Have a language expert improve your writing
Run a free plagiarism check in 10 minutes, generate accurate citations for free.
- Knowledge Base
Methodology
- What Is Non-Probability Sampling? | Types & Examples

What Is Non-Probability Sampling? | Types & Examples
Published on July 20, 2022 by Kassiani Nikolopoulou . Revised on June 22, 2023.
Non-probability sampling is a sampling method that uses non-random criteria like the availability, geographical proximity, or expert knowledge of the individuals you want to research in order to answer a research question.
Non-probability sampling is used when the population parameters are either unknown or not possible to individually identify. For example, visitors to a website that doesn’t require users to create an account could form part of a non-probability sample.
Note that this type of sampling is at higher risk for research biases than probability sampling, particularly sampling bias .
- In non-probability sampling , each unit in your target population does not have an equal chance of being included. Here, you can form your sample using other considerations, such as convenience or a particular characteristic.
- In probability sampling , each unit in your target population must have an equal chance of selection.
Table of contents
Types of non-probability sampling, non-probability sampling examples, probability vs. non-probability sampling, advantages and disadvantages of non-probability sampling, other interesting articles, frequently asked questions about non-probability sampling.
There are five common types of non-probability sampling:
Convenience sampling
Quota sampling, self-selection (volunteer) sampling, snowball sampling, purposive (judgmental) sampling.
Convenience sampling is primarily determined by convenience to the researcher.
This can include factors like:
- Ease of access
- Geographical proximity
- Existing contact within the population of interest
Convenience samples are sometimes called “accidental samples,” because participants can be selected for the sample simply because they happen to be nearby when the researcher is conducting the data collection .
In quota sampling , you select a predetermined number or proportion of units, called a quota. Your quota should comprise subgroups with specific characteristics (e.g., individuals, cases, or organizations) and should be selected in a non-random manner.
Your subgroups, called strata , should be mutually exclusive. Your estimation can be based on previous studies or on other existing data, if there are any. This helps you determine how many units should be chosen from each subgroup. In the data collection phase, you continue to recruit units until you reach your quota.
There are two types of quota sampling:
- Proportional quota sampling is used when the size of the population is known. This allows you to determine the quota of individuals that you need to include in your sample in order to be representative of your population.
- Non-proportional quota sampling is used when the size of the population is unknown. Here, it’s up to you to determine the quota of individuals that you are going to include in your sample in advance.
Note that quota sampling may sound similar to stratified sampling , a probability sampling method where you divide your population into subgroups that share a common characteristic.
The key difference here is that in stratified sampling, you take a random sample from each subgroup, while in quota sampling, the sample selection is non-random, usually via convenience sampling. In other words, who is included in the sample is left up to the subjective judgment of the researcher.
You stand at a convenient location, such as a busy shopping street, and randomly select people to talk to who appear to satisfy the age criterion. Once you stop them, you must first determine whether they do indeed fit the criteria of belonging to the predetermined age range and owning or renting a property in the suburb.
Self-selection sampling (also called volunteer sampling) relies on participants who voluntarily agree to be part of your research. This is common for samples that need people who meet specific criteria, as is often the case for medical or psychological research.
In self-selection sampling, volunteers are usually invited to participate through advertisements asking those who meet the requirements to sign up. Volunteers are recruited until a predetermined sample size is reached.
Self-selection or volunteer sampling involves two steps:
- Publicizing your need for subjects
- Checking the suitability of each subject and either inviting or rejecting them
Keep in mind that not all people who apply will be eligible for your research. There is a high chance that many applicants will not fully read or understand what your study is about, or may possess disqualifying factors. It’s important to double-check eligibility carefully before inviting any volunteers to form part of your sample.
Snowball sampling is used when the population you want to research is hard to reach, or there is no existing database or other sampling frame to help you find them. Research about socially marginalized groups such as drug addicts, homeless people, or sex workers often uses snowball sampling.
To conduct a snowball sample, you start by finding one person who is willing to participate in your research. You then ask them to introduce you to others.
Alternatively, your research may involve finding people who use a certain product or have experience in the area you are interested in. In these cases, you can also use networks of people to gain access to your population of interest.
In this way, the process of snowball sampling begins. You started by attending the meeting, where you met someone who could then put you in touch with others in the group.
Purposive sampling is a blanket term for several sampling techniques that choose participants deliberately due to qualities they possess. It is also called judgmental sampling, because it relies on the judgment of the researcher to select the units (e.g., people, cases, or organizations studied).
Purposive sampling is common in qualitative and mixed methods research designs, especially when considering specific issues with unique cases.
Common purposive sampling techniques include:
- Maximum variation (heterogeneous) sampling
Homogeneous sampling
Typical case sampling.
- Extreme (or deviant) case sampling
Critical case sampling
Expert sampling.
These can either be used on their own or in combination with other purposive sampling techniques.
Maximum variation sampling
The idea behind maximum variation sampling is to look at a subject from all possible angles in order to achieve greater understanding. Also known as heterogeneous sampling, it involves selecting candidates across a broad spectrum relating to the topic of study. This helps you capture a wide range of perspectives and identifies common themes evident across the sample.
Homogeneous sampling , unlike maximum variation sampling, aims to achieve a sample whose units share characteristics, such as a group of people that are similar in terms of age, culture, or job. The idea here is to focus on this similarity, investigating how it relates to the topic you are researching.
A typical case sample is composed of people who can be regarded as “typical” for a community or phenomenon. A typical case sample allows you to develop a profile of what would generally be agreed as being “average” or “normal.”
Typical case samples are often used when large communities or complex problems are investigated. In this way, you can gain an understanding in a relatively short time, even if you are not familiar with what’s going on yourself.
Note that the purpose of typical case sampling is to describe and illustrate what is typical to those unfamiliar with the setting or situation. The purpose is not to make generalized statements about the experiences of all participants. In other words, typical case sampling allows you to compare samples, not generalize samples to populations.
Extreme (deviant) case sampling
Extreme (or deviant) case sampling uses extreme cases of a particular phenomenon ( outliers ). This can mean remarkable failures, successes, or crises, as well as any event, organization, or individual that appears to be the “exception to the rule.” Extreme case sampling is most often used when researchers are developing best-practice guidelines.
Note that extreme case sampling usually occurs in combination with other sampling strategies. The process of identifying extreme or deviant cases usually occurs after some portion of data collection and analysis has already been completed.
Critical case sampling is used where a single case (or a small number of cases) can be critical or decisive in explaining the phenomenon of interest. It is often used in exploratory research , or in research with limited resources.
There are a few cues that can help show you whether or not a case is critical, such as:
- “If it happens here, it will happen anywhere”
- “If that group is having problems, then all groups are having problems”
It is critical to ensure that your cases fit these criteria prior to proceeding with this sampling method.
Expert sampling involves selecting a sample based on demonstrable experience, knowledge, or expertise of participants. This expertise may be a good way to compensate for a lack of observational evidence or to gather information during the exploratory phase of your research.
Alternatively, your research may be focused on individuals who possess exactly this expertise, similar to ethnographic research .
Receive feedback on language, structure, and formatting
Professional editors proofread and edit your paper by focusing on:
- Academic style
- Vague sentences
- Style consistency
See an example
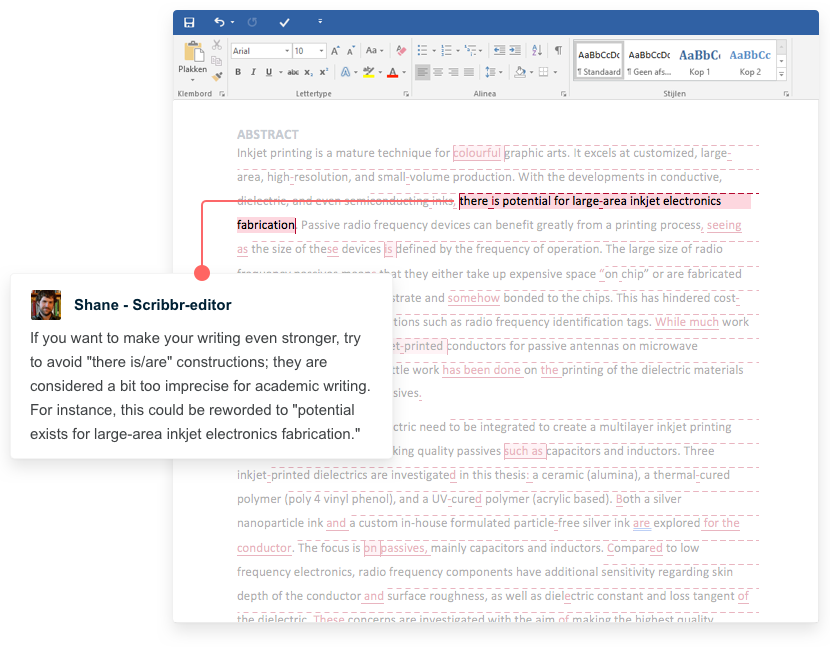
There are a few methods you can use to draw a non-probability sample, such as:
Social media
River sampling, street research.
Suppose you are researching the motivations of digital nomads (young professionals working solely in an online environment). You are curious what led them to adopt this lifestyle.
Since your population of interest is located all over the globe, it clearly isn’t feasible to conduct your study in person. Instead, you decide to use social media, finding your participants through snowball sampling.
You start by identifying social media sites that cater to digital nomads, such as Facebook groups, blogs, or freelance job sites. You ask the administrators for permission to post a call for participants with information about your research, encouraging readers to share the call with peers.
You are part of a research group investigating online behavior and cyberbullying, in particular among users aged 15 to 30 in your state. You are collecting data in two ways, using an online survey.
You first place a link to your survey in an online news article about cyber-hate published by local media. Second, you create an advertising campaign through social media, targeted at users aged 15 to 30 and linking back to your survey. To entice users to participate, a prize draw (movie tickets) is mentioned in all ads. The survey and the campaign are active for the same length of time.
These two data collection methods are river samples. The name refers to the idea of researchers dipping into the traffic flow of a website, catching some of the users floating by.
You are interested in the level of knowledge about myocardial infarction symptoms among the general population.
For a week, you stand in a shopping mall and stop passersby, asking them whether they would be willing to take part in your research. To try to allow as broad a range of respondents as possible to be included, you interview equal numbers of people from Monday to Friday during working hours.
Sampling methods can be broadly divided into two types:
- Probability sampling : When the sample is drawn in such a way that each unit in the population has an equal chance of selection
- Non-probability sampling : When you select the units for your sample with other considerations in mind, such as convenience or geographical proximity
Probability sampling
For many types of analysis, it is important that the statistical analysis is conducted from a random probability sample from the population of interest. For the sample to qualify as random, each unit must have an equal chance (i.e., equal probability) of being selected.
When you use a random selection method (e.g., a drawing) and ensure that you have a sufficiently large sample, your sample is more likely to be representative, and the results generalizable.
Non-probability sampling
Non-probability sampling designs are used when the sample needs to be collected based on a specific characteristic of the population (e.g., people with diabetes).
Unlike probability sampling, the goal is not to achieve objectivity in the selection of samples, or to make statistical inferences. Rather, the goal is to apply the results only to a certain subsection or organization. These are used in both quantitative and qualitative research.
It is important to be aware of the advantages and disadvantages of non-probability sampling and to understand how they can play a role in your study design.
Advantages of non-probability sampling
Depending on your research design, there are advantages to choosing non-probability sampling.
- Non-probability sampling does not require a sampling frame, so your subjects are often readily available. This can make non-probability sampling quicker and easier to carry out.
- Non-probability sampling allows you to target particular groups within your population. In certain types of research, it is vital that certain units be included in your sample. For example, many kinds of medical research rely on people with a specific health issue.
- Although it is not possible to make statistical inferences from the sample to the population, non-probability sampling methods can provide researchers with the data to make other types of generalizations from the sample being studied.
Disadvantages of non-probability sampling
Non-probability sampling has some downsides as well. These include the following:
- Non-probability samples are extremely unlikely to be representative of the population studied. This undermines the generalizability and validity of your results.
- As some units in the population have no chance of being included in the sample, undercoverage bias is likely.
- Furthermore, since the selection of units included in the sample is often based on ease of access, sampling bias is common as well.
- While the subjective judgment of the researcher in choosing who makes up the sample can be an advantage, it also increases the risk of observer bias .
You can mitigate the disadvantages of non-probability sampling by describing your choices in the methodology section of your dissertation . Specifically, explain how your sample would differ from one that was randomly selected and mention any subjects who might be excluded or overrepresented in your sample.
If you want to know more about statistics , methodology , or research bias , make sure to check out some of our other articles with explanations and examples.
- Student’s t -distribution
- Normal distribution
- Null and Alternative Hypotheses
- Chi square tests
- Confidence interval
- Quartiles & Quantiles
- Cluster sampling
- Stratified sampling
- Data cleansing
- Reproducibility vs Replicability
- Peer review
- Prospective cohort study
Research bias
- Implicit bias
- Cognitive bias
- Placebo effect
- Hawthorne effect
- Hindsight bias
- Affect heuristic
- Social desirability bias
When your population is large in size, geographically dispersed, or difficult to contact, it’s necessary to use a sampling method .
This allows you to gather information from a smaller part of the population (i.e., the sample) and make accurate statements by using statistical analysis. A few sampling methods include simple random sampling , convenience sampling , and snowball sampling .
A sampling frame is a list of every member in the entire population . It is important that the sampling frame is as complete as possible, so that your sample accurately reflects your population.
A sample is a subset of individuals from a larger population . Sampling means selecting the group that you will actually collect data from in your research. For example, if you are researching the opinions of students in your university, you could survey a sample of 100 students.
In statistics, sampling allows you to test a hypothesis about the characteristics of a population.
In stratified sampling , researchers divide subjects into subgroups called strata based on characteristics that they share (e.g., race, gender, educational attainment).
Once divided, each subgroup is randomly sampled using another probability sampling method.
Stratified and cluster sampling may look similar, but bear in mind that groups created in cluster sampling are heterogeneous , so the individual characteristics in the cluster vary. In contrast, groups created in stratified sampling are homogeneous , as units share characteristics.
Relatedly, in cluster sampling you randomly select entire groups and include all units of each group in your sample. However, in stratified sampling, you select some units of all groups and include them in your sample. In this way, both methods can ensure that your sample is representative of the target population .
Cite this Scribbr article
If you want to cite this source, you can copy and paste the citation or click the “Cite this Scribbr article” button to automatically add the citation to our free Citation Generator.
Nikolopoulou, K. (2023, June 22). What Is Non-Probability Sampling? | Types & Examples. Scribbr. Retrieved April 15, 2024, from https://www.scribbr.com/methodology/non-probability-sampling/
Is this article helpful?
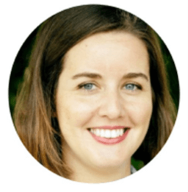
Kassiani Nikolopoulou
Other students also liked, sampling methods | types, techniques & examples, operationalization | a guide with examples, pros & cons, what is probability sampling | types & examples, "i thought ai proofreading was useless but..".
I've been using Scribbr for years now and I know it's a service that won't disappoint. It does a good job spotting mistakes”
- Privacy Policy
Buy Me a Coffee

Home » Non-probability Sampling – Types, Methods and Examples
Non-probability Sampling – Types, Methods and Examples
Table of Contents

Non-probability Sampling
Definition:
Non-probability sampling is a type of sampling method in which the probability of an individual or a group being selected from the population is not known. In other words, non-probability sampling is a method of sampling where the selection of participants is based on non-random criteria, such as convenience, availability, judgment, or quota.
Non-probability Sampling Methods
Non-probability Sampling Methods are as follows:
Convenience Sampling
This method involves selecting individuals or items that are easily accessible or convenient to the researcher. For example, a researcher conducting a study on college students may select participants from their own class or dormitory because they are convenient to access.
Snowball Sampling
This method involves selecting individuals who know other individuals who meet the criteria for the study. The researcher starts with a few participants and then asks them to refer others who may be interested in participating. This method is often used in studies where the population is difficult to access or identify.
Quota Sampling
This method involves selecting a sample that matches the characteristics of the population. The researcher sets quotas for each characteristic (such as age, gender, or occupation) and selects participants who fit into those quotas. This method is often used in market research studies.
Purposive Sampling
This method involves selecting individuals or items that meet specific criteria or have specific characteristics that the researcher is interested in studying. For example, a researcher studying the experiences of cancer survivors may purposively select individuals who have undergone chemotherapy.
Volunteer Sampling
This method involves selecting individuals who volunteer to participate in the study. This method is often used in studies where the population is difficult to access or identify.
How to Conduct Non-probability Sampling
To conduct a non-probability sampling, you should follow these general steps:
- Identify the target population: Identify the population you want to study. This can be a specific group of people, a geographic location, or any other defined population.
- Determine the sampling method: Choose the non-probability sampling method that is most appropriate for your study. Consider the advantages and disadvantages of each method and select the one that fits your research question and resources.
- Determine the sample size: Determine the appropriate sample size based on your research question, the available resources, and the sampling method you choose.
- Recruit participants : Recruit participants using the selected non-probability sampling method. For example, if you are using convenience sampling, you might approach people in a public place to participate in your study.
- Collect data: Collect data from the selected participants using the appropriate research methods, such as surveys, interviews, or observations.
- Analyze the data: Analyze the data collected from the sample to draw conclusions and make generalizations about the population.
Examples of Non-probability Sampling
- Convenience Sampling: In this type of sampling, participants are chosen because they are easy to reach or are readily available. For example, a researcher may choose to survey the first 100 people who enter a shopping mall.
- Quota Sampling : Quota sampling is a type of non-probability sampling in which participants are selected to ensure that the sample reflects the characteristics of the population in terms of certain traits. For example, if a researcher wants to conduct a study on the opinions of men and women about a certain product, they may select a sample that has an equal number of men and women.
- Purposive Sampling: In this type of sampling, participants are selected based on specific criteria such as age, gender, occupation, or experience. For example, a researcher may choose to interview only CEOs of Fortune 500 companies to study their leadership style.
- Snowball Sampling: Snowball sampling is a type of sampling in which the initial participants in a study are asked to refer others who they know that fit the criteria of the study. For example, a researcher may ask a person who has experienced homelessness to refer others they know who have experienced homelessness for a study on homelessness.
- Judgmental Sampling : In judgmental sampling, the researcher selects participants based on their own judgment about who would be the most appropriate for the study. For example, a researcher may select participants for a study on the effects of a new cancer drug based on their experience with the disease and their likelihood of benefiting from the treatment.
Applications of Non-probability Sampling
Here are some applications of non-probability sampling:
- Exploratory Studies: Non-probability sampling is commonly used in exploratory studies where the focus is on generating new ideas and insights rather than testing hypotheses. Exploratory studies often use a small sample size, and non-probability sampling is used to identify potential patterns or trends.
- Pilot Studies: Non-probability sampling is also used in pilot studies, which are small-scale studies conducted to evaluate the feasibility and potential outcomes of a larger study. Pilot studies often use a convenience sample or purposive sampling to identify potential issues or areas of improvement before conducting the larger study.
- Qualitative Research : Non-probability sampling is commonly used in qualitative research where the focus is on gaining an in-depth understanding of a particular phenomenon or context. Qualitative research often uses purposive sampling to identify participants who have the knowledge or experience needed to provide rich and detailed insights.
- Rare Populations : Non-probability sampling is used in studies of rare populations, where it may be difficult to obtain a large enough sample using a random sampling method. Snowball sampling is often used in studies of rare populations to identify potential participants through referrals from existing participants.
- Convenience Sampling : Non-probability sampling is also used in studies where the sample size is not a critical factor, and the focus is on convenience and efficiency. Convenience sampling is often used in market research, opinion polls, and customer satisfaction surveys.
- Ethnographic Research: Non-probability sampling is commonly used in ethnographic research, which involves studying the social and cultural practices of a particular group or community. Ethnographic research often uses purposive sampling to identify participants who can provide insights into the cultural practices and beliefs of the group being studied.
- Case Studies: Non-probability sampling is often used in case studies, which involve in-depth analysis of a single individual, organization, or event. Case studies often use purposive sampling to select the individual or organization that is most relevant to the study.
- Action Research: Non-probability sampling is also used in action research, which involves developing solutions to practical problems in real-world settings. Action research often uses purposive sampling to identify participants who can provide input and feedback on the proposed solutions.
- Behavioral Research: Non-probability sampling is used in behavioral research where the focus is on understanding human behavior, attitudes, and beliefs. Behavioral research often uses purposive sampling to identify participants who can provide insights into the behavior being studied.
- Historical Research: Non-probability sampling is used in historical research, which involves studying events and phenomena that occurred in the past. Historical research often uses purposive sampling to identify participants who have knowledge or experience relevant to the historical event or phenomenon being studied.
Purpose of Non-probability Sampling
The main purpose of non-probability sampling is to obtain a sample that is more convenient and practical than a random sample, particularly in situations where a random sample is not feasible, practical, or affordable. Non-probability sampling methods are often used in exploratory research, qualitative research, or in situations where researchers want to study a specific group or population.
When to use Non-probability Sampling
Here are some situations where non-probability sampling may be appropriate:
- Small or hard-to-reach populations: When the population of interest is small or difficult to access, non-probability sampling may be the only feasible option.
- Exploratory research: Non-probability sampling may be used in exploratory research studies where the objective is to generate hypotheses or insights for further investigation.
- Convenience sampling : This type of non-probability sampling is commonly used when the researcher selects the most convenient participants available, such as those who are nearby or easily accessible.
- Expert or judgmental sampling: When the researcher is interested in studying a specific group of individuals with specialized knowledge or expertise, expert or judgmental sampling may be used.
- Quota sampling: In quota sampling, the researcher identifies relevant characteristics of the population of interest and selects participants based on those characteristics in order to ensure a representative sample.
Characteristics of Non-probability Sampling
Here are some characteristics of non-probability sampling:
- Non-random selection : In non-probability sampling, the selection of participants is non-random and based on subjective criteria, such as convenience, availability, or judgment.
- Limited generalizability: Since non-probability sampling does not provide a representative sample of the population, the findings obtained from the sample may not be generalizable to the population as a whole.
- Biased sample: Non-probability sampling can result in a biased sample, which means that the sample is not representative of the population, leading to inaccurate or misleading conclusions.
- No sampling frame : Non-probability sampling does not require a sampling frame, which is a list of all the individuals or units in the population, making it easier and cheaper to conduct the sampling process.
- Subjective judgment: Non-probability sampling requires subjective judgment in selecting participants, which can introduce researcher bias and reduce the objectivity of the research findings.
- Less precision : Non-probability sampling generally provides less precision and accuracy compared to probability sampling methods, which may lead to lower statistical power and weaker inferential conclusions.
Advantages of Non-probability Sampling
Advantages of Non-probability Sampling are as follows:
- Easy to conduct: Non-probability sampling is relatively easy to conduct as it does not require a sampling frame or complex statistical calculations.
- Cost-effective: Non-probability sampling is usually less expensive than probability sampling methods as it does not require a large sample size or specialized equipment.
- Convenient: Non-probability sampling can be convenient as it allows researchers to select participants based on their availability or willingness to participate.
- More suitable for exploratory research : Non-probability sampling is more suitable for exploratory research where the focus is on generating new insights or hypotheses rather than making statistical inferences.
- Better for studying rare phenomena: Non-probability sampling can be more effective for studying rare or hard-to-reach populations, such as drug users or people with specific medical conditions, where a probability sample may be difficult to obtain.
- Allows for more diverse samples: Non-probability sampling can allow for a more diverse sample as it does not require strict randomization, allowing for the inclusion of participants who may not have been included in a probability sample.
Disadvantages of Non-probability Sampling
Disadvantages of Non-probability Sampling are as follows:
- Limited generalizability : Non-probability sampling does not provide a representative sample of the population, so the findings obtained from the sample may not be generalizable to the population as a whole.
- Difficulty in estimating sampling error : Non-probability sampling does not allow for the calculation of sampling error, which is the degree to which the sample estimates differ from the true population values.
- Difficult to replicate: Non-probability sampling can be difficult to replicate as the selection of participants is based on subjective criteria, making it challenging to obtain similar results in subsequent studies.
- Limited statistical power: Non-probability sampling generally provides less precision and accuracy compared to probability sampling methods, which may lead to lower statistical power and weaker inferential conclusions.
About the author
Muhammad Hassan
Researcher, Academic Writer, Web developer
You may also like

Probability Sampling – Methods, Types and...

Quota Sampling – Types, Methods and Examples

Sampling Methods – Types, Techniques and Examples

Simple Random Sampling – Types, Method and...

Convenience Sampling – Method, Types and Examples

Purposive Sampling – Methods, Types and Examples
- Skip to main content
- Skip to primary sidebar
- Skip to footer
- QuestionPro

- Solutions Industries Gaming Automotive Sports and events Education Government Travel & Hospitality Financial Services Healthcare Cannabis Technology Use Case NPS+ Communities Audience Contactless surveys Mobile LivePolls Member Experience GDPR Positive People Science 360 Feedback Surveys
- Resources Blog eBooks Survey Templates Case Studies Training Help center

Home Audience
Non-Probability Sampling: Types, Examples, & Advantages
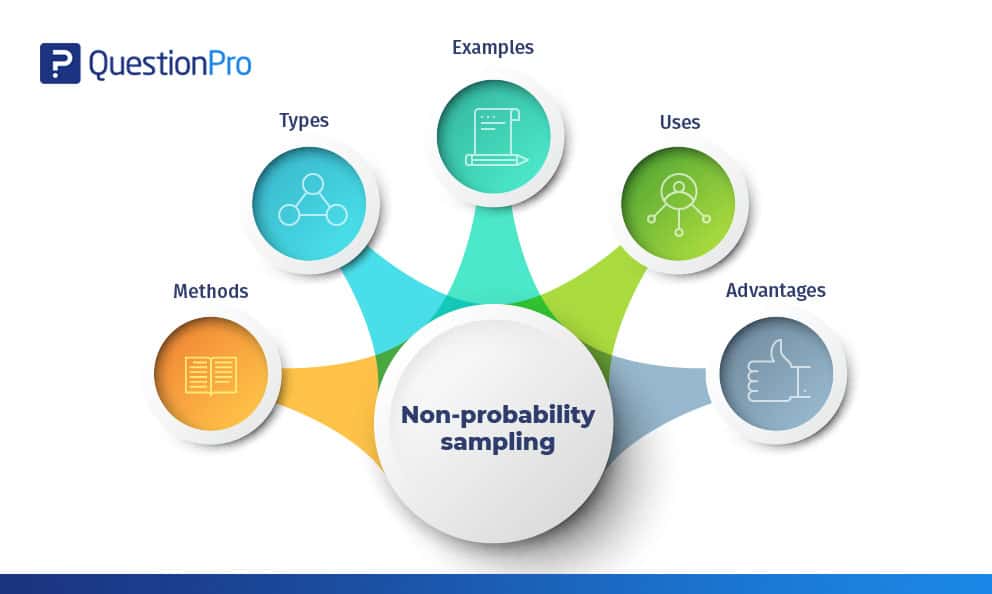
When we are going to do an investigation, and we need to collect data, we have to know the type of techniques we are going to use to be prepared. For this reason, there are two types of sampling : the random or probabilistic sample and the non-probabilistic one. In this case, we will talk in-depth about non-probability sampling. Keep reading!
What is non-probability sampling?
Definition: Non-probability sampling is defined as a sampling technique in which the researcher selects samples based on the subjective judgment of the researcher rather than random selection. It is a less stringent method. This sampling method depends heavily on the expertise of the researchers. It is carried out by observation, and researchers use it widely for qualitative research.
Non-probability sampling is a method in which not all population members have an equal chance of participating in the study, unlike probability sampling . Each member of the population has a known chance of being selected. Non-probability sampling is most useful for exploratory studies like a pilot survey (deploying a survey to a smaller sample compared to pre-determined sample size). Researchers use this method in studies where it is impossible to draw random probability sampling due to time or cost considerations.
LEARN ABOUT: Survey Sampling
Types of non-probability sampling
Here are the types of non-probability sampling methods:
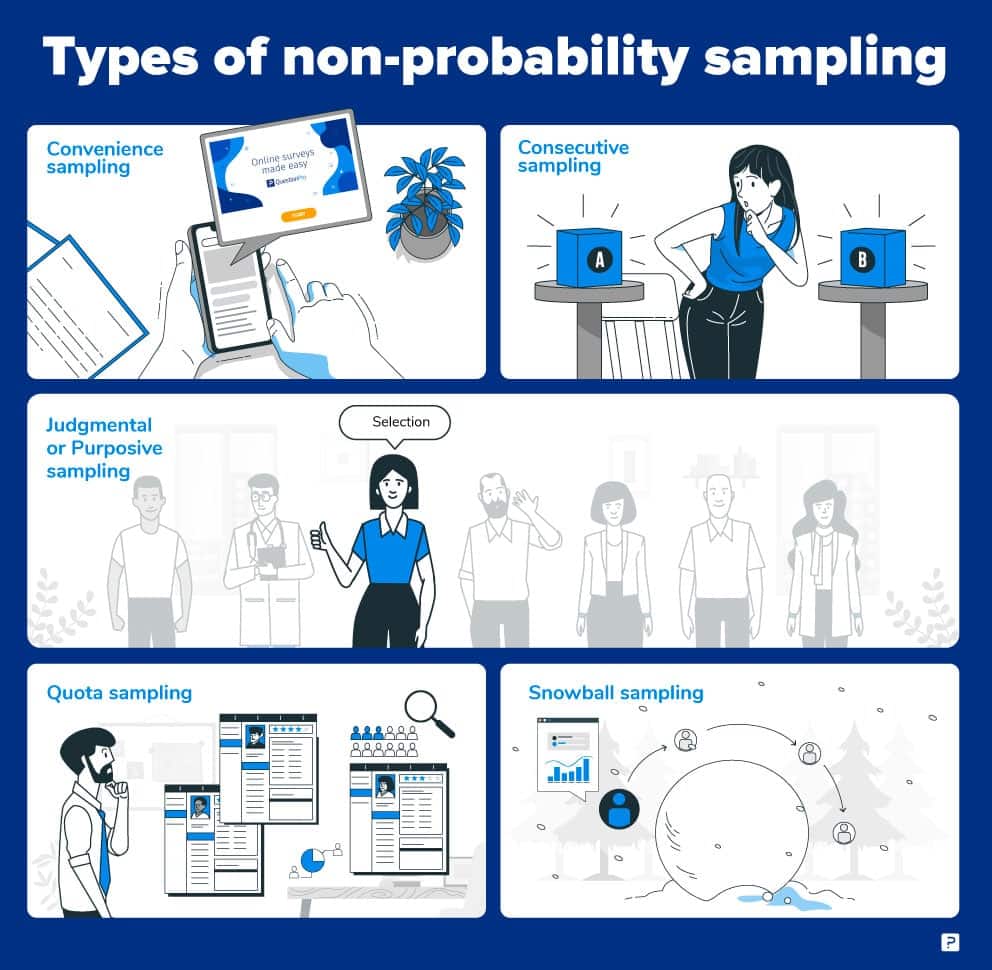
Convenience sampling
Convenience sampling is a non-probability sampling technique where samples are selected from the population only because they are conveniently available to the researcher. Researchers choose these samples just because they are easy to recruit, and the researcher did not consider selecting a sample that represents the entire population. Ideally, in research, it is good to test a sample that represents the population. But, in some research, the population is too large to examine and consider the entire population. It is one of the reasons why researchers rely on convenience sampling, which is the most common non-probability sampling method, because of its speed, cost-effectiveness, and ease of availability of the sample.
Consecutive sampling
This non-probability sampling method is very similar to convenience sampling, with a slight variation. Here, the researcher picks a single person or a group of a sample, conducts research over a period, analyzes the results, and then moves on to another subject or group if needed. Consecutive sampling technique gives the researcher a chance to work with many topics and fine-tune his/her research by collecting results that have vital insights.
Quota sampling
Hypothetically consider, a researcher wants to study the career goals of male and female employees in an organization. There are 500 employees in the organization, also known as the population. To understand better about a population, the researcher will need only a sample , not the entire population. Further, the researcher is interested in particular strata within the population. Here is where quota sampling helps in dividing the population into strata or groups.
Judgmental or Purposive sampling
In the judgmental sampling method, researchers select the samples based purely on the researcher’s knowledge and credibility. In other words, researchers choose only those people who they deem fit to participate in the research study. Judgmental or purposive sampling is not a scientific method of sampling, and the downside to this sampling technique is that the preconceived notions of a researcher can influence the results. Thus, this research technique involves a high amount of ambiguity.
Snowball sampling
Snowball sampling helps researchers find a sample when they are difficult to locate. Researchers use this technique when the sample size is small and not easily available. This sampling system works like the referral program. Once the researchers find suitable subjects, he asks them for assistance to seek similar subjects to form a considerably good size sample.
LEARN MORE: Simple Random Sampling
Non-probability sampling examples
Here are three simple examples of non-probability sampling to understand the subject better.
- An example of convenience sampling would be using student volunteers known to the researcher. Researchers can send the survey to students belonging to a particular school, college, or university, and act as a sample.
- In an organization, for studying the career goals of 500 employees, technically, the sample selected should have proportionate numbers of males and females. Which means there should be 250 males and 250 females. Since this is unlikely, the researcher selects the groups or strata using quota sampling.
- Researchers also use this type of sampling to conduct research involving a particular illness in patients or a rare disease. Researchers can seek help from subjects to refer to other subjects suffering from the same ailment to form a subjective sample to carry out the study.
When to use non-probability sampling?
- Use this type of sampling to indicate if a particular trait or characteristic exists in a population.
- Researchers widely use the non-probability sampling method when they aim at conducting qualitative research, pilot studies, or exploratory research.
- Researchers use it when they have limited time to conduct research or have budget constraints.
- When the researcher needs to observe whether a particular issue needs in-depth analysis , he applies this method.
- Use it when you do not intend to generate results that will generalize the entire population.
LEARN MORE: Population vs Sample
Advantages of non-probability sampling
Here are the advantages of using the non-probability technique
- Non-probability sampling techniques are a more conducive and practical method for researchers deploying surveys in the real world. Although statisticians prefer probability sampling because it yields data in the form of numbers, however, if done correctly, it can produce similar if not the same quality of results and avoid sampling errors .
- Getting responses using non-probability sampling is faster and more cost-effective than probability sampling because the sample is known to the researcher. The respondents respond quickly as compared to people randomly selected as they have a high motivation level to participate.
Select your respondents
Difference between non-probability sampling and probability sampling:
LEARN ABOUT: Sampling Frame
Sampling with QuestionPro Audience
Why restrict yourself to a limited population when you can access 22 million+ survey respondents around the globe? Every day, QuestionPro Audience enables researchers to collect actionable insights from pre-screened and mobile-ready respondents. Don’t let your survey receive research-biased answers. Good survey results are derived when the sample represents the population.
Now you know non-probability sampling is a great tool to extract information from a specific population. If you are a student or belong to a branch in which academic activities are developed, QuestionPro Audience is for you.
MORE LIKE THIS
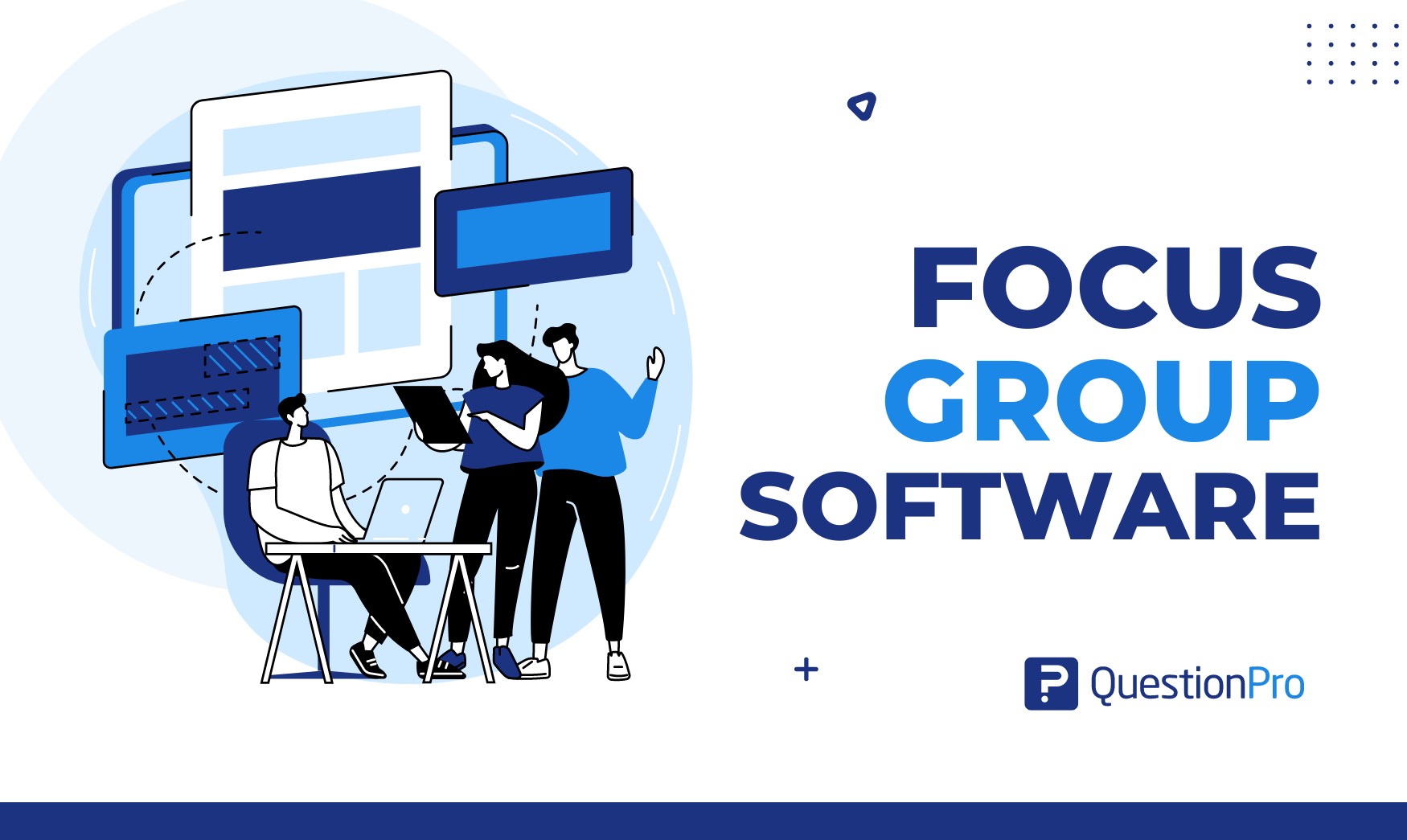
Top 7 Focus Group Software for Comprehensive Research
Apr 17, 2024
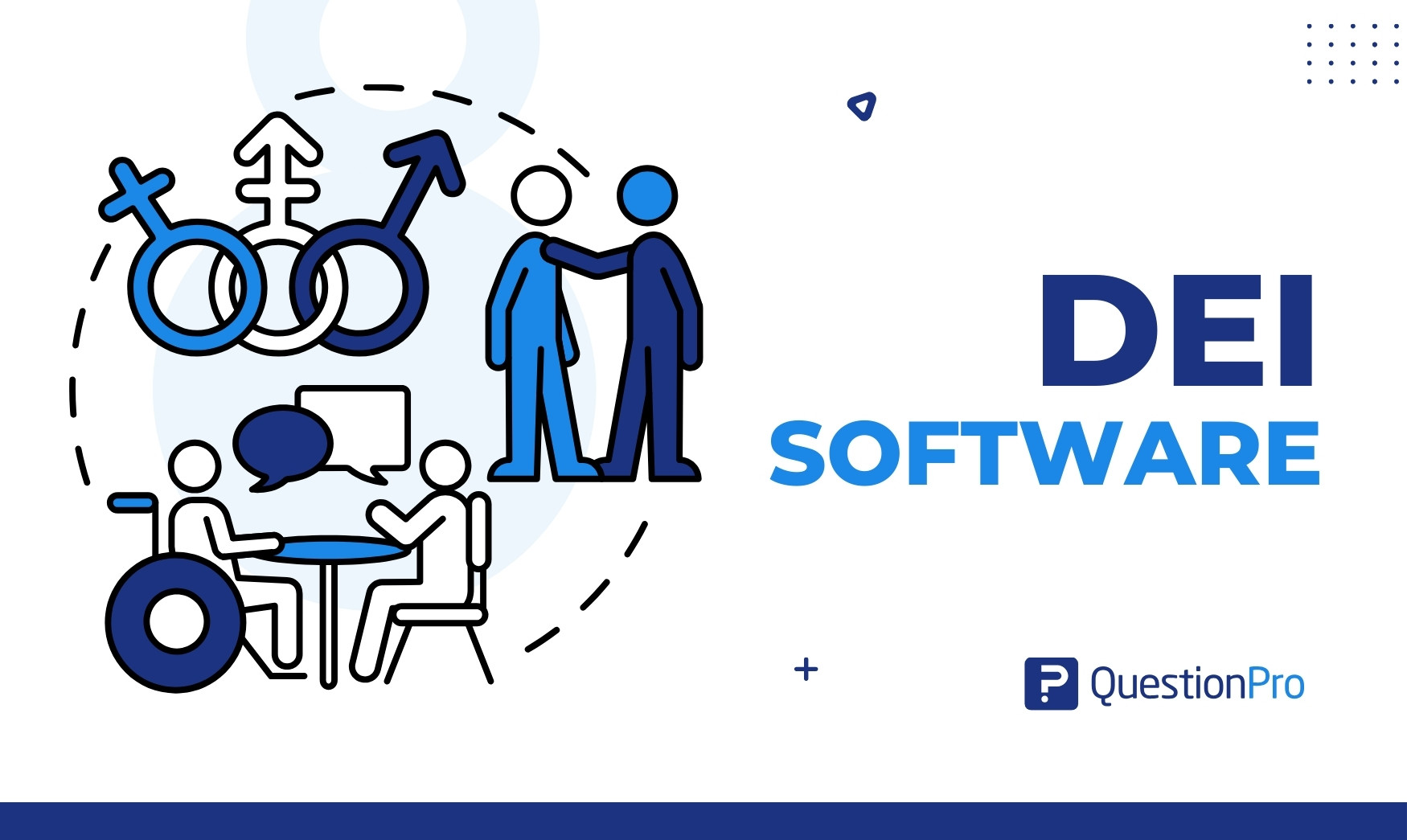
Top 7 DEI Software Solutions to Empower Your Workplace
Apr 16, 2024
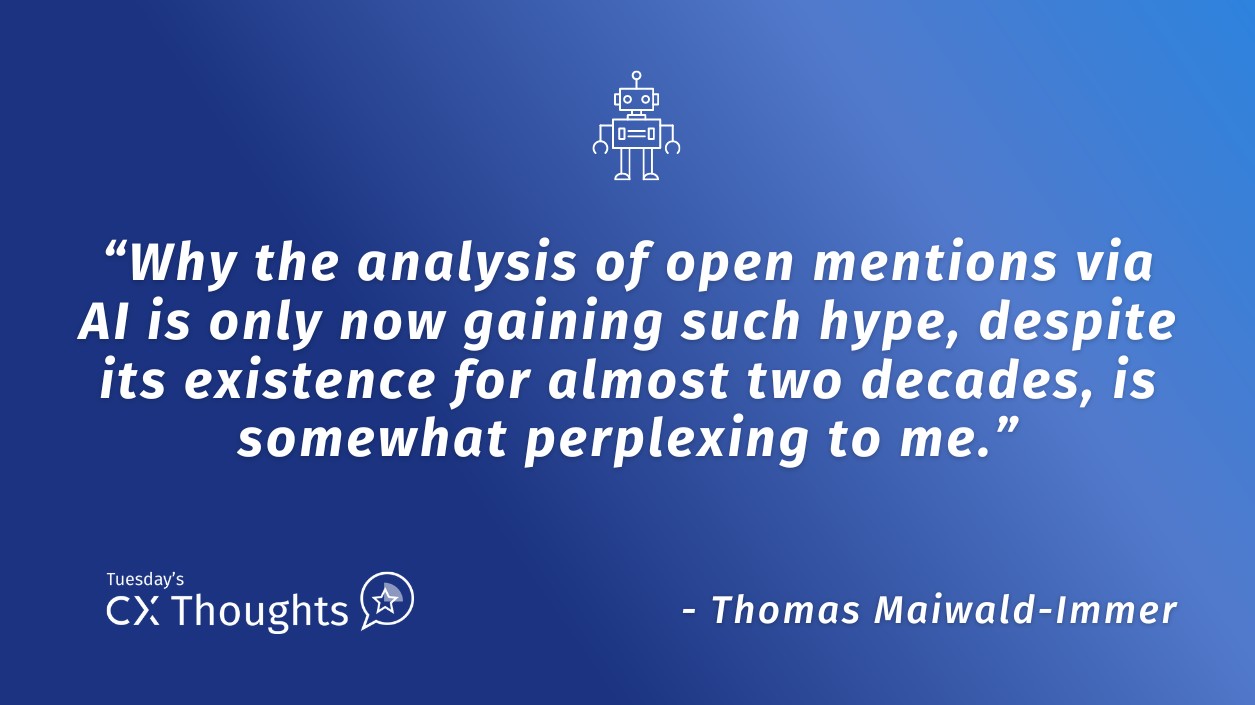
The Power of AI in Customer Experience — Tuesday CX Thoughts
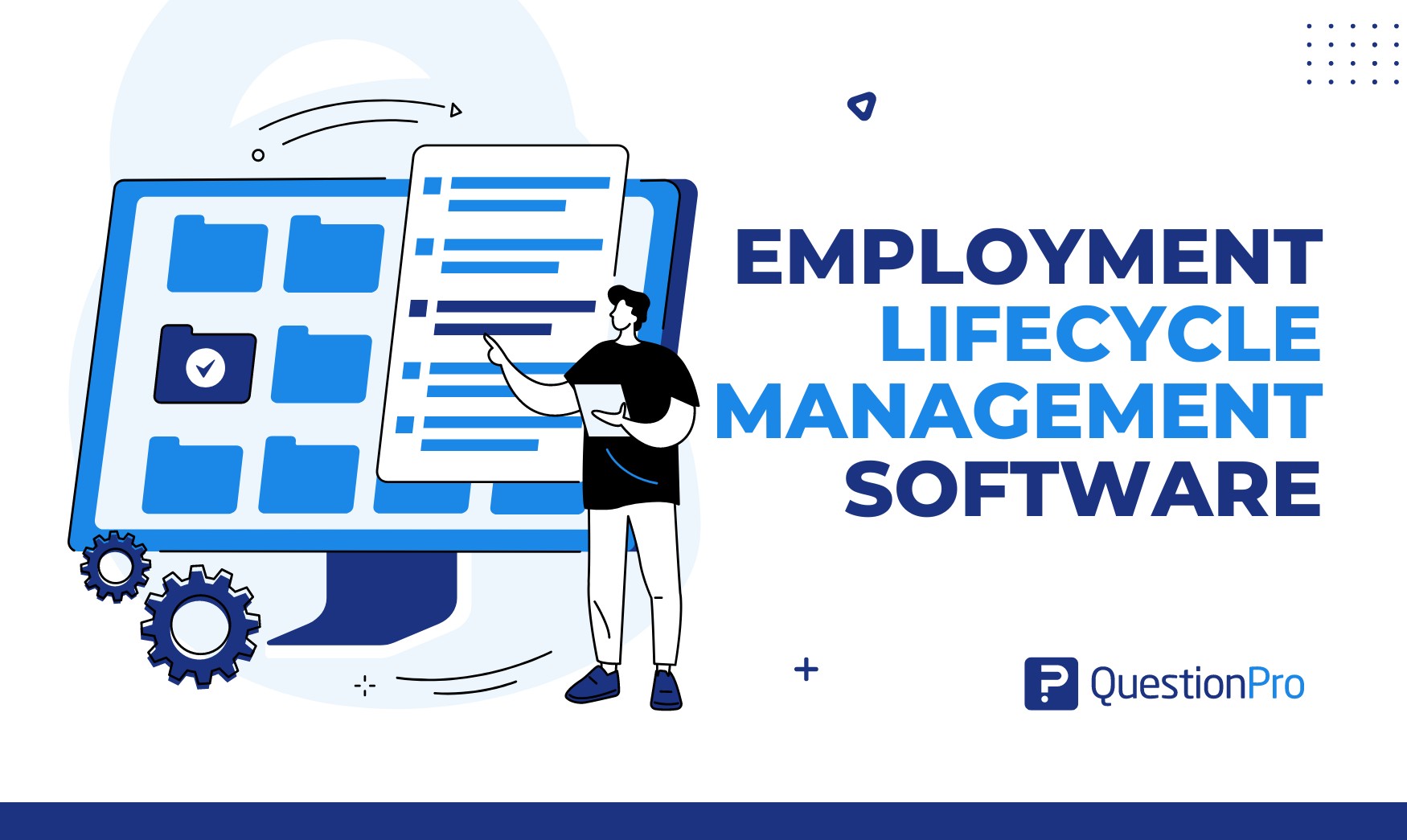
Employee Lifecycle Management Software: Top of 2024
Apr 15, 2024
Other categories
- Academic Research
- Artificial Intelligence
- Assessments
- Brand Awareness
- Case Studies
- Communities
- Consumer Insights
- Customer effort score
- Customer Engagement
- Customer Experience
- Customer Loyalty
- Customer Research
- Customer Satisfaction
- Employee Benefits
- Employee Engagement
- Employee Retention
- Friday Five
- General Data Protection Regulation
- Insights Hub
- Life@QuestionPro
- Market Research
- Mobile diaries
- Mobile Surveys
- New Features
- Online Communities
- Question Types
- Questionnaire
- QuestionPro Products
- Release Notes
- Research Tools and Apps
- Revenue at Risk
- Survey Templates
- Training Tips
- Uncategorized
- Video Learning Series
- What’s Coming Up
- Workforce Intelligence
Root out friction in every digital experience, super-charge conversion rates, and optimize digital self-service
Uncover insights from any interaction, deliver AI-powered agent coaching, and reduce cost to serve
Increase revenue and loyalty with real-time insights and recommendations delivered to teams on the ground
Know how your people feel and empower managers to improve employee engagement, productivity, and retention
Take action in the moments that matter most along the employee journey and drive bottom line growth
Whatever they’re are saying, wherever they’re saying it, know exactly what’s going on with your people
Get faster, richer insights with qual and quant tools that make powerful market research available to everyone
Run concept tests, pricing studies, prototyping + more with fast, powerful studies designed by UX research experts
Track your brand performance 24/7 and act quickly to respond to opportunities and challenges in your market
Explore the platform powering Experience Management
- Free Account
- For Digital
- For Customer Care
- For Human Resources
- For Researchers
- Financial Services
- All Industries
Popular Use Cases
- Customer Experience
- Employee Experience
- Employee Exit Interviews
- Net Promoter Score
- Voice of Customer
- Customer Success Hub
- Product Documentation
- Training & Certification
- XM Institute
- Popular Resources
- Customer Stories
- Market Research
- Artificial Intelligence
- Partnerships
- Marketplace
The annual gathering of the experience leaders at the world’s iconic brands building breakthrough business results, live in Salt Lake City.
- English/AU & NZ
- Español/Europa
- Español/América Latina
- Português Brasileiro
- REQUEST DEMO
- Experience Management
- Non-Probability sampling
Try Qualtrics for free
What is non-probability sampling everything you need to know.
17 min read Where can non-random sample selection be beneficial to your research? We explore non-probability sample types and explain how and why you might want to consider these for your next project.
What is non-probability sampling?
Non-probability sampling (sometimes nonprobability sampling) is a branch of sample selection that uses non-random ways to select a group of people to participate in research.
Unlike probability sampling and its methods , non-probability sampling doesn’t focus on accurately representing all members of a large population within a smaller sample group of participants. As a result, not all members of the population have an equal chance of participating in the study.
In fact, some research would deliver better results if non-probability sampling was used. For example, if you’re trying to access hard-to-reach social groups that aren’t usually visible, then a representative sample wouldn’t yield suitable candidates.
Instead, you may opt to select a sample based on your own reasons, including subjective judgment, sheer convenience, volunteers, or – in the above example – referrals from hidden members of society willing to speak out.
Free eBook: 2024 global market research trends report
When do you use non-probability sampling?
Non-probability sampling is typically used when access to a full population is limited or not needed, as well as in the following instances:
- You may want to gain the views of only a niche or targeted set of people, based on their location or characteristics . To ensure that there is plenty of data about the views of these specific people, it would make sense to have a sample full of people meeting the criteria.
- If there is a target market that you want to enter, it may be worthwhile doing a small pilot or exploratory research to see if new products and services are feasible to launch.
- If money and time are limited, non-probability sampling allows you to find sample candidates without investing a lot of resources.
- Where members are not represented traditionally in large populations or fly under the radar, like far-left and right-wing groups, it’s necessary to approach these subjects differently.
What’s the difference between non-probability and probability sampling?
Random vs deliberate choice.
Probability sampling, also known as random sampling , uses randomization rather than a deliberate choice to select a sample. This representative sample allows for statistical testing, where findings can be applied to the wider population in general.
What about non-probability sampling?
Non-probability sampling techniques, on the other hand, pick items or individuals for the sample based on your goals, knowledge, or experience . This eliminates the chance of users being picked at random but doesn’t offer the same bias-removal benefits as probability sampling.
Fair chance vs stacked chance
With probability sampling, there is an equal and fair chance of each member of the population being picked to be part of the smaller sample.
Conversely, in non-probability sampling, participants don’t have an equal chance of being selected. Instead, participants who hold desirable characteristics that fulfill your requirements are more likely to be selected.
Full knowledge of the population vs varying knowledge of the population
Probability sampling techniques require you to know who each member of the population is so that a representative sample size can be chosen. The population acts as the sampling frame — without it, creating a truly random sample can be difficult.
Non-probability sampling doesn’t need to know each member of the population before sampling. In some probability sampling methods, the sample grows on its own (snowballing) and sample participants can be sourced from one setting or location (convenience), irrespective of the total population.
Objectivity vs depth
Probability sampling aims to be objective in its sample selection method; it tries to remove bias by randomizing the selection and making it representative. Along with qualitative data , you’re more likely to get quantifiable data that can be scaled up to make models.
Non-probability sampling is the opposite, though it does aim to go deeper into one area, without consideration of the wider population. The level of detail in qualitative data will be greater, though any quantitative data is confined to the boundaries of that specific group and is hard to scale to other people in the population.
Faster to sample vs harder to sample
Probability sampling requires that a proportionate sample quota of representative yet diverse people be selected before the research can begin. This can be hard to do when response rates are low or there are no incentives to get involved.
Non-probability sampling avoids this problem. As the sample only needs to have the right amount of people before the research can begin, participant sourcing methods can be more creative and varied.
Also, as the ideal candidates will have similar traits, once you understand where to attract them from, you can repeat the process until you have the sample size you need.
Advantages of non-probability sampling
- This branch can be used where no sampling frame (full details of the total population) is known.
- It provides detailed descriptions about the sample in question, meaning if your research is interested in qualitative takeaways, non-probability sampling can provide you with more insights .
- It’s quick and cheap to carry out. You only need to invest a small amount of time to gather a sample size you feel is appropriate before you begin your research.
- It does not fall subject to low response rates — a common issue for probability sampling techniques.
- It can be a quick starting point to investigate or explore if there is an issue among a specific audience group or target market, leading to more investment or further research opportunities.
- The ability to connect with under-represented, hidden, or extreme groups makes this appealing for researchers interested in understanding niche viewpoints.
- In an online world, non-probability sampling becomes even easier to conduct, as the ability to connect with targeted sample members is faster and not constrained by physical geography.
- You and your researchers can react in real-time, meaning that analysis and research into world events can occur quicker.
Disadvantages of non-probability sampling
- The largest disadvantage would be the presence of sampling bias as the sample selection method gives an unfair advantage to certain members of a population.
- The results from non-probability sampling are not easily scaled up and used to make generalizations about the wider population.
- In some methods, such as volunteer or convenience sampling, samples can be filled with people who are more likely to agree to want to be part of research because they hold strong views that they want to share. This can skew the validity of the results.
- As you choose deliberate selection criteria to use to assess the suitability of participants for a sample, this can result in researcher or selection bias. Your views and opinions could influence the sample, which in turn, impacts the findings of the research.
- The sample may be subject to pre-screening checks or other hurdles that make it hard for some selected participants to get into the sample itself. This further adds complicated layers that could exclude suitable candidates from ending up in the sample.
- Since there is no way to measure the boundaries of a research-relevant population, the sample size is also unclear. You may find you need more participants or less at a later stage, which could waste time and money.
Types of non-probability sampling
There are four types of non-probability sampling techniques: convenience, quota, snowball and purposive — each of these sampling methods then have their own subtypes that provide different methods of analysis :
1. Convenience sampling (also called haphazard, grab, opportunity, or accidental sampling)
Convenience sampling is a common type of non-probability sampling where you choose participants for a sample, based on their convenience and availability.
You can see this type being used in public places, like malls or school campuses, where it’s easy to meet and select people as they ‘go by’ based on the characteristics and criteria that you think are important.
It is a cheap and quick way to collect people into a sample and run a survey to gather data. Because of this, it is usually used for quick user opinion polls or pilot testing.
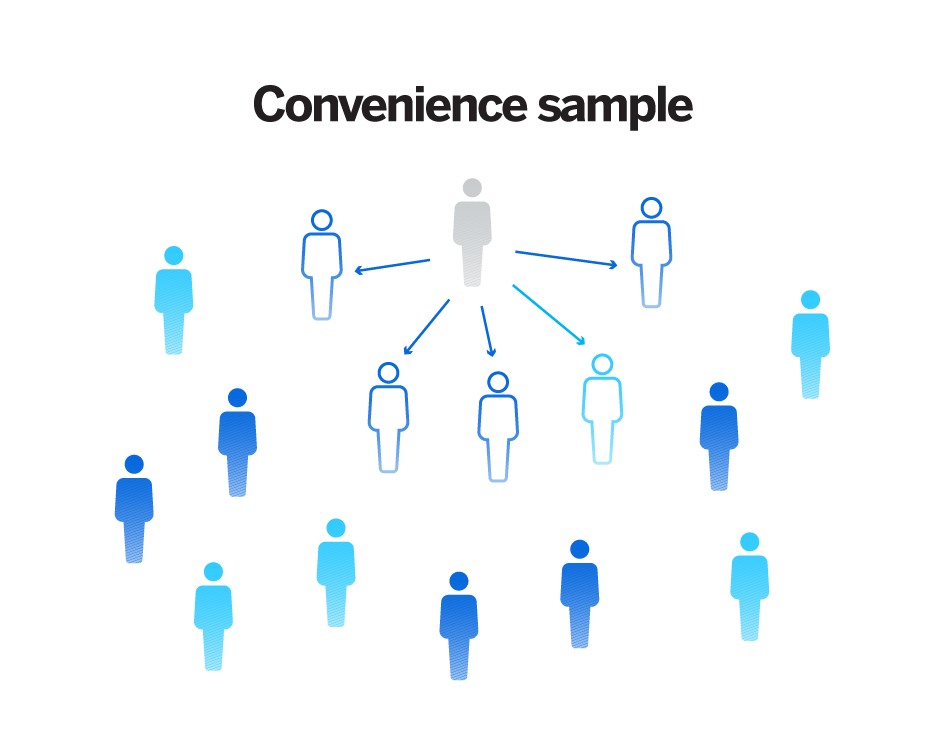
Convenience sampling also has two subtypes:
Consecutive sampling (also known as total enumerative sampling)
Consecutive sampling is the process of doing research with the sample members that meet the inclusion criteria and are conveniently available. You conduct research one after the other until you reach a conclusive result. Samples are chosen based on availability and each result is analyzed before you move onto the next sample or subject.
Self-selection (also known as volunteer sampling)
The self-selection sampling technique uses volunteers to fill in the sample size until it reaches a specified amount.
This requires less work contacting people, as volunteers sign up and opt-in to be part of the research if they meet your desired criteria. The insights gained will likely be based on strongly held opinions that these volunteers want to share. An example is medical research candidates that opt into medical studies because they fit the criteria of the research study and want to be involved for health reasons.
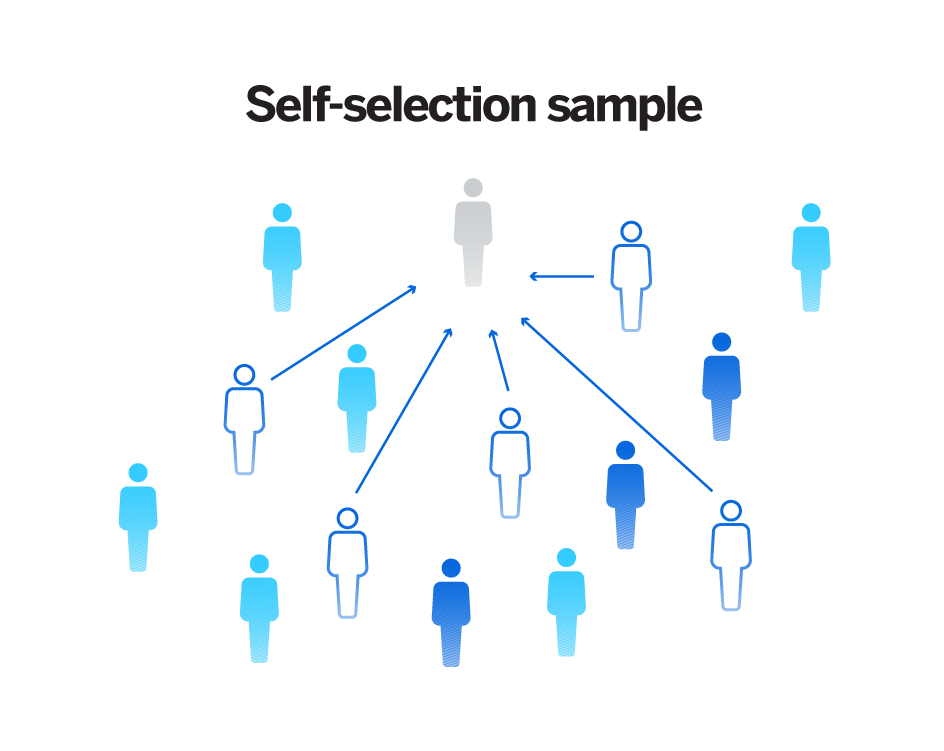
2. Quota sampling (also known as dimension sampling)
Quota sampling is a non-probability sampling technique similar to stratified sampling. In this method, the population is split into segments (strata) and you have to fill a quota based on people who match the characteristics of each stratum.
There are two types of quota sampling:
- Proportional quota sampling gives proportional numbers that represent segments in the wider population. For this, the population frame must be known.
- Non-proportional quota sampling uses stratum to divide a population, though only the minimum sample size per stratum is decided.
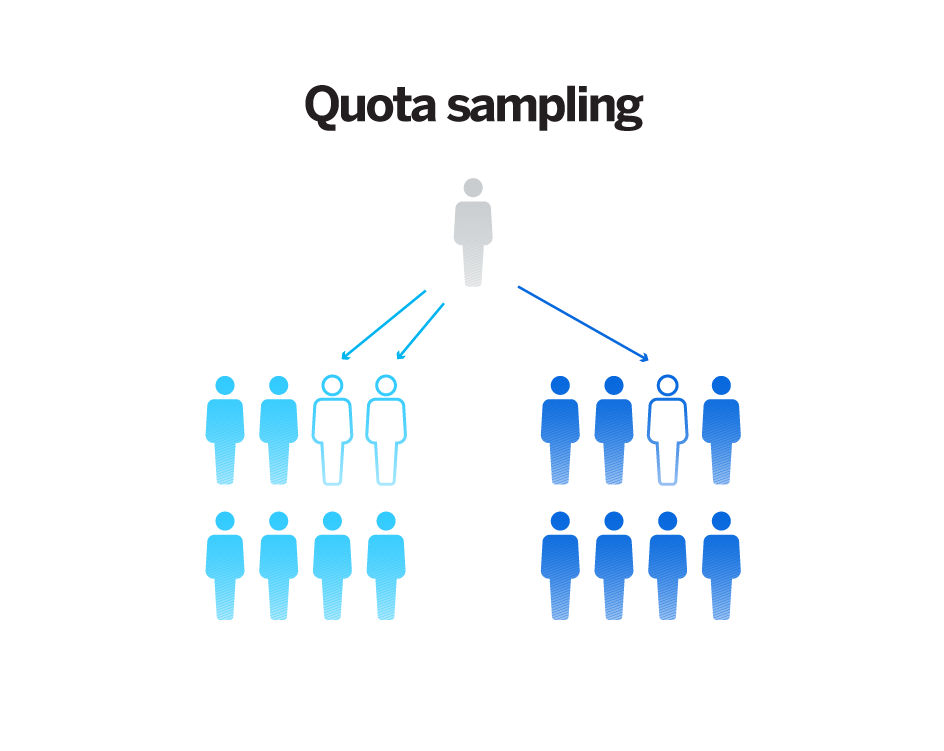
However, quota sampling techniques differ from probability-based sampling as there is no commitment from you to give an equal chance of participants being selected for the sample. Instead, you keep reaching out until the number in the stratum has been reached.
In general, quota sampling is conscious of the divisions in a population but still gives deep insights into each stratum.
3. Snowball sampling (also known as referral, respondent-driven, or chain referral sampling)
Snowball sampling is a non-probability sampling type that mimics a pyramid system in its selection pattern. You choose early sample participants, who then go on to recruit further sample participants until the sample size has been reached. This ongoing pattern can be perfectly described by a snowball rolling downhill: increasing in size as it collects more snow (in this case, participants).
This type of sampling is useful for getting in touch with hard-to-access communities of people, like sex workers, homeless people, or teenagers. An example of snowball sampling is recruiting sample members through social media channels who then promote your work to those in their network.
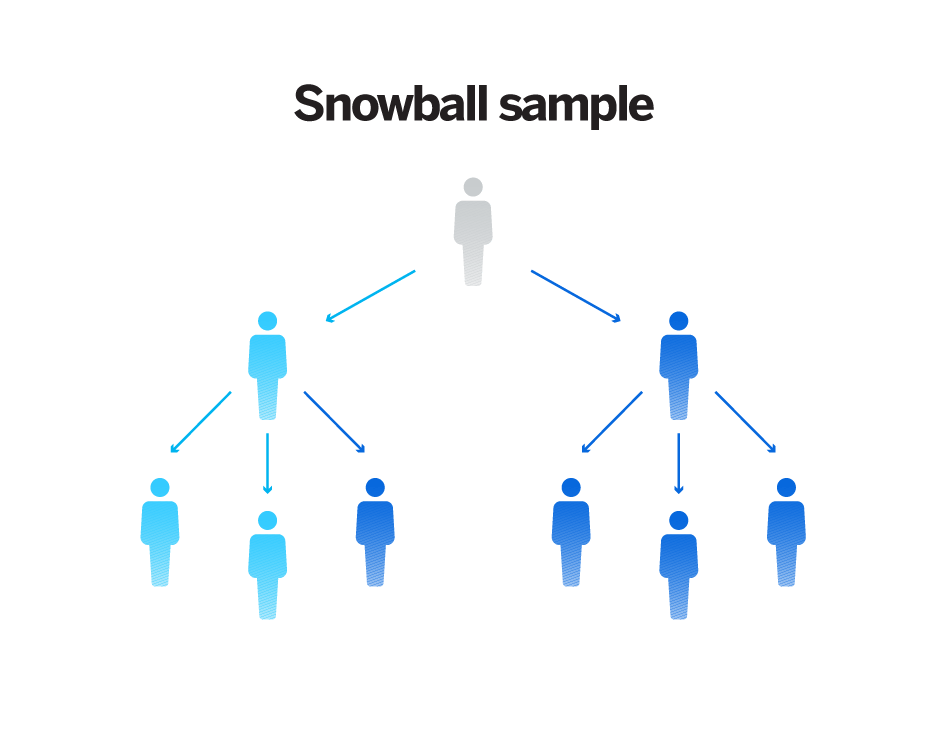
With this model, you are relying on who your initial sample members know to fulfill your ideal sample size . This can be quick to do when the chain of members develops past the first few levels. However, it does rely on the first members referring the research work to others.
Want to unlock more breakthrough insights? Use our research services and panels . With our proprietary online sample, you can get insights from any audience around the world and accurately track trends and shifts in your market over time.
4. Purposive sampling (also known as judgmental, selective, or subjective sampling)
Purposive sampling is a type of non-probability sampling where you make a conscious decision on what the sample needs to include and choose participants accordingly. In this way, you use your understanding of the research’s purpose and your knowledge of the population to judge what the sample needs to include to satisfy the research aims.
You must validate whether a prospective sample member fits the criteria you’re after, though if this is confirmed, the participant can be added to the sample. There are obvious bias issues with this type of sample selection method, though you have all the freedom to create the sample to fit the needs of your research.
That said, your credibility is at stake; even the smallest of mistakes can lead to incorrect data. However, because this is a fast and easy way to source a sample, you can redo the sample quite easily if there is a mistake.
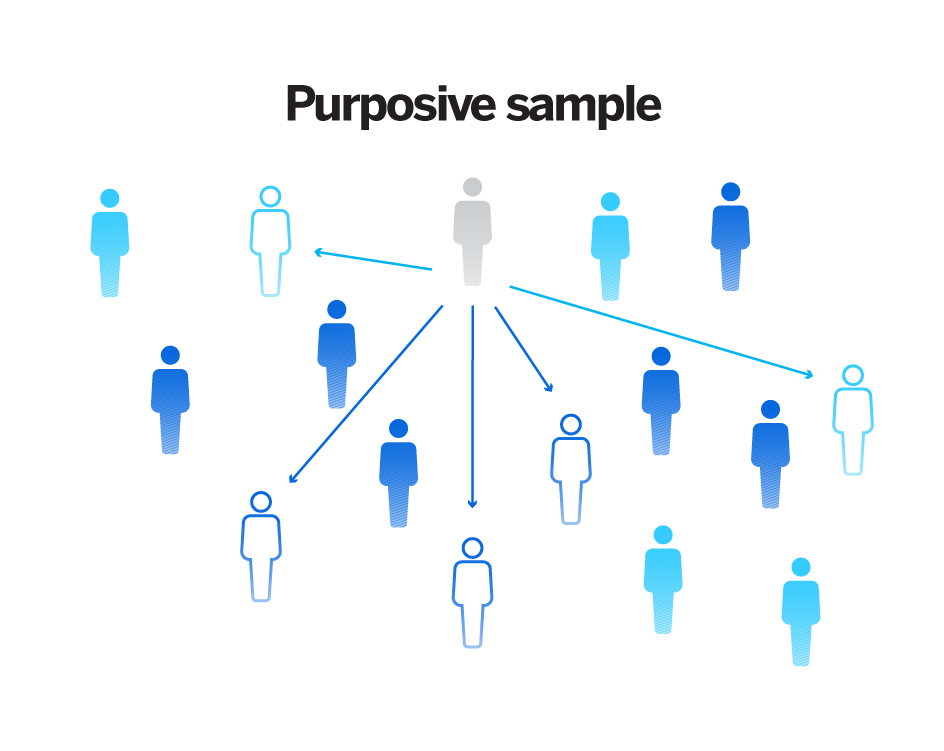
Some subtypes exist:
Heterogeneity sampling (also known as maximum variation sampling or sampling for diversity)
This is where you try to represent the widest range of views and opinions on the target topic of the research, regardless of proportional representation of the population. The main aims are to:
- make the research results as rich as they can be
- look at a topic from all perspectives
As such, having a broad spectrum of ideas from sample participants is key.
Homogeneous sampling (also known as modal instance sampling)
The opposite of heterogeneity sampling, homogenous sampling aims to get a sample of people who have similar or identical traits. For example, they might share the same views, beliefs, age, location, or employment. The traits selected are those that are useful to you in the research.
Instead of trying to see a topic from all angles, you focus on the research problem with a group of people who see it the same way and then go into detail.
Deviant sampling (also known as extreme sampling)
This is where you choose the sample based on cases or participant characteristics that are unusual or special in some way, such as outstanding successes or notable failures.
By allowing a group of non-traditional sample members to explore a topic, the insights will be unique and unpredictable, meaning that this could be valuable for ‘thinking outside the box’. Of course, you need to put in extra effort to find, connect and manage relationships with these sample members.
Expert sampling
When research goals call for a panel of specialists to help understand, discuss and elicit useful results, expert sampling could be useful. With expert sampling, the sample is chosen based on the knowledge of prospective sample members in a given area. This is best used in complex or highly technical research projects and where information is uncertain or unknown, though it can be used to validate other research findings by having an ‘expert’ vet the results.
Also, if you want to make sophisticated research easy, we can help. Just check out our solution that’s used by the world’s best brands to tackle research challenges and deliver the results that matter.
How to maximize your ROI
After reading through this guide, you should now have a better understanding of the different types of non-probability sampling techniques and how these sampling methods can be applied to your research.
But even with best practice, how can you maximize the ROI of the research that you do? With so much anxiety around financial and business health, many companies are reducing their research budgets and delaying projects. But with the speed at which consumers and employees are changing their behaviors, capturing insights and conducting targeted research has never been more important.
With access to real-time insights, you can empower your organization to make critical, data-driven decisions to drive breakthrough change. And this is where our eBook can help.
In it you’ll learn:
- How to improve research ROI through speed, agility, and consolidation
- Ways to get insights faster without sacrificing quality
- Tips for adjusting your research approach to be more nimble
Related resources
Stratified random sampling 12 min read, simple random sampling 9 min read, sampling methods 15 min read, how to determine sample size 12 min read, selection bias 11 min read, systematic random sampling 15 min read, convenience sampling 18 min read, request demo.
Ready to learn more about Qualtrics?
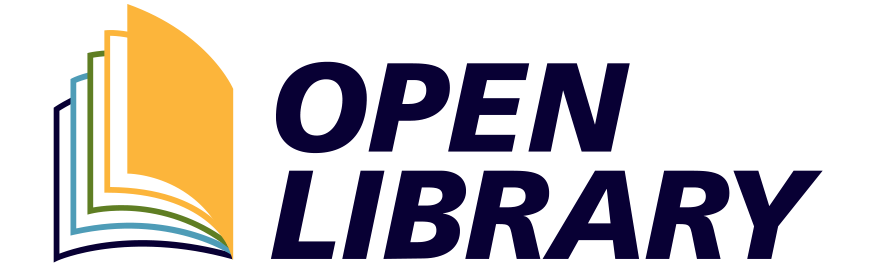
Want to create or adapt books like this? Learn more about how Pressbooks supports open publishing practices.
What constitutes an appropriate sample depends upon the research question(s), the research objectives, the researcher’s understanding of the phenomenon under study (developed through the literature review), and practical constraints (Palys & Atchison, 2014). These considerations will influence whether the researcher chooses to employ probabilistic or non-probabilistic sampling techniques. Probabilistic sampling techniques are employed to generate a formal or statistically representative sample. This technique is utilized when the researcher has a well-defined population to draw a sample from, as is often the case in quantitative research. This fact enables the researcher to generalize back to the broader population (Palys & Atchison, 2014). On the other hand, a non-probabilistic sampling technique is the method of choice when the population is not created equal and some participants are more desirable in advancing the research project´s objectives. Non-probability sampling techniques are the best approach for qualitative research. Because the researcher seeks a strategically chosen sample, generalizability is more of a theoretical or conceptual issue, and it is not possible to generalize back to the population (Palys & Atchison, 2014).
Probabilistic sampling techniques
As previously mentioned, probability sampling refers to sampling techniques for which a person’s (or event’s) likelihood of being selected for membership in the sample is known. You might ask yourself why we should care about a study element’s likelihood of being selected for membership in a researcher’s sample. The reason is that, in most cases, researchers who use probability sampling techniques are aiming to identify a representative sample from which to collect data. A representative sample is one that resembles the population from which it was drawn in all the ways that are important for the research being conducted. If, for example, you wish to be able to say something about differences between men and women at the end of your study, you must make sure that your sample doesn’t contain only women. That is a bit of an oversimplification, but the point with representativeness is that if your population varies in some way that is important to your study, your sample should contain the same sort of variation. While there is a formula to help you determine the sample size you will need to ensure representativeness, one of the easiest ways to do this is through an online sample size calculator. The calculator will do the work for you and tell you the minimum number of samples you will need in order to meet the desired statistical limitations (see https://www.calculator.net/sample-size-calculator.html )
Obtaining a representative sample is important in probability sampling because a key goal of studies that rely on probability samples is generalizability. In fact, generalizability is perhaps the key feature that distinguishes probability samples from nonprobability samples. Generalizability refers to the idea that a study’s results will tell us something about a group larger than the sample from which the findings were generated. In order to achieve generalizability, a core principle of probability sampling is that all elements in the researcher’s target population have an equal chance of being selected for inclusion in the study. In research, this is the principle of random selection. Random selection is a mathematical process that must meet two criteria. The first criterion is that chance governs the selection process. The second is that every sampling element has an equal probability of being selected (Palys & Atchison, 2014).
The core principal of probability sampling is random selection. If a researcher uses random selection techniques to draw a sample, he or she will be able to estimate how closely the sample represents the larger population from which it was drawn by estimating the sampling error.
Sampling error is the degree to which your sample deviates from the population’s characteristics. It is a statistical calculation of the difference between results from a sample and the actual parameters of a population. It is important to ensure that there is a minimum of sampling error (your sample needs to match the diversity of the population as closely as possible.) Sampling error comes from two main sources – systemic error and random error. Random error is due to chance, while systemic error means that there is some bias in the selection of the sample that makes particular individuals more likely to be selected than others. Here is an example to more fully explain the difference between a random and systemic error.
Example: Random and systemic errors
Consider the study of playground conditions for elementary school children. You would need a sampling frame (or list from which you sample) and select from that. Random sampling error would occur by chance and could not be controlled, but systemic error would be possible. Let us say that the list is designed in such a way that every 5th school is a private school. If you were to randomly sample every 5th school on the list, you would end up with a sample exclusively from private schools! Sampling error just means that an element of the population is more likely to be selected for the sample than another (in this case, the private schools are more likely to be sampled than the public schools).
Why is this discussion of error important? The use of the right techniques for sampling gives researchers the best chances at minimizing sampling error, and thus the strongest ability to say their results are reflective of the population. Research is done to benefit society in some way, so it is important that research results reflect what we might expect to see in society. Sample size also impacts sampling error. Generally, the bigger the sample, the smaller the error. However, there is a point of diminishing returns where only small reductions in error occur for increases in size. Cost and resources usually also prohibit very large samples, so ultimately the sample size is dependent upon a variety of factors, of which sampling error is only one Probability sampling techniques.
There are a variety of probability samples that researchers may use. For our purposes, we will focus on four: simple random samples, systematic samples, stratified samples, and cluster samples (see Table 6.1 for a summary of these four techniques). Simple random samples are the most basic type of probability sample, but their use is not particularly common. Part of the reason for this may be the work involved in generating a simple random sample. To draw a simple random sample, a researcher starts with a list of every single member, or element, of his or her population of interest. This list is sometimes referred to as a sampling frame . Once that list has been created, the researcher numbers each element sequentially and then randomly selects the elements from which he or she will collect data. To randomly select elements, researchers use a table of numbers that have been generated randomly. There are several possible sources for obtaining a random number table. Some statistics and research methods textbooks offer such tables as appendices to the text. Perhaps a more accessible source is one of the many free random number generators available on the Internet. A good online source is the website Stat Trek ( https://stattrek.com/ ), which contains a random number generator that you can use to create a random number table of whatever size you might need.
As you might have guessed, drawing a simple random sample can be quite tedious. S ystematic sampling techniques are somewhat less tedious but offer the benefits of a random sample. As with simple random samples, you must be able to produce a list of every one of your population elements. Once you have done that, to draw a systematic sample you would simply select every kth element on your list. But what is “k”, and where on the list of population elements does one begin the selection process? The symbol “k” is your selection interval or the distance between the elements you select for inclusion in your study. To begin the selection process, you would need to figure out how many elements you wish to include in your sample.
Let us say you want to interview 25 students from the Law program at your college or university. You do some research and discover that there are 150 students currently registered in the program. In this case, your selection interval, or k, is 6. To arrive at 6, simply divide the total number of population elements by your desired sample size. To determine where on your list of population elements to begin selecting the names of the 25 students you will interview, select a random number between 1 and k, and begin there. If we randomly select 3 as our starting point, we would begin by selecting the third student on the list and then select every sixth student from there.
There is one clear instance in which systematic sampling should not be employed. If your sampling frame has any pattern to it, you could inadvertently introduce bias into your sample by using a systemic sampling strategy. This is sometimes referred to as the problem of periodicity. Periodicity refers to the tendency for a pattern to occur at regular intervals. For example, suppose you want to observe how people use the outdoor public spaces in your city or town and you need to complete your observations within 28 days. During this time, you wish to conduct four observations on randomly chosen days. To determine which days you will conduct your observations, you will need to determine a selection interval. As you will recall from the preceding paragraphs, to do so you must divide your population size – in this case 28 days – by your desired sample size, in this case 4 days. This formula leads you to a selection interval of 7. If you randomly select 2 as your starting point and select every seventh day after that, you will wind up with a total of 4 days on which to conduct your observations. But what happens is that you are now observing on the second day of the week, being Tuesdays. As you have probably figured out, that is not such a good plan if you really wish to understand how public spaces in your city or town are used. Weekend use probably differs from weekday use, and that use may even vary during the week.
In cases such as this, where the sampling frame is cyclical, it would be better to use a stratified sampling technique . In stratified sampling, a researcher will divide the study population into relevant subgroups and then draw a sample from each subgroup. In this example, you might wish to first divide your sampling frame into two lists: weekend days and weekdays. Once you have your two lists, you can then apply either simple random or systematic sampling techniques to each subgroup.
Stratified sampling is a good technique to use when, as in the example, a subgroup of interest makes up a relatively small proportion of the overall sample. In the example of a study of use of public space in your city or town, you want to be sure to include weekdays and weekends in your sample. However, because weekends make up less than a third of an entire week, there is a chance that a simple random or systematic strategy would not yield sufficient weekend observation days. As you might imagine, stratified sampling is even more useful in cases where a subgroup makes up an even smaller proportion of the study population, say, for example, if you want to be sure to include both male and female perspectives in a study, but males make up only a small percentage of the population. There is a chance that simple random or systematic sampling strategy might not yield any male participants, but by using stratified sampling, you could ensure that your sample contained the proportion of males that is reflective of the larger population. Let us look at another example to help clarify things.
Example #1 Choosing a sampling technique
Suppose a researcher wanted to talk to police officers in Canada about their views on illegal drug use in the general population. A researcher could find a list of all Canadian police officers (a sampling frame) and do a simple random sample or a systematic sample with random start from that list. But what if the researcher wanted to ensure that female and male officers were included in the same proportions they are in the population of officers? Or if they wanted to ensure that urban and rural officers are represented as they are in the population of police? In these cases, stratified random sampling might be more appropriate. If the goal is to have the subgroups reflect the proportions in the population then proportional stratification should be used. With proportional stratification, the sample size of each subgroup is proportionate to the population size of the group. In other words, each subgroup has the same sampling fraction. The sampling fraction is the proportion of the population that the researcher wants included in the sample. It is equal to the sample size, divided by the population size (n/N) (see Palys & Atchison, 2014).
However, if the researcher wants to be able to compare male and female officers or rural and urban officers (or a more complicated concept: male and female officers within the rural and urban areas), a disproportional stratification may be used instead to ensure that the researcher has enough members of the subgroups to allow between group comparisons. With a disproportional sample, the size of the each sample subgroup does not need to be proportionate to the population size of the group. In other words, two or more strata will have different sampling fractions (see Palys & Atchison, 2014).
Up to this point in our discussion of probability samples, we have assumed that researchers will be able to access a list of population elements in order to create a sampling frame. This, as you might imagine, is not always the case. Let us say, for example, that you wish to conduct a study of bullying in high schools across Canada. Just imagine trying to create a list of every single high school student in the country. Even if you could find a way to generate such a list, attempting to do so might not be the most practical use of your time or resources. When this is the case, researchers turn to cluster sampling. Cluster sampling occurs when a researcher begins by sampling groups (or clusters) of population elements and then selects elements from within those groups. Here is an example of when a cluster sampling technique would be suitable:
Example #2 – Cluster sampling
Perhaps you are interested in the workplace experiences of college instructors. Chances are good that obtaining a list of all instructors that work for Canadian colleges would be rather difficult. You would be more likely, without too much hassle, to come up with a list of all colleges in Canada. Consequently, you could draw a random sample of Canadian colleges (your cluster) and then draw another random sample of elements (in this case, instructors) from within the colleges you initially selected. Cluster sampling works in stages. In this example we sampled in two stages. As you might have guessed, sampling in multiple stages does introduce the possibility of greater error (each stage is subjected to its own sampling error), but it is nevertheless a highly efficient method.
Now suppose colleges across the country were not willing to share their instructor lists? How might you sample then? Is it important that the instructors in your study are representative of all instructors? What happens if you need a representative sample, but you do not have a sampling frame? In these cases, multi-stage cluster sampling may be appropriate. This complex form of cluster sampling involves dividing the population into groups (or clusters ). The researcher chooses one or more clusters at random and samples everyone within the chosen cluster (see Palys & Atchison, 2014).
Table 7.1 Four Types of Probability Samples
Nonprobability Sampling Techniques.
Nonprobability sampling refers to sampling techniques for which a person’s (or event’s or researcher’s focus) likelihood of being selected for membership in the sample is unknown. Because we do not know the likelihood of selection, we do not know whether or not a nonprobability sample represents a larger population. Representing the population is not the goal with nonprobability samples, however the fact that nonprobability samples do not represent a larger population does not mean that they are drawn arbitrarily or without any specific purpose in mind. The following subsection, “Types of Nonprobability Samples,” examines more closely the process of selecting research elements when drawing a nonprobability sample. But first, let us consider why a researcher might choose to use a nonprobability sample.
One instance might be at the design stage of a research project. For example, if you are conducting survey research, you may want to administer the survey to a few people who seem to resemble the people you are interested in studying in order to help work out kinks in the survey. You might also use a nonprobability sample at the early stages of a research project if you are conducting a pilot study or exploratory research. Researchers also use nonprobability samples in full-blown research projects. These projects are usually qualitative in nature, where the researcher’s goal is in-depth, idiographic understanding rather than more general, nomothetic1 understanding. Evaluation researchers whose aim is to describe some very specific small group might use nonprobability sampling techniques. Researchers interested in contributing to our theoretical understanding of a phenomenon might also collect data from nonprobability samples. Researchers interested in contributing to social theories, by either expanding on them, modifying them, or poking holes in their propositions, might use nonprobability sampling techniques to seek out cases that seem anomalous in order to understand how theories can be improved.
In sum, there are many instances in which the use of nonprobability samples makes sense. The next subsection will examine several specific types of nonprobability samples.
Nonprobability sampling techniques
Researchers use several types of nonprobability samples, including: purposive samples, snowball samples, quota samples, and convenience samples. While the latter two strategies may be used by quantitative researchers from time to time, they are more typically employed in qualitative research; because they are both nonprobability methods, we include them in this section of the chapter.
To draw a purposive sample, researchers begin with specific perspectives that they wish to examine in mind, and then seek out research participants who cover that full range of perspectives. For example, if you are studying students’ level of satisfaction with their college or university program of study, you must include students from all programs, males and females, students of different ages, students who are working and those who are not, students who are studying online and those who are taking classes face-to-face, as well as past and present. While purposive sampling is often used when one’s goal is to include participants who represent a broad range of perspectives, purposive sampling may also be used when a researcher wishes to include only people who meet very narrow or specific criteria.
Qualitative researchers sometimes rely on snowball sampling techniques to identify study participants. In this case, a researcher might know of one or two people he or she would like to include in the study, but then relies on those initial participants to help identify additional study participants. Thus, the researcher’s sample builds and becomes larger as the study continues, much as a snowball builds and becomes larger as it rolls through the snow. Snowball sampling is an especially useful strategy when a researcher wishes to study some stigmatized group or behaviour. Having a previous participant vouch for the trustworthiness of the researcher may help new potential participants feel more comfortable about being included in the study. Snowball sampling is sometimes referred to as chain referral sampling. One research participant refers another, and that person refers another, and that person refers another—thus a chain of potential participants is identified. In addition to using this sampling strategy for potentially stigmatized populations, it is also a useful strategy to use when the researcher’s group of interest is likely to be difficult to find, not only because of some stigma associated with the group, but also because the group may be relatively rare.
When conducting quota sampling, a researcher identifies categories that are important to the study and for which there is likely to be some variation. Subgroups are created based on each category and the researcher decides how many people (or documents or whatever element happens to be the focus of the research) to include from each subgroup and collects data from that number for each subgroup. While quota sampling offers the strength of helping the researcher account for potentially relevant variation across study elements, we must remember that such a strategy does not yield statistically representative findings. And while this is important to note, it is also often the case that we do not really care about a statistically representative sample, because we are only interested in a specific case.
Let us go back to a previous example of student satisfaction with their college or university course of study, to look at an example of how a quota sampling approach would work in such a study.
Imagine you want to understand how student satisfaction varies across two types programs: the Emergency Services Management (ESM) degree program and the ESM diploma program. Perhaps you have the time and resources to interview 40 ESM students. Since you are interested in comparing the degree and the diploma program, you decide to interview 20 students from each program. In your review of literature on the topic before you began the study, you learned that degree and diploma experiences can vary by age of the students. Consequently, you decide on four important subgroup: males who are 29 years of age or younger, females who are 29 years of age or younger, males who are 30 years of age or older, and females who are thirty years of age or older. Your findings would not be representative of all students who enroll in degree or diploma programs at the college, or at other institutions; however, this is irrelevant to your purposes since you are solely interested in finding out about the satisfaction level of ESM students who are enrolled in either the ESM degree or diploma program.
Finally, convenience sampling is another nonprobability sampling strategy that is employed by both qualitative and quantitative researchers. To draw a convenience sample, a researcher simply collects data from those people or other relevant elements to which he or she has most convenient access. This method, also sometimes referred to as haphazard sampling, is most useful in exploratory research. It is also often used by journalists who need quick and easy access to people from their population of interest. If you have ever seen brief interviews of people on the street on the news, you have probably seen a haphazard sample being interviewed. While convenience samples offer one major benefit—convenience—we should be cautious about generalizing from research that relies on convenience sampling.
The following table provides a summary of the main differences between probability and non-probability sampling.
You will recall in Section 6.2 we discussed random assignment, which is different than random sampling. The following matrix will help differentiate the two.
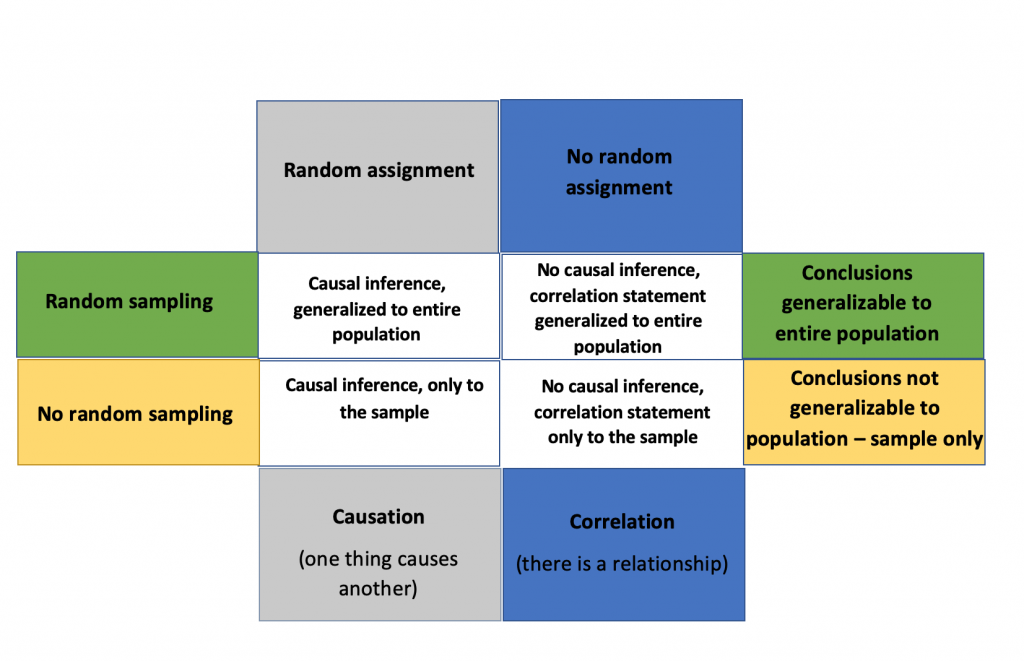
Adapted from Cetinkaya-Rundel, M. (n.d.). Random sampling vs. assignment. Retrieved ffrom https://www2.stat.duke.edu/courses/Fall12/sta101.001/resources/lecturettes/random_sample_assignment.pdf
A Word of Caution about Sampling: Questions to Ask about Samples
We read and hear about research results so often that we might overlook the need to ask important questions about where research participants come from and how they are identified for inclusion in a research project. It is easy to focus only on findings when we are busy and when the really interesting stuff is in a study’s conclusion, not its procedures. Now that you have some familiarity with the variety of procedures for selecting study participants, you are equipped to ask some very important questions about the findings you read, and to be a more responsible consumer of research.
Research Methods, Data Collection and Ethics Copyright © 2020 by Valerie Sheppard is licensed under a Creative Commons Attribution-NonCommercial-ShareAlike 4.0 International License , except where otherwise noted.
Share This Book
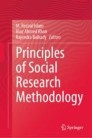
Principles of Social Research Methodology pp 415–426 Cite as
Sampling Techniques for Qualitative Research
- Heather Douglas 4
- First Online: 27 October 2022
2588 Accesses
2 Citations
This chapter explains how to design suitable sampling strategies for qualitative research. The focus of this chapter is purposive (or theoretical) sampling to produce credible and trustworthy explanations of a phenomenon (a specific aspect of society). A specific research question (RQ) guides the methodology (the study design or approach ). It defines the participants, location, and actions to be used to answer the question. Qualitative studies use specific tools and techniques ( methods ) to sample people, organizations, or whatever is to be examined. The methodology guides the selection of tools and techniques for sampling, data analysis, quality assurance, etc. These all vary according to the purpose and design of the study and the RQ. In this chapter, a fake example is used to demonstrate how to apply your sampling strategy in a developing country.
This is a preview of subscription content, log in via an institution .
Buying options
- Available as PDF
- Read on any device
- Instant download
- Own it forever
- Available as EPUB and PDF
- Compact, lightweight edition
- Dispatched in 3 to 5 business days
- Free shipping worldwide - see info
- Durable hardcover edition
Tax calculation will be finalised at checkout
Purchases are for personal use only
Douglas, H. (2010). Divergent orientations in social entrepreneurship organisations. In K. Hockerts, J. Robinson, & J. Mair (Eds.), Values and opportunities in social entrepreneurship (pp. 71–95). Palgrave Macmillan.
Chapter Google Scholar
Douglas, H., Eti-Tofinga, B., & Singh, G. (2018a). Contextualising social enterprise in Fiji. Social Enterprise Journal, 14 (2), 208–224. https://doi.org/10.1108/SEJ-05-2017-0032
Article Google Scholar
Douglas, H., Eti-Tofinga, B., & Singh, G. (2018b). Hybrid organisations contributing to wellbeing in small Pacific island countries. Sustainability Accounting, Management and Policy Journal, 9 (4), 490–514. https://doi.org/10.1108/SAMPJ-08-2017-0081
Douglas, H., & Borbasi, S. (2009). Parental perspectives on disability: The story of Sam, Anna, and Marcus. Disabilities: Insights from across fields and around the world, 2 , 201–217.
Google Scholar
Douglas, H. (1999). Community transport in rural Queensland: Using community resources effectively in small communities. Paper presented at the 5th National Rural Health Conference, Adelaide, South Australia, pp. 14–17th March.
Douglas, H. (2006). Action, blastoff, chaos: ABC of successful youth participation. Child, Youth and Environments, 16 (1). Retrieved from http://www.colorado.edu/journals/cye
Douglas, H. (2007). Methodological sampling issues for researching new nonprofit organisations. Paper presented at the 52nd International Council for Small Business (ICSB) 13–15 June, Turku, Finland.
Draper, H., Wilson, S., Flanagan, S., & Ives, J. (2009). Offering payments, reimbursement and incentives to patients and family doctors to encourage participation in research. Family Practice, 26 (3), 231–238. https://doi.org/10.1093/fampra/cmp011
Puamua, P. Q. (1999). Understanding Fijian under-achievement: An integrated perspective. Directions, 21 (2), 100–112.
Download references
Author information
Authors and affiliations.
The University of Queensland, The Royal Society of Queensland, Activation Australia, Brisbane, Australia
Heather Douglas
You can also search for this author in PubMed Google Scholar
Corresponding author
Correspondence to Heather Douglas .
Editor information
Editors and affiliations.
Centre for Family and Child Studies, Research Institute of Humanities and Social Sciences, University of Sharjah, Sharjah, United Arab Emirates
M. Rezaul Islam
Department of Development Studies, University of Dhaka, Dhaka, Bangladesh
Niaz Ahmed Khan
Department of Social Work, School of Humanities, University of Johannesburg, Johannesburg, South Africa
Rajendra Baikady
Rights and permissions
Reprints and permissions
Copyright information
© 2022 The Author(s), under exclusive license to Springer Nature Singapore Pte Ltd.
About this chapter
Cite this chapter.
Douglas, H. (2022). Sampling Techniques for Qualitative Research. In: Islam, M.R., Khan, N.A., Baikady, R. (eds) Principles of Social Research Methodology. Springer, Singapore. https://doi.org/10.1007/978-981-19-5441-2_29
Download citation
DOI : https://doi.org/10.1007/978-981-19-5441-2_29
Published : 27 October 2022
Publisher Name : Springer, Singapore
Print ISBN : 978-981-19-5219-7
Online ISBN : 978-981-19-5441-2
eBook Packages : Social Sciences
Share this chapter
Anyone you share the following link with will be able to read this content:
Sorry, a shareable link is not currently available for this article.
Provided by the Springer Nature SharedIt content-sharing initiative
- Publish with us
Policies and ethics
- Find a journal
- Track your research
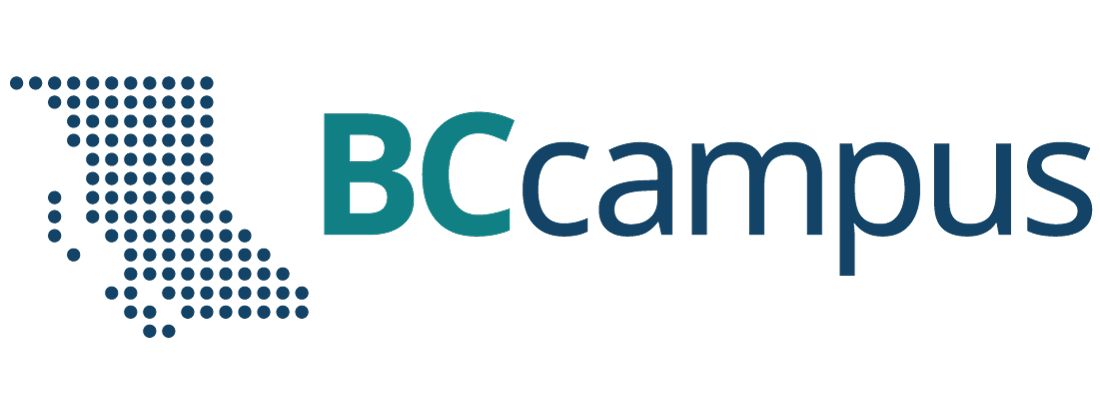
Want to create or adapt books like this? Learn more about how Pressbooks supports open publishing practices.
Sampling Techniques
39 Probabilistic and Non-Probabilistic Sampling Techniques
What constitutes an appropriate sample depends upon the research question(s), the research objectives, the researchers understanding of the phenomenon under study (developed through the literature review), and practical constraints (Palys & Atchison, 2014). These considerations will influence whether the researcher chooses to employ probabilistic or non-probabilistic sampling techniques. Probabilistic sampling techniques are employed to generate a formal or statistically representative sample. This technique is utilized when the researcher has a well-defined population to draw a sample from, as is often the case in quantitative research. This fact enables the researcher to generalize back to the broader population (Palys & Atchison, 2014). On the other hand, a non-probabilistic sampling technique is the method of choice when the population is not created equal and some participants are more desirable in advancing the research project´s objectives. Non-probability sampling techniques are the best approach for qualitative research. Because the researcher seeks a strategically chosen sample, generalizability is more of a theoretical or conceptual issue, and it is not possible to generalize back to the population (Palys & Atchison, 2014). In the following sections we will look at the types of sampling methods utilized with non-probabilistic and probabilistic samples.
Probabilistic sampling techniques
As previously mentioned, probability sampling refers to sampling techniques for which a person’s (or event’s) likelihood of being selected for membership in the sample is known. You might ask yourself why we should care about a study element’s likelihood of being selected for membership in a researcher’s sample. The reason is that, in most cases, researchers who use probability sampling techniques are aiming to identify a representative sample from which to collect data. A representative sample is one that resembles the population from which it was drawn in all the ways that are important for the research being conducted. If, for example, you wish to be able to say something about differences between men and women at the end of your study, you better make sure that your sample doesn’t contain only women. That is a bit of an oversimplification, but the point with representativeness is that if your population varies in some way that is important to your study, your sample should contain the same sorts of variation.
Obtaining a representative sample is important in probability sampling because a key goal of studies that rely on probability samples is generalizability. In fact, generalizability is perhaps the key feature that distinguishes probability samples from nonprobability samples. Generalizability refers to the idea that a study’s results will tell us something about a group larger than the sample from which the findings were generated. In order to achieve generalizability, a core principle of probability sampling is that all elements in the researcher’s target population have an equal chance of being selected for inclusion in the study. In research, this is the principle of random selection. Random selection is a mathematical process that must meet two criteria. The first criterium is that chance governs the section process. The second is that every sampling element has an equal probability of being selected (Palys & Atchison, 2014). The core principal of probability sampling is random selection. If a researcher uses random selection techniques to draw a sample, he or she will be able to estimate how closely the sample represents the larger population from which it was drawn by estimating the sampling error.
Sampling error is the degree to which your sample deviates from the population’s characteristics. It is a statistical calculation of the difference between results from a sample and the actual parameters of a population. It is important to ensure that there is a minimum of sampling error (your sample needs to match the diversity of the population as closely as possible.) Sampling error comes from two main sources – systemic error and random error. Random error is due to chance, while systemic error means that there is some bias in the selection of the sample that makes particular individuals more likely to be selected than others. Here is an example to more fully explain the difference between a random and systemic error.
Example: Random and systemic errors
Consider the study of playground conditions for the elementary school children. You would need a sampling frame (or list from which you sample) and select from that. Random sampling error would occur by chance and cannot be controlled. But systemic error would be a situation such as this. Let us say that the list is designed in such a way that every 5th school is a private school. If you were to randomly sample every 5th school on the list, you would end up with all private schools! Sampling error just means that an element of the population is more likely to be selected for the sample than another (in this case, the private schools are more likely to be sampled than the public schools).
Why is all of this error talk important? The use of the right techniques for sampling gives researchers the best chances at minimizing sampling error and thus the strongest ability to say their results are reflective of the population. Research is done to benefit society, in some way, so this is important that research results be reflective of what we might expect to see in society. Sample size also impacts sampling error. Generally, the bigger the sample is, the smaller the error. However, there is a point of diminishing returns where only small reductions in error are given for increases in size. Cost and resources usually also prohibit very large samples, so ultimately the sample size is dependent upon a variety of factors of which sampling error is only one.

Probability sampling techniques
There are a variety of probability samples that researchers may use. For our purposes we will focus on four: simple random samples, systematic samples, stratified samples, and cluster samples (see Table 6.1 for a summary of these four techniques). Simple random samples are the most basic type of probability sample, but their use is not particularly common. Part of the reason for this may be the work involved in generating a simple random sample. To draw a simple random sample, a researcher starts with a list of every single member, or element, of his or her population of interest. This list is sometimes referred to as a sampling frame . Once that list has been created, the researcher numbers each element sequentially and then randomly selects the elements from which he or she will collect data. To randomly select elements, researchers use a table of numbers that have been generated randomly. There are several possible sources for obtaining a random number table. Some statistics and research methods textbooks offer such tables as appendices to the text. Perhaps a more accessible source is one of the many free random number generators available on the Internet. A good online source is the website Stat Trek , which contains a random number generator that you can use to create a random number table of whatever size you might need.
As you might have guessed, drawing a simple random sample can be quite tedious. S ystematic sampling techniques are somewhat less tedious but offer the benefits of a random sample. As with simple random samples, you must be able to produce a list of every one of your population elements. Once you have done that, to draw a systematic sample you would simply select every kth element on your list. But what is k, and where on the list of population elements does one begin the selection process? k is your selection interval or the distance between the elements you select for inclusion in your study. To begin the selection process, you would need to figure out how many elements you wish to include in your sample.
Let us say you want to interview 25 students from the law program at your college or university. You do some research and discover that there are 150 students currently registered in the program. In this case, your selection interval, or k, is 6. To arrive at 6, simply divide the total number of population elements by your desired sample size. To determine where on your list of population elements to begin selecting the names of the 25 students you will interview, select a random number between 1 and k, and begin there. If we randomly select 3 as our starting point, we would begin by selecting the third student on the list and then select every fourth student from there.
There is one clear instance in which systematic sampling should not be employed. If your sampling frame has any pattern to it, you could inadvertently introduce bias into your sample by using a systemic sampling strategy. This is sometimes referred to as the problem of periodicity. Periodicity refers to the tendency for a pattern to occur at regular intervals. For example, suppose you wanted to observe how people use the outdoor public spaces in your city or town and you need to have your observations completed within 28 days. During this time, you wish to conduct four observations on randomly chosen days. To determine which days you will conduct your observations, you will need to determine a selection interval. As you will recall from the preceding paragraphs, to do so you must divide your population size – in this case 28 days – by your desired sample size, in this case 4 days. This formula leads you to a selection interval of 7. If you randomly select 2 as your starting point and select every seventh day after that, you will wind up with a total of 4 days on which to conduct your observations. But what happens is that you are now observing on the second day of the week, being Tuesdays. As you have probably figured out, that is not such a good plan if you really wish to understand how public spaces in your city or town are used. Weekend use probably differs from weekday use, and that use may even vary during the week.
In cases such as this, where the sampling frame is cyclical, it would be better to use a stratified sampling technique . In stratified sampling, a researcher will divide the study population into relevant subgroups and then draw a sample from each subgroup. In this example, you might wish to first divide your sampling frame into two lists: weekend days and weekdays. Once you have your two lists, you can then apply either simple random or systematic sampling techniques to each subgroup.
Stratified sampling is a good technique to use when, as in the example, a subgroup of interest makes up a relatively small proportion of the overall sample. In the example of a study of use of public space in your city or town, you want to be sure to include weekdays and weekends in your sample. However, because weekends make up less than a third of an entire week, there is a chance that a simple random or systematic strategy would not yield sufficient weekend observation days. As you might imagine, stratified sampling is even more useful in cases where a subgroup makes up an even smaller proportion of the study population, say, for example, if you want to be sure to include both male and female perspectives in a study, but males make up only a small percentage of the population. There is a chance simple random or systematic sampling strategy might not yield any male participants, but by using stratified sampling, you could ensure that your sample contained the proportion of males that is reflective of the larger population. Let us look at another example to help clarify things.
Example #1 Choosing a sampling technique
Suppose a researcher wanted to talk to police officers in Canada about their views on illegal drugs use in general population. A researcher could find a list of all police officers (a sampling frame) and do a simple random sample or a systematic sample with random start from that list. But what if the researcher wanted to ensure that female and male officers were included in the same proportions they are in the population of officers? Ot if they wanted to ensure that urban and rural officers are represented as they are in the population of police? In these cases, stratified random sampling might be more appropriate. If the goal is to have the subgroups reflect the proportions in the population then proportional stratification should be used. With proportional stratification, the sample size of each subgroup is proportionate to the population size of the group.In other words, each subgroup has the same sampling fraction. The sampling fraction is the proportion of the population that the researcher wants included in the sample. It is equal to the sample size, divided by the population size (n/N) (see Palys & Atchison, 2014).
However, if the researcher wanted to be able to compare male and female officer or rural and urban officers (or more complicated: male and female officers within the rural and urban areas), a disproportional stratification may be used instead to ensure that the researcher had enough members of the subgroups to allow between group comparisons. With a disproportional sample, the size of the each sample subgroup does not need to be proportionate to the population size of the group. In other words, two or more stratum will have different sampling fractions (see Palys & Atchison, 2014)
Up to this point in our discussion of probability samples, we have assumed that researchers will be able to access a list of population elements in order to create a sampling frame. This, as you might imagine, is not always the case. Let us say, for example, that you wish to conduct a study of bullying in high schools across Canada. Just imagine trying to create a list of every single high school student in the country. Basically, we are talking about a list of every high school student in the country. Even if you could find a way to generate such a list, attempting to do so might not be the most practical use of your time or resources. When this is the case, researchers turn to cluster sampling. Cluster sampling occurs when a researcher begins by sampling groups (or clusters) of population elements and then selects elements from within those groups. Here is an example of when a cluster sampling technique would be suitable.
Example #2 Cluster sampling
Perhaps you are interested in the workplace experiences of college instructors. Chances are good that obtaining a list of all instructors that work for Canadian colleges would be rather difficult. It would be more likely that you can come up with a list of all colleges inCanada without too much hassle. Consequently, you could dram a random sample of Canadian colleges (your cluster) and then draw another random sample of elements (in this case, instructors) from within the colleges you initially selected. Cluster sampling works in stages. In this sample, we sampled in two stages. As you might have guessed, sampling in multiple stages does introduce the possibility of greater error (each stage is subjected to its own sampling error), but it is nevertheless a highly efficient method.
Now suppose college across the country were not willing to share their instructor lists? How might you sample then? Is it important that the instructors in you study are representative of all instructors? What happens if you need a representative sample, but you do not have a sampling frame? In these cases, multi-stage cluster sampling may be appropriate. This complex form if cluster sampling involves dividing the population into groups (or clusters ). The researcher chooses one or more clusters ar random and everyone within the chosen cluster is sampled (see Palys & Atchison, 2014)
Nonprobability Sampling Techniques
Nonprobability sampling refers to sampling techniques for which a person’s (or event’s or researcher’s focus’) likelihood of being selected for membership in the sample is unknown. Because we do not know the likelihood of selection, we do not know with nonprobability samples whether a sample represents a larger population or not. But that is okay, because representing the population is not the goal with nonprobability samples. That said, the fact that nonprobability samples do not represent a larger population does not mean that they are drawn arbitrarily or without any specific purpose in mind. In the following subsection, “Types of Nonprobability Samples,” we will take a closer look at the process of selecting research elements when drawing a nonprobability sample. But first, let us consider why a researcher might choose to use a nonprobability sample.
One instance might be when we are designing a research project. For example, if we are conducting survey research, we may want to administer our survey to a few people who seem to resemble the people we are interested in studying in order to help work out kinks in the survey. We might also use a nonprobability sample at the early stages of a research project, if we are conducting a pilot study or exploratory research. Researchers also use nonprobability samples in full-blown research projects. These projects are usually qualitative in nature, where the researcher’s goal is in-depth, idiographic understanding rather than more general, nomothetic [1] understanding. Evaluation researchers whose aim is to describe some very specific small group might use nonprobability sampling techniques. Researchers interested in contributing to our theoretical understanding of some phenomenon might also collect data from nonprobability samples. Researchers interested in contributing to social theories, by either expanding on them, modifying them, or poking holes in their propositions, may use nonprobability sampling techniques to seek out cases that seem anomalous in order to understand how theories can be improved.
In sum, there are a number and variety of instances in which the use of nonprobability samples makes sense. We will examine several specific types of nonprobability samples in the next subsection.
Types of nonprobability samples
There are several types of nonprobability samples that researchers use. These include purposive samples, snowball samples, quota samples, and convenience samples. While the latter two strategies may be used by quantitative researchers from time to time, they are more typically employed in qualitative research, and because they are both nonprobability methods, we include them in this section of the chapter.
To draw a purposive sample , researchers begin with specific perspectives in mind that they wish to examine and then seek out research participants who cover that full range of perspectives. For example, if you are studying students’ level of satisfaction with their college or university program of study, you will want to be sure to include students from all programs, males and females, students of different ages, students who are working and those who are not, students who are studying online and those who are taking classes face-to-face, as well as past and present students (which would allow you to compare past and present students’ level of satisfaction). While purposive sampling is often used when one’s goal is to include participants who represent a broad range of perspectives, purposive sampling may also be used when a researcher wishes to include only people who meet very narrow or specific criteria.
Qualitative researchers sometimes rely on snowball sampling techniques to identify study participants. In this case, a researcher might know of one or two people h would like to include in his study but then relies on those initial participants to help identify additional study participants. Thus, the researcher’s sample builds and becomes larger as the study continues, much as a snowball builds and becomes larger as it rolls through the snow. Snowball sampling is an especially useful strategy when a researcher wishes to study some stigmatized group or behaviour. Having a previous participant vouch for the trustworthiness of the researcher may help new potential participants feel more comfortable about being included in the study. Snowball sampling is sometimes referred to as chain referral sampling. One research participant refers another, and that person refers another, and that person refers another—thus a chain of potential participants is identified. In addition to using this sampling strategy for potentially stigmatized populations, it is also a useful strategy to use when the researcher’s group of interest is likely to be difficult to find, not only because of some stigma associated with the group, but also because the group may be relatively rare.
When conducting quota sampling , a researcher identifies categories that are important to the study and for which there is likely to be some variation. Subgroups are created based on each category and the researcher decides how many people (or documents or whatever element happens to be the focus of the research) to include from each subgroup and collects data from that number for each subgroup. While quota sampling offers the strength of helping the researcher account for potentially relevant variation across study elements, we must remember that such a strategy does not yield statistically representative findings. And while this is important to note, it is also often the case that we do not really care about a statistically representative sample, because we are only interested in a specific case.
Let us go back to the example we considered previously of student satisfaction with their college or university course of study to look at an example of how a quota sampling approach would work in such a study.
Imagine you want to understand how student satisfaction varies across two types programs: the Emergency Services Management (ESM) degree program and the ESM diploma program. Perhaps you have the time and resources to interview 40 ESM students. As you are interested in comparing the degree and the diploma program, you decide to interview 20 from each program. In your review of literature on the topic before you began the study, you learned that degree and diploma experiences can vary by age of the students. Consequently, you decide on four important subgroup: males who are 29 years of age or younger, females who are 29 years of age or younger, males who are 30 years of age and older, and females who are thirty years of age and older. Your findings would not be representative of all students who enrol in degree or diploma programs at the college, or at other institution; however, this is irrelevant to your purposes as are solely interested in finding out about the satisfaction level of ESM students who are enrolled in either the ESM degree or diploma program.
Finally, convenience sampling is another nonprobability sampling strategy that is employed by both qualitative and quantitative researchers. To draw a convenience sample, a researcher simply collects data from those people or other relevant elements to which he or she has most convenient access. This method, also sometimes referred to as haphazard sampling, is most useful in exploratory research. It is also often used by journalists who need quick and easy access to people from their population of interest. If you have ever seen brief interviews of people on the street on the news, you have probably seen a haphazard sample being interviewed. While convenience samples offer one major benefit—convenience—we should be cautious about generalizing from research that relies on convenience sample.
Text Attributions
- This chapter is an adaptation of Chapter 7 in Principles of Sociological Inquiry , which was adapted by the Saylor Academy without attribution to the original authors or publisher, as requested by the licensor. © Creative Commons Attribution-NonCommercial-ShareAlike 3.0 License .
- idiographic understanding means the researcher attempts to explain or exhaustively describe the phenomenon under study. While the researcher might have to sacrifice some breadth of understanding, s/he will gain a much deeper, richer understanding of the phenomenon or group understudy. Whereas nomothetic understanding means the research attempts to provide a more general, sweeping explanation or description of the topic. In this case, depth of understanding may be sacrificed in favour of breadth of understanding. ↵
An Introduction to Research Methods in Sociology Copyright © 2019 by Valerie A. Sheppard is licensed under a Creative Commons Attribution-NonCommercial-ShareAlike 4.0 International License , except where otherwise noted.
Share This Book
Non-probability sampling: what it is and how to do it right
Last updated
14 May 2023
Reviewed by
Miroslav Damyanov
The process of choosing individuals to participate in a survey or an experiment is known as sampling. Getting the right sample requires careful thought and planning, as there are lots of ways to design, distribute, and collect data from surveys and experiments in ways that make extrapolating useful insights difficult, if not impossible.
Make research less tedious
Dovetail streamlines research to help you uncover and share actionable insights
- What is non-probability sampling?
Sampling can be categorized as either probability or non-probability sampling.
In probability sampling, you randomly select participants from your population, with every participant having an equal chance of being selected. In non-probability sampling, you choose non-random criteria upon which to base your sampling choices from a larger population—not everybody gets a chance at being selected.
There are four main subtypes of non-probability sampling (and variations of these subtypes) that researchers can commonly use in business and academic settings.
Convenience sampling
Convenience sampling involves engaging participants that are most convenient for you to access. For example, suppose you're looking to survey people's political opinions about a topic and decide to go door-to-door in your neighborhood to ask questions. In that case, you'd be creating a convenience sample.
One type of convenience subtype is consecutive sampling, in which a researcher first gathers their convenience sample and engages in research. When they complete it, they continue to recruit and engage other respondents who fit the study's screening criteria, forming secondary and tertiary convenience samples and studying them consecutively.
Purposive sampling
Also known as judgmental sampling, purposive sampling is a method by which a sample is selected based purely on the researcher’s knowledge and credibility. Several types of purposive sampling exist, including:
Critical case sampling
In critical case sampling, you're making a judgment call about which small group of participants or cases are most important to the study of your subject.
Deviant case sampling
Here, you're looking for the most extreme case representing a particular subject you're trying to study.
Expert sampling
When you engage in expert sampling, you gather a sample of those individuals with the greatest expertise relevant to your subject.
Homogenous sampling
If you're investigating an attribute that a group has in common, you may wish to assemble a group that strongly resembles each other in one or more aspects.
Maximum variation sampling
To look at a subject from all possible perspectives, you build a sample that’s as diverse as possible.
Typical case sampling
In a typical case sample, you're looking for participants who exemplify what the average subject would look like when it comes to a particular subject or phenomenon.
Quota sampling
Quota sampling involves selecting a sample representative of the population from which you're trying to collect feedback. For example, suppose you're surveying an audience of sports fans, a third of whom like teams A, B, and C, respectively. No matter how many people you choose to sample, you'd draw participants equally from the three different groups of sports fans.
However, it's important to note that in quota sampling, you're not randomly drawing participants from different subgroups. You're using some non-random attribute(s), such as proximity to you, to determine who participates.
Snowball sampling
Snowball sampling involves members of hard-to-reach populations. In such a sample, you start by engaging one member of this population willing to engage in your survey or experiment and ask them to introduce you to others in their group. Typically, researchers who've studied indigenous populations with little outside contact with the developed world must use this sampling method.
- What are the benefits and drawbacks of probability sampling and non-probability sampling?
Before determining which non-probability sampling method to use, it's important to understand what the difference between probability and non-probability sampling means for your research. As stated above, in probability sampling, you're randomly drawing participants from a population. When you do so, you're eliminating many forms of bias that may be found in the results.
Probability sampling doesn’t remove all forms of bias from a research project . For example, you could inadvertently exclude members from your research if the list of individuals you sample (your sampling frame) differs from the population. But there are far fewer potential biases when you use a probability sample than when you use a non-probability one.
However, probability sampling takes more effort and time than most non-probability samples. For example, say you're surveying a population and your sample includes 1,000 individuals, but only ten percent respond to your initial inquiry. To complete the research, you would need to spend time and money tracking down and encouraging the remaining participants to respond.
When you use a non-probability sample, you may find it easier to recruit willing participants. If you offer $5 coupons in a high-traffic area to participate in a survey, your results may not greatly reflect local area attitudes. But chances are you'll have a high participation rate.
With non-probability sampling, there are many forms of bias you may introduce to your study, including:
Healthy user bias
In studies regarding health and health interventions, healthy users are more likely to opt-in to these studies. This overrepresentation will skew results if the sampling frame isn’t weighted appropriately.
Non-response bias
If many respondents fail to participate and you form your conclusions based on those who do, the absence of those participants may skew the results.
Pre-screening bias
You may also introduce bias into a study based on how you pre-screen participants. If you advertise a study about weight loss, you may attract more people who are motivated to lose weight than the general population.
Self-selection bias
Respondents opting into particular studies may share characteristics that skew the data. For example, marijuana enthusiasts may volunteer to take a survey about attitudes toward marijuana at higher rates than members of the population at large, which may skew the results.
Undercoverage bias
Some population members are less likely to participate due to logistical issues. You might have difficulty recruiting participants in rural areas with inconsistent Internet access, resulting in under coverage of certain population segments.
Despite the risks of introducing biases in your research, there are many instances when using non-probability sampling rather than probability samples makes sense.
- When should you use non-probability sampling?
The best way to determine which sampling method to use is to examine your study and determine your desired outcomes. For example, if you're looking to study participants who typically don't respond to studies, you may have to resort to snowball sampling by necessity. Or, say you need to obtain feedback from a population, but only those with a specific attribute provide detailed feedback. You may want to oversample from that group to get the practical insights you need.
If you choose to use non-probability samples, you'll want to minimize the biases you introduce to the study to the greatest extent possible. Make sure that your screening process, research description, and questions don't create biases and skew results.
Oversample certain subgroups to avoid under-coverage. And make sure you spend appropriate time and resources recruiting participants to ensure that you attract the number of participants and level of engagement you need for your study.
- What are some examples of non-probability sampling?
Many common examples of non-probability sampling can be found in our day-to-day lives. Whenever you receive a customer feedback survey on a receipt, a company uses non-probability sampling. Political organizations that go door-to-door soliciting opinions are engaging in non-probability sampling. An employee survey that excludes managers from participation is another example.
Many businesses also use non-probability sampling when beta testing , conducting focus groups , or sending surveys to their entire customer base.
What is the difference between probability and non-probability sampling?
In probability sampling, every member of a population has an equal and non-zero chance of being selected for a study. In a non-probability sample, certain population members have a zero chance of being selected.
Is stratified sampling an example of non-probability sampling?
Stratified sampling is an example of probability sampling. In a stratified sample, a population is subdivided into different non-overlapping subpopulations known as strata. When sampling, a researcher randomly selects each element (aka member) of strata. If the populations overlap or elements aren’t chosen randomly, the researcher uses non-probability sampling.
What is random sampling versus non-random sampling?
In random sampling, each population element has an equal chance of being selected in the final. By contrast, certain elements are more likely to be selected than others in a non-random sample.
Is simple sampling non-probability?
Simple sampling (also known as simple random sampling) is an example of probability sampling, not non-probability sampling. In simple random sampling, a researcher chooses random elements from the sampling frame.
Does probability sampling or non-probability sampling lead to statistically significant results?
Statistical significance doesn’t depend on the type of sampling selected. Rather it depends on the effect size and the sample size. The effect size is the size of the difference in outcomes between two samples. The sample size or the number of participants in a study determines the amount of collected information, which affects the precision or level of confidence in the sample estimates.
The bigger the sample size, the more likely it is to find a statistically significant difference between the study groups. However, researchers should always perform a sample size calculation in advance to avoid wasting resources in over-recruiting, which may also unnecessarily inflate the study results.
Get started today
Go from raw data to valuable insights with a flexible research platform
Editor’s picks
Last updated: 21 December 2023
Last updated: 16 December 2023
Last updated: 6 October 2023
Last updated: 5 March 2024
Last updated: 25 November 2023
Last updated: 15 February 2024
Last updated: 11 March 2024
Last updated: 12 December 2023
Last updated: 6 March 2024
Last updated: 10 April 2023
Last updated: 20 December 2023
Latest articles
Related topics, log in or sign up.
Get started for free

Want to create or adapt books like this? Learn more about how Pressbooks supports open publishing practices.
Unit 12: Sampling. When enough is enough.
46 non-probability sampling.
Probably you’ve guessed it by now, right? If probability is random – NON colloquial random – the non-probability is non-random. Which is actually a lot closer to the concept of “random” as we understand it in ordinary, everyday, informal contexts. I know – potentially so confusing! Just commit to memory that Random Reduces Bias [in terms of sampling strategies]. NON-random/probability actually adds bias. So. Let’s say you’re doing your deconstruction and you head back to the original – OP – primary research. Mosey on over to the “procedure” and “participants” sections. And see that they used one of the methods below (which, PS, they do NOT always state the “name” of the strategy so you have to recognize it without the label)… SHOULD YOU BELIEVE IT? I’m not saying that you shouldn’t because getting random samples is pretty darned difficult, but you should definitely practice your critical thinking and add some of that healthy skepticism.
Learning Objectives
What is non-probability sampling?
- Non-Probability Sampling
Non-probability sampling is a non-random sampling technique. This means that it does not give every individual in a population or sampling frame equal chances of being selected. This means that the sample may be less representative of the target population compared to random sampling.
Types of Non-Probability Sampling
Types of Non-Random or Non-Probability Sampling (a selection. Yes, there are more…):
Convenience Volunteer Snowball Quota Network Purposive/Purposeful Theoretical Construct
- Convenience
- Ex: When deciding who to pull from a sample group you decide to pull members of your family because they are the easiest and most convenient group of people to study. ( Example from student Kailey Brown)
2. Volunteer
- Ex: The mass emails students receive from the University of Iowa to participate in studies. Whoever wants to participate responds to the email. (Example from student Kailey Brown)
3. Snowball
- Ex: One friend finds a survey on how much coffee she drinks. She knows a lot of coffee drinker friends and wants them to participate. Then those friends want to bring in more people to contribute. (Kailey Brown)
4 . Quota
- Ex: A researcher wants to mimic the population they are studying. The population is 70% people over 60. Therefore, the participants consisted of 70% people over 60.
5. Network
- Ex: Recruiting participants from social network sites. (Example from student Monica Bucholz)
6. Purposive/ Purposeful
- Ex: In a study about the experiences of adopted children, the researcher chooses to interview children who have been adopted (Example from student Wesley Woods)
7. Theoretical Construct
- Ex: In a study about adopted children, the researcher seeks to show the struggles many children go through. The researcher chooses to sample children who have had negative experiences.
Got ideas for questions to include on the exam?
Click this link to add them! [this course element is paused because ya’ll aren’t submitting many questions…]
… Unit 1 … Unit 2 …. Unit 3 … Unit 4 … Unit 5 … Unit 6 … Unit 7 … Unit 8 … Unit 9 … Unit 10 … Unit 11 … Unit 12 … Unit 13 … Unit 14 … Unit 15 … Unit 16 …
- Probability Sampling
- Considerations When Sampling
- On being skeptical [cuz they didn’t rep-re-sent]
non-random sampling technique
researcher chooses those in their sampling frame that are easiest to access
those who participate in the study choose on their own to do so
type of sampling is when someone starts the study and then refers more people to participate in it
like stratified sampling, quota sampling breaks up the sample into subcategories in ways the researcher determines
when a researcher utilizes their social network to recruit participants
researcher purposely and subjectively chooses participants based on how crucial the experiences they can share are to answering the researcher’s research questions
when the researcher selects participants that embody a theory they are interested in
Introduction to Social Scientific Research Methods in the field of Communication 3rd Ed - under construction for Fall 2023 Copyright © 2023 by Kate Magsamen-Conrad. All Rights Reserved.
Share This Book
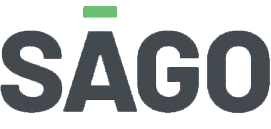
What We Offer
With a comprehensive suite of qualitative and quantitative capabilities and 55 years of experience in the industry, Sago powers insights through adaptive solutions.
- Recruitment
- Communities
- Methodify® Automated research
- QualBoard® Digital Discussions
- QualMeeting® Digital Interviews
- Global Qualitative
- Global Quantitative
- In-Person Facilities
- Research Consulting
- Europe Solutions
- Neuromarketing Tools
- Trial & Jury Consulting
Who We Serve
Form deeper customer connections and make the process of answering your business questions easier. Sago delivers unparalleled access to the audiences you need through adaptive solutions and a consultative approach.
- Consumer Packaged Goods
- Financial Services
- Media Technology
- Medical Device Manufacturing
- Marketing Research
With a 55-year legacy of impact, Sago has proven we have what it takes to be a long-standing industry leader and partner. We continually advance our range of expertise to provide our clients with the highest level of confidence.
- Global Offices
- Partnerships & Certifications
- News & Media
- Researcher Events
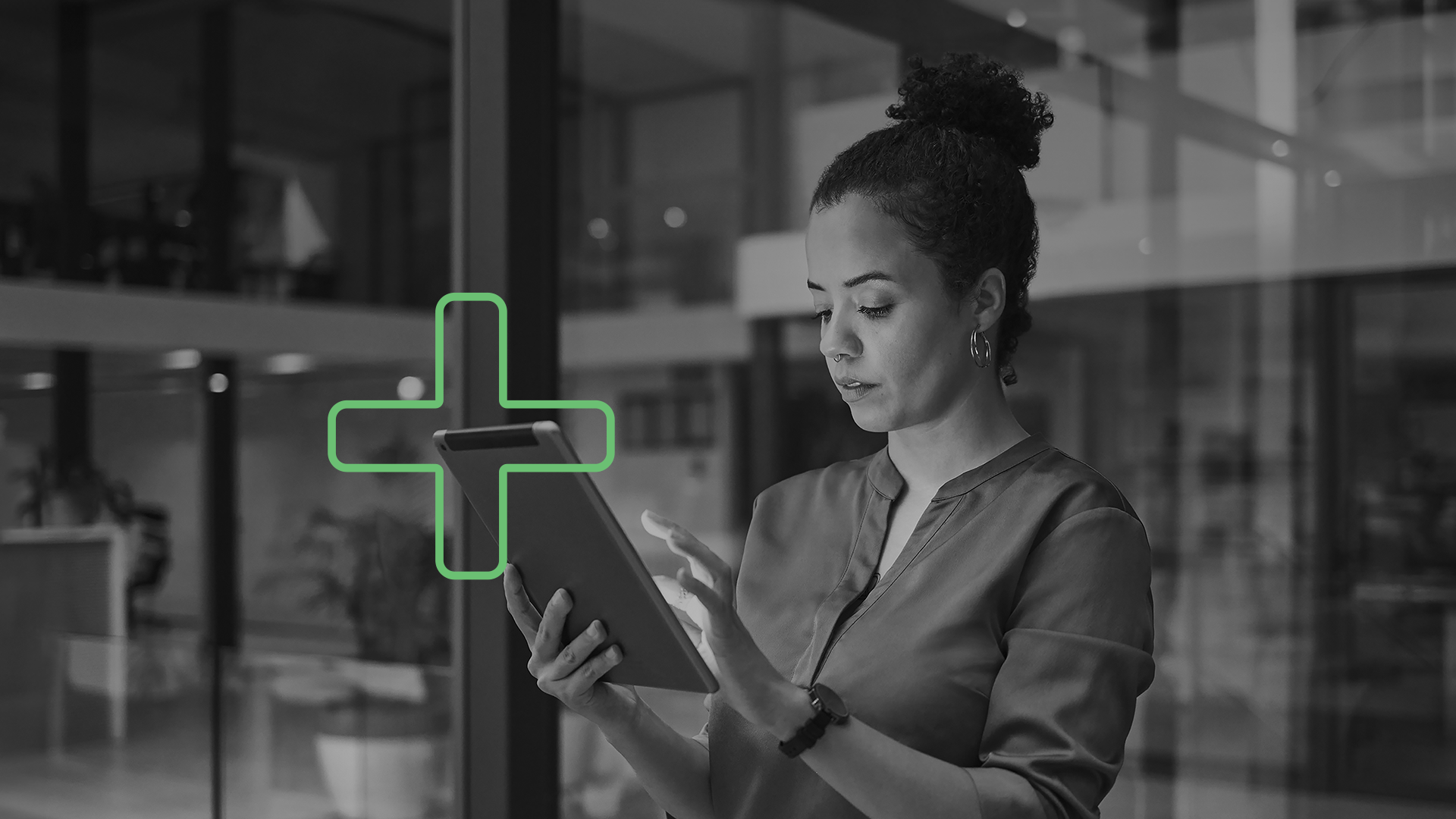
Sago Announces Launch of Sago Health to Elevate Healthcare Research
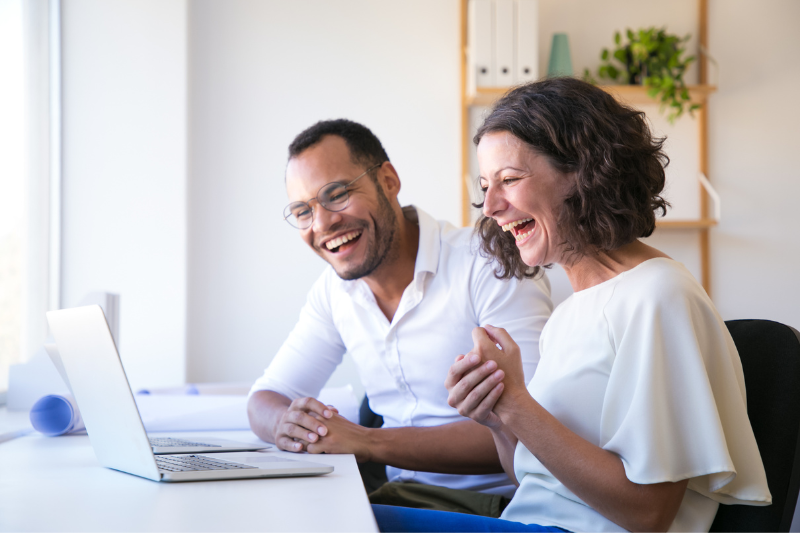
Sago Launches AI Video Summaries on QualBoard to Streamline Data Synthesis
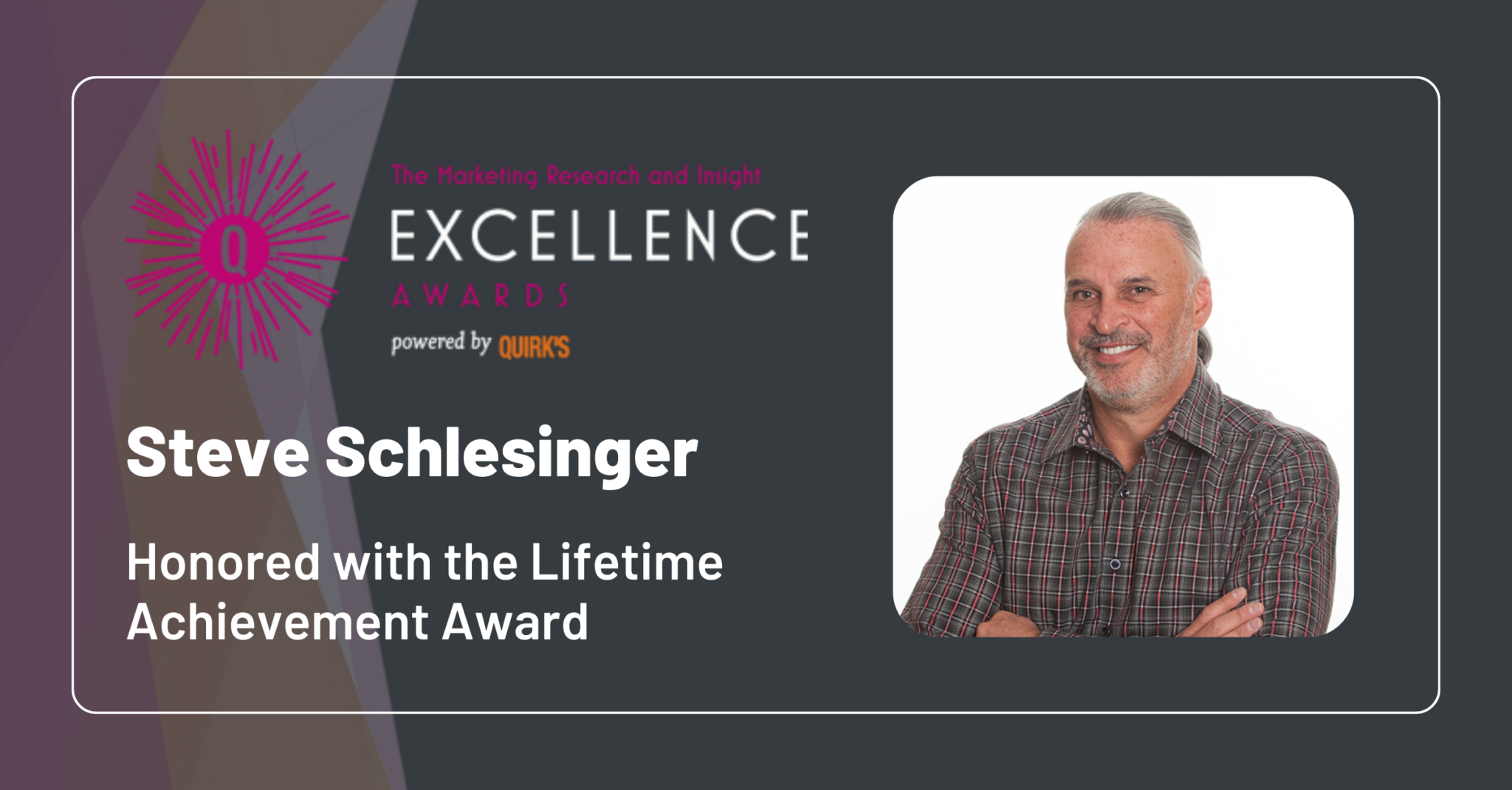
Sago Executive Chairman Steve Schlesinger to Receive Quirk’s Lifetime Achievement Award
Drop into your new favorite insights rabbit hole and explore content created by the leading minds in market research.
- Case Studies
- Knowledge Kit
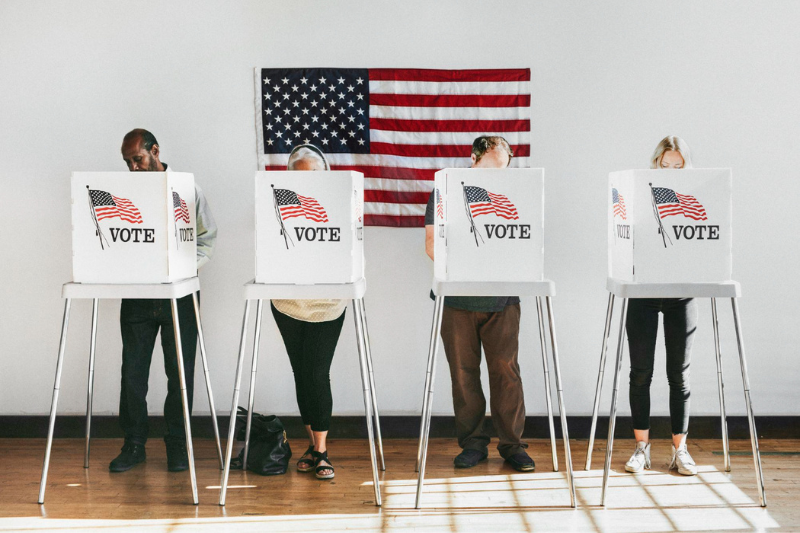
The Deciders April 2024: Michigan Voters in Union Households
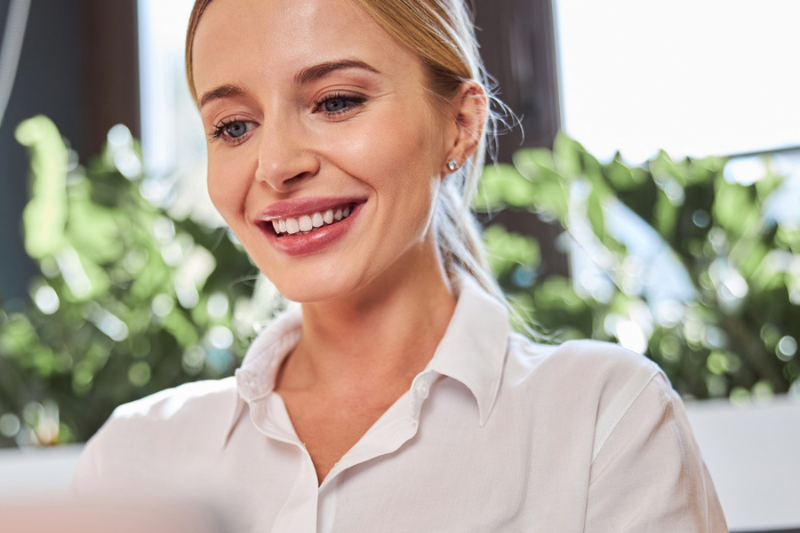
Navigating the Dynamic Future of Qualitative Research
Get in touch

- Account Logins

Different Types of Sampling Techniques in Qualitative Research
- Resources , Blog

Key Takeaways:
- Sampling techniques in qualitative research include purposive, convenience, snowball, and theoretical sampling.
- Choosing the right sampling technique significantly impacts the accuracy and reliability of the research results.
- It’s crucial to consider the potential impact on the bias, sample diversity, and generalizability when choosing a sampling technique for your qualitative research.
Qualitative research seeks to understand social phenomena from the perspective of those experiencing them. It involves collecting non-numerical data such as interviews, observations, and written documents to gain insights into human experiences, attitudes, and behaviors. While qualitative research can provide rich and nuanced insights, the accuracy and generalizability of findings depend on the quality of the sampling process. Sampling is a critical component of qualitative research as it involves selecting a group of participants who can provide valuable insights into the research questions.
This article explores different types of sampling techniques used in qualitative research. First, we’ll provide a comprehensive overview of four standard sampling techniques used in qualitative research. and then compare and contrast these techniques to provide guidance on choosing the most appropriate method for a particular study. Additionally, you’ll find best practices for sampling and learn about ethical considerations researchers need to consider in selecting a sample. Overall, this article aims to help researchers conduct effective and high-quality sampling in qualitative research.
In this Article:
- Purposive Sampling
- Convenience Sampling
- Snowball Sampling
- Theoretical Sampling
Factors to Consider When Choosing a Sampling Technique
Practical approaches to sampling: recommended practices, final thoughts, get expert guidance on your sample needs.
Want expert input on the best sampling technique for your qualitative research project? Book a consultation for trusted advice.
Request a consultation
4 Types of Sampling Techniques and Their Applications
Sampling is a crucial aspect of qualitative research as it determines the representativeness and credibility of the data collected. Several sampling techniques are used in qualitative research, each with strengths and weaknesses. In this section, let’s explore four standard sampling techniques used in qualitative research: purposive sampling, convenience sampling, snowball sampling, and theoretical sampling. We’ll break down the definition of each technique, when to use it, and its advantages and disadvantages.
1. Purposive Sampling
Purposive sampling, or judgmental sampling, is a non-probability sampling technique commonly used in qualitative research. In purposive sampling, researchers intentionally select participants with specific characteristics or unique experiences related to the research question. The goal is to identify and recruit participants who can provide rich and diverse data to enhance the research findings.
Purposive sampling is used when researchers seek to identify individuals or groups with particular knowledge, skills, or experiences relevant to the research question. For instance, in a study examining the experiences of cancer patients undergoing chemotherapy, purposive sampling may be used to recruit participants who have undergone chemotherapy in the past year. Researchers can better understand the phenomenon under investigation by selecting individuals with relevant backgrounds.
Purposive Sampling: Strengths and Weaknesses
Purposive sampling is a powerful tool for researchers seeking to select participants who can provide valuable insight into their research question. This method is advantageous when studying groups with technical characteristics or experiences where a random selection of participants may yield different results.
One of the main advantages of purposive sampling is the ability to improve the quality and accuracy of data collected by selecting participants most relevant to the research question. This approach also enables researchers to collect data from diverse participants with unique perspectives and experiences related to the research question.
However, researchers should also be aware of potential bias when using purposive sampling. The researcher’s judgment may influence the selection of participants, resulting in a biased sample that does not accurately represent the broader population. Another disadvantage is that purposive sampling may not be representative of the more general population, which limits the generalizability of the findings. To guarantee the accuracy and dependability of data obtained through purposive sampling, researchers must provide a clear and transparent justification of their selection criteria and sampling approach. This entails outlining the specific characteristics or experiences required for participants to be included in the study and explaining the rationale behind these criteria. This level of transparency not only helps readers to evaluate the validity of the findings, but also enhances the replicability of the research.
2. Convenience Sampling
When time and resources are limited, researchers may opt for convenience sampling as a quick and cost-effective way to recruit participants. In this non-probability sampling technique, participants are selected based on their accessibility and willingness to participate rather than their suitability for the research question. Qualitative research often uses this approach to generate various perspectives and experiences.
During the COVID-19 pandemic, convenience sampling was a valuable method for researchers to collect data quickly and efficiently from participants who were easily accessible and willing to participate. For example, in a study examining the experiences of university students during the pandemic, convenience sampling allowed researchers to recruit students who were available and willing to share their experiences quickly. While the pandemic may be over, convenience sampling during this time highlights its value in urgent situations where time and resources are limited.
Convenience Sampling: Strengths and Weaknesses
Convenience sampling offers several advantages to researchers, including its ease of implementation and cost-effectiveness. This technique allows researchers to quickly and efficiently recruit participants without spending time and resources identifying and contacting potential participants. Furthermore, convenience sampling can result in a diverse pool of participants, as individuals from various backgrounds and experiences may be more likely to participate.
While convenience sampling has the advantage of being efficient, researchers need to acknowledge its limitations. One of the primary drawbacks of convenience sampling is that it is susceptible to selection bias. Participants who are more easily accessible may not be representative of the broader population, which can limit the generalizability of the findings. Furthermore, convenience sampling may lead to issues with the reliability of the results, as it may not be possible to replicate the study using the same sample or a similar one.
To mitigate these limitations, researchers should carefully define the population of interest and ensure the sample is drawn from that population. For instance, if a study is investigating the experiences of individuals with a particular medical condition, researchers can recruit participants from specialized clinics or support groups for that condition. Researchers can also use statistical techniques such as stratified sampling or weighting to adjust for potential biases in the sample.
3. Snowball Sampling
Snowball sampling, also called referral sampling, is a unique approach researchers use to recruit participants in qualitative research. The technique involves identifying a few initial participants who meet the eligibility criteria and asking them to refer others they know who also fit the requirements. The sample size grows as referrals are added, creating a chain-like structure.
Snowball sampling enables researchers to reach out to individuals who may be hard to locate through traditional sampling methods, such as members of marginalized or hidden communities. For instance, in a study examining the experiences of undocumented immigrants, snowball sampling may be used to identify and recruit participants through referrals from other undocumented immigrants.
Snowball Sampling: Strengths and Weaknesses
Snowball sampling can produce in-depth and detailed data from participants with common characteristics or experiences. Since referrals are made within a network of individuals who share similarities, researchers can gain deep insights into a specific group’s attitudes, behaviors, and perspectives.
4. Theoretical Sampling
Theoretical sampling is a sophisticated and strategic technique that can help researchers develop more in-depth and nuanced theories from their data. Instead of selecting participants based on convenience or accessibility, researchers using theoretical sampling choose participants based on their potential to contribute to the emerging themes and concepts in the data. This approach allows researchers to refine their research question and theory based on the data they collect rather than forcing their data to fit a preconceived idea.
Theoretical sampling is used when researchers conduct grounded theory research and have developed an initial theory or conceptual framework. In a study examining cancer survivors’ experiences, for example, theoretical sampling may be used to identify and recruit participants who can provide new insights into the coping strategies of survivors.
Theoretical Sampling: Strengths and Weaknesses
One of the significant advantages of theoretical sampling is that it allows researchers to refine their research question and theory based on emerging data. This means the research can be highly targeted and focused, leading to a deeper understanding of the phenomenon being studied. Additionally, theoretical sampling can generate rich and in-depth data, as participants are selected based on their potential to provide new insights into the research question.
Participants are selected based on their perceived ability to offer new perspectives on the research question. This means specific perspectives or experiences may be overrepresented in the sample, leading to an incomplete understanding of the phenomenon being studied. Additionally, theoretical sampling can be time-consuming and resource-intensive, as researchers must continuously analyze the data and recruit new participants.
To mitigate the potential for bias, researchers can take several steps. One way to reduce bias is to use a diverse team of researchers to analyze the data and make participant selection decisions. Having multiple perspectives and backgrounds can help prevent researchers from unconsciously selecting participants who fit their preconceived notions or biases.
Another solution would be to use reflexive sampling. Reflexive sampling involves selecting participants aware of the research process and provides insights into how their biases and experiences may influence their perspectives. By including participants who are reflexive about their subjectivity, researchers can generate more nuanced and self-aware findings.
Choosing the proper sampling technique is one of the most critical decisions a researcher makes when conducting a study. The preferred method can significantly impact the accuracy and reliability of the research results.
For instance, purposive sampling provides a more targeted and specific sample, which helps to answer research questions related to that particular population or phenomenon. However, this approach may also introduce bias by limiting the diversity of the sample.
Conversely, convenience sampling may offer a more diverse sample regarding demographics and backgrounds but may also introduce bias by selecting more willing or available participants.
Snowball sampling may help study hard-to-reach populations, but it can also limit the sample’s diversity as participants are selected based on their connections to existing participants.
Theoretical sampling may offer an opportunity to refine the research question and theory based on emerging data, but it can also be time-consuming and resource-intensive.
Additionally, the choice of sampling technique can impact the generalizability of the research findings. Therefore, it’s crucial to consider the potential impact on the bias, sample diversity, and generalizability when choosing a sampling technique. By doing so, researchers can select the most appropriate method for their research question and ensure the validity and reliability of their findings.
Tips for Selecting Participants
When selecting participants for a qualitative research study, it is crucial to consider the research question and the purpose of the study. In addition, researchers should identify the specific characteristics or criteria they seek in their sample and select participants accordingly.
One helpful tip for selecting participants is to use a pre-screening process to ensure potential participants meet the criteria for inclusion in the study. Another technique is using multiple recruitment methods to ensure the sample is diverse and representative of the studied population.
Ensuring Diversity in Samples
Diversity in the sample is important to ensure the study’s findings apply to a wide range of individuals and situations. One way to ensure diversity is to use stratified sampling, which involves dividing the population into subgroups and selecting participants from each subset. This helps establish that the sample is representative of the larger population.
Maintaining Ethical Considerations
When selecting participants for a qualitative research study, it is essential to ensure ethical considerations are taken into account. Researchers must ensure participants are fully informed about the study and provide their voluntary consent to participate. They must also ensure participants understand their rights and that their confidentiality and privacy will be protected.
A qualitative research study’s success hinges on its sampling technique’s effectiveness. The choice of sampling technique must be guided by the research question, the population being studied, and the purpose of the study. Whether purposive, convenience, snowball, or theoretical sampling, the primary goal is to ensure the validity and reliability of the study’s findings.
By thoughtfully weighing the pros and cons of each sampling technique, researchers can make informed decisions that lead to more reliable and accurate results. In conclusion, carefully selecting a sampling technique is integral to the success of a qualitative research study, and a thorough understanding of the available options can make all the difference in achieving high-quality research outcomes.
If you’re interested in improving your research and sampling methods, Sago offers a variety of solutions. Our qualitative research platforms, such as QualBoard and QualMeeting, can assist you in conducting research studies with precision and efficiency. Our robust global panel and recruitment options help you reach the right people. We also offer qualitative and quantitative research services to meet your research needs. Contact us today to learn more about how we can help improve your research outcomes.
Find the Right Sample for Your Qualitative Research
Trust our team to recruit the participants you need using the appropriate techniques. Book a consultation with our team to get started .
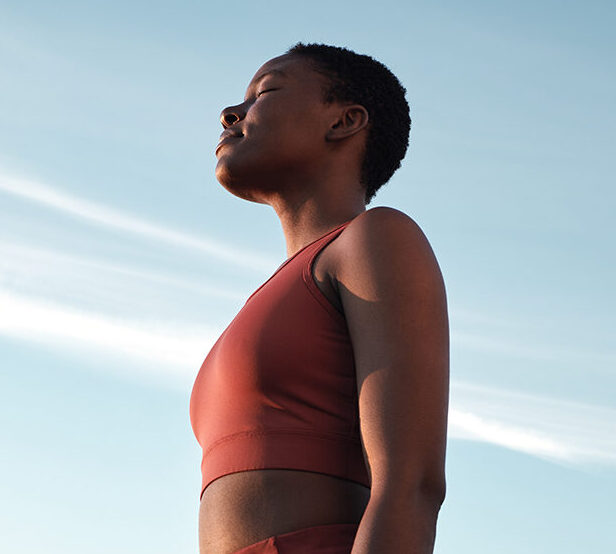
Quantifying Digital Detox Experience Across the Globe
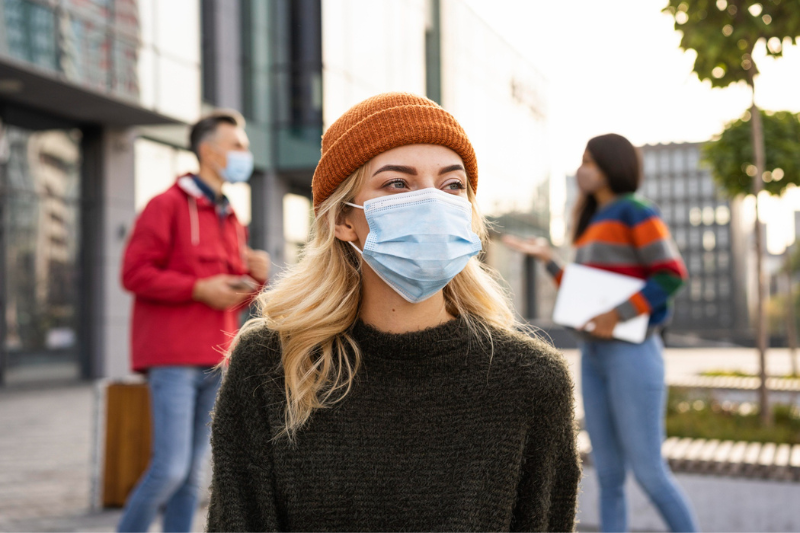
How Connecting with Gen C Can Help Your Brand Grow
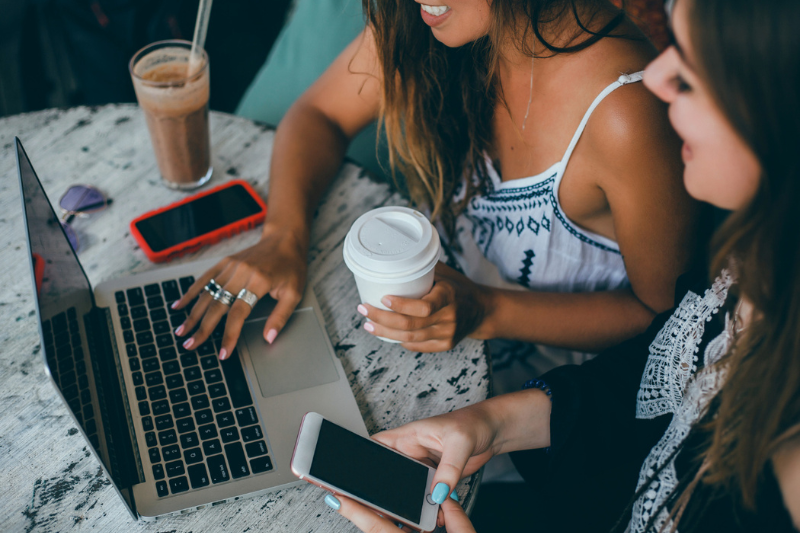
Digital Detox: How Different Generations Navigate Social Media Breaks
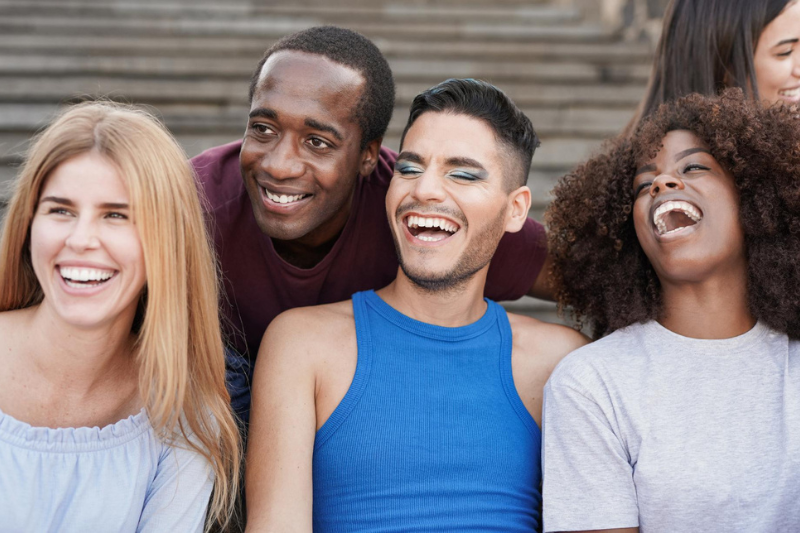
Building Trust Through Inclusive Healthcare Research Recruitment
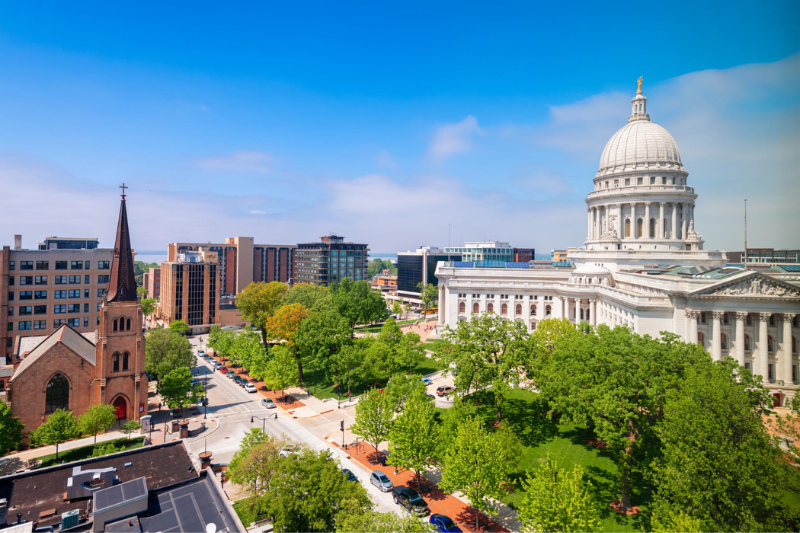
The Swing Voter Project Wisconsin: March 2024
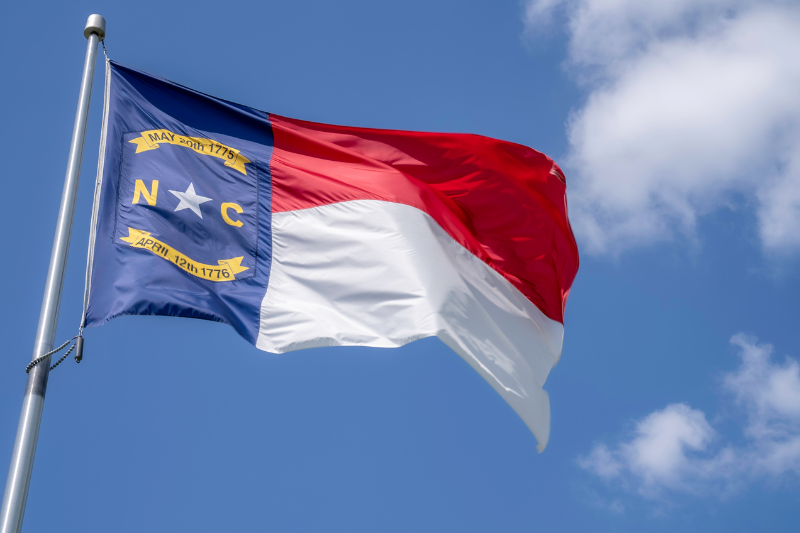
The Deciders February 2024: African American voters in North Carolina
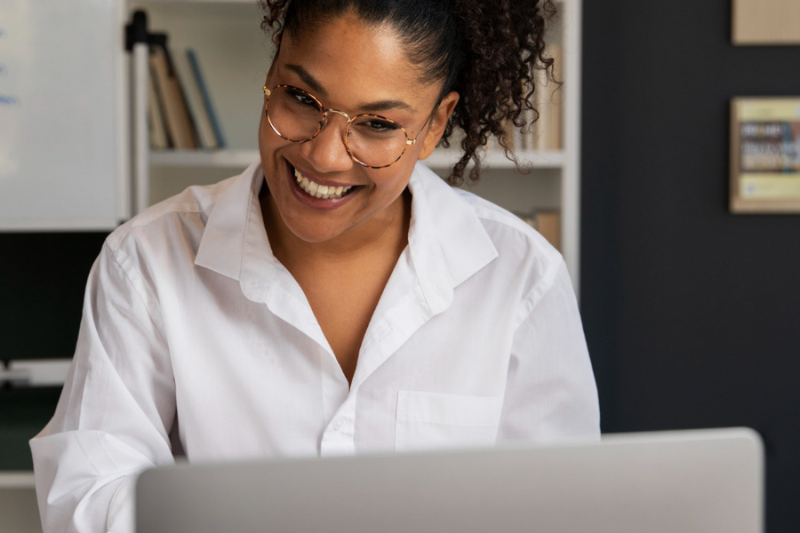
OnDemand: Moderator Minutes: Maximizing Efficiency with QualBoard – Streamline Your Projects with Support & AI
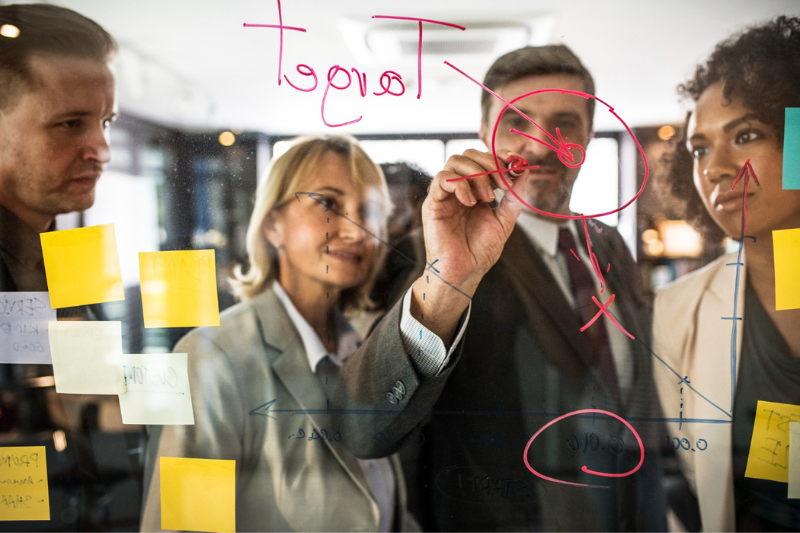
2024 Trends in Research: Fact or Fiction?
Take a deep dive into your favorite market research topics
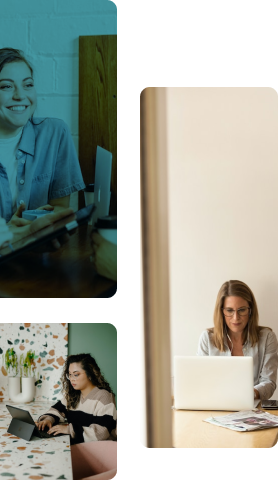
How can we help support you and your research needs?
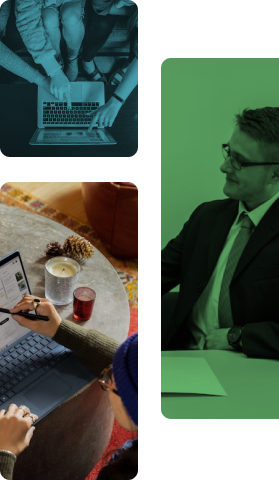
BEFORE YOU GO
Have you considered how to harness AI in your research process? Check out our on-demand webinar for everything you need to know


An official website of the United States government
The .gov means it’s official. Federal government websites often end in .gov or .mil. Before sharing sensitive information, make sure you’re on a federal government site.
The site is secure. The https:// ensures that you are connecting to the official website and that any information you provide is encrypted and transmitted securely.
- Publications
- Account settings
Preview improvements coming to the PMC website in October 2024. Learn More or Try it out now .
- Advanced Search
- Journal List
- Emerg (Tehran)
- v.5(1); 2017

Sampling methods in Clinical Research; an Educational Review
Mohamed elfil.
1 Faculty of Medicine, Alexandria University, Egypt.
Ahmed Negida
2 Faculty of Medicine, Zagazig University, Egypt.
Clinical research usually involves patients with a certain disease or a condition. The generalizability of clinical research findings is based on multiple factors related to the internal and external validity of the research methods. The main methodological issue that influences the generalizability of clinical research findings is the sampling method. In this educational article, we are explaining the different sampling methods in clinical research.
Introduction
In clinical research, we define the population as a group of people who share a common character or a condition, usually the disease. If we are conducting a study on patients with ischemic stroke, it will be difficult to include the whole population of ischemic stroke all over the world. It is difficult to locate the whole population everywhere and to have access to all the population. Therefore, the practical approach in clinical research is to include a part of this population, called “sample population”. The whole population is sometimes called “target population” while the sample population is called “study population. When doing a research study, we should consider the sample to be representative to the target population, as much as possible, with the least possible error and without substitution or incompleteness. The process of selecting a sample population from the target population is called the “sampling method”.
Sampling types
There are two major categories of sampling methods ( figure 1 ): 1; probability sampling methods where all subjects in the target population have equal chances to be selected in the sample [ 1 , 2 ] and 2; non-probability sampling methods where the sample population is selected in a non-systematic process that does not guarantee equal chances for each subject in the target population [ 2 , 3 ]. Samples which were selected using probability sampling methods are more representatives of the target population.

Sampling methods.
Probability sampling method
Simple random sampling
This method is used when the whole population is accessible and the investigators have a list of all subjects in this target population. The list of all subjects in this population is called the “sampling frame”. From this list, we draw a random sample using lottery method or using a computer generated random list [ 4 ].
Stratified random sampling
This method is a modification of the simple random sampling therefore, it requires the condition of sampling frame being available, as well. However, in this method, the whole population is divided into homogeneous strata or subgroups according a demographic factor (e.g. gender, age, religion, socio-economic level, education, or diagnosis etc.). Then, the researchers select draw a random sample from the different strata [ 3 , 4 ]. The advantages of this method are: (1) it allows researchers to obtain an effect size from each strata separately, as if it was a different study. Therefore, the between group differences become apparent, and (2) it allows obtaining samples from minority/under-represented populations. If the researchers used the simple random sampling, the minority population will remain underrepresented in the sample, as well. Simply, because the simple random method usually represents the whole target population. In such case, investigators can better use the stratified random sample to obtain adequate samples from all strata in the population.
Systematic random sampling (Interval sampling)
In this method, the investigators select subjects to be included in the sample based on a systematic rule, using a fixed interval. For example: If the rule is to include the last patient from every 5 patients. We will include patients with these numbers (5, 10, 15, 20, 25, ...etc.). In some situations, it is not necessary to have the sampling frame if there is a specific hospital or center which the patients are visiting regularly. In this case, the researcher can start randomly and then systemically chooses next patients using a fixed interval [ 4 ].
Cluster sampling (Multistage sampling)
It is used when creating a sampling frame is nearly impossible due to the large size of the population. In this method, the population is divided by geographic location into clusters. A list of all clusters is made and investigators draw a random number of clusters to be included. Then, they list all individuals within these clusters, and run another turn of random selection to get a final random sample exactly as simple random sampling. This method is called multistage because the selection passed with two stages: firstly, the selection of eligible clusters, then, the selection of sample from individuals of these clusters. An example for this, if we are conducting a research project on primary school students from Iran. It will be very difficult to get a list of all primary school students all over the country. In this case, a list of primary schools is made and the researcher randomly picks up a number of schools, then pick a random sample from the eligible schools [ 3 ].
Non-probability sampling method
Convenience sampling
Although it is a non-probability sampling method, it is the most applicable and widely used method in clinical research. In this method, the investigators enroll subjects according to their availability and accessibility. Therefore, this method is quick, inexpensive, and convenient. It is called convenient sampling as the researcher selects the sample elements according to their convenient accessibility and proximity [ 3 , 6 ]. For example: assume that we will perform a cohort study on Egyptian patients with Hepatitis C (HCV) virus. The convenience sample here will be confined to the accessible population for the research team. Accessible population are HCV patients attending in Zagazig University Hospital and Cairo University Hospitals. Therefore, within the study period, all patients attending these two hospitals and meet the eligibility criteria will be included in this study.
Judgmental sampling
In this method, the subjects are selected by the choice of the investigators. The researcher assumes specific characteristics for the sample (e.g. male/female ratio = 2/1) and therefore, they judge the sample to be suitable for representing the population. This method is widely criticized due to the likelihood of bias by investigator judgement [ 5 ].
Snow-ball sampling
This method is used when the population cannot be located in a specific place and therefore, it is different to access this population. In this method, the investigator asks each subject to give him access to his colleagues from the same population. This situation is common in social science research, for example, if we running a survey on street children, there will be no list with the homeless children and it will be difficult to locate this population in one place e.g. a school/hospital. Here, the investigators will deliver the survey to one child then, ask him to take them to his colleagues or deliver the surveys to them.
Conflict of interest:
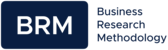
Non-Probability Sampling
In non-probability sampling (also known as non-random sampling) not all members of the population have a chance to participate in the study. In other words, this method is based on non-random selection criteria. This is contrary to probability sampling , where each member of the population has a known, non-zero chance of being selected to participate in the study.
Necessity for non-probability sampling can be explained in a way that for some studies it is not feasible to draw a random probability-based sample of the population due to time and/or cost considerations. In these cases, sample group members have to be selected on the basis of accessibility or personal judgment of the researcher. Therefore, the majority of non-probability sampling techniques include an element of subjective judgement. Non-probability sampling is the most helpful for exploratory stages of studies such as a pilot survey.
The issue of sample size in non-probability sampling is rather ambiguous and needs to reflect a wide range of research-specific factors in each case. Nevertheless, there are some considerations about the minimum sample sizes in non-probability sampling as illustrated in the table below:
Sizes of non-probability sampling [1]
The following is the list of the most popular non-probability sampling methods and their brief descriptions:
Non-probability sampling methods
Advantages of Non-Probability Sampling
- Possibility to reflect the descriptive comments about the sample
- Cost-effectiveness and time-effectiveness compared to probability sampling
- Effective when it is unfeasible or impractical to conduct probability sampling
Disadvantages of Non-Probability Sampling
- Unknown proportion of the entire population is not included in the sample group i.e. lack of representation of the entire population
- Lower level of generalization of research findings compared to probability sampling
- Difficulties in estimating sampling variability and identifying possible bias
My e-book, The Ultimate Guide to Writing a Dissertation in Business Studies: a step by step approach contains a detailed, yet simple explanation of sampling methods. The e-book explains all stages of the research process starting from the selection of the research area to writing personal reflection. Important elements of dissertations such as research philosophy, research approach, research design, methods of data collection and data analysis are explained in this e-book in simple words.
John Dudovskiy
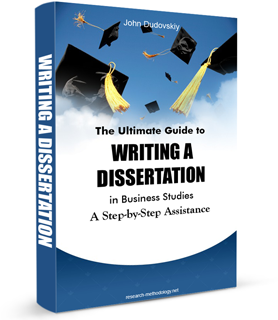
[1] Source: Saunders, M., Lewis, P. & Thornhill, A. (2012) “Research Methods for Business Students” 6 th edition, Pearson Education Limited
Have a language expert improve your writing
Run a free plagiarism check in 10 minutes, automatically generate references for free.
- Knowledge Base
- Methodology
- What Is Non-Probability Sampling? | Types & Examples
What Is Non-Probability Sampling? | Types & Examples
Published on 21 July 2022 by Kassiani Nikolopoulou . Revised on 10 October 2022.
Non-probability sampling is a sampling method that uses non-random criteria like the availability, geographical proximity, or expert knowledge of the individuals you want to research in order to answer a research question.
Non-probability sampling is used when the population parameters are either unknown or not possible to individually identify. For example, visitors to a website that doesn’t require users to create an account could form part of a non-probability sample.
- In non-probability sampling , each unit in your target population does not have an equal chance of being included. Here, you can form your sample using other considerations, such as convenience or a particular characteristic.
- In probability sampling , each unit in your target population must have an equal chance of selection. This minimises the risk of selection bias .
Table of contents
Types of non-probability sampling, non-probability sampling examples, probability vs. non-probability sampling, advantages and disadvantages of non-probability sampling, frequently asked questions about non-probability sampling.
There are five common types of non-probability sampling:
Convenience sampling
Quota sampling, self-selection (volunteer) sampling, snowball sampling, purposive (judgmental) sampling.
Convenience sampling is primarily determined by convenience to the researcher.
This can include factors like:
- Ease of access
- Geographical proximity
- Existing contact within the population of interest
Convenience samples are sometimes called “accidental samples,” because participants can be selected for the sample simply because they happen to be nearby when the researcher is conducting the data collection .
In quota sampling , you select a predetermined number or proportion of units, called a quota. Your quota should comprise subgroups with specific characteristics (e.g., individuals, cases, or organisations) and should be selected in a non-random manner.
Your subgroups, called strata , should be mutually exclusive. Your estimation can be based on previous studies or on other existing data, if there are any. This helps you determine how many units should be chosen from each subgroup. In the data collection phase, you continue to recruit units until you reach your quota.
There are two types of quota sampling:
- Proportional quota sampling is used when the size of the population is known. This allows you to determine the quota of individuals that you need to include in your sample in order to be representative of your population.
- Non-proportional quota sampling is used when the size of the population is unknown. Here, it’s up to you to determine the quota of individuals that you are going to include in your sample in advance.
Note that quota sampling may sound similar to stratified sampling , a probability sampling method where you divide your population into subgroups that share a common characteristic.
The key difference here is that in stratified sampling, you take a random sample from each subgroup, while in quota sampling, the sample selection is non-random, usually via convenience sampling. In other words, who is included in the sample is left up to the subjective judgment of the researcher.
You stand at a convenient location, such as a busy shopping street, and randomly select people to talk to who appear to satisfy the age criterion. Once you stop them, you must first determine whether they do indeed fit the criteria of belonging to the predetermined age range and owning or renting a property in the suburb.
Self-selection sampling (also called volunteer sampling) relies on participants who voluntarily agree to be part of your research. This is common for samples that need people who meet specific criteria, as is often the case for medical or psychological research.
In self-selection sampling, volunteers are usually invited to participate through advertisements asking those who meet the requirements to sign up. Volunteers are recruited until a predetermined sample size is reached.
Self-selection or volunteer sampling involves two steps:
- Publicizing your need for subjects
- Checking the suitability of each subject and either inviting or rejecting them
Keep in mind that not all people who apply will be eligible for your research. There is a high chance that many applicants will not fully read or understand what your study is about, or may possess disqualifying factors. It’s important to double-check eligibility carefully before inviting any volunteers to form part of your sample.
Snowball sampling is used when the population you want to research is hard to reach, or there is no existing database or other sampling frame to help you find them. Research about socially marginalised groups such as drug addicts, homeless people, or sex workers often uses snowball sampling.
To conduct a snowball sample, you start by finding one person who is willing to participate in your research. You then ask them to introduce you to others.
Alternatively, your research may involve finding people who use a certain product or have experience in the area you are interested in. In these cases, you can also use networks of people to gain access to your population of interest.
In this way, the process of snowball sampling begins. You started by attending the meeting, where you met someone who could then put you in touch with others in the group.
Purposive sampling is a blanket term for several sampling techniques that choose participants deliberately due to qualities they possess. It is also called judgmental sampling, because it relies on the judgment of the researcher to select the units (e.g., people, cases, or organizations studied).
Purposive sampling is common in qualitative and mixed methods research designs, especially when considering specific issues with unique cases.
Common purposive sampling techniques include:
- Maximum variation (heterogeneous) sampling
Homogeneous sampling
Typical case sampling.
- Extreme (or deviant) case sampling
Critical case sampling
Expert sampling.
These can either be used on their own or in combination with other purposive sampling techniques.
Maximum variation sampling
The idea behind maximum variation sampling is to look at a subject from all possible angles in order to achieve greater understanding. Also known as heterogeneous sampling, it involves selecting candidates across a broad spectrum relating to the topic of study. This helps you capture a wide range of perspectives and identifies common themes evident across the sample.
Homogeneous sampling , unlike maximum variation sampling, aims to achieve a sample whose units share characteristics, such as a group of people that are similar in terms of age, culture, or job. The idea here is to focus on this similarity, investigating how it relates to the topic you are researching.
A typical case sample is composed of people who can be regarded as “typical” for a community or phenomenon. A typical case sample allows you to develop a profile of what would generally be agreed as being “average” or “normal.”
Typical case samples are often used when large communities or complex problems are investigated. In this way, you can gain an understanding in a relatively short time, even if you are not familiar with what’s going on yourself.
Note that the purpose of typical case sampling is to describe and illustrate what is typical to those unfamiliar with the setting or situation. The purpose is not to make generalised statements about the experiences of all participants. In other words, typical case sampling allows you to compare samples, not generalise samples to populations.
Extreme (deviant) case sampling
Extreme (or deviant) case sampling uses extreme cases of a particular phenomenon ( outliers ). This can mean remarkable failures, successes, or crises, as well as any event, organisation, or individual that appears to be the “exception to the rule.” Extreme case sampling is most often used when researchers are developing best-practice guidelines.
Note that extreme case sampling usually occurs in combination with other sampling strategies. The process of identifying extreme or deviant cases usually occurs after some portion of data collection and analysis has already been completed.
Critical case sampling is used where a single case (or a small number of cases) can be critical or decisive in explaining the phenomenon of interest. It is often used in exploratory research , or in research with limited resources.
There are a few cues that can help show you whether or not a case is critical, such as:
- “If it happens here, it will happen anywhere”
- “If that group is having problems, then all groups are having problems”
It is critical to ensure that your cases fit these criteria prior to proceeding with this sampling method.
Expert sampling involves selecting a sample based on demonstrable experience, knowledge, or expertise of participants. This expertise may be a good way to compensate for a lack of observational evidence or to gather information during the exploratory phase of your research.
Alternatively, your research may be focused on individuals who possess exactly this expertise, similar to ethnographic research .
Prevent plagiarism, run a free check.
There are a few methods you can use to draw a non-probability sample, such as:
Social media
River sampling, street research.
Suppose you are researching the motivations of digital nomads (young professionals working solely in an online environment). You are curious what led them to adopt this lifestyle.
Since your population of interest is located all over the globe, it clearly isn’t feasible to conduct your study in person. Instead, you decide to use social media, finding your participants through snowball sampling.
You start by identifying social media sites that cater to digital nomads, such as Facebook groups, blogs, or freelance job sites. You ask the administrators for permission to post a call for participants with information about your research, encouraging readers to share the call with peers.
You are part of a research group investigating online behavior and cyberbullying, in particular among users aged 15 to 30 in your state. You are collecting data in two ways, using an online survey.
You first place a link to your survey in an online news article about cyber-hate published by local media. Second, you create an advertising campaign through social media, targeted at users aged 15 to 30 and linking back to your survey. To entice users to participate, a prize draw (movie tickets) is mentioned in all ads. The survey and the campaign are active for the same length of time.
These two data collection methods are river samples. The name refers to the idea of researchers dipping into the traffic flow of a website, catching some of the users floating by.
You are interested in the level of knowledge about myocardial infarction symptoms among the general population.
For a week, you stand in a shopping mall and stop passersby, asking them whether they would be willing to take part in your research. To try to allow as broad a range of respondents as possible to be included, you interview equal numbers of people from Monday to Friday during working hours.
Sampling methods can be broadly divided into two types:
- Probability sampling : When the sample is drawn in such a way that each unit in the population has an equal chance of selection
- Non-probability sampling : When you select the units for your sample with other considerations in mind, such as convenience or geographical proximity
Probability sampling
For many types of analysis, it is important that the statistical analysis is conducted from a random probability sample from the population of interest. For the sample to qualify as random, each unit must have an equal chance (i.e., equal probability) of being selected.
When you use a random selection method (e.g., a drawing) and ensure that you have a sufficiently large sample, your sample is more likely to be representative, and the results generalisable.
Non-probability sampling
Non-probability sampling designs are used when the sample needs to be collected based on a specific characteristic of the population (e.g., people with diabetes).
Unlike probability sampling, the goal is not to achieve objectivity in the selection of samples, or to make statistical inferences. Rather, the goal is to apply the results only to a certain subsection or organisation. These are used in both quantitative and qualitative research.
It is important to be aware of the advantages and disadvantages of non-probability sampling and to understand how they can play a role in your study design.
Advantages of non-probability sampling
Depending on your research design, there are advantages to choosing non-probability sampling.
- Non-probability sampling does not require a sampling frame, so your subjects are often readily available. This can make non-probability sampling quicker and easier to carry out.
- Non-probability sampling allows you to target particular groups within your population. In certain types of research, it is vital that certain units be included in your sample. For example, many kinds of medical research rely on people with a specific health issue.
- Although it is not possible to make statistical inferences from the sample to the population, non-probability sampling methods can provide researchers with the data to make other types of generalisations from the sample being studied.
Disadvantages of non-probability sampling
Non-probability sampling has some downsides as well. These include the following:
- Non-probability samples are extremely unlikely to be representative of the population studied. This undermines the generalisability of your results.
- As some units in the population have no chance of being included in the sample, undercoverage bias is likely.
- Furthermore, since the selection of units included in the sample is often based on ease of access, sampling bias is common as well.
- While the subjective judgment of the researcher in choosing who makes up the sample can be an advantage, it also increases the risk of researcher bias.
You can mitigate the disadvantages of non-probability sampling by describing your choices in the methodology section of your dissertation . Specifically, explain how your sample would differ from one that was randomly selected and mention any subjects who might be excluded or overrepresented in your sample.
When your population is large in size, geographically dispersed, or difficult to contact, it’s necessary to use a sampling method .
This allows you to gather information from a smaller part of the population, i.e. the sample, and make accurate statements by using statistical analysis. A few sampling methods include simple random sampling , convenience sampling , and snowball sampling .
A sampling frame is a list of every member in the entire population . It is important that the sampling frame is as complete as possible, so that your sample accurately reflects your population.
A sample is a subset of individuals from a larger population. Sampling means selecting the group that you will actually collect data from in your research.
For example, if you are researching the opinions of students in your university, you could survey a sample of 100 students.
Statistical sampling allows you to test a hypothesis about the characteristics of a population. There are various sampling methods you can use to ensure that your sample is representative of the population as a whole.
In stratified sampling , researchers divide subjects into subgroups called strata based on characteristics that they share (e.g., race, gender, educational attainment).
Once divided, each subgroup is randomly sampled using another probability sampling method .
Stratified and cluster sampling may look similar, but bear in mind that groups created in cluster sampling are heterogeneous , so the individual characteristics in the cluster vary. In contrast, groups created in stratified sampling are homogeneous , as units share characteristics.
Relatedly, in cluster sampling you randomly select entire groups and include all units of each group in your sample. However, in stratified sampling, you select some units of all groups and include them in your sample. In this way, both methods can ensure that your sample is representative of the target population .
Cite this Scribbr article
If you want to cite this source, you can copy and paste the citation or click the ‘Cite this Scribbr article’ button to automatically add the citation to our free Reference Generator.
Nikolopoulou, K. (2022, October 10). What Is Non-Probability Sampling? | Types & Examples. Scribbr. Retrieved 15 April 2024, from https://www.scribbr.co.uk/research-methods/nonprobability-sampling/
Is this article helpful?
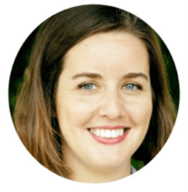
Kassiani Nikolopoulou
Other students also liked, sampling methods | types, techniques, & examples, operationalisation | a guide with examples, pros & cons, what is probability sampling | types & examples.
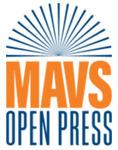
Want to create or adapt books like this? Learn more about how Pressbooks supports open publishing practices.
6.3 Probability sampling
Learning objectives.
- Describe how probability sampling differs from nonprobability sampling
- Define generalizability, and describe how it is achieved in probability samples
- Identify the various types of probability samples, and describe why a researcher may use one type over another
Quantitative researchers are often interested in making generalizations about groups larger than their study samples — that is, they are seeking nomothetic causal explanations. While there are certainly instances when quantitative researchers rely on nonprobability samples (e.g., when doing exploratory research), quantitative researchers tend to rely on probability sampling techniques. The goals and techniques associated with probability samples differ from those of nonprobability samples. We’ll explore those unique goals and techniques in this section.
Probability sampling
Unlike nonprobability sampling, probability sampling refers to sampling techniques for which a person’s likelihood of being selected from the sampling frame is known. You might ask yourself why we should care about a potential participant’s likelihood of being selected for the researcher’s sample. The reason is that, in most cases, researchers who use probability sampling techniques are aiming to identify a representative sample from which to collect data. A representative sample is one that resembles the population from which it was drawn in all the ways that are important for the research being conducted. If, for example, you wish to be able to say something about differences between men and women at the end of your study, you better make sure that your sample doesn’t contain only women. That’s a bit of an oversimplification, but the point with representativeness is that if your population contains variations that are important to your study, your sample should contain the same sorts of variation.
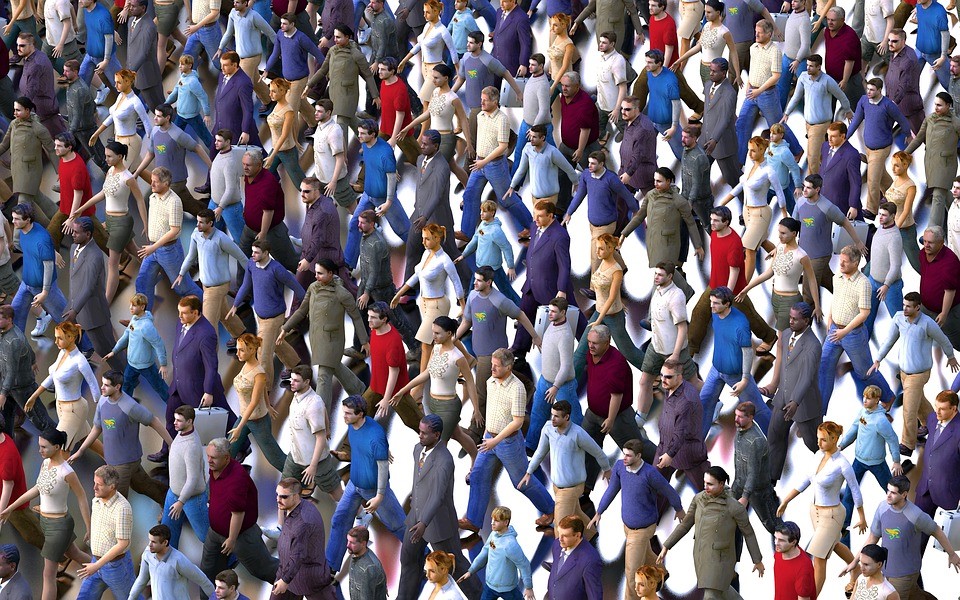
Obtaining a representative sample is important in probability sampling because of generalizability. In fact, generalizability is perhaps the key feature that distinguishes probability samples from nonprobability samples. Generalizability refers to the idea that a study’s results will tell us something about a group larger than the sample from which the findings were generated. In order to achieve generalizability, a core principle of probability sampling is that all elements in the researcher’s sampling frame have an equal chance of being selected for inclusion in the study. In research, this is the principle of random selection . Researchers often use a computer’s random number generator to determine which elements from the sampling frame get recruited into the sample.
Using random selection does not mean that the sample will be perfect. No sample is perfect. The only way to come with a sample that perfectly reflects the population would be to include everyone in the population in your sample, which defeats the whole point of sampling! Generalizing from a sample to a population always contains some degree of error. This is referred to as sampling error, the difference between results from a sample and the actual values in the population.
Generalizability is a pretty easy concept to grasp. Imagine a professor who takes a sample of individuals in your class to see if the material is too hard or too easy. The professor, however, only sampled individuals whose grades were over 90% in the class. Would that be a representative sample of all students in the class? That would be a case of sampling error—a mismatch between the results of the sample and the true feelings of the overall class. In other words, the results of the professor’s study don’t generalize to the overall population of the class.
Taking this one step further, imagine your professor is conducting a study on binge drinking among college students. The professor uses undergraduates at your school as her sampling frame. Even if that professor were to use probability sampling, perhaps your school differs from other schools in important ways. There are schools that are “party schools” where binge drinking may be more socially accepted, “commuter schools” at which there is little nightlife, and so on. If your professor plans to generalize her results to all college students, she will have to make an argument that her sampling frame (undergraduates at your school) is representative of the population (all undergraduate college students).
Types of probability samples
There are a variety of types of probability samples that researchers may use. These include simple random samples , systematic random samples , stratified random samples , and cluster samples . Let’s build on the previous example. Imagine we were concerned with binge drinking and chose the target population of fraternity members. How might you go about getting a probability sample of fraternity members that is representative of the overall population?
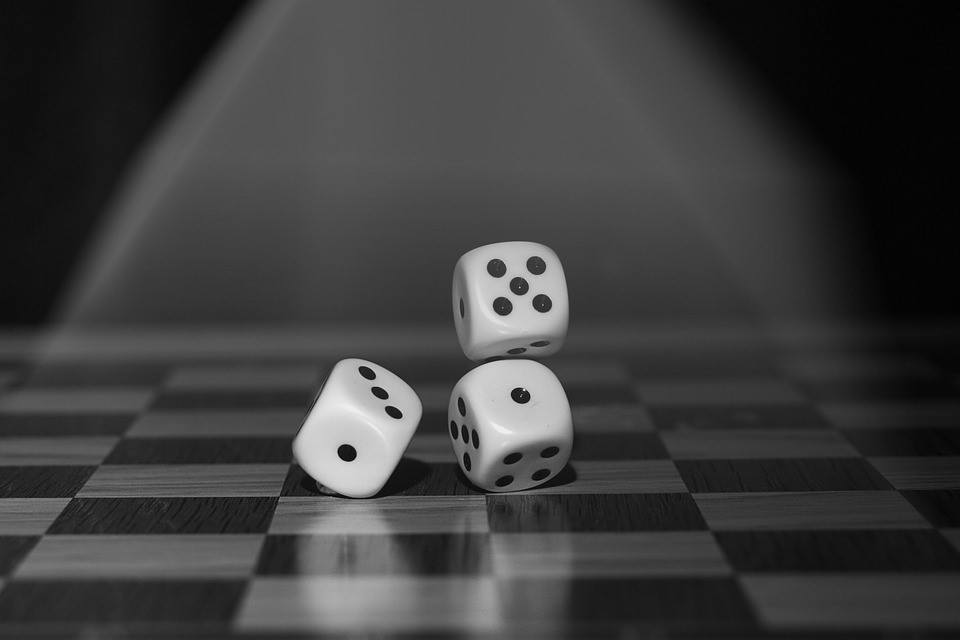
Simple random sampling
Simple random samples are the most basic type of probability sample. A simple random sample requires a sampling frame than contains a list of each person in the sampling frame. Your school likely has a list of all of the fraternity members on campus, as Greek life is subject to university oversight. You could use this as your sampling frame. Using the university’s list, you would number each fraternity member, or element , sequentially and then randomly select the elements from which you will collect data.
True randomness is difficult to achieve, and it takes complex computational calculations to do so. Although you think you can select things at random, human-generated randomness is actually quite predictable. To truly randomly select elements, researchers must rely on computer-generated help. Many free websites have good pseudo-random number generators. A good example is the website Random.org , which contains a random number generator that can also randomize lists of participants. Sometimes, researchers use a table of numbers that have been generated randomly. There are several possible sources for obtaining a random number table. Some statistics and research methods textbooks offer such tables as appendices to the text.
Systematic random sampling
As you might have guessed, drawing a simple random sample can be quite tedious. Systematic random sampling techniques are somewhat less tedious but offer the benefits of a random sample. As with simple random samples, you must possess a list of everyone in your sampling frame. Once you’ve done that, to draw a systematic sample you’d simply select every k th element on your list. But what is k , and where on the list of population elements does one begin the selection process? k is your selection interval or the distance between the elements you select for inclusion in your study. To begin the selection process, you’ll need to figure out how many elements you wish to include in your sample. Let’s say you want to interview 25 fraternity members on your campus, and there are 100 men on campus who are members of fraternities. In this case, your selection interval, or k , is 4. To arrive at 4, simply divide the total number of population elements by your desired sample size. This process is represented in Figure 6.2.
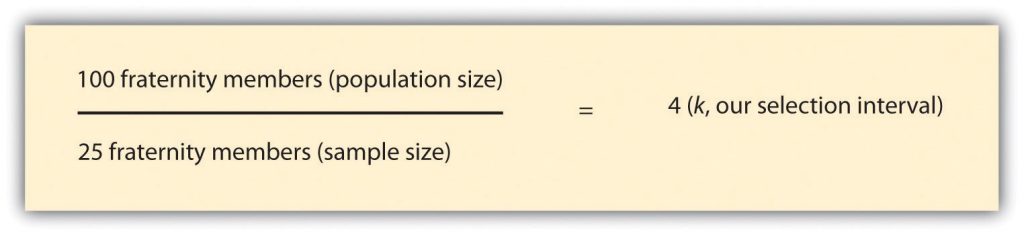
To determine where on your list of population elements to begin selecting the names of the 25 men you will interview, randomly select a number between 1 and k, and begin there. If we randomly select 3 as our starting point, we’d begin by selecting the third fraternity member on the list and then select every fourth member from there. This might be easier to understand if you can see it visually. Table 6.2 lists the names of our hypothetical 100 fraternity members on campus. You’ll see that the third name on the list has been selected for inclusion in our hypothetical study, as has every fourth name after that. A total of 25 names have been selected.
There is one clear instance in which systematic sampling should not be employed. If your sampling frame has any pattern to it, you could inadvertently introduce bias into your sample by using a systemic sampling strategy. (Bias will be discussed in more depth in the next section.) This is sometimes referred to as the problem of periodicity. Periodicity refers to the tendency for a pattern to occur at regular intervals. Let’s say, for example, that you wanted to observe binge drinking on campus each day of the week. Perhaps you need to have your observations completed within 28 days and you wish to conduct four observations on randomly chosen days. Table 6.3 shows a list of the population elements for this example. To determine which days we’ll conduct our observations, we’ll need to determine our selection interval. As you’ll recall from the preceding paragraphs, to do so we must divide our population size, in this case 28 days, by our desired sample size, in this case 4 days. This formula leads us to a selection interval of 7. If we randomly select 2 as our starting point and select every seventh day after that, we’ll wind up with a total of 4 days on which to conduct our observations. You’ll see how that works out in the following table.
Do you notice any problems with our selection of observation days in Table 6.3? Apparently, we’ll only be observing on Tuesdays. Moreover, Tuesdays may not be an ideal day to observe binge drinking behavior because binge drinking may be more likely to happen over the weekend.
Stratified random sampling
Another type of random sampling that could be helpful in cases such as this is stratified random sampling. In stratified random sampling , a researcher divides the sampling frame into relevant subgroups and then draw a sample from each subgroup. In this example, we might wish to first divide our sampling frame into two lists: weekend and weekdays. Once we have our two lists, we can then apply either simple random or systematic sampling techniques to each subgroup.
Stratified sampling is a good technique to use when, as in our example, a subgroup of interest makes up a relatively small proportion of the overall sample. In our example of a study of binge drinking, we want to include weekdays and weekends in our sample, but because weekends make up less than a third of an entire week, there’s a chance that a simple random or systematic strategy would not yield sufficient weekend observation days. As you might imagine, stratified sampling is even more useful in cases where a subgroup makes up an even smaller proportion of the sampling frame—for example, if we want to be sure to include in our study students who are in year five of their undergraduate program but this subgroup makes up only a small percentage of the population of undergraduates. There’s a chance simple random or systematic sampling strategy might not yield any fifth-year students, but by using stratified sampling, we could ensure that our sample contained the proportion of fifth-year students that is reflective of the larger population.
In this case, class year (e.g., freshman, sophomore, junior, senior, and fifth-year and higher) is our strata , or the characteristic by which the sample is divided. In using stratified sampling, we are often concerned with how well our sample reflects the population. A sample with too many freshmen may skew our results in one direction because perhaps they binge drink more (or less) than students in other class years. Proportionate stratified random sampling allows us to make sure our sample has the same proportion of people from each class year as the overall population of the school. Disproportionate stratified random samplin g allows us to over-sample smaller groups to ensure we have enough elements from the smaller group(s) for statistical analyses.
Cluster sampling
Up to this point in our discussion of probability samples, we’ve assumed that researchers will be able to access a list of population elements in order to create a sampling frame. This, as you might imagine, is not always the case. Let’s say, for example, that you wish to conduct a study of binge drinking across fraternity members at each undergraduate program in your state. Just imagine trying to create a list of every single fraternity member in the state. Even if you could find a way to generate such a list, attempting to do so might not be the most practical use of your time or resources. When this is the case, researchers turn to cluster sampling. Cluster sampling occurs when a researcher begins by randomly sampling groups (or clusters) of population elements and then selects elements from within those groups.
Let’s work through how we might use cluster sampling in our study of binge drinking. While creating a list of all fraternity members in your state would be next to impossible, you could easily create a list of all undergraduate colleges in your state. Thus, you could draw a random sample of undergraduate colleges (your cluster) and then draw another random sample of elements (in this case, fraternity members) from within the undergraduate college you initially selected. Cluster sampling works in stages. In this example, we sampled in two stages— (1) undergraduate colleges and (2) fraternity members at the undergraduate colleges we selected. However, we could add another stage if it made sense to do so. We could randomly select (1) undergraduate colleges (2) specific fraternities at each school and (3) individual fraternity members. As you might have guessed, sampling in multiple stages does introduce the possibility of greater error (each stage is subject to its own sampling error), but it is nevertheless highly efficien.
Jessica Holt and Wayne Gillespie (2008) used cluster sampling in their study of students’ experiences with violence in intimate relationships. Specifically, the researchers randomly selected 14 classes on their campus and then drew a random subsample of students from those classes. But you probably know from your experience with college classes that not all classes are the same size. So, if Holt and Gillespie had simply randomly selected 14 classes and then selected the same number of students from each class to complete their survey, then students in the smaller of those classes would have had a greater chance of being selected for the study than students in the larger classes. Keep in mind, with random sampling the goal is to make sure that each element has the same chance of being selected. When clusters are of different sizes, as in the example of sampling college classes, researchers often use a method called probability proportionate to size (PPS). This means that they take into account that their clusters are of different sizes. They do this by giving clusters different chances of being selected based on their size so that each element within those clusters winds up having an equal chance of being selected.
Comparing random sampling techniques
To summarize, probability samples are used to help a researcher make conclusions about larger groups. Probability samples require a sampling frame from which elements, usually human beings, can be selected at random from a list. The use of random selection reduces the error and bias present in the nonprobability sample types reviewed in the previous section, though some error will always remain. This strength is common to all probability sampling approaches summarized in Table 6.4.
In determining which probability sampling approach makes the most sense for your project, it helps to know more about your population. A simple random sample and systematic random sample are relatively similar to carry out. They both require a list of all elements in your sampling frame. Systematic random sampling is slightly easier in that it does not require you to use a random number generator for each element; instead it uses a sampling interval that is easy to calculate by hand.
The relative simplicity of both approaches is counter-weighted by their lack of sensitivity to characteristics in of your population. Stratified random samples help ensure that smaller subgroups are included in your sample, thus making the sample more representative of the overall population or allowing statistical analyses on subgroup differences possible. While these benefits are important, creating strata for this purpose requires knowing information about your population before beginning the sampling process. In our binge drinking example, we would need to know how many students are in each class year to make sure our sample contained the same proportions. We would need to know that, for example, fifth-year students make up 5% of the student population to make sure 5% of our sample is comprised of fifth-year students. If the true population parameters are unknown, stratified sampling becomes significantly more challenging.
Common to each of the previous probability sampling approaches is the necessity of using a real list of all elements in your sampling frame. Cluster sampling is different. It allows a researcher to perform probability sampling in cases for which a list of elements is not available or pragmatic to create. Cluster sampling is also useful for making claims about a larger population, in our example, all fraternity members within a state. However, because sampling occurs at multiple stages in the process, in our example at the university and student level, sampling error increases. For many researchers, this weakness is outweighed by the benefits of cluster sampling.
Key Takeaways
- In probability sampling, the aim is to identify a sample that resembles the population from which it was drawn.
- There are several types of probability samples including simple random samples, systematic samples, stratified samples, and cluster samples.
- Probability samples usually require a real list of elements in your sampling frame, though cluster sampling can be conducted without one.
- Cluster sampling- a sampling approach that begins by sampling groups (or clusters) of population elements and then selects elements from within those groups
- Disproportionate stratified random sampling-stratified random sampling where the proportion of elements from each group is not proportionate to that in the population (usually used to oversample small groups).
- Generalizability – the idea that a study’s results will tell us something about a group larger than the sample from which the findings were generated
- Periodicity- the tendency for a pattern to occur at regular intervals
- Probability proportionate to size- in cluster sampling, giving clusters different chances of being selected based on their size so that each element within those clusters has an equal chance of being selected
- Probability sampling- sampling approaches for which a person’s (or element’s) likelihood of being selected from the sampling frame is known
- Proportionate stratified random sampling-stratified random sampling where the proportion of elements from each group is proportionate to that in the population
- Random selection- using a randomly generated numbers to determine who from the sampling frame gets recruited into the sample
- Representative sample- a sample that resembles the population from which it was drawn in all the ways that are important for the research being conducted
- Sampling error- a statistical calculation of the difference between results from a sample and the actual parameters of a population
- Simple random sampling- selecting elements from a list using randomly generated numbers
- Strata- the characteristic by which the sample is divided
- Stratified random sampling- dividing the study population into relevant subgroups and then draw a sample from each subgroup
- Systematic random sampling- selecting every k th element from a list
Image attributions
crowd men women by DasWortgewand CC-0
roll the dice by 955169 CC-0
Figure 10.2 copied from Blackstone, A. (2012) Principles of sociological inquiry: Qualitative and quantitative methods. Saylor Foundation. Retrieved from: https://saylordotorg.github.io/text_principles-of-sociological-inquiry-qualitative-and-quantitative-methods/ Shared under CC-BY-NC-SA 3.0 License
Foundations of Social Work Research Copyright © 2020 by Rebecca L. Mauldin is licensed under a Creative Commons Attribution-NonCommercial-ShareAlike 4.0 International License , except where otherwise noted.
Share This Book

IMAGES
VIDEO
COMMENTS
Non-probability sampling is a sampling method that uses non-random criteria like the availability, geographical proximity, or expert knowledge of the individuals you want to research in order to answer a research question. Non-probability sampling is used when the population parameters are either unknown or not possible to individually identify.
Qualitative Research: Non-probability sampling is commonly used in qualitative research where the focus is on gaining an in-depth understanding of a particular phenomenon or context. Qualitative research often uses purposive sampling to identify participants who have the knowledge or experience needed to provide rich and detailed insights ...
What is a sampling plan? A sampling plan is a formal plan specifying a sampling method, a sample size, and procedure for recruiting participants (Box 1) [].A qualitative sampling plan describes how many observations, interviews, focus-group discussions or cases are needed to ensure that the findings will contribute rich data.
Definition:Non-probability sampling is defined as a sampling technique in which the researcher selects samples based on the subjective judgment of the researcher rather than random selection. It is a less stringent method. This sampling method depends heavily on the expertise of the researchers. It is carried out by observation, and researchers ...
Mine tends to start with a reminder about the different philosophical assumptions undergirding qualitative and quantitative research projects ( Staller, 2013 ). As Abrams (2010) points out, this difference leads to "major differences in sampling goals and strategies." (p.537). Patton (2002) argues, "perhaps nothing better captures the ...
Non-probability sampling (sometimes nonprobability sampling) is a branch of sample selection that uses non-random ways to select a group of people to participate in research. Unlike probability sampling and its methods, non-probability sampling doesn't focus on accurately representing all members of a large population within a smaller sample ...
Non-probability sampling techniques are the best approach for qualitative research. Because the researcher seeks a strategically chosen sample, generalizability is more of a theoretical or conceptual issue, and it is not possible to generalize back to the population (Palys & Atchison, 2014). Probabilistic sampling techniques
Abstract. Knowledge of sampling methods is essential to design quality research. Critical questions are provided to help researchers choose a sampling method. This article reviews probability and non-probability sampling methods, lists and defines specific sampling techniques, and provides pros and cons for consideration.
Purposive Sampling. Purposive (or purposeful) sampling is a non-probability technique used to deliberately select the best sources of data to meet the purpose of the study. Purposive sampling is sometimes referred to as theoretical or selective or specific sampling. Theoretical sampling is used in qualitative research when a study is designed ...
Nonprobability sampling. Nonprobability sampling refers to sampling techniques for which a person's likelihood of being selected for membership in the sample is unknown. Because we don't know the likelihood of selection, with nonprobability samples we don't know whether a sample is likely to represent a larger population. But that's okay.
Non-probability sampling techniques are the best approach for qualitative research. Because the researcher seeks a strategically chosen sample, generalizability is more of a theoretical or conceptual issue, and it is not possible to generalize back to the population (Palys & Atchison, 2014).
Many common examples of non-probability sampling can be found in our day-to-day lives. Whenever you receive a customer feedback survey on a receipt, a company uses non-probability sampling. Political organizations that go door-to-door soliciting opinions are engaging in non-probability sampling. An employee survey that excludes managers from ...
Non-Probability Sampling. Non-probability sampling is a non-random sampling technique. This means that it does not give every individual in a population or sampling frame equal chances of being selected. This means that the sample may be less representative of the target population compared to random sampling.
Key Takeaways: Sampling techniques in qualitative research include purposive, convenience, snowball, and theoretical sampling. Choosing the right sampling technique significantly impacts the accuracy and reliability of the research results. It's crucial to consider the potential impact on the bias, sample diversity, and generalizability when ...
Concerns with sampling in qualitative research focus on discovering the scope and the nature of the universe to be sampled. ... The newlyweds settle on whatever space is available but plan to move later to the more socially preferred (e.g., matralineal) sites. ... identify five major types of nonprobability sampling techniques for qualitative ...
Types Of Non-probability Sampling With Examples. 1. Convenience Sampling. Convenience sampling involves selecting participants based on accessibility and proximity to the researcher. It's handy when time and resources are limited. For example, a market research company posts a survey link across popular online communities and forums related to ...
A key stage in the qualitative research process is determining the nature of the study population and then sampling from this population so that data can then be collected. In qualitative research ...
Sampling types. There are two major categories of sampling methods ( figure 1 ): 1; probability sampling methods where all subjects in the target population have equal chances to be selected in the sample [ 1, 2] and 2; non-probability sampling methods where the sample population is selected in a non-systematic process that does not guarantee ...
Non-Probability Sampling. In non-probability sampling (also known as non-random sampling) not all members of the population have a chance to participate in the study. In other words, this method is based on non-random selection criteria. This is contrary to probability sampling, where each member of the population has a known, non-zero chance ...
Non-probability sampling is a sampling method that uses non-random criteria like the availability, geographical proximity, or expert knowledge of the individuals you want to research in order to answer a research question. Non-probability sampling is used when the population parameters are either unknown or not possible to individually identify.
of non -/ probabilistic sampling wich can be used with succes by the romanian firms in marketing research. Key words: non - probabilistic sampling, convenience sampling, volunteer sampling 1.
In order to achieve generalizability, a core principle of probability sampling is that all elements in the researcher's sampling frame have an equal chance of being selected for inclusion in the study. In research, this is the principle of random selection. Researchers often use a computer's random number generator to determine which ...