
- SUGGESTED TOPICS
- The Magazine
- Newsletters
- Managing Yourself
- Managing Teams
- Work-life Balance
- The Big Idea
- Data & Visuals
- Reading Lists
- Case Selections
- HBR Learning
- Topic Feeds
- Account Settings
- Email Preferences

Supply chain management
- Business management
- Operations and supply chain management
- Operations strategy

Souq.com’s CEO on Building an E-Commerce Powerhouse in the Middle East
- Ronaldo Mouchawar
- From the September–October 2017 Issue
Pandemic Preparedness: Who's Your Weak Link?
- George Abercrombie
- From the December 2007 Issue
The Japan Earthquake Rattles Supply Chains, Too
- Willy C. Shih
- March 23, 2011
Radically Simple IT
- David M. Upton
- Bradley Staats
- From the March 2008 Issue
Crime and Management: An Interview with New York City Police Commissioner Lee P. Brown
- Alan M. Webber
- From the May–June 1991 Issue
Inventory-Driven Costs
- Gianpaolo Callioni
- Xavier De Montgros
- Regine Slagmulder
- Luk N. Van Wassenhove
- Linda Wright
- From the March 2005 Issue
Innovate at Your Own Risk: Deborah Wince-Smith on Competitiveness
- Gardiner Morse
- From the May 2005 Issue

How Kenvue De-Risked Its Supply Chain
- Michael Altman
- Atalay Atasu
- Evren Özkaya
- October 18, 2023

The Latest Supply Chain Disruption: Plastics
- Bindiya Vakil
- March 26, 2021
Competing for Supply
- Bryan Stolle
- Bronwyn Fryer
- From the February 2001 Issue
Fit Products and Channels to Your Markets
- Robert E. Weigand
- From the January 1977 Issue
A New Way to Think About Office Lighting
- Iain Campbell
- Koben Calhoun
- James Mandel
- June 27, 2017
Why Sourcing Local Food Is So Hard for Restaurants
- Nicole Torres
- June 15, 2016

A Better Way for Employers to Procure Health Care
- Robert S. Mecklenburg M.D.
- November 17, 2016

The Circular Business Model
- Celine Dumas
- From the July–August 2021 Issue
Tiebreaker Selling
- James C. Anderson
- James A. Narus
- Marc Wouters
- From the March 2014 Issue
Break the Paper Jam in B2B Payments
- Steve Berez
- Arpan Sheth
- From the November 2007 Issue
Dangers of Modularity
- Olav Sorenson
- Lee Fleming
- From the September 2001 Issue

It's OK to Move Down (Yes, Down) the Value Chain
- Bradley R. Staats
- June 02, 2015
How I Did It: Timberland’s CEO on Standing Up to 65,000 Angry Activists
- Jeff Swartz
- From the September 2010 Issue

How Machine Learning Will Transform Supply Chain Management
- Narendra Agrawal
- Morris A. Cohen
- Rohan Deshpande
- Vinayak Deshpande
- From the March–April 2024 Issue

Building a Supplier Diversity Program? Learn from the U.S. Government.
- Chris Parker
- Dwaipayan Roy
- February 09, 2024

2023: A Strange, Tumultuous Year in Sustainability
- Andrew Winston
- December 28, 2023

How Global Companies Use AI to Prevent Supply Chain Disruptions
- Remko Van Hoek
- Mary Lacity
- November 21, 2023

Case Study: How Should We Diversify Our Supply Chain?
- Krishna G. Palepu
- From the November–December 2023 Issue

The Next Supply-Chain Challenge Isn’t a Shortage — It’s Inventory Glut
- PS Subramaniam
- September 29, 2023

Using Technology to Improve Supply-Chain Resilience
- Jonathan Colehower
- September 25, 2023

How Tony’s Chocolonely Created a Purpose-Driven (and Profitable) Supply Chain
- Frans Pannekoek
- Thomas Breugem
- September 11, 2023

Forecasting in Flux: Navigating Uncertainty through Strategic Supply Chain Flexibility
- June 15, 2023

Reducing Forecast Risk with Supply Chain Flexibility Webinar
- June 01, 2023

Rethinking Supply Chains for Greater Efficiency, Resiliency, and Sustainability
- April 27, 2023

How Tracking ESG Metrics Can Help Reduce Costs and Build Supply-Chain Resilience
- April 26, 2023

How to Build Supply Chain Resilience Without Sacrificing ESG Goals or Inflating Costs
- March 29, 2023

A Resilient Supply Chain Built for Competitive Advantage
- March 07, 2023

Marico’s Chairman on Innovating Across Every Part of the Business
- Harsh Mariwala
- From the January–February 2023 Issue

Crate & Barrel Holdings CEO Janet Hayes on Navigating Turbulent Times for Retail
- December 15, 2022

How Smaller Companies Can Bring Manufacturing Closer to Home
- Arnd Huchzermeier
- December 07, 2022

How American Eagle Reinvented Its Fulfillment Strategy
- Shekar Natarajan
- Thales S. Teixeira
- November 07, 2022

3 Ways to Build Trust with Your Suppliers
- Kate Vitasek
- Karl Manrodt
- Gerald Ledlow
- November 04, 2022

Sustainability at Siemens
- V. Kasturi Rangan
- Amy C. Edmondson
- Daniela Beyersdorfer
- Emer Moloney
- August 26, 2013
Horizontal Specialization and Modularity in the Semiconductor Industry
- Chintay Shih
- Chen-Fu Chien
- July 17, 2008
Worten Portugal: Becoming a Digital Marketplace
- Antonio Moreno
- Pedro Amorim
- Tonia Labruyere
- June 01, 2022
Intuit, Inc.: From Products to Services in the Information Age
- Chuck Holloway
- May 12, 2000
Haiti Hope: Innovating the Mango Value Chain
- Jean-Francois Harvey
- January 10, 2016

Supply Chain: Tools for Preparing Your Team for the Future
- Harvard Business Review
- Willy C Shih
- Christian Shuh
- Wolfgang Schnellbacher
- Daniel Weise
- December 31, 2023
- Robert D. Austin
- December 19, 2006
Supply Chain Optimization at Hugo Boss (A)
- Ananth Raman
- Nicole DeHoratius
- Zahra Kanji
- April 27, 2009
MedSource Technologies
- Robert S. Huckman
- November 07, 2002
Chai Point: Disrupting Chai
- Shikhar Ghosh
- Ramana Nanda
- Rachna Tahilyani
- September 04, 2017
New Jersey Temple Construction: Social Inequalities and Modern Slavery
- Pardeep Singh Attri
- Vivek Soundararajan
- Hari Bapuji
- September 18, 2022
Time is of the Essence: JP Landgoed Overcoming Challenges in the Citrus Industry
- Alicia Fourie
- Marianne Matthee
- January 18, 2024
adidas Russia/CIS and the Russian Crisis: Retrench or Double Down (B)
- Carlos Cordon
- Benoit Leleux
- Beverley Lennox
- September 14, 2016
Hu-Friedy: Evaluating Transportation Alternatives
- Maciek Nowak
- Thomas Scanlan
- May 01, 2018
- David E. Bell
- Natalie Kindred
- December 21, 2015
EMMA Safety Footwear (A): Designing a Circular Shoe
- Andre Calmon
- Luk Van Wassenhove
- Anne-Marie Carrick
- Anne Nai-Tien Huang
- March 08, 2021
Theo Chocolate: How Far Should Fair Trade Go?
- Andrew Hoffman
- February 27, 2015
Supply Chain Optimization at Hugo Boss (B) - The M-Ratio
Supply chain partners: virginia mason and owens & minor (a) (abridged).
- V.G. Narayanan
- April 14, 2010
H&M in China
- Andrew Inkpen
- Jonas Gamso
- August 31, 2021

Meta Abo Brewery: Creating a Sustainable Value Chain in Ethiopia, Teaching Note
- William E Youngdahl
- December 01, 2016
Popular Topics
Partner center.
- Browse All Articles
- Newsletter Sign-Up
SupplyChainManagement →
No results found in working knowledge.
- Were any results found in one of the other content buckets on the left?
- Try removing some search filters.
- Use different search filters.
Advertisement
Mapping research on healthcare operations and supply chain management: a topic modelling-based literature review
- Original Research
- Published: 31 March 2022
- Volume 315 , pages 29–55, ( 2022 )
Cite this article
- Imran Ali 1 &
- Devika Kannan 2
10k Accesses
31 Citations
Explore all metrics
The literature on healthcare operations and supply chain management has seen unprecedented growth over the past two decades. This paper seeks to advance the body of knowledge on this topic by utilising a topic modelling-based literature review to identify the core topics, examine their dynamic changes, and identify opportunities for further research in the area. Based on an analysis of 571 articles published until 25 January 2022, we identify numerous popular topics of research in the area, including patient waiting time, COVID-19 pandemic, Industry 4.0 technologies, sustainability, risk and resilience, climate change, circular economy, humanitarian logistics, behavioural operations, service-ecosystem, and knowledge management. We reviewed current literature around each topic and offered insights into what aspects of each topic have been studied and what are the recent developments and opportunities for more impactful future research. Doing so, this review help advance the contemporary scholarship on healthcare operations and supply chain management and offers resonant insights for researchers, research students, journal editors, and policymakers in the field.
Similar content being viewed by others
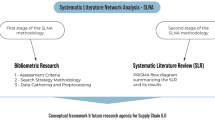
Towards Supply Chain 5.0: Redesigning Supply Chains as Resilient, Sustainable, and Human-Centric Systems in a Post-pandemic World
Alice Villar, Stefania Paladini & Oliver Buckley
Supply Chain “Flows” Management
Humanitarian supply chain management: a thematic literature review and future directions of research.
Abhishek Behl & Pankaj Dutta
Avoid common mistakes on your manuscript.
1 Introduction
Effective and efficient delivery of healthcare services is essential to counteract emergent diseases and promote healthy lives and the well-being of a population, particularly amid the COVID-19 outbreak. Many healthcare systems across the globe strive to efficiently utilise available resources and improve processes through more integrated operations involving all key stakeholders. As such, in the past few decades, research on healthcare has grown exponentially and has become a self-sustained discipline in operations and supply chain management (OSCM). Healthcare OSCM is a network of healthcare providers, pharmaceutical manufacturers, drug suppliers, distributors, and logistics service providers that are involved in the process of acquiring and transforming raw material into finished products, adding value, and facilitating the flow of products and services to the end-customer. With the rapid growth of research on healthcare OSCM, the extant studies have been conducted in various directions and expanded over a dozen of academic journals.
As the field has grown, a corresponding growth in systematic literature reviews in healthcare has occurred, especially in the past six years. For example, Narayana et al. ( 2014 ) reviewed 99 articles (published between 2000 to 2011) on the pharmaceutical supply chain and explore that most studies focus on efficiency improvement with growing interest in process analysis and technology implementation. Volland et al. ( 2017 ) presented a review of 145 articles (published between 1998 and 2014) on material logistics in hospitals and suggested further work on the concepts of supply and procurement, inventory management, and distribution and scheduling. Ali et al. ( 2018 ) conducted a review of 88 articles (published between 2009 and 2018) on cloud computing in hospitals and devised a framework on opportunities, issues, and applications of cloud computing-enabled healthcare systems. Malik et al. ( 2018 ) reviewed 22 articles (published between 2001 and 2015) on the application of data mining in healthcare service delivery and revealed that application of data mining is narrow-focused (workforce scheduling and quality of care), thereby suggesting further research on the broader application of data mining. Moons et al. ( 2019 ) presented a review of 56 articles (published between 2010 and 2016) on logistics performance measurement in the internal hospital supply chain, specifically inventory management and distribution activities in the operation theatre. Diwas Singh et al. ( 2020 ) offered a review of 70 empirical articles (published between 1999 and 2018) from three journals ( Management Science, Manufacturing and Service Operations Management, and Production and Operations Management ) and suggested the need for more empirical research on personalised medicine, value-based healthcare, and digitisation of healthcare. Keskinocak and Savva ( 2020 ) mapped the healthcare research published between 2009 and 2018 from a single journal ( Manufacturing and Service Operations Management ) and suggested the need to develop and apply new OM methods for the improvement of healthcare service.
The existing literature reviews have different scopes and emphases focusing on a specific topic (e.g., material logistics in hospitals, data mining, only empirical papers, etc.). In other words, they are not designed to capture latent topics (hidden topics) that reside in large volumes of scholarly data. Expanding upon some knowledge from the existing reviews, this paper seeks to advance our understanding of the key research topics that have been addressed in healthcare OSCM using a topic modelling-based literature review. Topic modelling with Latent Dirichlet Allocation (LDA) has been found as an effective method to algorithmically and automatically uncover the abstract topics that reside in a large and unstructured collection of articles (Antons et al., 2016 ; Blei, 2012 ; Zhang, 2012 ). LDA topic modelling builds upon rigorous statistical foundations which allows topic generation with little human intervention and/or manual processing (Blei, 2012 ; Zhang, 2012 ). Such an automatic method creates more meaningful and realistic topics and ensures the reliability and validity of outcomes as opposed to manual methods (Griffiths & Steyvers, 2004 ). LDA has been successfully applied for topic modelling in the information science field (Yan, 2014 ), marketing (Tirunillai & Tellis, 2014 ), statistics (De Battisti et al., 2015 ), tourism (Guo et al., 2017 ), decision sciences (Chae & Olson, 2018 ), computer science (Guo et al., 2017 ), and hydropower research (Jiang et al., 2016 ). However, to date, we have only seen a very limited application of topic modelling-base literature in OSCM fields (e.g., Chae & Olson, 2018 ; Lee & Kang, 2018 ), particularly in the healthcare context, to explore the significant topics hidden in a large sample of articles and to locate gaps for further research in each topic. As such, this study aims to: (1) uncover the latent topics residing in the articles published on healthcare OSCM, (2) examine the topic trend/evolution over time including hot, cold, and steady topics, and (3) find opportunities for more impactful research on each topic through a review of the literature around each topic. Driven by these core aims; the following research questions are posed:
What are the emergent topics of research in the field of healthcare OSCM?
How has research on each topic evolved over the past two decades?
What are the opportunities for more impactful future research in the area?
Our study is among the few that offers a topic modelling-based review of literature, which allows us objectively to uncover hidden patterns of research that pervade in a large collection of studies and to explore the development of literature on each topic. The paper advances our understanding of how the literature on different topics in the field of healthcare OSCM has evolved over time and what are the recent trends, knowledge flow, and opportunities for insightful research on each topic. We find an increasing focus and need for further investigation on some emergent topics, such as patient waiting time, COVID-19 pandemic, Industry 4.0 technologies, sustainability, risk and resilience, climate change, circular economy, humanitarian logistics, behavioural operations, service-ecosystem, and knowledge management. In terms of research methods, our analysis shows the increasing interest of researchers in the Markov method, mixed-integer programming, simulation, queueing model and stochastic programming in exploring multidimensional issues of healthcare OSCM. In addition, our analysis suggests that the past three years have also seen some empirical contributions in the area, including structural equation modelling, longitudinal study, and action research; thus, encouraging empirical researchers for more fruitful contributions on the topic.
Our study offers valuable research directions to researchers, journal editors, and policymakers in the field of healthcare OSCM. Researchers can observe whether their recent research topics are hot, cold, or steady, which will allow them to select appropriate topics for more productive forthcoming study in the area. Particularly, some early-career researchers find it difficult to keep up with the growing literature in their fields of research and familiarise themselves with a huge volume of literature in identifying the subject area on which they can focus or apply for research grants. The information from our topic modelling can be a useful tool for these researchers to understand recent developments in the field and then select more appropriate topics. Our findings can also be helpful to PhD students, who often face difficulties in topic selection due to time pressure. Journal editors can view if the extant research is consistent with their editorial policy, and they may even choose to launch a new editorial vision and direction. Our list of hot topics can be a valuable reference tool to launch special issues in the journals. Discovering underlying topics and tracking their evolution could also be of great interest to policymakers (e.g., government agencies) and industry. The information on which research themes and methods are growing or declining in popularity over time (Table 3 and Fig. 4 ) can support governmental funding agencies for grants allocation to promising areas. Likewise, firm managers and the industry as a whole can use this information to formulate promising R&D strategies and more informed investment decisions.
The rest of the paper is structured as follows. Section 2 elaborates on the research methods, covering data collection, screening and analysis procedures. Section 3 outlines results which include word cloud, key term matrix, topic labelling, and evolution of each topic over time. Section 4 provides discussion, while Sect. 5 offers a review of literature and opportunities for further research. The study is concluded in Sect. 6.
As mentioned earlier, this study employs the topic modelling method. The superiority of the topic modelling method has been evidenced in other fields, for example, Latent Semantic Analysis (LSA) (Deerwester et al., 1990 ), Non-Negative Matrix Factorization (NMF) (Lee & Seung, 1999 ), and probabilistic topic modelling. LSA uses singular value decomposition and a traditional matrix factorization technique to reduce the dimensionality of documents (Deerwester et al., 1990 ). Unlike singular value decomposition, in NMF, the transformed and reduced version of documents contains no negative elements (Lee & Seung, 1999 ). Probabilistic topic modelling is a recent development in topic modelling where words and documents are described through probabilistic topics, and a topic is described in terms of a probability distribution over a vocabulary of words (Hofmann, 2001 ; Lee & Kang, 2018 ). Latent Dirichlet Allocation (LDA) is the most popular probabilistic topic modelling algorithm. A comparison of LSA, NMF, and LDA suggests that LDA has the greatest ability to maintain topic coherence and to generate predominant topics that handle the issue of polysemy, the overlapping of words with multiple meanings (Stevens et al., 2012 ). LDA has been successfully applied in the information science field (Yan, 2014 ), marketing (Tirunillai & Tellis, 2014 ), statistics (De Battisti et al., 2015 ), tourism (Guo et al., 2017 ), decision sciences (Chae & Olson, 2018 ), computer science (Guo et al., 2017 ), and hydropower research (Jiang et al., 2016 ). Borrowing knowledge from adjacent areas, this study employs LDA in healthcare. The analysis is completed in four main steps: data collection; text pre-processing; word cloud; and topic modelling.
2.1 Data collection
The analysis aims to uncover the landscape of healthcare research published in good quality international journals in the field of operations and supply chains. The data were collected from Scopus on 25 January 2022, which is one of the largest abstracts and citation databases of academic papers (Ali & Gölgeci, 2019 ; Ferreira, 2018 ; Ali et al., 2021a , 2021b ). To retrieve a broad range of literature on the topic, a large set of keywords was developed based on the authors’ extensive knowledge of the field and review of the extant literature in the field. These keywords included: healthcare, AND operations AND “supply chain” OR hospital OR pharmaceutical OR drug OR medicine AND model OR framework OR Stochastic OR Simulation OR “linear programming” OR Heuristic OR mathematical OR optimization OR “numerical model” OR “nonlinear programming” OR “decision tool” OR “decision analysis” OR “discrete event” OR multi-objective OR multi-objective OR multi-attribute OR multi-criteria OR MCDM OR MCDA OR qualitative OR quantitative OR “case study” OR empirical. Quotation marks were placed on the keywords comprised of more than one word (e.g., “linear programming”) to ensure that search engines consider them as one word.
Initially, we did not limit the timespan because we sought to capture a wide range of publications. The search query generated 663 articles. We excluded the three articles published before 2000 to set the time interval between 2000 and 25 January 2022 (the date of data collection). Next, we carefully read the title and abstract of each article and excluded irrelevant articles. We also excluded articles in non-English languages. Extreme care was taken to include the articles that focus on healthcare OSCM. The inclusion and exclusion criteria resulted in the final set of 571 articles as shown in the supplementary file. Table 1 presents the distribution of these articles.
The articles selected from Scopus were validated using other databases, such as Web of Science, Google Scholar, ProQuest, and EBSCO to ensure that no important articles were missed in the review. The validation process also helped confirm that Scopus contains a greater number of related articles on the topic of healthcare OSCM compared to other databases, so it was used as a preferred database for this research.
2.2 Data pre-processing
Once the articles are collected from Scopus, some pre-processing of data collected is required to conduct further analysis. First, since our search result included the article’s title, keywords and abstract, we merged them as a single document. Second, we removed all punctuation and numbers and transformed all characters into lower-case. Third, we eliminated all “stop-words,” whose main role is to make a sentence grammatically correct, e.g., articles (a, an, the) and prepositions (of, by, from, etc.) and general words that repeatedly appear in most articles, e.g., “and,” “paper”, “find”, “effect”, and “discuss”. These words are not semantically meaningful, yet they create trouble in the analysis process. This step is important because without applying stop-word elimination, our analytical method could result in a big proportion of these unwanted words in the word cloud as well as in the topic matrix.

2.3 Word cloud
Word cloud with R-package is a text mining method to find the most frequently used keywords in sampled articles. A word cloud was designed to allow a visual interpretation of main themes and their trajectories in our review transcripts over the four different periods (2000–2004, 2005–2009, 2010–2014, 2015–2022). As mentioned earlier, we also included articles published until 25 January 2022. Data file for each period was imported into a popular R package, word cloud (Hornik & Grün, 2011 ) with default Dirichlet hyperparameters. The following functions in the R-package were applied to create word clouds: tm for text mining: snowballC for text stemming; Wordcloud2 for generating word cloud images; RColorBrewer for colour palettes.
2.4 Topics modelling
To extract the predominant topics from the 571 sampled articles, LDA’s generative process model was applied (Blei, 2012 ). Figure 1 demonstrates the process and its main variables where η is the parameter of the Dirichlet prior for the per-topic word distribution. K is the defined number of topics, while β k is a distribution of words over a topic. W represents the observable variable—the words in each article. W d,n is the n th word in article d . α is the parameter of the Dirichlet on the per-article topic distribution. θ d is a distribution of topics for the d th article. Z d,n is the topic assignment for the nth word in article d . Some variables are interdependent, that is, W (the observed word) depends on β (the distribution of topics) and Z (the topic assignment for the word), on θ (the topic proportion for the document). This process can be represented by the joint distribution of latent and observed variables through the following Figure and equations:
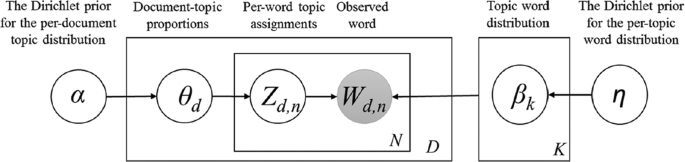
Adapted from Blei ( 2012 )
The graphical representation for LDA.
The generative process aims to explore the topic structure (θ, z , and β), which can explain W . Given the observed variable ( W ), the posterior distribution is estimated with the following equation:
The purpose of Fig. 1 and Eqs. 1 and 2 herein is to demonstrate the analysis process followed by LDA. To perform the analysis objectively and automatically, we used the R-package topic-models along with the tm package (Hornik & Grün, 2011 ). This analytical process produces a document term matrix for the desired number of topics.
3 Analysis and results
This section presents the analysis and the results of data processing, which consist of word cloud, topic modelling, and topic labelling.
3.1 Word cloud
As presented in Fig. 2 , the word clouds show some noticeable changes between 2000 and 2022 in the healthcare literature. In the first period (2000–2004), the popular terms include strategy, logistics, risk, simulation, integer, programming, operations, reengineering, information, inventory, and planning. While a few terms in the first period and second period (2005–2009) are similar (e.g., reengineering, outsourcing, supply, chains, healthcare, strategy, logistics), several new terms became popular in the second period which includes sustainability, warehousing, reverse, heuristic, stochastics, empirical, knowledge, humanitarian, among others.
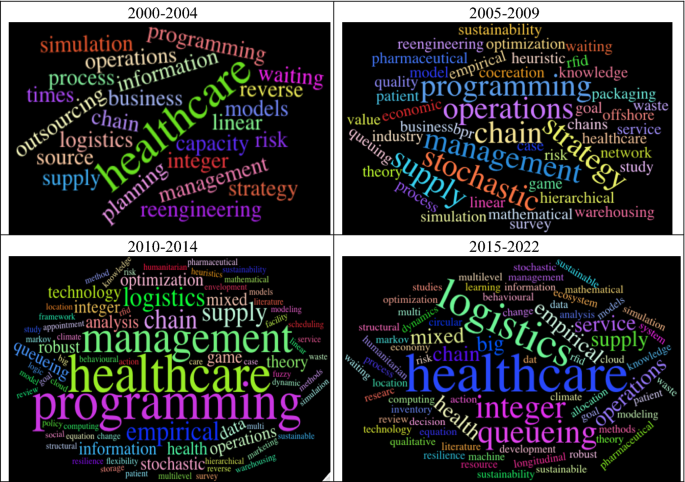
Word cloud demonstrating changing pattern of terms over time (2000–2022)
The third period (2010–2014) shows some important changes, where several new terms (e.g., behavioural, literature, reviews, multilevel, action, structural, equation, Markov) emerged, and other terms (e.g., reengineering, outsourcing, strategy) disappeared. Some other terms remain similar to the second period (e.g., sustainability, warehousing, reverse, heuristic, stochastics, empirical, knowledge, humanitarian, waiting time).
While many terms of the third period continued to appear in the fourth period (2015–2022), several new terms are generated: circular, economy, climate, change, longitudinal. Terms such as warehousing, marketing, strategy disappeared in this period. Understandably, the terms “healthcare”, “operations”, “supply”, “chain” appeared consistently across the four periods.
3.2 Topic modelling
While the visualisation of word clouds (observed words) provides a broad picture of the research dynamics in the literature motivating towards further analysis, it does not provide quantitative information on the relationship between different terms that are generated. Without having quantitative information on each key term, it would be difficult to merge the related terms and assign them into specific topics.
To derive the quantitative information on the nexus between different terms, we used LDA topic modelling that explores the hidden or latent structure and generates different topics by merging interrelated terms from the data set. Generating an appropriate number of topics ( K value) from the given sample of articles is always challenging (Roberts et al., 2014 ). Selecting too few topics would not provide a full and meaningful understanding of the field, while selecting too many topics often results in meaningless outcomes. Therefore, to select the appropriate number of topics, two measures were undertaken: (1) a different number of topics (K value) were tried and accuracies of outcome were checked for each set of topics, and (2) brainstorming among researchers was conducted to ensure topic quality and relatedness to the context of the study (Mimno & McCallum, 2012 ; Silge & Robinson, 2016 ). As a result, 34 relevant topics were produced using LDA with R-package (Fig. 3 ). For each topic, the top five terms were selected, where each term represents a distribution weight (per-topic term probability).
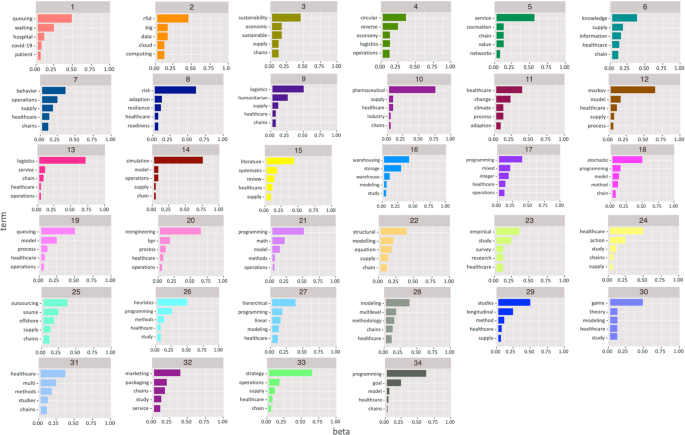
Topic matrix generated in R-package using LDA principle
3.3 Topic labelling
We conducted topic labelling based on the ‘LDA topic matrix’ that produced 34 topics (see Fig. 3 ). Each topic constitutes five terms with different distribution weights. Since the term “healthcare,” “operations,” “supply,” and “chain” appeared in most topics, we decided not to use any of them in labelling the topics. Further, while topic labelling in Table 2 , we used the numbering/sequence of topics exactly following the sequence of topics in the ‘LDA topic matrix’ (Fig. 3 ). To briefly illustrate the process of topic labelling, we discuss a few examples of topic labelling. From Fig. 3 , the top terms in Topic#1 are queuing and waiting, followed by hospital, patient, process; therefore, the topic is labelled the “patient’s waiting time”. Topic#2 includes top terms, such as RFID, big, data followed cloud, computing; therefore, we label the topic as “Industry 4.0”. Industry 4.0 refers to the fourth industrial revolution with the growing use of autonomous technologies such as cloud computing, big data analytics, internet supported RFID and others. Topic# 3 encompasses sustainability, economic, sustainable; hence, it is labelled as sustainability.
Likewise, Topic#8 is labelled “risk and resilience” since the top terms are risk, adaption, resilience supported by healthcare and readiness. Topic#18 is labelled “Stochastic programming” because its top terms are stochastics, programming, followed by model, method, and chain. We adopted a similar process for labelling all 34 topics as demonstrated in Table 2 .
3.4 Topic dynamic/evolution over time
In this section, we introduce the hot, cold, and steady topics (research themes and methods) to provide researchers with an idea of where trends are going (hot) and not going (cold). Exploring the dynamic changes in different topics over time can offer directions for more impactful research on healthcare. We identified three patterns of topic evolution including hot, cold, and steady. For this purpose, we employed a bivariate correlation between the frequency of keywords and time period using four different time periods, namely 2000–2004; 2005–2009; 2010–2014; 2015–2022 (Fig. 3 ). Scopus database was used to collect the information on the frequency of keywords (for each topic shown in Fig. 3 and Table 2 ) in each time interval.
Hot, cold, and steady topics (which include ‘research themes’ and ‘research methods’ as shown in Table 3 ) were identified based on the direction of the trendline on each topic and the value of slope (Fig. 4 ).
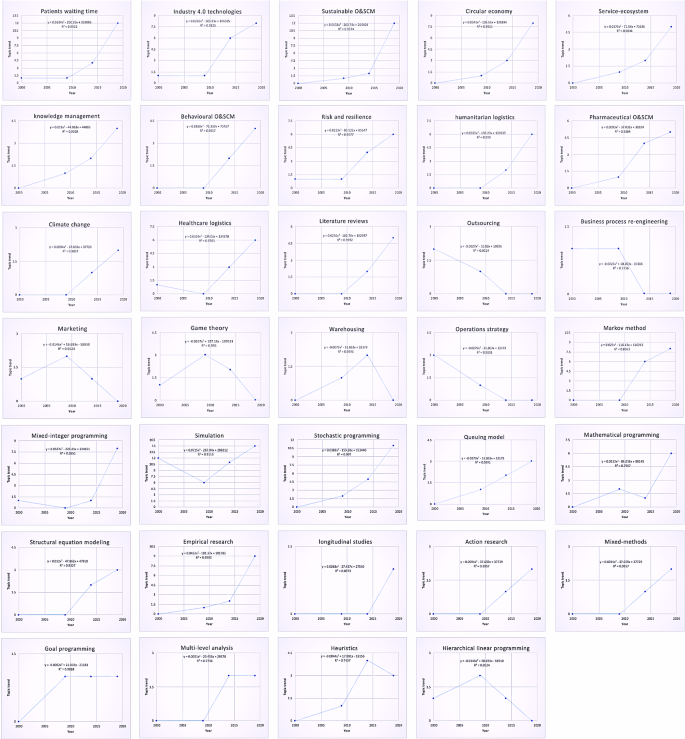
Topic trend/dynamic over time
Hot topics show a trendline moving upward and a positive slope (x-value). Examples of the hot topics related to research themes include the Patient’s waiting time, Industry 4.0 technologies, Sustainability, Circular economy, Behavioural OSCM (OSCM), Risk and resilience, Climate change, and research methods that constitute Markov method, Simulation, Stochastic programming, among others.
The number of contributions on these topics (themes and methods) increased almost continuously from 2010 to 2014, with a dramatic increase on some topics (e.g., patient waiting time, sustainability, circular economy, etc.) between 2015 and 2022.
Cold topics, on the other hand, imply the opposite pattern to these hot topics; they show declining trendline and negative slope values, perhaps due to the relative lack of interest of researchers in recent times.
Examples of cold topics associated with research themes include warehousing, marketing, outsourcing, business process reengineering, strategy, operation strategy, and research methods of heuristics and game theory model among others. These topics enjoyed significant attention between 2000 and 2015, but their proportions have been steadily decreasing over the past five years (Fig. 4 ).
Finally, steady topics show a rise in the beginning but there is no rise or fall in trendlines over the past 5 or more years; perhaps, their importance has not been increased over time.
Overall, two topics depicted a steady growth over the past 5 or more years including goal programming and multi-level analysis. As can be seen in Fig. 4 , the trendline for goal programming modelling increased up to 2009, while no remarkable increase was observed since then. Likewise, the trendline for multi-level analysis shows an upward trend until 2014; however, no growth has been demonstrated since then.
While not investigated in this study, it can be speculated that these topics may lose the interest of research and likely become a cold topic in future. Drawing upon trendline and slope for each topic in Fig. 4 , we have presented all 34 topics under three main categories: hot, cold, and steady (Table 3 ). For better clarity of expression, we have segregated the topics into themes and methods. While both themes and methods are both important, our focus is on research themes in this analysis and discussion.
4 Discussion
Over the last two decades, research on healthcare OSCM has seen spectacular growth. In light of the burgeoning literature on the topic, this study was motivated to explore recent developments, trends, and core topics of research in the area. The study was driven by three key research questions. Addressing the first research question, we explored 34 core topics on which extant research on the topic has been conducted over the past decades. For the second research question, our topic modelling analysis has resulted in the identification of hot, cold, and steady topics in empirical research healthcare OSCM (Table 3 and Fig. 4 ). Thirteen topics of research themes show an upward trend, and nine of them (Patient waiting time, Industry 4.0, Sustainable OSCM, Behavioural OSCM, Risk and resilience, Humanitarian logistics, Climate change, Healthcare logistics, and Literature reviews) start with a relatively flat value (close to 0) before showing a rising trend from around 2010. The other four topics (Circular economy, Service ecosystem, Knowledge management, and Pharmaceutical OSCM) show a gradual upward trend from the early 2000s. Five research topics belong to cold topics. Outsourcing and operations strategy shows an almost linear declining trend, while both Marketing and Warehousing show a bell-shaped trend whose popularity rose in the early 2000s but fell after 2010 and 2015, respectively. Business process reengineering was a popular topic during the early 2000s but, since 2010, shows a declining trend. It is important to note, however, that being identified as a cold topic does not suggest that the topics are not important and should be abandoned. The results provide more of an indication about the interest (trends) of researchers’ interests rather than the importance of the topics themselves. It is also interesting to see that most of the changes of the trends (either upward or downward) of the topics took place around 2010.
Finally, in response to the third research question, we offered a review of the literature and identified numerous opportunities for further research (Sect. 5). Our findings show a shift of current research from traditional topics of operations management (Business process reengineering, Marketing, Outsourcing, Heuristics) to emergent topics such as Patient waiting time, Industry 4.0 technologies, Sustainable operations, Risk and resilience, Climate change, and Circular economy. In general, the shift in topics seems to show a move away from traditional, efficiency-focused research toward broader goals of superior customer service and system’s effectiveness. While each of the three groups of topics deserves discussion, in this section, we discuss the hot research topics as well as provide recommendations of avenues for fruitful future research. It should be noted that although the topic “literature reviews” was a hot topic, we do not include it in our discussion below as the extant research related to it has already been discussed in the introduction section.
5 Literature review and opportunities for further research
The following section provides a brief synthesis of current literature around emergent research topics that are recovered through topic modelling, and it offers numerous opportunities for further research in the field.
5.1 Patients’ waiting time and COVID-19
This research topic covers two interrelated and emerging issues: patient waiting time and COVID-19 outbreak. With a rapidly growing population of aged people, healthcare systems have been facing pressure for more health services resulting in patients’ long waiting times. Therefore, determining methods to ameliorate waiting time has been high on the agenda of most developing and developed economies. Correspondingly, in recent years, mostly building upon simulation and Markov as the primary method of research, considerable studies appeared around the issues of patients’ waiting time, such as patient’s admission process (Chae, 2019 ; Vissers et al., 2007 ); surgery procedures (VanBerkel & Blake, 2007 ); patient inflow and outflow in the emergency department (Abo-Hamad & Arisha, 2013 ); no-shows of patients and overbooking (Topuz et al., 2018 ); outpatient appointment scheduling (Shehadeh et al., 2019 ); time overestimation for the emergency procedure (Gartner & Padman, 2019 ); walk-in patients treatment (Pan et al., 2019 ); patient readmission and discharge planning model (Gu et al., 2019 ); resource planning model to reduce waiting time of patients (Hejazi, 2021 ); load smoothing of scheduled admission to the reduced number of beds required and probability of delay (Asgari & Asgari, 2021 ), and modelled association between length of appointment interval and no-shows of patients (Pan et al., 2021 ).
Overall, although the existing studies have captured several important issues related to patient waiting time, we identify the need for further research on this significant topic. For example, there is potential to develop an integrated model investigating how multiple factors such as general service time, equipment breakdown, high attrition of hospital staff, variability in patient’s arrival time, and unpunctuality of already booked patients cumulatively contribute to patients’ waiting time. Further research can also devise a model at the intersection of patients’ waiting list management, operations theatre scheduling, and patient waiting time for surgery. While the literature on simulation and mathematical modelling continues to grow, there is also an opportunity to further expand empirical research for testing causal links between various factors and their combined effect on patient waiting time. For example, empirical research can test links among customers’ heterogeneous behaviour and their service time in scheduling appointments. Further, a unified model capturing non-value-added practices at both micro and meso levels would be fruitful future research in reducing patient waiting time. While research on bedding and staffing is growing, there is a need to optimise bed and staff requirements amid the global pandemic. Another potential research area is to examine the longitudinal effect of hospital staff’s training on patients’ waiting time.
Likewise, given the outbreak of COVID-19, the recent literature of healthcare OSCM has been rapidly growing on the topic. Pamucar et al. ( 2022 ) proposed a model for supplier selection amid COVID-19. Fan and Xie ( 2022 ) generated an optimization model for COVID-19 testing facility design and planning. Ghaderi ( 2022 ) offered a framework for public health intervention in the wake of the pandemic. Govindan et al. ( 2020 ) developed a decision support system and fuzzy interface system to help with demand management in the healthcare supply chain amid the COVID-19 crises. Nagurney ( 2021a ) modelled labour shortage due to COVID-19, resulting in reduced food harvest and supply. Tavana et al. ( 2021 ) presented a mixed-integer programming model for the equitable distribution of COVID-19 vaccine in developing countries. Salarpour and Nagurney ( 2021 ) constructed a stochastic model to study competition among different countries for medical supplies amid COVID-19 crises. Nagurney ( 2021b ) offered a supply chain network optimization model to manage labour in electronic commerce during the COVID-19 pandemic. Thakur ( 2021 ) developed a model to manage hospital waste during the COVID-19 outbreak. Choi ( 2021 ) used a sense-and-response specific OR model to demonstrate the specific actions needed to deal with the COVID-19 outbreak.
To sum up, while the past research continues to grow on COVID, it would be interesting to explore how to extenuate potential risks and develop shockproof healthcare systems to deal with unanticipated outbreaks such as COVID-19 and similar future incidents. In this regard, it is worth exploring how such a topic as Industry 4.0 technologies could be applied across the healthcare system to capture data from wide sources and help the decision-making process in the event of a pandemic, including contact tracing, the identification of the outbreak location. Some potential areas of research amid COVID-19 include but are not limited to patient inflow and outflow in the emergency department; outpatient appointment scheduling model; models on time estimation for the emergency procedure; walk-in patients’ treatment model; patient admission and discharge planning model; resource planning model to reduce waiting time of patients; load smoothing of scheduled admission to the reduced number of beds required and probability of delay. Besides, pharmaceutical SCM would be the ultimate hope for the recovery and possibly eradication of the virus that allows us to go back to normal life. It would be insightful to examine how pharmaceutical supply chains were affected by COVID-19 and develop a recovery model dealing with such a situation. In particular, if/when a pharmaceutical solution to COVID-19 emerges, research is needed to help understand the best ways to rapidly scale up and distribute the materials on a scale that has never been seen before. It is also of interest to study whether synergy in logistics could be achieved between the public organisations and the growing number of private companies that have entered the healthcare business. Future research should continue to incorporate the healthcare and hospital view into operations management and transfer established concepts from other industries into healthcare while accounting for industry specifics. Again, the links between this and COVID-19 are both obvious and critical.
5.2 Industry 4.0 technologies
Industry 4.0 or the fourth industrial revolution refers to fully automated and in-connected technologies such as the Internet of Things (IoT), cloud computing, big data analytics, blockchain, artificial intelligence, among others (Ali & Govindan, 2021 ; Ali et al., 2021a , 2021b ). Industry 4.0 technologies can enhance the level of service in the industry through real-time information sharing, visibility, traceability, agility, and connectivity within a firm’s boundary and across the supply chain (Govindan et al., 2022 ). Given this, growth in research on the role of Industry 4.0 technologies in healthcare service provision is evident, such as Sultan ( 2014 ) developed a conceptual framework on the potential of cloud computing in the advancement of healthcare services. Chong et al. ( 2015 ) modelled variables that influence the adoption of IoT (e.g., RFID) in healthcare. Priya and Ranjith Kumar ( 2015 ) used big data analytics to predict the progression of atherosclerotic disease, the narrowing and hardening of arteries due to the accumulation of plaque on the artery wall. Fan et al. ( 2018 ) recognised the factors affecting the adoption of artificial intelligence-based medical diagnosis support systems. Kochan et al. ( 2018 ) modelled on how cloud computing improves visibility and responsiveness in hospital SC. Galetsi et al. ( 2020 ) developed a theoretical framework for the realisation of big data analytics.
The research on Industry 4.0 is growing with the advent of new technologies and provides fruitful opportunities for further research. The models on big data should incorporate data of all inter-connected functions in healthcare, instead of just focusing on a single function or silo approach. For example, the impact of Industry 4.0 on the entire healthcare supply chain can be modelled. This is important to understand how interaction with different functions/partners through Industry 4.0 technologies influence healthcare productivity. Most of the current research focuses on big data and cloud computing which needs to be expanded to other Industry 4.0 technologies, such as blockchain, cyber-physical systems and the Internet of Things in healthcare OSCM. There is a lack of a holistic model testing the combined impact of all Industry 4.0 technologies on the healthcare system and an investigation into if there is a trade-off for investing between various digital technologies would be helpful. Future research can also investigate how machine learning techniques impact diagnostic, maintenance, and prognostics systems in healthcare settings. Research highlights the intricate issues of human–machine interaction at the workplace (Arslan et al., 2021 ); we suggest further research on how to maintain trustworthiness and to harmonise productive human–machine interactions and reduce workers’ resistance to automated machines in the healthcare settings. We also highlight the need for further research on the application of artificial intelligence in healthcare OSCM.
5.3 Sustainable operations and supply chain
Today sustainability is a growing concern of most organisations and therefore the topic of sustainability has received increasing focus from researchers from operations management. Healthcare systems worldwide face pressing challenges of service quality and cost issues as well as safe disposal of waste. As such, in recent times considerable attempts have been made to realise sustainability in the healthcare system. Chauhan and Singh ( 2016 ) employed a hybrid model for the selection of sustainable locations to dispose of healthcare waste. Anuar et al. ( 2018 ) offered a conceptual framework demonstrating the link between lean practices and a sustainable healthcare system. Malekpoor et al. ( 2018 ) modelled a sustainable healthcare treatment plan. Thorsen and McGarvey ( 2018 ) investigated the effectiveness of fixed and mobile dentistry for the financial sustainability of the healthcare system. Mousa and Othman ( 2020 ) found a positive impact of green human resource management practices on a sustainable healthcare system.
While research on healthcare sustainability is growing, there is still space for further research. For instance, more studies are needed that simultaneously investigate the impact of all three dimensions (social, environmental, economic) of sustainability through a single model. Prospective studies can also investigate the combined impact of synchronous business activities including green human resource management, green marketing, and green production on healthcare sustainability. There is also an opportunity to develop a sustainable performance measurement and management model in healthcare.
5.4 Circular economy
The circular economy is a recent concept aimed to eliminate waste through the make, use, recycle and reuse approach. It helps to overcome a take-make-dispose linear pattern of production and consumption. The healthcare industry inevitably generates waste that may become hazardous to both public health and the environment. Therefore, the circular economy has recently gained significant attention in healthcare literature. For example, Kumar and Rahman ( 2014 ) suggested RFID supported process reengineering for waste management ensuring circular economy. Jensen et al. ( 2019 ) discussed the healthcare refurbish system as a means to reduce waste. Dehghani et al. ( 2019 ) suggested that transhipment can reduce waste and save substantial costs compared to no-transhipment. Viegas et al. ( 2019 ) identified the need for research on the circularity of end-of-use medicine in the pharmaceutical supply chain.
Although research circular economy has witnessed mounting attention, there is still room for further research. For instance, there is a need to develop a model that could make the circularity of outdated medicine more visible through all stages of reverse flow and re-manufacturing. Also, healthcare literature lacks studies modelling processes and decision-making for circular economy implementation at micro and meso levels. Prospective research can also explore the healthcare staff’s perception of the circular economy. It would be interesting to investigate how healthcare professionals are prepared to deal with the circular economy and how they might contribute to reducing wastage in reverse flow.
5.5 Service ecosystem
With its root in marketing, the service ecosystems concept is defined as “relatively self-contained, self-adjusting systems of resource-integrating actors connected by shared institutional logics and mutual value creation through service exchange” (Vargo & Akaka, 2012 , p. 207). That is, the service ecosystem generates value for all the actors in a network through resource integration, value co-creation, and co-innovation. The service ecosystem concept has received a great deal of attention in healthcare literature. Nudurupati et al. ( 2015 ) elaborate on the process of value co-creation through strategic alliance and collaboration with multi-stakeholders. Albarune et al. ( 2015 ) suggested a value-based supply chain for integrated hospital management. Chae ( 2019 ) suggested digital innovation as a promising source of resource sharing and value creation among actors in an ecosystem. Lee et al. ( 2020 ) showed a positive relationship between the previous year value-based purchasing penalty and the current year care process improvement in the hospital.
Service ecosystem research is a growing area of research in the healthcare context; thus, offering opportunities for further expansion. For example, the influence of contextual behavioural factors in value co-creation and unlocking innovation needs further investigation. Also, service ecosystem research needs to be expanded from the organisational level to the entire healthcare system. For example, how resource integration practice can be integrated into a multitude of value co-creation processes in the healthcare networks. Moreover, the role of advanced technologies and servitisation in the healthcare service ecosystem has yet to be investigated.
5.6 Knowledge management
Knowledge management refers to the process of generating, transferring, and managing knowledge and information in an organisation or network. Knowledge management becomes a major driver of performance and productivity where front-line workers are required to provide customised services tailored to the need of individual clients such as healthcare. As such, the literature has witnessed considerable growth in literature on knowledge management in healthcare settings. Gagnon et al. ( 2016 ) empirically corroborated a positive association between knowledge management on staff practices and quality of healthcare. Mura et al. ( 2016 ) found that knowledge exchange and assets have a positive impact on workers’ innovation, such as idea generation, idea promotion and idea implementation. Avgerinos and Gokpinar ( 2017 ) argued that knowledge transfer capability in a team positively influences healthcare productivity. Hiranrithikorn and Sutduean ( 2019 ) expound that access to information and knowledge has a positive influence on healthcare supply chain skills. Despite considerable contributions to knowledge management, there is still significant room for further research. A few models are investigating the impact of knowledge sharing on the quality and productivity of healthcare performance. Qualitative research can be conducted to explore the barrier and enablers of knowledge sharing in healthcare. Future research can use multi-method research investigating the relationship between social capital, culture, and knowledge management.
5.7 Behavioural operations
Research on behavioural operations is well-received, particularly from healthcare service perspectives. Healthcare systems have two main stakeholders: staff and patients. The research suggests that the findings of healthcare modelling may not be reliable without considering the behaviours of the main stakeholders (Brailsford et al., 2012 ). For example, a simulation model suggesting the effectiveness of a new drug will be unreliable if it does not consider patient behaviour; some patients may not complete the prescribed course of medication, and potential side effects of medicine should be shown. As such, healthcare literature has seen an emergence of interest in understanding both human behaviour in practice and how to capture it in operations research models. Brailsford et al. ( 2012 ) used a simulation model capturing behavioural factors of a patient while treating breast cancer. Based on a review of literature, Kunc et al. ( 2018 ) identified scarcity of research on behavioural aspects of healthcare. Harper ( 2019 ) used a queuing model to assess how healthcare workers behave and treat patients, especially when such professionals encounter changing workloads, service queues, and other factors that impact service quality.
To sum, behavioural operational research has become an important research topic in healthcare but significant room for further research in the area remains intact. Specifically, we suggest more research with detailed models on the nexus between behavioural interventions on healthcare practice. Future research can model workforce adaptive behaviours and productivity. We agree that the incorporation of behavioural factors is important to increase the reliability of the healthcare model because of the increasing influence of human behaviours in healthcare operations. Future research can also examine the behavioural consequences of inter-organisational interaction in complex healthcare systems.
5.8 Risk and resilience
Healthcare systems are susceptible to operational failures in services offered to patients when a shortage of medicine and equipment occurs, or when the execution of such systems is incorrectly managed. Given the divergent risks emerging from a variety of sources, there is an increasing focus on risk mitigation and the development of resilient healthcare. Nemeth et al. ( 2011 ) developed a model for healthcare crises management and resilience. Mahjoub et al. ( 2014 ) found risk mitigation in healthcare through a risk-sharing agreement between pharmaceutical companies and healthcare service providers. Saedi et al. ( 2016 ) suggested an optimal inventory policy to reduce drug shortage risks. Rubbio et al. ( 2019 ) examined the role of digital technologies in developing healthcare resilience. Rahimian et al. ( 2019 ) investigated an appropriate level of risk-aversion through robust optimization. Zhang et al. ( 2020 ) formulated a model which helps to reduce overtime risk during surgery in a hospital.
While research on risk and resilience in healthcare is growing, there is still substantial room for more impactful future research. Most models investigate risk and resilience in isolation. Others focus on particular functions such as hospital surgery. As such, a quantitative model that simultaneously investigates risk and resilience focusing on the key supply chain actors in healthcare is lacking. Future research can also use a mixed-methods approach where qualitative research can explore various risk factors and resilience strategies, while quantitative research can test the interrelationship between risk and resilience in explaining healthcare performance.
5.9 Humanitarian logistics
Humanitarian logistics refers to the transportation, delivery, and distribution of supplies in the event of natural disasters or another emergency to the affected area and people. Given the unprecedented incidents such as floods, cyclones, disease outbursts, the humanitarian logistics in healthcare (e.g., supplies of medicines, medical equipment, sterile items, linen, and food) has gained significant attention in recent times. For example, Naor et al. ( 2018 ) found strategies for effective humanitarian logistics in healthcare, such as quickly sending a team to the disaster area to gather firsthand information on the unique situations of disaster followed by the main delegation staff carrying effective disaster relief service. Prasad et al. ( 2018 ) suggested effective healthcare intervention to deal with disease outbreaks in a community. Cherkesly et al. ( 2019 ) assist with the design of a community healthcare network, increasing the service coverage for underserved areas in adverse situations.
Although research on humanitarian logistics has been progressing steadily, we highlight the need for further research on healthcare OSCM. A fruitful future research avenue is modelling the role of volunteers and NGOs in humanitarian logistics from a healthcare perspective. Also, interviews with disaster area patients can provide additional insights into the real-world phenomenon. Further research can also develop a model of budget allocation as the community service demand increases drastically due to a natural disaster. It would be interesting to investigate the nexus among service compliance rate, disease rate, and total cost by disaster type.
5.10 Pharmaceutical OSCM
A pharmaceutical supply chain involves firms, processes, and operations involved in the design, development, and distribution of life-saving drugs. It is one of the critical supply chains since it is related to the life and health of people. The success of healthcare services directly depends on well-managed pharmaceutical supplies. Therefore, the management of the pharmaceutical supply chain has become a popular topic in healthcare literature. Jia and Zhao ( 2017 ) highlighted the issue of drug shortage due to lack of collaboration between pharmacies and pharmaceutical manufacturing and supplier. Nematollahi et al. ( 2018 ) also found a positive link between hospital service level and efficient pharmaceutical supply chain. Viegas et al. ( 2019 ) discussed the significance of a coordinated pharmaceutical supply chain for the reverse flow of end-of-life medicine. Recently, Zandkarimkhani et al. ( 2020 ) formulated a model to redesign the pharmaceutical supply chain network in the wake of demand uncertainty.
Despite growth in literature on the pharmaceutical supply chain, significant room for further research still exists, particularly in the context of a recent pandemic. Most of the existing research lacks a holistic view that involves all key stakeholders of the supply chain. There is less focus on upstream networks and processes (Narayana et al., 2014 ). There is also limited research on integrated new product development in the pharmaceutical supply chain. There is also an opportunity for research to model examining the link between healthcare financing and pharmaceutical supply chain financing such as investment in R&D, production and distribution, between waste management, environmental and pharmaceutical supply chains. Future studies can also model the behaviour of individuals in the robustness of pharmaceutical supply chains. Given that supply chain design is critical in the planning process (Govindan et al., 2017 ), we suggest designing integrated models that encompass all stages from drug manufacturing to final consumer for robust decision-making in the pharmaceutical supply chain. Another interesting avenue is formulating a model at the intersection of supplier selection and sustainable pharmaceutical supply chain networks.
5.11 Climate change and healthcare
The increased intensity and frequency of disasters due to extreme weather conditions draw our attention to the unprecedented changes in climate which are mainly attributed to excessive greenhouse gas emissions and carbon footprints. To deal with the climate change challenges, greenhouse gas mitigation targets were set to a minimum level in the historic Paris Agreement (Bodansky, 2016 ). While a lot of research spotlights the emissions reduction in the automotive, energy, and mining industries, the carbon footprint and climate change research has recently received attention. Rezali et al. ( 2018 ) discussed the importance of a green healthcare supply chain to reduce the environmental impact of the healthcare industry. Belkhir and Elmeligi ( 2019 ) examined 15 pharmaceutical supply chains across different countries and found that the pharmaceutical industry is more emission-intensive than the automotive industry. Tanwar et al. ( 2019 ) identify the potential risks and risk mitigation measures in implementing a green pharmaceutical supply chain. Over the past five years, the topic of climate change has received increasing attention in healthcare settings. Most studies on the topic focus on factors contributing to climate change (greenhouse gas emissions, carbon footprint), thus leaving a gap for research models incorporating the business impact of climate risks when they unfold and examining specific risk mitigation measures.
5.12 Healthcare logistics
Healthcare logistics refer to the physical flow of healthcare materials from the pharmaceutical production facility to the healthcare service provider. Logistics cost has been identified as the second largest cost after personnel in the healthcare system (Ross & Jayaraman, 2009 ). As such, the importance of material flow in healthcare service is recognised important to add value and reduce the cost of services. Correspondingly, a growth in literature on healthcare logistics is evident. Oumlil and Williams ( 2011 ) suggested strategic alliance and consolidation of logistics in the healthcare sector as a strategy to reduced logistics costs. Volland et al. ( 2017 ) identified four main domains in which healthcare logistics research exists, including procurement, inventory management, distribution, and supply chain management. Al-Sharhan et al. ( 2019 ) expounded on the significance of hospital-supplier integration of inefficient material logistics. Fathollahi-Fard et al. ( 2019 ) developed a green home healthcare supply chain model to reduce emissions in logistics. Pohjosenperä et al. ( 2019 ) discussed the impact of service modularity in value-creating and improving healthcare logistics service. Zabinsky et al. ( 2020 ) developed a model through mixed-integer linear programming to address healthcare scheduling and routing problem. Further research could examine an item-level analysis of different product categories in the regional healthcare units, together with their replenishment models. Such a study is needed to show the true potential of modularity in service offerings of the logistics support organisation.
6 Conclusion
Over the last two decades, research on healthcare OSCM has been continuously advancing making it a self-sustained discipline in the OSCM field. We argue that with this unprecedented development in healthcare literature, the current status and trends in the field need to be mapped. The use of robust statistical and automatic methods for extracting key topics from a large set of articles has been increasingly used in other fields such as finance, marketing, statistics, tourism, and computer sciences. Borrowing the knowledge from these adjacent fields, this study employs probabilistic topic modelling with LDA as an automatic text analytics approach to unpack dominant topics and development trajectories in a large collection of research articles on healthcare OSCM. In doing so, we contribute to the literature on new and rigorous methods for systematic literature review in the field of OSCM. Our sample included 571 articles retrieved from 20 journals in the field of OSCM. The results produced 34 topics on healthcare research. Analysis of dynamic changes in the 34 over the past twenty years suggested 24 hot, 8 cold, and 2 steady topics (see Table 3 ).
Our findings show a shift of current research from traditional topics of operations management (Business process reengineering, Marketing, Outsourcing, Heuristics) to emergent topics such as Patient waiting for time, Industry 4.0 technologies, Sustainable operations, Risk and resilience, Climate change, and Circular economy. In general, the shift in topics seems to show a move away from traditional, efficiency-focused research toward broader goals of superior customer service and system’s effectiveness. The data on research methods shows a larger dominance of modelling research (e.g., Markov, mixed-integer programming, simulation, queueing model, and stochastic) in exploring multidimensional issues of healthcare operations. However, the past three years have also seen some empirical research, including Structural equation modelling, Longitudinal study, and Action research. This shows a positive change in operations research journals from purely quantitative modelling studies to empirical research as well. This recent shift in a strategy not only offers a great opportunity to empirical researchers for publication in some OR journals but also provides sound premises on which to expand the scope and subscription of these journals.
The findings of this research offer useful research directions to researchers, journal editors, and policymakers in the field of healthcare research. Researchers can observe whether their recent research topics are hot, cold, or steady, and accordingly select appropriate topics for more impactful future research in the area. Particularly, some early-career researchers find it difficult to keep up with the growing literature in their fields of research and familiarise themselves with a huge volume of literature in identifying the subject area on which they can focus or apply for a research grant. The information from our topic modelling can be a useful tool for these researchers to understand recent developments in the field and then select more appropriate topics. Our findings can also be helpful to PhD students, who often face difficulties in topic selection due to time pressure. Journal editors can view if the extant research is consistent with their editorial policy, and they may like to launch a new editorial vision and direction. Our list of hot topics can be a valuable reference tool to launch special issues in the journals. Discovering underlying topics and tracking their evolution could also be of great interest to policymakers (e.g., government agencies) and industry. The information on which research themes and methods are growing or declining in popularity over time (Table 3 and Fig. 4 ) can support governmental funding agencies for grants allocation to promising areas. Likewise, firm managers and the industry as a whole can use this information to formulate promising R&D strategies and to make more informed investment decisions.
We believe that topic modelling is one of the most effective and reliable approaches in revealing the latent (hidden) structure and development of research topics in a field, and recommend that future research can combine the LDA-based models with other text analytics techniques from different text data (e.g., customer feedback, medical records, research reports, business reviews, company descriptions) and interconnection among various business areas (e.g., marketing, supply chain, information systems). For example, sentiment analysis (Pang & Lee, 2008 ) is deemed a popular text analytics tool in the field of finance (Sul et al., 2017 ), marketing (Liang et al., 2015 ), and operations/supply chain (Chae, 2015 ). The sentiment analysis can be integrated with topic modelling: a two-pronged method using topic modelling followed by sentiment analysis can offer more nuanced findings by revealing both positive and negative aspects of a product and/or service.
As with several studies, there are some limitations of our study. First, given the authors’ extensive knowledge of the field and review of the extant literature, full efforts were made to guarantee that all pertinent articles were included in the analysis. However, some articles might have been ignored. Nevertheless, two measures might help mitigate this limitation: (1) the topics are generated based on the frequencies of the keywords/key terms and, therefore, missing a few articles from such a large dataset (571 articles) will not have a significant impact on overall results; (2) the dataset is validated with other databases. Second, the research is limited to healthcare research published in OSCM journals. That is, we excluded the papers and related journals containing healthcare research but not from OSCM perspectives.
Abo-Hamad, W., & Arisha, A. (2013). Simulation-based framework to improve patient experience in an emergency department. European Journal of Operational Research, 224 (1), 154–166.
Article Google Scholar
Albarune, A. R. B., Farhat, N., & Afzal, F. (2015). The valued supply chain for integrated hospital management: A conceptual framework. International Journal of Supply Chain Management, 4 (3), 39–49.
Google Scholar
Ali, I. (2019). The impact of industry 4.0 on the nexus between supply chain risks and firm performance. In A. Guclu (Ed. 2019) Academy of Management Proceedings (1–6). Boston: Academy of Management.
Ali, I., Arslan, A., Khan, Z., & Tarba, S. Y. (2021a). The role of industry 4.0 technologies in mitigating supply chain disruption: Empirical evidence from the Australian Food Processing Industry. IEEE Transactions on Engineering Management, 1–11. https://doi.org/10.1109/TEM.2021.3088518
Ali, I., & Aboelmaged, M. G. S. (2021). Implementation of supply chain 4.0 in the food and beverage industry: Perceived drivers and barriers. International Journal of Productivity and Performance Management , ahead-of-print (ahead-of-print). https://doi.org/10.1108/IJPPM-07-2020-0393
Ali, I., & Gölgeci, I. (2019). Where is supply chain resilience research heading? A systematic and co-occurrence analysis. International Journal of Physical Distribution and Logistics Management, 49 (8), 793–815.
Ali, I., & Govindan, K. (2021). Extenuating operational risks through digital transformation of agri-food supply chains. Production Planning & Control . https://doi.org/10.1080/09537287.2021.1988177
Ali, I., Sultan, P., & Aboelmaged, M. (2021b). A bibliometric analysis of academic misconduct research in higher education: Current status and future research opportunities. Accountability in Research, 28 (6), 372–393.
Ali, O., Shrestha, A., Soar, J., & Wamba, S. F. (2018). Cloud computing-enabled healthcare opportunities, issues, and applications: A systematic review. International Journal of Information Management, 43 (6), 146–158.
Al-Sharhan, S., Omran, E., & Lari, K. (2019). An integrated holistic model for an eHealth system: A national implementation approach and a new cloud-based security model. International Journal of Information Management, 47 (1), 121–130.
Antons, D., Kleer, R., & Salge, T. O. (2016). Mapping the topic landscape of JPIM, 1984–2013: In search of hidden structures and development trajectories. Journal of Product Innovation Management, 33 (6), 726–749.
Anuar, A., Saad, R., & Yusoff, R. Z. (2018). Sustainability through lean healthcare and operational performance in the private hospitals: A proposed framework. Supply Chain Management, 7 (5), 221–227.
Arslan, A., Cooper, C., Khan, Z., Golgeci, I., & Ali, I. (2021). Artificial intelligence and human workers interaction at the team level: a conceptual assessment of the challenges and potential HRM strategies. International Journal of Manpower, ahead-of-print (ahead-of-print). https://doi.org/10.1108/IJM-01-2021-0052
Asgari, F., & Asgari, S. (2021). Addressing artificial variability in patient flow. Operations Research for Health Care, 28 , 100288. https://doi.org/10.1016/j.orhc.2021.100288
Avgerinos, E., & Gokpinar, B. (2017). Team familiarity and productivity in cardiac surgery operations: The effect of dispersion, bottlenecks, and task complexity. Manufacturing & Service Operations Management, 19 (1), 19–35.
Belkhir, L., & Elmeligi, A. (2019). Carbon footprint of the global pharmaceutical industry and relative impact of its major players. Journal of Cleaner Production, 214 (1), 185–194.
Blei, D. M. (2012). Probabilistic topic models. Communications of the ACM, 55 (4), 77–84.
Bodansky, D. (2016). The Paris climate change agreement: A new hope? American Journal of International Law, 110 (2), 288–319.
Brailsford, S. C., Harper, P. R., & Sykes, J. (2012). Incorporating human behaviour in simulation models of screening for breast cancer. European Journal of Operational Research, 219 (3), 491–507.
Chae, B., & Olson, D. (2018). A topical exploration of the intellectual development of decision sciences 1975–2016. Decision Sciences, Published (online) , 1–24.
Chae, B. K. (2015). Insights from hashtag# supplychain and Twitter Analytics: Considering Twitter and Twitter data for supply chain practice and research. International Journal of Production Economics, 165 (3), 247–259.
Chae, B. K. (2019). A General framework for studying the evolution of the digital innovation ecosystem: The case of big data. International Journal of Information Management, 45 (2), 83–94.
Chauhan, A., & Singh, A. (2016). A hybrid multi-criteria decision making method approach for selecting a sustainable location of healthcare waste disposal facility. Journal of Cleaner Production, 139 (4), 1001–1010.
Cherkesly, M., Rancourt, M. È., & Smilowitz, K. R. (2019). Community healthcare network in underserved areas: Design, mathematical models, and analysis. Production and Operations Management, 28 (7), 1716–1734.
Choi, T.-M. (2021). Fighting against COVID-19: What operations research can help and the sense-and-respond framework. Annals of Operations Research . https://doi.org/10.1007/s10479-021-03973-w
Chong, A.Y.-L., Liu, M. J., Luo, J., & Keng-Boon, O. (2015). Predicting RFID adoption in healthcare supply chain from the perspectives of users. International Journal of Production Economics, 159 (5), 66–75.
De Battisti, F., Ferrara, A., & Salini, S. (2015). A decade of research in statistics: A topic model approach. Scientometrics, 103 (2), 413–433.
Deerwester, S., Dumais, S. T., Furnas, G. W., Landauer, T. K., & Harshman, R. (1990). Indexing by latent semantic analysis. Journal of the American Society for Information Science, 41 (6), 391–407.
Dehghani, M., Abbasi, B., & Oliveira, F. (2019). Proactive transshipment in the blood supply chain: A stochastic programming approach. Omega , Published (online), 102–112.
Diwas Singh, K. C., Scholtes, S., & Terwiesch, C. (2020). Empirical research in healthcare operations: Past research, present understanding, and future opportunities. Manufacturing and Service Operations Management, 22 (1), 73–83.
Fan, Z., & Xie, X. (2022). A distributionally robust optimisation for COVID-19 testing facility territory design and capacity planning. International Journal of Production Research, 1–24.
Fan, W., Liu, J., Zhu, S., & Pardalos, P. M. (2018). Investigating the impacting factors for the healthcare professionals to adopt artificial intelligence-based medical diagnosis support system (AIMDSS). Annals of Operations Research . https://doi.org/10.1007/s10479-018-2818-y
Fathollahi-Fard, A. M., Govindan, K., Hajiaghaei-Keshteli, M. and Ahmadi, A. (2019). A green home health care supply chain: New modified simulated annealing algorithms. Journal of Cleaner Production, 240, pp. ahead-of-print.
Fattahi, M., Keyvanshokooh, E., Kannan, D., & Govindan, K. (2022). Resource planning strategies for healthcare systems during a pandemic. European Journal of Operational Research .
Ferreira, F. A., Kannan, D., Meidutė-Kavaliauskienė, I., & Vale, I. M. (2022). A sociotechnical approach to vaccine manufacturer selection as part of a global immunization strategy against epidemics and pandemics. Annals of Operations Research, 1–30.
Ferreira, F. A. (2018). Mapping the field of arts-based management: Bibliographic coupling and co-citation analyses. Journal of Business Research, 85 (2), 348–357.
Gagnon, M.-P., Simonyan, D., Godin, G., Labrecque, M., Ouimet, M., & Rousseau, M. (2016). Factors influencing electronic health record adoption by physicians: A multilevel analysis. International Journal of Information Management, 36 (3), 258–270.
Galetsi, P., Katsaliaki, K., & Kumar, S. (2020). Big data analytics in health sector: Theoretical framework, techniques and prospects. International Journal of Information Management, 50 (4), 206–216.
Gartner, D., & Padman, R. (2019). Machine learning for healthcare behavioural OR: Addressing waiting time perceptions in emergency care. Journal of the Operational Research Society, 71 (7), 1081–1101.
Ghaderi, M. (2022). Public health interventions in the face of pandemics: Network structure, social distancing, and heterogeneity. European Journal of Operational Research, 298 (3), 1016–1031.
Govindan, K., Nasr, A. K., Mostafazadeh, P., & Mina, H. (2021). Medical waste management during coronavirus disease 2019 (COVID-19) outbreak: A mathematical programming model. Computers & Industrial Engineering , 162 , 107668.
Govindan, K., Nasr, A. K., Saeed Heidary, M., Nosrati-Abargooee, S., & Mina, H. (2022). Prioritizing adoption barriers of platforms based on blockchain technology from balanced scorecard perspectives in healthcare industry: A structural approach. International Journal of Production Research, 1–15.
Govindan, K., Fattahi, M., & Keyvanshokooh, E. (2017). Supply chain network design under uncertainty: A comprehensive review and future research directions. European Journal of Operational Research, 263 (1), 108–141.
Govindan, K., Mina, H., & Alavi, B. (2020). A decision support system for demand management in healthcare supply chains considering the epidemic outbreaks: A case study of coronavirus disease 2019 (COVID-19). Transportation Research Part e: Logistics and Transportation Review . https://doi.org/10.1016/j.tre.2020.101967
Griffiths, T. L., & Steyvers, M. (2004). Finding scientific topics. Proceedings of the National Academy of Sciences, 101 (1), 5228–5235.
Gu, W., Fan, N., & Liao, H. (2019). Evaluating readmission rates and discharge planning by analyzing the length-of-stay of patients. Annals of Operations Research, 276 (1–2), 89–108.
Guo, Y., Barnes, S. J., & Jia, Q. (2017). Mining meaning from online ratings and reviews: Tourist satisfaction analysis using latent dirichlet allocation. Tourism Management, 59 (2), 467–483.
Harper, P. R. (2019). Server behaviours in healthcare queueing systems. Journal of the Operational Research Society, 71 (4), 1124–1136.
Hejazi, T.-H. (2021). State-dependent resource reallocation plan for health care systems: A simulation optimization approach. Computers & Industrial Engineering, 159 , 107502. https://doi.org/10.1016/j.cie.2021.107502
Hiranrithikorn, P., & Sutduean, J. (2019). Social capital predicting the supply chain skills: Mediating role of access to information and network resources. International Journal of Supply Chain Management, 8 (5), 842–853.
Hofmann, T. (2001). Unsupervised learning by probabilistic latent semantic analysis. Machine Learning, 42 (1–2), 177–196.
Hornik, K., & Grün, B. (2011). topicmodels: An R package for fitting topic models. Journal of Statistical Software, 40 (13), 1–30.
Jensen, J. P., Prendeville, S. M., Bocken, N. M., & Peck, D. (2019). Creating sustainable value through remanufacturing: Three industry cases. Journal of Cleaner Production, 218 (4), 304–314.
Jia, J., & Zhao, H. (2017). Mitigating the US drug shortages through pareto-improving contracts. Production and Operations Management, 26 (8), 1463–1480.
Jiang, H., Qiang, M., & Lin, P. (2016). A topic modeling based bibliometric exploration of hydropower research. Renewable and Sustainable Energy Reviews, 57 (3), 226–237.
Keskinocak, P., & Savva, N. (2020). A review of the healthcare-management (Modeling) literature published in manufacturing & service operations management. Manufacturing & Service Operations Management, 22 (1), 59–72.
Kochan, C. G., Nowicki, D. R., Sauser, B., & Randall, W. S. (2018). Impact of cloud-based information sharing on hospital supply chain performance: A system dynamics framework. International Journal of Production Economics, 195 (5), 168–185.
Kumar, A., & Rahman, S. (2014). RFID-enabled process reengineering of closed-loop supply chains in the healthcare industry of Singapore. Journal of Cleaner Production, 85 (2), 382–394.
Kunc, M., Harper, P., & Katsikopoulos, K. (2018). A review of implementation of behavioural aspects in the application of OR in healthcare. Journal of the Operational Research Society, 71 (7), 1055–1072.
Lee, D. D., & Seung, H. S. (1999). Learning the parts of objects by non-negative matrix factorization. Nature, 401 (6755), 788–791.
Lee, H., & Kang, P. (2018). Identifying core topics in technology and innovation management studies: A topic model approach. The Journal of Technology Transfer, 43 (5), 1291–1317.
Lee, S. J., Venkataraman, S., Heim, G. R., Roth, A. V., & Chilingerian, J. (2020). Impact of the value-based purchasing program on hospital operations outcomes: An econometric analysis. Journal of Operations Management, 66 (1–2), 151–175.
Liang, T.-P., Li, X., Yang, C.-T., & Wang, M. (2015). What in consumer reviews affects the sales of mobile apps: A multifacet sentiment analysis approach. International Journal of Electronic Commerce, 20 (2), 236–260.
Mahjoub, R., Odegaard, F., & Zaric, G. S. (2014). Health-based pharmaceutical pay-for-performance risk-sharing agreements. Journal of the Operational Research Society, 65 (4), 588–604.
Malekpoor, H., Mishra, N., & Kumar, S. (2018). A novel TOPSIS–CBR goal programming approach to sustainable healthcare treatment. Annals of Operations Research, 1–23.
Malik, M. M., Abdallah, S., & Ala’raj, M. (2018). Data mining and predictive analytics applications for the delivery of healthcare services: A systematic literature review. Annals of Operations Research, 270 (1–2), 287–312.
Mimno, D., & McCallum, A. (2012). Topic models conditioned on arbitrary features with Dirichlet-multinomial regression. UAI, 24 , 1–8.
Moons, K., Waeyenbergh, G., & Pintelon, L. (2019). Measuring the logistics performance of internal hospital supply chains—a literature study. Omega (united Kingdom), 82 (3), 205–217.
Mousa, S. K., & Othman, M. (2020). The impact of green human resource management practices on sustainable performance in healthcare organisations: A conceptual framework. Journal of Cleaner Production, 243 (2), 118595.
Mura, M., Lettieri, E., Radaelli, G., & Spiller, N. (2016). Behavioural operations in healthcare: A knowledge sharing perspective. International Journal of Operations & Production Management, 36 (10), 1222–1246.
Nagurney, A. (2021a). Supply chain game theory network modeling under labor constraints: Applications to the Covid-19 pandemic. European Journal of Operational Research, 293 (3), 880–891.
Naor, M., Dey, A., Meyer Goldstein, S., & Rosen, Y. (2018). Civilian-military pooling of health care resources in Haiti: A theory of complementarities perspective. International Journal of Production Research, 56 (21), 6741–6757.
Narayana, S. A., Pati, R. K., & Vrat, P. (2014). Managerial research on the pharmaceutical supply chain—a critical review and some insights for future directions. Journal of Purchasing and Supply Management, 20 (1), 18–40.
Nematollahi, M., Hosseini-Motlagh, S.-M., Ignatius, J., Goh, M., & Nia, M. S. (2018). Coordinating a socially responsible pharmaceutical supply chain under periodic review replenishment policies. Journal of Cleaner Production, 172 (3), 2876–2891.
Nemeth, C., Wears, R. L., Patel, S., Rosen, G., & Cook, R. (2011). Resilience is not control: healthcare, crisis management, and ICT. Cognition, Technology & Work , 13 (3), 189–202.
Nudurupati, S. S., Bhattacharya, A., Lascelles, D., & Caton, N. (2015). Strategic sourcing with multi-stakeholders through value co-creation: An evidence from global health care company. International Journal of Production Economics, 166 (5), 248–257.
Oumlil, A. B., & Williams, A. J. (2011). Strategic alliances and organisational buying: An empirical study of the healthcare industry. International Journal of Procurement Management, 4 (6), 610–626.
Pamucar, D., Torkayesh, A. E., & Biswas, S. (2022). Supplier selection in healthcare supply chain management during the COVID-19 pandemic: A novel fuzzy rough decision-making approach. Annals of Operations Research, 1–43.
Pan, X., Geng, N., Xie, X., & Wen, J. (2019). Managing appointments with waiting time targets and random walk-ins. Omega , 95 , 102062.
Pan, X., Geng, Na., & Xie, X. (2021). Appointment scheduling and real-time sequencing strategies for patient unpunctuality. European Journal of Operational Research, 295 (1), 246–260.
Pang, B., & Lee, L. (2008). Opinion mining and sentiment analysis. Foundations and Trends® in Information Retrieval, 2 (1–2), 1–135.
Pohjosenperä, T., Kekkonen, P., Pekkarinen, S., & Juga, J. (2019). Service modularity in managing healthcare logistics. The International Journal of Logistics Management, 3 (1), 174–191.
Prasad, S., Sundarraj, R., Tata, J., & Altay, N. (2018). Action-research-based optimisation model for health care behaviour change in rural India. International Journal of Production Research, 56 (21), 6774–6792.
Priya, M., & Ranjith Kumar, P. (2015). A novel intelligent approach for predicting atherosclerotic individuals from big data for healthcare. International Journal of Production Research, 53 (24), 7517–7532.
Rahimian, H., Bayraksan, G., & Homem-de-Mello, T. (2019). Controlling risk and demand ambiguity in newsvendor models. European Journal of Operational Research, 279 (3), 854–868.
Rezali, N., Ali, M. H., & Idris, F. (2018). Empowering green healthcare supply chain management practices challenges and future research. International Journal of Supply Chain Management, 7 (5), 282–289.
Roberts, M. E., Stewart, B. M., Tingley, D., Lucas, C., Leder-Luis, J., Gadarian, S. K., et al. (2014). Structural topic models for open-ended survey responses. American Journal of Political Science , 58 (4), 1064–1082.
Ross, A. D., & Jayaraman, V. (2009). Strategic purchases of bundled products in a health care supply chain environment. Decision Sciences, 40 (2), 269–293.
Rubbio, I., Bruccoleri, M., Pietrosi, A., & Ragonese, B. (2019). Digital health technology enhances resilient behaviour: Evidence from the ward. International Journal of Operations & Production Management, 40 (1), 34–67.
Saedi, S., Erhun Kundakcioglu, O., & Henry, A. C. (2016). Mitigating the impact of drug shortages for a healthcare facility: An inventory management approach. European Journal of Operational Research, 251 (1), 107–123.
Salarpour, M., & Nagurney, A. (2021). A multicountry, multicommodity stochastic game theory network model of competition for medical supplies inspired by the Covid-19 pandemic. International Journal of Production Economics, 236 , 108074. https://doi.org/10.1016/j.ijpe.2021.108074
Shehadeh, K. S., Cohn, A. E. M., & Epelman, M. A. (2019). Analysis of models for the Stochastic Outpatient Procedure Scheduling Problem. European Journal of Operational Research, 279 (3), 721–731.
Silge, J., & Robinson, D. (2016). tidytext: Text mining and analysis using tidy data principles in R. Journal of Open Source Software, 1 (3), 37–45.
Stevens, K., Kegelmeyer, P., Andrzejewski, D., & Buttler, D. (2012). Exploring topic coherence over many models and many topics . Paper presented at the proceedings of the 2012 joint conference on empirical methods in natural language processing and computational natural language learning .
Sul, H. K., Dennis, A. R., & Yuan, L. (2017). Trading on twitter: Using social media sentiment to predict stock returns. Decision Sciences, 48 (3), 454–488.
Sultan, N. (2014). Making use of cloud computing for healthcare provision: Opportunities and challenges. International Journal of Information Management, 34 (2), 177–184.
Tanwar, T., Kumar, U. D., & Mustafee, N. (2019). Optimal package pricing in healthcare services. Journal of the Operational Research Society , published online, 1–13.
Tavana, M., Govindan, K., Nasr, A. K., Heidary, M. S., & Mina, H. (2021). A mathematical programming approach for equitable COVID-19 vaccine distribution in developing countries. Annals of Operations Research . https://doi.org/10.1007/s10479-021-04130-z
Thakur, V. (2021). Framework for PESTEL dimensions of sustainable healthcare waste management: Learnings from COVID-19 outbreak. Journal of Cleaner Production, 287 , 125562. https://doi.org/10.1016/j.jclepro.2020.125562
Thorsen, A., & McGarvey, R. G. (2018). Efficient frontiers in a frontier state: Viability of mobile dentistry services in rural areas. European Journal of Operational Research, 268 (3), 1062–1076.
Tirunillai, S., & Tellis, G. J. (2014). Mining marketing meaning from online chatter: Strategic brand analysis of big data using latent dirichlet allocation. Journal of Marketing Research, 51 (4), 463–479.
Topuz, K., Uner, H., Oztekin, A., & Yildirim, M. B. (2018). Predicting pediatric clinic no-shows: A decision analytic framework using elastic net and Bayesian belief network. Annals of Operations Research, 263 (1–2), 479–499.
VanBerkel, P. T., & Blake, J. T. (2007). A comprehensive simulation for wait time reduction and capacity planning applied in general surgery. Health Care Management Science, 10 (4), 373–385.
Vargo, S. L., & Akaka, M. A. (2012). Value cocreation and service systems (re) formation: A service ecosystems view. Service Science, 4 (3), 207–217.
Viegas, C. V., Bond, A., Vaz, C. R., & Bertolo, R. J. (2019). Reverse flows within the pharmaceutical supply chain: A classificatory review from the perspective of end-of-use and end-of-life medicines. Journal of Cleaner Production , published online, 117719.
Vissers, J. M., Adan, I. J., & Dellaert, N. P. (2007). Developing a platform for comparison of hospital admission systems: An illustration. European Journal of Operational Research, 180 (3), 1290–1301.
Volland, J., Fügener, A., Schoenfelder, J., & Brunner, J. O. (2017). Material logistics in hospitals: A literature review. Omega (united Kingdom), 69 (3), 82–101.
Yan, E. (2014). Research dynamics: Measuring the continuity and popularity of research topics. Journal of Informetrics, 8 (1), 98–110.
Zabinsky, Z. B., Dulyakupt, P., Zangeneh-Khamooshi, S., Xiao, C., Zhang, P., Kiatsupaibul, S., & Heim, J. A. (2020). Optimal collection of medical specimens and delivery to central laboratory. Annals of Operations Research, 287 (1), 537–564.
Zandkarimkhani, S., Mina, H., Biuki, M., & Govindan, K. (2020). A chance constrained fuzzy goal programming approach for perishable pharmaceutical supply chain network design. Annals of Operations Research . https://doi.org/10.1007/s10479-020-03677-7
Zhang, Y., Wang, Y., Tang, J., & Lim, A. (2020). Mitigating overtime risk in tactical surgical scheduling. Omega, 93 , online 102024.
Zhang, L. (2012). Aspect and entity extraction from opinion documents . Springer.
Download references
Author information
Authors and affiliations.
School of Business and Law, CQ University, Rockhampton North Campus, Sydney, Australia
SDU- Center for Sustainable Supply Chain Engineering, Department of Technology and Innovation, University of Southern Denmark, Campusvej 55, Odense, Denmark
Devika Kannan
You can also search for this author in PubMed Google Scholar
Corresponding author
Correspondence to Devika Kannan .
Additional information
Publisher's note.
Springer Nature remains neutral with regard to jurisdictional claims in published maps and institutional affiliations.
Rights and permissions
Reprints and permissions
About this article
Ali, I., Kannan, D. Mapping research on healthcare operations and supply chain management: a topic modelling-based literature review. Ann Oper Res 315 , 29–55 (2022). https://doi.org/10.1007/s10479-022-04596-5
Download citation
Accepted : 14 February 2022
Published : 31 March 2022
Issue Date : August 2022
DOI : https://doi.org/10.1007/s10479-022-04596-5
Share this article
Anyone you share the following link with will be able to read this content:
Sorry, a shareable link is not currently available for this article.
Provided by the Springer Nature SharedIt content-sharing initiative
- Supply chain
- Topic modelling
- Literature review
- Find a journal
- Publish with us
- Track your research
Join for Free
Already on SCMDOJO? Log in
I accept SCMDOJO'S Terms of Use and Privacy Notice .
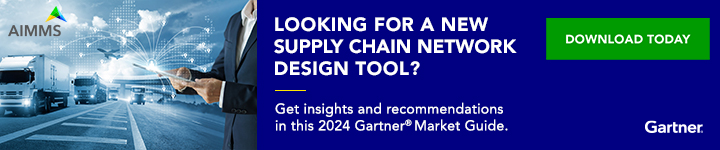
Supply Chain Management Thesis Topics- Top 40 Ideas
- Career Advice
One of the most frequently asked questions from SCMDOJO followers is, I am doing a Supply Chain Management Master from Europe, the UK or the USA, and I need some Master Thesis ideas in Supply Chain.
Key academic research areas in SCM are offering robust and implementable supply chain management thesis that are transforming worldwide trends. The increasing strength of global Supply Chain Management (SCM) is one functional area that shows several students are seeking a good start, especially in solving significant problems in the form of Masters and PhD thesis .
Nevertheless, with the changing trends in the industry, some students are likely to struggle with the early stages of academic writing. A significant reason for this problem is usually down to a lack of ideas or facing new topics with low research activity.
Old Industries and New Industries
The recent pattern shifts in academia, from the traditional research approach to other conventional methods, is taking a more student-centred view. Most of the supply chain management thesis is crafted by students, including dissertation, topic creation, research, and more with help of their supervisors.
With new industries, like Amazon and Apple, transforming old concepts with technological disruption, there are new trends to look out for to help narrow your supply chain management thesis.
The 7 Powerful Supply Chain Trends (I also dubbed “Supply Chain 7.0”) have the potential to become a powerful influence over time. These trends include Augmented reality (AR), Big Data, Gamification of the supply chain, moving the supply chain to the “Cloud,” and the Internet of Things (IoT) – Industry 4.0. Also, Artificial Intelligence (AI) and machine learning in the supply chain, alongside 3D Printing, are now needed to support the product life cycle.
Forbes also highlights the key 2020 Supply Chain Technology Trends that are receiving lots of buzz in Supply Chain Management. In this regard, students seeking top-notch research areas for supply chain management thesis can consider new trends to help create adequate research content.
40 Supply Chain Management Thesis Topics for 2024
On these premises, any supply chain management thesis should be comprehensive. There several topics and areas to consider, and below are 40 Supply Chain Management Thesis Topics for 2020 that students can do research on towards an excellent postgraduate study in SCM.
Digital Transformation
- Digital Transformation Key Attributes; Challenges; enablers & Success Factors.
- Smart Government Initiatives: How Governments are Driving Digital Change.
- Digital Leadership is linking to Virtual Teams or Self Organised Teams (Agile PM).
- Did COVID 19 impact the implementation of digital transformation?
- Cross-functional collaboration in the decision-making process.
- The value of data and interdependencies in decision-making.
- Machine learning techniques in supply chain management.
- Blockchain Technology in Supply Chain Transparency: Evaluating the Impact on Traceability and Trust.
- The Role of Artificial Intelligence in Predictive Analytics for Inventory Management.
- Sustainability in Digital Supply Chain Management: A Comparative Analysis of Environmental Impact.
- Digital Transformation in Warehouse Management: Enhancing Efficiency and Customer Satisfaction
Sustainable Project Management (SPM)
- Can apply the SPM model or any of its dimensions to any type of project
- Can Blockchain help with Sustainable Project Management?
- Factors affecting the application of an efficient supply management system.
IoT- Industry 4.0 and Big Data
- Application of IoT in Logistics – Challenges; enablers & Success Factors
- The practicability of intertwined supply networks with IoT.
- Implementation of IoT in 3PL/4PL Industry – Challenges; enablers & Success Factors
- Big data and impact in DDMRP
- Evaluation of technology use in modern supply chain management.
- The extension of supply chain resilience through Industry 4.0
- The Impact of Industry 4.0 on Supply Chain Management.
- Implementation of E-logistics in Supply Chain Operations.
- Predictive Maintenance Strategies in Industry 4.0-enabled Supply Chains: A Case Study Approach
- Optimizing Supply Chain Decision-Making through Real-time Big Data Analytics
- Cybersecurity Challenges in IoT-Enabled Supply Chains: A Comprehensive Analysis.
Operations and Supply Chain Management
- Risk Evaluation and Management involved in a supply chain
- Partnerships Perspective in Supply Chain Management
- Assessing Supply Chain Risk Management Capabilities
- Implementation of Green Supply Chain Management Practices
- Supply Chain Management Practices and Supply Chain Performance Effectiveness
- The Impact of Supply Chain Management Practices on the Overall Performance of the org
- The Influence of Environmental Management Practices and Supply Chain Integration on Technological Innovation Performance
- The Relationship between Total Quality Management Practices and their Effects on Firm Performance
- Level of Commitment to Top Management regarding the TQM Implementation
- Impact of Mobility Solutions (transportation / latest technologies) on logistics.
- Study on the roles of supply chain management in corporate outsourcing.
- Evaluating strategies for cost reduction in SCM relating to exports and imports.
- Circular Economy Practices in Supply Chain Management: A Case Study Approach
- Demand Forecasting in Omnichannel Retail: A Comparative Analysis of Forecasting Models.
- Ethical and Sustainable Procurement Practices: An Examination of Implementation Challenges and Benefits.
Watch my YouTube vlog explaining more on Top 10 HOT TOPICS for Supply Chain Research for Master Thesis or Ph.D. Thesis.
Get My PhD Thesis- Supplier Development Framework
The supply chain systems of today are more likely to see massive changes technologically in the coming years. Some selected supply chain management thesis topics may face limited data or access to real-time data in making proper research and forecast, including seasonality and trends. So, due diligence is necessary to ensure you not only pick an exciting supply chain management thesis, but you also have sufficient access to data, studies, and materials useful in such an area. The impact of these trends alongside technological advancement in the selected areas would certainly help your thesis stand out and unique.
If are looking for more articles in the education category, you can visit this page
Frequently Asked Questions (FAQ)
What are the emergent research topics in the field of supply chain management.
Blockchain Integration : Exploring the application of blockchain technology to enhance transparency, traceability, and security in supply chains.
Sustainable Supply Chains : Investigating strategies for integrating environmentally friendly practices, ethical sourcing, and social responsibility into supply chain management.
Digital Transformation : Studying the impact of emerging technologies like artificial intelligence, machine learning, and the Internet of Things on optimizing supply chain processes.
Resilience and Risk Managemen t: Analyzing approaches to build resilient supply chains capable of adapting to disruptions, such as pandemics, natural disasters, and geopolitical events.
Circular Economy : Examining supply chain models that minimize waste and promote the reuse, recycling, and repurposing of materials.
Supply Chain Visibility : Researching ways to improve real-time visibility into supply chain activities to enhance decision-making and responsiveness.
Last-Mile Delivery Optimization : Investigating innovative approaches to streamline and optimize the final stages of product delivery to consumers.
Supply Chain Finance : Exploring financial strategies and tools to optimize cash flow and working capital within supply chains.
Collaborative Supply Chains : Studying methods to enhance collaboration and information-sharing among supply chain partners to achieve mutual benefits.
E-commerce Integration : Examining the challenges and opportunities associated with integrating e-commerce platforms into traditional supply chain models.
What are the 4 areas of supply chain management?
What is the primary goal of the supply chain.
The main goal of a supply chain is to efficiently and effectively manage the flow of products or services from the point of origin to the point of consumption. This involves optimizing processes from procurement and production to distribution and delivery. The overarching objective is to meet customer demands with the right products, in the right quantity, at the right time, while minimizing costs and maximizing overall supply chain performance.
What is the difference between supply chain and supply chain management?
The term “supply chain” refers to the entire network of entities and activities involved in the creation and delivery of a product or service, encompassing suppliers, manufacturers, distributors, retailers, and customers. “Supply chain management,” on the other hand, is the strategic coordination and oversight of these interconnected processes to optimize efficiency, reduce costs, and enhance overall performance. While the supply chain is the broader concept, supply chain management specifically involves the planning, execution, and control of various elements within that network to achieve business objectives.
Recommended Books
How to get a phd: a handbook for students and their supervisors.
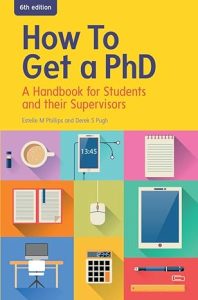
How to Get a PhD: How to Set Yourself Up for Success in the First 12 Months (Getting My PhD)

About the Author- Dr Muddassir Ahmed
Dr MuddassirAhmed is the Founder & CEO of SCMDOJO. He is a global speaker , vlogger and supply chain industry expert with 17 years of experience in the Manufacturing Industry in the UK, Europe, the Middle East and South East Asia in various Supply Chain leadership roles. Dr. Muddassir has received a PhD in Management Science from Lancaster University Management School. Muddassir is a Six Sigma black belt and founded the leading supply chain platform SCMDOJO to enable supply chain professionals and teams to thrive by providing best-in-class knowledge content, tools and access to experts.
You can follow him on LinkedIn , Facebook , Twitter or Instagram
- Research & Improvement

Free Supply Chain Digitalization Assessment
- Measure your organization's current digitalization readiness - 63 survey questions across six categories to achieve the objective
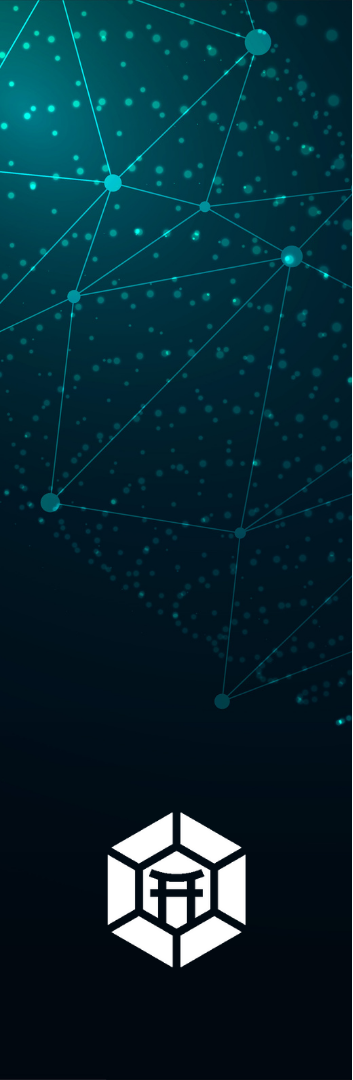
supply chain management Recently Published Documents
Total documents.
- Latest Documents
- Most Cited Documents
- Contributed Authors
- Related Sources
- Related Keywords
Effect of eco-innovation on green supply chain management, circular economy capability, and performance of small and medium enterprises
The mediating effect of digital supply chain management among the relationship between lean management and supply chain operations, construction and optimization of green supply chain management mode of agricultural enterprises in the digital economy.
Digital economy is a rapidly developing economic form under the background of the information age. This article introduces the construction and optimization of the green supply chain management model of agricultural enterprises under the digital economy, and intends to provide some ideas and directions for the development of green supply chain management of agricultural enterprises based on digital economy. This paper proposes the construction and optimization research methods of the green supply chain management model of agricultural enterprises under the digital economy, including the overview of green supply chain management, the construction of the optimization management model of the green supply chain of agricultural enterprises, and the digital economy under the construction of a green supply chain management practice index system for agricultural enterprises. Experimental results show that average Cronbachα value of each scale factor of the optimized management model in this paper is 0.876, and optimized decision-making coordination mechanism has high internal consistency.
Blockchain-Enabled IoT-BIM Platform for Supply Chain Management in Modular Construction
It resources and quality attributes: the impact on electronic green supply chain management implementation and performance, knowledge mapping of digital twin and physical internet in supply chain management: a systematic literature review, rfid-integrated blockchain-driven circular supply chain management: a system architecture for b2b tea industry, logistics 4.0 and smart supply chain management, ict utilization in supply chain environmental collaboration practices as the moderator of firms’ financial performance.
The purpose of this study is to verify whether increasing the intensity of ICT utilization in environmental collaboration practices (ECPs) in the supply chain has a positive impact on firms’ financial performance (FP). As such, it answers the call for investigating the moderators of firms’ FP in their environmental supply chain management. The paper presents the results of a study conducted among 500 firms located in Poland with the use of the CATI technique. The research results show that increasing the intensity of ICT utilization in ECPs, in most cases, does not affect the firms’ FP. Statistically significant relations were observed only in green design ECPs, which shows that increasing the intensity of ICT utilization in green design ECPs reduces the likelihood of revenue growth thanks to ECPs implementation. The paper fills a research gap in the moderating role of the intensity of ICT utilization in increasing financial benefits thanks to the implementation of ECPs in the supply chain. As such, it contributes to the environmental supply chain management literature. The results highlight the need for further research on the moderators of firms’ financial benefits from ECPs implementation, which should be studies in isolation but also jointly, to verify their combined effect. Also, the character of different ECPs categories should be studied to link them with the most appropriate ICT.
ANALISIS SISTEM INFORMASI AKUNTANSI DAN PENGENDALIAN INTERNAL PERSEDIAAN BAHAN BAKU PADA KEDAI KOPI LEDOKAN di BINANGUN
Abstrac The accounting system is a tool used to organize or collect, collect, and conduct information regarding all company transactions, where employees, company activities, materials and machines can be integrated in such a way that supervision can be carried out. This study aims to find out how to analyze information systems and internal control of raw material supplies at the Ledokan Coffee shop in Binangun Blitar. The data used are qualitative data in the form of some basic analysis from field interviews. The results showed that the Ledokan Coffee Shop has good supply chain management capabilities as evidenced by the existence of suppliers who have long-term cooperative relationships with them. Keywords: Accounting Information System, Inventory, Internal Contro Abstrak Sistem akuntansi adalah alat yang digunakan untuk mengatur atau mengumpulkan, mengumpulkan, dan melakukan informasi mengenai semua transaksi perusahaan, di mana karyawan, kegiatan perusahaan, bahan dan mesin dapat diintegrasikan sedemikian rupa sehingga pengawasan dapat dilakukan.Penelitian ini bertujuan untuk mengetahui bagaimana menganalisis system informasi dan pengendalian internal persedian bahan baku pada kedai Kopi Ledokan di Binangun Blitar. Data yang digunakan adalah data kualitatif berupa beberapa analisis yang mendasar dari wawancara dilapangan. Hasil penelitian menunjukkan bahwa Kedai Kopi Ledokan memiliki kemampuan supply chain management yang baik yang dibuktikan dengan adanya pemasok yang memiliki hubungan kerjasama jangka panjang dengan mereka. Kata kunci: Sistem Informasi Akuntansi, Persediaan, Pengendalian Internal
Export Citation Format
Share document.

An official website of the United States government
The .gov means it’s official. Federal government websites often end in .gov or .mil. Before sharing sensitive information, make sure you’re on a federal government site.
The site is secure. The https:// ensures that you are connecting to the official website and that any information you provide is encrypted and transmitted securely.
- Publications
- Account settings
Preview improvements coming to the PMC website in October 2024. Learn More or Try it out now .
- Advanced Search
- Journal List
- Springer Nature - PMC COVID-19 Collection

Mapping research on healthcare operations and supply chain management: a topic modelling-based literature review
1 School of Business and Law, CQ University, Rockhampton North Campus, Sydney, Australia
Devika Kannan
2 SDU- Center for Sustainable Supply Chain Engineering, Department of Technology and Innovation, University of Southern Denmark, Campusvej 55, Odense, Denmark
The literature on healthcare operations and supply chain management has seen unprecedented growth over the past two decades. This paper seeks to advance the body of knowledge on this topic by utilising a topic modelling-based literature review to identify the core topics, examine their dynamic changes, and identify opportunities for further research in the area. Based on an analysis of 571 articles published until 25 January 2022, we identify numerous popular topics of research in the area, including patient waiting time, COVID-19 pandemic, Industry 4.0 technologies, sustainability, risk and resilience, climate change, circular economy, humanitarian logistics, behavioural operations, service-ecosystem, and knowledge management. We reviewed current literature around each topic and offered insights into what aspects of each topic have been studied and what are the recent developments and opportunities for more impactful future research. Doing so, this review help advance the contemporary scholarship on healthcare operations and supply chain management and offers resonant insights for researchers, research students, journal editors, and policymakers in the field.
Introduction
Effective and efficient delivery of healthcare services is essential to counteract emergent diseases and promote healthy lives and the well-being of a population, particularly amid the COVID-19 outbreak. Many healthcare systems across the globe strive to efficiently utilise available resources and improve processes through more integrated operations involving all key stakeholders. As such, in the past few decades, research on healthcare has grown exponentially and has become a self-sustained discipline in operations and supply chain management (OSCM). Healthcare OSCM is a network of healthcare providers, pharmaceutical manufacturers, drug suppliers, distributors, and logistics service providers that are involved in the process of acquiring and transforming raw material into finished products, adding value, and facilitating the flow of products and services to the end-customer. With the rapid growth of research on healthcare OSCM, the extant studies have been conducted in various directions and expanded over a dozen of academic journals.
As the field has grown, a corresponding growth in systematic literature reviews in healthcare has occurred, especially in the past six years. For example, Narayana et al. ( 2014 ) reviewed 99 articles (published between 2000 to 2011) on the pharmaceutical supply chain and explore that most studies focus on efficiency improvement with growing interest in process analysis and technology implementation. Volland et al. ( 2017 ) presented a review of 145 articles (published between 1998 and 2014) on material logistics in hospitals and suggested further work on the concepts of supply and procurement, inventory management, and distribution and scheduling. Ali et al. ( 2018 ) conducted a review of 88 articles (published between 2009 and 2018) on cloud computing in hospitals and devised a framework on opportunities, issues, and applications of cloud computing-enabled healthcare systems. Malik et al. ( 2018 ) reviewed 22 articles (published between 2001 and 2015) on the application of data mining in healthcare service delivery and revealed that application of data mining is narrow-focused (workforce scheduling and quality of care), thereby suggesting further research on the broader application of data mining. Moons et al. ( 2019 ) presented a review of 56 articles (published between 2010 and 2016) on logistics performance measurement in the internal hospital supply chain, specifically inventory management and distribution activities in the operation theatre. Diwas Singh et al. ( 2020 ) offered a review of 70 empirical articles (published between 1999 and 2018) from three journals ( Management Science, Manufacturing and Service Operations Management, and Production and Operations Management ) and suggested the need for more empirical research on personalised medicine, value-based healthcare, and digitisation of healthcare. Keskinocak and Savva ( 2020 ) mapped the healthcare research published between 2009 and 2018 from a single journal ( Manufacturing and Service Operations Management ) and suggested the need to develop and apply new OM methods for the improvement of healthcare service.
The existing literature reviews have different scopes and emphases focusing on a specific topic (e.g., material logistics in hospitals, data mining, only empirical papers, etc.). In other words, they are not designed to capture latent topics (hidden topics) that reside in large volumes of scholarly data. Expanding upon some knowledge from the existing reviews, this paper seeks to advance our understanding of the key research topics that have been addressed in healthcare OSCM using a topic modelling-based literature review. Topic modelling with Latent Dirichlet Allocation (LDA) has been found as an effective method to algorithmically and automatically uncover the abstract topics that reside in a large and unstructured collection of articles (Antons et al., 2016 ; Blei, 2012 ; Zhang, 2012 ). LDA topic modelling builds upon rigorous statistical foundations which allows topic generation with little human intervention and/or manual processing (Blei, 2012 ; Zhang, 2012 ). Such an automatic method creates more meaningful and realistic topics and ensures the reliability and validity of outcomes as opposed to manual methods (Griffiths & Steyvers, 2004 ). LDA has been successfully applied for topic modelling in the information science field (Yan, 2014 ), marketing (Tirunillai & Tellis, 2014 ), statistics (De Battisti et al., 2015 ), tourism (Guo et al., 2017 ), decision sciences (Chae & Olson, 2018 ), computer science (Guo et al., 2017 ), and hydropower research (Jiang et al., 2016 ). However, to date, we have only seen a very limited application of topic modelling-base literature in OSCM fields (e.g., Chae & Olson, 2018 ; Lee & Kang, 2018 ), particularly in the healthcare context, to explore the significant topics hidden in a large sample of articles and to locate gaps for further research in each topic. As such, this study aims to: (1) uncover the latent topics residing in the articles published on healthcare OSCM, (2) examine the topic trend/evolution over time including hot, cold, and steady topics, and (3) find opportunities for more impactful research on each topic through a review of the literature around each topic. Driven by these core aims; the following research questions are posed:
- What are the emergent topics of research in the field of healthcare OSCM?
- How has research on each topic evolved over the past two decades?
- What are the opportunities for more impactful future research in the area?
Our study is among the few that offers a topic modelling-based review of literature, which allows us objectively to uncover hidden patterns of research that pervade in a large collection of studies and to explore the development of literature on each topic. The paper advances our understanding of how the literature on different topics in the field of healthcare OSCM has evolved over time and what are the recent trends, knowledge flow, and opportunities for insightful research on each topic. We find an increasing focus and need for further investigation on some emergent topics, such as patient waiting time, COVID-19 pandemic, Industry 4.0 technologies, sustainability, risk and resilience, climate change, circular economy, humanitarian logistics, behavioural operations, service-ecosystem, and knowledge management. In terms of research methods, our analysis shows the increasing interest of researchers in the Markov method, mixed-integer programming, simulation, queueing model and stochastic programming in exploring multidimensional issues of healthcare OSCM. In addition, our analysis suggests that the past three years have also seen some empirical contributions in the area, including structural equation modelling, longitudinal study, and action research; thus, encouraging empirical researchers for more fruitful contributions on the topic.
Our study offers valuable research directions to researchers, journal editors, and policymakers in the field of healthcare OSCM. Researchers can observe whether their recent research topics are hot, cold, or steady, which will allow them to select appropriate topics for more productive forthcoming study in the area. Particularly, some early-career researchers find it difficult to keep up with the growing literature in their fields of research and familiarise themselves with a huge volume of literature in identifying the subject area on which they can focus or apply for research grants. The information from our topic modelling can be a useful tool for these researchers to understand recent developments in the field and then select more appropriate topics. Our findings can also be helpful to PhD students, who often face difficulties in topic selection due to time pressure. Journal editors can view if the extant research is consistent with their editorial policy, and they may even choose to launch a new editorial vision and direction. Our list of hot topics can be a valuable reference tool to launch special issues in the journals. Discovering underlying topics and tracking their evolution could also be of great interest to policymakers (e.g., government agencies) and industry. The information on which research themes and methods are growing or declining in popularity over time (Table (Table3 3 and Fig. 4 ) can support governmental funding agencies for grants allocation to promising areas. Likewise, firm managers and the industry as a whole can use this information to formulate promising R&D strategies and more informed investment decisions.
Hot, cold, and steady topics/methods

Topic trend/dynamic over time
The rest of the paper is structured as follows. Section 2 elaborates on the research methods, covering data collection, screening and analysis procedures. Section 3 outlines results which include word cloud, key term matrix, topic labelling, and evolution of each topic over time. Section 4 provides discussion, while Sect. 5 offers a review of literature and opportunities for further research. The study is concluded in Sect. 6.
As mentioned earlier, this study employs the topic modelling method. The superiority of the topic modelling method has been evidenced in other fields, for example, Latent Semantic Analysis (LSA) (Deerwester et al., 1990 ), Non-Negative Matrix Factorization (NMF) (Lee & Seung, 1999 ), and probabilistic topic modelling. LSA uses singular value decomposition and a traditional matrix factorization technique to reduce the dimensionality of documents (Deerwester et al., 1990 ). Unlike singular value decomposition, in NMF, the transformed and reduced version of documents contains no negative elements (Lee & Seung, 1999 ). Probabilistic topic modelling is a recent development in topic modelling where words and documents are described through probabilistic topics, and a topic is described in terms of a probability distribution over a vocabulary of words (Hofmann, 2001 ; Lee & Kang, 2018 ). Latent Dirichlet Allocation (LDA) is the most popular probabilistic topic modelling algorithm. A comparison of LSA, NMF, and LDA suggests that LDA has the greatest ability to maintain topic coherence and to generate predominant topics that handle the issue of polysemy, the overlapping of words with multiple meanings (Stevens et al., 2012 ). LDA has been successfully applied in the information science field (Yan, 2014 ), marketing (Tirunillai & Tellis, 2014 ), statistics (De Battisti et al., 2015 ), tourism (Guo et al., 2017 ), decision sciences (Chae & Olson, 2018 ), computer science (Guo et al., 2017 ), and hydropower research (Jiang et al., 2016 ). Borrowing knowledge from adjacent areas, this study employs LDA in healthcare. The analysis is completed in four main steps: data collection; text pre-processing; word cloud; and topic modelling.
Data collection
The analysis aims to uncover the landscape of healthcare research published in good quality international journals in the field of operations and supply chains. The data were collected from Scopus on 25 January 2022, which is one of the largest abstracts and citation databases of academic papers (Ali & Gölgeci, 2019 ; Ferreira, 2018 ; Ali et al., 2021a , 2021b ). To retrieve a broad range of literature on the topic, a large set of keywords was developed based on the authors’ extensive knowledge of the field and review of the extant literature in the field. These keywords included: healthcare, AND operations AND “supply chain” OR hospital OR pharmaceutical OR drug OR medicine AND model OR framework OR Stochastic OR Simulation OR “linear programming” OR Heuristic OR mathematical OR optimization OR “numerical model” OR “nonlinear programming” OR “decision tool” OR “decision analysis” OR “discrete event” OR multi-objective OR multi-objective OR multi-attribute OR multi-criteria OR MCDM OR MCDA OR qualitative OR quantitative OR “case study” OR empirical. Quotation marks were placed on the keywords comprised of more than one word (e.g., “linear programming”) to ensure that search engines consider them as one word.
Initially, we did not limit the timespan because we sought to capture a wide range of publications. The search query generated 663 articles. We excluded the three articles published before 2000 to set the time interval between 2000 and 25 January 2022 (the date of data collection). Next, we carefully read the title and abstract of each article and excluded irrelevant articles. We also excluded articles in non-English languages. Extreme care was taken to include the articles that focus on healthcare OSCM. The inclusion and exclusion criteria resulted in the final set of 571 articles as shown in the supplementary file. Table Table1 1 presents the distribution of these articles.
Distribution of articles across 20 selected journals
The articles selected from Scopus were validated using other databases, such as Web of Science, Google Scholar, ProQuest, and EBSCO to ensure that no important articles were missed in the review. The validation process also helped confirm that Scopus contains a greater number of related articles on the topic of healthcare OSCM compared to other databases, so it was used as a preferred database for this research.
Data pre-processing
Once the articles are collected from Scopus, some pre-processing of data collected is required to conduct further analysis. First, since our search result included the article’s title, keywords and abstract, we merged them as a single document. Second, we removed all punctuation and numbers and transformed all characters into lower-case. Third, we eliminated all “stop-words,” whose main role is to make a sentence grammatically correct, e.g., articles (a, an, the) and prepositions (of, by, from, etc.) and general words that repeatedly appear in most articles, e.g., “and,” “paper”, “find”, “effect”, and “discuss”. These words are not semantically meaningful, yet they create trouble in the analysis process. This step is important because without applying stop-word elimination, our analytical method could result in a big proportion of these unwanted words in the word cloud as well as in the topic matrix.
Word cloud with R-package is a text mining method to find the most frequently used keywords in sampled articles. A word cloud was designed to allow a visual interpretation of main themes and their trajectories in our review transcripts over the four different periods (2000–2004, 2005–2009, 2010–2014, 2015–2022). As mentioned earlier, we also included articles published until 25 January 2022. Data file for each period was imported into a popular R package, word cloud (Hornik & Grün, 2011 ) with default Dirichlet hyperparameters. The following functions in the R-package were applied to create word clouds: tm for text mining: snowballC for text stemming; Wordcloud2 for generating word cloud images; RColorBrewer for colour palettes.
Topics modelling
To extract the predominant topics from the 571 sampled articles, LDA’s generative process model was applied (Blei, 2012 ). Figure 1 demonstrates the process and its main variables where η is the parameter of the Dirichlet prior for the per-topic word distribution. K is the defined number of topics, while β k is a distribution of words over a topic. W represents the observable variable—the words in each article. W d,n is the n th word in article d . α is the parameter of the Dirichlet on the per-article topic distribution. θ d is a distribution of topics for the d th article. Z d,n is the topic assignment for the nth word in article d . Some variables are interdependent, that is, W (the observed word) depends on β (the distribution of topics) and Z (the topic assignment for the word), on θ (the topic proportion for the document). This process can be represented by the joint distribution of latent and observed variables through the following Figure and equations:

The graphical representation for LDA.
Adapted from Blei ( 2012 )
The generative process aims to explore the topic structure (θ, z , and β), which can explain W . Given the observed variable ( W ), the posterior distribution is estimated with the following equation:
The purpose of Fig. 1 and Eqs. 1 and 2 herein is to demonstrate the analysis process followed by LDA. To perform the analysis objectively and automatically, we used the R-package topic-models along with the tm package (Hornik & Grün, 2011 ). This analytical process produces a document term matrix for the desired number of topics.
Analysis and results
This section presents the analysis and the results of data processing, which consist of word cloud, topic modelling, and topic labelling.
As presented in Fig. 2 , the word clouds show some noticeable changes between 2000 and 2022 in the healthcare literature. In the first period (2000–2004), the popular terms include strategy, logistics, risk, simulation, integer, programming, operations, reengineering, information, inventory, and planning. While a few terms in the first period and second period (2005–2009) are similar (e.g., reengineering, outsourcing, supply, chains, healthcare, strategy, logistics), several new terms became popular in the second period which includes sustainability, warehousing, reverse, heuristic, stochastics, empirical, knowledge, humanitarian, among others.

Word cloud demonstrating changing pattern of terms over time (2000–2022)
The third period (2010–2014) shows some important changes, where several new terms (e.g., behavioural, literature, reviews, multilevel, action, structural, equation, Markov) emerged, and other terms (e.g., reengineering, outsourcing, strategy) disappeared. Some other terms remain similar to the second period (e.g., sustainability, warehousing, reverse, heuristic, stochastics, empirical, knowledge, humanitarian, waiting time).
While many terms of the third period continued to appear in the fourth period (2015–2022), several new terms are generated: circular, economy, climate, change, longitudinal. Terms such as warehousing, marketing, strategy disappeared in this period. Understandably, the terms “healthcare”, “operations”, “supply”, “chain” appeared consistently across the four periods.
Topic modelling
While the visualisation of word clouds (observed words) provides a broad picture of the research dynamics in the literature motivating towards further analysis, it does not provide quantitative information on the relationship between different terms that are generated. Without having quantitative information on each key term, it would be difficult to merge the related terms and assign them into specific topics.
To derive the quantitative information on the nexus between different terms, we used LDA topic modelling that explores the hidden or latent structure and generates different topics by merging interrelated terms from the data set. Generating an appropriate number of topics ( K value) from the given sample of articles is always challenging (Roberts et al., 2014 ). Selecting too few topics would not provide a full and meaningful understanding of the field, while selecting too many topics often results in meaningless outcomes. Therefore, to select the appropriate number of topics, two measures were undertaken: (1) a different number of topics (K value) were tried and accuracies of outcome were checked for each set of topics, and (2) brainstorming among researchers was conducted to ensure topic quality and relatedness to the context of the study (Mimno & McCallum, 2012 ; Silge & Robinson, 2016 ). As a result, 34 relevant topics were produced using LDA with R-package (Fig. 3 ). For each topic, the top five terms were selected, where each term represents a distribution weight (per-topic term probability).

Topic matrix generated in R-package using LDA principle
Topic labelling
We conducted topic labelling based on the ‘LDA topic matrix’ that produced 34 topics (see Fig. 3 ). Each topic constitutes five terms with different distribution weights. Since the term “healthcare,” “operations,” “supply,” and “chain” appeared in most topics, we decided not to use any of them in labelling the topics. Further, while topic labelling in Table Table2, 2 , we used the numbering/sequence of topics exactly following the sequence of topics in the ‘LDA topic matrix’ (Fig. 3 ). To briefly illustrate the process of topic labelling, we discuss a few examples of topic labelling. From Fig. 3 , the top terms in Topic#1 are queuing and waiting, followed by hospital, patient, process; therefore, the topic is labelled the “patient’s waiting time”. Topic#2 includes top terms, such as RFID, big, data followed cloud, computing; therefore, we label the topic as “Industry 4.0”. Industry 4.0 refers to the fourth industrial revolution with the growing use of autonomous technologies such as cloud computing, big data analytics, internet supported RFID and others. Topic# 3 encompasses sustainability, economic, sustainable; hence, it is labelled as sustainability.
Likewise, Topic#8 is labelled “risk and resilience” since the top terms are risk, adaption, resilience supported by healthcare and readiness. Topic#18 is labelled “Stochastic programming” because its top terms are stochastics, programming, followed by model, method, and chain. We adopted a similar process for labelling all 34 topics as demonstrated in Table Table2 2 .
Topic dynamic/evolution over time
In this section, we introduce the hot, cold, and steady topics (research themes and methods) to provide researchers with an idea of where trends are going (hot) and not going (cold). Exploring the dynamic changes in different topics over time can offer directions for more impactful research on healthcare. We identified three patterns of topic evolution including hot, cold, and steady. For this purpose, we employed a bivariate correlation between the frequency of keywords and time period using four different time periods, namely 2000–2004; 2005–2009; 2010–2014; 2015–2022 (Fig. 3 ). Scopus database was used to collect the information on the frequency of keywords (for each topic shown in Fig. 3 and Table Table2) 2 ) in each time interval.
Hot, cold, and steady topics (which include ‘research themes’ and ‘research methods’ as shown in Table Table3) 3 ) were identified based on the direction of the trendline on each topic and the value of slope (Fig. 4 ).
Hot topics show a trendline moving upward and a positive slope (x-value). Examples of the hot topics related to research themes include the Patient’s waiting time, Industry 4.0 technologies, Sustainability, Circular economy, Behavioural OSCM (OSCM), Risk and resilience, Climate change, and research methods that constitute Markov method, Simulation, Stochastic programming, among others.
The number of contributions on these topics (themes and methods) increased almost continuously from 2010 to 2014, with a dramatic increase on some topics (e.g., patient waiting time, sustainability, circular economy, etc.) between 2015 and 2022.
Cold topics, on the other hand, imply the opposite pattern to these hot topics; they show declining trendline and negative slope values, perhaps due to the relative lack of interest of researchers in recent times.
Examples of cold topics associated with research themes include warehousing, marketing, outsourcing, business process reengineering, strategy, operation strategy, and research methods of heuristics and game theory model among others. These topics enjoyed significant attention between 2000 and 2015, but their proportions have been steadily decreasing over the past five years (Fig. 4 ).
Finally, steady topics show a rise in the beginning but there is no rise or fall in trendlines over the past 5 or more years; perhaps, their importance has not been increased over time.
Overall, two topics depicted a steady growth over the past 5 or more years including goal programming and multi-level analysis. As can be seen in Fig. 4 , the trendline for goal programming modelling increased up to 2009, while no remarkable increase was observed since then. Likewise, the trendline for multi-level analysis shows an upward trend until 2014; however, no growth has been demonstrated since then.
While not investigated in this study, it can be speculated that these topics may lose the interest of research and likely become a cold topic in future. Drawing upon trendline and slope for each topic in Fig. 4 , we have presented all 34 topics under three main categories: hot, cold, and steady (Table (Table3). 3 ). For better clarity of expression, we have segregated the topics into themes and methods. While both themes and methods are both important, our focus is on research themes in this analysis and discussion.
Over the last two decades, research on healthcare OSCM has seen spectacular growth. In light of the burgeoning literature on the topic, this study was motivated to explore recent developments, trends, and core topics of research in the area. The study was driven by three key research questions. Addressing the first research question, we explored 34 core topics on which extant research on the topic has been conducted over the past decades. For the second research question, our topic modelling analysis has resulted in the identification of hot, cold, and steady topics in empirical research healthcare OSCM (Table (Table3 3 and Fig. 4 ). Thirteen topics of research themes show an upward trend, and nine of them (Patient waiting time, Industry 4.0, Sustainable OSCM, Behavioural OSCM, Risk and resilience, Humanitarian logistics, Climate change, Healthcare logistics, and Literature reviews) start with a relatively flat value (close to 0) before showing a rising trend from around 2010. The other four topics (Circular economy, Service ecosystem, Knowledge management, and Pharmaceutical OSCM) show a gradual upward trend from the early 2000s. Five research topics belong to cold topics. Outsourcing and operations strategy shows an almost linear declining trend, while both Marketing and Warehousing show a bell-shaped trend whose popularity rose in the early 2000s but fell after 2010 and 2015, respectively. Business process reengineering was a popular topic during the early 2000s but, since 2010, shows a declining trend. It is important to note, however, that being identified as a cold topic does not suggest that the topics are not important and should be abandoned. The results provide more of an indication about the interest (trends) of researchers’ interests rather than the importance of the topics themselves. It is also interesting to see that most of the changes of the trends (either upward or downward) of the topics took place around 2010.
Finally, in response to the third research question, we offered a review of the literature and identified numerous opportunities for further research (Sect. 5). Our findings show a shift of current research from traditional topics of operations management (Business process reengineering, Marketing, Outsourcing, Heuristics) to emergent topics such as Patient waiting time, Industry 4.0 technologies, Sustainable operations, Risk and resilience, Climate change, and Circular economy. In general, the shift in topics seems to show a move away from traditional, efficiency-focused research toward broader goals of superior customer service and system’s effectiveness. While each of the three groups of topics deserves discussion, in this section, we discuss the hot research topics as well as provide recommendations of avenues for fruitful future research. It should be noted that although the topic “literature reviews” was a hot topic, we do not include it in our discussion below as the extant research related to it has already been discussed in the introduction section.
Literature review and opportunities for further research
The following section provides a brief synthesis of current literature around emergent research topics that are recovered through topic modelling, and it offers numerous opportunities for further research in the field.
Patients’ waiting time and COVID-19
This research topic covers two interrelated and emerging issues: patient waiting time and COVID-19 outbreak. With a rapidly growing population of aged people, healthcare systems have been facing pressure for more health services resulting in patients’ long waiting times. Therefore, determining methods to ameliorate waiting time has been high on the agenda of most developing and developed economies. Correspondingly, in recent years, mostly building upon simulation and Markov as the primary method of research, considerable studies appeared around the issues of patients’ waiting time, such as patient’s admission process (Chae, 2019 ; Vissers et al., 2007 ); surgery procedures (VanBerkel & Blake, 2007 ); patient inflow and outflow in the emergency department (Abo-Hamad & Arisha, 2013 ); no-shows of patients and overbooking (Topuz et al., 2018 ); outpatient appointment scheduling (Shehadeh et al., 2019 ); time overestimation for the emergency procedure (Gartner & Padman, 2019 ); walk-in patients treatment (Pan et al., 2019 ); patient readmission and discharge planning model (Gu et al., 2019 ); resource planning model to reduce waiting time of patients (Hejazi, 2021 ); load smoothing of scheduled admission to the reduced number of beds required and probability of delay (Asgari & Asgari, 2021 ), and modelled association between length of appointment interval and no-shows of patients (Pan et al., 2021 ).
Overall, although the existing studies have captured several important issues related to patient waiting time, we identify the need for further research on this significant topic. For example, there is potential to develop an integrated model investigating how multiple factors such as general service time, equipment breakdown, high attrition of hospital staff, variability in patient’s arrival time, and unpunctuality of already booked patients cumulatively contribute to patients’ waiting time. Further research can also devise a model at the intersection of patients’ waiting list management, operations theatre scheduling, and patient waiting time for surgery. While the literature on simulation and mathematical modelling continues to grow, there is also an opportunity to further expand empirical research for testing causal links between various factors and their combined effect on patient waiting time. For example, empirical research can test links among customers’ heterogeneous behaviour and their service time in scheduling appointments. Further, a unified model capturing non-value-added practices at both micro and meso levels would be fruitful future research in reducing patient waiting time. While research on bedding and staffing is growing, there is a need to optimise bed and staff requirements amid the global pandemic. Another potential research area is to examine the longitudinal effect of hospital staff’s training on patients’ waiting time.
Likewise, given the outbreak of COVID-19, the recent literature of healthcare OSCM has been rapidly growing on the topic. Pamucar et al. ( 2022 ) proposed a model for supplier selection amid COVID-19. Fan and Xie ( 2022 ) generated an optimization model for COVID-19 testing facility design and planning. Ghaderi ( 2022 ) offered a framework for public health intervention in the wake of the pandemic. Govindan et al. ( 2020 ) developed a decision support system and fuzzy interface system to help with demand management in the healthcare supply chain amid the COVID-19 crises. Nagurney ( 2021a ) modelled labour shortage due to COVID-19, resulting in reduced food harvest and supply. Tavana et al. ( 2021 ) presented a mixed-integer programming model for the equitable distribution of COVID-19 vaccine in developing countries. Salarpour and Nagurney ( 2021 ) constructed a stochastic model to study competition among different countries for medical supplies amid COVID-19 crises. Nagurney ( 2021b ) offered a supply chain network optimization model to manage labour in electronic commerce during the COVID-19 pandemic. Thakur ( 2021 ) developed a model to manage hospital waste during the COVID-19 outbreak. Choi ( 2021 ) used a sense-and-response specific OR model to demonstrate the specific actions needed to deal with the COVID-19 outbreak.
To sum up, while the past research continues to grow on COVID, it would be interesting to explore how to extenuate potential risks and develop shockproof healthcare systems to deal with unanticipated outbreaks such as COVID-19 and similar future incidents. In this regard, it is worth exploring how such a topic as Industry 4.0 technologies could be applied across the healthcare system to capture data from wide sources and help the decision-making process in the event of a pandemic, including contact tracing, the identification of the outbreak location. Some potential areas of research amid COVID-19 include but are not limited to patient inflow and outflow in the emergency department; outpatient appointment scheduling model; models on time estimation for the emergency procedure; walk-in patients’ treatment model; patient admission and discharge planning model; resource planning model to reduce waiting time of patients; load smoothing of scheduled admission to the reduced number of beds required and probability of delay. Besides, pharmaceutical SCM would be the ultimate hope for the recovery and possibly eradication of the virus that allows us to go back to normal life. It would be insightful to examine how pharmaceutical supply chains were affected by COVID-19 and develop a recovery model dealing with such a situation. In particular, if/when a pharmaceutical solution to COVID-19 emerges, research is needed to help understand the best ways to rapidly scale up and distribute the materials on a scale that has never been seen before. It is also of interest to study whether synergy in logistics could be achieved between the public organisations and the growing number of private companies that have entered the healthcare business. Future research should continue to incorporate the healthcare and hospital view into operations management and transfer established concepts from other industries into healthcare while accounting for industry specifics. Again, the links between this and COVID-19 are both obvious and critical.
Industry 4.0 technologies
Industry 4.0 or the fourth industrial revolution refers to fully automated and in-connected technologies such as the Internet of Things (IoT), cloud computing, big data analytics, blockchain, artificial intelligence, among others (Ali & Govindan, 2021 ; Ali et al., 2021a , 2021b ). Industry 4.0 technologies can enhance the level of service in the industry through real-time information sharing, visibility, traceability, agility, and connectivity within a firm’s boundary and across the supply chain (Govindan et al., 2022 ). Given this, growth in research on the role of Industry 4.0 technologies in healthcare service provision is evident, such as Sultan ( 2014 ) developed a conceptual framework on the potential of cloud computing in the advancement of healthcare services. Chong et al. ( 2015 ) modelled variables that influence the adoption of IoT (e.g., RFID) in healthcare. Priya and Ranjith Kumar ( 2015 ) used big data analytics to predict the progression of atherosclerotic disease, the narrowing and hardening of arteries due to the accumulation of plaque on the artery wall. Fan et al. ( 2018 ) recognised the factors affecting the adoption of artificial intelligence-based medical diagnosis support systems. Kochan et al. ( 2018 ) modelled on how cloud computing improves visibility and responsiveness in hospital SC. Galetsi et al. ( 2020 ) developed a theoretical framework for the realisation of big data analytics.
The research on Industry 4.0 is growing with the advent of new technologies and provides fruitful opportunities for further research. The models on big data should incorporate data of all inter-connected functions in healthcare, instead of just focusing on a single function or silo approach. For example, the impact of Industry 4.0 on the entire healthcare supply chain can be modelled. This is important to understand how interaction with different functions/partners through Industry 4.0 technologies influence healthcare productivity. Most of the current research focuses on big data and cloud computing which needs to be expanded to other Industry 4.0 technologies, such as blockchain, cyber-physical systems and the Internet of Things in healthcare OSCM. There is a lack of a holistic model testing the combined impact of all Industry 4.0 technologies on the healthcare system and an investigation into if there is a trade-off for investing between various digital technologies would be helpful. Future research can also investigate how machine learning techniques impact diagnostic, maintenance, and prognostics systems in healthcare settings. Research highlights the intricate issues of human–machine interaction at the workplace (Arslan et al., 2021 ); we suggest further research on how to maintain trustworthiness and to harmonise productive human–machine interactions and reduce workers’ resistance to automated machines in the healthcare settings. We also highlight the need for further research on the application of artificial intelligence in healthcare OSCM.
Sustainable operations and supply chain
Today sustainability is a growing concern of most organisations and therefore the topic of sustainability has received increasing focus from researchers from operations management. Healthcare systems worldwide face pressing challenges of service quality and cost issues as well as safe disposal of waste. As such, in recent times considerable attempts have been made to realise sustainability in the healthcare system. Chauhan and Singh ( 2016 ) employed a hybrid model for the selection of sustainable locations to dispose of healthcare waste. Anuar et al. ( 2018 ) offered a conceptual framework demonstrating the link between lean practices and a sustainable healthcare system. Malekpoor et al. ( 2018 ) modelled a sustainable healthcare treatment plan. Thorsen and McGarvey ( 2018 ) investigated the effectiveness of fixed and mobile dentistry for the financial sustainability of the healthcare system. Mousa and Othman ( 2020 ) found a positive impact of green human resource management practices on a sustainable healthcare system.
While research on healthcare sustainability is growing, there is still space for further research. For instance, more studies are needed that simultaneously investigate the impact of all three dimensions (social, environmental, economic) of sustainability through a single model. Prospective studies can also investigate the combined impact of synchronous business activities including green human resource management, green marketing, and green production on healthcare sustainability. There is also an opportunity to develop a sustainable performance measurement and management model in healthcare.
Circular economy
The circular economy is a recent concept aimed to eliminate waste through the make, use, recycle and reuse approach. It helps to overcome a take-make-dispose linear pattern of production and consumption. The healthcare industry inevitably generates waste that may become hazardous to both public health and the environment. Therefore, the circular economy has recently gained significant attention in healthcare literature. For example, Kumar and Rahman ( 2014 ) suggested RFID supported process reengineering for waste management ensuring circular economy. Jensen et al. ( 2019 ) discussed the healthcare refurbish system as a means to reduce waste. Dehghani et al. ( 2019 ) suggested that transhipment can reduce waste and save substantial costs compared to no-transhipment. Viegas et al. ( 2019 ) identified the need for research on the circularity of end-of-use medicine in the pharmaceutical supply chain.
Although research circular economy has witnessed mounting attention, there is still room for further research. For instance, there is a need to develop a model that could make the circularity of outdated medicine more visible through all stages of reverse flow and re-manufacturing. Also, healthcare literature lacks studies modelling processes and decision-making for circular economy implementation at micro and meso levels. Prospective research can also explore the healthcare staff’s perception of the circular economy. It would be interesting to investigate how healthcare professionals are prepared to deal with the circular economy and how they might contribute to reducing wastage in reverse flow.
Service ecosystem
With its root in marketing, the service ecosystems concept is defined as “relatively self-contained, self-adjusting systems of resource-integrating actors connected by shared institutional logics and mutual value creation through service exchange” (Vargo & Akaka, 2012 , p. 207). That is, the service ecosystem generates value for all the actors in a network through resource integration, value co-creation, and co-innovation. The service ecosystem concept has received a great deal of attention in healthcare literature. Nudurupati et al. ( 2015 ) elaborate on the process of value co-creation through strategic alliance and collaboration with multi-stakeholders. Albarune et al. ( 2015 ) suggested a value-based supply chain for integrated hospital management. Chae ( 2019 ) suggested digital innovation as a promising source of resource sharing and value creation among actors in an ecosystem. Lee et al. ( 2020 ) showed a positive relationship between the previous year value-based purchasing penalty and the current year care process improvement in the hospital.
Service ecosystem research is a growing area of research in the healthcare context; thus, offering opportunities for further expansion. For example, the influence of contextual behavioural factors in value co-creation and unlocking innovation needs further investigation. Also, service ecosystem research needs to be expanded from the organisational level to the entire healthcare system. For example, how resource integration practice can be integrated into a multitude of value co-creation processes in the healthcare networks. Moreover, the role of advanced technologies and servitisation in the healthcare service ecosystem has yet to be investigated.
Knowledge management
Knowledge management refers to the process of generating, transferring, and managing knowledge and information in an organisation or network. Knowledge management becomes a major driver of performance and productivity where front-line workers are required to provide customised services tailored to the need of individual clients such as healthcare. As such, the literature has witnessed considerable growth in literature on knowledge management in healthcare settings. Gagnon et al. ( 2016 ) empirically corroborated a positive association between knowledge management on staff practices and quality of healthcare. Mura et al. ( 2016 ) found that knowledge exchange and assets have a positive impact on workers’ innovation, such as idea generation, idea promotion and idea implementation. Avgerinos and Gokpinar ( 2017 ) argued that knowledge transfer capability in a team positively influences healthcare productivity. Hiranrithikorn and Sutduean ( 2019 ) expound that access to information and knowledge has a positive influence on healthcare supply chain skills. Despite considerable contributions to knowledge management, there is still significant room for further research. A few models are investigating the impact of knowledge sharing on the quality and productivity of healthcare performance. Qualitative research can be conducted to explore the barrier and enablers of knowledge sharing in healthcare. Future research can use multi-method research investigating the relationship between social capital, culture, and knowledge management.
Behavioural operations
Research on behavioural operations is well-received, particularly from healthcare service perspectives. Healthcare systems have two main stakeholders: staff and patients. The research suggests that the findings of healthcare modelling may not be reliable without considering the behaviours of the main stakeholders (Brailsford et al., 2012 ). For example, a simulation model suggesting the effectiveness of a new drug will be unreliable if it does not consider patient behaviour; some patients may not complete the prescribed course of medication, and potential side effects of medicine should be shown. As such, healthcare literature has seen an emergence of interest in understanding both human behaviour in practice and how to capture it in operations research models. Brailsford et al. ( 2012 ) used a simulation model capturing behavioural factors of a patient while treating breast cancer. Based on a review of literature, Kunc et al. ( 2018 ) identified scarcity of research on behavioural aspects of healthcare. Harper ( 2019 ) used a queuing model to assess how healthcare workers behave and treat patients, especially when such professionals encounter changing workloads, service queues, and other factors that impact service quality.
To sum, behavioural operational research has become an important research topic in healthcare but significant room for further research in the area remains intact. Specifically, we suggest more research with detailed models on the nexus between behavioural interventions on healthcare practice. Future research can model workforce adaptive behaviours and productivity. We agree that the incorporation of behavioural factors is important to increase the reliability of the healthcare model because of the increasing influence of human behaviours in healthcare operations. Future research can also examine the behavioural consequences of inter-organisational interaction in complex healthcare systems.
Risk and resilience
Healthcare systems are susceptible to operational failures in services offered to patients when a shortage of medicine and equipment occurs, or when the execution of such systems is incorrectly managed. Given the divergent risks emerging from a variety of sources, there is an increasing focus on risk mitigation and the development of resilient healthcare. Nemeth et al. ( 2011 ) developed a model for healthcare crises management and resilience. Mahjoub et al. ( 2014 ) found risk mitigation in healthcare through a risk-sharing agreement between pharmaceutical companies and healthcare service providers. Saedi et al. ( 2016 ) suggested an optimal inventory policy to reduce drug shortage risks. Rubbio et al. ( 2019 ) examined the role of digital technologies in developing healthcare resilience. Rahimian et al. ( 2019 ) investigated an appropriate level of risk-aversion through robust optimization. Zhang et al. ( 2020 ) formulated a model which helps to reduce overtime risk during surgery in a hospital.
While research on risk and resilience in healthcare is growing, there is still substantial room for more impactful future research. Most models investigate risk and resilience in isolation. Others focus on particular functions such as hospital surgery. As such, a quantitative model that simultaneously investigates risk and resilience focusing on the key supply chain actors in healthcare is lacking. Future research can also use a mixed-methods approach where qualitative research can explore various risk factors and resilience strategies, while quantitative research can test the interrelationship between risk and resilience in explaining healthcare performance.
Humanitarian logistics
Humanitarian logistics refers to the transportation, delivery, and distribution of supplies in the event of natural disasters or another emergency to the affected area and people. Given the unprecedented incidents such as floods, cyclones, disease outbursts, the humanitarian logistics in healthcare (e.g., supplies of medicines, medical equipment, sterile items, linen, and food) has gained significant attention in recent times. For example, Naor et al. ( 2018 ) found strategies for effective humanitarian logistics in healthcare, such as quickly sending a team to the disaster area to gather firsthand information on the unique situations of disaster followed by the main delegation staff carrying effective disaster relief service. Prasad et al. ( 2018 ) suggested effective healthcare intervention to deal with disease outbreaks in a community. Cherkesly et al. ( 2019 ) assist with the design of a community healthcare network, increasing the service coverage for underserved areas in adverse situations.
Although research on humanitarian logistics has been progressing steadily, we highlight the need for further research on healthcare OSCM. A fruitful future research avenue is modelling the role of volunteers and NGOs in humanitarian logistics from a healthcare perspective. Also, interviews with disaster area patients can provide additional insights into the real-world phenomenon. Further research can also develop a model of budget allocation as the community service demand increases drastically due to a natural disaster. It would be interesting to investigate the nexus among service compliance rate, disease rate, and total cost by disaster type.
Pharmaceutical OSCM
A pharmaceutical supply chain involves firms, processes, and operations involved in the design, development, and distribution of life-saving drugs. It is one of the critical supply chains since it is related to the life and health of people. The success of healthcare services directly depends on well-managed pharmaceutical supplies. Therefore, the management of the pharmaceutical supply chain has become a popular topic in healthcare literature. Jia and Zhao ( 2017 ) highlighted the issue of drug shortage due to lack of collaboration between pharmacies and pharmaceutical manufacturing and supplier. Nematollahi et al. ( 2018 ) also found a positive link between hospital service level and efficient pharmaceutical supply chain. Viegas et al. ( 2019 ) discussed the significance of a coordinated pharmaceutical supply chain for the reverse flow of end-of-life medicine. Recently, Zandkarimkhani et al. ( 2020 ) formulated a model to redesign the pharmaceutical supply chain network in the wake of demand uncertainty.
Despite growth in literature on the pharmaceutical supply chain, significant room for further research still exists, particularly in the context of a recent pandemic. Most of the existing research lacks a holistic view that involves all key stakeholders of the supply chain. There is less focus on upstream networks and processes (Narayana et al., 2014 ). There is also limited research on integrated new product development in the pharmaceutical supply chain. There is also an opportunity for research to model examining the link between healthcare financing and pharmaceutical supply chain financing such as investment in R&D, production and distribution, between waste management, environmental and pharmaceutical supply chains. Future studies can also model the behaviour of individuals in the robustness of pharmaceutical supply chains. Given that supply chain design is critical in the planning process (Govindan et al., 2017 ), we suggest designing integrated models that encompass all stages from drug manufacturing to final consumer for robust decision-making in the pharmaceutical supply chain. Another interesting avenue is formulating a model at the intersection of supplier selection and sustainable pharmaceutical supply chain networks.
Climate change and healthcare
The increased intensity and frequency of disasters due to extreme weather conditions draw our attention to the unprecedented changes in climate which are mainly attributed to excessive greenhouse gas emissions and carbon footprints. To deal with the climate change challenges, greenhouse gas mitigation targets were set to a minimum level in the historic Paris Agreement (Bodansky, 2016 ). While a lot of research spotlights the emissions reduction in the automotive, energy, and mining industries, the carbon footprint and climate change research has recently received attention. Rezali et al. ( 2018 ) discussed the importance of a green healthcare supply chain to reduce the environmental impact of the healthcare industry. Belkhir and Elmeligi ( 2019 ) examined 15 pharmaceutical supply chains across different countries and found that the pharmaceutical industry is more emission-intensive than the automotive industry. Tanwar et al. ( 2019 ) identify the potential risks and risk mitigation measures in implementing a green pharmaceutical supply chain. Over the past five years, the topic of climate change has received increasing attention in healthcare settings. Most studies on the topic focus on factors contributing to climate change (greenhouse gas emissions, carbon footprint), thus leaving a gap for research models incorporating the business impact of climate risks when they unfold and examining specific risk mitigation measures.
Healthcare logistics
Healthcare logistics refer to the physical flow of healthcare materials from the pharmaceutical production facility to the healthcare service provider. Logistics cost has been identified as the second largest cost after personnel in the healthcare system (Ross & Jayaraman, 2009 ). As such, the importance of material flow in healthcare service is recognised important to add value and reduce the cost of services. Correspondingly, a growth in literature on healthcare logistics is evident. Oumlil and Williams ( 2011 ) suggested strategic alliance and consolidation of logistics in the healthcare sector as a strategy to reduced logistics costs. Volland et al. ( 2017 ) identified four main domains in which healthcare logistics research exists, including procurement, inventory management, distribution, and supply chain management. Al-Sharhan et al. ( 2019 ) expounded on the significance of hospital-supplier integration of inefficient material logistics. Fathollahi-Fard et al. ( 2019 ) developed a green home healthcare supply chain model to reduce emissions in logistics. Pohjosenperä et al. ( 2019 ) discussed the impact of service modularity in value-creating and improving healthcare logistics service. Zabinsky et al. ( 2020 ) developed a model through mixed-integer linear programming to address healthcare scheduling and routing problem. Further research could examine an item-level analysis of different product categories in the regional healthcare units, together with their replenishment models. Such a study is needed to show the true potential of modularity in service offerings of the logistics support organisation.
Over the last two decades, research on healthcare OSCM has been continuously advancing making it a self-sustained discipline in the OSCM field. We argue that with this unprecedented development in healthcare literature, the current status and trends in the field need to be mapped. The use of robust statistical and automatic methods for extracting key topics from a large set of articles has been increasingly used in other fields such as finance, marketing, statistics, tourism, and computer sciences. Borrowing the knowledge from these adjacent fields, this study employs probabilistic topic modelling with LDA as an automatic text analytics approach to unpack dominant topics and development trajectories in a large collection of research articles on healthcare OSCM. In doing so, we contribute to the literature on new and rigorous methods for systematic literature review in the field of OSCM. Our sample included 571 articles retrieved from 20 journals in the field of OSCM. The results produced 34 topics on healthcare research. Analysis of dynamic changes in the 34 over the past twenty years suggested 24 hot, 8 cold, and 2 steady topics (see Table Table3 3 ).
Our findings show a shift of current research from traditional topics of operations management (Business process reengineering, Marketing, Outsourcing, Heuristics) to emergent topics such as Patient waiting for time, Industry 4.0 technologies, Sustainable operations, Risk and resilience, Climate change, and Circular economy. In general, the shift in topics seems to show a move away from traditional, efficiency-focused research toward broader goals of superior customer service and system’s effectiveness. The data on research methods shows a larger dominance of modelling research (e.g., Markov, mixed-integer programming, simulation, queueing model, and stochastic) in exploring multidimensional issues of healthcare operations. However, the past three years have also seen some empirical research, including Structural equation modelling, Longitudinal study, and Action research. This shows a positive change in operations research journals from purely quantitative modelling studies to empirical research as well. This recent shift in a strategy not only offers a great opportunity to empirical researchers for publication in some OR journals but also provides sound premises on which to expand the scope and subscription of these journals.
The findings of this research offer useful research directions to researchers, journal editors, and policymakers in the field of healthcare research. Researchers can observe whether their recent research topics are hot, cold, or steady, and accordingly select appropriate topics for more impactful future research in the area. Particularly, some early-career researchers find it difficult to keep up with the growing literature in their fields of research and familiarise themselves with a huge volume of literature in identifying the subject area on which they can focus or apply for a research grant. The information from our topic modelling can be a useful tool for these researchers to understand recent developments in the field and then select more appropriate topics. Our findings can also be helpful to PhD students, who often face difficulties in topic selection due to time pressure. Journal editors can view if the extant research is consistent with their editorial policy, and they may like to launch a new editorial vision and direction. Our list of hot topics can be a valuable reference tool to launch special issues in the journals. Discovering underlying topics and tracking their evolution could also be of great interest to policymakers (e.g., government agencies) and industry. The information on which research themes and methods are growing or declining in popularity over time (Table (Table3 3 and Fig. 4 ) can support governmental funding agencies for grants allocation to promising areas. Likewise, firm managers and the industry as a whole can use this information to formulate promising R&D strategies and to make more informed investment decisions.
We believe that topic modelling is one of the most effective and reliable approaches in revealing the latent (hidden) structure and development of research topics in a field, and recommend that future research can combine the LDA-based models with other text analytics techniques from different text data (e.g., customer feedback, medical records, research reports, business reviews, company descriptions) and interconnection among various business areas (e.g., marketing, supply chain, information systems). For example, sentiment analysis (Pang & Lee, 2008 ) is deemed a popular text analytics tool in the field of finance (Sul et al., 2017 ), marketing (Liang et al., 2015 ), and operations/supply chain (Chae, 2015 ). The sentiment analysis can be integrated with topic modelling: a two-pronged method using topic modelling followed by sentiment analysis can offer more nuanced findings by revealing both positive and negative aspects of a product and/or service.
As with several studies, there are some limitations of our study. First, given the authors’ extensive knowledge of the field and review of the extant literature, full efforts were made to guarantee that all pertinent articles were included in the analysis. However, some articles might have been ignored. Nevertheless, two measures might help mitigate this limitation: (1) the topics are generated based on the frequencies of the keywords/key terms and, therefore, missing a few articles from such a large dataset (571 articles) will not have a significant impact on overall results; (2) the dataset is validated with other databases. Second, the research is limited to healthcare research published in OSCM journals. That is, we excluded the papers and related journals containing healthcare research but not from OSCM perspectives.
Publisher's Note
Springer Nature remains neutral with regard to jurisdictional claims in published maps and institutional affiliations.
- Abo-Hamad W, Arisha A. Simulation-based framework to improve patient experience in an emergency department. European Journal of Operational Research. 2013; 224 (1):154–166. doi: 10.1016/j.ejor.2012.07.028. [ CrossRef ] [ Google Scholar ]
- Albarune ARB, Farhat N, Afzal F. The valued supply chain for integrated hospital management: A conceptual framework. International Journal of Supply Chain Management. 2015; 4 (3):39–49. [ Google Scholar ]
- Ali, I. (2019). The impact of industry 4.0 on the nexus between supply chain risks and firm performance. In A. Guclu (Ed. 2019) Academy of Management Proceedings (1–6). Boston: Academy of Management.
- Ali, I., Arslan, A., Khan, Z., & Tarba, S. Y. (2021a). The role of industry 4.0 technologies in mitigating supply chain disruption: Empirical evidence from the Australian Food Processing Industry. IEEE Transactions on Engineering Management, 1–11. 10.1109/TEM.2021.3088518
- Ali, I., & Aboelmaged, M. G. S. (2021). Implementation of supply chain 4.0 in the food and beverage industry: Perceived drivers and barriers. International Journal of Productivity and Performance Management , ahead-of-print (ahead-of-print). 10.1108/IJPPM-07-2020-0393
- Ali I, Gölgeci I. Where is supply chain resilience research heading? A systematic and co-occurrence analysis. International Journal of Physical Distribution and Logistics Management. 2019; 49 (8):793–815. doi: 10.1108/IJPDLM-02-2019-0038. [ CrossRef ] [ Google Scholar ]
- Ali I, Govindan K. Extenuating operational risks through digital transformation of agri-food supply chains. Production Planning & Control. 2021 doi: 10.1080/09537287.2021.1988177. [ CrossRef ] [ Google Scholar ]
- Ali I, Sultan P, Aboelmaged M. A bibliometric analysis of academic misconduct research in higher education: Current status and future research opportunities. Accountability in Research. 2021; 28 (6):372–393. doi: 10.1080/08989621.2020.1836620. [ PubMed ] [ CrossRef ] [ Google Scholar ]
- Ali O, Shrestha A, Soar J, Wamba SF. Cloud computing-enabled healthcare opportunities, issues, and applications: A systematic review. International Journal of Information Management. 2018; 43 (6):146–158. doi: 10.1016/j.ijinfomgt.2018.07.009. [ CrossRef ] [ Google Scholar ]
- Al-Sharhan S, Omran E, Lari K. An integrated holistic model for an eHealth system: A national implementation approach and a new cloud-based security model. International Journal of Information Management. 2019; 47 (1):121–130. doi: 10.1016/j.ijinfomgt.2018.12.009. [ CrossRef ] [ Google Scholar ]
- Antons D, Kleer R, Salge TO. Mapping the topic landscape of JPIM, 1984–2013: In search of hidden structures and development trajectories. Journal of Product Innovation Management. 2016; 33 (6):726–749. doi: 10.1111/jpim.12300. [ CrossRef ] [ Google Scholar ]
- Anuar A, Saad R, Yusoff RZ. Sustainability through lean healthcare and operational performance in the private hospitals: A proposed framework. Supply Chain Management. 2018; 7 (5):221–227. [ Google Scholar ]
- Arslan, A., Cooper, C., Khan, Z., Golgeci, I., & Ali, I. (2021). Artificial intelligence and human workers interaction at the team level: a conceptual assessment of the challenges and potential HRM strategies. International Journal of Manpower, ahead-of-print (ahead-of-print). 10.1108/IJM-01-2021-0052
- Asgari F, Asgari S. Addressing artificial variability in patient flow. Operations Research for Health Care. 2021; 28 :100288. doi: 10.1016/j.orhc.2021.100288. [ CrossRef ] [ Google Scholar ]
- Avgerinos E, Gokpinar B. Team familiarity and productivity in cardiac surgery operations: The effect of dispersion, bottlenecks, and task complexity. Manufacturing & Service Operations Management. 2017; 19 (1):19–35. doi: 10.1287/msom.2016.0597. [ CrossRef ] [ Google Scholar ]
- Belkhir L, Elmeligi A. Carbon footprint of the global pharmaceutical industry and relative impact of its major players. Journal of Cleaner Production. 2019; 214 (1):185–194. doi: 10.1016/j.jclepro.2018.11.204. [ CrossRef ] [ Google Scholar ]
- Blei DM. Probabilistic topic models. Communications of the ACM. 2012; 55 (4):77–84. doi: 10.1145/2133806.2133826. [ CrossRef ] [ Google Scholar ]
- Bodansky D. The Paris climate change agreement: A new hope? American Journal of International Law. 2016; 110 (2):288–319. doi: 10.5305/amerjintelaw.110.2.0288. [ CrossRef ] [ Google Scholar ]
- Brailsford SC, Harper PR, Sykes J. Incorporating human behaviour in simulation models of screening for breast cancer. European Journal of Operational Research. 2012; 219 (3):491–507. doi: 10.1016/j.ejor.2011.10.041. [ CrossRef ] [ Google Scholar ]
- Chae, B., & Olson, D. (2018). A topical exploration of the intellectual development of decision sciences 1975–2016. Decision Sciences, Published (online) , 1–24.
- Chae BK. Insights from hashtag# supplychain and Twitter Analytics: Considering Twitter and Twitter data for supply chain practice and research. International Journal of Production Economics. 2015; 165 (3):247–259. doi: 10.1016/j.ijpe.2014.12.037. [ CrossRef ] [ Google Scholar ]
- Chae BK. A General framework for studying the evolution of the digital innovation ecosystem: The case of big data. International Journal of Information Management. 2019; 45 (2):83–94. doi: 10.1016/j.ijinfomgt.2018.10.023. [ CrossRef ] [ Google Scholar ]
- Chauhan A, Singh A. A hybrid multi-criteria decision making method approach for selecting a sustainable location of healthcare waste disposal facility. Journal of Cleaner Production. 2016; 139 (4):1001–1010. doi: 10.1016/j.jclepro.2016.08.098. [ CrossRef ] [ Google Scholar ]
- Cherkesly M, Rancourt MÈ, Smilowitz KR. Community healthcare network in underserved areas: Design, mathematical models, and analysis. Production and Operations Management. 2019; 28 (7):1716–1734. [ Google Scholar ]
- Choi T-M. Fighting against COVID-19: What operations research can help and the sense-and-respond framework. Annals of Operations Research. 2021 doi: 10.1007/s10479-021-03973-w. [ PMC free article ] [ PubMed ] [ CrossRef ] [ Google Scholar ]
- Chong AY-L, Liu MJ, Luo J, Keng-Boon O. Predicting RFID adoption in healthcare supply chain from the perspectives of users. International Journal of Production Economics. 2015; 159 (5):66–75. doi: 10.1016/j.ijpe.2014.09.034. [ CrossRef ] [ Google Scholar ]
- De Battisti F, Ferrara A, Salini S. A decade of research in statistics: A topic model approach. Scientometrics. 2015; 103 (2):413–433. doi: 10.1007/s11192-015-1554-1. [ CrossRef ] [ Google Scholar ]
- Deerwester S, Dumais ST, Furnas GW, Landauer TK, Harshman R. Indexing by latent semantic analysis. Journal of the American Society for Information Science. 1990; 41 (6):391–407. doi: 10.1002/(SICI)1097-4571(199009)41:6<391::AID-ASI1>3.0.CO;2-9. [ CrossRef ] [ Google Scholar ]
- Dehghani, M., Abbasi, B., & Oliveira, F. (2019). Proactive transshipment in the blood supply chain: A stochastic programming approach. Omega , Published (online), 102–112.
- Diwas Singh KC, Scholtes S, Terwiesch C. Empirical research in healthcare operations: Past research, present understanding, and future opportunities. Manufacturing and Service Operations Management. 2020; 22 (1):73–83. doi: 10.1287/msom.2019.0826. [ CrossRef ] [ Google Scholar ]
- Fan, Z., & Xie, X. (2022). A distributionally robust optimisation for COVID-19 testing facility territory design and capacity planning. International Journal of Production Research, 1–24.
- Fan W, Liu J, Zhu S, Pardalos PM. Investigating the impacting factors for the healthcare professionals to adopt artificial intelligence-based medical diagnosis support system (AIMDSS) Annals of Operations Research. 2018 doi: 10.1007/s10479-018-2818-y. [ CrossRef ] [ Google Scholar ]
- Fathollahi-Fard, A. M., Govindan, K., Hajiaghaei-Keshteli, M. and Ahmadi, A. (2019). A green home health care supply chain: New modified simulated annealing algorithms. Journal of Cleaner Production, 240, pp. ahead-of-print.
- Fattahi, M., Keyvanshokooh, E., Kannan, D., & Govindan, K. (2022). Resource planning strategies for healthcare systems during a pandemic. European Journal of Operational Research . [ PMC free article ] [ PubMed ]
- Ferreira, F. A., Kannan, D., Meidutė-Kavaliauskienė, I., & Vale, I. M. (2022). A sociotechnical approach to vaccine manufacturer selection as part of a global immunization strategy against epidemics and pandemics. Annals of Operations Research, 1–30. [ PMC free article ] [ PubMed ]
- Ferreira FA. Mapping the field of arts-based management: Bibliographic coupling and co-citation analyses. Journal of Business Research. 2018; 85 (2):348–357. doi: 10.1016/j.jbusres.2017.03.026. [ CrossRef ] [ Google Scholar ]
- Gagnon M-P, Simonyan D, Godin G, Labrecque M, Ouimet M, Rousseau M. Factors influencing electronic health record adoption by physicians: A multilevel analysis. International Journal of Information Management. 2016; 36 (3):258–270. doi: 10.1016/j.ijinfomgt.2015.12.002. [ CrossRef ] [ Google Scholar ]
- Galetsi P, Katsaliaki K, Kumar S. Big data analytics in health sector: Theoretical framework, techniques and prospects. International Journal of Information Management. 2020; 50 (4):206–216. doi: 10.1016/j.ijinfomgt.2019.05.003. [ CrossRef ] [ Google Scholar ]
- Gartner D, Padman R. Machine learning for healthcare behavioural OR: Addressing waiting time perceptions in emergency care. Journal of the Operational Research Society. 2019; 71 (7):1081–1101. [ Google Scholar ]
- Ghaderi M. Public health interventions in the face of pandemics: Network structure, social distancing, and heterogeneity. European Journal of Operational Research. 2022; 298 (3):1016–1031. doi: 10.1016/j.ejor.2021.08.015. [ CrossRef ] [ Google Scholar ]
- Govindan, K., Nasr, A. K., Mostafazadeh, P., & Mina, H. (2021). Medical waste management during coronavirus disease 2019 (COVID-19) outbreak: A mathematical programming model. Computers & Industrial Engineering , 162 , 107668. [ PMC free article ] [ PubMed ]
- Govindan, K., Nasr, A. K., Saeed Heidary, M., Nosrati-Abargooee, S., & Mina, H. (2022). Prioritizing adoption barriers of platforms based on blockchain technology from balanced scorecard perspectives in healthcare industry: A structural approach. International Journal of Production Research, 1–15.
- Govindan K, Fattahi M, Keyvanshokooh E. Supply chain network design under uncertainty: A comprehensive review and future research directions. European Journal of Operational Research. 2017; 263 (1):108–141. doi: 10.1016/j.ejor.2017.04.009. [ CrossRef ] [ Google Scholar ]
- Govindan K, Mina H, Alavi B. A decision support system for demand management in healthcare supply chains considering the epidemic outbreaks: A case study of coronavirus disease 2019 (COVID-19) Transportation Research Part e: Logistics and Transportation Review. 2020 doi: 10.1016/j.tre.2020.101967. [ PMC free article ] [ PubMed ] [ CrossRef ] [ Google Scholar ]
- Griffiths TL, Steyvers M. Finding scientific topics. Proceedings of the National Academy of Sciences. 2004; 101 (1):5228–5235. doi: 10.1073/pnas.0307752101. [ PMC free article ] [ PubMed ] [ CrossRef ] [ Google Scholar ]
- Gu W, Fan N, Liao H. Evaluating readmission rates and discharge planning by analyzing the length-of-stay of patients. Annals of Operations Research. 2019; 276 (1–2):89–108. doi: 10.1007/s10479-018-2957-1. [ CrossRef ] [ Google Scholar ]
- Guo Y, Barnes SJ, Jia Q. Mining meaning from online ratings and reviews: Tourist satisfaction analysis using latent dirichlet allocation. Tourism Management. 2017; 59 (2):467–483. doi: 10.1016/j.tourman.2016.09.009. [ CrossRef ] [ Google Scholar ]
- Harper PR. Server behaviours in healthcare queueing systems. Journal of the Operational Research Society. 2019; 71 (4):1124–1136. [ Google Scholar ]
- Hejazi T-H. State-dependent resource reallocation plan for health care systems: A simulation optimization approach. Computers & Industrial Engineering. 2021; 159 :107502. doi: 10.1016/j.cie.2021.107502. [ CrossRef ] [ Google Scholar ]
- Hiranrithikorn P, Sutduean J. Social capital predicting the supply chain skills: Mediating role of access to information and network resources. International Journal of Supply Chain Management. 2019; 8 (5):842–853. [ Google Scholar ]
- Hofmann T. Unsupervised learning by probabilistic latent semantic analysis. Machine Learning. 2001; 42 (1–2):177–196. doi: 10.1023/A:1007617005950. [ CrossRef ] [ Google Scholar ]
- Hornik K, Grün B. topicmodels: An R package for fitting topic models. Journal of Statistical Software. 2011; 40 (13):1–30. [ Google Scholar ]
- Jensen JP, Prendeville SM, Bocken NM, Peck D. Creating sustainable value through remanufacturing: Three industry cases. Journal of Cleaner Production. 2019; 218 (4):304–314. doi: 10.1016/j.jclepro.2019.01.301. [ CrossRef ] [ Google Scholar ]
- Jia J, Zhao H. Mitigating the US drug shortages through pareto-improving contracts. Production and Operations Management. 2017; 26 (8):1463–1480. doi: 10.1111/poms.12697. [ CrossRef ] [ Google Scholar ]
- Jiang H, Qiang M, Lin P. A topic modeling based bibliometric exploration of hydropower research. Renewable and Sustainable Energy Reviews. 2016; 57 (3):226–237. doi: 10.1016/j.rser.2015.12.194. [ CrossRef ] [ Google Scholar ]
- Keskinocak P, Savva N. A review of the healthcare-management (Modeling) literature published in manufacturing & service operations management. Manufacturing & Service Operations Management. 2020; 22 (1):59–72. doi: 10.1287/msom.2019.0817. [ CrossRef ] [ Google Scholar ]
- Kochan CG, Nowicki DR, Sauser B, Randall WS. Impact of cloud-based information sharing on hospital supply chain performance: A system dynamics framework. International Journal of Production Economics. 2018; 195 (5):168–185. doi: 10.1016/j.ijpe.2017.10.008. [ CrossRef ] [ Google Scholar ]
- Kumar A, Rahman S. RFID-enabled process reengineering of closed-loop supply chains in the healthcare industry of Singapore. Journal of Cleaner Production. 2014; 85 (2):382–394. doi: 10.1016/j.jclepro.2014.04.037. [ PMC free article ] [ PubMed ] [ CrossRef ] [ Google Scholar ]
- Kunc M, Harper P, Katsikopoulos K. A review of implementation of behavioural aspects in the application of OR in healthcare. Journal of the Operational Research Society. 2018; 71 (7):1055–1072. doi: 10.1080/01605682.2018.1489355. [ CrossRef ] [ Google Scholar ]
- Lee DD, Seung HS. Learning the parts of objects by non-negative matrix factorization. Nature. 1999; 401 (6755):788–791. doi: 10.1038/44565. [ PubMed ] [ CrossRef ] [ Google Scholar ]
- Lee H, Kang P. Identifying core topics in technology and innovation management studies: A topic model approach. The Journal of Technology Transfer. 2018; 43 (5):1291–1317. doi: 10.1007/s10961-017-9561-4. [ CrossRef ] [ Google Scholar ]
- Lee SJ, Venkataraman S, Heim GR, Roth AV, Chilingerian J. Impact of the value-based purchasing program on hospital operations outcomes: An econometric analysis. Journal of Operations Management. 2020; 66 (1–2):151–175. doi: 10.1002/joom.1057. [ CrossRef ] [ Google Scholar ]
- Liang T-P, Li X, Yang C-T, Wang M. What in consumer reviews affects the sales of mobile apps: A multifacet sentiment analysis approach. International Journal of Electronic Commerce. 2015; 20 (2):236–260. doi: 10.1080/10864415.2016.1087823. [ CrossRef ] [ Google Scholar ]
- Mahjoub R, Odegaard F, Zaric GS. Health-based pharmaceutical pay-for-performance risk-sharing agreements. Journal of the Operational Research Society. 2014; 65 (4):588–604. doi: 10.1057/jors.2013.106. [ CrossRef ] [ Google Scholar ]
- Malekpoor, H., Mishra, N., & Kumar, S. (2018). A novel TOPSIS–CBR goal programming approach to sustainable healthcare treatment. Annals of Operations Research, 1–23.
- Malik MM, Abdallah S, Ala’raj, M. Data mining and predictive analytics applications for the delivery of healthcare services: A systematic literature review. Annals of Operations Research. 2018; 270 (1–2):287–312. doi: 10.1007/s10479-016-2393-z. [ CrossRef ] [ Google Scholar ]
- Mimno D, McCallum A. Topic models conditioned on arbitrary features with Dirichlet-multinomial regression. UAI. 2012; 24 :1–8. [ Google Scholar ]
- Moons K, Waeyenbergh G, Pintelon L. Measuring the logistics performance of internal hospital supply chains—a literature study. Omega (united Kingdom) 2019; 82 (3):205–217. [ Google Scholar ]
- Mousa, S. K., & Othman, M. (2020). The impact of green human resource management practices on sustainable performance in healthcare organisations: A conceptual framework. Journal of Cleaner Production, 243 (2), 118595.
- Mura M, Lettieri E, Radaelli G, Spiller N. Behavioural operations in healthcare: A knowledge sharing perspective. International Journal of Operations & Production Management. 2016; 36 (10):1222–1246. doi: 10.1108/IJOPM-04-2015-0234. [ CrossRef ] [ Google Scholar ]
- Nagurney A. Supply chain game theory network modeling under labor constraints: Applications to the Covid-19 pandemic. European Journal of Operational Research. 2021; 293 (3):880–891. doi: 10.1016/j.ejor.2020.12.054. [ PMC free article ] [ PubMed ] [ CrossRef ] [ Google Scholar ]
- Naor M, Dey A, Meyer Goldstein S, Rosen Y. Civilian-military pooling of health care resources in Haiti: A theory of complementarities perspective. International Journal of Production Research. 2018; 56 (21):6741–6757. doi: 10.1080/00207543.2017.1355121. [ CrossRef ] [ Google Scholar ]
- Narayana SA, Pati RK, Vrat P. Managerial research on the pharmaceutical supply chain—a critical review and some insights for future directions. Journal of Purchasing and Supply Management. 2014; 20 (1):18–40. doi: 10.1016/j.pursup.2013.09.001. [ CrossRef ] [ Google Scholar ]
- Nematollahi M, Hosseini-Motlagh S-M, Ignatius J, Goh M, Nia MS. Coordinating a socially responsible pharmaceutical supply chain under periodic review replenishment policies. Journal of Cleaner Production. 2018; 172 (3):2876–2891. doi: 10.1016/j.jclepro.2017.11.126. [ CrossRef ] [ Google Scholar ]
- Nemeth, C., Wears, R. L., Patel, S., Rosen, G., & Cook, R. (2011). Resilience is not control: healthcare, crisis management, and ICT. Cognition, Technology & Work , 13 (3), 189–202.
- Nudurupati SS, Bhattacharya A, Lascelles D, Caton N. Strategic sourcing with multi-stakeholders through value co-creation: An evidence from global health care company. International Journal of Production Economics. 2015; 166 (5):248–257. doi: 10.1016/j.ijpe.2015.01.008. [ CrossRef ] [ Google Scholar ]
- Oumlil AB, Williams AJ. Strategic alliances and organisational buying: An empirical study of the healthcare industry. International Journal of Procurement Management. 2011; 4 (6):610–626. doi: 10.1504/IJPM.2011.043002. [ CrossRef ] [ Google Scholar ]
- Pamucar, D., Torkayesh, A. E., & Biswas, S. (2022). Supplier selection in healthcare supply chain management during the COVID-19 pandemic: A novel fuzzy rough decision-making approach. Annals of Operations Research, 1–43. [ PMC free article ] [ PubMed ]
- Pan, X., Geng, N., Xie, X., & Wen, J. (2019). Managing appointments with waiting time targets and random walk-ins. Omega , 95 , 102062.
- Pan X, Geng Na, Xie X. Appointment scheduling and real-time sequencing strategies for patient unpunctuality. European Journal of Operational Research. 2021; 295 (1):246–260. doi: 10.1016/j.ejor.2021.02.055. [ CrossRef ] [ Google Scholar ]
- Pang, B., & Lee, L. (2008). Opinion mining and sentiment analysis. Foundations and Trends® in Information Retrieval, 2 (1–2), 1–135.
- Pohjosenperä T, Kekkonen P, Pekkarinen S, Juga J. Service modularity in managing healthcare logistics. The International Journal of Logistics Management. 2019; 3 (1):174–191. doi: 10.1108/IJLM-12-2017-0338. [ CrossRef ] [ Google Scholar ]
- Prasad S, Sundarraj R, Tata J, Altay N. Action-research-based optimisation model for health care behaviour change in rural India. International Journal of Production Research. 2018; 56 (21):6774–6792. doi: 10.1080/00207543.2017.1414329. [ CrossRef ] [ Google Scholar ]
- Priya M, Ranjith Kumar P. A novel intelligent approach for predicting atherosclerotic individuals from big data for healthcare. International Journal of Production Research. 2015; 53 (24):7517–7532. doi: 10.1080/00207543.2015.1087655. [ CrossRef ] [ Google Scholar ]
- Rahimian H, Bayraksan G, Homem-de-Mello T. Controlling risk and demand ambiguity in newsvendor models. European Journal of Operational Research. 2019; 279 (3):854–868. doi: 10.1016/j.ejor.2019.06.036. [ CrossRef ] [ Google Scholar ]
- Rezali N, Ali MH, Idris F. Empowering green healthcare supply chain management practices challenges and future research. International Journal of Supply Chain Management. 2018; 7 (5):282–289. [ Google Scholar ]
- Roberts, M. E., Stewart, B. M., Tingley, D., Lucas, C., Leder-Luis, J., Gadarian, S. K., et al. (2014). Structural topic models for open-ended survey responses. American Journal of Political Science , 58 (4), 1064–1082.
- Ross AD, Jayaraman V. Strategic purchases of bundled products in a health care supply chain environment. Decision Sciences. 2009; 40 (2):269–293. doi: 10.1111/j.1540-5915.2009.00228.x. [ CrossRef ] [ Google Scholar ]
- Rubbio I, Bruccoleri M, Pietrosi A, Ragonese B. Digital health technology enhances resilient behaviour: Evidence from the ward. International Journal of Operations & Production Management. 2019; 40 (1):34–67. doi: 10.1108/IJOPM-02-2018-0057. [ CrossRef ] [ Google Scholar ]
- Saedi S, Erhun Kundakcioglu O, Henry AC. Mitigating the impact of drug shortages for a healthcare facility: An inventory management approach. European Journal of Operational Research. 2016; 251 (1):107–123. doi: 10.1016/j.ejor.2015.11.017. [ CrossRef ] [ Google Scholar ]
- Salarpour M, Nagurney A. A multicountry, multicommodity stochastic game theory network model of competition for medical supplies inspired by the Covid-19 pandemic. International Journal of Production Economics. 2021; 236 :108074. doi: 10.1016/j.ijpe.2021.108074. [ PMC free article ] [ PubMed ] [ CrossRef ] [ Google Scholar ]
- Shehadeh KS, Cohn AEM, Epelman MA. Analysis of models for the Stochastic Outpatient Procedure Scheduling Problem. European Journal of Operational Research. 2019; 279 (3):721–731. doi: 10.1016/j.ejor.2019.06.023. [ CrossRef ] [ Google Scholar ]
- Silge J, Robinson D. tidytext: Text mining and analysis using tidy data principles in R. Journal of Open Source Software. 2016; 1 (3):37–45. doi: 10.21105/joss.00037. [ CrossRef ] [ Google Scholar ]
- Stevens, K., Kegelmeyer, P., Andrzejewski, D., & Buttler, D. (2012). Exploring topic coherence over many models and many topics . Paper presented at the proceedings of the 2012 joint conference on empirical methods in natural language processing and computational natural language learning .
- Sul HK, Dennis AR, Yuan L. Trading on twitter: Using social media sentiment to predict stock returns. Decision Sciences. 2017; 48 (3):454–488. doi: 10.1111/deci.12229. [ CrossRef ] [ Google Scholar ]
- Sultan N. Making use of cloud computing for healthcare provision: Opportunities and challenges. International Journal of Information Management. 2014; 34 (2):177–184. doi: 10.1016/j.ijinfomgt.2013.12.011. [ CrossRef ] [ Google Scholar ]
- Tanwar, T., Kumar, U. D., & Mustafee, N. (2019). Optimal package pricing in healthcare services. Journal of the Operational Research Society , published online, 1–13.
- Tavana M, Govindan K, Nasr AK, Heidary MS, Mina H. A mathematical programming approach for equitable COVID-19 vaccine distribution in developing countries. Annals of Operations Research. 2021 doi: 10.1007/s10479-021-04130-z. [ PMC free article ] [ PubMed ] [ CrossRef ] [ Google Scholar ]
- Thakur V. Framework for PESTEL dimensions of sustainable healthcare waste management: Learnings from COVID-19 outbreak. Journal of Cleaner Production. 2021; 287 :125562. doi: 10.1016/j.jclepro.2020.125562. [ PMC free article ] [ PubMed ] [ CrossRef ] [ Google Scholar ]
- Thorsen A, McGarvey RG. Efficient frontiers in a frontier state: Viability of mobile dentistry services in rural areas. European Journal of Operational Research. 2018; 268 (3):1062–1076. doi: 10.1016/j.ejor.2017.07.062. [ CrossRef ] [ Google Scholar ]
- Tirunillai S, Tellis GJ. Mining marketing meaning from online chatter: Strategic brand analysis of big data using latent dirichlet allocation. Journal of Marketing Research. 2014; 51 (4):463–479. doi: 10.1509/jmr.12.0106. [ CrossRef ] [ Google Scholar ]
- Topuz K, Uner H, Oztekin A, Yildirim MB. Predicting pediatric clinic no-shows: A decision analytic framework using elastic net and Bayesian belief network. Annals of Operations Research. 2018; 263 (1–2):479–499. doi: 10.1007/s10479-017-2489-0. [ CrossRef ] [ Google Scholar ]
- VanBerkel PT, Blake JT. A comprehensive simulation for wait time reduction and capacity planning applied in general surgery. Health Care Management Science. 2007; 10 (4):373–385. doi: 10.1007/s10729-007-9035-6. [ PubMed ] [ CrossRef ] [ Google Scholar ]
- Vargo SL, Akaka MA. Value cocreation and service systems (re) formation: A service ecosystems view. Service Science. 2012; 4 (3):207–217. doi: 10.1287/serv.1120.0019. [ CrossRef ] [ Google Scholar ]
- Viegas, C. V., Bond, A., Vaz, C. R., & Bertolo, R. J. (2019). Reverse flows within the pharmaceutical supply chain: A classificatory review from the perspective of end-of-use and end-of-life medicines. Journal of Cleaner Production , published online, 117719.
- Vissers JM, Adan IJ, Dellaert NP. Developing a platform for comparison of hospital admission systems: An illustration. European Journal of Operational Research. 2007; 180 (3):1290–1301. doi: 10.1016/j.ejor.2006.04.034. [ CrossRef ] [ Google Scholar ]
- Volland J, Fügener A, Schoenfelder J, Brunner JO. Material logistics in hospitals: A literature review. Omega (united Kingdom) 2017; 69 (3):82–101. [ Google Scholar ]
- Yan E. Research dynamics: Measuring the continuity and popularity of research topics. Journal of Informetrics. 2014; 8 (1):98–110. doi: 10.1016/j.joi.2013.10.010. [ CrossRef ] [ Google Scholar ]
- Zabinsky ZB, Dulyakupt P, Zangeneh-Khamooshi S, Xiao C, Zhang P, Kiatsupaibul S, Heim JA. Optimal collection of medical specimens and delivery to central laboratory. Annals of Operations Research. 2020; 287 (1):537–564. doi: 10.1007/s10479-019-03260-9. [ CrossRef ] [ Google Scholar ]
- Zandkarimkhani S, Mina H, Biuki M, Govindan K. A chance constrained fuzzy goal programming approach for perishable pharmaceutical supply chain network design. Annals of Operations Research. 2020 doi: 10.1007/s10479-020-03677-7. [ CrossRef ] [ Google Scholar ]
- Zhang, Y., Wang, Y., Tang, J., & Lim, A. (2020). Mitigating overtime risk in tactical surgical scheduling. Omega, 93 , online 102024.
- Zhang L. Aspect and entity extraction from opinion documents. Springer; 2012. [ Google Scholar ]

IMAGES
VIDEO
COMMENTS
We voice concerns, however, about both the supply of new supply chain scholars and the decreasing authorship of industry practitioners that had been a staple of our applied field. As a further contribution, we organize the most frequent keywords since 2020 to help scholars identify contemporary and understudied topics.
Harvard University aims to be fossil-fuel neutral by 2026 and totally free of fossil fuels by 2050. As part of this goal, the university is trying to decarbonize its supply chain and considers replacing cement with a low-carbon substitute called Pozzotive®, made with post-consumer recycled glass. A successful pilot project could jump start ...
Using Technology to Improve Supply-Chain Resilience. Supply chain management Digital Article. Jonathan Colehower. Companies need to do more than simply invest in building effective supply chains ...
Therefore, managing risk in SCs is an important topic of supply chain management and has been the focus of research through reviews (Ho et al. 2015; Kleindorfer and Saad 2005), ... Research on papers that focus on the ripple effect is dominated by the performance analysis of disruptions probabilities, especially for supplier failure. ...
Green supply chain management practices, supply chain quality practices, innovative management, lean management practices and industry 4.0 technologies are gaining prominence while market and financial performance outcomes of SCM practices have not been adequately researched..Conclusions followed by theoretical and practical implications are ...
Any aspects of global supply chain management. | Explore the latest full-text research PDFs, articles, conference papers, preprints and more on SUPPLY CHAIN MANAGEMENT. Find methods information ...
New research on supply chain management from Harvard Business School faculty on issues including what brands can do to monitor their suppliers' factory conditions, how Japan's earthquake and tsunami and caused havoc on retailers and car manufacturers, and the push to improve labor standards in global supply chains.
As incoming editors a year ago, our 2019 editorial described 14 topic areas deserving of research and professional practical attention in operations and supply chain management (O/SCM), that are still important and deserving of scholarly and practical work (Samson and Kalchschmidt, 2019).While the topics such as supply chain risk, sustainability and new technology have remained relevant, the ...
Supply chain manage ment (SCM) is the int egration of these activities through improved su pply. chain r elationships to achieve sustainable competitiv e advantage. In line with this Christopher ...
The 7 Powerful Supply Chain Trends (I also dubbed "Supply Chain 7.0") have the potential to become a powerful influence over time. These trends, including Augmented reality (AR), Big Data, Gamification of the supply chain, moving supply chain to "Cloud," and Internet of Things (IoT) - Industry 4.0.
Subsequent research has developed new schools of thought, books and articles have been published on the topic, trying to make new contributions to the supply chain topic and areas related with ...
Evidently, design and management of supply chain activities is a primary factor in promoting environmental sustainability. In this paper, we review the current state of academic research in designing and managing sustainable supply chains, and provide a discussion of future directions and research opportunities in this rapidly evolving field.
The STF on integrative literature reviews4 offers an ave-nue to synthesize important L&SCM research streams that create novel empirical, theoretical, or managerial insights. The papers published in 2021 also reflect a variety of data sources and the application of rigorous methodologi-cal approaches. Because of the novelty of many of the phe ...
The literature on healthcare operations and supply chain management has seen unprecedented growth over the past two decades. This paper seeks to advance the body of knowledge on this topic by utilising a topic modelling-based literature review to identify the core topics, examine their dynamic changes, and identify opportunities for further research in the area. Based on an analysis of 571 ...
40 Supply Chain Management Thesis Topics for 2024. On these premises, any supply chain management thesis should be comprehensive. There several topics and areas to consider, and below are 40 Supply Chain Management Thesis Topics for 2020 that students can do research on towards an excellent postgraduate study in SCM.
Get the latest supply chain trends and topics from trusted experts and backed by peer-based learnings with Gartner for Supply Chain. ... Learn key strategies for maximizing the impact of supply chain cost management. Supply chain leaders can use Gartner's research to identify spend reduction opportunities, benchmark current supply chain costs ...
Find the latest published documents for supply chain management, Related hot topics, top authors, the most cited documents, and related journals. ... The paper fills a research gap in the moderating role of the intensity of ICT utilization in increasing financial benefits thanks to the implementation of ECPs in the supply chain. As such, it ...
A supply chain is the set of entities that are involved in the design of new products and services, procuring raw materials, transforming them into semifinished and finished products and ...
Therefore, managing risk in SCs is an important topic of supply chain management and has been the focus of research through reviews (Ho et al. 2015; Kleindorfer and Saad 2005), ... Research on papers that focus on the ripple effect is dominated by the performance analysis of disruptions probabilities, especially for supplier failure. ...
Hence, research topics in procurement and supply chain management offer great scope and variety to students aiming to write up a paper in the discipline. Research topics in procurement management ...
The literature on healthcare operations and supply chain management has seen unprecedented growth over the past two decades. This paper seeks to advance the body of knowledge on this topic by utilising a topic modelling-based literature review to identify the core topics, examine their dynamic changes, and identify opportunities for further research in the area.
The textile supply chain is complex and global, with multiple players involved at every step. From sourcing the raw materials to manufacturing and distribution, the process can span continents. The direction for India is to become an international player playing an integral role in the global supply chain, something that no country has done.
Justin Chimka, associate professor of industrial engineering, was recently named director, while Claudia Rosales, an assistant professor of supply chain management, became co-director. Established in 2017, the center is a collaboration among J.B. Hunt Transport Services Inc., the College of Engineering and Sam M. Walton College of Business.