- Research article
- Open access
- Published: 27 August 2020

Type2 diabetes mellitus prediction using data mining algorithms based on the long-noncoding RNAs expression: a comparison of four data mining approaches
- Faranak Kazerouni 1 ,
- Azadeh Bayani 2 ,
- Farkhondeh Asadi 2 ,
- Leyla Saeidi 3 ,
- Nasrin Parvizi 4 &
- Zahra Mansoori 1
BMC Bioinformatics volume 21 , Article number: 372 ( 2020 ) Cite this article
6110 Accesses
33 Citations
3 Altmetric
Metrics details
About 90% of patients who have diabetes suffer from Type 2 DM (T2DM). Many studies suggest using the significant role of lncRNAs to improve the diagnosis of T2DM. Machine learning and Data Mining techniques are tools that can improve the analysis and interpretation or extraction of knowledge from the data. These techniques may enhance the prognosis and diagnosis associated with reducing diseases such as T2DM. We applied four classification models, including K-nearest neighbor (KNN), support vector machine (SVM), logistic regression, and artificial neural networks (ANN) for diagnosing T2DM, and we compared the diagnostic power of these algorithms with each other. We performed the algorithms on six LncRNA variables (LINC00523, LINC00995, HCG27_201, TPT1-AS1, LY86-AS1, DKFZP) and demographic data.
To select the best performance, we considered the AUC, sensitivity, specificity, plotted the ROC curve, and showed the average curve and range. The mean AUC for the KNN algorithm was 91% with 0.09 standard deviation (SD); the mean sensitivity and specificity were 96 and 85%, respectively. After applying the SVM algorithm, the mean AUC obtained 95% after stratified 10-fold cross-validation, and the SD obtained 0.05. The mean sensitivity and specificity were 95 and 86%, respectively. The mean AUC for ANN and the SD were 93% and 0.03, also the mean sensitivity and specificity were 78 and 85%. At last, for the logistic regression algorithm, our results showed 95% of mean AUC, and the SD of 0.05, the mean sensitivity and specificity were 92 and 85%, respectively. According to the ROCs, the Logistic Regression and SVM had a better area under the curve compared to the others.
We aimed to find the best data mining approach for the prediction of T2DM using six lncRNA expression. According to the finding, the maximum AUC dedicated to SVM and logistic regression, among others, KNN and ANN also had the high mean AUC and small standard deviations of AUC scores among the approaches, KNN had the highest mean sensitivity and the highest specificity belonged to SVM. This study’s result could improve our knowledge about the early detection and diagnosis of T2DM using the lncRNAs as biomarkers.
Diabetes mellitus (DM) is one of the most prevalent chronic non-communicable diseases (NCD) around the world; about 90% of the patients who have diabetes suffer from Type 2 DM (T2DM) [ 1 ]. The risk of developing T2DM is strongly associated with many predispositions, behavioral, and environmental risk factors and also genetic factors [ 1 , 2 , 3 , 4 ]. Besides the genetic factors, strong evidence indicates that factors such as obesity and physical inactivity are the main nongenetic determinants of the disease [ 5 , 6 ]. T2DM can range from predominant insulin resistance with relative insulin deficiency to dominant defective secretion with insulin resistance [ 4 ]. It is often related to metabolic syndrome problems. Individuals who have impaired glucose tolerance are high-risk subjects of type 2 diabetes [ 6 ].
Studies demonstrate a drastic increase of the disease in recent decades. The trends estimate that by 2035, more than 520 million people will be affected by the disease [ 7 ]. People who suffer from T2DM are susceptible to many forms of complications leading to morbidity and mortality in these patients. Many studies emphasize the genetic factors in the pathogenesis of T2DM [ 3 , 8 , 9 ]. Long non-coding RNAs (long ncRNAs, lncRNA) are subsets of RNA, specified as being transcripts with lengths exceeding 200 nucleotides that could not be translated into protein [ 10 ]. Long non-coding RNAs (lncRNAs) belong to a heterogeneous class of regulatory lncRNAs with transcript lengths > 200 nucleotides, which have a positive role in the development and growth of several various diseases including T2DM supporting the hypothesis that abnormal expression of LncRNAs is related to various diseases [ 11 ]. Besides, considering the significant role of lncRNAs in disease pathogenesis, increasing researches suggest using them to improve diagnosis, prognosis, and clinical management of T2DM. Genome-wide association studies (GWAS) have recently introduced several particular diabetes-related loci in the human genome [ 3 ]. Also, many studies discovered the relationship between more than 100 susceptible loci and T2DM at a genome-wide significant level [ 3 , 8 , 12 ]. Deregulation of genes located in GWAS defined loci may be risk factors for human diseases concerning which we applied the GWAS catalog to select six lncRNAs (LINC00523, LINC00995, CG27_201, TPT1-AS1,LY86-AS1, DKFZP) as our gene targets for the present study [ 3 ]. Knowledge Discovery in Databases (KDD) or data mining are techniques for the computational process of discovering patterns in large datasets containing various approaches such as artificial intelligence, machine learning, statistics, and database systems [ 13 ]. These methods are applied to recognize patterns in data, prediction, association, and classification problems [ 1 , 2 , 8 , 13 ]. Considering the importance of early detection of T2DM, machine learning and Data Mining techniques are tools that can improve the analysis and interpretation or extraction of knowledge from the data [ 14 , 15 ]. These techniques may enhance the prognosis and diagnosis associated with life quality, reducing diseases such as T2DM [ 15 , 16 ].
To date, several other studies tried to predict diabetes mellitus using outstanding data mining techniques [ 17 , 18 , 19 ]. Vijayan et al. [ 20 ] applied the expectation-maximization algorithm, KNN algorithm, K-means algorithm, amalgam KNN algorithm, and ANFIS algorithm to predict and diagnose Diabetes Mellitus. They used the UCI dataset containing blood test and demographic variables, and their results showed that EM possessed the least classification accuracy and amalgam KNN, and ANFIS provided better classification accuracy of more than 80 and 80%, respectively. Another study conducted by Saravananathan et al. [ 21 ] used popular classification algorithms, including J48, Support Vector Machines (SVM) Classification and Regression, Tree CART, and k-Nearest Neighbor (kNN) for diabetic data. Their performance indicators were accuracy, specificity, sensitivity, precision, error rate. They found that the J48 technique’s performance was remarkably superior to the other three techniques for the classification of diabetes data. Meng et al. [ 18 ] compared three data mining models of logistic regression, ANN, and decision tree for predicting diabetes mellitus or prediabetes by risk factors. They gathered information about demographic characteristics, family diabetes history, anthropometric measurements, and lifestyle risk. The decision tree model (C5.0) had the best classification performance with an accuracy of 77.87% with a sensitivity of 80.68% and specificity of 75.13%. Another study performed by Saeidi et al. [ 3 ] used logistic regression to assess the diagnostic value of LY86-AS1 and HCG27_201 as biomarkers for T2DM. They obtained a sensitivity of 64.6%, and specificity of 79.8%. Another study [ 2 ] used two other lncRNAs, including LINC00523 and LINC00994 expressions, for the evaluation of their potential diagnostic value for T2DM. They applied logistic regression and achieved a sensitivity of 81.44% and specificity of 61.11%. In our study, we combined six lncRNAs as variables for the first time and applied four classification models, including classification algorithms like K-nearest neighbor (KNN), support vector machine (SVM), logistic regression, and artificial neural networks (ANN) for diagnosing T2DM, and we compared the diagnostic power of these algorithms with each other. In the present study, we aimed to find the best data mining approach for the prediction of T2DM using six lncRNA expression. The result of this study could improve our knowledge about the early detection and diagnosis of T2DM using the lncRNAs as biomarkers [ 22 ].
The primary aim of the present study was to implement four models to predict DT2M applying data mining techniques based on the lncRNA variables. The research objectives of our study were:
Implementing data mining techniques for prediction of the DT2M.
Comparing the applied methods.
selecting the best model for the T2DM prediction.
We used the variables for predicting T2DM and comparing the performance of the various data mining techniques. For the implementation of the algorithms, we used ANACONDA3–5.2.0 64 bit a free and open-source platform distribution of python programming language with a vast number of modules, packages, and rich libraries that provide various methods for classification problems. For obtaining the best amount of performance in the models, 10-fold cross-validation performed on the dataset. In dealing with the small data sets, cross-validation is a prominent strategy for estimating the performance. Cross-Validation is a performance evaluation technique commonly used in practice. Here, the data set is repeatedly partitioned into two non-overlapping parts, a training set, and a hold-out set. For each partitioning, the hold-out set is used for testing, while the remainder is used for training. The two most popular variants are ten-fold cross-validation (10-fold CV), where the data is split into ten mutually disjoint folds [ 23 ].
Since our samples were more than 100, and to be sure that each fold contains the same proportion of healthy and diabetic individuals, we used the stratified 10-fold cross-validation approach [ 24 ]. Therefore, the results are reliable and more credible.
We applied four popular data mining approaches on the lncRNA variables, regression, k-nearest neighbors, SVM, and neural network classification algorithms.
KNN algorithm
The k-nearest neighbor’s algorithm (k-NN) is an algorithm for classifying variables regarding the closest training data in the feature space. K-NN uses an instance-based learning method, which is one of the simplest algorithms among data mining techniques. This method considers the nearest neighbors to each object and decides to dedicate the object to classes [ 22 , 25 ].
SVM algorithm
Support Vector Machine (SVM) is a supervised algorithm which divides the feature space called hyperplanes considering the target classes. SVM computes classification by maximizing the margin of the hyperplane that intercepts classes. This algorithm plots a multidimensional hyperplane that divides classes and increases the margin between classes to enhance the accuracy of classification. We used different kernel functions embedded in the SVM class of SVC library in python framework as a quadratic, polynomial, radial basis, etc. to classify the instance and to detect the best accuracy among them [ 25 , 26 , 27 ].
Artificial neural network
Artificial Neural Network is a data processing algorithm that simulates the biological neural network in its computations. A common problem in using ANN is that they act fundamentally as a black box and the parameters are set by the model so we cannot demonstrate them [ 28 ], we can just apply the model in our problems and obtain the high performance. We used Multilayer Perceptron Neural Networks (MLPNN). The structure of a multi-layer perceptron neural network has been demonstrated in Fig. 1 . It maps a set of input data into a set of appropriate output classes. It includes three layers input layer, hidden layer & output layer. The principal function of neurons of the input layer is to divide input Xi into neurons in the hidden layer. The neuron of the hidden layer adds the appropriate weights of Wij to the input variables. The output formula is:
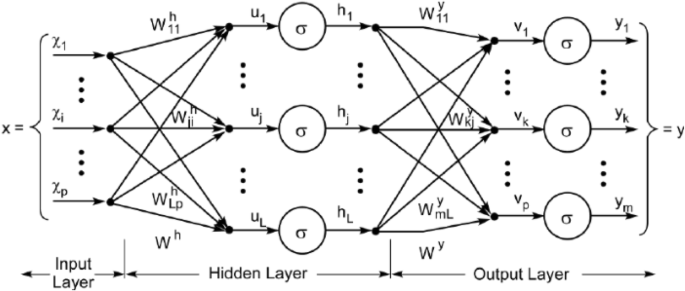
Artificial Neural Network structure
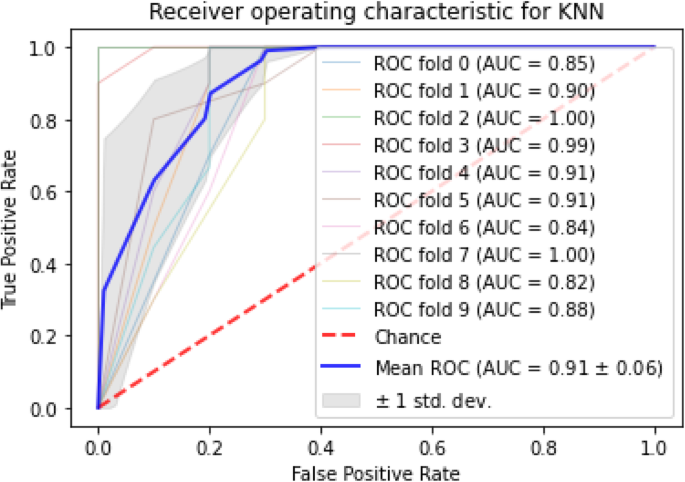
The ROC for KNN
Where f is a simple threshold function that we considered sigmoid and hyperbolic tangent function [ 25 ].
In the present study, a Multi-layer Perceptron Neural Networks (MLPNN) was performed. The structure of MLPNN is as shown in Fig. 1 . It makes a map of input data onto a set of suitable output data.
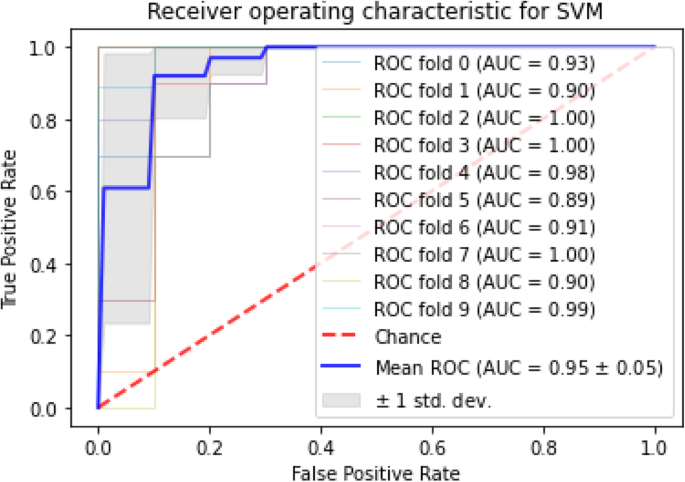
The ROC for SVM
The RBF networks are another type of neural network. In MLP, each neuron considers the weighted sum of its input values, in which each input value is multiplied by a coefficient, and the results are the sum of values. RBF is a more intuitive approach to MLP. An RBFN classifies the inputs by calculating the input’s similarity to examples from the training set. Each RBFN neuron stores one of the examples from the training set as a “prototype.” for classification of new input, in each neuron, the Euclidean distance between the input and its prototype is calculated. The input is dedicated to a class when it has more similar to that class than the other classes.
Logistic regression
Logistic regression is a common approach for predictive modeling practices. The function p(X) provides probability output between 0 and 1 for all values of X, where X1–Xp are the predictors. The coefficients β0–βp are estimated using maximum likelihood estimation
This study was based on the data obtained from three previous research conducted by Saeidi et al. and Mansoori et al. [ 2 , 3 ] and the research of Parvizi and colleagues, which is not published yet. We integrated these three studies, and our data mining analysis was implemented in their studies. The data were collected from 200 unrelated Iranian subjects, 100 T2DM patients, and 100 healthy individuals, matched for age and sex. T2DM patients were recruited from individuals who referred to the Diabetic Clinic at Shohada Hospital, Tehran, Iran. In the current study, we applied six lncRNAs expression and also six demographic variables, including sex, age, weight, height, BMI, and FBS for analysis and inputs of algorithms. For the preprocessing phase, we normalized the data inputs for KNN, SVM, and ANN models. We also had low missing variables, and we replaced them with zero (Table 1 ).
lncRNA extraction and selection
Increasing evidence has suggested several lncRNAs are implicated in T2DM pathogenesis. Recently, human β-cell transcriptome analysis showed lncRNAs dynamic regulation and abnormal expression of lncRNAs in T2DM [ 29 ]. However, the extent of lncRNA deregulation in T2DM has yet to be determined. To date, more than100 susceptibility loci have been identified as being associated with T2DM at a genome-wide significant level [ 2 , 30 ]. Considering this into account and by querying the GWAS catalog, we candidated 6 lncRNAs (LY86-AS1, HCG27_201, LINC00523, LINC00994, TPT1-AS1and DKFZP) as target genes for this study.
The large scale GWAS have recognized approximately 80 SNPs that were susceptible to T2DM [ 31 ]. From there, we used the GWAS catalog access in June 2017 to create a list of SNPs associated with T2DM. In the current study, we selected six lncRNA for expression analysis according to the scan carried out in the study of Mansoori et al. [ 2 ] and Saeedi et al. [ 3 ] We selected variants that had associations with increased risk of T2DM. We applied a quantitative PCR analysis of lncRNA expression levels in the 200 samples. We calculated the respective amount of each lncRNAs applying the 2-ΔΔct as means of duplicate measurements.
Analysis and evaluation criteria
To select the best performance data mining algorithms in predicting diabetic patients, we considered AUC, sensitivity, specificity, and plotted ROC curve for the folds we ran and showed the average curve and its range [ 19 , 26 ].
Table 2 shows the significant downregulation of PBMC expressions of the variables in the T2DM group compared with the control group. The AUC of each classification technique has been demonstrated in Table 3 .
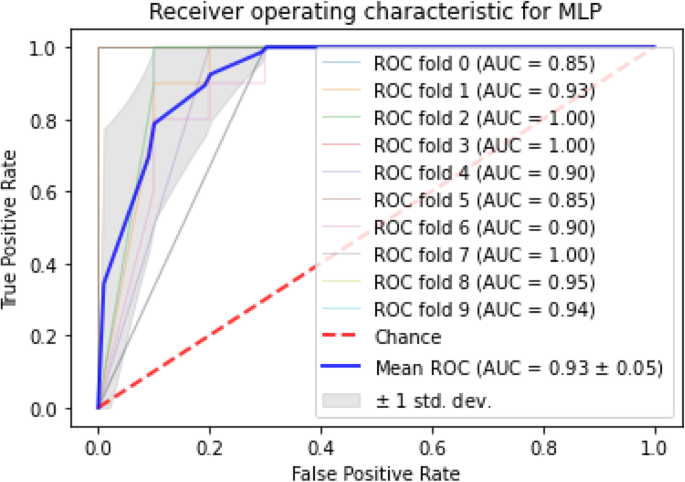
The ROC for MLP
AUC stands for “Area under the ROC Curve.” AUC computes the entire two-dimensional area under the whole ROC curve. According to the finding, the maximum AUC dedicated to SVM and logistic regression, among others, knn also had the highest mean AUC and minimum standard deviation of AUC scores among the approaches. The mean and standard deviation for AUC, sensitivity, and specificity of each algorithm is given in Table 4 . Apart from classification AUC, sensitivity, and specificity, the Receiver Operating Characteristic (ROC) with stratified cross-validation is shown for each approach in Figs. 2 , 3 , 4 and 5 .
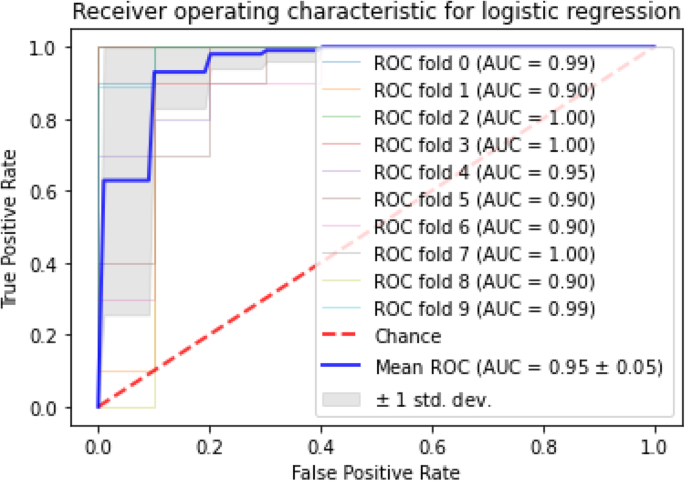
The ROC for logistic regression
ROC curves generally plot true positive rate on the Y-axis and false positive rate on the X-axis. In other words, a false positive rate of zero, and a true positive rate of one in the top left corner of the plot is called the ideal point. It means that a larger area under the curve (AUC) is usually better. According to the demonstrated ROCs, the KNN and SVM have a better area under the curve in comparison with the others.
For a medical diagnosis, optimized approaches to gain useful and accurate outcomes are essential. Applying machine learning and data mining methods to automate the process of diagnosis may assist practitioners to enhance the quality of their clinical decisions [ 32 , 33 ].
Since T2DM is one of the prevalent diseases with severe consequences [ 1 ], developing efficient methods for early detection of the disease was the primary purpose of our research.
Regardless of high number of lncRNAs in the RNA profile of human, a few numbers of them has been proved to be biologically active. The role of the few lncRNAs has been identified but several studies discussed the significant impact of lncRNAs in diabetic people, which may represent the role of abnormal expression of lncRNAs in the incidence of T2DM [ 3 ]. According to the possible function of lncRNAs in the development of T2DM, we considered the expression levels of six lncRNAs in addition to the demographic data in 200 diabetic and healthy individuals for our study. To measure the expression of the lncRNAs we applied PBMCs which demonstrate an extensive proportion of the genes encoded in the human genome [ 3 ]. Several studies have investigated different machine learning and data mining methods to predict different diseases [ 15 , 19 , 22 , 34 , 35 ] such as heart diseases, thyroid tumors, and also diabetes type 2 diabetes prediction. In the present study, we combined four commonly used data mining algorithms (KNN, SVM, neural networks, and regression) to predict type 2 diabetes using 6 Long non-coding RNAs expression and the demographic variables for the first time, because most of the previous studies used blood test variables or the demographic data for their analysis. Receiver operating characteristic (ROC) analysis, AUC, sensitivity, and specificity measure was used to assess the diagnostic value of the six biomarkers for T2DM. The mean AUC for the KNN algorithm was obtained 91% and with 0.06 standard deviation, and we obtained the highest sensitivity (96% with the standard deviation of 0.06), among other approaches. After applying the SVM algorithm, the mean AUC obtained 95% after 10-folds with the standard deviation of 0.05, and the highest specificity, among other approaches, obtained 86% with the standard deviation of 0.01. For the ANN, we applied a multi-layer perceptron with five hidden layers, and the mean AUC of folds was 93%, and the standard deviation was 0.03. At last, for the logistic regression algorithm, our results showed 95% of mean AUC, and the standard deviation of 0.05. The lower standard deviations in the AUC scores of computed folds means the algorithm has worked with more performance [ 15 , 17 , 36 ]. Other studies investigated data mining algorithms for several diseases. Saravananathan and Velmurugan [ 21 ] applied several classification algorithms in their study to analyze diabetes data, including KNN. Sadri Sa’di et al. [ 36 ] compared three data mining algorithms to predict T2DM and gained 73% precision for ANN. Sidiq et al. [ 15 ]. reported about 92% accuracy for KNN and 96% accuracy for SVM algorithms applying for the Diagnosis of Various Thyroid Ailments. In another study for the heart diseases. The data mining algorithms indicated more than 70% accuracy. The investigated studies are in line with the findings of our study that these algorithms have a strong power for prediction and early detection of many diseases, including T2DM, and we obtained remarkably better accuracy for prediction, for example, the SVM and logistic regression accuracy were 95%. In our study, we also obtained a better accuracy for logistic regression that was 95% and, in comparison with other studies, is a strong point, for example, Saeidi et al. [ 3 ] conducted a study to review two Long non-coding RNA expressions in type 2 diabetes mellitus and with applying regressions reported about 65% accuracy. Another research [ 2 ] used two different Long non-coding RNA expressions in type 2 diabetes mellitus and found 81% of accuracy with the regression algorithm. In the present study, for the first time, we performed four data mining algorithms on six Long non-coding RNAs and compared their power with each other. We demonstrated that Long non-coding RNAs are effective biomarkers for data mining algorithms and have a feasible power to be applied for prediction of T2DM. Also, in this research, we optimized the parameters of every algorithm and used stratified 10-fold cross-validation to gain the best performance. To be mentioned, in the nearest neighbor’s algorithm, the parameter k was varied between one and nine to find the best-optimized method, and we selected k = 3 to have the best performance and the lowest standard deviation in the accuracy of the folds. In addition, in choosing the parameters of the artificial neural network, the number of hidden layer neurons significantly affects the accuracy of the network, so we set the parameters with two hidden layers with five and three neurons respectively to yield the best accuracy. Considering the standard deviation of scores for each algorithm, the KNN had the lowest std. Moreover, the highest accuracy among the algorithms was the SVM algorithm and Logistic regression, which had the maximum accuracy in folds, among others. We should mention that the strong points of our study are using demographic data and six Long non-coding RNAs and combining them to get the best detection power of T2DM and performing four outstanding data mining algorithms and comparing their performances. As the limitations of this study, we should account for the limited number of samples, which is due to the high costs of measuring the Long non-coding RNAs. No doubt, the higher number of samples would lead to higher performance and more reliable results.
In this paper, the performance of conventional data mining classification techniques has been calculated and compared, for a dataset of patients referred for the screening of type 2 diabetes to the Shohada Hospital, Iran. The biomarker applied in this study demonstrated high diagnostic value, and the diagnostic process is suitable, which could help in the diagnosis of prediabetes and T2DM.
The classification techniques compared were support vector machine, artificial neural network, decision tree, nearest neighbors, and logistic regression. In data mining, it is not possible to say one classification technique will always work best, and it often depends on the number of samples, their distribution, and the choosing of the right algorithm. In this research work, SVM and Logistic Regression had the best Area Under Curve among methods of classification with the mean AUC of 95%. KNN and ANN also had the high mean AUC and small standard deviations of AUC scores among the approaches, KNN had the highest mean sensitivity, and the highest mean specificity belonged to SVM.
For future works, performing other data mining and machine learning methods and using higher numbers of samples are recommended to enhance the performance.
Availability of data and materials
The datasets used and/or analyzed during the current study are available from the corresponding author on reasonable request.
Li X, Zhao Z, Gao C, Rao L, Hao P, Jian D, Li W, Tang H, Li M. The diagnostic value of whole blood lncRNA ENST00000550337. 1 for prediabetes and type 2 diabetes mellitus. Exp Clin Endocrinol Diabetes. 2017;125(06):377–83.
Article CAS Google Scholar
Mansoori Z, Ghaedi H, Sadatamini M, Vahabpour R, Rahimipour A, Shanaki M, Kazerouni F. Downregulation of long non-coding RNAs LINC00523 and LINC00994 in type 2 diabetes in an Iranian cohort. Mol Biol Rep. 2018;45(5):1227–33.
Saeidi L, Ghaedi H, Sadatamini M, Vahabpour R, Rahimipour A, Shanaki M, Mansoori Z, Kazerouni F. Long non-coding RNA LY86-AS1 and HCG27_201 expression in type 2 diabetes mellitus. Mol Biol Rep. 2018;45(6):2601–8.
Petersmann A, Nauck M, Müller-Wieland D, Kerner W, Müller UA, Landgraf R, Freckmann G, Heinemann L. Definition, classification, and diagnosis of diabetes mellitus. Exp Clin Endocrinol Diabetes. 2018;126(07):406–10.
Armoon B, Karimy M. Epidemiology of childhood overweight, obesity and their related factors in a sample of preschool children from Central Iran. BMC Pediatr. 2019;19(1):159.
Article Google Scholar
Tuomilehto J, Lindström J, Eriksson JG, Valle TT, Hämäläinen H, Ilanne-Parikka P, Keinänen-Kiukaanniemi S, Laakso M, Louheranta A, Rastas M. Prevention of type 2 diabetes mellitus by changes in lifestyle among subjects with impaired glucose tolerance. N Engl J Med. 2001;344(18):1343–50.
Guariguata L, Whiting DR, Hambleton I, Beagley J, Linnenkamp U, Shaw JE. Global estimates of diabetes prevalence for 2013 and projections for 2035. Diabetes Res Clin Pract. 2014;103(2):137–49.
Leti F, DiStefano J. Long non-coding RNAs as diagnostic and therapeutic targets in type 2 diabetes and related complications. Genes. 2017;8(8):207.
Heydari M, Teimouri M, Heshmati Z, Alavinia SM. Comparison of various classification algorithms in the diagnosis of type 2 diabetes in Iran. International Journal of Diabetes in Developing Countries. 2016;36(2):167–73.
Perkel JM. Visiting “noncodarnia”. In: Future Science. 2013.
Kapranov P, Cheng J, Dike S, Nix DA, Duttagupta R, Willingham AT, Stadler PF, Hertel J, Hackermüller J, Hofacker IL. RNA maps reveal new RNA classes and a possible function for pervasive transcription. Science. 2007;316(5830):1484–8.
Cornelis F, Martin M, Saut O, Buy X, Kind M, Palussiere J, Colin T: Precision of manual two-dimensional segmentations of lung and liver metastases and its impact on tumour response assessment using RECIST 1.1. European radiology experimental 2017, 1(1):16.
Liao M, Liu Q, Li B, Liao W, Xie W, Zhang Y. A group of long non-coding RNAs identified by data mining can predict the prognosis of lung adenocarcinoma. Cancer Sci. 2018;109(12):4033.
Deshpande S, Thakare V. Data mining system and applications: a review. International Journal of Distributed and Parallel systems (IJDPS). 2010;1(1):32–44.
Umar Sidiq D, Aaqib SM, Khan RA. Diagnosis of various thyroid ailments using data mining classification techniques. Int J Sci Res Coput Sci Inf Technol. 2019;5:131–6.
Google Scholar
Zou Q, Qu K, Luo Y, Yin D, Ju Y, Tang H. Predicting diabetes mellitus with machine learning techniques. Front Genet. 2018;9.
Daghistani T, Alshammari R. Diagnosis of diabetes by applying data mining classification techniques. International Journal of Advanced Computer Science and Applications (IJACSA). 2016;7(7):329–32.
Meng X-H, Huang Y-X, Rao D-P, Zhang Q, Liu Q. Comparison of three data mining models for predicting diabetes or prediabetes by risk factors. Kaohsiung J Med Sci. 2013;29(2):93–9.
Wu H, Yang S, Huang Z, He J, Wang X. Type 2 diabetes mellitus prediction model based on data mining. Informatics in Medicine Unlocked. 2018;10:100–7.
Vijayan V, Ravikumar A: Study of data mining algorithms for prediction and diagnosis of diabetes mellitus. International journal of computer applications 2014, 95(17).
Saravananathan K, Velmurugan T. Analyzing diabetic data using classification algorithms in data mining. Indian J Sci Technol. 2016;9(43):196–1.
Nahar N, Ara F. Liver disease prediction by using different decision tree techniques. International Journal of Data Mining & Knowledge Management Process (IJDKP) Vol. 2018;8.
Airola A, Pahikkala T, Waegeman W, De Baets B, Salakoski T. An experimental comparison of cross-validation techniques for estimating the area under the ROC curve. Computational Statistics & Data Analysis. 2011;55(4):1828–44.
Purushotham S, Tripathy B: Evaluation of classifier models using stratified tenfold cross validation techniques. In: International Conference on Computing and Communication Systems: 2011. Springer: 680–690.
Abdar M, Kalhori SRN, Sutikno T, Subroto IMI, Arji G: Comparing Performance of Data Mining Algorithms in Prediction Heart Diseases. International Journal of Electrical & Computer Engineering (2088–8708) 2015, 5(6).
Sambyal RS, Javid T, Bansal A. Performance analysis of data mining classification algorithms to predict diabetes. International Journal of Scientific Research in Computer Science, Engineering and Information Technology. 2018;4(1):56–63.
Pradhan M, Kohale K, Naikade P, Pachore A, Palwe E. Design of classifier for detection of diabetes using neural network and fuzzy k-nearest neighbor algorithm. International Journal of Computational Engineering Research. 2012;2(5):1384–7.
Tzeng F-Y, Ma K-L. Opening the black box-data driven visualization of neural networks: IEEE; 2005.
Morán I, Akerman İ, Van De Bunt M, Xie R, Benazra M, Nammo T, Arnes L, Nakić N, García-Hurtado J, Rodríguez-Seguí S. Human β cell transcriptome analysis uncovers lncRNAs that are tissue-specific, dynamically regulated, and abnormally expressed in type 2 diabetes. Cell Metab. 2012;16(4):435–48.
Voight BF, Scott LJ, Steinthorsdottir V, Morris AP, Dina C, Welch RP, Zeggini E, Huth C, Aulchenko YS, Thorleifsson G. Twelve type 2 diabetes susceptibility loci identified through large-scale association analysis. Nat Genet. 2010;42(7):579.
Imamura M, Maeda S. Genetics of type 2 diabetes: the GWAS era and future perspectives. Endocr J. 2011:1107190592–2.
Soni J, Ansari U, Sharma D, Soni S. Predictive data mining for medical diagnosis: an overview of heart disease prediction. International Journal of Computer Applications. 2011;17(8):43–8.
Asadi F, Paydar S. Presenting an evaluation model of the trauma registry software. Int J Med Inform. 2018;112:99–103.
Dangare CS, Apte SS. Improved study of heart disease prediction system using data mining classification techniques. International Journal of Computer Applications. 2012;47(10):44–8.
Yuan F, Lu L, Zhang Y, Wang S, Cai Y-D. Data mining of the cancer-related lncRNAs GO terms and KEGG pathways by using mRMR method. Math Biosci. 2018;304:1–8.
Sa’di S, Maleki A, Hashemi R, Panbechi Z, Chalabi K. Comparison of data mining algorithms in the diagnosis of type II diabetes. International Journal on Computational Science & Applications (IJCSA). 2015;5(5):1–12.
Download references
Acknowledgments
Not applicable.
Author information
Authors and affiliations.
Department of Laboratory Medicine, School of Allied Medical Sciences, Shahid Beheshti University of Medical Sciences, Tehran, Iran
Faranak Kazerouni & Zahra Mansoori
Department of Health Information Technology and Management, School of Allied Medical Sciences, Shahid Beheshti University of Medical Sciences, Tehran, Iran
Azadeh Bayani & Farkhondeh Asadi
Department of Clinical Biochemistry, School of Medicine, Tehran University of Medical Sciences, Tehran, Iran
Leyla Saeidi
Department of Genetics, Faculty of Medicine, Babol University of Medical Sciences, Babol, Iran
Nasrin Parvizi
You can also search for this author in PubMed Google Scholar
Contributions
AB and FA designed the study. FA and AB collected the data and performed the statistical analysis. AB and NP interpreted the data. FK, ZM, and LS wrote and revised the paper. All authors read and approved the final manuscript.
Corresponding author
Correspondence to Farkhondeh Asadi .
Ethics declarations
Ethics approval and consent to participate.
Ethical approval was obtained from the Shahid Beheshti University of Medical Sciences Ethics Committee (IR.SBMU.RETECH.REC.1395.1036). We informed all participants that their participation was voluntary, and the study did not state any potential risk, and their identities will be private. Informed written consent forms were taken from all participants before participation.
Consent for publication
Competing interests.
The authors declare that they have no competing interests.
Additional information
Publisher’s note.
Springer Nature remains neutral with regard to jurisdictional claims in published maps and institutional affiliations.
Rights and permissions
Open Access This article is licensed under a Creative Commons Attribution 4.0 International License, which permits use, sharing, adaptation, distribution and reproduction in any medium or format, as long as you give appropriate credit to the original author(s) and the source, provide a link to the Creative Commons licence, and indicate if changes were made. The images or other third party material in this article are included in the article's Creative Commons licence, unless indicated otherwise in a credit line to the material. If material is not included in the article's Creative Commons licence and your intended use is not permitted by statutory regulation or exceeds the permitted use, you will need to obtain permission directly from the copyright holder. To view a copy of this licence, visit http://creativecommons.org/licenses/by/4.0/ . The Creative Commons Public Domain Dedication waiver ( http://creativecommons.org/publicdomain/zero/1.0/ ) applies to the data made available in this article, unless otherwise stated in a credit line to the data.
Reprints and permissions
About this article
Cite this article.
Kazerouni, F., Bayani, A., Asadi, F. et al. Type2 diabetes mellitus prediction using data mining algorithms based on the long-noncoding RNAs expression: a comparison of four data mining approaches. BMC Bioinformatics 21 , 372 (2020). https://doi.org/10.1186/s12859-020-03719-8
Download citation
Received : 13 December 2019
Accepted : 21 August 2020
Published : 27 August 2020
DOI : https://doi.org/10.1186/s12859-020-03719-8
Share this article
Anyone you share the following link with will be able to read this content:
Sorry, a shareable link is not currently available for this article.
Provided by the Springer Nature SharedIt content-sharing initiative
- Data mining
- Gene expression
- Machine learning algorithms
- Type 2 diabetes mellitus
BMC Bioinformatics
ISSN: 1471-2105
- General enquiries: [email protected]
Advertisement
Early Prediction of Diabetic Using Data Mining
- Survey Article
- Published: 17 January 2023
- Volume 4 , article number 169 , ( 2023 )
Cite this article
- Fayzeh Abdulkareem Jaber 1 &
- Joy Winston James ORCID: orcid.org/0000-0001-6693-3469 1
183 Accesses
Explore all metrics
The World Health Organization (WHO) reports that in 2018, 422 million people throughout the globe are living with diabetes, making it one of the most widespread chronic life-threatening conditions. Early diagnosis is often favoured for clinically relevant findings due to the comparatively longer asymptomatic period associated with diabetes. It is estimated that around 50% of people with diabetes go undiagnosed because of the length of time it takes for symptoms to appear. The appropriate evaluation of both common and less common sign symptoms, which may be present at various times between the onset of the illness and diagnosis, is essential for early detection of diabetes. Researchers have relied heavily on data mining-based categorization algorithms for illness risk prediction models. To estimate a person’s risk of developing diabetes, it is required to have access to data on people who have recently developed diabetes or who are at high risk of developing diabetes. A dataset of 768 instances was provided to us via Kaggle and was created by the National Institute of Diabetes and Digestive and Kidney Diseases. This set of examples was narrowed down from a bigger database using a variety of criteria. All our female patients are at least 21 years old and are indigenous Pima. We performed statistical analysis on the dataset using the Naïve–Bayes Algorithm, the Logistic Regression Algorithm, and the Random Forest Algorithm. We found that Random Forest provided the best accuracy for this dataset when evaluated using both ten-fold Cross- Validation and the percentage split method. The National Institute of Diabetes and Digestive and Kidney Diseases is the original source of this data. The goal is to diagnose a patient and then forecast whether they have diabetes based on those results.
This is a preview of subscription content, log in via an institution to check access.
Access this article
Price includes VAT (Russian Federation)
Instant access to the full article PDF.
Rent this article via DeepDyve
Institutional subscriptions
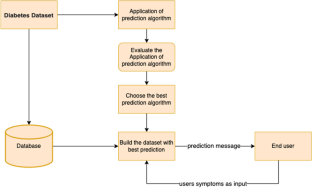
Similar content being viewed by others
Likelihood Prediction of Diabetes at Early Stage Using Data Mining Techniques
Analysis of the Performance of Data Mining Classification Algorithm for Diabetes Prediction
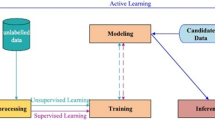
Predictive Supervised Machine Learning Models for Diabetes Mellitus
Bharath C, Saravanan N, Venkatalakshmi S. Assessment of knowledge related to diabetes mellitus among patients attending a dental college in Salem city-A cross sectional study. Braz Dent Sci. 2017;20:93–100.
Article Google Scholar
Dominic V, Gupta D. Khare S. Aggarwal A. Investigation of chronic disease correlation using data mining techniques. In: Proceedings of the 2015 2nd international conference on recent advances in engineering & computational sciences (RAECS), Chandigarh, India, 21–22 December 2015, pp 1–6.
Priyadarshini K, Lakshmi I. Predictive analysis of diabetes using Bayesian network and naive Bayes techniques. In: International conference on advancements in computing technologies - ICACT 2018, vol 4, issue 2. ISSN: 2454-4248.
Jegan C. Classification of diabetes disease using support vector machine. Int J Eng Res Appl. 2013;3:1797–801.
Google Scholar
Soni U, Behara S, Unni Krishnan K, Kumar R. Application of association rule mining in risk analysis for diabetes mellitus. Int J Adv Res Comput Commun Eng. 2016;5(4). ISSN (Online) 2278-1021. ISSN (Print) 2319 5940.
Harris MI, et al. Onset of NIDDM occurs at least 4–7 yr before clinical diagnosis. Diabetes Care. 1992;15(7):815–9.
Akter S, et al. Prevalence of diabetes and prediabetes and their risk factors among Bangladeshi adults: a nationwide survey. Bull World Health Organ. 2014;92:204-213A.
Statistics About Diabetes: American Diabetes Association 22 Mar 2018. https://www.diabetes.org .
Ramachandran A. Know the signs and symptoms of diabetes. Indian J Med Res. 2014;140(5):579.
Khan FA, Zeb K, Al-Rakhami M, Derhab A, Bukhari SAC. Detection and prediction of diabetes using data mining: a comprehensive review. IEEE Access. 2021;9:43711–35. https://doi.org/10.1109/ACCESS.2021.3059343 .
Fiarni C, Sipayung EM, Maemunah S. Analysis and prediction of diabetes complication disease using data mining algorithm. Procedia Comput Sci. 2019;161:449–57. https://doi.org/10.1016/j.procs.2019.11.144 .
Woldemichael FG, Menaria S “Prediction of diabetes using data mining techniques,” 2018 2nd International Conference on Trends in Electronics and Informatics (ICOEI), 2018; 414–418. https://doi.org/10.1109/ICOEI.2018.8553959 .
Yang H, Luo Y, Ren X, Wu M, He X, Peng B, Deng K, Yan D, Tang H, Lin H. Risk prediction of diabetes: big data mining with fusion of multifarious physical examination indicators. Inform Fusion. 2021. https://doi.org/10.1016/j.inffus.2021.02.015 .
Shuja M, Mittal S, Zaman M. Effective prediction of type II diabetes mellitus using data mining classifiers and SMOTE. In: Sharma H, Govindan K, Poonia R, Kumar S, El-Medany W, editors. Advances in computing and intelligent systems algorithms for intelligent systems. Singapore: Springer; 2020. https://doi.org/10.1007/978-981-15-0222-4_17 .
Chapter Google Scholar
Oladele TO, Ogundokun RO, Kayode AA, Adegun AA, Adebiyi MO. Application of Data mining algorithms for feature selection and prediction of diabetic retinopathy. In: , et al. Computational Science and Its Applications – ICCSA 2019. ICCSA 2019. Lecture Notes in Computer Science, vol 11623. Springer, Cham. 2019. https://doi.org/10.1007/978-3-030-24308-1_56 .
Deepika M, Kalaiselvi K. “A Empirical study on Disease Diagnosis using Data Mining Techniques,” 2018 Second International Conference on Inventive Communication and Computational Technologies (ICICCT). 2018; 615-620. https://doi.org/10.1109/ICICCT.2018.8473185 .
Download references
This study is not funded by any of the organization.
Author information
Authors and affiliations.
University of Technology Bahrain, Salmabad, Manama, Bahrain
Fayzeh Abdulkareem Jaber & Joy Winston James
You can also search for this author in PubMed Google Scholar
Corresponding author
Correspondence to Joy Winston James .
Ethics declarations
Conflict of interest.
On behalf of all authors, the corresponding author Dr. Joy Winston states that there is no conflict of interest.
Ethical Approval
This article does not contain any studies with human participants or animals performed by any of the authors.
Additional information
Publisher's note.
Springer Nature remains neutral with regard to jurisdictional claims in published maps and institutional affiliations.
This article is part of the topical collection “Advances in Computational Intelligence, Paradigms and Applications” guest edited by Young Lee and S. Meenakshi Sundaram.
Rights and permissions
Springer Nature or its licensor (e.g. a society or other partner) holds exclusive rights to this article under a publishing agreement with the author(s) or other rightsholder(s); author self-archiving of the accepted manuscript version of this article is solely governed by the terms of such publishing agreement and applicable law.
Reprints and permissions
About this article
Jaber, F.A., James, J.W. Early Prediction of Diabetic Using Data Mining. SN COMPUT. SCI. 4 , 169 (2023). https://doi.org/10.1007/s42979-022-01594-z
Download citation
Received : 01 October 2022
Accepted : 17 December 2022
Published : 17 January 2023
DOI : https://doi.org/10.1007/s42979-022-01594-z
Share this article
Anyone you share the following link with will be able to read this content:
Sorry, a shareable link is not currently available for this article.
Provided by the Springer Nature SharedIt content-sharing initiative
- Diabetes risk
- Data mining
- Evaluation model
- Supervised learning algorithms
- Unsupervised learning algorithms
- Mining tools
- Find a journal
- Publish with us
- Track your research

An official website of the United States government
The .gov means it’s official. Federal government websites often end in .gov or .mil. Before sharing sensitive information, make sure you’re on a federal government site.
The site is secure. The https:// ensures that you are connecting to the official website and that any information you provide is encrypted and transmitted securely.
- Publications
- Account settings
Preview improvements coming to the PMC website in October 2024. Learn More or Try it out now .
- Advanced Search
- Journal List
- J Healthc Eng
- v.2021; 2021

Machine Learning Based Diabetes Classification and Prediction for Healthcare Applications
Umair muneer butt.
1 School of Computer Sciences, Universiti Sains Malaysia, Penang, Malaysia
Sukumar Letchmunan
Mubashir ali.
2 Department of Management,Information and Production Engineering, University of Bergamo, Bergamo, Italy
Fadratul Hafinaz Hassan
Anees baqir.
3 Department of Environmental Sciences,Informatics,and Statistics, Ca'Foscari University of Venice, Venice, Italy
Hafiz Husnain Raza Sherazi
4 School of Computing and Engineering, University of West London, London, UK
Associated Data
The data used to support the findings of this study are included within the article.
The remarkable advancements in biotechnology and public healthcare infrastructures have led to a momentous production of critical and sensitive healthcare data. By applying intelligent data analysis techniques, many interesting patterns are identified for the early and onset detection and prevention of several fatal diseases. Diabetes mellitus is an extremely life-threatening disease because it contributes to other lethal diseases, i.e., heart, kidney, and nerve damage. In this paper, a machine learning based approach has been proposed for the classification, early-stage identification, and prediction of diabetes. Furthermore, it also presents an IoT-based hypothetical diabetes monitoring system for a healthy and affected person to monitor his blood glucose (BG) level. For diabetes classification, three different classifiers have been employed, i.e., random forest (RF), multilayer perceptron (MLP), and logistic regression (LR). For predictive analysis, we have employed long short-term memory (LSTM), moving averages (MA), and linear regression (LR). For experimental evaluation, a benchmark PIMA Indian Diabetes dataset is used. During the analysis, it is observed that MLP outperforms other classifiers with 86.08% of accuracy and LSTM improves the significant prediction with 87.26% accuracy of diabetes. Moreover, a comparative analysis of the proposed approach is also performed with existing state-of-the-art techniques, demonstrating the adaptability of the proposed approach in many public healthcare applications.
1. Introduction
Public health is a fundamental concern for protecting and preventing the community from health hazard diseases [ 1 ]. Governments are spending a considerable amount of their gross domestic product (GDP) for the welfare of the public, and initiatives such as vaccination have prolonged the life expectancy of people [ 2 ]. However, for the last many years, there has been a considerable emergence of chronic and genetic diseases affecting public health. Diabetes mellitus is one of the extremely life-threatening diseases because it contributes to other lethal diseases, i.e., heart, kidney, and nerve damage [ 3 ].
Diabetes is a metabolic disorder that impairs an individual's body to process blood glucose, known as blood sugar. This disease is characterized by hyperglycemia resulting from defects in insulin secretion, insulin action, or both [ 3 ]. An absolute deficiency of insulin secretion causes type 1 diabetes (T1D). Diabetes drastically spreads due to the patient's inability to use the produced insulin. It is called type 2 diabetes (T2D) [ 4 ]. Both types are increasing rapidly, but the ratio of increase in T2D is higher than T1D. 90 to 95% of cases of diabetes are of T2D.
Inadequate supervision of diabetes causes stroke, hypertension, and cardiovascular diseases [ 5 ]. To avoid and reduce the complications due to diabetes, a monitoring method of BG level plays a prominent role [ 6 ]. A combination of biosensors and advanced information and communication technology (ICT) provides an efficient real-time monitoring management system for the health condition of diabetic patients by using SMBG (self-monitoring of blood glucose) portable device. A patient can check the changes in glucose level in his blood by himself [ 7 ]. Users can better understand BG changes by using CGM (continuous glucose monitoring) sensors [ 4 ].
By exploiting the advantages of the advancement in modern sensor technology, IoT, and machine learning techniques, we have proposed an approach for the classification, early-stage identification, and prediction of diabetes in this paper. The primary objective of this study is twofold. First, to classify diabetes into predefined categories, we have employed three widely used classifiers, i.e., random forest, multilayer perceptron, and logistic regression. Second, for the predictive analysis of diabetes, long short-term memory (LSTM), moving averages (MA), and linear regression (LR) are used. To demonstrate the effectiveness of the proposed approach, PIMA Indian Diabetes is used for experimental evaluation. We concluded that, in experimental evaluation, MLP achieved an accuracy of 86.083% in diabetes classification as compared to the other classifiers and LSTM achieved a prediction accuracy of 87.26% for the prediction of diabetes. Moreover, we have also performed a comparative analysis of the proposed approach with existing state-of-the-art approaches. The accuracy results of our proposed approach demonstrate its adaptability in many healthcare applications.
Besides, we have also presented the IoT-based hypothetical diabetes self-monitoring system that uses BLE (Bluetooth Low Energy) devices and data processing in real-time. The latter technique used two applications: Apache Kafka (for streaming messages and data) and MongoDB (to store data). By utilizing BLE-based sensors, one can collect essential sign data about weight and blood glucose. These data will be handled by data processing techniques in a real-time environment. A BLE device will receive all the data produced by sensors and other necessary information about the patient that resides in the user application, installed on the cell phone. The raw data produced by sensors will be processed using the proposed approach to produce results, suggestions, and treatment from the patient's server-side.
The rest of the paper is organized as follows. In Section 2 , the paper presents the motivations for the proposed system by reviewing state-of-the-art techniques and their shortcomings. It covers the literature review about classification, prediction, and IoT-based techniques for healthcare. Section 3 highlights the role of physical activity in diabetes prevention and control. In Section 4 , we proposed the design and architecture of the diabetes classification and prediction systems. Section 5 discusses the results and performance of the proposed approach with state-of-the-art techniques. In Section 6 , an IoT-based hypothetical system is presented for real-time monitoring of diabetes. Finally, the paper is concluded in Section 7 , outlining the future research directions.
2. Literature Review
In this section, we discussed the classification and prediction algorithms for diabetes prediction in healthcare. Particularly, the significance of BLE-based sensors and machine learning algorithms is highlighted for self-monitoring of diabetes mellitus in healthcare. Machine learning plays an essential part in the healthcare industry by providing ease to healthcare professionals to analyze and diagnose medical data [ 8 – 12 ]. Moreover, intelligent healthcare systems are providing real-time clinical care to needy patients [ 13 , 14 ]. The features covered in this study are compared with the state-of-the-art studies ( Table 1 ).
Features' comparison of the proposed study vs. state-of-the-art studies.
2.1. Diabetes Classification for Healthcare
Health condition diagnosis is an essential and critical aspect for healthcare professionals. Classification of a diabetes type is one of the most complex phenomena for healthcare professionals and comprises several tests. However, analyzing multiple factors at the time of diagnosis can sometimes lead to inaccurate results. Therefore, interpretation and classification of diabetes are a very challenging task. Recent technological advances, especially machine learning techniques, are incredibly beneficial for the healthcare industry. Numerous techniques have been presented in the literature for diabetes classification.
Qawqzeh et al. [ 15 ] proposed a logistic regression model based on photoplethysmogram analysis for diabetes classification. They used 459 patients' data for training and 128 data points to test and validate the model. Their proposed system correctly classified 552 persons as nondiabetic and achieved an accuracy of 92%. However, the proposed technique is not compared with state-of-the-art techniques. Pethunachiyar [ 16 ] presented a diabetes mellitus classification system using a machine learning algorithm. Mainly, he used a support vector machine with different kernel functions and diabetes data from the UCI Machine Repository. He found SVM with linear function more efficient than naïve Bayes, decision tree, and neural networks. Nevertheless, the state-of-the-art comparison is missing and parameter selection is not elaborated.
Gupta et al. [ 17 ] exploited naïve Bayes and support vector machine algorithms for diabetes classification. They used the PIMA Indian Diabetes dataset. Besides, they used a feature selection based approach and k-fold cross-validation to improve the accuracy of the model. The experimental results showed the supremacy of the support vector machine over the naïve Bayes model. However, state-of-the-art comparison is missing along with achieved accuracy. Choubey et al. [ 18 ] presented a comparative analysis of classification techniques for diabetes classification. They used PIMA Indian data collected from the UCI Machine Learning Repository and a local diabetes dataset. They used AdaBoost, K-nearest neighbor regression, and radial basis function to classify patients as diabetic or not from both datasets. Besides, they used PCA and LDA for feature engineering, and it is concluded that both are useful with classification algorithms for improving accuracy and removing unwanted features.
Maniruzzaman et al. [ 19 ] used a machine learning paradigm to classify and predict diabetes. They utilized four machine learning algorithms, i.e., naive Bayes, decision tree, AdaBoost, and random forest, for diabetes classification. Also, they used three different partition protocols along with the 20 trials for better results. They used US-based National Health and Nutrition Survey data of diabetic and nondiabetic individuals and achieved promising results with the proposed technique. Ahuja et al. [ 20 ] performed a comparative analysis of various machine learning approaches, i.e., NB, DT, and MLP, on the PIMA dataset for diabetic classification. They found MLP superior as compared to other classifiers. The authors suggested that the performance of MLP can be enhanced by fine-tuning and efficient feature engineering. Recently, Mohapatra et al. [ 21 ] have also used MLP to classify diabetes and achieved an accuracy of 77.5% on the PIMA dataset but failed to perform state-of-the-art comparisons. MLP has been used in the literature for various healthcare disease classifications such as cardiovascular and cancer classification [ 35 , 36 ].
2.2. Predictive Analysis of Diabetes for Healthcare
Accurate classification of diabetes is a fundamental step towards diabetes prevention and control in healthcare. However, early and onset identification of diabetes is much more beneficial in controlling diabetes. The diabetes identification process seems tedious at an early stage because a patient has to visit a physician regularly. The advancement in machine learning approaches has solved this critical and essential problem in healthcare by predicting disease. Several techniques have been proposed in the literature for diabetes prediction.
Singh and Singh [ 22 ] proposed a stacking-based ensemble method for predicting type 2 diabetes mellitus. They used a publicly available PIMA dataset from the UCI Machine Learning Repository. The stacking ensemble used four base learners, i.e., SVM, decision tree, RBF SVM, and poly SVM, and trained them with the bootstrap method through cross-validation. However, variable selection is not explicitly mentioned and state-of-the-art comparison is missing.
Kumari et al. [ 23 ] presented a soft computing-based diabetes prediction system that uses three widely used supervised machine learning algorithms in an ensemble manner. They used PIMA and breast cancer datasets for evaluation purposes. They used random forest, logistic regression, and naïve Bayes and compared their performance with state-of-the-art individual and ensemble approaches, and their system outperforms with 79% accuracy.
Islam et al. [ 24 ] utilized data mining techniques, i.e., random forest, logistic regression, and naïve Bayes algorithm, to predict diabetes at the early or onset stage. They used 10-fold cross-validation and percentage split techniques for training purposes. They collected diabetic and nondiabetic data from 529 individuals directly from a hospital in Bangladesh through questionnaires. The experimental results show that random forest outperforms as compared to other algorithms. However, the state-of-the-art comparison is missing and achieved accuracy is not reported explicitly.
Malik et al. [ 25 ] performed a comparative analysis of data mining and machine learning techniques in early and onset diabetes mellitus prediction in women. They exploited traditional machine learning algorithms for proposing a diabetes prediction framework. The proposed system is evaluated on a diabetes dataset of a hospital in Germany. The empirical results show the superiority of K-nearest neighbor, random forest, and decision tree compared to other traditional algorithms.
Hussain and Naaz [ 26 ] presented a thorough review of machine learning models presented during 2010–2019 for diabetes prediction. They compared traditional supervised machine learning models with neural network-based algorithms in terms of accuracy and efficiency. They used Matthews correlation coefficient for evaluation purposes and observed naïve Bayes and random forest's supremacy compared to other algorithms.
2.3. Real-Time IoT-Based Processing of Healthcare Data
Real-time diabetes prediction is a complicated task. The emerging use of sensors in healthcare paved the path to handle fatal diseases [ 37 ]. Several techniques have been presented in the literature to classify and predict diabetes. Acciaroli et al. [ 4 ] exposed two accurate meters to measure diabetes in blood with less error rate. Furthermore, these commercial versions of glucometers are Accu-Chek with 6.5% error and CareSens with 4.0% error. Buckingham et al. [ 38 ] described the accuracy link of CGM with the calibration sensor. Alfian et al. [ 27 ] uncovered that the FDA had accepted CGM sensors for monitoring glucose in different trends and patterns. Moreover, at one particular time, one glucose reading should not be used to analyze the amount of insulin as not accepted in a glucometer. Rodríguez et al. [ 28 ] proposed a structural design containing a local gateway as a smartphone, cloud system, and sensors for advanced management of diabetes.
Filippoupolitis et al. [ 29 ] planned action to acknowledge a system using Bluetooth Low Energy (BLE) beacons and smartwatches. Mokhtari et al. considered technologies working with BLE for activity labeling and resident localization [ 30 ]. Gentili et al. [ 31 ] have used BLE with another application called Blue Voice, which can reveal the probability of multimedia communication of sensor devices and speech streaming service. Suárez et al. [ 32 ] projected a monitoring system based on the BLE device for air quality exposure with the environmental application. It aims at defining potential policy responses and studies the variables that are interrelated between societal level factors and diabetes prevalence [ 33 , 34 ].
Wang et al. [ 39 ] have given a general idea of the up-to-date BLE technology for healthcare systems based on a wearable sensor. They suggested that low-powered communication sensor technologies such as a BLE device can make it feasible for wearable systems of healthcare because it can be used without location constraints and is light in weight. Moreover, BLE is the first wireless technology in communication for healthcare devices in the form of a wearable device that meets expected operating requirements with low power, communication with cellular directly, secure data transmission, interoperability, electronic compatibility, and Internet communications. Rachim and Chung [ 40 ] have suggested one transmission system that used deficient power to observe the heart's activity through electrocardiograph signals using a BLE device for data transmission collecting by armband sensors and smartphones.
Mora et al. projected a dispersed structure using the IoT model to check human biomedically generated signals in reports using a BLE sensor device [ 41 ]. Cappon et al. [ 42 ] explored the study of CGM wearable sensors' prototypes and features of the commercial version currently used. Årsand et al. [ 43 ] offered the easiest method for monitoring blood glucose, physical activity, insulin injections, and nutritional information using smartphones and smartwatches. Morón et al. [ 44 ] observed the performance of the smartphone used in the medical field. Lee and Yoo [ 45 ] anticipated a structure using PDA (personal digital assistant) to manage diabetic patient's conditions better. It can also be used to send information about blood pressure, BG level, food consumption, and exercise plan of a patient with diabetes and give the direction of treatment by monitoring physical activity, food consumption, and insulin prescribed amount.
Rodríguez et al. [ 28 ] suggested an application for the smartphone, which can be used to receive the data from the sensor using a glucometer automatically. Rodríguez-Rodríguez et al. [ 46 ] suggested that checking the patient's glucose level and heart rate using sensors will produce colossal data, and analysis on big data can be used to solve this problem.
3. Role of Physical Activity in Prevention and Control of Diabetes Mellitus
Generally, physical activity is the first prevention and control strategy suggested by healthcare professionals to diabetic or prediabetic patients [ 47 ]. Among diet and medicine, exercise is a fundamental component in diabetes, cardiovascular disease, obesity, and lifestyle rescue programs. Nonetheless, dealing with all the fatal diseases has a significant economic burden. However, diabetes mellitus emerged as a devastating problem for the health sector and economy of a country of this century.
Recently, the international diabetes prevention and control federation predicts that diabetes can affect more than 366 million people worldwide [ 49 ]. The disease control and prevention center in the US alarmed the government that diabetes can affect more than 29 million people [ 50 ]. While these alarming numbers are continuously increasing, they will burden the economy around the globe. Therefore, researchers and healthcare professionals worldwide are researching and proposing guidelines to prevent and control this life-threatening disease. Sato [ 51 ] presented a thorough survey on the importance of exercise prescription for diabetes patients in Japan. He suggested that prolonged sitting should be avoided and physical activity should be performed every 30 minutes. Kirwan et al. [ 47 ] emphasized regular exercise to control and prevent type 2 diabetes. Particularly, they studied the metabolic effect on tissues of diabetic patients and found very significant improvements in individuals performing regular exercise. Moser et al. [ 48 ] have also highlighted the significance of regular exercise in improving the functionality of various organs of the body, as shown in Figure 1 .

Impact of regular exercise on metabolism of diabetic patients [ 48 ].
Yang et al. [ 52 ] focused on exercise therapy which plays a significant role in treating diabetes and its associated side effects. Specifically, they discovered cytokines which gives a novel insight into diabetes control, but the sequence is still under study. Kim and Jeon [ 53 ] presented a systematic overview of the effect of different exercises on the metabolism improvement of diabetic young individuals. They pointed out that several studies reported the significance of exercise on insulin, BP, and BG level improvement. However, none of these studies mentions the beta-cell improvement. Therefore, many challenges persist in diabetes prevention and control, which need serious attention from researchers worldwide.
4. Proposed Diabetic Classification and Prediction System for Healthcare
The proposed diabetes classification and prediction system has exploited different machine learning algorithms. First, to classify diabetes, we utilized logistic regression, random forest, and MLP. Notably, we fine-tuned MLP for classification due to its promising performance in healthcare, specifically in diabetes prediction [ 20 , 21 , 35 , 36 ]. The proposed MLP architecture and algorithm are shown in Figure 2 and Algorithm 1 , respectively.

Proposed MLP architecture with eight variables as input for diabetes classification.

Diabetes classification algorithm using MLP for healthcare.
Second, we implement three widely used machine learning algorithms for diabetes prediction, i.e., moving averages, linear regression, and LSTM. Mainly, we optimized LSTM for crime prediction due to its outstanding performance in real-world applications, particularly in healthcare [ 53 ]. The implementation details of the proposed algorithms are as follows.
4.1. Diabetes Classification Techniques
For diabetic classification, we fine-tuned three widely used state-of-the-art techniques. Mainly, a comparative analysis is performed among the proposed techniques for classifying an individual in either of the diabetes categories. The details of the proposed diabetes techniques are as follows.
4.1.1. Logistic Regression
It is appropriate to use logistic regression when the dependent variable is binary [ 54 ], as we have to classify an individual in either type 1 or type 2 diabetes. Besides, it is used for predictive analysis and explains the relationship between a dependent variable and one or many independent variables, as shown in equation ( 1 ). Therefore, we used the sigmoid cost function as a hypothesis function ( h θ ( x )). The aim is to minimize cost function J ( θ ). It always results in classifying an example either in class 1 or class 2.
4.1.2. Random Forest (RF)
As its name implies, it is a collection of models that operate as an ensemble. The critical idea behind RF is the wisdom of the crowd, each model predicts a result, and in the end, the majority wins. It has been used in the literature for diabetic prediction and was found to be effective [ 55 ]. Given a set of training examples X = x 1 , x 2 ,…, x m and their respective targets Y = y 1 , y 2 ,…, y m , RF classifier iterates B times by choosing samples with replacement by fitting a tree to the training examples. The training algorithm consists of the following steps depicted in equation ( 2 ).
- For b = 1... B , sample with replacement n training examples from X and Y .
- Train a classification tree f b on X b and Y b .
4.1.3. Multilayer Perceptron
For diabetes classification, we have fine-tuned multilayer perceptron in our experimental setup. It is a network where multiple layers are joined together to make a classification method, as shown in Figure 2 . The building block of this model is perceptron, which is a linear combination of input and weights. We used a sigmoid unit as an activation function shown in Algorithm 1 . The proposed algorithm consists of three main steps. First, weights are initialized and output is computed at the output layer ( δ k ) using the sigmoid activation function. Second, the error is computed at hidden layers ( δ h ) for all hidden units. Finally, in a backward manner, all network weights ( w i , j ) are updated to reduce the network error. The detailed procedure is outlined in Algorithm 1 for diabetes classification.
Figure 2 shows the multilayer perceptron classification model architecture where eight neurons are used in the input layer because we have eight different variables. The middle layer is the hidden layer where weights and input will be computed using a sigmoid unit. In the end, results will be computed at the output layer. Backpropagation is used for updating weights so that errors can be minimized for predicting class labels. For simplicity, only one hidden layer is shown in the architecture, which in reality is much denser.
Input data from the input layer are computed on the hidden layers with the input values and weights initialized. Every unit in the middle layer called the hidden layer takes the net input, applies activation function “sigmoid” on it, and transforms the massive data into a smaller range between 0 and 1. The calculation is functional for every middle layer. The same procedure is applied on the output layer, which leads to the results towards the prediction for diabetes.
4.2. Diabetes Prediction
It is more beneficial to identify the early symptoms of diabetes than to cure it after being diagnosed. Therefore, in this study, a diabetes prediction system is proposed where three state-of-the-art machine learning algorithms are exploited, and a comparative analysis is performed. The details of the proposed approaches are as follows.
4.2.1. Moving Averages
To predict diabetes, we used moving averages with the experimental setup due to its effectiveness in diabetes prediction for children [ 56 ]. It is based on a calculation that analyzes data points by creating a series of averages of the subset of the data randomly. The moving average algorithm is based on the “forward shifting” mechanism. It excludes the first number from the series and includes the next value in the dataset, as shown in equation ( 3 ). The input values are calculated by averaging ( P SM ) the train data at certain time stamps P M + P M + … P M −( n −1) . The algorithm used past observations as input and predicted future events.

4.2.2. Linear Regression
Second, a linear regression model is applied to the PIMA Indian dataset with the same experimental setup. We used this approach to model a relationship between a dependent variable, that is, outcome in our case, and one or more independent variables. The autonomous variable response affects a lot on the target/dependent variable, as shown in equation ( 4 ). We use a simplified hypothesis and cost function for multivariate linear regression, as there are eight different variables in our dataset [ 57 ]. We choose a very simplified hypothesis function ( h θ ( x )). The aim is to minimize cost function J ( θ ) by choosing the suitable weight ( θ T x ) parameters and minimizing sum of squared error (SSE).
4.2.3. Long Short-Term Memory
For diabetic forecasting, we have calibrated the long short-term memory algorithm with our experimental setup. The proposed approach outperformed as compared to other state-of-the-art techniques implemented, as shown in Table 2 . LSTM is based on recurrent neural network (RNN) architecture, and it has feedback connections that make it suitable for diabetes forecasting [ 58 ]. LSTM mainly consists of a cell, keep gate, write gate, and an output gate, as shown in Figure 3 . The key behind using LSTM for this problem is that the cell remembers the patterns over a long period, and three portals help regulate the information flow in and out of the system. The details are presented in Algorithm 2 .

BG prediction using long short-term memory (LSTM) algorithm.

Diabetes prediction algorithm by exploiting LSTM for healthcare.
Performance comparison of classifiers in diabetes classification.
Input to the algorithm is eight attributes enlisted in Table 3 , measured from healthy and diabetic patients. The proposed LSTM-based diabetes prediction algorithm is trained with 80% of the data, and the remaining 20% is used for testing. We fine-tuned the prediction model by using a different number of LSTM units in the cell state. This fine-tuning helps to identify more prominent features in the dataset. These features will be kept in the cell state of the keep gate of the LSTM and will be given more weightage because they provide more insights to predict BG level. After that, we updated the network's weights by pointwise addition of the cell state and passed only those essential attributes for BG prediction. At this stage, we captured the dependencies between diabetes parameters and the output variable. Finally, the output gate updates the cell state and outputs/forwards only those variables that can be mapped efficiently on the outcome variable.
Description of variables in the dataset.
The diabetes prediction algorithm consists of three fundamental steps. First, weights are initialized and a sigmoid unit is used in the forget/keep gate to decide which information should be retained from previous and current inputs ( C t −1 , h t −1 , and x t ). The input/write gate takes the necessary information from the keep gate and uses a sigmoid unit which outputs a value between 0 and 1. Besides, a Tan h unit is used to update the cell state C t and combine both outputs to update the old cell state to the new cell state.
Finally, inputs are processed at the output gate and again a sigmoid unit is applied to decide which cell state should be output. Also, Tan h is applied to the incoming cell state to push the output between 1 and −1. If the output of the gate is 1, then the memory cell is still relevant to the required production and should be kept for future results. If the output of the gate is 0, the memory cell is not appropriate, so it should be erased. For the write gate, the suitable pattern and type of information will be determined written into the memory cell. The proposed LSTM model predicts the BG level ( h t ) as output based on the patient's existing BG level ( X t ).
5. Experimental Studies
The proposed diabetes classification and prediction algorithm is evaluated on a publicly available PIMA Indian Diabetes dataset ( https://www.niddk.nih.gov/health-information/diabetes ). Besides, a comparative analysis is performed with state-of-the-art algorithms. The experimental results show the supremacy of the proposed algorithm as compared to state-of-the-art algorithms. The details of the dataset, performance measures, and comparative analysis performed are described in the following sections.
5.1. Dataset
This study used the PIMA Indian Diabetes (PID) dataset taken from the National Institute of Diabetes and Kidney Diseases center [ 59 ]. The primary objective of using this dataset is to build an intelligent model that can predict whether a person has diabetes or not, using some measurements included in the dataset. There are eight medical predictor variables and one target variable in the dataset. Diabetes classification and prediction are a binary classification problem. The details of the variables are shown in Table 3 .
The dataset consists of 768 records of different healthy and diabetic female patients of age greater than twenty-one, as shown in Figure 4 . The feature value distribution is shown in Figure 5 . The target variable outcome contains only two values, 0 and 1. The primary objective of using this dataset was to predict diabetes diagnostically. Whether a user has a chance of diabetes in the coming four years in women belongs to PIMA Indian. The dataset has a total of eight variables: glucose tolerance, no. of pregnancies, body mass index, blood pressure, age, insulin, and Diabetes Pedigree Function. All eight attributes shown in Table 3 are used for the training dataset in the classification model in this work.

PIMA data distribution.

Dataset features' distribution visualization.
5.2. Experimental Result and Discussion
This paper compares the proposed diabetes classification and prediction system with state-of-the-art techniques using the same experimental setup on the PIMA Indian dataset. The following sections highlighted the performance measure used and results attained for classification and prediction, and a comparative analysis with baseline studies is presented.
5.2.1. Performance Metrics
Three widely used state-of-the-art performance measures (Recall, Precision, and Accuracy) are used to evaluate the performance of proposed techniques, as shown in Table 4 . TP shows a person does not have diabetes and identified as a nondiabetic patient, and TN shows a diabetic patient correctly identified as a diabetic patient. FN shows the patient has diabetes but is predicted as a healthy person. Moreover, FP shows the patient is a healthy person but predicted as a diabetic patient. The algorithm utilized 10-fold cross-validation for training and testing the classification and prediction model.
Performance metrics for diabetes classification.
For diabetes prediction, the two most commonly used performance measures are the means correlation coefficient ( r /Pearson R ) and root mean square error (RMSE), as shown in Table 5 . R is mainly used to measure the linear dependence strength among the two variables. One variable is for actual value, and another variable is for predicted values. RMSE generates a hint of the overall correctness of the estimate. There can be three values for correlation: 0 for no relation, 1 for positive correlation, and −1 for the negative correlation. RMSE shows the difference between actual values and predicted values.
Performance measure for diabetes prediction.
5.2.2. Attained Results of Diabetic Classification Technique
For diabetic classification, three state-of-the-art classifiers are evaluated on the PIMA dataset. The results illustrate that the fine-tuned MLP algorithm obtained the highest accuracy of 86.083% as compared to state-of-the-art systems, as shown in Table 2 .
It is evident from the results that our proposed calibrated MLP model could be used for the effective classification of diabetes. The proposed classification approach can also be beneficial in the future with our proposed hypothetical system. Data of weight scales, blood pressure monitor, and blood glucometer will be collected through sensor devices such as BLE and input of user's demographic data (for example, date of birth, height, and age). The proposed MLP algorithm outperforms with 86.6% Precision, 85.1% Recall, and 86.083% Accuracy, as shown in Figure 6 . These results are outstanding for decision-making with the proposed hypothetical system to determine patient diabetes, T1D or T2D.

Performance comparison of classifiers.
We also have explored the dataset used in Andy Choens' study [ 27 ]. This dataset consists of records of only one patient. The information was recorded every five minutes. The collection of data was made by using a sensor device (a CGM device). This device allows the patient to store information about BG every five minutes. So, the recorded data by using this device are in massive amounts. Dataset was limited, and most data were noisy that can affect the accuracy of the proposed system, so we neglected it.
5.2.3. Achieved Results of Diabetic Prediction Techniques
For diabetic prediction, we implemented three state-of-the-art algorithms, i.e., linear regression, moving averages, and LSTM. Notably, we fine-tuned LSTM and compared its performance with other algorithms. It is evident from Figure 7 and Table 6 that the LSTM outperformed as compared to other algorithms implemented in this study.

Performance comparison of forecasting model.
Forecasting model comparison for BG.
Table 2 shows the performance values of prediction models with RMSE and r evaluation measures. The proposed fine-tuned LSTM produced the highest accuracy, 87.26%, compared to linear regression and moving average. We can see in Table 6 that the correlation coefficient value is 0.999 using LSTM, −0.071 for linear regression, and 0.710 for moving average, as shown in Figure 7 .
5.2.4. Comparison of the Proposed Method with Baseline Studies
Different baseline studies have been implemented and compared with the proposed system to verify the performance of the proposed diabetes classification and prediction system. Mainly, we focus on those studies that used the PIMA dataset.
First, we compare the state-of-the-art diabetes classification techniques with the proposed technique. All the baseline techniques [ 17 – 19 ] used the PIMA dataset and the same evaluation measures used in this study. In particular, the authors compared naïve Bayes [ 17 ], PCA_CVR (classification via regression) [ 18 ], and SVM [ 19 ] with different machine learning techniques for diabetes classification. However, the proposed fine-tuned MLP-based diabetes classification technique outperformed as compared to baseline studies, as shown in Figure 8 .

Proposed diabetes classification method vs. state-of-the-art techniques.
Several attempts have also been made in the literature for diabetic prediction due to its importance in real life. For this comparison, we have chosen the most recent and state-of-the-art techniques. We compare the proposed system performance with the recent state-of-the-art systems [ 60 – 65 ], as shown in Figure 9 and Table 7 . The proposed method outperformed as compared to state-of-the-art systems with an accuracy of 87.26%, all the compared systems evaluated on the PID with the same experimental setup.

Proposed diabetes prediction method vs. state-of-the-art systems.
Proposed prediction method vs. state-of-the-art systems.
6. Proposed Hypothetical IoT-Based Diabetic Monitoring System for Healthcare
This study has also proposed the architecture of a hypothetical diabetic monitoring system for diabetic patients. The proposed hypothetical system will enable a patient to control, monitor, and manage their chronic conditions in a better way at their homes. The monitoring system will store the health activities and create interaction between patients, smartphones, sensor medical devices, web servers, and medical teams by providing a platform having wireless communication devices, as shown in Figure 10 . The central theme of the proposed healthcare monitoring system is the collection of data from sensors using wireless devices and transmitting to a remote server for diagnosis and treatment of diabetes. Knowledge-based data are stored. Rule-based procedures will be applied for the suggestions and treatment of diabetes, informing the patient about his current health condition, prediction, and recommendation of future changes in BG.

The proposed hypothetical architecture of the healthcare monitoring system.
First, essential data about patient health will be collected from sensors such as BLE wireless devices. Data comprised weight, blood pressure, blood glucose, and heartbeat, along with some demographic information such as age, sex, name, and CNIC (Social Security Number). Some information is required in the application installed on the user's mobile and sensor data. All completed data in the application will be transferred to the real-time data processing system. On the other side, aggregate data will be stored in MongoDB for future processing. Analysis and prepossessing techniques are performed to extract rules from the knowledge base for the treatment and suggestions about the user. Results and treatment procedures will be sent to the monitoring system, and finally, the user can get the output by interacting with their android mobile phone. In the end, the patient will know about the health condition and risk prediction of diabetes based on the data transferred by their application and stored data from history about the user.
6.1. Tools and Technology for Implementation of Hypothetical System for Healthcare
The proposed structural design for hypothetical real-time processing and monitoring of diabetes is shown in Figure 11 . The data from the user's mobile will be transmitted in the JavaScript Object Notation (JSON) format to the Application Program Interface (API) in any language. The data produced at this stage will be in the form of messages, which are then transferred to the Kafka application [ 27 ]. Kafka will store all the data and messages and deliver the required data and processed output to the endpoints that could be a web server, monitoring system, or a database for permanent storage. In Kafka, application data are stored in different brokers, which can cause latency issues. Therefore, within the system architecture, it is vital to consider processing the readings from the sensors closer to the place where data are acquired, e.g., on the smartphone. The latency problem could be solved by placing sensors close to the place, such as a smartphone where data are sent and received.

Implementation level details of the proposed hypothetical system.
This inclusion will make the overall network architecture compliant to the emerging Edge and Fog computing paradigms, whose importance in critical infrastructures such as hospitals is gaining momentum. It is essential to consider the Edge and Fog computation paradigm while sending and receiving data from smartphones to increase the performance of the hypothetical system. Edge computing utilizes sensors and mobile devices to process, compute, and store data locally rather than cloud computing. Besides, Fog computing places resources near data sources such as gateways to improve latency problems [ 9 ].
Apache Kafka will be used in real time as a delivery agent for messages in a platform that allows fault-tolerant, tall throughput, and low-latency publication. The vital signs' data collected by the patients are placed using the JSON format and then transmitted using wireless devices with the help of an android application having HTTP along with REST API for the confined remote server for the design [ 28 ]. Moreover, Node.js for web design will be used as a REST API to collect sensor data. Kafka application will receive it in the form of streams of records.
The sensor data that comes from the Kafka application is continuously generated and stored on the server. In the proposed system, the MongoDB NoSQL database will be used for data storage due to its efficiency in handling and processing real-world data [ 29 ]. The stored diabetes patient data can be input into our proposed diabetes classification and prediction techniques to get useful insights.
7. Conclusion
In this paper, we have discussed an approach to assist the healthcare domain. The primary objective of this study is twofold. First, we proposed an MLP-based algorithm for diabetes classification and deep learning based LSTM for diabetes prediction. Second, we proposed an IOT-based hypothetical real-time diabetic monitoring system. The proposed theoretical diabetic monitoring system will use a smartphone, BLE-based sensor device, and machine learning based methods in the real-time data processing environment to predict BG levels and diabetes. The primary objective of the proposed system is to help the users monitor their vital signs using BLE-based sensor devices with the help of their smartphones.
Moreover, the proposed model will help the users to find out the risk of diabetes at a very early stage and help them gaining future predictions of their BG increase levels. For diabetic classification and prediction, MLP and LSTM are fine-tuned. The proposed approaches are evaluated on the PIMA Indian Diabetes dataset. Both approaches are compared with state-of-the-art approaches and outperformed with an accuracy of 86.083% and 87.26%, respectively.
As future work, we plan to implement the android application for the proposed hypothetical diabetic monitoring system with the proposed classification and prediction approaches. Genetic algorithms can also be explored with the proposed prediction mechanism for better monitoring [ 24 , 64 , 66 – 71 ].
Acknowledgments
This work was funded by the School of Computer Sciences, Universiti Sains Malaysia, Penang, Malaysia.
Data Availability
Conflicts of interest.
The authors declare that there are no conflicts of interest regarding the publication of this article.
- Open access
- Published: 20 December 2021
Machine learning and deep learning predictive models for type 2 diabetes: a systematic review
- Luis Fregoso-Aparicio ORCID: orcid.org/0000-0003-4986-5745 1 ,
- Julieta Noguez ORCID: orcid.org/0000-0002-6000-3452 2 ,
- Luis Montesinos ORCID: orcid.org/0000-0003-3976-4190 2 &
- José A. García-García ORCID: orcid.org/0000-0001-6876-4558 3
Diabetology & Metabolic Syndrome volume 13 , Article number: 148 ( 2021 ) Cite this article
19k Accesses
50 Citations
11 Altmetric
Metrics details
Diabetes Mellitus is a severe, chronic disease that occurs when blood glucose levels rise above certain limits. Over the last years, machine and deep learning techniques have been used to predict diabetes and its complications. However, researchers and developers still face two main challenges when building type 2 diabetes predictive models. First, there is considerable heterogeneity in previous studies regarding techniques used, making it challenging to identify the optimal one. Second, there is a lack of transparency about the features used in the models, which reduces their interpretability. This systematic review aimed at providing answers to the above challenges. The review followed the PRISMA methodology primarily, enriched with the one proposed by Keele and Durham Universities. Ninety studies were included, and the type of model, complementary techniques, dataset, and performance parameters reported were extracted. Eighteen different types of models were compared, with tree-based algorithms showing top performances. Deep Neural Networks proved suboptimal, despite their ability to deal with big and dirty data. Balancing data and feature selection techniques proved helpful to increase the model’s efficiency. Models trained on tidy datasets achieved almost perfect models.
Introduction
Diabetes mellitus is a group of metabolic diseases characterized by hyperglycemia resulting from defects in insulin secretion, insulin action, or both [ 1 ]. In particular, type 2 diabetes is associated with insulin resistance (insulin action defect), i.e., where cells respond poorly to insulin, affecting their glucose intake [ 2 ]. The diagnostic criteria established by the American Diabetes Association are: (1) a level of glycated hemoglobin (HbA1c) greater or equal to 6.5%; (2) basal fasting blood glucose level greater than 126 mg/dL, and; (3) blood glucose level greater or equal to 200 mg/dL 2 h after an oral glucose tolerance test with 75 g of glucose [ 1 ].
Diabetes mellitus is a global public health issue. In 2019, the International Diabetes Federation estimated the number of people living with diabetes worldwide at 463 million and the expected growth at 51% by the year 2045. Moreover, it is estimated that there is one undiagnosed person for each diagnosed person with a diabetes diagnosis [ 2 ].
The early diagnosis and treatment of type 2 diabetes are among the most relevant actions to prevent further development and complications like diabetic retinopathy [ 3 ]. According to the ADDITION-Europe Simulation Model Study, an early diagnosis reduces the absolute and relative risk of suffering cardiovascular events and mortality [ 4 ]. A sensitivity analysis on USA data proved a 25% relative reduction in diabetes-related complication rates for a 2-year earlier diagnosis.
Consequently, many researchers have endeavored to develop predictive models of type 2 diabetes. The first models were based on classic statistical learning techniques, e.g., linear regression. Recently, a wide variety of machine learning techniques has been added to the toolbox. Those techniques allow predicting new cases based on patterns identified in training data from previous cases. For example, Kälsch et al. [ 5 ] identified associations between liver injury markers and diabetes and used random forests to predict diabetes based on serum variables. Moreover, different techniques are sometimes combined, creating ensemble models to surpass the single model’s predictive performance.
The number of studies developed in the field creates two main challenges for researchers and developers aiming to build type 2 diabetes predictive models. First, there is considerable heterogeneity in previous studies regarding machine learning techniques used, making it challenging to identify the optimal one. Second, there is a lack of transparency about the features used to train the models, which reduces their interpretability, a feature utterly relevant to the doctor.
This review aims to inform the selection of machine learning techniques and features to create novel type 2 diabetes predictive models. The paper is organized as follows. “ Background ” section provides a brief background on the techniques used to create predictive models. “ Methods ” section presents the methods used to design and conduct the review. “ Results ” section summarizes the results, followed by their discussion in “ Discussion ” section, where a summary of findings, the opportunity areas, and the limitations of this review are presented. Finally, “ Conclusions ” section presents the conclusions and future work.
Machine learning and deep learning
Over the last years, humanity has achieved technological breakthroughs in computer science, material science, biotechnology, genomics, and proteomics [ 6 ]. These disruptive technologies are shifting the paradigm of medical practice. In particular, artificial intelligence and big data are reshaping disease and patient management, shifting to personalized diagnosis and treatment. This shift enables public health to become predictive and preventive [ 6 ].
Machine learning is a subset of artificial intelligence that aims to create computer systems that discover patterns in training data to perform classification and prediction tasks on new data [ 7 ]. Machine learning puts together tools from statistics, data mining, and optimization to generate models.
Representational learning, a subarea of machine learning, focuses on automatically finding an accurate representation of the knowledge extracted from the data [ 7 ]. When this representation comprises many layers (i.e., a multi-level representation), we are dealing with deep learning.
In deep learning models, every layer represents a level of learned knowledge. The nearest to the input layer represents low-level details of the data, while the closest to the output layer represents a higher level of discrimination with more abstract concepts.
The studies included in this review used 18 different types of models:
Deep Neural Network (DNN): DNNs are loosely inspired by the biological nervous system. Artificial neurons are simple functions depicted as nodes compartmentalized in layers, and synapses are the links between them [ 8 ]. DNN is a data-driven, self-adaptive learning technique that produces non-linear models capable of real-world modeling problems.
Support Vector Machines (SVM): SVM is a non-parametric algorithm capable of solving regression and classification problems using linear and non-linear functions. These functions assign vectors of input features to an n-dimensional space called a feature space [ 9 ].
k-Nearest Neighbors (KNN): KNN is a supervised, non-parametric algorithm based on the “things that look alike” idea. KNN can be applied to regression and classification tasks. The algorithm computes the closeness or similarity of new observations in the feature space to k training observations to produce their corresponding output value or class [ 9 ].
Decision Tree (DT): DTs use a tree structure built by selecting thresholds for the input features [ 8 ]. This classifier aims to create a set of decision rules to predict the target class or value.
Random Forest (RF): RFs merge several decision trees, such as bagging, to get the final result by a voting strategy [ 9 ].
Gradient Boosting Tree (GBT) and Gradient Boost Machine (GBM): GBTs and GBMs join sequential tree models in an additive way to predict the results [ 9 ].
J48 Decision Tree (J48): J48 develops a mapping tree to include attribute nodes linked by two or more sub-trees, leaves, or other decision nodes [ 10 ].
Logistic and Stepwise Regression (LR): LR is a linear regression technique suitable for tasks where the dependent variable is binary [ 8 ]. The logistic model is used to estimate the probability of the response based on one or more predictors.
Linear and Quadratic Discriminant Analysis (LDA): LDA segments an n-dimensional space into two or more dimensional spaces separated by a hyper-plane [ 8 ]. The aim of it is to find the principal function for every class. This function is displayed on the vectors that maximize the between-group variance and minimizes the within-group variance.
Cox Hazard Regression (CHR): CHR or proportional hazards regression analyzes the effect of the features to occur a specific event [ 11 ]. The method is partially non-parametric since it only assumes that the effects of the predictor variables on the event are constant over time and additive on a scale.
Least-Square Regression: (LSR) method is used to estimate the parameter of a linear regression model [ 12 ]. LSR estimators minimize the sum of the squared errors (a difference between observed values and predicted values).
Multiple Instance Learning boosting (MIL): The boosting algorithm sequentially trains several weak classifiers and additively combines them by weighting each of them to make a strong classifier [ 13 ]. In MIL, the classifier is logistic regression.
Bayesian Network (BN): BNs are graphs made up of nodes and directed line segments that prohibit cycles [ 14 ]. Each node represents a random variable and its probability distribution in each state. Each directed line segment represents the joint probability between nodes calculated using Bayes’ theorem.
Latent Growth Mixture (LGM): LGM groups patients into an optimal number of growth trajectory clusters. Maximum likelihood is the approach to estimating missing data [ 15 ].
Penalized Likelihood Methods: Penalizing is an approach to avoid problems in the stability of the estimated parameters when the probability is relatively flat, which makes it difficult to determine the maximum likelihood estimate using simple methods. Penalizing is also known as shrinkage [ 16 ]. Least absolute shrinkage and selection operator (LASSO), smoothed clipped absolute deviation (SCAD), and minimax concave penalized likelihood (MCP) are methods using this approach.
Alternating Cluster and Classification (ACC): ACC assumes that the data have multiple hidden clusters in the positive class, while the negative class is drawn from a single distribution. For different clusters of the positive class, the discriminatory dimensions must be different and sparse relative to the negative class [ 17 ]. Clusters are like “local opponents” to the complete negative set, and therefore the “local limit” (classifier) has a smaller dimensional subspace than the feature vector.
Some studies used a combination of multiple machine learning techniques and are subsequently labeled as machine learning-based method (MLB).
Systematic literature review methodologies
This review follows two methodologies for conducting systematic literature reviews: the Preferred Reporting Items for Systematic Reviews and Meta-Analyses (PRISMA) statement [ 18 ] and the Guidelines for performing Systematic Literature Reviews in Software Engineering [ 19 ]. Although these methodologies hold many similarities, there is a substantial difference between them. While the former was tailored for medical literature, the latter was adapted for reviews in computer science. Hence, since this review focuses on computer methods applied to medicine, both strategies were combined and implemented. The PRISMA statement is the standard for conducting reviews in the medical sciences and was the principal strategy for this review. It contains 27 items for evaluating included studies, out of which 23 are used in this review. The second methodology is an adaptation by Keele and Durham Universities to conduct systematic literature reviews in software engineering. The authors provide a list of guidelines to conduct the review. Two elements were adopted from this methodology. First, the protocol’s organization in three stages (planning, conducting, and reporting). Secondly, the quality assessment strategy to select studies based on the information retrieved by the search.
Related works
Previous reviews have explored machine learning techniques in diabetes, yet with a substantially different focus. Sambyal et al. conducted a review on microvascular complications in diabetes (retinopathy, neuropathy, nephropathy) [ 20 ]. This review included 31 studies classified into three groups according to the methods used: statistical techniques, machine learning, and deep learning. The authors concluded that machine learning and deep learning models are more suited for big data scenarios. Also, they observed that the combination of models (ensemble models) produced improved performance.
Islam et al. conducted a review with meta-analysis on deep learning models to detect diabetic retinopathy (DR) in retinal fundus images [ 21 ]. This review included 23 studies, out of which 20 were also included for meta-analysis. For each study, the authors identified the model, the dataset, and the performance metrics and concluded that automated tools could perform DR screening.
Chaki et al. reviewed machine learning models in diabetes detection [ 22 ]. The review included 107 studies and classified them according to the model or classifier, the dataset, the features selection with four possible kinds of features, and their performance. The authors found that text, shape, and texture features produced better outcomes. Also, they found that DNNs and SVMs delivered better classification outcomes, followed by RFs.
Finally, Silva et al. [ 23 ] reviewed 27 studies, including 40 predictive models for diabetes. They extracted the technique used, the temporality of prediction, the risk of bias, and validation metrics. The objective was to prove whether machine learning exhibited discrimination ability to predict and diagnose type 2 diabetes. Although this ability was confirmed, the authors did not report which machine learning model produced the best results.
This review aims to find areas of opportunity and recommendations in the prediction of diabetes based on machine learning models. It also explores the optimal performance metrics, the datasets used to build the models, and the complementary techniques used to improve the model’s performance.
Objective of the review
This systematic review aims to identify and report the areas of opportunity for improving the prediction of diabetes type 2 using machine learning techniques.
Research questions
Research Question 1 (RQ1): What kind of features make up the database to create the model?
Research Question 2 (RQ2): What machine learning technique is optimal to create a predictive model for type 2 diabetes?
Research Question 3 (RQ3): What are the optimal validation metrics to compare the models’ performance?
Information sources
Two search engines were selected to search:
PubMed, given the relationship between a medical problem such as diabetes and a possible computer science solution.
Web of Science, given its extraordinary ability to select articles with high affinity with the search string.
These search engines were also considered because they search in many specialized databases (IEEE Xplore, Science Direct, Springer Link, PubMed Central, Plos One, among others) and allow searching using keywords combined with boolean operators. Likewise, the database should contain articles with different approaches to predictive models and not specialized in clinical aspects. Finally, the number of articles to be included in the systematic review should be sufficient to identify areas of opportunity for improving models’ development to predict diabetes.
Search strategy
Three main keywords were selected from the research questions. These keywords were combined in strings as required by each database in their advanced search tool. In other words, these strings were adapted to meet the criteria of each database Table 1 .
Eligibility criteria
Retrieved records from the initial search were screened to check their compliance with eligibility criteria.
Firstly, papers published from 2017 to 2021 only were considered. Then, two rounds of screening were conducted. The first round focused mainly on the scope of the reported study. Articles were excluded if the study used genetic data to train the models, as this was not a type of data of interest in this review. Also, articles were excluded if the full text was not available. Finally, review articles were also excluded.
In the second round of screening, articles were excluded when machine learning techniques were not used to predict type 2 diabetes but other types of diabetes, treatments, or diseases associated with diabetes (complications and related diseases associated with metabolic syndrome). Also, studies using unsupervised learning were excluded as they cannot be validated using the same performance metrics as supervised learning models, preventing comparison.
Quality assessment
After retrieving the selected articles, three parameters were selected, each one generated by each research question. The eligibility criteria are three possible subgroups according to the extent to which the article satisfied it.
The dataset contains sociodemographic and lifestyle data, clinical diagnosis, and laboratory test results as attributes for the model.
Dataset contains only one kind of attributes.
Dataset contains similar kinds of attributes.
Dataset uses EHRs with multiple kinds of attributes.
The article presents a model with a machine learning technique to predict type 2 diabetes.
Machine Learning methods are not used at all.
The prediction method in the model is used as part of the prepossessing for the data to do data mining.
Model used a machine learning technique to predict type 2 diabetes.
The authors use supervised learning with validation metrics to contrast their results with previous work.
The authors used unsupervised methods.
The authors used a supervised method with one validation metric or several methods with supervised and unsupervised learning.
The authors used supervised learning with more than one metric to validate the model (accuracy, specificity, sensitivity, area under the ROC, F1-score).
Data extraction
After assessing the papers for quality, the intersection of the subgroups QA2.3 and QA1.1 or QA1.2 or QA1.3 and QA3.2 or QA3.3 were processed as follows.
First, the selected articles were grouped in two possible ways according to the data type (glucose forecasting or electronic health records). The first group contains models that screen the control levels of blood glucose, while the second group contains models that predict diabetes based on electronic health records.
The second classification was more detailed, applying for each group the below criteria.
The data extraction criteria are:
Machine learning model (specify which machine learning method use)
Validation parameter (accuracy, sensitivity, specificity, F1-score, AUC (ROC))
Complementary techniques (complementary statistics and machine learning techniques used for the models)
Data sampling (cross-validation, training-test set, complete data)
Description of the population (age, balanced or imbalance, population cohort size).
Risk of bias analyses
Risk of bias in individual studies.
The risk of bias in individual studies (i.e., within-study bias) was assessed based on the characteristics of the sample included in the study and the dataset used to train and test the models. One of the most common risks of bias is when the data is imbalanced. When the dataset has significantly more observations for one label, the probability of selecting that label increases, leading to misclassification.
The second parameter that causes a risk of bias is the age of participants. In most cases, diabetes onset would be in older people making possible bound between 40 to 80 years. In other cases, the onset occurs at early age generating another dataset with a range from 21 to 80.
A third parameter strongly related to age is the early age onset. Complications increase and appear early when a patient lives more time with the disease, making it harder to develop a model only for diabetes without correlation of their complications.
Finally, as the fourth risk of bias, according to Forbes [ 24 ] data scientists spend 80% of their time on data preparation, and 60% of it is in data cleaning and organization. A well-structured dataset is relevant to generate a good performance of the model. That can be check in the results from the data items extraction the datasets like PIMA dataset that is already clean and organized well generate a model with the recall of 1 [ 25 ] also the same dataset reach an accuracy of 0.97 [ 26 ] in another model. Dirty data can not achieve values as good as clean data.
Risk of bias across studies
The items considered to assess the risk of bias across the studies (i.e., between-study bias) were the reported validation parameters and the dataset and complementary techniques used.
Validation metrics were chosen as they are used to compare the performance of the model. The studies must be compared using the same metrics to avoid bias from the validation methods.
The complementary techniques are essential since they can be combined with the primary approach to creating a better performance model. It causes a bias because it is impossible to discern if the combination of the complementary and the machine learning techniques produces good performance or if the machine learning technique per se is superior to others.
Search results and reduction
The initial search generated 1327 records, 925 from PubMed and 402 from Web of Science. Only 130 records were excluded when filtering by publication year (2017–2021). Therefore, further searches were conducted using fine-tuned search strings and options for both databases to narrow down the results. The new search was carried out using the original keywords but restricting the word ‘diabetes’ to be in the title, which generated 517 records from both databases. Fifty-one duplicates were discarded. Therefore, 336 records were selected for further screening.
Further selection was conducted by applying the exclusion criteria to the 336 records above. Thirty-seven records were excluded since the study reported used non-omittable genetic attributes as model inputs, something out of this review’s scope. Thirty-eight records were excluded as they were review papers. All in all, 261 articles that fulfill the criteria were included in the quality assessment.
Figure 1 shows the flow diagram summarizing this process.
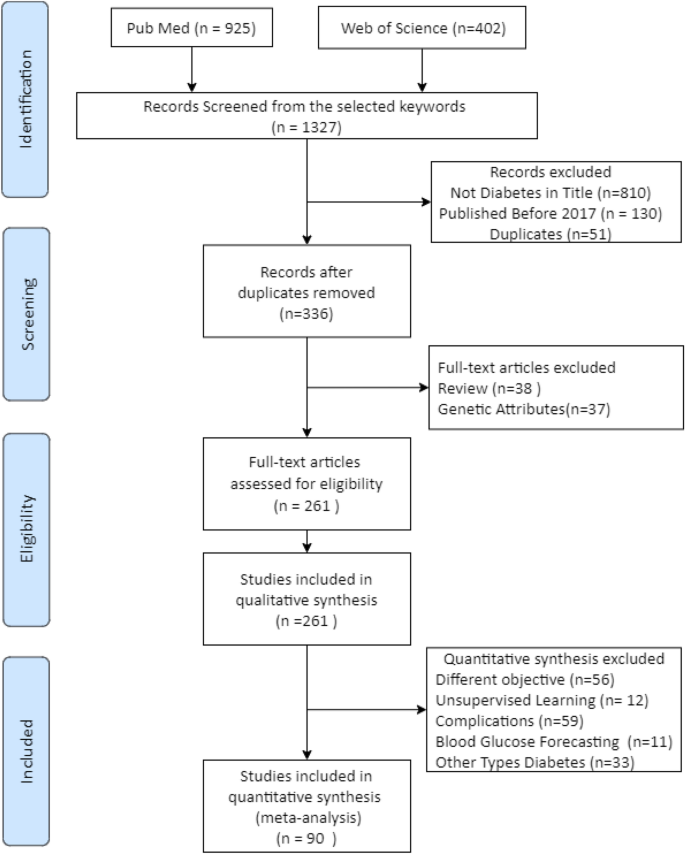
Flow diagram indicating the results of the systematic review with inclusions and exclusions
The 261 articles above were assessed for quality and classified into their corresponding subgroup for each quality question (Fig. 2 ).
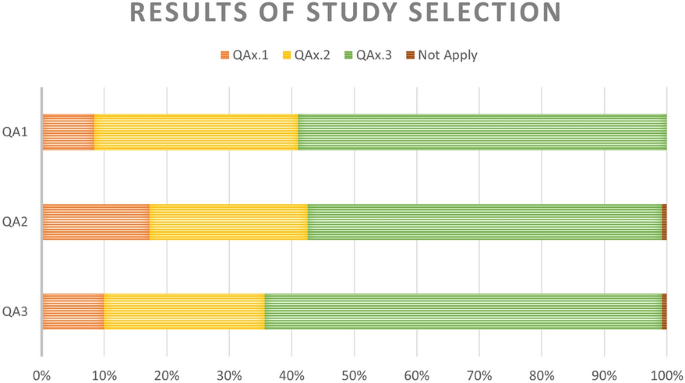
Percentage of each subgroup in the quality assessment. The criteria does not apply for two result for the Quality Assessment Questions 1 and 3
The first question classified the studies by the type of database used for building the models. The third subgroup represents the most desirable scenario. It includes studies where models were trained using features from Electronic Health Records or a mix of datasets including lifestyle, socio-demographic, and health diagnosis features. There were 22, 85, and 154 articles in subgroups one to three, respectively.
The second question classified the studies by the type of model used. Again, the third subgroup represents the most suitable subgroup as it contains studies where a machine learning model was used to predict diabetes onset. There were 46 studies in subgroup one, 66 in subgroup two, and 147 in subgroup three. Two studies were omitted from these subgroups: one used cancer-related model; another used a model of no interest to this review.
The third question clustered the studies based on their validation metrics. There were 25 studies in subgroup one (semi-supervised learning), 68 in subgroup two (only one validation metric), and 166 in subgroup three ( \(>1\) validation parameters). The criteria are not applied to two studies as they used special error metrics, making it impossible to compare their models with the rest.
Data extraction excluded 101 articles from the quantitative synthesis for two reasons. twelve studies used unsupervised learning. Nineteen studies focused on diabetes treatments, 33 in other types of diabetes (eighteen type 1 and fifteen Gestational), and 37 associated diseases.
Furthermore, 70 articles were left out of this review as they focus on the prediction of diabetes complications (59) or tried to forecast levels of glucose (11), not onset. Therefore, 90 articles were chosen for the next steps.
Table 2 summarize the results of the data extraction. These tables are divided into two main groups, each of them corresponding to a type of data.
For the risk of bias in the studies: unbalanced data means that the number of observations per class is not equally distributed. Some studies applied complementary techniques (e.g., SMOTE) to prevent the bias produced by unbalance in data. These techniques undersample the predominant class or oversample the minority class to produce a balanced dataset.
Other studies used different strategies to deal with other risks for bias. For instance, they might exclude specific age groups or cases presenting a second disease that could interfere with the model’s development to deal with the heterogeneity in some cohorts’ age.
For the risk of bias across the studies: the comparison between models was performed on those reporting the most frequently used validation metrics, i.e., accuracy and AUC (ROC). The accuracy is estimated to homogenize the criteria of comparison when other metrics from the confusion matrix were calculated, or the population’s knowledge is known. The confusion matrix is a two-by-two matrix containing four counts: true positives, true negatives, false positives, and false negatives. Different validation metrics such as precision, recall, accuracy, and F1-score are computed from this matrix.
Two kinds of complementary techniques were found. Firstly, techniques for balancing the data, including oversampling and undersampling methods. Secondly, feature selection techniques such as logistic regression, principal component analysis, and statistical testing. A comparison still can be performed between them with the bias caused by the improvement of the model.
This section discusses the findings for each of the research questions driving this review.
RQ1: What kind of features makes up the database to create the model?
Our findings suggest no agreement on the specific features to create a predictive model for type 2 diabetes. The number of features also differs between studies: while some used a few features, others used more than 70 features. The number and choice of features largely depended on the machine learning technique and the model’s complexity.
However, our findings suggest that some data types produce better models, such as lifestyle, socioeconomic and diagnostic data. These data are available in most but not all Electronic Health Records. Also, retinal fundus images were used in many of the top models, as they are related to eye vessel damage derivated from diabetes. Unfortunately, this type of image is no available in primary care data.
RQ2: What machine learning technique is optimal to create a predictive model for type 2 diabetes?
Figure 3 shows a scatter plot of studies that reported accuracy and AUC (ROC) values (x and y axes, respectively. The color of the dots represents thirteen of the eighteen types of model listed in the background. Dot labels represent the reference number of the study. A total of 30 studies is included in the plot. The studies closer to the top-right corner are the best ones, as they obtained high values for both validation metrics.
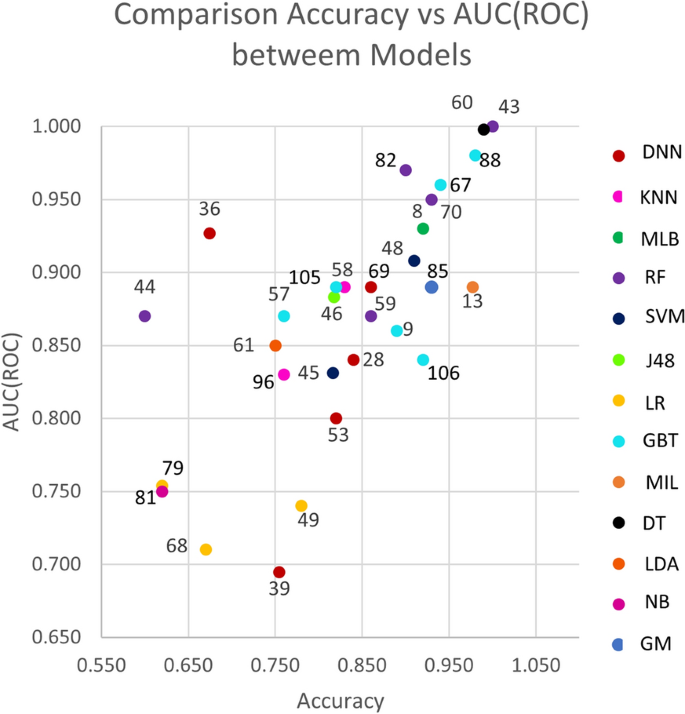
Scatterplot of AUC (ROC) vs. Accuracy for included studies. Numbers correspond to the number of reference and color dot the type of model, desired model has values of x-axis equal 1 and y-axis also equal 1
Figures 4 and 5 show the average accuracy and AUC (ROC) by model. Not all models from the background appear in both graphs since not all studies reported both metrics. Notably, most values represent a single study or the average of two studies. The exception is the average values for SVMs, RFs, GBTs, and DNNs, calculated with the results reported by four studies or more. These were the most popular machine learning techniques in the included studies.
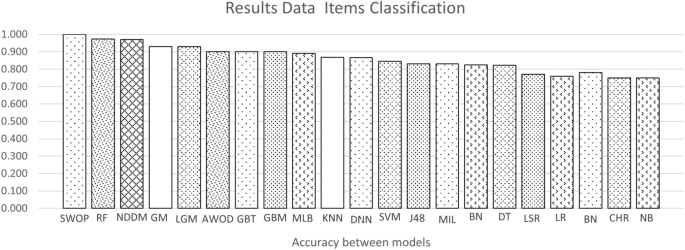
Average accuracy by model. For papers with more than one model the best score is the model selected to the graph. A better model has a higher value
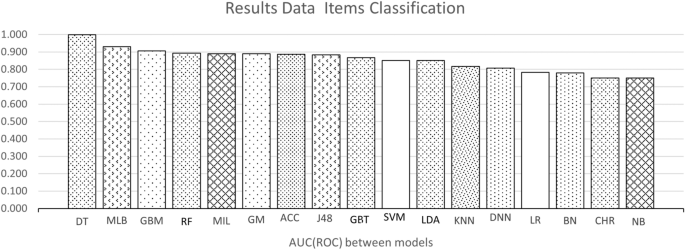
Average AUC (ROC) by model. For papers with more than one model the best score is the model selected to the graph. A better model has a higher value
RQ3: Which are the optimal validation metrics to compare the models’ improvement?
Considerable heterogeneity was found in this regard, making it harder to compare the performance between the models. Most studies reported some metrics computed from the confusion matrix. However, studies focused on statistical learning models reported hazard ratios and the c-statistic.
This heterogeneity remains an area of opportunity for further studies. To deal with it, we propose reporting at least three metrics from the confusion matrix (i.e., accuracy, sensitivity, and specificity), which would allow computing the rest. Additionally, the AUC (ROC) should be reported as it is a robust performance metric. Ideally, other metrics such as the F1-score, precision, or the MCC score should be reported. Reporting more metrics would enable benchmarking studies and models.
Summary of the findings
Concerning the datasets, this review could not identify an exact list of features given the heterogeneity mentioned above. However, there are some findings to report. First, the model’s performance is significantly affected by the dataset: the accuracy decreased significantly when the dataset became big and complex. Clean and well-structured datasets with a few numbers of samples and features make a better model. However, a low number of attributes may not reflect the real complexity of the multi-factorial diseases.
The top-performing models were the decision tree and random forest, with an similar accuracy of 0.99 and equal AUC (ROC) of one. On average, the best models for the accuracy metric were Swarm Optimization and Random Forest with a value of one in both cases. For AUC (ROC) decision tree with an AUC (ROC) of 0.98, respectively.
The most frequently-used methods were Deep Neural Networks, tree-type (Gradient Boosting and Random Forest), and support vector machines. Deep Neural Networks have the advantage of dealing well with big data, a solid reason to use them frequently [ 27 , 28 ]. Studies using these models used datasets containing more than 70,000 observations. Also, these models deal well with dirty data.
Some studies used complementary techniques to improve their model’s performance. First, resampling techniques were applied to otherwise unbalanced datasets. Second, feature selection techniques were used to identify the most relevant features for prediction. Among the latter, there is principal component analysis and logistic regression.
The model that has a good performance but can be improved is the Deep Neural Network. As shown in Figure 4 , their average accuracy is not top, yet some individual models achieved 0.9. Hence, they represent a technique worth further exploration in type 2 diabetes. They also have the advantage that can deal with large datasets. As shown in Table 2 many of the datasets used for DNN models were around 70,000 or more samples. Also, DNN models do not require complementary techniques for feature selection.
Finally, model performance comparison was challenging due to the heterogeneity in the metrics reported.
Conclusions
This systematic review analyzed 90 studies to find the main opportunity areas in diabetes prediction using machine learning techniques.
The review finds that the structure of the dataset is relevant to the accuracy of the models, regardless of the selected features that are heterogeneous between studies. Concerning the models, the optimal performance is for tree-type models. However, even tough they have the best accuracy, they require complementary techniques to balance data and reduce dimensionality by selecting the optimal features. Therefore, K nearest neighborhoods, and Support vector machines are frequently preferred for prediction. On the other hand, Deep Neural Networks have the advantage of dealing well with big data. However, they must be applied to datasets with more than 70,000 observations. At least three metrics and the AUC (ROC) should be reported in the results to allow estimation of the others to reduce heterogeneity in the performance comparison. Therefore, the areas of opportunity are listed below.
Areas of opportunity
First, a well-structured, balanced dataset containing different types of features like lifestyle, socioeconomically, and diagnostic data can be created to obtain a good model. Otherwise, complementary techniques can be helpful to clean and balance the data.
The machine learning model will depend on the characteristics of the dataset. When the dataset contains a few observations, machine learning techniques present a better performance; when observations are more than 70,000, Deep Learning has a good performance.
To reduce the heterogeneity in the validation parameters, the best way to do it is to calculate a minimum of three parameters from the confusion matrix and the AUC (ROC). Ideally, it should report five or more parameters (accuracy, sensitivity, specificity, precision, and F1-score) to become easier to compare. If one misses, it can be estimated from the other ones.
Limitations of the study
The study’s limitations are observed in the heterogeneity between the models that difficult to compare them. This heterogeneity is present in many aspects; the main is the populations and the number of samples used in each model. Another significant limitation is when the model predicts diabetes complications, not diabetes.
Availability of data and materials
All data generated or analysed during this study are included in this published article and its references.
Abbreviations
Deep Neural Network
Random forest
Support Vector Machine
k-Nearest Neighbors
Decision tree
Gradient Boosting Tree
Gradient Boost Machine
J48 decision tree
Logistic regression and stepwise regression
Linear and quadratric discriminant analysis
Multiple Instance Learning boosting
Bayesian Network
Latent growth mixture
Cox Hazard Regression
Least-Square Regression
Least absolute shrinkage and selection operator
Smoothed clipped absolute deviation
Minimax concave penalized likelihood
Alternating Cluster and Classification
Machine learning-based method
Synthetic minority oversampling technique
Area under curve (receiver operating characteristic)
Diabetic retinopathy
Gaussian mixture
Naive Bayes
Average weighted objective distance
Swarm Optimization
Newton’s Divide Difference Method
Root-mean-square error
AD Association. Classification and diagnosis of diabetes: standards of medical care in diabetes-2020. Diabetes Care. 2019. https://doi.org/10.2337/dc20-S002 .
Article Google Scholar
International Diabetes Federation. Diabetes. Brussels: International Diabetes Federation; 2019.
Google Scholar
Gregg EW, Sattar N, Ali MK. The changing face of diabetes complications. Lancet Diabetes Endocrinol. 2016;4(6):537–47. https://doi.org/10.1016/s2213-8587(16)30010-9 .
Article PubMed Google Scholar
Herman WH, Ye W, Griffin SJ, Simmons RK, Davies MJ, Khunti K, Rutten GEhm, Sandbaek A, Lauritzen T, Borch-Johnsen K, et al. Early detection and treatment of type 2 diabetes reduce cardiovascular morbidity and mortality: a simulation of the results of the Anglo-Danish-Dutch study of intensive treatment in people with screen-detected diabetes in primary care (addition-Europe). Diabetes Care. 2015;38(8):1449–55. https://doi.org/10.2337/dc14-2459 .
Article PubMed PubMed Central Google Scholar
Kälsch J, Bechmann LP, Heider D, Best J, Manka P, Kälsch H, Sowa J-P, Moebus S, Slomiany U, Jöckel K-H, et al. Normal liver enzymes are correlated with severity of metabolic syndrome in a large population based cohort. Sci Rep. 2015;5(1):1–9. https://doi.org/10.1038/srep13058 .
Article CAS Google Scholar
Sanal MG, Paul K, Kumar S, Ganguly NK. Artificial intelligence and deep learning: the future of medicine and medical practice. J Assoc Physicians India. 2019;67(4):71–3.
PubMed Google Scholar
Zhang A, Lipton ZC, Li M, Smola AJ. Dive into deep learning. 2020. https://d2l.ai .
Maniruzzaman M, Kumar N, Abedin MM, Islam MS, Suri HS, El-Baz AS, Suri JS. Comparative approaches for classification of diabetes mellitus data: machine learning paradigm. Comput Methods Programs Biomed. 2017;152:23–34. https://doi.org/10.1016/j.cmpb.2017.09.004 .
Muhammad LJ, Algehyne EA, Usman SS. Predictive supervised machine learning models for diabetes mellitus. SN Comput Sci. 2020;1(5):1–10. https://doi.org/10.1007/s42979-020-00250-8 .
Alghamdi M, Al-Mallah M, Keteyian S, Brawner C, Ehrman J, Sakr S. Predicting diabetes mellitus using smote and ensemble machine learning approach: the henry ford exercise testing (fit) project. PLoS ONE. 2017;12(7):e0179805. https://doi.org/10.1371/journal.pone.0179805 .
Article CAS PubMed PubMed Central Google Scholar
Mokarram R, Emadi M. Classification in non-linear survival models using cox regression and decision tree. Ann Data Sci. 2017;4(3):329–40. https://doi.org/10.1007/s40745-017-0105-4 .
Ivanova MT, Radoukova TI, Dospatliev LK, Lacheva MN. Ordinary least squared linear regression model for estimation of zinc in wild edible mushroom ( Suillus luteus (L.) roussel). Bulg J Agric Sci. 2020;26(4):863–9.
Bernardini M, Morettini M, Romeo L, Frontoni E, Burattini L. Early temporal prediction of type 2 diabetes risk condition from a general practitioner electronic health record: a multiple instance boosting approach. Artif Intell Med. 2020;105:101847. https://doi.org/10.1016/j.artmed.2020.101847 .
Xie J, Liu Y, Zeng X, Zhang W, Mei Z. A Bayesian network model for predicting type 2 diabetes risk based on electronic health records. Modern Phys Lett B. 2017;31(19–21):1740055. https://doi.org/10.1142/s0217984917400553 .
Hertroijs DFL, Elissen AMJ, Brouwers MCGJ, Schaper NC, Köhler S, Popa MC, Asteriadis S, Hendriks SH, Bilo HJ, Ruwaard D, et al. A risk score including body mass index, glycated haemoglobin and triglycerides predicts future glycaemic control in people with type 2 diabetes. Diabetes Obes Metab. 2017;20(3):681–8. https://doi.org/10.1111/dom.13148 .
Cole SR, Chu H, Greenland S. Maximum likelihood, profile likelihood, and penalized likelihood: a primer. Am J Epidemiol. 2013;179(2):252–60. https://doi.org/10.1093/aje/kwt245 .
Brisimi TS, Xu T, Wang T, Dai W, Paschalidis IC. Predicting diabetes-related hospitalizations based on electronic health records. Stat Methods Med Res. 2018;28(12):3667–82. https://doi.org/10.1177/0962280218810911 .
Moher D, Liberati A, Tetzlaff J, Altman DG. Preferred reporting items for systematic reviews and meta-analyses: the prisma statement. PLoS Med. 2009;6(7):e1000097. https://doi.org/10.1371/journal.pmed.1000097 .
Kitchenham B, Brereton OP, Budgen D, Turner M, Bailey J, Linkman S. Systematic literature reviews in software engineering—a systematic literature review. Inf Softw Technol. 2009;51(1):7–15. https://doi.org/10.1016/j.infsof.2008.09.009 .
Sambyal N, Saini P, Syal R. Microvascular complications in type-2 diabetes: a review of statistical techniques and machine learning models. Wirel Pers Commun. 2020;115(1):1–26. https://doi.org/10.1007/s11277-020-07552-3 .
Islam MM, Yang H-C, Poly TN, Jian W-S, Li Y-CJ. Deep learning algorithms for detection of diabetic retinopathy in retinal fundus photographs: a systematic review and meta-analysis. Comput Methods Programs Biomed. 2020;191:105320. https://doi.org/10.1016/j.cmpb.2020.105320 .
Chaki J, Ganesh ST, Cidham SK, Theertan SA. Machine learning and artificial intelligence based diabetes mellitus detection and self-management: a systematic review. J King Saud Univ Comput Inf Sci. 2020. https://doi.org/10.1016/j.jksuci.2020.06.013 .
Silva KD, Lee WK, Forbes A, Demmer RT, Barton C, Enticott J. Use and performance of machine learning models for type 2 diabetes prediction in community settings: a systematic review and meta-analysis. Int J Med Inform. 2020;143:104268. https://doi.org/10.1016/j.ijmedinf.2020.104268 .
Press G. Cleaning big data: most time-consuming, least enjoyable data science task, survey says. Forbes; 2016.
Prabhu P, Selvabharathi S. Deep belief neural network model for prediction of diabetes mellitus. In: 2019 3rd international conference on imaging, signal processing and communication (ICISPC). 2019. https://doi.org/10.1109/icispc.2019.8935838 .
Albahli S. Type 2 machine learning: an effective hybrid prediction model for early type 2 diabetes detection. J Med Imaging Health Inform. 2020;10(5):1069–75. https://doi.org/10.1166/jmihi.2020.3000 .
Maxwell A, Li R, Yang B, Weng H, Ou A, Hong H, Zhou Z, Gong P, Zhang C. Deep learning architectures for multi-label classification of intelligent health risk prediction. BMC Bioinform. 2017;18(S14):121–31. https://doi.org/10.1186/s12859-017-1898-z .
Nguyen BP, Pham HN, Tran H, Nghiem N, Nguyen QH, Do TT, Tran CT, Simpson CR. Predicting the onset of type 2 diabetes using wide and deep learning with electronic health records. Comput Methods Programs Biomed. 2019;182:105055. https://doi.org/10.1016/j.cmpb.2019.105055 .
Arellano-Campos O, Gómez-Velasco DV, Bello-Chavolla OY, Cruz-Bautista I, Melgarejo-Hernandez MA, Muñoz-Hernandez L, Guillén LE, Garduño-Garcia JDJ, Alvirde U, Ono-Yoshikawa Y, et al. Development and validation of a predictive model for incident type 2 diabetes in middle-aged Mexican adults: the metabolic syndrome cohort. BMC Endocr Disord. 2019;19(1):1–10. https://doi.org/10.1186/s12902-019-0361-8 .
You Y, Doubova SV, Pinto-Masis D, Pérez-Cuevas R, Borja-Aburto VH, Hubbard A. Application of machine learning methodology to assess the performance of DIABETIMSS program for patients with type 2 diabetes in family medicine clinics in Mexico. BMC Med Inform Decis Mak. 2019;19(1):1–15. https://doi.org/10.1186/s12911-019-0950-5 .
Pham T, Tran T, Phung D, Venkatesh S. Predicting healthcare trajectories from medical records: a deep learning approach. J Biomed Inform. 2017;69:218–29. https://doi.org/10.1016/j.jbi.2017.04.001 .
Spänig S, Emberger-Klein A, Sowa J-P, Canbay A, Menrad K, Heider D. The virtual doctor: an interactive clinical-decision-support system based on deep learning for non-invasive prediction of diabetes. Artif Intell Med. 2019;100:101706. https://doi.org/10.1016/j.artmed.2019.101706 .
Wang T, Xuan P, Liu Z, Zhang T. Assistant diagnosis with Chinese electronic medical records based on CNN and BILSTM with phrase-level and word-level attentions. BMC Bioinform. 2020;21(1):1–16. https://doi.org/10.1186/s12859-020-03554-x .
Kim YD, Noh KJ, Byun SJ, Lee S, Kim T, Sunwoo L, Lee KJ, Kang S-H, Park KH, Park SJ, et al. Effects of hypertension, diabetes, and smoking on age and sex prediction from retinal fundus images. Sci Rep. 2020;10(1):1–14. https://doi.org/10.1038/s41598-020-61519-9 .
Bernardini M, Romeo L, Misericordia P, Frontoni E. Discovering the type 2 diabetes in electronic health records using the sparse balanced support vector machine. IEEE J Biomed Health Inform. 2020;24(1):235–46. https://doi.org/10.1109/JBHI.2019.2899218 .
Mei J, Zhao S, Jin F, Zhang L, Liu H, Li X, Xie G, Li X, Xu M. Deep diabetologist: learning to prescribe hypoglycemic medications with recurrent neural networks. Stud Health Technol Inform. 2017;245:1277. https://doi.org/10.3233/978-1-61499-830-3-1277 .
Solares JRA, Canoy D, Raimondi FED, Zhu Y, Hassaine A, Salimi-Khorshidi G, Tran J, Copland E, Zottoli M, Pinho-Gomes A, et al. Long-term exposure to elevated systolic blood pressure in predicting incident cardiovascular disease: evidence from large-scale routine electronic health records. J Am Heart Assoc. 2019;8(12):e012129. https://doi.org/10.1161/jaha.119.012129 .
Kumar PS, Pranavi S. Performance analysis of machine learning algorithms on diabetes dataset using big data analytics. In: 2017 international conference on infocom technologies and unmanned systems (trends and future directions) (ICTUS). 2017. https://doi.org/10.1109/ictus.2017.8286062 .
Olivera AR, Roesler V, Iochpe C, Schmidt MI, Vigo A, Barreto SM, Duncan BB. Comparison of machine-learning algorithms to build a predictive model for detecting undiagnosed diabetes-ELSA-Brasil: accuracy study. Sao Paulo Med J. 2017;135(3):234–46. https://doi.org/10.1590/1516-3180.2016.0309010217 .
Peddinti G, Cobb J, Yengo L, Froguel P, Kravić J, Balkau B, Tuomi T, Aittokallio T, Groop L. Early metabolic markers identify potential targets for the prevention of type 2 diabetes. Diabetologia. 2017;60(9):1740–50. https://doi.org/10.1007/s00125-017-4325-0 .
Dutta D, Paul D, Ghosh P. Analysing feature importances for diabetes prediction using machine learning. In: 2018 IEEE 9th annual information technology, electronics and mobile communication conference (IEMCON). 2018. https://doi.org/10.1109/iemcon.2018.8614871 .
Alhassan Z, Mcgough AS, Alshammari R, Daghstani T, Budgen D, Moubayed NA. Type-2 diabetes mellitus diagnosis from time series clinical data using deep learning models. In: artificial neural networks and machine learning—ICANN 2018 lecture notes in computer science. 2018. p. 468–78. https://doi.org/10.1007/978-3-030-01424-7_46 .
Kuo K-M, Talley P, Kao Y, Huang CH. A multi-class classification model for supporting the diagnosis of type II diabetes mellitus. PeerJ. 2020;8:e9920. https://doi.org/10.7717/peerj.992 .
Pimentel A, Carreiro AV, Ribeiro RT, Gamboa H. Screening diabetes mellitus 2 based on electronic health records using temporal features. Health Inform J. 2018;24(2):194–205. https://doi.org/10.1177/1460458216663023 .
Talaei-Khoei A, Wilson JM. Identifying people at risk of developing type 2 diabetes: a comparison of predictive analytics techniques and predictor variables. Int J Med Inform. 2018;119:22–38. https://doi.org/10.1016/j.ijmedinf.2018.08.008 .
Perveen S, Shahbaz M, Keshavjee K, Guergachi A. Metabolic syndrome and development of diabetes mellitus: predictive modeling based on machine learning techniques. IEEE Access. 2019;7:1365–75. https://doi.org/10.1109/access.2018.2884249 .
Yuvaraj N, Sripreethaa KR. Diabetes prediction in healthcare systems using machine learning algorithms on Hadoop cluster. Cluster Comput. 2017;22(S1):1–9. https://doi.org/10.1007/s10586-017-1532-x .
Deo R, Panigrahi S. Performance assessment of machine learning based models for diabetes prediction. In: 2019 IEEE healthcare innovations and point of care technologies, (HI-POCT). 2019. https://doi.org/10.1109/hi-poct45284.2019.8962811 .
Jakka A, Jakka VR. Performance evaluation of machine learning models for diabetes prediction. Int J Innov Technol Explor Eng Regular Issue. 2019;8(11):1976–80. https://doi.org/10.35940/ijitee.K2155.0981119 .
Radja M, Emanuel AWR. Performance evaluation of supervised machine learning algorithms using different data set sizes for diabetes prediction. In: 2019 5th international conference on science in information technology (ICSITech). 2019. https://doi.org/10.1109/icsitech46713.2019.8987479 .
Choi BG, Rha S-W, Kim SW, Kang JH, Park JY, Noh Y-K. Machine learning for the prediction of new-onset diabetes mellitus during 5-year follow-up in non-diabetic patients with cardiovascular risks. Yonsei Med J. 2019;60(2):191. https://doi.org/10.3349/ymj.2019.60.2.191 .
Akula R, Nguyen N, Garibay I. Supervised machine learning based ensemble model for accurate prediction of type 2 diabetes. In: 2019 SoutheastCon. 2019. https://doi.org/10.1109/southeastcon42311.2019.9020358 .
Xie Z, Nikolayeva O, Luo J, Li D. Building risk prediction models for type 2 diabetes using machine learning techniques. Prev Chronic Dis. 2019. https://doi.org/10.5888/pcd16.190109 .
Lai H, Huang H, Keshavjee K, Guergachi A, Gao X. Predictive models for diabetes mellitus using machine learning techniques. BMC Endocr Disord. 2019;19(1):1–9. https://doi.org/10.1186/s12902-019-0436-6 .
Abbas H, Alic L, Erraguntla M, Ji J, Abdul-Ghani M, Abbasi Q, Qaraqe M. Predicting long-term type 2 diabetes with support vector machine using oral glucose tolerance test. bioRxiv. 2019. https://doi.org/10.1371/journal.pone.0219636 .
Sarker I, Faruque M, Alqahtani H, Kalim A. K-nearest neighbor learning based diabetes mellitus prediction and analysis for ehealth services. EAI Endorsed Trans Scalable Inf Syst. 2020. https://doi.org/10.4108/eai.13-7-2018.162737 .
Cahn A, Shoshan A, Sagiv T, Yesharim R, Goshen R, Shalev V, Raz I. Prediction of progression from pre-diabetes to diabetes: development and validation of a machine learning model. Diabetes Metab Res Rev. 2020;36(2):e3252. https://doi.org/10.1002/dmrr.3252 .
Garcia-Carretero R, Vigil-Medina L, Mora-Jimenez I, Soguero-Ruiz C, Barquero-Perez O, Ramos-Lopez J. Use of a k-nearest neighbors model to predict the development of type 2 diabetes within 2 years in an obese, hypertensive population. Med Biol Eng Comput. 2020;58(5):991–1002. https://doi.org/10.1007/s11517-020-02132-w .
Zhang L, Wang Y, Niu M, Wang C, Wang Z. Machine learning for characterizing risk of type 2 diabetes mellitus in a rural Chinese population: the Henan rural cohort study. Sci Rep. 2020;10(1):1–10. https://doi.org/10.1038/s41598-020-61123-x .
Haq AU, Li JP, Khan J, Memon MH, Nazir S, Ahmad S, Khan GA, Ali A. Intelligent machine learning approach for effective recognition of diabetes in e-healthcare using clinical data. Sensors. 2020;20(9):2649. https://doi.org/10.3390/s20092649 .
Article PubMed Central Google Scholar
Yang T, Zhang L, Yi L, Feng H, Li S, Chen H, Zhu J, Zhao J, Zeng Y, Liu H, et al. Ensemble learning models based on noninvasive features for type 2 diabetes screening: model development and validation. JMIR Med Inform. 2020;8(6):e15431. https://doi.org/10.2196/15431 .
Ahn H-S, Kim JH, Jeong H, Yu J, Yeom J, Song SH, Kim SS, Kim IJ, Kim K. Differential urinary proteome analysis for predicting prognosis in type 2 diabetes patients with and without renal dysfunction. Int J Mol Sci. 2020;21(12):4236. https://doi.org/10.3390/ijms21124236 .
Article CAS PubMed Central Google Scholar
Sarwar MA, Kamal N, Hamid W, Shah MA. Prediction of diabetes using machine learning algorithms in healthcare. In: 2018 24th international conference on automation and computing (ICAC). 2018. https://doi.org/10.23919/iconac.2018.8748992 .
Zou Q, Qu K, Luo Y, Yin D, Ju Y, Tang H. Predicting diabetes mellitus with machine learning techniques. Front Genet. 2018;9:515. https://doi.org/10.3389/fgene.2018.00515 .
Farran B, AlWotayan R, Alkandari H, Al-Abdulrazzaq D, Channanath A, Thanaraj TA. Use of non-invasive parameters and machine-learning algorithms for predicting future risk of type 2 diabetes: a retrospective cohort study of health data from Kuwait. Front Endocrinol. 2019;10:624. https://doi.org/10.3389/fendo.2019.00624 .
Xiong X-L, Zhang R-X, Bi Y, Zhou W-H, Yu Y, Zhu D-L. Machine learning models in type 2 diabetes risk prediction: results from a cross-sectional retrospective study in Chinese adults. Curr Med Sci. 2019;39(4):582–8. https://doi.org/10.1007/s11596-019-2077-4 .
Dinh A, Miertschin S, Young A, Mohanty SD. A data-driven approach to predicting diabetes and cardiovascular disease with machine learning. BMC Med Inform Decis Mak. 2019;19(1):1–15. https://doi.org/10.1186/s12911-019-0918-5 .
Liu Y, Ye S, Xiao X, Sun C, Wang G, Wang G, Zhang B. Machine learning for tuning, selection, and ensemble of multiple risk scores for predicting type 2 diabetes. Risk Manag Healthc Policy. 2019;12:189–98. https://doi.org/10.2147/rmhp.s225762 .
Tang Y, Gao R, Lee HH, Wells QS, Spann A, Terry JG, Carr JJ, Huo Y, Bao S, Landman BA, et al. Prediction of type II diabetes onset with computed tomography and electronic medical records. In: Multimodal learning for clinical decision support and clinical image-based procedures. Cham: Springer; 2020. p. 13–23. https://doi.org/10.1007/978-3-030-60946-7_2 .
Chapter Google Scholar
Maniruzzaman M, Rahman MJ, Ahammed B, Abedin MM. Classification and prediction of diabetes disease using machine learning paradigm. Health Inf Sci Syst. 2020;8(1):1–14. https://doi.org/10.1007/s13755-019-0095-z .
Boutilier JJ, Chan TCY, Ranjan M, Deo S. Risk stratification for early detection of diabetes and hypertension in resource-limited settings: machine learning analysis. J Med Internet Res. 2021;23(1):20123. https://doi.org/10.2196/20123 .
Li J, Chen Q, Hu X, Yuan P, Cui L, Tu L, Cui J, Huang J, Jiang T, Ma X, Yao X, Zhou C, Lu H, Xu J. Establishment of noninvasive diabetes risk prediction model based on tongue features and machine learning techniques. Int J Med Inform. 2021;149:104429. https://doi.org/10.1016/j.ijmedinf.2021.10442 .
Lam B, Catt M, Cassidy S, Bacardit J, Darke P, Butterfield S, Alshabrawy O, Trenell M, Missier P. Using wearable activity trackers to predict type 2 diabetes: machine learning-based cross-sectional study of the UK biobank accelerometer cohort. JMIR Diabetes. 2021;6(1):23364. https://doi.org/10.2196/23364 .
Deberneh HM, Kim I. Prediction of Type 2 diabetes based on machine learning algorithm. Int J Environ Res Public Health. 2021;18(6):3317. https://doi.org/10.3390/ijerph1806331 .
He Y, Lakhani CM, Rasooly D, Manrai AK, Tzoulaki I, Patel CJ. Comparisons of polyexposure, polygenic, and clinical risk scores in risk prediction of type 2 diabetes. Diabetes Care. 2021;44(4):935–43. https://doi.org/10.2337/dc20-2049 .
García-Ordás MT, Benavides C, Benítez-Andrades JA, Alaiz-Moretón H, García-Rodríguez I. Diabetes detection using deep learning techniques with oversampling and feature augmentation. Comput Methods Programs Biomed. 2021;202:105968. https://doi.org/10.1016/j.cmpb.2021.105968 .
Kanimozhi N, Singaravel G. Hybrid artificial fish particle swarm optimizer and kernel extreme learning machine for type-II diabetes predictive model. Med Biol Eng Comput. 2021;59(4):841–67. https://doi.org/10.1007/s11517-021-02333-x .
Article CAS PubMed Google Scholar
Ravaut M, Sadeghi H, Leung KK, Volkovs M, Kornas K, Harish V, Watson T, Lewis GF, Weisman A, Poutanen T, et al. Predicting adverse outcomes due to diabetes complications with machine learning using administrative health data. NPJ Digit Med. 2021;4(1):1–12. https://doi.org/10.1038/s41746-021-00394-8 .
De Silva K, Lim S, Mousa A, Teede H, Forbes A, Demmer RT, Jonsson D, Enticott J. Nutritional markers of undiagnosed type 2 diabetes in adults: findings of a machine learning analysis with external validation and benchmarking. PLoS ONE. 2021;16(5):e0250832. https://doi.org/10.1371/journal.pone.025083 .
Kim H, Lim DH, Kim Y. Classification and prediction on the effects of nutritional intake on overweight/obesity, dyslipidemia, hypertension and type 2 diabetes mellitus using deep learning model: 4–7th Korea national health and nutrition examination survey. Int J Environ Res Public Health. 2021;18(11):5597. https://doi.org/10.3390/ijerph18115597 .
Vangeepuram N, Liu B, Chiu P-H, Wang L, Pandey G. Predicting youth diabetes risk using NHANES data and machine learning. Sci Rep. 2021;11(1):1. https://doi.org/10.1038/s41598-021-90406- .
Recenti M, Ricciardi C, Edmunds KJ, Gislason MK, Sigurdsson S, Carraro U, Gargiulo P. Healthy aging within an image: using muscle radiodensitometry and lifestyle factors to predict diabetes and hypertension. IEEE J Biomed Health Inform. 2021;25(6):2103–12. https://doi.org/10.1109/JBHI.2020.304415 .
Ramesh J, Aburukba R, Sagahyroon A. A remote healthcare monitoring framework for diabetes prediction using machine learning. Healthc Technol Lett. 2021;8(3):45–57. https://doi.org/10.1049/htl2.12010 .
Lama L, Wilhelmsson O, Norlander E, Gustafsson L, Lager A, Tynelius P, Wärvik L, Östenson C-G. Machine learning for prediction of diabetes risk in middle-aged Swedish people. Heliyon. 2021;7(7):e07419. https://doi.org/10.1016/j.heliyon.2021.e07419 .
Shashikant R, Chaskar U, Phadke L, Patil C. Gaussian process-based kernel as a diagnostic model for prediction of type 2 diabetes mellitus risk using non-linear heart rate variability features. Biomed Eng Lett. 2021;11(3):273–86. https://doi.org/10.1007/s13534-021-00196-7 .
Kalagotla SK, Gangashetty SV, Giridhar K. A novel stacking technique for prediction of diabetes. Comput Biol Med. 2021;135:104554. https://doi.org/10.1016/j.compbiomed.2021.104554 .
Moon S, Jang J-Y, Kim Y, Oh C-M. Development and validation of a new diabetes index for the risk classification of present and new-onset diabetes: multicohort study. Sci Rep. 2021;11(1):1–10. https://doi.org/10.1038/s41598-021-95341-8 .
Ihnaini B, Khan MA, Khan TA, Abbas S, Daoud MS, Ahmad M, Khan MA. A smart healthcare recommendation system for multidisciplinary diabetes patients with data fusion based on deep ensemble learning. Comput Intell Neurosci. 2021;2021:1–11. https://doi.org/10.1155/2021/4243700 .
Rufo DD, Debelee TG, Ibenthal A, Negera WG. Diagnosis of diabetes mellitus using gradient boosting machine (LightGBM). Diagnostics. 2021;11(9):1714. https://doi.org/10.3390/diagnostics11091714 .
Haneef R, Fuentes S, Fosse-Edorh S, Hrzic R, Kab S, Cosson E, Gallay A. Use of artificial intelligence for public health surveillance: a case study to develop a machine learning-algorithm to estimate the incidence of diabetes mellitus in France. Arch Public Health. 2021. https://doi.org/10.21203/rs.3.rs-139421/v1 .
Wei H, Sun J, Shan W, Xiao W, Wang B, Ma X, Hu W, Wang X, Xia Y. Environmental chemical exposure dynamics and machine learning-based prediction of diabetes mellitus. Sci Tot Environ. 2022;806:150674. https://doi.org/10.1016/j.scitotenv.2021.150674 .
Leerojanaprapa K, Sirikasemsuk K. Comparison of Bayesian networks for diabetes prediction. In: International conference on computer, communication and computational sciences (IC4S), Bangkok, Thailand, Oct 20–21, 2018. 2019;924:425–434. https://doi.org/10.1007/978-981-13-6861-5_37 .
Subbaiah S, Kavitha M. Random forest algorithm for predicting chronic diabetes disease. Int J Life Sci Pharma Res. 2020;8:4–8.
Thenappan S, Rajkumar MV, Manoharan PS. Predicting diabetes mellitus using modified support vector machine with cloud security. IETE J Res. 2020. https://doi.org/10.1080/03772063.2020.178278 .
Sneha N, Gangil T. Analysis of diabetes mellitus for early prediction using optimal features selection. J Big Data. 2019;6(1):1–19. https://doi.org/10.1186/s40537-019-0175-6 .
Jain S. A supervised model for diabetes divination. Biosci Biotechnol Res Commun. 2020;13(14, SI):315–8. https://doi.org/10.21786/bbrc/13.14/7 .
Syed AH, Khan T. Machine learning-based application for predicting risk of type 2 diabetes mellitus (T2DM) in Saudi Arabia: a retrospective cross-sectional study. IEEE Access. 2020;8:199539–61. https://doi.org/10.1109/ACCESS.2020.303502 .
Nuankaew P, Chaising S, Temdee P. Average weighted objective distance-based method for type 2 diabetes prediction. IEEE Access. 2021;9:137015–28. https://doi.org/10.1109/ACCESS.2021.311726 .
Samreen S. Memory-efficient, accurate and early diagnosis of diabetes through a machine learning pipeline employing crow search-based feature engineering and a stacking ensemble. IEEE Access. 2021;9:134335–54. https://doi.org/10.1109/ACCESS.2021.311638 .
Fazakis N, Kocsis O, Dritsas E, Alexiou S, Fakotakis N, Moustakas K. Machine learning tools for long-term type 2 diabetes risk prediction. IEEE Access. 2021;9:103737–57. https://doi.org/10.1109/ACCESS.2021.309869 .
Omana J, Moorthi M. Predictive analysis and prognostic approach of diabetes prediction with machine learning techniques. Wirel Pers Commun. 2021. https://doi.org/10.1007/s11277-021-08274-w .
Ravaut M, Harish V, Sadeghi H, Leung KK, Volkovs M, Kornas K, Watson T, Poutanen T, Rosella LC. Development and validation of a machine learning model using administrative health data to predict onset of type 2 diabetes. JAMA Netw Open. 2021;4(5):2111315. https://doi.org/10.1001/jamanetworkopen.2021.11315 .
Lang L-Y, Gao Z, Wang X-G, Zhao H, Zhang Y-P, Sun S-J, Zhang Y-J, Austria RS. Diabetes prediction model based on deep belief network. J Comput Methods Sci Eng. 2021;21(4):817–28. https://doi.org/10.3233/JCM-20465 .
Gupta H, Varshney H, Sharma TK, Pachauri N, Verma OP. Comparative performance analysis of quantum machine learning with deep learning for diabetes prediction. Complex Intell Syst. 2021. https://doi.org/10.1007/s40747-021-00398-7 .
Roy K, Ahmad M, Waqar K, Priyaah K, Nebhen J, Alshamrani SS, Raza MA, Ali I. An enhanced machine learning framework for type 2 diabetes classification using imbalanced data with missing values. Complexity. 2021. https://doi.org/10.1155/2021/995331 .
Zhang L, Wang Y, Niu M, Wang C, Wang Z. Nonlaboratory-based risk assessment model for type 2 diabetes mellitus screening in Chinese rural population: a joint bagging-boosting model. IEEE J Biomed Health Inform. 2021;25(10):4005–16. https://doi.org/10.1109/JBHI.2021.307711 .
Turnea M, Ilea M. Predictive simulation for type II diabetes using data mining strategies applied to Big Data. In: Romanian Advanced Distributed Learning Association; Univ Natl Aparare Carol I; European Secur & Def Coll; Romania Partnership Ctr. 14th international scientific conference on eLearning and software for education - eLearning challenges and new horizons, Bucharest, Romania, Apr 19-20, 2018. 2018. p. 481-486. https://doi.org/10.12753/2066-026X-18-213 .
Vettoretti M, Di Camillo B. A variable ranking method for machine learning models with correlated features: in-silico validation and application for diabetes prediction. Appl Sci. 2021;11(16):7740. https://doi.org/10.3390/app11167740 .
Download references
Acknowledgements
We would like to thank Vicerrectoría de Investigación y Posgrado, the Research Group of Product Innovation, and the Cyber Learning and Data Science Laboratory, and the School of Engineering and Science of Tecnologico de Monterrey.
This study was funded by Vicerrectoría de Investigación y Posgrado and the Research Group of Product Innovation of Tecnologico de Monterrey, by a scholarship provided by Tecnologico de Monterrey to graduate student A01339273 Luis Fregoso-Aparicio, and a national scholarship granted by the Consejo Nacional de Ciencia y Tecnologia (CONACYT) to study graduate programs in institutions enrolled in the Padron Nacional de Posgrados de Calidad (PNPC) to CVU 962778 - Luis Fregoso-Aparicio.
Author information
Authors and affiliations.
School of Engineering and Sciences, Tecnologico de Monterrey, Av Lago de Guadalupe KM 3.5, Margarita Maza de Juarez, 52926, Cd Lopez Mateos, Mexico
Luis Fregoso-Aparicio
School of Engineering and Sciences, Tecnologico de Monterrey, Ave. Eugenio Garza Sada 2501, 64849, Monterrey, Nuevo Leon, Mexico
Julieta Noguez & Luis Montesinos
Hospital General de Mexico Dr. Eduardo Liceaga, Dr. Balmis 148, Doctores, Cuauhtemoc, 06720, Mexico City, Mexico
José A. García-García
You can also search for this author in PubMed Google Scholar
Contributions
Individual contributions are the following; conceptualization, methodology, and investigation: LF-A and JN; validation: LM and JAGG; writing—original draft preparation and visualization: LF-A; writing—review and editing: LM and JN; supervision: JAG-G; project administration: JN; and funding acquisition: LF and JN. All authors read and approved the final manuscript.
Corresponding author
Correspondence to Julieta Noguez .
Ethics declarations
Ethics approval and consent to participate.
Not applicable.
Consent for publication
Competing interests.
The authors declare that they have no competing interests.
Additional information
Publisher's note.
Springer Nature remains neutral with regard to jurisdictional claims in published maps and institutional affiliations.
Rights and permissions
Open Access This article is licensed under a Creative Commons Attribution 4.0 International License, which permits use, sharing, adaptation, distribution and reproduction in any medium or format, as long as you give appropriate credit to the original author(s) and the source, provide a link to the Creative Commons licence, and indicate if changes were made. The images or other third party material in this article are included in the article's Creative Commons licence, unless indicated otherwise in a credit line to the material. If material is not included in the article's Creative Commons licence and your intended use is not permitted by statutory regulation or exceeds the permitted use, you will need to obtain permission directly from the copyright holder. To view a copy of this licence, visit http://creativecommons.org/licenses/by/4.0/ . The Creative Commons Public Domain Dedication waiver ( http://creativecommons.org/publicdomain/zero/1.0/ ) applies to the data made available in this article, unless otherwise stated in a credit line to the data.
Reprints and permissions
About this article
Cite this article.
Fregoso-Aparicio, L., Noguez, J., Montesinos, L. et al. Machine learning and deep learning predictive models for type 2 diabetes: a systematic review. Diabetol Metab Syndr 13 , 148 (2021). https://doi.org/10.1186/s13098-021-00767-9
Download citation
Received : 06 July 2021
Accepted : 07 December 2021
Published : 20 December 2021
DOI : https://doi.org/10.1186/s13098-021-00767-9
Share this article
Anyone you share the following link with will be able to read this content:
Sorry, a shareable link is not currently available for this article.
Provided by the Springer Nature SharedIt content-sharing initiative
- Machine learning
- Deep learning
- Electronic health records
Diabetology & Metabolic Syndrome
ISSN: 1758-5996
- Submission enquiries: [email protected]
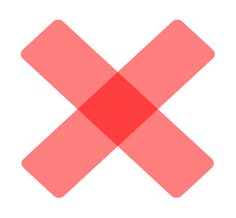
Something went wrong
An error has prevented the portal from working properly.
Please contact us .
You reached this page when trying to access MDI3MDQ5ZTAzYjcxLTcyNDgtZjNlNC03NDRiLWRhYzdkOTUy from 185.66.15.189 on April 28 2024, 13:14:35 UTC
Application of data mining methods in diabetes prediction
Ieee account.
- Change Username/Password
- Update Address
Purchase Details
- Payment Options
- Order History
- View Purchased Documents
Profile Information
- Communications Preferences
- Profession and Education
- Technical Interests
- US & Canada: +1 800 678 4333
- Worldwide: +1 732 981 0060
- Contact & Support
- About IEEE Xplore
- Accessibility
- Terms of Use
- Nondiscrimination Policy
- Privacy & Opting Out of Cookies
A not-for-profit organization, IEEE is the world's largest technical professional organization dedicated to advancing technology for the benefit of humanity. © Copyright 2024 IEEE - All rights reserved. Use of this web site signifies your agreement to the terms and conditions.

IMAGES
VIDEO
COMMENTS
Type 1 and Type 2 diabetes can cause heart disease, renal problems, and eye difficulties. In this paper, we propose a diabetes prediction model using data mining techniques. We apply four data mining techniques such as Random Forest, Support Vector Machine (SVM), Logistic Regression, and Naive Bayes.
In this paper, we present a comprehensive review of the state-of-the-art in the area of diabetes diagnosis and prediction using data mining. The aim of this paper is twofold; firstly, we explore ...
1. Introduction. Diabetes is a major health problem in Saudi Arabia, with the second-highest rate of diabetes in the Middle East and the seventh highest in the world, with an estimated population of 7 million living with diabetes and more than 3 million with pre-diabetes. [] The prevalence of type 2 diabetes in Saudi Arabia is 32.8%; however, it is predicted to reach 35.37% in 2020, 40.37% in ...
In this paper, we present a comprehensive review of the state-of-the-art in the area of diabetes diagnosis and prediction using data mining. The aim of this paper is twofold; firstly, we explore and investigate the data mining based diagnosis and prediction solutions in the field of glycemic control for diabetes. Secondly, in the light of this ...
Background About 90% of patients who have diabetes suffer from Type 2 DM (T2DM). Many studies suggest using the significant role of lncRNAs to improve the diagnosis of T2DM. Machine learning and Data Mining techniques are tools that can improve the analysis and interpretation or extraction of knowledge from the data. These techniques may enhance the prognosis and diagnosis associated with ...
The author hoped to predict the type of diabetes using a dataset containing the required data which would lead to be an added advantage for improving the accuracy. The RF and the J48 algorithm achieved an accuracy of 73.95% and 73.88%, respectively, on the Luzhou dataset and 71.44% and 71.67%, respectively, on the PIMA dataset.
Diabetes is a major concern, that arises with persistently elevated blood sugar levels leading to many health issues such as kidney damage, eyesight loss, and heart diseases, and also acting as a reason for death. These all risks are associated with both type 1 and type 2 diabetes. Data mining techniques will help us in the prediction and classification of diabetes. So, we can take the proper ...
A variety of studies that set out to use data mining for diabetes prediction are discussed here. Diabetes glycaemic management is discussed and studied by the authors of [], who go into the field of data mining to develop diagnostic and prognostic tools.After doing this research, they compare and contrast the many methods often used to diagnose and predict the course of diabetes based on key ...
Two data mining techniques were used to investigate the relationship between anthropometric predictors and binary response variables (diabetic and non-diabetic). So, the main objective of this study was to anticipate diabetes using the LR and DT models and to determine their associated factors, especially anthropometric markers.
The proposed LSTM-based diabetes prediction algorithm is trained with 80% of the data, and the remaining 20% is used for testing. We fine-tuned the prediction model by using a different number of LSTM units in the cell state. This fine-tuning helps to identify more prominent features in the dataset.
Diabetes Prediction Using Data Mining Techniques. Desmond Bala Bisandu 1*, Dorcas Dachollom Datiri, Eva Onokpasa, Godwin Thomas, Musa Maaji Haruna, Aminu. Aliyu, Jerry Zachariah Yakubu. 1,2,3,4,5 ...
Data mining can significantly help diabetes research and ultimately improve the quality of health care (14,15). Data mining methods in disease diagnosis using many complex machine-learning ...
This paper explores the early prediction of diabetes via five different data mining methods including: GMM, SVM, Logistic regression, ELM, ANN, and proves that ANN (Artificial Neural Network) provides the highest accuracy than other techniques. Data science methods have the potential to benefit other scientific fields by shedding new light on common questions. One such task is help to make ...
Diabetes mellitus is fourth most high mortality rate diseases in the world and it is also a cause of kidney disease, blindness, and heart diseases. Data mining techniques support a medical decision for a correct diagnosis, treatment of disease in such way it minimizes the workload of specialists. This study proposed to predict diabetes using data mining techniques. Back propagation algorithm ...
Diabetes Mellitus is a severe, chronic disease that occurs when blood glucose levels rise above certain limits. Over the last years, machine and deep learning techniques have been used to predict diabetes and its complications. However, researchers and developers still face two main challenges when building type 2 diabetes predictive models. First, there is considerable heterogeneity in ...
mining is the upcoming research area to solve various problems and classification is one of main problem in the field of data mining. In this paper, we use two classification algorithms J48 (which ...
integrated data set, and finally proposes an appropriate algorithm that can use the early symptoms of patients to predict diabetes. 2. METHODOLOGY The algorithm process proposed in this paper shown in Figure 1. First, the data set as input to the prediction algorithm, and then, though the evaluation model which is the method of introducing a
Mining the diabetes data in an efficient way is a crucial concern. The data mining techniques and methods will be discovered to find the appropriate approaches and techniques for efficient classification of Diabetes dataset and in extracting valuable patterns. In this study, medical bioinformatics analyses have been accomplished to predict ...
Data science methods have the potential to benefit other scientific fields by shedding new light on common questions. One such task is help to make predictions on medical data. Diabetes mellitus or simply diabetes is a disease caused due to the increase level of blood glucose. Various traditional methods, based on physical and chemical tests, are available for diagnosing diabetes. The methods ...
application was developed by using the data mining algorithm and they used the decision tree classifier to predict diabetes for the patient. The app also provides information about diabetes. The app uses the PIMA dataset for analysis of diabetes and adds a risk analysis feature to detect the level of diabetes.
The main aim of this paper is to provide brief prediction on both diabetes and liver disease and the goal is to provide a better final results when compared to the other algorithms, for the disease prediction. The growing occurrence of persistent liver disorder has been cited in current times. Similarly Diabetes, one of the frequently spreading disease is growing hastily in all of the Nations ...