Academia.edu no longer supports Internet Explorer.
To browse Academia.edu and the wider internet faster and more securely, please take a few seconds to upgrade your browser .
Enter the email address you signed up with and we'll email you a reset link.
- We're Hiring!
- Help Center
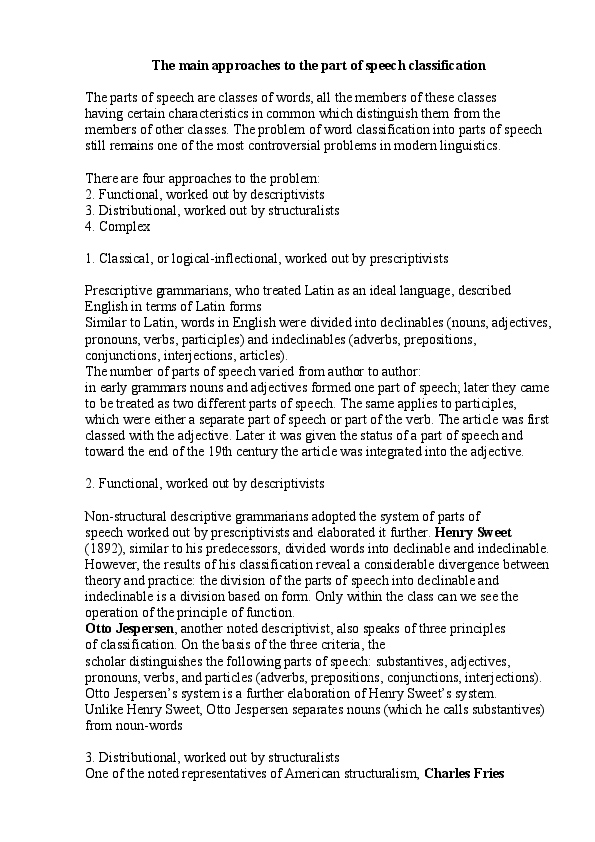

1. The main approaches to the part of speech classification

Related Papers
Kresta Niña Manipol
i n t ro d u c t i o n In every language we find groups of words that share grammatical characteristics. These groups are called " parts of speech, " and we examine them in this chapter and the next. Though many writers on language refer to " the eight parts of speech " (e.g., Weaver 1996: 254), the actual number of parts of speech we need to recognize in a language is determined by how fine-grained our analysis of the language is—the more fine-grained, the greater the number of parts of speech that will be distinguished. In this book we distinguish nouns, verbs, adjectives, and adverbs (the major parts of speech), and pronouns, wh-words, articles, auxiliary verbs, prepositions, intensifiers, conjunctions, and particles (the minor parts of speech). Every literate person needs at least a minimal understanding of parts of speech in order to be able to use such commonplace items as dictionaries and thesauruses, which classify words according to their parts (and sub-parts) of speech. For example, the American Heritage Dictionary (4 th edition, p. xxxi) distinguishes adjectives, adverbs, conjunctions, definite articles , indefinite articles, interjections, nouns, prepositions, pronouns, and verbs. It also distinguishes transitive, intransitive, and auxiliary verbs. Writers and writing teachers need to know about parts of speech in order to be able to use and teach about style manuals and school grammars. Regardless of their discipline, teachers need this information to be able to help students expand the contexts in which they can effectively communicate. A part of speech is a set of words with some grammatical characteristic(s) in common and each part of speech differs in grammatical characteristics from every other part of speech, e.g., nouns have different properties from verbs, which have different properties from adjectives, and so on. Part of speech analysis depends on knowing (or discovering) the distinguishing properties of the various word sets. This chapter describes several kinds of properties that separate the major parts of speech from each other and de
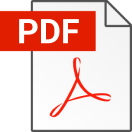
Maryna Pryadko
Aleksandra Zayats
Studies in Language
Luca Alfieri
Paco Aranda
mné ben hlima
Daniel García Velasco
In Martin Everaert, Marijana Marelj, and Eric Reuland (eds.), Concepts, Syntax and their Interfcae. MIT Press. 2016
Tal Siloni , Julia Horvath
Mohamad Nizar
Syntax for Bloomfield (1933), is the study of free forms which consist entirely of free forms. What Bloomfield thought was criticized by later scholars, they highlighted as a criterion form class membership (and hence syntactic equivalence) best expressed in terms of substitution. A form class is a set of forms (simple or complex, free or bound), any one of which can be replaced by another form in a particular construction or set of constructions throughout a language's sentences. Word class or part of speech is a group of words in a language unit based on categories of form, function and meaning in the grammatical system. In the previous article, the author explained that the lexeme bundle is not a large homogeneous collection, but consists of the categories of nouns, verbs, adjectives, prepositions, inflections, determiners, comparative adverbs and complements which according to Newson et.al (2004: 5-6) are word category. A speech contains structured categories of words and is a study of the context of words in phrases, clauses and sentences in the language system (syntax). Newson et al. (2004: 6-10) explained that word categories are divided into two typologies, they are thematic and functional. Nouns, verbs, adjectives, and prepositions are thematic categories. Function categories are inflections, determiners, comparative adverbs, and complements. Start investigating nouns and groups of nouns as the most common elements in sentence construction that can be structured in a complex or sophisticated way with a lot of information. Again, observing words is sophisticated and this is important for linguistic researchers who are responsible for maintaining the rules of English that are referred to by linguistic theories. The importance of research is aimed at teaching English. Teachers are responsible for guiding their students about how sentences behave in English, whether written or spoken, as a mother tongue or a foreign language. Teachers are also responsible for guiding how to interpret other languages into English accurately. This is the current concern of linguistic studies in English. This article only presents the thoughts of linguistic experts about nouns and also the case examples they provide. This will encourage researchers to explore a number of noun cases, which provides a wide field for syntactic research in English. That's why this article has written only as an introduction to word analysis, helping linguistic students or novice syntax researchers in English. Weaknesses in writing this article are the responsibility of the author and are open to criticism.
RELATED PAPERS
mulia zuhelmi
shahzad Masih
MEGARON / Yıldız Technical University, Faculty of Architecture E-Journal
fulya üstün demirkaya , MERVE YADİGAROĞLU YAVRU
The Journal of Adhesive Dentistry
Atsushi Kameyama
Ingeniería del agua
Mariângela Fontes Santiago
Seminars in Cell & Developmental Biology
Yasuhito Sakuraba
Sushant Shinde
IEEE Transactions on Image Processing
Pulak Purkait
İnformasiya təhlükəsizliyinin aktual multidissiplinar elmi-praktiki problemləri V respublika konfransının materialları
Məsumə Məmmədova
Endangered Species Research
Scott Roberton
IRA WIRASARI
Wilson Enderson López Cardona
Nusret Mutlu
Scientific reports
Sandeep Gupta
Muhammad Kholid
Francisco Elzevir Dantas Júnior
Hungarológiai Közlemények
Brazilian Creative Industries Journal
Planta medica
O. Potterat
Ludomir Jankowski
Emerald reach proceedings series
Teresa Beste
Manufacturing review
Catalin DUCU
Sigrid Rettenbacher
DHEA ALISYAH
- We're Hiring!
- Help Center
- Find new research papers in:
- Health Sciences
- Earth Sciences
- Cognitive Science
- Mathematics
- Computer Science
- Academia ©2024
- Tools and Resources
- Customer Services
- Applied Linguistics
- Biology of Language
- Cognitive Science
- Computational Linguistics
- Historical Linguistics
- History of Linguistics
- Language Families/Areas/Contact
- Linguistic Theories
- Neurolinguistics
- Phonetics/Phonology
- Psycholinguistics
- Sign Languages
- Sociolinguistics
- Share This Facebook LinkedIn Twitter
Article contents
Parts of speech, lexical categories, and word classes in morphology.
- Jaklin Kornfilt Jaklin Kornfilt Department of Languages, Literatures, and Linguistics, Syracuse University
- https://doi.org/10.1093/acrefore/9780199384655.013.606
- Published online: 30 January 2020
The term “part of speech” is a traditional one that has been in use since grammars of Classical Greek (e.g., Dionysius Thrax) and Latin were compiled; for all practical purposes, it is synonymous with the term “word class.” The term refers to a system of word classes, whereby class membership depends on similar syntactic distribution and morphological similarity (as well as, in a limited fashion, on similarity in meaning—a point to which we shall return). By “morphological similarity,” reference is made to functional morphemes that are part of words belonging to the same word class. Some examples for both criteria follow: The fact that in English, nouns can be preceded by a determiner such as an article (e.g., a book , the apple ) illustrates syntactic distribution. Morphological similarity among members of a given word class can be illustrated by the many adverbs in English that are derived by attaching the suffix – ly , that is, a functional morpheme, to an adjective ( quick, quick-ly ). A morphological test for nouns in English and many other languages is whether they can bear plural morphemes. Verbs can bear morphology for tense, aspect, and mood, as well as voice morphemes such as passive, causative, or reflexive, that is, morphemes that alter the argument structure of the verbal root. Adjectives typically co-occur with either bound or free morphemes that function as comparative and superlative markers. Syntactically, they modify nouns, while adverbs modify word classes that are not nouns—for example, verbs and adjectives.
Most traditional and descriptive approaches to parts of speech draw a distinction between major and minor word classes. The four parts of speech just mentioned—nouns, verbs, adjectives, and adverbs—constitute the major word classes, while a number of others, for example, adpositions, pronouns, conjunctions, determiners, and interjections, make up the minor word classes. Under some approaches, pronouns are included in the class of nouns, as a subclass.
While the minor classes are probably not universal, (most of) the major classes are. It is largely assumed that nouns, verbs, and probably also adjectives are universal parts of speech. Adverbs might not constitute a universal word class.
There are technical terms that are equivalents to the terms of major versus minor word class, such as content versus function words, lexical versus functional categories, and open versus closed classes, respectively. However, these correspondences might not always be one-to-one.
More recent approaches to word classes don’t recognize adverbs as belonging to the major classes; instead, adpositions are candidates for this status under some of these accounts, for example, as in Jackendoff (1977). Under some other theoretical accounts, such as Chomsky (1981) and Baker (2003), only the three word classes noun, verb, and adjective are major or lexical categories. All of the accounts just mentioned are based on binary distinctive features; however, the features used differ from each other. While Chomsky uses the two category features [N] and [V], Jackendoff uses the features [Subj] and [Obj], among others, focusing on the ability of nouns, verbs, adjectives, and adpositions to take (directly, without the help of other elements) subjects (thus characterizing verbs and nouns) or objects (thus characterizing verbs and adpositions). Baker (2003), too, uses the property of taking subjects, but attributes it only to verbs. In his approach, the distinctive feature of bearing a referential index characterizes nouns, and only those. Adjectives are characterized by the absence of both of these distinctive features.
Another important issue addressed by theoretical studies on lexical categories is whether those categories are formed pre-syntactically, in a morphological component of the lexicon, or whether they are constructed in the syntax or post-syntactically. Jackendoff (1977) is an example of a lexicalist approach to lexical categories, while Marantz (1997), and Borer (2003, 2005a, 2005b, 2013) represent an account where the roots of words are category-neutral, and where their membership to a particular lexical category is determined by their local syntactic context. Baker (2003) offers an account that combines properties of both approaches: words are built in the syntax and not pre-syntactically; however, roots do have category features that are inherent to them.
There are empirical phenomena, such as phrasal affixation, phrasal compounding, and suspended affixation, that strongly suggest that a post-syntactic morphological component should be allowed, whereby “syntax feeds morphology.”
- parts of speech
- word classes
- lexical categories
- functional categories
- closed versus open word classes
- features of lexical categories
- pre-syntactic word formation
- post-syntactic word formation
You do not currently have access to this article
Please login to access the full content.
Access to the full content requires a subscription
Printed from Oxford Research Encyclopedias, Linguistics. Under the terms of the licence agreement, an individual user may print out a single article for personal use (for details see Privacy Policy and Legal Notice).
date: 18 April 2024
- Cookie Policy
- Privacy Policy
- Legal Notice
- [66.249.64.20|193.7.198.129]
- 193.7.198.129
Character limit 500 /500
Have a language expert improve your writing
Run a free plagiarism check in 10 minutes, generate accurate citations for free.
- Knowledge Base
- Parts of speech
The 8 Parts of Speech | Chart, Definition & Examples
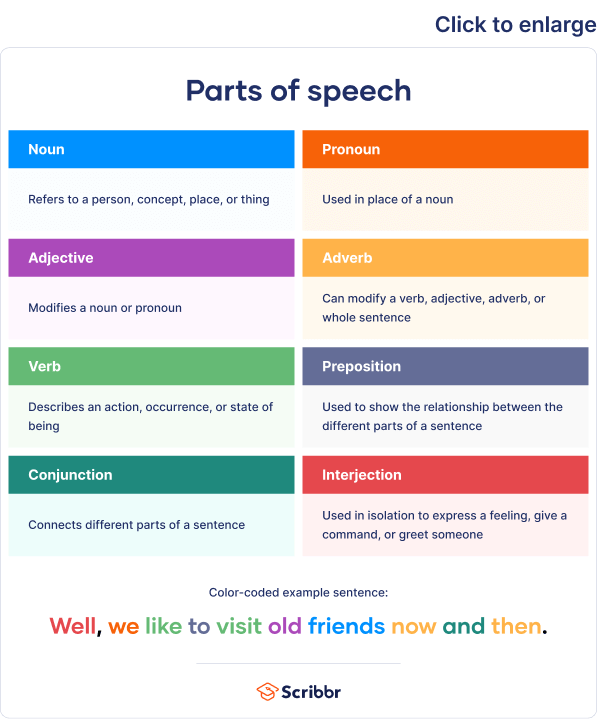
A part of speech (also called a word class ) is a category that describes the role a word plays in a sentence. Understanding the different parts of speech can help you analyze how words function in a sentence and improve your writing.
The parts of speech are classified differently in different grammars, but most traditional grammars list eight parts of speech in English: nouns , pronouns , verbs , adjectives , adverbs , prepositions , conjunctions , and interjections . Some modern grammars add others, such as determiners and articles .
Many words can function as different parts of speech depending on how they are used. For example, “laugh” can be a noun (e.g., “I like your laugh”) or a verb (e.g., “don’t laugh”).
Table of contents
- Prepositions
- Conjunctions
- Interjections
Other parts of speech
Interesting language articles, frequently asked questions.
A noun is a word that refers to a person, concept, place, or thing. Nouns can act as the subject of a sentence (i.e., the person or thing performing the action) or as the object of a verb (i.e., the person or thing affected by the action).
There are numerous types of nouns, including common nouns (used to refer to nonspecific people, concepts, places, or things), proper nouns (used to refer to specific people, concepts, places, or things), and collective nouns (used to refer to a group of people or things).
Ella lives in France .
Other types of nouns include countable and uncountable nouns , concrete nouns , abstract nouns , and gerunds .
Check for common mistakes
Use the best grammar checker available to check for common mistakes in your text.
Fix mistakes for free
A pronoun is a word used in place of a noun. Pronouns typically refer back to an antecedent (a previously mentioned noun) and must demonstrate correct pronoun-antecedent agreement . Like nouns, pronouns can refer to people, places, concepts, and things.
There are numerous types of pronouns, including personal pronouns (used in place of the proper name of a person), demonstrative pronouns (used to refer to specific things and indicate their relative position), and interrogative pronouns (used to introduce questions about things, people, and ownership).
That is a horrible painting!
A verb is a word that describes an action (e.g., “jump”), occurrence (e.g., “become”), or state of being (e.g., “exist”). Verbs indicate what the subject of a sentence is doing. Every complete sentence must contain at least one verb.
Verbs can change form depending on subject (e.g., first person singular), tense (e.g., simple past), mood (e.g., interrogative), and voice (e.g., passive voice ).
Regular verbs are verbs whose simple past and past participle are formed by adding“-ed” to the end of the word (or “-d” if the word already ends in “e”). Irregular verbs are verbs whose simple past and past participles are formed in some other way.
“I’ve already checked twice.”
“I heard that you used to sing .”
Other types of verbs include auxiliary verbs , linking verbs , modal verbs , and phrasal verbs .
An adjective is a word that describes a noun or pronoun. Adjectives can be attributive , appearing before a noun (e.g., “a red hat”), or predicative , appearing after a noun with the use of a linking verb like “to be” (e.g., “the hat is red ”).
Adjectives can also have a comparative function. Comparative adjectives compare two or more things. Superlative adjectives describe something as having the most or least of a specific characteristic.
Other types of adjectives include coordinate adjectives , participial adjectives , and denominal adjectives .
An adverb is a word that can modify a verb, adjective, adverb, or sentence. Adverbs are often formed by adding “-ly” to the end of an adjective (e.g., “slow” becomes “slowly”), although not all adverbs have this ending, and not all words with this ending are adverbs.
There are numerous types of adverbs, including adverbs of manner (used to describe how something occurs), adverbs of degree (used to indicate extent or degree), and adverbs of place (used to describe the location of an action or event).
Talia writes quite quickly.
Other types of adverbs include adverbs of frequency , adverbs of purpose , focusing adverbs , and adverbial phrases .
A preposition is a word (e.g., “at”) or phrase (e.g., “on top of”) used to show the relationship between the different parts of a sentence. Prepositions can be used to indicate aspects such as time , place , and direction .
I left the cup on the kitchen counter.
A conjunction is a word used to connect different parts of a sentence (e.g., words, phrases, or clauses).
The main types of conjunctions are coordinating conjunctions (used to connect items that are grammatically equal), subordinating conjunctions (used to introduce a dependent clause), and correlative conjunctions (used in pairs to join grammatically equal parts of a sentence).
You can choose what movie we watch because I chose the last time.
An interjection is a word or phrase used to express a feeling, give a command, or greet someone. Interjections are a grammatically independent part of speech, so they can often be excluded from a sentence without affecting the meaning.
Types of interjections include volitive interjections (used to make a demand or request), emotive interjections (used to express a feeling or reaction), cognitive interjections (used to indicate thoughts), and greetings and parting words (used at the beginning and end of a conversation).
Ouch ! I hurt my arm.
I’m, um , not sure.
The traditional classification of English words into eight parts of speech is by no means the only one or the objective truth. Grammarians have often divided them into more or fewer classes. Other commonly mentioned parts of speech include determiners and articles.
- Determiners
A determiner is a word that describes a noun by indicating quantity, possession, or relative position.
Common types of determiners include demonstrative determiners (used to indicate the relative position of a noun), possessive determiners (used to describe ownership), and quantifiers (used to indicate the quantity of a noun).
My brother is selling his old car.
Other types of determiners include distributive determiners , determiners of difference , and numbers .
An article is a word that modifies a noun by indicating whether it is specific or general.
- The definite article the is used to refer to a specific version of a noun. The can be used with all countable and uncountable nouns (e.g., “the door,” “the energy,” “the mountains”).
- The indefinite articles a and an refer to general or unspecific nouns. The indefinite articles can only be used with singular countable nouns (e.g., “a poster,” “an engine”).
There’s a concert this weekend.
If you want to know more about nouns , pronouns , verbs , and other parts of speech, make sure to check out some of our language articles with explanations and examples.
Nouns & pronouns
- Common nouns
- Proper nouns
- Collective nouns
- Personal pronouns
- Uncountable and countable nouns
- Verb tenses
- Phrasal verbs
- Types of verbs
- Active vs passive voice
- Subject-verb agreement
A is an indefinite article (along with an ). While articles can be classed as their own part of speech, they’re also considered a type of determiner .
The indefinite articles are used to introduce nonspecific countable nouns (e.g., “a dog,” “an island”).
In is primarily classed as a preposition, but it can be classed as various other parts of speech, depending on how it is used:
- Preposition (e.g., “ in the field”)
- Noun (e.g., “I have an in with that company”)
- Adjective (e.g., “Tim is part of the in crowd”)
- Adverb (e.g., “Will you be in this evening?”)
As a part of speech, and is classed as a conjunction . Specifically, it’s a coordinating conjunction .
And can be used to connect grammatically equal parts of a sentence, such as two nouns (e.g., “a cup and plate”), or two adjectives (e.g., “strong and smart”). And can also be used to connect phrases and clauses.
Is this article helpful?
Other students also liked, what is a collective noun | examples & definition.
- What Is an Adjective? | Definition, Types & Examples
- Using Conjunctions | Definition, Rules & Examples
More interesting articles
- Definite and Indefinite Articles | When to Use "The", "A" or "An"
- Ending a Sentence with a Preposition | Examples & Tips
- What Are Prepositions? | List, Examples & How to Use
- What Is a Determiner? | Definition, Types & Examples
- What Is an Adverb? Definition, Types & Examples
- What Is an Interjection? | Examples, Definition & Types
"I thought AI Proofreading was useless but.."
I've been using Scribbr for years now and I know it's a service that won't disappoint. It does a good job spotting mistakes”

Parts of Speech: The Ultimate Guide for Students and Teachers

This article is part of the ultimate guide to language for teachers and students. Click the buttons below to view these.
What are Parts of Speech ?
Just as a skilled bricklayer must get to grips with the trowel, brick hammer, tape measure, and spirit level, the student-writer must develop a thorough understanding of the tools of their trade too.
In English, words can be categorized according to their common syntactic function in a sentence, i.e. the job they perform.
We call these different categories Parts of Speech . Understanding the various parts of speech and how they work has several compelling benefits for our students.
Without first acquiring a firm grasp of the various parts of speech, students will struggle to fully comprehend how language works. This is essential not only for the development of their reading comprehension but their writing skills too.

Parts of speech are the core building blocks of grammar . To understand how a language works at a sentence and a whole-text level, we must first master parts of speech.
In English, we can identify eight of these individual parts of speech, and these will provide the focus for our Complete Guide to Parts of Speech .
THE EIGHT PARTS OF SPEECH (Click to jump to each section)
A complete unit on teaching figurative language.
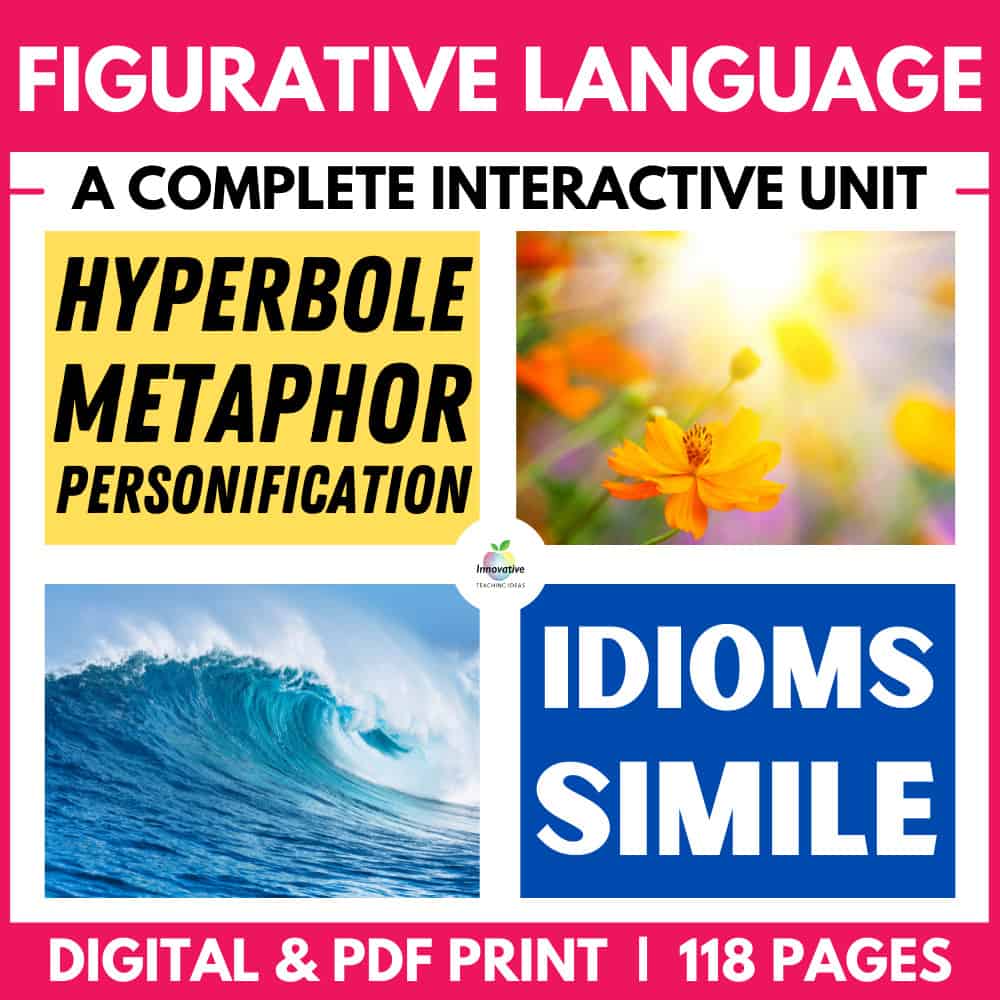
❤️The use of FIGURATIVE LANGUAGE is like “SPECIAL EFFECTS FOR AUTHORS.” It is a powerful tool to create VIVID IMAGERY through words. This HUGE 110 PAGE UNIT guides you through a complete understanding of FIGURATIVE LANGUAGE as both a READER and WRITER covering.

Often the first word a child speaks will be a noun, for example, Mum , Dad , cow , dog , etc.
Nouns are naming words, and, as most school kids can recite, they are the names of people, places, and things . But, what isn’t as widely understood by many of our students is that nouns can be further classified into more specific categories.
These categories are:
Common Nouns
Proper nouns, concrete nouns, abstract nouns, collective nouns, countable nouns, uncountable nouns.
All nouns can be classified as either common or proper .
Common nouns are the general names of people, places, and things. They are groups or classes on their own, rather than specific types of people, places, or things such as we find in proper nouns.
Common nouns can be further classified as abstract or concrete – more on this shortly!
Some examples of common nouns include:
People: teacher, author, engineer, artist, singer.
Places: country, city, town, house, garden.
Things: language, trophy, magazine, movie, book.
Proper nouns are the specific names for people, places, and things. Unlike common nouns, which are always lowercase, proper nouns are capitalized. This makes them easy to identify in a text.
Where possible, using proper nouns in place of common nouns helps bring precision to a student’s writing.
Some examples of proper nouns include:
People: Mrs Casey, J.K. Rowling, Nikola Tesla, Pablo Picasso, Billie Eilish.
Places: Australia, San Francisco, Llandovery, The White House, Gardens of Versailles.
Things: Bulgarian, The World Cup, Rolling Stone, The Lion King, The Hunger Games.
Nouns Teaching Activity: Common vs Proper Nouns
- Provide students with books suitable for their current reading level.
- Instruct students to go through a page or two and identify all the nouns.
- Ask students to sort these nouns into two lists according to whether they are common nouns or proper nouns.
As mentioned, all common and proper nouns can be further classified as either concrete or abstract .
A concrete noun is any noun that can be experienced through one of the five senses. In other words, if you can see, smell, hear, taste, or touch it, then it’s a concrete noun.
Some examples of concrete nouns include:
Abstract nouns refer to those things that can’t be experienced or identified through the five senses.
They are not physical things we can perceive but intangible concepts and ideas, qualities and states.
Some examples of abstract nouns include:
Nouns Teaching Activity: Concrete Vs. Abstract Nouns
- Provide students with a book suitable for their current reading level.
- Instruct students to go through a page or two and identify all the nouns (the lists from Practice Activity #1 may be suitable).
- This time, ask students to sort these nouns into two lists according to whether they are concrete or abstract nouns.
A collective noun is the name of a group of people or things. That is, a collective noun always refers to more than one of something.
Some examples of collective nouns include:
People: a board of directors, a team of football players, a cast of actors, a band of musicians, a class of students.
Places: a range of mountains, a suite of rooms, a union of states, a chain of islands.
Things: a bale of hay, a constellation of stars, a bag of sweets, a school of fish, a flock of seagulls.
Countable nouns are nouns that refer to things that can be counted. They come in two flavors: singular and plural .
In their singular form, countable nouns are often preceded by the article, e.g. a , an , or the .
In their plural form, countable nouns are often preceded by a number. They can also be used in conjunction with quantifiers such as a few and many .
Some examples of countable nouns include:
COUNTABLE NOUNS EXAMPLES
Also known as mass nouns, uncountable nouns are, as their name suggests, impossible to count. Abstract ideas such as bravery and compassion are uncountable, as are things like liquid and bread .
These types of nouns are always treated in the singular and usually do not have a plural form.
They can stand alone or be used in conjunction with words and phrases such as any , some , a little , a lot of , and much .
Some examples of uncountable nouns include:
UNCOUNTABLE NOUNS EXAMPLES
Nouns teaching activity: how many can you list .
- Organize students into small groups to work collaboratively.
- Challenge students to list as many countable and uncountable nouns as they can in ten minutes.
- To make things more challenging, stipulate that there must be an uncountable noun and a countable noun to gain a point.
- The winning group is the one that scores the most points.
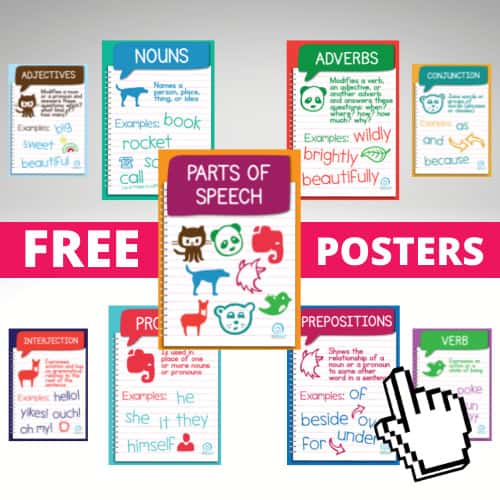
Without a verb, there is no sentence! Verbs are the words we use to represent both internal and external actions or states of being. Without a verb, nothing happens.
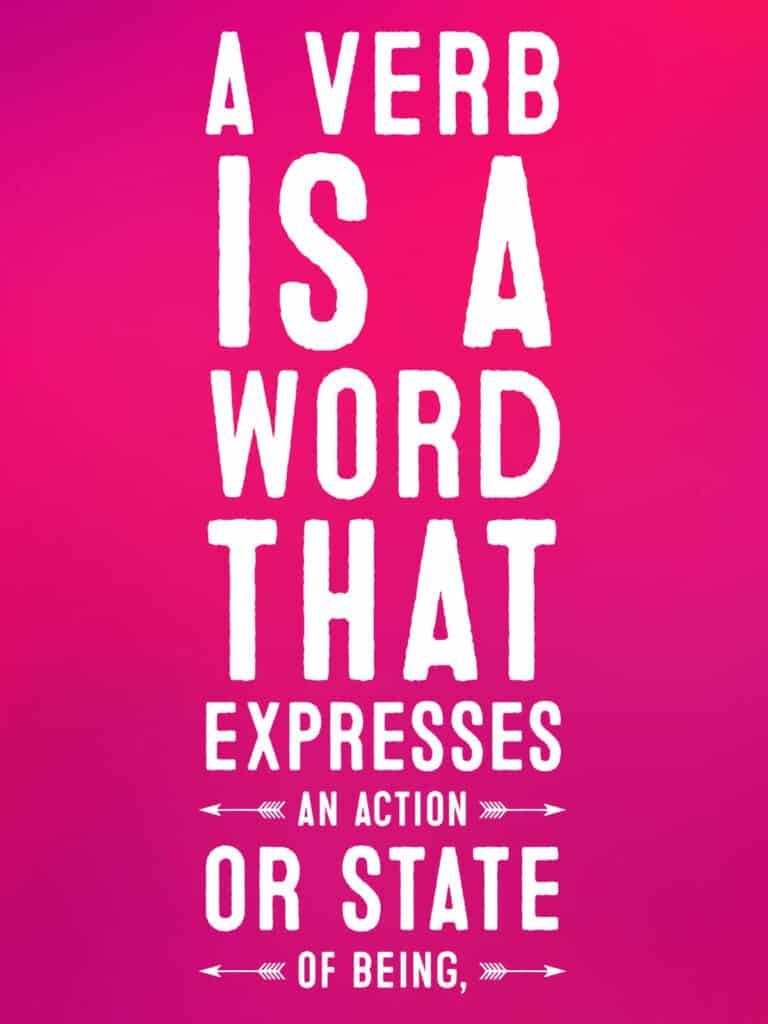
There are many different types of verbs. Here, we will look at five important verb forms organised according to the jobs they perform:
Dynamic Verbs
Stative verbs, transitive verbs, intransitive verbs, auxiliary verbs.
Each verb can be classified as being either an action or a stative verb.
Dynamic or action verbs describe the physical activity performed by the subject of a sentence. This type of verb is usually the first we learn as children.
For example, run , hit , throw , hide , eat , sleep , watch , write , etc. are all dynamic verbs, as is any action performed by the body.
Let’s see a few examples in sentences:
- I jogged around the track three times.
- She will dance as if her life depends on it.
- She took a candy from the bag, unwrapped it, and popped it into her mouth.
If a verb doesn’t describe a physical activity, then it is a stative verb.
Stative verbs refer to states of being, conditions, or mental processes. Generally, we can classify stative verbs into four types:
- Emotions/Thoughts
Some examples of stative verbs include:
Senses: hurt, see, smell, taste, hear, etc.
Emotions: love, doubt, desire, remember, believe, etc.
Being: be, have, require, involve, contain, etc.
Possession: want, include, own, have, belong, etc.
Here are some stative verbs at work in sentences:
- That is one thing we can agree on.
- I remember my first day at school like it was yesterday.
- The university requires students to score at least 80%.
- She has only three remaining.
Sometimes verbs can fit into more than one category, e.g., be , have , look , see , e.g.,
- She looks beautiful. (Stative)
- I look through the telescope. (Dynamic)
Each action or stative verb can also be further classified as transitive or intransitive .
A transitive verb takes a direct object after it. The object is the noun, noun phrase, or pronoun that has something done to it by the subject of the sentence.
We see this in the most straightforward English sentences, i.e., the Subject-Verb-Object or SVO sentence.
Here are two examples to illustrate. Note: the subject of each sentence is underlined, and the transitive verbs are in bold.
- The teacher answered the student’s questions.
- She studies languages at university.
- My friend loves cabbage.
Most sentences in English employ transitive verbs.
An intransitive verb does not take a direct object after it. It is important to note that only nouns, noun phrases, and pronouns can be classed as direct objects.
Here are some examples of intransitive verbs – notice how none of these sentences has direct objects after their verbs.
- Jane’s health improved .
- The car ran smoothly.
- The school opens at 9 o’clock.
Auxiliary verbs, also known as ‘helping’ verbs, work with other verbs to affect the meaning of a sentence. They do this by combining with a main verb to alter the sentence’s tense, mood, or voice.
Auxiliary verbs will frequently use not in the negative.
There are relatively few auxiliary verbs in English. Here is a list of the main ones:
- be (am, are, is, was, were, being)
- do (did, does, doing)
- have (had, has, having)
Here are some examples of auxiliary verbs (in bold) in action alongside a main verb (underlined).
She is working as hard as she can.
- You must not eat dinner until after five o’clock.
- The parents may come to the graduation ceremony.
The Subject-Auxiliary Inversion Test
To test whether or not a verb is an auxiliary verb, you can use the Subject-Auxiliary Inversion Test .
- Take the sentence, e.g:
- Now, invert the subject and the suspected auxiliary verb to see if it creates a question.
Is she working as hard as she can?
- Can it take ‘not’ in the negative form?
She is not working as hard as she can.
- If the answer to both of these questions is yes, you have an auxiliary verb. If not, you have a full verb.
Verbs Teaching Activity: Identify the Verbs
- Instruct students to go through an appropriate text length (e.g., paragraph, page, etc.) and compile a list of verbs.
- In groups, students should then discuss and categorize each verb according to whether they think they are dynamic or stative, transitive or intransitive, and/or auxiliary verbs.
The job of an adjective is to modify a noun or a pronoun. It does this by describing, quantifying, or identifying the noun or pronoun. Adjectives help to make writing more interesting and specific. Usually, the adjective is placed before the word it modifies.
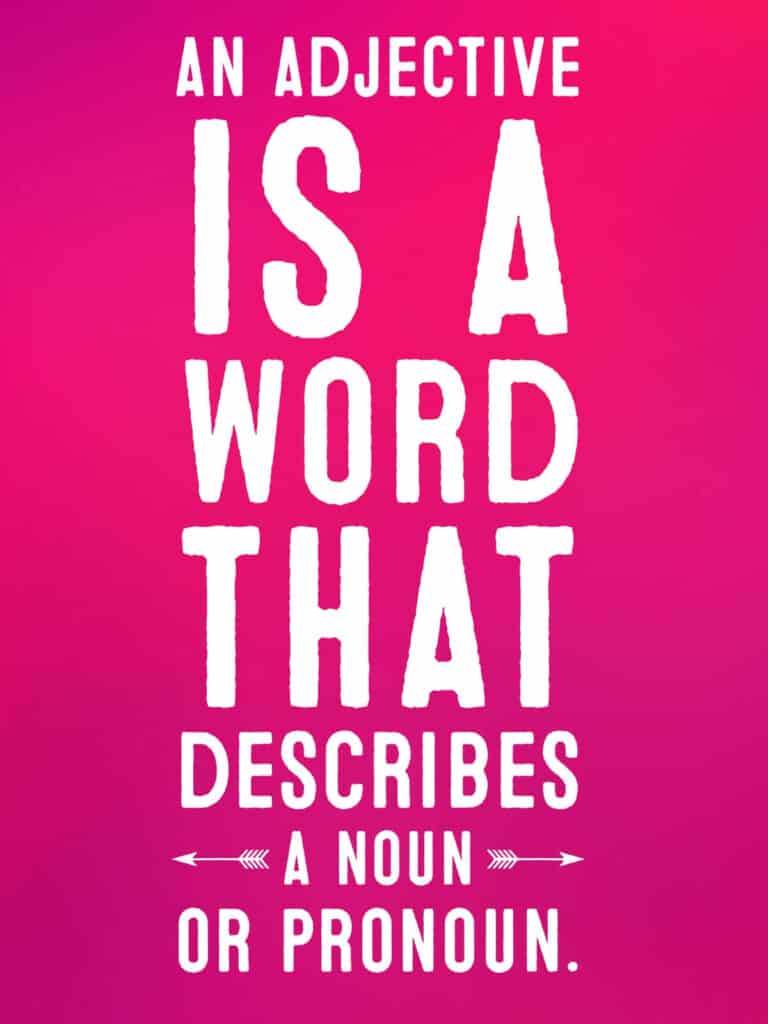
As with other parts of speech, not all adjectives are the same. There are many different types of adjectives and, in this article, we will look at:
Descriptive Adjectives
- Degrees of Adjectives
Quantitative Adjectives
Demonstrative adjectives, possessive adjectives, interrogative adjectives, proper adjectives.
Descriptive adjectives are what most students think of first when asked what an adjective is. Descriptive adjectives tell us something about the quality of the noun or pronoun in question. For this reason, they are sometimes referred to as qualitative adjectives .
Some examples of this type of adjective include:
- hard-working
In sentences, they look like this:
- The pumpkin was enormous .
- It was an impressive feat of athleticism I ever saw.
- Undoubtedly, this was an exquisite vase.
- She faced some tough competition.
Degrees of Adjectives
Descriptive adjectives have three degrees to express varying degrees of intensity and to compare one thing to another. These degrees are referred to as positive , comparative , and superlative .
The positive degree is the regular form of the descriptive adjective when no comparison is being made, e.g., strong .
The comparative degree is used to compare two people, places, or things, e.g., stronger .
There are several ways to form the comparative, methods include:
- Adding more or less before the adjective
- Adding -er to the end of one syllable adjectives
- For two-syllable adjectives ending in y , change the y to an i and add -er to the end.
The superlative degree is typically used when comparing three or more things to denote the upper or lowermost limit of a quality, e.g., strongest .
There are several ways to form the superlative, including:
- Adding most or least before the adjective
- Adding -est to the end of one syllable adjectives
- For two-syllable adjectives ending in y , change the y to an i and add -est to the end.
There are also some irregular adjectives of degree that follow no discernible pattern that must be learned off by students, e.g., good – better – best .
Let’s take a look at these degrees of adjectives in their different forms.
Let’s take a quick look at some sample sentences:
- It was a beautiful example of kindness.
Comparative
- The red is nice, but the green is prettier .
Superlative
- This mango is the most delicious fruit I have ever tastiest.
Quantitive adjectives provide information about how many or how much of the noun or pronoun.
Some quantitive adjectives include:
- She only ate half of her sandwich.
- This is my first time here.
- I would like three slices, please.
- There isn’t a single good reason to go.
- There aren’t many places like it.
- It’s too much of a good thing.
- I gave her a whole box of them.
A demonstrative adjective identifies or emphasizes a noun’s place in time or space. The most common demonstrative adjectives are this , that , these , and those .
Here are some examples of demonstrative adjectives in use:
- This boat is mine.
- That car belongs to her.
- These shoes clash with my dress.
- Those people are from Canada.
Possessive adjectives show ownership, and they are sometimes confused with possessive pronouns.
The most common possessive adjectives are my , your , his , her , our , and their .
Students need to be careful not to confuse these with possessive pronouns such as mine , yours , his (same in both contexts), hers , ours , and theirs .
Here are some examples of possessive adjectives in sentences:
- My favorite food is sushi.
- I would like to read your book when you have finished it.
- I believe her car is the red one.
- This is their way of doing things.
- Our work here is done.
Interrogative adjectives ask questions, and, in common with many types of adjectives, they are always followed by a noun. Basically, these are the question words we use to start questions. Be careful however, interrogative adjectives modify nouns. If the word after the question word is a verb, then you have an interrogative adverb on hand.
Some examples of interrogative adjectives include what , which , and whose .
Let’s take a look at these in action:
- What drink would you like?
- Which car should we take?
- Whose shoes are these?
Please note: Whose can also fit into the possessive adjective category too.
We can think of proper adjectives as the adjective form of proper nouns – remember those? They were the specific names of people, places, and things and need to be capitalized.
Let’s take the proper noun for the place America . If we wanted to make an adjective out of this proper noun to describe something, say, a car we would get ‘ American car’.
Let’s take a look at another few examples:
- Joe enjoyed his cup of Ethiopian coffee.
- My favorite plays are Shakespearean tragedies.
- No doubt about it, Fender guitars are some of the best in the world.
- The Mona Lisa is a fine example of Renaissance art.
Though it may come as a surprise to some, articles are also adjectives as, like all adjectives, they modify nouns. Articles help us determine a noun’s specification.
For example, ‘a’ and ‘an’ are used in front of an unspecific noun, while ‘the’ is used when referring to a specific noun.
Let’s see some articles as adjectives in action!
- You will find an apple inside the cupboard.
- This is a car.
- The recipe is a family secret.
Adjectives Teaching Activity: Types of Adjective Tally
- Choose a suitable book and assign an appropriate number of pages or length of a chapter for students to work with.
- Students work their way through each page, tallying up the number of each type of adjective they can identify using a table like the one below:
- Note how degrees of adjective has been split into comparative and superlative. The positive forms will take care of in the descriptive category.
- You may wish to adapt this table to exclude the easier categories to identify, such as articles and demonstrative, for example.

Traditionally, adverbs are defined as those words that modify verbs, but they do so much more than that. They can be used not only to describe how verbs are performed but also to modify adjectives, other adverbs, clauses, prepositions, or entire sentences.
With such a broad range of tasks at the feet of the humble adverb, it would be impossible to cover every possibility in this article alone. However, there are five main types of adverbs our students should familiarize themselves with. These are:
Adverbs of Manner
Adverbs of time, adverbs of frequency, adverbs of place, adverbs of degree.
Adverbs of manner describe how or the way in which something happens or is done. This type of adverb is often the first type taught to students. Many of these end with -ly . Some common examples include happily , quickly , sadly , slowly , and fast .
Here are a few taster sentences employing adverbs of manner:
- She cooks Chinese food well .
- The children played happily together.
- The students worked diligently on their projects.
- Her mother taught her to cross the road carefully .
- The date went badly .
Adverbs of time indicate when something happens. Common adverbs of time include before , now , then , after , already , immediately , and soon .
Here are some sentences employing adverbs of time:
- I go to school early on Wednesdays.
- She would like to finish her studies eventually .
- Recently , Sarah moved to Bulgaria.
- I have already finished my homework.
- They have been missing training lately .
While adverbs of time deal with when something happens, adverbs of frequency are concerned with how often something happens. Common adverbs of frequency include always , frequently , sometimes , seldom , and never .
Here’s what they look like in sentences:
- Harry usually goes to bed around ten.
- Rachel rarely eats breakfast in the morning.
- Often , I’ll go home straight after school.
- I occasionally have ketchup on my pizza.
- She seldom goes out with her friends.
Adverbs of place, as the name suggests, describe where something happens or where it is. They can refer to position, distance, or direction. Some common adverbs of place include above , below , beside , inside , and anywhere .
Check out some examples in the sentences below:
- Underneath the bridge, there lived a troll.
- There were pizzerias everywhere in the city.
- We walked around the park in the pouring rain.
- If the door is open, then go inside .
- When I am older, I would like to live nearby .
Adverbs of degree express the degree to which or how much of something is done. They can also be used to describe levels of intensity. Some common adverbs of degree include barely , little , lots , completely , and entirely .
Here are some adverbs of degree at work in sentences:
- I hardly noticed her when she walked into the room.
- The little girl had almost finished her homework.
- The job was completely finished.
- I was so delighted to hear the good news.
- Jack was totally delighted to see Diane after all these years.
Adverb Teaching Activity: The Adverb Generator
- Give students a worksheet containing a table divided into five columns. Each column bears a heading of one of the different types of adverbs ( manner , time , frequency , place , degree ).
- Challenge each group to generate as many different examples of each adverb type and record these in the table.
- The winning group is the one with the most adverbs. As a bonus, or tiebreaker, task the students to make sentences with some of the adverbs.

Pronouns are used in place of a specific noun used earlier in a sentence. They are helpful when the writer wants to avoid repetitive use of a particular noun such as a name. For example, in the following sentences, the pronoun she is used to stand for the girl’s name Mary after it is used in the first sentence.
Mary loved traveling. She had been to France, Thailand, and Taiwan already, but her favorite place in the world was Australia. She had never seen an animal quite as curious-looking as the duck-billed platypus.
We also see her used in place of Mary’s in the above passage. There are many different pronouns and, in this article, we’ll take a look at:
Subject Pronouns
Object pronouns, possessive pronouns, reflexive pronouns, intensive pronouns, demonstrative pronouns, interrogative pronouns.
Subject pronouns are the type of pronoun most of us think of when we hear the term pronoun . They operate as the subject of a verb in a sentence. They are also known as personal pronouns.
The subject pronouns are:
Here are a few examples of subject pronouns doing what they do best:
- Sarah and I went to the movies last Thursday night.
- That is my pet dog. It is an Irish Wolfhound.
- My friends are coming over tonight, they will be here at seven.
- We won’t all fit into the same car.
- You have done a fantastic job with your grammar homework!
Object pronouns operate as the object of a verb, or a preposition, in a sentence. They act in the same way as object nouns but are used when it is clear what the object is.
The object pronouns are:
Here are a few examples of object pronouns in sentences:
- I told you , this is a great opportunity for you .
- Give her some more time, please.
- I told her I did not want to do it .
- That is for us .
- Catherine is the girl whom I mentioned in my letter.
Possessive pronouns indicate ownership of a noun. For example, in the sentence:
These books are mine .
The word mine stands for my books . It’s important to note that while possessive pronouns look similar to possessive adjectives, their function in a sentence is different.
The possessive pronouns are:
Let’s take a look at how these are used in sentences:
- Yours is the yellow jacket.
- I hope this ticket is mine .
- The train that leaves at midnight is theirs .
- Ours is the first house on the right.
- She is the person whose opinion I value most.
- I believe that is his .
Reflexive pronouns are used in instances where the object and the subject are the same. For example, in the sentence, she did it herself , the words she and herself refer to the same person.
The reflexive pronoun forms are:
Here are a few more examples of reflexive pronouns at work:
- I told myself that numerous times.
- He got himself a new computer with his wages.
- We will go there ourselves .
- You must do it yourself .
- The only thing to fear is fear itself .
This type of pronoun can be used to indicate emphasis. For example, when we write, I spoke to the manager herself , the point is made that we talked to the person in charge and not someone lower down the hierarchy.
Similar to the reflexive pronouns above, we can easily differentiate between reflexive and intensive pronouns by asking if the pronoun is essential to the sentence’s meaning. If it isn’t, then it is used solely for emphasis, and therefore, it’s an intensive rather than a reflexive pronoun.
Often confused with demonstrative adjectives, demonstrative pronouns can stand alone in a sentence.
When this , that , these , and those are used as demonstrative adjectives they come before the noun they modify. When these same words are used as demonstrative pronouns, they replace a noun rather than modify it.
Here are some examples of demonstrative pronouns in sentences:
- This is delicious.
- That is the most beautiful thing I have ever seen.
- These are not mine.
- Those belong to the driver.
Interrogative pronouns are used to form questions. They are the typical question words that come at the start of questions, with a question mark coming at the end. The interrogative pronouns are:
Putting them into sentences looks like this:
- What is the name of your best friend?
- Which of these is your favourite?
- Who goes to the market with you?
- Whom do you think will win?
- Whose is that?
Pronoun Teaching Activity: Pronoun Review Table
- Provide students with a review table like the one below to revise the various pronoun forms.
- They can use this table to help them produce independent sentences.
- Once students have had a chance to familiarize themselves thoroughly with each of the different types of pronouns, provide the students with the headings and ask them to complete a table from memory.
Prepositions

Prepositions provide extra information showing the relationship between a noun or pronoun and another part of a sentence. These are usually short words that come directly before nouns or pronouns, e.g., in , at , on , etc.
There are, of course, many different types of prepositions, each relating to particular types of information. In this article, we will look at:
Prepositions of Time
Prepositions of place, prepositions of movement, prepositions of manner, prepositions of measure.
- Preposition of Agency
- Preposition of Possession
- Preposition of Source
Phrasal Prepositions
It’s worth noting that several prepositional words make an appearance in several different categories of prepositions.
Prepositions of time indicate when something happens. Common prepositions of time include after , at , before , during , in , on .
Let’s see some of these at work:
- I have been here since Thursday.
- My daughter was born on the first of September.
- He went overseas during the war.
- Before you go, can you pay the bill, please?
- We will go out after work.
Sometimes students have difficulty knowing when to use in , on , or at . These little words are often confused. The table below provides helpful guidance to help students use the right preposition in the right context.
The prepositions of place, in , at , on , will be instantly recognisable as they also double as prepositions of time. Again, students can sometimes struggle a little to select the correct one for the situation they are describing. Some guidelines can be helpful.
- If something is contained or confined inside, we use in .
- If something is placed upon a surface, we use on .
- If something is located at a specific point, we use at .
A few example sentences will assist in illustrating these:
- He is in the house.
- I saw it in a magazine.
- In France, we saw many great works of art.
- Put it on the table.
- We sailed on the river.
- Hang that picture on the wall, please.
- We arrived at the airport just after 1 pm.
- I saw her at university.
- The boy stood at the window.
Usually used with verbs of motion, prepositions of movement indicate movement from one place to another. The most commonly used preposition of movement is to .
Some other prepositions of movement include:
Here’s how they look in some sample sentences:
- The ball rolled across the table towards me.
- We looked up into the sky.
- The children ran past the shop on their way home.
- Jackie ran down the road to greet her friend.
- She walked confidently through the curtains and out onto the stage.
Preposition of manner shows us how something is done or how it happens. The most common of these are by , in , like , on , with .
Let’s take a look at how they work in sentences:
- We went to school by bus.
- During the holidays, they traveled across the Rockies on foot.
- Janet went to the airport in a taxi.
- She played soccer like a professional.
- I greeted her with a smile.
Prepositions of measure are used to indicate quantities and specific units of measurement. The two most common of these are by and of .
Check out these sample sentences:
- I’m afraid we only sell that fabric by the meter.
- I will pay you by the hour.
- She only ate half of the ice cream. I ate the other half.
- A kilogram of apples is the same weight as a kilogram of feathers.
Prepositions of Agency
These prepositions indicate the causal relationship between a noun or pronoun and an action. They show the cause of something happening. The most commonly used prepositions of agency are by and with .
Here are some examples of their use in sentences:
- The Harry Potter series was written by J.K. Rowling.
- This bowl was made by a skilled craftsman.
- His heart was filled with love.
- The glass was filled with water.
Prepositions of Possession
Prepositions of possessions indicate who or what something belongs to. The most common of these are of , to , and with .
Let’s take a look:
- He is the husband of my cousin.
- He is a friend of the mayor.
- This once belonged to my grandmother.
- All these lands belong to the Ministry.
- The man with the hat is waiting outside.
- The boy with the big feet tripped and fell.
Prepositions of Source
Prepositions of source indicate where something comes from or its origins. The two most common prepositions of source are from and by . There is some crossover here with prepositions of agency.
Here are some examples:
- He comes from New Zealand.
- These oranges are from our own orchard.
- I was warmed by the heat of the fire.
- She was hugged by her husband.
- The yoghurt is of Bulgarian origin.
Phrasal prepositions are also known as compound prepositions. These are phrases of two or more words that function in the same way as prepositions. That is, they join nouns or pronouns to the rest of the sentence.
Some common phrasal prepositions are:
- According to
- For a change
- In addition to
- In spite of
- Rather than
- With the exception of
Students should be careful of overusing phrasal prepositions as some of them can seem clichéd. Frequently, it’s best to say things in as few words as is necessary.
Preposition Teaching Activity: Pr eposition Sort
- Print out a selection of the different types of prepositions on pieces of paper.
- Organize students into smaller working groups and provide each group with a set of prepositions.
- Using the headings above as categories, challenge students to sort the prepositions into the correct groups. Note that some prepositions will comfortably fit into more than one group.
- The winning group is the one to sort all prepositions correctly first.
- As an extension exercise, students can select a preposition from each category and write a sample sentence for it.
ConjunctionS

Conjunctions are used to connect words, phrases, and clauses. There are three main types of conjunction that are used to join different parts of sentences. These are:
- Coordinating
- Subordinating
- Correlative
Coordinating Conjunctions
These conjunctions are used to join sentence components that are equal such as two words, two phrases, or two clauses. In English, there are seven of these that can be memorized using the mnemonic FANBOYS:
Here are a few example sentences employing coordinating conjunctions:
- As a writer, he needed only a pen and paper.
- I would describe him as strong but lazy.
- Either we go now or not at all.
Subordinating Conjunctions
Subordinating conjunctions are used to introduce dependent clauses in sentences. Basically, dependent clauses are parts of sentences that cannot stand as complete sentences on their own.
Some of the most common subordinate conjunctions are:
Let’s take a look at some example sentences:
- I will complete it by Tuesday if I have time.
- Although she likes it, she won’t buy it.
- Jack will give it to you after he finds it.
Correlative Conjunctions
Correlative conjunctions are like shoes; they come in pairs. They work together to make sentences work. Some come correlative conjunctions are:
- either / or
- neither / nor
- Not only / but also
Let’s see how some of these work together:
- If I were you, I would get either the green one or the yellow one.
- John wants neither pity nor help.
- I don’t know whether you prefer horror or romantic movies.
Conjunction Teaching Activity: Conjunction Challenge
- Organize students into Talking Pairs .
- Partner A gives Partner B an example of a conjunction.
- Partner B must state which type of conjunction it is, e.g. coordinating, subordinating, or correlative.
- Partner B must then compose a sentence that uses the conjunction correctly and tell it to Partner A.
- Partners then swap roles.
InterjectionS

Interjections focus on feelings and are generally grammatically unrelated to the rest of the sentence or sentences around them. They convey thoughts and feelings and are common in our speech. They are often followed by exclamation marks in writing. Interjections include expressions such as:
- Eww! That is so gross!
- Oh , I don’t know. I’ve never used one before.
- That’s very… err …generous of you, I suppose.
- Wow! That is fantastic news!
- Uh-Oh! I don’t have any more left.
Interjection Teaching Activity: Create a scenario
- Once students clearly understand what interjections are, brainstorm as a class as many as possible.
- Write a master list of interjections on the whiteboard.
- Partner A suggests an interjection word or phrase to Partner B.
- Partner B must create a fictional scenario where this interjection would be used appropriately.
With a good grasp of the fundamentals of parts of speech, your students will now be equipped to do a deeper dive into the wild waters of English grammar.
To learn more about the twists and turns of English grammar, check out our comprehensive article on English grammar here.
DOWNLOAD THESE 9 FREE CLASSROOM PARTS OF SPEECH POSTERS

PARTS OF SPEECH TUTORIAL VIDEOS

MORE ARTICLES RELATED TO PARTS OF SPEECH
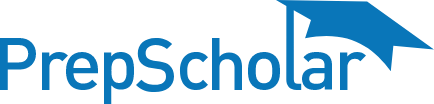
Choose Your Test
Sat / act prep online guides and tips, understanding the 8 parts of speech: definitions and examples.
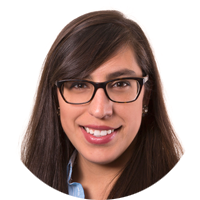
General Education
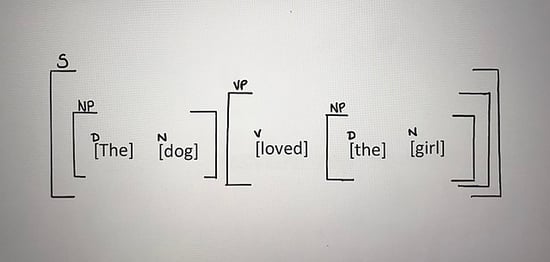
If you’re trying to learn the grammatical rules of English, you’ve probably been asked to learn the parts of speech. But what are parts of speech and how many are there? How do you know which words are classified in each part of speech?
The answers to these questions can be a bit complicated—English is a difficult language to learn and understand. Don’t fret, though! We’re going to answer each of these questions for you with a full guide to the parts of speech that explains the following:
- What the parts of speech are, including a comprehensive parts of speech list
- Parts of speech definitions for the individual parts of speech. (If you’re looking for information on a specific part of speech, you can search for it by pressing Command + F, then typing in the part of speech you’re interested in.)
- Parts of speech examples
- A ten question quiz covering parts of speech definitions and parts of speech examples
We’ve got a lot to cover, so let’s begin!
Feature Image: (Gavina S / Wikimedia Commons)
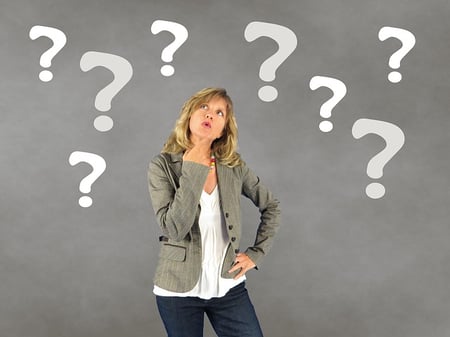
What Are Parts of Speech?
The parts of speech definitions in English can vary, but here’s a widely accepted one: a part of speech is a category of words that serve a similar grammatical purpose in sentences.
To make that definition even simpler, a part of speech is just a category for similar types of words . All of the types of words included under a single part of speech function in similar ways when they’re used properly in sentences.
In the English language, it’s commonly accepted that there are 8 parts of speech: nouns, verbs, adjectives, adverbs, pronouns, conjunctions, interjections, and prepositions. Each of these categories plays a different role in communicating meaning in the English language. Each of the eight parts of speech—which we might also call the “main classes” of speech—also have subclasses. In other words, we can think of each of the eight parts of speech as being general categories for different types within their part of speech . There are different types of nouns, different types of verbs, different types of adjectives, adverbs, pronouns...you get the idea.
And that’s an overview of what a part of speech is! Next, we’ll explain each of the 8 parts of speech—definitions and examples included for each category.
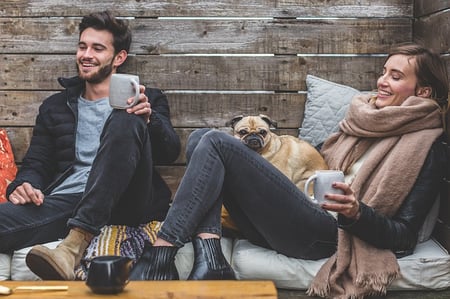
There are tons of nouns in this picture. Can you find them all?
Nouns are a class of words that refer, generally, to people and living creatures, objects, events, ideas, states of being, places, and actions. You’ve probably heard English nouns referred to as “persons, places, or things.” That definition is a little simplistic, though—while nouns do include people, places, and things, “things” is kind of a vague term. I t’s important to recognize that “things” can include physical things—like objects or belongings—and nonphysical, abstract things—like ideas, states of existence, and actions.
Since there are many different types of nouns, we’ll include several examples of nouns used in a sentence while we break down the subclasses of nouns next!
Subclasses of Nouns, Including Examples
As an open class of words, the category of “nouns” has a lot of subclasses. The most common and important subclasses of nouns are common nouns, proper nouns, concrete nouns, abstract nouns, collective nouns, and count and mass nouns. Let’s break down each of these subclasses!
Common Nouns and Proper Nouns
Common nouns are generic nouns—they don’t name specific items. They refer to people (the man, the woman), living creatures (cat, bird), objects (pen, computer, car), events (party, work), ideas (culture, freedom), states of being (beauty, integrity), and places (home, neighborhood, country) in a general way.
Proper nouns are sort of the counterpart to common nouns. Proper nouns refer to specific people, places, events, or ideas. Names are the most obvious example of proper nouns, like in these two examples:
Common noun: What state are you from?
Proper noun: I’m from Arizona .
Whereas “state” is a common noun, Arizona is a proper noun since it refers to a specific state. Whereas “the election” is a common noun, “Election Day” is a proper noun. Another way to pick out proper nouns: the first letter is often capitalized. If you’d capitalize the word in a sentence, it’s almost always a proper noun.
Concrete Nouns and Abstract Nouns
Concrete nouns are nouns that can be identified through the five senses. Concrete nouns include people, living creatures, objects, and places, since these things can be sensed in the physical world. In contrast to concrete nouns, abstract nouns are nouns that identify ideas, qualities, concepts, experiences, or states of being. Abstract nouns cannot be detected by the five senses. Here’s an example of concrete and abstract nouns used in a sentence:
Concrete noun: Could you please fix the weedeater and mow the lawn ?
Abstract noun: Aliyah was delighted to have the freedom to enjoy the art show in peace .
See the difference? A weedeater and the lawn are physical objects or things, and freedom and peace are not physical objects, though they’re “things” people experience! Despite those differences, they all count as nouns.
Collective Nouns, Count Nouns, and Mass Nouns
Nouns are often categorized based on number and amount. Collective nouns are nouns that refer to a group of something—often groups of people or a type of animal. Team , crowd , and herd are all examples of collective nouns.
Count nouns are nouns that can appear in the singular or plural form, can be modified by numbers, and can be described by quantifying determiners (e.g. many, most, more, several). For example, “bug” is a count noun. It can occur in singular form if you say, “There is a bug in the kitchen,” but it can also occur in the plural form if you say, “There are many bugs in the kitchen.” (In the case of the latter, you’d call an exterminator...which is an example of a common noun!) Any noun that can accurately occur in one of these singular or plural forms is a count noun.
Mass nouns are another type of noun that involve numbers and amount. Mass nouns are nouns that usually can’t be pluralized, counted, or quantified and still make sense grammatically. “Charisma” is an example of a mass noun (and an abstract noun!). For example, you could say, “They’ve got charisma, ” which doesn’t imply a specific amount. You couldn’t say, “They’ve got six charismas, ” or, “They’ve got several charismas .” It just doesn’t make sense!
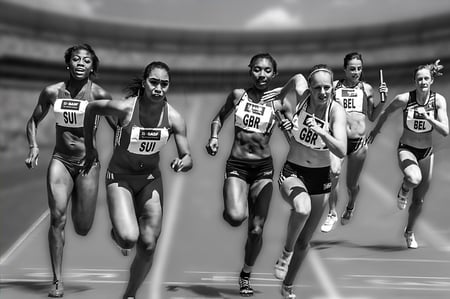
Verbs are all about action...just like these runners.
A verb is a part of speech that, when used in a sentence, communicates an action, an occurrence, or a state of being . In sentences, verbs are the most important part of the predicate, which explains or describes what the subject of the sentence is doing or how they are being. And, guess what? All sentences contain verbs!
There are many words in the English language that are classified as verbs. A few common verbs include the words run, sing, cook, talk, and clean. These words are all verbs because they communicate an action performed by a living being. We’ll look at more specific examples of verbs as we discuss the subclasses of verbs next!
Subclasses of Verbs, Including Examples
Like nouns, verbs have several subclasses. The subclasses of verbs include copular or linking verbs, intransitive verbs, transitive verbs, and ditransitive or double transitive verbs. Let’s dive into these subclasses of verbs!
Copular or Linking Verbs
Copular verbs, or linking verbs, are verbs that link a subject with its complement in a sentence. The most familiar linking verb is probably be. Here’s a list of other common copular verbs in English: act, be, become, feel, grow, seem, smell, and taste.
So how do copular verbs work? Well, in a sentence, if we said, “Michi is ,” and left it at that, it wouldn’t make any sense. “Michi,” the subject, needs to be connected to a complement by the copular verb “is.” Instead, we could say, “Michi is leaving.” In that instance, is links the subject of the sentence to its complement.
Transitive Verbs, Intransitive Verbs, and Ditransitive Verbs
Transitive verbs are verbs that affect or act upon an object. When unattached to an object in a sentence, a transitive verb does not make sense. Here’s an example of a transitive verb attached to (and appearing before) an object in a sentence:
Please take the clothes to the dry cleaners.
In this example, “take” is a transitive verb because it requires an object—”the clothes”—to make sense. “The clothes” are the objects being taken. “Please take” wouldn’t make sense by itself, would it? That’s because the transitive verb “take,” like all transitive verbs, transfers its action onto another being or object.
Conversely, intransitive verbs don’t require an object to act upon in order to make sense in a sentence. These verbs make sense all on their own! For instance, “They ran ,” “We arrived ,” and, “The car stopped ” are all examples of sentences that contain intransitive verbs.
Finally, ditransitive verbs, or double transitive verbs, are a bit more complicated. Ditransitive verbs are verbs that are followed by two objects in a sentence . One of the objects has the action of the ditransitive verb done to it, and the other object has the action of the ditransitive verb directed towards it. Here’s an example of what that means in a sentence:
I cooked Nathan a meal.
In this example, “cooked” is a ditransitive verb because it modifies two objects: Nathan and meal . The meal has the action of “cooked” done to it, and “Nathan” has the action of the verb directed towards him.
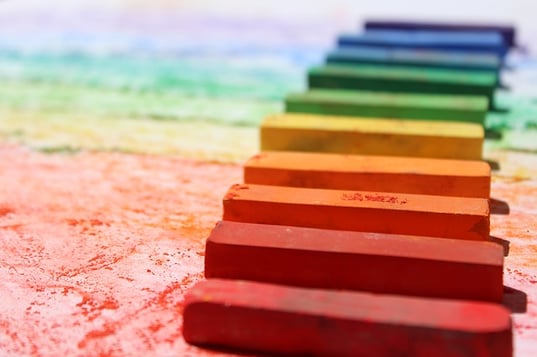
Adjectives are descriptors that help us better understand a sentence. A common adjective type is color.
#3: Adjectives
Here’s the simplest definition of adjectives: adjectives are words that describe other words . Specifically, adjectives modify nouns and noun phrases. In sentences, adjectives appear before nouns and pronouns (they have to appear before the words they describe!).
Adjectives give more detail to nouns and pronouns by describing how a noun looks, smells, tastes, sounds, or feels, or its state of being or existence. . For example, you could say, “The girl rode her bike.” That sentence doesn’t have any adjectives in it, but you could add an adjective before both of the nouns in the sentence—”girl” and “bike”—to give more detail to the sentence. It might read like this: “The young girl rode her red bike.” You can pick out adjectives in a sentence by asking the following questions:
- Which one?
- What kind?
- How many?
- Whose’s?
We’ll look at more examples of adjectives as we explore the subclasses of adjectives next!
Subclasses of Adjectives, Including Examples
Subclasses of adjectives include adjective phrases, comparative adjectives, superlative adjectives, and determiners (which include articles, possessive adjectives, and demonstratives).
Adjective Phrases
An adjective phrase is a group of words that describe a noun or noun phrase in a sentence. Adjective phrases can appear before the noun or noun phrase in a sentence, like in this example:
The extremely fragile vase somehow did not break during the move.
In this case, extremely fragile describes the vase. On the other hand, adjective phrases can appear after the noun or noun phrase in a sentence as well:
The museum was somewhat boring.
Again, the phrase somewhat boring describes the museum. The takeaway is this: adjective phrases describe the subject of a sentence with greater detail than an individual adjective.
Comparative Adjectives and Superlative Adjectives
Comparative adjectives are used in sentences where two nouns are compared. They function to compare the differences between the two nouns that they modify. In sentences, comparative adjectives often appear in this pattern and typically end with -er. If we were to describe how comparative adjectives function as a formula, it might look something like this:
Noun (subject) + verb + comparative adjective + than + noun (object).
Here’s an example of how a comparative adjective would work in that type of sentence:
The horse was faster than the dog.
The adjective faster compares the speed of the horse to the speed of the dog. Other common comparative adjectives include words that compare distance ( higher, lower, farther ), age ( younger, older ), size and dimensions ( bigger, smaller, wider, taller, shorter ), and quality or feeling ( better, cleaner, happier, angrier ).
Superlative adjectives are adjectives that describe the extremes of a quality that applies to a subject being compared to a group of objects . Put more simply, superlative adjectives help show how extreme something is. In sentences, superlative adjectives usually appear in this structure and end in -est :
Noun (subject) + verb + the + superlative adjective + noun (object).
Here’s an example of a superlative adjective that appears in that type of sentence:
Their story was the funniest story.
In this example, the subject— story —is being compared to a group of objects—other stories. The superlative adjective “funniest” implies that this particular story is the funniest out of all the stories ever, period. Other common superlative adjectives are best, worst, craziest, and happiest... though there are many more than that!
It’s also important to know that you can often omit the object from the end of the sentence when using superlative adjectives, like this: “Their story was the funniest.” We still know that “their story” is being compared to other stories without the object at the end of the sentence.
Determiners
The last subclass of adjectives we want to look at are determiners. Determiners are words that determine what kind of reference a noun or noun phrase makes. These words are placed in front of nouns to make it clear what the noun is referring to. Determiners are an example of a part of speech subclass that contains a lot of subclasses of its own. Here is a list of the different types of determiners:
- Definite article: the
- Indefinite articles : a, an
- Demonstratives: this, that, these, those
- Pronouns and possessive determiners: my, your, his, her, its, our, their
- Quantifiers : a little, a few, many, much, most, some, any, enough
- Numbers: one, twenty, fifty
- Distributives: all, both, half, either, neither, each, every
- Difference words : other, another
- Pre-determiners: such, what, rather, quite
Here are some examples of how determiners can be used in sentences:
Definite article: Get in the car.
Demonstrative: Could you hand me that magazine?
Possessive determiner: Please put away your clothes.
Distributive: He ate all of the pie.
Though some of the words above might not seem descriptive, they actually do describe the specificity and definiteness, relationship, and quantity or amount of a noun or noun phrase. For example, the definite article “the” (a type of determiner) indicates that a noun refers to a specific thing or entity. The indefinite article “an,” on the other hand, indicates that a noun refers to a nonspecific entity.
One quick note, since English is always more complicated than it seems: while articles are most commonly classified as adjectives, they can also function as adverbs in specific situations, too. Not only that, some people are taught that determiners are their own part of speech...which means that some people are taught there are 9 parts of speech instead of 8!
It can be a little confusing, which is why we have a whole article explaining how articles function as a part of speech to help clear things up .
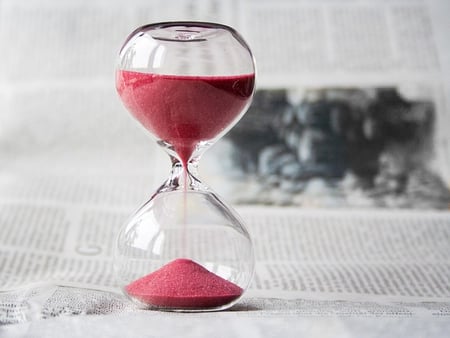
Adverbs can be used to answer questions like "when?" and "how long?"
Adverbs are words that modify verbs, adjectives (including determiners), clauses, prepositions, and sentences. Adverbs typically answer the questions how?, in what way?, when?, where?, and to what extent? In answering these questions, adverbs function to express frequency, degree, manner, time, place, and level of certainty . Adverbs can answer these questions in the form of single words, or in the form of adverbial phrases or adverbial clauses.
Adverbs are commonly known for being words that end in -ly, but there’s actually a bit more to adverbs than that, which we’ll dive into while we look at the subclasses of adverbs!
Subclasses Of Adverbs, Including Examples
There are many types of adverbs, but the main subclasses we’ll look at are conjunctive adverbs, and adverbs of place, time, manner, degree, and frequency.
Conjunctive Adverbs
Conjunctive adverbs look like coordinating conjunctions (which we’ll talk about later!), but they are actually their own category: conjunctive adverbs are words that connect independent clauses into a single sentence . These adverbs appear after a semicolon and before a comma in sentences, like in these two examples:
She was exhausted; nevertheless , she went for a five mile run.
They didn’t call; instead , they texted.
Though conjunctive adverbs are frequently used to create shorter sentences using a semicolon and comma, they can also appear at the beginning of sentences, like this:
He chopped the vegetables. Meanwhile, I boiled the pasta.
One thing to keep in mind is that conjunctive adverbs come with a comma. When you use them, be sure to include a comma afterward!
There are a lot of conjunctive adverbs, but some common ones include also, anyway, besides, finally, further, however, indeed, instead, meanwhile, nevertheless, next, nonetheless, now, otherwise, similarly, then, therefore, and thus.
Adverbs of Place, Time, Manner, Degree, and Frequency
There are also adverbs of place, time, manner, degree, and frequency. Each of these types of adverbs express a different kind of meaning.
Adverbs of place express where an action is done or where an event occurs. These are used after the verb, direct object, or at the end of a sentence. A sentence like “She walked outside to watch the sunset” uses outside as an adverb of place.
Adverbs of time explain when something happens. These adverbs are used at the beginning or at the end of sentences. In a sentence like “The game should be over soon,” soon functions as an adverb of time.
Adverbs of manner describe the way in which something is done or how something happens. These are the adverbs that usually end in the familiar -ly. If we were to write “She quickly finished her homework,” quickly is an adverb of manner.
Adverbs of degree tell us the extent to which something happens or occurs. If we were to say “The play was quite interesting,” quite tells us the extent of how interesting the play was. Thus, quite is an adverb of degree.
Finally, adverbs of frequency express how often something happens . In a sentence like “They never know what to do with themselves,” never is an adverb of frequency.
Five subclasses of adverbs is a lot, so we’ve organized the words that fall under each category in a nifty table for you here:
It’s important to know about these subclasses of adverbs because many of them don’t follow the old adage that adverbs end in -ly.
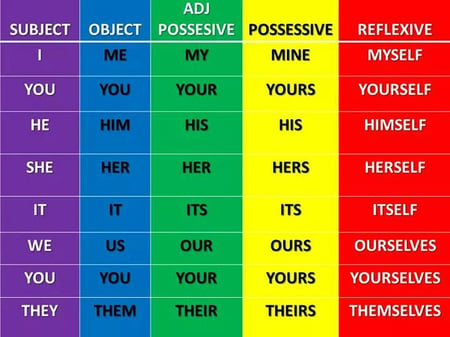
Here's a helpful list of pronouns. (Attanata / Flickr )
#5: Pronouns
Pronouns are words that can be substituted for a noun or noun phrase in a sentence . Pronouns function to make sentences less clunky by allowing people to avoid repeating nouns over and over. For example, if you were telling someone a story about your friend Destiny, you wouldn’t keep repeating their name over and over again every time you referred to them. Instead, you’d use a pronoun—like they or them—to refer to Destiny throughout the story.
Pronouns are typically short words, often only two or three letters long. The most familiar pronouns in the English language are they, she, and he. But these aren’t the only pronouns. There are many more pronouns in English that fall under different subclasses!
Subclasses of Pronouns, Including Examples
There are many subclasses of pronouns, but the most commonly used subclasses are personal pronouns, possessive pronouns, demonstrative pronouns, indefinite pronouns, and interrogative pronouns.
Personal Pronouns
Personal pronouns are probably the most familiar type of pronoun. Personal pronouns include I, me, you, she, her, him, he, we, us, they, and them. These are called personal pronouns because they refer to a person! Personal pronouns can replace specific nouns in sentences, like a person’s name, or refer to specific groups of people, like in these examples:
Did you see Gia pole vault at the track meet? Her form was incredible!
The Cycling Club is meeting up at six. They said they would be at the park.
In both of the examples above, a pronoun stands in for a proper noun to avoid repetitiveness. Her replaces Gia in the first example, and they replaces the Cycling Club in the second example.
(It’s also worth noting that personal pronouns are one of the easiest ways to determine what point of view a writer is using.)
Possessive Pronouns
Possessive pronouns are used to indicate that something belongs to or is the possession of someone. The possessive pronouns fall into two categories: limiting and absolute. In a sentence, absolute possessive pronouns can be substituted for the thing that belongs to a person, and limiting pronouns cannot.
The limiting pronouns are my, your, its, his, her, our, their, and whose, and the absolute pronouns are mine, yours, his, hers, ours, and theirs . Here are examples of a limiting possessive pronoun and absolute possessive pronoun used in a sentence:
Limiting possessive pronoun: Juan is fixing his car.
In the example above, the car belongs to Juan, and his is the limiting possessive pronoun that shows the car belongs to Juan. Now, here’s an example of an absolute pronoun in a sentence:
Absolute possessive pronoun: Did you buy your tickets ? We already bought ours .
In this example, the tickets belong to whoever we is, and in the second sentence, ours is the absolute possessive pronoun standing in for the thing that “we” possess—the tickets.
Demonstrative Pronouns, Interrogative Pronouns, and Indefinite Pronouns
Demonstrative pronouns include the words that, this, these, and those. These pronouns stand in for a noun or noun phrase that has already been mentioned in a sentence or conversation. This and these are typically used to refer to objects or entities that are nearby distance-wise, and that and those usually refer to objects or entities that are farther away. Here’s an example of a demonstrative pronoun used in a sentence:
The books are stacked up in the garage. Can you put those away?
The books have already been mentioned, and those is the demonstrative pronoun that stands in to refer to them in the second sentence above. The use of those indicates that the books aren’t nearby—they’re out in the garage. Here’s another example:
Do you need shoes? Here...you can borrow these.
In this sentence, these refers to the noun shoes. Using the word these tells readers that the shoes are nearby...maybe even on the speaker’s feet!
Indefinite pronouns are used when it isn’t necessary to identify a specific person or thing . The indefinite pronouns are one, other, none, some, anybody, everybody, and no one. Here’s one example of an indefinite pronoun used in a sentence:
Promise you can keep a secret?
Of course. I won’t tell anyone.
In this example, the person speaking in the second two sentences isn’t referring to any particular people who they won’t tell the secret to. They’re saying that, in general, they won’t tell anyone . That doesn’t specify a specific number, type, or category of people who they won’t tell the secret to, which is what makes the pronoun indefinite.
Finally, interrogative pronouns are used in questions, and these pronouns include who, what, which, and whose. These pronouns are simply used to gather information about specific nouns—persons, places, and ideas. Let’s look at two examples of interrogative pronouns used in sentences:
Do you remember which glass was mine?
What time are they arriving?
In the first glass, the speaker wants to know more about which glass belongs to whom. In the second sentence, the speaker is asking for more clarity about a specific time.
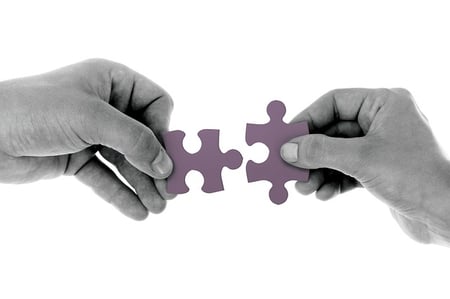
Conjunctions hook phrases and clauses together so they fit like pieces of a puzzle.
#6: Conjunctions
Conjunctions are words that are used to connect words, phrases, clauses, and sentences in the English language. This function allows conjunctions to connect actions, ideas, and thoughts as well. Conjunctions are also used to make lists within sentences. (Conjunctions are also probably the most famous part of speech, since they were immortalized in the famous “Conjunction Junction” song from Schoolhouse Rock .)
You’re probably familiar with and, but, and or as conjunctions, but let’s look into some subclasses of conjunctions so you can learn about the array of conjunctions that are out there!
Subclasses of Conjunctions, Including Examples
Coordinating conjunctions, subordinating conjunctions, and correlative conjunctions are three subclasses of conjunctions. Each of these types of conjunctions functions in a different way in sentences!
Coordinating Conjunctions
Coordinating conjunctions are probably the most familiar type of conjunction. These conjunctions include the words for, and, nor, but, or, yet, so (people often recommend using the acronym FANBOYS to remember the seven coordinating conjunctions!).
Coordinating conjunctions are responsible for connecting two independent clauses in sentences, but can also be used to connect two words in a sentence. Here are two examples of coordinating conjunctions that connect two independent clauses in a sentence:
He wanted to go to the movies, but he couldn’t find his car keys.
They put on sunscreen, and they went to the beach.
Next, here are two examples of coordinating conjunctions that connect two words:
Would you like to cook or order in for dinner?
The storm was loud yet refreshing.
The two examples above show that coordinating conjunctions can connect different types of words as well. In the first example, the coordinating conjunction “or” connects two verbs; in the second example, the coordinating conjunction “yet” connects two adjectives.
But wait! Why does the first set of sentences have commas while the second set of sentences doesn’t? When using a coordinating conjunction, put a comma before the conjunction when it’s connecting two complete sentences . Otherwise, there’s no comma necessary.
Subordinating Conjunctions
Subordinating conjunctions are used to link an independent clause to a dependent clause in a sentence. This type of conjunction always appears at the beginning of a dependent clause, which means that subordinating conjunctions can appear at the beginning of a sentence or in the middle of a sentence following an independent clause. (If you’re unsure about what independent and dependent clauses are, be sure to check out our guide to compound sentences.)
Here is an example of a subordinating conjunction that appears at the beginning of a sentence:
Because we were hungry, we ordered way too much food.
Now, here’s an example of a subordinating conjunction that appears in the middle of a sentence, following an independent clause and a comma:
Rakim was scared after the power went out.
See? In the example above, the subordinating conjunction after connects the independent clause Rakim was scared to the dependent clause after the power went out. Subordinating conjunctions include (but are not limited to!) the following words: after, as, because, before, even though, one, since, unless, until, whenever, and while.
Correlative Conjunctions
Finally, correlative conjunctions are conjunctions that come in pairs, like both/and, either/or, and neither/nor. The two correlative conjunctions that come in a pair must appear in different parts of a sentence to make sense— they correlate the meaning in one part of the sentence with the meaning in another part of the sentence . Makes sense, right?
Here are two examples of correlative conjunctions used in a sentence:
We’re either going to the Farmer’s Market or the Natural Grocer’s for our shopping today.
They’re going to have to get dog treats for both Piper and Fudge.
Other pairs of correlative conjunctions include as many/as, not/but, not only/but also, rather/than, such/that, and whether/or.
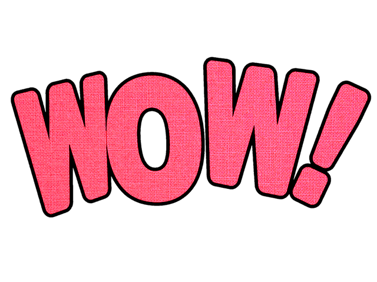
Interjections are single words that express emotions that end in an exclamation point. Cool!
#7: Interjections
Interjections are words that often appear at the beginning of sentences or between sentences to express emotions or sentiments such as excitement, surprise, joy, disgust, anger, or even pain. Commonly used interjections include wow!, yikes!, ouch!, or ugh! One clue that an interjection is being used is when an exclamation point appears after a single word (but interjections don’t have to be followed by an exclamation point). And, since interjections usually express emotion or feeling, they’re often referred to as being exclamatory. Wow!
Interjections don’t come together with other parts of speech to form bigger grammatical units, like phrases or clauses. There also aren’t strict rules about where interjections should appear in relation to other sentences . While it’s common for interjections to appear before sentences that describe an action or event that the interjection helps explain, interjections can appear after sentences that contain the action they’re describing as well.
Subclasses of Interjections, Including Examples
There are two main subclasses of interjections: primary interjections and secondary interjections. Let’s take a look at these two types of interjections!
Primary Interjections
Primary interjections are single words, like oh!, wow!, or ouch! that don’t enter into the actual structure of a sentence but add to the meaning of a sentence. Here’s an example of how a primary interjection can be used before a sentence to add to the meaning of the sentence that follows it:
Ouch ! I just burned myself on that pan!
While someone who hears, I just burned myself on that pan might assume that the person who said that is now in pain, the interjection Ouch! makes it clear that burning oneself on the pan definitely was painful.
Secondary Interjections
Secondary interjections are words that have other meanings but have evolved to be used like interjections in the English language and are often exclamatory. Secondary interjections can be mixed with greetings, oaths, or swear words. In many cases, the use of secondary interjections negates the original meaning of the word that is being used as an interjection. Let’s look at a couple of examples of secondary interjections here:
Well , look what the cat dragged in!
Heck, I’d help if I could, but I’ve got to get to work.
You probably know that the words well and heck weren’t originally used as interjections in the English language. Well originally meant that something was done in a good or satisfactory way, or that a person was in good health. Over time and through repeated usage, it’s come to be used as a way to express emotion, such as surprise, anger, relief, or resignation, like in the example above.
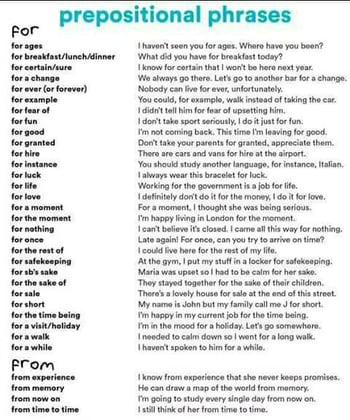
This is a handy list of common prepositional phrases. (attanatta / Flickr)
#8: Prepositions
The last part of speech we’re going to define is the preposition. Prepositions are words that are used to connect other words in a sentence—typically nouns and verbs—and show the relationship between those words. Prepositions convey concepts such as comparison, position, place, direction, movement, time, possession, and how an action is completed.
Subclasses of Prepositions, Including Examples
The subclasses of prepositions are simple prepositions, double prepositions, participle prepositions, and prepositional phrases.
Simple Prepositions
Simple prepositions appear before and between nouns, adjectives, or adverbs in sentences to convey relationships between people, living creatures, things, or places . Here are a couple of examples of simple prepositions used in sentences:
I’ll order more ink before we run out.
Your phone was beside your wallet.
In the first example, the preposition before appears between the noun ink and the personal pronoun we to convey a relationship. In the second example, the preposition beside appears between the verb was and the possessive pronoun your.
In both examples, though, the prepositions help us understand how elements in the sentence are related to one another. In the first sentence, we know that the speaker currently has ink but needs more before it’s gone. In the second sentence, the preposition beside helps us understand how the wallet and the phone are positioned relative to one another!
Double Prepositions
Double prepositions are exactly what they sound like: two prepositions joined together into one unit to connect phrases, nouns, and pronouns with other words in a sentence. Common examples of double prepositions include outside of, because of, according to, next to, across from, and on top of. Here is an example of a double preposition in a sentence:
I thought you were sitting across from me.
You see? Across and from both function as prepositions individually. When combined together in a sentence, they create a double preposition. (Also note that the prepositions help us understand how two people— you and I— are positioned with one another through spacial relationship.)
Prepositional Phrases
Finally, prepositional phrases are groups of words that include a preposition and a noun or pronoun. Typically, the noun or pronoun that appears after the preposition in a prepositional phrase is called the object of the preposition. The object always appears at the end of the prepositional phrase. Additionally, prepositional phrases never include a verb or a subject. Here are two examples of prepositional phrases:
The cat sat under the chair .
In the example above, “under” is the preposition, and “the chair” is the noun, which functions as the object of the preposition. Here’s one more example:
We walked through the overgrown field .
Now, this example demonstrates one more thing you need to know about prepositional phrases: they can include an adjective before the object. In this example, “through” is the preposition, and “field” is the object. “Overgrown” is an adjective that modifies “the field,” and it’s quite common for adjectives to appear in prepositional phrases like the one above.
While that might sound confusing, don’t worry: the key is identifying the preposition in the first place! Once you can find the preposition, you can start looking at the words around it to see if it forms a compound preposition, a double preposition of a prepositional phrase.
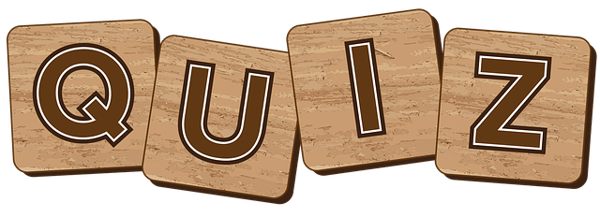
10 Question Quiz: Test Your Knowledge of Parts of Speech Definitions and Examples
Since we’ve covered a lot of material about the 8 parts of speech with examples ( a lot of them!), we want to give you an opportunity to review and see what you’ve learned! While it might seem easier to just use a parts of speech finder instead of learning all this stuff, our parts of speech quiz can help you continue building your knowledge of the 8 parts of speech and master each one.
Are you ready? Here we go:
1) What are the 8 parts of speech?
a) Noun, article, adverb, antecedent, verb, adjective, conjunction, interjection b) Noun, pronoun, verb, adverb, determiner, clause, adjective, preposition c) Noun, verb, adjective, adverb, pronoun, conjunction, interjection, preposition
2) Which parts of speech have subclasses?
a) Nouns, verbs, adjectives, and adverbs b) Nouns, verbs, adjectives, adverbs, conjunctions, and prepositions c) All of them! There are many types of words within each part of speech.
3) What is the difference between common nouns and proper nouns?
a) Common nouns don’t refer to specific people, places, or entities, but proper nouns do refer to specific people, places, or entities. b) Common nouns refer to regular, everyday people, places, or entities, but proper nouns refer to famous people, places, or entities. c) Common nouns refer to physical entities, like people, places, and objects, but proper nouns refer to nonphysical entities, like feelings, ideas, and experiences.
4) In which of the following sentences is the emboldened word a verb?
a) He was frightened by the horror film . b) He adjusted his expectations after the first plan fell through. c) She walked briskly to get there on time.
5) Which of the following is a correct definition of adjectives, and what other part of speech do adjectives modify?
a) Adjectives are describing words, and they modify nouns and noun phrases. b) Adjectives are describing words, and they modify verbs and adverbs. c) Adjectives are describing words, and they modify nouns, verbs, and adverbs.
6) Which of the following describes the function of adverbs in sentences?
a) Adverbs express frequency, degree, manner, time, place, and level of certainty. b) Adverbs express an action performed by a subject. c) Adverbs describe nouns and noun phrases.
7) Which of the following answers contains a list of personal pronouns?
a) This, that, these, those b) I, you, me, we, he, she, him, her, they, them c) Who, what, which, whose
8) Where do interjections typically appear in a sentence?
a) Interjections can appear at the beginning of or in between sentences. b) Interjections appear at the end of sentences. c) Interjections appear in prepositional phrases.
9) Which of the following sentences contains a prepositional phrase?
a) The dog happily wagged his tail. b) The cow jumped over the moon. c) She glared, angry that he forgot the flowers.
10) Which of the following is an accurate definition of a “part of speech”?
a) A category of words that serve a similar grammatical purpose in sentences. b) A category of words that are of similar length and spelling. c) A category of words that mean the same thing.
So, how did you do? If you got 1C, 2C, 3A, 4B, 5A, 6A, 7B, 8A, 9B, and 10A, you came out on top! There’s a lot to remember where the parts of speech are concerned, and if you’re looking for more practice like our quiz, try looking around for parts of speech games or parts of speech worksheets online!
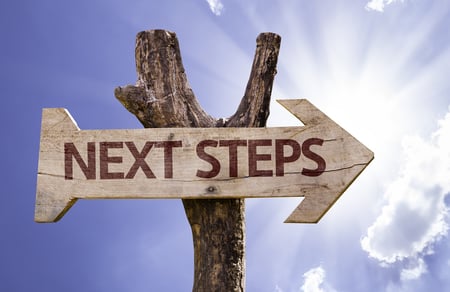
What’s Next?
You might be brushing up on your grammar so you can ace the verbal portions of the SAT or ACT. Be sure you check out our guides to the grammar you need to know before you tackle those tests! Here’s our expert guide to the grammar rules you need to know for the SAT , and this article teaches you the 14 grammar rules you’ll definitely see on the ACT.
When you have a good handle on parts of speech, it can make writing essays tons easier. Learn how knowing parts of speech can help you get a perfect 12 on the ACT Essay (or an 8/8/8 on the SAT Essay ).
While we’re on the topic of grammar: keep in mind that knowing grammar rules is only part of the battle when it comes to the verbal and written portions of the SAT and ACT. Having a good vocabulary is also important to making the perfect score ! Here are 262 vocabulary words you need to know before you tackle your standardized tests.
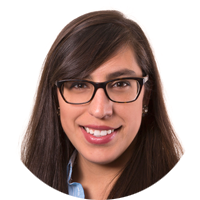
Ashley Sufflé Robinson has a Ph.D. in 19th Century English Literature. As a content writer for PrepScholar, Ashley is passionate about giving college-bound students the in-depth information they need to get into the school of their dreams.
Student and Parent Forum
Our new student and parent forum, at ExpertHub.PrepScholar.com , allow you to interact with your peers and the PrepScholar staff. See how other students and parents are navigating high school, college, and the college admissions process. Ask questions; get answers.

Ask a Question Below
Have any questions about this article or other topics? Ask below and we'll reply!
Improve With Our Famous Guides
- For All Students
The 5 Strategies You Must Be Using to Improve 160+ SAT Points
How to Get a Perfect 1600, by a Perfect Scorer
Series: How to Get 800 on Each SAT Section:
Score 800 on SAT Math
Score 800 on SAT Reading
Score 800 on SAT Writing
Series: How to Get to 600 on Each SAT Section:
Score 600 on SAT Math
Score 600 on SAT Reading
Score 600 on SAT Writing
Free Complete Official SAT Practice Tests
What SAT Target Score Should You Be Aiming For?
15 Strategies to Improve Your SAT Essay
The 5 Strategies You Must Be Using to Improve 4+ ACT Points
How to Get a Perfect 36 ACT, by a Perfect Scorer
Series: How to Get 36 on Each ACT Section:
36 on ACT English
36 on ACT Math
36 on ACT Reading
36 on ACT Science
Series: How to Get to 24 on Each ACT Section:
24 on ACT English
24 on ACT Math
24 on ACT Reading
24 on ACT Science
What ACT target score should you be aiming for?
ACT Vocabulary You Must Know
ACT Writing: 15 Tips to Raise Your Essay Score
How to Get Into Harvard and the Ivy League
How to Get a Perfect 4.0 GPA
How to Write an Amazing College Essay
What Exactly Are Colleges Looking For?
Is the ACT easier than the SAT? A Comprehensive Guide
Should you retake your SAT or ACT?
When should you take the SAT or ACT?
Stay Informed

Get the latest articles and test prep tips!
Looking for Graduate School Test Prep?
Check out our top-rated graduate blogs here:
GRE Online Prep Blog
GMAT Online Prep Blog
TOEFL Online Prep Blog
Holly R. "I am absolutely overjoyed and cannot thank you enough for helping me!”
Please enable JavaScript.
Coggle requires JavaScript to display documents.
Part of speech tagging: a systematic review of deep learning and machine learning approaches
- Alebachew Chiche ORCID: orcid.org/0000-0003-2668-6509 1 &
- Betselot Yitagesu 2
Journal of Big Data volume 9 , Article number: 10 ( 2022 ) Cite this article
31k Accesses
65 Citations
Metrics details
Natural language processing (NLP) tools have sparked a great deal of interest due to rapid improvements in information and communications technologies. As a result, many different NLP tools are being produced. However, there are many challenges for developing efficient and effective NLP tools that accurately process natural languages. One such tool is part of speech (POS) tagging, which tags a particular sentence or words in a paragraph by looking at the context of the sentence/words inside the paragraph. Despite enormous efforts by researchers, POS tagging still faces challenges in improving accuracy while reducing false-positive rates and in tagging unknown words. Furthermore, the presence of ambiguity when tagging terms with different contextual meanings inside a sentence cannot be overlooked. Recently, Deep learning (DL) and Machine learning (ML)-based POS taggers are being implemented as potential solutions to efficiently identify words in a given sentence across a paragraph. This article first clarifies the concept of part of speech POS tagging. It then provides the broad categorization based on the famous ML and DL techniques employed in designing and implementing part of speech taggers. A comprehensive review of the latest POS tagging articles is provided by discussing the weakness and strengths of the proposed approaches. Then, recent trends and advancements of DL and ML-based part-of-speech-taggers are presented in terms of the proposed approaches deployed and their performance evaluation metrics. Using the limitations of the proposed approaches, we emphasized various research gaps and presented future recommendations for the research in advancing DL and ML-based POS tagging.
Introduction
Natural language processing (NLP) has become a part of daily life and a crucial tool today. It aids people in many areas, such as information retrieval, information extraction, machine translation, question-answering speech synthesis and recognition, and so on. In particular, NLP is an automatic approach to analyzing texts using a different set of technologies and theories with the help of a computer. It is also defined as a computerized approach to process and understand natural language. Thus, it improves human-to-human communication, enables human-to-machine communication by doing useful processing of texts or speeches. Part-of-speech (POS) tagging is one of the most important addressed areas and main building block and application in the natural language processing discipline [ 1 , 2 , 3 ]. So, Part of Speech (POS) Tagging is a notable NLP topic that aims in assigning each word of a text the proper syntactic tag in its context of appearance [ 4 , 5 , 6 , 7 , 8 ]. Part-of-speech (POS) tagging, also called grammatical tagging, is the automatic assignment of part-of-speech tags to words in a sentence [ 9 , 10 , 11 ]. A POS is a grammatical classification that commonly includes verbs, adjectives, adverbs, nouns, etc. POS tagging is an important natural language processing application used in machine translation, word sense disambiguation, question answering parsing, and so on. The genesis of POS tagging is based on the ambiguity of many words in terms of their part of speech in a context.
Manually tagging part-of-speech to words is a tedious, laborious, expensive, and time-consuming task; therefore, widespread interest is becoming in automating the tagging process [ 12 ]. As stated by Pisceldo et al. [ 4 ], the main issue that must be addressed in part of speech tagging is that of ambiguity: words behave differently given different contexts in most languages, and thus the difficulty is to identify the correct tag of a word appearing in a particular sentence. Several approaches have been deployed to automatic POS tagging, like transformational-based, rule-based and probabilistic approaches. Rule-based part of speech taggers assign a tag to a word based on manually created linguistic rules; for instance, a word that follows adjectives is tagged as a noun [ 12 ]. And probabilistic approaches [ 12 ] determine the frequent tag of a word in a given context based on probability values calculated from a tagged corpus which is tagged manually. On the other hand, a combination of probabilistic and rule-based approaches is the transformational-based approach to automatically calculate symbolic rules from a corpus.
To accomplish the requirements of an efficient POS tagger, the researchers have explored the possibility of using Deep learning (DL) and Machine learning (ML) techniques. Under the big umbrella of artificial intelligence, both ML and DL aim to learn meaningful information from the given big language resources [ 13 , 14 ]. Because of the growth of powerful graphics processor units (GPUs), these techniques have gained widespread recognition and appeal in the field of natural language processing, notably part of speech tagging (POST), throughout the previous decade. [ 13 , 15 ]. Both ML and DL are powerful tools for extracting valuable and hidden features from the given corpus and assigning the correct POS tags to words based on the patterns discovered. To learn valuable information from the corpus, the ML-based POS tagger relies mostly on feature engineering [ 16 ]. On the other hand, DL-based POS taggers are better at learning complicated features from raw data without relying on feature engineering because of their deep structure [ 17 ].
Different researchers forwarded numerous ML and DL-based solutions to make POS taggers effective in tagging part of speech of words in their context. However, the extensive use of POS tagging and the resulting complications have generated several challenges for POS tagging systems to appropriately tag the word class. The research on using the DL methods for POS tagging is currently in its early stage, and there is still a gap to further explore this approach within POS tagging to effectively assign part of speech within the sentence.
The main contributions of this paper are addressed in three phases. Phase I; we selected recent journal articles focusing on DL- and ML-based POS tagging (published between 2017 and February 2021). Phase II; we extensively reviewed and discussed each article from various parameters such as proposed methods and techniques, weakness, strength, and evaluation metrics. Phase III; in this phase, recent trends in POS tagging using AI methods are provided, challenges in DL/ML-based POS tagging are highlighted, and we provided future research directions in this domain. This review paper is explored based on three aspects: (i) Systematic article selection process is followed to obtain more related research articles on POS tagging implementation using Artificial Intelligence methods, while others reviewed without using the systematic approach. (ii) Our study emphasized the research articles published between 2017 and July 2021 to provide a piece of updated information in the design of AI-oriented POST. (iii) A recent POS tagging model based on the DL and ML approach is reviewed according to their methods and techniques, and evaluation metrics. The intent is to provide new researchers with more updated knowledge on AI-oriented POS tagging in one place.
Therefore, this paper aims to review Artificial Intelligence oriented POS tagging and related studies published from 2017 to 2021 by examining what methods and techniques have been used, what experiments have been conducted, and what performance metrics have been used for evaluation. The research paper provides a comprehensive overview of the advancement and recent trends in DL- and ML-based solutions for POS tagger Systems. The key idea is to provide up-to-date information on recent DL-based and ML-based POS taggers that provide a ground for the new researchers who want to start exploring this research domain.
The rest of the paper is organized as follows: “ Methodology ” section describes the research methodology deployed for the study. “ POS tagging approaches ” section presents the basic POS tagging approaches. “ Artificial Intelligence methods for POS tagging ” section describes the ML and DL methodologies used. The details about the evaluation metrics are shown in “ Evaluation metrics ” section. Recent observations in POS implementation, research challenges, and future research directions are also presented in “ Remarks, challenges, and future trends ” section. Finally, the Conclusion of the review article is presented in “ Conclusion ” section.
Methodology
This study explores a systematic literature review of various DL and ML-based POS tagging and examines the research articles published from 2017 to 2021. A systematic article review is a research methodology conducted to identify, extract, and examine useful literature related to a particular research area. We followed two stages process in this systematic review.
Stage-1 identifies the information resource and keywords to execute query related to "POST" and obtain an initial list of articles. Stage-2 applies certain criteria on the initial list to select the most related and core articles and store them into a final list reviewed in this paper. The main aim of this review paper is to answer some of the following questions: (i) What is state-of-the-art in the design of AI-oriented POS tagging? (ii) What are the current ML and DL methodologies deployed for designing POS tagging? (iii) What are the strengths and weaknesses of deployed methods and techniques? (iv)? What are the most common evaluation metrics used for testing? And (v) What are the future research trends in AI-oriented POS tagging?
In the first phase, keywords and search engines are selected for searching articles. As a potential search engine, Scopus document search is selected due to searching all well-known databases. The search query is executed using the initial keyword like "Part of speech tagging" and filter the publication duration that showed between 2017 and 2021. The initial query search results from articles that proposed POS tagging using different methods like AI-oriented, rule-based stochastic etc., for different applications. Then the query keyword is redefined by combining the keyword deep learning or machine learning to get more important research articles. Accordingly, important articles from query search based on the defined keywords were taken and stored as an initial list of articles. The process of stage-1 is presented in Fig. 1 .
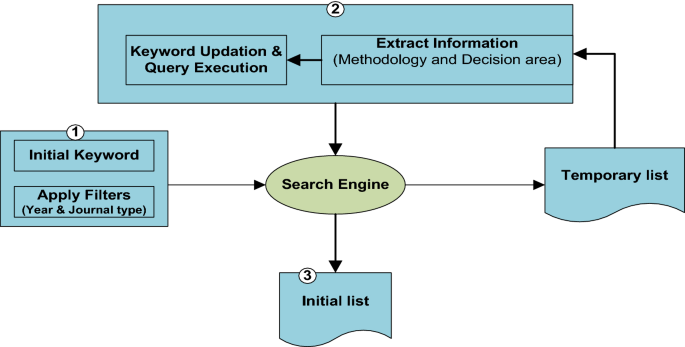
Stage one methodology
Whereas in stage-2, we defined criteria to get a more focused article from the initial list used for analysis. As a result, articles were selected that proposed new ML and DL methods written in English. In this review, we did not include papers with keywords like survey, review, and analysis. Based on these criteria, we selected articles for this review and stored them in the final article list, then used them for analysis. All selected articles which are stored in the final list were analyzed based on the DL or ML methodology proposed and the strengths and weaknesses of the proposed methodology. And also analyzed performance metrics used for evaluation and testing purposes. At last, future research directions and challenges in the design of effective and efficient AI-based POS tagging are identified. The complete process used in stage-1 and stage-2 is summarized in Figs. 1 and 2 , respectively.

Stage two methodology
POS tagging approaches
This section first describes the details about approaches of POS tagging based on its methods and techniques deployed for tagging the given the word. Several POS tagging approaches have been proposed to automatically tag words with part-of-speech tags in a sentence. The most familiar approaches are rule-based [ 18 , 19 ], artificial neural network [ 20 ], stochastic [ 21 , 22 ] and hybrid approaches [ 22 , 23 , 24 ]. The most commonly used part of speech tagging approaches is presented as follows.
A rule-based approach for POS tagging uses hand-crafted rules to assign tags to words in a sentence. According to [ 19 , 25 ], the rules generated mostly depend on linguistic features of the language, such as lexical, morphological, and syntactical information. Linguistic experts may construct these rules or use machine learning on an annotated corpus [ 10 , 11 ]. The first way of getting rules is tedious, prone to error, and time-consuming. Besides, it needs highly a language expert on the language being tagged. For the second process, a model built using experts then learns and stores a sequence of rules using a training corpus without expert rule [ 19 ].
Artificial neural network
Artificial Neural Network is an algorithm inspired by biological neurons and is used to estimate functions that can depend on a large number of inputs, and they are generally unknown [ 29 , 30 ]. It is presented as interconnected systems of "neurons" that are used to exchange messages. The associations between neurons have numeric loads that can be changed dependent on experience, making neural organizations versatile to sources of info and ready to learn. It is an assortment of an enormous number of interconnected handling neurons cooperating to tackle given issues (Fig. 3 ).
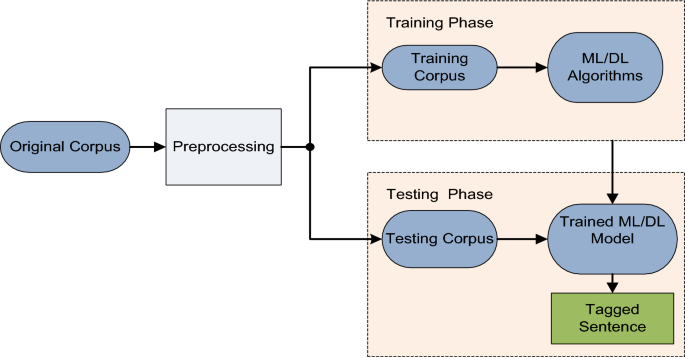
ML/DL Based POS Tagging Model
Like other approaches, an ANN approach that can be used for POS tagger developments requires a pre-processing activity before working on the actual ANN-based tagger [ 11 , 14 ]. The output from the pre-processing task would be taken as an input for the input layer of the neural network. From this pre-processed input, the neural network trains itself by adopting the value of the numeric weights of the connection between input layers until the correct POS tag is provided.
Hidden Markov Model
The hidden Markov model is the most widely implemented POS tagging method under the stochastic approach [ 6 , 23 , 31 ]. It follows a factual Markov model in which the tagger framework being demonstrated is thought to be explored from one state to another with an inconspicuous state. Unlike the Markov model, in HMM, the state is not directly observable to the observer, but the output that depends on the hidden state is visible. As stated in [ 23 , 32 , 33 ], Hidden Markov Model is a familiar statistical model that is used to find the most frequent tag sequence T = {t1, t2, t3… tn} for a word sequence in sentence W = {w1, w2, w3…wn} [ 33 ]. The Viterbi algorithm is a well-known method for tagging the most likely tag sequence for each word in a sentence when using a hidden Markov model.
Maximum Entropy Markov Model
Maximum Entropy Markov is a conditional probabilistic sequence model [ 12 , 34 , 35 ]. Maximum entropy modeling aims to take the probabilistic lexical distribution that scores maximum entropy out of the distributions to complement a certain set of constraints. The constraints limit the model to perform as per a set of measurements collected from the training corpus.
The most commonly deployed statistics for POS tagging are: how often a word was annotated in a certain way and how often labels showed up in a sequence. On the other hand, unlike HMM in the maximum entropy approach, it is likely to effortlessly characterize and include much more complex measurements, which are not confined to n-gram sequences [ 36 ]. Also, the problem of HMM is solved by the Maximum Entropy Markov model (MEMM) because it is possible to include random features sets. However, the MEMM approach has a business problem in labeling because it normalizes not the whole sequence; rather, it normalizes per state [ 35 ].
Artificial intelligence methods for POS tagging
This section provides a general methodology of the AI-based POS tagging along with the details of the most commonly deployed DL and ML algorithms used to implement an effective POS tagging. Both DL and ML are broadly classified into supervised and unsupervised algorithms [ 22 , 32 , 37 , 38 ]. In supervised learning algorithms, the hidden information is extracted from the labeled data. In contrast, unsupervised learning algorithms find useful features and information from the unlabeled data.
Machine Learning Algorithms
Machine Learning could be a set of AI that has all the strategies and algorithms that enable the machines to learn automatically by using mathematical models to extract relevant knowledge from the given datasets [ 15 , 38 , 39 , 40 , 41 , 42 ]. The most common ML algorithms used for POS taggers are Neural Network, Naïve Bayes, HMM, Support Vector Machine (SVM), ANN, Conditional Random Field (CRF), Brill, and TnT.
Naive Bayes
In some circumstances, statistical dependencies between system variables exist. Notwithstanding, it may be hard to definitively communicate the probabilistic connections among these factors [ 43 ]. A probabilistic graph model can be used to exploit these casual dependencies or relationships between the variables of a problem, which is called Naïve Bayesian Networks (NB). The probabilistic model provides an answer for "What is the probability of a given word occurrence before the other words in a given sentence?" by following conditional probability [ 44 ].
Hirpassa et al. [ 39 ] proposed an automatic prediction of POS tags of words in the Amharic language to address the POS tagging problem. The statistical-based POS taggers are compared. The performances of all these taggers, which are Conditional Random Field (CRF), Naive Bays (NB), Trigrams'n'Tags (TnT) Tagger, and an HMM-based tagger, are compared with the same training and testing datasets. The empirical result shows that CRF-based tagger has outperformed the performance of others. The CRF-based tagger has achieved the best accuracy of 94.08% during the experiment.

Support vector machine
Support vector machines (SVM) is first proposed by Vapnik (1998). SVM is a machine learning algorithm used in applications that need binary classification, adopted for various kinds of domain problems, including NLP [ 16 , 45 ]. Basically, an SVM algorithm learns a linear hyperplane that splits the set of positive collections from the set of negative collections with the highest boundary. Surahio and Maha [ 45 ] have tried to develop a prediction System for Sindhi Parts of Speech Tags using the Support Vector Machine learning algorithm. Rule-Based Approach (RBA) and SVM experiment on the same dataset. Based on the experiments, SVM has achieved better detection accuracy when compared to RBA tagging techniques.
Conditional random field (CRF)
A conditional random field (CRF) is a method used for building discriminative probabilistic models that segment and label a given sequential data [ 12 , 33 , 46 , 47 , 48 ]. A conditional random field is an undirected x, y graphical model in which each yi vertex represents a random variable whose distribution is dependent on some observation variable X, and each margin characterizes a dependency between xi and yi random variables. The dependency of Yi on Xi is defined in a set of functions of f(Yi-1,Yi,X,i). Khan et al. [ 22 ] proposed a conditional random field (CRF)-based Urdu POS tagger with both language dependent and independent feature sets.
It used both deep learning and machine learning approaches with the language-dependent feature set using two datasets to compare the effectiveness of ML and DL approaches. Also, Hirpassa et al. [ 39 ] proposed an automatic prediction of POS tags of words in the Amharic language to address the POS tagging problem. The statistical-based POS taggers are compared. The performances of all these taggers, which are Conditional Random Field (CRF), Naive Bays (NB), Trigrams'n'Tags (TnT) Tagger, and an HMM-based tagger, are compared with the same training and testing datasets. The empirical result shows that CRF-based tagger has outperformed the performance of others. The CRF-based tagger has achieved the best accuracy of 94.08% during the experiment.
Hidden Markov model (HMM)
The Hidden Markov model is the most commonly used model for part of speech tagging appropriate [ 49 , 50 , 51 , 52 ]. HMM is appropriate in cases where something is hidden while another is observed. In this case, the observed ones are words, and the hidden one is tagged. Demilie [ 53 ] proposed an Awngi language part of speech tagger using the Hidden Markov Model. They created 23 hand-crafted tag sets and collected 94,000 sentences. A tenfold cross-validation mechanism was used to evaluate the performance of the Awngi HMM POS tagger. The empirical result shows that uni-gram and bi-gram taggers achieve 93.64% and 94.77% tagging accuracy, respectively. The other author, Hirpassa et al. [ 39 ], proposed an automatic prediction of POS tags of words in the Amharic language to address the POS tagging problem. The statistical-based POS taggers are compared. The performances of all these taggers, which are Conditional Random Field (CRF), Naive Bays (NB), Trigrams'n'Tags (TnT) Tagger, and an HMM-based tagger, are compared with the same training and testing datasets. As the empirical result shows, CRF-based tagger has outperformed the performance of others. The CRF-based tagger has achieved the highest accuracy of 94.08% during the experiment.
Deep learning algorithms
Currently, deep learning methods are the most common word in machine learning to automatically extract complex data representation at a high level of abstraction, especially used for extremely complex problems. It is a data-intensive approach to come with a better result than traditional methods (Naïve Bayes, SVM, HMM, etc.). During the text-based corpora, deep learning sequential models are better than feed-forward methods. In this paper, some of the common sequential deep learning methods such as FNN, MLP, GRU, CNN, RNN, LSTM, and BLSTM are discussed.
Multilayer perceptron (MLP)
The neural network (NN) is a machine learning algorithm that mimics the neurons of the human brain for processing information (Haykin, 1999). One of the widely deployed neural network techniques is Multilayer perceptron (MLP) in many NLP and other pattern recognition problems. An MLP neural network consists of three layers: an input layer as input nodes, one or more hidden layers, and an output layer of computation nodes. Besides, the backpropagation learning algorithm is often used to train an MLP neural network, which is also called backpropagation NN. In the beginning, randomly assigned weights are set at the beginning of algorithm training. Then, the MLP algorithm automatically performs weight changing to define the hidden layer unit representation is mostly good at minimizing the misclassification [ 54 , 55 , 56 ]. Besharati et al. [ 54 ] proposed a POS tagging model for the Persian language using word vectors as the input for MLP and LSTM neural networks. Then the proposed model is compared with the results of the other neural network models and with a second-order HMM tagger, which is used as a benchmark.
Long short-term memory
A Long Short-Term Memory (LSTM) is a special kind of RNN network architecture, which has the capability of learning long-term dependencies. An LSTM can also learn to fill the gap in time intervals in more than1000 steps [ 14 , 57 , 58 ].
Bidirectional long short-term memory
Bidirectional LSTM contains two separate hidden layers to process information in both directions. The first hidden layer processes the forward input sequences, while the other hidden layer processes it backward; both are then connected to the same output layer, which provides access to the future and past context of every point in the sequence. Hence BLSTM beat both standard LSTMs and RNNs, and it also significantly provides a faster and more accurate model [ 14 , 58 ].
Gate recurrent unit
Gated recurrent unit (GRU) is an extension of recurrent neural network which aims to process memories of sequence of data by storing prior input state of the network, which they plan to target vectors based on the prior input [ 14 , 58 ].
Feed-forward neural network
A feed-forward neural network (FNN) is one artificial neural network in which connections between the neuron units do not form a cycle. Also, in Feedforward neural networks, information processing is passed through the network input layers to output layers [ 59 ].
Recurrent neural network (RNN)
On the other hand, a recurrent neural network (RNN) is among an artificial neural network model where connections between the processing units form cyclic paths. It is recurrent since they receive inputs, update the hidden layers depending on the prior computations, and that make predictions for all elements of a sequence [ 33 , 46 , 60 , 61 , 62 ].
Deep neural network
In a normal Recurrent Neural Network (RNN), the information pipes through only one layer to the output layer before processing. But Deep Neural Networks (DNN) is a combination of both deep neural networks (DNN) and RNNs concepts [ 33 , 63 ].
Convolutional neural network
A convolutional neural network (CNN) is a deep learning network structure that is more suitable for the information stored in the array's data structure. Like other neural network structures, CNN comprises an input layer, the memory stack of pooling and convolutional layers for extracting feature sets, and then a fully connected layer with a softmax classifier in the classification layer [ 64 , 65 , 66 , 67 , 68 ].
Evaluation metrics
This section describes the most commonly deployed performance metrics for validating the performance of ML and DL methods for POS tagging. All the evaluation metrics are based on the different metrics used in the Confusion Matrix, which is a confusion matrix providing information about the Actual and Predicted class which are; True Positive (TP)—assigns correct tags to the given words, false positive (FP)—assigns incorrect tags to the given words, false negative (FN)—not assign any tags to given words [ 14 , 55 , 72 ].
True Positive (TP): The word correctly tagged as labelled by experts
False Negative (FN): The given word is not tagged to any of the tag sets.
False Positive (FP): The given word tagged wrongly.
True Negative (TN): The occurrences correctly categorized as normal instances.
In addition to these, the various evaluation metrics used in the previous works are,
Precision: The ratio of correctly tagged part of speech to all the samples tagged words:
Recall: The ratio of all samples correctly tagged as tagged to all the samples that are tagged by expert (aka a Detection Rate).
False alarm rate: the false positive rate is defined as the ratio of wrongly tagged word samples to all the samples.
True negative rate: The ratio of the number of correctly tagged samples to all the samples.
Accuracy: The ratio of correctly tagged part of speech to the total number of instances (aka Detection accuracy).
F-Measure: It is the harmonic mean of the Precision and Recall.
Remarks, challenges, and future trends
This section first presents the researcher's observation in POS tagging based on their proposed methodology and performance criteria. It also highlights the potential research gap and challenges and lastly forwards the future trends for the researchers to come up with a robust, efficient, and effective POS tagger.
Observations and state of art
The effectiveness of AI-oriented POS tagging depends on the learning phase using appropriate corpora. For classical machine Learning techniques, the algorithms could be trained under a small corpus to come with better results. But in the presence of a larger corpus size, deep learning methods are preferable compared to the classical machine learning techniques. These methods learn and uncover useful knowledge from given raw datasets. To make POS tagging efficient in tagging unknown words, it needs to be trained with known corpus. In nature, deep learning algorithms are resource hungry in terms of computational resources and time consumption, so the large corpus and deep nature of the algorithms make the learning process difficult.
Table 1 highlights the summary of the strengths and weaknesses of the reviewed articles. It is observed that deep learning-oriented POS tagging methodologies are preferred by researchers nowadays over the machine learning methods because of their efficiency in learning from the large-size corpus in an unlabeled text.
The introduction of GPUs and cloud-based platforms nowadays has eased the implementation of the deep learning method due to the need for extensive computational resources by Deep Learning (DL).
Based on the reviewed article, we observed that for the past three years, the majority of the researchers preferred Deep Learning (DL) tools for developing the POS tagging model, as depicted in Fig. 4 . It is observed that 68% of the proposed approaches are based on the deep learning approaches, 12% of proposed solutions use a hybrid approach by combining machine learning with deep learning algorithms, and the remaining 20% of proposed POS tagger models are implemented based on machine learning methods.
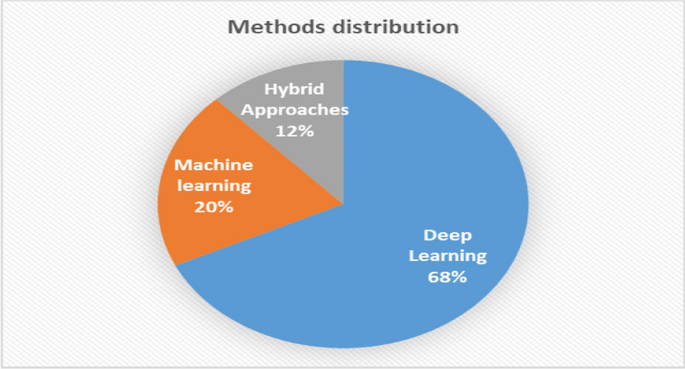
Methods distribution
Besides, Table 2 shows the frequency of Deep Learning and Machine Learning algorithms deployed by different researchers to design an effective POS tagger model. It is shown that the three most frequent deep learning algorithms used are LSTM, RNN, and BiLSTM, respectively. Then the machine learning approaches like CRF and HMM come into the list and are most commonly deployed in the hybrid approach to improve deep learning algorithms. Also, machine learning algorithms like KNN, MLP, and SVM are less frequently used algorithms during this period.
The analysis of the evaluation metrics used in various researches for evaluating the performance of the methodology is presented in Fig. 5 . It is well known that the most commonly deployed performance metrics are Accuracy and Recall (Detection rate). For efficient POS tagging, the model needs a higher Accuracy and Recall. It is observed that the most widely used metrics are accuracy, recall, precision, and F-measure. So, to examine the effectiveness and efficiency of the proposed methodology, these four-evaluation metrics should be taken as performance metrics. For a typical POS tagger developed using machine learning and deep learning algorithms, Accuracy, Recall, F-measure, and Precision should be the compulsory metric to evaluate the methodology (Table 3 ).
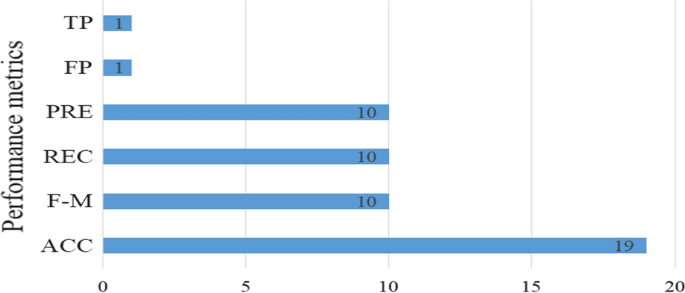
Research challenges
This subsection presents the research challenges that existed in the field of POS tagging.
Lack of Enough and standard dataset: Most recent research studies indicated the unavailability of enough standard corpus for building better POS taggers for a particular language. The proposed methodologies faced difficulties in getting a balanced corpus size for some part of speech within the corpus. To come up with a better POS tagger, it needs to be trained and tested using a balanced and verified corpus. By incorporating a balanced and maximum number of tokens within a corpus, it should enable the DL and ML-based POS tagger to learn more patterns. Then the POS tagger could label words with an appropriate part of speech. But preparing a suitable language corpus is a tedious process that needs plenty of language resources and language experts' knowledge to verify. Therefore, the research challenge for developing an efficient POS tagging model is the preparation of enough and standard corpus with enough tokens of almost all balanced parts of speech. The corpus should be released publicly to help reduce the resource scarcity of the research community.
Lower detection accuracy: It is observed that most of the proposed POS tagging methodologies reveal lower detection accuracy of the POS tagging model as a whole, for some parts of speech tags in particular. This low detection accuracy problem is faced because of the imbalanced nature of the corpus. The ML/DL-based POS tagger trained with less frequent part of speech tags provides low detection accuracy than part of speech with more part of speech. To overcome these problems, it should come up with a balanced corpus and also an efficient technique like Synthetic Minority Over-sampling Technique (SMOTE), RandomOverSampler; which are techniques used to balance unbalanced classes of the corpus. These techniques can be used to increase the number of minority parts of speech tag instances to come up with a balanced corpus. But there is still a research gap to improve accuracy and demands more research effort in this arena.
Resource requirement: Most recent POS tagging methodologies proposed are based on very complex models that need high computing resources and time for processing. These can be solved by using a multi-core high-performance GPU to fasten the computation process and reduce time, but it will incur a high amount of money. The deployment of these complex models may experience an extra processing overhead that will affect the performance of the POS tagger. Besides alleviating the overhead of processing units and computational processes, the most important features must be selected to speed up the processing by using an efficient feature selection algorithm. Although various research works have been explored to come up with the best feature selection algorithm, there is still room for improvement in this direction.
Future directions
This part of the article provides the area which needs further improvement in ML/ DL-oriented POS tagging research.
Efficient POS Tagging Model: As stated, POS tagging is one of the most important and groundwork for any other natural language processing tools like information extraction, information retrieval, machine translation. Recent research works show that there is a constraint in automatically tagging "Unknown" words with a high false positive rate. To this end, the performance of the POS tagger can be improved by using a balanced, up-to-date systematic dataset. An attempt to propose an efficient and complete POS tagging model for most under resource languages using ML/DL methodologies is almost null. So, research can be explored in this area to come up with an efficient POS tagging model that can automatically label parts of speech to words. The POS tagging model should incorporate sentences from different domains in a corpus and repeatedly train the model with the updated corpus to enable the model to learn the new features. This mechanism will ultimately improve the POS tagging model in identifying UNKNOWN words and then minimize false positive rates. Despite the fact that several research studies are being conducted in order to develop an efficient and successful POS tagging strategy, there is still room for improvement.
Way forwards to complex models: Recently, like other domains, ML/DL-oriented POS tagging has been popular because of the ability to learn the feature deeply so as to generate excellent patterns in identifying parts of speech to words. Obviously, the DL-oriented POS tagging models are too complex that need high storage capacity, computational power, and time. This complex nature of the DL-based POS tagging implementation challenges the real-world scenario. The solution to address this problem is to use GPU-based high-performance computers, but GPU-based devices are costly. So, to reduce computational costs, the model can be trained and explored on cloud-based GPU platforms. The second solution forwarded is to use efficient and intelligent feature selection algorithms for reducing the complex nature of deep learning algorithms. This will use less computing resources by selecting the main features while the same detection accuracy is achieved using the whole set of features.
This review paper presents a comprehensive assessment of the part of speech tagging approaches based on the deep learning (DL) and machine learning (ML) methods to provide interested and new researchers with up-to-date knowledge, recent researcher's inclinations, and advancement of the arena. As a research methodology, a systematic approach is followed to prioritize and select important research articles in the field of artificial intelligence-based POS tagging. At the outset, the theoretical concept of NLP and POS tagging and its various POS tagging approaches are explained comprehensively based on the reviewed research articles. Then the methodology that is followed by each article is presented, and strong points and weak points of each article are provided in terms of the capability and difficulty of the POS tagging model. Based on this review, the recent development of research shows the use of deep learning (DL) oriented methodologies improves the efficiency and effectiveness of POS tagging in terms of accuracy and reduction in false-positive rate. Almost 68% of the proposed POS tagging solutions were deep learning (DL) based methods, with LSTM, RNN, and BiLSTM being the three topmost frequently used DL algorithms. The remaining 20% and 12% of proposed POS tagging models are machine learning (ML) and Hybrid approaches, respectively. However, deep learning methods have shown much better tagging performance than the machine learning-oriented methods in terms of learning features by themselves. But these methods are more complex and need high computing resources. So, these difficulties should be solved to improve POS tagging performance. Given the increasing application of DL and ML techniques in POS tagging, this paper can provide a valuable reference and a baseline for researches in both ML and DL fields that want to pull the potential of these techniques in the POS tagging arena. Proposing an efficient POS tagging model by adopting less complex deep learning algorithms and an effective POS tagging in terms of detection mechanism is a potential future research area. Further, the researcher will use this knowledge to propose a new and efficient deep learning-based POS tagging which will effectively identify a part of the speech of words within the sentences.
Availability of data and materials
Not applicable.
Abbreviations
Autoencoder
Artificial Intelligence
Artificial Neural Network
Bidirectional Long Short-Term Memory
Convolutional Neural Network
Conditional Random Field
Deep Belief Network
Deep Learning
Deep Neural Network
False Alarm Rate
False Negative
Feedforward Neural Network
False Positive
Gated Recurrent Unit
Synthetic Minority Over-sampling Technique
K-Nearest Neighbor
Long Short-Term Memory
Machine Learning
Multilayer Perceptron
Naïve Bayes
Natural Language Processing
Part of Speech
Part of Speech Tagging
Recurrent Neural Network
Support Vector Machine
True Negative
True Positive
Alharbi R, Magdy W, Darwish K, AbdelAli A, Mubarak H. Part-of-speech tagging for Arabic Gulf dialect using Bi-LSTM. Int Conf Lang Resour Eval. 2018;3925–3932:2019.
Google Scholar
Demilie WB. Analysis of implemented part of speech tagger approaches: the case of Ethiopian languages. Indian J Sci Technol. 2020;13(48):4661–71.
Article Google Scholar
Sánchez-Martínez F, Pérez-Ortiz JA, Forcada ML. Using target-language information to train part-of-speech taggers for machine translation. Mach Transl. 2008;22(1–2):29–66.
Singh J, Joshi N, Mathur I. Part of speech tagging of marathi text using trigram method. Int J Adv Inf Technol. 2013;3(2):35–41.
Marques NC, Lopes GP. Using Neural Nets for Portuguese Part-of-Speech Tagging. In: Proc. Fifth Int. Conf. Cogn. Sci. Nat. Lang. Process., no. August, 1996.
Kumawat D, Jain V. POS tagging approaches: a comparison. Int J Comput Appl. 2015;118(6):32–8.
Chungku C, Rabgay J, Faaß G. Building NLP resources for Dzongkha: a tagset and a tagged corpus. in: Proceedings of the 8th Workshop on Asian Language Resources, pp. 103–110. 2010.
Singh J, Joshi N, Mathur I. Development of Marathi part of speech tagger using statistical approach. In: Proc. 2013 Int. Conf. Adv. Comput. Commun. Informatics, ICACCI 2013, no. October 2013, pp. 1554–1559, 2013.
Cutting D. A Practical Part-of-Speech Tagger Doug Cutting and Julian Kupiec and Jan Pedersen and Penelope Sibun Xerox Palo Alto Research Center 3333 Coyote. In: Proc. Conf., pp. 133–140, 1992.
Lv C, Liu H, Dong Y, Chen Y. Corpus based part-of-speech tagging. Int J Speech Technol. 2016;19(3):647–54.
Divyapushpalakshmi M, Ramalakshmi R. An efficient sentimental analysis using hybrid deep learning and optimization technique for Twitter using parts of speech (POS) tagging. Int J Speech Technol. 2021;24(2):329–39.
Pisceldo F, Adriani M, and R. Manurung R. Probabilistic Part of Speech Tagging for Bahasa Indonesia. In: Proc. 3rd Int. MALINDO Work. Coloca. event ACL-IJCNLP. 2009.
Alzubaidi L, et al. Review of deep learning: concepts, CNN architectures, challenges, applications. Fut Direct. 2021;8:1.
Deshmukh RD, Kiwelekar A. Deep Learning Techniques for Part of Speech Tagging by Natural Language Processing. In: 2nd Int. Conf. Innov. Mech. Ind. Appl. ICIMIA 2020 - Conf. Proc., no. Icimia, pp. 76–81, 2020.
Crawford M, Khoshgoftaar TM, Prusa JD, Richter AN, Al Najada H. Survey of review spam detection using machine learning techniques. J Big Data. 2015;2:1.
Antony PJ, Mohan SP, Soman KP. SVM based part of speech tagger for Malayalam. In: ITC 2010 - 2010 Int. Conf. Recent Trends Information Telecommunication Computer. p. 339–341, 2010.
Najafabadi MM, Villanustre F, Khoshgoftaar TM, Seliya N, Wald R, Muharemagic E. Deep learning applications and challenges in big data analytics. J Big Data. 2015;2(1):1–21.
Brill E. Transformation-based error-driven learning and natural language processing: a case study in part-of-speech tagging. Comput Linguist. 1995;21(4):543–66.
Brill E. Rule-Based Part of Speech. In: Proc. third Conf. Appl. Nat. Lang. Process. (ANLC ’92), pp. 152–155; 1992.
Brill E. A Simple Rule-Based Part Of Speech Tagger. In: Proceedings of the Third Conference on Applied Computational Linguistics (ACL), Trento, Italy, 1992, pp. 1–14; 1992.
Mamo G, Meshesha M. Parts of speech tagging for Afaan Oromo. Int J Adv Comput Sci Appl. 2011;1(3):1–5.
Hall J. A Probabilistic Part-of-Speech Tagger with Suffix Probabilities A Probabilistic Part-of-Speech Tagger with Suffix Probabilities. MSc: Thesis, Växjö University; 2003.
Zin KK. Hidden markov model with rule based approach for part of speech tagging of Myanmar language. In: Proc. 3rd Int. Conf. Commun. Inf. Technol. CIT’09 , pp. 123–128; 2009.
Altunyurt L, Orhan Z, Güngör T. A composite approach for part of speech tagging in Turkish. InProceeding of International Scientific Conference on Computer Science, Istanbul, Turkey 2006.
Pham B. Parts of Speech Tagging : Rule-Based. https://digitalcommons.harrisburgu.edu/cisc_student-coursework/2 , February, 2020.
Mekuria Z. Design and development of part-of-speech tagger for Kafi-noonoo Language. MSc: Thesis, Addis Ababa University, Ethiopia; 2013.
Farhat NH. Photonit neural networks and learning mathines the role of electron-trapping materials. IEEE Expert Syst their Appl. 1992;7(5):63–72.
Chen CLP, Zhang CY, Chen L, Gan M. Fuzzy restricted boltzmann machine for the enhancement of deep learning. IEEE Trans Fuzzy Syst. 2015;23(6):2163–73.
Chen T. An innovative fuzzy and artificial neural network approach for forecasting yield under an uncertain learning environment. J Ambient Intell Humaniz Comput. 2018;9(4):1013–25.
Lu BL, Ma Q, Ichikawa M, Isahara H. Efficient part-of-speech tagging with a min-max modular neural-network model. Appl Intell. 2003;19(1–2):65–81.
Article MATH Google Scholar
Nisheeth J, Hemant D, Iti M. HMM based POS tagger for Hindi. In: Proceeding of 2013 International Conference on Artificial Intelligence and Soft Computing. pp. 341–349, 2013. http://doi: https://doi.org/10.5121/csit.2013.3639
Getinet Y. Unsupervised Part Of Speech Tagging For Amharic. MSc: Thesis, University of Gondar Ethiopia; 2015.
Khan W, et al. Part of speech tagging in urdu: comparison of machine and deep learning approaches. IEEE Access. 2019;7:38918–36.
Silfverberg M, Ruokolainen T, Kurimo M, Linden K. PVS A, Karthik G. Part-of-speech tagging and chunking using conditional random fields and transformation based learning. Shallow Parsing for South Asian Languages. 2007; pp. 259–264.
Wang G, Sun J, Ma J, Xu K, Gu J. Sentiment classification: the contribution of ensemble learning. Decis Support Syst. 2014;57(1):77–93.
Xia R, Zong C, Li S. Ensemble of feature sets and classification algorithms for sentiment classification. Inf Sci (Ny). 2011;181(6):1138–52.
Biemann C. Unsupervised part-of-speech tagging in the large. Res Lang Comput. 2009;7(2):101–35.
Moraboena S, Ketepalli G, Ragam P. A deep learning approach to network intrusion detection using deep autoencoder. Rev d’Intelligence Artif. 2020;34(4):457–63.
Hirpssa S, Lehal GS. POS tagging for amharic text: a machine learning approach. INFOCOMP. 2020;19(1):1–8.
Gupta V, Singh VK, Mukhija P, Ghose U. Aspect-based sentiment analysis of mobile reviews. J Intell Fuzzy Syst. 2019;36(5):4721–30.
Mansour RF, Escorcia-Gutierrez J, Gamarra M, Gupta D, Castillo O, Kumar S. Unsupervised deep learning based variational autoencoder model for COVID-19 diagnosis and classification. Pattern Recognit Lett. 2021;151:267–74.
Jacob SS, Vijayakumar R. Sentimental analysis over twitter data using clustering based machine learning algorithm. J Ambient Intelligence Humanized Computing. 2021;4:1–2.
Tseng C, Patel N, Paranjape H, Lin TY, Teoh S. Classifying Twitter Data with Naive Bayes Classifier. In: 2012 IEEE International Conference on Granular Computing Classifying , 2012; pp. 1–6.
Kumar S, Nezhurina MI. An ensemble classification approach for prediction of user’s next location based on Twitter data. J Ambient Intell Humaniz Comput. 2019;10(11):4503–13.
Surahio FA, Mahar JA. Prediction system for sindhi parts of speech tags by using support vector machine. In: 2018 International Conference on Computing, Mathematics and Engineering Technologies (iCoMET) 2018; pp. 1-6.
Gashaw I, Shashirekha H. Machine Learning Approaches for Amharic Parts-of-speech Tagging,” in Proc. of ICON-2018, Patiala, India, pp.69–74, December 2018.
Suraksha NM, Reshma K, Kumar KS. “Part-Of-Speech Tagging And Parsing Of Kannada Text Using Conditional Random Fields ( CRFs ),” 2017 International Conference on Intelligent Computing and Control (I2C2) , 2017.
Sutton C, McCallum A. An introduction to conditional random fields. Found Trends Mach Learn. 2011;4(4):267–373.
Khorjuvenkar DN, Ainapurkar M, Chagas S. Parts of speech tagging for Konkani language. In: Proc. 2nd Int. Conf. Comput. Methodol. Commun. ICCMC 2018, no. ICCMC, pp. 605–607, 2018.
Ankita, Abdul Nazeer KA. Part-of-speech tagging and named entity recognition using improved hidden markov model and bloom filter. In: 2018 Int. Conf. Comput. Power Commun. Technol. GUCON 2018, pp. 1072–1077, 2019.
Mohammed S. Using machine learning to build POS tagger for under-resourced language: the case of Somali. Int J Inf Technol. 2020;12(3):717–29.
Mathew W, Raposo R, Martins B. Predicting future locations with hidden Markov models. In: Proceedings of the 2012 ACM conference on ubiquitous computing; 2012, p. 911–18.
Demilie WB. Parts of Speech Tagger for Awngi Language. Int J Eng Sci Comput. 2019;9:1.
Besharati S, Veisi H, Darzi A, Saravani SHH. A hybrid statistical and deep learning based technique for Persian part of speech tagging. Iran J Comput Sci. 2021;4(1):35–43.
Argaw M. Amharic Parts-of-Speech Tagger using Neural Word Embeddings as Features Amharic Parts-of-Speech Tagger using Neural Word Embeddings as Features. MSc.Thesis: Addis Ababa University, Ethiopia; 2019.
Singh A, Verma C, Seal S, Singh V. Development of part of speech tagger using deep learning. Int J Eng Adv Technol. 2019;9(1):3384–91.
Bahcevan CA, Kutlu E, Yildiz T. Deep Neural Network Architecture for Part-of-Speech Tagging for Turkish Language. UBMK 2018 - 3rd Int. Conf. Comput. Sci. Eng. , pp. 235–238, 2018.
Gopalakrishnan A, Soman KP, Premjith B. Part-of-speech tagger for biomedical domain using deep neural network architecture. In: 2019 10th International Conference on Computing, Communication and Networking Technologies (ICCCNT) 2019, pp. 1-5.
Anastasyev D, Gusev I, Indenbom E. Improving part-of-speech tagging via multi-task learning and character-level word representations. Komp’juternaja Lingvistika i Intellektual’nye Tehnol. , vol. 2018-May, no. 17, pp. 14–27, 2018.
Prabha G, Jyothsna PV, Shahina KK, Premjith B, Soman KP. “A Deep Learning Approach for Part-of-Speech Tagging in Nepali Language,” 2018 Int. Conf. Adv. Comput. Commun. Informatics, ICACCI 2018 , pp. 1132–1136, 2018.
Sayami S, Shakya S. Nepali POS Tagging Using Deep Learning Approaches. Int J Sci. 2020;17:69–84.
Attia M, Samih Y, Elkahky A, Mubarak H, Abdelali A, Darwish K. POS Tagging for Improving Code-Switching Identification in Arabic. no. August, pp. 18–29, 2019.
Srivastava P, Chauhan K, Aggarwal D, Shukla A, Dhar J, Jain VP. Deep learning based unsupervised POS tagging for Sanskrit. In: Proceedings of the 2018 International Conference on Algorithms, Computing and Artificial Intelligence 2018; pp. 1-6.
Pasupa K, Ayutthaya TS. Thai sentiment analysis with deep learning techniques: a comparative study based on word embedding, POS-tag, and sentic features. Sustain Cities Soc. 2019;50:101615.
Meftah S, Semmar N, Sadat F, Hx KA. A neural network model for part-of-speech tagging of social media texts. In Proceedings of the Eleventh International Conference on Language Resources and Evaluation (LREC-2018).pdf,” pp. 2821–2828, 2018.
Mishra P. Building a Kannada POS Tagger Using Machine Learning and Neural Network Models. arXiv:1808.03175.
Gupta V, Jain N, Shubham S, Madan A, Chaudhary A, Xin Q. “Toward Integrated CNN-based Sentiment Analysis of Tweets for Scarce-resource Language—Hindi”, ACM Trans . Asian Low-Resource Lang Inf Process. 2021;20(5):1–23.
Gupta V, Juyal S, Singh GP, Killa C, Gupta N. Emotion recognition of audio/speech data using deep learning approaches. J Inf Optim Sci. 2020;41(6):1309–17.
Kumar S, Kumar MA, Soman KP. Deep learning based part-of-speech tagging for Malayalam Twitter data (Special issue: deep learning techniques for natural language processing). J Intelligent Syst. 2019;28(3):423–35.
Baig A, Rahman MU, Kazi H, Baloch A. Developing a pos tagged corpus of urdu tweets. Computers. 2020;9(4):1–13.
Bonchanoski M, Zdravkova K. Machine learning-based approach to automatic POS tagging of macedonian language. In: ACM Int. Conf. Proceeding Ser. , vol. Part F1309, 2017.
Kumar S, Kumar MA, Soman KP. Deep learning based part-of-speech tagging for Malayalam twitter data (Special issue: Deep learning techniques for natural language processing). J Intell Syst. 2019;28(3):423–35.
Kabir MF, Abdullah-Al-Mamun K, Huda MN. Deep learning based parts of speech tagger for Bengali. In: 2016 5th International Conference on Informatics, Electronics and Vision (ICIEV) 2016; pp. 26-29.
Patoary AH, Kibria MJ, Kaium A. Implementation of Automated Bengali Parts of Speech Tagger: An Approach Using Deep Learning Algorithm. In: 2020 IEEE Region 10 Symposium (TENSYMP) 2020; pp. 308-311.
Akhil KK, Rajimol R, Anoop VS. Parts-of-Speech tagging for Malayalam using deep learning techniques. Int J Inf Technol. 2020;12(3):741–8.
Download references
Acknowledgements
Author information, authors and affiliations.
Department of Information Systems, College of Computing, Debre Berhan University, Debre Berhan, Ethiopia
Alebachew Chiche
Department of Computer Science, College of Computing, Debre Berhan University, Debre Berhan, Ethiopia
Betselot Yitagesu
You can also search for this author in PubMed Google Scholar
Contributions
AC prepared the manuscript including summarizing some of the surveyed work. BY prepared the technical report upon which the manuscript is based and summarized several of the surveyed work. Both authors read and approved the final manuscript.
Corresponding author
Correspondence to Alebachew Chiche .
Ethics declarations
Ethics approval and consent to participate, consent for publication, competing interests.
The authors declare that they have no competing interests.
Additional information
Publisher's note.
Springer Nature remains neutral with regard to jurisdictional claims in published maps and institutional affiliations.
Rights and permissions
Open Access This article is licensed under a Creative Commons Attribution 4.0 International License, which permits use, sharing, adaptation, distribution and reproduction in any medium or format, as long as you give appropriate credit to the original author(s) and the source, provide a link to the Creative Commons licence, and indicate if changes were made. The images or other third party material in this article are included in the article's Creative Commons licence, unless indicated otherwise in a credit line to the material. If material is not included in the article's Creative Commons licence and your intended use is not permitted by statutory regulation or exceeds the permitted use, you will need to obtain permission directly from the copyright holder. To view a copy of this licence, visit http://creativecommons.org/licenses/by/4.0/ .
Reprints and permissions
About this article
Cite this article.
Chiche, A., Yitagesu, B. Part of speech tagging: a systematic review of deep learning and machine learning approaches. J Big Data 9 , 10 (2022). https://doi.org/10.1186/s40537-022-00561-y
Download citation
Received : 22 September 2021
Accepted : 10 January 2022
Published : 24 January 2022
DOI : https://doi.org/10.1186/s40537-022-00561-y
Share this article
Anyone you share the following link with will be able to read this content:
Sorry, a shareable link is not currently available for this article.
Provided by the Springer Nature SharedIt content-sharing initiative
- Machine learning
- Deep learning
- Hybrid approach
- Part of speech
- Part of speech tagging
- Performance metrics
“Parts of Speech” and “Word Classes”: Defining Basic Categories for Grammatical Analysis
- First Online: 25 November 2019
Cite this chapter
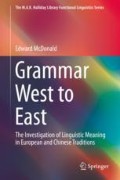
- Edward McDonald 4
Part of the book series: The M.A.K. Halliday Library Functional Linguistics Series ((TMAKHLFLS))
298 Accesses
Although for the most part, as noted earlier, questions of “grammar”, of the “meaningful arrangement” (cf. McDonald 2008) of wordings, did not figure prominently in the Chinese linguistic tradition, there was one outstanding exception. From very early times, Chinese scholars noted the presence in the language of special kinds of words that eventually came to be known as “empty words” xūzì 虛字, or what would now be called “function words” or “grammatical words”. Such words, as we saw in Chap. 3 , are a prominent feature of Old Chinese, where the bulk of grammatical meanings are expressed through them. For example, if we take an extract from the opening of the Analects ,
This is a preview of subscription content, log in via an institution to check access.
Access this chapter
- Available as PDF
- Read on any device
- Instant download
- Own it forever
- Available as EPUB and PDF
- Durable hardcover edition
- Dispatched in 3 to 5 business days
- Free shipping worldwide - see info
Tax calculation will be finalised at checkout
Purchases are for personal use only
Institutional subscriptions
The Chinese surname Mă 馬 is literally ‘horse’
Suggestions for further reading
Chen B (2015) [A study of linguistic methodology in 20th century China]. 2.1 [Word class theory]. The Commercial Press, Beijing. 陳保亞《20世紀中國語言學方法論研究》2.1 詞類論, 商務印書館, 北京。
Google Scholar
Robins RH (1966) The development of the word class system of the European grammatical tradition. Found Lang 2(1):3–19
Yang X (2005) The Pragmatic Turn: Articulating Communicative Practice in the Analects. Oriens Extremus 45:235–254
Download references
Author information
Authors and affiliations.
Sydney, NSW, Australia
Edward McDonald
You can also search for this author in PubMed Google Scholar
Rights and permissions
Reprints and permissions
Copyright information
© 2020 Springer Nature Singapore Pte Ltd.
About this chapter
McDonald, E. (2020). “Parts of Speech” and “Word Classes”: Defining Basic Categories for Grammatical Analysis. In: Grammar West to East. The M.A.K. Halliday Library Functional Linguistics Series. Springer, Singapore. https://doi.org/10.1007/978-981-13-7597-2_12
Download citation
DOI : https://doi.org/10.1007/978-981-13-7597-2_12
Published : 25 November 2019
Publisher Name : Springer, Singapore
Print ISBN : 978-981-13-7595-8
Online ISBN : 978-981-13-7597-2
eBook Packages : Social Sciences Social Sciences (R0)
Share this chapter
Anyone you share the following link with will be able to read this content:
Sorry, a shareable link is not currently available for this article.
Provided by the Springer Nature SharedIt content-sharing initiative
- Publish with us
Policies and ethics
- Find a journal
- Track your research
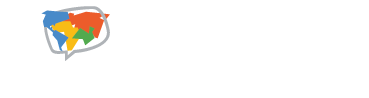
Explore More
Stay in our orbit.
Stay connected with industry news, resources for English teachers and job seekers, ELT events, and more.
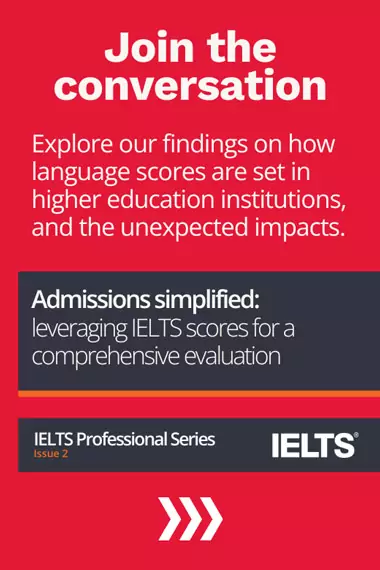
Explore Topics
- Global Elt News
- Job Resources
- Industry Insights
- Teaching English Online
- Classroom Games / Activities
- Teaching English Abroad
- Professional Development
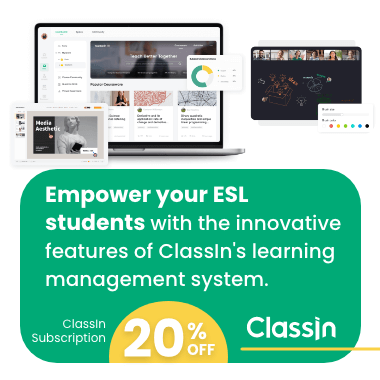
Popular Articles
- 5 Popular ESL Teaching Methods Every Teacher Should Know
- 10 Fun Ways to Use Realia in Your ESL Classroom
- How to Teach ESL Vocabulary: Top Methods for Introducing New Words
- Advice From an Expert: TEFL Interview Questions & How to Answer Them
- What Is TESOL? What Is TEFL? Which Certificate Is Better – TEFL or TESOL?
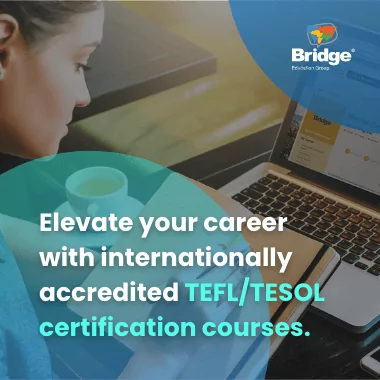
How to Teach Parts of Speech: Lesson Tips & Activities for ESL Teachers
Coleen monroe.
- March 22, 2021
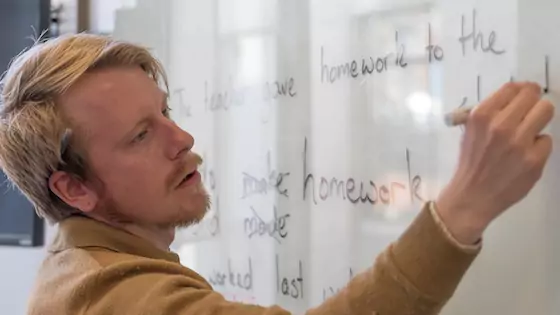
Bridge grad Coleen Monroe previously taught English in South Korea and Chile. She has since gone on to earn her Master’s in Linguistics from University College London and is currently teaching English in China. As a seasoned teacher, we asked Coleen to again share her expertise — this time on how to teach parts of speech. A version of this post also appeared on her personal blog, Reverse Retrograde , about travel, TEFL, and more.
If you’re new to teaching, you’ll want to get initial training and qualification with a TEFL certificate . You can explore our online TEFL courses to get started!
Okay, so you’ve realized that grammar is a thing and that your new job as a TEFL/TESOL teacher requires you to know something about how to teach it. Good start! But you won’t get far unless you’re able to guide students to label the parts of the sentences that you use in daily speech, and especially in writing. The parts of speech are a foundation for all the other skills you need as a teacher in the ESL classroom, so let’s get started with how to teach parts of speech.
Want more ideas for teaching grammar? Earn a Specialized TEFL/TESOL Certificate in Teaching English Grammar!
Why teach parts of speech?
In order to teach parts of speech to ESL learners, you first need to know why this grammar topic is essential. The tips below will help you to be able to show your students why it’s important to learn parts of speech in the ESL classroom.
- Parts of speech are a part of universal human grammar. In other words, they exist in every human language as categories.
- Parts of speech are essential to being able to use other grammar in a new language.
- Students will need to be able to identify and manipulate parts of speech in order to conjugate verbs. This is particularly important for verb agreement, which is a common problem for ESL learners.
- In some parts of the world, grammar is considered to be the most important part of ESL. Using the activities in this article will demonstrate to parents and other teachers that you’re putting in the effort to teach grammar in your classroom.
- Whatever your learners’ goals, they’ll need to be able to understand the basics of English. Even kindergartners can learn parts of speech in a simple way and use them to help further their English understanding.
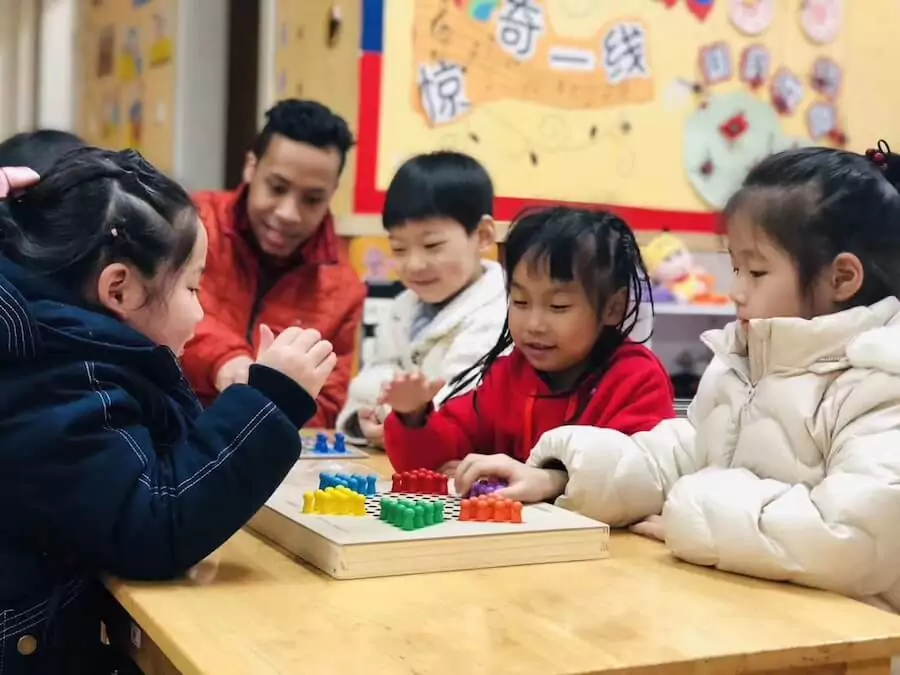
Teacher Stefano, from Jamaica , uses games to teach ESL to his students.
What are the types of parts of speech?
Before learning how to teach parts of speech to ESL students, let’s have a refresher on what the parts of speech are.
I made it a goal to teach my students the parts of speech in every lesson. For the past few months, at some point in the lesson, I write “Parts of Speech” on the board. Underneath, I write the following:
- Noun: a person, place, or thing
- Verb: an action
- Adjective: describes a noun
- Adverb: describes a verb (-ly)
That’s basically all you need to know about the parts of speech as well. You don’t need to know about how they “work” in theory to effectively teach this topic. You can use these simplified definitions to teach parts of speech to most levels of students.
If necessary, you can add more complicated parts of speech:
- Prepositions: on, after, in, etc. (shows where something/someone is)
- Pronouns: she, he, they, etc. (not a name)
- Articles : a, an, the
- Conjunctions: or, but, and, because, so, etc. (connect ideas)
These descriptions are designed for low- to intermediate-level learners and will help you to teach parts of speech in a fun and clear way. This is not an exhaustive guide, but it should help you to be able to write your objectives for a parts of speech lesson.
Get more tips on using objectives in ESL lesson plans.
How do you teach parts of speech?
Teaching parts of speech lessons to your ESL students doesn’t have to be boring. You can make it as interesting or as intricate as you need. The following activities for teaching parts of speech involve little to no prep. You can use them in your lessons frequently, as repetition builds familiarity!
1. Classroom treasure hunt
Elicit the parts of speech by giving examples for each.
Teacher: What’s a noun? (Pointing to a trash can): Oh, look! A noun! (Pointing to a chair): Oh, look! A noun! (Pointing to self): Oh, look! A noun!
Students will then be able to give more examples.
Use this as a basis for a new game. Students should be in small groups or pairs. Set a timer and have them write down as many of a certain part of speech as they can see in the classroom. Then, switch to a different part of speech and have them attempt to write more words. If you like, you can make it so that it’s harder each round or you can eliminate those who don’t write a certain number of words in a round.
- If you’re teaching online, you can still have students hunt for parts of speech in their own homes.
Learn the most popular ESL teaching methods to use in your classroom.
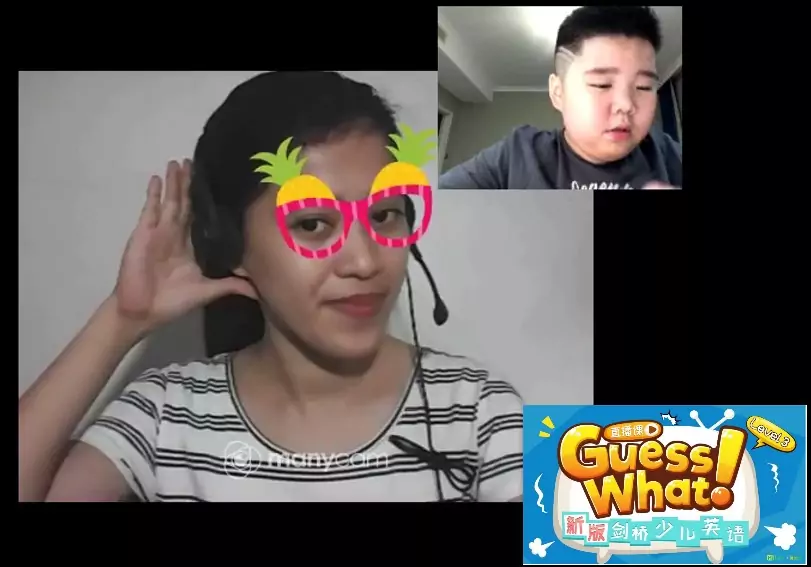
Teacher Juicy Mae, from the Philippines , plays a game with her ESL student online.
2. Grammar by numbers
Use a coloring sheet with a “Paint by Numbers” scheme based on words and their part of speech. This is a good lesson plan for parts of speech for young learners. This works really well for getting students to work together and makes a nice project to show parents, too! Just be aware that some English words can play many roles in a sentence.
For example:
Dream A dream: noun form To dream: verb form Dream job: adjective form
This is a good opportunity to remind your students that English grammar is not a precise science and that the “rules” they learn in school may or may not actually hold up in real life. The ambiguity may cause their heads to temporarily explode, but I promise it’s better for them in the long run. (“WHAT DO YOU MEAN THERE ISN’T A RIGHT ANSWER???” Ahhhhhhhhhhh!”)
- Older learners can do a version of this without coloring. Simply create a worksheet that involves matching the parts of speech with words. Online teachers can email their worksheets to students and have them complete the activity for homework.
Read these top tips for creating materials for the EFL classroom.
3. Sorting race
Before class, create a table in Word, PowerPoint, or something similar. It should be a grid that has different categories for parts of speech and words that exemplify these categories. Print out the table, cut it up, and put the papers into a box or bag.
Pass out the box(es) and set a timer or play some music. The objective of this activity is for students to sort the pieces of paper correctly into the different categories.
- To make it more competitive, put the students into teams, for example, Team Noun or Team Verb. Give each team their own box and have them race to find all of the words that fit into their part of speech category.
Check out more team-based activities for the ESL classroom or online.
4. Swat words like flies
This activity requires a text in English. You can use a class textbook or article or you can prepare a text of your own to bring to class. Each person or team in the classroom needs to have a copy of the text.
There are two ways to play this game, but both involve hitting the text very hard with one’s hand. The idea is that the word they find on the page should be treated like a fly or a mosquito that they’re trying to kill. This makes it a “beat the buzzer” style game.
- In the first version, the teacher should say a word that appears in the text. The students can search the text for the word and when they find it, slap the book or paper. Whoever finds it first should tell the teacher what part of speech that word is.
- In the second version, the teacher says a part of speech. For example, the teacher might say “verb.” The students have to hit the book when they find a verb in the text and then say which word they found. This is a fast-paced activity that will help you to teach parts of speech in a fun way.
- Online teachers can send the text to the students before class via email or messaging. Rather than swatting the paper, students could raise their hand or hold something up to their webcam when they’ve found the right word.
Get ideas for last-minute EFL lesson plans.
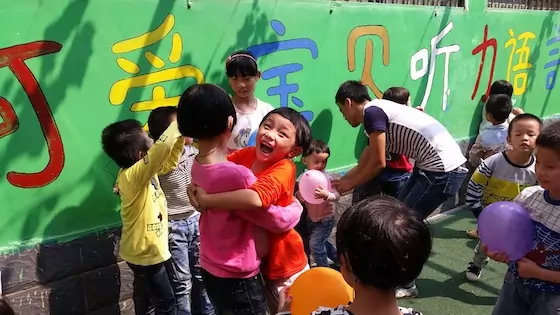
Kindergarten ESL students play a game in China.
5. Guided discovery with vocabulary
Whenever you encounter a new set of vocabulary words in your lesson, use it as an opportunity to reinforce and teach parts of speech. Keep blank pages around the room with the labels for each part of speech you want the students to know, and ask them which parts of speech they think the new words are.
Allow time for the students to be able to explore their new vocabulary together, in pairs or individually. You can set a timer if you want to keep them on task. Students should look at the new vocabulary and attempt to sort them into the parts of speech categories. Then, add the words to the correct lists.
- You can use digital parts of speech lists in the virtual classroom. Keep them up to date via a classroom blog or other platform where you can upload documents or publish lists.
Learn more about teaching English with guided discovery for ESL.
Additional, last-minute activities for teaching parts of speech
Here are some other examples of how you can incorporate parts of speech into any lesson, even when they aren’t the focus or main topic:
- When you play Bingo, have the students shout out the part of speech every time you say a new word.
- Instead of saying, “Rock, paper, scissor!” say “Noun, verb, adjective!” in order to get more practice speaking the words out loud.
- Whenever you play a new song, ask students what parts of speech appear in the title.
- Instead of saying words that you’ve written on the board, use parts of speech. For example, say, “Noun!” Ask a student to come up to the board and touch the noun.
There you have it — how to teach parts of speech in a fun way! Keeping things lively with these activities will help your ESL students to enjoy the lesson while learning this necessary grammar topic.
Get more ideas for teaching grammar topics to ESL students in the Bridge TEFL/TESOL Grammar Advisor Certification course.
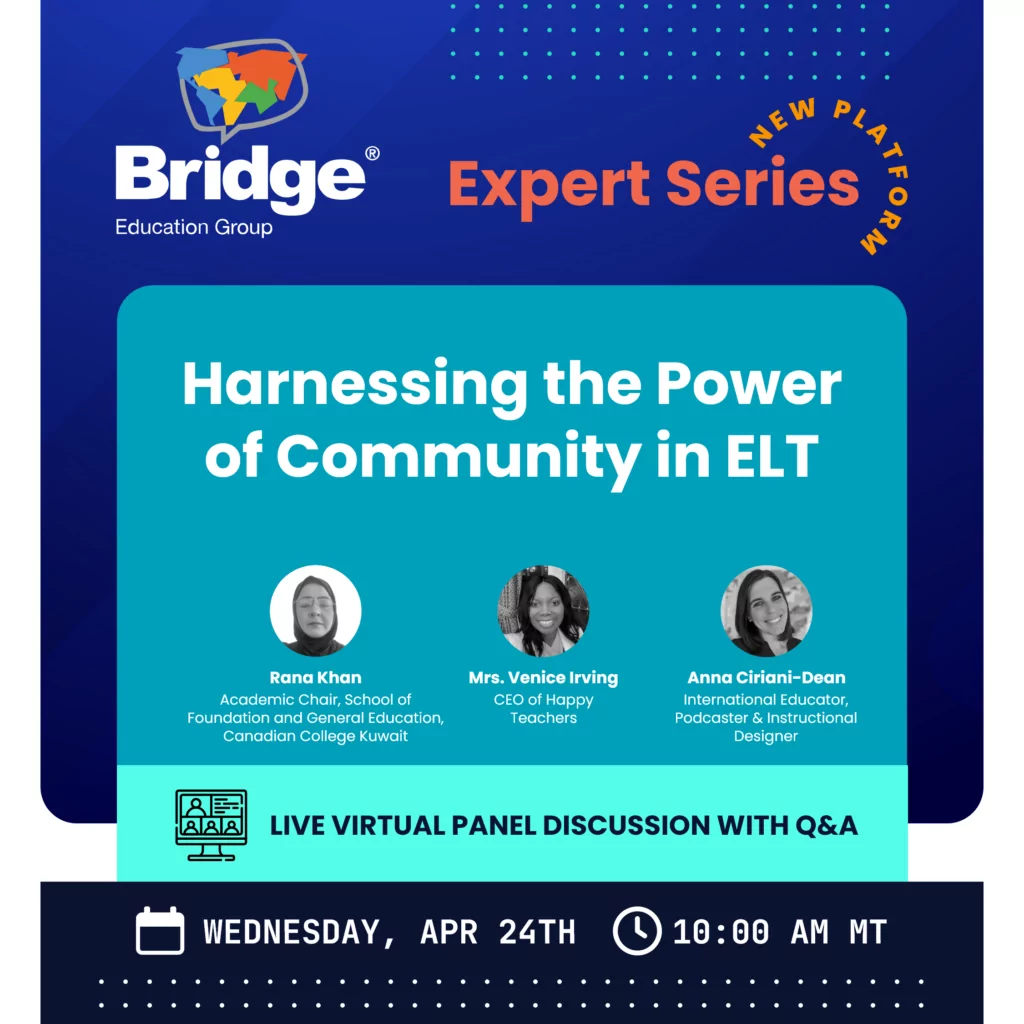
Coleen Monroe is a Colorado native who has left a trail of new homes for herself around the world. She's set foot in 30 countries and lived on four continents in the last eleven years. Her nomad homes have been in Chilean Patagonia, France, Italy, Switzerland, South Korea, England, and Iceland. Her latest travel adventures took her to Yunnan, Beijing, Jiangxi, and Southern China, where she's currently teaching.
- Search Menu
- Browse content in Arts and Humanities
- Browse content in Archaeology
- Anglo-Saxon and Medieval Archaeology
- Archaeological Methodology and Techniques
- Archaeology by Region
- Archaeology of Religion
- Archaeology of Trade and Exchange
- Biblical Archaeology
- Contemporary and Public Archaeology
- Environmental Archaeology
- Historical Archaeology
- History and Theory of Archaeology
- Industrial Archaeology
- Landscape Archaeology
- Mortuary Archaeology
- Prehistoric Archaeology
- Underwater Archaeology
- Urban Archaeology
- Zooarchaeology
- Browse content in Architecture
- Architectural Structure and Design
- History of Architecture
- Residential and Domestic Buildings
- Theory of Architecture
- Browse content in Art
- Art Subjects and Themes
- History of Art
- Industrial and Commercial Art
- Theory of Art
- Biographical Studies
- Byzantine Studies
- Browse content in Classical Studies
- Classical History
- Classical Philosophy
- Classical Mythology
- Classical Literature
- Classical Reception
- Classical Art and Architecture
- Classical Oratory and Rhetoric
- Greek and Roman Papyrology
- Greek and Roman Epigraphy
- Greek and Roman Law
- Greek and Roman Archaeology
- Late Antiquity
- Religion in the Ancient World
- Digital Humanities
- Browse content in History
- Colonialism and Imperialism
- Diplomatic History
- Environmental History
- Genealogy, Heraldry, Names, and Honours
- Genocide and Ethnic Cleansing
- Historical Geography
- History by Period
- History of Emotions
- History of Agriculture
- History of Education
- History of Gender and Sexuality
- Industrial History
- Intellectual History
- International History
- Labour History
- Legal and Constitutional History
- Local and Family History
- Maritime History
- Military History
- National Liberation and Post-Colonialism
- Oral History
- Political History
- Public History
- Regional and National History
- Revolutions and Rebellions
- Slavery and Abolition of Slavery
- Social and Cultural History
- Theory, Methods, and Historiography
- Urban History
- World History
- Browse content in Language Teaching and Learning
- Language Learning (Specific Skills)
- Language Teaching Theory and Methods
- Browse content in Linguistics
- Applied Linguistics
- Cognitive Linguistics
- Computational Linguistics
- Forensic Linguistics
- Grammar, Syntax and Morphology
- Historical and Diachronic Linguistics
- History of English
- Language Evolution
- Language Reference
- Language Acquisition
- Language Variation
- Language Families
- Lexicography
- Linguistic Anthropology
- Linguistic Theories
- Linguistic Typology
- Phonetics and Phonology
- Psycholinguistics
- Sociolinguistics
- Translation and Interpretation
- Writing Systems
- Browse content in Literature
- Bibliography
- Children's Literature Studies
- Literary Studies (Romanticism)
- Literary Studies (American)
- Literary Studies (Asian)
- Literary Studies (European)
- Literary Studies (Eco-criticism)
- Literary Studies (Modernism)
- Literary Studies - World
- Literary Studies (1500 to 1800)
- Literary Studies (19th Century)
- Literary Studies (20th Century onwards)
- Literary Studies (African American Literature)
- Literary Studies (British and Irish)
- Literary Studies (Early and Medieval)
- Literary Studies (Fiction, Novelists, and Prose Writers)
- Literary Studies (Gender Studies)
- Literary Studies (Graphic Novels)
- Literary Studies (History of the Book)
- Literary Studies (Plays and Playwrights)
- Literary Studies (Poetry and Poets)
- Literary Studies (Postcolonial Literature)
- Literary Studies (Queer Studies)
- Literary Studies (Science Fiction)
- Literary Studies (Travel Literature)
- Literary Studies (War Literature)
- Literary Studies (Women's Writing)
- Literary Theory and Cultural Studies
- Mythology and Folklore
- Shakespeare Studies and Criticism
- Browse content in Media Studies
- Browse content in Music
- Applied Music
- Dance and Music
- Ethics in Music
- Ethnomusicology
- Gender and Sexuality in Music
- Medicine and Music
- Music Cultures
- Music and Media
- Music and Religion
- Music and Culture
- Music Education and Pedagogy
- Music Theory and Analysis
- Musical Scores, Lyrics, and Libretti
- Musical Structures, Styles, and Techniques
- Musicology and Music History
- Performance Practice and Studies
- Race and Ethnicity in Music
- Sound Studies
- Browse content in Performing Arts
- Browse content in Philosophy
- Aesthetics and Philosophy of Art
- Epistemology
- Feminist Philosophy
- History of Western Philosophy
- Metaphysics
- Moral Philosophy
- Non-Western Philosophy
- Philosophy of Language
- Philosophy of Mind
- Philosophy of Perception
- Philosophy of Science
- Philosophy of Action
- Philosophy of Law
- Philosophy of Religion
- Philosophy of Mathematics and Logic
- Practical Ethics
- Social and Political Philosophy
- Browse content in Religion
- Biblical Studies
- Christianity
- East Asian Religions
- History of Religion
- Judaism and Jewish Studies
- Qumran Studies
- Religion and Education
- Religion and Health
- Religion and Politics
- Religion and Science
- Religion and Law
- Religion and Art, Literature, and Music
- Religious Studies
- Browse content in Society and Culture
- Cookery, Food, and Drink
- Cultural Studies
- Customs and Traditions
- Ethical Issues and Debates
- Hobbies, Games, Arts and Crafts
- Lifestyle, Home, and Garden
- Natural world, Country Life, and Pets
- Popular Beliefs and Controversial Knowledge
- Sports and Outdoor Recreation
- Technology and Society
- Travel and Holiday
- Visual Culture
- Browse content in Law
- Arbitration
- Browse content in Company and Commercial Law
- Commercial Law
- Company Law
- Browse content in Comparative Law
- Systems of Law
- Competition Law
- Browse content in Constitutional and Administrative Law
- Government Powers
- Judicial Review
- Local Government Law
- Military and Defence Law
- Parliamentary and Legislative Practice
- Construction Law
- Contract Law
- Browse content in Criminal Law
- Criminal Procedure
- Criminal Evidence Law
- Sentencing and Punishment
- Employment and Labour Law
- Environment and Energy Law
- Browse content in Financial Law
- Banking Law
- Insolvency Law
- History of Law
- Human Rights and Immigration
- Intellectual Property Law
- Browse content in International Law
- Private International Law and Conflict of Laws
- Public International Law
- IT and Communications Law
- Jurisprudence and Philosophy of Law
- Law and Politics
- Law and Society
- Browse content in Legal System and Practice
- Courts and Procedure
- Legal Skills and Practice
- Primary Sources of Law
- Regulation of Legal Profession
- Medical and Healthcare Law
- Browse content in Policing
- Criminal Investigation and Detection
- Police and Security Services
- Police Procedure and Law
- Police Regional Planning
- Browse content in Property Law
- Personal Property Law
- Study and Revision
- Terrorism and National Security Law
- Browse content in Trusts Law
- Wills and Probate or Succession
- Browse content in Medicine and Health
- Browse content in Allied Health Professions
- Arts Therapies
- Clinical Science
- Dietetics and Nutrition
- Occupational Therapy
- Operating Department Practice
- Physiotherapy
- Radiography
- Speech and Language Therapy
- Browse content in Anaesthetics
- General Anaesthesia
- Neuroanaesthesia
- Clinical Neuroscience
- Browse content in Clinical Medicine
- Acute Medicine
- Cardiovascular Medicine
- Clinical Genetics
- Clinical Pharmacology and Therapeutics
- Dermatology
- Endocrinology and Diabetes
- Gastroenterology
- Genito-urinary Medicine
- Geriatric Medicine
- Infectious Diseases
- Medical Toxicology
- Medical Oncology
- Pain Medicine
- Palliative Medicine
- Rehabilitation Medicine
- Respiratory Medicine and Pulmonology
- Rheumatology
- Sleep Medicine
- Sports and Exercise Medicine
- Community Medical Services
- Critical Care
- Emergency Medicine
- Forensic Medicine
- Haematology
- History of Medicine
- Browse content in Medical Skills
- Clinical Skills
- Communication Skills
- Nursing Skills
- Surgical Skills
- Browse content in Medical Dentistry
- Oral and Maxillofacial Surgery
- Paediatric Dentistry
- Restorative Dentistry and Orthodontics
- Surgical Dentistry
- Medical Ethics
- Medical Statistics and Methodology
- Browse content in Neurology
- Clinical Neurophysiology
- Neuropathology
- Nursing Studies
- Browse content in Obstetrics and Gynaecology
- Gynaecology
- Occupational Medicine
- Ophthalmology
- Otolaryngology (ENT)
- Browse content in Paediatrics
- Neonatology
- Browse content in Pathology
- Chemical Pathology
- Clinical Cytogenetics and Molecular Genetics
- Histopathology
- Medical Microbiology and Virology
- Patient Education and Information
- Browse content in Pharmacology
- Psychopharmacology
- Browse content in Popular Health
- Caring for Others
- Complementary and Alternative Medicine
- Self-help and Personal Development
- Browse content in Preclinical Medicine
- Cell Biology
- Molecular Biology and Genetics
- Reproduction, Growth and Development
- Primary Care
- Professional Development in Medicine
- Browse content in Psychiatry
- Addiction Medicine
- Child and Adolescent Psychiatry
- Forensic Psychiatry
- Learning Disabilities
- Old Age Psychiatry
- Psychotherapy
- Browse content in Public Health and Epidemiology
- Epidemiology
- Public Health
- Browse content in Radiology
- Clinical Radiology
- Interventional Radiology
- Nuclear Medicine
- Radiation Oncology
- Reproductive Medicine
- Browse content in Surgery
- Cardiothoracic Surgery
- Gastro-intestinal and Colorectal Surgery
- General Surgery
- Neurosurgery
- Paediatric Surgery
- Peri-operative Care
- Plastic and Reconstructive Surgery
- Surgical Oncology
- Transplant Surgery
- Trauma and Orthopaedic Surgery
- Vascular Surgery
- Browse content in Science and Mathematics
- Browse content in Biological Sciences
- Aquatic Biology
- Biochemistry
- Bioinformatics and Computational Biology
- Developmental Biology
- Ecology and Conservation
- Evolutionary Biology
- Genetics and Genomics
- Microbiology
- Molecular and Cell Biology
- Natural History
- Plant Sciences and Forestry
- Research Methods in Life Sciences
- Structural Biology
- Systems Biology
- Zoology and Animal Sciences
- Browse content in Chemistry
- Analytical Chemistry
- Computational Chemistry
- Crystallography
- Environmental Chemistry
- Industrial Chemistry
- Inorganic Chemistry
- Materials Chemistry
- Medicinal Chemistry
- Mineralogy and Gems
- Organic Chemistry
- Physical Chemistry
- Polymer Chemistry
- Study and Communication Skills in Chemistry
- Theoretical Chemistry
- Browse content in Computer Science
- Artificial Intelligence
- Computer Architecture and Logic Design
- Game Studies
- Human-Computer Interaction
- Mathematical Theory of Computation
- Programming Languages
- Software Engineering
- Systems Analysis and Design
- Virtual Reality
- Browse content in Computing
- Business Applications
- Computer Security
- Computer Games
- Computer Networking and Communications
- Digital Lifestyle
- Graphical and Digital Media Applications
- Operating Systems
- Browse content in Earth Sciences and Geography
- Atmospheric Sciences
- Environmental Geography
- Geology and the Lithosphere
- Maps and Map-making
- Meteorology and Climatology
- Oceanography and Hydrology
- Palaeontology
- Physical Geography and Topography
- Regional Geography
- Soil Science
- Urban Geography
- Browse content in Engineering and Technology
- Agriculture and Farming
- Biological Engineering
- Civil Engineering, Surveying, and Building
- Electronics and Communications Engineering
- Energy Technology
- Engineering (General)
- Environmental Science, Engineering, and Technology
- History of Engineering and Technology
- Mechanical Engineering and Materials
- Technology of Industrial Chemistry
- Transport Technology and Trades
- Browse content in Environmental Science
- Applied Ecology (Environmental Science)
- Conservation of the Environment (Environmental Science)
- Environmental Sustainability
- Environmentalist Thought and Ideology (Environmental Science)
- Management of Land and Natural Resources (Environmental Science)
- Natural Disasters (Environmental Science)
- Nuclear Issues (Environmental Science)
- Pollution and Threats to the Environment (Environmental Science)
- Social Impact of Environmental Issues (Environmental Science)
- History of Science and Technology
- Browse content in Materials Science
- Ceramics and Glasses
- Composite Materials
- Metals, Alloying, and Corrosion
- Nanotechnology
- Browse content in Mathematics
- Applied Mathematics
- Biomathematics and Statistics
- History of Mathematics
- Mathematical Education
- Mathematical Finance
- Mathematical Analysis
- Numerical and Computational Mathematics
- Probability and Statistics
- Pure Mathematics
- Browse content in Neuroscience
- Cognition and Behavioural Neuroscience
- Development of the Nervous System
- Disorders of the Nervous System
- History of Neuroscience
- Invertebrate Neurobiology
- Molecular and Cellular Systems
- Neuroendocrinology and Autonomic Nervous System
- Neuroscientific Techniques
- Sensory and Motor Systems
- Browse content in Physics
- Astronomy and Astrophysics
- Atomic, Molecular, and Optical Physics
- Biological and Medical Physics
- Classical Mechanics
- Computational Physics
- Condensed Matter Physics
- Electromagnetism, Optics, and Acoustics
- History of Physics
- Mathematical and Statistical Physics
- Measurement Science
- Nuclear Physics
- Particles and Fields
- Plasma Physics
- Quantum Physics
- Relativity and Gravitation
- Semiconductor and Mesoscopic Physics
- Browse content in Psychology
- Affective Sciences
- Clinical Psychology
- Cognitive Psychology
- Cognitive Neuroscience
- Criminal and Forensic Psychology
- Developmental Psychology
- Educational Psychology
- Evolutionary Psychology
- Health Psychology
- History and Systems in Psychology
- Music Psychology
- Neuropsychology
- Organizational Psychology
- Psychological Assessment and Testing
- Psychology of Human-Technology Interaction
- Psychology Professional Development and Training
- Research Methods in Psychology
- Social Psychology
- Browse content in Social Sciences
- Browse content in Anthropology
- Anthropology of Religion
- Human Evolution
- Medical Anthropology
- Physical Anthropology
- Regional Anthropology
- Social and Cultural Anthropology
- Theory and Practice of Anthropology
- Browse content in Business and Management
- Business Ethics
- Business Strategy
- Business History
- Business and Technology
- Business and Government
- Business and the Environment
- Comparative Management
- Corporate Governance
- Corporate Social Responsibility
- Entrepreneurship
- Health Management
- Human Resource Management
- Industrial and Employment Relations
- Industry Studies
- Information and Communication Technologies
- International Business
- Knowledge Management
- Management and Management Techniques
- Operations Management
- Organizational Theory and Behaviour
- Pensions and Pension Management
- Public and Nonprofit Management
- Strategic Management
- Supply Chain Management
- Browse content in Criminology and Criminal Justice
- Criminal Justice
- Criminology
- Forms of Crime
- International and Comparative Criminology
- Youth Violence and Juvenile Justice
- Development Studies
- Browse content in Economics
- Agricultural, Environmental, and Natural Resource Economics
- Asian Economics
- Behavioural Finance
- Behavioural Economics and Neuroeconomics
- Econometrics and Mathematical Economics
- Economic History
- Economic Systems
- Economic Methodology
- Economic Development and Growth
- Financial Markets
- Financial Institutions and Services
- General Economics and Teaching
- Health, Education, and Welfare
- History of Economic Thought
- International Economics
- Labour and Demographic Economics
- Law and Economics
- Macroeconomics and Monetary Economics
- Microeconomics
- Public Economics
- Urban, Rural, and Regional Economics
- Welfare Economics
- Browse content in Education
- Adult Education and Continuous Learning
- Care and Counselling of Students
- Early Childhood and Elementary Education
- Educational Equipment and Technology
- Educational Strategies and Policy
- Higher and Further Education
- Organization and Management of Education
- Philosophy and Theory of Education
- Schools Studies
- Secondary Education
- Teaching of a Specific Subject
- Teaching of Specific Groups and Special Educational Needs
- Teaching Skills and Techniques
- Browse content in Environment
- Applied Ecology (Social Science)
- Climate Change
- Conservation of the Environment (Social Science)
- Environmentalist Thought and Ideology (Social Science)
- Natural Disasters (Environment)
- Social Impact of Environmental Issues (Social Science)
- Browse content in Human Geography
- Cultural Geography
- Economic Geography
- Political Geography
- Browse content in Interdisciplinary Studies
- Communication Studies
- Museums, Libraries, and Information Sciences
- Browse content in Politics
- African Politics
- Asian Politics
- Chinese Politics
- Comparative Politics
- Conflict Politics
- Elections and Electoral Studies
- Environmental Politics
- European Union
- Foreign Policy
- Gender and Politics
- Human Rights and Politics
- Indian Politics
- International Relations
- International Organization (Politics)
- International Political Economy
- Irish Politics
- Latin American Politics
- Middle Eastern Politics
- Political Behaviour
- Political Economy
- Political Institutions
- Political Methodology
- Political Communication
- Political Philosophy
- Political Sociology
- Political Theory
- Politics and Law
- Public Policy
- Public Administration
- Quantitative Political Methodology
- Regional Political Studies
- Russian Politics
- Security Studies
- State and Local Government
- UK Politics
- US Politics
- Browse content in Regional and Area Studies
- African Studies
- Asian Studies
- East Asian Studies
- Japanese Studies
- Latin American Studies
- Middle Eastern Studies
- Native American Studies
- Scottish Studies
- Browse content in Research and Information
- Research Methods
- Browse content in Social Work
- Addictions and Substance Misuse
- Adoption and Fostering
- Care of the Elderly
- Child and Adolescent Social Work
- Couple and Family Social Work
- Developmental and Physical Disabilities Social Work
- Direct Practice and Clinical Social Work
- Emergency Services
- Human Behaviour and the Social Environment
- International and Global Issues in Social Work
- Mental and Behavioural Health
- Social Justice and Human Rights
- Social Policy and Advocacy
- Social Work and Crime and Justice
- Social Work Macro Practice
- Social Work Practice Settings
- Social Work Research and Evidence-based Practice
- Welfare and Benefit Systems
- Browse content in Sociology
- Childhood Studies
- Community Development
- Comparative and Historical Sociology
- Economic Sociology
- Gender and Sexuality
- Gerontology and Ageing
- Health, Illness, and Medicine
- Marriage and the Family
- Migration Studies
- Occupations, Professions, and Work
- Organizations
- Population and Demography
- Race and Ethnicity
- Social Theory
- Social Movements and Social Change
- Social Research and Statistics
- Social Stratification, Inequality, and Mobility
- Sociology of Religion
- Sociology of Education
- Sport and Leisure
- Urban and Rural Studies
- Browse content in Warfare and Defence
- Defence Strategy, Planning, and Research
- Land Forces and Warfare
- Military Administration
- Military Life and Institutions
- Naval Forces and Warfare
- Other Warfare and Defence Issues
- Peace Studies and Conflict Resolution
- Weapons and Equipment

- < Previous chapter
- Next chapter >

24 Part-of-Speech Tagging
Dan Tufiș is Professor of Computational Linguistics and Director of the Institute of Artificial Intelligence in Bucharest (since 2002). He graduated from the faculty of Computer Science of the ‘Politehnica’ University of Bucharest in 1979, obtaining a PhD from the same university in 1992. His contributions in NLP (paradigmatic morphology, POS tagging, WSD, QA, MT, word alignment, large mono- and multilingual corpora and dictionaries, wordnet, etc.) have been published in more than 300 scientific papers.
Radu Ion is a Senior Researcher at the Research Institute for Artificial Intelligence in Bucharest. He graduated from the Faculty of Computer Science at the Politehnica University of Bucharest in 2001, and received his PhD from the Romanian Academy in 2007. Among his research interests are ML for NLP, NLU, MT, and CL problems such as WSD and dependency parsing. He has co-authored 76 publications in peer-reviewed conferences and journals.
- Published: 05 October 2017
- Cite Icon Cite
- Permissions Icon Permissions
One of the fundamental tasks in natural-language processing is the morpho-lexical disambiguation of words occurring in text. Over the last twenty years or so, approaches to part-of-speech tagging based on machine learning techniques have been developed or ported to provide high-accuracy morpho-lexical annotation for an increasing number of languages. Due to recent increases in computing power, together with improvements in tagging technology and the extension of language typologies, part-of-speech tags have become significantly more complex. The need to address multilinguality more directly in the web environment has created a demand for interoperable, harmonized morpho-lexical descriptions across languages. Given the large number of morpho-lexical descriptors for a morphologically complex language, one has to consider ways to avoid the data sparseness threat in standard statistical tagging, yet ensure that full lexicon information is available for each word form in the output. The chapter overviews the current major approaches to part-of-speech tagging.
24.1 Introduction
Lexical ambiguity resolution is a procedure by which a computer program reads an arbitrary text, segments it into tokens, and attaches to each token the information characterizing the lexical and contextual properties of the respective word. 1 This information can be explicitly specified or encoded in a more compact way by a uniquely interpretable label. Such a description is called a part-of-speech tag (or POS tag for short). The set of all possible tags is called the tagset of the lexical ambiguity resolution process. For example, the sentence ‘We can can a can.’ might have a labelling such as shown in Table 24.1 .
We use here the notion of token to refer to a string of characters from a text that a word identification program would return as a single processing unit. Typically, each string of non-blank characters constitutes a token, but in some cases, a sequence such as New York or back and forth might be more appropriately processed as a single token. In other cases, words like damelo (‘give it to me’ in Italian) might be split into several tokens in order to distinguish the relevant pieces of the string for adequate syntactic processing. The procedure that figures out what the tokens are in the input is called a tokenizer . The complexity of this process varies depending on the language family, and tokenization is a research topic in itself for many languages (e.g. for Asian languages which do not use white spaces between words, agglutinative languages, or even for compound productive languages). We do not address the problem of tokenization in this chapter, but the reader should be aware that tokenizing a sentence might not merely involve white-space identification and a multiword dictionary lookup.
Lexical ambiguity resolution is applied to the tokenized input, in order to assign the appropriate POS tag to each token. The POS assignment is achieved by a program called a POS tagger . While some tokens—such as ‘we’ and ‘a’ in the example above—are easier to interpret, other tokens, such as the token ‘can’ in the example, may be harder to disambiguate. An ambiguous lexical item is one that may be classified differently depending on its context. The set of all possible tags/descriptions a lexical token may receive is called the (lexical) ambiguity class (AC) of that token. In general, many tokens may share the same ambiguity class. It is intuitive that not all the tags in the ambiguity class of a word 2 are equally probable, and to a large extent, the surrounding tags reduce the interpretation possibilities for the current token or even fully disambiguate it. Information about the ambiguity class of each lexical token, the probabilities of the tags in an ambiguity class, as well as the interdependencies between tokens’ tags are knowledge sources for the tagger’s decision-making. The majority of the available POS taggers have this a priori knowledge constructed (at least partially) in a statistical manner. For the sake of uniformity, let us call a language model (LM) all the a priori information a tagger needs for its job. The construction of a language model may be achieved manually by human experts who write dictionary entries, and grammar rules to decide the right interpretation in context for ambiguous lexical items. Another option is the data-driven one, where a specialized part of the tagger (called the learner ) learns the language model from the training data .
These tags are compliant with the Multext-East morpho-lexical specifications ( Erjavec 2010 )
Depending on the way the language model is created/learned, one distinguishes two major approaches: supervised versus unsupervised methods. As usually happens with dichotomized distinctions, there are also mixture approaches, sometimes called semi-supervised , partially supervised , or hybrid methods. The supervised learners construct the LMs from annotated corpora (sometimes supplemented by human-made lexicons) while the unsupervised learners rely only on raw corpora with or without a lexicon that specifies the ambiguity classes for the lexical stock (some approaches use only a few frequent lexical entries as learning seeds). Although for most national languages annotated corpora do exist, this is definitely not the case for many other languages or dialects of interest for researchers and even commercial developers. Because annotated corpora are expensive to build, the interest in unsupervised learning for POS tagging has increased significantly. For an interesting account on unsupervised learning research for POS tagging, see Christodoulopoulos et al. (2010) .
In general, data-driven LMs are unreadable for humans and they are represented in a codified manner, interpretable and used by computer programs according to a specific data model. The handmade LMs, more often than not expressed as IF-THEN-ELSE rules, are human-readable, and thus the language experts can interpret and modify/extend them. We will review one of the rule-based taggers: Brill’s transformation-based POS tagger, known for its high accuracy.
Among the data-driven models for POS tagging, in this chapter we will discuss only N-gram models with Hidden Markov Models (HMM; see Chapter 11 for more details) as the classical representative of the data-driven approach; Maximum Entropy models (ME; see Chapter 11 ) with the more recent Conditional Random Fields models (CRF) and the Bidirectional Long Short-Term Memory deep neural network with a CRF layer (BI-LSTM-CRF) as representatives of the state of the art in English POS tagging; 3 and Transformation-Based Error-Driven models as a hybrid rule-based approach.
There are several other types of tagging models, most of them data-driven, but due to space limitation they are not dealt with here. However, for the interested reader we provide some reference points: decision trees ( Black et al. 1992 ; see also Chapter 13 of this volume and Schmid 1994 ); other approaches using neural networks ( Collins 2002 ; Boroș et al. 2013 ; Zheng et al. 2013 ; dos Santos and Zadrozny 2014 ; Akbik et al. 2018 ); Bayesian Nets ( Maragoudakis et al. 2003 ); Case-Based ( Daelemans et al. 1996 ), Inductive Logic Programming ( Cussens 1997 ); and Support Vector Machines ( Herbst and Joachims 2007 ), etc.
The chapter is organized as follows: first, we will discuss the basic model, the N-grams (section 24.2 ). Then, we will address a general problem for any data-driven model, namely data sparseness and various ways to mitigate its consequences (section 24.3 ). Section 24.4 introduces the generative/discriminative dichotomy for statistical tagging models. HMM generative models are discussed in section 24.4.1 while ME, CRF, and BI-LSTM-CRF discriminative models are reviewed in section 24.4.2 . Rule-based tagging is briefly covered in section 24.4.3 with two models: a pure grammar approach and a hybrid one. The last section gives several web addresses for downloadable taggers or tagging web services.
24.2 N-gram Models
The tagging problem is defined as the assignment to each word in an input sequence w 1 , w 2 , …, w k a unique label representing the appropriate part-of-speech interpretation, thus getting the output w 1 /t 1 , w 2 /t 2 , …, w k /t k . As the sequence of words and their associated tags is arbitrary, it is convenient to describe the process in statistical terms: let us consider a text of k words as a sequence of random variables X 1 , X 2 , …, X k , each of these variables taking arbitrary values from a set V called the lexicon. In a similar way, we consider the sequence of tags attached to the k words of a text as a sequence of k variables Y 1 , Y 2 , …, Y k , each of them taking values from the tagset T. Our linguistic intuition says that once a variable X i has been instantiated by a specific word w i , the value of the associated tag t i is restricted to one of its ambiguity class. That is t i ∈AC(w i ) = {t i1 , t i2 , …, t im }. However, not all of them are equally likely. We might write this by using different probabilities of the assignment: p(w i |t i1 ), p(w i |t i2 ) … p(w i |t im ); for our previous example t i (can) ϵ AC(can) = {Voip, Vmn, Ncns} and we have the probabilities p(can|Voip), p(can|Vmn), and p(can|Ncns). Such probabilities can be easily estimated from an annotated corpus by counting how many times the tag t ik has been assigned to the word w i out of the total number of occurrences of the tag t ik :
Going one step further, linguistic intuition says that deciding on the appropriate tag from the ambiguity class of a given word depends on its context. Let us call the context of the tag t i , C(t i ) = <t i-1 , t i-2 , …, t 1 >, the sequence of tags assigned to the words preceding the word w i . At this point, we have two major intuitions to accommodate: the tag of a given word depends on the word itself and on the tags of its context . Assuming that the context dependency of a tag is restricted to a number of n-1 preceding tags, one can make the following estimations:
However, this approach is impractical because of the lack of tagged data to cover the huge number of possible contexts (T N ). Most N-gram models, for tractability reasons, limit the contexts by considering only one or two preceding words/tags:
Assuming that the probabilities p ˜ ( w i | t i ) and p ˜ ( t i | t i − 1 ) are reliably estimated, one may solve the problem of assigning an input string w 1 , w 2 , …, w n the part-of-speech annotation w 1 /t 1 , w 2 /t 2 , …, w n /t n . What is desired is that this annotation should be the most linguistically appropriate, i.e. the tag’s assignment should have the highest probability among a huge number of annotations:
Finding the most likely tag’s assignment for the input string is an optimization problem and it can be done in different ways, such as using a sliding window of k≤N words and choosing the best assignment in the window ( Tufiș and Mason 1998 ), using (binary) decision trees ( Schmid 1994 ), or by dynamic programming ( Church 1989 ). The sliding-window approach is very simple and very fast, but might not find the global (sentence-level) optimal solution. The decision-tree-based search is more flexible (allows for variable length of contexts, including negative restrictions), but might also get stuck into a local maximum. Dynamic programming, with its famous Viterbi algorithm ( Viterbi 1967 ; see Chapter 11 ), relies on fixed length contexts but is guaranteed to find the global optimal solution ( assuming that the conditional independences hold and that all conditional probabilities are reliably estimated).
24.3 Data Sparseness
This is an unavoidable problem for any model that makes probability estimations based on corpus counting. Equation (24.4) shows a product of probability estimates which would be zero in case the input string contains one or more words or tag sequences unseen in the training corpus. This would render such an input string impossible (p = 0) which certainly is wrong. On the other hand, to be reliable, the estimations should be made based on a minimal number of observations, empirically say five or ten. Yet this is practically impossible, because whatever large training corpus one reasonably assumes, according to Zipf Rank-Frequency Law there will always exist a long tail of rare words. Avoiding the ‘ data sparseness curse ’ in POS tagging requires finding a way to associate whatever unknown word from an input string with the most likely tags and their probability distribution and using the knowledge acquired from the training corpus to estimate probabilities for tag bigrams/trigrams not seen during the training.
A naive method of treating out-of-vocabulary words (OOV—words not seen during the training phase) is to automatically associate as an ambiguity class the list of tags for open-class categories (nouns, verbs, adjectives, adverbs) 4 and their probabilities uniformly distributed. A better approach is to do a morphological analysis of the OOVs or a simpler ‘prefix’ or ‘suffix’ investigation. Here ‘ prefix ’ and ‘ suffix ’ are used in a loose way, namely as a string of k letters that start or end an input word. For instance, capitalization of a sentence-internal word might be a strong clue that it is a noun (in German) or a proper noun (in most languages). In languages with significant inflectional morphology the suffixes carry a lot of information that might help in inducing a reasonably reduced ambiguity class. Tag probabilities are assigned according to the frequency of the endings observed in the training corpus. For instance, words in the Wall Street Journal part of the Penn Treebank ending in able are adjectives in 98% of cases ( fashionable, variable ), with the remaining 2% being nouns ( cable, variable ) ( Brants 2000 ). The probability distribution for a particular suffix may be generated from the words in the training corpus that share the same suffix. A usual assumption is that the distribution of unseen words is similar to the distribution of rare words, and consequently, from the words in the training corpus that share the same suffix, to consider only those that have a small frequency is a good heuristic ( Weischedel et al. 1993 ). Thorsten Brants implemented these heuristics in his well-known TnT tagger and the reported accuracy for tagging OOVs is 89% ( Brants 2000 ). A maximum likelihood estimation (see also Chapter 11 ) of a tag t given a suffix of length i c i c i-1 … c 1 is derived from the corpus by the relation:
where count th (α k c i c i-1 … c 1 /t) is the number of words ending in c i c i-1 … c 1 which were tagged by t and their frequency is above the threshold th; count th (α k c i c i-1 … c 1 ) is the total number of words ending in c i c i-1 … c 1 with frequency above the threshold th . These probabilities are smoothed by successive substrings of the suffix according to the recursive formula:
The weights θ i may be taken independently of context and set to sample variance ( s N-1 ) of the unconditional maximum likelihood probabilities of the tags from the training corpus:
The length of the ‘prefix’ was empirically set to ten occurrences. The procedure described above can be further refined in several ways. One possibility ( Ion 2007 ) is to consider not all substrings c i c i-1 … c 1, but only those substrings that are real linguistic suffixes. This way, the set of possible tags for an OOV will be significantly reduced and the probability estimations will be more robust. Having a list of linguistic suffixes would also eliminate the arbitrary length of the longest ‘suffix’ (in TnT this is ten characters), resulting in speeding up the process.
The second problem in dealing with the data sparseness is related to estimating probabilities for tag n-grams t i t i-1 … t i-n + 1 unseen in the training corpus. This problem is more general and its solution is known as smoothing . We already saw a particular type of smoothing above for processing OOVs. The basic idea is to adjust the maximum likelihood estimate of probabilities by modifying (increasing or decreasing) the actual counts for the n-grams seen in the training corpus and assigning the probability mass gained this way to unobserved n-grams. An excellent study of the smoothing techniques for language modelling is Chen and Goodman (1998) . They discuss several methods among which are the following: additive smoothing, Good–Turing Estimate, Church–Gale smoothing, Jelinek–Mercer linear interpolation (also Witten–Bell smoothing and absolute discounting as instantiations of the Jelinek–Mercer method), Katz backoff , and Kneser–Ney smoothing . The Kneser–Ney method is generally acknowledged as the best-performing smoothing technique. These methods are reviewed in more detail in Tufiș (2016) .
24.4 Generative versus Discriminative Tagging Models
The model with its parameters established would allow for finding the most likely tag sequence to a new sequence of input words. In machine learning, one distinguishes between generative and discriminative models ( Sutton and McCallum 2006 ).
A generative model is based on a model of the joint distribution { P θ ( W , T ) : θ ∈ Θ } . The best-known generative model for POS tagging is the Hidden Markov Model.
A discriminative model is based on a model of the conditional distribution { P θ ( W | T ) : θ ∈ Θ } . The discriminative models are also called conditional models (Maximum Entropy and Conditional Random Fields among others).
The parameters θ are estimated by specialized methods from the training data. For tractability reasons, both generative and discriminative models make simplifying assumptions on the T sequences, but only the generative models need to make additional assumptions on W sequences. This is the main difference between the two types of model and because of this, from a theoretical point of view, the discriminative models are more powerful, although, as noticed by other researchers ( Johnson 2001 ; Toutanova 2006 ), the improvements may not be statistically significant for the practical tagging task. Additionally, training the discriminative models is more computationally intensive.
24.4.1 Hidden Markov Models
Hidden Markov Models (HMM) is a generative n-gram model that allows treating the tagging of a sequence of words W (the observables) as the problem of finding the most probable (the best explanation for the observables) path traversal S (from an initial state to a final state) of a finite-state system. The system’s states are not directly observable. In terms of the notions introduced in section 24.2 , we define a first-order Hidden Markov Model (the definition is easily generalized to n-order HMMs) as a probabilistic finite-state formalism characterized by a tuple:
HMM = λ T ( π, A, B) T = finite set of states (a state corresponds to a tag of the underlying tagset) π = initial state probabilities (this is the set of probabilities of the tags to be assigned to the first word of sentences; to make sense of the probabilities p(t 1 |t o ) the sentences are headed by a dummy word, always tagged BOS ( Begin of Sentence ) so that p(t 1 |t o ) = p(t 1 |BOS) = π(t 1 )). A = transition matrix (encoding the probabilities a ij to move from s i to s j , that is, the probability p(t j |t i ) that given the tag t i of the previous word, the current word is tagged with t j ). B = emission matrix (encoding the lexical probabilities p(w i |t i ), that is, the probability that being in the state s i —tagged t i —observes the word w i ).
24.4.1.1 HMM parameters
The parameter estimation/learning of an HMM designed for POS tagging depends on the type of available resources, along the dichotomy supervised vs unsupervised training.
24.4.1.2 Supervised training
For supervised training, a POS-corpus annotated as accurately as possible is the prerequisite resource. The minimal size of the training corpus is a very difficult question to answer because there are several factors to be taken into account: the language, the tagset size, the minimal number of occurrences of a word/tag association, etc.
The set of all tags seen in the training corpus will make the tagset, based on which T parameter of the HMM is defined. All the other parameters are set by most likelihood estimations (MLE):
π = { p ˜ ( t i | B O S ) } ; p ˜ ( t i | B O S ) = c o u n t ( B O S , t i ) c o u n t ( B O S ) that is, we count what fraction of the number of sentences have their proper first word tagged with t i .
A = { p ˜ ( t j | t i ) } ; p ˜ ( t j | t i ) = c o u n t ( t i , t j ) c o u n t ( t i ) that is, we count what fraction of the number of words tagged with t j were preceded by words tagged with t i .
B = { p ˜ ( w i | t i ) } ; p ˜ ( w i | t i ) = c o u n t ( w i , t i ) c o u n t ( t i ) that is, we count what fraction of the number of words tagged with t i were w i .
It is interesting to note that knowing only the B parameter (that is a lexicon with attached probabilities for various tags of a word), then systematically choosing the highest probability tag, would ensure more than 92% and even higher accuracy for POS disambiguating texts in most of the languages. For instance, by assigning each word its highest probability tag, Lee et al. (2010) report a tagging accuracy as high as 94.6% 5 on the WSJ portion of the Penn Treebank.
24.4.1.3 Inference to the best tagging solution
Once the parameters of the HMM have been determined (either by supervised or unsupervised training) one can proceed to solve the proper tagging problem: finding the most likely hidden transition path s 1 , s 2 , …, s N (corresponding to the sequence of tags t 1 , t 2 , …, t N ) that generated the input string w 1 , w 2 , …, w N . A simple-minded solution would be to try all the possible paths (in the range of T N ) and on each state path compute p(w j |t i )*p(t i |t i-1 ). This way, we would need O(2N*T N ) computations. This number is enormous: to get a feeling, consider a usual tagset T of 100 tags and a modest-sized corpus to be tagged, containing 1,000 sentences each made up on average of 20 words. The upper limit of the number of computations for tagging this corpus would be 1,000*2*20*100 20 = 4*10 44 . Fortunately, there are many ways to make the computation tractable.
The best-known algorithm for solving this problem is Viterbi’s, which has been shown to find the optimal sequence of hidden states by doing at most N*T 2 computations (see Chapter 11 for mathematical details). To take the previous example, Viterbi’s algorithm would need no more than 2*10 8 computations, that is, 2*10 36 fewer! The algorithm looks at each state j at time t which could emit the word w t (that is, for which b j (w t ) is non-null), and for all the transitions that lead into that state, it decides which of them, say i , was the most likely to occur, i.e. the transition with the greatest accumulated score γ t-1 (i). The state i from which originated the transition with the highest accumulated score is stored as a back pointer to the current state j and it is assigned the accumulated score γ t (j) = b j (w t )*γ t−1 (i). When the algorithm reaches the end of the sentence (time t = N), it determines the final state as before and computes the Viterbi path by following the back pointers (tracking back the optimal path). The probability of this path is the accumulated score of the final state.
24.4.2 Discriminative Models
This is a class of models that were defined for solving problems where the two fundamental hypotheses used by the generative models are too weak or grossly inapplicable. The conditional models also consider more realistically the inherent lack of full data sets required to build a robust and wide-coverage statistical model. The joint probability distributions are replaced with conditional probability distributions where conditioning is restricted to available data and they are enabled to consider not only the identity of observables (word forms) but many other relevant properties they may have, such as prefixes, suffixes, embedded hyphens, starting with an uppercase letter, etc. Dependencies among non-adjacent input words can also be taken into account.
Among the conditional models, the most successful for POS tagging are Maximum Entropy (ME) models (including Maximum Entropy Markov models) and Conditional Random Fields (CRF) models (with different variants Linear-Chain CRF and Skip-Chain CRF ).
24.4.2.1 Maximum Entropy models
Maximum Entropy language modelling was first used by Della Pietra et al. (1992) . Maximum Entropy (ME) based taggers are among the best-performing since they take into account ‘diverse forms of contextual information in a principled manner, and do not impose any distributional assumptions on the training data’ ( Ratnaparkhi 1996 ). If one denoted by H the set of contextual hints (or histories ) for predicting tags and by T the set of possible tags, then one defines the event space as E ⊆ H ⊗ T. The common way to represent contextual information is by means of binary valued features (constraint functions) f i on event space f i : E→{0,1}, subject to a set of restrictions. The model requires that the expected value of each feature f i according to the model p should match the observed value 6 and, moreover, it should be a constant:
Computing this expected value according to p requires summing over all events (h, t) which is not practically possible. The standard approximation ( Curran and Clark 2003 ; Rosenfeld 1996 ) is to use the observed relative frequencies of the events p ˜ ( h , t ) with the simplification that this is zero for unseen events: ∑ { ( h , t ) } p ˜ ( h ) p ( t | h ) f i ( h , t ) .
It may be shown (see Ratnaparkhi 1996 ) that the probability of tag t given the context h for an ME probability distribution has the form:
where π ( h ) = 1 Σ t Π i = 1 k α i f i ( h , t ) is a normalization constant, { α 1 , …, α k } are the positive model parameters, and {f 1 , …, f k } are the binary features used to contextually predict the tag t . The history h i is defined as follows: h i = { w i , w i+1 , w i+2 , w i-1 , w i-2 , t i-1 , t i-2 } with w i the current word for which the tag is to be predicted, w i-1 , w i-2 , t i-1 , and t i-2 the preceding two words and their respective predicted tags and w i+1 , w i+2 the following two words. For a given history h and a considered tag t for the current word w i , a feature f i (h, t) may refer to any word or tag in the history and should encode information that might help predict t . Example features taken from Ratnaparkhi (1996) look like:
The first example feature says that if the current word w i ends with the ‘ ing ’ string of letters, the probability that the current word is a Verb Gerund is enhanced by this feature (more precisely, the probability p(t i |h i ) is contributed by α j —see equation (24.9) ).
The second exemplified feature says that if the current word w i is ‘about’ and the preceding words were tagged as Determiner (DT) and Plural Noun (NNS), the predicted tag Preposition (IN) is enhanced by this feature.
24.4.2.2 ME parameters
The parameters { α 1 , …, α k } act as weights for each active feature which together contribute to predicting a tag t i for a word w i . They are chosen to maximize the likelihood of the training data (a tagged corpus of N words):
The algorithm for finding the parameters of the distribution that uniquely maximizes the likelihood L(p) over distributions of the form shown in equation (24.9) that satisfy the constraints specified by the features is called Generalized Iterative Scaling (GIS; Darroch and Ratcliff 1972 ).
24.4.2.3 Inference to the best tagging solution
This is basically a beam search algorithm (the beam size is a parameter; the larger the beam, the longer the tagging time is; Ratnaparkhi 1996 recommends the value of 5). The algorithm finds the MLE tag sequence t 1 … t N for the input string:
For a detailed presentation of the algorithm, see Ratnaparkhi (1996) .
There are various available ME taggers with tagging accuracy ranging between 97% and 98% (MaxEnt, Stanford tagger, NLTK tagger to name just a few). The popularity of the ME taggers is due to the wide range of context dependencies that may be encoded via the features. Nevertheless, as has already been noticed by other researchers ( Rosenfeld 1996 ), selection of the features is very important and this is a matter of human expertise 7 (the same training data but different features would more often than not lead to different performances). On top of this, most of the best discriminating features are language-dependent. While the GIS algorithm is guaranteed to converge (provided the constraints are consistent) there is no theoretical bound on the number of iterations required and, correlated with the intensive computation, one may decide to stop the iterations before reaching the optimal solution.
24.4.2.4 Conditional Random Field model
The Conditional Random Field (CRF) model was introduced in Lafferty et al. (2001) . It is a very general discriminative model that uses the exponential conditional distribution, similar to the Maximum Entropy model. As in ME models, binary features are used as triggers for contextual information that might support a prediction.
The generalization consists of giving access to the entire sequence of observables to any feature, so that it can be activated to support prediction t i for the observable w i , taking into account whatever attribute is needed from the sequence w 1 , w 2 , …, w N . The tagging problem has been addressed with a particular form of the model, called Linear Chain Conditional Random Field, which combines the advantages of discriminative modelling (feature-based) and sequence modelling (order-based). The major motivation for CRF was to deal with one weakness of discriminative models based on finite states, namely the label bias problem . This problem is related to the computing of transition probabilities which, ignoring the observables, are biased towards states which have fewer outgoing transitions. Unlike previous non-generative finite-state models, which use per state exponential models for the conditional probabilities of next state given the current state, a CRF has a single exponential model for the probability of the entire sequence of states given the observation sequence ( Lafferty et al. 2001 ). CRF uses a normalization function which is not a constant but a function of the observed input string.
The Skip-Chain CRF is a Linear Chain CRF with additional long-distance edges ( skip edges ) between related words. The features on skip edges may incorporate information from the context of both endpoints, so that strong evidence at one endpoint can influence the label at the other endpoint ( Lafferty et al. 2001 ). The relatedness of the words connected by a skip edge may be judged based on orthography similarity (e.g. Levenshtein distance) or semantic similarity (e.g. WordNet-based synonymy or hyponymy).
The procedure for the estimation of CRF model parameters is an improved version of the GIS, due to Della Pietra et al. (1997) . The inference procedure is a modified version of the Viterbi algorithm (see Lafferty et al. 2001 for details).
24.4.2.5 Bidirectional Long Short-Term Memory Deep Neural Network with a CRF layer
These models successfully fuse CRF modelling with deep neural network learning of sequence tagging. Specifically, the Bidirectional Long Short-Term Memory Deep Neural Network with a CRF layer on the output (BI-LSTM-CRF; Huang et al. 2015 ) is a neural network that is able to learn the POS tag of a given word w i in a sequence W using features from the left and right contexts of the word as well as sentence-wide features.
An LSTM neural network is a kind of recursive neural network (RNN). We will introduce the RNN for POS tagging first and then provide the differentiating factor between an LSTM and an RNN.
An RNN is a neural network with one hidden layer h which is connected to itself through a feedback loop. It is called a ‘loop’ because the output of the neural network at time step i (e.g. the output for the word at index i in the sequence W ) depends on the output of the hidden layer at time step i which, in turn, is computed using the output of the same hidden layer at the previous time point, i − 1. Thus, when the RNN is unfolded through time, the ‘loop’ is actually a chain of dependencies for the hidden layer through time (see Figure 24.1 in which the word ‘We’ is at time step i = 1, word ‘can’ is at time step i = 2, etc.). Mathematically, the hidden layer h at time step i is given by h ( i ) = f ( U x ( i ) ) + Z h ( i − 1 ) and the output layer y at time step i is given by y ( i ) = g ( V h ( i ) ) where U, Z , and V are the weight matrices linking the input vector x to the hidden layer vector h , the previous hidden layer h to the current hidden layer h , and the current hidden layer h to the output vector y respectively ( f is the sigmoid function and g is the softmax function). y is a proper probability distribution over the tagset for the network in Figure 24.1 and the input vector x is a feature vector of the input word at a certain point in time. Usually, it is a one-hot vector encoding of the word in its context, e.g. place 1 on the corresponding position in the 0-initialized vector if the word and its POS label are in the vocabulary. The reader should note that there are many other ways in which x can be encoded (e.g. one may reserve bits for specifying if the word is upper case, if it is a proper name, if it ends with a specified suffix, etc.) and the performance of the POS tagger largely depends on it.
An RNN for POS tagging
In an RNN, the choice of the POS tag at time step i is only supported by evidence in the left context of the word, through the time dependency of the hidden layer h . It would be very helpful if we could use the evidence in the right context of the word, i.e. from the ‘future’ hidden layer h at time step i + 1. To achieve this, we could enforce the feedback mechanism on the reverse of the input sequence W , or equivalently, reading the sequence from right to left. We introduce a new hidden layer k in the RNN, thus obtaining a bidirectional RNN or BI-RNN (see Figure 24.2 ). The computation of the new hidden layer k at time step i is similar to the computation of hidden layer h , only using the dependency from the future: k ( i ) = f ( U x ( i ) + Y h ( i + 1 ) ) and the output layer y now depends on both h and k : y ( i ) = g ( V L R h ( i ) + V R L k ( i ) ) where V L R and V R L are the left-to-right and right-to-left weight matrices, linking the hidden layers h and k to the output layer y . In practice, we need to pre-compute the values of the hidden layers h and k , reading the sequence left to right and right to left, before computing the values of the output layer.
A BI-RNN computes the probability of a POS tag sequence T , t 1 , … , t N , given the word sequence W , w 1 , … , w N as P ( T | W ) = Π i = 1 N y ( t i ) where y ( t i ) is the learnt probability distribution of the POS tags (the output of the BI-RNN) at time step i . The best POS tagging assignment T ˜ = argmax t i y ( t i ) , 1 ≤ i ≤ N is computed one tag at a time, independently of the already-made decisions. At this point, it would be very nice if we could incorporate already-made decisions into the best POS tagging assignment discovery and the key to this development is the use of a CRF model on the output of the BI-RNN.
A BI-RNN for POS tagging
The deep neural network obtained by constructing a CRF model over the output of the BI-RNN is called a BI-RNN-CRF neural network. The advantage of using a CRF model is that it can use sentence-wide features and we can model the POS tag N-gram sequences directly (as we did for the HMM models), with the addition of the tag-to-tag transition matrix A (temporally invariant, i.e. the transition score from tag t i to tag t i + 1 does not depend on the time step i ). When searching for the best tagging assignment T ˜ , we should now solve the problem T ˜ = argmax t 1 , … , t n Σ i = 1 N − 1 ( A t i t i + 1 + y ( t i ) ) which can be done efficiently with the Viterbi decoder.
At the beginning of this section we mentioned that there is one differentiating factor between the RNN and the LSTM neural networks and the difference is in the structure of a hidden layer neuron: RNN has classic, sigmoid-based neurons while LSTM develops a more complicated structure of a ‘memory cell’ designed to remember information from a long history ( Huang et al. 2015 ; Figure 24.2 ). Reviewing the mathematics of an LSTM network is beyond the scope of this chapter (see more details in Chapter 14 ) but it is worth mentioning that training and running BI-LSTM-CRF networks is done in the exact same way as with BI-RNN-CRF networks.
Using a BI-LSTM-CRF network, Huang et al. (2015) report an all-token POS tagging accuracy of 97.55% on the standard test part of the Wall Street Journal , release 3 (see n. 3 ), the best accuracy of a POS tagging algorithm (the absolute best POS tagging algorithm is a BI-LSTM-CRF network with a character-based input word encoding; see Akbik et al. 2018 ). For an exact account of the BI-LSTM-CRF deep neural network used to obtain this result, including a detailed description of the features that were used and the training procedure (which is quite involved), we direct the reader to Huang et al. (2015) .
24.4.3 Rule-Based Methods
Historically, the first taggers were based on handwritten grammars. Building them was time-consuming and the taggers were hardly adaptable to other languages or even the same language but a different universe of discourse. Their accuracy was significantly lower than that of the early statistical taggers. These were major drawbacks which, complemented by the increasingly better performance of stochastic taggers, made them obsolete for a while.
Hindle (1989) implemented a parser-based (Fiddich) part-of-speech disambiguator which was probably the first rule-based tagger (Fiddich used 700 handwritten disambiguation rules for 46 lexical categories) with performance close to the one of statistical taggers of the late 1980s ( Garside et al. 1987 ; Church 1989 ). Although its development time was significantly longer than that necessary for building a statistical tagger and the rule writing required linguistic expertise, Hindle’s tagger was innovative as it included for the first time the ability to learn additional disambiguation rules from training data.
This idea has been further explored, developed, and implemented in the tagger created by Eric Brill ( 1992 , 1995 ). Brill’s tagger is the best-known and most used data-driven rule-based tagger and it will be reviewed in the section on Transformation-Based Tagging . In the following section we will discuss a pure rule-based, grammar-based system, which challenges all the current statistical taggers.
24.4.3.1 Constraint Grammar tagging
Constraint Grammar (CG) is a linguistic formalism proposed by Fred Karlsson (1990) based on pattern-action rules. CG is the underlying model for the EngCG tagger ( Vuotilainen and Tapanainen 1993 ; Tapanainen and Vuotilainen 1994 ).
The tagger is ‘reductionistic’ in the sense that it iteratively reduces the morphological ambiguity for words, the context of which matches the pattern of one or more grammar rules. In an initial phase, a two-level morphological analyser generates all possible interpretations (the ambiguity class) for each word in the input sentences. The words out of the system’s lexicon are associated with the interpretations generated by a rule-based guesser.
The proper disambiguation phase is achieved by a collection of several pattern-action reductionistic rules. The pattern of such a rule defines one or more contexts (constraints) where a tag from the ambiguity class is illegitimate.
For instance, a rule ‘REMOVE (V) IF (−1C (ART))’ will remove the verb reading of an ambiguous word (which has in its ambiguity class a verb interpretation) if it is immediately preceded by a word unambiguously tagged as an article.
The disambiguator avoids risky predictions and about 3–7% of the words remain partially disambiguated, with an average of 1.04–1.08 tags per output word ( Tapanainen and Voutilainen 1994 ). EngCG-2 tagger uses 3,600 constraints and disambiguates arbitrary English texts with an accuracy of 99.7%. The development of the grammar rules took several years. A word which is left partially disambiguated is considered correctly tagged if the correct tag is among the remaining ones. When EngCG-2 tagger’s output is further processed by a finite-state parser that eliminates the remaining ambiguities, the final accuracy drops a little bit and is reported to be 99.26%, higher than that of any reported statistical tagger.
However, these results have been seriously questioned. One major issue was related to the notion of correct analysis ( Church 1992 ) given that even human annotators after negotiation, in double-blind manually tagging tasks, usually disagree on at least 3% of all words (cf. Samuelsson and Vuotilainen 1997 ). According to this opinion, it would be irrelevant to speak about accuracy higher than 97%. There are several arguments against such a criticism, some of them provided in Samuelsson and Vuotilainen (1997) . They show that in their experiments with double-blind manual tagging ‘an interjudge agreement virtually of 100% is possible, at least with the EngCG tagset if not with the original Brown Corpus tag set’.
A second reservation concerned the EngCG tagset which, being underspecified, was thought to make the tagging task easier than it might be. Samuelsson and Vuotilainen (1997) provide evidence that this suspicion is unjustified by training a state-of-the-art trigram statistical tagger on 357,000 words from the Brown Corpus re-annotated with the EngCG tagset. On a test corpus of 55,000 words of journalistic, scientific, and manual texts, the best accuracy obtained by the statistical tagger was 95.32%.
24.4.3.2 Transformation-based tagging
This approach was pioneered by Eric Brill (1992) and it hybridizes rule-based and data-driven methods for part-of-speech tagging. The proper tagging process is rule-based while the rules controlling the process are learned from a training corpus, part-of-speech annotated. The learning process is error-driven, resulting in an ordered list of rules which, when applied on a preliminary tagged new text, reduces the number of tagging errors by repeatedly transforming the tags, until the tagger’s scoring function reports no further improvement possible. The initial tagging could be simply the assignment of the most frequent tag for each word. If the most frequent tag of a word is not known, the initial tagging could resort to an arbitrary tag from the ambiguity class of that word.
The tagger training instantiates a set of predefined ‘patch’ templates by observing the differences between the current annotation of a word and the gold-standard annotation for the same word. A patch template has two parts: a rewrite rule and a triggering environment. A rewrite rule is simply ‘ change tag A to tag B ’. A triggering context might be verbalized as:
The preceding (following) word is tagged z
The preceding (following) word is w
The word two before (after) is w
One of the two preceding (following) words is tagged z
The current word is w and preceding (following) word is x
The current word is w and preceding (following) word is tagged z
The version reported in Brill (1995) used 21 patch templates which, after training on 600,000 words from the tagged Wall Street Journal Corpus, were instantiated by 447 transformation rules. Examples of instantiated rules, learnt after the training, are: 8
Change from NN to VB if the previous tag is TO
Change from VBP to VB if one of the previous three tags is MD
Change from VBD to VBN if one of the previous two tags is VB
The initial annotation and the gold standard annotation (of the same text) are compared word by word and, where they differ, a learning event is launched. To learn a transformation, the learner checks all possible instantiations of the transformation templates and counts the number of tagging errors after each one is applied over the entire text. A transformation rule improves one or more tags but it might also wrongly change some others. The transformation that has resulted in the greatest error reduction is chosen and appended to the ordered list of transformation rules. The learning process stops when no transformations can be found whose application reduces errors beyond some specified threshold ( Brill 1995 ). The accuracy of the tagging process (the percentage of the correct tags out of the total number of tags) was 97.2% when all the 447 learnt rules were applied. Brill (1995) notices that the rules towards the end of the learnt list have a modest contribution to the overall accuracy. When only the first 200 rules were applied the accuracy dropped only a little to 97%. For this experiment all the words in the test set were known to the tagger. To deal with unknown words, Brill’s tagger makes use of different transformation templates ( Brill 1995 ):
Change the tag of an unknown word (from X) to Y if: • Deleting the prefix (suffix) x, |x|≤ 4, results in a word • The first (last) (1,2,3,4) characters of the word are x • Adding the character string x as a prefix (suffix) results in a word (|x|≤ 4) • Word w ever appears immediately to the left (right) of the current word • Character z appears in the word.
A corpus of 350,000 words was used to learn 243 transformation rules for unknown words and on the test set (150,000 words) the tagging accuracy of unknown words was 82.2% and the overall accuracy was 96.6%.
Brill’s tagger could be optimized to run extremely fast. Roche and Shabes (1995) describe a method to compile the transformation rules into a finite-state transducer with one state transition taken for each word in the input string, with the resulting tagger running ten times faster than a Markov model tagger (cf. Brill 1995 ).
24.5 Conclusions
In this chapter we addressed the problem of part-of-speech tagging and its most popular approaches. We briefly discussed the data sparseness problem and the most effective methods to limit the inherent lack of sufficient training data for statistical modelling. The average performance of the state-of-the-art taggers for most languages is 97–98%. This figure might sound impressive, yet if we consider an average sentence length of 30 words it means that on average every third sentence 9 may contain two to three tagging errors which could be harmful for its higher-level processing (syntactic, semantic, discourse). With a limited number of ambiguities (k-best tagging) left in the output, for subsequent linguistically more informed processors, such as the EngCG-2 tagger, the accuracy of POS tagging could be almost 100%.
The multilinguality and interoperability requirements for modern tagging technology as well as the availability of more lexical resources has led to larger tagsets than those used earlier, and consequently it has become important to give the tagsets an appropriate design. The maximally informative linguistic encodings found in standardized computational lexicons are too numerous to be directly used as POS tagsets. Tiered tagging methodology ( Tufiș 1999 ) is one way of coping with large lexical tagsets in POS tagging, while still ensuring robustness and high accuracy of the tagging process.
Further Reading and Relevant Resources
One of the most comprehensive textbooks on tagging (both on implementation and user issues) is Syntactic Wordclass Tagging, edited by von Halteren in 1999. High-quality papers on various models of the tagging process can be found in the proceedings of conferences organized by the Association for Computational Linguistics (ACL, EACL, and EMNLP), ELDA/ELRA (LREC), and the International Committee for Computational Linguistics (COLING), as well as in several regional conferences. A web search (POS tagging + language of interest) is always a good way of finding preliminary information. A useful source of information on some of the available tools for text preprocessing (tokenization, lemmatization, tagging, and shallow parsing) can be found at < http://nlp.stanford.edu/links/statnlp.html#Taggers > and < https://aclweb.org/aclwiki/POS_Tagging_(State_of_the_art) >. Complementary information can be obtained from: < http://acopost.sourceforge.net/ >, < http://sourceforge.net/projects/acopost/ >, < http://ilk.uvt.nl/mbt/ >, < http://ucrel.lancs.ac.uk/claws/ >, < http://nlp.postech.ac.kr/~project/DownLoad >, < http://www.ims.uni-stuttgart.de/projekte/corplex/TreeTagger/DecisionTreeTagger.html >, < http://search.cpan.org/~acoburn/Lingua-EN-Tagger/ >, < http://alias-i.com/lingpipe/ >, < http://code.google.com/p/hunpos/ > and several other places such as the web pages of various infrastructural European projects on language and speech technology: CLARIN ( http://www.clarin.eu ), FlaReNet (< http://www.flarenet.eu >, < http://www.resourcebook.eu >), MetaNet ( http://www.meta-net.eu ), etc. The tiered tagging methodologies have been implemented by METT ( Ceauşu 2006 ) and TTL ( Ion 2007 ). TTL has been turned into a SOAP-based web service ( Tufiș et al. 2008 ), available at < http://ws.racai.ro/ttlws.wsdl >.
Akbik, Alan , Duncan Blythe , and Roland Vollgraf (2018). ‘Contextual String Embeddings for Sequence Labeling’. In Proceedings of the 27th International Conference on Computational Linguistics , Santa Fe, New Mexico, USA, 1638–1649. Stroudsburg, PA: Association for Computational Linguistics.
Black, Ezra , Fred Jelinek , John Lafferty , Robert Mercer , and Salim Roukos (1992). ‘Decision Tree Models Applied to the Labeling of Text with Parts-of-Speech’. In Proceedings of the HLT’91 Workshop on Speech and Natural Language , Harriman, New York, 117–121. Stroudsburg, PA: Association for Computational Linguistics.
Boroș, Tiberiu , Radu Ion , and Dan Tufiș (2013). ‘Large Tagset Labeling Using Feed Forward Neural Networks: Case Study on Romanian Language’. In Proceedings of the 51st Annual Meeting of the Association for Computational Linguistics , Sofia, Bulgaria, 692–700. Stroudsburg, PA: Association for Computational Linguistics.
Brants, Thorsten (2000). ‘TnT: A Statistical Part-of-Speech Tagger’. In Proceedings of the Sixth Conference on Applied Natural Language Processing , Seattle, WA, 224–231. Stroudsburg, PA: Association for Computational Linguistics.
Brill, Eric (1992). ‘A Simple Rule-Based Part of Speech Tagger’. In Proceedings of the Third Conference on Applied Natural Language Processing , Trento, Italy, 152–155. Stroudsburg, PA: Association for Computational Linguistics.
Brill, Eric ( 1995 ). ‘ Transformation-Based Error-Driven Learning and Natural Language Processing: A Case Study in Part-of-Speech Tagging ’, Computational Linguistics , 21(4): 543–565.
Google Scholar
Ceauşu, Alexandru ( 2006 ). ‘ Maximum Entropy Tiered Tagging ’. In Proceedings of the Eleventh ESSLLI Student Session , Malaga, Spain, 173–179. European Association for Logic, Language, and Information.
Google Preview
Chen, Stanley F. and Joshua Goodman (1998). ‘An Empirical Study of Smoothing Techniques for Language Modelling’. Technical Report TR-10-98, August, Computer Science Group, Harvard University, Cambridge, MA.
Christodoulopoulos, Christos , Sharon Goldwater , and Mark Steedman (2010). ‘Two Decades of Unsupervised POS Induction: How Far Have We Come?’. In Proceedings of the Conference on Empirical Methods in Natural Language Processing (EMNLP 2010) , MIT, Cambridge, MA, 575–584. Stroudsburg, PA: Association for Computational Linguistics.
Choi, Jinho D. (2016). ‘Dynamic Feature Induction: The Last Gist to the State-of-the-Art’. In Proceedings of the Conference of the North American Chapter of the Association for Computational Linguistics: Human Language Technologies , San Diego, CA, 271–281. Stroudsburg, PA: Association for Computational Linguistics.
Church, Kenneth (1989). ‘A Stochastic Parts Program and Noun Phrase for Unrestricted Text’. In Proceedings of IEEE 1989 International Conference on Acoustics, Speech and Signal Processing , Glasgow, 695–698. IEEE Press.
Church, Kenneth ( 1992 ). ‘Current Practice in Part of Speech Tagging and Suggestions for the Future’. In C. F. Simmons (ed.), Sborn í k Pr á ci: In Honor of Henry Ku č era , 13–48. Ann Arbor, MI: Michigan Slavic Studies.
Collins, Michael (2002). ‘Discriminative Training Methods for Hidden Markov Models: Theory and Experiments with the Perceptron Algorithm’. In Proceedings of the Conference on Empirical Methods in Natural Language Processing (EMNLP 2002) , Philadelphia, PA, 1–8. Stroudsburg, PA: Association for Computational Linguistics.
Curran, James R. and Stephen Clark (2003). ‘Investigating GIS and Smoothing for Maximum Entropy Taggers’. In Proceedings of the Tenth Conference of the European Chapter of the Association for Computational Linguistics (EACL ’03) , Budapest, Hungary, 91–98. Stroudsburg, PA: Association for Computational Linguistics.
Cussens, James ( 1997 ). ‘Part-of-Speech Tagging Using Progol’. In N. Lavrač and S. Džeroski (eds), Inductive Logic Programming: 7th International Workshop, ILP-97 , 93–108. Lecture Notes in Computer Science, 1297. Berlin and Heidelberg: Springer.
Daelemans, Walter , Jakub Zavrel , Peter Berck , and Steven Gillis (1996). ‘MBT: A Memory-Based Part-of-Speech Tagger Generator’. In Proceedings of 4th Workshop on Very Large Corpora , Copenhagen, Denmark, 14–27. Stroudsburg, PA: Association for Computational Linguistics.
Darroch, John N. , and Douglas Ratcliff ( 1972 ). ‘ Generalized Iterative Scaling for Log-linear Models ’. The Annals of Mathematical Statistics , 43(5): 1470–1480.
Della Pietra, Stephen A. , Vincent J. Della Pietra , Robert Mercer , and Salim Roukos (1992). ‘Adaptive Language Modeling Using Minimum Discriminant Estimation’. In Proceedings of the IEEE International Conference on Acoustics, Speech and Signal Processing , San Francisco, 633–636. IEEE Press.
Della Pietra, Stephen A. , Vincent J. Della Pietra , and John Lafferty ( 1997 ). ‘ Inducing Features of Random Fields ’, IEEE Transactions on Pattern Analysis and Machine Intelligence , 19(4): 380–393.
Erjavec, Tomaž ( 2010 ). ‘MULTEXT-East XML: An Investigation of a Schema for Language Engineering and Corpus Linguistics’. In D. Tufiș and C. Forăscu (eds), Multilinguality and Interoperability in Language Processing with Emphasis on Romanian , 15–38. Bucharest: Romanian Academy Publishing House.
Garside, Roger , Geoffrey Leech , and Geoffrey Sampson ( 1987 ). The Computational Analysis of English: A Corpus-Based Approach . London: Longman.
van Halteren, Hans (ed.) ( 1999 ). Syntactic Wordclass Tagging . Text, Speech and Language Technology, 9. Dordrecht: Kluwer Academic Publishers.
Herbst, Evan and Joachims Thorsten (2007). ‘SVMhmm Sequence Tagging with Structural Support Vector Machines and its Application to Part-of-Speech Tagging’. < http://www.cs.cornell.edu/people/tj/svm_light/old/svm_hmm_v2.13.html >.
Hindle, Donald (1989). ‘Acquiring Disambiguation Rules from Text’. Proceedings of the 27th Annual Meeting of the Association for Computational Linguistics , Vancouver, Canada, 118–125. Stroudsburg, PA: Association for Computational Linguistics.
Huang, Zhiheng , Xu, Wei , and Yu, Kai (2015). ‘Bidirectional LSTM-CRF Models for Sequence Tagging’. < https://www.arxiv.org/abs/1508.01991 >, CoRR, 2015, abs/1508.01991
Ion, Radu (2007). ‘Word Sense Disambiguation Methods Applied to English and Romanian’ [in Romanian]. PhD thesis, Romanian Academy, Bucharest.
Johnson, Mark (2001). ‘Joint and Conditional Estimation of Tagging and Parsing Models’. In Proceedings of the 39th Annual Meeting on Association for Computational Linguistics (ACL '01) , Toulouse, France, 322–329. Stroudsburg, PA: Association for Computational Linguistics.
Karlsson, Fred ( 1990 ). ‘Constraint Grammar as a Framework for Parsing Running Text’. In Proceedings of 13th Conference on Computational Linguistics (COLING ’90) , Helsinki, Finland, vol. 3, 168–173. Stroudsburg, PA: Association for Computational Linguistics.
Lafferty, John , Andrew McCallum , and Fernando Pereira (2001). ‘Conditional Random Fields: Probabilistic Models for Segmenting and Labeling Sequence Data’. In Proceedings of the 18th International Conference in Machine Learning (ICML ‘01) , Berkshires, MA, 282–289. San Francisco: Morgan Kaufmann Publishers.
Lee, Keok Yoong , Aria Haghigi and Regina Barzilay (2010). ‘Simple Type-Level Unsupervised POS Tagging’. In Proceedings of the 2010 Conference on Empirical Methods in Natural Language Processing , MIT, MA, 853–861.
Maragoudakis, Manolis , Katia Kermanidis , and Nikos Fakotakis (2003). ‘Towards a Bayesian Stochastic Part-of-Speech and Case Tagger of Natural Language Corpora’. In Proceedings of the Conference on Corpus Linguistics , Lancaster: 486–495. Centre for Computer Corpus Research on Language Technical Papers, University of Lancaster.
Ratnaparkhi, Adwait (1996). ‘A Maximum Entropy Part of Speech Tagger’. In Proceedings of the Conference on Empirical Methods in Natural Language Processing (EMNLP 1996) , Philadelphia, PA, 133–142. Stroudsburg, PA: Association for Computational Linguistics.
Roche, Emmanuel and Yves Shabes ( 1995 ). ‘ Deterministic Part of Speech Tagging with Finite State Transducers ’, Computational Linguistics , 21(2): 227–253.
Rosenfeld, Ronald ( 1996 ). ‘ A Maximum Entropy Approach to Statistical Language Modelling ’, Computer Speech and Language , 10: 187–228.
dos Santos, Cicero Nogueira and Bianca Zadrozny (2014). Learning Character-Level Representations for Part-of-Speech Tagging. In Proceedings of the 31st International Conference on Machine Learning , Beijing, China, 1818–1826. JMLR: Workshop and Conference Proceedings, 32. Brookline, MA: Microtome Publishing.
Samuelsson, Chris and Atro Vuotilainen (1997). ‘Comparing a Linguistic and a Stochastic Tagger’. In Proceedings of the 35th Annual Meeting of the Association for Computational Linguistics and Eighth Conference of the European Chapter of the ACL , Madrid, Spain, 246–253. Stroudsburg, PA: Association for Computational Linguistics.
Schmid, Helmut (1994). ‘Probabilistic Part-of-Speech Tagging Using Decision Trees’. In Proceedings of International Conference on New Methods in Language Processing , 44–49. Manchester: University of Manchester. < http://www.ims.uni-stuttgart.de/projekte/corplex/TreeTagger/ >.
Sutton, Charles and Andrew McCallum ( 2006 ). ‘An Introduction to Conditional Random Fields for Relational Learning’. In L. Getoor and B. Taskar (eds), Introduction to Statistical Relation Learning , 93–128. Cambridge, MA: MIT Press.
Tapanainen, Pasi and Atro Voutilainen (1994). ‘Tagging Accurately: Don’t Guess if You Know’. In Proceedings of the 4th Conference on Applied Natural Language Processing , Stuttgart, Germany, 47–52. Stroudsburg, PA: Association for Computational Linguistics.
Toutanova, Kristina (2006). ‘Competitive Generative Models with Structure Learning for NLP Classification Tasks’. In Proceedings of the Conference on Empirical Methods in Natural Language Processing (EMNLP 2006) , Sydney, Australia, 576–584. Stroudsburg, PA: Association for Computational Linguistics.
Tufiș, Dan ( 1999 ). ‘Tiered Tagging and Combined Classifiers’. In F. Jelinek and E. Nöth (eds), Text, Speech and Dialogue , 28–33. Lecture Notes in Artificial Intelligence, 1692. Berlin and Heidelberg: Springer.
Tufiș, Dan ( 2016 ). ‘ An Overview of Data-Driven Part-of-Speech Tagging ’, Romanian Journal of Information Science and Technology , 19(1–2): 78–97.
Tufiș, Dan and Oliver Mason (1998). ‘Tagging Romanian Texts: A Case Study for QTAG, a Language Independent Probabilistic Tagger’. In Proceedings of the First International Conference on Language Resources and Evaluation , Granada, Spain, 589–596. Paris: European Language Resources Association.
Tufiș, Dan , Radu Ion , Alexandru Ceauşu , and Dan Ştefănescu (2008). ‘RACAI’s Linguistic Web Services’. In Proceedings of the Sixth International Conference on Language Resources and Evaluation , Marrakech, Morocco, 327–333. Paris: European Language Resources Association.
Viterbi, Andrew J. ( 1967 ). ‘ Error Bounds for Convolutional Codes and an Asymptotically Optimum Decoding Algorithm ’, IEEE Transactions on Information Theory , 13(2): 260–269.
Voutilainen, Atro and Pasi Tapanainen (1993). ‘Ambiguity Resolution in a Reductionistic Parser’. In Proceedings of the Sixth Conference of the European Chapter of the Association for Computational Linguistics , Utrecht, 394–403. Stroudsburg, PA: Association for Computational Linguistics.
Weischedel, Ralph , Marie Meteer , Richard Schwartz , Lance Ramshaw , and Jeff Palmucci ( 1993 ). ‘ Coping with Ambiguity and Unknown Words through Probabilistic Models ’, Computational Linguistics , 19(2): 219–242.
Zheng, Xiaoqing , Hanyang Chen , and Tianyu Xu (2013). ‘Deep Learning for Chinese Word Segmentation and POS Tagging’. In Proceedings of the Conference on Empirical Methods in Natural Language Processing (EMNLP 2013) , Seattle, WA, 647–657. Stroudsburg, PA: Association for Computational Linguistics.
This chapter is an adapted and extended version of the article by Tufiș (2016) .
We will use interchangeably the terms ‘word’ and ‘token’, but be aware of the differences, as discussed at the beginning of this section.
The Association of Computational Linguistics maintains a list of the best EN POS taggers at < https://aclweb.org/aclwiki/POS_Tagging_(State_of_the_art) >. Because all of them have been trained and tested on the same corpora, their performances are directly comparable. The BI-LSTM-CRF tagger is listed with an accuracy of 97.55% over all tokens of the test set, 1.09% better than the TnT HMM tagger. The best current POS tagger is actually a Cyclic Dependency Network tagger with a novel feature discovery algorithm ( Choi 2016 ).
The number of words in closed-class categories being limited, it is reasonable to assume that they are already in the tagger’s lexicon.
This didn’t count words that were not in the dictionary. We did the same experiment on Orwell’s novel Nineteen Eighty-Four for English and Romanian and obtained 92.88% and respectively 94.19% accuracy, using the Multext-Est compliant tagsets.
That is, E p f j = E p ˜ f j .
However, for automatic feature selection from a given candidate set, see Della Pietra et al. (1997) .
NN = noun, singular or mass; VB = verb, base form; TO = ‘to’; VBP = verb, non-3rd person singular present; MD = modal; VBD = verb, past tense; VBN = verb, past participle.
Due to local dependencies, tagging errors tend to cluster in the same sentences.
- About Oxford Academic
- Publish journals with us
- University press partners
- What we publish
- New features
- Open access
- Institutional account management
- Rights and permissions
- Get help with access
- Accessibility
- Advertising
- Media enquiries
- Oxford University Press
- Oxford Languages
- University of Oxford
Oxford University Press is a department of the University of Oxford. It furthers the University's objective of excellence in research, scholarship, and education by publishing worldwide
- Copyright © 2024 Oxford University Press
- Cookie settings
- Cookie policy
- Privacy policy
- Legal notice
Process-Oriented Profiling of Speech Sound Disorders
Sanne diepeveen.
1 HAN University of Applied Sciences, 6524 TM Nijmegen, The Netherlands
2 Donders Institute for Brain, Cognition and Behaviour, Department of Rehabilitation, Radboud University Medical Center, 6525 AJ Nijmegen, The Netherlands
Hayo Terband
3 Department of Communication Sciences and Disorders, University of Iowa, Iowa City, IA 52242, USA
Leenke van Haaften
Anne marie van de zande.
4 Rijndam Rehabilitation Centre Rotterdam, 3001 KD Rotterdam, The Netherlands
Charlotte Megens-Huigh
Bert de swart, ben maassen.
5 Centre for Language and Cognition, Groningen University, 9712 EK Groningen, The Netherlands
Associated Data
The data presented in this study are available on request from the corresponding author. The data are not publicly available due to articles in preparation.
The differentiation between subtypes of speech sound disorder (SSD) and the involvement of possible underlying deficits is part of ongoing research and debate. The present study adopted a data-driven approach and aimed to identify and describe deficits and subgroups within a sample of 150 four to seven-year-old Dutch children with SSD. Data collection comprised a broad test battery including the Computer Articulation Instrument (CAI). Its tasks Picture Naming (PN), NonWord Imitation (NWI), Word and NonWord Repetition (WR; NWR) and Maximum Repetition Rate (MRR) each render a variety of parameters (e.g., percentage of consonants correct) that together provide a profile of strengths and weaknesses of different processes involved in speech production. Principal Component Analysis on the CAI parameters revealed three speech domains: (1) all PN parameters plus three parameters of NWI; (2) the remaining parameters of NWI plus WR and NWR; (3) MRR. A subsequent cluster analysis revealed three subgroups, which differed significantly on intelligibility, receptive vocabulary, and auditory discrimination but not on age, gender and SLPs diagnosis. The clusters could be typified as three specific profiles: (1) phonological deficit; (2) phonological deficit with motoric deficit; (3) severe phonological and motoric deficit. These results indicate that there are different profiles of SSD, which cover a spectrum of degrees of involvement of different underlying problems.
1. Introduction
A substantial part of the caseload of speech and language pathologists (SLPs) consists of children with a speech sound disorder (SSD). Prevalence estimates vary, ranging from approximately 3.4% to 24.6% of children in the age of 4 to 8 years being diagnosed with an SSD [ 1 , 2 , 3 ]. Children with SSD are a heterogenous group in terms of symptoms and severity as well as regarding (suspected) underlying deficits (and comorbidities), which makes diagnosing children with SSD a complicated affair [ 4 , 5 ].
1.1. Speech Development
Speech is the product of a variety of linguistic and speech motor processes working together [ 6 , 7 , 8 , 9 ]. During speech production, the first process is the conceptualization of a preverbal message from memory or from perception, for example seeing a picture of a cat in a naming task. Next is the formation of an utterance (word or sentence), which is executed by two lexicalization steps: the selection of a lemma, which contains meaning and grammatical word information, and the related lexeme or word form. This lexeme is the input for the next phase, phonological encoding, which consists of generating the sequence of speech sounds and the syllabic and prosodic structures. The selected syllables are the basic elements of the next phase: articulomotor planning and programming. Here, the motor plans and programs for the different speech movements are formed. Motor planning involves the selection and sequencing of articulatory movement goals which are then implemented in muscle specific motor programs (motor programming). Finally, the articulatory movements are executed (motor execution). The neural signals are sent to peripheral systems and transformed into coordinated muscle activity, resulting in an acoustic speech signal [ 10 , 11 , 12 , 13 ].
Children develop adult-like speech both through the development of motor skills and through the expansion of the language system, especially the storage of words with their associated phonemes (lexeme) and the sound system (phonology). Around the age of 24 months, an expressive vocabulary spurt is observed in typically developing (TD) children. During this spurt, a temporary increase in the variability of jaw movements is found, which is believed to be due to the speech motor system rearranging itself to match the rapid cognitive and linguistic development [ 14 , 15 , 16 ]. Saletta et al. [ 17 ] found that a task with a higher linguistic load was associated with increased speech motor variability in TD children’s speech. Thus, linguistic/phonological development influences the speech motor system and vice versa. Both developmental systems can present problems in children with SSD and in intervention an SLP should use different therapy methods for the two systems. An SLP has to investigate both systems in the diagnostic phase. Problems of interpretation arise when an SLP uses only a naming task in the assessment process. Naming the picture of, for example, a cat in a speech assessment does not provide enough information to differentiate a linguistic deficit from a speech motor deficit based on speech errors alone (overt symptoms). If, in the example of the target word ‘cat’, the /k/ is substituted into [t] this may be interpreted as the phonological process of fronting; the child substitutes a sound produced with the tongue further back in the mouth for one made with the tongue tip just behind the teeth, at the front of the mouth. However, this substitution can also be seen as a simplification of the word ‘cat’; the child uses not two different articulatory movement goals, /k/ and /t/, but only one which is easier to produce. The present study set out to investigate the results of a process-oriented speech assessment in a large sample of children with SSD. Using a data-driven approach we investigated if subgroups can be distinguished and how they compare.
1.2. Current Practice in Speech Assessments and Interpretation
As mentioned above, diagnosing children with SSD is a hard task due to the ambiguity of the diagnostic markers for SSD subtypes and the overlap of speech symptoms between the different diagnostic labels. According to SLPs’ reports, a wide variety of different speech assessments are used to diagnose children with SSD and often more than one assessment is used for a single child/per case [ 18 , 19 , 20 , 21 , 22 , 23 , 24 ]. The obtained assessment data are interpreted based on the SLP’s own clinical experience and not on the basis of a clearly formulated set of objectified criteria, as evidenced from data from the Netherlands [ 18 ] and the United Kingdom [ 18 , 19 ]. From questionnaires and interviews of a total of 170 SLPs in the Netherlands, Diepeveen et al. [ 18 ] found that there is no consensus on the terminology and there are many idiosyncrasies in diagnosis and treatment planning of SSDs. A reported 85 different diagnostic labels were used for children with SSD and the speech symptoms associated with these labels showed large overlap. Furthermore, the reports indicated that intervention methods were used for a variety of different diagnostic labels and methods incongruent with their described purpose. The Nuffield Dyspraxia Programme, for example, was also used with children who had been diagnosed with a phonological problem [ 18 ]. Overall, the study concluded that there is no consensus among SLPs in the Netherlands on the terminology and there are many idiosyncrasies in diagnosis and treatment planning of SSDs.
SLPs have different classification systems at their disposal that differentiate subtypes of speech disorders in children (see Waring and Knight [ 25 ] for an overview). Two of the systems that are commonly used are Shriberg’s Speech Disorders Classification System (SDCS) [ 26 ] and Dodd’s Model of Differential Diagnosis (MDD) [ 27 , 28 ]. These two systems have a different approach on classifying SSD. The SDCS is based on the behavioral phenotype of the child’s speech and etiological background, whereas the MDD is based on a descriptive-linguistic approach. SDCS and MDD have been subject of prevalence studies, which are shortly summarized below.
The SDCS is an organized framework to distinguish between several subtypes of SSD. It has four levels: etiological processes (distal causes), speech processes (proximal causes), clinical typology (behavioral phenotypes) and diagnostic markers (critical signs of phenotype). At the clinical typology level, three different types are described; each characterized by a specific set of disorders. The three main groups are: speech delay (SD), speech errors (SE) and motor speech disorder (MSD [ 29 ]). In a study of 97 children with SSD, Vick et al. [ 30 ] discovered two groups of children based on five speech tasks and also non-speech tasks. One group (76%) met the criteria of SD and a smaller group (10.3%) met the criteria of motor speech disorder—not otherwise specified (MSD-NOS). Differences between the groups were on atypical speech movements such as a higher variability in measures of articulatory kinematics and a poor performance on iambic lexical stress word imitation in the MSD-NOS group. To further examine the use of the SDCS for the motor speech disorder group and to estimate the prevalence of the types of motor speech disorders, Shriberg et al. [ 29 ] used a sample of 415 children with idiopathic speech delay. A conversational speech sample of each child was used to complete a narrow phonetic transcription, a prosody-voice coding, and an acoustic analysis. These were then entered into the SDCS analysis program and based on the outcomes of the three measures a child was classified in a group. The classification of MSD applied, was Speech Motor Delay, Childhood Dysarthria, Childhood Apraxia of Speech (CAS), and concurrent Childhood Dysarthria and CAS. The following results emerged: 82.2% of the children that met the SDCS criterion for SD at assessment had no MSD; 17.8% with SD met criteria of one of the subgroups of MSD. Of the latter group, 12% was classified has having a Speech Motor Delay; 3.4% met criteria for Childhood Dysarthria and 2.4% children were classified with CAS. None of the children were classified has having the combination Childhood Dysarthria and CAS.
Another model that is often used by SLPs is Dodd’s [ 27 ] Model for Differential Diagnosis (MDD). The MDD model contains the following diagnostic labels: (1) articulation disorder: substitutions or distortions of sound (e.g., lateral lisp); (2) phonological delay: speech error patterns typical of younger children; (3) consistent atypical phonological disorder: consistent error patterns of unusual non-developmental errors; (4) inconsistent phonological disorder: inconsistent error pattern of the same lexical item and no oromotor difficulties; and (5) CAS: inconsistency in speech, oromotor signs, slow speech rate, disturbed articulation, short utterance length, poorer performance in imitation. For each of these labels a description is given of the speech problems that can be seen during assessment (Dodd, 2014). Ttofari-Eecen et al. [ 28 ] conducted a validation study for the MDD model and assessed a group of children who speak standard Australian English with the Goldman-Fristoe Test of Articulation 2 (GFTA-2) (Sounds-in-Words and Stimulability sections [ 31 ]), the Diagnostic Evaluation of Articulation and Phonology (DEAP Inconsistency Assessment) [ 32 ] and the Verbal Motor Production Assessment for Children (VMPAC) [ 33 ]. A total of 126 children were eventually divided into the five groups: suspected atypical speech motor control (10%); inconsistent phonological disorder (15%); consistent atypical phonological disorder (20%); phonological delay (55%); and articulation disorder (0%). Ttofari-Eecen et al. [ 28 ] concluded that although the model was designed for the use of children with an articulation or phonological delay or disorder only, the model can be used by SLPs in clinical practice to differentiate children with suspected SSD including children with motor speech disorder such as dysarthria or CAS.
In the MDD and the SDCS, classification is done through the description of the error patterns of the speech output and these errors are compared with typically developing children. Within the SDCS, the extensive use of etiological criteria is also included [ 25 ]. The question is whether an SLP can differentiate between the different diagnostic labels based on the error pattern and/or etiology. Both the MDD and the SDCS models leave little room for selecting multiple diagnoses per child, as shown in the two studies described above; all 415 children in Shriberg et al. [ 29 ] and all 126 children in Ttofari-Eecen et al. [ 28 ] received only one diagnosis. Speech errors and/or the etiological background are matched to a specific diagnostic label in both models, and thus these classification systems seem to leave no room for diagnosing the gradual involvement of multiple underlying deficits belonging to one or more different diagnostic labels [ 9 ].
1.3. Diagnostic Profiling within the Psycholinguistic Framework
As mentioned above, some children with SSD present problems in multiple processes, both linguistic and speech motor [ 34 ]. An SLP should therefore assess these multiple processes in a child with SSD to find out which one or more of these underlying processes show deficient functioning. The Psycholinguistic Framework aids SLPs to examine at a cognitive or psycholinguistic level where in the speech and language process the impairment is situated [ 35 ]. This framework is a psycholinguistic speech-processing model and comprises a ‘box and arrow’ model of speech processing skills and representations that serves as a guide for compiling individual profiles of strengths and weaknesses [ 12 ]. By comparing speech symptoms under different elicitation conditions within this framework, the proximal causes of SSD can be studied since involvement of underlying processes is different in different speech conditions. In a nonword imitation setting, for example, an alternative speech production route starts from auditory input. Since the child has no lexical representation of the target nonword available, the child must use either the phonological decoding and encoding system (analyze and select combinations of familiar consonants and vowels, possibly syllables) or the auditory-to-motor-planning pathway (repeating the sounds without phonological interpretation, such as in repeating click-sounds).
The problems experienced by children with SSD can be at the level of word-form retrieval, phonological encoding, motor planning and programming, and/or articulation (motor execution). Systematic comparison of speech symptoms under varying conditions allows for assessing a profile of intact and deficient processes. This calls for a shift in the clinical reasoning skills of SLPs from a more diagnostic classification system such as the MDD or the SDCS model (diagnostic categories based on error patterns within a naming task or spontaneous speech) to a process-orientated view [ 9 ]. In other words, an SLP should identify the possible deficiencies of the underlying speech processes [ 7 , 8 , 9 ]. Unfortunately, current diagnostic instruments are not designed to provide fine-grained information about the involvement of the different underlying speech production processes [ 9 ]. For example, Geronikou and Rees [ 36 ] conducted a small study to profile four Greek speaking children with SSD based on nonword auditory discrimination, mispronunciation detection, naming, real word repetition and nonword repetition. The children could be profiled as having issues with either phonological or motor representations and the authors concluded that there is a need for a study with a wider range of consonants and clusters in different positions in words in the diagnostic instrument and they also advised to use a larger group of children. Such a study is possible with a new diagnostic instrument developed and released in the Netherlands, the Computer Articulation Instrument (CAI) [ 37 ]. The basic idea of the CAI is that speech is elicited in different contexts, which each tap into different levels of the production process such that functioning of production processes can be assessed by comparing performances. In addition, the sample of elicited words and nonwords contain all consonants and clusters in different positions, in most cases in at least two different words/nonwords, depending on the frequency of occurrence of the consonants and clusters in the Dutch language. Thus, the instrument yields comprehensive speech profiles from several speech tasks that reflect the functioning of different speech production processes—including phonological skills and speech motor skills; a comparison of those speech profiles gives an indication of possible underlying deficits.
The first aim of the present study was to determine which components emerge in a sample of 150 children with SSD with Principal Component Analysis (PCA) of the speech measures (20 parameters) of the CAI. This analysis was previously conducted for a norm group of 1524 typically developing Dutch-speaking children aged between 2;0 and 7;0 (years;months) indicated five meaningful components: (1) picture naming (PN); (2) segmental quality of nonword imitation (NWI); (3) quality of syllabic structure of NWI; (4) word and nonword proportion of whole-word variability (PWV), based on word- and nonword repetition (WR and NWR); and (5) mono- and multi-syllabic sequences of maximum repetition rate (MRR) [ 13 ]. PCA is not premised on average skills, but on the variation of skills and particularly on covariance. In a typical population, variation of skills may not be expressed in specific underlying components, due to a ceiling effect. In contrast, in an SSD population, underlying deficits may cause large covariance. If the components are similar, this could mean that children with SSD go through similar developmental milestones as typically developing children, which could be interpreted as an overall speech delay. In contrast, a different component structure could imply a deviant speech profile, which would indicate specific speech deficits. The components can also provide information about the tasks in which specific speech symptoms appear, which helps interpretation regarding the psycholinguistic processes involved.
The second aim was to test whether profiles can be differentiated and identified with the CAI test battery [ 37 ] in the same sample of children with SSD. To this end, we conducted k-means cluster analysis, an unsupervised machine learning method to partition data into a k number of groups (clusters) by minimizing variances within clusters, maximizing group similarity. This analysis was exploratory with no preconceived hypotheses about how children would group.
2. Materials and Methods
2.1. participants.
Participants were recruited in collaboration with the SPEECH study [ 38 ]. 150 children aged 4;0 to 6;6 (years;months, M = 5;2) participated in this study. The sample consisted of 94 boys and 56 girls; this ratio between boys and girls is consistent with other international studies [ 2 , 29 ]. The children were recruited through private practices ( n = 60), special schools for language- and hearing-impaired children ( n = 60), a rehabilitation center ( n = 16), regular schools ( n = 12) and an audiological center ( n = 2) in the Netherlands. The children lived in different regions of the Netherlands (North, n = 13; East, n = 44; South, n = 20; West, n = 73). Three children also spoke a language other than Dutch: German, English, and Spanish.
Inclusion criteria were as follows:
- Aged 4;0 to 6;11 (years;monthts);
- Dutch as the primary language as indicated by parental report;
- No history of hearing problems based on parents’ or caregivers’ information (further indicated by care givers) about the child’s hearing status;
- A speech sound disorder (SSD) diagnosed by the referring SLP.
At the time of the study 138 children received speech and language therapy. One of these children had scores on the CAI above percentile 16 (see below) and was excluded for this study. Twelve children were recruited through regular schools and had no history of speech or language therapy; they were recruited for the control group of another study [ 38 ] and were found to have an SSD. These cases were referred to an SLP and were added to the SSD group.
Diagnoses were based on clinical observation and/or a Dutch speech assessment (note that no normalized and standardized assessments were available at the time) and determined by the child’s SLP. The majority of the children were diagnosed with a phonological disorder ( n = 105), seventeen children with CAS, nine children with a phonetic articulation disorder, five children with dysarthria and the diagnosis of two children was not further specified by the SLP. Eleven children (those recruited through regular schools and not receiving speech therapy at the time of the study) were not previously diagnosed and were referred to an SLP after the diagnostic session; these children did not receive a diagnostic label. Of all children, thirty-two children received more than one diagnosis; sixteen children were diagnosed with a phonological disorder in combination with a phonetic disorder; ten children with CAS and a phonological disorder; two children with dysarthria and a phonological disorder; one child with CAS and a phonetic disorder. Three children received three diagnoses (CAS, phonological disorder, and a phonetic disorder).
Receptive vocabulary of 123 children was determined with the Peabody Picture Vocabulary Test-III-NL [ 39 ] ( n = 79) or another comprehension test ( n = 44) was available in the child’s file. Ninety-one children had a quotient score above 85 (range 85–129; 32 children had a score below the 85 (range 66–84). The other children ( n = 26) were judged to have a normal comprehension level of the Dutch language, as determined by a professional (teacher, daycare employee and/or SLP), caregivers and the examiner. Comprehension language scores within normal range were not an inclusion criterion, since a comorbidity of a language impairment is common for children with SSD [ 1 ].
2.2. Data Collection
Caregivers were first asked to complete a questionnaire containing questions about their child’s speech and language development, and health condition. They also completed the Intelligibility in Context Scale (ICS [ 40 ]). If the child already received speech therapy, the SLP was also asked to fill out a questionnaire about the child’s speech and language abilities. The children were subsequently seen during one or two sessions by 12 student-SLPs or SLPs specifically trained in the administration of the different assessments. The assessment took place at school, private practice, rehabilitation center, or audiological center facilities, in a quiet room.
2.3. Materials
During the one or two sessions a receptive vocabulary task, the Peabody Picture Vocabulary Test-III-NL (PPVT-III-NL [ 39 ]); an auditory discrimination test (phonemic judgement) part of the Testinstrumentarium Taalontwikkelingsstoornissen (TTOS-ADT [ 41 ]) and the Computer Articulation Instrument (CAI) were conducted. The framework of the CAI is an integrated model of the cognitive and sensorimotor functions involved in speech production and perception (see Figure 1 [ 9 , 11 ]).

The speech production processes assessed in the four tasks of the Computer Articulation Instrument (Maassen & Terband [ 11 ]; Figure 15.2). MRR = maximum repetition rate. Printed with permission.
The first task of the CAI, picture naming (PN), examines the child’s ability to retrieve the stored information about a real word and contains the whole chain of the speech production process, from preverbal visual-conceptual processing to lemma access, word-form retrieval, phonological encoding, motor planning, and articulation (motor execution; see Figure 1 [ 11 ]). In the second task, the child is asked to imitate nonwords (NWI). Due to the nature of the task, the child has no lexical representation of the target utterance available, which means the child must use either the phonological decoding and encoding system or the auditory-to-motor-planning pathway. For the word (WR) and nonword repetition (NWR) tasks, the child is asked to repeat five words or nonwords five times to assess the variability of the speech of the child, which taps into the stages of motor planning and motor programming and stability of the phonological representation of the word form. The final task, maximum repetition rate (MRR), provides a window into the child’s motor execution by examining the child’s ability to repeat six different sequences as fast as possible (e.g., patakapataka…). For more information on the reliability, validation, and collection of the norms of the CAI, see van Haaften et al. [ 13 ].
2.4. Data Analysis
A computer or laptop with the CAI, which automatically stored the acoustic signal on the hard disk, was used. The children were seated in front of a microphone and wore open-back headphones to provide a good sound level of the automated instructions.
The recordings were transcribed (broad phonetic transcription) and analyzed according to the CAI examiner’s manual [ 37 ] on the computer by the student-SLPs or SLPs. The student-SLPs worked in pairs and the SLPs worked alone. Following the psychometric evaluation guidelines [ 13 ], all student SLPs and SLPs were required to practice the transcription and other analyses of the CAI with two practice-examples of children with SSD. After the training session, the results of the transcription and the analysis corresponded between the student-SLPs or SLPs. The transcriptions of the CAI of all children in this study were checked and differences were discussed between the student-SLPs or SLPs. The transcriptions were also checked by the first author (SD) or Anniek van Doornik (collaboration partner in collecting the data). After the transcription and analysis, an automated report was generated of several outcome measures of all CAI-tasks. The outcome measures (percentiles) were based on the data of the norm group [ 37 ]. Table 1 contains the outcome measures per speech task (parameters) used in the statistical analysis and the number of completed tasks per age group.
Parameters/outcome measures per speech task.
Note. PN = Picture Naming; NWI = NonWord Imitation; WR = Word Repetition; NWR = NonWord Repetition; MRR = Maximum Repetition Rate. Note. Level 4 and 5 are part of the five degrees of complexity of phonological contrasts of Dutch syllable-initial consonants described by Beers [ 42 ].
2.5. Statistical Analysis
All statistical analyses were performed using SPSS Version 26. All raw scores were transformed per age group (four/five/six years old) into z-scores to control for speech development to be able to compare the different variables with each other in a single analysis; the z-scores were calculated only with the raw scores of the 150 children with an SSD. To also control for outliers, z-scores lower than −2.33 or higher than 2.33 were replaced by −2.33 or 2.33, respectively; these were the lowest/highest z-scores observed in the CAI norm group. This was the case for eight z-scores in the entire database. Not all children could perform a correct sequence for the MRR task, due to speech–motor difficulties and/or due to shyness or inattentiveness of the child. Additionally, some recordings could not be analyzed due to the low acoustic quality. In cases where children made speech errors, for example replacing a sound with another sound, the missing score was replaced by the lowest z-score (−2.33) of the norm group. This was the case for ten children for the sequence pa; 15 for ta; 19 for ka; 59 for pataka; 29 for pata and 35 for taka.
A principal component analysis (PCA) with varimax rotation (listwise exclusion) was conducted to determine which components are present and to identify clusters of items.
The Kaiser-Meyer-Olkin (KMO) measure was calculated prior to the PCA to determine whether the sample size was adequate; a value larger than 0.5 is deemed acceptable [ 43 ]. The number of principals components (PC) was determined on the criterion for eigenvalues greater than 1. Components were retained if they featured at least three parameters. The CAI-parameters were considered for a PC if they had an absolute factor loading value of more than 0.4. The parameters with the highest factor loading on a PC were included in that PC [ 43 ].
Using the same procedure and criteria, a series of additional PCAs was performed subsequently on each of the subsets of variables loading significantly on one PC in the first analysis (see Table 2 ). There were several reasons to conduct this additional series. Because PCA necessarily applies listwise exclusion, the relatively large number of missing values in the MRR task also limited the number of datapoints for the other components. In the complementary PCAs per subset, all available data for that PC could be included. Factor loadings could thus be verified on all available data and composite performance scores could be obtained for the maximum number of children, including those with missing values on other PCs. These additional PCAs per subset also functioned as a check if the PCs should not be broken down into sub-components on the larger sample. Next, Pearson product-moment correlations were calculated to determine relationships between PCs. A split-half reliability of the PCs (comparing the outcomes when using half of the dataset, randomly selected, with the outcomes using the full dataset) was conducted to check whether the results were stable. If the results of the split-half procedure are similar to the results of the whole group this confirms the outcomes of the results of the conducted analysis.
Principal Component Analysis results for Picture Naming (PN), NonWord Imitation (NWI), Word (WR) and NonWord (NWR) Repetition, and Maximum Repetition Rate (MRR). The highest component loading of each parameter is displayed in boldface.
Note. PN = Picture Naming; NWI = NonWord Imitation; WR = Word Repetition; NWR = NonWord Repetition; MRR = Maximum Repetition Rate; PCCI = Percentage of consonants correct in syllable-initial position; PVC = Percentage of vowels correct; Level 4 = percentage of correct consonants /b/, /f/ and /ʋ/; Level 5 = percentage of correct consonants /l/ and /R/; RedClus = percentage of reduction of initial consonant clusters from 2 consonants to 1; CV = percentage of correct syllable structure CV; CVC = percentage of correct syllable structure CVC; CCVC = percentage of correct syllable structure CCVC; SP = Simplification processes, total score of the processes: fronting, stopping, voicing, devoicing and gliding; UP = Unusual processes, total score of the processes: backing, atypical stopping, Hsation, nasalisation and denasalisation; WR-PWV = Proportion of whole-word variability—Word Repetition; NWR-PWV = Proportion of whole-word variability—NonWord Repetition; MRR-pa = number of syllables per second of sequence /pa/; MRR-ta = number of syllables per second of sequence /ta/; MRR-ka = number of syllables per second of sequence /ka/; MRR-pataka = number of syllables per second of sequence /pataka/; MRR-pata = number of syllables per second of sequence /pata/; MRR-taka = number of syllables per second of sequence /taka/.
Subsequently, we conducted an exploratory k-means cluster analysis with the z-scores of all CAI parameters to test whether distinctive profiles could be identified in our sample of children with SSD. K-means clustering is an unsupervised machine learning method to partition data into a predetermined k number of clusters. In an iterative manner, the observations are divided into groups in a way that minimizes the within-cluster variance and maximizes the variance between clusters. To determine which number of clusters provided the best fit, a comparison was made between analyses with two to four clusters. First, the Iteration History of every number of clusters was compared to determine the best solution. After this procedure, the graphs of the clusters were observed to see how the outcomes of the parameters were combined in the different clusters. For example, a two cluster-composition could mean the outcomes of the parameters are clustered in a group with children that score reasonably well and a group with children that score very low. Finally, the number of children in the different clusters was observed to see if there were clusters with a very small number of children in them.
In order to check for possible bias due to age or gender, the distributions of age and gender were compared across clusters. The construct validity was examined by comparing the clusters with respect to parameters of the CAI. The external validity (criterion) was also examined by comparing the clusters with the outcomes of the ICS (objective measure of severity), receptive vocabulary (PPVT-III-NL), auditory discrimination test (T-TOS), indication of the severity of the speech problem judged by the SLP and care givers (subjective measure of severity), the diagnosis given by the SLP and setting of the child (for example a private practice). This was analyzed with an ANOVA or a Chi-squared test, depending on the level of measurement of the variable; significance was defined as p < 0.05 for all tests.
The results of the PCAs are presented first, along with the analysis of correlations between the PCs. Next, we describe the results of the cluster analysis, followed by a comparison between clusters of the PCs identified in the PCA as well as all the non-CAI variables. Note that all children in our sample have atypical speech development, which was verified with the percentage of consonants correct in syllable-initial position (PCCI) scores of the tasks Picture Naming and NonWord Imitation. The scores on these tasks were transformed into z-scores compared to the norm group. Note that these are different z-scores than used in the analysis of this study and were calculated with the average and the standard deviation of the norm group of the CAI [ 37 ]. All children scored below a z-score of −1.5 on at least one of the two parameters (PN-PCCI z-score M = −4.79, SD = 4.77; NWI-PCCI z-score M = −2.91, SD = 2.68), and no z-score higher than 1 occurred thus confirming the diagnosis of SSD for all children.
3.1. Principal Component Analysis
A PCA with orthogonal rotation (varimax) was conducted on all speech parameters of the CAI. The KMO measure confirmed adequacy of the sample for the analysis (KMO = 0.870). The analysis yielded a solution in which three components had an eigenvalue higher than 1, (12.7, 2.64 and 1.94 respectively). This three-component solution explained 61.7% of the variance. All principal components had a Cronbach’s alpha’s higher than 0.74, which indicates the internal consistency of the components were acceptable. The results of the PCA are presented in Table 2 . Parameters loading high on the first PC were all the parameters of the PN task plus the following parameters of the NWI task: Level 5, Simplification processes and the Unusual processes (PN+) (an explanation of the parameters can be found in Table 1 ). The second PC included WR, NWR and almost all the parameters of the NWI task except for Level 5, Simplification processes and the Unusual processes (NWI/PWV). The last PC contained all the parameters of the MRR. It should be noted that the parameters NWI-PCCI, NWI-level 4, NWI-SP and NWI-UP also had high loadings (above 0.4) on one of the other two components; these parameters were included with the PC on which the highest loading was calculated. The grouping was confirmed by repeating the analysis with half of the SSD group.
A complementary series of PCAs was performed to obtain composite performance scores for all children, including those with missing values on other components, and to verify factor loadings and check if the PCs should not be broken down into sub-components on the larger sample. All three PCAs yielded a one-component solution. Within this additional PCA, the first component (PN+), comprising the PN parameters and the phonological processes of the NWI task explained 63.2% of the variance (KMO = 0.884); the second PC (NWI/PWV) comprising the remaining NWI parameters and the two repetition tasks (WR and NWR) explained 61.8% of the variance (KMO = 0.889), and the third PC (MRR) containing all MRR parameters explained 50.2% of the variance (KMO = 0.788). Pearson product-moment correlations between the components of the second PCA were calculated. Moderate and significant correlations were found between PN+ (PC 1) and MRR (PC 3), and between NWI/PWV (PC 2) and MRR (PC 3). The correlation between PN+ and NWI/PWV was high. The results are shown in Table 3 .
Pearson correlations between factors, n = 100.
Note. PN = Picture Naming; NWI = NonWord Imitation; PWV = proportion of whole-word variability, Word and NonWord Repetition; MRR = Maximum Repetition Rate. * Correlation of factor scores is significant at the 0.01 level (two-tailed).
3.2. Cluster Analysis
A k-means cluster analysis was conducted with the same CAI parameters as used in the PCA (see Table 2 ). Forty-nine children out of a total 149 children were not included due to listwise exclusion (exclusion because of missing data); some children did not complete all the tasks due to failure or refusal. To check which number of clusters would fit best, the remaining 100 children were each allocated to one of either two, three or four clusters. The three-cluster analysis yielded the clearest results, which are shown in Table 4 and Figure 2 . The two-cluster analysis yielded one group of children who performed poorly on all parameters and one group who performed slightly better on all parameters. The four-cluster analysis yielded one group that scored significantly worse and one group that performed significantly better, each compared to the other three clusters. However, no clear interpretation could be made of the profiles of the other two, intermediate clusters. Therefore, the analysis of three clusters was chosen to be described here. To validate this choice the same procedure was applied on a random selection of half of the 100 cases. The clusters yielded approximately the same mean scores for the three clusters as for the one based on all 100 children for the k-means cluster analysis, and the same components emerged for the PCA.

Overview of the distribution of the parameters across the clusters in z-scores.
Measures age, gender, parameters of the CAI in three subgroups of children with SSD identified by cluster analysis ( n = 100).
Note. PN = Picture naming; NWI = Nonword imitation; WR = Word repetition; NWR = Nonword repetition; MRR = Maximum repetition rate; PCCI = Percentage of consonants correct in syllable-initial position; PVC = Percentage of vowels correct; Level 4 = percentage of correct consonants /b/, /f/ and /ʋ/; Level 5 = percentage of correct consonants /l/ and /R/; RedClus = percentage of reduction of initial consonant clusters from 2 consonants to 1; CV = percentage of correct syllable structure CV; CVC = percentage of correct syllable structure CVC; CCVC = percentage of correct syllable structure CCVC; SP = Simplification processes, total score of the processes: fronting, stopping, voicing, devoicing and gliding; UP = Unusual processes, total score of the processes: backing, atypical stopping, Hsation, nasalisation and denasalisation; WR-PWV = Proportion of whole-word variability—Word repetition; NWR-PWV = Proportion of whole-word variability—Nonword repetition; MRR-pa = number of syllables per second of sequence /pa/; MRR-ta = number of syllables per second of sequence /ta/; MRR-ka = number of syllables per second of sequence /ka/; MRR-pataka = number of syllables per second of sequence /pataka/; MRR-pata = number of syllables per second of sequence /pata/; MRR-taka = number of syllables per second of sequence /taka/, ~ no score because of a ceiling effect in the norm group. Note. Redclus for the norm group is inverted. Note. SP and UP: a lower score means better performance. Note. * ANOVA is significant at the 0.01 level. Note. The coding below the p -value are the results of the post-hoc analysis, e.g., I = II, I/II > III means: differences between cluster I and II are not significant, whereas I and II outperform III.
The three clusters that emerged differed significantly from each other with respect to the parameters PN-level 5, PN-CCVC, NWI-Level 4, NWI-Level 5, and all MRR parameters with all differences showing large effect sizes ( η 2 > 0.14). The children in cluster I outperformed children in cluster II and III, and children in cluster II scored better than children in cluster III. However, most of the CAI-parameters were not normally distributed, therefore, if a difference between the three groups was found to be significant at the 5% level, the comparison was reanalyzed using the Bonferroni corrected listwise comparisons for the non-normally distributed parameters. When this was applied, clusters I and II were not significantly different from each other on these parameters (Picture naming: PCCI, PVC, Level 4, RedClus, CV, CVC, SP, UP; NonWord Imitation: PCCI, PVC, RedClus, CV, CVC, SP, UP and the Word/NonWord Repetition), whereas cluster III was significantly different from clusters I and II. Children in cluster III scored lower than children in cluster I and II on all parameters. In Figure 2 the performance of the children on the tasks for the three clusters is shown.
3.3. Cluster Comparison with Non-CAI Variables
Two Chi-squared tests indicated that age and gender did not differ between the three clusters (see Table 4 ). A series of ANOVAs with post hoc pairwise comparisons indicated that the clusters did differ in the performance of the children on some of the additional assessments. With respect to the receptive vocabulary assessment (PPVT-III-NL), the auditory discrimination task (TTOS-ADT) and the speech intelligibility (ICS), the children in cluster I outperformed the children in clusters II and III while the cluster II children in turn also outperformed the children in cluster III (I > II > III; see Table 5 ).
Measures PPVT-III-NL, TTOS-ADT, ICS, intelligibility level SLP and parents, diagnosis and setting in three subgroups of children with SSD identified by cluster analysis ( n = 100).
Note. PPVT-III-NL = quotient of word comprehension; TTOS-ADT = percentile of auditory discrimination test. ICS = average score on the Intelligibility of Context Scale. Note. Data missing in this group: $ 16 children, $$ 15 children, $$$ 8 children; + 13 children; ++ 11 children, +++ 7 children; ^ 13 children, ^^ 5 children, ^^^ 1 child Note. Diagnosis: some children did not get a diagnosis, because the SLP did not include it in the questionnaire or the child was part of the control group. Note. * ANOVA is significant at the 0.01 level.
The SLPs and caregivers were asked to rate the child’s speech problem in the questionnaire. SLPs and caregivers were asked ‘How would you estimate the severity of the speech problem?’ and they could answer with Mild, Moderate or Severe. To see if the SLP’s judgement correlated with the distribution in the clusters, a comparison (Chi-squared test) was made between the clusters with respect to the judgement of the severity of the SSD. There was a significant difference between the three clusters on the three severity levels; these differences showed a moderate effect size ( V between 0.3 and 0.5) (see Table 5 ). Most of the children in cluster III were judged to have a severe speech problem, 19 (59.4%) children were considered to have a severe speech problem judged by SLPs and 13 (52.0%) children judged by their caregivers. The label moderate was mostly given to the children in cluster I and II. The label mild was given by the SLPs to 12 (80.0%) and by their caregivers to 16 (76.2%) children in cluster I; three children in cluster I were labeled by their caregivers as having no speech problem.
For 88 children, the diagnosis that they had received from their SLP was known (the diagnosis by the SLP based on the SLP’s assessment of the child); for 12 children, the SLP’s diagnosis was not known. The label phonological disorder was most often given by the SLPs followed by the diagnosis of CAS; phonetic disorder and dysarthria were the least frequent diagnoses. The result of the Chi-squared test showed no interaction between the diagnostic labels and the clusters.
The analysis also included the number of children attending a particular setting. The clusters differed significantly regarding setting. In clusters I and II, the largest category consisted of children who received speech therapy in a private practice. In cluster III, however, the largest category was special education for children with speech and language disorders. The settings audiologic center and rehabilitation center were divided roughly equally across the three clusters. The children who were initially recruited for the control group, but who turned out to have a speech problem, were mainly placed in Cluster I.
3.4. Comparison of Clusters and Components
To see if the clusters differed from each other on the scores on the three principal components (PC) identified in the PCA, a single multivariate ANOVA was conducted. The clusters differed significantly on each PC: PN+ (PC 1; F = 144.15, p = 0.000, η 2 = 0.748); NWI/PWV (PC 2; F = 57.15, p = 0.000, η 2 = 0.541) and MRR (PC 3; F = 88.66, p = 0.000, η 2 = 0.646). Table 6 presents the mean PC scores for the three clusters. Children in the largest cluster (I, n = 46) scored best on all components. Children in cluster II ( n = 28) showed a different pattern: they scored similar to children in cluster I on the PN+ PC and on the NWI/PWV, but they scored weak on the MRR. The children in cluster III ( n = 26) scored very low on all the PCs.
Mean factor scores and standard deviation of the three clusters per factor.
4. Discussion
The overall aim of this study was to determine the possibility of profiling children with SSD based on underlying deficits. For this, the CAI was administered, and a two-step analysis procedure was conducted, comprising a Principal Component Analysis (PCA) to find components, followed by a cluster analysis (k-means clustering) to find distinct profiles.
4.1. Step 1. Which Components Emerged and How Do These Compare to Norm Group Outcomes?
The PCA yielded three stable and meaningful components. The first component (labeled PN+) consisted of all picture naming (PN) parameters plus three parameters of the nonword imitation (NWI) task: NWI-level5 and the phonological processes (see Table 1 for explanation of the parameters). The second component (labeled NWI/PWV) consisted of the remaining NWI parameters and the two-proportion whole-word variability (PWV) parameters, based on word repetition (WR) and nonword repetition (NWR). The third component (labeled MRR) contained all maximum repetition rate (MRR) parameters. The results of the PCA in the current group of children with SSD differed from the results of the PCA in the CAI norm group, which consisted of Dutch children with typical development ( n = 1.524) aged two to seven years [ 13 ]. In the norm group, five components were discovered: PN; segmental quality of NWI; quality of syllabic structure of NWI; word and nonword proportion of whole-word variability (PWV) and MRR. Note that the phonological processes were not included in the norm group, probably because their frequency of occurrence was too low among the 4–7-year-olds. The component MRR emerged as one component in both samples.
The five components from the norm group [ 13 ] were used in a previous study to compare the scores of 41 children with SSD [ 44 ]. That is, the components’ weights obtained from the PCA of the norm group were used to calculate component scores for the children with SSD. In this study, the child’s SLP had scored the severity of the speech disorder as moderate or severe (mild did not occur). Children in the moderate group obtained better scores than children in the severe group on parameters of the Picture Naming and NonWord Imitation tasks, whereas word and nonword repetition consistency were equal for these two groups. Furthermore, the moderate and severe groups differed with respect to the MRR-bi-and trisyllabic parameters, but not with respect to the MRR-monosyllabic sequences. Thus, this study provided evidence that comparison of performance on the different speech tasks of the CAI can provide distinct profiles which are different from the norm group and related to severity of SSD.
In the present study, not only the number of components in the clinical sample was smaller than that of the norm group, but also the composition of the first two components was different. In the norm group, all the parameters of the PN task loaded onto one component, and segmental quality of NWI and quality of syllabic structure of NWI on two separate components. In contrast, in the SSD group the specific phonological parameters of the NWI task, namely the NWI-level5, and the two phonological processes, loaded onto the PN component rather than on the NWI. Thus, the first component in the SSD group comprised both segmental and syllabic aspects of picture naming as well as specific phonological aspects of nonword imitation. Therefore, phonological encoding is a stronger component in the SSD group. The second component in the SSD group contained the remaining parameters of NWI, reflecting overall segmental quality (PCC, PVC) and quality of syllable structure (CV, CVC, CCVC), plus the percentage whole-word variability (WR-PWV and NWR-PWV). Interpretation? Related to the chain: auditory—memory—encoding/assembly. The difference between the norm group and the children with SSD regarding the parameters WR-PWV and NWR-PWV could be due to the fact that the typical children are consistent in this task already at an early developmental stage, resulting in a ceiling effect, whereas large differences were found between the SSD subgroups. Overall, the two components in the children with SSD as compared to four components in the norm group, seem to indicate a much clearer dissociation in the SSD group as compared to the norm group between phonological processes of speech production (word form retrieval and phonological encoding) and the processes that follow (motor planning, programming, and the stability of those processes). For naming pictures, children use the whole chain of the speech production process, and thereby rely on their vocabulary and –for the speech production process– specifically on the stored word forms (lexemes). In contrast, for repeating nonwords speakers use either the phonological decoding and encoding systems, or the auditory-to-motor-planning pathway (or both). The statistical result that PN and NWI-parameters load largely on different components, indicates that this distinction in underlying processing has significant impact on the quality of production. This implies that it is important to assess both tasks to get a broad view on the whole speech production process and on parts of the chain. Children who make relatively few errors in speech production when imitating nonwords may have relatively little difficulty in pronouncing new words they are learning, which could be a starting point for a method of intervention.
4.2. Which Clusters Emerged?
After the PCA analysis, a cluster analysis (k-means clustering) was conducted to see if subgroups would emerge from the data. Three clusters were found. The children in cluster I ( n = 46) outperformed the children in the other two clusters on all parameters, while the children in cluster III ( n = 26) scored lowest on all parameters. However, compared to the norm group, the children in cluster I scored lower on all parameters of PN and NWI. Although the cluster I group shows little or no vowel replacement in their speech as well as few errors in the simple syllable structures (CV and CVC), these children do make cluster reduction errors and phonological processes do still occur in more complex syllables. Therefore, this cluster can be labelled as phonological deficit. The children in cluster II ( n = 28) showed a different pattern: they scored similar to the cluster I children on the PN+ and NWI/PWV principal components, but they scored weak on the MRR. As such, this cluster could be labeled as a phonological deficit with motoric deficit. The children in cluster III ( n = 26) scored very low on all components, and this cluster could thus best be labeled as severe phonological and motoric deficit.
4.3. How Do the Different Clusters Compare to Each Other and to Norm Data?
McLeod [ 45 ] concluded in her review that 11 studies found a weak to moderately significant correlation between ICS and PCC. In our study this correlation calculation was not part of the research question, but we found a severity trend as well. As discussed above for each task of the CAI a difference can be observed between the clusters. This can be further supported by data on the intelligibility of the children as assessed by the caregivers and the SLPs. The intelligibility on the ICS is significantly different between the three clusters; the intelligibility of children in cluster I is better than that of cluster II and the children in cluster III show the lowest intelligibility. This was also confirmed by the responses of the speech therapists to the question of how severe they thought the speech problem was. Here, too, the clusters differed significantly from each other; the severity of the SSD is rated as least severe for the children in cluster I and more severe for the children in cluster II, and the children in 670 cluster III are the most severe cases according to the SLPs (severity: III > II > I).
With respect to error patterns, a first difference between the three clusters that can be observed is in vowel production. PN-PVC and NWI-PVC in cluster I and II showed a fairly high score and do not differ much from the children with a typical speech development. In typical development, five-year-old children achieve a mean PVC of 97.0 (SD = 3.9) in naming pictures and 90.5 (SD = 7.5) in repeating nonwords (see Table 3 ) [ 37 ]. The cluster I and II children in the present study showed similar averages and did not differ significantly from each other. However, the children in cluster III obtained significantly lower PVC-scores compared to the norm data. Roepke and Brosseau-Lapré [ 46 ] also observed differences in vowel production for 39 typically developing children compared to 45 children with SSD. They concluded that no conclusion could be drawn from their study as to whether these speech errors are systematic and reflect speech severity because the children were not matched on language ability but on age; another pattern might have been obtained if children were matched on language ability. However, a clear pattern was visible in our study: the children with the most severe speech disorder (cluster III; severe phonological and motoric deficit) showed lower PVCs than the other two less severe speech disorder groups.
Regarding consonant production, the results showed a similar profile among the clusters in the SSD group, cluster I and II children had similar averages of PCCI on both PN and NWI while the children in cluster III scored lower on both tasks. In the case of PCCI, however, all children with SSD scored lower compared to the norm group data (percentage for the five-year-old: PCCI-PN = 95.2, SD = 5.2; PCCI-NWI = 82.5, SD = 10.1). These findings indicate once more that measures such as the percentage consonants correct can serve as a severity index [ 47 , 48 ].
Consistency of errors was also measured in the present study, by means of the proportion whole-word variability when repeating five words and five nonwords five times (PWV-WR and PWV-NWR respectively). The children in cluster I and II scored the same and the children in cluster III were significantly less consistent in repeating the five words and nonwords. Compared to children in the norm group the mean inconsistency scores of the two tasks were slightly higher for the children in cluster I and II, and the children in cluster III showed the largest variability.
The last task of the CAI is the Maximum Repetition Rate (MRR). The results showed that children in cluster I outperformed children in cluster II and III on all MRR parameters and that the cluster II children outperformed the children in cluster III, all with a large effect size. In comparison to the norm group (mean of the five years old ranges from 3.74 syll/s to 4.29 syll/s for the different sequences in the norm group [ 37 , 49 ]), the children in cluster I scored similar on all MRR parameters. The children in cluster II produced the monosyllabic sequences slightly slower than the children of the norm group, and the bi- and trisyllabic sequences were produced at least one syllable per second slower than the norm group. The cluster III children produced the /pa/ sequences somewhat slower than the norm group as well and produced all other sequences with at least one syllable per second slower [ 37 , 49 ]. Children in cluster II and III were slightly better on the mono syllabic sequences compared to the bi-tri syllabic sequences. This difference may be a predictor of motor planning and programming problems. Ozanne [ 50 ] performed a cluster analysis of 18 behaviors that could reveal an underlying speech motor planning and programming problem on a dataset in a study of 100 children (ages 3;0–5;6 years;months) with SSD of unknown origin. The most common problems of the children were incorrect DDK sequences (38%), slow DDK rate (35%) and an increase in errors with increased linguistic load (27%), which corroborates our findings.
In the past, several debates have taken place about the potential value of nonspeech oral motor tasks such as the MRR [ 51 ]. Criticism has mainly come from the field of adult acquired disorders, but most studies with children conclude that MRR should be part of the assessment of SSD [ 52 , 53 , 54 ]. The current study confirms that MRR performance has a distinctive contribution to the diagnosis of SSD. The distinction between Cluster I and II is primarily based on MRR, and the distinction between Cluster I and III on both MRR and the phonological components. Across clusters, the correlations between the phonological components are high, and the correlations between these clusters and MRR are moderate. This shows that MRR contributes to diagnostic classification as an indicator of speech motor involvement (Cluster 1 versus Cluster 2) and can be considered an indicator of severity (Clusters 2 and 3).
In summary, three conclusions can be drawn from the analysis of the clusters: (1) there are different profiles of SSD; (2) in which severity plays a role and (3) that cover a spectrum of degrees of involvement of different underlying problems.
In this study, the group of children with missing values in the MRR, because children could not or refused to perform a sequence, was not included in the cluster analysis. Why the children refused is not known; children were not asked to give an explanation. They might have refused out of boredom of the session. In addition, not all typically developing children in the MRR norm group performed a sequence either [ 48 ]. In the future, qualitative analysis (e.g., 0 = no MRR; 1 = could not perform a long sequence; 2 = could not perform a sequence correctly due to a speech error, etc.) could be used to assess the number of children who performed a sequence.
4.4. How Do These Relate to Diagnostic Classification Systems?
We cannot make a direct, quantitative comparison, between our results and the results of the previously mentioned two studies in the introduction classifying children with the SCDC [ 29 ] and with Dodd’s model [ 28 ], due to the large differences in tasks and data analysis method. This study applied a data-driven cluster analysis, while the other two studies aimed to classify the children according to pre-determined profiles that (are assumed to) correspond to certain subtypes of speech disorders. Furthermore, in our data, severity of the speech disorder also plays a role in clustering the outcomes of the CAI, while speech severity is not included in the validity studies of SCDC and in Dodd’s model.
4.4.1. Dodd’s Model for Differential Diagnosis (MDD)
The children in the consistent atypical phonological disorder group and the children in the phonological delay group in the study of Ttofari-Eecen et al. [ 28 ] had at least one (a)typical phonological error pattern and had no difficulty repeating the 25 words of the DEAP Inconsistency Assessment [ 32 ] multiple times. This group could be compared to the children in cluster I, who also had at least one typical and/or atypical phonological error pattern. However, children in cluster I had a higher mean score on the Word and NonWord repetition tasks of the CAI compared to the norm group of the CAI; children in cluster I scored les consistent. Therefore, they might not be similar to the consistent atypical phonological disorder group and the phonological delay group of the MDD model; these children do not have a lower score on an inconsistency assessment compared to a norm group. The children in cluster II performed similar to the children in the inconsistent phonological disorder group of the Ttofari-Eecen-study; they had a typical and/or atypical phonological error pattern and were inconsistent in their speech. The children in cluster III can be compared with the suspected atypical speech motor control group based on the overall low scores on the CAI-parameters, including the MRR-task. Ttofari-Eecen et al. [ 28 ] also found oromotor problems in their population; unfortunately, the results of the Dutch oromotor task was not known for all children in our study.
4.4.2. Speech Disorders Classification System (SDCS)
Comparison with the SDCS is even more complicated as the different categories of the SDCS are defined at different levels: etiological processes (distal causes), speech processes (proximal causes), and clinical typology (behavioral phenotype) [ 55 ]. Focusing on the categories based on clinical typology, the children in cluster I (phonological deficit cluster) can probably best be compared to children in the Speech Delay group (SD), as they showed no evidence of motor involvement (scores on PWV and MRR that are only slightly below the norm). The children in the other two clusters, with poor MRR would probably fall within the Motor Speech Disorder group (MSD). Further differentiation between subgroups of MSD requires additional speech motor tasks, which is beyond the scope of this study.
4.5. Clinical Implications and Future Research
In the future, the tasks of the CAI will be supplemented with components that can provide a more detailed view of problems with motor planning and programming. Examples of these components: are systematic manipulation of conditions during speech such as speeding up; blocking auditory feedback and exercises to determine a short-term learning effect [ 9 ]; as well as acoustic measurements of coarticulation and variability [ 56 ]. The aim of the CAI is to provide SLPs with sufficient information to plan a well-fitting intervention that is specifically tailored to the individual child. In 2010, Williams et al. reported 23 different interventions for children with SSD [ 57 ]. There are currently even more interventions available that were not included in that article, for example the Dynamic Temporal and Tactile Cueing (DTTC) [ 58 ] and since 2010 a few new interventions entered the market, for example Rapid Syllable Transition Treatment (ReST) [ 59 ]. More fine-grained analyses of underlying processing deficits could give a large contribution to the design of tailor-made therapy plans.
A classification of the different interventions and mapping these onto the outcomes of the process-oriented assessment might be a solution, as already described by several authors [ 12 , 60 , 61 ]. In their review, Wren and colleagues [ 61 ] proposed a framework of five different categories of interventions: (1) environmental, (2) auditory–perceptual, (3) cognitive–linguistic, (4) production, and (5) integrated. For the children in the present study, it would perhaps be best to offer the children in cluster I (phonological deficit cluster) an intervention in the auditory-perceptual category or the cognitive-linguistics category because as the results show these children showed problems mainly with the tasks PN and NWI. This suggests that these children experience problems primarily in lemma access, word form retrieval, and phonological encoding. To treat these problems, the SLP can choose an intervention that falls under the auditory perceptual interventions or the cognitive-linguistic interventions. The auditory perceptual interventions target the perceptual skills of the child to change the speech output. The aim is to immerse the child in an auditory stimulation of word targets as well as auditory discrimination exercises that stimulate the child’s phonemic awareness, for example cycles approach. The cognitive-linguistics interventions stimulate the higher-level processing to promote change in the speech through confronting a child with their reduced set of contrasts or increasing awareness of sounds in speech, for example Metaphon [ 61 ]. To help SLPs make a choice between these two interventions, Bron et al. [ 62 ] developed a flowchart for Dutch SLPs in which, for example, age is one of the factors. Younger children could have more difficulty with a cognitive-linguistic intervention (such as Metaphon), because this form of intervention relies more on the child’s cognitive abilities; the children must learn to hear the differences between their pronunciation of the word and the correct pronunciation and they must also understand that their pronunciation refers to a different concept than the word they mean to pronounce, for example the difference between ‘hat’ and ‘rat’. Younger children or children with lower cognitive abilities and/or children with an inconsistent error pattern tend to benefit more from a phonological cycles approach then from Metaphon [ 62 ].
The second group of children (cluster II, phonological and motoric deficit) scored worse on the speech motor tasks of the CAI (sequences of the MRR with more problems with the bi-tri syllabic sequences) than the children in cluster I; they also have more problems with the pronunciation of /l/ and /r/ (level 5) and the CCVC structures. These children have problems with the following underlying speech processes: lemma access, word form selection, phonological encoding and speech motor planning and programming (see Figure 1 ). The interventions in the category production could be a good choice; they can benefit from the guidance on phonetic placement or manner and imitation in combination with one of the interventions in the auditory perceptual or the cognitive-linguistic group.
The last group of children (cluster III, severe phonological and motoric deficit) score low on all the tasks and especially on the speech motor tasks. What also distinguishes this group from the other two clusters is the additional lower score on the auditory discrimination task. Integrating an auditory perceptual intervention with one that is more focused on the motor speech system (production) could help to fill the child’s phonological system and reduce the speech motor difficulties. Currently, SLPs combine interventions and usually choose the intervention based on availability and own experience [ 18 , 19 ]. Hopefully this will change in the future and SLPs will make their decisions during clinical reasoning on a process-oriented assessment and the framework described by Wren et al. [ 61 ]. Further development of treatment planning frameworks, flow charts and decision trees on additional assessments leading to specific treatment recommendation/prescription are warranted.
5. Conclusions
In summary, the results of this study demonstrated three underlying principal components of the CAI-parameters for a group of children with SSD. The components showed a different pattern compared to a study with typically developing children with the same CAI-parameters. Three different clusters of children could be identified. The largest group showed problems compared to the norm group only at the phonological level and could be characterized as having a phonological deficit. The second, much smaller group had the same problems but also experienced some difficulties at the speech motor level. This group was termed as having phonological and motor deficits. The third group, equal in number to the second, showed extensive problems at both the phonological and speech motor level and could be characterized as having severe phonological and motor deficits. This data-driven clustering shows that there seems to be a difference in severity of the speech disorders amongst the three clusters, and different profiles of speech processing problems could be detected in our sample. The profiles are informative with respect to treatment planning in that each profile implies a specific intervention approach. More comparative research is needed to test the diagnostic accuracy of process-orientated diagnosis methods including more and different children, for example children with dysarthria, and controlling for possible additional factors such as behavioral characteristics and language impairment [ 63 ].
Acknowledgments
The authors would like to thank all children that participated in this study and their parents or caregivers for their time and effort, Anniek van Doornik, student-SLPs and SLPs for their help with participant recruitment and test administration, and two anonymous reviewers for their constructive comments on an earlier version of this manuscript.
Funding Statement
This research received no external funding.
Author Contributions
Conceptualization, S.D., B.M., H.T. and B.d.S.; methodology, S.D., B.M. and H.T.; data collection, S.D., L.v.H., A.M.v.d.Z. and C.M.-H.; data analyses, S.D., B.M. and H.T.; writing—original draft preparation, S.D.; writing—review and editing, S.D., B.M. and H.T.; visualization, S.D., B.M. and H.T.; supervision, B.M., H.T. and B.d.S.; project administration, S.D. All authors have read and agreed to the published version of the manuscript.
Institutional Review Board Statement
The study was conducted in accordance with the Declaration of Helsinki, and approved by the research ethics committee of the Radboud University Nijmegen Medical Centre (file number: CMO 2016-2985), and the internal review board ETCL of de faculty of Humanities, Utrecht University (file number: doorn026-01-2017).
Informed Consent Statement
Informed consent was obtained from all subjects involved in the study.
Data Availability Statement
Conflicts of interest.
The authors declare no conflict of interest.
Publisher’s Note: MDPI stays neutral with regard to jurisdictional claims in published maps and institutional affiliations.

IMAGES
VIDEO
COMMENTS
The number of parts of speech varied from author to author: in early grammars nouns and adjectives formed one part of speech; later they came to be treated as two different parts of speech. The same applies to participles, which were either a separate part of speech or part of the verb. The article was first classed with the adjective.
Most traditional and descriptive approaches to parts of speech draw a distinction between major and minor word classes. The four parts of speech just mentioned—nouns, verbs, adjectives, and adverbs—constitute the major word classes, while a number of others, for example, adpositions, pronouns, conjunctions, determiners, and interjections ...
Just like y is sometimes a vowel and sometimes a consonant, there are words that are sometimes one part of speech and other times another. Here are a few examples: "I went to work " (noun). "I work in the garden" (verb). "She paints very well " (adverb). "They are finally well now, after weeks of illness" (adjective).
1 Open classes. The open parts-of-speech classes that may occur in a language are the classes of nouns, verbs, adjectives, and adverbs. Typically, each of these classes may be divided into a number of subclasses on the basis of certain distinctive gram-matical properties. For example, the class of nouns in English may be divided into such ...
'An implicational map of parts of speech' complements Hengeveld's (1992) original classification of part-of-speech systems (see 'Word Classes' in Language and Linguistics Compass (2007) 1-6), which has been presented in a greater detail in two subsequent articles: Hengeveld, Kees, and Jan Rijkhoff and Anna Siewierska. 2004.
A part of speech (also called a word class) is a category that describes the role a word plays in a sentence.Understanding the different parts of speech can help you analyze how words function in a sentence and improve your writing. The parts of speech are classified differently in different grammars, but most traditional grammars list eight parts of speech in English: nouns, pronouns, verbs ...
In natural language processing, POS tagging is the automatic annotation of words with grammatical categories, also called POS tags. Parts of speech are also sometimes called lexical categories. Most European languages have inherited the Greek and Latin part-of-speech classification with a few adaptations.
The concept of part of speech dates back to the classical antiquity philosophy and teaching. Plato made a distinction between the verb and the noun. After him, the word classification further evolved and parts of speech grew in number until Dionysius Thrax fixed and formulated them under a form that we still use today.
Other grammar texts prefer to think of parts of speech in terms of form and structure classes. The form classes are composed of the major parts of speech: nouns, verbs, adjectives, and adverbs. These are the words that carry the content or meaning of a sentence. The structure classes are composed of the minor parts of
The parts of speech refer to categories to which a word belongs. In English, there are eight of them : verbs , nouns, pronouns, adjectives, adverbs, prepositions, conjunctions, and interjections. Many English words fall into more than one part of speech category. Take the word light as an example.
Parts of Speech: The Ultimate Guide for Students and Teachers. By Shane Mac Donnchaidh September 11, 2021March 5, 2024 March 5, 2024. This article is part of the ultimate guide to language for teachers and students. Click the buttons below to view these.
In the English language, it's commonly accepted that there are 8 parts of speech: nouns, verbs, adjectives, adverbs, pronouns, conjunctions, interjections, and prepositions. Each of these categories plays a different role in communicating meaning in the English language. Each of the eight parts of speech—which we might also call the "main ...
The problem of word classification into parts of speech still remains one of the most controversial problems in modern linguistics. The attitude of grammarians with regard to parts of speech and the basis of their classification varied a good deal at different times. Only in English grammarians have been vacillating between 3 and 13 parts of ...
A distributional approach to the parts to the parts of speech classification can be illustrated by the classification introduced by Charles Fries. He wanted to avoid the traditional terminology and establish a classification of words based on distributive analysis, that is, the ability of words to combine with other words of different types.
The notional parts of speech are open classes of words, with established basic semantic, formal and functional characteristics. There are only four notional classes of words, which correlate with the four main syntactic positions in the sentence: nouns, verbs, adjectives, and adverbs. They are interconnected by the four stages of the lexical ...
Part-of-speech (POS) tagging, also called grammatical tagging, is the automatic assignment of part-of-speech tags to words in a sentence [9,10,11]. A POS is a grammatical classification that commonly includes verbs, adjectives, adverbs, nouns, etc. POS tagging is an important natural language processing application used in machine translation ...
From "parts of the sentence" to "parts of speech" to "word classes" On the other side of Eurasia, as already examined briefly in Chap. 5, a different trajectory of linguistic analysis was being explored. According to the account given in Halliday 1977/2003, in Greek tradition it was the rhetoricians, the Sophists, who
Parts of speech are a part of universal human grammar. In other words, they exist in every human language as categories. Parts of speech are essential to being able to use other grammar in a new language. Students will need to be able to identify and manipulate parts of speech in order to conjugate verbs.
As the current state-of-the-art feature selection approaches work on question classification in English, the Thai language has yet to investigate question classification. The feature selection approaches considering the Part-of-Speech (POS) tag can boost performance for classifying question type from the sentence in Thai texts.
Abstract. One of the fundamental tasks in natural-language processing is the morpho-lexical disambiguation of words occurring in text. Over the last twenty years or so, approaches to part-of-speech tagging based on machine learning techniques have been developed or ported to provide high-accuracy morpho-lexical annotation for an increasing number of languages.
There are four approaches to the problem: 1) The classical parts of speech theory goes back to ancient times. It is based on Latin grammar. According to the Latin classification of the parts of speech all words were divided dichotomically into declinable and indeclinable parts of speech. This system was reproduced in the earliest English grammars.
Abstract. The differentiation between subtypes of speech sound disorder (SSD) and the involvement of possible underlying deficits is part of ongoing research and debate. The present study adopted a data-driven approach and aimed to identify and describe deficits and subgroups within a sample of 150 four to seven-year-old Dutch children with SSD.