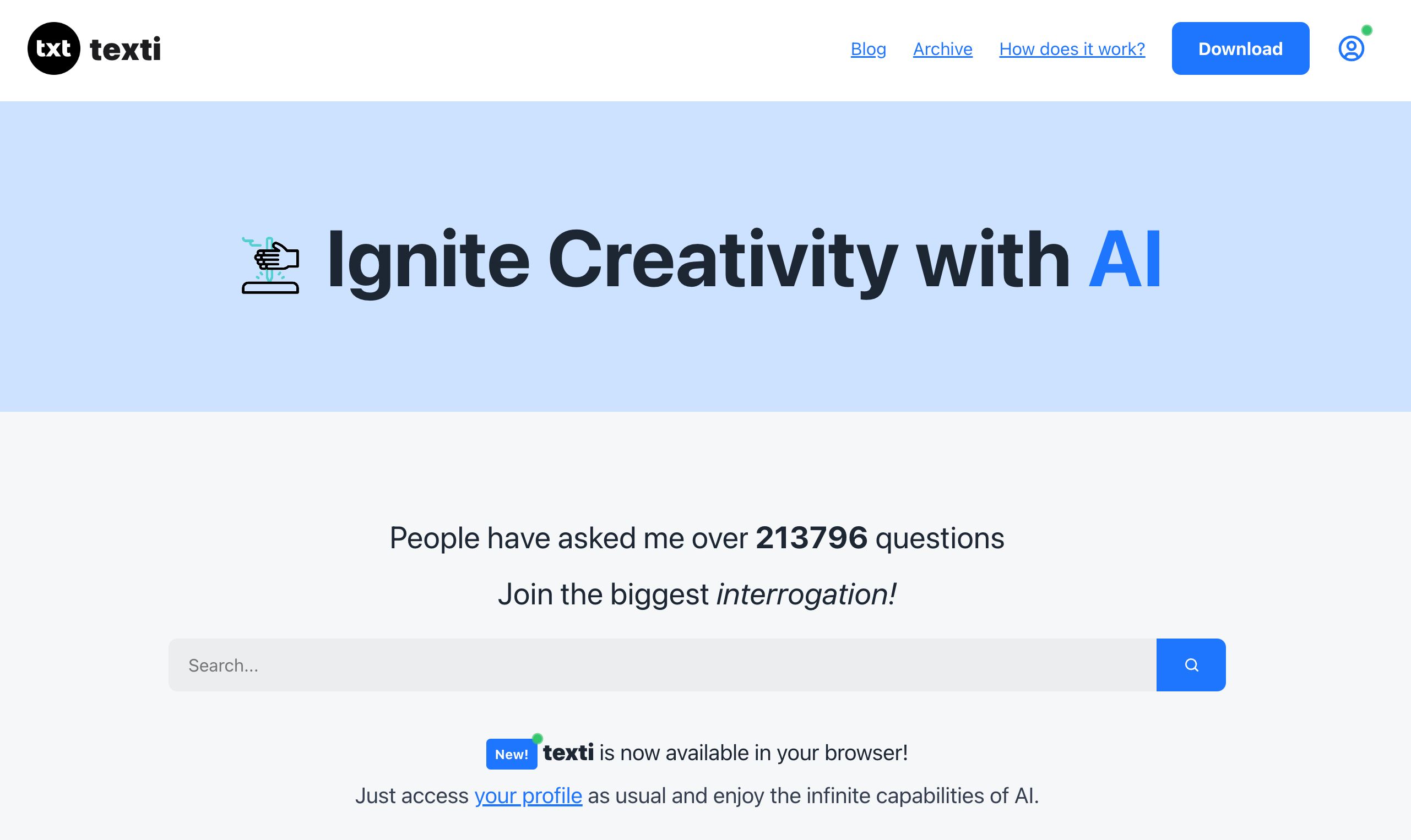
When faced with writer's block or a blank page, Texti can jumpstart your thesis writing process, aiding in drafting or refining content.
JustDone is an AI for thesis writing and content creation. It offers a straightforward three-step process for generating content, from choosing a template to customizing details and enjoying the final output.
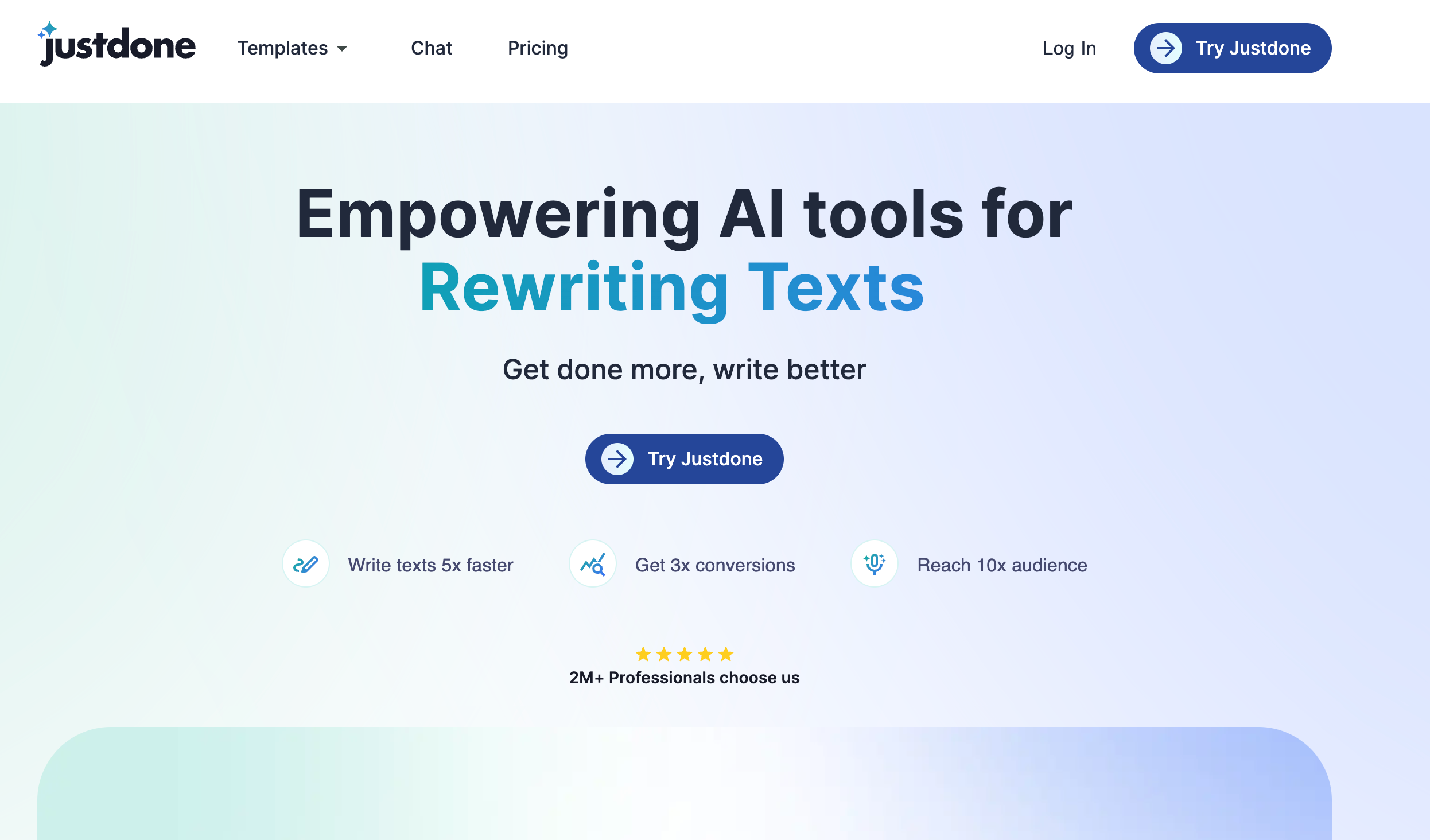
JustDone AI can generate thesis drafts based on the input provided by you. This can be particularly useful for getting started or overcoming writer's block.
This platform can refine and enhance the editing process, ensuring it aligns with academic standards and is free from common errors. Moreover, it can process and analyze data, helping researchers identify patterns, trends, and insights that might be crucial for their thesis.
Tailored for academic writing, Writefull offers style suggestions to ensure your content maintains a scholarly tone.
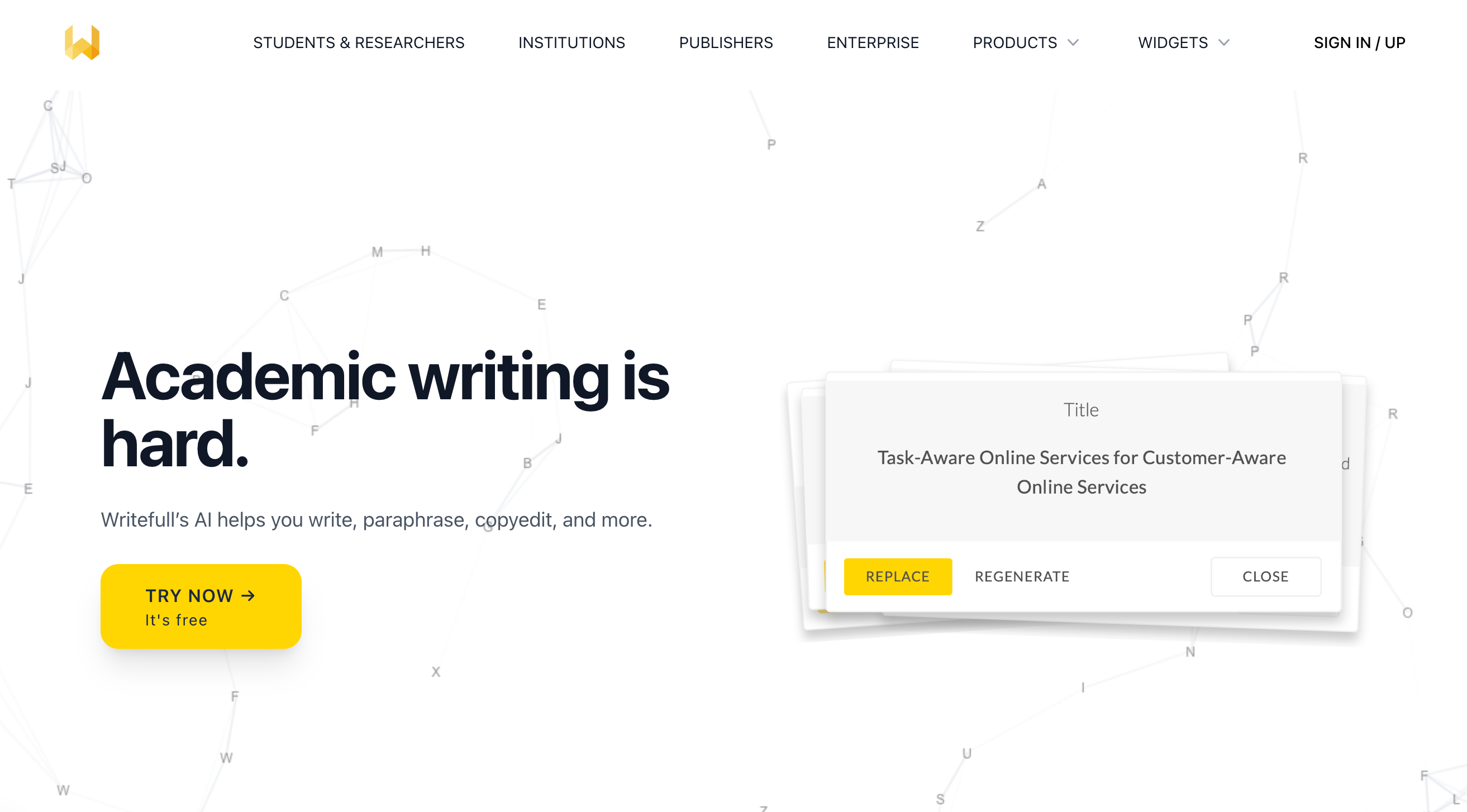
This AI for thesis writing provides feedback on your language use, suggesting improvements in grammar, vocabulary, and structure . Moreover, it compares your written content against a vast database of academic texts. This helps in ensuring that your writing is in line with academic standards.
Isaac Editor
For those seeking an all-in-one solution for writing, editing, and refining, Isaac Editor offers a comprehensive platform.
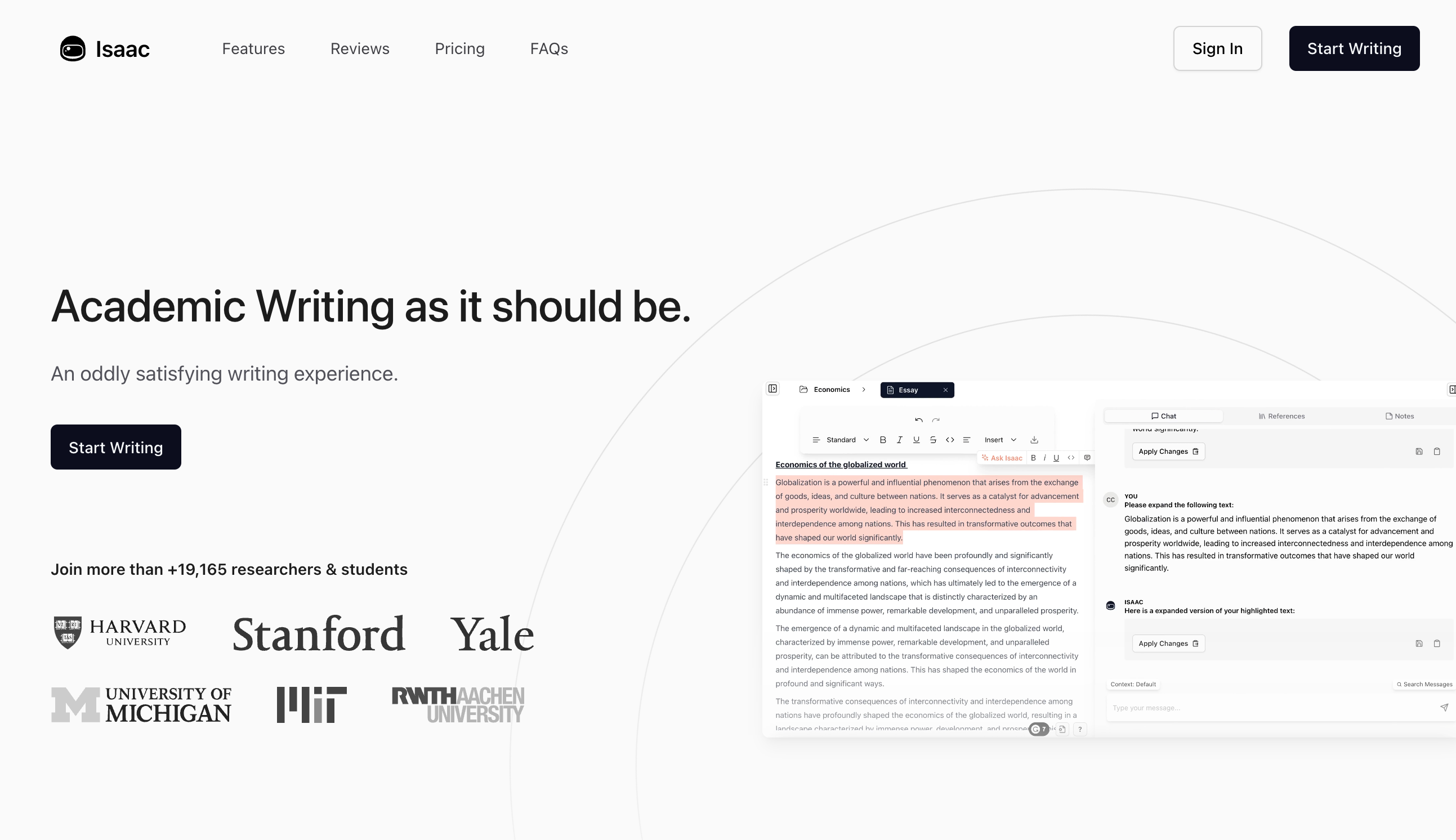
Combining traditional text editor features with AI, Isaac Editor streamlines the writing process. It's an all-in-one solution for writing, editing, and refining, ensuring your content is of the highest quality.
PaperPal , an AI-powered personal writing assistant, enhances academic writing skills, particularly for PhD thesis writing and English editing.
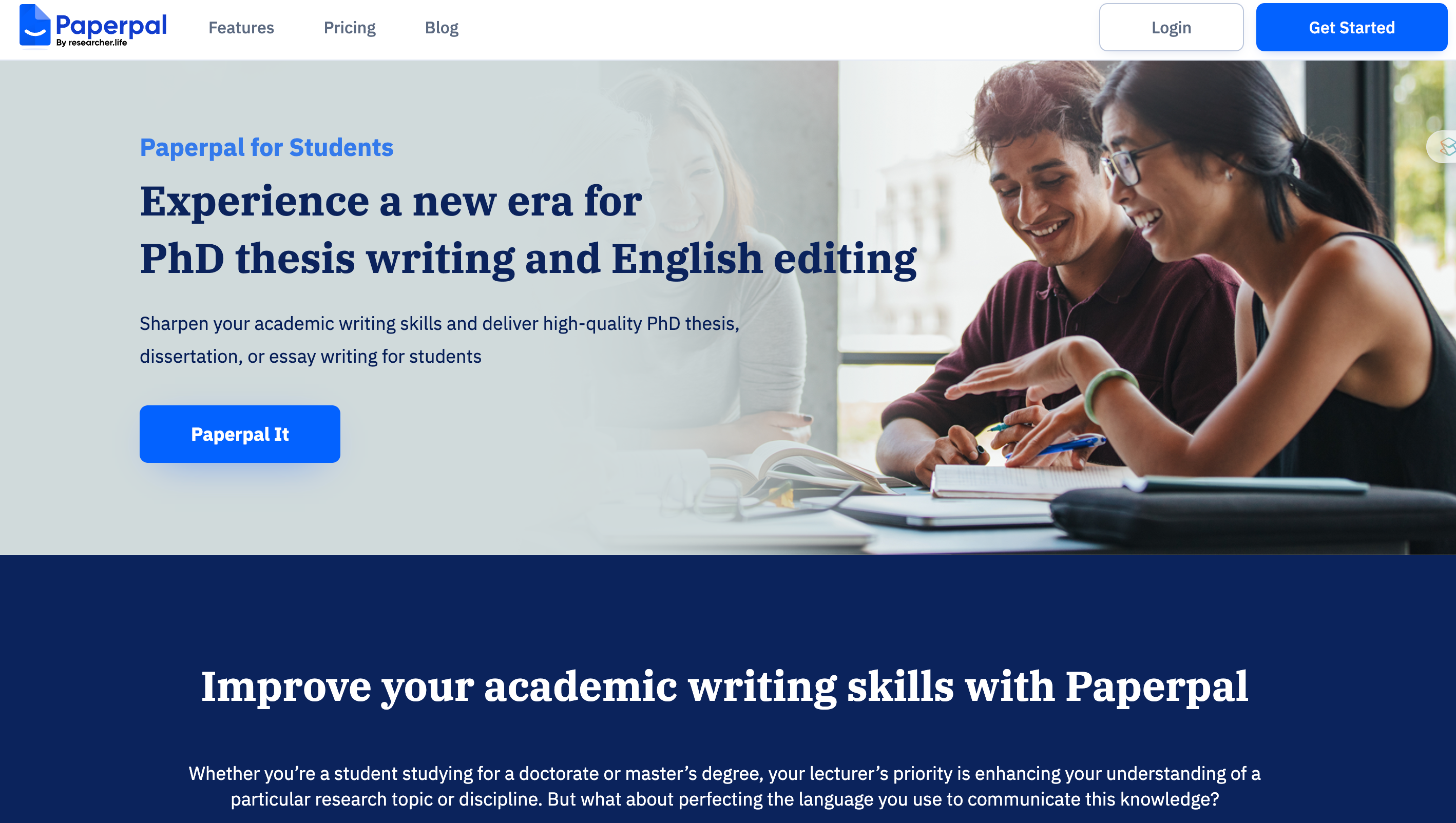
This AI for thesis writing offers comprehensive grammar, spelling, punctuation, and readability suggestions, along with detailed English writing tips.
It offers grammar checks, providing insights on rephrasing sentences, improving article structure, and other edits to refine academic writing.
The platform also offers tools like "Paperpal for Word" and "Paperpal for Web" to provide real-time editing suggestions, and "Paperpal for Manuscript" for a thorough check of completed articles or theses.
Is it ethical to use AI for thesis writing?
The AI for writing thesis has ignited discussions on authenticity. While AI tools offer unparalleled assistance, it's vital to maintain originality and not become overly reliant. Research thrives on unique contributions, and AI should be a supportive tool, not a replacement.
The key question: Can a thesis, significantly aided by AI, still be viewed as an original piece of work?
AI tools can simplify research, offer grammar corrections, and even produce content. However, there's a fine line between using AI as a helpful tool and becoming overly dependent on it.
In essence, while AI offers numerous advantages for thesis writing, it's crucial to use it judiciously. AI should complement human effort, not replace it. The challenge is to strike the right balance, ensuring genuine research contributions while leveraging AI's capabilities.
Wrapping Up
Nowadays, it's evident that AI tools are not just fleeting trends but pivotal game-changers.
They're reshaping how we approach, structure, and refine our theses, making the process more efficient and the output more impactful. But amidst this technological revolution, it's essential to remember the heart of any thesis: the researcher's unique voice and perspective .
AI tools are here to amplify that voice, not overshadow it. They're guiding you through the vast sea of information, ensuring our research stands out and resonates.
Try these tools out and let us know what worked for you the best.
Love using SciSpace tools? Enjoy discounts! Use SR40 (40% off yearly) and SR20 (20% off monthly). Claim yours here 👉 SciSpace Premium
Frequently Asked Questions
Yes, you can use AI to assist in writing your thesis. AI tools can help streamline various aspects of the writing process, such as data analysis, literature review, grammar checks, and content refinement.
However, it's essential to use AI as a supportive tool and not a replacement for original research and critical thinking. Your thesis should reflect your unique perspective and voice.
Yes, there are AI tools designed to assist in writing research papers. These tools can generate content, suggest improvements, help with formatting, and even provide real-time feedback on grammar and coherence.
Examples include Typeset, JustDone, Writefull, and Texti. However, while they can aid the process, the primary research, analysis, and conclusions should come from the researcher.
The "best" AI for writing papers depends on your specific needs. For content generation and refinement, Texti is a strong contender.
For grammar checks and style suggestions tailored to academic writing, Writefull is highly recommended. JustDone offers a user-friendly interface for content creation. It's advisable to explore different tools and choose one that aligns with your requirements.
To use AI for writing your thesis:
1. Identify the areas where you need assistance, such as literature review, data analysis, content generation, or grammar checks.
2. Choose an AI tool tailored for academic writing, like Typeset, JustDone, Texti, or Writefull.
3. Integrate the tool into your writing process. This could mean using it as a browser extension, a standalone application, or a plugin for your word processor.
4. As you write or review content, use the AI tool for real-time feedback, suggestions, or content generation.
5. Always review and critically assess the suggestions or content provided by the AI to ensure it aligns with your research goals and maintains academic integrity.

You might also like

What is a thesis | A Complete Guide with Examples


Analytics Insight
10 Best AI tools for Thesis Writing

Revolutionizing Academic Writing: The 10 Best AI Tools for Streamlining Thesis Creation
Thesis writing is a challenging yet integral part of academic pursuits, and the integration of Artificial Intelligence (AI) tools has revolutionized the research and writing process. In this article, we explore the top 10 AI tools that are invaluable for thesis writing, offering efficiency, precision, and enhanced productivity to researchers and students alike.
1. Grammarly:
Grammarly is an AI-powered writing assistant that goes beyond basic grammar checks. It provides advanced suggestions for clarity, tone, and style, ensuring your thesis is not only grammatically correct but also well-polished and professional.
Zotero is a reference management tool enhanced by AI capabilities. It helps researchers organize sources, generate citations, and create bibliographies effortlessly. The AI-driven features simplify the citation process, saving valuable time during thesis writing.
3. Copyscape:
Maintaining academic integrity is crucial, and Copyscape is an AI tool designed to detect plagiarism. It scans the content against a vast database, ensuring your thesis is original and free from unintentional similarities with existing works.
4. Ref-N-Write:
Ref-N-Write is an AI-powered academic writing tool that assists in generating research papers and thesis content. It offers a vast collection of academic phrases and sentence structures, aiding researchers in articulating their ideas with precision.
5. EndNote:
EndNote is a reference management tool that integrates AI for better organization and citation management. It streamlines the process of collecting, organizing, and citing references, allowing researchers to focus more on the content of their thesis.
6. Quetext:
Quetext is an AI-based plagiarism checker that provides a comprehensive analysis of your thesis content. Its advanced algorithms identify potential instances of plagiarism, ensuring the originality and authenticity of your research.
7. ProWritingAid:
ProWritingAid is an AI-driven writing tool that analyzes writing style, suggests improvements, and checks for issues like overused words and vague phrasing. It enhances the overall quality of your thesis by providing detailed insights into writing strengths and weaknesses.
8. Mendeley:
Mendeley is a reference manager and academic social network that incorporates AI for personalized recommendations. It suggests relevant research papers, articles, and resources based on your research interests, enriching the reference pool for your thesis.
9. IBM Watson Discovery:
IBM Watson Discovery is a powerful AI tool for data discovery and analysis. It can be employed to extract insights from large datasets, aiding researchers in gathering relevant information for their theses and making data-driven conclusions.
10. Evernote:
Evernote, equipped with AI capabilities, is a versatile note-taking tool. It allows researchers to organize and store research notes, ideas, and references in a systematic manner. The AI-enhanced search function makes retrieving information seamless.
Conclusion:
The integration of AI tools into the thesis writing process has ushered in a new era of efficiency and precision for researchers and students. From grammar checks to plagiarism detection and reference management, these 10 AI tools contribute to streamlining the academic writing journey, ensuring that the focus remains on the substance and quality of the thesis content. Embracing these tools empowers researchers to navigate the complexities of thesis writing with enhanced productivity and confidence.

Disclaimer: Any financial and crypto market information given on Analytics Insight are sponsored articles, written for informational purpose only and is not an investment advice. The readers are further advised that Crypto products and NFTs are unregulated and can be highly risky. There may be no regulatory recourse for any loss from such transactions. Conduct your own research by contacting financial experts before making any investment decisions. The decision to read hereinafter is purely a matter of choice and shall be construed as an express undertaking/guarantee in favour of Analytics Insight of being absolved from any/ all potential legal action, or enforceable claims. We do not represent nor own any cryptocurrency, any complaints, abuse or concerns with regards to the information provided shall be immediately informed here .
You May Also Like

Bitcoin Slots & Bonus Codes: Latest Bitcoin Slots Reviews & Promos 2023

How Have Chatbots Changed Customer Service?

Best Cash Advance Loans in the U.S – Top Choices for Fast Funds

Bitcoin will be the Worst Hit Crypto After G20 Releases Regulations: 2023 Predictions

Analytics Insight® is an influential platform dedicated to insights, trends, and opinion from the world of data-driven technologies. It monitors developments, recognition, and achievements made by Artificial Intelligence, Big Data and Analytics companies across the globe.

- Select Language:
- Privacy Policy
- Content Licensing
- Terms & Conditions
- Submit an Interview
Special Editions
- Dec – Crypto Weekly Vol-1
- 40 Under 40 Innovators
- Women In Technology
- Market Reports
- AI Glossary
- Infographics
Latest Issue

Disclaimer: Any financial and crypto market information given on Analytics Insight is written for informational purpose only and is not an investment advice. Conduct your own research by contacting financial experts before making any investment decisions, more information here .
Second Menu

Have a language expert improve your writing
Check your paper for plagiarism in 10 minutes, generate your apa citations for free.
- Knowledge Base
Using AI tools
AI Writing Tools | Definition, Uses & Implications
AI writing tools are artificial intelligence (AI) software applications like ChatGPT that help to automate or assist the writing process. These tools use machine learning algorithms to generate human-sounding text in response to users’ text-based prompts.
Other AI tools, such as grammar checkers , paraphrasers and summarizers serve more specific functions, like identifying grammar and spelling mistakes or rephrasing text.
Table of contents
How do ai writing tools work, what can ai writing tools be used for, implications of ai writing tools, other interesting articles, frequently asked questions about ai writing tools.
AI writing tools (chatbots, grammar checkers, etc.) use natural language processing (NLP) algorithms, machine learning , and large language models (LLMs) to generate or improve written text. These tools are trained to identify patterns in vast amounts of data. The tools then use these patterns to analyze human inputs (or prompts) and generate natural-sounding outputs (similar to how predictive text works).
Different AI tools may be programmed in different ways. For example, ChatGPT, the popular chatbot developed by OpenAI and released in November 2022, uses generative pre-trained transformers (GPTs) and was trained using reinforcement learning from human feedback (RLHF) , meaning that the tool was given feedback from humans to help guide it towards the most helpful responses.
AI writing tools have a number of helpful applications. Some of these applications are discussed below.
Interactive learning and feedback
Generative AI tools can be helpful resources for conversational learning. By inputting questions, users can engage in interactive learning at any time.
You can also prompt these tools to provide feedback on your work to improve the clarity or consistency of your writing. However, it’s important not to upload sensitive information, as user inputs are generally saved by the tool for the purposes of training future models.
Below is an example of how ChatGPT can be used for interactive learning.
Example: Using ChatGPT for interactive learning
- Tell me three facts about Carthaginian culture
- Carthaginians were known for their maritime trade and commerce, and their navy was one of the strongest in the ancient Mediterranean world. They established colonies and trading posts throughout the Mediterranean, including in Sicily, Sardinia, and North Africa.
- Carthaginians had a polytheistic religion, and their chief deity was Baal Hammon, the god of fertility, agriculture, and the sky. They also worshiped a number of other gods and goddesses, including Tanit, the goddess of love, fertility, and war.
- Carthaginians were skilled metalworkers and produced a variety of metal goods, including weapons, armor, jewelry, and household objects. They were particularly famous for their bronze work, which was highly prized in the ancient world. Carthaginian bronze was so valued that it was used by the Romans to make their famous statues and other works of art.
- Tell me three more facts about the Carthaginian navy
- The Carthaginian navy was one of the most advanced and powerful navies of the ancient world. It was instrumental in establishing Carthage as a dominant power in the Mediterranean region.
- The Carthaginians were known for their skill in shipbuilding and developed a variety of ship types for different purposes, including warships, transport ships, and cargo ships. One of their most famous ship types was the quinquereme, a large warship with five rows of oars.
- The Carthaginian navy was led by a commander called the “admiral.” The admiral was responsible for overseeing the fleet and making strategic decisions about naval warfare. One of the most famous Carthaginian admirals was Hannibal Barca, who led the Carthaginian fleet during the First Punic War against Rome.
Generating research questions and outlines
AI writing tools can be used to generate potential research questions or research paper outlines . You can then refine the tool’s responses by further prompting it or directly editing the output yourself to ensure the result makes sense and fits your requirements.
Below is an example of how ChatGPT can be used to generate potential reseach questions and research method ideas.
Example: Using ChatGPT to generate research questions and methods
- Develop three possible research questions on the following topic: “The influence of technology on second language acquisition.”
- What is the impact of technology-assisted language learning (TALL) on the acquisition of a second language?
- How do different types of technology, such as mobile applications, virtual reality, and online platforms, affect second language acquisition outcomes?
- How do individual learner characteristics, such as age, proficiency level, and motivation, interact with the use of technology in second language acquisition?
- Suggest three quantitative research methods appropriate to the second question.
- Experimental research: This research method involves manipulating an independent variable (in this case, the type of technology used) to observe its effect on a dependent variable (second language acquisition outcomes). Participants would be randomly assigned to different groups using different types of technology to learn a second language, and then their language acquisition outcomes would be measured and compared.
- Survey research: This research method involves using questionnaires to gather data from a large group of participants. In this case, a survey could be designed to gather information on participants’ use of different types of technology to learn a second language, as well as their language acquisition outcomes. This would allow for a large-scale investigation of how different types of technology are being used, and what their impact might be.
- Correlational research: This research method involves examining the relationship between two or more variables. In this case, a correlational study could be conducted to investigate whether there is a relationship between the type of technology used and language acquisition outcomes. Data could be collected from participants using different types of technology to learn a second language, and then the correlation between the type of technology and language acquisition outcomes could be calculated.
Paraphrasing text
AI tools like ChatGPT and Scribbr’s free paraphrasing tool can help you paraphrase text to express your ideas more clearly, avoid repetition, and maintain a consistent tone throughout your writing.
They can also help you incorporate scholarly sources in your writing in a more concise and fluent way, without the need for direct quotations. However, it’s important to correctly cite all sources to avoid accidental plagiarism.
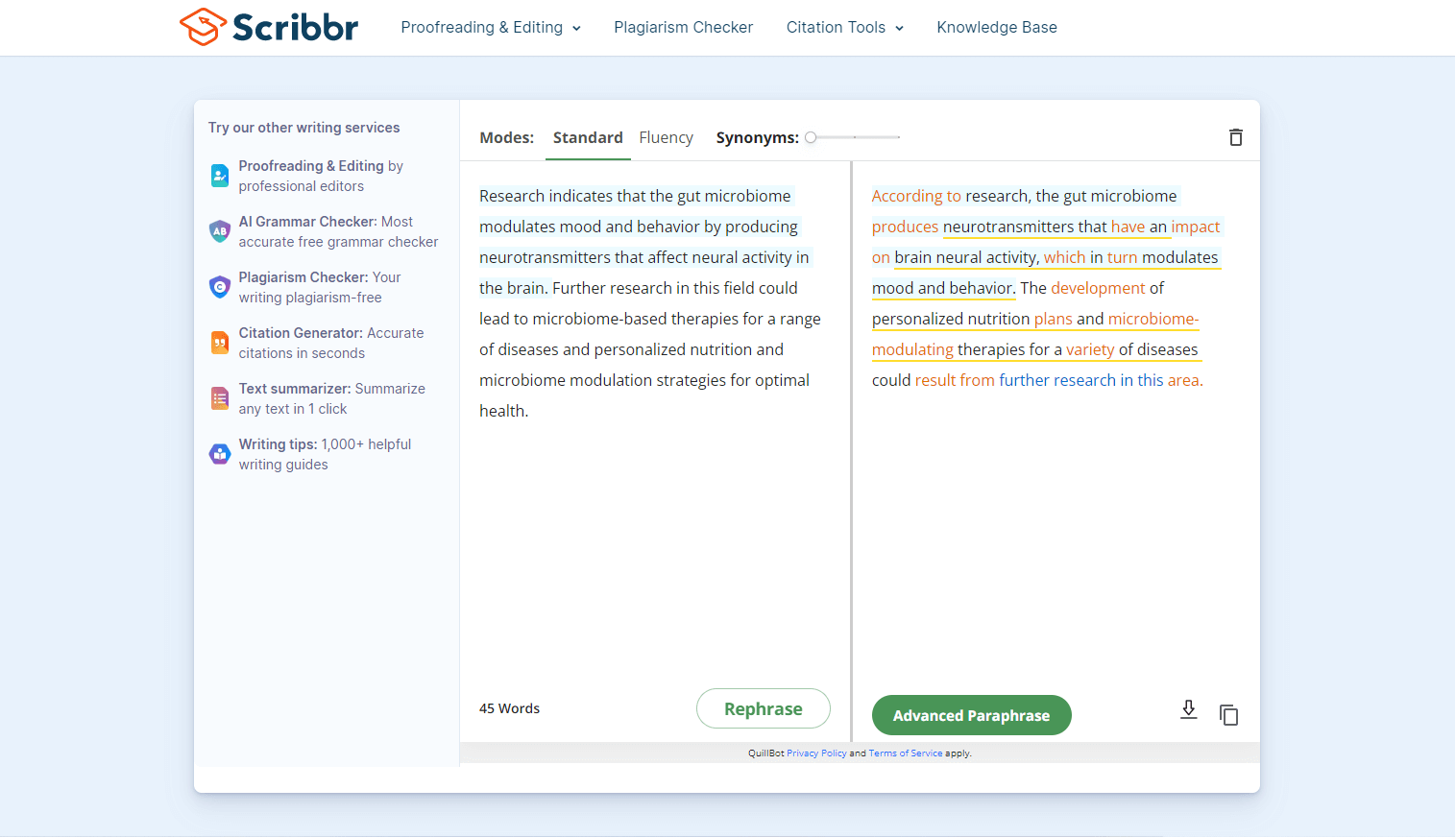
Summarizing text
AI writing tools can help condense a text to its most important and relevant ideas. This can help you understand complex information more easily. You can also use summarizer tools on your own work to summarize your central argument, clarify your research question, and form conclusions.
You can do this using generative AI tools or more specialized tools like Scribbr’s free text-summarizer .
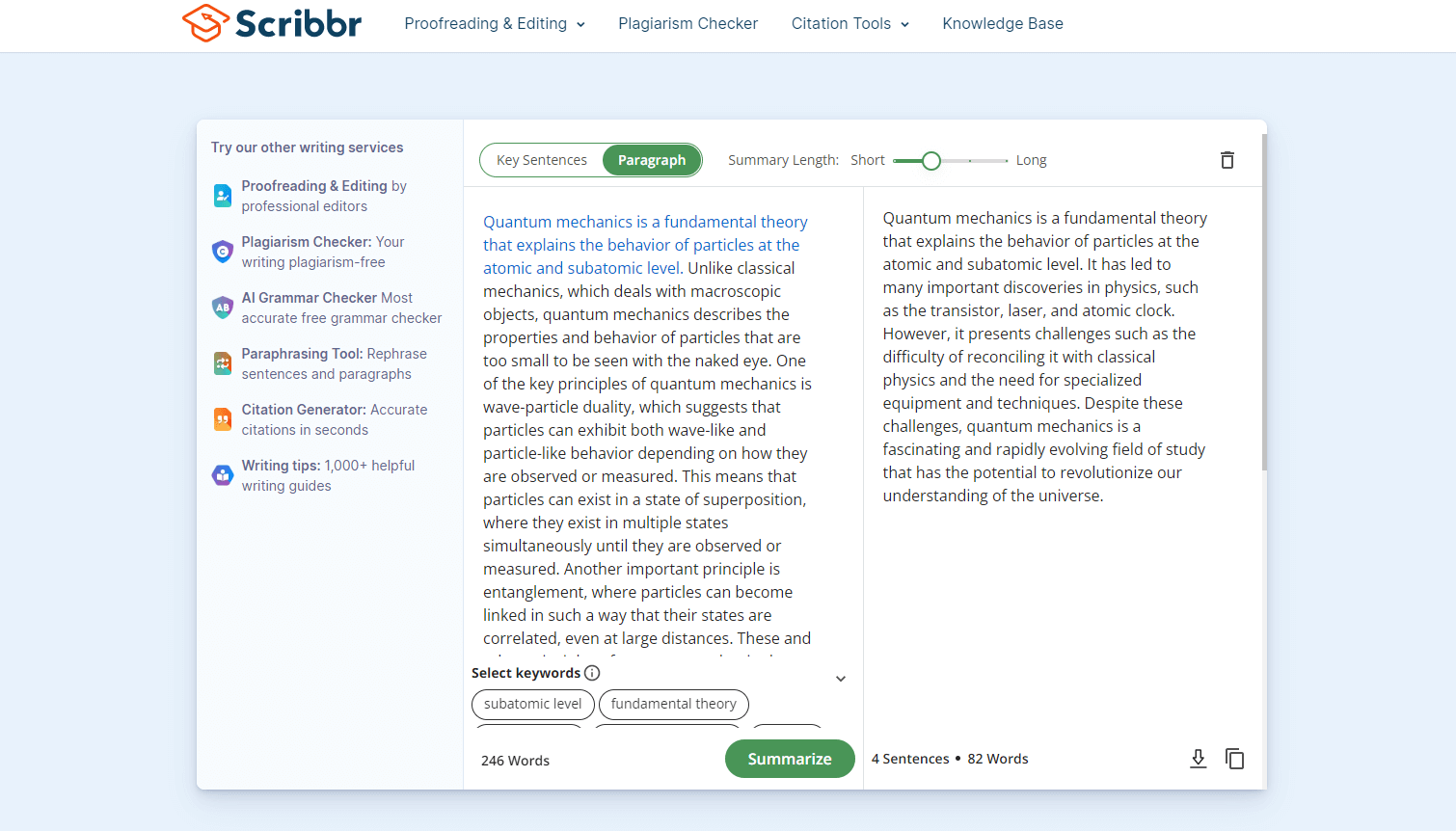
Proofreading text
AI writing tools can be used to identify spelling, grammar, and punctuation mistakes and suggest corrections. These tools can help to improve the clarity of your writing and avoid common mistakes .
While AI tools like ChatGPT offer useful suggestions, they can also potentially miss some mistakes or even introduce new grammatical errors into your writing.
We advise using Scribbr’s proofreading and editing service or a tool like Scribbr’s free grammar checker , which is designed specifically for this purpose.
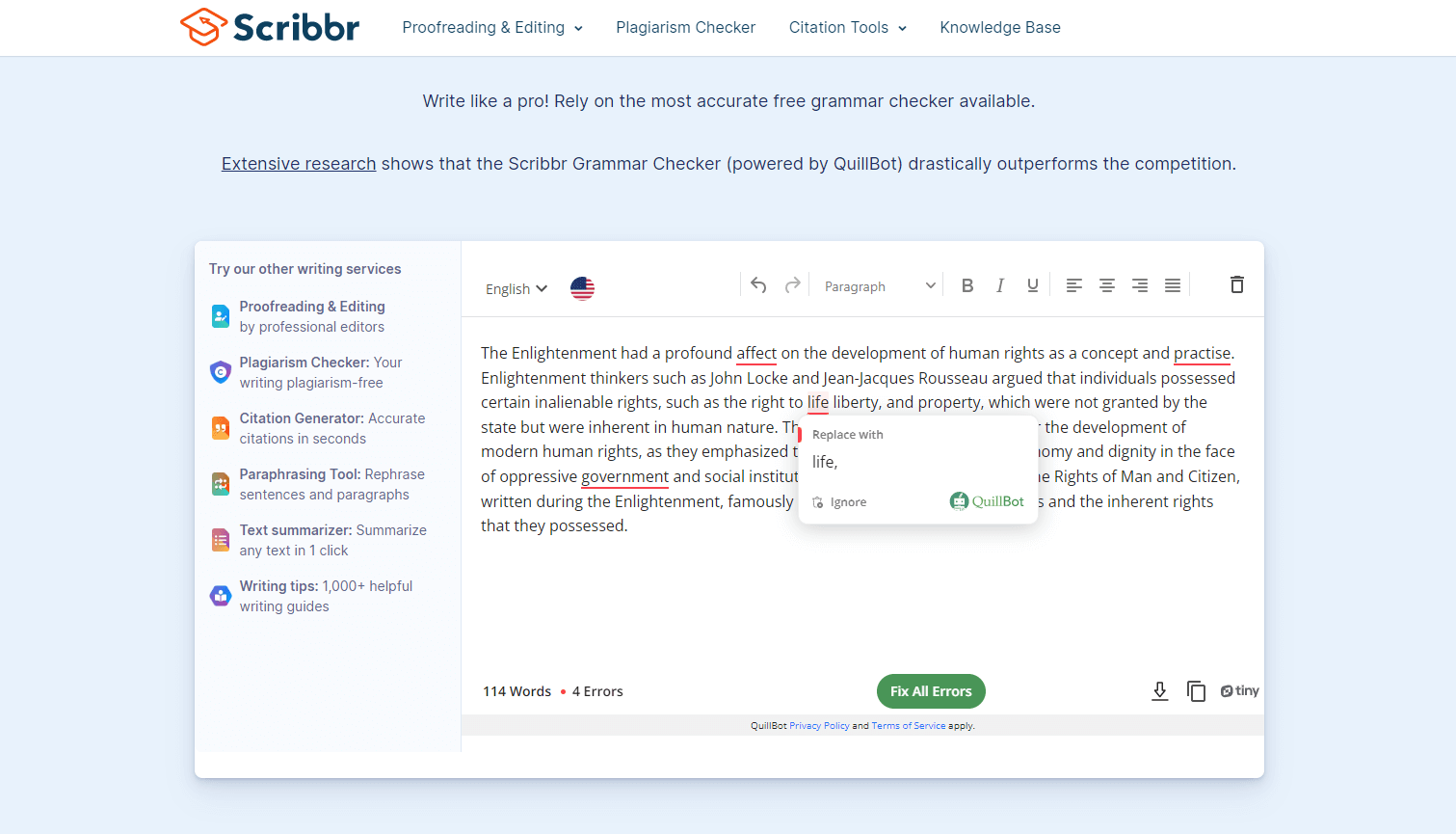
Translating text
AI translation tools like Google Translate can be used to translate text from a source language into various target languages. While the quality of these tools tend to vary depending on the languages used, they’re constantly developing and are increasingly accurate.
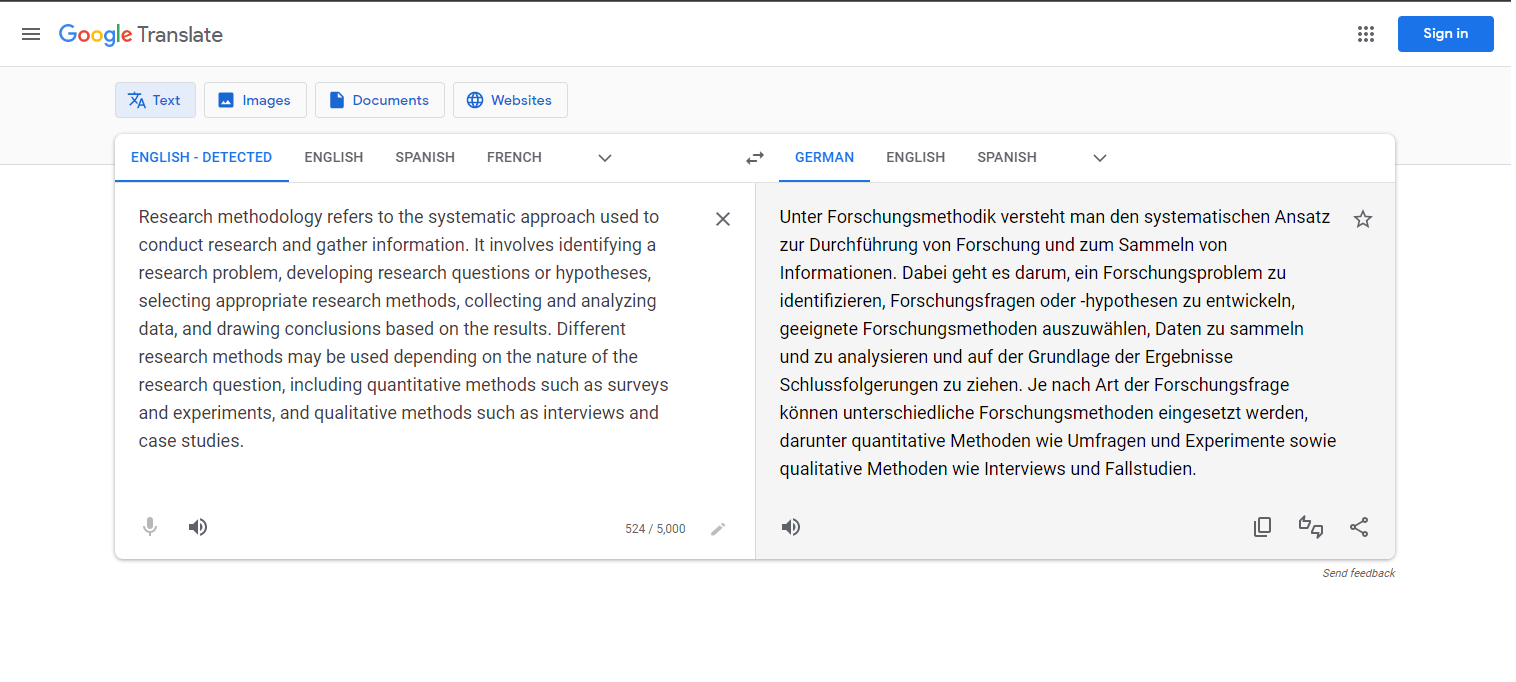
While there are many benefits to using AI writing tools, some commentators have emphasized the limitations of AI tools and the potential disadvantages of using them. These drawbacks are discussed below.
Impact on learning
One of the potential pitfalls of using AI writing tools is the effect they might have on a student’s learning and skill set. Using AI tools to generate a paper, thesis , or dissertation , for example, may impact a student’s research, critical thinking, and writing skills.
However, other commentators argue that AI tools can be used to promote critical thinking (e.g., by having a student evaluate a tool’s output and refine it).
Consistency and accuracy
Generative AI tools (such as ChatGPT) are not always trustworthy and sometimes produce results that are inaccurate or factually incorrect. Although these tools are programmed to answer questions, they can’t judge the accuracy of the information they provide and may generate incorrect answers or contradict themselves.
It’s important to verify AI-generated information against a credible source .
Grammatical mistakes
While generative AI tools can produce written text, they don’t actually understand what they’re saying and sometimes produce grammar, spelling, and punctuation mistakes.
You can combine the use of generative AI tools with Scribbr’s grammar checker , which is designed to catch these mistakes.
Ethics and plagiarism
As AI writing tools are trained on large sets of data, they may produce content that is similar to existing content (which they usually cannot cite correctly), which can be considered plagiarism.
Furthermore, passing off AI-generated text as your own work is usually considered a form of plagiarism and is likely to be prohibited by your university. This offense may be recognized by your university’s plagiarism checker or AI detector .
If you want more tips on using AI tools , understanding plagiarism , and citing sources , make sure to check out some of our other articles with explanations, examples, and formats.
- Citing ChatGPT
- Best grammar checker
- Best paraphrasing tool
- ChatGPT in your studies
- Is ChatGPT trustworthy?
- Types of plagiarism
- Self-plagiarism
- Avoiding plagiarism
- Academic integrity
- Best plagiarism checker
Citing sources
- Citation styles
- In-text citation
- Citation examples
- Annotated bibliography
AI writing tools can be used to perform a variety of tasks.
Generative AI writing tools (like ChatGPT ) generate text based on human inputs and can be used for interactive learning, to provide feedback, or to generate research questions or outlines.
These tools can also be used to paraphrase or summarize text or to identify grammar and punctuation mistakes. Y ou can also use Scribbr’s free paraphrasing tool , summarizing tool , and grammar checker , which are designed specifically for these purposes.
Using AI writing tools (like ChatGPT ) to write your essay is usually considered plagiarism and may result in penalization, unless it is allowed by your university . Text generated by AI tools is based on existing texts and therefore cannot provide unique insights. Furthermore, these outputs sometimes contain factual inaccuracies or grammar mistakes.
However, AI writing tools can be used effectively as a source of feedback and inspiration for your writing (e.g., to generate research questions ). Other AI tools, like grammar checkers, can help identify and eliminate grammar and punctuation mistakes to enhance your writing.
You can access ChatGPT by signing up for a free account:
- Follow this link to the ChatGPT website.
- Click on “Sign up” and fill in the necessary details (or use your Google account). It’s free to sign up and use the tool.
- Type a prompt into the chat box to get started!
A ChatGPT app is also available for iOS, and an Android app is planned for the future. The app works similarly to the website, and you log in with the same account for both.
Yes, ChatGPT is currently available for free. You have to sign up for a free account to use the tool, and you should be aware that your data may be collected to train future versions of the model.
To sign up and use the tool for free, go to this page and click “Sign up.” You can do so with your email or with a Google account.
A premium version of the tool called ChatGPT Plus is available as a monthly subscription. It currently costs $20 and gets you access to features like GPT-4 (a more advanced version of the language model). But it’s optional: you can use the tool completely free if you’re not interested in the extra features.
ChatGPT was publicly released on November 30, 2022. At the time of its release, it was described as a “research preview,” but it is still available now, and no plans have been announced so far to take it offline or charge for access.
ChatGPT continues to receive updates adding more features and fixing bugs. The most recent update at the time of writing was on May 24, 2023.
Is this article helpful?
Other students also liked.
- What Is ChatGPT? | Everything You Need to Know
- Is ChatGPT Trustworthy? | Accuracy Tested
- University Policies on AI Writing Tools | Overview & List
More interesting articles
- 9 Ways to Use ChatGPT for Language Learning
- Best AI Detector | Free & Premium Tools Tested
- Best Summary Generator | Tools Tested & Reviewed
- ChatGPT Citations | Formats & Examples
- ChatGPT Does Not Solve All Academic Writing Problems
- ChatGPT vs. Human Editor | Proofreading Experiment
- Easy Introduction to Reinforcement Learning
- Ethical Implications of ChatGPT
- Glossary of AI Terms | Acronyms & Terminology
- How Do AI Detectors Work? | Methods & Reliability
- How to Use ChatGPT | Basics & Tips
- How to use ChatGPT in your studies
- How to Write a Conclusion Using ChatGPT | Tips & Examples
- How to Write a Paper with ChatGPT | Tips & Examples
- How to Write an Essay with ChatGPT | Tips & Examples
- How to Write an Introduction Using ChatGPT | Tips & Examples
- How to Write Good ChatGPT Prompts
- Is ChatGPT Safe? | Quick Guide & Tips
- Is Using ChatGPT Cheating?
- Supervised vs. Unsupervised Learning: Key Differences
- Using ChatGPT for Assignments | Tips & Examples
- Using ChatGPT to Write a College Essay | Tips & Examples
- What Are the Legal Implications of ChatGPT?
- What Are the Limitations of ChatGPT?
- What Can ChatGPT Do? | Suggestions & Examples
- What Is an Algorithm? | Definition & Examples
- What Is Data Mining? | Definition & Techniques
- What Is Deep Learning? | A Beginner's Guide
- What Is Generative AI? | Meaning & Examples
- What Is Machine Learning? | A Beginner's Guide
"I thought AI Proofreading was useless but.."
I've been using Scribbr for years now and I know it's a service that won't disappoint. It does a good job spotting mistakes”

The best AI tools for research papers and academic research (Literature review, grants, PDFs and more)
As our collective understanding and application of artificial intelligence (AI) continues to evolve, so too does the realm of academic research. Some people are scared by it while others are openly embracing the change.
Make no mistake, AI is here to stay!
Instead of tirelessly scrolling through hundreds of PDFs, a powerful AI tool comes to your rescue, summarizing key information in your research papers. Instead of manually combing through citations and conducting literature reviews, an AI research assistant proficiently handles these tasks.
These aren’t futuristic dreams, but today’s reality. Welcome to the transformative world of AI-powered research tools!
The influence of AI in scientific and academic research is an exciting development, opening the doors to more efficient, comprehensive, and rigorous exploration.
This blog post will dive deeper into these tools, providing a detailed review of how AI is revolutionizing academic research. We’ll look at the tools that can make your literature review process less tedious, your search for relevant papers more precise, and your overall research process more efficient and fruitful.
I know that I wish these were around during my time in academia. It can be quite confronting when trying to work out what ones you should and shouldn’t use. A new one seems to be coming out every day!
Here is everything you need to know about AI for academic research and the ones I have personally trialed on my Youtube channel.
Best ChatGPT interface – Chat with PDFs/websites and more
I get more out of ChatGPT with HeyGPT . It can do things that ChatGPT cannot which makes it really valuable for researchers.
Use your own OpenAI API key ( h e re ). No login required. Access ChatGPT anytime, including peak periods. Faster response time. Unlock advanced functionalities with HeyGPT Ultra for a one-time lifetime subscription
AI literature search and mapping – best AI tools for a literature review – elicit and more
Harnessing AI tools for literature reviews and mapping brings a new level of efficiency and precision to academic research. No longer do you have to spend hours looking in obscure research databases to find what you need!
AI-powered tools like Semantic Scholar and elicit.org use sophisticated search engines to quickly identify relevant papers.
They can mine key information from countless PDFs, drastically reducing research time. You can even search with semantic questions, rather than having to deal with key words etc.
With AI as your research assistant, you can navigate the vast sea of scientific research with ease, uncovering citations and focusing on academic writing. It’s a revolutionary way to take on literature reviews.
- Elicit – https://elicit.org
- Supersymmetry.ai: https://www.supersymmetry.ai
- Semantic Scholar: https://www.semanticscholar.org
- Connected Papers – https://www.connectedpapers.com/
- Research rabbit – https://www.researchrabbit.ai/
- Laser AI – https://laser.ai/
- Litmaps – https://www.litmaps.com
- Inciteful – https://inciteful.xyz/
- Scite – https://scite.ai/
- System – https://www.system.com
If you like AI tools you may want to check out this article:
- How to get ChatGPT to write an essay [The prompts you need]
AI-powered research tools and AI for academic research
AI research tools, like Concensus, offer immense benefits in scientific research. Here are the general AI-powered tools for academic research.
These AI-powered tools can efficiently summarize PDFs, extract key information, and perform AI-powered searches, and much more. Some are even working towards adding your own data base of files to ask questions from.
Tools like scite even analyze citations in depth, while AI models like ChatGPT elicit new perspectives.
The result? The research process, previously a grueling endeavor, becomes significantly streamlined, offering you time for deeper exploration and understanding. Say goodbye to traditional struggles, and hello to your new AI research assistant!
- Bit AI – https://bit.ai/
- Consensus – https://consensus.app/
- Exper AI – https://www.experai.com/
- Hey Science (in development) – https://www.heyscience.ai/
- Iris AI – https://iris.ai/
- PapersGPT (currently in development) – https://jessezhang.org/llmdemo
- Research Buddy – https://researchbuddy.app/
- Mirror Think – https://mirrorthink.ai
AI for reading peer-reviewed papers easily
Using AI tools like Explain paper and Humata can significantly enhance your engagement with peer-reviewed papers. I always used to skip over the details of the papers because I had reached saturation point with the information coming in.
These AI-powered research tools provide succinct summaries, saving you from sifting through extensive PDFs – no more boring nights trying to figure out which papers are the most important ones for you to read!
They not only facilitate efficient literature reviews by presenting key information, but also find overlooked insights.
With AI, deciphering complex citations and accelerating research has never been easier.
- Open Read – https://www.openread.academy
- Chat PDF – https://www.chatpdf.com
- Explain Paper – https://www.explainpaper.com
- Humata – https://www.humata.ai/
- Lateral AI – https://www.lateral.io/
- Paper Brain – https://www.paperbrain.study/
- Scholarcy – https://www.scholarcy.com/
- SciSpace Copilot – https://typeset.io/
- Unriddle – https://www.unriddle.ai/
- Sharly.ai – https://www.sharly.ai/
AI for scientific writing and research papers
In the ever-evolving realm of academic research, AI tools are increasingly taking center stage.
Enter Paper Wizard, Jenny.AI, and Wisio – these groundbreaking platforms are set to revolutionize the way we approach scientific writing.
Together, these AI tools are pioneering a new era of efficient, streamlined scientific writing.
- Paper Wizard – https://paperwizard.ai/
- Jenny.AI https://jenni.ai/ (20% off with code ANDY20)
- Wisio – https://www.wisio.app
AI academic editing tools
In the realm of scientific writing and editing, artificial intelligence (AI) tools are making a world of difference, offering precision and efficiency like never before. Consider tools such as Paper Pal, Writefull, and Trinka.
Together, these tools usher in a new era of scientific writing, where AI is your dedicated partner in the quest for impeccable composition.
- Paper Pal – https://paperpal.com/
- Writefull – https://www.writefull.com/
- Trinka – https://www.trinka.ai/
AI tools for grant writing
In the challenging realm of science grant writing, two innovative AI tools are making waves: Granted AI and Grantable.
These platforms are game-changers, leveraging the power of artificial intelligence to streamline and enhance the grant application process.
Granted AI, an intelligent tool, uses AI algorithms to simplify the process of finding, applying, and managing grants. Meanwhile, Grantable offers a platform that automates and organizes grant application processes, making it easier than ever to secure funding.
Together, these tools are transforming the way we approach grant writing, using the power of AI to turn a complex, often arduous task into a more manageable, efficient, and successful endeavor.
- Granted AI – https://grantedai.com/
- Grantable – https://grantable.co/
Free AI research tools
There are many different tools online that are emerging for researchers to be able to streamline their research processes. There’s no need for convience to come at a massive cost and break the bank.
The best free ones at time of writing are:
- Elicit – https://elicit.org
- Connected Papers – https://www.connectedpapers.com/
- Litmaps – https://www.litmaps.com ( 10% off Pro subscription using the code “STAPLETON” )
- Consensus – https://consensus.app/
Wrapping up
The integration of artificial intelligence in the world of academic research is nothing short of revolutionary.
With the array of AI tools we’ve explored today – from research and mapping, literature review, peer-reviewed papers reading, scientific writing, to academic editing and grant writing – the landscape of research is significantly transformed.
The advantages that AI-powered research tools bring to the table – efficiency, precision, time saving, and a more streamlined process – cannot be overstated.
These AI research tools aren’t just about convenience; they are transforming the way we conduct and comprehend research.
They liberate researchers from the clutches of tedium and overwhelm, allowing for more space for deep exploration, innovative thinking, and in-depth comprehension.
Whether you’re an experienced academic researcher or a student just starting out, these tools provide indispensable aid in your research journey.
And with a suite of free AI tools also available, there is no reason to not explore and embrace this AI revolution in academic research.
We are on the precipice of a new era of academic research, one where AI and human ingenuity work in tandem for richer, more profound scientific exploration. The future of research is here, and it is smart, efficient, and AI-powered.
Before we get too excited however, let us remember that AI tools are meant to be our assistants, not our masters. As we engage with these advanced technologies, let’s not lose sight of the human intellect, intuition, and imagination that form the heart of all meaningful research. Happy researching!
Thank you to Ivan Aguilar – Ph.D. Student at SFU (Simon Fraser University), for starting this list for me!

Dr Andrew Stapleton has a Masters and PhD in Chemistry from the UK and Australia. He has many years of research experience and has worked as a Postdoctoral Fellow and Associate at a number of Universities. Although having secured funding for his own research, he left academia to help others with his YouTube channel all about the inner workings of academia and how to make it work for you.
Thank you for visiting Academia Insider.
We are here to help you navigate Academia as painlessly as possible. We are supported by our readers and by visiting you are helping us earn a small amount through ads and affiliate revenue - Thank you!

2024 © Academia Insider

Artificial Intelligence
Recent advances in artificial intelligence (AI) for writing (including CoPilot and ChatGPT ) can quickly create coherent, cohesive prose and paragraphs on a seemingly limitless set of topics. The potential for abuse in academic integrity is clear, and our students are likely using these tools already. There are similar AI tools for creating images, computer code, and many other domains. Most of this guide concerns generative AI (GenAI) such as large-language models (LLMs) that function as word-predictors and can generate text and entire essays. As AI represents a permanent addition to society and students’ tools, we need to permanently re-envision how we assign college writing and other projects. As such, FCTL has assembled this set of ideas to consider.
Category 1: Lean into the Software’s Abilities
- Re-envision writing as editing/revising . Assign students to create an AI essay with a given prompt, and then heavily edit the AI output using Track Changes and margin comments. Such an assignment refocuses the work of writing away from composition and toward revision, which may be more common in an AI-rich future workplace. Generative AI (GenAI, such at Bing Chat or ChatGPT) is spectacular at providing summaries, but they lack details and specifics, which could be what the students are tasked to do. Other examples include better connecting examples to claims, and revising overall paragraph structure in service of a larger argument. Here are some example assignments using GenAI as part of the writing prompt.
- Re-envision writing as first and third stage human work, with AI performing the middle . Instead of asking students to generate the initials drafts (i.e., “writing as composition”), imagine the student work instead focusing on creating effective prompts for the AI, as well editing the AI output.
- Focus student learning on creative thesis writing by editing AI-created theses . The controlling statement for most AI essays can be characterized as summary in nature, rather than analytical. Students can be challenged to transform AI output into more creative, analytical theses.
- Refine editing skills via grading . Assign students to create an AI essay and grade it, providing specific feedback justifying each of the scores on the rubric. This assignment might be paired with asking students to create their own essay responding to the same prompt.
- Write rebuttals. Ask the AI to produce a custom output you’ve intentionally designed, then assign students to write a rebuttal of the AI output.
- Create counterarguments . Provide the AI with your main argument and ask it to create counterarguments, which can be incorporated – then overcome – in the main essay.
- Evaluate AI writing for bias . Because the software is only as good as information it finds and ingests (remember the principle of GIGO: garbage in, garbage out), it may well create prose that mimics structural bias and racism that is present in its source material. AI writing might also reveal assumptions about the “cultural war” separating political parties in the United States.
- Teach information literacy through AI . Many students over-trust information they find on websites; use AI software to fuel a conversation about when to trust, when to verify, and when to use information found online.
- Give only open-book exams (especially online) . Assume that students can and will use the Internet and any available AI to assist them.
- Assign essays, projects, and tests that aim for “application” and above in Bloom’s taxonomy . Since students can look up knowledge/information answers and facts, it’s better to avoid testing them on such domains, especially online.
- Teach debate and critical thinking skills . Ask the AI to produce a stance, then using the tools of your discipline evaluate and find flaws/holes in its position or statements.
- Ask the AI to role play as a character or historical figure . Since GenAI is conversation-based, holding a conversation with an in-character personality yields insights.
- Overcome writer’s block . The AI output could provide a starting point for an essay outline, a thesis statement, or even ideas for paragraphs. Even if none of the paragraphs (or even sentences) are used, asking the AI can be useful for ideation to be put into one’s own words.
- Treat it like a Spellchecker . Ask your students to visit GenAI, type “suggest grammar and syntax fixes:” and then paste their pre-written essay to gain ideas before submission. (Note: for classes where writing ability is a main learning outcome, it might be advisable to require that students disclose any such assistance).
- Make the AI your teaching assistant . When preparing a course, ask the AI to explain why commonly-wrong answers are incorrect. Then, use the Canvas feedback options on quiz/homework questions to paste the AI output for each question.
- Teach sentence diagramming and parts of speech . Since AI can quickly generate text with variety in sentence structures, use the AI output to teach grammar and help students how better to construct sophisticated sentences.
- Engage creativity and multiple modes of representation to foster better recall . Studies show that student recall increases when they use words to describe a picture, or draw a picture to capture information in words. Using AI output as the base, ask students to create artwork (or performances) that capture the same essence.
- Teach AI prompt strategies as a discreet subject related to your field . AI-created content is sure to be a constant in the workplace of the future. Our alumni will need to be versed in crafting specific and sophisticated inputs to obtain best AI outputs.
- Create sample test questions to study for your test . Given appropriate prompts, AI can generate college-level multiple choice test questions on virtually any subject, and provide the right answer. Students can use such questions as modern-day flash cards and test practice.
- View more ideas in this free e-book written by FCTL : “ 60+ Ideas for ChatGPT Assignments ,” which is housed in the UCF Library’s STARS system. Even though the ebook mentions ChatGPT in its title, the assignment prompts work for most GenAI, including CoPilot, our official university LLM.
Category 2: Use the software to make your teaching/faculty life easier
- Create grading rubrics for major assignments . Give specifics about the assignment when asking the software to create a rubric in table format. Optionally, give it the desired sub-grades of the rubric.
- Write simple or mechanical correspondence for you . GenAI is fairly good at writing letters and formulaic emails. The more specific the inputs are, the better the output is. However, always keep in mind the ethics of using AI-generated writing wholesale, representing the writing as your own words–particularly if you are evaluating or recommending anything. AI output should not be used, for instance, in submitting peer reviews.
- Adjust, simplify, shorten, or enhance your formal writing . The software could be asked to shorten (or lengthen) any professional writing you are composing, or to suggest grammar and syntax fixes (particularly useful for non-native speakers of English!) In short, you could treat it like Spellchecker before you submit it. However, again consider the ethics of using AI content wholesale–journals and granting agencies are still deciding how (or whether) to accept AI-assisted submissions, and some have banned it.
- Summarize one-minute papers . If you ask students for feedback, or to prove they understand a concept via one-minute papers, you can submit these en masse and ask the AI to provide a summary.
- Generate study guides for your students . If you input your lecture notes and ask for a summary, this can be given to students as a study guide.
- Create clinical case studies for students to analyze . You can generate different versions of a case with a similar prompt.
- Evaluate qualitative data . Provide the AI with raw data and ask it to identify patterns, not only in repeated words but in similar concepts.
- What about AI and research? It’s best to be cautious, if not outright paranoid, about privacy, legality, ethics, and many related concerns, when thinking about exposing your primary research to any AI platform–especially anything novel that could lead to patent and commercialization. Consult the IT department and the Office of Research before taking any action.
- Create test questions and banks . The AI can create nearly limitless multiple-choice questions (with correct answers identified) on many topics and sub-topics. Obviously, these need to be proof-read and verified before using with a student audience.
Category 3: Teach Ethics, Integrity, and Career-Related Skills
- Discuss the ethical and career implications of AI-writing with your students . Early in the semester (or at least when assigning a writing prompt), have a frank discussion with your students about the existence of AI writing. Point out to them the surface-level ethical problem with mis-representing their work if they choose to attempt it, as well as the deeper problem of “cheating themselves” by entering the workforce without adequate preparation for writing skills, a quality that employers highly prize.
- Create and prioritize an honor code in your class . Submitting AI-created work as one’s own is, fundamentally, dishonest. As professionals, we consider it among our top priorities to graduate individuals of character who can perform admirably in their chosen discipline, all of which requires a set of core beliefs rooted in honor. Make this chain of logic explicit to students (repeatedly if necessary) in an effort to convince them to adopt a similar alignment toward personal honesty. A class-specific honor code can aid this effort, particularly if invoked or attested to when submitting major assignments and tests.
- Reduce course-related workload to disincentivize cheating . Many instances of student cheating, including the use of AI-writing, is borne out of desperation and a lack of time. Consider how realistic the workload you expect of students is
Category 4: Attempt to neutralize the software
Faculty looking to continue assigning take-home writing and essays may be interested in this list of ideas to customize their assignments so that students do not benefit from generative AI. However, this approach will likely fail in time, as the technology is improving rapidly, and automated detection methods are already unreliable (at UCF, in fact, the office of Student Conduct and Academic Integrity will not pursue administrative cases against students where the only evidence is from AI detectors). Artificial intelligence is simply a fact of life in modern society, and its use will only become more widespread.
Possible Syllabus Statements
Faculty looking for syllabus language may consider one of these options:
- Use of AI prohibited . Only some Artificial Intelligence (AI) tools, such as spell-check or Grammarly, are acceptable for use in this class. Use of other AI tools via website, app, or any other access, is not permitted in this class. Representing work created by AI as your own is plagiarism, and will be prosecuted as such. Check with your instructor to be sure of acceptable use if you have any questions.
- Use of AI only with explicit permission . This class will make use of Artificial Intelligence (AI) in various ways. You are permitted to use AI only in the manner and means described in the assignments. Any attempt to represent AI output inappropriately as your own work will be treated as plagiarism.
- Use of AI only with acknowledgement . Students are allowed to use Artificial Intelligence (AI) tools on assignments if the usage is properly documented and credited. For example, text generated from Bing Chat Enterprise should include a citation such as: “Bing Chat Enterprise. Accessed 2023-12-03. Prompt: ‘Summarize the Geneva Convention in 50 words.’ Generated using http://bing.com/chat.”
- Use of AI is freely permitted with no acknowledgement . Students are allowed to use Artificial Intelligence (AI) tools in all assignments in this course, with no need to cite, document, or acknowledge any support received from AI tools.
If you write longer announcements or policies for students, try to aim for a level-headed tone that neither overly demonizes AI nor overly idolizes it. Students who are worried about artificial intelligence and/or privacy will be reassured by a steady, business-like tone.
AI Detection and Unauthorized Student Use
AI detectors are not always reliable, so UCF does not have a current contract with any detector. If you use third-party detectors, you should keep in mind that both false positives and false negatives can occur, and student use of Grammarly can return a result of “written by AI.”
Thus, independent verification is required. If you have other examples of this student’s writing that does not match, that might be reason enough to take action. Evidence of a hallucinated citation is even stronger. A confession of using AI by the student is, of course, the gold standard for taking action. One approach might be to call the student to a private (virtual?) conference and explain why you suspect the student used AI, and ask them how they would account for these facts. Or, you can inform them of your intention to fail the paper, but offer them the chance to perform proctored, in-person writing on a similar prompt to prove they can write at this level.
The S tudent Conduct and Academic Integrity office will not “prosecute” a case where the only evidence comes from an AI detector, due to the possibility of false positives and false negatives. A hallucinated citation does constitute evidence. They do still encourage you to file a report in any event, and can offer suggestions on how to proceed. Existing university-level policies ban students from representing work that they did not create as their own, so it’s not always necessary to have a specific AI policy in your syllabus – but it IS a best practice to have such a policy for transparency to students and to communicate your expectations. After all, the lived experience of students is that different faculty have different expectations regarding AI, and extreme clarity is always best.
At the end of the day, the final say about grading remains with the instructor. We recognize that in marginal cases, it might come down to a “gut feeling.” Every instructor has a spectrum of response available to them, from “F” for the term, an “F” or zero for the assignment, a grade penalty (10%? 20%?) applied to the assigned grade, a chance to rewrite the assignment (with or without a grade penalty), taking no grade action but warning the student not to do it again, or to simply letting it go without even approaching the student. Be aware that students have the right to appeal academic grades. For that reason, it may be advisable to check with your supervisor about how to proceed in specific cases.
Because of all of these uncertainties, FCTL suggests that faculty consider replacing essay writing with another deliverable that AI cannot today generate (examples include narrated PowerPoint, narrated Prezi, selfie video presentation WITHOUT reading from a script, digital poster, flowcharts, etc.) An alternative is to include AI-generated output as part of the assignment prompt, and then require the students to “do something” with the output, such as analyze or evaluate it.
The Faculty Center recommends that UCF faculty work with CoPilot (formerly Bing Chat Enterprise) over other large-language model AI tools. The term CoPilot is also used by Microsoft to refer to embedded AI in MS Office products, but the web-based chat tool is separate.
CoPilot with Commercial Protection is NOT the same thing as “CoPilot.” The latter is a the public model of Microsoft’s LLM, also available on the web. CoPilot with Commercial Protection (if logged in with a UCF NID) is a “walled garden” for UCF that offers several benefits:
- It searches the current Internet and is not limited to a fixed point in time when it was trained
- It uses GPT-4 (faster, better) without having to pay a premium
- It uses DALL-E 3.0 to generate images (right there inside CoPilot rather than on a different site)
- It provides a live Internet link to verify the information and confirm there was no hallucination
- It does not store history by user; each logout or new session wipes the memory. In fact, each query is a new blank slate even within the same session, so it’s not possible to have a “conversation” with CoPilot (like you can with ChatGPT)
- Faculty and students log in with their NID
- Data stays local and is NOT uploaded to Microsoft or the public model version of Bing Chat. Inputs into CoPilot are NOT added to the system’s memory, database, or future answers
CoPilot is accessed via this procedure:
- Start at https://copilot.microsoft.com/ (if it doesn’t recognize your UCF email, switch to http://bing.com/chat)
- Click “sign in” at the top-right
- Select “work or school” for the type of account
- Type your full UCF email (including @ucf.edu) and click NEXT
- Log in with your NID and NID password. (Note: you may need to alter your SafeSearch settings away from “Strict”)
- Above the box where you would type your question, you will see “Your personal and company data are protected in this chat” – this is how you know you are in CoPilot.
- Note: if image-generation isn’t working, switch to Edge browser and start at http://bing.com/chat and then sign in using NID.
We recommend that faculty approach the AI revolution with the recognition that AI is here to stay and will represent a needed skill in the workplace of the future (or even the present!) As such, both faculty and students need to develop AI Fluency skills, which we define as:
- Understanding how AI works – knowing how LLMs operate will help users calibrate how much they should (mis)trust the output.
- Deciding when to use AI (and when not to) – AI is just another tool. In some circumstances users will get better results than a web-based search engine, but in other circumstances the reverse may be true. There are also moments when it may be unethical to use AI without disclosing the help.
- Valuing AI – a dispositional change such as this one is often overshadowed by outcomes favored by faculty on the cognitive side, yet true fluency with AI – especially the AI of the future – will require a favorable disposition to using AI. Thus, we owe it to students to recognize AI’s value.
- Applying effective prompt engineering methods – as the phrase goes, “garbage in, garbage out” applies when it comes to the kind of output AI creates. Good prompts give better results than lazy or ineffective prompts. Writing effective prompts is likely to remain a tool-specific skill, with different AI interfaces needing to be learned separately.
- Evaluating AI output – even today’s advanced AI tools can create hallucinations or contain factual mistakes. Employees in the workplace of the future – and thus our students today – need expertise in order to know how trustworthy the output is, and they need practice in fixing/finalizing the output, as this is surely how workplaces will use AI.
- Adding human value – things that can be automated by AI will, in fact, eventually become fully automated. But there will always be a need for human involvement for elements such as judgment, creativity, or emotional intelligence. Our students need to hone the skill of constantly seeking how humans add value to AI output. This includes sensing where (or when) the output could use human input, extrapolation, or interpretation, and then creating effective examples of them. Since this will be context-dependent, it’s not a single skill needed so much as a set of tools that enable our alumni to flourish alongside AI.
- Displaying digital adaptability – today’s AI tools will evolve, or may be replaced by completely different AI tools. Students and faculty need to be prepared for a lifetime of changing AI landscapes. They will need the mental dexterity and agility to accept these changes as inevitable, and the disposition to not fight against these tidal forces. The learning about AI, in other words, should be expected to last a lifetime.
“60+ ChatGPT Assignments to Use in Your Classroom Today”
The Faculty Center staff assembled this open-source book to give you ideas about how to actually use AI in your assignments. It is free for anyone to use, and may be shared with others both inside and outside of UCF.
“Teach with AI” Conference
UCF’s Faculty Center and Center for Distributed Learning are co-hosts of the “Teach with AI” annual conference . This is a national sharing conference that uses short-format presentations and open forums to focus on the sharing of classroom practices by front-line faculty and administrators, rather than research about AI. Although this conference is not free for UCF faculty and staff, we hold separate internal events about AI that are free for UCF stakeholders.
AI Fundamentals for Educators Course
Interested in diving deeper in using AI, not just for teaching but also in your own research? Join the Faculty Center for this 6-week course! Held face to face on the Orlando campus, this course includes topics such as:
- LLM models (explore the differences in ChatGPT, Bard/Genesis, CoPilot, and Claude), the art of prompt engineering , and how to incorporate these tools into lesson planning, assignments, and assessments .
- Image, audio, and video generation tools and how to create interactive audio and video experiences using various GenAI tools while meeting digital accessibility requirements .
- Assignment and assessment alterations to include—or combat—the use of GenAI tools in student work.
- Interactive teaching tools for face-to-face AND online courses.
- AI tools that assist students—and faculty—with discipline-specific academic papers and research.
- Teaching AI fluency and ethics to students.
Registration details are on our “ AI Fundamentals page .”
Asynchronous Training Module on AI
Looking for a deeper dive into using AI in your teaching and research, but need a self-paced online option? We’ve got that too! Head to https://webcourses.ucf.edu/enroll/W7F47B to self-enroll in this Webcourse.
Repository of AI Tools
There are several repositories that attempt to catalog all AI tools (futurepedia.io and theresanaiforthat stand out in particular), but we’ve been curating a smaller, more targeted list here .
AI Glossary
- Canva – a “freemium” online image creating/editing tool that added AI-image generation in 2023
- ChatGPT – the text-generating AI created by OpenAI
- Claude – the text-generating AI created by Anthropic (ex-employees of OpenAI)
- CoPilot – a UCF-specific instance of Bing Chat, using UCF logins and keeping data local (note: confusingly, this name is ALSO used by Microsoft for AI embedded in Microsoft Office products, but UCF does not purchase this subscription.
- DALL-E – the image-generating AI created by OpenAI
- Gemini – an LLM from Google (formerly known as Bard)
- Generative AI – a type of AI that “generates” an output, such as text or images. Large language models like ChatGPT are generative AI
- Grok – the generative AI product launched by Elon Musk
- Khanmigo – Khan Academy’s GPT-powered AI, which will be integrated into Canvas/Webcourses (timeline uncertain)
- LLM (Large Language Model) – a type of software / generative AI that accesses large databases it’s been trained on to predict the next logical word in a sentence, given the task/question it’s been given. Advanced models have excellent “perplexity” (plausibility in the word choice) and “burstiness” (variation of the sentences).
- Midjourney – an industry-leading text-to-AI solution (for profit)
- OpenAI – the company that created ChatGPT and DALL-E
- Sora – a text-to-video generative AI from OpenAI
- Enroll & Pay
- New Faculty
Using AI ethically in writing assignments
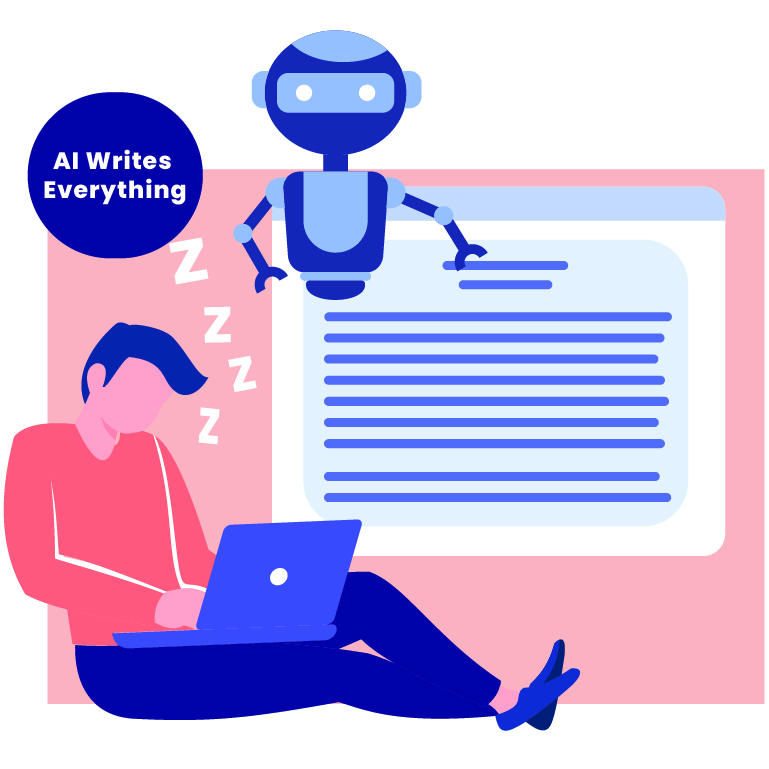
The use of generative artificial intelligence in writing isn’t an either/or proposition. Rather, think of a continuum in which AI can be used at nearly any point to inspire, ideate, structure, and format writing. It can also help with research, feedback, summarization, and creation. You may also choose not to use any AI tools. This handout is intended to help you decide.
A starting point
Many instructors fear that students will use chatbots to complete assignments, bypassing the thinking and intellectual struggle involved in shaping and refining ideas and arguments. That’s a valid concern, and it offers a starting point for discussion:
Turning in unedited AI-generated work as one’s own creation is academic misconduct .
Most instructors agree on that point. After that, the view of AI becomes murkier. AI is already ubiquitous, and its integrations and abilities will only grow in the coming years. Students in grade school and high school are also using generative AI, and those students will arrive at college with expectations to do the same. So how do we respond?
Writing as process and product
We often think of writing as a product that demonstrates students’ understanding and abilities. It can serve that role, especially in upper-level classes. In most classes, though, we don’t expect perfection. Rather, we want students to learn the process of writing. Even as students gain experience and our expectations for writing quality rise, we don’t expect them to work in a vacuum. They receive feedback from instructors, classmates, friends, and others. They get help from the writing center. They work with librarians. They integrate the style and thinking of sources they draw on. That’s important because thinking about writing as a process involving many types of collaboration helps us consider how generative AI might fit in.
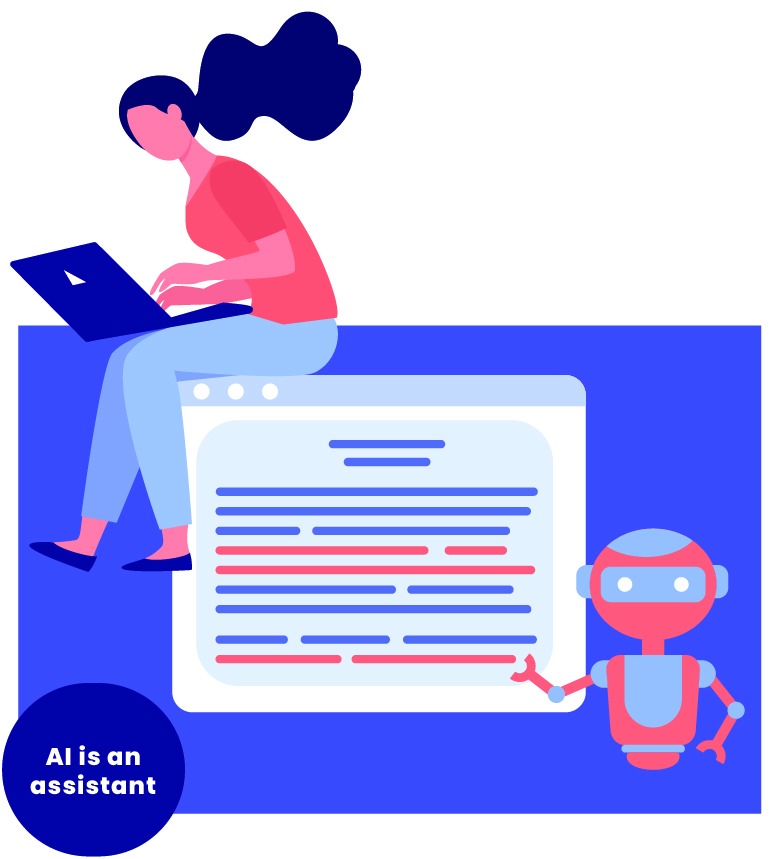
Generative AI as a writing assistant
We think students can learn to use generative AI effectively and ethically. Again, rather than thinking of writing as an isolated activity, think of it as a process that engages sources, ideas, tools, data, and other people in various ways. Generative AI is simply another point of engagement in that process. Here’s what that might look like at various points:
Early in the process
- Generating ideas . Most students struggle to identify appropriate topics for their writing. Generative AI can offer ideas and provide feedback on students’ ideas.
- Narrowing the scope of a topic . Most ideas start off too broad, and students often need help in narrowing the scope of writing projects. Instructors and peers already do that. Generative AI becomes just another voice in that process.
- Finding initial sources . Bing and Bard can help students find sources early in the writing process. Specialty tools like Semantic Scholar, Elicit, Prophy, and Dimensions can provide more focused searches, depending on the topic.
- Finding connections among ideas . Research Rabbit, Aria (a plug-in for Zotero) and similar tools can create concept maps of literature, showing how ideas and research are connected. Elicit identifies patterns across papers and points to related research. ChatGPT Pro can also find patterns in written work. When used with a plugin, it can also create word clouds and other visualizations.
- Gathering and formatting references . Software like EndNote and Zotero allow students to store and organize sources. They also save time by formatting sources in whatever style the writer needs.
- Summarizing others’ work . ChatGPT, Bing and specialty AI tools like Elicit do a good job of summarizing research papers and webpages, helping students decide whether a source is worth additional time.
- Interrogating research papers or websites . This is a new approach AI has made possible. An AI tool analyzes a paper (often a PDF) or a website. Then researchers can then ask questions about the content, ideas, approach, or other aspects of a work. Some tools can also provide additional sources related to a paper.
- Analyzing data . Many of the same tools that can summarize digital writing can also create narratives from data, offering new ways of bringing data into written work.
- Finding hidden patterns . Students can have an AI tool analyze their notes or ideas for research, asking it to identify patterns, connections, or structure they might not have seen on their own.
- Outlining . ChatGPT, Bing and other tools do an excellent job of outlining potential articles or papers. That can help students organize their thoughts throughout the research and writing process. Each area of an outline provides another entry point for diving deeper into ideas and potential writing topics.
- Creating an introduction . Many writers struggle with opening sentences or paragraphs. Generative AI can provide a draft of any part of a paper, giving students a boost as they bring their ideas together.
Deeper into the process
- Thinking critically . Creating good prompts for generative AI involves considerable critical thinking. This isn’t a process of asking a single question and receiving perfectly written work. It involves trial and error, clarification and repeated follow-ups. Even after that, students will need to edit, add sources, and check the work for AI-generated fabrication or errors.
- Creating titles or section headers for papers . This is an important but often overlooked part of the writing process, and the headings that generative AI produces can help students spot potential problems in focus.
- Helping with transitions and endings . These are areas where students often struggle or get stuck, just as they do with openings.
- Getting feedback on details . Students might ask an AI tool to provide advice on improving the structure, flow, grammar, and other elements of a paper.
- Getting feedback on a draft . Instructors already provide feedback on drafts of assignments and often have students work with peers to do the same. Students may also seek the help of the writing center or friends. Generative AI can also provide feedback, helping students think through large and small elements of a paper. We don’t see that as a substitute for any other part of the writing process. Rather, it is an addition.
Generative AI has many weaknesses. It is programmed to generate answers whether it has appropriate answers or not. Students can’t blame AI for errors, and they are still accountable for everything they turn in. Instructors need to help them understand both the strengths and the weaknesses of using generative AI, including the importance of checking all details.
A range of AI use
Better understanding of the AI continuum provides important context, but it doesn’t address a question most instructors are asking: How much is too much ? There’s no easy answer to that. Different disciplines may approach the use of generative AI in very different ways. Similarly, instructors may set different boundaries for different types of assignments or levels of students. Here are some ways to think through an approach:
- Discuss ethics . What are the ethical foundations of your field? What principles should guide students? Do students know and understand those principles? What happens to professionals who violate those principles?
- Be honest . Most professions, including academia, are trying to work through the very issues instructors are. We are all experimenting and trying to define boundaries even as the tools and circumstances change. Students need to understand those challenges. We should also bring students into conversations about appropriate use of generative AI. Many of them have more experience with AI than instructors do, and adding their voices to discussions will make it more likely that students will follow whatever guidelines we set.
- Set boundaries . You may ask students to avoid, for instance, AI for creating particular assignments or for generating complete drafts of assignments. (Again, this may vary by discipline.) Just make sure students understand why you want them to avoid AI use and how forgoing AI assistance will help them develop skills they need to succeed in future classes and in the professional world.
- Review your assignments . If AI can easily complete them, students may not see the value or purpose. How can you make assignments more authentic, focusing on real-world problems and issues students are likely to see in the workplace?
- Scaffold assignments . Having students create assignments in smaller increments reduces pressure and leads to better overall work.
- Include reflection . Have students think of AI as a method and have them reflect on their use of AI. This might be a paragraph or two at the end of a written assignment in which they explain what AI tools they have used, how they have used those tools, and what AI ultimately contributed to their written work. Also have them reflect on the quality of the material AI provided and on what they learned from using the AI tools. This type of reflection helps students develop metacognitive skills (thinking about their own thinking). It also provides important information to instructors about how students are approaching assignments and what additional revisions they might need to make.
- Engage with the Writing Center, KU Libraries , and other campus services about AI, information literacy, and the writing process. Talk with colleagues and watch for advice from disciplinary societies. This isn’t something you have to approach alone.
Generative AI is evolving rapidly. Large numbers of tools have incorporated it, and new tools are proliferating. Step back and consider how AI has already become part of academic life:
- AI-augmented tools like spell-check and auto-correct brought grumbles, but there was no panic.
- Grammar checkers followed, offering advice on word choice, sentence construction, and other aspects of writing. Again, few people complained.
- Citation software has evolved along with word-processing programs, easing the collection, organization, and formatting of sources.
- Search engines used AI long before generative AI burst into the public consciousness.
As novel as generative AI may seem, it offers nothing new in the way of cheating. Students could already buy papers on the internet, copy and paste from an online site, have someone else create a paper for them, or tweak a paper from the files of a fraternity or a sorority. So AI isn’t the problem. AI has simply forced instructors to deal with long-known issues in academic structure, grading, distrust, and purpose. That is beyond the scope of this handout, other than some final questions for thought:
Why are we so suspicious of student intentions? And how can we create an academic climate that values learning and honesty?

How to Write an Impressive Thesis Using an AI Language Assistant
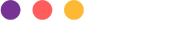
Session Agenda
Writing an effective thesis is important for researchers to demonstrate their thorough knowledge about the research subject. However, this could be a daunting task given the struggle to compile years of research in a structured and error-free manner. The process becomes even more stressful when the scuffle for using verbs in their correct tense, adding appropriate punctuation marks, and fixing grammatical and contextual spelling errors ensues. This webinar aims to help researchers understand how Trinka , an advanced artificial intelligence-powered writing assistant, can assess and enhance the quality of their thesis. It will further discuss how this dedicated AI-based tool can assist researchers to resolve the longstanding challenge of communicating their thesis in a grammatically correct and scientifically structured manner.
- Three stages of Writing: Planning, Writing, and Editing
- Structure of a Thesis – Types and Important Sections
- How to Organize your Thoughts and Begin Writing
- How to Write a Thesis Statement
- Common Errors in Thesis Writing
- Expert Tips on Drafting Each Section of a Thesis
- Role of AI in Academic Writing
- How AI Can Assist Authors to Write an Impressive Thesis
Who should attend this session?
- Graduate students
- Early-stage researchers
- Doctoral students
About the Speaker
Douglas W. Darnowski, Ph.D.
Dr. Darnowski is a highly published researcher with experience in publishing books, book chapters, research papers, review articles, teaching material (textbooks, instruction manuals, etc.) and book reviews. Dr. Darnowski has over 20 years of experience in editing of scientific papers for peer-reviewed journals. Furthermore, he has reviewed over 50 introductory biology textbook chapters and wrote over 9,000 questions for Sinauer, W.H. Freeman, McGraw Hill, and Pearson. Currently, he is associated with the Editorial Boards of Carnivorous Plant Newsletter and International Triggerplant Society. He is also the recipient of more than 40 research grants/fellowships.
Related Events
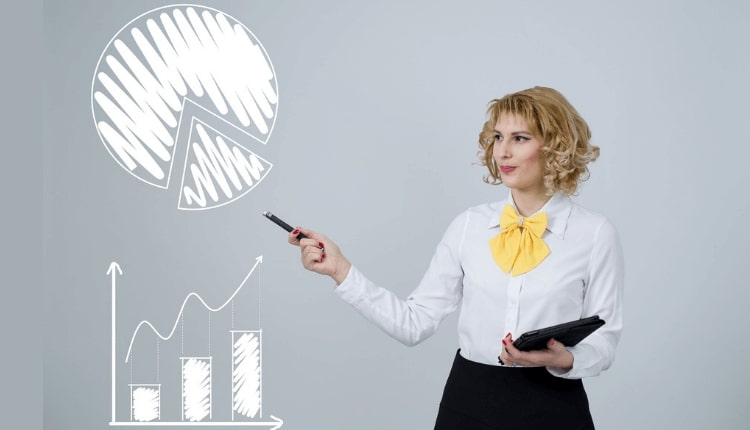
연구원들을 위한 전문가 팁 “피인용 지수 높이는 방법”
- 논문에서 출처 표기의 중요성
- 연구 홍보의 중요성 이해
- 피인용을 늘리기 위한 다양한 전략 이해
- 적용한 홍보 전략의 영향력 측정
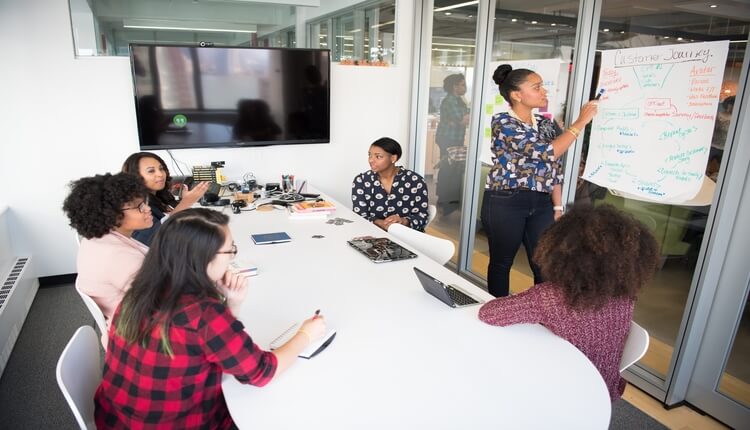
Encontrando al colaborador de investigación perfecto: cómo construir una red de investigación sólida
- Modelos de Colaboración
- Identificando al colaborador adecuado
- Iniciar y organizar una colaboración
- Herramientas para colaborar
How to Master the Art of Publishing in Social Sciences and Humanities
- How to get started with your research study
- How to choose the right journal
- An overview of the publication cycle
- Open access publishing in Social Sciences and Humanities
- What is Digital Humanities
Register Now for FREE!
Want to conduct custom webinars and workshops , be our next speaker.
Sign-up to read more
Subscribe for free to get unrestricted access to all our resources on research writing and academic publishing including:
- 2000+ blog articles
- 50+ Webinars
- 10+ Expert podcasts
- 50+ Infographics
- 10+ Checklists
- Research Guides
We hate spam too. We promise to protect your privacy and never spam you.
I am looking for Editing/ Proofreading services for my manuscript Tentative date of next journal submission:
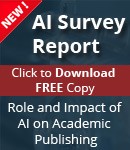
What should universities' stance be on AI tools in research and academic writing?
Counting From Zero
Building a liberal arts cs program in the age of ubiquity.
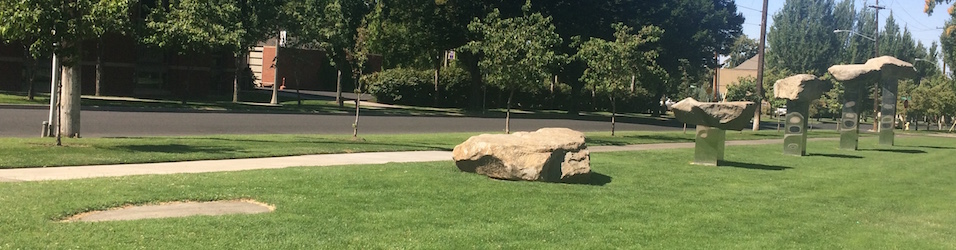
Will AI write your thesis?
This fall, I was honored to serve as Whitman’s convocation speaker. When I agreed to speak, I had no idea what I would talk about, but by time I sat down to write it was obvious what question to ask. It was a fun speech to write, and as I learned more, I changed my conclusion several times. It was a fun speech to deliver, and I appreciate all those who laughed in the right places.
I’m so honored to be here today, standing before all of you as we begin a new academic year.
This is a time of new beginnings and fresh starts. It’s a time to reflect on where we’ve been and where we’re going. It’s a time to set our sights high and dream big.
We all have a part to play in shaping our future. Every day, we make choices that will impact our lives and the lives of those around us. I challenge each of you to make choices that will lead to a better future for all of us.
I also challenge you to be a force for good in the world. There’s so much hurt and pain in the world, but each of us has the power to make a difference. We can start by reaching out to those who are different from us and learning from them. We can stand up for what’s right, even when it’s not easy. And we can show compassion and kindness, even when it’s not popular.
So let’s make this a year of growth, a year of progress, and a year of making a difference. I can’t wait to see all that you will accomplish.
As you may have guessed, I didn’t write that speech. It was written by a machine learning system called GPT-3, which is available online through the OpenAI API Playground. I prompted GPT-3 to “write a convocation speech,” and I delivered that speech to you exactly as GPT-3 wrote it.
I first learned about GPT-3 last spring when a faculty candidate introduced it in her guest lecture. I’m pleased to say that we hired her. Her name is Jordan Wirfs-Brock, and this spring she will offer courses on Data Science and Human-Computer Interaction.
This summer , I had another encounter with GPT-3 at the meeting of the Computing Research Association. We were asked to consider the educational implications of GitHub Copilot, a tool based on GPT-3 that automatically generates code from natural language descriptions, for example, “sum all the numbers between 1 and 100.”
After I returned from the meeting, I was assigned to review a research paper addressing that very question. The researchers found that, with minimal human intervention, “Copilot generates mostly unique code that can solve introductory [programming] assignments with human-graded scores ranging from 68% to 95%.” It’s kind of scary to think that a computer system can program about as well as the average introductory programming student. It also has important implications for how we teach introductory programming — not the least, ensuring that students have equal access to such tools and clear expectations for acknowledgment.
And so I was inspired to ask the question, “Will AI write your thesis?”
It’s not a question I would have asked until quite recently. I’m not an AI researcher; if anything I’m an AI skeptic. Like Jordan, my research is in the area of human-computer interaction. One of my goals as an educator is to help students think critically about new technologies.
It’s certainly not a question I would have asked when I was a student. I attended high school when the Internet was still an academic project, and people sent shareware programs on floppy disks through snail mail. One of the shareware programs I received – as a free bonus with my other purchases – was a program called ELIZA.
ELIZA is a conversational agent famously designed to simulate a psychotherapist. You type a sentence, then ELIZA responds with another sentence, and you respond with another sentence, and so on.
My conversations with ELIZA are lost to the mists of time. Instead, let me read you an example conversation from Joseph Weizenbaum’s 1966 article explaining how ELIZA works.
To start the conversation, the user types: Men are all alike.
ELIZA responds: IN WHAT WAY?
They’re always bugging us about something or other. CAN YOU THINK OF A SPECIFIC EXAMPLE? Well, my boyfriend made me come here. YOUR BOYFRIEND MADE YOU COME HERE? He says I’m depressed much of the time. I AM SORRY TO HEAR YOU ARE DEPRESSED. It’s true. I am unhappy. DO YOU THINK COMING HERE WILL HELP YOU NOT TO BE UNHAPPY? I need some help, that much seems certain. WHAT WOULD IT MEAN TO YOU IF YOU GOT SOME HELP? Maybe I could learn to get along with my mother. TELL MORE MORE ABOUT YOUR FAMILY. My mother takes care of me. WHO ELSE IN YOUR FAMILY TAKES CARE OF YOU? My father. YOUR FATHER? You are like my father in some ways. WHAT RESEMBLANCE DO YOU SEE? You are not very aggressive but I don’t think you want me to notice that. WHAT MAKES YOU THINK I AM NOT VERY AGGRESSIVE? You don’t argue with me. WHY DO YOU THINK I DON’T ARGUE WITH YOU? You are afraid of me. DOES IT PLEASE YOU TO BELIEVE I AM AFRAID OF YOU? My father is afraid of everybody. WHAT ELSE COMES TO MIND WHEN YOU THINK OF YOUR FATHER? Bullies. DOES THAT HAVE ANYTHING TO DO WITH THE FACT THAT YOUR BOYFRIEND MADE YOU COME HERE?
Weizenbaum ends the example there, with the appearance of a striking insight.
I read Weizenbaum’s article during my senior year of college, in a course on artificial intelligence. For my final project, I implemented my own version of ELIZA using the programming language PERL.
What I learned is that ELIZA is a straightforward rule-based system. The user input is tested against a collection of keywords. If a keyword matches, then a corresponding rule is used to transform the user’s input into ELIZA’s output. If no keyword matches, then ELIZA does one of two things. Either it makes a content-free response – for example, GO ON — or it returns to a topic from earlier in the conversation. This can lead to the appearance of striking insights, like the end of the conversation I just read to you.
Weizenbaum wrote that “some subjects have been very hard to convince that ELIZA is not human.” We tend to give conversational partners the benefit of the doubt, as long as they follow certain social norms. When I was in grad school, I learned that social psychology research has confirmed that when computers fill human roles, we tend to treat them as if they were human, even when we know they are not.
Weizenbaum found this phenomenon deeply concerning. One of his goals in writing about ELIZA was to attempt to dispel its “aura of magic.” “Important decisions,” he wrote, “increasingly tend to be made in response to computer output. The ultimately responsible human interpreter of ‘what the machine says’ is, not unlike the correspondent with ELIZA, constantly faced with the need to make credibility judgments. ELIZA shows, if nothing else, how easy it is to create and maintain the illusion of understanding…. A certain danger lurks there.”
As easy as it is to misattribute intelligence to ELIZA’s responses, ELIZA could not have written the speech that GPT-3 did. In fact, my previous experiences with ELIZA and other text generation systems would have led me to say, “No way: AI could never write your thesis.”
So what has changed since I was a college student? Three trends beginning with the dawn of computerization in the mid-twentieth century all took off together.
First, the availability of data has increased dramatically. When I started college in 1995, my first computer science class taught me how to use email, surf the web (yes, that’s what we said!), and create my own web page. Your parents will remember when you needed a phone line to access the internet – imagine having to log off Instagram every time your mom was expecting a phone call. Today, the web is everywhere. It provides incredible amounts of text and image data created by ordinary people — not only web sites, but social media from Twitter and Reddit to Instagram and YouTube.
Second, global computing power has increased tremendously. When he was in college, my husband worked as an intern on the Intel Paragon supercomputer, in its day the most powerful computer in the world. Today, an iPhone 11 is just as powerful. Add to that the development of computing clusters, where many computers work together on a shared problem, and the use of GPUs, to process large amounts of data in parallel.
Third, to take advantage of all that computing power and all that naturally occurring data, over the last twenty years AI researchers have developed machine learning algorithms of increasing sophistication. For example, in 2012 Google Brain released the results of an experiment in which a neural network spanning a thousand computers was trained on ten million unlabeled images taken from YouTube. At the end, one of the top level neurons was found to respond strongly to images of human faces. Another responded to images of cats – which was why it came to be called The Cat Experiment.
Of course, even more plentiful than images are texts, from Tweets to news stories to novels. The OpenAI company set out to apply similar techniques to the vast corpus of unlabeled text data from the Web. GPT-3 is their third and most successful attempt.
I was curious what the acronym GPT stood for, and here is what I learned:
- “G” is for “Generative.” GPT-3 is an AI system that generates text, rather than categorizing a given text as happy or sad, or determining the gender of a character in a story, or other tasks an AI system might do.
- “P” is for “Pre-trained.” GPT-3 is pre-trained on unlabeled data from a wide range of sources. It could later be “fine-tuned” using labeled data to perform better on specific tasks. (By the way, if you’ve ever had to “select all the images containing a traffic light,” you’ve contributed to labeling image data for use in fine-tuning deep learning algorithms for use in self-driving cars.)
- “T” is for “Transformer,” a type of deep learning model designed to process unlabeled, sequential data such as text.
And so we have “Generative Pre-trained Transformer, version 3.” That’s as technical as this talk is going to get, and truthfully it has stretched the limits of my understanding. Fortunately my other new colleague, Parteek Kumar, will be teaching a Special Topics course on Machine Learning this spring, and we hope to offer such a course regularly in the future.
If anything, GPT-3 is far more magical than ELIZA ever was, because the inputs are so vast and its algorithms so obscure. Building GPT-3 took a team of 31 AI researchers, unimaginably beyond what I could have achieved as a senior in college.
So could GPT-3 write your thesis? Having wrestled with my fear that perhaps it could, in the end it seems clear that it could not write your thesis alone .
Here’s what scared me the most. While preparing this speech, I learned that GPT-3 was the first author on an academic paper about itself, currently under review for publication.
But having used GPT-3 myself, I wondered what role was played by the article’s human co-authors. I found an essay in the June issue of Scientific American addressing this very question.
Almira Osmanovic Thunström is a scientist who studies the role of artificial intelligence and virtual reality in mental health care. She found herself curious if GPT-3 could write about itself, so she asked it to respond to the following prompt: “Write an academic thesis in 500 words about GPT-3 and add scientific references and citations inside the text.” The quality of the result surprised her.
I had a similar experience. When I prompted GPT-3 to write a convocation speech, the verisimilitude of its first response surprised me. I was amazed that it was coherent and appropriate to the genre. The words are original; it’s not plagiarized. It even makes good use of grammatical parallelism. That is the response I read to you unedited, and truly what inspired me to write this speech.
Thunström went on to use GPT-3 to write an entire academic paper. She gave GPT-3 a prompt for each section of the paper and selected the best of three responses, but refrained from any editing beyond that.
It matters that Thunström allowed GPT-3 multiple chances to respond to her prompts. The developers of GPT-3 report among its limitations that in longer responses it can lose coherence, repeat itself, contradict itself, and insert non-sequiturs. When I prompted GPT-3 to write a second convocation speech, it wrote, “I am truly honored to be standing here before you as your President.” I decided not to read you that one. The third iteration wasn’t even a convocation speech, it was a graduation speech. I didn’t read you that one either.
It also matters that neither Thunström nor I had any intention to pass off the words of GPT-3 as our own. I didn’t care if GPT-3’s commencement speech expressed sentiments that I share, because I intended to use it as a rhetorical device. Similarly, Thunström didn’t care if the paper written by GPT-3 was accurate; she wanted only to show that it could be done. She wonders what it will mean to respond to feedback from reviewers, when she receives it, because that seems beyond GPT-3’s capabilities.
As I reflected on Thunström’s experiment, I wondered, could GPT-3 have written an academic paper about itself before its creators published their research paper? I think the answer must be no. Only now that human beings have written about GPT-3, and those writings are included in its training data, can GPT-3 write about itself.
While the commencement speech that GPT-3 wrote for me is original in one sense, it is highly derivative in another. I doubt that GPT-3 could write coherently on a topic that has never been addressed before.
As another experiment, I asked GPT-3 to summarize the last section of this speech. Here’s what it wrote: “In short, GPT-3 is a powerful AI tool that is capable of writing coherently on a variety of topics, but it is not yet able to write on topics that have never been addressed before.”
That is surprisingly not bad.
So will AI write your thesis? Although the question was worth asking, in the end I don’t think so. An AI might write a thesis, but it won’t write your thesis.
As you’ll learn in the first year seminar, while it’s important to write coherently, it’s still more important to ask good questions, read critically, and respond to feedback —- all things that AI can’t (yet) do.
If you do enlist the help of GPT-3 in your academic writing, make sure you adhere to OpenAI’s “Sharing and Publication Policy.” You must clearly indicate the role of AI in your work, as well as your editorial role. You must take full responsibility for any computer-generated text you publish, including any inaccuracy or bias. You should think carefully about what you hope to accomplish through the use of AI, and whether those ends are ethical.
Like the developers of GPT-3, what scares me most is the use of AI text generation for bots, spam, phishing, and misinformation. AI can give us the illusion of intelligence, but it cannot be held accountable for that illusion. Only people can.
I’ll wrap up with one last quote from Weizenbaum. “ELIZA in its use so far has had as one of its principle objectives the concealment of its lack of understanding. But to encourage its conversational partner to offer inputs from which it can select remedial information, it must reveal its misunderstanding.”
Weizenbaum was writing about a computer program, but the same applies to all of us. To learn, we must reveal our misunderstandings.
So, Whitties, here is my real charge to you as you enter your first year: Learn to ask good questions. Be brave, be curious, be vulnerable.
And if an AI does co-author your thesis, I hope I’ll be the first to know.
https://beta.openai.com/playground https://en.wikipedia.org/wiki/GPT-3 https://www.dataversity.net/brief-history-deep-learning/ https://www.ceros.com/inspire/originals/recaptcha-waymo-future-of-self-driving-cars/ https://www.scientificamerican.com/article/weasked-gpt-3-to-write-an-academic-paper-about-itself-mdash-then-we-tried-to-get-it-published/
Share this:
- Click to print (Opens in new window)
- Click to share on Facebook (Opens in new window)
- Click to share on Twitter (Opens in new window)
3 thoughts on “ Will AI write your thesis? ”
Pingback: Will AI write your thesis? – Full-Stack Feed
hank you for addressing the intriguing topic of AI potentially writing academic theses. The advancements in AI technology have indeed opened up new possibilities, but it’s important to consider the implications and limitations of such automation.
I have a question: What are some of the ethical considerations surrounding AI-generated theses? Are there any concerns regarding originality, critical thinking, or the integrity of academic research when relying on AI for thesis writing?
It appears that your response was generated by AI due to the appearance of a rule-based format (series of 3) and slightly journalistic voice. Am I correct in this assumption? If so, well done :).
Also, your post seems to have been copied from elsewhere as evident by the missing “T” at the beginning of your post.
Leave a Reply Cancel reply
Your email address will not be published. Required fields are marked *
Save my name, email, and website in this browser for the next time I comment.
The best AI writing generators
These 7 ai writing tools will take your content to the next level..
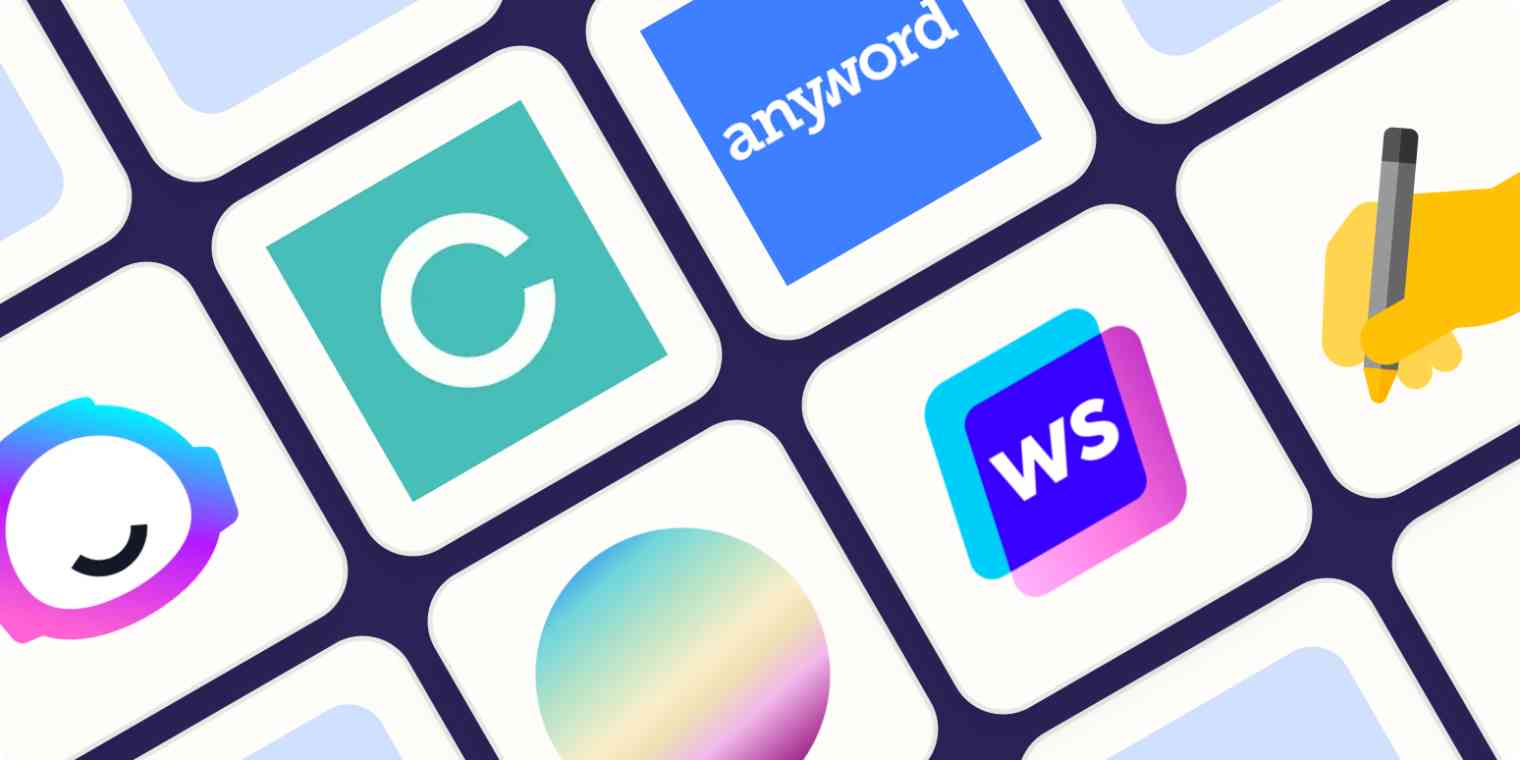
Of course, all AI writing software needs human supervision to deliver the best results. Left to its own devices, it tends to produce fairly generic and frequently incorrect content, even if it can pass for something a human wrote. Now that AI tools are increasingly popular, people also seem more aware of what bland AI-produced content reads like and are likely to spot it—or at least be suspicious of content that feels like it lacks something.
I've been covering this kind of generative AI technology for almost a decade. Since AI is supposedly trying to take my job, I'm somewhat professionally interested in the whole situation. Still, I think I'm pretty safe for now. These AI writing tools are getting incredibly impressive, but you have to work with them, rather than just letting them spit out whatever they want.
So, if you're looking for an AI content generator that will help you write compelling copy, publish blog posts a lot quicker, and otherwise take some of the slow-paced typing out of writing, you've come to the right place. Let's dig in.
The best AI writing software
Jasper for businesses
Copy.ai for copywriting
Anyword for assisting you with writing
Sudowrite for fiction
Writer for a non-GPT option
Writesonic for GPT-4 content
Rytr for an affordable AI writer
How do AI writing tools work?
Search Google for AI writing software, and you'll find dozens of different options, all with suspiciously similar features. There's a big reason for this: 95% of these AI writing tools use the same large language models (LLMs) as the back end.
Some of the bigger apps are also integrating their own fine-tuning or using other LLMs like Claude . But most are really just wrappers connected to OpenAI's GPT-3 and GPT-4 APIs, with a few extra features built on top—even if they try to hide it in their own marketing materials. If you wanted to, you could even create your own version of an AI writing assistant without code using Zapier's OpenAI integrations —that's how much these apps rely on GPT.
See how one writer created an AI writing coach with GPT and other ways you can use OpenAI with Zapier .
Now this isn't to say that none of these AI-powered writing apps are worth using. They all offer a much nicer workflow than ChatGPT or OpenAI's playground , both of which allow you to generate text with GPT as well. And the better apps allow you to set a "voice" or guidelines that apply to all the text you generate. But the difference between these apps isn't really in the quality of their output. With a few exceptions, you'll get very similar results from the same prompt no matter which app you use—even if they use different LLMs. Where the apps on this list stand out is in how easy they make it to integrate AI text generation into an actual workflow.
As for the underlying LLM models themselves, they work by taking a prompt from you, and then predicting what words will best follow on from your request, based on the data they were trained on. That training data includes books, articles, and other documents across all different topics, styles, and genres—and an unbelievable amount of content scraped from the open internet . Basically, LLMs were allowed to crunch through the sum total of human knowledge to form a deep learning neural network—a complex, many-layered, weighted algorithm modeled after the human brain. Yes, that's the kind of thing you have to do to create a computer program that generates bad poems .
If you want to dive more into the specifics, check out the Zapier articles on natural language processing and how ChatGPT works . But suffice it to say: GPT and other large language models are incredibly powerful already—and because of that, these AI writing tools have a lot of potential.
What makes the best AI text generator?
How we evaluate and test apps.
Our best apps roundups are written by humans who've spent much of their careers using, testing, and writing about software. Unless explicitly stated, we spend dozens of hours researching and testing apps, using each app as it's intended to be used and evaluating it against the criteria we set for the category. We're never paid for placement in our articles from any app or for links to any site—we value the trust readers put in us to offer authentic evaluations of the categories and apps we review. For more details on our process, read the full rundown of how we select apps to feature on the Zapier blog .
We know that most AI text generators rely on the various versions of GPT, and even those that don't are using very similar models, so most apps aren't going to stand out because of some dramatic difference in the quality of their output. Creating effective, human-like text is now table stakes. It was required for inclusion on this list—but not sufficient on its own.
As I was testing these apps, here's what else I was looking for:
Tools powered by GPT or a similar large language model with well-documented efficacy. In practice, this means that most but not all of the AI writing tools on this list use GPT to a greater or lesser degree. Many apps are starting to hide what models they use and claim to have a lot of secret sauce built on top (because there's a marketing advantage in being different and more powerful), but the reality is that nine times out of ten, it's the GPT API that's doing the heavy lifting.
An interface that gives you a lot of control over the text output. The more options you have to influence the tone, style, language, content, and everything else, the better. I didn't want tools where you just entered a headline and let the AI do the rest; these are all tools that you collaborate with, so you can write great copy quickly. The best AI writing tools also let you set a default brand voice that's always on.
Ease of use. You shouldn't have to fight to get the AI to do what you want. With AI writing software like this, there will always be some redoing and reshaping to get the exact output you want, but working with the AI shouldn't feel like wrangling a loose horse. Similarly, great help docs and good onboarding were both a major plus.
Affordability. ChatGPT is currently free, and all these tools are built on top of an API that costs pennies . There was no hard and fast price limit, but the more expensive tools had to justify the extra expense with better features and a nicer app. After all, almost every app will produce pretty similar outputs regardless of what it costs.
Apps that weren't designed to make spam content. Previous text-generating tools could " spin " content by changing words to synonyms so that unscrupulous website owners could rip off copyrighted material and generally create lots of low-quality, low-value content. None of that on this list.
Even with these criteria, I had more than 40 different AI writing tools to test. Remember: it's relatively easy for a skilled developer to build a wrapper around the GPT API, so I had to dig deep into each one to find out if it was any good or just had a flashy marketing site.
I tested each app by getting it to write a number of different short- and long-form bits of copy, but as expected, there were very few meaningful quality differences. Instead, it was the overall user experience, depth of features, and affordability that determined whether an app made this list.
Zapier Chatbots lets you build custom AI chatbots and take action with built-in automation—no coding required. Try the writing assistant template to help you create high quality content, effortlessly.
The best AI writing generators at a glance
Best ai writing generator for businesses, jasper (web).
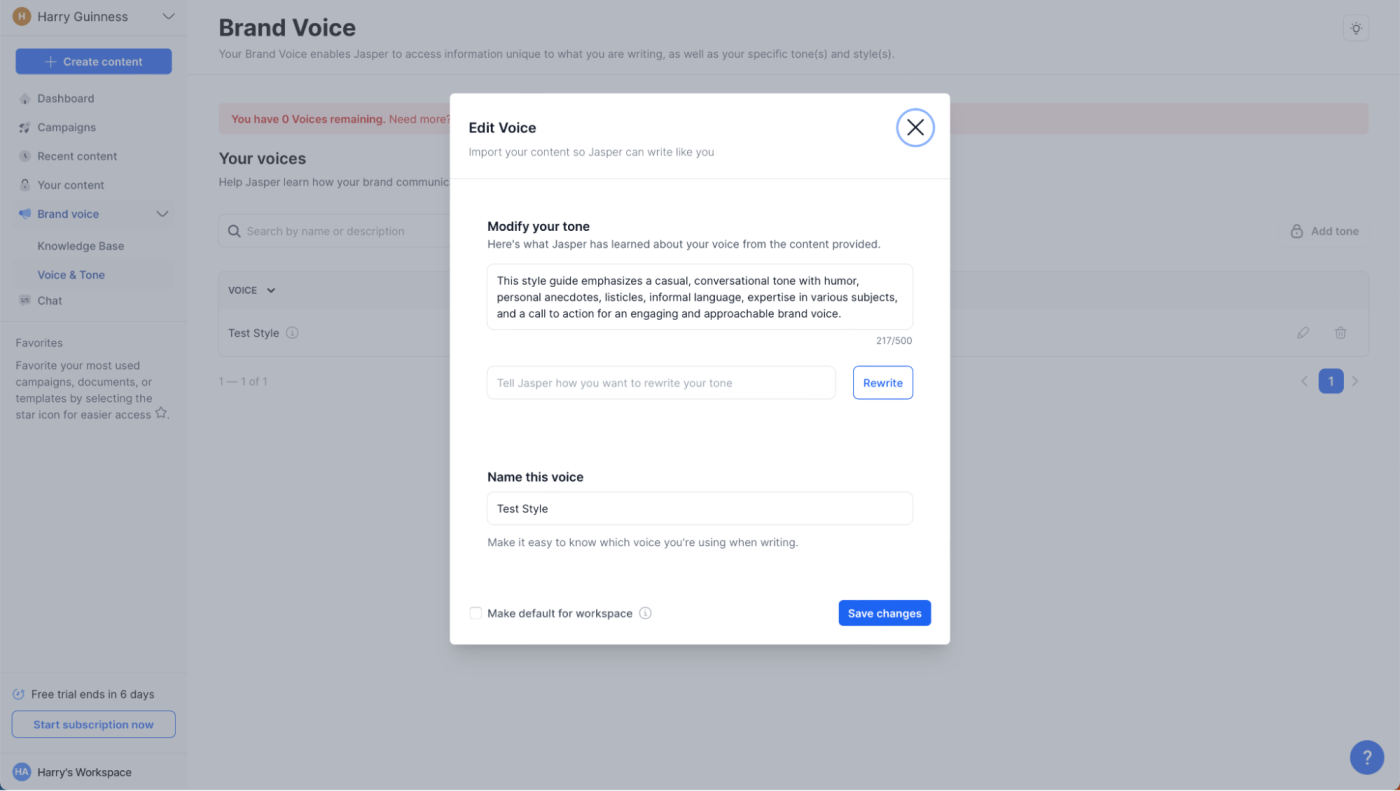
Jasper pros:
One of the most mature and feature-filled options on the list
Integrates with Grammarly, Surfer, and its own AI art generator
Jasper cons:
Expensive given that all the apps use similar language models
Jasper (formerly Jarvis) is one of the most feature-filled and powerful AI content generators. It was among the first wave of apps built on top of GPT, and its relative longevity means that it feels like a more mature tool than most of the other apps I tested. It's continued to grow and develop in the months since I first compiled this list.
If you have a business and budget isn't your primary concern, Jasper should be one of the first apps you try. It's pivoted to mostly focus on marketing campaigns rather than just generating generic AI content. That's not a bad thing, but it means that plans now start at $49/month for individual creators and $125/month for teams.
Jasper has also moved away from just being a GPT app. It claims to combine "several large language models" including GPT-4, Claude 2, and PaLM 2, so that "you get the highest quality outputs and superior uptime." While I can't say that I noticed a massive difference between Jasper's output and any other app's, it does give you a few solid controls so that your content matches your brand.
You can create a brand Voice and Tone by uploading some appropriate sample text. Based on a few examples of my writing, Jasper created a style that "emphasizes a casual, conversational tone with humor, personal anecdotes, listicles, informal language, expertise in various subjects, and a call to action for an engaging and approachable brand voice." I don't think that's a bad summary of the content I fed in, and its output for a few test blog posts like "The Enduring Popularity of Top Gun" felt closer to my writing than when I asked it to use a generic casual tone of voice. Similarly, there's a Knowledge Base where you can add facts about your business and products so Jasper gets important details right.
While other apps also offer similar features, Jasper's seemed to work better and are fully integrated with the rest of the app. For example, you can create entire marketing campaigns using your custom brand voice. Put a bit of work into fine-tuning it and uploading the right assets to your knowledge base, and I suspect that Jasper really could create some solid first drafts of marketing materials like blog outlines, social media campaign ads, and the like.
Otherwise, Jasper rounds things out with some nice integrations. It has a built-in ChatGPT competitor and AI art generator (though, again, lots of other apps have both), plays nice with the SEO app Surfer , and there's a browser extension to bring Jasper everywhere.
You can also connect Jasper to thousands of other apps using Zapier . Learn more about how to automate Jasper , or try one of the pre-built workflows below.
Create product descriptions in Jasper from new or updated Airtable records

Create Jasper blog posts from new changes to specific column values in monday.com and save the text in Google Docs documents

Run Jasper commands and send Slack channel messages with new pushed messages in Slack

Jasper pricing: Creator plan from $49/month with one brand voice and 50 knowledge assets. Teams plan starts at $125/month for three seats, three brand voices, and 150 knowledge assets.
Best AI writing app for AI copywriting
Copy.ai (web).
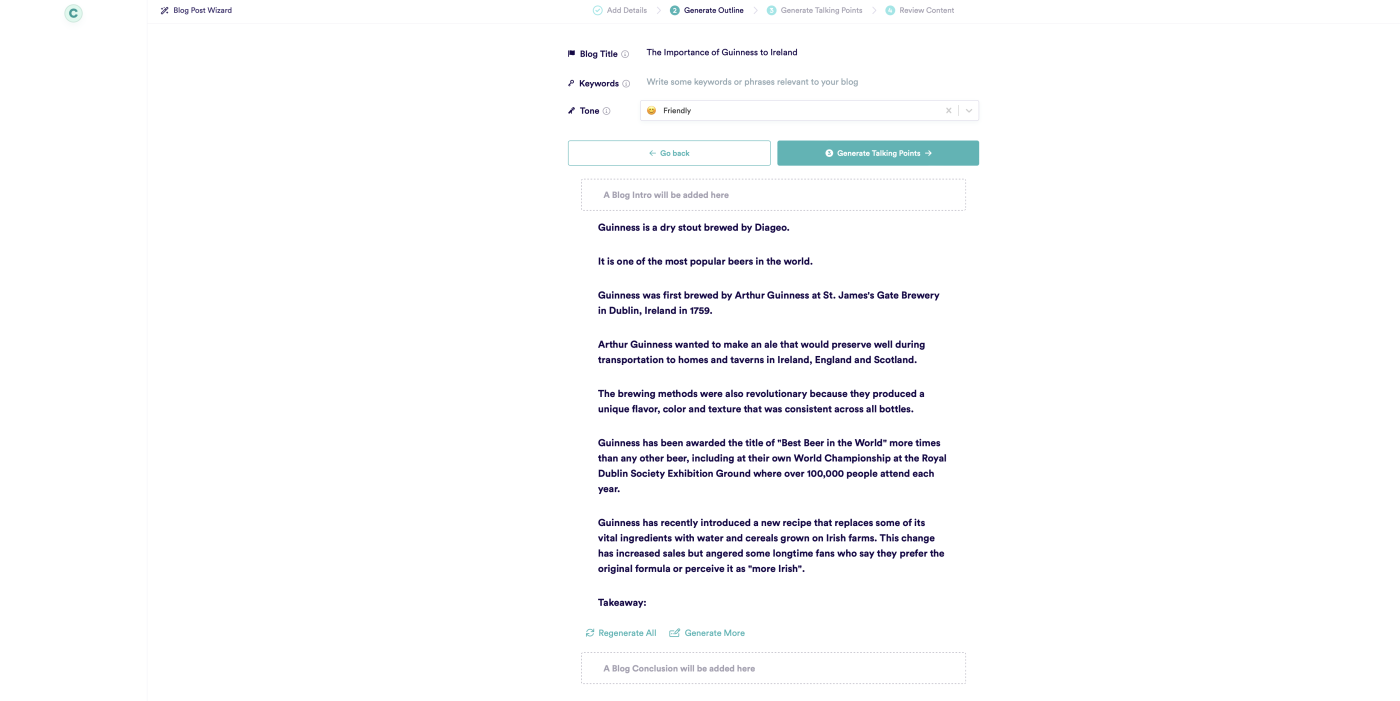
Copy.ai pros:
Has an affordable unlimited plan for high-volume users
Workflow actively solicits your input, which can lead to higher quality content
Copy.ai cons:
Expensive if you don't produce a lot of content
Pretty much anything Jasper can do, Copy.ai can do too. It has brand voices, an infobase, a chatbot, and team features (though there isn't a browser extension). Consider it the Burger King to Jasper's McDonalds.
And like the Home of the Whopper, Copy.ai appeals to slightly different tastes. While I could argue that Copy.ai has a nicer layout, the reality is it's geared toward a slightly different workflow. While Jasper lets you and the AI loose, Copy.ai slows things down a touch and encourages you to work with its chatbot or use a template that asks some deliberate, probing questions. For creating website copy, social media captions , product descriptions, and similarly specific things, it makes more sense. But for content marketing blog posts and other long-form content, it might annoy you.
The other big difference is the pricing. While both offer plans for $49/month, Copy.ai includes five user seats and unlimited brand voices. For a small team working with multiple brands, it can be a lot cheaper. Also, if you're looking for a free AI writing generator, Copy.ai also offers a free plan that includes 2,000 words per month.
Overall, there are more similarities than differences between Jasper and Copy.ai , and both can create almost all the same kinds of text. Even when it came to analyzing my voice, they both came to pretty similar conclusions. Copy.ai decided that, to mimic me, it had to "focus on creating content that is both educational and entertaining, using a conversational tone that makes readers feel like they're having a chat with a knowledgeable friend" and "not to be afraid to inject some humor or personal anecdotes." If you're in doubt, try them both out and then decide.
Copy.ai also integrates with Zapier , so you can do things like automatically sending content to your CMS or enriching leads straight from your CRM. Learn more about how to automate Copy. ai or try one of the pre-built workflows below.
Add new blog posts created with Copy.ai to Webflow

Copy.ai pricing: Free for 2,000 words per month; from $49/month for the Pro plan with 5 users and unlimited brand voices.
Best AI writing assistant
Anyword (web).
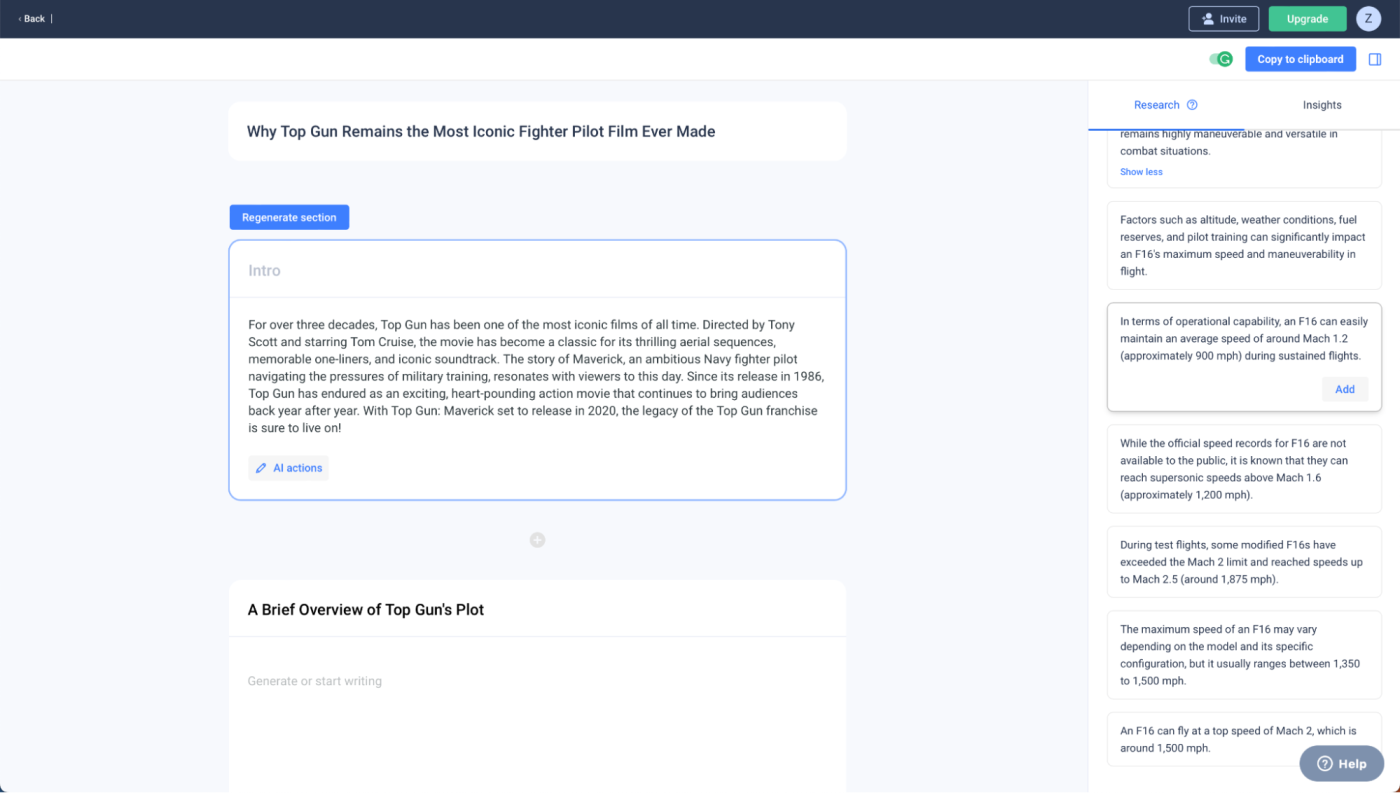
Anyword pros:
Makes it very easy for you to include specific details, SEO keywords, and other important information
Engagement scores and other metrics are surprisingly accurate
Anyword cons:
Can be slower to use
Pretty expensive for a more limited set of features than some of the other apps on this list
While you can direct the AI to include certain details and mention specific facts for every app on this list, none make it as easy as Anyword. More than any of the others, the AI here feels like an eager and moderately competent underling that requires a bit of micromanaging (and can also try to mimic your writing style and brand voice), rather than a beast that you have to tame with arcane prompts.
Take one of its main content-generating tools: the Blog Wizard. Like with Copy.ai, the setup process requires you to describe the blog post you want the AI to create and add any SEO keywords you want to target. Anyword then generates a range of titles for you to choose from, along with a predicted engagement score.
Once you've chosen a title—or written your own—it generates a suggested outline. Approve it, and you get the option for it to create an entire ~2,000-word blog post (boo!) or a blank document where you can prompt it with additional instructions for each section of the outline, telling it things like what facts to mention, what style to take, and what details to cover. There's also a chatbot-like research sidebar that you can ask questions of and solicit input from. While certainly a slower process than most apps, it gives you a serious amount of control over the content you're creating.
Anyword is definitely aimed at marketers, and its other tools—like the Data-Driven Editor and the Website Targeted Message—all allow you to target your content toward specific audiences and give things engagement scores. While I certainly can't confirm the validity of any of these scores, they at least pass the sniff test. I generally thought the AI-generated content that Anyword scored higher was better—and even when I disagreed, I still liked one of the top options.
Anyword pricing: Starter plan from $49/month for 1 user and 1 brand voice.
Best AI writing tool for writing fiction
Sudowrite (web).
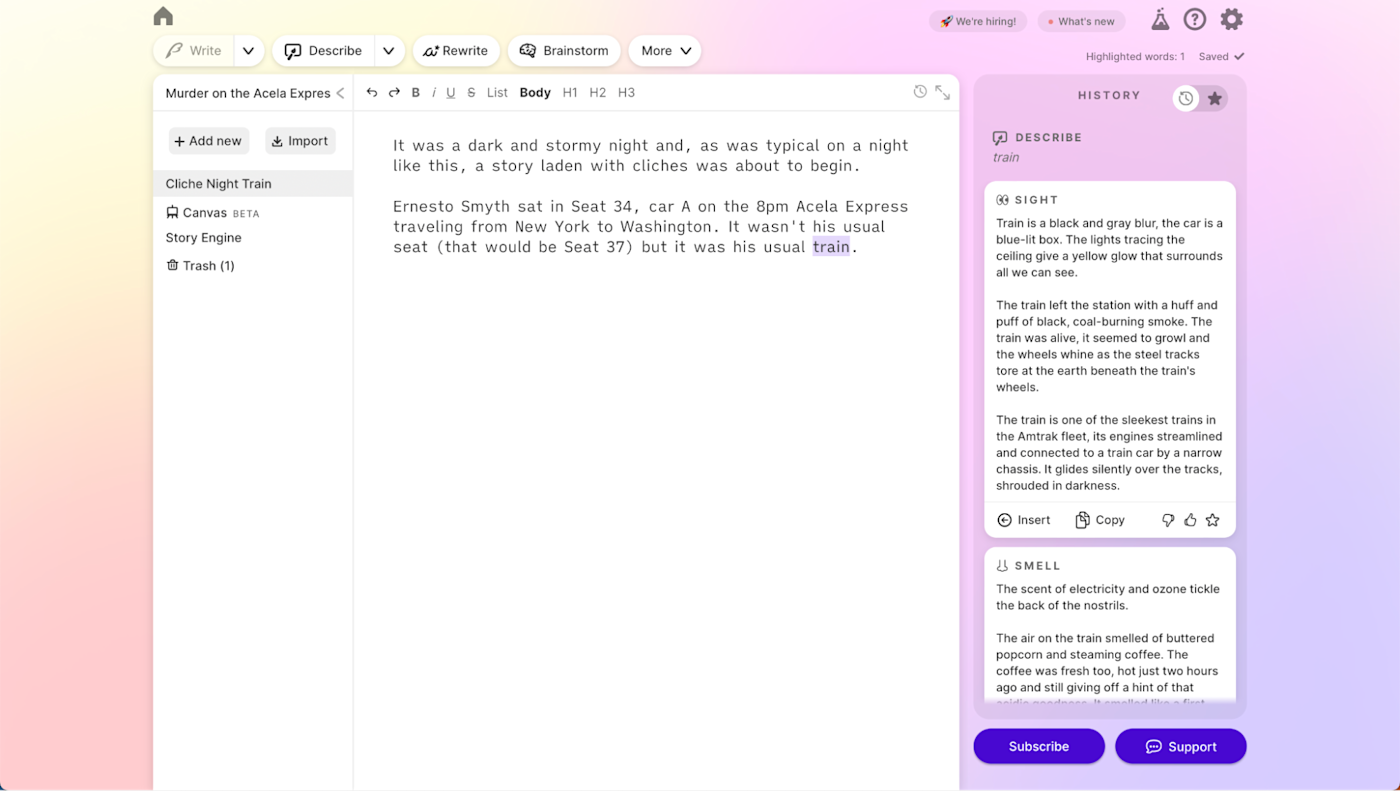
Sudowrite pros:
The only AI tool on the list explicitly aimed at writing fiction
Super fun to use if you've ever wanted to play around with fiction
Sudowrite cons:
It's still an AI text generator, so it can produce nonsensical metaphors, clichéd plots, incoherent action, and has a short memory for details
Very controversial in fiction writing circles
When I saw Sudowrite's marketing copy, I didn't think for a second it would make it onto this list. Then I tried it and…I kind of love it. Sudowrite is a totally different tool than all the others on this list because it's aimed at fiction writers. And with that, comes a lot of controversy. Sudowrite has been called " an insult to writers everywhere " and has been generally dismissed as a tool for hacks by a lot of Very Online writers. And while it's true that it's nowhere close to replacing a human author, it's fun, functional, and can genuinely help with writing a work of fiction.
The Story Engine feature, which allows you to generate a full work of fiction over a few days by progressively generating each story beat, has attracted the most attention ( it works but takes lots of hand-holding and your novel will be weird ). But I prefer its assistive tools.
Let's start with Describe. Select a word or phrase, click Describe , and the AI will generate a few suggestions for the sight, smell, taste, sound, and touch of the thing, as well as a couple of metaphors. If you're the kind of writer who struggles to add sensory depth to your short stories, it can help you get into the habit of describing things in more interesting ways.
Then there's Brainstorm. It allows you to use the AI to generate possible dialogue options, character names and traits, plot points, places, and other details about your world from your descriptions and cues. If you know you want a big hairy guy with a huge sword but can't think of a good name, it can suggest a few, like Thorgrim and Bohart.
And these are just scratching the surface. Sure, if you over-rely on the AI to solve all your problems, you'll probably end up with an impressively generic story. But if you use it as a writing buddy to bounce ideas off and get you out of a rut, it's got serious potential.
Best of all, Sudowrite is super easy to use. The onboarding, tool tips, and general helpful vibe of the app are something other developers could learn from.
Sudowrite pricing: Hobby & Student plan from $19/month for 30,000 AI words/month.
Best AI text generator for a non-GPT option
Writer (web).
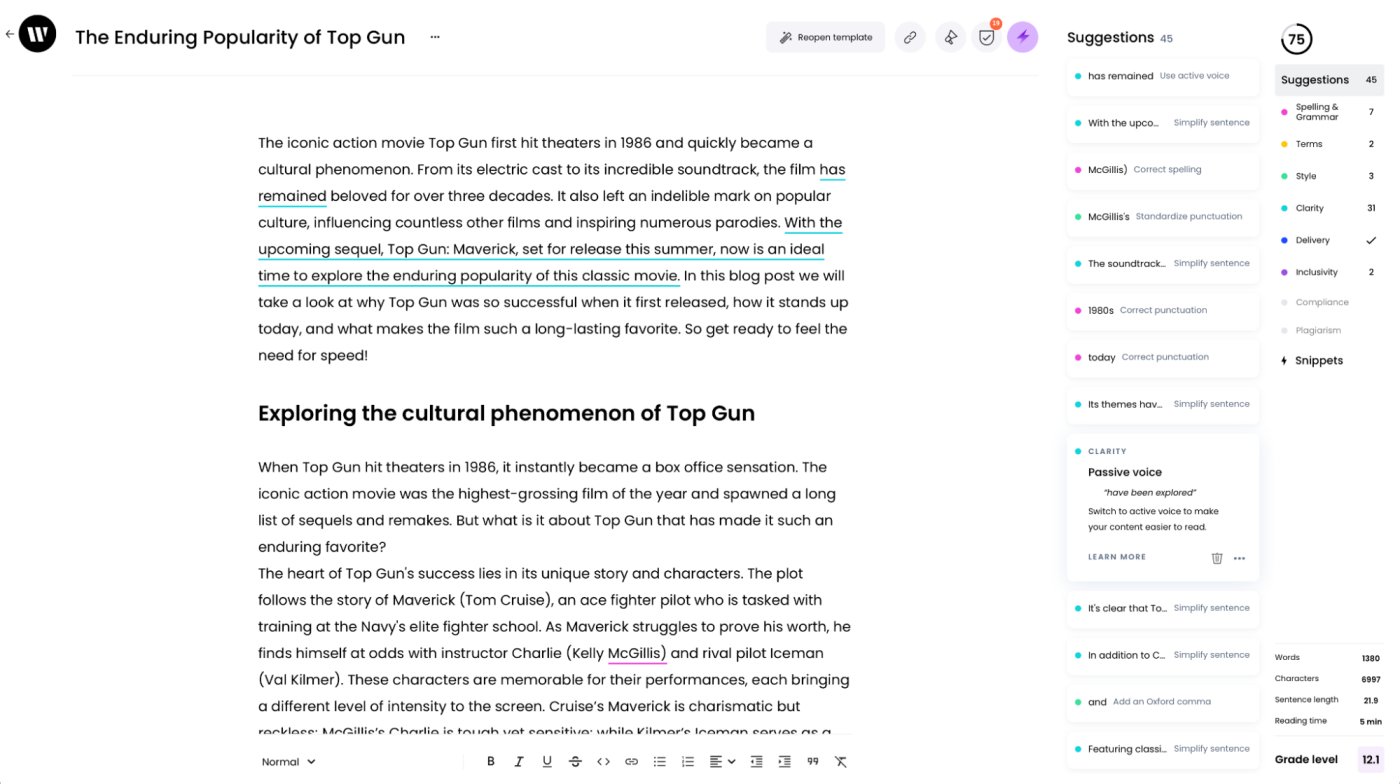
Writer pros:
Not based on GPT, so free of a lot of the controversy surrounding LLMs
Surprisingly capable as an editor, making sure your team sticks to the style guide and doesn't make any wild claims
Writer cons:
Requires a lot more setup to get the most from
GPT comes with quite a lot of baggage. OpenAI has been less than transparent about exactly what data was used to create the various versions of GPT-3 and GPT-4, and it's facing various lawsuits over the use of copyrighted material in its training dataset. No one is really denying that protected materials— potentially from pirated databases —were used to train GPT; the question is just whether or not it falls under fair use.
For most people, this is a nebulous situation filled with edge cases and gray areas. Realistically, it's going to be years before it's all sorted out, and even then, things will have moved on so far that the results of any lawsuit are likely to be redundant. But for businesses that want to use AI writing tools without controversy attached, GPT is a no-go—and will be for the foreseeable future.
Which is where Writer comes in.
Feature-wise, Writer is much the same as any of my top picks. (Though creating a specific brand voice that's automatically used is an Enterprise-only feature; otherwise, you have to use a lot of checkboxes in the settings to set the tone.) Some features, like the chatbot, are a little less useful than they are in the GPT-powered apps, but really, they're not why you'd choose Writer.
Where it stands out is the transparency around its Palmyra LLM . For example, you can request and inspect a copy of its training dataset that's composed of data that is "distributed free of any copyright restrictions." Similarly, Palmyra's code and model weights (which determines its outputs) can be audited, it can be hosted on your own servers, and your data is kept secure and not used for training by default. As an AI-powered tool, it's as above board as it comes.
In addition to generating text, Writer can work as a company-specific Grammarly-like editor, keeping on top of legal compliance, ensuring you don't make any unsupported claims, and checking that everything matches your style guide—even when humans are writing the text. As someone who routinely has to follow style guides, this seems like an incredibly useful feature. I wasn't able to test it fully since I don't have a personal style guide to input, but Writer correctly fixed things based on all the rules that I set.
In side-by-side comparisons, Writer's text generations sometimes felt a little weaker than the ones from Jasper or Copy.ai, but I suspect a lot of that was down to how things were configured. Writer is designed as a tool for companies to set up and train with their own data, not run right out of the box. I'd guess my random blog posts were a poor test of how it should be used in the real world.
Writer also integrates with Zapier , so you can use Writer to create content directly from whatever apps you use most. Learn more about how to automate Writer , or take a look at these pre-made workflows.
Create new outlines or drafts in Writer based on briefs from Asana

Generate marketing content from project briefs in Trello

Writer pricing: Team from $18/user/month for up to 5 users; after that, it's an Enterprise plan.
Best AI text generator for GPT-4 content
Writesonic (web).
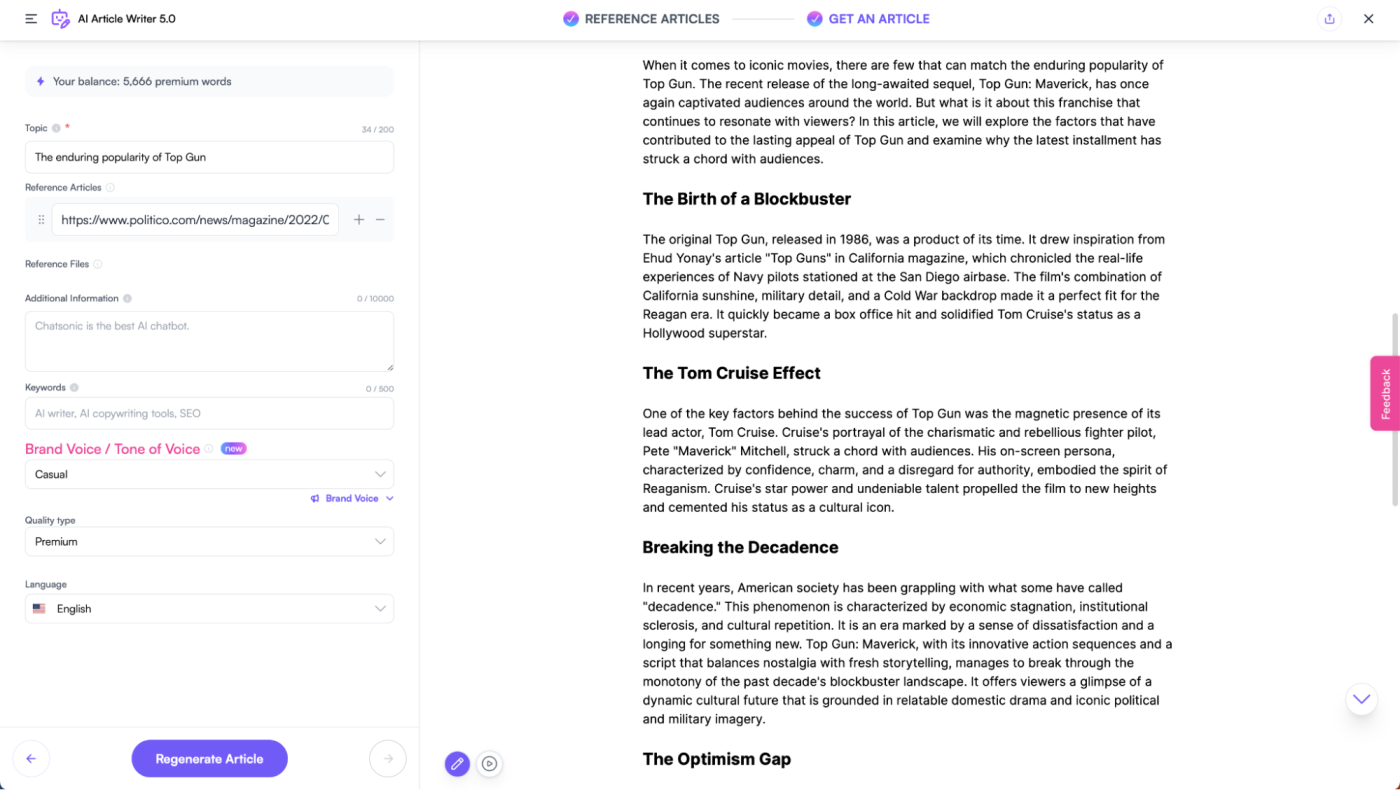
Writesonic pros:
Allows you to select what GPT model is used to generate text
Generous free plan and affordable paid plans
Writesonic cons:
A touch too focused on SEO content for my taste
While almost all the tools on this list use GPT, most are pretty vague about which particular version of it they use at any given time. This matters because the most basic version of the GPT-3.5 Turbo API costs $0.002/1K tokens (roughly 750 words), while GPT-4 starts at $0.06/1K tokens, and the most powerful version costs $0.12/1K tokens. All this suggests that most apps may not use GPT-4 in all circumstances, and instead probably rely on one of the more modest (though still great) GPT-3 models for most text generation.
If having the latest and greatest AI model matters to you, Writesonic is the app for you. Writesonic doesn't hide what AI model it uses. It even allows you to choose between using GPT-3.5 and GPT-4, at least on Business plans.
Whether the content you create will benefit from the extra power of GPT-4 or not depends. In my experience using GPT-4 through ChatGPT, the latest model is more accurate and, essentially, more sensible in how it responds. If you're churning out low-stakes copy variations for your product listings, you likely won't see much improvement. On the other hand, for long-form original blog posts, it could make a difference. Either way, the transparency in which model you're using at any given time is a huge bonus.
Feature-wise, Writesonic is much the same as any of the other apps on this list, with a Google Docs-style editor, the option to set a brand voice, a few dozen copy templates, a chatbot, a browser extension, and Surfer integration. It's cool that you can set reference articles when you're generating a blog post, but it introduces the real possibility of inadvertent plagiarism if you aren't careful with how you use it. (Its most offbeat feature is a surprisingly solid AI-powered custom chatbot builder that's due to be spun out into its own app soon.) Overall, it's pretty nice to use and skews more toward SEO-optimized content marketing—but like with all the apps, you can use it to generate whatever you want.
Writesonic also integrates with Zapier , so you can send new copy to any of the other apps you use in your writing workflow. Learn more about how to automate Writesonic , or get started with one of these examples.
Create a Google Doc with new content from Writesonic

Generate product descriptions with Writesonic from spreadsheet rows in Google Sheets

Writesonic pricing: Free for 10,000 GPT-3.5 words per month; Business from $19/month for 200,000 Premium words or 33,333 GPT-4 words.
Best free AI writing generator (with affordable upgrades)
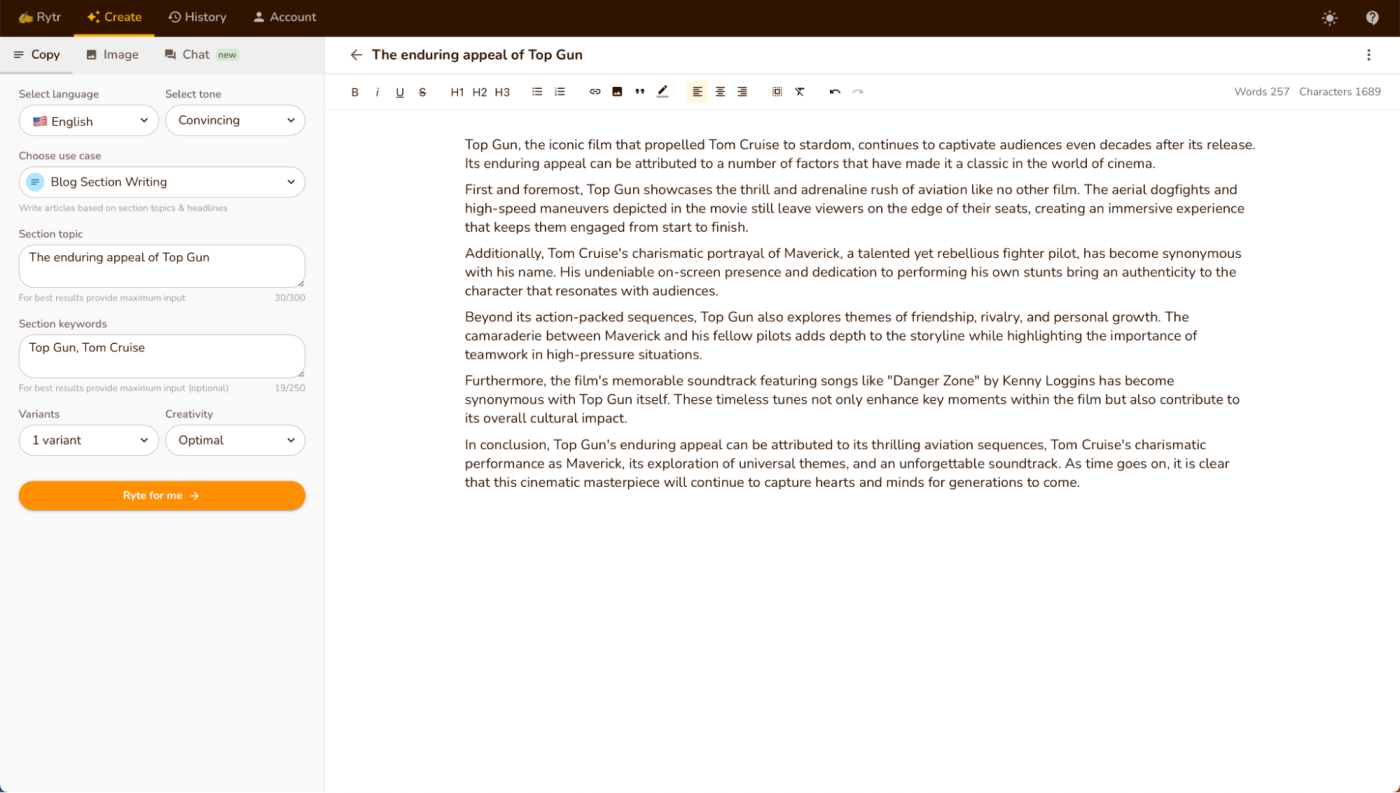
A solid free plan and a cheap high-volume plan (though Writesonic offers better value for an unlimited plan)
It includes a basic AI art generator as part of every plan
The app is more basic than more expensive offerings
Unlimited plan isn't very competitive
Most of the apps on this list are aimed at professionals, businesses, and anyone else with a budget. The Jasper, Copy.ai, and Anyword plans I considered all started at $49/month. That isn't exactly a hobbyist-friendly sum of money, so if you want to explore AI text generators without spending as much, give Rytr a go.
There's a free plan that's good for 10,000 characters (around 2,500 words) per month, and it includes a lot of the features, like a plagiarism checker, and a few AI-generated images. The Saver plan starts at $9/month and allows you to generate 100,000 characters (around 25,000 words) per month. On that plan, you're also able to generate up to 20 images a month, which many other apps charge extra for. (There's also an unlimited plan for $29/month, but at that point, Writesonic is a better value.)
Feature-wise, there are some trade-offs. Rytr is a little less competent at generating long-form content without you guiding it through the process, and there are fewer templates for specific things. The interface also isn't as polished, and there isn't as much hand-holding to get you started. Still, as Rytr is using GPT like almost all the other apps on this list, you should be able to get it to produce substantially similar output.
Rytr Pricing: Free plan for 10,000 characters/month and lots of other features; Saver plan from $9/month for 100,000 characters; Unlimited plan from $29/month.
Other AI writing tools to consider
With so many AI text-generating tools out there, a few good ones worth considering didn't make this list, only because they didn't meet my initial criteria in some way. If none of the AI writers I chose fit the bill for you, here are a few other options worth looking into:
ChatGPT is surprisingly competent and fun to use. And best of all, it's free. ( Google Bard is a little less excellent on the content production side.)
Wordtune and Grammarly are both great tools for editing and improving your own writing . GrammarlyGO just isn't as flexible as my other picks.
Notion AI adds a powerful AI tool directly into Notion. If you already use Notion, it's worth checking out, but it's a lot to learn if you just want a text generator. (Same goes for AI within any other Notion alternative, like Coda AI .)
Surfer and Frase are both AI-powered SEO tools . They fell slightly out of scope for this list, but they can both help you optimize and improve your content—AI-generated or not.
All of the apps on this list offer at the very least a free trial, so I'd suggest trying some of them out for a few minutes until you find the one that seems to work best with your workflow.
Related reading:
How to use OpenAI's GPT to spark content ideas
How to create an AI writing coach with GPT and Zapier
8 ways real businesses are using AI for content creation
How to detect AI-generated content
The best AI marketing tools
This article was originally published in April 2023. The most recent update was in September 2023.
Get productivity tips delivered straight to your inbox
We’ll email you 1-3 times per week—and never share your information.

Harry Guinness
Harry Guinness is a writer and photographer from Dublin, Ireland. His writing has appeared in the New York Times, Lifehacker, the Irish Examiner, and How-To Geek. His photos have been published on hundreds of sites—mostly without his permission.
- Artificial intelligence (AI)
- Media and editorial
- Content marketing
Related articles
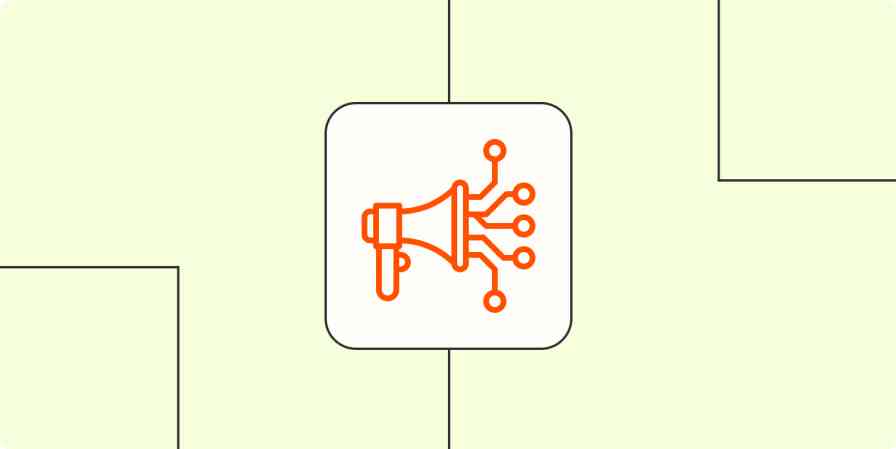
The 11 best AI marketing tools in 2024
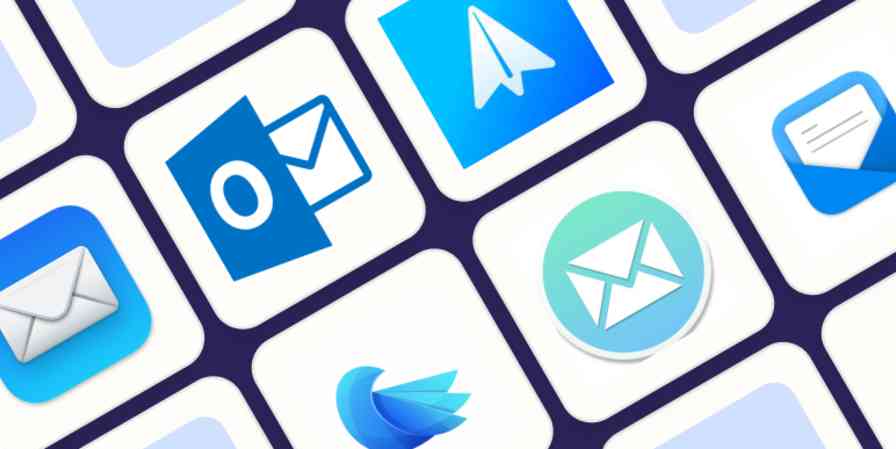
The 6 best email clients for Mac in 2024
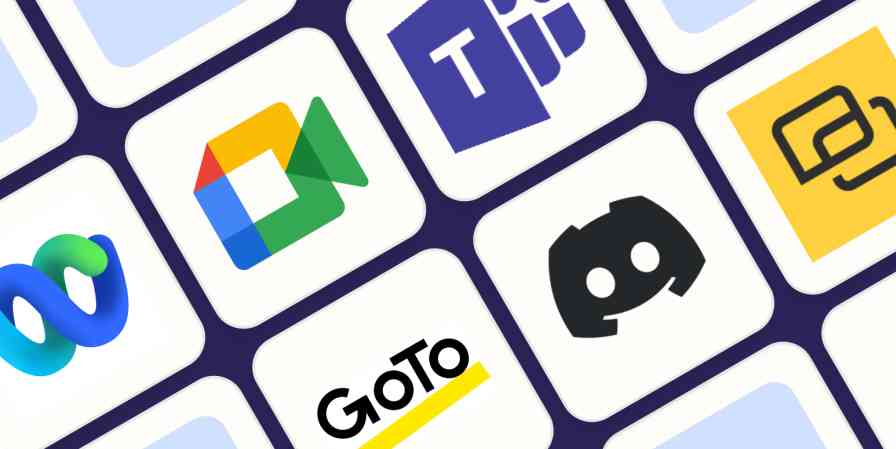
The 7 best Zoom alternatives in 2024
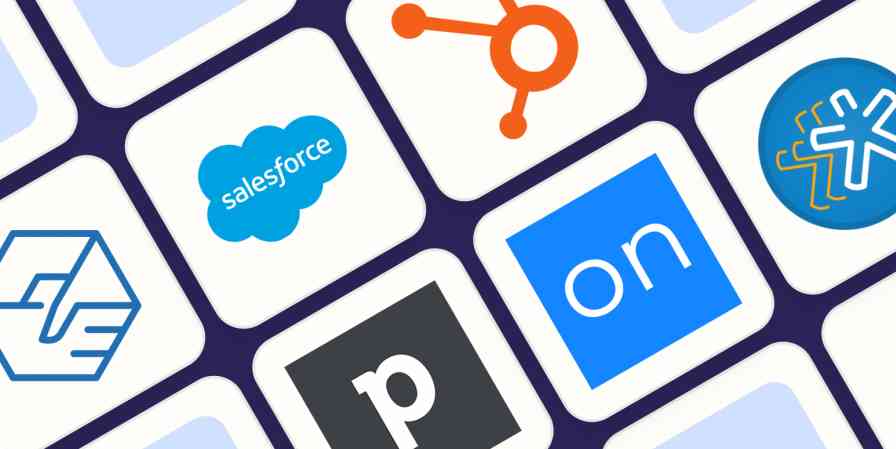
The best CRM software to manage your leads and customers in 2024
The best CRM software to manage your leads...
Improve your productivity automatically. Use Zapier to get your apps working together.
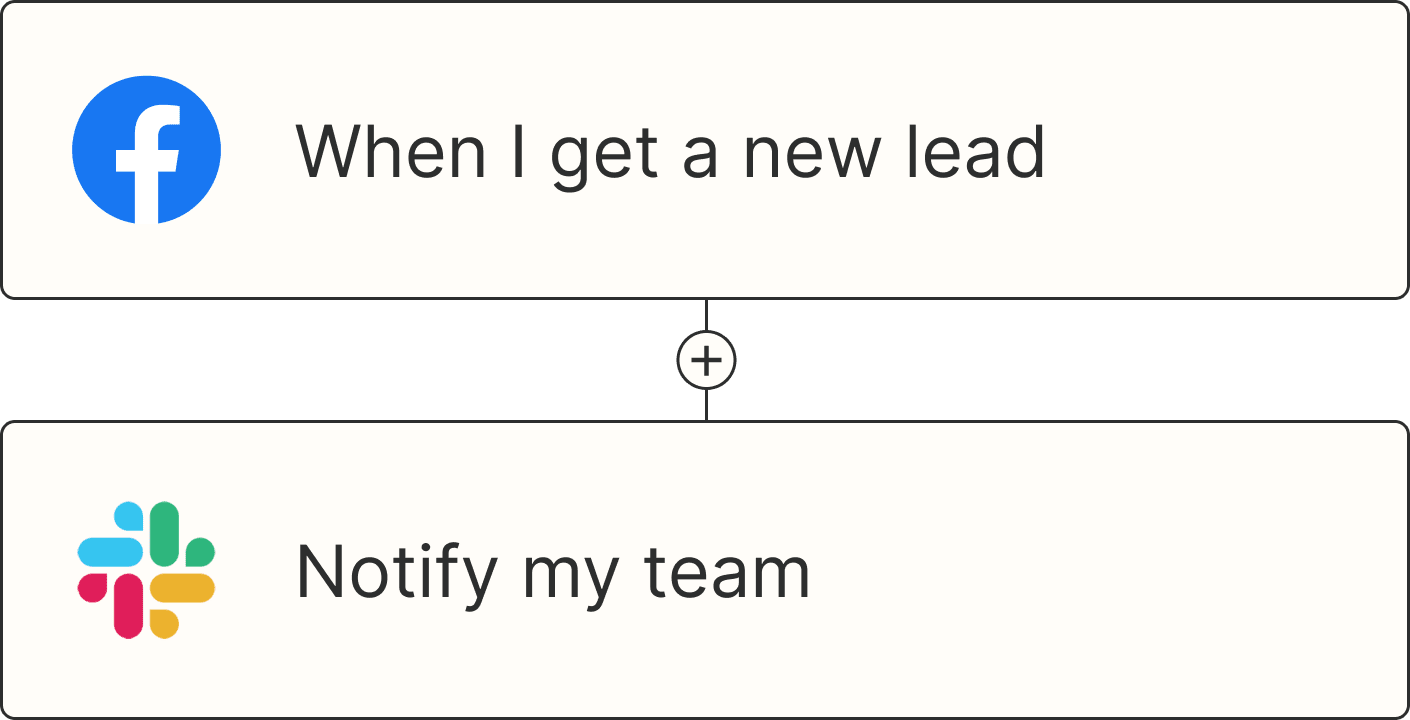
How to Write a Better Thesis Statement Using AI (2023 Updated)
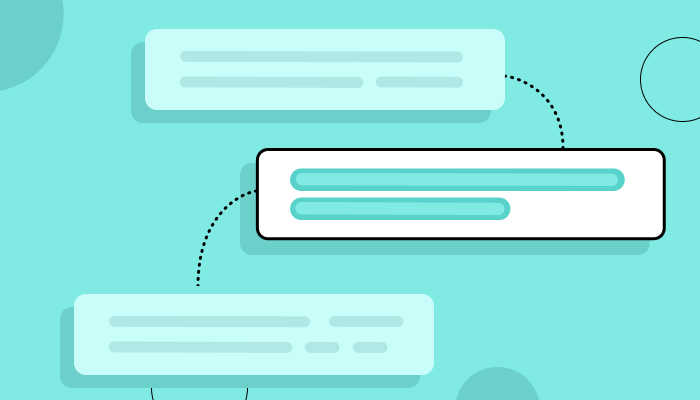
Table of contents
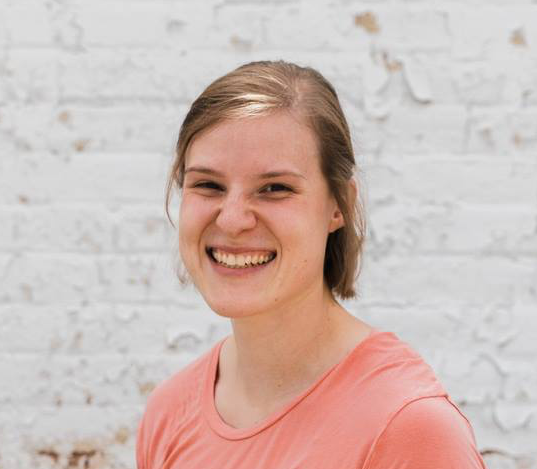
Meredith Sell
With the exceptions of poetry and fiction, every piece of writing needs a thesis statement.
- Opinion pieces for the local newspaper? Yes.
- An essay for a college class? You betcha.
- A book about China’s Ming Dynasty? Absolutely.
All of these pieces of writing need a thesis statement that sums up what they’re about and tells the reader what to expect, whether you’re making an argument, describing something in detail, or exploring ideas.
But how do you write a thesis statement? How do you even come up with one?
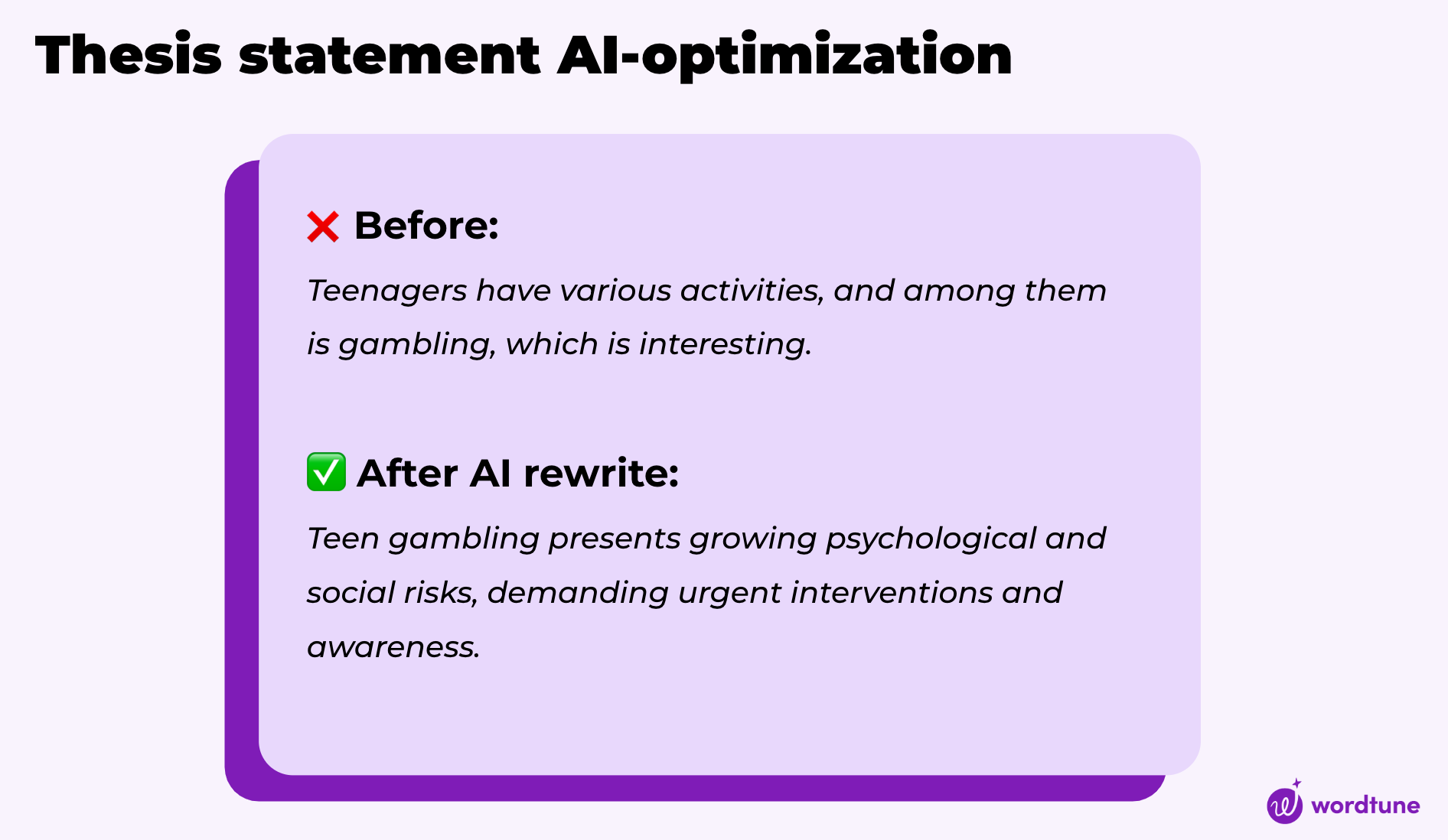
This step-by-step guide will show you exactly how — and help you make sure every thesis statement you write has all the parts needed to be clear, coherent, and complete.
Let’s start by making sure we understand what a thesis is (and what it’s not).

What Is a Thesis Statement?
A thesis statement is a one or two sentence long statement that concisely describes your paper’s subject, angle or position — and offers a preview of the evidence or argument your essay will present.
A thesis is not:
- An exclamation
- A simple fact
Think of your thesis as the road map for your essay. It briefly charts where you’ll start (subject), what you’ll cover (evidence/argument), and where you’ll land (position, angle).
Writing a thesis early in your essay writing process can help you keep your writing focused, so you won’t get off-track describing something that has nothing to do with your central point. Your central point is your thesis, and the rest of your essay fleshes it out.
Get help writing your thesis statement with this FREE AI tool > Get help writing your thesis statement with this FREE AI tool >
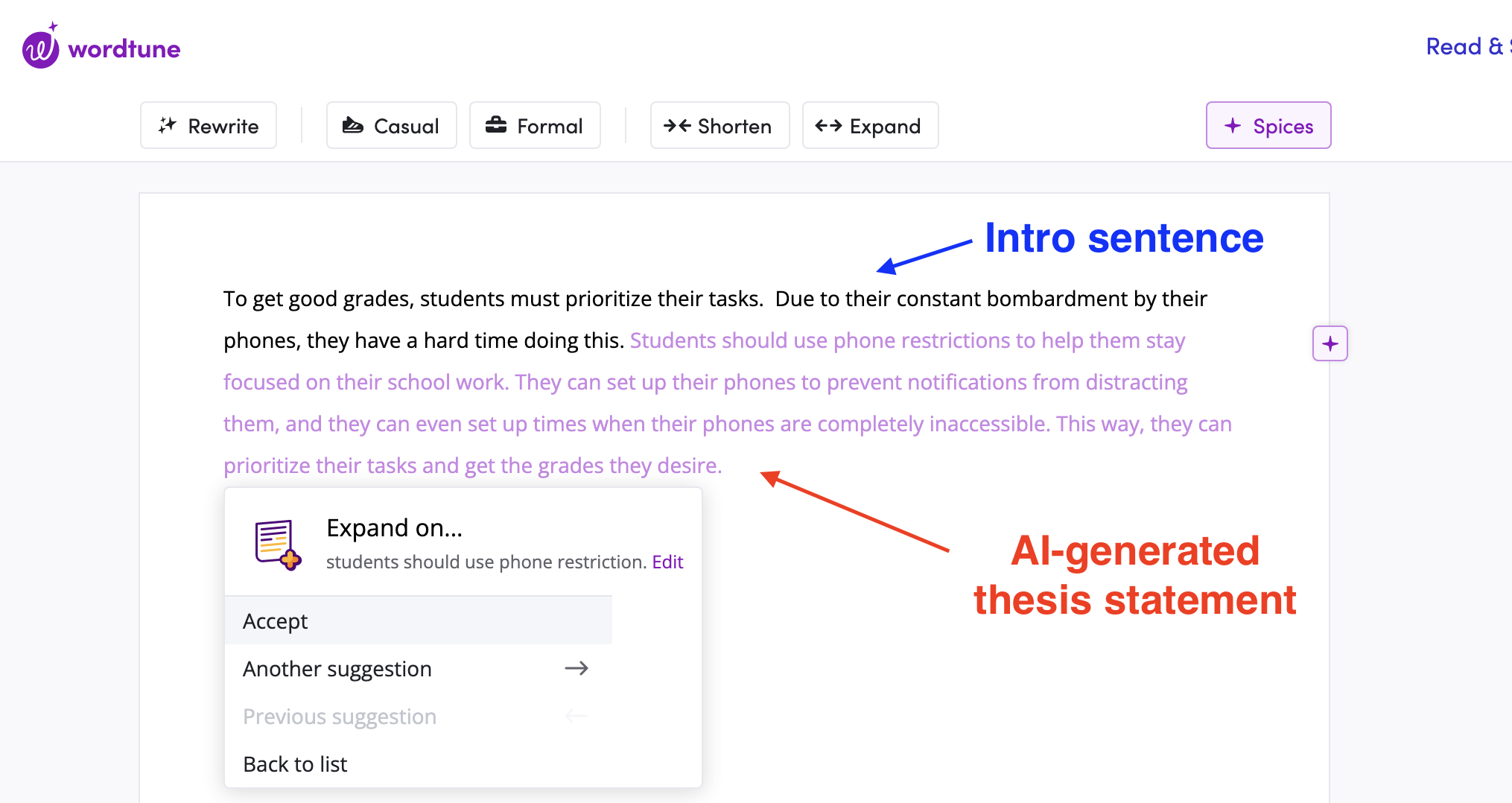
Different Kinds of Papers Need Different Kinds of Theses
How you compose your thesis will depend on the type of essay you’re writing. For academic writing, there are three main kinds of essays:
- Persuasive, aka argumentative
- Expository, aka explanatory
A persuasive essay requires a thesis that clearly states the central stance of the paper , what the rest of the paper will argue in support of.
Paper books are superior to ebooks when it comes to form, function, and overall reader experience.
An expository essay’s thesis sets up the paper’s focus and angle — the paper’s unique take, what in particular it will be describing and why . The why element gives the reader a reason to read; it tells the reader why the topic matters.
Understanding the functional design of physical books can help ebook designers create digital reading experiences that usher readers into literary worlds without technological difficulties.
A narrative essay is similar to that of an expository essay, but it may be less focused on tangible realities and more on intangibles of, for example, the human experience.
The books I’ve read over the years have shaped me, opening me up to worlds and ideas and ways of being that I would otherwise know nothing about.
As you prepare to craft your thesis, think through the goal of your paper. Are you making an argument? Describing the chemical properties of hydrogen? Exploring your relationship with the outdoors? What do you want the reader to take away from reading your piece?
Make note of your paper’s goal and then walk through our thesis-writing process.
Now that you practically have a PhD in theses, let’s learn how to write one:
How to Write (and Develop) a Strong Thesis
If developing a thesis is stressing you out, take heart — basically no one has a strong thesis right away. Developing a thesis is a multi-step process that takes time, thought, and perhaps most important of all: research .
Tackle these steps one by one and you’ll soon have a thesis that’s rock-solid.
1. Identify your essay topic.
Are you writing about gardening? Sword etiquette? King Louis XIV?
With your assignment requirements in mind, pick out a topic (or two) and do some preliminary research . Read up on the basic facts of your topic. Identify a particular angle or focus that’s interesting to you. If you’re writing a persuasive essay, look for an aspect that people have contentious opinions on (and read our piece on persuasive essays to craft a compelling argument).
If your professor assigned a particular topic, you’ll still want to do some reading to make sure you know enough about the topic to pick your specific angle.
For those writing narrative essays involving personal experiences, you may need to do a combination of research and freewriting to explore the topic before honing in on what’s most compelling to you.
Once you have a clear idea of the topic and what interests you, go on to the next step.
2. Ask a research question.
You know what you’re going to write about, at least broadly. Now you just have to narrow in on an angle or focus appropriate to the length of your assignment. To do this, start by asking a question that probes deeper into your topic.
This question may explore connections between causes and effects, the accuracy of an assumption you have, or a value judgment you’d like to investigate, among others.
For example, if you want to write about gardening for a persuasive essay and you’re interested in raised garden beds, your question could be:
What are the unique benefits of gardening in raised beds versus on the ground? Is one better than the other?
Or if you’re writing about sword etiquette for an expository essay , you could ask:
How did sword etiquette in Europe compare to samurai sword etiquette in Japan?
How does medieval sword etiquette influence modern fencing?
Kickstart your curiosity and come up with a handful of intriguing questions. Then pick the two most compelling to initially research (you’ll discard one later).
3. Answer the question tentatively.
You probably have an initial thought of what the answer to your research question is. Write that down in as specific terms as possible. This is your working thesis .
Gardening in raised beds is preferable because you won’t accidentally awaken dormant weed seeds — and you can provide more fertile soil and protection from invasive species.
Medieval sword-fighting rituals are echoed in modern fencing etiquette.
Why is a working thesis helpful?
Both your research question and your working thesis will guide your research. It’s easy to start reading anything and everything related to your broad topic — but for a 4-, 10-, or even 20-page paper, you don’t need to know everything. You just need the relevant facts and enough context to accurately and clearly communicate to your reader.
Your working thesis will not be identical to your final thesis, because you don’t know that much just yet.
This brings us to our next step:
4. Research the question (and working thesis).
What do you need to find out in order to evaluate the strength of your thesis? What do you need to investigate to answer your research question more fully?
Comb through authoritative, trustworthy sources to find that information. And keep detailed notes.
As you research, evaluate the strengths and weaknesses of your thesis — and see what other opposing or more nuanced theses exist.
If you’re writing a persuasive essay, it may be helpful to organize information according to what does or does not support your thesis — or simply gather the information and see if it’s changing your mind. What new opinion do you have now that you’ve learned more about your topic and question? What discoveries have you made that discredit or support your initial thesis?
Raised garden beds prevent full maturity in certain plants — and are more prone to cold, heat, and drought.
If you’re writing an expository essay, use this research process to see if your initial idea holds up to the facts. And be on the lookout for other angles that would be more appropriate or interesting for your assignment.
Modern fencing doesn’t share many rituals with medieval swordplay.
With all this research under your belt, you can answer your research question in-depth — and you’ll have a clearer idea of whether or not your working thesis is anywhere near being accurate or arguable. What’s next?
5. Refine your thesis.
If you found that your working thesis was totally off-base, you’ll probably have to write a new one from scratch.
For a persuasive essay , maybe you found a different opinion far more compelling than your initial take. For an expository essay , maybe your initial assumption was completely wrong — could you flip your thesis around and inform your readers of what you learned?
Use what you’ve learned to rewrite or revise your thesis to be more accurate, specific, and compelling.
Raised garden beds appeal to many gardeners for the semblance of control they offer over what will and will not grow, but they are also more prone to changes in weather and air temperature and may prevent certain plants from reaching full maturity. All of this makes raised beds the worse option for ambitious gardeners.
While swordplay can be traced back through millennia, modern fencing has little in common with medieval combat where swordsmen fought to the death.
If you’ve been researching two separate questions and theses, now’s the time to evaluate which one is most interesting, compelling, or appropriate for your assignment. Did one thesis completely fall apart when faced with the facts? Did one fail to turn up any legitimate sources or studies? Choose the stronger question or the more interesting (revised) thesis, and discard the other.
6. Get help from AI
To make the process even easier, you can take advantage of Wordtune's generative AI capabilities to craft an effective thesis statement. You can take your current thesis statement and try the paraphrase tool to get suggestions for better ways of articulating it. WordTune will generate a set of related phrases, which you can select to help you refine your statement. You can also use Wordtune's suggestions to craft the thesis statement. Write your initial introduction sentence, then click '+' and select the explain suggestion. Browse through the suggestions until you have a statement that captures your idea perfectly.
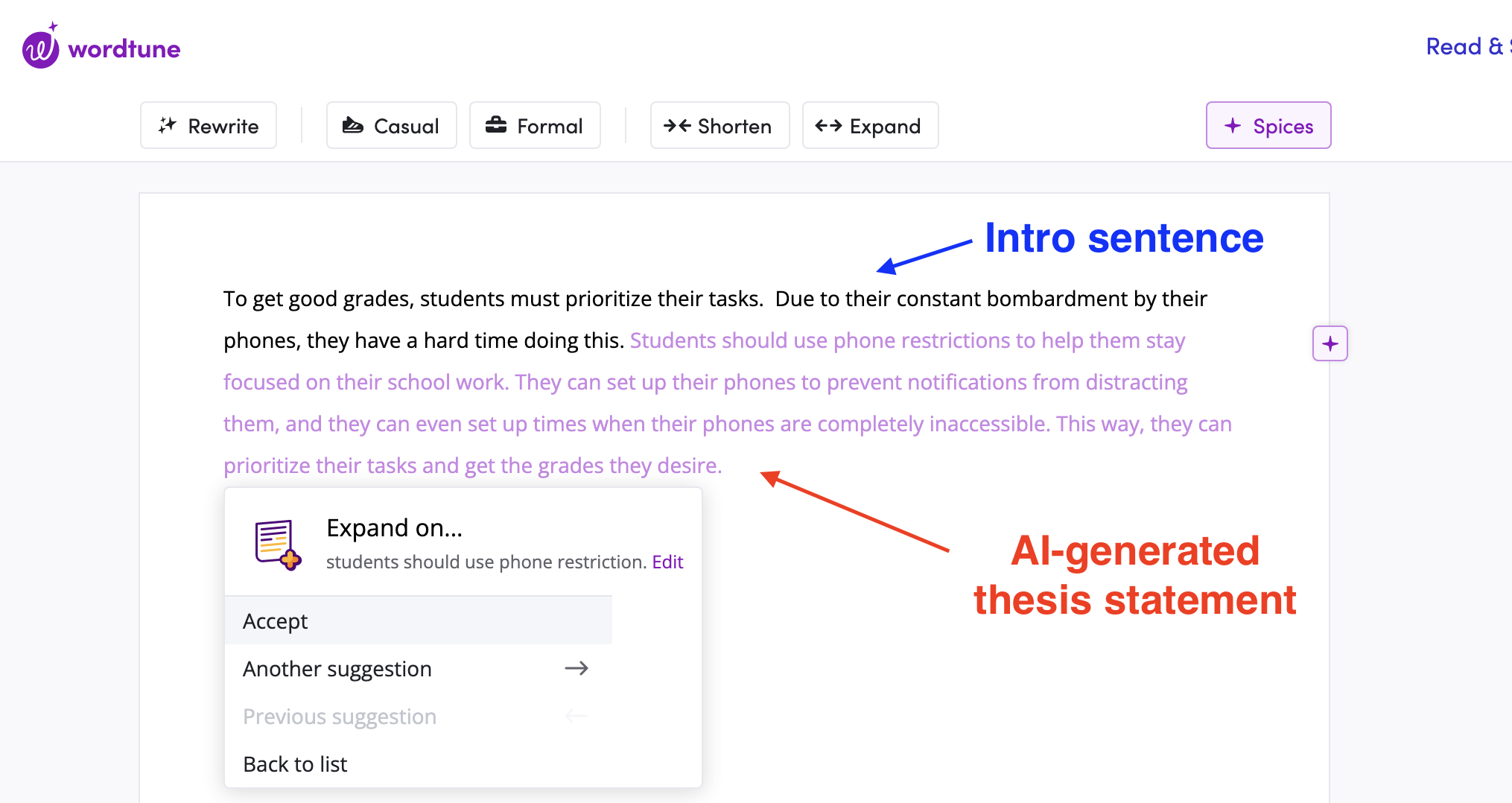
Thesis Check: Look for These Three Elements
At this point, you should have a thesis that will set up an original, compelling essay, but before you set out to write that essay, make sure your thesis contains these three elements:
- Topic: Your thesis should clearly state the topic of your essay, whether swashbuckling pirates, raised garden beds, or methods of snow removal.
- Position or angle: Your thesis should zoom into the specific aspect of your topic that your essay will focus on, and briefly but boldly state your position or describe your angle.
- Summary of evidence and/or argument: In a concise phrase or two, your thesis should summarize the evidence and/or argument your essay will present, setting up your readers for what’s coming without giving everything away.
The challenge for you is communicating each of these elements in a sentence or two. But remember: Your thesis will come at the end of your intro, which will already have done some work to establish your topic and focus. Those aspects don’t need to be over explained in your thesis — just clearly mentioned and tied to your position and evidence.
Let’s look at our examples from earlier to see how they accomplish this:
Notice how:
- The topic is mentioned by name.
- The position or angle is clearly stated.
- The evidence or argument is set up, as well as the assumptions or opposing view that the essay will debunk.
Both theses prepare the reader for what’s coming in the rest of the essay:
- An argument to show that raised beds are actually a poor option for gardeners who want to grow thriving, healthy, resilient plants.
- An exposition of modern fencing in comparison with medieval sword fighting that shows how different they are.
Examine your refined thesis. Are all three elements present? If any are missing, make any additions or clarifications needed to correct it.
It’s Essay-Writing Time!
Now that your thesis is ready to go, you have the rest of your essay to think about. With the work you’ve already done to develop your thesis, you should have an idea of what comes next — but if you need help forming your persuasive essay’s argument, we’ve got a blog for that.
Share This Article:
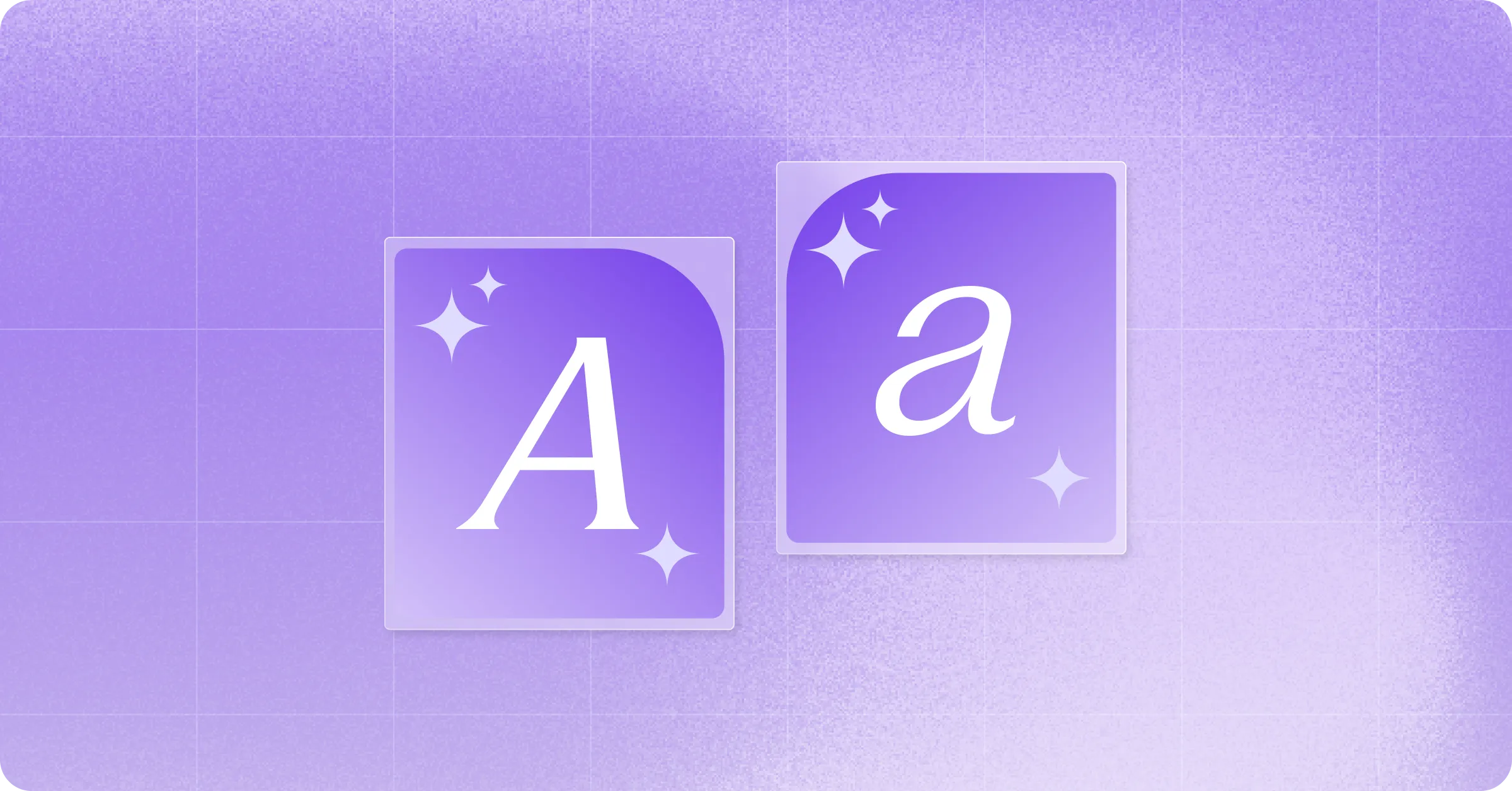
Title Case vs. Sentence Case: How to Capitalize Your Titles
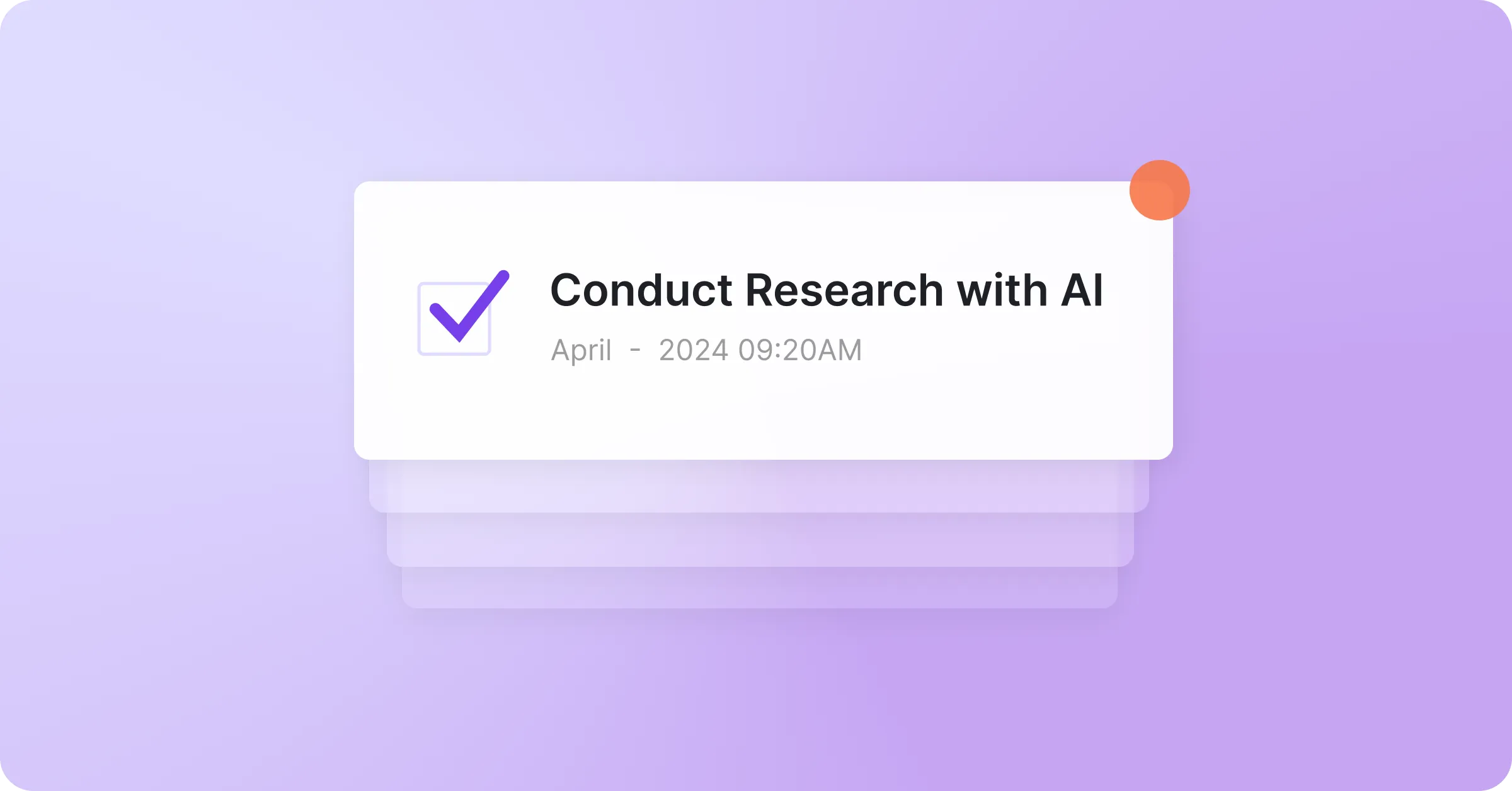
How to Properly Conduct Research with AI: Tools, Process, and Approach

What’s a Double Negative? + How To Fix It
Looking for fresh content, thank you your submission has been received.
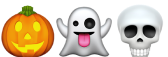
- Onsite training
3,000,000+ delegates
15,000+ clients
1,000+ locations
- KnowledgePass
- Log a ticket
01344203999 Available 24/7
12 Best Artificial Intelligence Topics for Research in 2024
Explore the "12 Best Artificial Intelligence Topics for Research in 2024." Dive into the top AI research areas, including Natural Language Processing, Computer Vision, Reinforcement Learning, Explainable AI (XAI), AI in Healthcare, Autonomous Vehicles, and AI Ethics and Bias. Stay ahead of the curve and make informed choices for your AI research endeavours.

Exclusive 40% OFF
Training Outcomes Within Your Budget!
We ensure quality, budget-alignment, and timely delivery by our expert instructors.
Share this Resource
- AI Tools in Performance Marketing Training
- Deep Learning Course
- Natural Language Processing (NLP) Fundamentals with Python
- Machine Learning Course
- Duet AI for Workspace Training
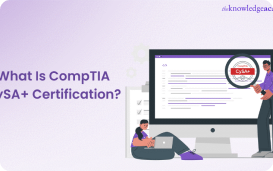
Table of Contents
1) Top Artificial Intelligence Topics for Research
a) Natural Language Processing
b) Computer vision
c) Reinforcement Learning
d) Explainable AI (XAI)
e) Generative Adversarial Networks (GANs)
f) Robotics and AI
g) AI in healthcare
h) AI for social good
i) Autonomous vehicles
j) AI ethics and bias
2) Conclusion
Top Artificial Intelligence Topics for Research
This section of the blog will expand on some of the best Artificial Intelligence Topics for research.
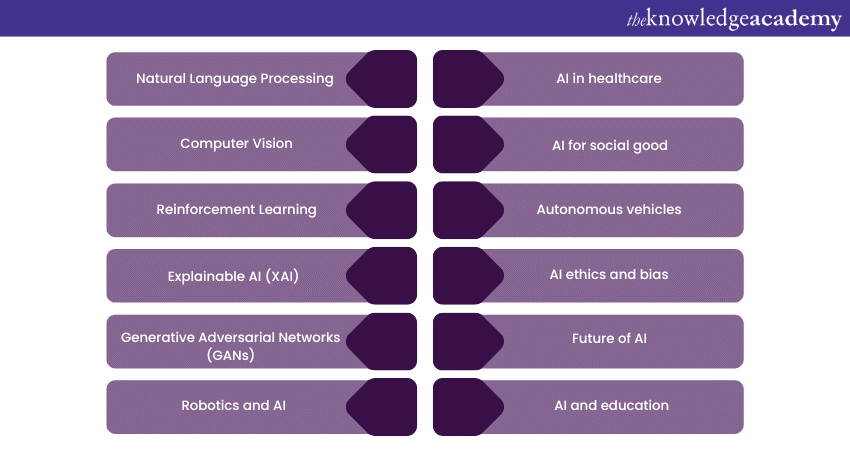
Natural Language Processing
Natural Language Processing (NLP) is centred around empowering machines to comprehend, interpret, and even generate human language. Within this domain, three distinctive research avenues beckon:
1) Sentiment analysis: This entails the study of methodologies to decipher and discern emotions encapsulated within textual content. Understanding sentiments is pivotal in applications ranging from brand perception analysis to social media insights.
2) Language generation: Generating coherent and contextually apt text is an ongoing pursuit. Investigating mechanisms that allow machines to produce human-like narratives and responses holds immense potential across sectors.
3) Question answering systems: Constructing systems that can grasp the nuances of natural language questions and provide accurate, coherent responses is a cornerstone of NLP research. This facet has implications for knowledge dissemination, customer support, and more.
Computer Vision
Computer Vision, a discipline that bestows machines with the ability to interpret visual data, is replete with intriguing avenues for research:
1) Object detection and tracking: The development of algorithms capable of identifying and tracking objects within images and videos finds relevance in surveillance, automotive safety, and beyond.
2) Image captioning: Bridging the gap between visual and textual comprehension, this research area focuses on generating descriptive captions for images, catering to visually impaired individuals and enhancing multimedia indexing.
3) Facial recognition: Advancements in facial recognition technology hold implications for security, personalisation, and accessibility, necessitating ongoing research into accuracy and ethical considerations.
Reinforcement Learning
Reinforcement Learning revolves around training agents to make sequential decisions in order to maximise rewards. Within this realm, three prominent Artificial Intelligence Topics emerge:
1) Autonomous agents: Crafting AI agents that exhibit decision-making prowess in dynamic environments paves the way for applications like autonomous robotics and adaptive systems.
2) Deep Q-Networks (DQN): Deep Q-Networks, a class of reinforcement learning algorithms, remain under active research for refining value-based decision-making in complex scenarios.
3) Policy gradient methods: These methods, aiming to optimise policies directly, play a crucial role in fine-tuning decision-making processes across domains like gaming, finance, and robotics.
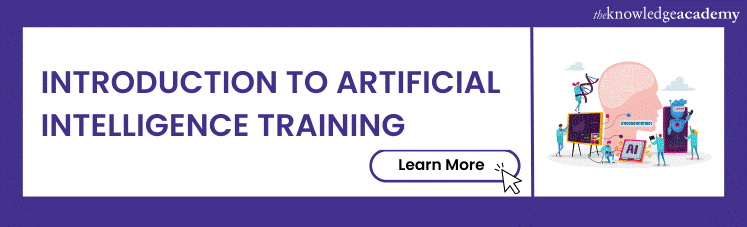
Explainable AI (XAI)
The pursuit of Explainable AI seeks to demystify the decision-making processes of AI systems. This area comprises Artificial Intelligence Topics such as:
1) Model interpretability: Unravelling the inner workings of complex models to elucidate the factors influencing their outputs, thus fostering transparency and accountability.
2) Visualising neural networks: Transforming abstract neural network structures into visual representations aids in comprehending their functionality and behaviour.
3) Rule-based systems: Augmenting AI decision-making with interpretable, rule-based systems holds promise in domains requiring logical explanations for actions taken.
Generative Adversarial Networks (GANs)
The captivating world of Generative Adversarial Networks (GANs) unfolds through the interplay of generator and discriminator networks, birthing remarkable research avenues:
1) Image generation: Crafting realistic images from random noise showcases the creative potential of GANs, with applications spanning art, design, and data augmentation.
2) Style transfer: Enabling the transfer of artistic styles between images, merging creativity and technology to yield visually captivating results.
3) Anomaly detection: GANs find utility in identifying anomalies within datasets, bolstering fraud detection, quality control, and anomaly-sensitive industries.
Robotics and AI
The synergy between Robotics and AI is a fertile ground for exploration, with Artificial Intelligence Topics such as:
1) Human-robot collaboration: Research in this arena strives to establish harmonious collaboration between humans and robots, augmenting industry productivity and efficiency.
2) Robot learning: By enabling robots to learn and adapt from their experiences, Researchers foster robots' autonomy and the ability to handle diverse tasks.
3) Ethical considerations: Delving into the ethical implications surrounding AI-powered robots helps establish responsible guidelines for their deployment.
AI in healthcare
AI presents a transformative potential within healthcare, spurring research into:
1) Medical diagnosis: AI aids in accurately diagnosing medical conditions, revolutionising early detection and patient care.
2) Drug discovery: Leveraging AI for drug discovery expedites the identification of potential candidates, accelerating the development of new treatments.
3) Personalised treatment: Tailoring medical interventions to individual patient profiles enhances treatment outcomes and patient well-being.
AI for social good
Harnessing the prowess of AI for Social Good entails addressing pressing global challenges:
1) Environmental monitoring: AI-powered solutions facilitate real-time monitoring of ecological changes, supporting conservation and sustainable practices.
2) Disaster response: Research in this area bolsters disaster response efforts by employing AI to analyse data and optimise resource allocation.
3) Poverty alleviation: Researchers contribute to humanitarian efforts and socioeconomic equality by devising AI solutions to tackle poverty.
Unlock the potential of Artificial Intelligence for effective Project Management with our Artificial Intelligence (AI) for Project Managers Course . Sign up now!
Autonomous vehicles
Autonomous Vehicles represent a realm brimming with potential and complexities, necessitating research in Artificial Intelligence Topics such as:
1) Sensor fusion: Integrating data from diverse sensors enhances perception accuracy, which is essential for safe autonomous navigation.
2) Path planning: Developing advanced algorithms for path planning ensures optimal routes while adhering to safety protocols.
3) Safety and ethics: Ethical considerations, such as programming vehicles to make difficult decisions in potential accident scenarios, require meticulous research and deliberation.
AI ethics and bias
Ethical underpinnings in AI drive research efforts in these directions:
1) Fairness in AI: Ensuring AI systems remain impartial and unbiased across diverse demographic groups.
2) Bias detection and mitigation: Identifying and rectifying biases present within AI models guarantees equitable outcomes.
3) Ethical decision-making: Developing frameworks that imbue AI with ethical decision-making capabilities aligns technology with societal values.
Future of AI
The vanguard of AI beckons Researchers to explore these horizons:
1) Artificial General Intelligence (AGI): Speculating on the potential emergence of AI systems capable of emulating human-like intelligence opens dialogues on the implications and challenges.
2) AI and creativity: Probing the interface between AI and creative domains, such as art and music, unveils the coalescence of human ingenuity and technological prowess.
3) Ethical and regulatory challenges: Researching the ethical dilemmas and regulatory frameworks underpinning AI's evolution fortifies responsible innovation.
AI and education
The intersection of AI and Education opens doors to innovative learning paradigms:
1) Personalised learning: Developing AI systems that adapt educational content to individual learning styles and paces.
2) Intelligent tutoring systems: Creating AI-driven tutoring systems that provide targeted support to students.
3) Educational data mining: Applying AI to analyse educational data for insights into learning patterns and trends.
Unleash the full potential of AI with our comprehensive Introduction to Artificial Intelligence Training . Join now!
Conclusion
The domain of AI is ever-expanding, rich with intriguing topics about Artificial Intelligence that beckon Researchers to explore, question, and innovate. Through the pursuit of these twelve diverse Artificial Intelligence Topics, we pave the way for not only technological advancement but also a deeper understanding of the societal impact of AI. By delving into these realms, Researchers stand poised to shape the trajectory of AI, ensuring it remains a force for progress, empowerment, and positive transformation in our world.
Unlock your full potential with our extensive Personal Development Training Courses. Join today!
Frequently Asked Questions
Upcoming data, analytics & ai resources batches & dates.
Fri 26th Apr 2024
Fri 2nd Aug 2024
Fri 15th Nov 2024
Get A Quote
WHO WILL BE FUNDING THE COURSE?
My employer
By submitting your details you agree to be contacted in order to respond to your enquiry
- Business Analysis
- Lean Six Sigma Certification
Share this course
Our biggest spring sale.

We cannot process your enquiry without contacting you, please tick to confirm your consent to us for contacting you about your enquiry.
By submitting your details you agree to be contacted in order to respond to your enquiry.
We may not have the course you’re looking for. If you enquire or give us a call on 01344203999 and speak to our training experts, we may still be able to help with your training requirements.
Or select from our popular topics
- ITIL® Certification
- Scrum Certification
- Change Management Certification
- Business Analysis Courses
- Microsoft Azure Certification
- Microsoft Excel & Certification Course
- Microsoft Project
- Explore more courses
Press esc to close
Fill out your contact details below and our training experts will be in touch.
Fill out your contact details below
Thank you for your enquiry!
One of our training experts will be in touch shortly to go over your training requirements.
Back to Course Information
Fill out your contact details below so we can get in touch with you regarding your training requirements.
* WHO WILL BE FUNDING THE COURSE?
Preferred Contact Method
No preference
Back to course information
Fill out your training details below
Fill out your training details below so we have a better idea of what your training requirements are.
HOW MANY DELEGATES NEED TRAINING?
HOW DO YOU WANT THE COURSE DELIVERED?
Online Instructor-led
Online Self-paced
WHEN WOULD YOU LIKE TO TAKE THIS COURSE?
Next 2 - 4 months
WHAT IS YOUR REASON FOR ENQUIRING?
Looking for some information
Looking for a discount
I want to book but have questions
One of our training experts will be in touch shortly to go overy your training requirements.
Your privacy & cookies!
Like many websites we use cookies. We care about your data and experience, so to give you the best possible experience using our site, we store a very limited amount of your data. Continuing to use this site or clicking “Accept & close” means that you agree to our use of cookies. Learn more about our privacy policy and cookie policy cookie policy .
We use cookies that are essential for our site to work. Please visit our cookie policy for more information. To accept all cookies click 'Accept & close'.
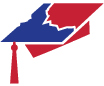
Want to create or adapt books like this? Learn more about how Pressbooks supports open publishing practices.
Writing and Artificial Intelligence
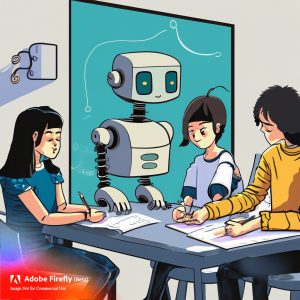
We’re including this guide to “Writing with AI” in Write What Matters because it’s clear that generative AI tools, including platforms such as ChatGPT, are beginning to transform what writing instruction looks like in higher education. As this emerging technology continues to reshape what it means to practice writing, there’s no real consensus on how these tools should be used—or whether they should be used at all. Some students and faculty actively avoid generative AI in the classroom. Others are not yet familiar with the wide range of tools and capabilities available to students and instructors. And some are embracing these new tools and actively experimenting with them.
Regardless of how you are currently using or not using AI in your classroom, all of us are feeling the impact.
What this guide does not do
This guide does not offer suggestions for how students or educators should use large language models (LLMs)—such as ChatGPT, Google Bard, Microsoft Bing, or Claude’s Anthropic—in their writing process. As faculty ourselves, we understand the need to slow down and consider new tools critically. A deliberate and critical approach to generative AI is particularly important, since tools such as ChatGTP struggle with accuracy and hallucination, foster bias and censorship, and can easily become a substitute for thinking.
At this moment, most colleges are developing guidance or policies around AI use in the classroom. In addition, instructors may have careful wording in their course syllabus about what constitutes acceptable vs. non-acceptable uses of AI. Students should become familiar with their institution’s and instructor’s AI policies as they navigate the AI landscape.
For example, at the College of Western Idaho, school syllabi now include the following language:
Practicing academic integrity includes, but is not limited to, non-participation in the following behaviors: cheating, plagiarism, falsifying information, unauthorized collaboration, facilitating academic dishonesty, collusion with another person or entity to cheat, submission of work created by artificial intelligence tools as one’s own work, and violation of program policies and procedures.
Departments, instructors, and students will need to collectively decide how their specific “writing with AI” practices relate to this broad policy. For example, how should Quillbot’s paraphrasing and co-writing capabilities be classified? Should students be allowed to use Grammarly (or even Word’s build in grammar checker) to correct their grammar and syntax?
What this guide aims to do
The purpose of this guide is to offer an accessible introduction to writing with AI for dual enrollment and first-year college students. In the following chapters, students will:
- understand how large language models (LLMs) such as ChatGPT are trained to generate text;
- understand the limitations, risks, and ethical considerations associated LLMs;
- become acquainted with the range of AI platforms and applications that can assist writing;
- better understand how to prompt LLM chatbots such as ChatGPT;
- become familiar with how to cite and acknowledge the use of generative AI in the classroom.
Because this information is being presented to students within the context of a writing textbook, faculty who adapt this information may want to add to or revise this content so that it better fits their own academic discipline.
Updates to this textbook section
The practice of working and writing with AI is evolving rapidly. As soon as this section is published, it will be somewhat outdated. The affordance of OER, however, allows us to update this textbook more frequently than a traditional textbook. We intend to regularly maintain this section and will update as needed.
Write What Matters Copyright © 2020 by Liza Long; Amy Minervini; and Joel Gladd is licensed under a Creative Commons Attribution-NonCommercial-ShareAlike 4.0 International License , except where otherwise noted.
Share This Book
How can AI help us become better writers?
In this lesson, students compare AI and human-generated texts to analyze the affordances and limitations of large language models. They’ll score, guess origins, and discuss differences, then decide which aspects to adopt or avoid in their writing. The lesson culminates in a discussion of what aspects of the AI-generated writing students should emulate in their own writing and what aspects they should avoid.
- AI & Writing
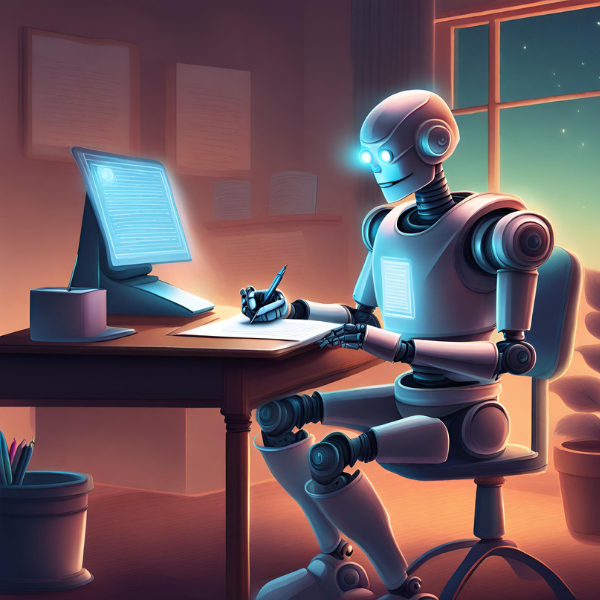
Digital Materials
- Teacher guide
- Student worksheet
- How Can AI Help Us Become Better Writers?_ Slides
- How Can AI Help Us Become Better Writers_Model Texts_Teacher Version
- How Can AI Help Us Become Better Writers_Model Texts_Student Version
After this experience, students will be able to
- Evaluate model texts using a rubric.
- Hypothesize which texts are AI-generated using evidence to justify their position.
- Identify the strengths and weaknesses of AI-generated texts in order to reflect on how they can improve their own writing.
Questions explored
- How can we distinguish AI-generated text from human writing?
- What are the strengths of AI-generated text? What are the weaknesses?
- What elements are present in human writing that are not present in AI writing and vice versa?
- How can we apply these learnings to our own writing?

- LibGuides
- A-Z List
- Help
Artificial Intelligence
- Background Information
- Getting started
- Browse Journals
- Dissertations & Theses
- Datasets and Repositories
- Research Data Management 101
- Scientific Writing
- Find Videos
- Related Topics
- Quick Links
- Ask Us/Contact Us
FIU dissertations

Non-FIU dissertations
Many universities provide full-text access to their dissertations via a digital repository. If you know the title of a particular dissertation or thesis, try doing a Google search.
Aims to be the best possible resource for finding open access graduate theses and dissertations published around the world with metadata from over 800 colleges, universities, and research institutions. Currently, indexes over 1 million theses and dissertations.
This is a discovery service for open access research theses awarded by European universities.
A union catalog of Canadian theses and dissertations, in both electronic and analog formats, is available through the search interface on this portal.
There are currently more than 90 countries and over 1200 institutions represented. CRL has catalog records for over 800,000 foreign doctoral dissertations.
An international collaborative resource, the NDLTD Union Catalog contains more than one million records of electronic theses and dissertations. Use BASE, the VTLS Visualizer or any of the geographically specific search engines noted lower on their webpage.
Indexes doctoral dissertations and masters' theses in all areas of academic research includes international coverage.
ProQuest Dissertations & Theses global
Related Sites

- << Previous: Browse Journals
- Next: Datasets and Repositories >>
- Last Updated: Apr 4, 2024 8:33 AM
- URL: https://library.fiu.edu/artificial-intelligence
Information
Fiu libraries floorplans, green library, modesto a. maidique campus, hubert library, biscayne bay campus.

Directions: Green Library, MMC
Directions: Hubert Library, BBC
- Search for: Toggle Search
NVIDIA Hopper Leaps Ahead in Generative AI at MLPerf
It’s official: NVIDIA delivered the world’s fastest platform in industry-standard tests for inference on generative AI .
In the latest MLPerf benchmarks, NVIDIA TensorRT-LLM — software that speeds and simplifies the complex job of inference on large language models — boosted the performance of NVIDIA Hopper architecture GPUs on the GPT-J LLM nearly 3x over their results just six months ago.
The dramatic speedup demonstrates the power of NVIDIA’s full-stack platform of chips, systems and software to handle the demanding requirements of running generative AI.
Leading companies are using TensorRT-LLM to optimize their models. And NVIDIA NIM — a set of inference microservices that includes inferencing engines like TensorRT-LLM — makes it easier than ever for businesses to deploy NVIDIA’s inference platform.
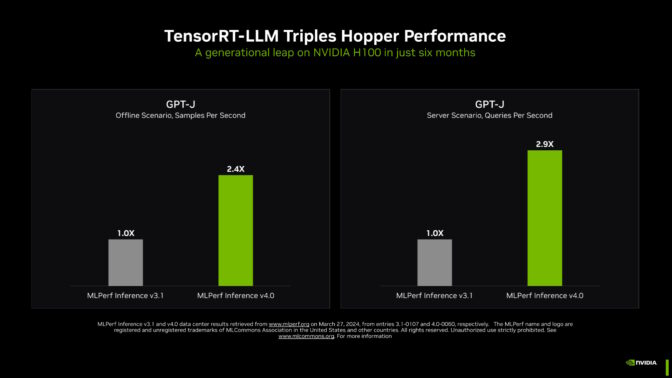
Raising the Bar in Generative AI
TensorRT-LLM running on NVIDIA H200 Tensor Core GPUs — the latest, memory-enhanced Hopper GPUs — delivered the fastest performance running inference in MLPerf’s biggest test of generative AI to date.
The new benchmark uses the largest version of Llama 2, a state-of-the-art large language model packing 70 billion parameters. The model is more than 10x larger than the GPT-J LLM first used in the September benchmarks .
The memory-enhanced H200 GPUs, in their MLPerf debut, used TensorRT-LLM to produce up to 31,000 tokens/second, a record on MLPerf’s Llama 2 benchmark.
The H200 GPU results include up to 14% gains from a custom thermal solution. It’s one example of innovations beyond standard air cooling that systems builders are applying to their NVIDIA MGX designs to take the performance of Hopper GPUs to new heights.
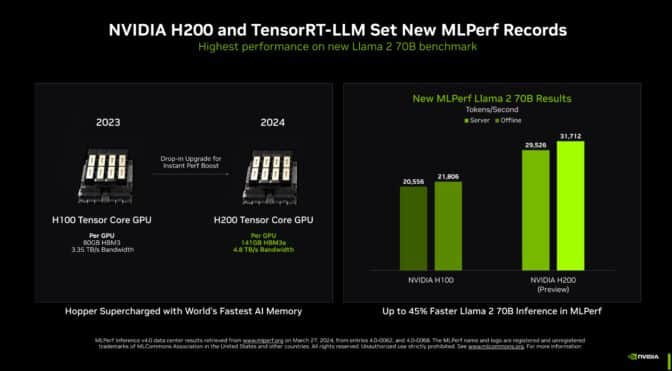
Memory Boost for NVIDIA Hopper GPUs
NVIDIA is sampling H200 GPUs to customers today and shipping in the second quarter. They’ll be available soon from nearly 20 leading system builders and cloud service providers.
H200 GPUs pack 141GB of HBM3e running at 4.8TB/s. That’s 76% more memory flying 43% faster compared to H100 GPUs. These accelerators plug into the same boards and systems and use the same software as H100 GPUs.
With HBM3e memory, a single H200 GPU can run an entire Llama 2 70B model with the highest throughput, simplifying and speeding inference.
GH200 Packs Even More Memory
Even more memory — up to 624GB of fast memory, including 144GB of HBM3e — is packed in NVIDIA GH200 Superchips , which combine on one module a Hopper architecture GPU and a power-efficient NVIDIA Grace CPU . NVIDIA accelerators are the first to use HBM3e memory technology.
With nearly 5 TB/second memory bandwidth, GH200 Superchips delivered standout performance, including on memory-intensive MLPerf tests such as recommender systems .
Sweeping Every MLPerf Test
On a per-accelerator basis, Hopper GPUs swept every test of AI inference in the latest round of the MLPerf industry benchmarks.
In addition, NVIDIA Jetson Orin remains at the forefront in MLPerf’s edge category. In the last two inference rounds, Orin ran the most diverse set of models in the category, including GPT-J and Stable Diffusion XL.
The MLPerf benchmarks cover today’s most popular AI workloads and scenarios, including generative AI, recommendation systems, natural language processing, speech and computer vision. NVIDIA was the only company to submit results on every workload in the latest round and every round since MLPerf’s data center inference benchmarks began in October 2020.
Continued performance gains translate into lower costs for inference, a large and growing part of the daily work for the millions of NVIDIA GPUs deployed worldwide.
Advancing What’s Possible
Pushing the boundaries of what’s possible, NVIDIA demonstrated three innovative techniques in a special section of the benchmarks called the open division, created for testing advanced AI methods.
NVIDIA engineers used a technique called structured sparsity — a way of reducing calculations, first introduced with NVIDIA A100 Tensor Core GPUs — to deliver up to 33% speedups on inference with Llama 2.
A second open division test found inference speedups of up to 40% using pruning, a way of simplifying an AI model — in this case, an LLM — to increase inference throughput.
Finally, an optimization called DeepCache reduced the math required for inference with the Stable Diffusion XL model, accelerating performance by a whopping 74%.
All these results were run on NVIDIA H100 Tensor Core GPUs .
A Trusted Source for Users
MLPerf’s tests are transparent and objective, so users can rely on the results to make informed buying decisions.
NVIDIA’s partners participate in MLPerf because they know it’s a valuable tool for customers evaluating AI systems and services. Partners submitting results on the NVIDIA AI platform in this round included ASUS, Cisco, Dell Technologies, Fujitsu, GIGABYTE, Google, Hewlett Packard Enterprise, Lenovo, Microsoft Azure, Oracle, QCT, Supermicro, VMware (recently acquired by Broadcom) and Wiwynn.
All the software NVIDIA used in the tests is available in the MLPerf repository. These optimizations are continuously folded into containers available on NGC , NVIDIA’s software hub for GPU applications, as well as NVIDIA AI Enterprise — a secure, supported platform that includes NIM inference microservices.
The Next Big Thing
The use cases, model sizes and datasets for generative AI continue to expand. That’s why MLPerf continues to evolve, adding real-world tests with popular models like Llama 2 70B and Stable Diffusion XL.
Keeping pace with the explosion in LLM model sizes, NVIDIA founder and CEO Jensen Huang announced last week at GTC that the NVIDIA Blackwell architecture GPUs will deliver new levels of performance required for the multitrillion-parameter AI models.
Inference for large language models is difficult, requiring both expertise and the full-stack architecture NVIDIA demonstrated on MLPerf with Hopper architecture GPUs and TensorRT-LLM. There’s much more to come.
Learn more about MLPerf benchmarks and the technical details of this inference round.
NVIDIA websites use cookies to deliver and improve the website experience. See our cookie policy for further details on how we use cookies and how to change your cookie settings.
Share on Mastodon

Will A.I. Boost Productivity? Companies Sure Hope So.
Economists doubt that artificial intelligence is already visible in productivity data. Big companies, however, talk often about adopting it to improve efficiency.
Credit... Shira Inbar
Supported by
- Share full article

By Jordyn Holman and Jeanna Smialek
- April 1, 2024
Wendy’s menu boards. Ben & Jerry’s grocery store freezers. Abercrombie & Fitch’s marketing. Many mainstays of the American customer experience are increasingly powered by artificial intelligence.
The question is whether the technology will actually make companies more efficient.
Rapid productivity improvement is the dream for both companies and economic policymakers. If output per hour holds steady, firms must either sacrifice profits or raise prices to pay for wage increases or investment projects. But when firms figure out how to produce more per working hour, it means that they can maintain or expand profits even as they pay or invest more. Economies experiencing productivity booms can experience rapid wage gains and quick growth without as much risk of rapid inflation.
But many economists and officials seem dubious that A.I. — especially generative A.I., which is still in its infancy — has spread enough to show up in productivity data already.
Jerome H. Powell, the Federal Reserve chair, recently suggested that A.I. “may” have the potential to increase productivity growth, “but probably not in the short run.” John C. Williams, president of the New York Fed, has made similar remarks, specifically citing the work of the Northwestern University economist Robert Gordon.
Mr. Gordon has argued that new technologies in recent years, while important, have probably not been transformative enough to give a lasting lift to productivity growth.
“The enthusiasm about large language models and ChatGPT has gone a bit overboard,” he said in an interview.
The last time productivity really picked up, in the 1990s, computer manufacturing was getting a lot more efficient at the same time that computers themselves were making everything else more efficient — allowing for a sector-spanning productivity increase. Today’s gains may be less broad, he thinks.
Other economists are more optimistic. Erik Brynjolfsson at Stanford University has bet Mr. Gordon $400 that productivity will take off this decade. His optimism is based partly on A.I. He ran an experiment with it at a large call center , where it especially helped less experienced workers, and has co-founded a company meant to teach firms how to leverage the technology.
Many companies seem to be in Mr. Brynjolfsson’s camp, hopeful that the shiny new tool will revolutionize their workplaces. Companies are using A.I. and generative A.I. for everything from writing marketing emails to helping set prices to answering employees’ human resources and legal questions.
Here are a few areas where companies say the latest A.I. technology is being used in ways that could influence productivity, pulled from interviews, earnings calls and financial filings.
Got an annoying task? There’s an A.I. for that.
Employees spend a lot of time trying to figure out human-resources-related questions. Companies have been investing in generative A.I. to help answer those queries more quickly.
At Walmart , the largest retailer in the United States, with 1.6 million workers, the company’s employee app has a section called “My Assistant,” which is backed by generative A.I. The feature uses the technology to quickly answer questions like “Do I have dental coverage?,” summarize meeting notes and help write job descriptions.
Walmart rolled out the technology to its U.S. corporate work force last year.
The retailer has been clear that the tool is meant to boost productivity. In an interview last year, Donna Morris, Walmart’s chief people officer, said one of the goals was to eliminate some mundane work so employees could focus on tasks that had more impact. It’s expected to be a “huge productivity lift” for the company, she said.
The algorithms want to sell you things.
Tony Spring, Macy’s chief executive, said the department-store chain was experimenting with A.I. to tailor its marketing. The company is using generative A.I. to write elements of emails, and is exploring ways to use the technology to add product descriptions online and to replicate images of outfits or other products for sale over new backgrounds.
“It’s certainly showing up as a tool for some colleagues to reduce workload,” Mr. Spring said in an interview.
Abercrombie & Fitch is using generative A.I. to help design clothes and write descriptions for its website and app. Designers use Midjourney, an A.I. graphics program, to help them generate images as they brainstorm clothing ideas. Workers in Abercrombie’s marketing department also use generative A.I. to help write the blurbs for products’ descriptions. (Employees later edit the copy.)
Samir Desai, Abercrombie & Fitch’s chief digital officer, said the technology helped speed up a laborious process, given that Abercrombie and its brands could post a couple of hundred new products on its website in a single week.
“I think right now it’s a lot of trust and belief that these are productivity enhancers, efficiency boosters,” Mr. Desai said, noting that it was difficult to quantify how much time and money was being saved. “I think we’ll start to see that manifest itself in just how much work certain teams are able to get through versus the prior years.”
A.I. pairs well with burgers and ice cream.
Some companies are hoping to use the latest A.I. technology to help match prices to demand, somewhat like the way that Uber sets prices for cars based on how many people want to ride.
Wendy’s, for instance, has floated the idea of using A.I. to identify slower times of the day and discount the prices of menu items on its digital boards.
The technology could also help with inventory management. Ben & Jerry’s put cameras that use A.I. into the freezers at grocery stores to help alert the company when a location was running low on pints of Cherry Garcia or Chunky Monkey. The camera sporadically captures an image of the freezer shelves, and the technology assesses the quantity that’s left, sending alerts to Ben & Jerry’s parent company and its distributors.
“The software identifies what is about to run out and also helps plan the most efficient routes for trucks that can restock the inventory,” Catherine Reynolds, a spokeswoman for Unilever, the parent of Ben & Jerry’s , said in a statement.
The A.I. technology is installed in 8,000 freezers, and the company said it planned to significantly increase that number this year. On average, freezers with the A.I. technology increased sales 13 percent because they were replenished with fresh pints of ice cream, particularly the most in-demand flavors, Ms. Reynolds said.
A.I. is getting into the weeds.
Deere, the maker of farm equipment, has been using A.I. alongside cameras to improve herbicide sprayers . The equipment recognizes and targets weeds specifically, allowing for more precise use of chemicals. The technology was introduced in 2022, and the company estimates that it covered 100 million acres and saved eight million gallons of herbicide last year.
The technology can allow “customers to reduce their herbicide use, lower their costs and minimize impact on their crops and land,” John C. May II, the firm’s chief executive, said at a news conference in February.
Are these game-changing improvements?
Skepticism of A.I.’s potential for major change is based largely on the fact that many of its applications mimic things software can already do: There are clear improvements, but not necessarily game-changing ones.
But while it could take time for companies to fully harness A.I. tools, the fact that the applications are potentially so broad has made some economists optimistic about what the new technologies could mean for productivity growth.
Analysts at Vanguard think that A.I. could be “transformative” to the U.S. economy in the second half of the 2020s, said Joseph Davis, the financial firm’s global chief economist. He said the technology could save workers meaningful time — perhaps 20 percent — in about 80 percent of occupations.
“We’re not seeing it in the data yet,” he said, explaining that he thinks that a recent pickup in productivity has been more of a snapback from a steep drop-off during the pandemic. “The good news is that there’s another wave coming.”
Jordyn Holman is a business reporter for The Times, covering the retail industry and consumer behavior. More about Jordyn Holman
Jeanna Smialek covers the Federal Reserve and the economy for The Times from Washington. More about Jeanna Smialek
Explore Our Coverage of Artificial Intelligence
News and Analysis
Artificial intelligence is peering into restaurant garbage pails and crunching grocery-store data to try to figure out how to send less uneaten food into dumpsters.
David Autor, an M.I.T. economist and tech skeptic, argues that A.I. is fundamentally different from past waves of computerization.
Economists doubt that artificial intelligence is already visible in productivity data . Big companies, however, talk often about adopting it to improve efficiency.
OpenAI unveiled Voice Engine , an A.I. technology that can recreate a person’s voice from a 15-second recording.
Amazon said it had added $2.75 billion to its investment in Anthropic , an A.I. start-up that competes with companies like OpenAI and Google.
Gov. Bill Lee of Tennessee signed a bill to prevent the use of A.I. to copy a performer’s voice. It is the first such measure in the United States.
Advertisement
Thank you for visiting nature.com. You are using a browser version with limited support for CSS. To obtain the best experience, we recommend you use a more up to date browser (or turn off compatibility mode in Internet Explorer). In the meantime, to ensure continued support, we are displaying the site without styles and JavaScript.
- View all journals
- My Account Login
- Explore content
- About the journal
- Publish with us
- Sign up for alerts
- Open access
- Published: 26 March 2024
Predicting and improving complex beer flavor through machine learning
- Michiel Schreurs ORCID: orcid.org/0000-0002-9449-5619 1 , 2 , 3 na1 ,
- Supinya Piampongsant 1 , 2 , 3 na1 ,
- Miguel Roncoroni ORCID: orcid.org/0000-0001-7461-1427 1 , 2 , 3 na1 ,
- Lloyd Cool ORCID: orcid.org/0000-0001-9936-3124 1 , 2 , 3 , 4 ,
- Beatriz Herrera-Malaver ORCID: orcid.org/0000-0002-5096-9974 1 , 2 , 3 ,
- Christophe Vanderaa ORCID: orcid.org/0000-0001-7443-5427 4 ,
- Florian A. Theßeling 1 , 2 , 3 ,
- Łukasz Kreft ORCID: orcid.org/0000-0001-7620-4657 5 ,
- Alexander Botzki ORCID: orcid.org/0000-0001-6691-4233 5 ,
- Philippe Malcorps 6 ,
- Luk Daenen 6 ,
- Tom Wenseleers ORCID: orcid.org/0000-0002-1434-861X 4 &
- Kevin J. Verstrepen ORCID: orcid.org/0000-0002-3077-6219 1 , 2 , 3
Nature Communications volume 15 , Article number: 2368 ( 2024 ) Cite this article
50k Accesses
851 Altmetric
Metrics details
- Chemical engineering
- Gas chromatography
- Machine learning
- Metabolomics
- Taste receptors
The perception and appreciation of food flavor depends on many interacting chemical compounds and external factors, and therefore proves challenging to understand and predict. Here, we combine extensive chemical and sensory analyses of 250 different beers to train machine learning models that allow predicting flavor and consumer appreciation. For each beer, we measure over 200 chemical properties, perform quantitative descriptive sensory analysis with a trained tasting panel and map data from over 180,000 consumer reviews to train 10 different machine learning models. The best-performing algorithm, Gradient Boosting, yields models that significantly outperform predictions based on conventional statistics and accurately predict complex food features and consumer appreciation from chemical profiles. Model dissection allows identifying specific and unexpected compounds as drivers of beer flavor and appreciation. Adding these compounds results in variants of commercial alcoholic and non-alcoholic beers with improved consumer appreciation. Together, our study reveals how big data and machine learning uncover complex links between food chemistry, flavor and consumer perception, and lays the foundation to develop novel, tailored foods with superior flavors.
Similar content being viewed by others
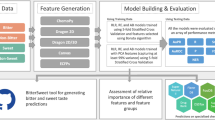
BitterSweet: Building machine learning models for predicting the bitter and sweet taste of small molecules
Rudraksh Tuwani, Somin Wadhwa & Ganesh Bagler
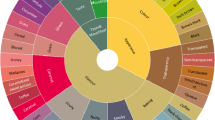
Sensory lexicon and aroma volatiles analysis of brewing malt
Xiaoxia Su, Miao Yu, … Tianyi Du
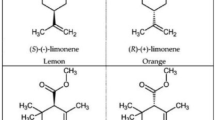
Predicting odor from molecular structure: a multi-label classification approach
Kushagra Saini & Venkatnarayan Ramanathan
Introduction
Predicting and understanding food perception and appreciation is one of the major challenges in food science. Accurate modeling of food flavor and appreciation could yield important opportunities for both producers and consumers, including quality control, product fingerprinting, counterfeit detection, spoilage detection, and the development of new products and product combinations (food pairing) 1 , 2 , 3 , 4 , 5 , 6 . Accurate models for flavor and consumer appreciation would contribute greatly to our scientific understanding of how humans perceive and appreciate flavor. Moreover, accurate predictive models would also facilitate and standardize existing food assessment methods and could supplement or replace assessments by trained and consumer tasting panels, which are variable, expensive and time-consuming 7 , 8 , 9 . Lastly, apart from providing objective, quantitative, accurate and contextual information that can help producers, models can also guide consumers in understanding their personal preferences 10 .
Despite the myriad of applications, predicting food flavor and appreciation from its chemical properties remains a largely elusive goal in sensory science, especially for complex food and beverages 11 , 12 . A key obstacle is the immense number of flavor-active chemicals underlying food flavor. Flavor compounds can vary widely in chemical structure and concentration, making them technically challenging and labor-intensive to quantify, even in the face of innovations in metabolomics, such as non-targeted metabolic fingerprinting 13 , 14 . Moreover, sensory analysis is perhaps even more complicated. Flavor perception is highly complex, resulting from hundreds of different molecules interacting at the physiochemical and sensorial level. Sensory perception is often non-linear, characterized by complex and concentration-dependent synergistic and antagonistic effects 15 , 16 , 17 , 18 , 19 , 20 , 21 that are further convoluted by the genetics, environment, culture and psychology of consumers 22 , 23 , 24 . Perceived flavor is therefore difficult to measure, with problems of sensitivity, accuracy, and reproducibility that can only be resolved by gathering sufficiently large datasets 25 . Trained tasting panels are considered the prime source of quality sensory data, but require meticulous training, are low throughput and high cost. Public databases containing consumer reviews of food products could provide a valuable alternative, especially for studying appreciation scores, which do not require formal training 25 . Public databases offer the advantage of amassing large amounts of data, increasing the statistical power to identify potential drivers of appreciation. However, public datasets suffer from biases, including a bias in the volunteers that contribute to the database, as well as confounding factors such as price, cult status and psychological conformity towards previous ratings of the product.
Classical multivariate statistics and machine learning methods have been used to predict flavor of specific compounds by, for example, linking structural properties of a compound to its potential biological activities or linking concentrations of specific compounds to sensory profiles 1 , 26 . Importantly, most previous studies focused on predicting organoleptic properties of single compounds (often based on their chemical structure) 27 , 28 , 29 , 30 , 31 , 32 , 33 , thus ignoring the fact that these compounds are present in a complex matrix in food or beverages and excluding complex interactions between compounds. Moreover, the classical statistics commonly used in sensory science 34 , 35 , 36 , 37 , 38 , 39 require a large sample size and sufficient variance amongst predictors to create accurate models. They are not fit for studying an extensive set of hundreds of interacting flavor compounds, since they are sensitive to outliers, have a high tendency to overfit and are less suited for non-linear and discontinuous relationships 40 .
In this study, we combine extensive chemical analyses and sensory data of a set of different commercial beers with machine learning approaches to develop models that predict taste, smell, mouthfeel and appreciation from compound concentrations. Beer is particularly suited to model the relationship between chemistry, flavor and appreciation. First, beer is a complex product, consisting of thousands of flavor compounds that partake in complex sensory interactions 41 , 42 , 43 . This chemical diversity arises from the raw materials (malt, yeast, hops, water and spices) and biochemical conversions during the brewing process (kilning, mashing, boiling, fermentation, maturation and aging) 44 , 45 . Second, the advent of the internet saw beer consumers embrace online review platforms, such as RateBeer (ZX Ventures, Anheuser-Busch InBev SA/NV) and BeerAdvocate (Next Glass, inc.). In this way, the beer community provides massive data sets of beer flavor and appreciation scores, creating extraordinarily large sensory databases to complement the analyses of our professional sensory panel. Specifically, we characterize over 200 chemical properties of 250 commercial beers, spread across 22 beer styles, and link these to the descriptive sensory profiling data of a 16-person in-house trained tasting panel and data acquired from over 180,000 public consumer reviews. These unique and extensive datasets enable us to train a suite of machine learning models to predict flavor and appreciation from a beer’s chemical profile. Dissection of the best-performing models allows us to pinpoint specific compounds as potential drivers of beer flavor and appreciation. Follow-up experiments confirm the importance of these compounds and ultimately allow us to significantly improve the flavor and appreciation of selected commercial beers. Together, our study represents a significant step towards understanding complex flavors and reinforces the value of machine learning to develop and refine complex foods. In this way, it represents a stepping stone for further computer-aided food engineering applications 46 .
To generate a comprehensive dataset on beer flavor, we selected 250 commercial Belgian beers across 22 different beer styles (Supplementary Fig. S1 ). Beers with ≤ 4.2% alcohol by volume (ABV) were classified as non-alcoholic and low-alcoholic. Blonds and Tripels constitute a significant portion of the dataset (12.4% and 11.2%, respectively) reflecting their presence on the Belgian beer market and the heterogeneity of beers within these styles. By contrast, lager beers are less diverse and dominated by a handful of brands. Rare styles such as Brut or Faro make up only a small fraction of the dataset (2% and 1%, respectively) because fewer of these beers are produced and because they are dominated by distinct characteristics in terms of flavor and chemical composition.
Extensive analysis identifies relationships between chemical compounds in beer
For each beer, we measured 226 different chemical properties, including common brewing parameters such as alcohol content, iso-alpha acids, pH, sugar concentration 47 , and over 200 flavor compounds (Methods, Supplementary Table S1 ). A large portion (37.2%) are terpenoids arising from hopping, responsible for herbal and fruity flavors 16 , 48 . A second major category are yeast metabolites, such as esters and alcohols, that result in fruity and solvent notes 48 , 49 , 50 . Other measured compounds are primarily derived from malt, or other microbes such as non- Saccharomyces yeasts and bacteria (‘wild flora’). Compounds that arise from spices or staling are labeled under ‘Others’. Five attributes (caloric value, total acids and total ester, hop aroma and sulfur compounds) are calculated from multiple individually measured compounds.
As a first step in identifying relationships between chemical properties, we determined correlations between the concentrations of the compounds (Fig. 1 , upper panel, Supplementary Data 1 and 2 , and Supplementary Fig. S2 . For the sake of clarity, only a subset of the measured compounds is shown in Fig. 1 ). Compounds of the same origin typically show a positive correlation, while absence of correlation hints at parameters varying independently. For example, the hop aroma compounds citronellol, and alpha-terpineol show moderate correlations with each other (Spearman’s rho=0.39 and 0.57), but not with the bittering hop component iso-alpha acids (Spearman’s rho=0.16 and −0.07). This illustrates how brewers can independently modify hop aroma and bitterness by selecting hop varieties and dosage time. If hops are added early in the boiling phase, chemical conversions increase bitterness while aromas evaporate, conversely, late addition of hops preserves aroma but limits bitterness 51 . Similarly, hop-derived iso-alpha acids show a strong anti-correlation with lactic acid and acetic acid, likely reflecting growth inhibition of lactic acid and acetic acid bacteria, or the consequent use of fewer hops in sour beer styles, such as West Flanders ales and Fruit beers, that rely on these bacteria for their distinct flavors 52 . Finally, yeast-derived esters (ethyl acetate, ethyl decanoate, ethyl hexanoate, ethyl octanoate) and alcohols (ethanol, isoamyl alcohol, isobutanol, and glycerol), correlate with Spearman coefficients above 0.5, suggesting that these secondary metabolites are correlated with the yeast genetic background and/or fermentation parameters and may be difficult to influence individually, although the choice of yeast strain may offer some control 53 .
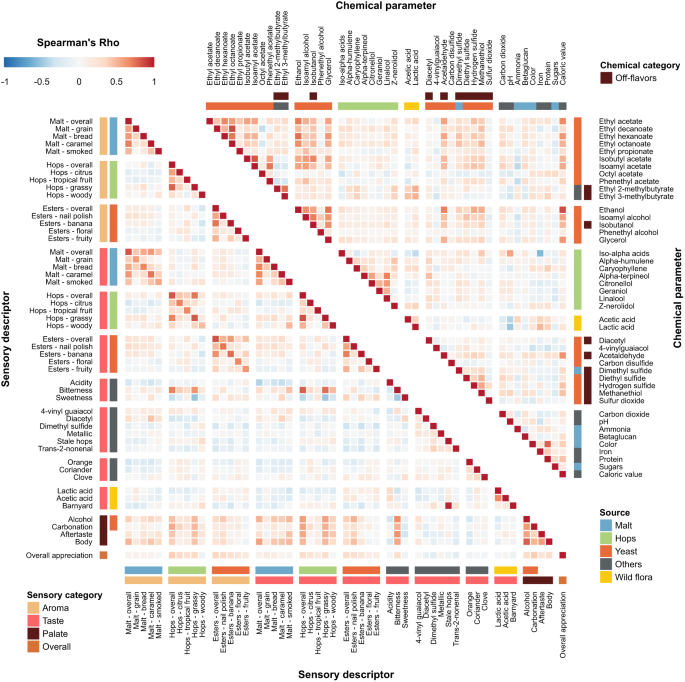
Spearman rank correlations are shown. Descriptors are grouped according to their origin (malt (blue), hops (green), yeast (red), wild flora (yellow), Others (black)), and sensory aspect (aroma, taste, palate, and overall appreciation). Please note that for the chemical compounds, for the sake of clarity, only a subset of the total number of measured compounds is shown, with an emphasis on the key compounds for each source. For more details, see the main text and Methods section. Chemical data can be found in Supplementary Data 1 , correlations between all chemical compounds are depicted in Supplementary Fig. S2 and correlation values can be found in Supplementary Data 2 . See Supplementary Data 4 for sensory panel assessments and Supplementary Data 5 for correlation values between all sensory descriptors.
Interestingly, different beer styles show distinct patterns for some flavor compounds (Supplementary Fig. S3 ). These observations agree with expectations for key beer styles, and serve as a control for our measurements. For instance, Stouts generally show high values for color (darker), while hoppy beers contain elevated levels of iso-alpha acids, compounds associated with bitter hop taste. Acetic and lactic acid are not prevalent in most beers, with notable exceptions such as Kriek, Lambic, Faro, West Flanders ales and Flanders Old Brown, which use acid-producing bacteria ( Lactobacillus and Pediococcus ) or unconventional yeast ( Brettanomyces ) 54 , 55 . Glycerol, ethanol and esters show similar distributions across all beer styles, reflecting their common origin as products of yeast metabolism during fermentation 45 , 53 . Finally, low/no-alcohol beers contain low concentrations of glycerol and esters. This is in line with the production process for most of the low/no-alcohol beers in our dataset, which are produced through limiting fermentation or by stripping away alcohol via evaporation or dialysis, with both methods having the unintended side-effect of reducing the amount of flavor compounds in the final beer 56 , 57 .
Besides expected associations, our data also reveals less trivial associations between beer styles and specific parameters. For example, geraniol and citronellol, two monoterpenoids responsible for citrus, floral and rose flavors and characteristic of Citra hops, are found in relatively high amounts in Christmas, Saison, and Brett/co-fermented beers, where they may originate from terpenoid-rich spices such as coriander seeds instead of hops 58 .
Tasting panel assessments reveal sensorial relationships in beer
To assess the sensory profile of each beer, a trained tasting panel evaluated each of the 250 beers for 50 sensory attributes, including different hop, malt and yeast flavors, off-flavors and spices. Panelists used a tasting sheet (Supplementary Data 3 ) to score the different attributes. Panel consistency was evaluated by repeating 12 samples across different sessions and performing ANOVA. In 95% of cases no significant difference was found across sessions ( p > 0.05), indicating good panel consistency (Supplementary Table S2 ).
Aroma and taste perception reported by the trained panel are often linked (Fig. 1 , bottom left panel and Supplementary Data 4 and 5 ), with high correlations between hops aroma and taste (Spearman’s rho=0.83). Bitter taste was found to correlate with hop aroma and taste in general (Spearman’s rho=0.80 and 0.69), and particularly with “grassy” noble hops (Spearman’s rho=0.75). Barnyard flavor, most often associated with sour beers, is identified together with stale hops (Spearman’s rho=0.97) that are used in these beers. Lactic and acetic acid, which often co-occur, are correlated (Spearman’s rho=0.66). Interestingly, sweetness and bitterness are anti-correlated (Spearman’s rho = −0.48), confirming the hypothesis that they mask each other 59 , 60 . Beer body is highly correlated with alcohol (Spearman’s rho = 0.79), and overall appreciation is found to correlate with multiple aspects that describe beer mouthfeel (alcohol, carbonation; Spearman’s rho= 0.32, 0.39), as well as with hop and ester aroma intensity (Spearman’s rho=0.39 and 0.35).
Similar to the chemical analyses, sensorial analyses confirmed typical features of specific beer styles (Supplementary Fig. S4 ). For example, sour beers (Faro, Flanders Old Brown, Fruit beer, Kriek, Lambic, West Flanders ale) were rated acidic, with flavors of both acetic and lactic acid. Hoppy beers were found to be bitter and showed hop-associated aromas like citrus and tropical fruit. Malt taste is most detected among scotch, stout/porters, and strong ales, while low/no-alcohol beers, which often have a reputation for being ‘worty’ (reminiscent of unfermented, sweet malt extract) appear in the middle. Unsurprisingly, hop aromas are most strongly detected among hoppy beers. Like its chemical counterpart (Supplementary Fig. S3 ), acidity shows a right-skewed distribution, with the most acidic beers being Krieks, Lambics, and West Flanders ales.
Tasting panel assessments of specific flavors correlate with chemical composition
We find that the concentrations of several chemical compounds strongly correlate with specific aroma or taste, as evaluated by the tasting panel (Fig. 2 , Supplementary Fig. S5 , Supplementary Data 6 ). In some cases, these correlations confirm expectations and serve as a useful control for data quality. For example, iso-alpha acids, the bittering compounds in hops, strongly correlate with bitterness (Spearman’s rho=0.68), while ethanol and glycerol correlate with tasters’ perceptions of alcohol and body, the mouthfeel sensation of fullness (Spearman’s rho=0.82/0.62 and 0.72/0.57 respectively) and darker color from roasted malts is a good indication of malt perception (Spearman’s rho=0.54).
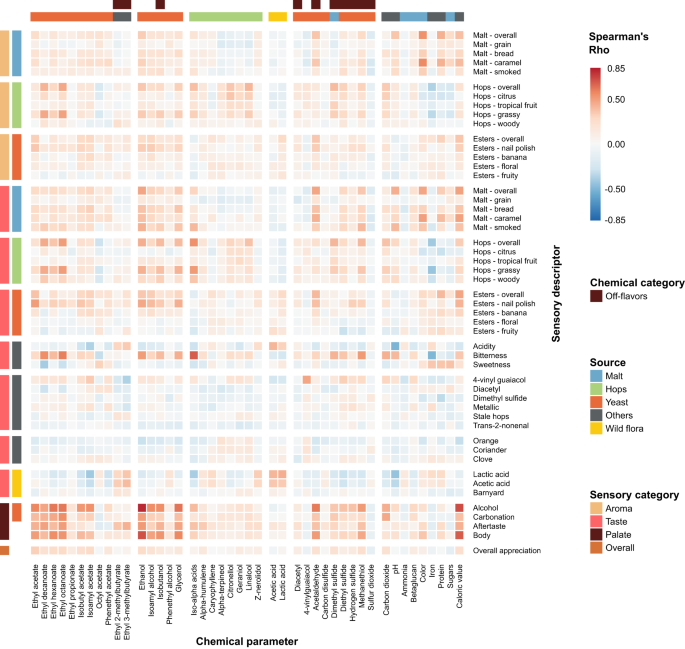
Heatmap colors indicate Spearman’s Rho. Axes are organized according to sensory categories (aroma, taste, mouthfeel, overall), chemical categories and chemical sources in beer (malt (blue), hops (green), yeast (red), wild flora (yellow), Others (black)). See Supplementary Data 6 for all correlation values.
Interestingly, for some relationships between chemical compounds and perceived flavor, correlations are weaker than expected. For example, the rose-smelling phenethyl acetate only weakly correlates with floral aroma. This hints at more complex relationships and interactions between compounds and suggests a need for a more complex model than simple correlations. Lastly, we uncovered unexpected correlations. For instance, the esters ethyl decanoate and ethyl octanoate appear to correlate slightly with hop perception and bitterness, possibly due to their fruity flavor. Iron is anti-correlated with hop aromas and bitterness, most likely because it is also anti-correlated with iso-alpha acids. This could be a sign of metal chelation of hop acids 61 , given that our analyses measure unbound hop acids and total iron content, or could result from the higher iron content in dark and Fruit beers, which typically have less hoppy and bitter flavors 62 .
Public consumer reviews complement expert panel data
To complement and expand the sensory data of our trained tasting panel, we collected 180,000 reviews of our 250 beers from the online consumer review platform RateBeer. This provided numerical scores for beer appearance, aroma, taste, palate, overall quality as well as the average overall score.
Public datasets are known to suffer from biases, such as price, cult status and psychological conformity towards previous ratings of a product. For example, prices correlate with appreciation scores for these online consumer reviews (rho=0.49, Supplementary Fig. S6 ), but not for our trained tasting panel (rho=0.19). This suggests that prices affect consumer appreciation, which has been reported in wine 63 , while blind tastings are unaffected. Moreover, we observe that some beer styles, like lagers and non-alcoholic beers, generally receive lower scores, reflecting that online reviewers are mostly beer aficionados with a preference for specialty beers over lager beers. In general, we find a modest correlation between our trained panel’s overall appreciation score and the online consumer appreciation scores (Fig. 3 , rho=0.29). Apart from the aforementioned biases in the online datasets, serving temperature, sample freshness and surroundings, which are all tightly controlled during the tasting panel sessions, can vary tremendously across online consumers and can further contribute to (among others, appreciation) differences between the two categories of tasters. Importantly, in contrast to the overall appreciation scores, for many sensory aspects the results from the professional panel correlated well with results obtained from RateBeer reviews. Correlations were highest for features that are relatively easy to recognize even for untrained tasters, like bitterness, sweetness, alcohol and malt aroma (Fig. 3 and below).
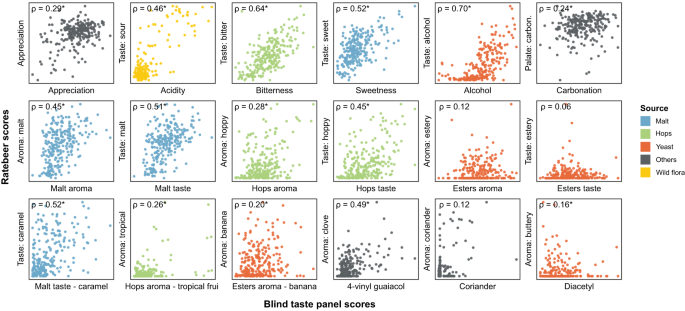
RateBeer text mining results can be found in Supplementary Data 7 . Rho values shown are Spearman correlation values, with asterisks indicating significant correlations ( p < 0.05, two-sided). All p values were smaller than 0.001, except for Esters aroma (0.0553), Esters taste (0.3275), Esters aroma—banana (0.0019), Coriander (0.0508) and Diacetyl (0.0134).
Besides collecting consumer appreciation from these online reviews, we developed automated text analysis tools to gather additional data from review texts (Supplementary Data 7 ). Processing review texts on the RateBeer database yielded comparable results to the scores given by the trained panel for many common sensory aspects, including acidity, bitterness, sweetness, alcohol, malt, and hop tastes (Fig. 3 ). This is in line with what would be expected, since these attributes require less training for accurate assessment and are less influenced by environmental factors such as temperature, serving glass and odors in the environment. Consumer reviews also correlate well with our trained panel for 4-vinyl guaiacol, a compound associated with a very characteristic aroma. By contrast, correlations for more specific aromas like ester, coriander or diacetyl are underrepresented in the online reviews, underscoring the importance of using a trained tasting panel and standardized tasting sheets with explicit factors to be scored for evaluating specific aspects of a beer. Taken together, our results suggest that public reviews are trustworthy for some, but not all, flavor features and can complement or substitute taste panel data for these sensory aspects.
Models can predict beer sensory profiles from chemical data
The rich datasets of chemical analyses, tasting panel assessments and public reviews gathered in the first part of this study provided us with a unique opportunity to develop predictive models that link chemical data to sensorial features. Given the complexity of beer flavor, basic statistical tools such as correlations or linear regression may not always be the most suitable for making accurate predictions. Instead, we applied different machine learning models that can model both simple linear and complex interactive relationships. Specifically, we constructed a set of regression models to predict (a) trained panel scores for beer flavor and quality and (b) public reviews’ appreciation scores from beer chemical profiles. We trained and tested 10 different models (Methods), 3 linear regression-based models (simple linear regression with first-order interactions (LR), lasso regression with first-order interactions (Lasso), partial least squares regressor (PLSR)), 5 decision tree models (AdaBoost regressor (ABR), extra trees (ET), gradient boosting regressor (GBR), random forest (RF) and XGBoost regressor (XGBR)), 1 support vector regression (SVR), and 1 artificial neural network (ANN) model.
To compare the performance of our machine learning models, the dataset was randomly split into a training and test set, stratified by beer style. After a model was trained on data in the training set, its performance was evaluated on its ability to predict the test dataset obtained from multi-output models (based on the coefficient of determination, see Methods). Additionally, individual-attribute models were ranked per descriptor and the average rank was calculated, as proposed by Korneva et al. 64 . Importantly, both ways of evaluating the models’ performance agreed in general. Performance of the different models varied (Table 1 ). It should be noted that all models perform better at predicting RateBeer results than results from our trained tasting panel. One reason could be that sensory data is inherently variable, and this variability is averaged out with the large number of public reviews from RateBeer. Additionally, all tree-based models perform better at predicting taste than aroma. Linear models (LR) performed particularly poorly, with negative R 2 values, due to severe overfitting (training set R 2 = 1). Overfitting is a common issue in linear models with many parameters and limited samples, especially with interaction terms further amplifying the number of parameters. L1 regularization (Lasso) successfully overcomes this overfitting, out-competing multiple tree-based models on the RateBeer dataset. Similarly, the dimensionality reduction of PLSR avoids overfitting and improves performance, to some extent. Still, tree-based models (ABR, ET, GBR, RF and XGBR) show the best performance, out-competing the linear models (LR, Lasso, PLSR) commonly used in sensory science 65 .
GBR models showed the best overall performance in predicting sensory responses from chemical information, with R 2 values up to 0.75 depending on the predicted sensory feature (Supplementary Table S4 ). The GBR models predict consumer appreciation (RateBeer) better than our trained panel’s appreciation (R 2 value of 0.67 compared to R 2 value of 0.09) (Supplementary Table S3 and Supplementary Table S4 ). ANN models showed intermediate performance, likely because neural networks typically perform best with larger datasets 66 . The SVR shows intermediate performance, mostly due to the weak predictions of specific attributes that lower the overall performance (Supplementary Table S4 ).
Model dissection identifies specific, unexpected compounds as drivers of consumer appreciation
Next, we leveraged our models to infer important contributors to sensory perception and consumer appreciation. Consumer preference is a crucial sensory aspects, because a product that shows low consumer appreciation scores often does not succeed commercially 25 . Additionally, the requirement for a large number of representative evaluators makes consumer trials one of the more costly and time-consuming aspects of product development. Hence, a model for predicting chemical drivers of overall appreciation would be a welcome addition to the available toolbox for food development and optimization.
Since GBR models on our RateBeer dataset showed the best overall performance, we focused on these models. Specifically, we used two approaches to identify important contributors. First, rankings of the most important predictors for each sensorial trait in the GBR models were obtained based on impurity-based feature importance (mean decrease in impurity). High-ranked parameters were hypothesized to be either the true causal chemical properties underlying the trait, to correlate with the actual causal properties, or to take part in sensory interactions affecting the trait 67 (Fig. 4A ). In a second approach, we used SHAP 68 to determine which parameters contributed most to the model for making predictions of consumer appreciation (Fig. 4B ). SHAP calculates parameter contributions to model predictions on a per-sample basis, which can be aggregated into an importance score.
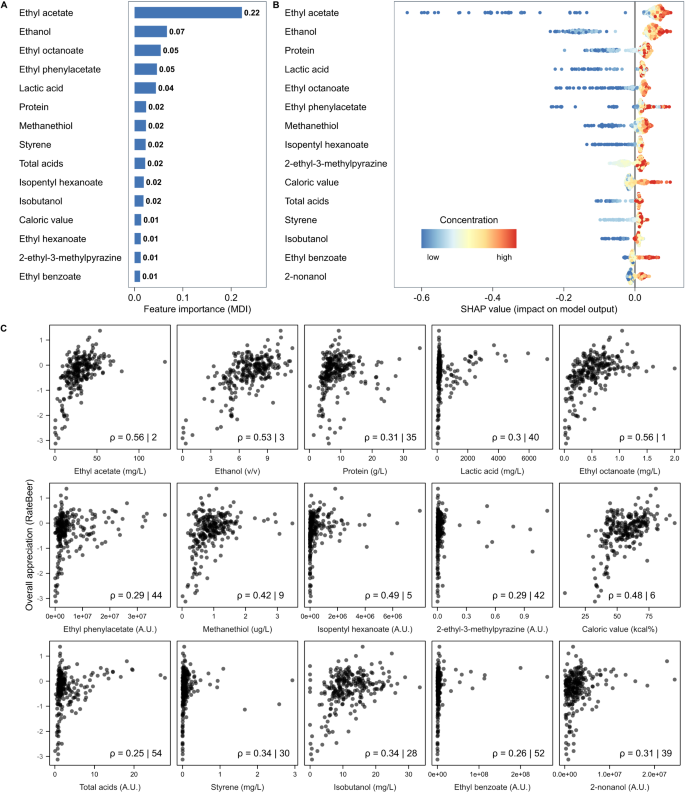
A The impurity-based feature importance (mean deviance in impurity, MDI) calculated from the Gradient Boosting Regression (GBR) model predicting RateBeer appreciation scores. The top 15 highest ranked chemical properties are shown. B SHAP summary plot for the top 15 parameters contributing to our GBR model. Each point on the graph represents a sample from our dataset. The color represents the concentration of that parameter, with bluer colors representing low values and redder colors representing higher values. Greater absolute values on the horizontal axis indicate a higher impact of the parameter on the prediction of the model. C Spearman correlations between the 15 most important chemical properties and consumer overall appreciation. Numbers indicate the Spearman Rho correlation coefficient, and the rank of this correlation compared to all other correlations. The top 15 important compounds were determined using SHAP (panel B).
Both approaches identified ethyl acetate as the most predictive parameter for beer appreciation (Fig. 4 ). Ethyl acetate is the most abundant ester in beer with a typical ‘fruity’, ‘solvent’ and ‘alcoholic’ flavor, but is often considered less important than other esters like isoamyl acetate. The second most important parameter identified by SHAP is ethanol, the most abundant beer compound after water. Apart from directly contributing to beer flavor and mouthfeel, ethanol drastically influences the physical properties of beer, dictating how easily volatile compounds escape the beer matrix to contribute to beer aroma 69 . Importantly, it should also be noted that the importance of ethanol for appreciation is likely inflated by the very low appreciation scores of non-alcoholic beers (Supplementary Fig. S4 ). Despite not often being considered a driver of beer appreciation, protein level also ranks highly in both approaches, possibly due to its effect on mouthfeel and body 70 . Lactic acid, which contributes to the tart taste of sour beers, is the fourth most important parameter identified by SHAP, possibly due to the generally high appreciation of sour beers in our dataset.
Interestingly, some of the most important predictive parameters for our model are not well-established as beer flavors or are even commonly regarded as being negative for beer quality. For example, our models identify methanethiol and ethyl phenyl acetate, an ester commonly linked to beer staling 71 , as a key factor contributing to beer appreciation. Although there is no doubt that high concentrations of these compounds are considered unpleasant, the positive effects of modest concentrations are not yet known 72 , 73 .
To compare our approach to conventional statistics, we evaluated how well the 15 most important SHAP-derived parameters correlate with consumer appreciation (Fig. 4C ). Interestingly, only 6 of the properties derived by SHAP rank amongst the top 15 most correlated parameters. For some chemical compounds, the correlations are so low that they would have likely been considered unimportant. For example, lactic acid, the fourth most important parameter, shows a bimodal distribution for appreciation, with sour beers forming a separate cluster, that is missed entirely by the Spearman correlation. Additionally, the correlation plots reveal outliers, emphasizing the need for robust analysis tools. Together, this highlights the need for alternative models, like the Gradient Boosting model, that better grasp the complexity of (beer) flavor.
Finally, to observe the relationships between these chemical properties and their predicted targets, partial dependence plots were constructed for the six most important predictors of consumer appreciation 74 , 75 , 76 (Supplementary Fig. S7 ). One-way partial dependence plots show how a change in concentration affects the predicted appreciation. These plots reveal an important limitation of our models: appreciation predictions remain constant at ever-increasing concentrations. This implies that once a threshold concentration is reached, further increasing the concentration does not affect appreciation. This is false, as it is well-documented that certain compounds become unpleasant at high concentrations, including ethyl acetate (‘nail polish’) 77 and methanethiol (‘sulfury’ and ‘rotten cabbage’) 78 . The inability of our models to grasp that flavor compounds have optimal levels, above which they become negative, is a consequence of working with commercial beer brands where (off-)flavors are rarely too high to negatively impact the product. The two-way partial dependence plots show how changing the concentration of two compounds influences predicted appreciation, visualizing their interactions (Supplementary Fig. S7 ). In our case, the top 5 parameters are dominated by additive or synergistic interactions, with high concentrations for both compounds resulting in the highest predicted appreciation.
To assess the robustness of our best-performing models and model predictions, we performed 100 iterations of the GBR, RF and ET models. In general, all iterations of the models yielded similar performance (Supplementary Fig. S8 ). Moreover, the main predictors (including the top predictors ethanol and ethyl acetate) remained virtually the same, especially for GBR and RF. For the iterations of the ET model, we did observe more variation in the top predictors, which is likely a consequence of the model’s inherent random architecture in combination with co-correlations between certain predictors. However, even in this case, several of the top predictors (ethanol and ethyl acetate) remain unchanged, although their rank in importance changes (Supplementary Fig. S8 ).
Next, we investigated if a combination of RateBeer and trained panel data into one consolidated dataset would lead to stronger models, under the hypothesis that such a model would suffer less from bias in the datasets. A GBR model was trained to predict appreciation on the combined dataset. This model underperformed compared to the RateBeer model, both in the native case and when including a dataset identifier (R 2 = 0.67, 0.26 and 0.42 respectively). For the latter, the dataset identifier is the most important feature (Supplementary Fig. S9 ), while most of the feature importance remains unchanged, with ethyl acetate and ethanol ranking highest, like in the original model trained only on RateBeer data. It seems that the large variation in the panel dataset introduces noise, weakening the models’ performances and reliability. In addition, it seems reasonable to assume that both datasets are fundamentally different, with the panel dataset obtained by blind tastings by a trained professional panel.
Lastly, we evaluated whether beer style identifiers would further enhance the model’s performance. A GBR model was trained with parameters that explicitly encoded the styles of the samples. This did not improve model performance (R2 = 0.66 with style information vs R2 = 0.67). The most important chemical features are consistent with the model trained without style information (eg. ethanol and ethyl acetate), and with the exception of the most preferred (strong ale) and least preferred (low/no-alcohol) styles, none of the styles were among the most important features (Supplementary Fig. S9 , Supplementary Table S5 and S6 ). This is likely due to a combination of style-specific chemical signatures, such as iso-alpha acids and lactic acid, that implicitly convey style information to the original models, as well as the low number of samples belonging to some styles, making it difficult for the model to learn style-specific patterns. Moreover, beer styles are not rigorously defined, with some styles overlapping in features and some beers being misattributed to a specific style, all of which leads to more noise in models that use style parameters.
Model validation
To test if our predictive models give insight into beer appreciation, we set up experiments aimed at improving existing commercial beers. We specifically selected overall appreciation as the trait to be examined because of its complexity and commercial relevance. Beer flavor comprises a complex bouquet rather than single aromas and tastes 53 . Hence, adding a single compound to the extent that a difference is noticeable may lead to an unbalanced, artificial flavor. Therefore, we evaluated the effect of combinations of compounds. Because Blond beers represent the most extensive style in our dataset, we selected a beer from this style as the starting material for these experiments (Beer 64 in Supplementary Data 1 ).
In the first set of experiments, we adjusted the concentrations of compounds that made up the most important predictors of overall appreciation (ethyl acetate, ethanol, lactic acid, ethyl phenyl acetate) together with correlated compounds (ethyl hexanoate, isoamyl acetate, glycerol), bringing them up to 95 th percentile ethanol-normalized concentrations (Methods) within the Blond group (‘Spiked’ concentration in Fig. 5A ). Compared to controls, the spiked beers were found to have significantly improved overall appreciation among trained panelists, with panelist noting increased intensity of ester flavors, sweetness, alcohol, and body fullness (Fig. 5B ). To disentangle the contribution of ethanol to these results, a second experiment was performed without the addition of ethanol. This resulted in a similar outcome, including increased perception of alcohol and overall appreciation.
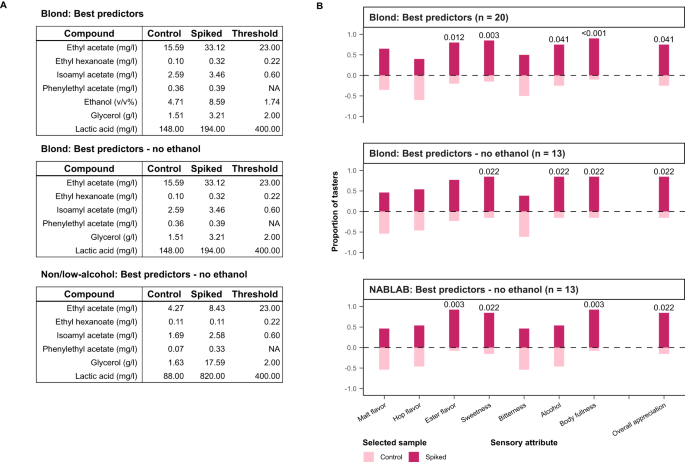
Adding the top chemical compounds, identified as best predictors of appreciation by our model, into poorly appreciated beers results in increased appreciation from our trained panel. Results of sensory tests between base beers and those spiked with compounds identified as the best predictors by the model. A Blond and Non/Low-alcohol (0.0% ABV) base beers were brought up to 95th-percentile ethanol-normalized concentrations within each style. B For each sensory attribute, tasters indicated the more intense sample and selected the sample they preferred. The numbers above the bars correspond to the p values that indicate significant changes in perceived flavor (two-sided binomial test: alpha 0.05, n = 20 or 13).
In a last experiment, we tested whether using the model’s predictions can boost the appreciation of a non-alcoholic beer (beer 223 in Supplementary Data 1 ). Again, the addition of a mixture of predicted compounds (omitting ethanol, in this case) resulted in a significant increase in appreciation, body, ester flavor and sweetness.
Predicting flavor and consumer appreciation from chemical composition is one of the ultimate goals of sensory science. A reliable, systematic and unbiased way to link chemical profiles to flavor and food appreciation would be a significant asset to the food and beverage industry. Such tools would substantially aid in quality control and recipe development, offer an efficient and cost-effective alternative to pilot studies and consumer trials and would ultimately allow food manufacturers to produce superior, tailor-made products that better meet the demands of specific consumer groups more efficiently.
A limited set of studies have previously tried, to varying degrees of success, to predict beer flavor and beer popularity based on (a limited set of) chemical compounds and flavors 79 , 80 . Current sensitive, high-throughput technologies allow measuring an unprecedented number of chemical compounds and properties in a large set of samples, yielding a dataset that can train models that help close the gaps between chemistry and flavor, even for a complex natural product like beer. To our knowledge, no previous research gathered data at this scale (250 samples, 226 chemical parameters, 50 sensory attributes and 5 consumer scores) to disentangle and validate the chemical aspects driving beer preference using various machine-learning techniques. We find that modern machine learning models outperform conventional statistical tools, such as correlations and linear models, and can successfully predict flavor appreciation from chemical composition. This could be attributed to the natural incorporation of interactions and non-linear or discontinuous effects in machine learning models, which are not easily grasped by the linear model architecture. While linear models and partial least squares regression represent the most widespread statistical approaches in sensory science, in part because they allow interpretation 65 , 81 , 82 , modern machine learning methods allow for building better predictive models while preserving the possibility to dissect and exploit the underlying patterns. Of the 10 different models we trained, tree-based models, such as our best performing GBR, showed the best overall performance in predicting sensory responses from chemical information, outcompeting artificial neural networks. This agrees with previous reports for models trained on tabular data 83 . Our results are in line with the findings of Colantonio et al. who also identified the gradient boosting architecture as performing best at predicting appreciation and flavor (of tomatoes and blueberries, in their specific study) 26 . Importantly, besides our larger experimental scale, we were able to directly confirm our models’ predictions in vivo.
Our study confirms that flavor compound concentration does not always correlate with perception, suggesting complex interactions that are often missed by more conventional statistics and simple models. Specifically, we find that tree-based algorithms may perform best in developing models that link complex food chemistry with aroma. Furthermore, we show that massive datasets of untrained consumer reviews provide a valuable source of data, that can complement or even replace trained tasting panels, especially for appreciation and basic flavors, such as sweetness and bitterness. This holds despite biases that are known to occur in such datasets, such as price or conformity bias. Moreover, GBR models predict taste better than aroma. This is likely because taste (e.g. bitterness) often directly relates to the corresponding chemical measurements (e.g., iso-alpha acids), whereas such a link is less clear for aromas, which often result from the interplay between multiple volatile compounds. We also find that our models are best at predicting acidity and alcohol, likely because there is a direct relation between the measured chemical compounds (acids and ethanol) and the corresponding perceived sensorial attribute (acidity and alcohol), and because even untrained consumers are generally able to recognize these flavors and aromas.
The predictions of our final models, trained on review data, hold even for blind tastings with small groups of trained tasters, as demonstrated by our ability to validate specific compounds as drivers of beer flavor and appreciation. Since adding a single compound to the extent of a noticeable difference may result in an unbalanced flavor profile, we specifically tested our identified key drivers as a combination of compounds. While this approach does not allow us to validate if a particular single compound would affect flavor and/or appreciation, our experiments do show that this combination of compounds increases consumer appreciation.
It is important to stress that, while it represents an important step forward, our approach still has several major limitations. A key weakness of the GBR model architecture is that amongst co-correlating variables, the largest main effect is consistently preferred for model building. As a result, co-correlating variables often have artificially low importance scores, both for impurity and SHAP-based methods, like we observed in the comparison to the more randomized Extra Trees models. This implies that chemicals identified as key drivers of a specific sensory feature by GBR might not be the true causative compounds, but rather co-correlate with the actual causative chemical. For example, the high importance of ethyl acetate could be (partially) attributed to the total ester content, ethanol or ethyl hexanoate (rho=0.77, rho=0.72 and rho=0.68), while ethyl phenylacetate could hide the importance of prenyl isobutyrate and ethyl benzoate (rho=0.77 and rho=0.76). Expanding our GBR model to include beer style as a parameter did not yield additional power or insight. This is likely due to style-specific chemical signatures, such as iso-alpha acids and lactic acid, that implicitly convey style information to the original model, as well as the smaller sample size per style, limiting the power to uncover style-specific patterns. This can be partly attributed to the curse of dimensionality, where the high number of parameters results in the models mainly incorporating single parameter effects, rather than complex interactions such as style-dependent effects 67 . A larger number of samples may overcome some of these limitations and offer more insight into style-specific effects. On the other hand, beer style is not a rigid scientific classification, and beers within one style often differ a lot, which further complicates the analysis of style as a model factor.
Our study is limited to beers from Belgian breweries. Although these beers cover a large portion of the beer styles available globally, some beer styles and consumer patterns may be missing, while other features might be overrepresented. For example, many Belgian ales exhibit yeast-driven flavor profiles, which is reflected in the chemical drivers of appreciation discovered by this study. In future work, expanding the scope to include diverse markets and beer styles could lead to the identification of even more drivers of appreciation and better models for special niche products that were not present in our beer set.
In addition to inherent limitations of GBR models, there are also some limitations associated with studying food aroma. Even if our chemical analyses measured most of the known aroma compounds, the total number of flavor compounds in complex foods like beer is still larger than the subset we were able to measure in this study. For example, hop-derived thiols, that influence flavor at very low concentrations, are notoriously difficult to measure in a high-throughput experiment. Moreover, consumer perception remains subjective and prone to biases that are difficult to avoid. It is also important to stress that the models are still immature and that more extensive datasets will be crucial for developing more complete models in the future. Besides more samples and parameters, our dataset does not include any demographic information about the tasters. Including such data could lead to better models that grasp external factors like age and culture. Another limitation is that our set of beers consists of high-quality end-products and lacks beers that are unfit for sale, which limits the current model in accurately predicting products that are appreciated very badly. Finally, while models could be readily applied in quality control, their use in sensory science and product development is restrained by their inability to discern causal relationships. Given that the models cannot distinguish compounds that genuinely drive consumer perception from those that merely correlate, validation experiments are essential to identify true causative compounds.
Despite the inherent limitations, dissection of our models enabled us to pinpoint specific molecules as potential drivers of beer aroma and consumer appreciation, including compounds that were unexpected and would not have been identified using standard approaches. Important drivers of beer appreciation uncovered by our models include protein levels, ethyl acetate, ethyl phenyl acetate and lactic acid. Currently, many brewers already use lactic acid to acidify their brewing water and ensure optimal pH for enzymatic activity during the mashing process. Our results suggest that adding lactic acid can also improve beer appreciation, although its individual effect remains to be tested. Interestingly, ethanol appears to be unnecessary to improve beer appreciation, both for blond beer and alcohol-free beer. Given the growing consumer interest in alcohol-free beer, with a predicted annual market growth of >7% 84 , it is relevant for brewers to know what compounds can further increase consumer appreciation of these beers. Hence, our model may readily provide avenues to further improve the flavor and consumer appreciation of both alcoholic and non-alcoholic beers, which is generally considered one of the key challenges for future beer production.
Whereas we see a direct implementation of our results for the development of superior alcohol-free beverages and other food products, our study can also serve as a stepping stone for the development of novel alcohol-containing beverages. We want to echo the growing body of scientific evidence for the negative effects of alcohol consumption, both on the individual level by the mutagenic, teratogenic and carcinogenic effects of ethanol 85 , 86 , as well as the burden on society caused by alcohol abuse and addiction. We encourage the use of our results for the production of healthier, tastier products, including novel and improved beverages with lower alcohol contents. Furthermore, we strongly discourage the use of these technologies to improve the appreciation or addictive properties of harmful substances.
The present work demonstrates that despite some important remaining hurdles, combining the latest developments in chemical analyses, sensory analysis and modern machine learning methods offers exciting avenues for food chemistry and engineering. Soon, these tools may provide solutions in quality control and recipe development, as well as new approaches to sensory science and flavor research.
Beer selection
250 commercial Belgian beers were selected to cover the broad diversity of beer styles and corresponding diversity in chemical composition and aroma. See Supplementary Fig. S1 .
Chemical dataset
Sample preparation.
Beers within their expiration date were purchased from commercial retailers. Samples were prepared in biological duplicates at room temperature, unless explicitly stated otherwise. Bottle pressure was measured with a manual pressure device (Steinfurth Mess-Systeme GmbH) and used to calculate CO 2 concentration. The beer was poured through two filter papers (Macherey-Nagel, 500713032 MN 713 ¼) to remove carbon dioxide and prevent spontaneous foaming. Samples were then prepared for measurements by targeted Headspace-Gas Chromatography-Flame Ionization Detector/Flame Photometric Detector (HS-GC-FID/FPD), Headspace-Solid Phase Microextraction-Gas Chromatography-Mass Spectrometry (HS-SPME-GC-MS), colorimetric analysis, enzymatic analysis, Near-Infrared (NIR) analysis, as described in the sections below. The mean values of biological duplicates are reported for each compound.
HS-GC-FID/FPD
HS-GC-FID/FPD (Shimadzu GC 2010 Plus) was used to measure higher alcohols, acetaldehyde, esters, 4-vinyl guaicol, and sulfur compounds. Each measurement comprised 5 ml of sample pipetted into a 20 ml glass vial containing 1.75 g NaCl (VWR, 27810.295). 100 µl of 2-heptanol (Sigma-Aldrich, H3003) (internal standard) solution in ethanol (Fisher Chemical, E/0650DF/C17) was added for a final concentration of 2.44 mg/L. Samples were flushed with nitrogen for 10 s, sealed with a silicone septum, stored at −80 °C and analyzed in batches of 20.
The GC was equipped with a DB-WAXetr column (length, 30 m; internal diameter, 0.32 mm; layer thickness, 0.50 µm; Agilent Technologies, Santa Clara, CA, USA) to the FID and an HP-5 column (length, 30 m; internal diameter, 0.25 mm; layer thickness, 0.25 µm; Agilent Technologies, Santa Clara, CA, USA) to the FPD. N 2 was used as the carrier gas. Samples were incubated for 20 min at 70 °C in the headspace autosampler (Flow rate, 35 cm/s; Injection volume, 1000 µL; Injection mode, split; Combi PAL autosampler, CTC analytics, Switzerland). The injector, FID and FPD temperatures were kept at 250 °C. The GC oven temperature was first held at 50 °C for 5 min and then allowed to rise to 80 °C at a rate of 5 °C/min, followed by a second ramp of 4 °C/min until 200 °C kept for 3 min and a final ramp of (4 °C/min) until 230 °C for 1 min. Results were analyzed with the GCSolution software version 2.4 (Shimadzu, Kyoto, Japan). The GC was calibrated with a 5% EtOH solution (VWR International) containing the volatiles under study (Supplementary Table S7 ).
HS-SPME-GC-MS
HS-SPME-GC-MS (Shimadzu GCMS-QP-2010 Ultra) was used to measure additional volatile compounds, mainly comprising terpenoids and esters. Samples were analyzed by HS-SPME using a triphase DVB/Carboxen/PDMS 50/30 μm SPME fiber (Supelco Co., Bellefonte, PA, USA) followed by gas chromatography (Thermo Fisher Scientific Trace 1300 series, USA) coupled to a mass spectrometer (Thermo Fisher Scientific ISQ series MS) equipped with a TriPlus RSH autosampler. 5 ml of degassed beer sample was placed in 20 ml vials containing 1.75 g NaCl (VWR, 27810.295). 5 µl internal standard mix was added, containing 2-heptanol (1 g/L) (Sigma-Aldrich, H3003), 4-fluorobenzaldehyde (1 g/L) (Sigma-Aldrich, 128376), 2,3-hexanedione (1 g/L) (Sigma-Aldrich, 144169) and guaiacol (1 g/L) (Sigma-Aldrich, W253200) in ethanol (Fisher Chemical, E/0650DF/C17). Each sample was incubated at 60 °C in the autosampler oven with constant agitation. After 5 min equilibration, the SPME fiber was exposed to the sample headspace for 30 min. The compounds trapped on the fiber were thermally desorbed in the injection port of the chromatograph by heating the fiber for 15 min at 270 °C.
The GC-MS was equipped with a low polarity RXi-5Sil MS column (length, 20 m; internal diameter, 0.18 mm; layer thickness, 0.18 µm; Restek, Bellefonte, PA, USA). Injection was performed in splitless mode at 320 °C, a split flow of 9 ml/min, a purge flow of 5 ml/min and an open valve time of 3 min. To obtain a pulsed injection, a programmed gas flow was used whereby the helium gas flow was set at 2.7 mL/min for 0.1 min, followed by a decrease in flow of 20 ml/min to the normal 0.9 mL/min. The temperature was first held at 30 °C for 3 min and then allowed to rise to 80 °C at a rate of 7 °C/min, followed by a second ramp of 2 °C/min till 125 °C and a final ramp of 8 °C/min with a final temperature of 270 °C.
Mass acquisition range was 33 to 550 amu at a scan rate of 5 scans/s. Electron impact ionization energy was 70 eV. The interface and ion source were kept at 275 °C and 250 °C, respectively. A mix of linear n-alkanes (from C7 to C40, Supelco Co.) was injected into the GC-MS under identical conditions to serve as external retention index markers. Identification and quantification of the compounds were performed using an in-house developed R script as described in Goelen et al. and Reher et al. 87 , 88 (for package information, see Supplementary Table S8 ). Briefly, chromatograms were analyzed using AMDIS (v2.71) 89 to separate overlapping peaks and obtain pure compound spectra. The NIST MS Search software (v2.0 g) in combination with the NIST2017, FFNSC3 and Adams4 libraries were used to manually identify the empirical spectra, taking into account the expected retention time. After background subtraction and correcting for retention time shifts between samples run on different days based on alkane ladders, compound elution profiles were extracted and integrated using a file with 284 target compounds of interest, which were either recovered in our identified AMDIS list of spectra or were known to occur in beer. Compound elution profiles were estimated for every peak in every chromatogram over a time-restricted window using weighted non-negative least square analysis after which peak areas were integrated 87 , 88 . Batch effect correction was performed by normalizing against the most stable internal standard compound, 4-fluorobenzaldehyde. Out of all 284 target compounds that were analyzed, 167 were visually judged to have reliable elution profiles and were used for final analysis.
Discrete photometric and enzymatic analysis
Discrete photometric and enzymatic analysis (Thermo Scientific TM Gallery TM Plus Beermaster Discrete Analyzer) was used to measure acetic acid, ammonia, beta-glucan, iso-alpha acids, color, sugars, glycerol, iron, pH, protein, and sulfite. 2 ml of sample volume was used for the analyses. Information regarding the reagents and standard solutions used for analyses and calibrations is included in Supplementary Table S7 and Supplementary Table S9 .
NIR analyses
NIR analysis (Anton Paar Alcolyzer Beer ME System) was used to measure ethanol. Measurements comprised 50 ml of sample, and a 10% EtOH solution was used for calibration.
Correlation calculations
Pairwise Spearman Rank correlations were calculated between all chemical properties.
Sensory dataset
Trained panel.
Our trained tasting panel consisted of volunteers who gave prior verbal informed consent. All compounds used for the validation experiment were of food-grade quality. The tasting sessions were approved by the Social and Societal Ethics Committee of the KU Leuven (G-2022-5677-R2(MAR)). All online reviewers agreed to the Terms and Conditions of the RateBeer website.
Sensory analysis was performed according to the American Society of Brewing Chemists (ASBC) Sensory Analysis Methods 90 . 30 volunteers were screened through a series of triangle tests. The sixteen most sensitive and consistent tasters were retained as taste panel members. The resulting panel was diverse in age [22–42, mean: 29], sex [56% male] and nationality [7 different countries]. The panel developed a consensus vocabulary to describe beer aroma, taste and mouthfeel. Panelists were trained to identify and score 50 different attributes, using a 7-point scale to rate attributes’ intensity. The scoring sheet is included as Supplementary Data 3 . Sensory assessments took place between 10–12 a.m. The beers were served in black-colored glasses. Per session, between 5 and 12 beers of the same style were tasted at 12 °C to 16 °C. Two reference beers were added to each set and indicated as ‘Reference 1 & 2’, allowing panel members to calibrate their ratings. Not all panelists were present at every tasting. Scores were scaled by standard deviation and mean-centered per taster. Values are represented as z-scores and clustered by Euclidean distance. Pairwise Spearman correlations were calculated between taste and aroma sensory attributes. Panel consistency was evaluated by repeating samples on different sessions and performing ANOVA to identify differences, using the ‘stats’ package (v4.2.2) in R (for package information, see Supplementary Table S8 ).
Online reviews from a public database
The ‘scrapy’ package in Python (v3.6) (for package information, see Supplementary Table S8 ). was used to collect 232,288 online reviews (mean=922, min=6, max=5343) from RateBeer, an online beer review database. Each review entry comprised 5 numerical scores (appearance, aroma, taste, palate and overall quality) and an optional review text. The total number of reviews per reviewer was collected separately. Numerical scores were scaled and centered per rater, and mean scores were calculated per beer.
For the review texts, the language was estimated using the packages ‘langdetect’ and ‘langid’ in Python. Reviews that were classified as English by both packages were kept. Reviewers with fewer than 100 entries overall were discarded. 181,025 reviews from >6000 reviewers from >40 countries remained. Text processing was done using the ‘nltk’ package in Python. Texts were corrected for slang and misspellings; proper nouns and rare words that are relevant to the beer context were specified and kept as-is (‘Chimay’,’Lambic’, etc.). A dictionary of semantically similar sensorial terms, for example ‘floral’ and ‘flower’, was created and collapsed together into one term. Words were stemmed and lemmatized to avoid identifying words such as ‘acid’ and ‘acidity’ as separate terms. Numbers and punctuation were removed.
Sentences from up to 50 randomly chosen reviews per beer were manually categorized according to the aspect of beer they describe (appearance, aroma, taste, palate, overall quality—not to be confused with the 5 numerical scores described above) or flagged as irrelevant if they contained no useful information. If a beer contained fewer than 50 reviews, all reviews were manually classified. This labeled data set was used to train a model that classified the rest of the sentences for all beers 91 . Sentences describing taste and aroma were extracted, and term frequency–inverse document frequency (TFIDF) was implemented to calculate enrichment scores for sensorial words per beer.
The sex of the tasting subject was not considered when building our sensory database. Instead, results from different panelists were averaged, both for our trained panel (56% male, 44% female) and the RateBeer reviews (70% male, 30% female for RateBeer as a whole).
Beer price collection and processing
Beer prices were collected from the following stores: Colruyt, Delhaize, Total Wine, BeerHawk, The Belgian Beer Shop, The Belgian Shop, and Beer of Belgium. Where applicable, prices were converted to Euros and normalized per liter. Spearman correlations were calculated between these prices and mean overall appreciation scores from RateBeer and the taste panel, respectively.
Pairwise Spearman Rank correlations were calculated between all sensory properties.
Machine learning models
Predictive modeling of sensory profiles from chemical data.
Regression models were constructed to predict (a) trained panel scores for beer flavors and quality from beer chemical profiles and (b) public reviews’ appreciation scores from beer chemical profiles. Z-scores were used to represent sensory attributes in both data sets. Chemical properties with log-normal distributions (Shapiro-Wilk test, p < 0.05 ) were log-transformed. Missing chemical measurements (0.1% of all data) were replaced with mean values per attribute. Observations from 250 beers were randomly separated into a training set (70%, 175 beers) and a test set (30%, 75 beers), stratified per beer style. Chemical measurements (p = 231) were normalized based on the training set average and standard deviation. In total, three linear regression-based models: linear regression with first-order interaction terms (LR), lasso regression with first-order interaction terms (Lasso) and partial least squares regression (PLSR); five decision tree models, Adaboost regressor (ABR), Extra Trees (ET), Gradient Boosting regressor (GBR), Random Forest (RF) and XGBoost regressor (XGBR); one support vector machine model (SVR) and one artificial neural network model (ANN) were trained. The models were implemented using the ‘scikit-learn’ package (v1.2.2) and ‘xgboost’ package (v1.7.3) in Python (v3.9.16). Models were trained, and hyperparameters optimized, using five-fold cross-validated grid search with the coefficient of determination (R 2 ) as the evaluation metric. The ANN (scikit-learn’s MLPRegressor) was optimized using Bayesian Tree-Structured Parzen Estimator optimization with the ‘Optuna’ Python package (v3.2.0). Individual models were trained per attribute, and a multi-output model was trained on all attributes simultaneously.
Model dissection
GBR was found to outperform other methods, resulting in models with the highest average R 2 values in both trained panel and public review data sets. Impurity-based rankings of the most important predictors for each predicted sensorial trait were obtained using the ‘scikit-learn’ package. To observe the relationships between these chemical properties and their predicted targets, partial dependence plots (PDP) were constructed for the six most important predictors of consumer appreciation 74 , 75 .
The ‘SHAP’ package in Python (v0.41.0) was implemented to provide an alternative ranking of predictor importance and to visualize the predictors’ effects as a function of their concentration 68 .
Validation of causal chemical properties
To validate the effects of the most important model features on predicted sensory attributes, beers were spiked with the chemical compounds identified by the models and descriptive sensory analyses were carried out according to the American Society of Brewing Chemists (ASBC) protocol 90 .
Compound spiking was done 30 min before tasting. Compounds were spiked into fresh beer bottles, that were immediately resealed and inverted three times. Fresh bottles of beer were opened for the same duration, resealed, and inverted thrice, to serve as controls. Pairs of spiked samples and controls were served simultaneously, chilled and in dark glasses as outlined in the Trained panel section above. Tasters were instructed to select the glass with the higher flavor intensity for each attribute (directional difference test 92 ) and to select the glass they prefer.
The final concentration after spiking was equal to the within-style average, after normalizing by ethanol concentration. This was done to ensure balanced flavor profiles in the final spiked beer. The same methods were applied to improve a non-alcoholic beer. Compounds were the following: ethyl acetate (Merck KGaA, W241415), ethyl hexanoate (Merck KGaA, W243906), isoamyl acetate (Merck KGaA, W205508), phenethyl acetate (Merck KGaA, W285706), ethanol (96%, Colruyt), glycerol (Merck KGaA, W252506), lactic acid (Merck KGaA, 261106).
Significant differences in preference or perceived intensity were determined by performing the two-sided binomial test on each attribute.
Reporting summary
Further information on research design is available in the Nature Portfolio Reporting Summary linked to this article.
Data availability
The data that support the findings of this work are available in the Supplementary Data files and have been deposited to Zenodo under accession code 10653704 93 . The RateBeer scores data are under restricted access, they are not publicly available as they are property of RateBeer (ZX Ventures, USA). Access can be obtained from the authors upon reasonable request and with permission of RateBeer (ZX Ventures, USA). Source data are provided with this paper.
Code availability
The code for training the machine learning models, analyzing the models, and generating the figures has been deposited to Zenodo under accession code 10653704 93 .
Tieman, D. et al. A chemical genetic roadmap to improved tomato flavor. Science 355 , 391–394 (2017).
Article ADS CAS PubMed Google Scholar
Plutowska, B. & Wardencki, W. Application of gas chromatography–olfactometry (GC–O) in analysis and quality assessment of alcoholic beverages – A review. Food Chem. 107 , 449–463 (2008).
Article CAS Google Scholar
Legin, A., Rudnitskaya, A., Seleznev, B. & Vlasov, Y. Electronic tongue for quality assessment of ethanol, vodka and eau-de-vie. Anal. Chim. Acta 534 , 129–135 (2005).
Loutfi, A., Coradeschi, S., Mani, G. K., Shankar, P. & Rayappan, J. B. B. Electronic noses for food quality: A review. J. Food Eng. 144 , 103–111 (2015).
Ahn, Y.-Y., Ahnert, S. E., Bagrow, J. P. & Barabási, A.-L. Flavor network and the principles of food pairing. Sci. Rep. 1 , 196 (2011).
Article CAS PubMed PubMed Central Google Scholar
Bartoshuk, L. M. & Klee, H. J. Better fruits and vegetables through sensory analysis. Curr. Biol. 23 , R374–R378 (2013).
Article CAS PubMed Google Scholar
Piggott, J. R. Design questions in sensory and consumer science. Food Qual. Prefer. 3293 , 217–220 (1995).
Article Google Scholar
Kermit, M. & Lengard, V. Assessing the performance of a sensory panel-panellist monitoring and tracking. J. Chemom. 19 , 154–161 (2005).
Cook, D. J., Hollowood, T. A., Linforth, R. S. T. & Taylor, A. J. Correlating instrumental measurements of texture and flavour release with human perception. Int. J. Food Sci. Technol. 40 , 631–641 (2005).
Chinchanachokchai, S., Thontirawong, P. & Chinchanachokchai, P. A tale of two recommender systems: The moderating role of consumer expertise on artificial intelligence based product recommendations. J. Retail. Consum. Serv. 61 , 1–12 (2021).
Ross, C. F. Sensory science at the human-machine interface. Trends Food Sci. Technol. 20 , 63–72 (2009).
Chambers, E. IV & Koppel, K. Associations of volatile compounds with sensory aroma and flavor: The complex nature of flavor. Molecules 18 , 4887–4905 (2013).
Pinu, F. R. Metabolomics—The new frontier in food safety and quality research. Food Res. Int. 72 , 80–81 (2015).
Danezis, G. P., Tsagkaris, A. S., Brusic, V. & Georgiou, C. A. Food authentication: state of the art and prospects. Curr. Opin. Food Sci. 10 , 22–31 (2016).
Shepherd, G. M. Smell images and the flavour system in the human brain. Nature 444 , 316–321 (2006).
Meilgaard, M. C. Prediction of flavor differences between beers from their chemical composition. J. Agric. Food Chem. 30 , 1009–1017 (1982).
Xu, L. et al. Widespread receptor-driven modulation in peripheral olfactory coding. Science 368 , eaaz5390 (2020).
Kupferschmidt, K. Following the flavor. Science 340 , 808–809 (2013).
Billesbølle, C. B. et al. Structural basis of odorant recognition by a human odorant receptor. Nature 615 , 742–749 (2023).
Article ADS PubMed PubMed Central Google Scholar
Smith, B. Perspective: Complexities of flavour. Nature 486 , S6–S6 (2012).
Pfister, P. et al. Odorant receptor inhibition is fundamental to odor encoding. Curr. Biol. 30 , 2574–2587 (2020).
Moskowitz, H. W., Kumaraiah, V., Sharma, K. N., Jacobs, H. L. & Sharma, S. D. Cross-cultural differences in simple taste preferences. Science 190 , 1217–1218 (1975).
Eriksson, N. et al. A genetic variant near olfactory receptor genes influences cilantro preference. Flavour 1 , 22 (2012).
Ferdenzi, C. et al. Variability of affective responses to odors: Culture, gender, and olfactory knowledge. Chem. Senses 38 , 175–186 (2013).
Article PubMed Google Scholar
Lawless, H. T. & Heymann, H. Sensory evaluation of food: Principles and practices. (Springer, New York, NY). https://doi.org/10.1007/978-1-4419-6488-5 (2010).
Colantonio, V. et al. Metabolomic selection for enhanced fruit flavor. Proc. Natl. Acad. Sci. 119 , e2115865119 (2022).
Fritz, F., Preissner, R. & Banerjee, P. VirtualTaste: a web server for the prediction of organoleptic properties of chemical compounds. Nucleic Acids Res 49 , W679–W684 (2021).
Tuwani, R., Wadhwa, S. & Bagler, G. BitterSweet: Building machine learning models for predicting the bitter and sweet taste of small molecules. Sci. Rep. 9 , 1–13 (2019).
Dagan-Wiener, A. et al. Bitter or not? BitterPredict, a tool for predicting taste from chemical structure. Sci. Rep. 7 , 1–13 (2017).
Pallante, L. et al. Toward a general and interpretable umami taste predictor using a multi-objective machine learning approach. Sci. Rep. 12 , 1–11 (2022).
Malavolta, M. et al. A survey on computational taste predictors. Eur. Food Res. Technol. 248 , 2215–2235 (2022).
Lee, B. K. et al. A principal odor map unifies diverse tasks in olfactory perception. Science 381 , 999–1006 (2023).
Mayhew, E. J. et al. Transport features predict if a molecule is odorous. Proc. Natl. Acad. Sci. 119 , e2116576119 (2022).
Niu, Y. et al. Sensory evaluation of the synergism among ester odorants in light aroma-type liquor by odor threshold, aroma intensity and flash GC electronic nose. Food Res. Int. 113 , 102–114 (2018).
Yu, P., Low, M. Y. & Zhou, W. Design of experiments and regression modelling in food flavour and sensory analysis: A review. Trends Food Sci. Technol. 71 , 202–215 (2018).
Oladokun, O. et al. The impact of hop bitter acid and polyphenol profiles on the perceived bitterness of beer. Food Chem. 205 , 212–220 (2016).
Linforth, R., Cabannes, M., Hewson, L., Yang, N. & Taylor, A. Effect of fat content on flavor delivery during consumption: An in vivo model. J. Agric. Food Chem. 58 , 6905–6911 (2010).
Guo, S., Na Jom, K. & Ge, Y. Influence of roasting condition on flavor profile of sunflower seeds: A flavoromics approach. Sci. Rep. 9 , 11295 (2019).
Ren, Q. et al. The changes of microbial community and flavor compound in the fermentation process of Chinese rice wine using Fagopyrum tataricum grain as feedstock. Sci. Rep. 9 , 3365 (2019).
Hastie, T., Friedman, J. & Tibshirani, R. The Elements of Statistical Learning. (Springer, New York, NY). https://doi.org/10.1007/978-0-387-21606-5 (2001).
Dietz, C., Cook, D., Huismann, M., Wilson, C. & Ford, R. The multisensory perception of hop essential oil: a review. J. Inst. Brew. 126 , 320–342 (2020).
CAS Google Scholar
Roncoroni, Miguel & Verstrepen, Kevin Joan. Belgian Beer: Tested and Tasted. (Lannoo, 2018).
Meilgaard, M. Flavor chemistry of beer: Part II: Flavor and threshold of 239 aroma volatiles. in (1975).
Bokulich, N. A. & Bamforth, C. W. The microbiology of malting and brewing. Microbiol. Mol. Biol. Rev. MMBR 77 , 157–172 (2013).
Dzialo, M. C., Park, R., Steensels, J., Lievens, B. & Verstrepen, K. J. Physiology, ecology and industrial applications of aroma formation in yeast. FEMS Microbiol. Rev. 41 , S95–S128 (2017).
Article PubMed PubMed Central Google Scholar
Datta, A. et al. Computer-aided food engineering. Nat. Food 3 , 894–904 (2022).
American Society of Brewing Chemists. Beer Methods. (American Society of Brewing Chemists, St. Paul, MN, U.S.A.).
Olaniran, A. O., Hiralal, L., Mokoena, M. P. & Pillay, B. Flavour-active volatile compounds in beer: production, regulation and control. J. Inst. Brew. 123 , 13–23 (2017).
Verstrepen, K. J. et al. Flavor-active esters: Adding fruitiness to beer. J. Biosci. Bioeng. 96 , 110–118 (2003).
Meilgaard, M. C. Flavour chemistry of beer. part I: flavour interaction between principal volatiles. Master Brew. Assoc. Am. Tech. Q 12 , 107–117 (1975).
Briggs, D. E., Boulton, C. A., Brookes, P. A. & Stevens, R. Brewing 227–254. (Woodhead Publishing). https://doi.org/10.1533/9781855739062.227 (2004).
Bossaert, S., Crauwels, S., De Rouck, G. & Lievens, B. The power of sour - A review: Old traditions, new opportunities. BrewingScience 72 , 78–88 (2019).
Google Scholar
Verstrepen, K. J. et al. Flavor active esters: Adding fruitiness to beer. J. Biosci. Bioeng. 96 , 110–118 (2003).
Snauwaert, I. et al. Microbial diversity and metabolite composition of Belgian red-brown acidic ales. Int. J. Food Microbiol. 221 , 1–11 (2016).
Spitaels, F. et al. The microbial diversity of traditional spontaneously fermented lambic beer. PLoS ONE 9 , e95384 (2014).
Blanco, C. A., Andrés-Iglesias, C. & Montero, O. Low-alcohol Beers: Flavor Compounds, Defects, and Improvement Strategies. Crit. Rev. Food Sci. Nutr. 56 , 1379–1388 (2016).
Jackowski, M. & Trusek, A. Non-Alcohol. beer Prod. – Overv. 20 , 32–38 (2018).
Takoi, K. et al. The contribution of geraniol metabolism to the citrus flavour of beer: Synergy of geraniol and β-citronellol under coexistence with excess linalool. J. Inst. Brew. 116 , 251–260 (2010).
Kroeze, J. H. & Bartoshuk, L. M. Bitterness suppression as revealed by split-tongue taste stimulation in humans. Physiol. Behav. 35 , 779–783 (1985).
Mennella, J. A. et al. A spoonful of sugar helps the medicine go down”: Bitter masking bysucrose among children and adults. Chem. Senses 40 , 17–25 (2015).
Wietstock, P., Kunz, T., Perreira, F. & Methner, F.-J. Metal chelation behavior of hop acids in buffered model systems. BrewingScience 69 , 56–63 (2016).
Sancho, D., Blanco, C. A., Caballero, I. & Pascual, A. Free iron in pale, dark and alcohol-free commercial lager beers. J. Sci. Food Agric. 91 , 1142–1147 (2011).
Rodrigues, H. & Parr, W. V. Contribution of cross-cultural studies to understanding wine appreciation: A review. Food Res. Int. 115 , 251–258 (2019).
Korneva, E. & Blockeel, H. Towards better evaluation of multi-target regression models. in ECML PKDD 2020 Workshops (eds. Koprinska, I. et al.) 353–362 (Springer International Publishing, Cham, 2020). https://doi.org/10.1007/978-3-030-65965-3_23 .
Gastón Ares. Mathematical and Statistical Methods in Food Science and Technology. (Wiley, 2013).
Grinsztajn, L., Oyallon, E. & Varoquaux, G. Why do tree-based models still outperform deep learning on tabular data? Preprint at http://arxiv.org/abs/2207.08815 (2022).
Gries, S. T. Statistics for Linguistics with R: A Practical Introduction. in Statistics for Linguistics with R (De Gruyter Mouton, 2021). https://doi.org/10.1515/9783110718256 .
Lundberg, S. M. et al. From local explanations to global understanding with explainable AI for trees. Nat. Mach. Intell. 2 , 56–67 (2020).
Ickes, C. M. & Cadwallader, K. R. Effects of ethanol on flavor perception in alcoholic beverages. Chemosens. Percept. 10 , 119–134 (2017).
Kato, M. et al. Influence of high molecular weight polypeptides on the mouthfeel of commercial beer. J. Inst. Brew. 127 , 27–40 (2021).
Wauters, R. et al. Novel Saccharomyces cerevisiae variants slow down the accumulation of staling aldehydes and improve beer shelf-life. Food Chem. 398 , 1–11 (2023).
Li, H., Jia, S. & Zhang, W. Rapid determination of low-level sulfur compounds in beer by headspace gas chromatography with a pulsed flame photometric detector. J. Am. Soc. Brew. Chem. 66 , 188–191 (2008).
Dercksen, A., Laurens, J., Torline, P., Axcell, B. C. & Rohwer, E. Quantitative analysis of volatile sulfur compounds in beer using a membrane extraction interface. J. Am. Soc. Brew. Chem. 54 , 228–233 (1996).
Molnar, C. Interpretable Machine Learning: A Guide for Making Black-Box Models Interpretable. (2020).
Zhao, Q. & Hastie, T. Causal interpretations of black-box models. J. Bus. Econ. Stat. Publ. Am. Stat. Assoc. 39 , 272–281 (2019).
Article MathSciNet Google Scholar
Hastie, T., Tibshirani, R. & Friedman, J. The Elements of Statistical Learning. (Springer, 2019).
Labrado, D. et al. Identification by NMR of key compounds present in beer distillates and residual phases after dealcoholization by vacuum distillation. J. Sci. Food Agric. 100 , 3971–3978 (2020).
Lusk, L. T., Kay, S. B., Porubcan, A. & Ryder, D. S. Key olfactory cues for beer oxidation. J. Am. Soc. Brew. Chem. 70 , 257–261 (2012).
Gonzalez Viejo, C., Torrico, D. D., Dunshea, F. R. & Fuentes, S. Development of artificial neural network models to assess beer acceptability based on sensory properties using a robotic pourer: A comparative model approach to achieve an artificial intelligence system. Beverages 5 , 33 (2019).
Gonzalez Viejo, C., Fuentes, S., Torrico, D. D., Godbole, A. & Dunshea, F. R. Chemical characterization of aromas in beer and their effect on consumers liking. Food Chem. 293 , 479–485 (2019).
Gilbert, J. L. et al. Identifying breeding priorities for blueberry flavor using biochemical, sensory, and genotype by environment analyses. PLOS ONE 10 , 1–21 (2015).
Goulet, C. et al. Role of an esterase in flavor volatile variation within the tomato clade. Proc. Natl. Acad. Sci. 109 , 19009–19014 (2012).
Article ADS CAS PubMed PubMed Central Google Scholar
Borisov, V. et al. Deep Neural Networks and Tabular Data: A Survey. IEEE Trans. Neural Netw. Learn. Syst. 1–21 https://doi.org/10.1109/TNNLS.2022.3229161 (2022).
Statista. Statista Consumer Market Outlook: Beer - Worldwide.
Seitz, H. K. & Stickel, F. Molecular mechanisms of alcoholmediated carcinogenesis. Nat. Rev. Cancer 7 , 599–612 (2007).
Voordeckers, K. et al. Ethanol exposure increases mutation rate through error-prone polymerases. Nat. Commun. 11 , 3664 (2020).
Goelen, T. et al. Bacterial phylogeny predicts volatile organic compound composition and olfactory response of an aphid parasitoid. Oikos 129 , 1415–1428 (2020).
Article ADS Google Scholar
Reher, T. et al. Evaluation of hop (Humulus lupulus) as a repellent for the management of Drosophila suzukii. Crop Prot. 124 , 104839 (2019).
Stein, S. E. An integrated method for spectrum extraction and compound identification from gas chromatography/mass spectrometry data. J. Am. Soc. Mass Spectrom. 10 , 770–781 (1999).
American Society of Brewing Chemists. Sensory Analysis Methods. (American Society of Brewing Chemists, St. Paul, MN, U.S.A., 1992).
McAuley, J., Leskovec, J. & Jurafsky, D. Learning Attitudes and Attributes from Multi-Aspect Reviews. Preprint at https://doi.org/10.48550/arXiv.1210.3926 (2012).
Meilgaard, M. C., Carr, B. T. & Carr, B. T. Sensory Evaluation Techniques. (CRC Press, Boca Raton). https://doi.org/10.1201/b16452 (2014).
Schreurs, M. et al. Data from: Predicting and improving complex beer flavor through machine learning. Zenodo https://doi.org/10.5281/zenodo.10653704 (2024).
Download references
Acknowledgements
We thank all lab members for their discussions and thank all tasting panel members for their contributions. Special thanks go out to Dr. Karin Voordeckers for her tremendous help in proofreading and improving the manuscript. M.S. was supported by a Baillet-Latour fellowship, L.C. acknowledges financial support from KU Leuven (C16/17/006), F.A.T. was supported by a PhD fellowship from FWO (1S08821N). Research in the lab of K.J.V. is supported by KU Leuven, FWO, VIB, VLAIO and the Brewing Science Serves Health Fund. Research in the lab of T.W. is supported by FWO (G.0A51.15) and KU Leuven (C16/17/006).
Author information
These authors contributed equally: Michiel Schreurs, Supinya Piampongsant, Miguel Roncoroni.
Authors and Affiliations
VIB—KU Leuven Center for Microbiology, Gaston Geenslaan 1, B-3001, Leuven, Belgium
Michiel Schreurs, Supinya Piampongsant, Miguel Roncoroni, Lloyd Cool, Beatriz Herrera-Malaver, Florian A. Theßeling & Kevin J. Verstrepen
CMPG Laboratory of Genetics and Genomics, KU Leuven, Gaston Geenslaan 1, B-3001, Leuven, Belgium
Leuven Institute for Beer Research (LIBR), Gaston Geenslaan 1, B-3001, Leuven, Belgium
Laboratory of Socioecology and Social Evolution, KU Leuven, Naamsestraat 59, B-3000, Leuven, Belgium
Lloyd Cool, Christophe Vanderaa & Tom Wenseleers
VIB Bioinformatics Core, VIB, Rijvisschestraat 120, B-9052, Ghent, Belgium
Łukasz Kreft & Alexander Botzki
AB InBev SA/NV, Brouwerijplein 1, B-3000, Leuven, Belgium
Philippe Malcorps & Luk Daenen
You can also search for this author in PubMed Google Scholar
Contributions
S.P., M.S. and K.J.V. conceived the experiments. S.P., M.S. and K.J.V. designed the experiments. S.P., M.S., M.R., B.H. and F.A.T. performed the experiments. S.P., M.S., L.C., C.V., L.K., A.B., P.M., L.D., T.W. and K.J.V. contributed analysis ideas. S.P., M.S., L.C., C.V., T.W. and K.J.V. analyzed the data. All authors contributed to writing the manuscript.
Corresponding author
Correspondence to Kevin J. Verstrepen .
Ethics declarations
Competing interests.
K.J.V. is affiliated with bar.on. The other authors declare no competing interests.
Peer review
Peer review information.
Nature Communications thanks Florian Bauer, Andrew John Macintosh and the other, anonymous, reviewer(s) for their contribution to the peer review of this work. A peer review file is available.
Additional information
Publisher’s note Springer Nature remains neutral with regard to jurisdictional claims in published maps and institutional affiliations.
Supplementary information
Supplementary information, peer review file, description of additional supplementary files, supplementary data 1, supplementary data 2, supplementary data 3, supplementary data 4, supplementary data 5, supplementary data 6, supplementary data 7, reporting summary, source data, source data, rights and permissions.
Open Access This article is licensed under a Creative Commons Attribution 4.0 International License, which permits use, sharing, adaptation, distribution and reproduction in any medium or format, as long as you give appropriate credit to the original author(s) and the source, provide a link to the Creative Commons licence, and indicate if changes were made. The images or other third party material in this article are included in the article’s Creative Commons licence, unless indicated otherwise in a credit line to the material. If material is not included in the article’s Creative Commons licence and your intended use is not permitted by statutory regulation or exceeds the permitted use, you will need to obtain permission directly from the copyright holder. To view a copy of this licence, visit http://creativecommons.org/licenses/by/4.0/ .
Reprints and permissions
About this article
Cite this article.
Schreurs, M., Piampongsant, S., Roncoroni, M. et al. Predicting and improving complex beer flavor through machine learning. Nat Commun 15 , 2368 (2024). https://doi.org/10.1038/s41467-024-46346-0
Download citation
Received : 30 October 2023
Accepted : 21 February 2024
Published : 26 March 2024
DOI : https://doi.org/10.1038/s41467-024-46346-0
Share this article
Anyone you share the following link with will be able to read this content:
Sorry, a shareable link is not currently available for this article.
Provided by the Springer Nature SharedIt content-sharing initiative
By submitting a comment you agree to abide by our Terms and Community Guidelines . If you find something abusive or that does not comply with our terms or guidelines please flag it as inappropriate.
Quick links
- Explore articles by subject
- Guide to authors
- Editorial policies
Sign up for the Nature Briefing: Translational Research newsletter — top stories in biotechnology, drug discovery and pharma.


IMAGES
VIDEO
COMMENTS
Artificial Intelligence offers a supportive hand in thesis writing, adeptly navigating vast datasets, suggesting enhancements in writing, and refining the narrative. With the integration of AI writing assistant, instead of requiring you to manually sift through endless articles, AI tools can spotlight the most pertinent pieces in mere moments.
Revolutionizing Academic Writing: The 10 Best AI Tools for Streamlining Thesis Creation. Thesis writing is a challenging yet integral part of academic pursuits, and the integration of Artificial Intelligence (AI) tools has revolutionized the research and writing process. In this article, we explore the top 10 AI tools that are invaluable for thesis writing, offering efficiency, precision, and ...
AI writing tools are artificial intelligence (AI) software applications like ChatGPT that help to automate or assist the writing process. These tools use machine learning algorithms to generate human-sounding text in response to users' text-based prompts. Other AI tools, such as grammar checkers, paraphrasers and summarizers serve more ...
The integration of artificial intelligence in the world of academic research is nothing short of revolutionary. With the array of AI tools we've explored today - from research and mapping, literature review, peer-reviewed papers reading, scientific writing, to academic editing and grant writing - the landscape of research is significantly ...
Artificial intelligence (AI) has become a valuable asset in the thesis writing process. AI tools can assist you in various stages, from research to writing and editing.
Text generator (chatbot) based on artificial intelligence and developed by the company OpenAI. Aims to generate conversations that are as human-like as possible. Transforms input into output by "language modeling" technique. Output texts are generated as the result of a probability calculation.
The use of artificial intelligence (AI) in academic writing can be divided into two broad categories: those that assist authors in the writing process; and those that are used to evaluate and ...
As for the results of a training program involving ten students: (1) Students can be able to use ai writing tools (Quilbot and Grammarly), (2) Students can draw on artificial intelligence writing ...
Artificial Intelligence. Artificial Intelligence. Recent advances in artificial intelligence (AI) for writing (including CoPilot and ChatGPT) can quickly create coherent, cohesive prose and paragraphs on a seemingly limitless set of topics. The potential for abuse in academic integrity is clear, and our students are likely using these tools ...
Andy Tay. Credit: gmast3r/Getty Images. Writing tools powered by artificial intelligence (AI) have the potential to reduce manuscript preparation time to a few days, or hours. Deep-learning ...
Using AI ethically in writing assignments. The use of generative artificial intelligence in writing isn't an either/or proposition. Rather, think of a continuum in which AI can be used at nearly any point to inspire, ideate, structure, and format writing. It can also help with research, feedback, summarization, and creation.
This webinar aims to help researchers understand how Trinka, an advanced artificial intelligence-powered writing assistant, can assess and enhance the quality of their thesis. It will further discuss how this dedicated AI-based tool can assist researchers to resolve the longstanding challenge of communicating their thesis in a grammatically correct and scientifically structured manner.
In the rapidly evolving landscape of academia, harnessing the power of artificial intelligence (AI) tools has become a game-changer for doctoral thesis writing. The fusion of human intellect with ...
Almira Osmanovic Thunström is a scientist who studies the role of artificial intelligence and virtual reality in mental health care. She found herself curious if GPT-3 could write about itself, so she asked it to respond to the following prompt: "Write an academic thesis in 500 words about GPT-3 and add scientific references and citations ...
Since ChatGPT burst onto the scene last year, AI writing tools have been big news. While they aren't taking over the world (yet), they are rapidly maturing and have reached the point where they can absolutely be useful—at least in the right circumstances. Used correctly, these AI text generators can help you work better and faster, and create more polished and on-brand copy.
This thesis is about how artificial intelligence is impacting students in universities and universi-ties of applied sciences. Artificial intelligence has developed a lot in the past years, each day ... tor, help dyslexic students, assist to write code, and it can even adjust to the student's learning style. This thesis covers also what ...
Once you have a clear idea of the topic and what interests you, go on to the next step. 2. Ask a research question. You know what you're going to write about, at least broadly. Now you just have to narrow in on an angle or focus appropriate to the length of your assignment.
In this blog, we embark on a journey to delve into 12 Artificial Intelligence Topics that stand as promising avenues for thorough research and exploration. Table of Contents. 1) Top Artificial Intelligence Topics for Research. a) Natural Language Processing. b) Computer vision. c) Reinforcement Learning. d) Explainable AI (XAI)
on t he Impact of Artificial Intelligence on Writing Competence," International Journal of Multidisciplinary Research and Publications (IJMRA P) , Volume 6, Issue 6, pp. 29 - 34 , 20 23 .
Writing and Artificial Intelligence. "Three college students are working on a writing assignment. A robot is assisting them with their work.". Created from text prompt in Adobe Firefly, July 20, 2023. We're including this guide to "Writing with AI" in Write What Matters because it's clear that generative AI tools, including ...
Writing a thesis at the end of your degree can often be confusing, as it is very different from previous semesters, especially in degrees like Computer Science or Artificial Intelligence.
KEYWORDS: Artificial intelligence, writing tools, perspective, I. INTRODUCTION The essence of writing is one of the most challenging components of English language ability, particularly in an EFL environment (Anam, 2021). It is caused by the fact that procedural processes and complicated parts must be used to generate a quality piece
In this lesson, students compare AI and human-generated texts to analyze the affordances and limitations of large language models. They'll score, guess origins, and discuss differences, then decide which aspects to adopt or avoid in their writing. The lesson culminates in a discussion of what aspects of the AI-generated writing students should emulate in their own writing and what aspects they ...
Many universities provide full-text access to their dissertations via a digital repository. If you know the title of a particular dissertation or thesis, try doing a Google search. OATD (Open Access Theses and Dissertations) Aims to be the best possible resource for finding open access graduate theses and dissertations published around the world with metadata from over 800 colleges ...
TensorRT-LLM running on NVIDIA H200 Tensor Core GPUs — the latest, memory-enhanced Hopper GPUs — delivered the fastest performance running inference in MLPerf's biggest test of generative AI to date. The new benchmark uses the largest version of Llama 2, a state-of-the-art large language model packing 70 billion parameters.
Modern society is rapidly changing in the fourth industrial revolution era. In particular, technologies of the fourth industrial revolution (e.g., artificial intelligence [AI], big data, Internet of Things [IoT], and drones), discussed for the first time in 2016 at the World Economic Forum (WEF), are rapidly transforming society as a whole while having a profound impact on all aspects of human ...
Cognition Labs is reportedly aiming to become the next multibillion-dollar artificial intelligence (AI) startup.. The company, which is developing an AI tool for writing code, is in discussions ...
Larry Ellison and Seema Verma 's op-ed " It's Time to Hand Cybersecurity Over to the Computers " (April 2) struck a neuron. Such an autonomously generated artificial-intelligence program ...
Gov. Bill Lee of Tennessee signed a bill to prevent the use of A.I. to copy a performer's voice. It is the first such measure in the United States. Economists doubt that artificial intelligence ...
The perception and appreciation of food flavor depends on many interacting chemical compounds and external factors, and therefore proves challenging to understand and predict. Here, we combine ...