REVIEW article
Environmental and health impacts of air pollution: a review.
- 1 Delphis S.A., Kifisia, Greece
- 2 Laboratory of Hygiene and Environmental Protection, Faculty of Medicine, Democritus University of Thrace, Alexandroupolis, Greece
- 3 Centre Hospitalier Universitaire Vaudois (CHUV), Service de Médicine Interne, Lausanne, Switzerland
- 4 School of Social and Political Sciences, University of Glasgow, Glasgow, United Kingdom
One of our era's greatest scourges is air pollution, on account not only of its impact on climate change but also its impact on public and individual health due to increasing morbidity and mortality. There are many pollutants that are major factors in disease in humans. Among them, Particulate Matter (PM), particles of variable but very small diameter, penetrate the respiratory system via inhalation, causing respiratory and cardiovascular diseases, reproductive and central nervous system dysfunctions, and cancer. Despite the fact that ozone in the stratosphere plays a protective role against ultraviolet irradiation, it is harmful when in high concentration at ground level, also affecting the respiratory and cardiovascular system. Furthermore, nitrogen oxide, sulfur dioxide, Volatile Organic Compounds (VOCs), dioxins, and polycyclic aromatic hydrocarbons (PAHs) are all considered air pollutants that are harmful to humans. Carbon monoxide can even provoke direct poisoning when breathed in at high levels. Heavy metals such as lead, when absorbed into the human body, can lead to direct poisoning or chronic intoxication, depending on exposure. Diseases occurring from the aforementioned substances include principally respiratory problems such as Chronic Obstructive Pulmonary Disease (COPD), asthma, bronchiolitis, and also lung cancer, cardiovascular events, central nervous system dysfunctions, and cutaneous diseases. Last but not least, climate change resulting from environmental pollution affects the geographical distribution of many infectious diseases, as do natural disasters. The only way to tackle this problem is through public awareness coupled with a multidisciplinary approach by scientific experts; national and international organizations must address the emergence of this threat and propose sustainable solutions.

Approach to the Problem
The interactions between humans and their physical surroundings have been extensively studied, as multiple human activities influence the environment. The environment is a coupling of the biotic (living organisms and microorganisms) and the abiotic (hydrosphere, lithosphere, and atmosphere).
Pollution is defined as the introduction into the environment of substances harmful to humans and other living organisms. Pollutants are harmful solids, liquids, or gases produced in higher than usual concentrations that reduce the quality of our environment.
Human activities have an adverse effect on the environment by polluting the water we drink, the air we breathe, and the soil in which plants grow. Although the industrial revolution was a great success in terms of technology, society, and the provision of multiple services, it also introduced the production of huge quantities of pollutants emitted into the air that are harmful to human health. Without any doubt, the global environmental pollution is considered an international public health issue with multiple facets. Social, economic, and legislative concerns and lifestyle habits are related to this major problem. Clearly, urbanization and industrialization are reaching unprecedented and upsetting proportions worldwide in our era. Anthropogenic air pollution is one of the biggest public health hazards worldwide, given that it accounts for about 9 million deaths per year ( 1 ).
Without a doubt, all of the aforementioned are closely associated with climate change, and in the event of danger, the consequences can be severe for mankind ( 2 ). Climate changes and the effects of global planetary warming seriously affect multiple ecosystems, causing problems such as food safety issues, ice and iceberg melting, animal extinction, and damage to plants ( 3 , 4 ).
Air pollution has various health effects. The health of susceptible and sensitive individuals can be impacted even on low air pollution days. Short-term exposure to air pollutants is closely related to COPD (Chronic Obstructive Pulmonary Disease), cough, shortness of breath, wheezing, asthma, respiratory disease, and high rates of hospitalization (a measurement of morbidity).
The long-term effects associated with air pollution are chronic asthma, pulmonary insufficiency, cardiovascular diseases, and cardiovascular mortality. According to a Swedish cohort study, diabetes seems to be induced after long-term air pollution exposure ( 5 ). Moreover, air pollution seems to have various malign health effects in early human life, such as respiratory, cardiovascular, mental, and perinatal disorders ( 3 ), leading to infant mortality or chronic disease in adult age ( 6 ).
National reports have mentioned the increased risk of morbidity and mortality ( 1 ). These studies were conducted in many places around the world and show a correlation between daily ranges of particulate matter (PM) concentration and daily mortality. Climate shifts and global planetary warming ( 3 ) could aggravate the situation. Besides, increased hospitalization (an index of morbidity) has been registered among the elderly and susceptible individuals for specific reasons. Fine and ultrafine particulate matter seems to be associated with more serious illnesses ( 6 ), as it can invade the deepest parts of the airways and more easily reach the bloodstream.
Air pollution mainly affects those living in large urban areas, where road emissions contribute the most to the degradation of air quality. There is also a danger of industrial accidents, where the spread of a toxic fog can be fatal to the populations of the surrounding areas. The dispersion of pollutants is determined by many parameters, most notably atmospheric stability and wind ( 6 ).
In developing countries ( 7 ), the problem is more serious due to overpopulation and uncontrolled urbanization along with the development of industrialization. This leads to poor air quality, especially in countries with social disparities and a lack of information on sustainable management of the environment. The use of fuels such as wood fuel or solid fuel for domestic needs due to low incomes exposes people to bad-quality, polluted air at home. It is of note that three billion people around the world are using the above sources of energy for their daily heating and cooking needs ( 8 ). In developing countries, the women of the household seem to carry the highest risk for disease development due to their longer duration exposure to the indoor air pollution ( 8 , 9 ). Due to its fast industrial development and overpopulation, China is one of the Asian countries confronting serious air pollution problems ( 10 , 11 ). The lung cancer mortality observed in China is associated with fine particles ( 12 ). As stated already, long-term exposure is associated with deleterious effects on the cardiovascular system ( 3 , 5 ). However, it is interesting to note that cardiovascular diseases have mostly been observed in developed and high-income countries rather than in the developing low-income countries exposed highly to air pollution ( 13 ). Extreme air pollution is recorded in India, where the air quality reaches hazardous levels. New Delhi is one of the more polluted cities in India. Flights in and out of New Delhi International Airport are often canceled due to the reduced visibility associated with air pollution. Pollution is occurring both in urban and rural areas in India due to the fast industrialization, urbanization, and rise in use of motorcycle transportation. Nevertheless, biomass combustion associated with heating and cooking needs and practices is a major source of household air pollution in India and in Nepal ( 14 , 15 ). There is spatial heterogeneity in India, as areas with diverse climatological conditions and population and education levels generate different indoor air qualities, with higher PM 2.5 observed in North Indian states (557–601 μg/m 3 ) compared to the Southern States (183–214 μg/m 3 ) ( 16 , 17 ). The cold climate of the North Indian areas may be the main reason for this, as longer periods at home and more heating are necessary compared to in the tropical climate of Southern India. Household air pollution in India is associated with major health effects, especially in women and young children, who stay indoors for longer periods. Chronic obstructive respiratory disease (CORD) and lung cancer are mostly observed in women, while acute lower respiratory disease is seen in young children under 5 years of age ( 18 ).
Accumulation of air pollution, especially sulfur dioxide and smoke, reaching 1,500 mg/m3, resulted in an increase in the number of deaths (4,000 deaths) in December 1952 in London and in 1963 in New York City (400 deaths) ( 19 ). An association of pollution with mortality was reported on the basis of monitoring of outdoor pollution in six US metropolitan cities ( 20 ). In every case, it seems that mortality was closely related to the levels of fine, inhalable, and sulfate particles more than with the levels of total particulate pollution, aerosol acidity, sulfur dioxide, or nitrogen dioxide ( 20 ).
Furthermore, extremely high levels of pollution are reported in Mexico City and Rio de Janeiro, followed by Milan, Ankara, Melbourne, Tokyo, and Moscow ( 19 ).
Based on the magnitude of the public health impact, it is certain that different kinds of interventions should be taken into account. Success and effectiveness in controlling air pollution, specifically at the local level, have been reported. Adequate technological means are applied considering the source and the nature of the emission as well as its impact on health and the environment. The importance of point sources and non-point sources of air pollution control is reported by Schwela and Köth-Jahr ( 21 ). Without a doubt, a detailed emission inventory must record all sources in a given area. Beyond considering the above sources and their nature, topography and meteorology should also be considered, as stated previously. Assessment of the control policies and methods is often extrapolated from the local to the regional and then to the global scale. Air pollution may be dispersed and transported from one region to another area located far away. Air pollution management means the reduction to acceptable levels or possible elimination of air pollutants whose presence in the air affects our health or the environmental ecosystem. Private and governmental entities and authorities implement actions to ensure the air quality ( 22 ). Air quality standards and guidelines were adopted for the different pollutants by the WHO and EPA as a tool for the management of air quality ( 1 , 23 ). These standards have to be compared to the emissions inventory standards by causal analysis and dispersion modeling in order to reveal the problematic areas ( 24 ). Inventories are generally based on a combination of direct measurements and emissions modeling ( 24 ).
As an example, we state here the control measures at the source through the use of catalytic converters in cars. These are devices that turn the pollutants and toxic gases produced from combustion engines into less-toxic pollutants by catalysis through redox reactions ( 25 ). In Greece, the use of private cars was restricted by tracking their license plates in order to reduce traffic congestion during rush hour ( 25 ).
Concerning industrial emissions, collectors and closed systems can keep the air pollution to the minimal standards imposed by legislation ( 26 ).
Current strategies to improve air quality require an estimation of the economic value of the benefits gained from proposed programs. These proposed programs by public authorities, and directives are issued with guidelines to be respected.
In Europe, air quality limit values AQLVs (Air Quality Limit Values) are issued for setting off planning claims ( 27 ). In the USA, the NAAQS (National Ambient Air Quality Standards) establish the national air quality limit values ( 27 ). While both standards and directives are based on different mechanisms, significant success has been achieved in the reduction of overall emissions and associated health and environmental effects ( 27 ). The European Directive identifies geographical areas of risk exposure as monitoring/assessment zones to record the emission sources and levels of air pollution ( 27 ), whereas the USA establishes global geographical air quality criteria according to the severity of their air quality problem and records all sources of the pollutants and their precursors ( 27 ).
In this vein, funds have been financing, directly or indirectly, projects related to air quality along with the technical infrastructure to maintain good air quality. These plans focus on an inventory of databases from air quality environmental planning awareness campaigns. Moreover, pollution measures of air emissions may be taken for vehicles, machines, and industries in urban areas.
Technological innovation can only be successful if it is able to meet the needs of society. In this sense, technology must reflect the decision-making practices and procedures of those involved in risk assessment and evaluation and act as a facilitator in providing information and assessments to enable decision makers to make the best decisions possible. Summarizing the aforementioned in order to design an effective air quality control strategy, several aspects must be considered: environmental factors and ambient air quality conditions, engineering factors and air pollutant characteristics, and finally, economic operating costs for technological improvement and administrative and legal costs. Considering the economic factor, competitiveness through neoliberal concepts is offering a solution to environmental problems ( 22 ).
The development of environmental governance, along with technological progress, has initiated the deployment of a dialogue. Environmental politics has created objections and points of opposition between different political parties, scientists, media, and governmental and non-governmental organizations ( 22 ). Radical environmental activism actions and movements have been created ( 22 ). The rise of the new information and communication technologies (ICTs) are many times examined as to whether and in which way they have influenced means of communication and social movements such as activism ( 28 ). Since the 1990s, the term “digital activism” has been used increasingly and in many different disciplines ( 29 ). Nowadays, multiple digital technologies can be used to produce a digital activism outcome on environmental issues. More specifically, devices with online capabilities such as computers or mobile phones are being used as a way to pursue change in political and social affairs ( 30 ).
In the present paper, we focus on the sources of environmental pollution in relation to public health and propose some solutions and interventions that may be of interest to environmental legislators and decision makers.
Sources of Exposure
It is known that the majority of environmental pollutants are emitted through large-scale human activities such as the use of industrial machinery, power-producing stations, combustion engines, and cars. Because these activities are performed at such a large scale, they are by far the major contributors to air pollution, with cars estimated to be responsible for approximately 80% of today's pollution ( 31 ). Some other human activities are also influencing our environment to a lesser extent, such as field cultivation techniques, gas stations, fuel tanks heaters, and cleaning procedures ( 32 ), as well as several natural sources, such as volcanic and soil eruptions and forest fires.
The classification of air pollutants is based mainly on the sources producing pollution. Therefore, it is worth mentioning the four main sources, following the classification system: Major sources, Area sources, Mobile sources, and Natural sources.
Major sources include the emission of pollutants from power stations, refineries, and petrochemicals, the chemical and fertilizer industries, metallurgical and other industrial plants, and, finally, municipal incineration.
Indoor area sources include domestic cleaning activities, dry cleaners, printing shops, and petrol stations.
Mobile sources include automobiles, cars, railways, airways, and other types of vehicles.
Finally, natural sources include, as stated previously, physical disasters ( 33 ) such as forest fire, volcanic erosion, dust storms, and agricultural burning.
However, many classification systems have been proposed. Another type of classification is a grouping according to the recipient of the pollution, as follows:
Air pollution is determined as the presence of pollutants in the air in large quantities for long periods. Air pollutants are dispersed particles, hydrocarbons, CO, CO 2 , NO, NO 2 , SO 3 , etc.
Water pollution is organic and inorganic charge and biological charge ( 10 ) at high levels that affect the water quality ( 34 , 35 ).
Soil pollution occurs through the release of chemicals or the disposal of wastes, such as heavy metals, hydrocarbons, and pesticides.
Air pollution can influence the quality of soil and water bodies by polluting precipitation, falling into water and soil environments ( 34 , 36 ). Notably, the chemistry of the soil can be amended due to acid precipitation by affecting plants, cultures, and water quality ( 37 ). Moreover, movement of heavy metals is favored by soil acidity, and metals are so then moving into the watery environment. It is known that heavy metals such as aluminum are noxious to wildlife and fishes. Soil quality seems to be of importance, as soils with low calcium carbonate levels are at increased jeopardy from acid rain. Over and above rain, snow and particulate matter drip into watery ' bodies ( 36 , 38 ).
Lastly, pollution is classified following type of origin:
Radioactive and nuclear pollution , releasing radioactive and nuclear pollutants into water, air, and soil during nuclear explosions and accidents, from nuclear weapons, and through handling or disposal of radioactive sewage.
Radioactive materials can contaminate surface water bodies and, being noxious to the environment, plants, animals, and humans. It is known that several radioactive substances such as radium and uranium concentrate in the bones and can cause cancers ( 38 , 39 ).
Noise pollution is produced by machines, vehicles, traffic noises, and musical installations that are harmful to our hearing.
The World Health Organization introduced the term DALYs. The DALYs for a disease or health condition is defined as the sum of the Years of Life Lost (YLL) due to premature mortality in the population and the Years Lost due to Disability (YLD) for people living with the health condition or its consequences ( 39 ). In Europe, air pollution is the main cause of disability-adjusted life years lost (DALYs), followed by noise pollution. The potential relationships of noise and air pollution with health have been studied ( 40 ). The study found that DALYs related to noise were more important than those related to air pollution, as the effects of environmental noise on cardiovascular disease were independent of air pollution ( 40 ). Environmental noise should be counted as an independent public health risk ( 40 ).
Environmental pollution occurs when changes in the physical, chemical, or biological constituents of the environment (air masses, temperature, climate, etc.) are produced.
Pollutants harm our environment either by increasing levels above normal or by introducing harmful toxic substances. Primary pollutants are directly produced from the above sources, and secondary pollutants are emitted as by-products of the primary ones. Pollutants can be biodegradable or non-biodegradable and of natural origin or anthropogenic, as stated previously. Moreover, their origin can be a unique source (point-source) or dispersed sources.
Pollutants have differences in physical and chemical properties, explaining the discrepancy in their capacity for producing toxic effects. As an example, we state here that aerosol compounds ( 41 – 43 ) have a greater toxicity than gaseous compounds due to their tiny size (solid or liquid) in the atmosphere; they have a greater penetration capacity. Gaseous compounds are eliminated more easily by our respiratory system ( 41 ). These particles are able to damage lungs and can even enter the bloodstream ( 41 ), leading to the premature deaths of millions of people yearly. Moreover, the aerosol acidity ([H+]) seems to considerably enhance the production of secondary organic aerosols (SOA), but this last aspect is not supported by other scientific teams ( 38 ).
Climate and Pollution
Air pollution and climate change are closely related. Climate is the other side of the same coin that reduces the quality of our Earth ( 44 ). Pollutants such as black carbon, methane, tropospheric ozone, and aerosols affect the amount of incoming sunlight. As a result, the temperature of the Earth is increasing, resulting in the melting of ice, icebergs, and glaciers.
In this vein, climatic changes will affect the incidence and prevalence of both residual and imported infections in Europe. Climate and weather affect the duration, timing, and intensity of outbreaks strongly and change the map of infectious diseases in the globe ( 45 ). Mosquito-transmitted parasitic or viral diseases are extremely climate-sensitive, as warming firstly shortens the pathogen incubation period and secondly shifts the geographic map of the vector. Similarly, water-warming following climate changes leads to a high incidence of waterborne infections. Recently, in Europe, eradicated diseases seem to be emerging due to the migration of population, for example, cholera, poliomyelitis, tick-borne encephalitis, and malaria ( 46 ).
The spread of epidemics is associated with natural climate disasters and storms, which seem to occur more frequently nowadays ( 47 ). Malnutrition and disequilibration of the immune system are also associated with the emerging infections affecting public health ( 48 ).
The Chikungunya virus “took the airplane” from the Indian Ocean to Europe, as outbreaks of the disease were registered in Italy ( 49 ) as well as autochthonous cases in France ( 50 ).
An increase in cryptosporidiosis in the United Kingdom and in the Czech Republic seems to have occurred following flooding ( 36 , 51 ).
As stated previously, aerosols compounds are tiny in size and considerably affect the climate. They are able to dissipate sunlight (the albedo phenomenon) by dispersing a quarter of the sun's rays back to space and have cooled the global temperature over the last 30 years ( 52 ).
Air Pollutants
The World Health Organization (WHO) reports on six major air pollutants, namely particle pollution, ground-level ozone, carbon monoxide, sulfur oxides, nitrogen oxides, and lead. Air pollution can have a disastrous effect on all components of the environment, including groundwater, soil, and air. Additionally, it poses a serious threat to living organisms. In this vein, our interest is mainly to focus on these pollutants, as they are related to more extensive and severe problems in human health and environmental impact. Acid rain, global warming, the greenhouse effect, and climate changes have an important ecological impact on air pollution ( 53 ).
Particulate Matter (PM) and Health
Studies have shown a relationship between particulate matter (PM) and adverse health effects, focusing on either short-term (acute) or long-term (chronic) PM exposure.
Particulate matter (PM) is usually formed in the atmosphere as a result of chemical reactions between the different pollutants. The penetration of particles is closely dependent on their size ( 53 ). Particulate Matter (PM) was defined as a term for particles by the United States Environmental Protection Agency ( 54 ). Particulate matter (PM) pollution includes particles with diameters of 10 micrometers (μm) or smaller, called PM 10 , and extremely fine particles with diameters that are generally 2.5 micrometers (μm) and smaller.
Particulate matter contains tiny liquid or solid droplets that can be inhaled and cause serious health effects ( 55 ). Particles <10 μm in diameter (PM 10 ) after inhalation can invade the lungs and even reach the bloodstream. Fine particles, PM 2.5 , pose a greater risk to health ( 6 , 56 ) ( Table 1 ).
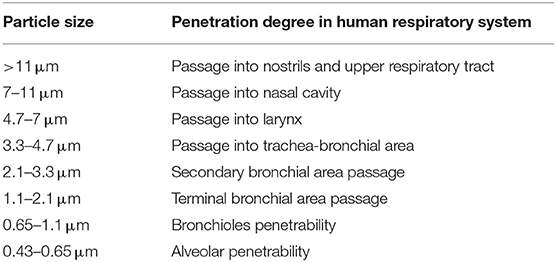
Table 1 . Penetrability according to particle size.
Multiple epidemiological studies have been performed on the health effects of PM. A positive relation was shown between both short-term and long-term exposures of PM 2.5 and acute nasopharyngitis ( 56 ). In addition, long-term exposure to PM for years was found to be related to cardiovascular diseases and infant mortality.
Those studies depend on PM 2.5 monitors and are restricted in terms of study area or city area due to a lack of spatially resolved daily PM 2.5 concentration data and, in this way, are not representative of the entire population. Following a recent epidemiological study by the Department of Environmental Health at Harvard School of Public Health (Boston, MA) ( 57 ), it was reported that, as PM 2.5 concentrations vary spatially, an exposure error (Berkson error) seems to be produced, and the relative magnitudes of the short- and long-term effects are not yet completely elucidated. The team developed a PM 2.5 exposure model based on remote sensing data for assessing short- and long-term human exposures ( 57 ). This model permits spatial resolution in short-term effects plus the assessment of long-term effects in the whole population.
Moreover, respiratory diseases and affection of the immune system are registered as long-term chronic effects ( 58 ). It is worth noting that people with asthma, pneumonia, diabetes, and respiratory and cardiovascular diseases are especially susceptible and vulnerable to the effects of PM. PM 2.5 , followed by PM 10 , are strongly associated with diverse respiratory system diseases ( 59 ), as their size permits them to pierce interior spaces ( 60 ). The particles produce toxic effects according to their chemical and physical properties. The components of PM 10 and PM 2.5 can be organic (polycyclic aromatic hydrocarbons, dioxins, benzene, 1-3 butadiene) or inorganic (carbon, chlorides, nitrates, sulfates, metals) in nature ( 55 ).
Particulate Matter (PM) is divided into four main categories according to type and size ( 61 ) ( Table 2 ).
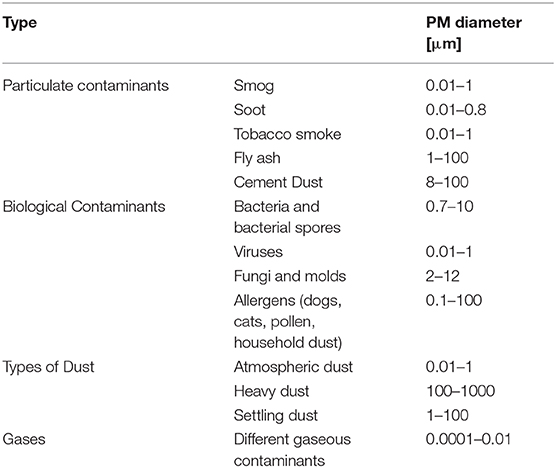
Table 2 . Types and sizes of particulate Matter (PM).
Gas contaminants include PM in aerial masses.
Particulate contaminants include contaminants such as smog, soot, tobacco smoke, oil smoke, fly ash, and cement dust.
Biological Contaminants are microorganisms (bacteria, viruses, fungi, mold, and bacterial spores), cat allergens, house dust and allergens, and pollen.
Types of Dust include suspended atmospheric dust, settling dust, and heavy dust.
Finally, another fact is that the half-lives of PM 10 and PM 2.5 particles in the atmosphere is extended due to their tiny dimensions; this permits their long-lasting suspension in the atmosphere and even their transfer and spread to distant destinations where people and the environment may be exposed to the same magnitude of pollution ( 53 ). They are able to change the nutrient balance in watery ecosystems, damage forests and crops, and acidify water bodies.
As stated, PM 2.5 , due to their tiny size, are causing more serious health effects. These aforementioned fine particles are the main cause of the “haze” formation in different metropolitan areas ( 12 , 13 , 61 ).
Ozone Impact in the Atmosphere
Ozone (O 3 ) is a gas formed from oxygen under high voltage electric discharge ( 62 ). It is a strong oxidant, 52% stronger than chlorine. It arises in the stratosphere, but it could also arise following chain reactions of photochemical smog in the troposphere ( 63 ).
Ozone can travel to distant areas from its initial source, moving with air masses ( 64 ). It is surprising that ozone levels over cities are low in contrast to the increased amounts occuring in urban areas, which could become harmful for cultures, forests, and vegetation ( 65 ) as it is reducing carbon assimilation ( 66 ). Ozone reduces growth and yield ( 47 , 48 ) and affects the plant microflora due to its antimicrobial capacity ( 67 , 68 ). In this regard, ozone acts upon other natural ecosystems, with microflora ( 69 , 70 ) and animal species changing their species composition ( 71 ). Ozone increases DNA damage in epidermal keratinocytes and leads to impaired cellular function ( 72 ).
Ground-level ozone (GLO) is generated through a chemical reaction between oxides of nitrogen and VOCs emitted from natural sources and/or following anthropogenic activities.
Ozone uptake usually occurs by inhalation. Ozone affects the upper layers of the skin and the tear ducts ( 73 ). A study of short-term exposure of mice to high levels of ozone showed malondialdehyde formation in the upper skin (epidermis) but also depletion in vitamins C and E. It is likely that ozone levels are not interfering with the skin barrier function and integrity to predispose to skin disease ( 74 ).
Due to the low water-solubility of ozone, inhaled ozone has the capacity to penetrate deeply into the lungs ( 75 ).
Toxic effects induced by ozone are registered in urban areas all over the world, causing biochemical, morphologic, functional, and immunological disorders ( 76 ).
The European project (APHEA2) focuses on the acute effects of ambient ozone concentrations on mortality ( 77 ). Daily ozone concentrations compared to the daily number of deaths were reported from different European cities for a 3-year period. During the warm period of the year, an observed increase in ozone concentration was associated with an increase in the daily number of deaths (0.33%), in the number of respiratory deaths (1.13%), and in the number of cardiovascular deaths (0.45%). No effect was observed during wintertime.
Carbon Monoxide (CO)
Carbon monoxide is produced by fossil fuel when combustion is incomplete. The symptoms of poisoning due to inhaling carbon monoxide include headache, dizziness, weakness, nausea, vomiting, and, finally, loss of consciousness.
The affinity of carbon monoxide to hemoglobin is much greater than that of oxygen. In this vein, serious poisoning may occur in people exposed to high levels of carbon monoxide for a long period of time. Due to the loss of oxygen as a result of the competitive binding of carbon monoxide, hypoxia, ischemia, and cardiovascular disease are observed.
Carbon monoxide affects the greenhouses gases that are tightly connected to global warming and climate. This should lead to an increase in soil and water temperatures, and extreme weather conditions or storms may occur ( 68 ).
However, in laboratory and field experiments, it has been seen to produce increased plant growth ( 78 ).
Nitrogen Oxide (NO 2 )
Nitrogen oxide is a traffic-related pollutant, as it is emitted from automobile motor engines ( 79 , 80 ). It is an irritant of the respiratory system as it penetrates deep in the lung, inducing respiratory diseases, coughing, wheezing, dyspnea, bronchospasm, and even pulmonary edema when inhaled at high levels. It seems that concentrations over 0.2 ppm produce these adverse effects in humans, while concentrations higher than 2.0 ppm affect T-lymphocytes, particularly the CD8+ cells and NK cells that produce our immune response ( 81 ).It is reported that long-term exposure to high levels of nitrogen dioxide can be responsible for chronic lung disease. Long-term exposure to NO 2 can impair the sense of smell ( 81 ).
However, systems other than respiratory ones can be involved, as symptoms such as eye, throat, and nose irritation have been registered ( 81 ).
High levels of nitrogen dioxide are deleterious to crops and vegetation, as they have been observed to reduce crop yield and plant growth efficiency. Moreover, NO 2 can reduce visibility and discolor fabrics ( 81 ).
Sulfur Dioxide (SO 2 )
Sulfur dioxide is a harmful gas that is emitted mainly from fossil fuel consumption or industrial activities. The annual standard for SO 2 is 0.03 ppm ( 82 ). It affects human, animal, and plant life. Susceptible people as those with lung disease, old people, and children, who present a higher risk of damage. The major health problems associated with sulfur dioxide emissions in industrialized areas are respiratory irritation, bronchitis, mucus production, and bronchospasm, as it is a sensory irritant and penetrates deep into the lung converted into bisulfite and interacting with sensory receptors, causing bronchoconstriction. Moreover, skin redness, damage to the eyes (lacrimation and corneal opacity) and mucous membranes, and worsening of pre-existing cardiovascular disease have been observed ( 81 ).
Environmental adverse effects, such as acidification of soil and acid rain, seem to be associated with sulfur dioxide emissions ( 83 ).
Lead is a heavy metal used in different industrial plants and emitted from some petrol motor engines, batteries, radiators, waste incinerators, and waste waters ( 84 ).
Moreover, major sources of lead pollution in the air are metals, ore, and piston-engine aircraft. Lead poisoning is a threat to public health due to its deleterious effects upon humans, animals, and the environment, especially in the developing countries.
Exposure to lead can occur through inhalation, ingestion, and dermal absorption. Trans- placental transport of lead was also reported, as lead passes through the placenta unencumbered ( 85 ). The younger the fetus is, the more harmful the toxic effects. Lead toxicity affects the fetal nervous system; edema or swelling of the brain is observed ( 86 ). Lead, when inhaled, accumulates in the blood, soft tissue, liver, lung, bones, and cardiovascular, nervous, and reproductive systems. Moreover, loss of concentration and memory, as well as muscle and joint pain, were observed in adults ( 85 , 86 ).
Children and newborns ( 87 ) are extremely susceptible even to minimal doses of lead, as it is a neurotoxicant and causes learning disabilities, impairment of memory, hyperactivity, and even mental retardation.
Elevated amounts of lead in the environment are harmful to plants and crop growth. Neurological effects are observed in vertebrates and animals in association with high lead levels ( 88 ).
Polycyclic Aromatic Hydrocarbons(PAHs)
The distribution of PAHs is ubiquitous in the environment, as the atmosphere is the most important means of their dispersal. They are found in coal and in tar sediments. Moreover, they are generated through incomplete combustion of organic matter as in the cases of forest fires, incineration, and engines ( 89 ). PAH compounds, such as benzopyrene, acenaphthylene, anthracene, and fluoranthene are recognized as toxic, mutagenic, and carcinogenic substances. They are an important risk factor for lung cancer ( 89 ).
Volatile Organic Compounds(VOCs)
Volatile organic compounds (VOCs), such as toluene, benzene, ethylbenzene, and xylene ( 90 ), have been found to be associated with cancer in humans ( 91 ). The use of new products and materials has actually resulted in increased concentrations of VOCs. VOCs pollute indoor air ( 90 ) and may have adverse effects on human health ( 91 ). Short-term and long-term adverse effects on human health are observed. VOCs are responsible for indoor air smells. Short-term exposure is found to cause irritation of eyes, nose, throat, and mucosal membranes, while those of long duration exposure include toxic reactions ( 92 ). Predictable assessment of the toxic effects of complex VOC mixtures is difficult to estimate, as these pollutants can have synergic, antagonistic, or indifferent effects ( 91 , 93 ).
Dioxins originate from industrial processes but also come from natural processes, such as forest fires and volcanic eruptions. They accumulate in foods such as meat and dairy products, fish and shellfish, and especially in the fatty tissue of animals ( 94 ).
Short-period exhibition to high dioxin concentrations may result in dark spots and lesions on the skin ( 94 ). Long-term exposure to dioxins can cause developmental problems, impairment of the immune, endocrine and nervous systems, reproductive infertility, and cancer ( 94 ).
Without any doubt, fossil fuel consumption is responsible for a sizeable part of air contamination. This contamination may be anthropogenic, as in agricultural and industrial processes or transportation, while contamination from natural sources is also possible. Interestingly, it is of note that the air quality standards established through the European Air Quality Directive are somewhat looser than the WHO guidelines, which are stricter ( 95 ).
Effect of Air Pollution on Health
The most common air pollutants are ground-level ozone and Particulates Matter (PM). Air pollution is distinguished into two main types:
Outdoor pollution is the ambient air pollution.
Indoor pollution is the pollution generated by household combustion of fuels.
People exposed to high concentrations of air pollutants experience disease symptoms and states of greater and lesser seriousness. These effects are grouped into short- and long-term effects affecting health.
Susceptible populations that need to be aware of health protection measures include old people, children, and people with diabetes and predisposing heart or lung disease, especially asthma.
As extensively stated previously, according to a recent epidemiological study from Harvard School of Public Health, the relative magnitudes of the short- and long-term effects have not been completely clarified ( 57 ) due to the different epidemiological methodologies and to the exposure errors. New models are proposed for assessing short- and long-term human exposure data more successfully ( 57 ). Thus, in the present section, we report the more common short- and long-term health effects but also general concerns for both types of effects, as these effects are often dependent on environmental conditions, dose, and individual susceptibility.
Short-term effects are temporary and range from simple discomfort, such as irritation of the eyes, nose, skin, throat, wheezing, coughing and chest tightness, and breathing difficulties, to more serious states, such as asthma, pneumonia, bronchitis, and lung and heart problems. Short-term exposure to air pollution can also cause headaches, nausea, and dizziness.
These problems can be aggravated by extended long-term exposure to the pollutants, which is harmful to the neurological, reproductive, and respiratory systems and causes cancer and even, rarely, deaths.
The long-term effects are chronic, lasting for years or the whole life and can even lead to death. Furthermore, the toxicity of several air pollutants may also induce a variety of cancers in the long term ( 96 ).
As stated already, respiratory disorders are closely associated with the inhalation of air pollutants. These pollutants will invade through the airways and will accumulate at the cells. Damage to target cells should be related to the pollutant component involved and its source and dose. Health effects are also closely dependent on country, area, season, and time. An extended exposure duration to the pollutant should incline to long-term health effects in relation also to the above factors.
Particulate Matter (PMs), dust, benzene, and O 3 cause serious damage to the respiratory system ( 97 ). Moreover, there is a supplementary risk in case of existing respiratory disease such as asthma ( 98 ). Long-term effects are more frequent in people with a predisposing disease state. When the trachea is contaminated by pollutants, voice alterations may be remarked after acute exposure. Chronic obstructive pulmonary disease (COPD) may be induced following air pollution, increasing morbidity and mortality ( 99 ). Long-term effects from traffic, industrial air pollution, and combustion of fuels are the major factors for COPD risk ( 99 ).
Multiple cardiovascular effects have been observed after exposure to air pollutants ( 100 ). Changes occurred in blood cells after long-term exposure may affect cardiac functionality. Coronary arteriosclerosis was reported following long-term exposure to traffic emissions ( 101 ), while short-term exposure is related to hypertension, stroke, myocardial infracts, and heart insufficiency. Ventricle hypertrophy is reported to occur in humans after long-time exposure to nitrogen oxide (NO 2 ) ( 102 , 103 ).
Neurological effects have been observed in adults and children after extended-term exposure to air pollutants.
Psychological complications, autism, retinopathy, fetal growth, and low birth weight seem to be related to long-term air pollution ( 83 ). The etiologic agent of the neurodegenerative diseases (Alzheimer's and Parkinson's) is not yet known, although it is believed that extended exposure to air pollution seems to be a factor. Specifically, pesticides and metals are cited as etiological factors, together with diet. The mechanisms in the development of neurodegenerative disease include oxidative stress, protein aggregation, inflammation, and mitochondrial impairment in neurons ( 104 ) ( Figure 1 ).
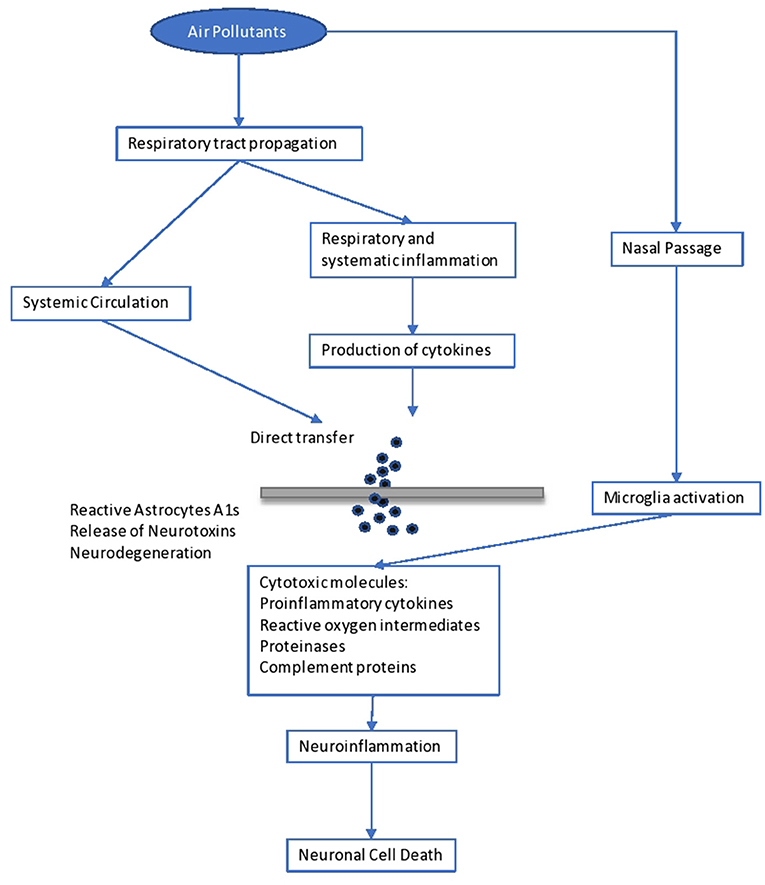
Figure 1 . Impact of air pollutants on the brain.
Brain inflammation was observed in dogs living in a highly polluted area in Mexico for a long period ( 105 ). In human adults, markers of systemic inflammation (IL-6 and fibrinogen) were found to be increased as an immediate response to PNC on the IL-6 level, possibly leading to the production of acute-phase proteins ( 106 ). The progression of atherosclerosis and oxidative stress seem to be the mechanisms involved in the neurological disturbances caused by long-term air pollution. Inflammation comes secondary to the oxidative stress and seems to be involved in the impairment of developmental maturation, affecting multiple organs ( 105 , 107 ). Similarly, other factors seem to be involved in the developmental maturation, which define the vulnerability to long-term air pollution. These include birthweight, maternal smoking, genetic background and socioeconomic environment, as well as education level.
However, diet, starting from breast-feeding, is another determinant factor. Diet is the main source of antioxidants, which play a key role in our protection against air pollutants ( 108 ). Antioxidants are free radical scavengers and limit the interaction of free radicals in the brain ( 108 ). Similarly, genetic background may result in a differential susceptibility toward the oxidative stress pathway ( 60 ). For example, antioxidant supplementation with vitamins C and E appears to modulate the effect of ozone in asthmatic children homozygous for the GSTM1 null allele ( 61 ). Inflammatory cytokines released in the periphery (e.g., respiratory epithelia) upregulate the innate immune Toll-like receptor 2. Such activation and the subsequent events leading to neurodegeneration have recently been observed in lung lavage in mice exposed to ambient Los Angeles (CA, USA) particulate matter ( 61 ). In children, neurodevelopmental morbidities were observed after lead exposure. These children developed aggressive and delinquent behavior, reduced intelligence, learning difficulties, and hyperactivity ( 109 ). No level of lead exposure seems to be “safe,” and the scientific community has asked the Centers for Disease Control and Prevention (CDC) to reduce the current screening guideline of 10 μg/dl ( 109 ).
It is important to state that impact on the immune system, causing dysfunction and neuroinflammation ( 104 ), is related to poor air quality. Yet, increases in serum levels of immunoglobulins (IgA, IgM) and the complement component C3 are observed ( 106 ). Another issue is that antigen presentation is affected by air pollutants, as there is an upregulation of costimulatory molecules such as CD80 and CD86 on macrophages ( 110 ).
As is known, skin is our shield against ultraviolet radiation (UVR) and other pollutants, as it is the most exterior layer of our body. Traffic-related pollutants, such as PAHs, VOCs, oxides, and PM, may cause pigmented spots on our skin ( 111 ). On the one hand, as already stated, when pollutants penetrate through the skin or are inhaled, damage to the organs is observed, as some of these pollutants are mutagenic and carcinogenic, and, specifically, they affect the liver and lung. On the other hand, air pollutants (and those in the troposphere) reduce the adverse effects of ultraviolet radiation UVR in polluted urban areas ( 111 ). Air pollutants absorbed by the human skin may contribute to skin aging, psoriasis, acne, urticaria, eczema, and atopic dermatitis ( 111 ), usually caused by exposure to oxides and photochemical smoke ( 111 ). Exposure to PM and cigarette smoking act as skin-aging agents, causing spots, dyschromia, and wrinkles. Lastly, pollutants have been associated with skin cancer ( 111 ).
Higher morbidity is reported to fetuses and children when exposed to the above dangers. Impairment in fetal growth, low birth weight, and autism have been reported ( 112 ).
Another exterior organ that may be affected is the eye. Contamination usually comes from suspended pollutants and may result in asymptomatic eye outcomes, irritation ( 112 ), retinopathy, or dry eye syndrome ( 113 , 114 ).
Environmental Impact of Air Pollution
Air pollution is harming not only human health but also the environment ( 115 ) in which we live. The most important environmental effects are as follows.
Acid rain is wet (rain, fog, snow) or dry (particulates and gas) precipitation containing toxic amounts of nitric and sulfuric acids. They are able to acidify the water and soil environments, damage trees and plantations, and even damage buildings and outdoor sculptures, constructions, and statues.
Haze is produced when fine particles are dispersed in the air and reduce the transparency of the atmosphere. It is caused by gas emissions in the air coming from industrial facilities, power plants, automobiles, and trucks.
Ozone , as discussed previously, occurs both at ground level and in the upper level (stratosphere) of the Earth's atmosphere. Stratospheric ozone is protecting us from the Sun's harmful ultraviolet (UV) rays. In contrast, ground-level ozone is harmful to human health and is a pollutant. Unfortunately, stratospheric ozone is gradually damaged by ozone-depleting substances (i.e., chemicals, pesticides, and aerosols). If this protecting stratospheric ozone layer is thinned, then UV radiation can reach our Earth, with harmful effects for human life (skin cancer) ( 116 ) and crops ( 117 ). In plants, ozone penetrates through the stomata, inducing them to close, which blocks CO 2 transfer and induces a reduction in photosynthesis ( 118 ).
Global climate change is an important issue that concerns mankind. As is known, the “greenhouse effect” keeps the Earth's temperature stable. Unhappily, anthropogenic activities have destroyed this protecting temperature effect by producing large amounts of greenhouse gases, and global warming is mounting, with harmful effects on human health, animals, forests, wildlife, agriculture, and the water environment. A report states that global warming is adding to the health risks of poor people ( 119 ).
People living in poorly constructed buildings in warm-climate countries are at high risk for heat-related health problems as temperatures mount ( 119 ).
Wildlife is burdened by toxic pollutants coming from the air, soil, or the water ecosystem and, in this way, animals can develop health problems when exposed to high levels of pollutants. Reproductive failure and birth effects have been reported.
Eutrophication is occurring when elevated concentrations of nutrients (especially nitrogen) stimulate the blooming of aquatic algae, which can cause a disequilibration in the diversity of fish and their deaths.
Without a doubt, there is a critical concentration of pollution that an ecosystem can tolerate without being destroyed, which is associated with the ecosystem's capacity to neutralize acidity. The Canada Acid Rain Program established this load at 20 kg/ha/yr ( 120 ).
Hence, air pollution has deleterious effects on both soil and water ( 121 ). Concerning PM as an air pollutant, its impact on crop yield and food productivity has been reported. Its impact on watery bodies is associated with the survival of living organisms and fishes and their productivity potential ( 121 ).
An impairment in photosynthetic rhythm and metabolism is observed in plants exposed to the effects of ozone ( 121 ).
Sulfur and nitrogen oxides are involved in the formation of acid rain and are harmful to plants and marine organisms.
Last but not least, as mentioned above, the toxicity associated with lead and other metals is the main threat to our ecosystems (air, water, and soil) and living creatures ( 121 ).
In 2018, during the first WHO Global Conference on Air Pollution and Health, the WHO's General Director, Dr. Tedros Adhanom Ghebreyesus, called air pollution a “silent public health emergency” and “the new tobacco” ( 122 ).
Undoubtedly, children are particularly vulnerable to air pollution, especially during their development. Air pollution has adverse effects on our lives in many different respects.
Diseases associated with air pollution have not only an important economic impact but also a societal impact due to absences from productive work and school.
Despite the difficulty of eradicating the problem of anthropogenic environmental pollution, a successful solution could be envisaged as a tight collaboration of authorities, bodies, and doctors to regularize the situation. Governments should spread sufficient information and educate people and should involve professionals in these issues so as to control the emergence of the problem successfully.
Technologies to reduce air pollution at the source must be established and should be used in all industries and power plants. The Kyoto Protocol of 1997 set as a major target the reduction of GHG emissions to below 5% by 2012 ( 123 ). This was followed by the Copenhagen summit, 2009 ( 124 ), and then the Durban summit of 2011 ( 125 ), where it was decided to keep to the same line of action. The Kyoto protocol and the subsequent ones were ratified by many countries. Among the pioneers who adopted this important protocol for the world's environmental and climate “health” was China ( 3 ). As is known, China is a fast-developing economy and its GDP (Gross Domestic Product) is expected to be very high by 2050, which is defined as the year of dissolution of the protocol for the decrease in gas emissions.
A more recent international agreement of crucial importance for climate change is the Paris Agreement of 2015, issued by the UNFCCC (United Nations Climate Change Committee). This latest agreement was ratified by a plethora of UN (United Nations) countries as well as the countries of the European Union ( 126 ). In this vein, parties should promote actions and measures to enhance numerous aspects around the subject. Boosting education, training, public awareness, and public participation are some of the relevant actions for maximizing the opportunities to achieve the targets and goals on the crucial matter of climate change and environmental pollution ( 126 ). Without any doubt, technological improvements makes our world easier and it seems difficult to reduce the harmful impact caused by gas emissions, we could limit its use by seeking reliable approaches.
Synopsizing, a global prevention policy should be designed in order to combat anthropogenic air pollution as a complement to the correct handling of the adverse health effects associated with air pollution. Sustainable development practices should be applied, together with information coming from research in order to handle the problem effectively.
At this point, international cooperation in terms of research, development, administration policy, monitoring, and politics is vital for effective pollution control. Legislation concerning air pollution must be aligned and updated, and policy makers should propose the design of a powerful tool of environmental and health protection. As a result, the main proposal of this essay is that we should focus on fostering local structures to promote experience and practice and extrapolate these to the international level through developing effective policies for sustainable management of ecosystems.
Author Contributions
All authors listed have made a substantial, direct and intellectual contribution to the work, and approved it for publication.
Conflict of Interest
IM is employed by the company Delphis S.A.
The remaining authors declare that the present review paper was conducted in the absence of any commercial or financial relationships that could be construed as a potential conflict of interest.
1. WHO. Air Pollution . WHO. Available online at: http://www.who.int/airpollution/en/ (accessed October 5, 2019).
Google Scholar
2. Moores FC. Climate change and air pollution: exploring the synergies and potential for mitigation in industrializing countries. Sustainability . (2009) 1:43–54. doi: 10.3390/su1010043
CrossRef Full Text | Google Scholar
3. USGCRP (2009). Global Climate Change Impacts in the United States. In: Karl TR, Melillo JM, Peterson TC, editors. Climate Change Impacts by Sectors: Ecosystems . New York, NY: United States Global Change Research Program. Cambridge University Press.
4. Marlon JR, Bloodhart B, Ballew MT, Rolfe-Redding J, Roser-Renouf C, Leiserowitz A, et al. (2019). How hope and doubt affect climate change mobilization. Front. Commun. 4:20. doi: 10.3389/fcomm.2019.00020
5. Eze IC, Schaffner E, Fischer E, Schikowski T, Adam M, Imboden M, et al. Long- term air pollution exposure and diabetes in a population-based Swiss cohort. Environ Int . (2014) 70:95–105. doi: 10.1016/j.envint.2014.05.014
PubMed Abstract | CrossRef Full Text | Google Scholar
6. Kelishadi R, Poursafa P. Air pollution and non-respiratory health hazards for children. Arch Med Sci . (2010) 6:483–95. doi: 10.5114/aoms.2010.14458
7. Manucci PM, Franchini M. Health effects of ambient air pollution in developing countries. Int J Environ Res Public Health . (2017) 14:1048. doi: 10.3390/ijerph14091048
8. Burden of Disease from Ambient and Household Air Pollution . Available online: http://who.int/phe/health_topics/outdoorair/databases/en/ (accessed August 15, 2017).
9. Hashim D, Boffetta P. Occupational and environmental exposures and cancers in developing countries. Ann Glob Health . (2014) 80:393–411. doi: 10.1016/j.aogh.2014.10.002
10. Guo Y, Zeng H, Zheng R, Li S, Pereira G, Liu Q, et al. The burden of lung cancer mortality attributable to fine particles in China. Total Environ Sci . (2017) 579:1460–6. doi: 10.1016/j.scitotenv.2016.11.147
11. Hou Q, An XQ, Wang Y, Guo JP. An evaluation of resident exposure to respirable particulate matter and health economic loss in Beijing during Beijing 2008 Olympic Games. Sci Total Environ . (2010) 408:4026–32. doi: 10.1016/j.scitotenv.2009.12.030
12. Kan H, Chen R, Tong S. Ambient air pollution, climate change, and population health in China. Environ Int . (2012) 42:10–9. doi: 10.1016/j.envint.2011.03.003
13. Burroughs Peña MS, Rollins A. Environmental exposures and cardiovascular disease: a challenge for health and development in low- and middle-income countries. Cardiol Clin . (2017) 35:71–86. doi: 10.1016/j.ccl.2016.09.001
14. Kankaria A, Nongkynrih B, Gupta S. Indoor air pollution in india: implications on health and its control. Indian J Comm Med . 39:203–7. doi: 10.4103/0970-0218.143019
15. Parajuli I, Lee H, Shrestha KR. Indoor air quality and ventilation assessment of rural mountainous households of Nepal. Int J Sust Built Env . (2016) 5:301–11. doi: 10.1016/j.ijsbe.2016.08.003
16. Saud T, Gautam R, Mandal TK, Gadi R, Singh DP, Sharma SK. Emission estimates of organic and elemental carbon from household biomass fuel used over the Indo-Gangetic Plain (IGP), India. Atmos Environ . (2012) 61:212–20. doi: 10.1016/j.atmosenv.2012.07.030
17. Singh DP, Gadi R, Mandal TK, Saud T, Saxena M, Sharma SK. Emissions estimates of PAH from biomass fuels used in rural sector of Indo-Gangetic Plains of India. Atmos Environ . (2013) 68:120–6. doi: 10.1016/j.atmosenv.2012.11.042
18. Dherani M, Pope D, Mascarenhas M, Smith KR, Weber M BN. Indoor air pollution from unprocessed solid fuel use and pneumonia risk in children aged under five years: a systematic review and meta-analysis. Bull World Health Organ . (2008) 86:390–4. doi: 10.2471/BLT.07.044529
19. Kassomenos P, Kelessis A, Petrakakis M, Zoumakis N, Christides T, Paschalidou AK. Air Quality assessment in a heavily-polluted urban Mediterranean environment through Air Quality indices. Ecol Indic . (2012) 18:259–68. doi: 10.1016/j.ecolind.2011.11.021
20. Dockery DW, Pope CA, Xu X, Spengler JD, Ware JH, Fay ME, et al. An association between air pollution and mortality in six U.S. cities. N Engl J Med . (1993) 329:1753–9. doi: 10.1056/NEJM199312093292401
21. Schwela DH, Köth-Jahr I. Leitfaden für die Aufstellung von Luftreinhalteplänen [Guidelines for the Implementation of Clean Air Implementation Plans]. Landesumweltamt des Landes Nordrhein Westfalen. State Environmental Service of the State of North Rhine-Westphalia (1994).
22. Newlands M. Environmental Activism, Environmental Politics, and Representation: The Framing of the British Environmental Activist Movement . Ph.D. thesis. University of East London, United Kingdom (2015).
23. NEPIS (National Service Center for Environmental Publications) US EPA (Environmental Protection Agency) (2017). Available online at: https://www.epa.gov/clean-air-act-overview/air-pollution-current-and-future-challenges (accessed August 15, 2017).
24. NRC (National Research Council). Available online at: https://www.nap.edu/read/10728/chapter/1,2014 (accessed September 17, 2019).
25. Bull A. Traffic Congestion: The Problem and How to Deal With It . Santiago: Nationes Unidas, Cepal (2003).
26. Spiegel J, Maystre LY. Environmental Pollution Control, Part VII - The Environment, Chapter 55, Encyclopedia of Occupational Health and Safety . Available online at: http://www.ilocis.org/documents/chpt55e.htm (accessed September 17, 2019).
27. European Community Reports. Assessment of the Effectiveness of European Air Quality Policies and Measures: Case Study 2; Comparison of the EU and US Air Quality Standards and Planning Requirements. (2004). Available online at: https://ec.europa.eu/environment/archives/cafe/activities/pdf/case_study2.pdf (accessed September 22, 2019).
28. Gibson R, Ward S. Parties in the digital age; a review. J Represent Democracy . (2009) 45:87–100. doi: 10.1080/00344890802710888
29. Kaun A, Uldam J. Digital activism: after the hype. New Media Soc. (2017) 20:2099–106. doi: 10.1177/14614448177319
30. Sivitanides M, Shah V. The era of digital activism. In: 2011 Conference for Information Systems Applied Research(CONISAR) Proceedings Wilmington North Carolina, USA . Available online at: https://www.arifyildirim.com/ilt510/marcos.sivitanides.vivek.shah.pdf (accessed September 22, 2019).
31. Möller L, Schuetzle D, Autrup H. Future research needs associated with the assessment of potential human health risks from exposure to toxic ambient air pollutants. Environ Health Perspect . (1994) 102(Suppl. 4):193–210. doi: 10.1289/ehp.94102s4193
32. Jacobson MZ, Jacobson PMZ. Atmospheric Pollution: History, Science, and Regulation. Cambridge University Press (2002). p. 206. doi: 10.1256/wea.243.02
33. Stover RH. Flooding of soil for disease control. In: Mulder D, editor. Chapter 3. Developments in Agricultural and Managed Forest Ecology . Elsevier (1979). p. 19–28. Available online at: http://www.sciencedirect.com/science/article/pii/B9780444416926500094 doi: 10.1016/B978-0-444-41692-6.50009-4 (accessed July 1, 2019).
34. Maipa V, Alamanos Y, Bezirtzoglou E. Seasonal fluctuation of bacterial indicators in coastal waters. Microb Ecol Health Dis . (2001) 13:143–6. doi: 10.1080/089106001750462687
35. Bezirtzoglou E, Dimitriou D, Panagiou A. Occurrence of Clostridium perfringens in river water by using a new procedure. Anaerobe . (1996) 2:169–73. doi: 10.1006/anae.1996.0022
36. Kjellstrom T, Lodh M, McMichael T, Ranmuthugala G, Shrestha R, Kingsland S. Air and Water Pollution: Burden and Strategies for Control. DCP, Chapter 43. 817–32 p. Available online at: https://www.dcp-3.org/sites/default/files/dcp2/DCP43.pdf (accessed September 17, 2017).
37. Pathak RK, Wang T, Ho KF, Lee SC. Characteristics of summertime PM2.5 organic and elemental carbon in four major Chinese cities: implications of high acidity for water- soluble organic carbon (WSOC). Atmos Environ . (2011) 45:318–25. doi: 10.1016/j.atmosenv.2010.10.021
38. Bonavigo L, Zucchetti M, Mankolli H. Water radioactive pollution and related environmental aspects. J Int Env Appl Sci . (2009) 4:357–63
39. World Health Organization (WHO). Preventing Disease Through Healthy Environments: Towards an Estimate of the Environmental Burden of Disease . 1106 p. Available online at: https://www.who.int/quantifying_ehimpacts/publications/preventingdisease.pdf (accessed September 22, 2019).
40. Stansfeld SA. Noise effects on health in the context of air pollution exposure. Int J Environ Res Public Health . (2015) 12:12735–60. doi: 10.3390/ijerph121012735
41. Ethical Unicorn. Everything You Need To Know About Aerosols & Air Pollution. (2019). Available online at: https://ethicalunicorn.com/2019/04/29/everything-you-need-to-know-about-aerosols-air-pollution/ (accessed October 4, 2019).
42. Colbeck I, Lazaridis M. Aerosols and environmental pollution. Sci Nat . (2009) 97:117–31. doi: 10.1007/s00114-009-0594-x
43. Incecik S, Gertler A, Kassomenos P. Aerosols and air quality. Sci Total Env . (2014) 355, 488–9. doi: 10.1016/j.scitotenv.2014.04.012
44. D'Amato G, Pawankar R, Vitale C, Maurizia L. Climate change and air pollution: effects on respiratory allergy. Allergy Asthma Immunol Res . (2016) 8:391–5. doi: 10.4168/aair.2016.8.5.391
45. Bezirtzoglou C, Dekas K, Charvalos E. Climate changes, environment and infection: facts, scenarios and growing awareness from the public health community within Europe. Anaerobe . (2011) 17:337–40. doi: 10.1016/j.anaerobe.2011.05.016
46. Castelli F, Sulis G. Migration and infectious diseases. Clin Microbiol Infect . (2017) 23:283–9. doi: 10.1016/j.cmi.2017.03.012
47. Watson JT, Gayer M, Connolly MA. Epidemics after natural disasters. Emerg Infect Dis . (2007) 13:1–5. doi: 10.3201/eid1301.060779
48. Fenn B. Malnutrition in Humanitarian Emergencies . Available online at: https://www.who.int/diseasecontrol_emergencies/publications/idhe_2009_london_malnutrition_fenn.pdf . (accessed August 15, 2017).
49. Lindh E, Argentini C, Remoli ME, Fortuna C, Faggioni G, Benedetti E, et al. The Italian 2017 outbreak Chikungunya virus belongs to an emerging Aedes albopictus –adapted virus cluster introduced from the Indian subcontinent. Open Forum Infect Dis. (2019) 6:ofy321. doi: 10.1093/ofid/ofy321
50. Calba C, Guerbois-Galla M, Franke F, Jeannin C, Auzet-Caillaud M, Grard G, Pigaglio L, Decoppet A, et al. Preliminary report of an autochthonous chikungunya outbreak in France, July to September 2017. Eur Surveill . (2017) 22:17-00647. doi: 10.2807/1560-7917.ES.2017.22.39.17-00647
51. Menne B, Murray V. Floods in the WHO European Region: Health Effects and Their Prevention . Copenhagen: WHO; Weltgesundheits organisation, Regionalbüro für Europa (2013). Available online at: http://www.euro.who.int/data/assets/pdf_file/0020/189020/e96853.pdf (accessed 15 August 2017).
52. Schneider SH. The greenhouse effect: science and policy. Science . (1989) 243:771–81. doi: 10.1126/science.243.4892.771
53. Wilson WE, Suh HH. Fine particles and coarse particles: concentration relationships relevant to epidemiologic studies. J Air Waste Manag Assoc . (1997) 47:1238–49. doi: 10.1080/10473289.1997.10464074
54. US EPA (US Environmental Protection Agency) (2018). Available online at: https://www.epa.gov/pm-pollution/particulate-matter-pm-basics (accessed September 22, 2018).
55. Cheung K, Daher N, Kam W, Shafer MM, Ning Z, Schauer JJ, et al. Spatial and temporal variation of chemical composition and mass closure of ambient coarse particulate matter (PM10–2.5) in the Los Angeles area. Atmos Environ . (2011) 45:2651–62. doi: 10.1016/j.atmosenv.2011.02.066
56. Zhang L, Yang Y, Li Y, Qian ZM, Xiao W, Wang X, et al. Short-term and long-term effects of PM2.5 on acute nasopharyngitis in 10 communities of Guangdong, China. Sci Total Env. (2019) 688:136–42. doi: 10.1016/j.scitotenv.2019.05.470.
57. Kloog I, Ridgway B, Koutrakis P, Coull BA, Schwartz JD. Long- and short-term exposure to PM2.5 and mortality using novel exposure models, Epidemiology . (2013) 24:555–61. doi: 10.1097/EDE.0b013e318294beaa
58. New Hampshire Department of Environmental Services. Current and Forecasted Air Quality in New Hampshire . Environmental Fact Sheet (2019). Available online at: https://www.des.nh.gov/organization/commissioner/pip/factsheets/ard/documents/ard-16.pdf (accessed September 22, 2019).
59. Kappos AD, Bruckmann P, Eikmann T, Englert N, Heinrich U, Höppe P, et al. Health effects of particles in ambient air. Int J Hyg Environ Health . (2004) 207:399–407. doi: 10.1078/1438-4639-00306
60. Boschi N (Ed.). Defining an educational framework for indoor air sciences education. In: Education and Training in Indoor Air Sciences . Luxembourg: Springer Science & Business Media (2012). 245 p.
61. Heal MR, Kumar P, Harrison RM. Particles, air quality, policy and health. Chem Soc Rev . (2012) 41:6606–30. doi: 10.1039/c2cs35076a
62. Bezirtzoglou E, Alexopoulos A. Ozone history and ecosystems: a goliath from impacts to advance industrial benefits and interests, to environmental and therapeutical strategies. In: Ozone Depletion, Chemistry and Impacts. (2009). p. 135–45.
63. Villányi V, Turk B, Franc B, Csintalan Z. Ozone Pollution and its Bioindication. In: Villányi V, editor. Air Pollution . London: Intech Open (2010). doi: 10.5772/10047
64. Massachusetts Department of Public Health. Massachusetts State Health Assessment . Boston, MA (2017). Available online at: https://www.mass.gov/files/documents/2017/11/03/2017%20MA%20SHA%20final%20compressed.pdf (accessed October 30, 2017).
65. Lorenzini G, Saitanis C. Ozone: A Novel Plant “Pathogen.” In: Sanitá di Toppi L, Pawlik-Skowrońska B, editors. Abiotic Stresses in Plant Springer Link (2003). p. 205–29. doi: 10.1007/978-94-017-0255-3_8
66. Fares S, Vargas R, Detto M, Goldstein AH, Karlik J, Paoletti E, et al. Tropospheric ozone reduces carbon assimilation in trees: estimates from analysis of continuous flux measurements. Glob Change Biol . (2013) 19:2427–43. doi: 10.1111/gcb.12222
67. Harmens H, Mills G, Hayes F, Jones L, Norris D, Fuhrer J. Air Pollution and Vegetation . ICP Vegetation Annual Report 2006/2007. (2012)
68. Emberson LD, Pleijel H, Ainsworth EA, den Berg M, Ren W, Osborne S, et al. Ozone effects on crops and consideration in crop models. Eur J Agron . (2018) 100:19–34. doi: 10.1016/j.eja.2018.06.002
69. Alexopoulos A, Plessas S, Ceciu S, Lazar V, Mantzourani I, Voidarou C, et al. Evaluation of ozone efficacy on the reduction of microbial population of fresh cut lettuce ( Lactuca sativa ) and green bell pepper ( Capsicum annuum ). Food Control . (2013) 30:491–6. doi: 10.1016/j.foodcont.2012.09.018
70. Alexopoulos A, Plessas S, Kourkoutas Y, Stefanis C, Vavias S, Voidarou C, et al. Experimental effect of ozone upon the microbial flora of commercially produced dairy fermented products. Int J Food Microbiol . (2017) 246:5–11. doi: 10.1016/j.ijfoodmicro.2017.01.018
71. Maggio A, Fagnano M. Ozone damages to mediterranean crops: physiological responses. Ital J Agron . (2008) 13–20. doi: 10.4081/ija.2008.13
72. McCarthy JT, Pelle E, Dong K, Brahmbhatt K, Yarosh D, Pernodet N. Effects of ozone in normal human epidermal keratinocytes. Exp Dermatol . (2013) 22:360–1. doi: 10.1111/exd.12125
73. WHO. Health Risks of Ozone From Long-Range Transboundary Air Pollution . Available online at: http://www.euro.who.int/data/assets/pdf_file/0005/78647/E91843.pdf (accessed August 15, 2019).
74. Thiele JJ, Traber MG, Tsang K, Cross CE, Packer L. In vivo exposure to ozone depletes vitamins C and E and induces lipid peroxidation in epidermal layers of murine skin. Free Radic Biol Med. (1997) 23:365–91. doi: 10.1016/S0891-5849(96)00617-X
75. Hatch GE, Slade R, Harris LP, McDonnell WF, Devlin RB, Koren HS, et al. Ozone dose and effect in humans and rats. A comparison using oxygen- 18 labeling and bronchoalveolar lavage. Am J Respir Crit Care Med . (1994) 150:676–83. doi: 10.1164/ajrccm.150.3.8087337
76. Lippmann M. Health effects of ozone. A critical review. JAPCA . (1989) 39:672–95. doi: 10.1080/08940630.1989.10466554
77. Gryparis A, Forsberg B, Katsouyanni K, Analitis A, Touloumi G, Schwartz J, et al. Acute effects of ozone on mortality from the “air pollution and health: a European approach” project. Am J Respir Crit Care Med . (2004) 170:1080–7. doi: 10.1164/rccm.200403-333OC
78. Soon W, Baliunas SL, Robinson AB, Robinson ZW. Environmental effects of increased atmospheric carbon dioxide. Climate Res . (1999) 13:149–64 doi: 10.1260/0958305991499694
79. Richmont-Bryant J, Owen RC, Graham S, Snyder M, McDow S, Oakes M, et al. Estimation of on-road NO2 concentrations, NO2/NOX ratios, and related roadway gradients from near-road monitoring data. Air Qual Atm Health . (2017) 10:611–25. doi: 10.1007/s11869-016-0455-7
80. Hesterberg TW, Bunn WB, McClellan RO, Hamade AK, Long CM, Valberg PA. Critical review of the human data on short-term nitrogen dioxide (NO 2 ) exposures: evidence for NO2 no-effect levels. Crit Rev Toxicol . (2009) 39:743–81. doi: 10.3109/10408440903294945
81. Chen T-M, Gokhale J, Shofer S, Kuschner WG. Outdoor air pollution: nitrogen dioxide, sulfur dioxide, and carbon monoxide health effects. Am J Med Sci . (2007) 333:249–56. doi: 10.1097/MAJ.0b013e31803b900f
82. US EPA. Table of Historical SO 2 NAAQS, Sulfur US EPA . Available online at: https://www3.epa.gov/ttn/naaqs/standards/so2/s_so2_history.html (accessed October 5, 2019).
83. WHO Regional Office of Europe (2000). Available online at: https://euro.who.int/_data/assets/pdf_file/0020/123086/AQG2ndEd_7_4Sulfuroxide.pdf
84. Pruss-Ustun A, Fewrell L, Landrigan PJ, Ayuso-Mateos JL. Lead exposure. Comparative Quantification of Health Risks . World Health Organization. p. 1495–1542. Available online at: https://www.who.int/publications/cra/chapters/volume2/1495-1542.pdf?ua=1
PubMed Abstract | Google Scholar
85. Goyer RA. Transplacental transport of lead. Environ Health Perspect . (1990) 89:101–5. doi: 10.1289/ehp.9089101
86. National Institute of Environmental Health Sciences (NIH). Lead and Your Health . (2013). 1–4 p. Available online at: https://www.niehs.nih.gov/health/materials/lead_and_your_health_508.pdf (accessed September 17, 2019).
87. Farhat A, Mohammadzadeh A, Balali-Mood M, Aghajanpoor-Pasha M, Ravanshad Y. Correlation of blood lead level in mothers and exclusively breastfed infants: a study on infants aged less than six months. Asia Pac J Med Toxicol . (2013) 2:150–2.
88. Assi MA, Hezmee MNM, Haron AW, Sabri MYM, Rajion MA. The detrimental effects of lead on human and animal health. Vet World . (2016) 9:660–71. doi: 10.14202/vetworld.2016.660-671
89. Abdel-Shafy HI, Mansour MSM. A review on polycyclic aromatic hydrocarbons: source, environmental impact, effect on human health and remediation. Egypt J Pet . (2016) 25:107–23. doi: 10.1016/j.ejpe.2015.03.011
90. Kumar A, Singh BP, Punia M, Singh D, Kumar K, Jain VK. Assessment of indoor air concentrations of VOCs and their associated health risks in the library of Jawaharlal Nehru University, New Delhi. Environ Sci Pollut Res Int . (2014) 21:2240–8. doi: 10.1007/s11356-013-2150-7
91. Molhave L, Clausen G, Berglund B, Ceaurriz J, Kettrup A, Lindvall T, et al. Total Volatile Organic Compounds (TVOC) in Indoor Air Quality Investigations. Indoor Air . 7:225–240. doi: 10.1111/j.1600-0668.1997.00002.x
92. Gibb T. Indoor Air Quality May be Hazardous to Your Health . MSU Extension. Available online at: https://www.canr.msu.edu/news/indoor_air_quality_may_be_hazardous_to_your_health (accessed October 5, 2019).
93. Ebersviller S, Lichtveld K, Sexton KG, Zavala J, Lin Y-H, Jaspers I, et al. Gaseous VOCs rapidly modify particulate matter and its biological effects – Part 1: simple VOCs and model PM. Atmos Chem Phys Discuss . (2012) 12:5065–105. doi: 10.5194/acpd-12-5065-2012
94. WHO (World Health Organization). Dioxins and Their Effects on Human Health. Available online at: https://www.who.int/news-room/fact-sheets/detail/dioxins-and-their-effects-on-human-health (accessed October 5, 2019).
95. EEA (European Environmental Agency). Air Quality Standards to the European Union and WHO . Available online at: https://www.eea.europa.eu/themes/data-and-maps/figures/air-quality-standards-under-the
96. Nakano T, Otsuki T. [Environmental air pollutants and the risk of cancer]. (Japanese). Gan To Kagaku Ryoho . (2013) 40:1441–5.
97. Kurt OK, Zhang J, Pinkerton KE. Pulmonary health effects of air pollution. Curr Opin Pulm Med . (2016) 22:138–43. doi: 10.1097/MCP.0000000000000248
98. Guarnieri M, Balmes JR. Outdoor air pollution and asthma. Lancet . (2014) 383:1581–92. doi: 10.1016/S0140-6736(14)60617-6
99. Jiang X-Q, Mei X-D, Feng D. Air pollution and chronic airway diseases: what should people know and do? J Thorac Dis . (2016) 8:E31–40.
100. Bourdrel T, Bind M-A, Béjot Y, Morel O, Argacha J-F. Cardiovascular effects of air pollution. Arch Cardiovasc Dis . (2017) 110:634–42. doi: 10.1016/j.acvd.2017.05.003
101. Hoffmann B, Moebus S, Möhlenkamp S, Stang A, Lehmann N, Dragano N, et al. Residential exposure to traffic is associated with coronary atherosclerosis. Circulation . (2007) 116:489–496. doi: 10.1161/CIRCULATIONAHA.107.693622
102. Katholi RE, Couri DM. Left ventricular hypertrophy: major risk factor in patients with hypertension: update and practical clinical applications. Int J Hypertens . (2011) 2011:495349. doi: 10.4061/2011/495349
103. Leary PJ, Kaufman JD, Barr RG, Bluemke DA, Curl CL, Hough CL, et al. Traffic- related air pollution and the right ventricle. the multi-ethnic study of atherosclerosis. Am J Respir Crit Care Med . (2014) 189:1093–100. doi: 10.1164/rccm.201312-2298OC
104. Genc S, Zadeoglulari Z, Fuss SH, Genc K. The adverse effects of air pollution on the nervous system. J Toxicol . (2012) 2012:782462. doi: 10.1155/2012/782462
105. Calderon-Garciduenas L, Azzarelli B, Acuna H, et al. Air pollution and brain damage. Toxicol Pathol. (2002) 30:373–89. doi: 10.1080/01926230252929954
106. Rückerl R, Greven S, Ljungman P, Aalto P, Antoniades C, Bellander T, et al. Air pollution and inflammation (interleukin-6, C-reactive protein, fibrinogen) in myocardial infarction survivors. Environ Health Perspect . (2007) 115:1072–80. doi: 10.1289/ehp.10021
107. Peters A, Veronesi B, Calderón-Garcidueñas L, Gehr P, Chen LC, Geiser M, et al. Translocation and potential neurological effects of fine and ultrafine particles a critical update. Part Fibre Toxicol . (2006) 3:13–8. doi: 10.1186/1743-8977-3-13
108. Kelly FJ. Dietary antioxidants and environmental stress. Proc Nutr Soc . (2004) 63:579–85. doi: 10.1079/PNS2004388
109. Bellinger DC. Very low lead exposures and children's neurodevelopment. Curr Opin Pediatr . (2008) 20:172–7. doi: 10.1097/MOP.0b013e3282f4f97b
110. Balbo P, Silvestri M, Rossi GA, Crimi E, Burastero SE. Differential role of CD80 and CD86 on alveolar macrophages in the presentation of allergen to T lymphocytes in asthma. Clin Exp Allergy J Br Soc Allergy Clin Immunol . (2001) 31:625–36. doi: 10.1046/j.1365-2222.2001.01068.x
111. Drakaki E, Dessinioti C, Antoniou C. Air pollution and the skin. Front Environ Sci Eng China . (2014) 15:2–8. doi: 10.3389/fenvs.2014.00011
112. Weisskopf MG, Kioumourtzoglou M-A, Roberts AL. Air pollution and autism spectrum disorders: causal or confounded? Curr Environ Health Rep . (2015) 2:430–9. doi: 10.1007/s40572-015-0073-9
113. Mo Z, Fu Q, Lyu D, Zhang L, Qin Z, Tang Q, et al. Impacts of air pollution on dry eye disease among residents in Hangzhou, China: a case-crossover study. Environ Pollut . (2019) 246:183–9. doi: 10.1016/j.envpol.2018.11.109
114. Klopfer J. Effects of environmental air pollution on the eye. J Am Optom Assoc . (1989) 60:773–8.
115. Ashfaq A, Sharma P. Environmental effects of air pollution and application of engineered methods to combat the problem. J Indust Pollut Control . (2012) 29.
116. Madronich S, de Gruijl F. Skin cancer and UV radiation. Nature . (1993) 366:23–9. doi: 10.1038/366023a0
117. Teramura A. Effects of UV-B radiation on the growth and yield of crop plants. Physiol Plant . (2006) 58:415–27. doi: 10.1111/j.1399-3054.1983.tb04203.x
118. Singh E, Tiwari S, Agrawal M. Effects of elevated ozone on photosynthesis and stomatal conductance of two soybean varieties: a case study to assess impacts of one component of predicted global climate change. Plant Biol Stuttg Ger . (2009) 11(Suppl. 1):101–8. doi: 10.1111/j.1438-8677.2009.00263.x
119. Manderson L. How global Warming is Adding to the Health Risks of Poor People . The Conversation. University of the Witwatersrand. Available online at: http://theconversation.com/how-global-warming-is-adding-to-the-health-risks-of-poor-people-109520 (accessed October 5, 2019).
120. Ministers of Energy and Environment. Federal/Provincial/Territorial Ministers of Energy and Environment (Canada), editor. The Canada-Wide Acid Rain Strategy for Post-2000 . Halifax: The Ministers (1999). 11 p.
121. Zuhara S, Isaifan R. The impact of criteria air pollutants on soil and water: a review. (2018) 278–84. doi: 10.30799/jespr.133.18040205
122. WHO. First WHO Global Conference on Air Pollution and Health. (2018). Available online at: https://www.who.int/airpollution/events/conference/en/ (accessed October 6, 2019).
123. What is the Kyoto Protocol? UNFCCC . Available online at: https://unfccc.int/kyoto__protocol (accessed October 6, 2019).
124. CopenhagenClimate Change Conference (UNFCCC) . Available online at: https://unfccc.int/process-and-meetings/conferences/past-conferences/copenhagen-climate-change-conference-december-2009/copenhagen-climate-change-conference-december-2009 (accessed October 6, 2019).
125. Durban Climate Change Conference,. UNFCCC (2011). Available online at: https://unfccc.int/process-and-meetings/conferences/past-conferences/copenhagen-climate-change-conference-december-2009/copenhagen-climate-change-conference-december-2009 (accessed October 6, 2019).
126. Paris Climate Change Agreement,. (2016). Available online at: https://unfccc.int/process-and-meetings/the-paris-agreement/the-paris-agreement
Keywords: air pollution, environment, health, public health, gas emission, policy
Citation: Manisalidis I, Stavropoulou E, Stavropoulos A and Bezirtzoglou E (2020) Environmental and Health Impacts of Air Pollution: A Review. Front. Public Health 8:14. doi: 10.3389/fpubh.2020.00014
Received: 17 October 2019; Accepted: 17 January 2020; Published: 20 February 2020.
Reviewed by:
Copyright © 2020 Manisalidis, Stavropoulou, Stavropoulos and Bezirtzoglou. This is an open-access article distributed under the terms of the Creative Commons Attribution License (CC BY) . The use, distribution or reproduction in other forums is permitted, provided the original author(s) and the copyright owner(s) are credited and that the original publication in this journal is cited, in accordance with accepted academic practice. No use, distribution or reproduction is permitted which does not comply with these terms.
*Correspondence: Ioannis Manisalidis, giannismanisal@gmail.com ; Elisavet Stavropoulou, elisabeth.stavropoulou@gmail.com
† These authors have contributed equally to this work
Disclaimer: All claims expressed in this article are solely those of the authors and do not necessarily represent those of their affiliated organizations, or those of the publisher, the editors and the reviewers. Any product that may be evaluated in this article or claim that may be made by its manufacturer is not guaranteed or endorsed by the publisher.
Click through the PLOS taxonomy to find articles in your field.
For more information about PLOS Subject Areas, click here .
Loading metrics
Open Access
Peer-reviewed
Research Article
Exposure to outdoor air pollution and its human health outcomes: A scoping review
Contributed equally to this work with: Zhuanlan Sun, Demi Zhu
Roles Writing – original draft
Affiliation Department of Management Science and Engineering, School of Economics and Management, Tongji University, Shanghai, China

Roles Writing – review & editing
* E-mail: [email protected]
Affiliation Department of Comparative Politics, School of International and Public Affairs, Shanghai Jiaotong University, Shanghai, China
- Zhuanlan Sun,
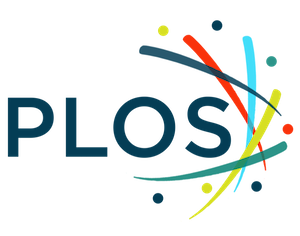
- Published: May 16, 2019
- https://doi.org/10.1371/journal.pone.0216550
- Reader Comments
Despite considerable air pollution prevention and control measures that have been put into practice in recent years, outdoor air pollution remains one of the most important risk factors for health outcomes. To identify the potential research gaps, we conducted a scoping review focused on health outcomes affected by outdoor air pollution across the broad research area. Of the 5759 potentially relevant studies, 799 were included in the final analysis. The included studies showed an increasing publication trend from 1992 to 2008, and most of the studies were conducted in Asia, Europe, and North America. Among the eight categorized health outcomes, asthma (category: respiratory diseases) and mortality (category: health records) were the most common ones. Adverse health outcomes involving respiratory diseases among children accounted for the largest group. Out of the total included studies, 95.2% reported at least one statistically positive result, and only 0.4% showed ambiguous results. Based on our study, we suggest that the time frame of the included studies, their disease definitions, and the measurement of personal exposure to outdoor air pollution should be taken into consideration in any future research. The main limitation of this study is its potential language bias, since only English publications were included. In conclusion, this scoping review provides researchers and policy decision makers with evidence taken from multiple disciplines to show the increasing prevalence of outdoor air pollution and its adverse effects on health outcomes.
Citation: Sun Z, Zhu D (2019) Exposure to outdoor air pollution and its human health outcomes: A scoping review. PLoS ONE 14(5): e0216550. https://doi.org/10.1371/journal.pone.0216550
Editor: Mathilde Body-Malapel, University of Lille, FRANCE
Received: December 15, 2018; Accepted: April 10, 2019; Published: May 16, 2019
Copyright: © 2019 Sun, Zhu. This is an open access article distributed under the terms of the Creative Commons Attribution License , which permits unrestricted use, distribution, and reproduction in any medium, provided the original author and source are credited.
Data Availability: All relevant data are within the manuscript and its Supporting Information files.
Funding: This work received support from Major projects of the National Social Science Fund of China, Award Number: 13&ZD176, Grant recipient: Demi Zhu.
Competing interests: The authors have declared that no competing interests exist.
Introduction
In recent years, despite considerable improvements in air pollution prevention and control, outdoor air pollution has remained a major environmental health hazard to human beings. In some developing countries, the concentrations of air quality far exceed the upper limit announced in the World Health Organization guidelines [ 1 ]. Moreover, it is widely acknowledged that outdoor air pollution increases the incidence rates of multiple diseases, such as cardiovascular disease, lung cancer, respiratory symptoms, asthma, negatively affected pregnancy, and poor birth outcomes [ 2 – 6 ].
The influence of outdoor air pollution exposure and its mechanisms continue to be hotly debated [ 7 – 11 ]. Some causal inference studies have been conducted to examine these situations [ 12 ]; these have indicated that an increase in outdoor air exposure affects people’s health outcomes both directly and indirectly [ 13 ]. However, few studies in the existing literature have examined the extent, range, and nature of the influence of outdoor air pollution with regard to human health outcomes. Thus, such research gaps need to be identified, and related fields of study need to be mapped.
Systematic reviews and meta-analyses, the most commonly used traditional approach to synthesize knowledge, use quantified data from relevant published studies in order to aggregate findings on a specific topic [ 14 ]; furthermore, they formally assesses the quality of these studies to generate precise conclusions related to the focused research question [ 15 ]. In comparison, scoping review is a more narrative type of knowledge synthesis, and it focuses on a broader area [ 16 ] of the evidence pertaining to a given topic. It is often used to systematically summarize the evidence available (main sources, types, and research characteristics), and it tends to be more comprehensive and helpful to policymakers at all levels.
Scoping reviews have already been used to examine a variety of health related issues [ 17 ]. As an evidence synthesis approach that is still in the midst of development, the methodology framework for scoping reviews faces some controversy with regard to its conceptual clarification and definition [ 18 , 19 ], the necessity of quality assessment [ 20 – 22 ], and the time required for completion [ 19 , 21 , 23 ]. Comparing this approach with other knowledge synthesis methods, such as evidence gap map and rapid review, the scoping review has become increasingly influential for efficient evidence-based decision-making because it offers a very broad topic scope [ 15 ].
To our knowledge, few studies have systematically reviewed the literature in the broad field of outdoor air pollution exposure research, especially with regard to related health outcomes. To fill this gap, we conducted a comprehensive scoping review of the literature with a focus on health outcomes affected by outdoor air pollution. The purposes of this study were as follows: 1) provide a systematic overview of relevant studies; 2) identify the different types of outdoor air pollution and related health outcomes; and 3) summarize the publication characteristics and explore related research gaps.
Materials and methods
The methodology framework used in this study was initially outlined by Arksey and O’Malley [ 23 ] and further advanced by Levac et al. [ 20 ], Daudt et al. [ 21 ], and the Joanna Briggs Institute [ 24 ]. The framework was divided into six stages: identifying the research question; identifying relevant studies; study selection; charting the data; collating, summarizing and reporting the results; and consulting exercise.
Stage one: Research question identification
As recommended, we combined broader research questions with a clearly articulated scope of inquiry [ 20 ]; this included defining the concept, target population, and outcomes of interest in order to disseminate an effective search strategy. Thus, an adaptation of the “PCC” (participants, concept, context) strategy was used to guide the construction of research questions and inclusion criteria [ 24 ].
Types of participants.
There were no strict restrictions on ages, genders, ethnicity, or regions of participants. Everyone, including newborns, children, adults, pregnant women, and the elderly, suffer from health outcomes related to exposure to outdoor air pollution; hence, all groups were included in the study to ensure that the inquiry was sufficiently comprehensive.
The core concept was clearly articulated in order to guide the scope and breadth of the inquiry [ 24 ]. A list of outdoor air pollution and health outcome related terms were compiled by reviewing potential text words in the titles or abstracts of the most pertinent articles [ 25 – 33 ]; we also read the most cited literature reviews on air pollution related health outcomes. To classify the types of air pollution and health outcomes, we consulted researchers from different air pollution related disciplines. The classified results are shown in Table 1 .
- PPT PowerPoint slide
- PNG larger image
- TIFF original image
https://doi.org/10.1371/journal.pone.0216550.t001
Our scoping review included studies from peer-reviewed journals. There were no restrictions in terms of the research field, time period, and geographical coverage. The intended audiences of our scoping review were researchers, physicians, and public policymakers.
Stage two: Relevant studies identification
We followed Joanna Briggs Institute’s instructions [ 24 ] to launch three-step search strategies to identify all relevant published and unpublished studies (grey literature) across the multi-disciplinary topic in an iterative way. The first step included a limited search of the entire database using keywords relevant to the topic and conducting an abstract and indexing categorizations analysis. The second step was a further search of all included databases based on the newly identified keywords and index terms. The final step was to search the reference list of the identified reports and literatures.
Electronic databases.
We conducted comprehensive literature searches by consulting with an information specialist. We searched the following three electronic databases from their inception until now: PubMed, Web of Science, and Scopus. The language of the studies included in our sample was restricted to English.
Search terms.
The search terms we used were broad enough to uncover any related literature and prevent chances of relevant information being overlooked. This process was conducted iteratively with different search item combinations to ensure that all relevant literature was captured ( S1 Table ).
The search used combinations of the following terms: 1) outdoor air pollution (ozone, sulfur dioxide, carbon monoxide, nitrogen dioxide, PM 2.5 , PM 10 , total suspended particle, suspended particulate matter, toxic air pollutant, volatile organic pollutant, nitrogen oxide) and 2) health outcomes (asthma, lung cancer, respiratory infection, respiratory disorder, diabetes, chronic respiratory disease, chronic obstructive pulmonary disease, hypertension, heart rate variability, heart attack, cardiopulmonary disease, ischemic heart disease, blood coagulation, deep vein thrombosis, stroke, morbidity, hospital admission, outpatient visit, emergency room visit, mortality, DNA methylation change, neurobehavioral function, inflammatory disease, skin disease, abortion, Alzheimer’s disease, disability, cognitive function, Parkinson’s disease).
Additional studies search.
Key, important, and top journals were read manually, reference lists and citation tracing were used to collect studies and related materials, and suggestions from specialists were considered to guarantee that the research was as comprehensive as possible.
Bibliographies Management Software (Mendeley) was used to remove duplicated literatures and manage thousands of bibliographic references that needed to be appraised to check whether they should be included in the final study selection.
Our literature retrieval generated a total of 5759 references; the majority of these (3567) were found on the Scopus electronic database, which emphasized the importance of collecting the findings on this broad topic.
Stage three: Studies selection
Our study identification picked up a large number of irrelevant studies; we needed a mechanism to include only the studies that best fit the research question. The study selection stage should be an iterative process of searching the literature, refining the search strategy, and reviewing articles for inclusion. Study inclusion and exclusion criteria were discussed by the team members at the beginning of the process, then two inter-professional researchers applied the criteria to independently review the titles and abstracts of all studies [ 21 ]. If there were any ambiguities, the full article was read to make decision about whether it should be chosen for inclusion. When disagreements on study inclusion occurred, a third specialist reviewer made the final decision. This process should be iterative to guarantee the inclusion of all relevant studies.
Inclusion and exclusion criteria.
The inclusion criteria used in our scoping study ensured that the articles were considered only if they were: 1) long-term and short-term exposure, perspective or prospective studies; 2) epidemiological time series studies; 3) meta-analysis and systematic review articles rather than the primary studies that contained the main parameters we were concerned with; 4) economic research studies using causality inference with observational data; and 5) etiology research studies on respiratory disease, cancer, and cardiovascular disease.
Articles were removed if they 1) focused exclusively on indoor air pollution exposure and 2) did not belong to peer-reviewed journals or conference papers (such as policy documents, proposals, and editorials).
Stage four: Data charting
The data extracted from the final articles were entered into a “data charting form” using the database, programmed Excel, so that the following relevant data could be recorded and charted according to the variables of interest ( Table 2 ).
https://doi.org/10.1371/journal.pone.0216550.t002
Stage five: Results collection, summarization, and report
The extracted data were categorized into topics such as people’s health types and regions of diseases caused by outdoor air exposure. Each reported topic should be provided with a clear explanation to enable future research. Finally, the scoping review results were tabulated in order to find research gaps to either enable meaningful research or obtain good pointers for policymaking.
Stage six: Consultation exercise
Our scoping review took into account the consultation phase of sharing preliminary findings with experts, all of whom are members of the Committee on Public Health and Urban Environment Management in China. This enabled us to identify additional emerging issues related to health outcomes.
The original search was conducted in May of 2018; the Web of Science, PubMed, and Scopus databases were searched, resulting in a total of 5759 potentially relevant studies. After a de-duplication of 1451 studies and the application of the inclusion criteria, 3027 studies were assessed as being irrelevant and excluded based on readings of the titles and the abstracts. In the end, 1281 studies were assessed for in-depth full-text screening. To prevent overlooking potentially relevant papers, we manually screened the top five impact factor periodicals in the database we were searching. We traced the reference lists and the cited literatures of the included studies, and then we reviewed the newly collected literatures to generate more relevant studies. Further, after preliminary consultation with experts, we included studies on two additional health outcome categories, pregnancy and children and mental disorders. Hence, 214 more potential studies were included during this process. Besides, 379 original studies of the inclusive meta-analysis and systematic review studies were removed for duplication. In total, 1116 studies were included for in-depth full-text screening analysis and 799 eligible studies were included in the end. The detailed articles selection process was shown in Fig 1 .
https://doi.org/10.1371/journal.pone.0216550.g001
The included air pollution related health outcome studies increased between 1992 and 2018, as shown in Fig 2 . Most studies were published in the last decade and more than 75% of studies (614/76.9%) were published after 2011.
The included studies increased during this period, more than 75% of studies were published after 2011.
https://doi.org/10.1371/journal.pone.0216550.g002
The general characteristics summary of all included studies are shown in Table 3 . Most studies were carried out in Asia, Europe and North America (280/35.0%, 261/32.7% and 219/27.4%, respectively). According to the category system of journal citation reports (JCR) in the Web of Science, 323/40.4% of all studies on health outcomes came from environmental science, 213/26.7% came from the field of medicine, and 24/3.0% were from economics. The top three research designs of the included studies were cohort studies, systematic reviews and meta-analyses, and time series studies (116/14.5%, 107/13.4% and 76/9.5%, respectively). Almost all included studies were published in journals (794/99.4%). The lengths of the included studies ranged from four pages [ 34 ] to over thirty-nine pages [ 35 ].
https://doi.org/10.1371/journal.pone.0216550.t003
Regions of studies
Table 4 outlines the locations in which health outcomes were affected by outdoor air pollution. The continents of Asia (277/34.7%), Europe (219/27.4%), and North America (168/21.0%) account for most of these studies. As the word cloud in Fig 3 illustrates, most of the included studies had been mainly conducted in the United States and China. About 62.8% of the studies (502) had been especially conducted in developed countries.
https://doi.org/10.1371/journal.pone.0216550.t004
Word cloud representing the country of included studies, the size of each term is in proportion to its frequency.
https://doi.org/10.1371/journal.pone.0216550.g003
Most authors (573/799) evaluated the air pollution health outcomes of their own continent, at a proportion of 71.7%.
Types of air pollution and related health outcomes
We categorized the health outcomes, by consulting with experts, into respiratory diseases, chronic diseases, cardiovascular diseases, health records, cancer, mental disorders, pregnancy and children, and other diseases ( Table 5 ). We also divided the outdoor air pollution into general air pollution gas, fine particulate matter, other hazardous substances, and a mixture of them.
https://doi.org/10.1371/journal.pone.0216550.t005
Most of the health records showed that mortality (163/286; 57.0%) was the most common health outcome related to outdoor air pollution, as is visually represented in Fig 4 . Respiratory diseases (e.g., asthma and respiratory symptoms) and cardiovascular diseases (e.g., heart disease) that resulted from exposure to outdoor air pollution were also common (69/199, 63/199 and 23/90; or 34.7%, 31.7% and 25.6%; respectively).
Word cloud representing the health outcomes of included studies, the size of each term is in proportion to its frequency.
https://doi.org/10.1371/journal.pone.0216550.g004
Types of affected groups
The population of included studies was categorized into seven subgroups: birth and infant, children, women and pregnancy, adults, elderly, all ages and not specified ( Table 6 ). The largest air pollution proportion fell under the groups of all ages and children (261/799; 32.7% and 165/799; 20.7%), health outcomes of respiratory diseases in children account for the largest groups (114/199; 57.3%).
https://doi.org/10.1371/journal.pone.0216550.t006
There were 121 research studies in the “Not Specified” group. As shown in Table 6 , the “Birth & Infant,” “Women & Pregnancy,” “Children,” and “elderly” groups occupied the subject areas of more than half of the total included studies, which means that air pollution affected these population groups more acutely. Moreover, age is a confounding factor for the prevalence of cancer and cardiovascular diseases. However, there were only 2 studies (2/38, 5.3%) on cancer and 14 studies (14/90, 15.6%) on cardiovascular diseases in the elderly group.
Summary of results
Of all included studies, 95.2% reported at least one statistically positive result, 4.4% were convincingly negative, and only 0.4% showed ambiguous results ( Table 7 ).
https://doi.org/10.1371/journal.pone.0216550.t007
There were 27 primary studies that showed no association between air pollution and disease, including cancer (n = 1), chronic diseases (n = 1), cardiovascular diseases (n = 6), health records (n = 7), pregnancy and children (n = 2), respiratory diseases (n = 6), mental disorders (n = 1), and other diseases (n = 3). Moreover, eight meta-analyses showed no evidence for any association between air pollution and disease prevalence (childhood asthma, chronic bronchitis, asthma, cardio-respiratory mortality, acute respiratory distress syndrome and acute lung injury, mental disorder, cardiovascular disease, and daily respiratory death). Three meta-analyses showed ambiguous results for mental health, venous thromboembolism, and hypertension.
Our scoping review provided an overview on the subject of outdoor air pollution and health outcomes. We adhered to the methodology outlined for publishing guidelines and used the six steps outlined by the scoping review protocol. The guiding principle ensured that our methods were transparent and free from potential bias. The strengths of the included studies are that they tend to focus on large sample sizes and broad geographical coverage. This research helped us to identify research gaps and disseminate research findings [ 23 ] to policymakers, practitioners, and consumers for further missing or potentially valuable investigations.
Principal findings
Among the included studies, we identified various health outcomes of outdoor air pollution, including respiratory diseases, chronic diseases, cardiovascular diseases, health records, cancer, mental disorders, pregnancy and children, and other diseases. Among them, asthma in respiratory diseases and mortality in health records were the most common ones. The study designs contained cohort, meta-analysis, time series, crossover, cross-sectional, and other qualitative methods. In addition, we included economically relevant studies [ 12 , 36 , 37 ] to investigate the causal inference of outdoor air pollution on health outcomes. Further, pregnancy and children, mental disorders, and other diseases are health outcomes that might have uncertain or inconsistent effects. For example, Kirrane et al. [ 38 ] reported that PM 2.5 had positive associations with Parkinson’s disease; however, some studies report that there is no statistically significant overall association between PM exposure and such diseases [ 39 ]. Overall, the majority of these studies suggested a potential positive association between outdoor air pollution and health outcomes, although several recent studies revealed no significant correlations [ 40 – 42 ].
Time frame of included studies
The time frame of included studies is one of the most important characteristics of air pollution research. Even in the same country or region, industrialization and modernization caused by air pollution is distinguished between different time periods [ 43 , 44 ]. In addition, the more the public understands environment science, the more people will take preventative measures to protect themselves. This is also influenced by time. Although air pollution should not be seen as an inevitable side effect of economic growth, time period should be considered in future studies. The publication trends with regard to air pollution related health outcome research increased sharply after 2010. In recent times, published studies have begun to pay more attention to controlling confounding factors such as socioeconomic factors and human behavior.
Population and country
More than 50% of the studies on the relationship between air pollution and health outcomes originated from high income countries. There was less research (<25%) from developing countries and poor countries [ 45 – 48 ], which may result from inadequate environmental monitoring systems and public health surveillance systems. Less cohesive policies and inadequate scientific research may be another reason. In this regard, stratified analysis by regional income will be helpful for exploring the real estimates. It is reported that the stroke incidence is largely associated with low and middle income countries rather than with high income countries [ 49 ]. More studies are urgently needed in highly populated regions, such as Eastern Asia and North and Central Africa.
It is worth noting that rural and urban differences in air pollution research have been neglected. There are only eight studies focused on the difference of spatial variability of air pollution [ 50 – 57 ]. Variation is common even across relatively small areas due to geographical, topographical, and meteorological factors. For example, an increase in PM 2.5 in Northern China was predominantly from abundant coal combustion used for heating in the winter months [ 58 ]. These differences should be considered with caution by urbanization and by region. Data analysis adjustment for spatial autocorrelation will provide a more accurate estimate of the differences in air. What’s more, in some countries such as China, migrants are not able to access healthcare within the cities; this has resulted in misleading conclusions about a “healthier” population and null based bias was introduced [ 59 ].
Other studies (including systematic reviews and economic studies) on outdoor air pollution
Our scoping review included a large number of systematic reviews and meta-analyses. Of the included 107 systematic review and meta-analyses, the most discussed topics were respiratory diseases influenced by mixed outdoor air pollution [ 60 – 62 ]. Little systematic review research focused on chronic diseases, cancer, and mental disorders, which are current research gaps and potential research directions. A large overlap remains between the primary studies included in the systematic reviews. However, some systematic reviews that focused on the same topic have conflicting results, which were mainly caused by different inclusion criteria and subgroup analyses [ 63 , 64 ]. To solve this problem, it is critical that reporting of systematic reviews should retrieve all related published systematic reviews and meta-analyses.
As for the 24 included economic studies, two kinds of health outcomes—morbidity [ 65 ] and economic cost [ 66 ]—were discussed separately using regression approaches. The economic methods were different from those used in the epidemiology; the study focused on causal inference and provided a new perspective for examining the relevant environmental health problems. Furthermore, meta-regression methodology, an economic synthesis approach, proved to be very effective for evaluating the outcomes in a comprehensive way [ 67 ].
Diagnostic criteria for diseases
The diagnostic criteria for diseases forms an important aspect of health-related outcomes. The diagnostic criteria for stroke and mental disorders might be less reliable than those for cancer, mobility, and cardiovascular diseases [ 68 ]. Few studies provided detailed disease diagnostic information on how the disease was measured. Thus, the overall effect estimation of outdoor air pollution might be overestimated. It is recommended that ICD-10 or ICD-11 classification should be adopted as the health outcome classification criterion to ensure consistency among studies in different disciplines considered in future research [ 69 ].
In spite of these broad disease definitions, studies in healthy people or individuals with chronic diseases were not conducted separately. People with chronic diseases were more susceptible to air pollution [ 70 ]. It is obvious that air pollution related population mobility might be underestimated. However, the obvious association of long-term exposure to air pollution with chronic disease related mortality has been reported by prospective cohort studies [ 71 ]. It should be translated to other diverse air pollution related effect research. The population with pre-existing diseases should be analyzed as subgroups.
Except for the overall population, subgroups of people with outdoor occupations and athletes [ 72 , 73 ], sensitive groups such as infants and children, older adults [ 74 , 75 ], and people with respiratory or cardiovascular diseases, should be analyzed separately.
Measurement of personal exposure
The measurement of personal exposure to air pollutants (e.g., measurement of errors associated with the monitoring instruments, heterogeneity in the amount of time spent outdoors, and geographic variation) was lacking in terms of accurate determination. There is a need for clear reporting of these measurements. The key criterion to determine if there is causal relationship between air pollution and negative health outcomes was that at least one aspect of these could be measured in an unbiased manner.
Pollutant dispersion factor
It is well known that the association between air pollution and stroke, and respiratory and cardiovascular disease subtype might be caused by many other factors such as temperature, humidity, season, barometric pressure, and even wind speed and rain [ 76 – 78 ]. These confounding factors related to aspects of energy, transportation, and socioeconomic status, may explain the varying effect size of the association between air pollution and diseases.
While the associations reported in epidemiological studies were significant, proving a causal relationship between the different air pollutants affected by any other factors and adverse effects has been more challenging. To avoid bias, these modifier effects should be compared with previous localized studies. In fact, how the confounding variables account for the heterogeneity should be explored by case-controlled study design or other causal interference research designs.
Study limitations
The following limitations should not be overlooked. First, scoping reviews are based on a knowledge synthesis approach that allows for the mapping of gaps in the existing literature; however, they lack quality assessment for the included studies, which may be an obstacle for precise interpretation. Some improvements have been made by adding a quality assessment [ 15 , 22 , 79 ] to increase the reliability of the findings, and other included studies control for quality by including only peer-reviewed publications [ 80 ]; however, this is not a requirement for scoping reviews. While our paper aimed to comprehensively present a broader range of global-level current published literatures related to outdoor air pollution health outcomes, we did not assess the quality of the analyzed literature. The conclusions of this scoping review were based on the existence of the selected studies rather than their intrinsic qualities.
Second, bias is an inevitable problem from the perspectives of languages, disciplines, and literatures in knowledge synthesis. We included literatures from electronic databases, key journals, and reference lists to avoid “selection bias” and then included unpublished literature to avoid “publication bias”; further, we also conscientiously sampled among the studies to ensure that there was a safeguard against “researcher bias.” We only took English language articles into account because of the cost and time involved in translating the material, which might have led to a potential language bias [ 23 ]. However, in scoping reviews, language restriction does not have the importance that it does in meta-analysis [ 81 ].
Conclusions
In all, the topic of outdoor air pollution exposure related health outcomes is discussed across multiple-disciplines. The various characteristics and contexts of different disciplines suggest different underlying mechanisms worth of the attention of researchers and policymakers. The presentation of the diversity of health outcomes and its relationship to outdoor exposure air pollution is the purpose of this scoping review for new findings in future investigations.
Supporting information
S1 table. literature search strategies..
https://doi.org/10.1371/journal.pone.0216550.s001
S2 Table. PRISMA-ScR checklist.
https://doi.org/10.1371/journal.pone.0216550.s002
Acknowledgments
The authors would like to thank Miaomiao Liu, an assistant professor in School of the Environment of Nanjing University, for her valuable advice with regard to this article.
- View Article
- PubMed/NCBI
- Google Scholar
- 24. The Joanna Briggs Institute. The Joanna Briggs Institute Reviewers’ Manual 2015: Methodology for JBI scoping reviews. Joanne Briggs Inst. 2015; 1–24.
- Open access
- Published: 06 November 2008
Health effects of ambient air pollution – recent research development and contemporary methodological challenges
- Cizao Ren 1 , 2 &
- Shilu Tong 1
Environmental Health volume 7 , Article number: 56 ( 2008 ) Cite this article
39k Accesses
57 Citations
1 Altmetric
Metrics details
Exposure to high levels of air pollution can cause a variety of adverse health outcomes. Air quality in developed countries has been generally improved over the last three decades. However, many recent epidemiological studies have consistently shown positive associations between low-level exposure to air pollution and health outcomes. Thus, adverse health effects of air pollution, even at relatively low levels, remain a public concern. This paper aims to provide an overview of recent research development and contemporary methodological challenges in this field and to identify future research directions for air pollution epidemiological studies.
Peer Review reports
Introduction
It is well known that exposure to high levels of air pollution can adversely affect human health. A number of air pollution catastrophes occurred in industrial countries between 1950s and 1970s, such as the London smog of 1952 [ 1 ]. Air quality in western countries has significantly improved since the 1970s. However, adverse health effects of exposure to relatively low level of air pollution remain a public concern, motivated largely by a number of recent epidemiological studies that have shown the positive associations between air pollution and health outcomes using sophisticated time-series and other designs [ 2 ].
This review highlights the key findings from major epidemiological study designs (including time-series, case-crossover, panel, cohort, and birth outcome studies) in estimating the associations of exposure to ambient air pollution with health outcomes over the last two decades, and identifies future research opportunities. We do not intend for this to be a formal systematic literature review or meta-analysis, but to discuss issues we feel are vitally important based on the recent literature and our own experience. This paper is divided into two parts: firstly to summarize recent findings from major epidemiological studies, and secondly to discuss key methodological challenges in this field and to identify research opportunities for future air pollution epidemiological studies.
Health effects of ambient air pollution
Time-series studies.
There are a large number of time-series studies on the short-term health effects of air pollution, with the emphasis on mortality and hospital admissions by means of fitting Poisson regression models at a community level or ecological level. This type of time-series design is a major approach to estimating short-term health effects of air pollution in epidemiological studies for the last two decades. Many studies have found associations between daily changes in ambient particulate air pollution and increased cardiorespiratory hospital admissions [ 3 – 6 ], along with cardiorespiratory mortality [ 7 – 9 ] and all cause mortality [ 10 ]. Because numerous air pollution time-series studies show that exposure to air pollution is associated with different kinds of human health outcomes, it is impossible to list results from all studies. Table 1 only selects major time-series studies on short-term health effects of particulate matter (PM) and ozone from different countries around the world published over the last two decades because these two air pollutants are important toxic agents and widely explored by the majority of air pollution epidemiological studies. Early findings have been systematically and thoroughly reviewed by other authors [ 11 , 12 ].
Single-site time-series studies have been criticized because of exposure measurement errors, substantial variation of the air pollution effects and the heterogeneity of the statistical approaches used in different studies [ 13 ]. Recently, several multi-site time-series studies have been conducted in Europe and the United States. Two large collaborative air pollution projects in Europe and U.S. are summarised below.
In Europe, the APHEA (Air Pollution and Health: a European Approach) studies have provided many new insights. Initial studies were based on older data (APHEA-1) [ 14 ] and a new series of studies (APHEA-2) used data of the PM 10 fraction since the late 1990s [ 15 ]. The APHEA-2 mortality studies covered over 43 million people and 29 European cities, which were all studied for more than 5 years in the 1990s. The combined effect estimate showed that all-cause daily mortality increased by 0.6% (95% CI: 0.4%, 0.8%) for each 10 μg/m 3 increase in PM 10 from data involving 21 cities. It was found that there was heterogeneity between cities with different levels of NO 2 . The estimated increase in daily mortality for an increase of 10 μg/m 3 in PM 10 were 0.2% (95% CI: 0.0%, 0.4%), and 0.8% (95% CI: 0.7%, 0.9%) in cities with low and high average NO 2 , respectively [ 16 ]. The APHEA-2 hospital admission study involved 38 million people living in eight European cities. Hospital admissions for asthma and chronic obstructive pulmonary disease (COPD) increased by 1.0% (95% CI: 0.4%, 1.5%) per 10 μg/m 3 PM 10 increment among people older than 65 years [ 15 ].
In the United States, the National Morbidity, Mortality and Air Pollution Studies (NMMAPS) focused on the 20 largest metropolitan areas in the USA, involving 50 million inhabitants, during 1987–94 [ 2 ]. All-cause mortality was increased by 0.5% (95% CI: 0.1%, 0.9%) for each increase of 10 μg/m 3 in PM 10 . The estimated increase in the relative rate of death from cardiovascular and respiratory disease was 0.7% (95% CI: 0.2%, 1.2%). Effects on hospital admissions were studied in ten cities with a combined population of 1 843 000 individuals older than 65 years [ 17 ]. The model used considered simultaneously the effects of PM 10 up to the lag of 5 days and effects of PM 10 on chronic obstructive pulmonary disease admissions to be 2.5% (95% CI: 1.8%, 3.3%) and on cardiovascular disease admissions to be 1.3% (95% CI: 1.0%, 1.5%) for an increase of 10 μg/m 3 in PM 10 . Bell et al. [ 18 ] analysed 95 NMMAPS community data to examine the association between ozone concentration and mortality, showing that a 10-ppb increase in the previous week's ozone was associated with a 0.5% (95% posterior interval (PI), 0.3%, 0.8%) increase in daily mortality and a 0.64% (95% PI, 0.31%, 0.98%) increase in cardiovascular and respiratory mortality. The effect estimates of the exposure over the previous week were larger than those considering only a single day's exposure. Recently, Dominici et al. [ 13 ] examined the short-term association between fine particulate air pollution and hospital admissions and found that exposure to PM 2.5 was associated with different health outcomes. The largest association was observed for heart failure, and a 10 μg/m 3 increase in PM 2.5 was found to be associated with a 1.3% (95% PI: 0.8%, 1.8%) increase in hospital admissions from heart failure on the same day.
Although time-series studies have shown that day-to-day variations in air pollutant concentrations are associated with daily deaths and hospital admissions, it is still unclear how many days, weeks or months of air pollution have brought such events forward. Harvesting or mortality/morbidity displacement means that some cases are occurring only in those to whom it would have happened in a few days anyway [ 19 ]. If so, the increase in cases immediately after exposure would be offset by a deficit in daily deaths a few days later [ 19 , 20 ]. If air pollution has harvesting effects, normal time-series models are unable to estimate the effects due to the issues of collinearity and statistical power. The polynomial distributed lag (PDL) model [ 21 ] and the time-scale model [ 19 ] have been adopted to explore whether air pollution has harvesting or displacement effects on daily deaths or hospital admissions. A few studies suggested potential harvesting effects of ambient air pollution while other studies have shown that there is no evidence for harvesting effects [ 19 , 22 , 23 ]. Although one study shows that potential bias might occur in PDL model [ 24 ], the estimated effects of ambient air pollutants seem to increase when longer lags of air pollution are included [ 19 , 20 ].
Case-crossover studies
Case-crossover study design is an alternative approach to estimating short-term health effects of air pollution in epidemiological studies. In the last two decades, the case-crossover design has been applied in a large number of studies of air pollution and health [ 25 – 28 ]. For example, Neas et al. [ 27 ] used a case-crossover study design to estimate the association between air pollution and mortality in Philadelphia and found a 100 μg/m 3 increment in the 48 hours mean level of TSP was associated with increased all-cause mortality (odds ratio (OR) = 1.06; 95% CI: 1.03, 1.09). A similar association was observed for deaths in individuals over 65 years of age (OR: 1.07; 95% CI: 1.04, 1.11). Levy et al. [ 28 ] estimated the effect of short-term changes in exposure to particulate matter on the rate of sudden cardiac arrest. The cases were obtained from a previously conducted population-based case-control study and were combined with ambient air monitoring data. The results did not show any evidence of a short-term effect of particulate air pollution on the risk of sudden cardiac arrest in people without previously recognised heart disease. Schwartz [ 26 ] conducted a case-crossover study to examine the sensitivity of the association between ozone and mortality when adjusted for temperature and found that 10-ppb increase of maximum hourly ozone was associated with 0.23% (95% CI: 0.01% ~0.44%) increase in daily deaths after adjusting for temperature in 14 US cities. Barnett et al. [ 25 ] examined the association between air pollution and cardio-respiratory hospital admissions in Australia and New Zealand cities. The results show that air pollution arising from common emission sources was significantly associated with cardiovascular health outcomes in the elderly. For example, for a 0.9-ppm increase in CO, there were significant increases in elderly hospital admissions for 2.2% (95% CI: 0.9%, 3.4%) increase of total cardiovascular disease and 2.8% (95% CI: 1.3%, 4.4%) increase of all cardiac disease.
Panel studies
Many air pollution panel studies have been conducted, including several large longitudinal studies of air pollution and health effects such as the Southern California Children's Health Study [ 29 , 30 ], in which children from grades 4, 7, and 10 residing in twelve communities near Los Angeles were followed annually. The results indicated that exposure to ambient particles, NO 2 , and inorganic acid vapour was associated with reduced lung function in children. Another large panel study, the Pollution Effects on Asthmatic Children in Europe (PEACE), was designed to examine the relationship between short-term changes in air pollution and lung function, respiratory symptoms and medication use [ 31 ]. This project was conducted in 14 centres using a common protocol in the winter of 1993–1994. Each PEACE centre involved an urban and a rural panel of symptomatic children and followed at least seventy-five 6–12 year old children [ 31 ]. The pooled estimates of two literature reviews which were separately conducted about the PEACE study and showed that no clear relation could be established for changes in PM 10 , black smoke, SO 2 and NO 2 and changes in respiratory health. The non-significant effects were thought to be possibly due to the short observation period. Ward and Ayres [ 32 ] reviewed 22 panel studies published in the 1990s to estimate the overall effects of ambient particles on children. Results show that the majority of identified panel studies indicated an adverse effect of particulate air pollution. Several recent panel studies also show that particulate air pollution is associated with human health [ 33 – 37 ].
Cohort studies
Compared to time-series and case-crossover studies, there are only a few large cohort studies. About a dozen cohort studies have been conducted in the United States [ 38 – 44 ], Europe [ 45 – 48 ] and Australia [ 49 ]. A cohort study conducted by Dockery et al. [ 39 ] in six U.S. cities shows that there was a statistically significant and robust association between air pollution and mortality. The adjusted mortality rate ratio for the most polluted city was 1.26 (95% CI: 1.08–1.47) compared with the least polluted city. Air pollution was also associated with deaths from lung cancer and cardiopulmonary diseases. Abbey et al. [ 38 ] conducted a cohort study during 1973–1992 to estimate effect of exposure to long-term ambient concentrations of PM 10 and other air pollutants, and show that PM 10 was strongly associated with mortality from respiratory disease for both sexes adjusting for a wide range of potentially confounding factors. The relative risk (RR) for an interquartile range (IQR) difference of PM 10 was 1.18 (95% CI: 1.42, 3.97). Ozone was strongly associated with lung cancer mortality for males for the IQR difference (RR: 4.19; 95% CI: 1.81, 9.69). Sulphur dioxide was also strongly associated with lung cancer mortality for both sexes. Pope et al. [ 44 ] conducted one cohort study in the US to examine the long-term effect of exposure to fine particulate air pollution. They found that fine particulate and sulphur oxide-related pollution were associated with all-cause, lung cancer and cardiopulmonary mortality. A 10 μg/m 3 increase in fine particulate air pollution was associated with an increase of 4%, 6%, and 8% for all-cause, cardiopulmonary, and lung cancer mortality, respectively. Hoek et al. [ 48 ] investigated a random sample of 5000 people and 489 of 4492 (11%) died during 1986–1994 in the Netherlands fining that cardiopulmonary mortality was associated with living near a major road with relative risk of 1.95 (95% CI: 1.09–3.52). A cohort study conducted by Filleul et al. [ 46 ] in France found that urban air pollution to be associated with increased mortality over 25 years in France. Frostad et al. [ 47 ], in a 30-year follow-up cohort study in Norway, found that respiratory symptoms were a significant predictor of mortality from all causes. In Australia, Jalaludin et al.[ 49 ] enrolled a cohort of primary school children with a history of wheeze (n = 148) in an 11-month longitudinal study to examine the association between ambient air pollution and respiratory morbidity. They found that PM 10 and NO 2 , but not ozone, were significantly associated with doctor visits for asthma.
Birth outcome studies
Even though effects of exposure to ambient air pollution on mortality and hospital admissions have been increasingly demonstrated over the past 30 years, exploring its adverse impact on pregnant outcomes has only begun since the last decade [ 50 ]. Because pregnancy is a period of human development particularly susceptible to the influence of many environmental factors due to high cell proliferation, organ develop and the changes of capabilities of fetal metabolism, the relative short-term period provides a unique opportunity to study the adverse effects of ambient toxins on human health [ 51 ]. The majority of birth outcome studies are based on large datasets routinely collected from air pollution monitoring systems and birth registration processes, and therefore, in general, the statistical power is strong [ 52 – 59 ]. Logistic regression models or linear regression models at the individual level are usually adopted to assess the effects of ambient air pollution on adverse birth outcomes adjusting for potential confounders including maternal age, maternal race, parity, fetal gender, season, gestational period, etc. Birth outcomes usually include low birth weight, preterm delivery and other biomarkers such as birth defect and ultrasound measures of head circumference. Personal exposures are often estimated at different terms, including the full gestation, trimesters, month after the pregnancy or before the time of delivery, etc.
Many studies have shown that there are significant associations between exposure to ambient air pollutants and adverse birth outcomes [ 52 – 60 ]. For example, Liu et al. [ 53 ] found that 5-ppb increase of sulfur dioxide was associated with an 11% (95% CI: 1%, 22%) increase of low birth weight (< 2500 grams) during the first month gestation and with a 9% increase of preterm delivery in Vancouver, Canada. A 1.0 ppm increase of carbon monoxide during the last month of pregnancy was associated with an 8% increase of preterm delivery. Parker et al. [ 60 ] selected population within 5 miles of over 40 air pollution monitoring sites across 28 California counties to estimate the adverse effects of air pollution and found that per 10 μg/m 3 PM increase was associated with 13 g (95% CI: 7.6 g, 18.3 g) decrease of birth weight. Similarly, Ritz et al. [ 59 ] conducted a population-nested case-control study to examine associations between air pollution and birth outcomes in Los Angeles and found that air pollution exposure was associated with preterm birth. Hansen et al. [ 58 ] examined the associations of exposure to ambient air pollution during early pregnancy with fetal ultrasonic measurements during mid-pregnancy in Australia. They found that a reduction in fetal abdominal circumference was associated with exposure to O 3 during the days 31–60 of pregnancy (-1.42 mm, 95% CI: -2.74, -0.09), SO2 during the days 61–90 (-1.67 mm, 95% CI: -2.94, -0.40), and PM 10 during the days 90–120 (-0.78 mm, 95% CI: -1.49, -0.08).
Implications of weak health effects
Even though the association of air pollution with health outcomes is weak, it still has strong public health implications. One reason is that air pollution is ubiquitous and affects the whole population in most metropolitan cities. Another reason is that residents are continuously and permanently exposed to air pollution, which may have both short- and long-term effects on health outcomes. Some intervention studies have shown that the reduction in air pollution has resulted in an improvement in population health [ 55 , 61 ]. For example, Hedley et al. [ 61 ] reported that cardiovascular, respiratory and all cause mortality reduced by 2.0% (p < 0.05), 3.9% (p < 0.05) and 2.1% (p < 0.05) respectively in the first 12 months after an introduction of the restrictions on sulphur content of fuel in Hong Kong.
Contemporary methodological challenges
Air pollution epidemiologic research is challenged by the complexity of human exposure to environmental agents and by the difficulty of accurately measuring exposure. Residents are usually ubiquitously exposed to air pollution. In order to detect small effects of air pollution, both high statistical power and sophisticated study design are required. In addition, the characteristics of air pollutants vary and their concentrations change both spatially and temporally. Although everyone is susceptible to high concentration of pollution, its concentrations are not evenly distributed across populations. Due to such complexities, there are still many research questions to be addressed by future air pollution epidemiological studies. The following section discusses these issues.
Shape of exposure response curve
The shape of the exposure and response curve is very important. A key research question to be addressed is whether a threshold exists below which a certain air pollutant has no effect on population health. If such a threshold could be identified, public health benefits would be expected from bringing the pollutant below this level. Both theoretical and empirical works have been done to shed light on this issue [ 62 , 63 ]. In the analysis of NMMAPS data, no threshold evidence was found for the relationship between PM 10 and daily all-cause and cardiorespiratory mortality [ 63 ]. By contrast, a threshold of about 50 μg/m 3 was indicated for non-cardiorespiratory causes of death – viz, below this point, PM 10 had little influence on non-cardiorespiratory mortality. These issues remain to be clarified.
Model uncertainty and bias
The process of model selection includes how to select covariates (eg, meteorological variables and co-pollutants), lag structure for air pollutants and the number of degrees of freedom for smoothing functions to adjust for long-term trend, short fluctuation, seasonality, other covariates and the determination of referent in case-crossover design. Studies have shown that the model choice will impact on estimates of relative risk [ 64 ]. As a result, many authors attempted to estimate the effects using the best single lag or combination of lags for meteorological factors and/or air pollutants and to identify the best degree of freedom for smoothing to adjust for different potential confounders. Some types of data can use several different models. Some authors do not clearly state why they select models and how they conduct data analyses. For example, when we estimate associations between exposure to air pollution and recurrent asthma episodes, based on different assumptions, at least five survival Cox models could be applied to estimate the associations between exposure to air pollution and asthma episodes [ 65 ]. Different assumptions or models may result in different estimates, and sometimes the difference is considerable. The choice of software options may cause this kind of uncertainty as well [ 65 ].
Results presented by the "best" final models are likely to cause publication bias because stronger and positive estimates tend to be published but negative results are usually difficult to be published. Multi-site time series design in which all data are analysed using the same model is one way to solve this problem. However, model uncertainty still exists in a multi-site study to some extent due to the model choice. Some studies have used Bayesian Model Averaging (BMA) to take into account uncertainties in model choice when making an inference [ 64 ]. BMA uses hierarchical models. The predictions and inferences are based on multiple models rather than a single model. Predictions are obtained by forming weighted averages of predictions over the different models where weights depend on the degree to which the data support each model.
Measurement errors of exposure to air pollution and potential confounders usually exist in air pollution epidemiological studies and it is impossible to be solved in most air pollution studies [ 66 ]. Due to spatial and temporal variations, data obtained in air pollution central monitors are not well representative of individual exposures. Some models are used to assess individual exposure to air pollution [ 66 , 67 ], but they could not efficiently adjust for measurement errors. Therefore, potential misclassification bias of exposure is one of the main concerns in air pollution studies.
There are both spatial and temporal variations for exposure and outcomes in air pollution studies [ 68 ]. Both times-series and case-crossover designs at a community level can efficiently adjust for some measured and unmeasured time-invariant characteristics of the subjects (such as gender, age, smoking status and spatial characteristics) via matching, and therefore, the potential confounding from these measured and unmeasured characteristics is minimised [ 69 , 70 ]. The key concern for these designs is how to control for temporal confounding and meteorological variables, such as seasonality, short-term variations and weather conditions (eg, temperature and humidity). In a prospective cohort study design, a major issue is how to identify a cohort with a sufficient variation in cumulative exposures, particularly when data recorded in central monitoring stations are used to measure ambient air pollution levels [ 44 ]. However, in maximizing the geographical variability of exposure the relative risk estimates from cohort studies are likely to be confounded by area-specific characteristics [ 68 ]. Due to collection of relatively detailed individual characters and sufficient adjustment for potential individual social and economic status, such confounding might be efficiently adjusted for.
Birth outcome studies are mainly based on routinely collected data, including exposure, outcome and potential confounders [ 52 – 60 ]. Most studies use pollution data obtained from the different monitors and the closest residential monitoring data are used as exposure proxies [ 58 , 60 , 71 , 72 ]. In general, information related to birth outcomes is well recorded in birth registration systems. However, the data may not include complete and accurate information on other potential confounders, such as maternal social and economic status and life styles. Birth outcome data analyses are usually conducted at an individual level. Therefore, this design is inherently vulnerable to some potential biases, including both temporal and spatial misclassification bias. Ritz and Wilhelm [ 73 ] has discussed the methodological issues of birth outcome designs in detail, and this review would not repeat these issues but rather than focus on potential bias in relation to spatial variation, which was ignored in their review, in the following section.
In birth outcome studies, both exposure and outcome data include temporal and spatial variations to some extent. The majority of birth outcome studies have adjusted for temporal and other confounders which are related to delivery information, including season, maternal age and race, fetal gender, parity, and maternal education attainment [ 52 – 60 , 71 , 72 ]. However, so far, few studies have paid much attention to the potential spatial confounding. Unlike time-series or case-crossover studies, most birth outcome studies lack the ability for automatic adjustment for measured and unmeasured time-invariant spatial variations. Unlike cohort studies, birth outcome study designs also lack the ability to efficiently adjust for personal life styles and social and economic status due to the lack of the detailed information available in routinely collected data. Because both exposure to air pollution and birth outcomes are influenced by some geographic characteristics, such as land use, forest, public infrastructure, and residential social and economic status, etc, the previous birth outcome studies might introduce bias to some extent due to the failure to consider spatial variation. In general, these spatial-related factors are favourable to links between air pollution exposure and birth outcomes. Therefore, we presume that the stronger associations reported in the previous birth outcome studies might partially attribute to this kind of bias. The simple way to adjust for the spatial variation is to add a categorical variable for individual residential areas to fit a fixed effect model or to include the residential areas to fit a mixed model or a random effect model.
Interaction of temperature and air pollution
In many locations, patterns of air pollution are driven by weather. Therefore, concentrations of air pollutants may be associated with temperature. Therefore, it may be possible that temperature and air pollution interact to affect health outcomes. Although effect modification has important public health implications [ 74 ], this issue has so far received limited attention, probably because of methodological complexity and the difficulty in data interpretation. Several studies examined whether or not ambient air pollution and temperature interact to affect human health outcomes, but they produced conflicting results [ 75 – 78 ]). For example, Samet et al. [ 78 ] investigated the sensitivity of the particulate air pollution mortality effect estimate to alternative methods of controlling weather and did not find any evidence that weather conditions modified the associations of particulate air pollution and sulphur dioxide with mortality, regardless of approaches of synoptic weather conditions. Katsouyanni et al. [ 75 ] used a multiple linear regression to investigate the interaction between air pollution and high temperature during a heat wave in Athens in July 1987. They found that while the main effects of air pollution index were not statistically significant, there was statistically significant synergistic effect between high levels of sulphur dioxide and high temperature (P < 0.05). Roberts [ 77 ] found evidence that the effect of particulate air pollution on mortality might depend on temperature but the synergistic effect was sensitive to the number of degrees of freedom used in confounder adjustments. Recently, we found that temperature and particulate matter symmetrically enhanced the effect [ 76 ]. Since then, several multiple-site studies have found evidence that temperature and air pollutants interacted to impact human health but the nature and magnitude of such an interaction varied with geographic area [ 79 – 82 ]. Thus, further research is needed to examine the interactive effects between air pollutants and temperature on mortality and morbidity, especially in different spatial settings.
Many time-series, case-crossover and panel studies have shown that there are consistent short-term effects of air pollution on health outcomes (hospital admissions or deaths). Some cohort studies have also shown long-term health effects of air pollution. In spite of the weak associations of air pollution with human morbidity or mortality, its public health implications are strong because exposure to air pollution is ubiquitous and widespread. However, there are several key methodological challenges in the estimation of the health effects of low-level exposure to air pollution, such as the shape of the exposure response curve, threshold of air pollution, interactive effects of air pollution and weather conditions, and model uncertainty and potential bias. Future research efforts should focus on these important issues.
Ministry of Health: Mortality and morbidity during the London fog of December 1952. Reports on Public Health and Medical Subjects. 1954, London: HMSO, 95
Google Scholar
Samet J, Dominici J, Curriero F, Coursac I, Zeger SL: Fine particulate air pollution and mortality in 20 US cities, 1987–1994. N Engl J Med. 2000, 343: 1742-1749. 10.1056/NEJM200012143432401.
Article CAS Google Scholar
Anderson H, Ponce de Leon A, Bland J, Bower JS, Strachan DP: Air pollution and daily mortality in London: 1987–92. BMJ. 1996, 312: 665-669.
Burnett B, Brook J, Yung W, Dales RE, Krewski D: Association between ozone and hospitalization for respiratory diseases in 16 Canadian cities. Environ Res. 1997, 72: 24-31. 10.1006/enrs.1996.3685.
Linn W, Szlachcic Y, Gong H, Kinney PL, Berhane KT: Air pollution and daily hospital admissions in metropolitan Los Angeles. Environ Health Perspect. 2000, 108: 427-434. 10.2307/3454383.
Moolgavkar SH, Luebeck EG, Anderson EL: Air pollution and hospital admissions for respiratory causes in Minneapolis-St. Paul and Birmingham. Epidemiology. 1997, 8: 364-370. 10.1097/00001648-199707000-00003.
Hoek G, Brunekreef B, Fischer P, Wijnen JV: The association between air pollution and heart failure, arrhythmia, embolism, thrombosis, and other cardiovascular causes of death in a time series study. Epidemiology. 2001, 12: 355-357. 10.1097/00001648-200105000-00017.
Mar T, Norris G, Koenig J, Larson TV: Associations between air pollution and mortality in Phoenix, 1995–1997. Environ Health Perspect. 2000, 108: 347-353. 10.2307/3454354.
Rossi G, Vigotti MA, Zanobetti A: Air pollution and cause specific mortality in Milan, Italy, 1980–1989. Arch Environ Health. 1999, 54: 158-164.
Roemer W, van Wijnen J: Daily mortality and air pollution along busy streets in Amsterdam, 1987–1998. Epidemiology. 2001, 12: 649-653. 10.1097/00001648-200111000-00012.
Brunekreef B, Holgate ST: Air pollution and health. Lancet. 2002, 360: 1233-1242. 10.1016/S0140-6736(02)11274-8.
Nyberg F, Pershagen G: Epidemiological studies on the health effects of ambient particulate air pollution. Scan J Work Environ Health. 2000, 26 (suppl 1): 49-89.
CAS Google Scholar
Dominici F, Peng R, Bell M, Pham L, McDermott A, Zeger SL, Samet JM: Fine particulate air pollution and hospital admission for cardiovascular and respiratory diseases. JAMA. 2006, 295: 1127-1134. 10.1001/jama.295.10.1127.
Katsouyanni K, Touloumi G, Spix C, Schwartz J, Balducci F, Medina S, Rossi G, Wojtyniak B, Sunyer J, Bacharova L, Schouten JP, Ponka A, Anderson HR: Short-term effects of ambient sulphur dioxide and particulate matter on mortality in 12 European cities: results from time series data from the APHEA project. BMJ. 1997, 314: 1658-1663.
Atkinson R, Anderson H, Sunyer J, Ayres J, Baccini M, Vonk J, Boumghar A, Forastiere F, Forsbert B, Touloumi G, Schwartz J, Katsouyanni K: Acute effects of particulate air pollution on respiratory admissions: results from APHEA 2 project. Am J Respir Crit Care Med. 2001, 164: 1860-1866.
Katsouyanni K, Touloumi G, Samol E, Gryparis A, Tertre AL, Monopolis Y, Rossi G, Zmirou D, Ballester F, Boumghar A, Anderson HR, Wojtyniak B, Paldy A, Braunstein R, Pekkanen J, Schindler C, Schwartzet J: Confounding and effect modification in the short-term effects of ambient particles on total mortality: results from 29 European cities within the APHEA-2 project. Epidemiology. 2001, 12: 521-531. 10.1097/00001648-200109000-00011.
Zanobetti A, Schwartz J, Dockery D: Airborne particles are a risk factor for hospital admissions for heart and lung disease. Environ Health Perspect. 2000, 108: 1071-1077. 10.2307/3434961.
Bell M, McDermott A, Zeger S, Samet JM, Dominici F: Ozone and short-term mortality in 95 US urban communities, 1987–2000. JAMA. 2004, 292: 2372-2378. 10.1001/jama.292.19.2372.
Zanobetti A, Schwartz J, Samoli E, Gryparis A, Touloumi G, Atkinson R, Tertre AL, Bobros J, Celko M, Goren A, Forsberg B, Michelozzi P, Rabczenko D, Ruiz EA, Katsouyanni K: The temporal pattern of mortality responses to air pollution: a multicity assessment of mortality displacement. Epidemiology. 2002, 13: 87-89. 10.1097/00001648-200201000-00014.
Article Google Scholar
Schwartz J: Is there harvesting in the association of airborne particles with daily deaths and hospital admission?. Epidemiology. 2001, 12: 55-61. 10.1097/00001648-200101000-00010.
Schwartz J: The distributed lag between air pollution and daily deaths. Epidemiology. 2000, 11: 320-326. 10.1097/00001648-200005000-00016.
Zanobetti A, Schwartz J, Samoli E, Gryparis A, Touloumi G, Peacock J, Anderson RH, Lertre AL, Bobros J, Celko M, Goren A, Forsberg B, Michelozzi P, Rabczenko D, Hoyos SP, Wichmann HE, Katsouyanni K: The temporal pattern of respiratory and heart disease mortality in response to air pollution. Environ Health Perspect. 2003, 111 (9): 1188-1193.
Goodman PG, Dockery DW, Clancy L: Cause-specific mortality and the extended effects of particulate pollution and temperature exposure. Environ Health Perspect. 2004, 112: 179-185.
Roberts S, Switzer P: Mortality Displacement and Distributed Lag Models. Inhal Toxicol. 2004, 16: 879-888. 10.1080/08958370490519598.
Barnett A, Williams G, Schwartz J, Best TL, Neller AH, Petroeschevsky AL, Simpson RW: The effects of air pollution on hospitalizations for cardiovascular disease in elderly people in Australia and New Zealand cities. Environ Health Perspect. 2006, 114: 1018-1023.
Schwartz J: How sensitive is the association between ozone and daily deaths to control for temperature?. Am J Respir Crit Care Med. 2005, 171: 627-631. 10.1164/rccm.200407-933OC.
Neas L, Schwartz J, Dockery D: A case-crossover analysis of air pollution and mortality in Philadelphia. Environ Health Perspect. 1999, 107: 629-631. 10.2307/3434453.
Levy D, Sheppard L, Checkoway H, Kaufman J, Lumley T, Koenig J, Siscovick D: A case-crossover analysis of particulate amtter air pollution and out-of-hospital primary cardiac arrest. Epidemiology. 2001, 12: 193-199. 10.1097/00001648-200103000-00011.
Guaderman W, McConnell R, Gilliland F, London S, Thomas D, Avol E, Vora H, Berhane K, Rappaport EB, Lurmann F, Margolis HG, Peters J: Association between air pollution and lung function growth in southern California children. Am J Respir Crit Care Med. 2000, 162: 1383-1390.
Peters J, Avol E, Gaundman W, Linn WS, Navidi W, London SJ, Margolis H, Rappaport E, Vora H, Gong H, Thomas DC: A study of twelve southern California communities with differing levels and types of air pollution: II. Effects on pulmonary function. Am J Respir Crit Care Med. 1999, 159: 768-775.
Roemer W, Hoek G, Brunekreef B: Pollution effects on asthmatic children in Europe, the PEACE study. Clinical and Experimental Allergy. 2000, 30: 1067-1075. 10.1046/j.1365-2222.2000.00851.x.
Ward D, Ayres J: Particulate air pollution and panel studies in children: a systematically review. Occup Environ Med. 2004, 61: e13-10.1136/oem.2003.007088.
Lagorio S, Forastiere F, Pistelli R, Iavarone I, Michelozzi P, Fano V, Marconi A, Ziemacki G, Ostro BD: Air pollution and lung function among susceptible adult subjects: a panel study. Environ Health. 2006, 5: 11-10.1186/1476-069X-5-11.
Mar T, Koenig J, Jansen K, Sullivan J, Kaufman J, Trenga CA, Siahpush SH, Liu LS, Neas L: Fine particulate air pollution and cardiorespiratory effects in the elderly. Epidemiology. 2005, 16: 681-687. 10.1097/01.ede.0000173037.83211.d6.
Pope CA, Hansen ML, Long RW, Nielsen KR, Eatough NL, Wilson WE, Eatough DJ: Ambient particulate air pollution, heart rate variability, and blood markers of inflammation in a panel of elderly subjects. Environ Health Perspect. 2004, 112: 339-345.
Trenga CA, Sullivan JH, Schilderout JS, Shepherd KP, Shapiro GG, Liu LJS, Kaufman JD, Koenig JQ: Effect of particulate air pollution on lung function in a Seattle panel study. Chest. 2006, 129: 1614-1622. 10.1378/chest.129.6.1614.
Zanobetti A, Canner M, Stone P, Schwartz J, Sher D, Eagan-Bengston E, Gates KA, Hartley LH, Suh H, Gold DR: Ambient pollution and blood pressure in cardiac rehabilitation patients. Circulation. 2004, 110: 2184-2189. 10.1161/01.CIR.0000143831.33243.D8.
Abbey D, Nishino N, McDonnell WF, Burchetter PJ, Knutsen SF, Beeson WL, Yang JX: Long-term inhalable particles and other air pollutants related to mortality in nonsmokers. Am J Respir Crit Care Med. 1999, 159: 373-382.
Dockery DW, Pope C, Spengler JD, Ware JH, Fay ME, Ferris BG, Speizer FE: An association between air pollution and mortality in six U.S. cities. N Engl J Med. 1993, 329: 1753-1759. 10.1056/NEJM199312093292401.
Enstrom J: Fine particulate air pollution and total mortality among elderly Californians, 1973–2002. Inhal Toxicol. 2005, 17: 803-816. 10.1080/08958370500240413.
Lipfert F, Perry H, Miler J, Baty JD, Wyzga RE, Carmody SE: Air pollution, blood pressure, and their long-term associations with mortality. Inhal Toxicol. 2003, 15: 493-512. 10.1080/08958370304463.
McConnell R, Berhane K, Gilliland F, London SJ, Islam T, Gauderman WJ, Avol E, Margolis HG, Peters JM: Asthma in exercising children exposed to ozone: a cohort study. Lancet. 2002, 359: 386-391. 10.1016/S0140-6736(02)07597-9.
Pope C, Thun M, Namboodiri M, Dockery DW, Evans JS, Speizer FE, Heath CW: Particulate air pollution as a predictor of mortality in a prospective study of U.S. adults. Am J Respir Crit Care Med. 1995, 151 (3 Pt 1): 669-674.
Pope C, Burnett R, Thun M, Calle EE, Krewski D, Ito K, Thurston GD: Lung cancer, cardiopulmonary mortality, and long-term exposure to fine particulate air pollution. JAMA. 2002, 287: 1132-1141. 10.1001/jama.287.9.1132.
Clancy L, Goodman P, Sinclair H, Dockery DW: Effect of air pollution control on death in Dublin, Ireland: an intervention study. Lancet. 2002, 360: 1210-1214. 10.1016/S0140-6736(02)11281-5.
Filleul L, Rondeau V, Vandentorren S, Moual NL, Cantagrel A, Annesi-Maesano I, Charpin D, Declercq C, Neukirch F, Paris C, Vervloet D, Brochard P, Tessier JF, Kauffmann F, Baldiet I: Twenty five year mortality and air pollution: results from French PAARC survey. Occup Environ Med. 2005, 62: 453-460. 10.1136/oem.2004.014746.
Frostad A, Søyseth V, Andersen A, Gulsvik A: Respiratory symptoms as predictors of all-cause mortality in an urban community: a 30-year follow-up. J Int Med. 2006, 259: 520-529. 10.1111/j.1365-2796.2006.01631.x.
Hoek G, Brunekreef B, Goldbohm S, Fischer P, Brandt PA: Association between mortality and indicators of traffic-related air pollution in the Netherlands. Lancet. 2002, 360: 1203-1209. 10.1016/S0140-6736(02)11280-3.
Jalaludin B, O'Toole B, Leeder S: Acute effects of urban ambient air pollution on respiratory symptoms, asthma medication use, and doctor visits for asthma in a cohort of Australia children. Environ Res. 2004, 95: 32-42. 10.1016/S0013-9351(03)00038-0.
Šrám RJ, Binková B, Dejmek J, Bobak M: Ambient air pollution and pregnancy outcomes: a review of the literature. Environ Health Perspect. 2005, 113 (4): 375-382.
Selevan SG, Kimmel CA, Mendola P: Identifying Critical Windows of Exposure for Children's Health. Environ Health Perspect. 2000, 108 (suppl): 451-455. 10.2307/3454536.
Maisonet M, Bush TJ, Correa A, Jaakkol JJ: Relation between ambient air pollution and low birth weight in the northeastern United States. Environ Health Perspect. 2001, 109 (suppl 3): 351-356. 10.2307/3434782.
Liu S, Krewski D, Shi Y, Chen Y, Burnett RT: Association between gaseous ambient air pollutants and adverse pregnancy outcomes in Vancouver, Canada. Environ Health Perspect. 2003, 111: 1773-1778.
Woodruff TJ, Parker JD, Schoendorf KC: Fine particulate matter (PM 2.5 ) air pollution and selected causes of postneonatal infant mortality in California. Environ Health Perspect. 2006, 114: 786-790.
Hansen C, Neller A, Williams G, Simpson R: Maternal exposure to low levels of ambient air pollution and preterm birth in Brisbane, Australia. BJOG. 2006, 113: 935-941. 10.1111/j.1471-0528.2006.01010.x.
Slama R, Morgenstern V, Cyrys J, Zutavern A, Herbarth O, Wichmann HE, Heinrich J, the LISA Study Group: Traffic-related atmospheric pollutants levels during pregnancy and offspring's term birth weight: a study relying on a land-use regression exposure model. Environ Health Perspect. 2007, 115: 1283-1292.
Bell ML, Ebisu K, Belanger K: Ambient air pollution and low birth weight in Connecticut and Massachusetts. Environ Health Perspect. 2007, 115: 1118-1125.
Hansen CA, Barnett AG, Pritchard G: The effect of ambient air pollution during early pregnancy on fetal ultrasonic measurements during mid-pregnancy. Environ Health Perspect. 2008, 116: 362-369.
Ritz B, Wilhelm M, Hoggatt KJ, Ghosh JKC: Ambient air pollution and preterm birth in the environment and pregnancy outcomes study at the University of California, Los Angles. Am J Epidemiol. 2007, 166: 1045-1052. 10.1093/aje/kwm181.
Parker JD, Woodruff TJ, Basu R, Schoendorf KC: Air pollution and birth weight among term infants in California. Pediatrics. 2005, 115: 121-128.
Hedley A, Wong C, Thach T, Ma S, Lam TH, Anderson HR: Cardiovascular and all-cause mortality after restrictions on sulphur content of fuel in Hong Kong: an intervention study. Lancet. 2002, 360: 1646-1652. 10.1016/S0140-6736(02)11612-6.
Cakmak S, Burnett R, Krewski D: Methods for detecting and estimating population threshold concentrations for air pollution-related mortality with exposure measurement error. Risk Anal. 1999, 19: 487-496.
Daniels M, Dominci F, Samet J, Zeger SL: Estimating particulate matter-mortality dose-response curves and threshold levels: an analysis of daily time-series for the 20 largest US cities. Am J Epidemiol. 2000, 152: 397-406. 10.1093/aje/152.5.397.
Clyde M: Model uncertainty and health effect studies for particulate matter. Environmetrics. 2000, 11: 745-763. 10.1002/1099-095X(200011/12)11:6<745::AID-ENV431>3.0.CO;2-N.
SAS Institute Inc: SAS/STATE 9.1 User's Guide. 2004, Cary, NC: SAS Institute Inc, 3213-3332.
Sarnat JA, Wilson WE, Strand M, Brook J, Wyzga R, Lumley T: Panel discussion review: session one – exposure assessment and related errors in air pollution epidemiologic studies. J Expo Sci Environ Epidemiol. 2007, 17: s75-s82. 10.1038/sj.jes.7500621.
Jerret M, Arain A, Kanaroglou P, Beckerman B, Potoglou D, Sahsuvaroglu T, Morrison J, Giovis C: A review and evaluation of intraurban air pollution exposure models. J Expo Anal Environ Epidemiol. 2005, 15: 185-204. 10.1038/sj.jea.7500388.
Dominici F, Sheppard L, Clyde M: Health effects of air pollution: a statistical review. International Statistic Review. 2003, 71: 243-276.
Maclure M: The case-crossover design: a method for study transient effects on the risk of acute events. Am J Epidemiol. 1991, 133: 144-153.
Hastie TJ, Tibshirani RJ: Generalized Additive Models. 1990, New York: Chapman & Hall
Ritz B, Yu F: The Effect of Ambient Carbon Monoxide on Low Birth Weight among Children Born in Southern California between 1989 and 1993. Environ Health Perspect. 1999, 107: 17-25. 10.2307/3434285.
Wilhelm M, Ritz B: Local variations in CO and particulate air pollution and adverse birth outcomes in Los Angeles County, California, USA. Environ Health Perspect. 2005, 113: 1212-1221.
Ritz B, Wilhelm M: Ambient air pollution and adverse birth outcomes: methodological issues in an emerging field. Basic Clin Pharmacol Toxicol. 2008, 102: 182-190.
Rotheman KJ, Greenland S: Modern Epidemiology. 1998, New York: Lippincott Williams & Wilkins, 2
Katsouyanni K, Pantazopoulou A, Touloumi G: Evidence for interaction between air pollution and high temperature in the causation of excess mortality. Arch Environ Health. 1993, 48: 235-242.
Ren C, Tong S: Temperature modified he health effects of particulate matter in Brisbane, Australia. Int j Biometeorol. 2006, 51: 87-96. 10.1007/s00484-006-0054-7.
Roberts S: Interactions between particulate air pollution and temperature in air pollution mortality time series studies. Environ Res. 2004, 96: 328-337. 10.1016/j.envres.2004.01.015.
Samet J, Zeger S, Kelshall J, Xu J, Kalkstein L: Does weather confound or modify the association of particulate air pollution with mortality? An analysis of the Philadelphia data, 1973–1980. Environ Res. 1998, 77: 9-19. 10.1006/enrs.1997.3821.
Ren C, Williams GM, Mengersen K, Morawska L, Tong S: Does temperature modify short-term effects of ozone on total mortality in 60 large eastern US communities? – an assessment using NMMAPS data. Environ Int. 2008, 34: 451-458. 10.1016/j.envint.2007.10.001.
Stafoggia M, Schwartz J, Forastiere F, Perucci CA, the SISTI group: Does temperature modify the association between air pollution and mortality? A multicity case-crossover analysis in Italy. Am J Epidemiol. 2008, 167: 1476-1485. 10.1093/aje/kwn074.
Ren C, Williams G, Tong S: Does Particulate Matter Modify the Association between Temperature and Cardiorespiratory Diseases?. Environ Health Perspect. 2006, 114: 1690-1696.
Ren C, Williams GM, Morawska L, Mengersen K, Tong S: Ozone modifies associations between temperature and cardiovascular mortality: analysis of the NMMAPS data. Occup Environ Med. 2008, 65: 255-260. 10.1136/oem.2007.033878.
Peters A, Skorkovsky J, Kotesovec F, Brynda J, Spix C, Wichmann HE, Joachim Heinrich J: Associations between mortality and air pollution in central Europe. Environ Health Perspect. 2000, 108: 283-287. 10.2307/3454344.
Schwartz J: Assessing confounding, effect modification, and threshold in the association between ambient particles and daily deaths. Environ Health Perspect. 2000, 108: 563-568. 10.2307/3454620.
Hales S, Salmond C, Town GI, Kjellstrom T, Woodward A: Daily mortality in relation to weather and air pollution in Christchurch, New Zealand. Aust ZN Public Health. 2000, 24: 89-91. 10.1111/j.1467-842X.2000.tb00731.x.
Wong TW, Tam WS, Yu TS, Wong AHS: Associations between daily mortalities from respiratory and cardiovascular diseases and air pollution in Hong Kong, China. Occup Environ Med. 2002, 59: 30-35. 10.1136/oem.59.1.30.
Kim H, Kim Y, Hong YC: The lag-effect pattern in the relationship of particulate air pollution to daily mortality in Seoul, Korea. Int J Biometeorol. 2003, 48: 25-30. 10.1007/s00484-003-0176-0.
Kan H, Chen B: Air pollution and daily mortality in Shanghai: a time-series study. Arch Environ Health. 2003, 58: 360-367.
Petroeschevsky A, Simpson RW, Thalib L, Rutherford S: Associations between outdoor air pollution and hospital admissions in Brisbane, Australia. Arch Environ Health. 2001, 56: 37-52.
Braga AL, Saldiva PH, Pereira LA, Menezes JC, Conceicai JM, Lin CA, Zanobetti A, Schwartz J, Dockery DW: Health effects of air pollution exposure on children and adolescents in Sao Paulo, Brazil. Pediatr Pulmonol. 2001, 31: 106-113. 10.1002/1099-0496(200102)31:2<106::AID-PPUL1017>3.0.CO;2-M.
Download references
Acknowledgements
We thank Prof. Gail Williams, School of Population Health, University of Queensland for the comments on the earlier version of the manuscript. We also thank two reviewers for their insightful and constructive comments.
Author information
Authors and affiliations.
School of Public Health and Institute of Health and Biomedical Innovation, Queensland University of Technology, Kelvin Grove, QLD 4059, Australia
Cizao Ren & Shilu Tong
Harvard School of Public Health, Exposure, Epidemiology and Risk Program, Landmark Center West, Suite 415, 401 Park Dr, Boston, MA 02215, USA
You can also search for this author in PubMed Google Scholar
Corresponding author
Correspondence to Cizao Ren .
Additional information
Competing interests.
The authors declare that they have no competing interests.
Authors' contributions
CR conceived of the study, participated in its design, and is responsible for the draft of the manuscript. ST participated in the study design and revised the draft of the manuscript.
Rights and permissions
This article is published under license to BioMed Central Ltd. This is an Open Access article distributed under the terms of the Creative Commons Attribution License ( http://creativecommons.org/licenses/by/2.0 ), which permits unrestricted use, distribution, and reproduction in any medium, provided the original work is properly cited.
Reprints and permissions
About this article
Cite this article.
Ren, C., Tong, S. Health effects of ambient air pollution – recent research development and contemporary methodological challenges. Environ Health 7 , 56 (2008). https://doi.org/10.1186/1476-069X-7-56
Download citation
Received : 29 January 2008
Accepted : 06 November 2008
Published : 06 November 2008
DOI : https://doi.org/10.1186/1476-069X-7-56
Share this article
Anyone you share the following link with will be able to read this content:
Sorry, a shareable link is not currently available for this article.
Provided by the Springer Nature SharedIt content-sharing initiative
- Birth Outcome
- Bayesian Model Average
- Lung Cancer Mortality
- Chronic Obstructive Pulmonary Disease Admission
- Birth Outcome Study
Environmental Health
ISSN: 1476-069X
- General enquiries: [email protected]
ENCYCLOPEDIC ENTRY
Air pollution.
Air pollution consists of chemicals or particles in the air that can harm the health of humans, animals, and plants. It also damages buildings.
Biology, Ecology, Earth Science, Geography
Loading ...

Air pollution consists of chemicals or particles in the air that can harm the health of humans, animals, and plants. It also damages buildings. Pollutants in the air take many forms. They can be gases , solid particles, or liquid droplets. Sources of Air Pollution Pollution enters the Earth's atmosphere in many different ways. Most air pollution is created by people, taking the form of emissions from factories, cars, planes, or aerosol cans . Second-hand cigarette smoke is also considered air pollution. These man-made sources of pollution are called anthropogenic sources . Some types of air pollution, such as smoke from wildfires or ash from volcanoes , occur naturally. These are called natural sources . Air pollution is most common in large cities where emissions from many different sources are concentrated . Sometimes, mountains or tall buildings prevent air pollution from spreading out. This air pollution often appears as a cloud making the air murky. It is called smog . The word "smog" comes from combining the words "smoke" and " fog ." Large cities in poor and developing nations tend to have more air pollution than cities in developed nations. According to the World Health Organization (WHO) , some of the worlds most polluted cities are Karachi, Pakistan; New Delhi, India; Beijing, China; Lima, Peru; and Cairo, Egypt. However, many developed nations also have air pollution problems. Los Angeles, California, is nicknamed Smog City. Indoor Air Pollution Air pollution is usually thought of as smoke from large factories or exhaust from vehicles. But there are many types of indoor air pollution as well. Heating a house by burning substances such as kerosene , wood, and coal can contaminate the air inside the house. Ash and smoke make breathing difficult, and they can stick to walls, food, and clothing. Naturally-occurring radon gas, a cancer -causing material, can also build up in homes. Radon is released through the surface of the Earth. Inexpensive systems installed by professionals can reduce radon levels. Some construction materials, including insulation , are also dangerous to people's health. In addition, ventilation , or air movement, in homes and rooms can lead to the spread of toxic mold . A single colony of mold may exist in a damp, cool place in a house, such as between walls. The mold's spores enter the air and spread throughout the house. People can become sick from breathing in the spores. Effects On Humans People experience a wide range of health effects from being exposed to air pollution. Effects can be broken down into short-term effects and long-term effects . Short-term effects, which are temporary , include illnesses such as pneumonia or bronchitis . They also include discomfort such as irritation to the nose, throat, eyes, or skin. Air pollution can also cause headaches, dizziness, and nausea . Bad smells made by factories, garbage , or sewer systems are considered air pollution, too. These odors are less serious but still unpleasant . Long-term effects of air pollution can last for years or for an entire lifetime. They can even lead to a person's death. Long-term health effects from air pollution include heart disease , lung cancer, and respiratory diseases such as emphysema . Air pollution can also cause long-term damage to people's nerves , brain, kidneys , liver , and other organs. Some scientists suspect air pollutants cause birth defects . Nearly 2.5 million people die worldwide each year from the effects of outdoor or indoor air pollution. People react differently to different types of air pollution. Young children and older adults, whose immune systems tend to be weaker, are often more sensitive to pollution. Conditions such as asthma , heart disease, and lung disease can be made worse by exposure to air pollution. The length of exposure and amount and type of pollutants are also factors. Effects On The Environment Like people, animals, and plants, entire ecosystems can suffer effects from air pollution. Haze , like smog, is a visible type of air pollution that obscures shapes and colors. Hazy air pollution can even muffle sounds. Air pollution particles eventually fall back to Earth. Air pollution can directly contaminate the surface of bodies of water and soil . This can kill crops or reduce their yield . It can kill young trees and other plants. Sulfur dioxide and nitrogen oxide particles in the air, can create acid rain when they mix with water and oxygen in the atmosphere. These air pollutants come mostly from coal-fired power plants and motor vehicles . When acid rain falls to Earth, it damages plants by changing soil composition ; degrades water quality in rivers, lakes and streams; damages crops; and can cause buildings and monuments to decay . Like humans, animals can suffer health effects from exposure to air pollution. Birth defects, diseases, and lower reproductive rates have all been attributed to air pollution. Global Warming Global warming is an environmental phenomenon caused by natural and anthropogenic air pollution. It refers to rising air and ocean temperatures around the world. This temperature rise is at least partially caused by an increase in the amount of greenhouse gases in the atmosphere. Greenhouse gases trap heat energy in the Earths atmosphere. (Usually, more of Earths heat escapes into space.) Carbon dioxide is a greenhouse gas that has had the biggest effect on global warming. Carbon dioxide is emitted into the atmosphere by burning fossil fuels (coal, gasoline , and natural gas ). Humans have come to rely on fossil fuels to power cars and planes, heat homes, and run factories. Doing these things pollutes the air with carbon dioxide. Other greenhouse gases emitted by natural and artificial sources also include methane , nitrous oxide , and fluorinated gases. Methane is a major emission from coal plants and agricultural processes. Nitrous oxide is a common emission from industrial factories, agriculture, and the burning of fossil fuels in cars. Fluorinated gases, such as hydrofluorocarbons , are emitted by industry. Fluorinated gases are often used instead of gases such as chlorofluorocarbons (CFCs). CFCs have been outlawed in many places because they deplete the ozone layer . Worldwide, many countries have taken steps to reduce or limit greenhouse gas emissions to combat global warming. The Kyoto Protocol , first adopted in Kyoto, Japan, in 1997, is an agreement between 183 countries that they will work to reduce their carbon dioxide emissions. The United States has not signed that treaty . Regulation In addition to the international Kyoto Protocol, most developed nations have adopted laws to regulate emissions and reduce air pollution. In the United States, debate is under way about a system called cap and trade to limit emissions. This system would cap, or place a limit, on the amount of pollution a company is allowed. Companies that exceeded their cap would have to pay. Companies that polluted less than their cap could trade or sell their remaining pollution allowance to other companies. Cap and trade would essentially pay companies to limit pollution. In 2006 the World Health Organization issued new Air Quality Guidelines. The WHOs guidelines are tougher than most individual countries existing guidelines. The WHO guidelines aim to reduce air pollution-related deaths by 15 percent a year. Reduction Anybody can take steps to reduce air pollution. Millions of people every day make simple changes in their lives to do this. Taking public transportation instead of driving a car, or riding a bike instead of traveling in carbon dioxide-emitting vehicles are a couple of ways to reduce air pollution. Avoiding aerosol cans, recycling yard trimmings instead of burning them, and not smoking cigarettes are others.
Downwinders The United States conducted tests of nuclear weapons at the Nevada Test Site in southern Nevada in the 1950s. These tests sent invisible radioactive particles into the atmosphere. These air pollution particles traveled with wind currents, eventually falling to Earth, sometimes hundreds of miles away in states including Idaho, Utah, Arizona, and Washington. These areas were considered to be "downwind" from the Nevada Test Site. Decades later, people living in those downwind areascalled "downwinders"began developing cancer at above-normal rates. In 1990, the U.S. government passed the Radiation Exposure Compensation Act. This law entitles some downwinders to payments of $50,000.
Greenhouse Gases There are five major greenhouse gases in Earth's atmosphere.
- water vapor
- carbon dioxide
- nitrous oxide
London Smog What has come to be known as the London Smog of 1952, or the Great Smog of 1952, was a four-day incident that sickened 100,000 people and caused as many as 12,000 deaths. Very cold weather in December 1952 led residents of London, England, to burn more coal to keep warm. Smoke and other pollutants became trapped by a thick fog that settled over the city. The polluted fog became so thick that people could only see a few meters in front of them.
Media Credits
The audio, illustrations, photos, and videos are credited beneath the media asset, except for promotional images, which generally link to another page that contains the media credit. The Rights Holder for media is the person or group credited.
Illustrators
Educator reviewer, last updated.
March 6, 2024
User Permissions
For information on user permissions, please read our Terms of Service. If you have questions about how to cite anything on our website in your project or classroom presentation, please contact your teacher. They will best know the preferred format. When you reach out to them, you will need the page title, URL, and the date you accessed the resource.
If a media asset is downloadable, a download button appears in the corner of the media viewer. If no button appears, you cannot download or save the media.
Text on this page is printable and can be used according to our Terms of Service .
Interactives
Any interactives on this page can only be played while you are visiting our website. You cannot download interactives.
Related Resources

An official website of the United States government
The .gov means it’s official. Federal government websites often end in .gov or .mil. Before sharing sensitive information, make sure you’re on a federal government site.
The site is secure. The https:// ensures that you are connecting to the official website and that any information you provide is encrypted and transmitted securely.
- Publications
- Account settings
Preview improvements coming to the PMC website in October 2024. Learn More or Try it out now .
- Advanced Search
- Journal List
- Int J Environ Res Public Health

Indoor Air Pollution, Related Human Diseases, and Recent Trends in the Control and Improvement of Indoor Air Quality
Vinh van tran.
1 Department of BioNano Technology, Gachon University, 1342 Seongnam-Daero, Sujeong-Gu, Seongnam-Si, Gyeonggi-do 13120, Korea; moc.liamg@03ktmhkhnivnav
2 Institute of Research and Development, Duy Tan University, Da Nang 550000, Vietnam
Duckshin Park
3 Korea Railroad Research Institute (KRRI), 176 Cheoldobakmulkwan-ro, Uiwang-si 16105, Gyeonggi-do, Korea
Young-Chul Lee
Indoor air pollution (IAP) is a serious threat to human health, causing millions of deaths each year. A plethora of pollutants can result in IAP; therefore, it is very important to identify their main sources and concentrations and to devise strategies for the control and enhancement of indoor air quality (IAQ). Herein, we provide a critical review and evaluation of the major sources of major pollutant emissions, their health effects, and issues related to IAP-based illnesses, including sick building syndrome (SBS) and building-related illness (BRI). In addition, the strategies and approaches for control and reduction of pollutant concentrations are pointed out, and the recent trends in efforts to resolve and improve IAQ, with their respective advantages and potentials, are summarized. It is predicted that the development of novel materials for sensors, IAQ-monitoring systems, and smart homes is a promising strategy for control and enhancement of IAQ in the future.
1. Introduction
Indoor environment conditions contribute greatly to human wellbeing, as most people spend around 90% of their time indoors, mainly at home or in the workplace [ 1 ]. According to the World Health Organization (WHO), indoor air pollution (IAP) is responsible for the deaths of 3.8 million people annually [ 2 ]. IAP can be generated inside homes or buildings through occupants’ activities, such as cooking, smoking, use of electronic machines, use of consumer products, or emission from building materials. Harmful pollutants inside buildings include carbon monoxide (CO), volatile organic compounds (VOCs), particulate matter (PM), aerosol, biological pollutants, and others [ 3 ]. Therefore, over the past decade, research on air quality control has begun to shift from outdoor to indoor environments, as reflects lifestyle changes linked to increased levels of urbanization [ 4 ]. It was indicated that decreased IAQ can negatively affect human health by causing building-associated illness [ 5 ]. Both short- and long-term IAP exposure can cause a wide range of diseases [ 6 ]. Therefore, the development of monitoring systems has a vital role to play in IAQ control.
IAP normally is a complex mixture of particulate and various gaseous components. IAP compositions differ significantly depending on sources, emission rates, and ventilation conditions [ 7 ]. For effective control of IAQ, therefore, it is necessary to determine the sources of air pollution. Moreover, the development of monitoring systems for the measurement of indoor pollutant concentrations as well as key strategies for control and enhancement of IAQ are considered essential. In this paper, we provide a comprehensive overview of the major IAP sources and IAQ-control strategies; we emphasize the sources, characteristics, and health effects of each IAP; we identify and discuss health issues and building-associated illnesses related to an IAQ decrease; and finally, we present the recent and trending strategies for the control and reduction of pollutant concentrations and better IAQ. It is expected that promising strategies for monitoring and control of IAQ in the future will include novel materials-based sensors, smart monitoring systems, and smart homes.
2. Indoor Air Quality (IAQ) and Indoor Air Pollution (IAP)
According to the EPA’s definition, IAQ is the air quality within and around buildings and structures, especially as it relates to the health and comfort of building occupants [ 8 ]. IAP, meanwhile, refers to the existence of pollutants, such as volatile organic compounds (VOCs), particulate matter (PM), inorganic compounds, physical chemicals, and biological factors, all of which are at high concentrations in the indoor air of non-industrial buildings, and all of which can have negative impacts on the human body. In order to protect people from such pollutants, IAQ has emerged and been developed as a research field [ 9 ]. The main parameters for evaluation of IAQ include pollutant concentrations, thermal conditions (temperature, airflow, relative humidity), light, and noise. Thermal conditions are crucial aspects of IAQ, for two basic reasons [ 10 , 11 ]: (i) Several problems related to poor IAQ can be solved simply by adjusting the relative humidity or temperature, and (ii) building materials in high-temperature buildings can be highly released.
It has been indicated that IAQ in residential areas or buildings is significantly affected by three primary factors [ 12 , 13 ]: (i) Outdoor air quality, (ii) human activity in buildings, and (iii) building and construction materials, equipment, and furniture. It is known that outdoor contaminant concentrations and building airtightness have a great influence on IAQ, due to the possibility of transportation of contaminants from outdoors to indoors [ 14 ]. As outdoor pollutants’ concentrations increase, they are transported from outdoors to the indoor environment via ventilation. Hence, the correlation of outdoor air pollution with IAQ highly depends on the ventilation rate additionally to the lifetimes and mixing ratios of such pollutants [ 15 ]. Human daily activities generally cause IAP by the discharge of waste gases, tobacco smoke, pesticides, solvents, cleaning agents, particulates, dust, mold, fibers, and allergens [ 16 ]. Humans also create favorable conditions for the development of millions of mold, fungus, pollen, spores, bacteria, viruses, and insects, such as dust mites and roaches. Combustion sources and cooking activates contribute to carbon dioxide (CO 2 ), sulfur dioxide (SO 2 ), CO, nitrogen dioxide (NO 2 ), and particulate matter (PM) emissions into indoor air environments [ 17 , 18 ]. In addition, equipment, such as computers, photocopy machines, printers, and other office machines, emit ozone (O3) and volatile compounds. Common building materials, such as poly(vinyl chloride) PVC floor covering, parquet, linoleum, rubber carpet, adhesive, lacquer, paint, sealant, and particle board, can shed toxic compounds (i.e., alkanes, aromatic compounds, 2-ethylhexanol, acetophenone, alkylated aromatic compounds, styrene, toluene, glycols, glycolesters, texanol, ketones, esters, siloxane, and formaldehyde) [ 10 ].
Importantly, the design and operation of ventilation systems also have a significant influence on IAQ. Due to superseding the stale indoor air by the fresh outdoor air, ventilation creates suitable IAQ and a healthy indoor environment. There are several benefits for the operation of ventilation in a building [ 19 ], including: (i) Providing oxygen and fresh air for human respiration; (ii) diluting indoor air pollutants to reach the short-term exposure limits of harmful contaminants as well as odors and vapors; (iii) using outdoor air with a low aerosol concentration to control aerosols inside buildings; (iv) controlling internal humidity; and (v) creating proper air distribution and promoting healthy and comfortable environment. Ventilation systems can be classified into two types [ 20 ], including: (i) Mechanical ventilation systems that use mechanical equipment, such as fans or blowers; and (ii) natural ventilation systems, which are the exchange processes between indoor air and out indoor without using mechanical equipment. Although natural ventilation systems may be well adopted by the occupants, they are insufficient in some buildings or climates. These days, mechanical ventilation systems have been commonly used in buildings, which significantly increases energy consumption. Thus, hybrid ventilation systems are designed to take advantage of both mechanical and natural ventilation systems, in order to decrease energy consumption and increase the use of sustainable technologies [ 21 ]. In hybrid ventilation systems, the shortcomings of natural ventilation will be compensated by mechanical components [ 22 ]. In summary, in heating, ventilation, and air conditioning (HVAC) systems of buildings, ventilation plays a key role in creating suitable IAQ, but it is also responsible for energy consumption. Therefore, improving ventilation systems in buildings is the key issue not only for enhancing energy efficiency but also for providing better IAQ to the occupants and minimizing the possibility of health problems as a consequence [ 21 ].
3. Main Pollutants in Indoor Air Environment
Numerous indoor air pollutants have been recognized to have harmful impacts on IAQ and human health [ 23 ]. The main indoor air pollutants include NOx, volatile and semi-volatile organic compounds (VOCs), SO 2 , O 3 , CO, PM, radon, toxic metals, and microorganisms. The sources and health effects of some common pollutants are listed in Table 1 . Some of them can be present in both indoor and outdoor environments, while others originate from the outdoor environment. Generally, indoor air pollutants are able to be classified into organic, inorganic, biological, or radioactive [ 24 ].
Common indoor pollutants and their effects on human health.
3.1. Particulate Matters
PM is defined as carbonaceous particles in association with adsorbed organic chemicals and reactive metals. PM’s main components are sulfates, nitrates, endotoxin, polycyclic aromatic hydrocarbons, and heavy metals (iron, nickel, copper, zinc, and vanadium) [ 7 ]. Depending on the particle size, PM generally is classified into (i) coarse particles, PM 10 of diameter <10 µm; (ii) fine particles, PM 2.5 of diameter <2.5 µm; and (iii) ultrafine particles, PM 0.1 of diameter <0.1 µm. PM is especially concerning, as it is sometimes inhalable, affecting the lungs and heart and causing serious health effects. It has been shown that indoor PM levels often exceed outdoor ones [ 25 ]. Indoor PM sources include (i) particles that migrate from the outdoor environment and (ii) particles generated by indoor activities. Cooking, fossil fuel combustion activities, smoking, machine operation, and residential hobbies are the main reasons why PM is distributed inside of buildings. Compared with PM 10 and PM 2.5 , PM 0.1 created by fossil fuel combustion represents a greater threat to health due to its penetrability into the small airways as well as alveoli [ 26 , 27 ]. According to research about the concentration of major indoor pollutants, it has been indicated that cooking and cigarette smoking are the largest sources of indoor air PM, whereas cleaning activities often have a lesser contribution to indoor PM [ 28 ]. Smoking is known as a major source of indoor PM 2.5, with estimated increases in homes with smokers ranging from 25 to 45 µg /m 3 and the concentration in winter is greater than in summer [ 29 ]. For cooking activity, it was shown that cooking activities enable the emission of millions of particles (~10 6 particles/cm 3 ) through the burning of oil, wood, and food and most of them are ultra-fine particles [ 30 , 31 ]. In addition, these fine particles can distribute not only to the kitchen but also spread to the living room and other areas in the building, thereby causing adverse effects to the occupants’ health [ 31 , 32 ]. Meanwhile, other normal human activities, such as walking around and sitting on furniture, are likely to resuspend house dust and contribute to 25% of the indoor PM concentrations [ 29 ]. In summary, it has been found that the source strengths for human activities ranged from 0.03 to 0.5 mg.min −1 for PM 2.5 and from 0.1 and 1.4 mg.min −1 for PM 10 [ 33 ].
Volatile organic compounds (VOCs) are recognized as gases containing a variety of chemicals emitted from liquids or solids [ 34 ]. Formaldehyde, a colorless gas with an acrid smell and which is released from many building materials, such as particleboard, plywood, and glues, is one of the most widespread VOCs. VOC concentrations in indoor environments are at least 10 times higher than outdoors, regardless of the building location [ 34 , 35 ]. Generally, indoor VOCs are generated from four main sources: (i) Human activities, including cooking, smoking, and the use of cleaning and personal care products; (ii) generation from indoor chemical reactions; (iii) penetration of outdoor air through infiltration and ventilation systems; and (iv) originating from building materials [ 35 , 36 , 37 , 38 , 39 ]. The VOCs concentration is able to be affected by air exchange rates, house age and size, building renovations, outdoor VOC levels, and door and window opening [ 40 ]. Moreover, it has been demonstrated that about 50 different VOCs are identified during 90-min cooking periods [ 35 ]. Because VOCs are organic chemicals that possess a low boiling point (T b ) and are easily volatilized even at room temperature, the WHO classified them into four groups: (i) Very volatile organic compounds (VVOCs) with T b : 50–100 °C; (ii) volatile organic compounds (VOCs) with 100 °C < T b < 240 °C; (iii) semi-volatile inorganic compounds (SVOCs) with 240 °C < T b < 380 °C; and (iv) particulate organic matter (POM) with T b > 380 °C [ 41 , 42 ]. Normally, exposure to VOCs released from consumer products is incurred via three main pathways: Inhalation, ingestion, or dermal contact. Most people are not seriously affected by short-term exposure to low concentrations of VOCs, but in cases of long-term exposure, some VOCs are considered to be harmful risks to human health, potentially causing cancer [ 43 ]. As for SVOCs, transdermal uptake directly from air has a higher contribution compared with intake via inhalation [ 44 , 45 ].
The two principal nitrogen oxides are nitric oxide (NO) and nitrogen dioxide (NO 2 ), both of which are associated with combustion sources, such as cooking stoves and heaters [ 46 ]. Ambient concentrations of NO and NO 2 vary widely depending on local sources and sinks. Their average concentration in buildings without combustion activities is half that in the outdoors, but when gas stoves and heaters are used, indoor levels often exceed outdoor levels. Under ambient conditions, NO is rapidly oxidized to form NO 2 ; hence, NO 2 is usually considered as a primary pollutant. The reaction of NO 2 with water produces nitrous acid (HONO), a strong oxidant and common pollutant of indoor environments [ 47 ]. It has been indicated that indoor levels of NO 2 are a function of both outdoor and indoor sources; hence, indoor levels can be influenced by high outdoor levels originating from combustion or local traffic sources. It was reported that the distance between buildings and roadways has a significant influence on indoor NO 2 levels [ 48 ]. Besides, the air exchange between outdoors and indoors also affects NO 2 levels in buildings [ 49 ]. Additionally, the major indoor sources include smoking and wood-, gas-, oil-, coal-, and kerosene-burning appliances, such as stoves, space, ovens, and water heaters and fireplaces [ 47 ].
Ozone is a powerful oxidizing agent mainly produced by photochemical reactions of O 2 , NOx, and VOCs in the atmosphere. However, it cannot be used to eliminate other indoor chemical pollutants, due to its slow reaction with most airborne pollutants [ 50 , 51 ]. Ozone enables rapid reaction with several indoor pollutants, but the reaction products can irritate humans and damage materials. The main sources of indoor ozone mainly come from the outdoor atmosphere and the operation of electrical devices [ 52 ]. The machines commonly emitting indoor ozone gas include photocopiers, disinfecting devices, air-purifying devices, and other office devices [ 53 , 54 , 55 , 56 ]. The ozone emission mechanisms of these devices can be divided into two categories: Corona discharge and photochemical mechanisms. It has been shown that indoor ozone levels depend on various factors: (i) The outdoor ozone level; (ii) indoor emission rates; (iii) air-exchange rates; (iv) surface-removal rates, and (v) reactions between other chemicals and ozone in the air [ 50 ]. Indoor ozone levels generally fluctuate between 20% and 80% of the outdoor ozone level according to the air-exchange rate [ 57 ]. Humans are exposed to ozone primarily by inhalation, but skin exposure is also a recognized vector [ 58 ].
Sulfur dioxide (SO 2 ) is the most common gas among the group of sulfur oxides (SO x ) present in the atmosphere. SO 2 is primarily produced by the combustion process of fossil fuels, and combines with aerosols and PMs to form a complex group of distinct air [ 59 ]. Indoor sources of SO 2 emissions include vented gas appliances, oil furnaces, tobacco smoke, kerosene heaters, and coal or wood stoves [ 60 ]. In addition, outdoor air is also regarded as a main source of indoor SO 2 [ 61 ]. Indoor SO 2 levels are often lower than outdoor levels. SO 2 emission indoors is usually small, owing to its reactivation, which can be easily absorbed by indoor surfaces. It is known that the hourly concentration of SO 2 in buildings is often below 20 ppb [ 62 ]. Human exposure to SO 2 , which can impair respiratory function, is only via inhalation.
Carbon monoxide (CO) in indoor air is produced mainly by combustion processes, such as cooking or heating. Besides, CO can also enter into indoor environments through infiltration from outdoor air [ 63 ]. The important sources of indoor CO emissions include unvented kerosene and gas space heaters; leaking chimneys and furnaces; back-drafting from furnaces, gas water heaters, wood stoves, and fireplaces; gas stoves; generators and other gasoline-powered equipment; and tobacco smoke [ 47 ]. The average concentration of CO in a building without any gas stoves is about 0.5–5 ppm, while the concentration in areas near gas stoves ranges from 5 to 15 ppm and even 30 ppm or higher. CO exposure can cause adverse health effects, such as (i) at low concentrations, there are impacts on cardiovascular and neurobehavioral processes; and (ii) at high concentrations, unconsciousness or death [ 64 ].
Carbon dioxide (CO 2 ), a colorless and odorless gas, is a well-known constituent of the earth’s atmosphere and also a major human metabolite [ 65 ]. The average CO 2 concentration in ambient air is about 400 ppm, which is primarily the result of the combustion of fossil fuels [ 65 , 66 ]. Recently, the indoor CO 2 level has been applied as a reference for the assessment of IAQ as well as for ventilation control [ 66 , 67 , 68 ]. According to the ASHRAE standard, it is recommended that indoor CO 2 concentrations are below 700 ppm to ensure human health [ 69 ]. It is established that exposure to a a CO 2 concentration of 3000 ppm increases headache intensity, sleepiness, fatigue, and concentration difficulty [ 65 , 70 ].
3.7. Toxic Metals
Heavy metals are released into the atmosphere through either human activities or natural processes [ 71 ]. IAP by heavy metals has various causes, including infiltration of outdoor pollutants (dust and soil), smoking, fuel consumption products, and building materials [ 55 ]. Heavy metals in indoor dust, entering the human body through inhalation, ingestion, or dermal contact, can have adverse effects on human health [ 72 , 73 ]. According to the International Agency for Research on Cancer (IARC), heavy metals in indoor air are classified into two main groups based on their effects on humans: (i) Non-carcinogenic elements, including cobalt (Co), aluminum (Al), copper (Cu), nickel (Ni), iron (Fe), and zinc (Zn); and (ii) both carcinogenic and non-carcinogenic elements encompassing arsenic (As), chromium (Cr), cadmium (Cd), and lead (Pb) [ 74 ]. These common heavy metals (i.e., As, Cr, Cd, Pb) are likely to cause cancers [ 75 , 76 ], while Cd and Pb, along with some others, can cause carcinogenic effects, such as cardiovascular disease, slow growth development, and damage to the nervous system [ 73 , 77 , 78 ]. It has been reported that Pb levels in indoor air can fluctuate from 5.80 to 639.10 μg/g, while the highest levels of As, Al, Cr, Cd, Co, Cu, Ni, Fe, and Zn are about 486.80, 7150.00, 254.00, 8.48, 43.40, 513.00, 471.00, 4801.00, and 2293.56 μg/g, respectively [ 74 ].
3.8. Aerosols
Indoor aerosols are either primary aerosols originating from different indoor sources or secondary aerosols formed by indoor gas-to-particle reactions [ 79 ]. Moreover, outdoor particles infiltrating indoors are also likely to be a source of indoor aerosols. Secondary inorganic aerosols are PMs consisting of inorganic elements, including anthropogenic or crustal sources and water-soluble ions [ 6 ], while secondary organic aerosols (SOAs) are formed in the gas-to-particle conversion process of VOCs [ 80 ]. Additionally, carbonaceous aerosols, which comprise SOAs and elemental carbons released in incomplete combustion, are well-known species in PM 2.5 [ 81 ]. Biological aerosols (bioaerosols) are a subset of atmospheric PMs comprising dispersal units (fungal spores and plant pollen), microorganisms (bacteria and archaea), or cellular materials [ 82 ]. Due to their diversity in terms of compounds and phases (gas, liquid, or solid), aerosols can be regarded as dynamic systems [ 83 ]. As such, their particle size distribution varies from the nucleation mode (<30 nm in vacuum cleaning condition) to the accumulation mode (~100 nm, indoor combustion aerosols from smoking, cooking, or incense burning), and to the fine and coarse modes (>1 µm, resuspension aerosols) [ 84 , 85 ]. Aerosol exposure through inhalation in the indoor environment has been linked to numerous adverse health effects, mainly in the lungs (the entrance to the human body) and other important target organs, such as the heart and brain [ 79 ].
The primary sources of indoor radon include building materials, soil gas, and tap water [ 86 ]. As soil contains radium at trace concentrations, radon is likely to be one of the constituents in the gas filling soil pores. As for radon emissions from building materials, all materials holding trace amounts of radium can release radon. Among building materials, masonry materials (i.e., stone, concrete, and brick) are the main sources for indoor radon emission, in that tons of such materials are used in building construction. Indoor radon can be released through the usage of water from underground water sources containing granite or other radium-bearing rock, and such water sources commonly contain radon concentrations above 10,000 pCi/L [ 87 ]. Finally, outdoor air is also regarded as a source of indoor radon [ 88 ]. Human exposure to radon in buildings is incurred mainly through the permeation pathways of underlying soil gas [ 89 ]. Epidemiological studies have demonstrated that indoor radon can cause lung cancer risk increases of 3% to 14%, depending on the average radon level [ 90 ].
3.10. Pesticides
These days, inorganic and organic pesticides have commonly been utilized as protectants for wooden building materials by impregnation or surface coating [ 91 ]. Pesticides are also used to control and prevent pests, including bacteria, fungi, insects, rodents, and other organisms [ 92 , 93 ]. In the indoor environment, pesticides are usually semi-volatile compounds that may exist in either gas or particulate form according to properties, such as the vapor pressure, product viscosity, and water solubility [ 94 ]. In addition, it has been indicated that carpet and textiles are likely to play the role of long-term reservoirs for organochlorine pesticides [ 95 , 96 ]. It is supposed that when used in carpets, textiles, and cushioned furniture, pesticides in fibers will migrate into polyurethane foam pads [ 97 , 98 ], and thus carpet, textiles, and cushioned furniture can reflect an integrated pesticide exposure during their lifetime. Moreover, pesticides are able to enter buildings from outdoors. Once inside, they can persist for months or years due to their protection against sunlight, extreme temperatures, rain, and other factors [ 97 ]. Dermal uptake, ingestion, and inhalation of particles or volatile compounds containing pesticides are believed to be potential exposure routes in the indoor environment [ 92 ]. Pesticide exposure is associated with adverse health risks, including (i) short-term skin and eye irritation, dizziness, headaches, and nausea; and (ii) long-term chronic impacts, such as cancer, asthma, and diabetes [ 99 ].
3.11. Biological Pollutants
Biological pollutants in indoor environments include biological allergens (e.g., animal dander and cat saliva, house dust, cockroaches, mites, and pollen) and microorganisms (viruses, fungi, and bacteria) [ 100 ]. Biological allergens, known as antigens, originate from a number of insects, animals, mites, plants, or fungi, and will induce an allergic state in reacting with specific immunoglobulin E (IgE) antibodies [ 101 ]. Indoor sources of allergens mainly include furred pets (dog and cat dander), house dust mites, molds, plants, cockroaches, and rodents [ 102 ], and there are outdoor sources as well [ 101 ]. Viruses and bacteria often originate from or are carried by people and animals. It has been demonstrated that exposure to biological allergens can result in sensitization, respiratory infections, respiratory allergic diseases, and wheezing [ 103 ], while exposure to bacteria and viruses indoors is likely to cause noninfectious and infectious adverse health outcomes [ 104 ].
4. IAQ Guidelines and Standards
It is obvious that the combination of long-term exposure and anthropogenic indoor activities can cause degradation of IAQ and significant risks to human health, even at low air pollutant concentrations. To face these IAQ problems, relevant organizations as well as the scientific community have attempted to develop and apply IAQ standards and guidelines. After many efforts, the world community established IAQ guidelines and standards based on an integrated building approach [ 110 ]. According to the WHO and USEPA, the role of IAQ guidelines is to provide a critical database as a reference for the prevention of harmful consequences of IAP and protection of public health; hence, the goal is to eliminate, or at least minimize, possible risks to human populations [ 111 ]. Table 2 summarizes the IAQ guidelines of the WHO and USEPA for some common pollutants [ 112 ]. However, it is necessary to distinguish non-occupational (i.e., residential houses, schools, offices) guidelines from occupational (industrial) standards [ 113 ]. Generally, the WHO and USEPA guidelines are the maximum concentrations during specific durations (i.e., 1 h, 24 h, or 1 year). Moreover, the IAQ guidelines of the WHO and USEPA seem non-applicable to occupational sectors [ 114 ], as they usually are applied for the control of IAQ inside households, schools, hospitals, public buildings, and offices [ 47 ]. In addition, each country will formulate specific standards or guidelines suitable to their own particular circumstances [ 115 , 116 ].
Indoor air quality guidelines for major indoor air pollutants.
(Source: European Commission DG XVII: https://www.europeansources.info/corporate-author/european-commission-dg-xvii/ ).
5. The Oxidative Capacity of Indoor Environment
The oxidative capacity has been significantly less explored in indoor environments. However, recent studies in atmospheric science have started looking at indoor oxidants and their precursors, in which the combination of indoor oxidants and their precursors will be referred to as “oxidants*” [ 117 ]. It has been supposed that ozone (O 3 ), the hydroxyl radical (OH), and the nitrate radical (NO 3 ) are major oxidants * [ 118 ]. In addition, hydrogen peroxide (H 2 O 2 ), nitrogen dioxide (NO 2 ), hydroperoxy radicals (HO 2 ), chlorine atoms (Cl), and alkylperoxy radicals (RO 2 ) can be important indoor oxidants* under certain conditions. Oxidation is regarded as the dominant process in ozone reactions indoors; thus, most research into indoor oxidants* has focused solely on ozone. Recently, it was demonstrated that the formation of OH radicals by formaldehyde (HCHO) and photolysis of nitrous acid (HONO) is an important source of indoor oxidants* ( Figure 1 ) [ 117 ]. In the atmosphere, the OH radical is known as the key species in photo-oxidation cycles; it can oxidize VOCs to form secondary aerosols or other gas species, which can cause toxic and carcinogenic effects in humans [ 119 ]. As for the important formation pathways of OH radicals, there are several different reactions productive of OH radicals, such as (i) NO and hydroperoxyl (HO 2 ), (ii) ozone and alkenes, and (iii) the photolysis reaction of ozone (λ < 320 nm), HONO (λ < 400 nm), and H 2 O 2 (λ < 360 nm) [ 120 ].

Schematic illustration of the important formation pathways of OH radicals indoors. Reproduced with permission from [ 118 ].
The primary sources of emissions of indoor oxidants* are building materials [ 121 , 122 ] and electronics [ 123 ]. Besides, acetaldehyde and other carbonyls can be emitted from human and microbial occupants [ 121 , 124 , 125 ], and moreover, human activities (i.e., cleaning or disinfection of surfaces, the use of air fresheners, cooking, smoking, vaping, bleach cleaning) are likely to be major sources of indoor oxidants* [ 117 ]. Other important sources are secondary oxidant formation indoors and transport from outdoors [ 50 ]. Greater outdoor-to-indoor transport of O 3 and NO can lead to heightened NO/HO 2 and O 3 /alkene reactions that result in higher levels of OH radicals.
6. The Effects of Indoor Air Pollution to Human Health
6.1. building-associated illness.
Over the past decades, various symptoms and illnesses have been linked to diminished IAQ in buildings and houses. Indoor exposure to inorganic, organic, physical, and biological contaminants, though often at low levels, is common, ubiquitous, and sustained. Therefore, the harmful effects of IAP on human health have always attracted great attention and concern. According to the WHO, building-associated illness refers to any illness caused by indoor environmental factors, which commonly are divided into two categories: Sick building syndrome (SBS) and building-related illness (BRI) [ 10 ]. Their associated symptoms are shown in Figure 2 .

The common symptoms of sick building syndrome (SBS) and building related illness (BRI).
6.1.1. Sick Building Syndrome (SBS)
SBS often refers to a group of symptoms that are linked to the physical environments of specific buildings [ 126 ]. Acute health and comfort effects of SBS will appear when patients spend a certain amount or duration of time in a building, but they and their causes are difficult to clearly identify [ 126 ]. These effects are either localized in particular areas or widespread throughout a building [ 127 , 128 ]. It has been reported that symptoms tend to worsen as a function of the exposure time in buildings and can disappear as people spend more time away from the building [ 129 ]. According to the WHO, SBS symptoms caused by IAP can be divided into four categories: (i) Mucous-membrane irritation: Eye, throat, and nose irritation; (ii) neurotoxic effects: Headaches, irritability, and fatigue; (iii) asthma and asthma-like symptoms: Chest tightness and wheezing; and (iv) skin irritation and dryness, gastrointestinal problems (i.e., diarrhea), and others [ 130 , 131 ]. The International Labour Organization (ILO) reported that infants, the elderly, persons with chronic disease, and most urban dwellers of any age have higher health risks linked with IAP-associated SBS symptoms [ 132 ]. A low ventilation rate, building dampness, and high room temperature also tend to increase the likelihood of SBS prevalence [ 133 ]. Moreover, other risk factors, such as gender, atopy, and psychosocial factors, also have a significant influence on SBS symptom prevalence [ 134 ].
6.1.2. Building-Related Illness (BRI)
BRI describes illnesses and symptoms with an identified causative agent directly related to exposure to poor air quality in buildings. It is known that causative agents can be chemicals, such as formaldehyde, xylene, pesticides, and benzene, but biological agents are more widespread. In buildings, the typical sources for indoor emissions of biological contaminants are cooling towers, humidification systems, filters, drain pans, wet surfaces, and water-damaged building materials [ 10 ]. BRI symptoms have been associated with the flu, including fever, chills, chest tightness, muscle aches, and cough. In addition, serious lung and respiratory problems are likely to occur. Common BRI illnesses include Legionnaires’ disease, hypersensitivity pneumonitis, and humidifier fever [ 10 ]. It was reported that indoor environmental pollutants can cause BRI symptoms via four major mechanisms: (i) Immunologic, (ii) infectious, (iii) toxic, and (iv) irritant [ 135 ]. An irritant effect is often BRI’s initial insult, but toxic, allergic, or infectious mechanisms can arise subsequently, depending on the pollutant type and individual susceptibility. Psychologic mechanisms are often not paid significant attention but are demonstrably likely to increase the overall morbidity of building-related diseases as well [ 136 ].
Four main factors have been linked to BRI, including: (i) Physical-environmental factors, (ii) chemical factors, (iii) biological factors, and (iv) psychosocial factors. As for the physical-environmental factors, BRI can be influenced by the temperature, humidity, lighting, air movement, and dust concentration. The chemical factors, meanwhile, include various pollutants released from human activities and products, such as carpets, paint, new furniture, smoking, cosmetics, asbestos, drapes, and insecticides. Finally, major biological factors associated with BRI are microorganisms as mentioned in Section 3.11 .
6.2. Acute Respiratory Infection
The respiratory system is frequently the primary target of IAP effects because pollutants often enter into the human body through inhalation. Depending on the area of the affected respiratory tract, acute respiratory infections can be classified into acute lower respiratory infections (ALRIs) and upper respiratory infections (URIs) [ 137 ]. URIs are illnesses involving the upper respiratory with common symptoms, such as cough, sinusitis, and otitis media [ 138 ], and they are often mild in nature and caused by biological pollutants (viruses, bacteria, fungi, fungal spores, and mites). Meanwhile, ALRI, an acute infection of the lung, is caused by viruses or bacteria, resulting in lung inflammation [ 137 ]. It has been found that IAP increases the risk of childhood ALRI by 78%, which leads to a million deaths in children under 5 years of age every year [ 139 ]. This may be because children have a relatively large lung surface area [ 140 ]. It has been demonstrated that children living in buildings using solid fuels possess a higher risk of developing ALRI by 2-3 times more than those combusting clean fuels [ 141 ]. IAP adversely affects specific and nonspecific host defenses of the respiratory tract against pathogens. In addition, IAP enhances the severity of respiratory infections and also results in high rates of chronic bronchitis of cooking mothers [ 142 ].
6.3. Pulmonary Diseases
Inhaled air pollutants are associated with allergic diseases and pulmonary diseases, such as asthma, atopic dermatitis, and allergic rhinitis. Moreover, smoking activity is regarded as one of the most important factors in the development of chronic inflammatory pulmonary diseases, including chronic obstructive pulmonary disease (COPD), asthma, and lung cancer [ 143 ]. Maximal obtainment of lung function can be significantly affected by IAP exposure; subsequently, lung function is declined. Noxious particles, including PM and CO, may influence lung development starting in utero [ 144 ].
COPD diseases are featured by an enhanced chronic inflammatory response in the airways and the lung to toxic PM or indoor air pollutants. It has been demonstrated that women, especially in developing countries, have a great risk for COPD because of exposure to household smoke from cooking [ 145 ]. PMs from fossil fuel combustion induce inflammation in the lung and highly reduce the pulmonary function. It has been indicated that fuel smoke in the household can cause COPD diseases with clinical signs and mortality similar to that of tobacco smokers [ 146 ]. Fuel burning generates chemical compounds with a high oxidative capacity that induce oxidative stress and DNA damage, which are pointed as the main mechanism responsible for the pathogenesis of COPD [ 147 ]. Additionally, biological factors, such as allergens, viruses, and bacterial substances, are likely to induce severe inflammatory reactions and cause immune dysfunction and chronic inflammation, which leads to COPD diseases [ 148 , 149 , 150 ].
Exposure to indoor air pollutants is able to result in asthma symptoms or cause asthma exacerbations [ 151 ]. It has been indicated that acute exposure to combustion smoke can induce bronchial irritation, inflammation, and enhance bronchial reactivity, which is regarded as the main mechanism responsible for asthma exacerbation, especially in children [ 152 ]. It has been demonstrated that children between 5 and 14 years living in houses combusting coal, wood, and kerosene have a relative risk of 1.6 in asthma exacerbation [ 153 ].
Lung cancer is usually associated with smoking. Recently, it has been demonstrated that lung cancer is highly linked to IAP exposure in females due to spending long times cooking [ 154 ] and thus lung cancer is more popular in females than males in non-smoking cases. Moreover, it has also been indicated that there is a considerable difference in the percentages of non-smoking females with lung cancer based on the various regions. For illustration, more than 80% of female lung cancer cases in east and south Asia are not related to smoking, whereas it is only 15% in the USA [ 155 ]. Emissions from the combustion of solid fuels for cooking or heating have been associated with a high risk of lung cancer [ 156 ]. It has been demonstrated that people using solid fuel, such as coal and wood, for heating and cooking throughout their life have a 4 times higher risk of lung cancer as compared with those combusting clean energy [ 156 ]. It is the incomplete combustion that highly emits various particle and gaseous carcinogens, including SO 2 , CO, NO 2 , PAHs, formaldehyde, heavy metals, and PM 2.5 [ 157 ]. These pollutants have been found to be related to morbidity and mortality from respiratory diseases, especially lung cancer [ 158 ].
6.4. Cardiovascular Diseases (CVDs)
The use of solid fuels in households can emit various pollutants that are linked to CVDs, including PM, PAHs, CO, heavy metals, and other organic pollutants [ 159 ]. Exposure to PM 2.5 increases the incidences of specific acute CVDs, such as ischemic stroke, myocardial infarction, cardiac arrhythmia, heart failure, and atrial fibrillation [ 160 , 161 ]. It has been reported that PM can cause CVDs due to inducing oxidative stress, systemic inflammation, increased blood coagulability, and autonomic and vascular imbalance [ 162 ]. PM is also a main factor causing significant increases in fibrinogen, platelet activation, plasma viscosity, and release of endothelins, a family of potent vasoconstrictor molecules. Moreover, CO in the indoor air environment is likely to influence tissue oxygenation through carboxyhemoglobin production, which results in a high impact on cardiovascular function [ 141 ]. Additionally, exposure to PAH and Pb from the use of fuel for cooking also enhances oxidative damage, stimulates the renin-angiotensin system, and downregulates nitric oxide [ 163 ]. These mechanisms may cause increased vascular tone and peripheral vascular resistance.
7. Current Strategies for Monitoring and Control of IAQ
7.1. development of materials for iaq sensors.
During the past decade, two-dimensional (2-D) nanostructured materials, due to their unique physical and chemical properties, have attracted great attention for their utility in the design and production of gas-sensing devices. It was demonstrated that 2-D materials possess outstanding features, such as a large surface-to-volume ratio, excellent semiconducting properties, and high surface sensitivity. Moreover, the combination of 2-D nanostructured materials with other-dimensional materials is also proposed as a promising approach to the development of high-performance IAQ-monitoring sensors [ 164 ]. It has been demonstrated that 2-D nanostructured materials can be combined with 3-D bulk materials, 2-D nanostructures, 1-D nanostructures, and 0-D nanomaterials to produce 4 novel architectures for IAQ monitoring ( Figure 3 ). Due to their special optical and electrochemical properties, 0-D nanomaterials of nanoscale (<100 nm) size, such as quantum dots (QDs), nanoparticles, nanocrystals, and nanoclusters, have been applied to the production of photonic, electronic, and chemical-sensing devices [ 165 , 166 ]. The use of 1-D nanostructures, such as nanotubes, nanowires (NWs), nanofibers, and nanorods, in combination with 2-D materials has been demonstrated to be effective in numerous IAQ-sensing applications, owing especially to their fast electron-transport capability, special morphology, and high surface-to-volume ratio [ 167 ]. Meanwhile, due to the formation of Van der Waals heterostructures through direct stacking of different 2-D materials, various types of 2-D–2-D heterostructures, including black phosphorus/MoSe 2 , graphene/MoS 2 , and graphene/WS 2 /graphene, have been applied in novel devices for IAQ monitoring [ 168 , 169 ]. Finally, by growing or transferring 2-D materials onto bulk 3-D semiconductors, layered 2-D-nanostructured semiconductors can be integrated with traditional bulk 3-D semiconductor substrates in the design of new gas-sensing devices [ 167 ]. In summary, heterostructure-based devices, given the superior properties of their constituent materials, could be utilized for a wide range of IAQ detection applications.

Schematic overview of 2-D nanostructured materials and different types of heterostructures with 0-D, 1-D, 2D, and 3-D materials used for gas-sensing applications. Reproduced with permission from [ 164 ].
The development of inexpensive sensor networks and systems has emerged as a key strategy for the monitoring of IAQ. Metal oxide (MOx) sensors are currently regarded as one of several outstanding technologies, especially in consideration of their low cost, high sensitivity, simplicity, and compatibility with modern electronic devices [ 170 ]. With their small size and low cost, they are ideally suited for remote and portable monitoring systems. Recently, metal oxide semiconductor gas sensors have been considered as a viable platform for the detection of VOCs and CO in the indoor air environment [ 171 , 172 , 173 ]. It is reported that several metal oxides, including SnO 2 , ZnO, TiO 2 , In 2 O 3 , Fe 2 O 3 , MoO 3 , Co 3 O 4 , CuO, NiO, and CdO, have been utilized in IAQ-sensing applications.
7.2. Advanced Technologies for Monitoring of IAQ
There have been various techniques invented and introduced for real-time monitoring [ 174 ]. Among them, however, wireless sensor network (WSN) and Internet of Things (IoT)-based systems are the most popular technologies developed for IAQ monitoring due to their rising scope in the Industry 4.0 revolution.
7.2.1. Internet of Thing (IoT)-Based Systems
In recent years, with the development of mobile technologies, the Internet of Things (IoT), and big data, machine-learning technologies have been introduced as trending technologies that offer great capability for real-time IAQ monitoring. By the introduction of IoT-based portable IAQ-monitoring devices, these days, air quality can be easily monitored and controlled in real time. Many IoT-based IAQ-monitoring systems and devices have been designed and introduced, including open-source technologies for data processing and transmission [ 175 ].
One of the important applications of IoT in IAQ monitoring is “electronic noses” (E-noses), which are biomimetic-type devices that mimic the functionalities of mammals’ olfaction system [ 176 ]. Generally, an E-nose system consists of four basic components: (i) A multi-sensor array; (ii) software with digital pattern recognition algorithms; (iii) an information-processing unit (i.e., an artificial neural network (ANN)), and (iv) reference library databases [ 177 ]. By using the chemical sensor arrays in combination with the classification algorithms, E-noses can easily monitor target gases by detecting and discriminating types and concentrations [ 178 ]. Therefore, E-noses have globally attracted great interest for their low-cost portable IAQ-monitoring utility [ 179 ]. For example, Tastan et al. proposed a low-cost, portable, IoT-based, and real-time monitoring E-nose system [ 180 ], by which a range of sensors can measure different air pollutants (i.e., CO 2 , CO, PM10, and NO 2 ) and air parameters (i.e., temperature and humidity). Especially, the proposed E-nose is produced with open-source, low-cost, and easy installation software and four detection units, including GP2Y1010AU (dust sensor), MH-Z14A (CO 2 sensor), MICS-4514 (NO 2 and CO sensor), and DHT22 (temperature sensor and humidity sensor). These sensors monitor via the 32-bit ESP32 Wi-Fi controller, while a mobile device interface based on the Blynk IoT platform receives data and records it in a cloud server ( Figure 4 A). This concept is anticipated to serve as a model for future IAQ-monitoring devices. In other research, Chen et al. developed a high-performance smart E-nose system comprising a multiplexed tin oxide (SnO 2 ) nanotube sensor array, a read-out electronic circuit, a mobile phone receiver, a wireless data transmission unit, and a data processing application [ 181 ]. Compared with conventional devices, this nanotube sensor exhibited higher sensitivity gas detection and discrimination at room temperature, owing to the use of nanotube sensors possessing a large surface area for interaction with gas molecules. It was demonstrated that this E-nose device can detect indoor target air pollutants by only a simple vector-matching recognition algorithm; hence, it can be regarded as possessing state-of-the-art sensitivity for air pollutant detection at room temperature based on metal oxide sensors. Importantly, therefore, the smart E-nose device and system can be applied for high-performance monitoring of the indoor environment’s quality in smart buildings, smart homes, or even smart cities in the present and future ( Figure 4 B).

Illustration of the proposed electronics nose (E-nose) system architecture ( A ); the proposed E-nose application in future smart buildings ( B ); primitive concept design of Smart-Air ( C ); and a smart ventilation system for optimizing indoor air quality (IAQ) Levels in buildings ( D ). Reproduced with permission from [ 180 , 181 , 183 , 184 ].
Recently, smart devices based on the integration of cloud computing and IoT have been developed to precisely monitor IAQ and efficiently transmit real-time data to a cloud computing-based web server using an IoT sensor network [ 182 , 183 ]. Jo et al. (2020) introduced a Smart-Air device to collect reliable and accurate data for IAQ monitoring ( Figure 4 C) [ 184 ]. The Smart-Air device includes three components: A pollutant detection sensor array, a microcontroller, and an LTE modem. The sensors in the Smart-Air device include a VOC sensor, a laser PM sensor, a CO 2 sensor, a CO sensor, and a temperature/humidity sensor. The Smart-Air platform additionally relies on cloud computing technology and IoT technology to monitor and control IAQ anytime and anywhere. Importantly, the Korean Ministry of Environment testing has demonstrated that the device is highly reliable. All received data is stored in the web server’s cloud to provide resources for further analysis of IAQ [ 184 ].
According to the concept of the combination of ventilation system activation with IAQ-variable-level detection, a strategy for IAP reduction by demand-controlled ventilation systems (DCVs) based on CO 2 detection was recently developed [ 185 ]. This system consists of four basic components, including the hardware, backend, mobile app, and IAQ index computation. The system operation depends on several real-time sensors and algorithms to accurately and automatically control the ventilation system and maintain a suitable concentration of air pollutants by balancing indoor and outdoor parameters [ 183 ]. As shown in Figure 4 D, this sensing platform provides an IoT-based system for the control of IAQ levels in a building based on recorded data. Some parameters, such as the CO 2 , VOCs, humidity, and temperature of both the indoor and outdoor environments, are measured simultaneously; then, this data is applied for computation of the IAQ index, which is used for algorithm-based ventilation control. Additionally, the system allows for observation and management via a mobile app.

7.2.2. Wireless Sensor Network (WSN)-Based Systems
In the past few years, the wireless sensor network (WSN) has gained great attention in many different monitoring situations [ 186 ]. Generally, WSN is composed of tiny devices or nodes having an important role in collecting information by sensors from the environment as well as wirelessly communicating with other nodes in the system [ 174 ]. It has been indicated that in the development strategies of the WSN system, ZigBee, a wireless standard based on the IEEE 802.15.4 specification, is regarded as the most reliable communication protocol because of its low cost, low consumption, and low data rate [ 187 , 188 ]. In addition, use of the ZigBee protocol allows networks to work in power-saving mode [ 189 , 190 ].
In practical applications, WSN systems usually include a distributed sensor network connected to a cloud system [ 191 ]. ZigBee-based sensor nodes transmit field measurement data to the cloud system through a gateway. The optimized cloud computing systems have often been utilized to monitor, store, process, and visualize the obtained data from the sensor network [ 187 ]. Additionally, the obtained data is processed and analyzed by artificial intelligence techniques for optimization of the air pollutant detection. Due to the great number of nodes deployed to provide relevant information of the IAQ distribution in different areas, the WSN systems may significantly enhance the efficiency of air quality monitoring and measurement. The WSN is an automatic IAQ monitoring system with an autonomous, accurate, and simultaneous measurement of IAQ in different areas, which allows the user or the building manager to get data in real time [ 192 ]. It has been found that the WSN systems are usually applied for the monitoring and detection of numerous common VOCs, such as benzene, ethylbenzene, toluene, and xylene [ 191 ], and a variety of environmental parameters, including, temperature, relative humidity, CO, CO 2 , and luminosity [ 192 ].
7.3. Air Purification Technologies for IAQ Improvement
Control of emission sources, development of air purification technologies, and optimization of ventilation systems are regarded as three main approaches to improve IAQ [ 193 ]. Among them, the investigation of novel technologies to filter and purify indoor air pollutants has attracted great attention over the last decade. Adsorption technologies using carbon-based filter media have been known as the leading technologies for removing gas pollutants [ 194 ]. There are numerous residential and commercial HVAC filters that have been developed and used in various buildings, i.e., household, shopping mall, school, and office building. It was reported that adsorptive HVAC filters may effectively remove several noxious pollutants, including O 3 , aerosols, VOCs, and PMs [ 195 ]. The HVAC filters can be designed in various shapes, such as filter cassettes made of flat sheet filter media and cartridges inserted by granulated activated carbon or bag filters [ 196 ]. However, this technology has exhibited a significant limitation in terms of the adsorption efficiency because its equilibrium adsorption capacity is significantly decreased at sub-ppm concentrations of pollutants [ 197 ]. Additionally, it has been demonstrated that the adsorption efficiency of carbon-based filters is remarkably reduced in the presence of humidity. Therefore, improvement of the long-term operation and performance of this air filter system is a potential approach in the development strategies of HVAC filters.
Long-term operation can induce the proliferation of molds and the growth of germs, which lead to the effective decrease of the air filters [ 198 ]. Non-thermal plasma (NTP) technologies have been demonstrated as an effective solution to resolve these problems. The operation of NTP techniques is based on the generation of a quasi-neutral environment, such as radicals, ions, electrons, and UV photons [ 199 ]. Several non-thermal discharge plasma techniques have been developed for the removal of indoor air contaminants, such as NO x , SO x , and VOCs, including corona discharge [ 200 ], dielectric barrier discharge [ 201 ], and surface discharge. It has been shown that ozone formed by the electric discharge exhibits a significant germicidal power and the air filter can function as an effective electric precipitator due to electrostatic force. However, NTP technologies also have some disadvantages: (i) Poor energy efficiency; (ii) strong influence of humidity on the performance; and (iii) the formation of secondary hazardous pollutants, such as CO, HCHO, and NO x [ 202 ]. These drawbacks have limited practical applications of this technique in the indoor air purification.
In the development strategies for indoor air pollution control, advanced oxidation processes (AOPs)-based technologies have attracted great attention due to the minimum generation of secondary pollution. Among them, photocatalysis, which is the process by which indoor air pollutants are disintegrated due to exposing semiconductor photocatalysts under sufficiently energetic irradiation, has been considered as a promising technology [ 203 ]. Compared with conventional adsorption technologies, photocatalysis exhibits several outstanding features: (i) Direct degradation of gaseous pollutants (particularly VOCs) into CO 2 and H 2 O under ambient conditions; and (ii) applicability for the removal of low-concentration pollutants (sub-ppm levels) [ 204 ]. The semiconductors used as photocatalysts in the photocatalysis technology include titanium dioxide (TiO 2 ), polymeric (or graphitic), tungsten oxide (WO 3 ), carbon nitride (CN), Bi, Ag, cadmium sulfide (CdS), metal oxides MO x (M = Fe, Zn, V), perovskite, and others [ 204 ]. However, due to the low cost, strong oxidative power, and chemical stability, TiO 2 has gained more attention for IAP purification [ 205 ].
7.4. Smart Home for IAQ Control
In recent years, home automation generally and the “smart home” particularly have attracted great interest. Now, the smart home, controllable via smartphone applications and IoT-based wearable devices, is a vital element of smart cities. Its foundation was the proposed home energy management system (HEMS) [ 206 , 207 ]. In fact, originally, the smart home was developed simply to enhance the energy-usage efficiency [ 208 , 209 ] and optimize ventilation technology [ 21 ]. However, the term ‘smart home’ these days is most often associated with various home automation systems, such as home appliances, communications, and entertainment electronics. The future development of smart home technology is likely to proceed in two general directions: (i) The above-mentioned control of ventilation, blinds, and appliances for a reduction of energy costs and enhancement of health, safety, and comfortability; and (ii) assistance of elderly and disabled people by utilization of robotic machines and smart sensor technologies [ 210 ]. However, the smart home also offers opportunities to reduce the negative impacts of IAP on human health. Certainly, as smart home technology is increasingly applied in buildings, IAQ and air hygiene will be taken more seriously. Normally, a smart building system consists of three main components: (i) User gateway media, (ii) power supply media, and (iii) controller and devices [ 206 ]. User gateway media is a combination of a smartphone application and a supervisory control and data acquisition (SCADA) interface, which allows occupants to monitor and manage appliances remotely through smartphones or computers using Wi-Fi or a wireless module. Power supply media is a novel system that optimizes energy efficiency by using renewable energy sources. Finally, controller and devices provide automatic control of the fan speed, temperature, and air pollutant concentrations. The key to smart buildings is modern sensor technology [ 211 ]. By the use of multiple sensors, all climatic parameters and concentrations of polluting substances are recorded automatically and constantly in the SCADA system. Especially, based on the data recorded thereby, smart sensor systems can take appropriate action (e.g., adjustment of ventilation settings and/or temperature) to improve IAQ and reduce IAP [ 210 ].
8. Conclusions
In conclusion, pollutants in the indoor air environment are significant contributing causes of human diseases. There are numerous indoor air pollutants, including PM, VOCs, CO, CO2, ozone, radon, heavy metals, aerosols, pesticides, biological allergens, and microorganisms, all of which can lead to diminished IAQ and thereby harmful effects on human health. Most of these pollutants usually originate from two main sources: (i) Human activities in buildings, such as combustion, cleaning, use of certain building materials in the course of construction or renovation, and operation of electronic machines; and (ii) transportation from outdoor sources. Although these pollutants are often present at only low concentrations in buildings, long-term exposure can cause significant risks to human health. Generally, there are two categories of building-associated illness: Sick building syndrome (SBS) and building-related illness (BRI). To reduce IAP’s impacts, many strategies and approaches for the control and reduction of pollutant concentrations have been taken. It is expected that the development of advanced materials for sensors, IAQ-monitoring systems, and the smart home will prove to be effective for the control and enhancement of IAQ into the future.
Author Contributions
Conceptualization, V.V.T. and Y.-C.L.; writing—original draft preparation, V.V.T. and D.P.; writing—review and editing, D.P. and Y.-C.L.; visualization, D.P.; supervision, Y.-C.L.; project administration, D.P. and Y.-C.L.; funding acquisition, D.P. All authors have read and agreed to the published version of the manuscript.
This research was funded by a grant from the Subway Fine Dust Reduction Technology Development Project of the Ministry of Land Infrastructure and Transport (19QPPW-B152306-01) and the Ministry of Environment as “Korea Environmental Industry & Technology Institute (KEITI) (No. 2018000120004)”.
Conflicts of Interest
The authors declare no conflict of interest.
Article
- Volume 16, issue 5
- ESSD, 16, 2385–2405, 2024
- Peer review
- Related articles
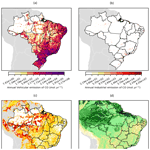
Brazilian Atmospheric Inventories – BRAIN: a comprehensive database of air quality in Brazil
Leonardo hoinaski, robson will, camilo bastos ribeiro.
Developing air quality management systems to control the impacts of air pollution requires reliable data. However, current initiatives do not provide datasets with large spatial and temporal resolutions for developing air pollution policies in Brazil. Here, we introduce the Brazilian Atmospheric Inventories (BRAIN), the first comprehensive database of air quality and its drivers in Brazil. BRAIN encompasses hourly datasets of meteorology, emissions, and air quality. The emissions dataset includes vehicular emissions derived from the Brazilian Vehicular Emissions Inventory Software (BRAVES), industrial emissions produced with local data from the Brazilian environmental agencies, biomass burning emissions from FINN – Fire INventory from the National Center for Atmospheric Research (NCAR), and biogenic emissions from the Model of Emissions of Gases and Aerosols from Nature (MEGAN) ( https://doi.org/10.57760/sciencedb.09858 , Hoinaski et al., 2023a; https://doi.org/10.57760/sciencedb.09886 , Hoinaski et al., 2023b). The meteorology dataset has been derived from the Weather Research and Forecasting Model (WRF) ( https://doi.org/10.57760/sciencedb.09857 , Hoinaski and Will, 2023a; https://doi.org/10.57760/sciencedb.09885 , Hoinaski and Will, 2023c). The air quality dataset contains the surface concentration of 216 air pollutants produced from coupling meteorological and emissions datasets with the Community Multiscale Air Quality Modeling System (CMAQ) ( https://doi.org/10.57760/sciencedb.09859 , Hoinaski and Will, 2023b; https://doi.org/10.57760/sciencedb.09884 , Hoinaski and Will, 2023d). We provide gridded data in two domains, one covering the Brazilian territory with 20×20 km spatial resolution and another covering southern Brazil with 4×4 km spatial resolution. This paper describes how the datasets were produced, their limitations, and their spatiotemporal features. To evaluate the quality of the database, we compare the air quality dataset with 244 air quality monitoring stations, providing the model's performance for each pollutant measured by the monitoring stations. We present a sample of the spatial variability of emissions, meteorology, and air quality in Brazil from 2019, revealing the hotspots of emissions and air pollution issues. By making BRAIN publicly available, we aim to provide the required data for developing air quality policies on municipal and state scales, especially for under-developed and data-scarce municipalities. We also envision that BRAIN has the potential to create new insights into and opportunities for air pollution research in Brazil.
- Article (PDF, 13528 KB)
- Supplement (215138 KB)
- Article (13528 KB)
- Full-text XML

Hoinaski, L., Will, R., and Ribeiro, C. B.: Brazilian Atmospheric Inventories – BRAIN: a comprehensive database of air quality in Brazil, Earth Syst. Sci. Data, 16, 2385–2405, https://doi.org/10.5194/essd-16-2385-2024, 2024.
It is consensus that air pollution threatens public health (OECD, 2024), economic progress (OECD, 2016), and climate (US EPA, 2023a). The negative outcomes associated with air pollution are not uniform within populations, and the impacts may be greater for more susceptible and exposed individuals (Makri and Stilianakis, 2008). Due to their social vulnerability and increasing emissions, developing countries urgently require reliable databases to provide information for designing air quality management systems to control air pollution (Sant'Anna et al., 2021).
Brazil has continental dimensions, is the seventh most populous country in the world, and has the 12th largest gross domestic product (IBGE, 2024). The combination of poorly planned development and the huge socioeconomic discrepancy has led to air quality impacts in Brazil. Air-pollution-related problems are not only restricted to great Brazilian cities and industrialized areas. Vehicular fleets and fuel consumption have also increased in small municipalities (CEIC, 2021; MME, 2023), posing a challenge to controlling vehicular emissions. In preserved and rural areas, large fire emissions have occurred due to illegal deforestation and poor soil management (Escobar, 2019; Rajão et al., 2020).
Following the practices of developed countries, Brazilian air quality policies have been enforced through legislative laws, using air quality standards as key components. However, the air quality management system remains incomplete in Brazil, with policies falling short of effectiveness due to a lack of air quality monitoring data across much of the country, primarily limited to well-developed areas (Sant'Anna et al., 2021). Moreover, Brazilian environmental agencies have not provided enough data and guidance to permit progress. Air quality consultants are still struggling to find mandatory inputs to understand and predict air quality for regulatory purposes. Efforts toward the permanent improvement of high-spatiotemporal-resolution emissions inventories and of meteorological and air quality data are needed.
An effective air quality management system must provide data to determine what emission reductions are needed to achieve the air quality standards (US EPA, 2023b). It requires air quality monitoring, a robust and detailed emissions inventory, reliable meteorological datasets, and methodologies to adapt the state-of-the-art air quality models to Brazil's reality. Moreover, it is crucial to undertake ongoing evaluation and to fully understand the air quality problem to design and implement the programs for pollution control. Currently, available initiatives including reanalysis and satellite products are still not providing datasets with large spatial and temporal resolutions for developing air pollution policies in Brazil. Global reanalyses such as the Copernicus Atmospheric Monitoring Service (CAMS) (Inness et al., 2019) and the second version of the Modern-Era Retrospective Analysis for Research and Applications (MERRA-2) (GMAO, 2015a, b) can provide estimates of air pollutants by combining chemical transport models (CTMs) with satellite and ground-based observations and physical information, assimilating data to constrain the results. However, the currently available reanalysis products do not provide data with high spatial resolution (up to 0.75° × 0.75° and 0.5° × 0.625°) and could be biased toward representing local and regional air quality (Arfan Ali et al., 2022). Moreover, they provide data for only a small list of air pollutants. Satellite-based products such as Sentinel-5P TROPOMI (Veefkind et al., 2012) and the Moderate-Resolution Imaging Spectroradiometer (MODIS) (Levy et al., 2015; Platnick et al., 2015) are still challenging due to their low temporal resolution, data gaps due to cloud coverage, and uncertainties (Shin et al., 2020). Besides, satellites rely on total tropospheric column measurements, which do not represent surface concentrations (Shin et al., 2019).
In this article, we present the Brazilian Atmospheric Inventories (BRAIN), the first comprehensive database to elaborate upon air quality management systems in Brazil. BRAIN combines local inventories, consolidated datasets, and the usage of internationally recommended models to provide hourly emissions and meteorological and air quality data covering the entire country.
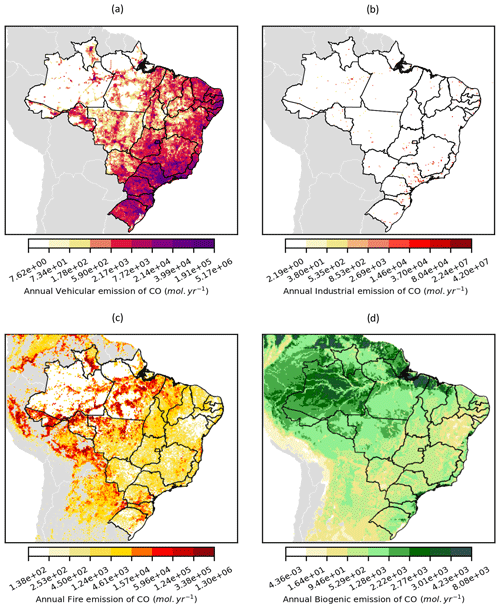
Figure 1 Spatial distribution of CO emissions from (a) vehicles, (b) industries, (c) biomass burning, and (d) biogenic sources, as provided by BRAIN.
BRAIN contains three types of hourly datasets: emissions, meteorology, and air quality. The emissions inventories include vehicular, industrial, biogenic, and biomass burning emissions. We provide meteorological data derived from the Weather Research and Forecasting (WRF) model. Coupling emissions, WRF, and the Community Multiscale Air Quality Modeling System (CMAQ) version 5.3.2, we provide air quality gridded data. All datasets are available on two spatial resolutions: the largest (Fig. S1 in the Supplement – d01) covers the entire country, while the smallest covers southern Brazil (Fig. S1 – d02). The BRAIN datasets in d01 are freely available at https://doi.org/10.57760/sciencedb.09858 (Hoinaski et al., 2023a), https://doi.org/10.57760/sciencedb.09857 (Hoinaski and Will, 2023a), and https://doi.org/10.57760/sciencedb.09859 (Hoinaski and Will, 2023b). The BRAIN datasets in d02 are available at https://doi.org/10.57760/sciencedb.09886 (Hoinaski et al., 2023b), https://doi.org/10.57760/sciencedb.09885 (Hoinaski and Will, 2023c), and https://doi.org/10.57760/sciencedb.09884 (Hoinaski and Will, 2023d). The Federal University of Santa Catarina (UFSC) institutional repository, https://brain.ens.ufsc.br/ (last access: 8 May 2024), and the web platform, https://hoinaski.prof.ufsc.br/BRAIN/ (last access: 8 May 2024), have served the BRAIN database since 2019. We envision making available more detailed datasets for other Brazilian regions, especially in the southeast, where the anthropogenic emission effects are more prominent. Future versions will also provide more detailed modeling outputs to properly cover medium- and small-sized cities.
BRAIN is intended to fill the gaps in those cases where adequately representative monitoring data to characterize the air quality are not available. BRAIN would be useful in providing background concentrations for a good procedure for licensing new sources of air pollution.
2.1 Emissions inventory
The BRAIN emissions inventory allows the spatiotemporal analysis of vehicular, industrial, biomass burning, and biogenic emissions in Brazil. The present version of this database does not account for other South American countries' emissions, apart from biomass burning and biogenic sources. We envision implementing other sources and a more detailed emissions inventory from other South American countries in a future version. Figure 1 presents a sample of the inventory, showing the annual carbon monoxide (CO) emissions by source. Section S2 in the Supplement (Table S1) summarizes the species in each emission source inventory. More information on each emissions dataset can be found in Sect. 2.1.1 to 2.1.5.
We observed higher vehicular emission rates of CO in urban areas with large population and vehicle fleet densities, mainly in the south and southeast (Fig. 1a). High industrial emission rates have been detected in the Brazilian regions, with large stationary sources such as refining units, thermoelectric power plants, and cement and paper industries (Fig. 1b) (Kawashima et al., 2020). In general, the north shows a higher concentration of biogenic and fire emissions. While the hotspots of biogenic emissions are predominately in the extreme north, the hotspots of fire emissions turn up in the midwestern, northern, and southern regions, as well as on the Brazilian western border (Fig. 1c–d).
2.1.1 Vehicular emissions
BRAIN uses the multispecies and high-spatiotemporal-resolution database of vehicular emissions from the Brazilian Vehicular Emission Inventory Software (BRAVES) (Hoinaski et al., 2022; Vasques and Hoinaski, 2021). The BRAVES database disaggregates municipality-aggregated emissions using the road density approach and temporal disaggregation based on vehicular flow profiles. SPECIATE 5.1 (Eyth et al., 2020) from the United States Environmental Protection Agency (USEPA, https://www.epa.gov/air-emissions-modeling/speciate , last access: 8 May 2024) speciates conventional pollutants in 41 species. BRAVES considers local studies (Nogueira et al., 2015) and data from Companhia Ambiental do Estado de São Paulo (CETESB) ( https://cetesb.sp.gov.br/veicular/relatorios-e-publicacoes/ , last access: 8 May 2024) to speciate acetaldehydes, formaldehyde, ethanol, and aldehydes in order to account for biofuel particularities in Brazil.
In BRAVES, vehicular activity is defined by fuel consumption in each municipality using data provided by the Brazilian National Agency for Oil, Natural Gas and Biofuel (ANP) ( https://www.gov.br/anp/pt-br/centrais-de-conteudo/dados-abertos/vendas-de-derivados-de-petroleo-e-biocombustiveis , last access: 8 May 2024). A fraction of fuel consumed by road transportation is based on data from the National Energy Balance (BEN) ( https://www.epe.gov.br/pt/publicacoes-dados-abertos/publicacoes/balanco-energetico-nacional-ben , last access: 8 May 2024), and MMA (2014). BRAVES calculates the weighted emission factor (EF) to address the effect of the fleet composition in terms of category, model year, and fuel utilization.
Vasques and Hoinaski (2021) compared BRAVES with different vehicular emission inventories from a local to national scale. On a national scale, vehicular emission rates from BRAVES underestimate the Emission Database for Global Atmospheric Research (EDGAR) and are slightly higher for CO (14 %) and non-methane volatile organic compounds (NMVOCs) (9 %) compared with the national inventory from Ministério do Meio Ambiente (MMA). The differences between estimates from BRAVES and from well-developed state inventories vary from −1 % to 35 % in São Paulo and from −2 % to 52 % in Minas Gerais. In addition, a relatively small bias between BRAVES and the Vehicular Emission Inventory (VEIN) was observed in São Paulo and Vale do Paraiba (Vasques and Hoinaski, 2021).
2.1.2 Industrial emissions
We derived the industrial emissions inventory by combining data from the state environmental agencies of Espírito Santo, Minas Gerais, and Santa Catarina. The emission rates of point sources from Espírito Santo and Minas Gerais are publicly provided by Instituto de Meio Ambiente e Recursos Hídricos do Espírito Santo (IEMA-ES) ( https://iema.es.gov.br/qualidadedoar/inventariodefontes , last access: 8 May 2024) and Fundação Estadual de Meio Ambiente (FEAM) ( http://www.feam.br/qualidade-do-ar/emissao-de-fontes-fixas , last access: 8 May 2024). Data from IEMA-ES contain emissions from the metropolitan region of Vitória from 2015, compiling measurements from regulatory procedures and emissions estimates. We did not convert the emissions inventory to the current modeling year since the data are not continuously updated. Therefore, we assumed that all emissions from these sources occurred in 2019.
In Santa Catarina, industrial emission data have been provided by Instituto de Meio Ambiente (IMA) ( https://www.ima.sc.gov.br/index.php , last access: 8 May 2024). These data are collected in the licensing process of potentially polluting industries. The base year of emission rates varies according to the availability. Summary information about the industrial sector types, the number of industries, and the respective emission rates in Santa Catarina can be found in Hoinaski et al. (2020) and at https://github.com/leohoinaski/IND_Inventory/blob/main/Inputs/BR_Ind.xlsx (last access: 8 May 2024). Emissions from large stationary sources (refining units, thermoelectric power plants, cement, and paper industries) provided by Kawashima et al. (2020) have been included when not encountered in the environmental agencies' inventories.
We chemically speciated the industrial emission rates by adopting the following steps: (i) grouping each point source using the same categories as in the Emission Database for Global Atmospheric Research (EDGAR) (Crippa et al., 2018) and the Intergovernmental Panel on Climate Change (IPCC) industrial segments, (ii) selecting compatible profiles in SPECIATE 5.1 for each group (Eyth et al., 2020), (iii) averaging the speciation factor by group and pollutant, and (iv) applying the speciation factor for the targeted pollutant (PM, NO x , VOCs). The SPECIATE 5.1 profiles used in this work are listed at https://github.com/leohoinaski/IND_Inventory/tree/main/IndustrialSpeciation (last access: 8 May 2024). The speciation factors by industrial group and pollutant are available at https://github.com/leohoinaski/IND_Inventory/blob/main/IndustrialSpeciation/IND_speciation.csv (last access: 8 May 2024).
We also vertically allocate the industrial emissions according to the plume's effective height, estimated by the sum of the geometric height and the superelevation of the plume. The plume superelevation was estimated by the Briggs method (Briggs, 1975, 1969). The initial vertical distribution of the plume has been estimated by disaggregating the emissions using a Gaussian approach, as proposed in the Sparse Matrix Operator Kernel Emissions (SMOKE) model (Bieser et al., 2011; Gordon et al., 2018; Guevara et al., 2014). Python code to estimate the plume's effective height and the initial vertical disaggregation of industrial emissions is available at https://doi.org/10.5281/zenodo.11167115 (Hoinaski, 2024a).
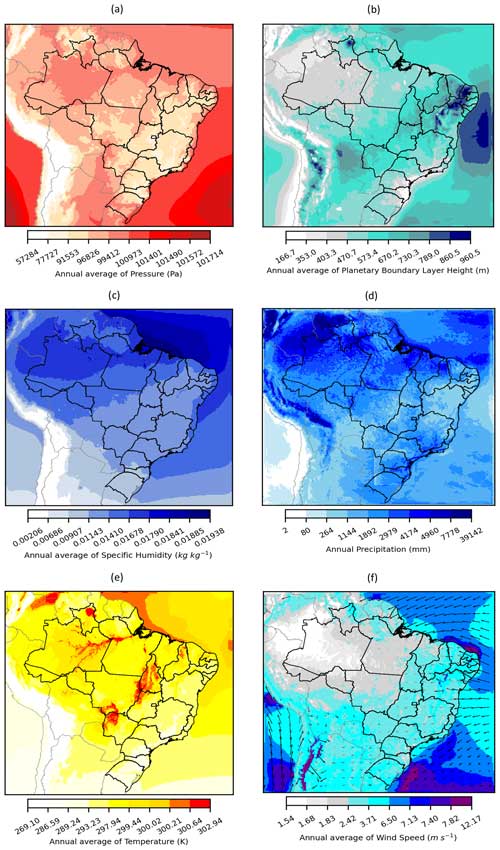
Figure 2 Annual average of meteorological variables in 2019, simulated by the WRF with 20 × 20 km resolution. (a) Atmospheric pressure, (b) planetary boundary layer height, (c) specific humidity, (d) annual accumulated precipitation, (e) temperature, (f) wind intensity and direction. All variables are annual averages except for precipitation, which represents the annual accumulated total.
2.1.3 Biomass burning emissions
The Fire INventory from NCAR (FINN) version 1.5 (Wiedinmyer et al., 2011) provides data on biomass burning emissions in BRAIN. FINN outputs contain daily emissions of trace gas and particle emissions from wildfires, agricultural fires, and prescribed burnings and do not include biofuel use and trash burning. Datasets have a 1 km spatial resolution and are available at https://www.acom.ucar.edu/Data/fire/ (last access: 8 May 2024).
Since CMAQ requires hourly emissions, a Python code ( https://github.com/barronh/finn2cmaq , last access: 8 May 2024) temporally disaggregates daily emissions into hourly emissions. The same code vertically splits the fire emissions to consider the plume rise effect and represents the vertical distribution (Henderson, 2022), converting text files into hourly 3D netCDF files.
Pereira et al. (2016) suggest that fire emissions estimated by FINN are strongly related to deforestation in many Brazilian regions. FINN estimates have a high correlation with both the Brazilian Biomass Burning Emission Model (3BEM) (0.86) and the Global Fire Assimilation System (GFAS) (0.84). The emissions estimated from FINN are commonly overestimated in comparison to other biomass burning emission inventories. An overestimation also occurs when FINN is used in air quality models and compared with observations. However, the use of FINN as input in air quality models can capture the temporal variability of pollutants emitted by biomass burning (Vongruang et al., 2017).
We have implemented the FINN v1.5 in this first version of BRAIN. However, FINN version 2.5 (Wiedinmyer et al., 2023) will be included in our emissions inventory in future work; this version uses an updated algorithm for determining fire size based on MODIS and Visible Infrared Imaging Radiometer Suite (VIIRS) satellite instruments. We also provide data from 2020 with the same modeling grid upgraded to FINN v2.5.
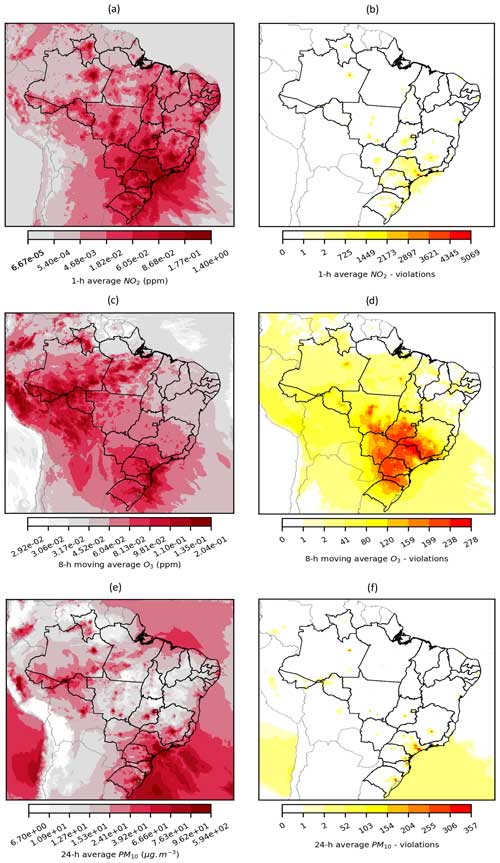
Figure 3 Spatial distribution of air pollutant concentration (a, c, e) and number of violations of air quality standards (b, d, f) for NO 2 (a–b) , O 3 (c–d) , and PM 10 (e–f) .
2.1.4 Biogenic emissions
We derived the biogenic emissions using the Model of Emissions of Gases and Aerosols from Nature (MEGAN) version 3.2 (Guenther et al., 2012; Silva et al., 2020). MEGAN is based on the leaf area index and plant functional groups. The model estimates emissions of gases and aerosols for different meteorological conditions and land cover types (Guenther et al., 2012). The leaf-level temperature and photosynthetically active radiation, as well as the vegetative stress conditions implemented in MEGAN, provide more physically realistic parameterizations for biosphere–atmosphere interactions (Silva et al., 2020). Input datasets, emission factor processors, and emission estimation modules are available at https://bai.ess.uci.edu/megan/data-and-code (last access: 8 May 2024). Data from WRF and the Meteorology-Chemistry Interface Processor (MCIP) have been used in MEGAN simulations.
MEGAN is commonly adopted to estimate emissions from biogenic fluxes, which constitute an important input for air quality modeling in many regions worldwide (Hogrefe et al., 2011; Kitagawa et al., 2022; Kota et al., 2015). Although MEGAN overestimates nighttime biogenic fluxes, the modeled emissions are correlated with measurements in the Amazon during both wet and dry seasons. The model is capable of capturing relatively well the seasonal variability of important organic pollutants in tropical forests (Sindelarova et al., 2014).
2.1.5 Sea spray aerosol emissions
Sea spray aerosol (SSA) is an important source of particles in the atmosphere. Due to its properties, SSA influences gas–particle partitioning in coastal environments (Gantt et al., 2015). SSA has been implemented in CMAQ as an inline source and requires the input of an ocean mask file (OCEAN) to identify the fractional coverage in each model grid cell allocated to the open ocean (OPEN) or surf zone (SURF). CMAQ uses this coverage information to calculate sea spray emission fluxes from the model's grid cells (US EPA, 2022). Detailed information on the mechanism of sea spray aerosol emissions and its implementation in CMAQ can be found in Gantt et al. (2015).
We provide a Python code ( https://github.com/leohoinaski/CMAQrunner/blob/master/hoinaskiSURFZONEv2.py , last access: 8 May 2024) to reproduce the OCEAN time-independent Input/Output Applications Programming Interface (I/O API) ( https://www.cmascenter.org/ioapi/ , last access: 8 May 2024) file so that it is ready to use in CMAQ. This code uses a shoreline Environmental Systems Research Institute (ESRI) shapefile from the National Oceanic and Atmospheric Administration (NOAA), available at https://www.ngdc.noaa.gov/mgg/shorelines/ (last access: 8 May 2024).
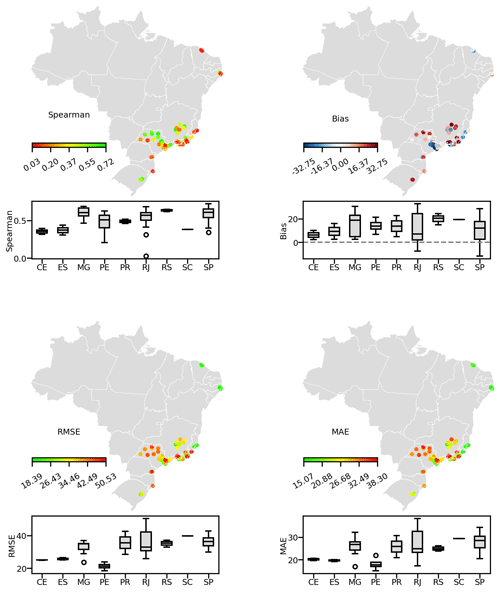
Figure 4 Spearman rank, bias, root mean squared error (RMSE), and mean absolute error (MAE) of O 3 dataset of BRAIN vs. observed values. Box plots of statistical metric by Brazilian state (considering only states with monitoring stations with representative data in 2019).
2.2 Meteorology
The WRF model has been used in this work to produce inputs for CMAQ and for meteorology characterization in Brazil. We provide hourly simulations in netCDF files. WRF has been set up to reproduce 36 h simulations, where the initial 12 h have been dedicated to model stabilization; these are excluded from the analysis. Thirty-three vertical levels have been employed, spaced at 50 hPa intervals. The parameterizations used in this work are described in Sect. S3. The remaining vertical levels followed a hybrid modeling scheme, accounting for terrain in the lower layers and gradually minimizing its influence at the higher levels. Details of WRF outputs can be found in Sect. S4 (Table S2).
The Global Forecast System (GFS) from the National Center for Atmospheric Research (NCAR) provided inputs with a spatial resolution of 0.25° × 0.25° and a temporal resolution of 6 h for the WRF simulations (Skamarock et al., 2008). Land use data and classification parameters are from the United States Geological Survey's (USGS) Moderate Resolution Imaging Spectroradiometer (MODIS).
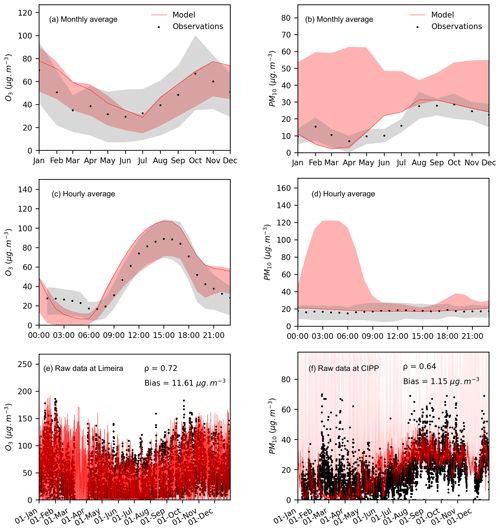
Figure 5 Time series of O 3 and PM 10 modeled and measured at Limeira (a, c, e) and CIPP (b, d, f) monitoring stations.
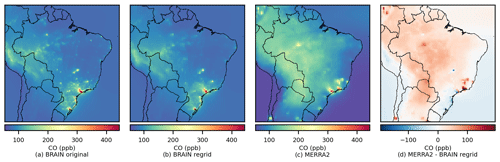
Figure 6 Annual average concentration of CO from BRAIN with its original resolution (a) , from BRAIN regridded to MERRA-2 resolution (b) , and from MERRA-2 (c) and the difference between MERRA-2 and BRAIN (d) .
Table 1 BRAIN datasets freely available.
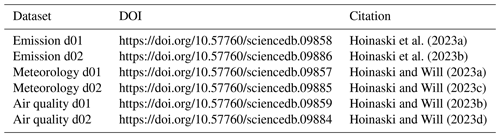
Download Print Version | Download XLSX
The Brazilian regions (north, northeast, midwest, southeast, and south) encompass three distinct climatic zones, namely the equatorial, tropical, and subtropical zones. The climatic diversity in Brazil is also shaped by topographical variations, landscape or vegetation, and the coastal areas. The temperature in Brazil follows a latitudinal pattern, increasing from south to north (Fig. 2e). The highest average temperatures are observed in the Amazon region, matching the historic data (Cavalcanti, 2016). The southern region exhibits the lowest average temperatures, which is also consistent with historical data (Cavalcanti, 2016).
The highest values of atmospheric pressure occurred in the northern region and in the extreme south of the country, and the lowest values were between the southeastern and southern regions (Fig. 2a). The planetary boundary layer height (PBLH) reaches the highest levels in the northeastern region and the lowest levels at the southern and southeastern coasts (Fig. 2b). The highest values of wind speed occurred in part of the northern and southern region. The Amazon region presented the lowest values of surface wind speed (Fig. 2f).
Humidity and precipitation exhibit similar patterns in the northern and northeastern regions (Fig. 2c, d) due to the trade winds that transport moisture from the tropical Atlantic (Mendonça and Danni-Oliveira, 2017). Except for the coast, the northeastern region is characterized by low humidity and drought during half of the year. The southern and southeastern regions have well-distributed rainfall throughout the year, as well as intermediate levels of humidity, except for the northern coast of the southern region, which has an elevated level of precipitation and humidity throughout the year.
The WRF model demonstrated the ability to reproduce diurnal and seasonal variability in winds in the Brazilian northeastern region (Souza et al., 2022a), although it underestimated the height of the planetary boundary layer (PBLH) by up to 20 %, as well as the temperature and humidity at 4 °C and 15 %, respectively. Pedruzzi et al. (2022) tested several model configurations, including an alternative land use scheme, and found a WRF tendency to overestimate temperature and humidity in the Brazilian southeastern region. Macedo et al. (2016) also evaluated the model's ability to predict extreme precipitation events. Although the WRF reasonably predicts the main meteorological aspects of the Brazilian southern region, the precipitation extremes were underestimated. A wind mapping study (Souza et al., 2022b) using WRF indicated that the average errors presented by the model in Brazil are minor, with an average bias of 2 m s −1 at 200 m in terms of wind intensity and errors at temperatures of 2 °C and humidity of approximately 10 %. Winds at lower levels tended to be overestimated, whereas PBLH was generally underestimated during the day.
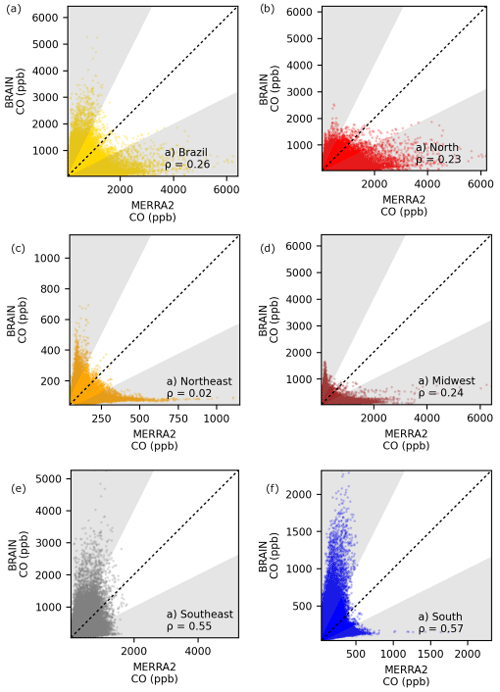
Figure 7 Concentration of CO from BRAIN vs. MERRA-2 in Brazil (a) , northern Brazil (b) , northeastern Brazil (c) , midwestern Brazil (d) , southeastern Brazil (e) , and southern Brazil (f) .
2.3 Air quality
We coupled emissions inventories, WRF, and CMAQ to produce the BRAIN air quality dataset for Brazil. CMAQ version 5.3.2 was set up using the third version of the Carbon Bond 6 chemical mechanism (cb6r3_ae7_aq) (Yarwood et al., 2010; Emery et al., 2015) with AERO7 treatment of secondary organic aerosol for standard cloud chemistry (Appel et al., 2021). The other model configurations used in this work can be found in Sect. S5 and at https://github.com/leohoinaski/CMAQrunner (last access: 8 May 2024). The pollutant list in CMAQ outputs, containing 216 species, can be found in Sect. S6 (Table S3).
The CMAQ standard profile of boundary conditions is used in the larger domain (d01), which provides the boundary conditions for the smaller one (d02). Further improvements to the database could include the boundary conditions derived from the GEOS-Chem model (Bey et al., 2001) ( https://geoschem.github.io/ , last access: 8 May 2024) or other better alternatives for the largest domain. The simulations have 24 h length and a time step interval of 1 h. The last hour of the previous simulation has been set up as the initial condition of the next one. We used the standard profile for the first hour of the first simulation (00:00:00 GMT on 1 January 2019). The figures with the spatial distribution and violations of criteria pollutants can be found in Sect. S7. Section S8 also presents the time series of criteria pollutants in Brazilian cities.
Using the BRAIN air quality dataset, we can observe the highest concentrations of NO 2 (Fig. 3a–b), O 3 (Fig. 3c–d), and PM 10 (Fig. 3e–f) in southeastern and southern Brazil. The concentration of O 3 violates the World Health Organization (WHO) air quality standards in multiple locations all over the country, while for NO 2 and PM 10 , this occurred mostly in southeastern and southern Brazil.
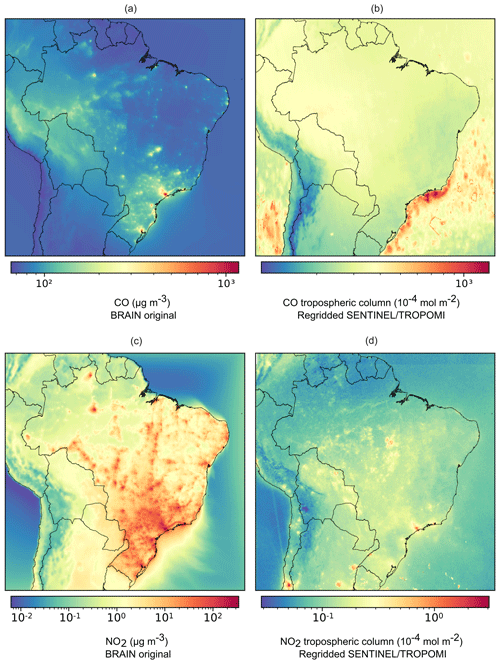
Figure 8 Annual average concentration of CO and NO 2 from BRAIN at its original resolution (a, c) and from Sentinel-5P TROPOMI spatially aligned to the BRAIN resolution (b, d) .
2.3.1 Models' performance
We sampled pixels around the monitoring station using a buffer of 0.5° to calculate the Spearman rank, bias, root mean squared error (RMSE), and mean absolute error (MAE) of the sampled pixels. We selected the highest Spearman rank of each pixel to demonstrate the model's performance in Figs. 4 and 5. Section S10 presents the box plots with overall statistical metrics for all stations. Section S11 presents statistical metrics by means of a monitoring station and pollutant, considering the pixel with the highest Spearman rank around each monitoring station. Section S12 presents the scatter plots comparing the BRAIN air quality dataset and the observations of each monitoring station. We used the simulations with domain d01 in the statistical analysis.
We observed the highest Spearman rank (0.72) in the state of São Paulo for O 3 concentration. Bias analysis revealed an underestimation in the São Paulo metropolitan area, while an overestimation occurred in Minas Gerais, Santa Catarina, Rio Grande do Sul, and the interior of São Paulo. In the northeast and in the state of Espírito Santo, bias is closer to zero. In Rio de Janeiro, the model over- and underestimated the observations. Regarding RMSE and MAE, the model performed better in coastal areas (maps in Fig. 4).
Comparing the states with air quality monitoring stations, the Spearman correlation of the O 3 dataset of BRAIN is higher in São Paulo, Minas Gerais, and Rio de Janeiro. However, these states also have a higher range of bias values, which could be negative and positive in São Paulo and Rio de Janeiro and are only positive in Minas Gerais (box plots in Fig. 4).
The heterogeneity in the stations' types and the insufficient spatial representativeness of observations in the Brazilian states must be considered while evaluating the model performance. According to the IEMA (2022), the strategic planning for the implementation of air quality monitoring stations, the financing and political efforts, and the technical characteristics (from installation to calibration and maintenance) vary significantly between Brazilian states. The lack of data quality assurance may compromise the credibility of the available air quality observations in Brazil.
BRAIN reproduced well the concentrations in moderately urbanized areas, such as Limeira and Piracicaba (Sect. S12). The database reached moderate performance in highly urbanized areas such as Copacabana and Rio de Janeiro (RJ) and at Marginal Tietê in the megacity of São Paulo (Sect. S12). Regarding the temporal profiles of O 3 and PM 10 , the seasonal and daily profiles are captured for both modeled pollutants, showing a suitable fit with the observations at the Limeira and Pecém Industrial and Port Complex (CIPP) air quality monitoring stations (Fig. 5). This reveals that the database can capture temporal patterns of air pollutant concentrations in urbanized and industrialized areas.
Figures with statistical metrics for other pollutants can be found in Sect. S13. Figures of modeled and observed time series for all monitoring stations can be found in Sect. S14.
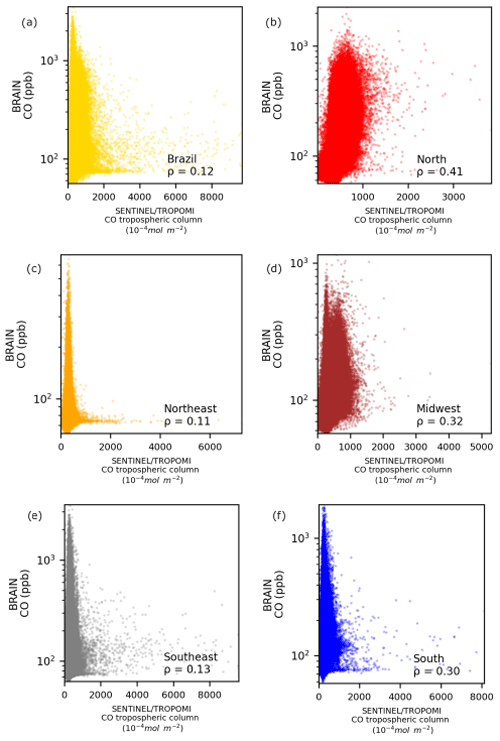
Figure 9 Concentration of CO from BRAIN vs. Sentinel-5P TROPOMI in Brazil (a) , northern Brazil (b) , northeastern Brazil (c) , midwestern Brazil (d) , southeastern Brazil (e) , and southern Brazil (f) .
Overall, the average concentrations are well simulated by CMAQ in BRAIN, with fair to good correlations (up to ∼ 0.7) between modeling and local measurements in São Paulo. Similar results have been reported by Albuquerque et al. (2018). Kitagawa et al. (2021) simulated PM 2.5 in a Brazilian coastal–urban area and showed that the CMAQ results commonly overestimated the observations, which agrees with the BRAIN air quality dataset. In another comparison between observations and CMAQ simulations (Kitagawa et al., 2022), the model overestimated the PM and NO 2 concentrations in the metropolitan region of Vitória (MRV) and underestimated O 3 . The authors suggest that the CMAQ simulations are suitable over the MRV, even though the model could not capture some local variabilities in air pollutant concentrations. It is already reported that the short-time abrupt variations are difficult to reproduce by means of air quality models (Albuquerque et al., 2018). The complex task of predicting air quality is associated with multiple error factors, including the lack of an emissions inventory, meteorology parameterizations, initial and boundary conditions, chemical mechanisms, numerical routines, etc. (Cheng et al., 2019; Albuquerque et al., 2018; Park et al., 2006; Pedruzzi et al., 2019).
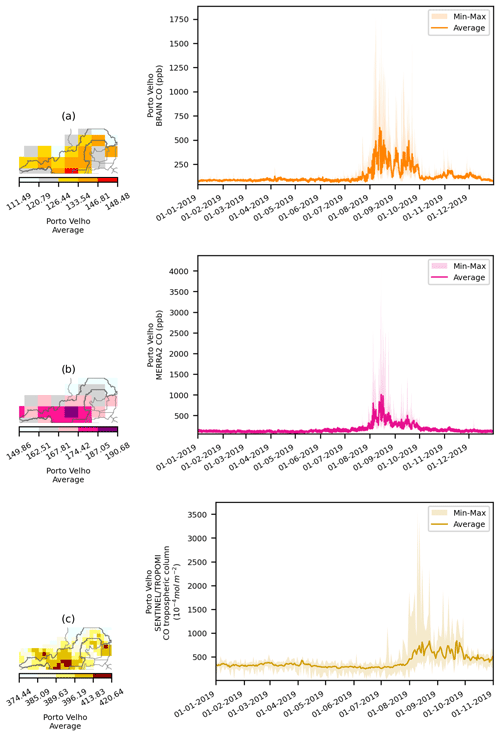
Figure 10 Annual average and hourly time series of CO from BRAIN (a) , MERRA-2 (b) , and Sentinel-5P TROPOMI (daily averages) (c) in Porto Velho, Brazil.
We analyzed the performances of 4×4 km simulations for CO, NO 2 , O 3 , and SO 2 , drawing a buffer of 0.5° degrees around monitoring station positions in southern Brazil. Our findings indicated higher Spearman values for the spatial resolution of 20×20 km for CO, O 3 , and SO 2 . Specifically, for O 3 , the best result at 20×20 km was 0.76, whereas the same point at 4×4 km resolution showed a correlation of 0.46. This pattern was also observed for CO, with the best result at 20×20 km being a Spearman value of 0.47 and 0.23 at the same point at 4×4 km resolution. The smallest differences in Spearman rank were observed for SO 2 (0.22: 20×20 , 0.19: 4×4 ). Even though improving the spatial resolution did not increase the correlation with measured data, we found the best results for bias, RMSE, and MAE for almost all pollutants at a 4×4 km resolution, except for CO. Please refer to Sect. S15 for the complete statistical analysis of 4×4 km simulations.
BRAIN captures seasonal patterns and the absolute magnitude of PM 2.5 in the northwest of the Amazonas state (near the Amazon Tall Tower Observatory – ATTO), as presented by Artaxo et al. (2013). This shows that our database can reproduce the concentrations in background areas (far from highly urbanized centers). Comparing BRAIN with observations at heavily biomass-burning-impacted sites in southwestern Amazonia (Porto Velho) (Artaxo et al., 2013) revealed that BRAIN can capture seasonal variations caused by wet and dry seasons, as well as the magnitude of average and peak concentrations. However, BRAIN PM 2.5 estimates are closer to the coarse mode of the time series rather than the fine mode shown in Artaxo et al. (2013). Even though BRAIN has captured the O 3 pattern observed by Artaxo et al. (2013), the estimates are around 2.7 times higher than the observations in the dry season and a factor of 2 higher for the wet season. It is worth mentioning that BRAIN uses 2019 data, while the study by Artaxo et al. (2013) consists of a sampling campaign from 2008 to 2012.
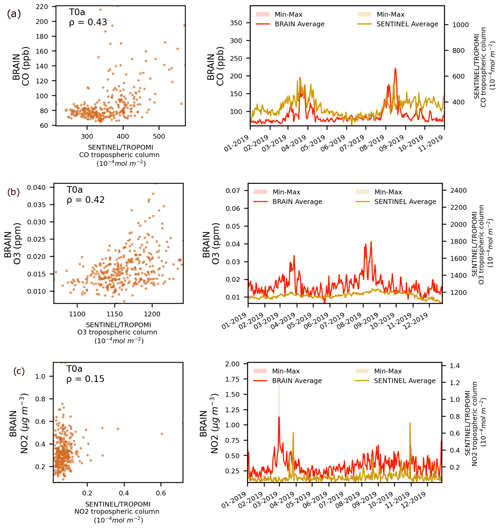
Figure 11 Scatter plot and daily time series of CO (a) , O 3 (b) , and NO 2 (c) from BRAIN and Sentinel-5P TROPOMI at T0a (GoAmazon reference). Values extracted using a buffer of 0.2° around the site.
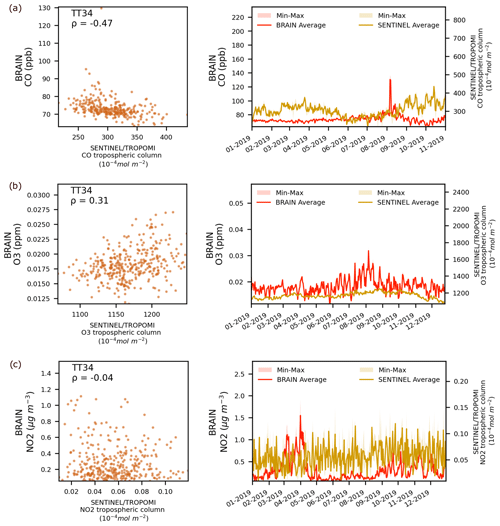
Figure 12 Scatterplot and daily time series of CO, O 3 , and NO 2 from BRAIN and Sentinel-5P TROPOMI at T0t/TT34 (GoAmazon reference). Values extracted using a buffer of 0.2° around the site.
BRAIN has a similar spatial pattern compared with MERRA-2 (GMAO, 2015a b), capturing hotspots in higher populated areas located in the southeast, south, and midwest. In the Amazon region, BRAIN can also capture hotspots similarly to MERRA-2 (Fig. 6). BRAIN estimates for carbon monoxide are lower than those of MERRA-2, except in the southern region and in some urban centers in the southeast and midwest (Fig. 6). Carbon monoxide concentrations estimated by BRAIN are moderately correlated with MERRA-2, mainly in the south (0.57) and southeast (0.55), while in the midwest, north, and northeast, the correlation is weaker (Fig. 7). Compared with the consolidated MERRA-2 database, BRAIN has the advantage since it uses local and more refined information and provides data at a higher spatial resolution for multiple species. We provide a detailed comparison between the MERRA-2 and BRAIN datasets for PM 2.5 , SO 2 , O 3 , and CO in Sect. S16.
We also compare our database with Sentinel-5P TROPOMI (Veefkind et al., 2012) data to demonstrate BRAIN's ability to capture the spatiotemporal variability of air pollutants in unmonitored areas (Fig. 8). We spatially realign Sentinel-5P TROPOMI products to the BRAIN resolution ( 20×20 km) using data from the NASA Goddard Earth Sciences Data and Information Services Center (GES-DISC) ( https://disc.gsfc.nasa.gov/ , last access: 8 May 2024). We merged all layers of the same day and interpolated them to match the BRAIN resolution. We computed the daily averages for both datasets. In this evaluation, we must consider the differences between the datasets since Sentinel-5P TROPOMI relies on tropospheric column measurements and BRAIN surface concentrations. BRAIN captured the hotspots of CO and NO 2 similarly to Sentinel-5P TROPOMI products, especially in southeastern Brazil. However, the hotspots of CO are dislocated towards the ocean in Sentinel-5P TROPOMI. NO 2 estimates from BRAIN present a higher number of hotspots. We emphasize that surface concentration data are more suitable than tropospheric column data in representing air quality. In this analysis, we removed negative values from Sentinel-5P TROPOMI products since they represent low-quality measurements (Eskes et al., 2022).
When we compared CO daily datasets from BRAIN and Sentinel-5P TROPOMI by Brazilian regions, we observed a moderate correlation in the north (0.41), midwest (0.32), and south (0.3). This analysis shows that BRAIN can reasonably detect temporal and spatial patterns of air pollutants. The complete comparison of CO and NO 2 from Sentinel-5P TROPOMI and BRAIN can be found in Sect. S17.
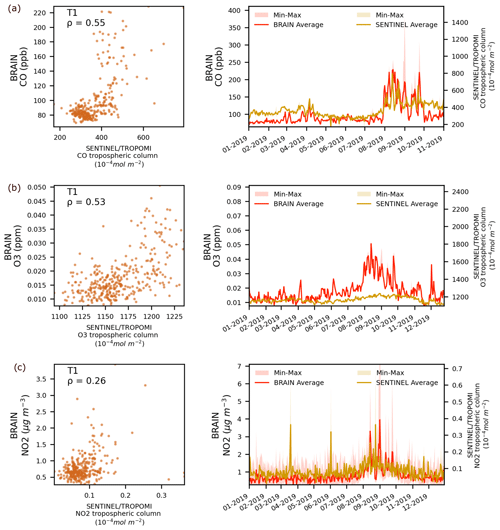
Figure 13 Scatter plot and daily time series of CO (a) , O 3 (b) , and NO 2 (c) from BRAIN and Sentinel-5P TROPOMI at T1 (GoAmazon reference). Values extracted using a buffer of 0.2° around the site.
We highlight that BRAIN, MERRA-2, and Sentinel-5P TROPOMI can capture similar temporal patterns of air pollutant concentrations in heavily biomass-burning-impacted sites such as Porto Velho in Rondônia (Fig. 10) and in urban areas such as São Paulo. We provide time series (minimum–maximum and average) of BRAIN, MERRA-2, and Sentinel-5P TROPOMI data spatially averaged within Brazilian capitals in Sect. S18. Section S19 contains time series (only average) of BRAIN data in Brazilian capitals.
To analyze BRAIN's performance in background regions (with low anthropogenic influence), we extracted data from two forested sites in the Brazilian northern region. As reference, we used the sampling sites of the GoAmazon experiment (Martin et al., 2016), named T0a (forested site situated 154.1 km from the Manaus urban area) and T0t/TT34 (a broken-canopy forested site situated 60.9 km from the city of Manaus). Sentinel-5P TROPOMI data spatially aligned to the BRAIN resolution were also extracted for comparison. A buffer of 0.2° around the sites selected the data of CO, O 3 , and NO 2 from both datasets. The results revealed that BRAIN captured the seasonal profile at T0a (Fig. 11), showing a moderate correlation with the tropospheric column measurements of Sentinel-5P TROPOMI, especially for CO and O 3 .
BRAIN estimates are slightly higher than the observed concentrations in background areas of CO, O 3 , and NO 2 in TT34 (Fig. 12) and T0a (Fig. 11). While O 3 concentrations simulated by BRAIN range around 18 ppb (average in 2019) at the TT34 site, observed concentrations in 2013 (Artaxo et al., 2013) were around 8.5 ppb ± 1.9 ppb. In T0a, BRAIN simulated concentrations around 16 ppb, overestimating the observations (7 ppb ± 2 ppb during the wet season from March to April 2013–2020) (Nascimento et al., 2022). Concerning CO, the concentrations simulated by BRAIN are slightly lower, ranging around 73 ppb (average) at TT34 compared to the 130 ppb observed during the GoAmazon experiment from 2010 to 2011 (Artaxo et al., 2013). We emphasize that the BRAIN and GoAmazon datasets are reported in different periods and, consequently, are influenced by different emission rates. For instance, fire emissions have changed significantly since 2011 in the Amazon (Copernicus, 2022; Naus et al., 2022).
We also analyzed BRAIN results in the Manaus urban area. We adopted the sampling site of the GoAmazon experiment (Martin et al., 2016) named T1 (INPA campus in Manaus). Compared with Sentinel-5P TROPOMI data, BRAIN reproduced fairly the temporal pattern of CO, O 3 , and NO 2 in the T1 site (Fig. 13). Abou Rafee et al. (2017) reported mean concentrations of 88.7 ppb for NO x and 382.6 pbb for CO in the Manaus urban area, while BRAIN reached 79 and 99 ppb (maximum of 383 ppb), revealing an underestimation in this area. Again, the sampling campaign presented by Abou Rafee et al. (2017) and the BRAIN simulations use different base years. Comparing BRAIN at T0a/TT34 (background sites) and T1 (urbanized), the database has reached consistent results, with lower concentration levels in preserved areas.
The inability to better predict the observations is mostly due to the quality of the emissions inventory. The lack of information on industrial emissions and their temporal variability is an important source of errors. Moreover, the vehicular emissions inventory also needs improvements to properly disaggregate the emissions in high-flow roads. Future versions of BRAIN could address these issues and incorporate other emission sources.
Emission data are available at https://doi.org/10.57760/sciencedb.09858 (Hoinaski et al., 2023a) and https://doi.org/10.57760/sciencedb.09886 (Hoinaski et al., 2023b). Meteorology data are available at https://doi.org/10.57760/sciencedb.09857 (Hoinaski and Will, 2023a) and https://doi.org/10.57760/sciencedb.09885 (Hoinaski and Will, 2023c). Air quality data are available at https://doi.org/10.57760/sciencedb.09859 (Hoinaski and Will, 2023b) and https://doi.org/10.57760/sciencedb.09884 (Hoinaski and Will, 2023d).
Code to generate the database, statistics, and figures is available at https://github.com/leohoinaski/CMAQrunner (last access: 8 May 2024) or https://doi.org/10.5281/zenodo.11166975 (Hoinaski, 2024b) and https://github.com/leohoinaski/IND_Inventory (last access: 8 May 2024) or https://doi.org/10.5281/zenodo.11167115 (Hoinaski, 2024a).
In this paper, we present BRAIN, the first comprehensive database for air quality management in Brazil. BRAIN provides emissions, meteorology, and air quality datasets for the entire country at a reliable spatiotemporal resolution. The BRAIN database covers a wide range of pollutant species (emissions and ambient concentrations) and atmospheric variables. So far, Brazil has lacked a comprehensive and easily accessible database for developing air quality management systems in urbanized and rural areas. This work contributes to overcoming this gap. BRAIN is a step forward toward a good procedure for licensing new sources of air pollution in Brazil.
Using a sample of BRAIN, we observed several violations of WHO air quality recommendations. The violations are not restricted to densely populated areas but also occur in rural ones. This reinforces the need for better air quality policies and a deep restructuring of the environmental agencies' procedures and data management in Brazil.
Compared with observations, the BRAIN air quality dataset has achieved good overall performance in predicting the criteria pollutants. However, there is plenty of room for improvement, mainly in relation to the quality of the emissions inventory. The lack of information on industrial emissions and their temporal variability is an important source of error. Moreover, the vehicular emissions inventory also needs improvements to properly disaggregate the emissions in high-flow roads. Improvements in boundary conditions and the inclusion of emission sources from other Latin American countries could also enhance the CMAQ performance. The influence of long-range transport will be addressed in a future version of the database by implementing boundary contributions from GEOSCHEM and other tools. Future versions of BRAIN could address these issues, incorporate other emission sources, and provide CMAQ outputs using different chemical mechanisms. We envision providing enough data to reproduce the historical pattern and future scenarios of air pollution in Brazil through a web platform to facilitate the access and usage of our database. We believe in an ongoing process that will improve the database.
The supplement related to this article is available online at: https://doi.org/10.5194/essd-16-2385-2024-supplement .
LH designed the methodology and developed the software. LH, RW, and CBR processed the data curation, conducted the formal analysis, and created the figures. LH, RW, and CBR prepared the original draft and revised the paper. LH is the project administrator and laboratory supervisor.
The contact author has declared that none of the authors has any competing interests.
Publisher’s note: Copernicus Publications remains neutral with regard to jurisdictional claims made in the text, published maps, institutional affiliations, or any other geographical representation in this paper. While Copernicus Publications makes every effort to include appropriate place names, the final responsibility lies with the authors.
The authors would like to thank the Secretaria de Estado do Desenvolvimento Econômico Sustentável do governo de Santa Catarina. The authors are grateful for the doctoral scholarships provided by the Coordenação de Aperfeiçoamento de Pessoal de Nível Superior – Brasil (CAPES).
This research has been supported by the Fundação de Amparo à Pesquisa e Inovação do Estado de Santa Catarina (grant no. 2018/TR/499; “Avaliação do impacto das emissões veiculares, queimadas, industriais e naturais na qualidade do ar em Santa Catarina”).
This paper was edited by Jing Wei and reviewed by two anonymous referees.
Abou Rafee, S. A., Martins, L. D., Kawashima, A. B., Almeida, D. S., Morais, M. V. B., Souza, R. V. A., Oliveira, M. B. L., Souza, R. A. F., Medeiros, A. S. S., Urbina, V., Freitas, E. D., Martin, S. T., and Martins, J. A.: Contributions of mobile, stationary and biogenic sources to air pollution in the Amazon rainforest: a numerical study with the WRF-Chem model, Atmos. Chem. Phys., 17, 7977–7995, https://doi.org/10.5194/acp-17-7977-2017 , 2017.
Albuquerque, T. T. A., de Fátima Andrade, M., Ynoue, R. Y., Moreira, D. M., Andreão, W. L., dos Santos, F. S., and Nascimento, E. G. S.: WRF-SMOKE-CMAQ modeling system for air quality evaluation in São Paulo megacity with a 2008 experimental campaign data, Environ. Sci. Pollut. Res., 25, 36555–36569, https://doi.org/10.1007/S11356-018-3583-9 , 2018.
Brazilian National Agency for Oil, Natural Gas and Biofuel (ANP): Vendas de derivados de petróleo e biocombustíveis, https://www.gov.br/anp/pt-br/centrais-de-conteudo/dados-abertos/vendas-de-derivados-de-petroleo-e-biocombustiveis (last access: 8 May 2024).
Appel, K. W., Bash, J. O., Fahey, K. M., Foley, K. M., Gilliam, R. C., Hogrefe, C., Hutzell, W. T., Kang, D., Mathur, R., Murphy, B. N., Napelenok, S. L., Nolte, C. G., Pleim, J. E., Pouliot, G. A., Pye, H. O. T., Ran, L., Roselle, S. J., Sarwar, G., Schwede, D. B., Sidi, F. I., Spero, T. L., and Wong, D. C.: The Community Multiscale Air Quality (CMAQ) model versions 5.3 and 5.3.1: system updates and evaluation, Geosci. Model Dev., 14, 2867–2897, https://doi.org/10.5194/gmd-14-2867-2021 , 2021.
Arfan Ali, Md., Bilal, M., Wang, Y., Nichol, J. E., Mhawish, A., Qiu, Z., de Leeuw, G., Zhang, Y., Zhan, Y., Liao, K., Almazroui, M., Dambul, R., Shahid, S., and Islam, M. N.: Accuracy assessment of CAMS and MERRA-2 reanalysis PM 2.5 and PM 10 concentrations over China, Atmos. Environ., 288, 119297, https://doi.org/10.1016/j.atmosenv.2022.119297 , 2022.
Artaxo, P., Rizzo, L. V., Brito, J. F., Barbosa, H. M., Arana, A., Sena, E. T., Cirino, G. G., Bastos, W., Martin, S. T., and Andreae, M. O.: Atmospheric aerosols in Amazonia and land use change: from natural biogenic to biomass burning conditions, Faraday Discuss., 165, 203–235, https://doi.org/10.1039/C3FD00052D , 2013.
Bey, I., Jacob, D. J., Yantosca, R. M., Logan, J. A., Field, B. D., Fiore, A. M., Li, Q., Liu, H. Y., Mickley, L. J., and Schultz, M. G.: Global modeling of tropospheric chemistry with assimilated meteorology: Model description and evaluation, J. Geophys. Res.-Atmos., 106, 23073–23095, https://doi.org/10.1029/2001JD000807 , 2001.
Bieser, J., Aulinger, A., Matthias, V., Quante, M., and Denier Van Der Gon, H. A. C.: Vertical emission profiles for Europe based on plume rise calculations, Environ. Pollut., 159, 2935–2946, https://doi.org/10.1016/J.ENVPOL.2011.04.030 , 2011.
Briggs, G. A.: Plume Rise: A Critical Survey, https://doi.org/10.2172/4743102 , 1969.
Briggs, G. A.: Plume rise predictions, TENNESSEE, U.S.A., Environ. Res. Labs., 59–111, https://doi.org/10.1007/978-1-935704-23-2_3 , 1975.
Cavalcanti, I. F.: Tempo e clima no Brasil, Oficina de textos, eISBN 978-85-7975-234-6, 2016.
CEIC: Brazil Vehicel Fleet: by Region, https://www.ceicdata.com/en/brazil/vehicle-fleet-by-region (last access: 8 May 2024) 2021.
Cheng, J., Su, J., Cui, T., Li, X., Dong, X., Sun, F., Yang, Y., Tong, D., Zheng, Y., Li, Y., Li, J., Zhang, Q., and He, K.: Dominant role of emission reduction in PM 2.5 air quality improvement in Beijing during 2013–2017: a model-based decomposition analysis, Atmos. Chem. Phys., 19, 6125–6146, https://doi.org/10.5194/acp-19-6125-2019 , 2019.
Copernicus: Wildfires: Amazonas records highest emissions in 20 years, https://atmosphere.copernicus.eu/wildfires-amazonas-records-highest-emissions-20-years (last access: 8 May 2024), 2022.
Crippa, M., Guizzardi, D., Muntean, M., Schaaf, E., Dentener, F., van Aardenne, J. A., Monni, S., Doering, U., Olivier, J. G. J., Pagliari, V., and Janssens-Maenhout, G.: Gridded emissions of air pollutants for the period 1970–2012 within EDGAR v4.3.2, Earth Syst. Sci. Data, 10, 1987–2013, https://doi.org/10.5194/essd-10-1987-2018 , 2018.
Eskes, H., Van Geffen, J., Boersma, F., Eichmann, K.U., Apituley, A., Pedergnana, M., Sneep, M., Veefkind, J.P., and Loyola, D.: Sentinel-5 precursor/TROPOMI Level 2 Product User Manual Nitrogendioxide document number: S5P-KNMI-L2-0021-MA, https://sentinels.copernicus.eu/documents/247904/2474726/Sentinel-5P-Level-2-Product-User-Manual-Nitrogen-Dioxide.pdf/ad25ea4c-3a9a-3067-0d1c-aaa56eb1746b?t=1658312035057 (last access: 8 May 2024), 2022.
Emery, C., Jung, J., Koo, B., and Yarwood, G.: Final Report, Improvements to CAMx Snow Cover Treatments and Carbon Bond Chemical Mechanism for Winter Ozone, Tech. rep., Ramboll Environ, Novato, CA, USA, https://www.camx.com/files/udaq_snowchem_final_6aug15.pdf (last access: 8 May 2024), 2015.
Escobar, H.: Amazon fires clearly linked to deforestation, scientists say, Science, 80, 853, https://doi.org/10.1126/science.365.6456.853 , 2019.
Eyth, A., Strum, M., Murphy, B., Epa, U.S., Shah, T., Shi, Y., Beardsley, R., Yarwood, G., and Houyoux, M.: Speciation Tool User's Guide Speciation Tool User's Guide Version 5.0 Ramboll-Speciation Tool User's Guide, https://www.cmascenter.org/speciation_tool/documentation/5.1/Ramboll_sptool_users_guide_V5.pdf (last access: 8 May 2024), 2020.
Gantt, B., Kelly, J. T., and Bash, J. O.: Updating sea spray aerosol emissions in the Community Multiscale Air Quality (CMAQ) model version 5.0.2, Geosci. Model Dev., 8, 3733–3746, https://doi.org/10.5194/gmd-8-3733-2015 , 2015.
Global Modeling and Assimilation Office (GMAO): MERRA-2 tavg1_2d_chm_Nx: 2d, 1-Hourly, Time-Averaged, Single-Level, Assimilation, Carbon Monoxide and Ozone Diagnostics V5.12.4, Greenbelt, MD, USA, Goddard Earth Sciences Data and Information Services Center (GES DISC) [data set], https://doi.org/10.5067/3RQ5YS674DGQ , 2015a.
Global Modeling and Assimilation Office (GMAO): MERRA-2 tavg1_2d_aer_Nx: 2d, 1-Hourly, Time-averaged, Single-Level, Assimilation, Aerosol Diagnostics V5.12.4, Greenbelt, MD, USA, Goddard Earth Sciences Data and Information Services Center (GES DISC) [data set], https://doi.org/10.5067/KLICLTZ8EM9D , 2015b.
Gordon, M., Makar, P. A., Staebler, R. M., Zhang, J., Akingunola, A., Gong, W., and Li, S.-M.: A comparison of plume rise algorithms to stack plume measurements in the Athabasca oil sands, Atmos. Chem. Phys., 18, 14695–14714, https://doi.org/10.5194/acp-18-14695-2018 , 2018.
Guenther, A. B., Jiang, X., Heald, C. L., Sakulyanontvittaya, T., Duhl, T., Emmons, L. K., and Wang, X.: The Model of Emissions of Gases and Aerosols from Nature version 2.1 (MEGAN2.1): an extended and updated framework for modeling biogenic emissions, Geosci. Model Dev., 5, 1471–1492, https://doi.org/10.5194/gmd-5-1471-2012 , 2012.
Guevara, M., Soret, A., Arévalo, G., Martínez, F., and Baldasano, J. M.: Implementation of plume rise and its impacts on emissions and air quality modelling, Atmos. Environ., 99, 618–629, https://doi.org/10.1016/J.ATMOSENV.2014.10.029 , 2014.
Henderson, B.: [Fire emission pre-processor for CMAQ], finntocmaq, GitHub [code], https://github.com/barronh/finn2cmaq (last access: 8 May 2024), 2022.
Hogrefe, C., Isukapalli, S. S., Tang, X., Georgopoulos, P. G., He, S., Zalewsky, E. E., Hao, W., Ku, J. Y., Key, T., and Sistla, G.: Impact of Biogenic Emission Uncertainties on the Simulated Response of Ozone and Fine Particulate Matter to Anthropogenic Emission Reductions, J. Air Waste Manage., 61, 92–108, https://doi.org/10.3155/1047-3289.61.1.92 , 2011.
Hoinaski, L.: leohoinaski/IND_Inventory: IND2CMAQ_v1.0 (IND2CMAQ_v1.0), Zenodo [code], https://doi.org/10.5281/zenodo.11167115 , 2024a.
Hoinaski, L.: CMAQrunner_v1.0, Zenodo [code], https://doi.org/10.5281/zenodo.11166975 , 2024b.
Hoinaski, L. and Will, R.: Brazilian Atmospheric Inventories – BRAIN version 1: meteorology dataset in Brazil, V1, Science Data Bank [data set], https://doi.org/10.57760/sciencedb.09857 , 2023a.
Hoinaski, L. and Will, R.: Brazilian Atmospheric Inventories – BRAIN version 1: air quality dataset in Brazil, V1, Science Data Bank [data set], https://doi.org/10.57760/sciencedb.09859 , 2023b.
Hoinaski, L., and Will, R.: Brazilian Atmospheric Inventories – BRAIN version 1: meteorology dataset in Southern Brazil, V1, Science Data Bank [data set], https://doi.org/10.57760/sciencedb.09885 , 2023c.
Hoinaski, L. and Will, R.: Brazilian Atmospheric Inventories – BRAIN version 1: air quality dataset in Southern Brazil, V1, Science Data Bank [data set], https://doi.org/10.57760/sciencedb.09884 , 2023d.
Hoinaski, L., Ribeiro, C. B., Santos, O. N., Vasques, T. V., Meotti, B., Will, R., and Rodella, F. H. C.: Avaliação do impacto das emissões veiculares, queimadas, industriais e naturais na qualidade do ar em Santa Catarina, 2020.
Hoinaski, L., Vasques, T. V., Ribeiro, C. B., and Meotti, B.: Multispecies and high-spatiotemporal-resolution database of vehicular emissions in Brazil, Earth Syst. Sci. Data, 14, 2939–2949, https://doi.org/10.5194/essd-14-2939-2022 , 2022.
Hoinaski, L., Will, R., and Ribeiro, C. B.: Brazilian Atmospheric Inventories – BRAIN version 1: emission dataset in Brazil, V1, Science Data Bank [data set], https://doi.org/10.57760/sciencedb.09858 , 2023a.
Hoinaski, L., Will, R., and Ribeiro, C. B.: Brazilian Atmospheric Inventories – BRAIN version 1: emission dataset in Southern Brazil, V1, Science Data Bank [data set], https://doi.org/10.57760/sciencedb.09886 , 2023b.
Inness, A., Ades, M., Agustí-Panareda, A., Barré, J., Benedictow, A., Blechschmidt, A.-M., Dominguez, J. J., Engelen, R., Eskes, H., Flemming, J., Huijnen, V., Jones, L., Kipling, Z., Massart, S., Parrington, M., Peuch, V.-H., Razinger, M., Remy, S., Schulz, M., and Suttie, M.: The CAMS reanalysis of atmospheric composition, Atmos. Chem. Phys., 19, 3515–3556, https://doi.org/10.5194/acp-19-3515-2019 , 2019.
Instituto Brasileiro de Geografia e Estatística (IBGE): Brasil em síntese, https://brasilemsintese.ibge.gov.br/territorio.html , last access: 8 May 2024.
Instituto de Energia e Meio Ambiente (IEMA): Recomendações para a expansão e a continuidade das redes de monitoramento da qualidade do ar no Brasil, https://energiaeambiente.org.br/wp-content/uploads/2022/07/IEMA_policypaper_qualidadedoar.pdf (last access: 8 May 2024), 2022.
Instituto de Energia e Meio Ambiente (IEMA): Plataforma de qualidade do ar, https://energiaeambiente.org.br/qualidadedoar/ , last access: 8 May 2024.
Kawashima, A. B., Martins, L. D., Abou Rafee, S. A., Rudke, A. P., de Morais, M. V., and Martins, J. A.: Development of a spatialized atmospheric emission inventory for the main industrial sources in Brazil, Environ. Sci. Pollut. Res., 27, 35941–35951, https://doi.org/10.1007/S11356-020-08281-7 , 2020.
Kitagawa, Y. K. L., Pedruzzi, R., Galvão, E. S., de Araújo, I. B., de Almeira Alburquerque, T. T., Kumar, P., Nascimento, E. G. S., and Moreira, D. M.: Source apportionment modelling of PM 2.5 using CMAQ-ISAM over a tropical coastal-urban area, Atmos. Pollut. Res., 12, 101250, https://doi.org/10.1016/J.APR.2021.101250 , 2021.
Kitagawa, Y. K. L., Kumar, P., Galvão, E. S., Santos, J. M., Reis, N. C., Nascimento, E. G. S., and Moreira, D. M.: Exposure and dose assessment of school children to air pollutants in a tropical coastal-urban area, Sci. Total Environ., 803, 149747, https://doi.org/10.1016/J.SCITOTENV.2021.149747 , 2022.
Kota, S. H., Schade, G., Estes, M., Boyer, D., and Ying, Q.: Evaluation of MEGAN predicted biogenic isoprene emissions at urban locations in Southeast Texas, Atmos. Environ., 110, 54–64, https://doi.org/10.1016/J.ATMOSENV.2015.03.027 , 2015.
Levy, R. and Hsu, C.: MODIS Atmosphere L2 Aerosol Product, NASA MODIS Adaptive Processing System, Goddard Space Flight Center, USA [data set], https://doi.org/10.5067/MODIS/MOD04_L2.006 , 2015.
Macedo, L. R., Basso, J. L. M., Yamasaki, Y., Macedo, L. R., Basso, J. L. M., and Yamasaki, Y.: Evaluation of the WRF Weather Forecasts over the Southern Region of Brazil, Am. J. Clim. Chang., 5, 103–115, https://doi.org/10.4236/AJCC.2016.51011 , 2016.
Makri, A. and Stilianakis, N. I.: Vulnerability to air pollution health effects, Int. J. Hyg. Environ. Health, 211, 326–336, https://doi.org/10.1016/J.IJHEH.2007.06.005 , 2008.
Martin, S. T., Artaxo, P., Machado, L. A. T., Manzi, A. O., Souza, R. A. F., Schumacher, C., Wang, J., Andreae, M. O., Barbosa, H. M. J., Fan, J., Fisch, G., Goldstein, A. H., Guenther, A., Jimenez, J. L., Pöschl, U., Silva Dias, M. A., Smith, J. N., and Wendisch, M.: Introduction: Observations and Modeling of the Green Ocean Amazon (GoAmazon2014/5), Atmos. Chem. Phys., 16, 4785–4797, https://doi.org/10.5194/acp-16-4785-2016 , 2016.
Mendonça, F. and Danni-Oliveira, I. M.: Climatologia: noções básicas e climas do Brasil, Oficina de textos, eISBN 978-85-7975-114-1, 2017.
Ministério de Minas e Energia (MME): Dados estatísticos, https://www.gov.br/anp/pt-br/centrais-de-conteudo/dados-estatisticos (last access: 8 May 2024).
Ministério do Meio Ambiente (MMA): Inventário Nacional de Emissões Atmosféricas por Veículos Automotores Rodoviários 2013, https://antigo.mma.gov.br/images/arquivo/80060/Inventario_de_Emissoes_por_Veiculos_Rodoviarios_2013.pdf (last access: 8 May 2024), 2014.
National Energy Balance (BEN): Balanço Energético Nacional, https://www.epe.gov.br/pt/publicacoes-dados-abertos/publicacoes/balanco-energetico-nacional-ben , last access: 8 May 2024.
Nascimento, J. P., Barbosa, H. M. J., Banducci, A. L., Rizzo, L. V., Vara-Vela, A. L., Meller, B. B., Gomes, H., Cezar, A., Franco, M. A., Ponczek, M., Wolff S., Bela, M. M., and Artaxo, P.: Major Regional-Scale Production of O 3 and Secondary Organic Aerosol in Remote Amazon Regions from the Dynamics and Photochemistry of Urban and Forest Emissions, Environ. Sci. Technol., 56, 9924–9935, https://doi.org/10.1021/acs.est.2c01358 , 2022.
Naus, S., Domingues, L. G., Krol, M., Luijkx, I. T., Gatti, L. V., Miller, J. B., Gloor, E., Basu, S., Correia, C., Koren, G., Worden, H. M., Flemming, J., Pétron, G., and Peters, W.: Sixteen years of MOPITT satellite data strongly constrain Amazon CO fire emissions, Atmos. Chem. Phys., 22, 14735–14750, https://doi.org/10.5194/acp-22-14735-2022 , 2022.
Nogueira, T., de Souza, K. F., Fornaro, A., de Fatima Andrade, M., and de Carvalho, L. R. F.: On-road emissions of carbonyls from vehicles powered by biofuel blends in traffic tunnels in the Metropolitan Area of Sao Paulo, Brazil, Atmos. Environ., 108, 88–97, https://doi.org/10.1016/J.ATMOSENV.2015.02.064 , 2015.
Organization of Economic Co-operation and Development (OECD): The economic consequences of outdoor air pollution, https://www.oecd.org/env/the-economic-consequences-of-outdoor-air-pollution-9789264257474-en.htm (last access: 8 May 2024), 2016.
Organization of Economic Co-operation and Development (OECD): Air pollution, https://www.oecd.org/environment/air-pollution/ , last access: 8 May 2024.
Park, S. K., Evan Cobb, C., Wade, K., Mulholland, J., Hu, Y., and Russell, A. G.: Uncertainty in air quality model evaluation for particulate matter due to spatial variations in pollutant concentrations, Atmos. Environ., 40, 563–573, https://doi.org/10.1016/J.ATMOSENV.2005.11.078 , 2006.
Pedruzzi, R., Baek, B. H., Henderson, B. H., Aravanis, N., Pinto, J. A., Araujo, I. B., Nascimento, E. G. S., Reis Junior, N. C., Moreira, D. M., and de Almeida Albuquerque, T. T.: Performance evaluation of a photochemical model using different boundary conditions over the urban and industrialized metropolitan area of Vitória, Brazil, Environ. Sci. Pollut. Res., 26, 16125–16144, https://doi.org/10.1007/S11356-019-04953-1 , 2019.
Pedruzzi, R., Andreão, W. L., Baek, B. H., Hudke, A. P., Glotfelty, T. W., Dias de Freitas, E., Martins, J. A., Bowden, J. H., Pinto, J. A., Alonso, M. F., and de Almeida Abuquerque, T. T.: Update of land use/land cover and soil texture for Brazil: Impact on WR F modeling results over São Paulo, Atmos. Environ., 268, 118760, https://doi.org/10.1016/J.ATMOSENV.2021.118760 , 2022.
Pereira, G., Siqueira, R., Rosário, N. E., Longo, K. L., Freitas, S. R., Cardozo, F. S., Kaiser, J. W., and Wooster, M. J.: Assessment of fire emission inventories during the South American Biomass Burning Analysis (SAMBBA) experiment, Atmos. Chem. Phys., 16, 6961–6975, https://doi.org/10.5194/acp-16-6961-2016 , 2016.
Platnick, S., Hubanks, P., Meyer, K., and King, M. D.: MODIS Atmosphere L3 Monthly Product (08_L3), NASA MODIS Adaptive Processing System, Goddard Space Flight Center [data set], https://doi.org/10.5067/MODIS/MOD08_M3.006 , 2015.
Rajão, R., Soares-Filho, B., Nunes, F., Börner, J., Machado, L., Assis, D., Oliveira, A., Pinto, L., Ribeiro, V., Rausch, L., Gibbs, H., and Figueira, D.: The rotten apples of Brazil's agribusiness, Science, 80, 369, 246–248, https://doi.org/10.1126/SCIENCE.ABA6646 , 2020.
Sant'Anna, A., Alencar, A., Araújo, C., Vormittag, E., Wicher, H., Cunha, K. B. da, Faria, M., de Fatima Andrade, M., Porto, P., Artaxo, P., Rocha, R., Simoni, W. De, Pinheiro, B., and Esturba, T.: O Estado da Qualidade do Ar no Brasil, https://www.wribrasil.org.br/sites/default/files/wri-o-estado-da-_qualidade-do-ar-no-brasil.pdf (last access: 8 May 2024), 2021.
Shin, M., Kang, Y., Park, S., Im, J., Yoo, C., and Quackenbush, L. J.: Estimating ground-level particulate matter concentrations using satellite-based data: a review, GISci. Remote Sens., 57, 174–189, https://doi.org/10.1080/15481603.2019.1703288 , 2020.
Skamarock, W. C., Klemp, J. B., Dudhia, J., Gill, D. O., Barker, D., Duda, M. G., and Powers, J. G.: A Description of the Advanced Research WRF Version 3 (No. NCAR/TN-475 + STR), University Corporation for Atmospheric Research, https://doi.org/10.5065/D68S4MVH , 2008.
Silva, S. J., Heald, C. L., and Guenther, A. B.: Development of a reduced-complexity plant canopy physics surrogate model for use in chemical transport models: a case study with GEOS-Chem v12.3.0, Geosci. Model Dev., 13, 2569–2585, https://doi.org/10.5194/gmd-13-2569-2020 , 2020.
Sindelarova, K., Granier, C., Bouarar, I., Guenther, A., Tilmes, S., Stavrakou, T., Müller, J.-F., Kuhn, U., Stefani, P., and Knorr, W.: Global data set of biogenic VOC emissions calculated by the MEGAN model over the last 30 years, Atmos. Chem. Phys., 14, 9317–9341, https://doi.org/10.5194/acp-14-9317-2014 , 2014.
Souza, N. B. P., Cardoso dos Santos, J. V., Sperandio Nascimento, E. G., Bandeira Santos, A. A., and Moreira, D. M.: Long-range correlations of the wind speed in a northeast region of Brazil, Energy, 243, 122742, https://doi.org/10.1016/J.ENERGY.2021.122742 , 2022a.
Souza, N. B. P., Sperandio, N. E. G., Santos, A. A. B., and Moreira, D. M.: Wind mapping using the mesoscale WRF model in a tropical region of Brazil, Energy, 240, 122491, https://doi.org/10.1016/J.ENERGY.2021.122491 , 2022b.
United States Environmental Protection Agency (U.S. EPA): Creating an OCEAN file for input to CMAQ, CMAQ, GitHub [code], https://github.com/U.S.EPA/CMAQ/blob/main/DOCS/Users_Guide/Tutorials/CMAQ_UG_tutorial_oceanfile.md (last access: 8 May 2024), 2022.
United States Environmental Protection Agency (U.S. EPA): Air Quality and Climate Change Research, https://www.epa.gov/air-research/air-quality-and-climate-change-research (last access: 8 May 2024), 2023a.
United States Environmental Protection Agency (U.S. EPA): Air Quality System (AQS), https://www.epa.gov/aqs (last access: 8 May 2024), 2023b.
Vasques, T. V., and Hoinaski, L.: Brazilian vehicular emission inventory software – BRAVES, Transp. Res. Part D Transp. Environ., 100, 103041, https://doi.org/10.1016/J.TRD.2021.103041 , 2021.
Veefkind, J. P., Aben, I., McMullan, K., Förster, H., de Vries, J., Otter, G., Claas, J., Eskes, H. J., de Haan, J. F., Kleipool, Q., van Weele, M., Hasekamp, O., Hoogeveen, R., Landgraf, J., Snel, R., Tol, P., Ingmann, P., Voors, R., Kruizinga, B., Vink, R., Visser, H., and Levelt, P. F.: TROPOMI on the ESA Sentinel-5 Precursor: A GMES mission for global observations of the atmospheric composition for climate, air quality and ozone layer applications, Remote Sens. Environ., 120, 70–83, https://doi.org/10.1016/j.rse.2011.09.027 , 2012.
Vongruang, P., Wongwises, P., and Pimonsree, S.: Assessment of fire emission inventories for simulating particulate matter in Upper Southeast Asia using WRF-CMAQ, Atmos. Pollut. Res., 8, 921–929, https://doi.org/10.1016/J.APR.2017.03.004 , 2017.
Wiedinmyer, C., Akagi, S. K., Yokelson, R. J., Emmons, L. K., Al-Saadi, J. A., Orlando, J. J., and Soja, A. J.: The Fire INventory from NCAR (FINN): a high resolution global model to estimate the emissions from open burning, Geosci. Model Dev., 4, 625–641, https://doi.org/10.5194/gmd-4-625-2011 , 2011.
Wiedinmyer, C., Kimura, Y., McDonald-Buller, E. C., Emmons, L. K., Buchholz, R. R., Tang, W., Seto, K., Joseph, M. B., Barsanti, K. C., Carlton, A. G., and Yokelson, R.: The Fire Inventory from NCAR version 2.5: an updated global fire emissions model for climate and chemistry applications, Geosci. Model Dev., 16, 3873–3891, https://doi.org/10.5194/gmd-16-3873-2023 , 2023.
Yarwood, G., Jung, J., Whitten, G., Heo, G., Mellberg, J., and Estes, M..: Updates to the Carbon Bond Mechanism for Version 6 (CB6), in: 9th Annual CMAS Conference, Chapel Hill, NC, 11–13, 1-4, https://www.cmascenter.org/conference/2010/abstracts/emery_updates_carbon_2010.pdf (last access: 8 May 2024), 2010.
- Introduction
- BRAIN database
- Data availability
- Code availability
- Author contributions
- Competing interests
- Acknowledgements
- Financial support
- Review statement
Thank you for visiting nature.com. You are using a browser version with limited support for CSS. To obtain the best experience, we recommend you use a more up to date browser (or turn off compatibility mode in Internet Explorer). In the meantime, to ensure continued support, we are displaying the site without styles and JavaScript.
- View all journals
- My Account Login
- Explore content
- About the journal
- Publish with us
- Sign up for alerts
- Open access
- Published: 06 May 2024
Robots, firm relocation, and air pollution: unveiling the unintended spatial spillover effects of emerging technology
- Yanying Wang 1 , 2 &
- Qingyang Wu 3
Humanities and Social Sciences Communications volume 11 , Article number: 577 ( 2024 ) Cite this article
239 Accesses
2 Altmetric
Metrics details
- Development studies
- Environmental studies
Amidst the global upsurge in industrial robot deployment, there remains a notable gap in our understanding of their environmental impact. This paper explores how the introduction of industrial robots has changed air quality at both the local and neighborhood levels in China. Using the Spatial Durbin Model, we investigate the regional spillovers of PM 2.5 concentration and the diffusion of this innovative technology. Our findings reveal that the rise of robots significantly reduces air pollution in the local area, while exacerbating it in neighboring regions. This contrast is mainly because pollution-intensive industries are more inclined to relocate to neighboring regions than their cleaner counterparts, after the local use of robots increases. Throughout the process, internal costs rather than external costs dominate firms’ relocation decisions. This study provides novel insights into the complex environmental externalities associated with the spread of industrial robots and highlights the critical issue of growing environmental inequality in the era of emerging technologies.
Similar content being viewed by others
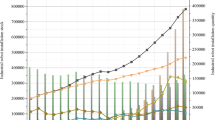
Impact of industrial robots on environmental pollution: evidence from China
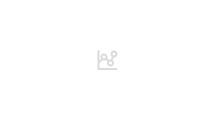
The impact of industrial robot adoption on corporate green innovation in China
Automation and labour market inequalities: a comparison between cities and non-cities, introduction.
Among all the emerging technologies, the development of industrial robots stands out significantly. Since the 1990s, robotics technology has made significant advancements. In recent years, a number of developing nations have capitalized on the wave of the ongoing Industrial 4.0 revolution (Kozul-Wright, 2016 ). This trend has facilitated a rapid dissemination of robotic utilization across developing countries, exhibiting a nearly tenfold increase from 2000 to 2015. This increase is significantly faster than the growth seen in developed countries (World Bank, 2021). As shown in Fig. 1 , by the end of 2019, the global inventory of industrial robots amounted to 2.7 million units, with applications spanning various sectors such as electronics, logistics, chemicals, and processing and manufacturing. Notably, China has emerged as the leading purchaser of industrial robots starting from 2013, reflecting its remarkable economic growth over the past four decades.
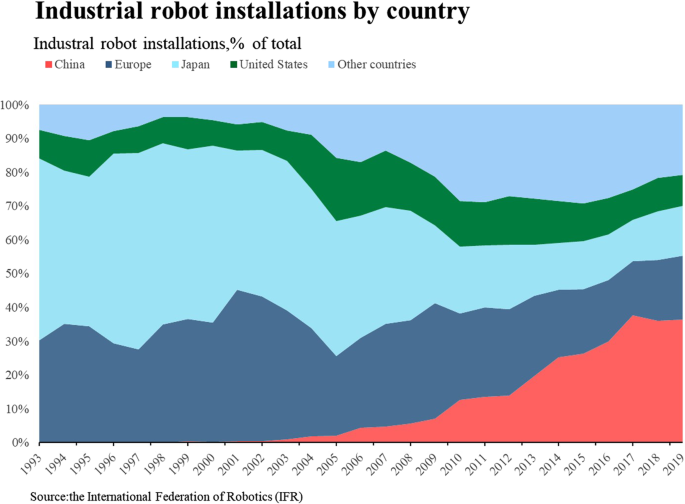
Note: calculated and made by the author.
The widespread adoption of industrial robots is expected to alter economic structure (Aghion et al. 2019 ) and enhance productivity (Acemoglu and Restrepo, 2020 ; Dauth et al. 2021 ; Gihleb et al. 2022 ). However, the potential for harmonizing economic growth and environmental improvement remains uncertain. While numerous studies have explored the influence of technological progress on environmental quality, findings have been inconsistent. Specifically, research on the environmental effects of industrial robots is limited, often focusing on the average effects rather than specific impacts (Luan et al. 2022 ). Given the potential disparities in global technological progress, the environmental externalities of uneven diffusion of new technologies should be well studied.
This paper attempts to fill this gap by investigating whether the use of industrial robots generates environmental externalities from a spatial perspective. We argue that the introduction of robots could reshape the uneven distribution of production efficiency across different regions (Luan et al. 2022 ). Greater robot penetration may improve local air quality by directly improving industrial structures, increasing energy efficiency, and reducing local pollution abatement costs, which is identified as the first environmental externality (Wang et al. 2022 ; Li et al. 2023 ; Wu, 2023 ). Furthermore, a regional increase in robot usage can also make former high-polluting industries uncompetitive locally, prompting physical or operational relocation to other regions with less emerging technology coverage (Acemoglu and Restrepo, 2020 ). This scenario represents the second environmental externality.
It is particularly important for China to discuss these environmental externalities in depth. China has experienced significant regional imbalances alongside notable economic growth. In the case of industrial robots, China’s industrial robots are mainly concentrated in the economically developed coastal regions. At the same time, air quality varies widely from region to region. As society develops, people’s demand for health and environmental comfort increases, while air pollution remains the most important environmental risk factor contributing to the burden of disease, disproportionately impacting vulnerable groups in China. It is therefore important to understand whether and to what extent this emerging technology contributes to uneven pollution distribution in China.
We use the Spatial Durbin Model (SDM) to test the whether the rising industrial robots in one area would reduce local air pollution while exacerbate it in neighboring areas. We transformed industry-level robot exposure data from the International Federation of Robotics (IFR) into a measure of robot penetration rate in Chinese cities. The spatial distance between cities, and the PM2.5 concentration of the cities were also mapped out. The results suggest a negative and significant relationship between robot exposure and local PM2.5 concentration, indicating that the adoption of industrial robots was accompanied by a decrease in PM2.5 concentration by about 6.89 μg/m 3 during 2006 to 2019. However, considering the possibility of the pollution nearby transfer, the net effect of industrial robots on overall air quality may not be as straightforward. There is a positive spillover effect of robot use on PM2.5 concentrations in neighboring areas, suggesting that the increasing exposure to robots in the local area actually “pollutes” their neighborhood. Through rigorous analysis, alternative explanations were discounted. By revealing the complexity of environmental consequences associated with increased robot exposure, our study provides insights into equitable and sustainable policies in an era of emerging technologies.
This study fills in a significant gap in existing literature by offering a novel and comprehensive understanding of the complex environmental ramifications of emerging technologies. Previous research has focused on robots’ effects on carbon emissions, but the nuanced spillover effects on air pollution and their micro-mechanisms have been largely underexplored. Our paper introduces the concept of two-sided externalities from a micro perspective by examining the influence of technological advancements on corporate investment and location decisions using Chinese data samples. In addition, we delve into the theoretical underpinnings of industrial robots’ impact on environmental pollution and empirically validate two primary mechanisms: the industrial structure effect and the internal cost effect. Furthermore, our findings suggest that emerging technologies may intensify environmental inequality, supporting observations that developing countries bear a disproportionate burden of environmental pollution.
In addition to the above-mentioned, this study also contributes to numerous empirical literatures on assessing the environmental impact of industrial robots (Liu et al. 2022 ; Ding et al. 2023 ), the drivers of environmental inequality (Bell and Ebisu, 2012 ; Zheng et al. 2022 ), the analysis of externalities using spatial econometrics (Anselin, 2003 ; Autant‐Bernard and LeSage, 2011 ; Wu et al. 2023 ; Chiu et al. 2024 ), and the study of pollution industry transfer dynamics (Ben Kheder and Zugravu, 2012 ; Dou and Han, 2019 ).
The rest of the paper is organized as follows. Section “Literature review and research hypothesis” presents a theoretical model, formulates the hypotheses, and summarizes the two-sided externalities of industrial robots on air pollution. The data descriptions, variable definitions, and spatial econometric specifications are described in Section “Materials and methods”. Section “Empirics results” presents the empirical results with robustness checks. Further discussion on the space-time characteristics of industrial robot induced pollution near transfer (IR-PNT), with the embodied mechanism, is presented in Section “Further discussion on IR-PNT”. The last section concludes the paper.
Literature review and research hypothesis
This study focuses on the environmental externalities of industrial robots in China from a spatial perspective, including both local and neighboring impacts. Recognizing the multifaceted ways in which emerging technologies influence air quality, we analyze these impacts as two-sided externalities. First, industrial robots directly affect local air quality by improving the overall industrial structure of the local area, increasing energy efficiency, and reducing the cost of pollution control. Second, the diffusion of this technology may indirectly worsen the ambient quality of the neighborhood by facilitating the relocation of pollution-intensive business (Chun et al. 2015 ). The specific mechanism is discussed below.
First externality: cleaning the air
It is supposed that the industrial robots have a direct impact on local air quality by improving the local industrial structures, boosting energy efficiency, and reducing pollution control expenses.
First, the introduction of industrial robots can lead to an advanced industrial structure, which is widely acknowledged for promoting cleaner production and reducing pollutant emissions (Gao and Yuan, 2021 ). The incorporation of industrial robots facilitates knowledge and technological spillovers, accelerating the development of new technologies and products across diverse sectors, thereby reshaping the industrial landscape. As supported by a growing body of research, the introduction of robots plays a pivotal role in industrial upgrading, fostering industrialization and modernization (Hägele et al. 2016 ; Jung and Lim, 2020 ). On a detailed technological level, the application of industrial robots exhibits a dual impact on labor demand, characterized by substitution and heterogeneity. Automation primarily targets the replacement of repetitive tasks and positions typically held by low-skilled workers, consequently shifting the industrial structure towards a more capital-intensive configuration (Graetz and Michaels, 2018 ). Aghion et al. ( 2017 ) demonstrated how the integration of artificial intelligence and traditional production methods in different industrial sectors influences the evolution of industrial structure. This effect is dominant in the manufacturing sector, as the application of industrial robots promotes the expansion of this very manufacturing sector, where the use of industrial robots also stimulates growth in related service industries through the manufacturing scale effect (Acemoglu and Restrepo, 2020 ).
Second, the integration of robots in the initial stages of the production process can enhance the energy transition and boost energy efficiency, thereby reducing enterprises’ pollution emissions. China faces significant environmental pollution problems resulting from inefficient energy consumption (Zhang et al. 2013 ). Energy inefficiencies are prevalent across various stages, including development, processing, conversion, transmission, distribution, and end-use. Emerging technologies offer a pathway to the energy transition by bolstering the efficacy of energy systems and reducing transition costs (Bocca et al. 2021 ). They can also accelerate the adoption and application of renewable energy sources and equipment. For example, the application of robots in enterprises can optimize technological processes of coal combustion or encourage the adoption of cleaner energy alternatives, subsequently enhancing energy efficiency and reducing pollution emissions (Sheng and Bu, 2022 ). Overall, by facilitating the energy transition at the production level, industrial robots reduce pollution emissions.
In addition, the clean effect of robotics can be further explained from a microscopic perspective through the variation of abatement costs. For example, industrial robots can reduce material losses in manufacturing and supply chain operations. They also facilitate the implementation of digital environmental monitoring and accounting systems, enabling efficient measurement, reporting, and verification of environmental impacts. Concepts like smart recycling systems, which repurpose waste into high-quality reusable materials, further illustrate this point (Dusík et al. 2018 ; Wilts et al. 2021 ). Consequently, the adoption of industrial robots is associated with reduced abatement costs (Wang and Feng, 2022 ). However, abatement costs are not constant across firms. In regions where the majority of firms do not utilize industrial robots, abatement costs will be relatively higher due to less efficient in terms of profitability and resource efficiency. Faced with this disparity, companies will generally consider whether to leave or stay according to the level of abatement costs (Shen et al. 2017 ). They may either invest in eco-friendly technologies to manage their emissions or relocate robots to areas with lower relative emission costs to gain operational and abatement advantages. This decision-making process is further detailed in the following subsection (see the next subsection for details).
Moreover, these effects demonstrate variability linked to local developmental stages and industrial configurations. In some contexts, the rebound effect of robot usage on environment have been found in nations of different development stages and industrial structures (see, e.g., Li et al. 2022 ; Luan et al. 2022 ; Zhu et al. 2023 ). For instance, sectors such as manufacturing, agriculture, and utilities, including electricity, gas, and water supply, the deployment of industrial robots markedly enhances the efficiency of energy equipment. This leads to a notable reduction in emissions, especially in high-pollution enterprises and those in regions with more relaxed environmental regulations, as identified by Zhu et al. ( 2023 ). Therefore, Proposition 1 can be summarized as follows.
Proposition 1: The diffusion of industrial robots could generally improve local air quality, but the impact may vary due to heterogeneity in regional development stages and industrial structures.
Second externality: industrial robot induced pollution nearby transfer (IR- PNT)
From a micro perspective, technological progress not only drives local enterprises to further innovate and reduce pollution emissions, but also affects the location decisions of enterprises. According to the new economic geography theory, technological progress is a critical determinant of the location of firms (Fujita and Krugman, 2004 ), while migration (in a broad sense, including the relocation in the physical sense and transfer of heavy pollution production business) is one of the main strategies with which firms respond to the penetration of new technologies (Pellenbarg et al. 2002 ; Aduba and Asgari, 2020 ). Then, intuitively, this technological advancement intuitively reshapes the uneven distribution of production efficiency across regions (Florida et al. 2008 ). A higher local adoption rate of emerging technologies corresponds to an increased likelihood of the relocation of highly polluting firms, which tend to have lower production capacities, to neighboring areas (Milani, 2017 ). Subsequently, the movement of these polluting entities on a micro level result in a substantial reduction of local air pollution. However, this also gives rise to a contrasting trend in neighboring regions, where the risk of environmental degradation emerges (Bommer, 1999 ). Realistic examples confirm the possibility of the existence of this effect: in Langfang and Zunhua, Hebei, which are adjacent to Beijing, the share of value added in the secondary sector is still rising from 2014 to 2017.
In other words, the introduction of industrial robots will also lead to the so-called “Baumol’s disease”, where the application of new technologies changes the local industrial layout and factor endowment, leading to huge variations in the competitive advantages of different regions (Bartelsman and Doms, 2000 ; Juhász et al. 2020 ; Benhabib et al. 2021 ). Even if only a limited number of firms adopt industrial robots in their operations in a given area, the rest of the firms can more easily learn from the “forerunners” and thus benefit much more from the positive externality of knowledge diffusion through the industrial chain and business ecosystem (Aghion et al. 2017 ). In this way, the adoption of industrial robots in a certain region can affect the costs and returns of firms, which can further encourage firms to innovate or relocate.
Next, a theoretical illustration of this view is provided. The model proposed by Levinson and Taylor ( 2008 ) and Shen et al. ( 2017 ) is extended to a multi-regional model, where the penetration of industrial robots is considered as the dominant factor influencing the variation in production costs, as it determines whether it is feasible and realistic for a firm to implement automation in its production, as well as the significant savings in labor costs that can be achieved by replacing humans with robots (Soergel, 2015 ; Graetz and Michaels, 2018 ).
Three regions, numbered “I”, “II”, “III” are considered. The model is partial equilibrium, and the price of production factors and is exogenous. Without loss of generality, we have made the following three assumptions: From a regional perspective, the production cost is greatly reduced due to the improvement in productivity; 2) For a representative company without industrial robots, the relative cost of emitting pollutants is χ I > χ II > χ III . The introduction of robots by other firms can increase the relative cost of pollution for firms without robots; 3) The demand of investment in abatement technologies is generally higher for pollution-intensive enterprises. The intuition is that the average productivity of enterprises will increase after the popularization of industrial robots. But for representative enterprises without robots, it is equivalent to losing their relative productivity and facing a higher cost of emitting pollutants. In the initial state, the continuum of industries of region I is indexed by \(\nu \in [0,1]\) while there are no industries in regions II and III; The pollution intensity of industry \(\nu\) is \(\sigma (\nu )\) and \({\sigma }^{{\prime} }(\nu ) > 0,0 < \sigma (\nu ) < 1\) . The production cost per unit product in regions are \({c}_{I},{c}_{{II}},{c}_{{III}}\) , and \({c}_{I} < {c}_{{II}} < {c}_{{III}}\) . Labor and capital flow freely between regions, and the relocation costs of fixed assets should be taken into consideration when industry \(\nu\) in region I moves to regions II and III; The distance between region I and region III is greater than that between region II and region III, so the production cost per unit product of industry \(\nu\) in region II is higher.
θ is the share of enterprises’ inputs used in environmental-friendly technologies. The production function \(F\left(K\left(\nu \right),L\left(\nu \right)\right)\) satisfies the constant returns to scale (CRTS). The output \(Q(\nu )\) and the pollution discharge \(E(\nu )\) are as follows:
The representative enterprise in region I aims at the maximum profit by choosing θ :
The first order derivative w.r.t θ is:
This is consistent with our intuition above that the greater the penetration of local industrial robots, the greater the pollution abatement efforts of enterprises in the area, thus improving air quality.
Based on Eq. ( 1 ), the production function with pollution emission and finished products as input factors can be written as:
As \({{\rm{\chi }}}_{I}\) and \({c}_{I}\) are the shadow prices of pollution emissions and production, respectively. The first order derivative w.r.t \(\nu\) is:
For the same reason, the unit costs of enterprise in regions II and III are:
The condition for an enterprise to relocate from region I to region II is:
Suppose an interior threshold industry level \(\widetilde{v}\) meets \({C}_{{II}}(\widetilde{v})={C}_{I}(\widetilde{v})\) :
As \({\sigma }^{{\prime} }(v) > 0,0 < \sigma (v) < 1\) , the EQ. (7) holds under \(v > \widetilde{v}\) , suggesting that the rational decision for enterprise in region I is to relocate to region II.
And Eq. ( 9 ) show the negative association between \(\widetilde{v}\) and \({{\rm{\chi }}}_{I}\) :
Hence the following Propositions 2a and 2b is straightforward:
Proposition 2a: Considering the penetration of industrial robots, the pollution-intensive enterprises would choose either to innovate locally or to relocate to the neighborhood; moreover, the greater the comparative disadvantage of the pollution-intensive enterprises, the more likely they are to choose to relocate to the neighborhood.
Proposition 2b: As the penetration of industrial robots increases, the likelihood that highly polluting enterprises will relocate nearby will increase, resulting in worse air quality in the neighborhood (IR-PNT effect).
Extend the conclusion to three areas. Consider the interior threshold industries \(\overline{\overline{v}}\) meets \({C}_{I}(v) < {C}_{II}(\overline{\overline{v}})={C}_{III}(\overline{\overline{v}})\) :
As \({{\rm{\chi }}}_{{II}}\) > \({{\rm{\chi }}}_{{III}}\) , \({\sigma }^{{\prime} }\left(v\right) > 0,\,0 < \sigma (v) < 1\) , the enterprise would like to move again from region II to region III.
In practice, a company’s decision to relocate relies heavily on an overall cost-benefit analysis. The costs of relocation can generally be divided into external and internal costs. For example, the external cost of firm relocation usually includes transportation links and information infrastructure, which are crucial for maintaining connectivity and operational efficiency post-relocation (Audirac, 2005 ); Internal costs, on the other hand, encompass aspects like the reallocation of current assets and employee wages, which significantly influence a firm’s financial capabilities and willingness to relocate (Pennings and Sleuwaegen, 2000 ). Additionally, the distance for relocation emerges as a pivotal factor, influencing both the feasibility and cost-effectiveness of the move. This aspect forms the basis for the spatial econometric models used in our analysis (Ben Kheder and Zugravu, 2012 ). Based on the conclusion of this part of the analysis, Propositions 3a and 3b can be proposed as follows:
Proposition 3a: The IR-PNT effect is influenced by geographical distance. Firms tend to relocate to closer areas due to lower overall migration costs, resulting in a more pronounced concentration of industrial activities in nearby regions, and thereby intensifying the IR-PNT effect.
Proposition 3b: The heterogeneity of the IR-PNT effect is significantly shaped by the varying levels of external and internal migration costs faced by local firms. These costs influence firm decisions on relocation, affecting the distribution and intensity of the IR-PNT effect across different regions.
Materials and methods
Data sources.
The raw data is obtained from the IFR, which contains the number of robots by industry, country and year, based on the annual surveys of robot suppliers. The data of China used in this paper is extracted from 2006 to 2019, because the robot industry in China was undeveloped in the 90s and its boom started in 2006, the industry-specific data was only provided in 2006. Therefore, the consistent data of robots in 7 broad industries (roughly at the two-digit level), i.e., agriculture, forestry, and fishing; mining; manufacturing; utilities; construction; education, research, and development; and non-specific industries, are adopted. In manufacturing, consistent data on the use of robots are collected for 11 industries for more detail (roughly at the 3-digit level): food and beverage, plastics and chemical products, rubber and plastic products (non-automotive), fabricated metal products (non-automotive), electrical machinery, electronic components/devices, semiconductors, liquid crystal display (LCD), light-emitting diode (LED), automotive, motor vehicles, engines and bodies, unspecified auto parts, and other vehicles.
In terms of outcome variables, surface PM2.5 data from van Donkelaar et al. ( 2021 ), who lead an annual global satellite-based estimate combining the instruments of NASA MODIS, MISR, and SeaWIFS and calibrated using a geographically weighted regression, are used to construct measures of annual PM2.5 concentration for each prefecture-level city (For details, see: https://sites.wustl.edu/acag/datasets/surface-pm2-5/ ).
To control for the potentially confounding factors that are not of interest here, information from the China Statistical Yearbook, the China Energy Statistics Yearbook, the China Environmental Statistics Yearbook, and the China Energy Statistics Yearbook is collected, and a set of economic and environmental indicators are obtained to primarily mitigate the bias from omitted variables (Wu et al. 2023 ; Jiang et al. 2024 ). See Table 1 for the detailed definition of the covariates.
Indicators of exposure to robots
We focus on the industrial robot use at the city level and mainly use the IFR dataset. As the most detailed data source available on industrial robots, IFR data have been popular in many studies such as those by Giuntella and Wang ( 2019 ), Anelli et al. ( 2019 ), and Acemoglu and Restrepo ( 2020 ). However, there are still some challenges in calculating the number of industrial robots per worker using IFR data. First, the different industrial classification methods make it difficult to map the raw data one-to-one to the Chinese Industry Classification System (CICS). Second, data on the distribution of labor in some industries are not available in China. In view of this, the IFR data are mapped to CICS, and the focus is only on the robotic installations with available labor data, which account for 29.2% of the total raw data.
In light of these challenges, our methodology aligns with established practices in the existing literature (Acemoglu and Restrepo, 2020 ), where the robot usage at the city level is calculated to represent the technological impact of robots. The first step is to calculate the exposure to robots at the industry level:
where \({\rm{MR}}_{{it}}\) donates the stock of industrial robots of industry i in year t, and \({L}_{i,t=2005}\) is the number of labors of i industry based on 2005.
Second is to construct the city-level industry robot usage index:
where I represents the collection of various industries. \({L}_{i,j,t=2005}\) is the number of labors of industry i in city j in 2005. \({\rm{Mean}}\,{L}_{i,t=2005}\) is the mean of labors of industry i in 2005. It is notable that \({L}_{i,j,t=2005}/{\rm{Mean}}\,{L}_{i,t=2005}\) is the proportion of number of labors in industry \(i\) and region j to the average number of labors of the industry, representing the relative labor force proportion.
Since the industry classification standard in China’s statistical system is not exactly the same as the IFR, we apply the employment data at the city and industry level from the China Labor Statistics Yearbook and merge it with the major industries provided by the IFR, so as to obtain the relative share of labor in industry i at the city level in year t . Then, we calculate and obtain the target variable \({\rm{Robot}}_{{jt}}\) .
Finally, since the distribution of cities’ exposure to robots is substantially right-skewed, we adopt its logarithmic version, which is essentially the normal distribution in the empirics.
Spatial econometric model
We selected the SDM for its effectiveness in addressing the issue of spatial dependence in the study of the environmental impacts of emerging technologies. The SDM’s spatial weight matrix captures the geographical distribution and interactions of economic activities, which is essential for understanding the diffusion of pollutants and the influence of environmental policies across regions. Furthermore, its flexibility allows it to be adapted to specific research contexts, making it suitable for exploring the micro-mechanisms of spillover effects. In addition, our dataset fits well with the data requirements of the SDM, promising insightful results. The accuracy and theoretical relevance of this model outperform other models considered, making it the optimal choice for our study.
Spatial correlation test
Spatial correlation test reflects whether there is a correlation between any two geographical entities. The global Moran’s I value (GMI) is used to test the existence of spatial correlations, defined as:
where N is the number of observations, i and j are the two dimensions of coordinates. x i i are the observations of cell i with the mean \(\bar{x}\) . And there is a theoretical possibility that the global spatial correlation test may ignore the features of atypical local area, Anselin ( 1995 ) therefore proposed a local version Moran’s I value (LMI) formulated as follows:
where w ij is the spatial weight of two adjacent regions based on the specific spatial relationships and \(W\) is the double summation of w ij on i and j that \(W=\mathop{\sum }\nolimits_{i=1}^{n}\mathop{\sum }\nolimits_{j=1}^{n}{w}_{{ij}}\) . Take the geographical adjacency weight matrix \({w}_{{ij}}^{a}\) for instance, it follows the rule that it is equal to 1 if regions i and j are spatially adjacent, and 0 otherwise. An alternative method used in this paper is to assign weights using the decay functions, such as the geographical distance weight matrix \({w}_{{ij}}^{d}\) :
where \({\phi }_{i}\) and \({\phi }_{j}\) donate the spherical coordinates of the centroids of regions i and j respectively, \(\Delta \tau\) is the difference of coordinates between regions i and j. \(R\) is the earth radius, approximately 3958.761 miles. The geographical distance weight matrix is standardized by rows and its diagonal elements are set to 0.
Spatial autoregressive model (SAM) and spatial error model (SEM) are two typical categories for spatial econometric setting (LeSage and Pace, 2009 ). And LeSage et al. ( 2015 ) construct a spatial panel data model called SDM, in consideration of the spatial correlation of the dependent variables as well as the independent variable by adding the spatial lag terms of endogenous and exogenous factors. Since the spatial effect of industrial robot exposure and environmental pollution in a certain region may both be correlated with environmental pollution in nearby regions, we apply the SDM, and the model of this paper is expressed as follows:
where \({\mathrm{ln}{PM}}_{{it}}\) denotes the particulate matter concentrations in the logs of each city I in year t ; \({{IR}\exp }_{{jt}}\) denotes the industrial robots’ exposure in the logs of each city j in year t ; X consists of a vector of control variables, and as noted above. \({w}_{{ij}}\) represents the elements of the n×n order spatial weight matrix and the geographical adjacency weight matrices and geographical distance weight matrix is used in this paper. λ includes the prefecture-level city and year fixed effects. Besides, the \(\rho\) and \(\theta\) in Eq. ( 17 ) represent the spatial regression coefficient of the explanatory variable and the explained variable, respectively. Specifically, \(\rho\) reflects the spatial dependence of environmental pollution in neighboring regions, and \(\theta\) reflects the degree of influence of industrial robot exposure in neighboring regions on environmental pollution in the local area.
After analyzing the impact of industrial robots on environmental pollution using the spatial econometric model in Section “Empirics results”, the IR-PNT effects are further discussed in Section “Further discussion on IR-PNT”. By using SDM, it is also shown that by changing the factors of comparative resource abundance of a region, the installation of robots will inevitably affect the relocation decision of enterprises and factories, and then the air quality of neighboring areas.
Empirics results
Spatial correlation tests.
The results of the GMI (see Table 2 ) show that all Moran’s I indices of PM 2.5 from 2006 to 2019 are significantly greater than zero, indicating that the air pollution levels of cities in China are basically spatially autocorrelated. Moreover, the GMI of PM 2.5 is relatively stable during the study period, indicating the consistency of the level of spatial agglomeration. Therefore, the spatial econometric model is necessary to explore the relationship between air pollutants and other factors.
The above results are consistent with the previous literature, generally Chen et al. ( 2017 ) and Feng et al. ( 2019 ). Urban air quality in China is spatially autocorrelated with a stable trend. It is widely recognized that due to geographical relationships, the mutual transmission of pollutants exacerbates regional pollution. In fact, the formation of PM 2.5 is not only related to physical and chemical factors (such as atmospheric conditions, temperature and humidity, etc.), but also related to anthropogenic economic activities, such as the distribution of heavily polluting and energy-consuming enterprises. In this case, since neighboring cities tend to have similar socio-economic characteristics, and the PM 2.5 of these cities are also quite similar.
To investigate the local characteristics of PM 2.5, the local Moran’s I scatterplots of PM 2.5 at the beginning and end of the study period are also presented (see Fig. 2 ). The scatterplots can be divided into four quadrants, which indicate the local spatial correlations between regions (Feng et al. 2019 ). The first and third quadrants represent positive spatial correlations, while the second and fourth quadrants represent negative spatial correlations. From Fig. 2 , it is clear that PM 2.5 is positively correlated in most cities, with a shorter spatial distance between different regions indicating a similar concentration of PM 2.5. Overall, highly polluted cities in China tend to cluster together, as do clean cities throughout the study period.
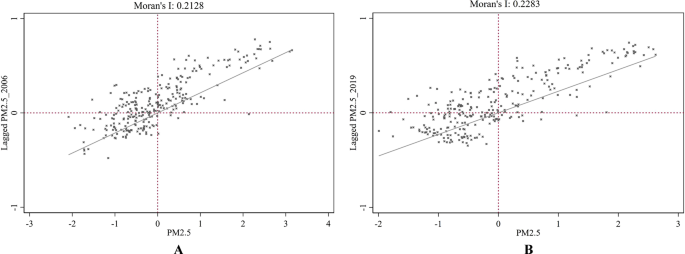
A Local Moran’s I in 2006 B Local Moran’s I in 2019. Note: Due to the length of the paper, only part of the results are shown, but we accept any request for results.
Baseline regressions
In order to clarify the relationship between industrial robots and local air pollution, we first estimate how the presence of industrial robots affects the average level of air pollution, and present the results in Table 3 . Columns (1) and (3) show the case without control variables, while columns (2) and (4) include them sequentially. To test the robustness of the results, the first two columns use the (0–1) adjacency matrix, while the last two columns use the geographical distance matrix.
Unlike traditional non-spatial models, the spatial econometric model incorporates circular feedbacks between independent and dependent variables in local and nearby areas. Therefore, the estimated coefficients in the first two rows of Table 3 cannot reflect the marginal effects of the independent variables on the dependent variable. As outlined by Elhorst ( 2014 ), the marginal effects should be explained by the direct and indirect effects in the SDM, respectively, and we estimate these effects accordingly.
For the direct effect, the coefficients of IRexp indicate a negative and significant correlation between industrial robot exposure and PM2.5 after controlling for socio-economic variables and city-level fixed effects, which verifies the proposition of the first environmental externality (local cleaning effect), i.e., Proposition 1 . Taking column (4) as an example, cities with the highest exposure to industrial robots experience a 61% (0.0184 × (2.22 - 0.065) / 0.065) reduction in PM2.5 concentrations compared to that of cities with average exposure to industrial robots, simply due to the increasing exposure to industrial robots in the local area (with the highest and average values of exposure to industrial robots being 2.22 and 0.065, respectively). Moreover, the resulting value suggests that during the period 2006 - 2019, the introduction of industrial robots will reduce the average PM2.5 concentration by approximately 6.89 μg/m 3 (50.6*0.0184 × ((0.126 - 0.015) / 0.015), where 50.6 μg/m 3 is the actual PM2.5 concentration in 2006. Based on the empirical dose-response relationship estimated by Burnett et al. ( 1999 ) and Pope et al. ( 2008 ), this reduction of 6.89 μg/m 3 from 2006 to 2019 averted the risk of hospitalization for cardiopulmonary disease and heart failure by 2.27% (6.89 × (0.33%) and 9.03% (6.89 × (1.31%), respectively, nationally.
However, considering the possibility of IR-PNT, the overall cleaning effect of industrial robots may be limited. For the indirect effect, the coefficients of W_IRexp indicate that the industrial robot exerts a positive spillover effect on PM2.5 in neighboring regions, which means that the higher the exposure to industrial robots in the local area, the higher the PM2.5 in the neighboring areas will be. It can be understood as the fact that the higher exposure to industrial robots will “pollute” its neighborhood. This result confirms the proposition of the second environmental externality (IR-PNT) proposed hereby, i.e., Propositions 2a and 2b . Specifically, column 4 shows that the 1% increase in exposure to industrial robots in neighboring regions increases the PM 2.5 in the local area by 0.11% on average, although this effect is insignificant. It will be further specified in the next section.
Additionally, the coefficients of S_rho show that air pollution has a significant positive spillover effect: The 1% increase in PM 2.5 in neighboring regions leads to at least 0.23% higher PM 2.5 in the local area, which is consistent with the spatial correlation test mentioned above in Table 2 .
Overall, industrial robots have a spillover effect on air quality, leading to increased pollution. This finding is consistent with Nguyen et al. ( 2020 ) and Liu et al. ( 2022 ), which indicate that the development of new and emerging technologies is not always good for the environment. Our finding is also similar to Chen et al. ( 2022 ), which found that emission reduction efforts in clean energy development are offset by CO 2 transfer effect.
Heterogeneity analysis
In this section, the heterogeneous effects of industrial robots on air pollution are analyzed. Column (2) in Table 4 performs the spatial regression according to the location of the cities, while column (3) in Table 4 performs the spatial regression according to the size of the cities. For the direct effect, the coefficients of East_ IRexp and Metro_ IRexp are negative, unlike W_ IRexp in the baseline, indicating that as the number of industrial robots increases in the east and in the metropolis, the local cleaning effect is even greater. For the indirect effect, combining the coefficients of IRexp , East_ IRexp and Metro_ IRexp , there will be a “cleaning” effect in the metropolis and a small one in the eastern areas, although not significant. This result differs from the baseline. This may be because there is a stronger climate in the east that encourages firms to invest in green and innovative technologies rather than relocating to pollute neighboring areas (Zhang et al. 2024 ).
Our findings are not consistent with Ding et al. ( 2023 ), whose heterogeneity analysis suggests that AI reduces carbon emissions through spatial spillovers, with the effect being stronger in the Midwest. In contrast, our analysis indicates a stronger effect in the Eastern regions. This discrepancy may be attributed to differences in the data scales used: Ding et al. ( 2023 ) employed provincial panel data, our study is based on city-level data. At the same time, the firms that emit large amounts of carbon and cause air pollution do not exactly overlap. This variation in the distribution of high-emission firms may significantly influence the regional impact of AI on environmental outcomes.
From Table 5 , we can see that the regression results of PM2.5 at different quartiles show significant variations, and the local cleaning effect of industrial robots is stronger in areas with higher PM2.5. From the perspective of effect decomposition, the indirect effect dominates the total effect of the pollution reduction effect of industrial robots in the cities with PM2.5 at different quantiles. Surprisingly, the increase in robots in clean areas increases the pollution of neighboring regions considerably, and this effect gradually declines as PM2.5 is mitigated into a cleaning effect in neighboring regions. This suggests that the IR-PNT effect that we find in the baseline regression is mainly caused by the introduction of industrial robots in the cleaning area. This may be because polluters in cleaner areas already face high marginal abatement costs, exacerbating their relative local disadvantage. As a result, they are more likely to relocate to nearby areas to avoid competition.
Robustness checks
To assess the robustness of the effect of industrial robots on air pollution, the sample window from 2009 to 2017 in column (1) is narrowed to eliminate the boundary effect of the data, as shown in Table 6 . In addition, a region-based placebo test is adopted in column (2) by randomly assigning the exposure to industrial robots of each region in a normal distribution manner. Column (3) includes the first and second lags of the dependent variables to overcome the serial correlation. Lastly, in column (4), a difference-in-difference model is set up to test the effect of industrial robots on air pollution, taking the document of Guiding Opinions of the Ministry of Industry and Information Technology on Promoting the Development of Industrial Robot Industry as a shock that occurred in 2013 and taking the proportion of employees in tertiary industries as the policy treatment effect, a difference-in-difference model is established to check the effects of industrial robots on air pollution.
In summary, the result drawn here has passed all the robustness checks, and the pseudo-shock cannot reject the null hypothesis even at the 10% significance level. It is clear that the effect of industrial robots on PM2.5 is still significant and negative, which is consistent with the previous result and the main conclusion here.
Alternative interpretations
This section discusses several latent competing stories that may also create a spurious link between industrial robots and pollution. Possible confounding factors fall into three categories: differentiated environmental regulation (including formal or informal), contemporaneous historical events (e.g., the Air Pollution Prevention and Control Action Plan, carbon emission trading system, low-carbon city pilots), and other emerging technologies (the development of information and communication technology).
Environmental regulations
Increasing environmental awareness and the quest for better living conditions is an inevitable trend that accompanies socio-economic development. To meet the demand for a better environment, the Chinese government has implemented a series of policies to control pollution and improve environmental quality. This process is synchronized with socio-economic development, and may be a latent factor in comprehensively reducing environmental pollution. If the increase in environmental awareness and regulation overlaps with the penetration of industrial robots, the resulting air pollution control effect of robots is likely to be overestimated.
To assess the potential problem, the effect of the level of local environmental regulation (broadly defined) on PM2.5 is examined. Environmental regulation includes not only the mandatory environmental policies set by the government (formal regulation), but also the participation of environmentalists and the general public, who assume environmental rights and responsibilities, and will negotiate and consult with polluters (informal regulation). Theoretically, the level of environmental regulation can exert pressure on the behavior of heavy polluters and residents. The frequency of environmental-related terms, such as “green,” “low-carbon,” “ecology,” “pollution discharge,” “emission reduction,” “environmental protection,”, etc., is collected from the Government Work Reports of each city as a proxy for formal environmental regulation. In addition, following the work of Pargal and Wheeler ( 1996 ), indicators such as income level, education level, population density, and age structure are selected to comprehensively measure the intensity of informal regulation in each city. The results of columns (1) and (2) in Table 6 show that the estimated cleaning effect of industrial robots on PM 2.5 remains highly significant, suggesting that it is unlikely that the present results can be explained by the effect of environmental regulations.
Contemporaneous historical events
Some might argue that it is not industrial robots that have improved local air quality, but rather the impact of concurrent events, such as the introduction of more direct air pollution policies during the study period. In China, the Air Pollution Prevention and Control Action Plan (Action Plan) from 2013 to 2017 sets clear and quantifiable targets for the reduction of atmospheric particulate matter, and is considered a milestone for the improvement of China’s ecological environment (Geng et al. 2021 ), while the introduction of the carbon emission trading scheme (ETS) market is considered an effective way to reduce carbon emissions (Cui et al. 2021 ). In turn, carbon dioxide has a similar source with PM2.5, and promoting carbon markets may have synergistic effects in reducing PM2.5. Similarly, the two waves of low-carbon city pilot projects launched in 2010 and 2012 are also considered to be the driving force for improving air quality (Yu and Zhang, 2021 ).
In order to assess the potential influence of the above contemporaneous events, the data in the year after 2013 are excluded in the baseline specification when the Action Plan was implemented. A number of carbon ETS market and low-carbon city pilots are also excluded by assigning a value of 0 to cities with a carbon ETS market or low-carbon cities and a value of 1 to cities without a carbon ETS market or non-low-carbon cities, in order to control for the effect. The results in columns (3), (4), and (5) of Table 6 indicate that the estimated cleaning effect of industrial robots remains significant. Although the magnitudes become somewhat smaller when excluding the carbon ETS market and low-carbon cities, this does not indicate that the impact of industrial robots can be ignored.
Other emerging technologies
A potential concern is that the increase in industrial robots may be accompanied by other emerging technologies that have actually been the main contributors to pollution reduction. For example, the development of information and communication technology (ICT) is considered to be a feasible possibility to reduce PM2.5 concentrations, as it increases production efficiency and technological innovation, and promotes the evolution of the regional industrial structure in a progressive way (Gouvea et al. 2018 ). Moreover, ICT is almost synchronized with the development of industrial robots. To exclude the potential inference of ICT in the present baseline model, the level of ICT development in cities is calculated by extracting the first principal component of total telecommunication services, the number of local telephone users, and the number of internet users. Cities with an above-average level of digital economy are then assigned a value of 1 and otherwise 0 to eliminate the confounding effect of ICT development. The results in column (6) of Table 7 show that the estimated cleaning effect of industrial robots on PM2.5 remains highly significant, although its magnitudes become smaller. Overall, there is convincing evidence that the main results are unlikely to be overturned by the alternative interpretations mentioned above.
Further discussion on IR-PNT
In order to unravel the more specific characteristics of IR-PNT, this section discusses its spatial and temporal variations, as well as the main driving factors behind, including the changing industrial layout (from a macro perspective) and the relocation of firms (from a micro perspective).
Spatial variation of IR-PNT
Based on the spatial weight matrix of reciprocal geographical distance, we set different thresholds d * to study the pollution nearby transfer effects. Specifically, starting from 25 km, we withhold the spatial weight of neighboring cities with distance d ij smaller than the threshold d * , and set the weight of more distant cities to 0. This geographical distance threshold model is expressed as follows:
where \({{PR}}_{{it}}\) denotes the PM2.5 concentrations in logs of each city i in year t , Wij is the spatial weight as defined above, \({{AI}\exp }_{{jt}}\) denotes the industrial robots’s exposure in logs of each city j in year t , \({\boldsymbol{X}}\) consists of a vector of control variables, \({\boldsymbol{\lambda }}\) i ncludes the city and year fixed effects at the prefecture level. Other variables are defined as in Eq. ( 17 ). In this part, we focus on the coefficient of \({\beta }_{d}^{\dagger }\) in this specification.
Figure 3 shows the estimated coefficient results of the PNT effect of industrial robots. It can be seen that the effect of IR-PNT shows an inverted U-shaped curve, which first increases and then decreases with the increase of geographical distance, and the peak point is around 90 km. This result confirms the Proposition 3a . The reasons for this curve may be that: (1) due to technology spillovers, firms in local and very nearby areas tend to experience similar impacts of industrial robots, as well as similar environmental regulations, so there is less motivation for polluting enterprises to relocate their business within a certain distance (Porter, 2000 ); (2) when the distance exceeds 150 km, the IR-PNT effect gradually decreases, probably due to the fact that as the distance increases, the costs of supporting facilities and relocation increase, so heavy polluters are reluctant to relocate. Examples of this can be seen in reality. The IR-PNT effect is more pronounced in neighboring regions, such as Langfang near Beijing and Kaifeng near Zhengzhou, when industrial robots penetrate.
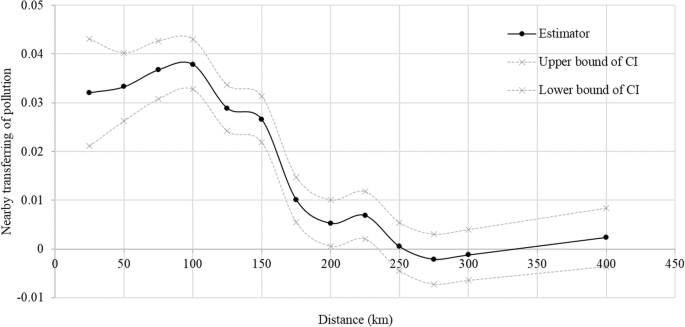
Note: The black solid line is the estimated coefficient of IR-PNT, and the gray dashed lines are the lower and upper bounds of its confidence interval (CI) at the 95% level of statistical significance.
Temporal Variation of IR-PNT
In this part, the cross-terms between the spatial lag terms of industrial robots and the year dummy variables are introduced into the regression to study the temporal variation of the IR-PNT effect. The model is expressed as follows:
where the variables are all defined as in Eq. ( 17 ). We focus on the coefficient of β k in this specification.
The results are shown in Fig. 4 . According to the estimated results of the full sample, the overall magnitudes of IR-PNT vary in the same pattern, and there is an inverted U-shaped curve of IR-PNT with the increase of the geographical distance as shown in Fig. 3 . However, over time, the effect increases after an initial decrease, with the extreme value in 2014. Specifically, the IR-PNT effect is similar to that of the overall estimate, showing a downward trend from 2006 to 2013, which becomes an upward trend from 2014 to 2019. The possible explanations are that the Chinese government started to attach importance to environmental protection and introduced a package of laws and regulations during this period, including the inclusion of air quality in the evaluation of local officials’ promotion, which led to an increase in PNT penalties (Zhang et al. 2013 ). However, the IR-PNT effect has rebounded since 2015, showing that more attention needs to be paid to these years and justifying the practical significance of the present research.
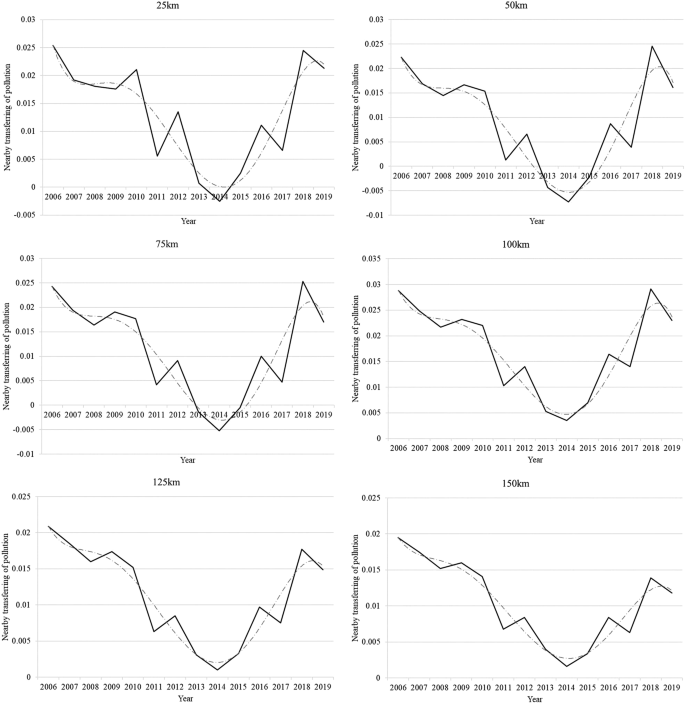
Note: The black solid line is the estimated coefficient of IR-PNT, and the gray dashed line is the fit line smoothed with a sixth-order polynomial function.
Scale-structure trade-offs and mechanism embodied in IR-PNT
The PNT can be attributed to the economic scale and industrial structure change (Antweiler et al. 2001 ). Therefore, we try to explore the scale-structure trade-offs embodied in the IR-PNT mechanism: As industrial robots become more widespread, does the city, to which companies are relocated, changes in the absolute number of pollution-intensive industries (scale effect) or rather in the relative amount (structural effect)? A priori, if the relative share of pollution-intensive industries in neighboring cities is not significantly affected, but the absolute number is, the scale effect dominates; otherwise, the structural effect dominates.
Therefore, two indicators, i.e., P_ratio and P_scale , are constructed with the proportion and amount of the output value of pollution-intensive industries, respectively. The selection of pollution-intensive industries includes 11 industries from the First National Pollution Source Census released by the China’s State Council in 2006. The data are from China Bureau of Statistics.
Table 8 shows the results of the scale structure trade-offs embodied in the IR-PNT mechanism. Columns (1) and (2) show that in the local area, the installation of industrial robots can promote the transformation of the local industrial structure and make it cleaner, while it can significantly worsen the pollution intensities of the local industrial structure in neighboring regions. There is significant impact of the penetration of industrial robots on the proportion of the output value of polluting industries, but no significant impact on the absolute amount of the output value of polluting industries, suggesting that the effects of IR-PNT are led by the structural effect.
Further examination is conducted over whether the structure and scale of the pollution-intensive industries have a significant impact on the PM2.5 concentrations. As shown in Columns (3) and (5), the increase in the proportion of the output value of pollution-intensive industries will significantly increase the local PM2.5 concentrations at a 1% statistical level, and the rise in the amount of the output value of pollution-intensive industries in adjacent areas can also benefit the local ambient air quality at a 10% statistical level. In any case, considering the changes of the absolute and relative quantity of the output value of pollution-intensive industries, it is found that the increase in the PM2.5 concentration in the adjacent areas is dominated by the fact that the industrial structure changes toward a more pollution-intensive state.
At the firm level, as the penetration of industrial robots increases, the pollution-intensive firms will choose either to innovate locally or to relocate to the neighborhood. There are two factors that can affect their relocation decision, thus forming the mechanism of IR-PNT, namely external and internal costs. For the external cost of relocation, two principal components are used as proxies. The first principal component is extracted from the volume of freight among road, air, water and rail to proxy the degree of transport connectivity. In addition, the second principal component is extracted from the gross telecommunications business, telephone and internet users to proxy the level of information infrastructure. We assume that the more transport links and information infrastructures there are, the lower the barriers to relocation. For the internal costs of relocation, we focus on the inertia of production factor inputs. The share of current assets and employee wages are constructed to measure the internal costs of relocation.
Table 9 shows the results for external costs in the IR-PNT mechanism. According to columns (1) and (3), the estimated coefficients of W_IRexp×W_Trans and W_IRexp×W_ICT are negative, although the coefficients of W_IRexp×W_Trans are not significant, indicating that on average the higher the external costs of relocation, the more reluctant the pollution-intensive firms would be to relocate, thus there will be no strong effect of IN-PNT.
Table 10 shows the results for internal costs in the IR-PNT mechanism. The estimated coefficients of W_IRexp×W_Quick are positive at the 5% statistical level, while those of W_IRexp×W_Wages are negative at the 1% statistical level. The results in columns (1) and (2) indicate that the higher the current asset ratio of pollution-intensive industries, the higher the probability of relocation. However, the conclusion from column (3) that rising wages can prevent the relocation of pollution-intensive industries is relatively unexpected. One explanation is that higher wages imply a higher level of human capital, which makes firms reluctant to relocate to neighboring regions to avoid the sunk costs of employee training (Weiss, 1995 ; Philippon and Reshef, 2012 ). Overall, compared to the coefficients of external costs, the internal nature is more dominant in the willingness to relocate. This result confirms the Proposition 3b .
These findings complement the literature on the relationship between firm location behavior and pollution (see, e.g., Dou and Han, 2019 ). We verify that, in addition to environmental regulations, firms make location decisions that take into account their relative competitiveness in emerging technologies and adapt by relocating their operations or flexing their industrial layout, which affects local and neighboring air quality.
Conclusions and policy implications
Environmental problems are frequently the result of externalities. With the rapid development of industrial robots in China, this emerging technology is anticipated to profoundly transform economic structure and productivity. However, whether it can harmonize the goals of economic growth and environmental improvement remains a question. Grounded in the new economic geography theory, this study focuses on the two-sided environmental externalities caused by industrial robots and investigates how the greater penetration of robots in the economy affects local and neighboring air quality using the spatial econometric model.
Our theoretical model suggests that the level of penetration of industrial robots in different regions will shape the locational choices of diverse firm types by changing their relative competitive advantages. The empirical results proved that the presence of robots significantly mitigates air pollution in the local area, yet paradoxically intensify it in neighboring areas. This phenomenon, termed IR-PNT, underscores the presence of pollution transfer at a regional level. To investigate the cause of the IR-PNT, a mechanism analysis is further conducted. Consistent with our theoretical model, it is found that the structural effect mainly contributes to the increase of PM2.5, which shows that as the penetration of industrial robots increases, pollution-intensive business is more likely than others to relocate to neighboring cities, often replacing cleaning firms in their neighboring cities. It is shown that developed cities with advanced technologies tend to relocate highly polluting business to neighboring cities, imposing an unfair burden on their “neighbors”.
Furthermore, we identified both heterogeneous and rebound effects of robots on air quality. Firstly, an increase in industrial robots in the east and in the metropolis has larger local cleaning and IR-PNT effect. Conversely, cities with initially cleaner air tend to experience a rebound effect post robot integration, linked to their distinct industrial structures and advanced robotic technologies. Notably, operational robots can produce waste, like discarded batteries, and their deployment necessitates considerable material use. If these materials are sourced or processed in a way that harms the environment, it can counteract the initial environmental gains. In China, the penetration rate of robots in cleaner areas is relatively lower and more likely to be in the stage of triggering rebound effects.
Due to limitations in data availability, the analyses in this study do not cover all industries in China, nor do they address the spatial spillovers of robotics across sectors. In addition, although not a central aspect of this study, the identification of causality remains potentially underdeveloped. This study has improved causal validity to some extent through robustness tests and the exclusion of competing hypotheses. Future research should aim to more definitively establish and confirm the relationship between industrial robotics and environmental quality using more robust causal inference methods. In addition, it should be emphasized that our conclusions regarding the local cleaning effect and the IR-PNT effect are derived from a specific Chinese sample. In order to formulate more generalizable findings, further verification through cross-national studies is essential.
In general, considering the IR-PNT effect, policy makers may need to consider how to minimize, reduce and offset the externalities caused by the unequal distribution of industrial robots. First, researchers are expected to assess the pollution nearby transfer effect of emerging technologies in different policy scenarios (Moni et al. 2020 ). For example, the tradable permit policy, such as cap-and-trade, can improve the optimal allocation of resources in technological change through the improvement of pollutant property rights, making it easier to achieve the balanced goals of pollution control and economic development (Liu et al. 2020 ; Wu and Wang, 2022 ).
Second, we find that the IR-PNT effect is mainly caused by the relocation of polluting firms from clean areas to neighboring regions. This suggests that regional cooperation in pollution reduction is crucial. Particular attention must be paid to the air quality around the clean area., to avoid cleaning at the expense of polluting someone else’s backyard. To achieve this, a synergistic approach is essential, integrating technological, financial and administrative strategies in regional cooperation.
Lastly, the study shows that IR-PNT effects are lower in metropolitan areas and urban agglomerations, and there is even a cleaning effect. In this case, the expansion of urban agglomerations tends to reduce the environmental inequality caused by technological progress. On the one hand, the externalities of pollution are resolved by internalizing them in larger urban clusters; on the other hand, the expanded urban agglomerations increase the area of cities and the distance and costs of relocating polluting firms (Tabuchi, 1998 ), thus reducing pollution transfer and reducing environmental inequality.
Data availability
The datasets generated during and/or analyzed during the current study are available in the Harvard Dataverse repository, https://doi.org/10.7910/DVN/9BYJYD .
Acemoglu D, Restrepo P (2017) Secular stagnation? The effect of aging on economic growth in the age of automation. Am Econ Rev 107:174–179. https://doi.org/10.1257/aer.p20171101
Article Google Scholar
Acemoglu D, Restrepo P (2019) Automation and new tasks: how technology displaces and reinstates labor. J Econ Perspect 33:3–30. https://doi.org/10.1257/jep.33.2.3
Acemoglu D, Restrepo P (2020) Robots and jobs: evidence from US labor markets. J Polit Econ 128:2188–2244. https://doi.org/10.1086/705716
Aduba JJ, Asgari B (2020) Productivity and technological progress of the Japanese manufacturing industries, 2000–2014: estimation with data envelopment analysis and log-linear learning model. Asia-Pacific. J Reg Sci 4(2):343–387
Google Scholar
Aghion P, Jones BF, Jones CI (2017) Artificial Intelligence and Economic Growth. Working Paper Series. https://doi.org/10.3386/w23928
Aghion P, Antonin C, Bunel S (2019) Artificial intelligence, growth and employment: the role of policy. Econ Stat 510(1):149–164
Anderson D (2001) Technical progress and pollution abatement: an economic view of selected technologies and practices. Environ Dev Econ 6:283–311. https://doi.org/10.1017/S1355770X01000171
Anelli M, Colantone I, Stanig P (2019) We were the robots: automation and voting behavior in western europe. BAFFI CAREFIN Centre Research Paper, (2019-115)
Anselin L (1995) Local indicators of spatial association—LISA. Geogr Anal 27(2):93–115
Anselin L (2003) Spatial externalities, spatial multipliers, and spatial econometrics. Int Reg Sci Rev 26(2):153–166
Antweiler W, Copeland BR, Taylor MS (2001) Is free trade good for the environment? Am Econ Rev 91:877–908. https://doi.org/10.1257/aer.91.4.877
Arbia, G, Espa, G, Giuliani, D (2021) Spatial microeconometrics. Routledge
Atanu S, Love HA, Schwart R (1994) Adoption of emerging technologies under output uncertainty. Am J Agric Econ 76(4):836–846
Audirac I (2005) Information technology and urban form: challenges to smart growth. Int Reg Sci Rev 28(2):119–145
Autant-Bernard C, LeSage JP (2011) Quantifying knowledge spillovers using spatial econometric models. J Reg Sci 51(3):471–496
Autor, D, Chin, C, Salomons, AM, Seegmiller, B, 2022. New Frontiers: The Origins and Content of New Work, 1940–2018. Working Paper Series. https://doi.org/10.3386/w30389
Bartelsman EJ, Doms M (2000) Understanding productivity: lessons from longitudinal microdata. J Econ Lit 38(3):569–594
Bell ML, Ebisu K (2012) Environmental inequality in exposures to airborne particulate matter components in the United States. Environ Health Perspect 120(12):1699–1704
Article CAS PubMed PubMed Central Google Scholar
Benhabib J, Perla J, Tonetti C (2021) Reconciling models of diffusion and innovation: a theory of the productivity distribution and technology frontier. Econometrica 89(5):2261–2301
Article MathSciNet Google Scholar
Ben Kheder S, Zugravu N (2012) Environmental regulation and French firms location abroad: an economic geography model in an international comparative study. Ecol Econ 77:48–61. https://doi.org/10.1016/j.ecolecon.2011.10.005
Bommer R (1999) Environmental policy and industrial competitiveness: the pollution‐haven hypothesis reconsidered. Rev Int Econ 7(2):342–355
Bocca, R, Ashraf, M, Jamison, S (2021) Fostering Effective Energy Transition 2021 Edition. In World Economic Forum
Buera, FJ, Fattal Jaef, RN (2018) The dynamics of development: innovation and reallocation. World Bank Policy Research Working Paper (8505)
Burnett RT, Smith-doiron M, Stieb D, Cakmak S, Brook JR (1999) Effects of particulate and gaseous air pollution on cardiorespiratory hospitalizations. Arch Environ Health 54:130–139. https://doi.org/10.1080/00039899909602248
Article CAS PubMed Google Scholar
Chen SM, He LY (2014) Welfare loss of China’s air pollution: How to make personal vehicle transportation policy. China Econ Rev 31:106–118
Chen X, Shao S, Tian Z, Xie Z, Yin P (2017) Impacts of air pollution and its spatial spillover effect on public health based on China’s big data sample. J Clean Prod 142:915–925. https://doi.org/10.1016/j.jclepro.2016.02.119 . Special Volume on Improving natural resource management and human health to ensure sustainable societal development based upon insights gained from working within ‘Big Data Environments’
Article CAS Google Scholar
Chen Y, Shao S, Fan M, Tian Z, Yang L (2022) One man’s loss is another’s gain: does clean energy development reduce CO2 emissions in China? Evidence based on the spatial Durbin model. Energy Econ 107:105852
Cheng H, Jia R, Li D, Li H (2019) The rise of robots in China. J Econ Perspect 33(2):71–88
Chiu S-H, Lin T-Y, Wang W-C (2024) Investigating the spatial effect of operational performance in China’s regional tourism system. Human Soc Sci Commun 11(1):14. https://doi.org/10.1057/s41599-024-02741-y
Chun H, Kim JW, Lee J (2015) How does information technology improve aggregate productivity? A new channel of productivity dispersion and reallocation. Res Policy 44(5):999–1016
Cui J, Wang C, Zhang J, Zheng Y (2021) The effectiveness of China’s regional carbon market pilots in reducing firm emissions. Proc Natl Acad Sci USA 118(52):e2109912118
Dauth W, Findeisen S, Suedekum J, Woessner N (2021) The adjustment of labor markets to robots. J Eur Econ Assoc 19:3104–3153. https://doi.org/10.1093/jeea/jvab012
Dusík, J, Fischer, T, Sadler, B, Therivel, R, Saric, I, (2018) Strategic Environmental and Social Assessment of Automation: Scoping Working Paper
Ding T, Li J, Shi X, Li X, Chen Y (2023) Is artificial intelligence associated with carbon emissions reduction? Case of China. Resour Policy 85:103892. https://doi.org/10.1016/j.resourpol.2023.103892
Dou J, Han X (2019) How does the industry mobility affect pollution industry transfer in China: empirical test on Pollution Haven Hypothesis and Porter Hypothesis. J Clean Prod 217:105–115. https://doi.org/10.1016/j.jclepro.2019.01.147
Elhorst JP (2014) Spatial Econometrics: From Cross-Sectional Data to Spatial Panels. Springer, Berlin, Heidelberg, 10.1007/978-3-642-40340-8
Book Google Scholar
Ehrlich PR, Holdren JP (1971) Impact of population growth. Science 171:1212–1217. cq9zm8
Article ADS CAS PubMed Google Scholar
Feng Y, Cheng J, Shen J, Sun H (2019) Spatial effects of air pollution on public health in China. Environ Resour Econ 73:229–250. https://doi.org/10.1007/s10640-018-0258-4
Florida R, Mellander C, Stolarick K (2008) Inside the black box of regional development—human capital, the creative class and tolerance. J Econ Geogr 8(5):615–649
Fujita M, Krugman P (2004) The new economic geography: past, present and the future. In Fifty years of regional science (pp. 139-164). Springer, Berlin, Heidelberg
Gao K, Yuan Y (2021) The effect of innovation-driven development on pollution reduction: empirical evidence from a quasi-natural experiment in China. Technol Forecast Soc Change 172:121047
Geng G, Zheng Y, Zhang Q, Xue T, Zhao H, Tong D, Davis SJ (2021) Drivers of PM2. 5 air pollution deaths in China 2002–2017. Nat Geosci 14(9):645–650
Article ADS CAS Google Scholar
Gihleb R, Giuntella O, Stella L, Wang T (2022) Industrial robots, Workers’ safety, and health. Labour Econ 78:102205. https://doi.org/10.1016/j.labeco.2022.102205
Giuntella O, Wang T (2019) Is an Army of Robots Marching on Chinese Jobs? https://doi.org/10.2139/ssrn.3390271
Gouvea R, Kapelianis D, Kassicieh S (2018) Assessing the nexus of sustainability and information & communications technology. Technol Forecast Soc Change 130:39–44
Graetz G, Michaels G (2018) Robots at work. Rev Econ Stat 100:753–768. https://doi.org/10.1162/rest_a_00754
Grossman GM, Krueger AB (1991) Environmental Impacts of a North American Free Trade Agreement. Working Paper Series. https://doi.org/10.3386/w3914
Hägele M, Nilsson K, Pires JN, Bischoff R (2016) Industrial robotics. In Springer handbook of robotics (pp. 1385-1422). Springer, Cham
IFR (2020) World Robotics 2020. International Federation of Robotics and the United Nations
Jiang L, Yang Y, Wu Q, Yang L, Yang Z (2024) Hotter days, dirtier air: the impact of extreme heat on energy and pollution intensity in China. Energy Econ 130:107291
Juhász R, Squicciarini MP, Voigtländer N (2020) Technology adoption and productivity growth: Evidence from industrialization in France (No. w27503). National Bureau of Economic Research
Jung JH, Lim DG (2020) Industrial robots, employment growth, and labor cost: a simultaneous equation analysis. Technol Forecast Soc Change 159:120202
Kozul-Wright R (2016, October). Robots and industrialization in developing countries. In United Nations Conference on Trade and Development (No. 60)
Kromann L, Malchow-Møller N, Skaksen JR, Sørensen A (2020) Automation and productivity—a cross-country, cross-industry comparison. Ind Corp Change 29:265–287. https://doi.org/10.1093/icc/dtz039
LeSage J, Pace RK (2009) Introduction to Spatial Econometrics. Chapman and Hall/CRC, New York, p 340. 10.1201/9781420064254
LeSage J (2015) Spatial econometrics. In Handbook of research methods and applications in economic geography (pp. 23-40). Edward Elgar Publishing
Levinson A, Taylor MS (2008) Unmasking the pollution haven effect. Int Econ Rev 49(1):223–254
Li J, Ma S, Qu Y, Wang J (2023) The impact of artificial intelligence on firms’ energy and resource efficiency: empirical evidence from China. Resour Policy 82:103507. https://doi.org/10.1016/j.resourpol.2023.103507
Li Y, Zhang Y, Pan A, Han M, Veglianti E (2022) Carbon emission reduction effects of industrial robot applications: Heterogeneity characteristics and influencing mechanisms. Technol Soc 70:102034
Lin F (2017) Trade openness and air pollution: city-level empirical evidence from China. China Econ Rev 45:78–88
Liu H, Owens KA, Yang K, Zhang C (2020) Pollution abatement costs and technical changes under different environmental regulations. China Econ Rev 62:101497
Liu J, Yu Q, Chen Y, Liu J (2022) The impact of digital technology development on carbon emissions: a spatial effect analysis for China. Resour Conserv Recycl 185:106445. https://doi.org/10.1016/j.resconrec.2022.106445
Liu J, Liu L, Qian Y, Song S (2022) The effect of artificial intelligence on carbon intensity: evidence from China’s industrial sector. Socioecon Plann Sci 83:101002. https://doi.org/10.1016/j.seps.2020.101002
Liu Y, Ren T, Liu L, Ni J, Yin Y (2022) Heterogeneous industrial agglomeration, technological innovation and haze pollution. China Econ Rev https://doi.org/10.1016/j.chieco.2022.101880
Luan F, Yang X, Chen Y, Regis PJ (2022) Industrial robots and air environment: a moderated mediation model of population density and energy consumption. Sustain Prod Consum. 30:870–888
Luo Z, Wan G, Wang C, Zhang X (2018) Urban pollution and road infrastructure: a case study of China. China Econ Rev 49:171–183
Milani S (2017) The impact of environmental policy stringency on industrial R&D conditional on pollution intensity and relocation costs. Environ Resour Econ 68:595–620
Moni SM, Mahmud R, High K, Carbajales‐Dale M (2020) Life cycle assessment of emerging technologies: a review. J Ind Ecol 24(1):52–63
Nguyen TT, Pham TAT, Tram HTX (2020) Role of information and communication technologies and innovation in driving carbon emissions and economic growth in selected G-20 countries. J Environ Manag 261:110162. https://doi.org/10.1016/j.jenvman.2020.110162
Pargal S, Wheeler D (1996) Informal regulation of industrial pollution in developing countries: evidence from Indonesia. J Polit Econ 104:1314–1327. https://doi.org/10.1086/262061
Pellenbarg PH, Van Wissen LJ, Van Dijk J (2002) Firm Relocation: State of the Art and Research Prospects. University of Groningen, Groningen, p 1–42
Pennings E, Sleuwaegen L (2000) International relocation: firm and industry determinants. Economics Letters 67(2):179–186
Philippon T, Reshef A (2012) Wages and human capital in the US finance industry: 1909–2006. Q J Econ 127(4):1551–1609
Pires JN (2007) Introduction to the Industrial Robotics World. https://doi.org/10.1007/978-0-387-23326-0_1
Pope III CA, Renlund DG, Kfoury AG, May HT, Horne BD (2008) Relation of heart failure hospitalization to exposure to fine particulate air pollution. Am J Cardiol 102(9):1230–1234
Article PubMed Google Scholar
Porter ME (2000) Locations, clusters, and company strategy. The Oxford Handbook of Economic Geography 253:274
Shen K, Gang J, Xian F, University N, University F (2017) Does environmental regulation cause pollution to transfer nearby? Econ Res J 52:44–59
Sheng D, Bu W (2022) The usage of robots and enterprieses’ pollution emissions in China. J Quant Technol Econ 2022(9):157–176
Shi B, Feng C, Qiu M, Ekeland A (2018) Innovation suppression and migration effect: The unintentional consequences of environmental regulation. China Econ Rev 49:1–23
Soergel A (2015) Robots could cut labor costs 16 percent by 2025 - US News & World Report. Retrieved August 7, 2022, from https://www.usnews.com/news/articles/2015/02/10/robots-could-cut-international-labor-costs-16-percent-by-2025-consulting-group-says
Tabuchi T (1998) Urban agglomeration and dispersion: a synthesis of Alonso and Krugman. J Urb Econ 44(3):333–351
Uhlmann E, Reinkober S, Hollerbach T (2016) Energy efficient usage of industrial robots for machining processes. Proc CIRP 48:206–211. https://doi.org/10.1016/j.procir.2016.03.241
Van Donkelaar A, Hammer MS, Bindle L, Brauer M, Brook JR, Garay MJ, Martin RV (2021) Monthly global estimates of fine particulate matter and their uncertainty. Environ Sci Technol 55(22):15287–15300
Article ADS PubMed Google Scholar
Wang E-Z, Lee C-C, Li Y (2022) Assessing the impact of industrial robots on manufacturing energy intensity in 38 countries. Energy Econ 105:105748. https://doi.org/10.1016/j.eneco.2021.105748
Wang Y, Feng J (2022) The Adoption Of Industrial Robots And Pollution Abatement In China
Weiss, Andrew (1995) Human Capital vs. Signalling Explanations of Wages. Journal of Economic Perspectives 9(4):133–154
Wilts H, Garcia BR, Garlito RG, Gómez LS, Prieto EG (2021) Artificial intelligence in the sorting of municipal waste as an enabler of the circular economy. Resources 10:28. https://doi.org/10.3390/resources10040028
World Health Organization. (2021) New WHO Global Air Quality Guidelines aim to save millions of lives from air pollution. Air Pollution is One of the Biggest Environmental Threats to Human Health, Alongside Climate Change
Wu Q, Wang Y (2022) How does carbon emission price stimulate enterprises’ total factor productivity? Insights from China’s emission trading scheme pilots. Energy Econ 109:105990. https://doi.org/10.1016/j.eneco.2022.105990
Wu Q (2023) Sustainable growth through industrial robot diffusion: quasi-experimental evidence from a Bartik shift-share design. Econ Transit Inst Change 31:1107–1133. https://doi.org/10.1111/ecot.12367
Wu Q, Sun Z, Jiang L, Jiang L (2023) “Bottom-up” abatement on climate from the “top-down” design: lessons learned from China’s low-carbon city pilot policy. Empir Econ 66:1223–1257
Wu Y, Al-Duais ZAM, Peng B (2023) Towards a low-carbon society: spatial distribution, characteristics and implications of digital economy and carbon emissions decoupling. Human Soc Sci Commun 10:1–13. https://doi.org/10.1057/s41599-023-02233-5
Yu Y, Zhang N (2021) Low-carbon city pilot and carbon emission efficiency: quasi-experimental evidence from China. Energy Econ 96:105125
Zhang X, Wu L, Zhang R, Deng S, Zhang Y, Wu J, Li Y, Lin L, Li L, Wang Y, Wang L (2013) Evaluating the relationships among economic growth, energy consumption, air emissions and air environmental protection investment in China. Renew Sustain Energy Rev 18:259–270. https://doi.org/10.1016/j.rser.2012.10.029
Zhang Z, Zhang W, Wu Q, Liu J, Jiang L (2024) Climate adaptation through trade: evidence and mechanism from heatwaves on firms’ imports. China Econ Rev 84:102–133
Zheng W, Chen P (2020) The political economy of air pollution: Local development, sustainability, and political incentives in China. Energy Res Soc Sci 69:101707
Zheng S, Yao R, Zou K (2022) Provincial environmental inequality in China: measurement, influence, and policy instrument choice. Ecol Econ 200:107537. https://doi.org/10.1016/j.ecolecon.2022.107537
Zhu H, Sang B, Zhang C, Guo L (2023) Have industrial robots improved pollution reduction? A theoretical approach and empirical analysis. China World Econ 31(4):153–172
Download references
Author information
Authors and affiliations.
College of Environmental Sciences and Engineering, Peking University, Beijing, 100871, China
Yanying Wang
Centre for Environment, Energy and Natural Resource Governance (CEENRG), Department of Land Economy, University of Cambridge, Cambridge, CB2 3QZ, UK
Department of Environmental Health Sciences, Fielding School of Public Health, University of California, Los Angeles, LA, CA, 90095, USA
Qingyang Wu
You can also search for this author in PubMed Google Scholar
Contributions
The authors confirm their contribution to the paper as follows: study conception and design: Q Wu; data collection: Y Wang and Q Wu; analysis and interpretation of the results: Y Wang and Q Wu; draft the paper preparation and revision: Y Wang and Q Wu. All authors reviewed the results and approved the final version of the paper.
Corresponding author
Correspondence to Qingyang Wu .
Ethics declarations
Competing interests.
The authors declare no competing interests.
Ethical approval
This article does not contain any studies with human participants performed by any of the authors.
Informed consent
Additional information.
Publisher’s note Springer Nature remains neutral with regard to jurisdictional claims in published maps and institutional affiliations.
Rights and permissions
Open Access This article is licensed under a Creative Commons Attribution 4.0 International License, which permits use, sharing, adaptation, distribution and reproduction in any medium or format, as long as you give appropriate credit to the original author(s) and the source, provide a link to the Creative Commons licence, and indicate if changes were made. The images or other third party material in this article are included in the article’s Creative Commons licence, unless indicated otherwise in a credit line to the material. If material is not included in the article’s Creative Commons licence and your intended use is not permitted by statutory regulation or exceeds the permitted use, you will need to obtain permission directly from the copyright holder. To view a copy of this licence, visit http://creativecommons.org/licenses/by/4.0/ .
Reprints and permissions
About this article
Cite this article.
Wang, Y., Wu, Q. Robots, firm relocation, and air pollution: unveiling the unintended spatial spillover effects of emerging technology. Humanit Soc Sci Commun 11 , 577 (2024). https://doi.org/10.1057/s41599-024-03100-7
Download citation
Received : 28 November 2023
Accepted : 17 April 2024
Published : 06 May 2024
DOI : https://doi.org/10.1057/s41599-024-03100-7
Share this article
Anyone you share the following link with will be able to read this content:
Sorry, a shareable link is not currently available for this article.
Provided by the Springer Nature SharedIt content-sharing initiative
Quick links
- Explore articles by subject
- Guide to authors
- Editorial policies

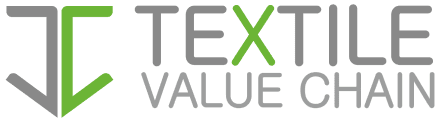
Design and Development of Children’s Wear Using Pre-consumer Textile Waste: A Sustainable Approach
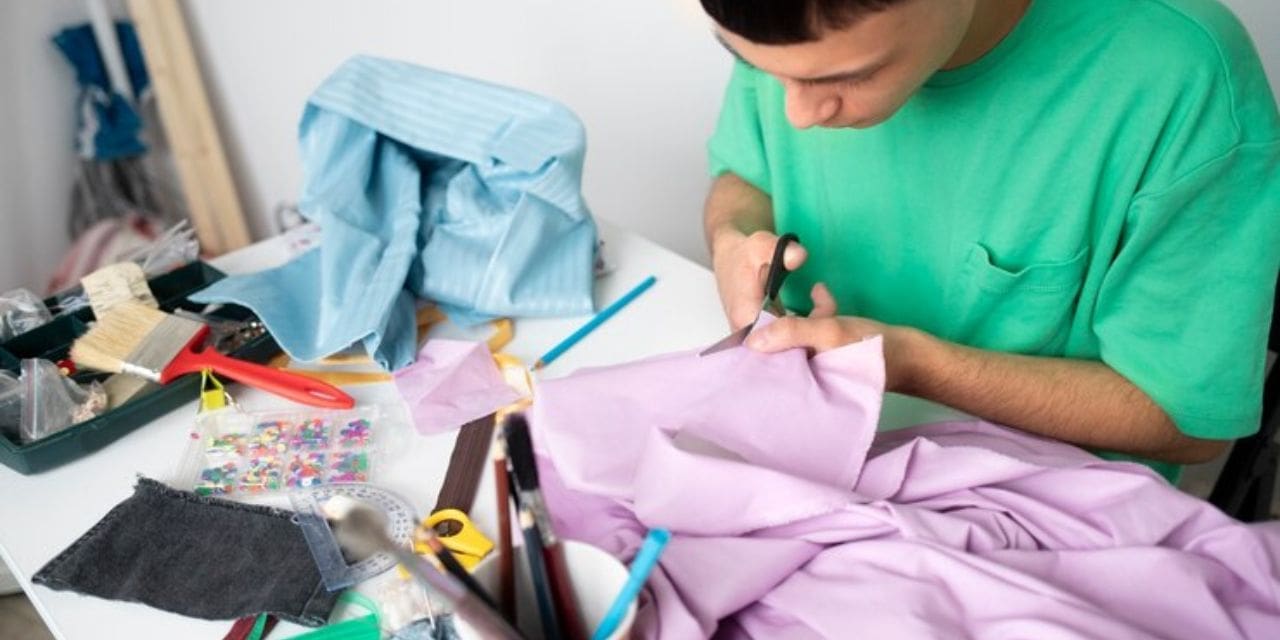
Harshita Bhown
Assistant Professor
Department of Fashion & Textiles
IIS (deemed to be University), Jaipur
Abstract: The textile and apparel industry in India is a significant contributor to waste generation and environmental pollution. To address this issue, sustainable practices such as textile recycling and upcycling have gained attention. This research paper focuses on utilizing pre-consumer textile waste to design and develop children’s wear, aiming to reduce the environmental impact of fashion products. The paper explores the concept of waste, particularly textile waste, and its environmental implications. It also discusses the types of textile waste and the need for textile waste recovery. The study emphasizes the process of refashioning and highlights the stages of the product design and development process. The significance of the study lies in promoting the use of pre-consumer textile waste in children’s clothing, encouraging sustainable practices in the fashion industry, and reducing environmental pollution. The objectives include identifying potential sources of waste fabric, developing a new fabric material, designing and creating a collection of children’s wear, and evaluating the market acceptability. The limitations of the study are also acknowledged, including the focus on boutique waste and a specific region.
INTRODUCTION
Textile and apparel industry in india.
The textile industry is a critical consumer goods industry, but it is also one of the most polluting industries. Waste is generated at every stage of textile production, including consumption. To address this problem, the textile industry has implemented measures to reduce its negative impact on the environment, including textile recycling and upcycling as a sustainability measure.
In India, the textile and clothing industry is the second-largest employer after agriculture and one of the largest producers of waste. However, human desires for fashion and technological advancements have resulted in an overflowing closet of garments, many of which are not being used. As a result, terms like upcycling, remade, reclaimed, and re-fashioned have emerged to promote sustainability. The disposal of textile waste, which often ends up in incinerators or landfills, is harming the environment, especially with the production of harmful gases.
Developed countries have become aware of the environmental degradation caused by our consumerist approach, and every manufacturing activity has come under scrutiny for sustainability, especially the textile industry. Recycling is essential because the clothing industry is the second-largest contributor to pollution. By processing textile waste and converting pre-consumer apparel waste into material or products for the development of kids wear, we can reduce the environmental impact of fashion products and contribute to the economic development of our community. The aim of this product development is to create kids wear with materials that decrease the environmental impact of fashion products and are loved by consumers, have a connection to them, and are designed to be kept and used for longer.
The concept of waste is an important one in many fields, including environmental science, economics, and sustainability. Waste can be defined as any product or substance that is no longer useful or valuable to the person or organization that owns it, and which is, or will be, discarded. This can include a wide range of materials, from household trash to industrial waste.
The concept of waste is closely linked to the idea of consumption. As humans, we consume natural resources and produce waste as a byproduct. This waste can take many forms, including physical waste (such as discarded food or packaging), chemical waste (such as industrial pollutants or hazardous materials), and even waste in the form of energy (such as unused heat or electricity).
One of the major challenges associated with waste is the environmental impact. Many types of waste can be harmful to the environment, either directly or indirectly. For example, organic waste can contribute to the release of greenhouse gases, while hazardous waste can contaminate soil and water sources.
To address the problem of waste, many organizations and individuals are focusing on reducing waste through practices such as recycling, composting, and conservation. By minimizing waste, we can reduce the impact on the environment and promote sustainability for future generations.
Textile waste
Textile waste refers to the material generated during the production and sale of clothing, which is no longer useful for its original purpose and is deemed unusable by the owner. It can come in the form of fabric and garment leftovers, or items that have reached the end of their life cycle and are destined for landfill or recycling.
Textile waste is a growing problem in today’s society due to the increasing production and consumption of clothing. According to the Environmental Protection Agency (EPA), the US alone generates around 16 million tons of textile waste each year, with only 15% of that being recycled or donated. The rest ends up in landfills, where it can take hundreds of years to decompose.
There are several sources of textile waste. Fashion and textile industry waste is created during the manufacturing process, when scraps of fabric and materials are left over from the production of clothing and accessories. Retailers also contribute to the problem by overstocking and discarding unsold or out-of-season items. Consumer waste is another significant source, as people discard clothing that they no longer want or need.
Types of Textile Waste
Textile waste can be categorized into two main types: pre-consumer waste and post-consumer waste. Pre-consumer waste, also known as factory waste or production waste, refers to materials discarded during the manufacturing process. This can include fabric scraps, cutting waste, and defective or rejected garments. Post-consumer waste, on the other hand, refers to clothing and textiles that are discarded by consumers after use.
Textile Waste Recovery
Textile waste recovery refers to the process of diverting textile waste from landfills and finding alternative uses for it. This can involve recycling, upcycling, or repurposing the waste materials to create new products or materials. By recovering textile waste, we can reduce the demand for virgin resources, conserve energy, and minimize the environmental impact of the textile industry.
Refashioning
Refashioning is a creative and sustainable approach to fashion design that involves transforming existing garments or textile waste into new, updated pieces. It focuses on reimagining and repurposing materials to extend their lifespan and reduce waste. Refashioning allows designers to experiment with different techniques, cuts, and styles, giving new life to discarded garments.
Product Design & Development from Waste
Designing and developing products from waste materials requires a systematic approach that encompasses various stages. This includes identifying potential sources of waste fabric, assessing their quality and suitability for reuse, developing innovative fabric materials, designing and creating new products, and evaluating their market acceptability. By incorporating waste materials into the design and development process, we can create unique and sustainable products that contribute to a circular economy and reduce the environmental impact of the textile industry.
In conclusion, the textile and apparel industry’s impact on the environment necessitates sustainable approaches to minimize waste generation and promote responsible consumption. This research paper focuses on utilizing pre-consumer textile waste to design and develop children’s wear, emphasizing the concept of waste, the types of textile waste, the need for textile waste recovery, the process of refashioning, and the stages of the product design and development process. By embracing sustainable practices and promoting the use of textile waste in children’s clothing, we can contribute to a greener and more environmentally conscious fashion industry.
METHODOLOGY:
The methodology for the research paper involves the following steps:
Research Design:
The research design is developed to obtain first-hand information and integrate different components of the study in a systematic manner.
Research Process:
The research process consists of three phases: exploratory phase, development of fabric and garment phase, and evaluation phase.
Exploratory Phase:
- A survey is conducted in Mansarovar, Jaipur, to gather information from 50 boutiques through a self-structured questionnaire and interview schedule.
- Data collection is carried out through the survey process.
Development of Fabric and Garment Phase:
- Fabric waste is collected from 50 boutiques in Mansarovar, Jaipur.
- The collected fabric waste is segregated into biodegradable and non-biodegradable materials.
- Design of kids’ wear is developed using CAD software, with 10 designs created and selected through a 5-point rating.
- Fabric is developed from the waste materials using the patchwork technique.
- Four final designs are chosen for construction.
- Garments for children aged 3-5 are constructed using the developed fabric.
Evaluation Phase:
- The evaluation of sketches and designs is done through a 5-point ranking scale completed by 100 consumers (mothers).
- Market acceptability is checked.
- A pilot study is conducted, including fabric waste collection, sketch development, fabric development, creation of prototypes, assessment of acceptability by mothers, and data analysis.
- The methodology aims to reduce environmental pollution, save resources, and repurpose textile waste into children’s wear. It involves data collection, analysis, and evaluation to ensure the developed garments meet customer needs and preferences. The research is conducted in Mansarovar, Jaipur, focusing on boutiques in the area.
Result Analysis:
The research paper presents the results of three different surveys: a boutique survey, a consumer survey, and a survey on the acceptability of CAD designs for kids’ frocks. Here is a summary of the key findings from each survey:
Boutique Survey:
- 92% of respondents are familiar with the concept of recycling discarded fabrics.
- The types of discarded fabrics generated in the boutiques include small cutting pieces (36%), very small pieces (26%), decorative pieces (16%), and remaining fabric (12%).
- Preferred methods for disposing of discarded fabrics include upcycling into other items and clothing swaps (24% each), donating (16%), and disposing into trash (12%).
- Reasons for getting rid of discarded fabrics include the need for more space (26%), inconvenience (30%), and difficulty in recycling or upcycling (28%).
- 50% of respondents dispose of discarded fabrics in the trash once a week, while 26% do so once a month.
- 62% of respondents dispose of discarded fabrics into the trash because it saves time, and 34% do so because it saves labor.
- All types of fabrics are commonly discarded in boutiques (100%).
- 7% of respondents classify discarded fabrics before disposing of them, while 40.8% do not.
- 46% of respondents consider it very important to utilize discarded fabrics.
- Prototype : According to sketched designs four product were constructed by own
Consumer Survey:
- 6% of respondents are willing to buy sustainable garments for their kids.
- Fabindia is the most well-known sustainable clothing brand (60% awareness).
- 54% of respondents are willing to spend a little more than usual on sustainable apparel for kids.
- The most common problems faced with existing apparel for kids include fit (50%), comfort (50%), durability (50%), quality (50%), and design varieties (50%).
- The majority of respondents spend between Rs. 2,500 and Rs. 10,000 on kids’ clothes in 6 months.
- The most preferred style of dressing for kids is contemporary (32.7%), followed by eclectic (35.4%).
- Abstract prints are the most preferred for kids’ clothing (38%).
- The important criteria while purchasing kids’ clothing include price (50%), quality (50%), comfort (50%), patterns (59%), and colors (50%).
Acceptability of CAD Designs for Kids’ Frocks:
- The most preferred CAD designs for kids’ frocks include GD 01 (78.8%), GD 02 (60.6%), GD 03 (67.7%), GD 10 (62.6%), and GD 04 (14.1%).
- These are the main findings from the research paper, highlighting the preferences, opinions, and behaviors of both boutiques and consumers regarding discarded fabrics, sustainable clothing, and CAD designs for kids’ frocks.
Summary and Conclusion:
The textile industry is a significant contributor to pollution, generating waste at every stage of production and consumption. To address this issue, the concept of textile recycling and upcycling has emerged as a sustainable solution. In the context of India, which has a large textile and clothing industry and produces substantial waste, the need for sustainable practices is crucial. This research focused on developing children’s wear using waste fabric, with the aim of reducing the environmental impact of fashion products and promoting economic development.
The study categorized textile waste into three main types: pre-consumer waste, post-consumer waste, and industrial/production waste. The objectives of the study were to identify potential sources of waste fabric in Jaipur, develop a new fabric material from the waste fabric, design and create a collection of children’s wear using the new fabric, and evaluate the market acceptability of the collection.
Waste fabric, known as chindi fabric, was collected from boutiques in Mansarovar, Jaipur. Sketches of kids’ frocks were developed based on current fashion trends and forecasts using CAD software. The collected fabric waste was sorted and transformed through patchwork techniques to create the fabric for the garments. The developed garments were constructed using sustainable materials and techniques, ensuring both practicality and fashion appeal.
To assess the market acceptability, a convenient sampling approach was used, and mothers of kids participated in a survey using a questionnaire. The survey evaluated various aspects of the developed kidswear, including design, quality, and pricing. The results indicated a positive attitude toward sustainable garment development using waste fabric for kidswear, with respondents appreciating the concept of recycled clothing.
The market survey provided valuable insights into customer preferences and expectations, allowing for improvements in future collections. Overall, this research demonstrates the potential of upcycling waste fabric to create environmentally responsible and aesthetically pleasing children’s wear. It emphasizes the importance of sustainable textile practices and the need to reduce textile waste through innovative approaches.
Result Analysis: The research paper focuses on utilizing pre-consumer textile waste to design and develop children’s wear, aiming to reduce the environmental impact of fashion products.
The methodology involves three phases: exploratory phase, development of fabric and garment phase, and evaluation phase .
The evaluation phase includes a 5-point ranking scale completed by 100 consumers (mothers) to assess market acceptability.
The research paper presents the results of three different surveys: a boutique survey, a consumer survey, and a survey on the acceptability of CAD designs for kids’ frocks .
The research is conducted in Mansarovar, Jaipur, focusing on boutiques in the area.
The research aims to reduce environmental pollution, save resources, and repurpose textile waste into children’s wear.
Works Cited
Pandey, N., & Gupta, A. (2022). Textile recycling and upcycling as sustainability measures: A case study of developing kidswear using textile waste. Journal of Cleaner Production, 330, 129757. https://doi.org/10.1016/j.jclepro.2020.129757
Gupta, A. (2017). Exploring the potential of chindi (textile waste) for producing quality products for women empowerment.
Dave, J., & Babel, S. (n.d.). Disposing Pattern Of Boutiques And Tailor Shops Cutting Waste.
Jain, P., & Gupta, C. (2018). Finding treasure out of textile trash generated by garment manufacturing units in Delhi/NCR.
Aparna, V., & Adnan, M. (2021). To design a capsule wardrobe with size adjustable clothing for toddlers using dead stock and eco-friendly fabrics. International Journal of Aquatic Science. Retrieved from http://www.journalaquaticscience.com/article_136012_6f77785ce9a4ce646ef8067c8061adca.pdf
Roy, N. C., Hannan, M. A., Uddin, K. M., Uddin, M. N., & Rana, M. R. (2015). Recycling garments fabric waste.
Jain, M. ( 2018) . Challenges for sustainability in textile craft and fashion design.
Selvaraj, R., & Priyanka,R., ( 2015). Study on Recycled Waste Cloth in Concrete
Rani, S., & Jamal.Z., (2018). Recycling of textiles waste for environmental protection.
Tripathi, V.M., (2019). An Empirical Study on the Indian kids apparel market
Shaharuddin, S., & Jalil, M., (2021) Multifunctional Children Clothing Design Process Based on the Eco-Fashion Design Model.
Islam, J., & Islam S., ( 2021 ). Sources and Fates of Textile Solid Wastes and Their Sustainable Management
Gam, H., & Cao, H., & Farr, C., & Kang, M., ( 2010 ). Quest for the eco-apparel market: A study of mothers’ willingness to purchase organic cotton clothing for their children
Related Posts

Autefa At ITMA – Innovative fiber recycling solutions for sustainable textile circularity

SECOND INFUSION OF RS 600 CRORE BY IKEA IN ITS INDIA ENTITY

TORAY’S COMPOSITES USED IN ESA’S PAYLOAD DISPENSER

PM to inaugurate The India Toy Fair 2021 on 27th February
In-depth characterization of particulate matter in a highly polluted urban environment at the foothills of Himalaya–Karakorum Region
- Research Article
- Published: 13 May 2024
Cite this article
- Bahadar Zeb 1 ,
- Khan Alam ORCID: orcid.org/0000-0001-7674-811X 2 , 3 ,
- Zhongwei Huang 2 ,
- Fatma Öztürk 4 ,
- Peng Wang 5 ,
- Lyudmila Mihaylova 6 ,
- Muhammad Fahim Khokhar 7 &
- Said Munir 8
56 Accesses
Explore all metrics
In recent years, the rising levels of atmospheric particulate matter (PM) have an impact on the earth’s system, leading to undesirable consequences on various aspects like human health, visibility, and climate. The present work is carried out over an insufficiently studied but polluted urban area of Peshawar, which lies at the foothills of the famous Himalaya and Karakorum area, Northern Pakistan. The particulate matter with an aerodynamic diameter of less than 10 µm, i.e., PM 10 are collected and analyzed for mineralogical, morphological, and chemical properties. Diverse techniques were used to examine the PM 10 samples, for instance, Fourier transform infrared spectroscopy, x-ray diffraction, and scanning electron microscopy along with energy-dispersive x-ray spectroscopy, proton-induced x-ray emission, and an OC/EC carbon analyzer. The 24 h average PM 10 mass concentration along with standard deviation was investigated to be 586.83 ± 217.70 µg/m 3 , which was around 13 times greater than the permissible limit of the world health organization (45 µg/m 3 ) and 4 times the Pakistan national environmental quality standards for ambient PM 10 (150 µg/m 3 ). Minerals such as crystalline silicate, carbonate, asbestiform minerals, sulfate, and clay minerals were found using FTIR and XRD investigations. Microscopic examination revealed particles of various shapes, including angular, flaky, rod-like, crystalline, irregular, rounded, porous, chain, spherical, and agglomeration structures. This proved that the particles had geogenic, anthropogenic, and biological origins. The average value of organic carbon, elemental carbon, and total carbon is found to be 91.56 ± 43.17, 6.72 ± 1.99, and 102.41 ± 44.90 µg/m 3 , respectively. Water-soluble ions K + and OC show a substantial association ( R = 0.71). Prominent sources identified using Principle component analysis (PCA) are anthropogenic, crustal, industrial, and electronic combustion. This research paper identified the potential sources of PM 10 , which are vital for preparing an air quality management plan in the urban environment of Peshawar.
This is a preview of subscription content, log in via an institution to check access.
Access this article
Price includes VAT (Russian Federation)
Instant access to the full article PDF.
Rent this article via DeepDyve
Institutional subscriptions
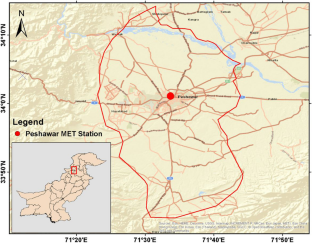
Similar content being viewed by others
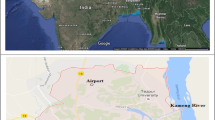
Morphology and mineralogy of ambient particulate matter over mid-Brahmaputra Valley: application of SEM–EDX, XRD, and FTIR techniques
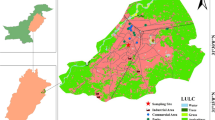
Assessment and characterization of particulate matter during the winter season in the urban environment of Lahore, Pakistan
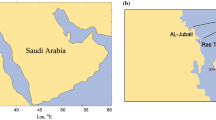
Characteristics of PM10 at industrial cities using integrated analytical techniques: Al-Jubail and Ras Tanura case study
Availability of data and materials.
The relevant author is agreeable to grant the data utilized in this research work on behalf of realistic appeal.
Alam K, Blaschke T, Madl P, Mukhtar A, Hussain M, Trautmann T, Rahman S (2011) Aerosol size distribution and mass concentration measurements in various cities of Pakistan. J Environ Monit 13:1944–1952
Article CAS Google Scholar
Alam K, Mukhtar A, Shahid I, Blaschke T, Majid H, Rahman S et al (2014) Source apportionment and characterization of particulate matter (PM10) in urban environment of Lahore. Aerosol Air Qual Res 14(7):1851–1861
Alam K, Rahman N, Khan HU, Haq BS, Rahman S (2015) Particulate matter and its source apportionment in Peshawar, Northern Pakistan. Aerosol Air Qual Res 15:634–647
Aldape F, Flores MJ, Diaz RV, Crumpton D (1996a) Upgrading of the PIXE system at ININ (Mexico) and report on elemental composition of atmospheric aerosols from 1990 in the ZMCM. Nucl Instr Meth B 109:459–464
Article Google Scholar
Aldape F, Flores MJ, García RG, Nelson JW (1996b) PIXE analysis of atmospheric aerosols from a simultaneous three site sampling during the autumn of 1993 in Mexico City. Nucl Instr Meth B 109:502–505
Aldape F (2004) Uso de los Aceleradores en la Búsqueda de Soluciones a la Problemática Ambiental: Trascendencia Social. In: Experiencia Mexicana en Aceleradores de Partículas. Serie: Ciencia y Tecnología en la Historia de Mexico. Editorial Siglo XXI. Primera Edición, p 203
Google Scholar
Alexis NE et al (2006) Biological material on inhaled coarse fraction particulate matter activates airway phagocytes in vivo in healthy volunteers. J Allergy Clin Immunol 117:1396–1403
Allen DT, Palen EJ, Haimov MI, Hering SV, Young JR (1994) Fourier transforms infrared spectroscopy of aerosol collected in a low pressure impactor (LPI/FTIR): method development and field calibration. Aerosol Sci Technol 21:325–342
Andreae MO, Rosenfeld DJESR (2008) Aerosol–cloud–precipitation interactions. Part 1. The nature and sources of cloud-active aerosols. Earth-Sci Rev 89(1–2):13–41
Antao SM, Hassan I, Wang J, Lee PL, Toby BH (2008) State-of-the-art high-resolution powder X-ray diffraction (HRPXRD) illustrated with Rietveld structure refinement of quartz, sodalite, tremolite, and meionite. Can Miner 46(6):1501–1509
Bahadur R, Uplinger T, Russell LM, Sive BC, Cliff SS, Millet DB, Goldstein A, Bates TS (2010) Phenol groups in northeastern US submicrometer aerosol particles produced from seawater sources. Environ Sci Technol 44:2542–2548
Balakrishna G, Pervez S, Bisht DS (2011) Source apportionment of arsenic in atmospheric dust fall out in an urban residential area, Raipur, central India. Atmos Chem Phys 11:5141–5151. https://doi.org/10.5194/acp-11-5141-2011
Balducci D, Valerio F (1986) Qualitative and quantitative evaluation of chrysotile and crocidolite fibers with IR-spectroscopy: application to asbestos-cement products. Int J Environ Anal Chem 27(4):315–323
Bernardoni V, Vecchi R, Valli G, Piazzalunga A, Fermo P (2011) PM10 source apportionment in Milan (Italy) using time-resolved data. Sci Total Environ 409:4788–4795. https://doi.org/10.1016/j.scitotenv.2011.07.048
Bharti SK, Kumar D, Anand S, Barman SC, Kumar N (2017) Characterization and morphological analysis of individual aerosol of PM10 in urban area of Lucknow, India. Micron 103:90–98
Bhuyan P, Deka P, Prakash A, Balachandran S, Hoque RR (2018) Chemical characterization and source apportionment of aerosol over mid Brahmaputra Valley, India. Environ Pollut 234:997–1010
Bora J, Deka P, Bhuyan P, Sarma KP, Hoque RR (2021) Morphology and mineralogy of ambient particulate matter over mid Brahmaputra Valley: application of SEM–EDX, XRD, and FTIR techniques. SN Appl Sci 3:137
Brauer M, Amann M, Burnett RT, Cohen A, Dentener F, Ezzati M, Henderson SB, Krzyzanowski M, Martin RV, Van Dingenen R, Van Donkelaar A (2012) Exposure assessment for estimation of the global burden of disease attributable to outdoor air pollution. Environ Sci Technol 46(2):652–660
Braun RA, Dadashazar H, MacDonald AB, Aldhaif AM, Maudlin LC, Crosbie E, Aghdam MA, Hossein Mardi A, Sorooshian A (2017) Impact of wildfire emissions on chloride and bromide depletion in marine aerosol particles. Environ Sci Technol 51:9013–9021
Cachier H, Bremond MP, Buat-Menard PATRICK (1989) Determination of atmospheric soot carbon with a simple thermal method. Tellus B: Chem Phys Meteorol 41(3):379–390
Calvo AI, Alves C, Castro A, Pont V, Vicente AM, Fraile R (2013) Research on aerosol sources and chemical composition: past, current and emerging issues. Atmos Res 120:1–28
Chang Y, Huang K, Xie M, Deng C, Zou Z, Liu S, Zhang Y (2018) First long-term and near real-time measurement of trace elements in China’s urban atmosphere: temporal variability, source apportionment and precipitation effect. Atmos Chem Phys 18:11793–11812. https://doi.org/10.5194/acp-18-11793-2018
Chen Y, Zhi G, Feng Y, Fu J, Feng J, Sheng G, Simoneit BR (2006) Measurements of emission factors for primary carbonaceous particles from residential raw-coal combustion in China. Geophys Res Lett 33(20)
Choobari OA, Zawar-Reza P, Sturman A (2014) The global distribution of mineral dust and its impacts on the climate system: a review. Atmos Res 138:152–165
Chow JC, Watson JG, Lu Z, Lowenthal DH, Frazier CA, Solomon PA, Thuillier RH, Magliano K (1996) Descriptive analysis of PM2.5 and PM10 at regionally representative locations during SJVAQS/AUSPEX. Atmos Environ 30:2079–2112
Chowdhury S, Dey S, Smith KR (2018) Ambient PM2. 5 exposure and expected premature mortality to 2100 in India under climate change scenarios. Nature Commun 9(1):318
Colthup NB, Daly LH, Wiberley SE (1990) Introduction to infrared and Raman spectroscopy, 3rd edn. Acadamic press, Newyork
Coury C, Dillner AM (2008) A method to quantify organic functional groups and inorganic compounds in ambient aerosols using attenuated total reflectance FTIR spectroscopy and multivariate chemometric techniques. Atmos Environ 42:5923–5932
Crosbie E, Sorooshian A, Monfared NA, Shingler R, Esmaili O (2014) A multi-year aerosol characterization for the greater Tehran Area using satellite, surface, and modeling data. Atmos 5(2):178–197
De Marco A, Proietti C, Anav A, Ciancarella L, D’Elia I, Fares S, Fornasierb MF, Fusaroe L, Manese MGF, Marchettof A, Mirceac M, Paolettig E, Piersantic A, Rogoraf M, Salvatid L, Salvatorie E, Screpantih A, Vialettoc G, Vitalee M, Leonardi C (2019) Impacts of air pollution on human and ecosystem health, and implications for the National Emission Ceilings Directive: insights from Italy. Environ Int 125:320–333
Dockery CA, Hueckel-Weng R, Birbaumer N, Plewnia C (2009) Enhancement of planning ability by transcranial direct current stimulation. J Neurosci 29(22):7271–7277
Draxler RR (2003) HYSPLIT (hybrid single-particle Lagrangian integrated trajectory) model. http://www.arl.noaa.gov/HYSPLIT.php
EPA, U (2004) EPA air quality criteria for particulate matter (final report, Oct 2004). US Environmental Protection Agency, Washington EPA 600/P-99/002aF-bF
Falkovich AH, Ganor E, Levin Z, Formenti P, Rudich Y (2001) Chemical and mineralogical analysis of individual mineral dust particles. J Geophys Res Atmos 106(D16):18029–18036
Fang Y, Naik V, Horowitz LW, Mauzerall DL (2013) Air pollution and associated human mortality: the role of air pollutant emissions, climate change and methane concentration increases from the pre industrial period to present. Atmos Chem Phys 13:1377–1394
Farsani MH, Shirmardi M, Alavi N, Maleki H, Sorooshian A, Babaei A et al (2018) Evaluation of the relationship between PM10 concentrations and heavy metals during normal and dusty days in Ahvaz. Iran Aeolian Res 33:12–22
Flores MJ, Aldape F (2001) PIXE study of airborne particulate matter in northern Mexico City. Int J PIXE 11(1–2):61–67
Flores MJ, Aldape F, DÍAZ, Crumpton D (1993) Set-up and improvements of the PIXE facility at ININ, Mexico. Nucl Instr Meth B 75(1–4):116–119
Flores MJ, Aldape F, Diaz RV, Hernández-Méndez B (1999) PIXE analysis of airborne particulate matter from Xalostoc, Mexico: winter to summer comparison. Nucl Instr Meth B 150(1–4):445–449
Götschi T, Oglesby L, Mathys P, Monn C, Manalis N, Koistinen K et al (2002) Comparison of black smoke and PM2. 5 levels in indoor and outdoor environments of four European cities. Environ Sci Technol 36(6):1191–1197
Gualtieri AF, Venturelli P (1999) In situ study of the goethite-hematite phase transformation by real time synchrotron powder diffraction. Am Mineral 84(5–6):895–904
Gugamsetty B, Wei H, Liu CN, Awasthi A, Hsu SC, Tsai CJ, Roam GD, Wu YC, Chen CF (2012) Source characterization and apportionment of PM10, PM2.5 and PM0.1 by using positive matrix factorization. Aerosol Air Qual Res 12:476–491
Gupta I, Kumar R (2006) Trends of particulate matter in four cities in India. Atmos Environ 40:2552–2566
Hansen LP, Sargent TJ (2001) Robust control and model uncertainty. Am Econ Rev 91(2):60–66
Harper M (2008) 10th Anniversary critical review: naturally occurring asbestos. J Environ Monit 10(12):1394–1408
Herman JR, Bhartia PK, Torres O, Hsu C, Seftor C, Celarier E (1997) Global distribution of UV- absorbing aerosols from Nimbus 7/TOMS data. J Geophys Res 102:16911–16922
Houghton JT, Ding Y, Griggs DJ, Noguer M, van der Linden PJ, Dai X, Maskell K, Johnson CA (2001) Climate change. Cambridge University Press, Cambridge, p 881
Khan S, Zeb B, Ullah S, Huraira MM, Ali G, Zahid S, Rahman Z (2024) Assessment and characterization of particulate matter during the winter season in the urban environment of Lahore, Pakistan. Int J Environ Sci Technol 21(1):833–844
Khaniabadi YO, Daryanoosh SM, Hopke PK, Ferrante M, De Marco A, Sicard P, Conti GO, Goudarzi G, Basiri H, Mohammadi MJ, Keishams F (2017) Acute myocardial infarction and COPD attributed to ambient SO2 in Iran. Environ Res 156:683–687
Khokhar MF, Yasmin N, Chishtie F, Shahid I (2016) Temporal variability and characterization of aerosols across the Pakistan region during the winter fog periods. Atmosphere 7(5):67
Kleshtanova V, Tonchev V, Stoycheva A, Angelov C (2023) Cloud condensation nuclei and backward trajectories of air masses at Mt. Moussala in two months of 2016. J Atmos Solar-Terrestrial Phys 243:106004
Kłosek-Wawrzyn E, Małolepszy J, Murzyn P (2013) Sintering behavior of kaolin with calcite. Procedia Eng 57:572–582
Kodama H, Oinuma K (1962) Identification of kaolin minerals in the presence of chlorite by X-ray diffraction and infrared absorption spectra. Clays Clay Miner 11(1):236–249
Kulkarni G, Mei F, Shilling JE, Wang J, Reveggino RP, Flynn C, Zelenyuk A, Fast J (2023) Cloud condensation nuclei closure study using airborne measurements over the Southern Great Plains. J Geophys Res: Atmos 128(5):e2022JD037964
Kulshrestha UC, Saxena A, Kumar N, Kumari KM, Srivastava SS (1998) Chemical composition and association of size differentiated aerosols at a suburban site in a semi arid tract of India. J Atmos Chem 29:109–118
Kumar B, Verma K, Kulshrestha U (2014) Deposition and mineralogical characteristics of atmospheric dust in relation to land use and land cover change in Delhi (India). Geography J. https://doi.org/10.1155/2014/325612
Kuzmichev AA, Loboyko VF (2016) Impact of the polluted air on the appearance of buildings and architectural monuments in the area of town planning. Procedia Eng 150:2095–2101
Lafuente B, Downs RT, Yang H, Stone N, Armbruster T, Danisi RM (2015) The power of databases: the RRUFF project. In: Highlights in mineralogical crystallography, vol 1, p 25
Lafuente R, García-Blàquez N, Jacquemin B, Checa MA (2016) Outdoor air pollution and sperm quality. Fertil Steril 106(4):880–896
Li W, Bai Z (2009) Characteristics of organic and elemental carbon in atmospheric fine particles in Tianjin, China. Particuology 7(6):432–437
Li WJ, Shao LY (2009) Observation of nitrate coatings on atmospheric mineral dust particles. Atmos Chem Phys 9:1863–1871
Lim SS, Vos T, Flaxman AD, Danaei G, Shibuya K, Adair-Rohani H, Aryee M (2012) A comparative risk assessment of burden of disease and injury attributable to 67 risk factors and risk factor clusters in 21 regions, 1990–2010: a systematic analysis for the Global Burden of Disease Study 2010. Lancet 380:2224–2260
Liu B, Li Y, Wang L, Bi X, Dong H, Sun X, Xiao Z, Zhang Y, Feng Y (2020) Source directional apportionment of ambient PM2. 5 in urban and industrial sites at a megacity in China. Atmos Res 235:104764
Lough GC, Schauer JJ, Park JS, Shafer MM, Deminter JT, Weinstein JP (2005) Emissions of metals associated with motor vehicle roadways. Environ Sci Technol 39:826–836
Madejova J, Komadel P (2001) Baseline studies of the clay minerals society source clays: infrared methods. Clays Clay Miner 49(5):410–432
Maenhaut W, Francois F, Cafmeyer J (1994) The “Gent” stacked filter unit (SFU) sampler for the collection of atmospheric aerosols in two size fractions: Description and instructions for installation and use (No. NAHRES--19)
Mahapatra PS, Sinha PR, Boopathy R, Das T, Mohanty S, Sahu SC, Gurjar BR (2018) Seasonal progression of atmospheric particulate matter over an urban coastal region in peninsular India: role of local meteorology and long-range transport. Atmos Res 199:145–158
Maleki H, Sorooshian A, Goudarzi G, Nikfal AH, Baneshi MM (2016) Temporal profile of PM10 and associated health effects in one of the most polluted cities of the world (Ahvaz, Iran) between 2009 and 2014. Aeolian Res 22:135–140
Manzoor S, Kulshrestha U (2015) Atmospheric aerosols: air quality and climate change perspectives. Curr World Environ 10(3):738–746
Maria SF, Russell LM, Turpin BJ, Porcja RJ (2002) FTIR measurements of functional groups and organic mass in aerosol samples over the Caribbean. Atmos Environ 36:5185–5196
Mariani LR, Mello W (2007) PM2.5–10, PM2.5 and associated water-soluble inorganic species at a coastal urban site in the metropolitan region of Rio de Janeiro. Atmos Environ 41:2887–2892
Marzouni MB, Moradi M, Zarasvandi A, Akbaripoor S, Hassanvand MS, Neisi A, Goudarzi G, Mohammadi MJ, Sheikhi R, Kermani M, Shirmardi M (2017) Health benefits of PM 10 reduction in Iran. Int j Biometeorol 61:1389–1401
Memhood T, Tianle Z, Ahmad I, Li X, Shen F, Akram W, Dong L (2018) Variations of PM2. 5, PM10 mass concentration and health assessment in Islamabad, Pakistan. In: IOP conference series: earth and environmental science, vol 133. IOP Publishing, p 012031
Miranda J, López-Suárez A, Paredes-Gutiérrez R, González S, De Lucio OG, Andrade E, Morales JR, Avila-Sobarzo MJ (1998) A study of atmospheric aerosols from five sites in Mexico city using PIXE. Nucl Instr Meth B 136(1–4):970–974
Misiūnas A, Niaura G, Talaikytė Z, Eicher-Lorka O, Razumas V (2005) Infrared and Raman bands of phytantriol as markers of hydrogen bonding and interchain interaction. Spectrochimica Acta Part A: Mol Biomol Spectrosc 62(4–5):945–957
Mogo S, Cachorro VE, de Frutos AM (2005) Morphological, chem- ical and optical absorbing characterization of aerosols in the urban atmosphere of Valladolid. Atmos Chem Phys Eur Geosci Union 5(10):2739–2748
Mukherjee A, Agrawal M (2017) World air particulate matter: sources, distribution and health effects. Environ Chem Let 15:283–309
Murr LE, Bang JJ (2003) Electron microscope comparisons of fine and ultra-fine carbonaceous and non-carbonaceous, airborne particulates. Atmos Environ 37:4795–4806
Nagendra SS, Diya M, Chithra VS, Menon JS, Peter AE (2016) Characteristics of air pollutants at near and far field regions of a national highway located at an industrial complex. Transport Res D:Trans Environ 48:1–13
Neupane BB, Amita S, Basant G, Mahesh KJ (2020) Characterization of airborne dust samples collected from core areas of Kathmandu Valley. Heliyon 6(4):e03791
Ojima J (2003) Determining of crystalline silica in respirable dust samples by infrared spectrophotometry in the presence of interferences. J Occup Health 45(2):94–103
Osinubi OY, Gochfeld M, Kipen HM (2000) Health effects of asbestos and nonasbestos fibers. Environ Health Persp 108:665–674
CAS Google Scholar
Öztürk F, Keleş M (2016) Wintertime chemical compositions of coarse and fine fractions of particulate matter in Bolu, Turkey. Environ Sci Pollut Res 23(14):4157–14172
Pachauri T, Singla V, Satsangi A, Lakhani A, Kumari KM (2013) SEM-EDX characterization of individual coarse particles in Agra, India. Aerosol Air Qual Res 13:523–536
Panda S, Nagendra SS (2018) Chemical and morphological characterization of respirable suspended particulate matter (PM10) and associated heath risk at a critically polluted industrial cluster. Atmos Pollut Res 9(5):791–803
Parmar RS, Satsangi GS, Kumari M, Lakhani A, Srivastava SS, Prakash S (2001) Study of size distribution of atmospheric aerosol at Agra. Atmos Environ 35:693–702
Pipal AS, Kulshrestha A, Taneja A (2011) Characterization and morphological analysis of airborne P M2.5 and P M10 in Agra located in north central India. Atmos Environ 45:3621–3630. https://doi.org/10.1016/j.atmosenv.2011.03.062
Pipal AS, Jan R, Satsangi PG, Tiwari S, Taneja A (2014) Study of surface morphology, elemental composition and origin of atmospheric aerosols (PM2.5 and P M10) over Agra, India. Aerosol Air Qual Res 14(6):1685–1700
Prabhu V, Shridhar V, Choudhary A (2019) Investigation of the source, morphology, and trace elements associated with atmospheric PM10 and human health risks due to inhalation of carcinogenic elements at Dehradun, an indo-Himalayan city. SN Appl Sci 1(5):429
Rahman SA, Hamzah MS, Elias MS, Salim NAA, Hashim A, Shukor S, Siong WB, Wood AK (2015) A long term study on characterization and source apportionment of particulate pollution in Klang Valley, Kuala Lumpur. Aerosol Air Qual Res 15(6):2291–2304
Rai P, Furger M, El Haddad I, Kumar V, Wang L, Singh A, Dixit K, Bhattu D, Petit JE, Ganguly D, Rastogi N (2020) Real-time measurement and source apportionment of elements in Delhi’s atmosphere. Sci Total Environ 742:140332
Ram K, Sarin MM (2010) Spatio-temporal variability in atmospheric abundances of EC, OC and WSOC over Northern India. J Aerosol Sci 41:88–98
Reizer M, Juda-Rezler K (2016) Explaining the high PM10 concentrations observed in Polish urban areas. Air Qual Atmos Hlth 9(5):517–531
Ristić M, Czakó-Nagy I, Musić S, Vértes A (2011) Spectroscopic characterization of chrysotile asbestos from different regions. J Mol Struc 993(1–3):120–126
Rodríguez I, Galí S, Marcos C (2009) Atmospheric inorganic aero- sol of a non-industrial city in the centre of an industrial region of the North of Spain, and its possible influence on the climate on a regional scale. Environ Geol 56(8):1551–1561
Schauer JJ, Kleeman MJ, Cass GR, Simoneit BRT (2001) Measurement of emissions from air pollution sources. 3. C1–C29 organic compounds from fireplace combustion of wood. Environ Sci Technol 35:1716–1728
Schauer JJ, Kleeman MJ, Cass GR, Simoneit BRT (2002) Measurement of emissions from air pollution sources. 5. C1–C32 organic compounds from gasoline-powered motor vehicles. Environ Sci Tech 36:1169–1180
See SW, Balasubramanium R (2008) Chemical characteristics of fine particles emitted from different gas cooking methods. Atmos Environ 42:8852–8862
Seinfeld JH, Pandis SN (2016) Atmospheric chemistry and physics: from air pollution to climate change. John Wiley & Sons
Seinfeld J, Pandis S (2006) Atmospheric chemistry and physics, from air pollution to climate change, 2nd edn. Willey Interscience Publication, USA
Shahid I, Chishtie F, Bulbul G, Shahid MZ, Shafique S, Lodhi A (2019) State of air quality in twin cities of Pakistan: Islamabad and Rawalpindi. Atmósfera 32(1):71–84
Shakya KM, Rupakheti M, Shahi A, Maskey R, Pradhan B, Panday A, Puppala SP, Lawrence M, Peltier RE (2017) Near-road sampling of PM 2. 5, BC, and fine-particle chemical components in Kathmandu Valley, Nepal. Atmos Chem Phys 17(10):6503–6516
Sharma SK, Mandal TK, Saxena M, Sharma A, Gautam R (2014) Source apportionment of PM10 by using positive matrix factorization at an urban site of Delhi, India. Urban Clim 10:656–670
Sharma A, Singh S, Kulshrestha UC (2017) Determination of urban dust signatures through chemical and mineralogical characterization of atmospheric dustfall in East Delhi (India). J Ind Geophys Union 21(2):140–147
Siddiqui MA, Ahmed Z (2005) Mineralogy of the Swat kaolin deposits, Pakistan. Arab J Sci Eng 30:195–218
Simao J, Agudo RE, Navarro RC (2006) Effects of particulate matter from gasoline and diesel vehicle exhaust emissions on silicate stones sulfation. Atmos Environ 40:6905–6917
Simonsen ME, Sønderby C, Li Z, Søgaard EG (2009) XPS and FT-IR investigation of silicate polymers. J Mater Sci 44(8):2079–2088
Singh R, Sharma BS (2012) Composition, seasonal variation, andsources of PM10 from world heritage site Taj Mahal, Agra. Environ Monit Assess 184:5945–5956
Steiner AL, Brooks SD, Deng C, Thornton DC, Pendleton MW, Bryant V (2015) Pollen as atmospheric cloud condensation nuclei. Geophys Res Lett 42(9):3596–3602
Tidblad J, Kreislová K, Faller M, De la Fuente D, Yates T, Verney-Carron A, Grøntoft T, Gordon A, Hans U (2017) ICP materials trends in corrosion, soiling and air pollution (1987–2014). Materials 10:969
Tsai YI, Kuo SC (2005) PM2.5 aerosol water content and chemical composition in a metropolitan and a coastal area in Southern Taiwan. Atmos Environ 39:4827–4839
Usman F, Zeb B, Alam K, Huang Z, Shah A, Ahmad I, Ullah S (2022a) In-depth analysis of physicochemical properties of particulate matter (PM10, PM2. 5 and PM1) and its characterization through FTIR, XRD and SEM–EDX techniques in the foothills of the Hindu Kush region of northern Pakistan. Atmosphere 13(1):124
Usman F, Zeb B, Alam K, Valipour M, Ditta A, Sorooshian A et al (2022b) Exploring the mass concentration of particulate matter and its relationship with meteorological parameters in the HinduKush range. Atmosphere 13(10):1628
Vempati RK, Loeppert RH, Sittertz-Bhatkar H, Burghardt RC (1990) Infrared vibrations of hematite formed froam aqueous-and dry-thermal incubation of Si-containing ferrihydrite. Clays Clay Miner 38(3):294–298
Wang J, Becker U (2009) Structure and carbonate orientation of vaterite (CaCO3). Am Mineral 94(2–3):380–386
Wang X, Zhang L, Yao Z, Ai S, Qian ZM, Wang H, BeLue R, Liu T, Xiao J, Li X, Zeng W (2018) Ambient coarse particulate pollution and mortality in three Chinese cities: association and attributable mortality burden. Sci Total Environ 628:1037–1042
Watson JG, Chow JC (2001) PM2.5 chemical source profiles for vehicle exhaust, vegetative burning, geological material, and coal burning in northwestern Colorado during 1995. Chemosphere 43:1141–1151
Wu Z, Liu F, Fan W (2015) Characteristics of PM10 and PM2.5 at Mount Wutai Buddhism scenic spot, Shanxi, China. Atmosphere 6(8):1195–1210
Yadav R, Sahu LK, Jaaffrey SNA, Beig G (2014) Temporal variation of particulate matter (PM) and potential sources at an urban site of Udaipur in Western India. Aerosol Air Qual Res 14(6):1613–1629
Yadav S, Gettu N, Swain B, Kumari K, Ojha N, Gunthe SS (2020) Bioaerosol impact on crop health over India due to emerging fungal diseases (EFDs): an important missing link. Environ Sci Pollut Res 27:12802–12829
Yassin A, Yebesi F, Tingle R (2005) Occupational exposure to crystalline silica dust in the United States, 1988–2003. Environ Health Perspec 113(3):255–260
Zeb B, Alam K, Sorooshian A, Blaschke T, Ahmad I, Shahid I (2018) On the morphology and composition of particulate matter in an urban environment. Aerosol Air Qual Res 18:1431–1447
Zeb B, Alam K, Ditta A, Ullah S, Ali HM, Ibrahim M, Salem MZ (2022) Variation in coarse particulate matter (PM10) and its characterization at multiple locations in the semiarid region. Front Environ Sci 10:843582
Zhang Y, Shao M, Zhang Y, Zeng L, He L, Zhu B, Wei Y, Zhu X (2007) Source profiles of particulate organic matters emitted from cereal straw burnings. J Environ Sci 19:167–175
Zhang L, Chen Z, Guo J, Xu Z (2021) Distribution of heavy metals and release mechanism for respirable fine particles incineration ashes from lignite. Resour Conserv Recycl 166:105282
Zhou J, Xing Z, Deng J, Du K (2016) Characterizing and sourcing ambient PM2. 5 over key emission regions in China I: water-soluble ions and carbonaceous fractions. Atmos Environ 135:20–30
Zuśka Z, Kopcińska J, Dacewicz E, Skowera B, Wojkowski J, Ziernicka-Wojtaszek A (2019) Application of the principal component analysis (PCA) method to assess the impact of meteorological elements on concentrations of particulate matter (PM10): a case study of the mountain valley (the Sącz Basin, Poland). Sustainability 11(23):6740
Download references
Acknowledgements
The authors are very much thankful to the Director Accelerator at the National Centre for Physics, Quaid-i-Azam University (Islamabad) for the PIXE analysis of PM 10 samples. It is noted that the Pakistan Meteorological Department (Regional Office Peshawar) provided meteorological data as well as graciously supporting and facilitating the collecting of PM 10 data. The authors appreciate the anonymous reviewers’ helpful criticism and improvement of the paper
Author information
Authors and affiliations.
Department of Mathematics, Shaheed Benazir Bhutto University, Sheringal, Dir (Upper), Pakistan
Bahadar Zeb
Collaborative Innovation Centre for Western Ecological Safety, College of Atmospheric Sciences, Lanzhou University, Lanzhou, 730000, China
Khan Alam & Zhongwei Huang
Department of Physics, University of Peshawar, Peshawar, 25120, Pakistan
Faculty of Engineering, Environmental Engineering Department, Bolu Abant İzzet Baysal University, Gölköy Campus 14030, Bolu, Turkey
Fatma Öztürk
Department of Computing and Mathematics, Manchester Metropolitan University, Manchester, M15 6BH, UK
Department of Automatic Control and Systems Engineering, The University of Sheffield, Sheffield, S10 2TN, UK
Lyudmila Mihaylova
Institute of Environmental Sciences and Engineering, National University of Sciences and Technology, Islamabad, 44000, Pakistan
Muhammad Fahim Khokhar
Institute for Transport Studies, University of Leeds, Leeds, LS2 9JT, UK
You can also search for this author in PubMed Google Scholar
Contributions
Bahadar Zeb: formal analysis, investigation, and writing original draft; Khan Alam: conceptualization, methodology, validation, writing—review and editing; Zhongwei Huang: formal analysis and investigation; Fatma Öztürk: methodology and formal analysis; Peng Wang: formal analysis and reviewing and editing; Lyudmila Mihaylova: reviewing and editing; Muhammad Fahim Khokhar: methodology and formal analysis; Said Munir: validation.
Corresponding author
Correspondence to Khan Alam .
Ethics declarations
Ethical approval.
The authors confirm that the current research study is novel and carried out morally. All authors have given their approval to the manuscript and the manuscript does not feature any research involving either animals or humans.
Consent to participate
The authors have given their approval to take part in this study.
Consent for publication
The authors provide their permission for publication in the ESPR journal for the present study.
Competing interests
The authors declare no competing interests.
Additional information
Responsible Editor: Gerhard Lammel
Publisher's Note
Springer Nature remains neutral with regard to jurisdictional claims in published maps and institutional affiliations.
Supplementary Information
Below is the link to the electronic supplementary material.
Supplementary file1 (DOCX 1081 KB)
Rights and permissions.
Springer Nature or its licensor (e.g. a society or other partner) holds exclusive rights to this article under a publishing agreement with the author(s) or other rightsholder(s); author self-archiving of the accepted manuscript version of this article is solely governed by the terms of such publishing agreement and applicable law.
Reprints and permissions
About this article
Zeb, B., Alam, K., Huang, Z. et al. In-depth characterization of particulate matter in a highly polluted urban environment at the foothills of Himalaya–Karakorum Region. Environ Sci Pollut Res (2024). https://doi.org/10.1007/s11356-024-33487-4
Download citation
Received : 12 December 2023
Accepted : 23 April 2024
Published : 13 May 2024
DOI : https://doi.org/10.1007/s11356-024-33487-4
Share this article
Anyone you share the following link with will be able to read this content:
Sorry, a shareable link is not currently available for this article.
Provided by the Springer Nature SharedIt content-sharing initiative
- Elemental carbon
- Organic carbon
- Total carbon
- Principle component analysis, Air quality analysis
- Find a journal
- Publish with us
- Track your research

IMAGES
VIDEO
COMMENTS
Pollution is defined as the introduction into the environment of substances harmful to humans and other living organisms. Pollutants are harmful solids, liquids, or gases produced in higher than usual concentrations that reduce the quality of our environment. ... In the present paper, we focus on the sources of environmental pollution in ...
Tribute to Martin L. Williams. Professor Martin L. Williams. Martin Williams, one of the organisers of the Discussion meeting leading to this collection of papers and an author of one with Paul Monks, has been a leader of research and policy development to solve the problems of poor air quality in the UK and more widely through Europe throughout the last four decades.
Abstract. Air pollution is a pressing global environmental challenge with far-reaching consequences for human health and well-being. This research paper presents an extensive examination of air ...
Moreover, air pollution seems to have various malign health effects in early human life, such as respiratory, cardiovascular, mental, and perinatal disorders ( 3 ), leading to infant mortality or chronic disease in adult age ( 6 ). National reports have mentioned the increased risk of morbidity and mortality ( 1 ).
Air pollution is a cause of disease for millions around the world and now more than ever urgent action is required to tackle the burden of its impacts. Doing so will not only improve both life ...
Clean air is a fundamental requirement for the existence of life on earth. However, with the rapid rate of economic development, globalization, and increasing energy demand, large amount of emissions and waste are generated, leading to severe air pollution. This paper surveys the literature to provide an overview of the impact of air pollution on various aspects of human life. The impact is ...
Purpose of Review During the past decade, weather and climate extremes, enhanced by climate change trends, have received tremendous attention because of their significant impacts on socio-economy, public health, and ecosystems. At the same time, many parts of the world still suffer from severe air pollution issues. However, whether and how air pollutants play a role in weather and climate ...
Air pollution is high on the global agenda and is widely recognised as a threat to both public health and economic progress. The World Health Organization (WHO) estimates that 4.2 million deaths ...
When we use less extreme (i.e., higher) poverty thresholds, the number of air pollution and poverty-exposed people increases significantly. We estimate that around 1.8 billion people living on ...
There are primarily two sources of air pollution in the atmosphere; (1) Natural emissions; and (2) Anthropogenic emissions. Anthropogenic emissions are produced by internal combustion (IC) engines and furnaces via combustion. IC engine has made our life more convenient; however, in return for this convenience, automobiles have caused air ...
Air pollution refers to the modification of the characteristics of the atmosphere induced by contaminants [1]. Sources of air pollution are, for example, any kind of combustion, vehicles, and ...
Introduction. Air pollution is almost a century-old challenge in India. However, the last few decades have been severe, ... At the time of analysis (February 2020), the repository was composed of approximately 1670 research papers, 30 reports and case studies, 66 court trials, and over 7 statutes, compiled with data from 1950 to February 2020. ...
Despite considerable air pollution prevention and control measures that have been put into practice in recent years, outdoor air pollution remains one of the most important risk factors for health outcomes. To identify the potential research gaps, we conducted a scoping review focused on health outcomes affected by outdoor air pollution across the broad research area. Of the 5759 potentially ...
This paper aims to provide an overview of recent research development and contemporary methodological challenges in this field and to identify future research directions for air pollution epidemiological studies. ... and 2.1% (p < 0.05) respectively in the first 12 months after an introduction of the restrictions on sulphur content of fuel in ...
Environmental air pollution is a major risk factor for morbidity and mortality worldwide. Environmental air pollution has a direct impact on human health, being responsible for an increase in the incidence of and number of deaths due to cardiopulmonary, neoplastic, and metabolic diseases; it also contributes to global warming and the consequent climate change associated with extreme events and ...
Air. pollution may be described as contamination o f the atmosphere by gaseous, liquid, or solid wastes or. by-products that can endang er human health and welfare o f plants and animals, at tack ...
In conclusion, this umbrella review confirms that air pollution and climate change represent intertwined elements of the main global crisis of our time. The study extends the vast list of adverse effects of exposures to air pollution and climate change hazards on physical health by indicating that they are also a threat to global mental health.
TLDR. This unique textbook examines the basic health and environmental issues associated with air pollution including the relevant toxicology and epidemiology from a fundamental science viewpoint and provides a foundation for the sampling and analysis of air pollutants as well as an understanding of international air quality regulations. Expand.
The study period 1975 to 2022 was selected because research of air pollution sharply increased in the 1970s and this 48-year period permits the identification of trends. ... Zhou M, Iems H, Paper W. The effect of air pollution on mortality in China: evidence from the 2008 Beijing Olympic Games. ... Introduction of local air quality management ...
Air pollution consists of chemicals or particles in the air that can harm the health of humans, animals, and plants. It also damages buildings. Pollutants in the air take many forms. They can be gases, solid particles, or liquid droplets. Sources of Air Pollution Pollution enters the Earth's atmosphere in many different ways. Most air pollution is created by people, taking the form of ...
1. Introduction. Indoor environment conditions contribute greatly to human wellbeing, as most people spend around 90% of their time indoors, mainly at home or in the workplace [].According to the World Health Organization (WHO), indoor air pollution (IAP) is responsible for the deaths of 3.8 million people annually [].IAP can be generated inside homes or buildings through occupants ...
Abstract. Air pollution occurs when gases, dust particles, fumes (or smoke) or odour are introduced into the atmosphere in a way that makes it harmful to humans, animals and plant. Air pollution ...
Section 2 presents the literature survey with a comparative analysis of the literary works in the realm of air quality prediction with ML. Section 3 describes the dataset being studied, preprocessing, and feature selection techniques applied. Section 4 deals with observing hidden patterns in the dataset through data visualisation. Section 5 is dedicated to the experimental design, analysis of ...
Abstract. Developing air quality management systems to control the impacts of air pollution requires reliable data. However, current initiatives do not provide datasets with large spatial and temporal resolutions for developing air pollution policies in Brazil. Here, we introduce the Brazilian Atmospheric Inventories (BRAIN), the first comprehensive database of air quality and its drivers in ...
Amidst the global upsurge in industrial robot deployment, there remains a notable gap in our understanding of their environmental impact. This paper explores how the introduction of industrial ...
Air pollution problem has become a major concern globally! High concentration of air pollutants are putting millions of people's health at risk. India is one of the countries that is severely ...
The methodology for the research paper involves the following steps: Research Design: The research design is developed to obtain first-hand information and integrate different components of the study in a systematic manner. Research Process: The research process consists of three phases: exploratory phase, development of fabric and garment ...
In recent years, the rising levels of atmospheric particulate matter (PM) have an impact on the earth's system, leading to undesirable consequences on various aspects like human health, visibility, and climate. The present work is carried out over an insufficiently studied but polluted urban area of Peshawar, which lies at the foothills of the famous Himalaya and Karakorum area, Northern ...