Mobile Cloud Computing: Issues, Applications and Scope in COVID-19
- Conference paper
- First Online: 27 March 2022
- Cite this conference paper
- Hariket Sukesh Kumar Sheth ORCID: orcid.org/0000-0001-5283-7716 15 &
- Amit Kumar Tyagi ORCID: orcid.org/0000-0003-2657-8700 16
Part of the book series: Lecture Notes in Networks and Systems ((LNNS,volume 418))
Included in the following conference series:
- International Conference on Intelligent Systems Design and Applications
1933 Accesses
12 Citations
As the world is transitioning into a tech-savvy era, the twenty-first century is evidence of many technological advancements in the field of AI, IoT, ML, etc. Mobile Cloud computing (MCC) is one such emerging technology, providing services regardless of the time and place, contours the limitations of mobile devices to process bulk data, providing multi-platform support and dynamic provisioning. Not only there is an enhancement in computation speed, energy efficiency, execution, integration, but also incorporates considerate issues in terms of client-to-cloud and cloud-to-client authentication, privacy, trust, and security. Reviewing and overcoming addressed concerns is essential to provide reliable yet efficient service in nearing future. Mobile Cloud Computing has the potential to bring wonders in the fields such as education, medical science, biometry, forensics, and automobiles, which could counter the challenges faced in the ongoing COVID-19 Pandemic. To combat the prevailing challenges due to COVID-19, it has become critical that more efficient and specialized technologies like Mobile Cloud Computing are accepted that enable appropriate reach and delivery of vital services by involving gamification, cloud rendering, and collaborative practices. This paper provides a detailed study about MCC, mitigated security and deployment attacks, issues, applications of MCC, providing developers and practitioners opportunities for future enhancements.
This is a preview of subscription content, log in via an institution to check access.

Access this chapter
Subscribe and save.
- Get 10 units per month
- Download Article/Chapter or eBook
- 1 Unit = 1 Article or 1 Chapter
- Cancel anytime
- Available as PDF
- Read on any device
- Instant download
- Own it forever
- Available as EPUB and PDF
- Compact, lightweight edition
- Dispatched in 3 to 5 business days
- Free shipping worldwide - see info
Tax calculation will be finalised at checkout
Purchases are for personal use only
Institutional subscriptions
Similar content being viewed by others
Security Frameworks for Green Mobile Cloud Computing
Security Frameworks in Mobile Cloud Computing
A Review on Mobile Cloud Computing
Conflict of interest.
The authors have thoroughly discussed about the contents of these research paper and declare that they surely do not have any conflicts of interest.
Shahzad, A., Hussain, M: Security issues and challenges of mobile cloud computing. Int. J. Grid Distrib. Comput. 6 , 37–50 (2013). www.sersc.org
Mobile Cloud Market – Growth, Trends, COVID-19 Impact, and Forecasts (2021–26). https://www.mordorintelligence.com/industry-reports/global-mobile-cloud-market-industry
Nguyen, D.C., Pathirana, P.N., Ding, M., Seneviratne, A.: Blockchain for secure EHRs sharing of mobile cloud based E-Health systems. IEEE Access 7 , 66792–66806 (2019). https://doi.org/10.1109/ACCESS.2019.2917555
Article Google Scholar
Dubovitskaya, A., Xu, Z., Ryu, S., Schumacher, M., Wang, F.: Secure and trustable electronic medical records sharing using blockchain. AMIA Annu. Symp. Proc. 2017 , 650–659 (2018). PMID: 29854130; PMCID: PMC5977675
Google Scholar
Holbl, M., Kompara, M., Kamisalic, A., Zlatolas, L.N.: A systematic review of the use of blockchain in healthcare. Symmetry 10 (10), 470 (2018). https://doi.org/10.3390/sym10100470
Elazhary, H.: Internet of Things (IoT), mobile cloud, cloudlet, mobile IoT, IoT cloud, fog, mobile edge, and edge emerging computing paradigms: disambiguation and research directions. J. Netw. Comput. Appl. 128 , 105–140 (2019). https://doi.org/10.1016/j.jnca.2018.10.021
Eom, H., Figueiredo, R., Cai, H., Zhang, Y., Huang, G.: MALMOS: machine learning-based mobile offloading scheduler with online training. In: 2015 3rd IEEE International Conference on Mobile Cloud Computing, Services, and Engineering, pp. 51–60 (2015). https://doi.org/10.1109/MobileCloud.2015.19
Talavera, L.E., Endler, M., Vasconcelos, I., Vasconcelos, R., Cunha, M., Silva, F.J.d.S.e.: The mobile hub concept: enabling applications for the internet of mobile things. In: 2015 IEEE International Conference on Pervasive Computing and Communication Workshops (PerCom Workshops), pp. 123–128 (2015). https://doi.org/10.1109/PERCOMW.2015.7134005
Hernandez, L., Cao, H., Wachowicz, M.: Implementing an Edge-fog-cloud Architecture for Stream Data Management. Cornell University Library (2017). https://arxiv.org/abs/1708.00352
UST Global. Internet of Medical Things (IoMT) Connecting Healthcare for a Better Tomorrow (2017). https://www.ust-global.com/sites/default/files/internet_of_medical_things_iomt.pdf
Alvi, S.A., Shah, G.A., Mahmood, W.: Energy efficient green routing protocol for internet of multimedia things. In: 2015 IEEE Tenth International Conference on Intelligent Sensors, Sensor Networks and Information Processing (ISSNIP), pp. 1–6 (2015). https://doi.org/10.1109/ISSNIP.2015.7106958
Amit Kumar, T., Shamila, M.: Spy in the crowd: how user’s privacy is getting affected with the integration of Internet of Thing’s devices (March 20, 2019). In: Proceedings of International Conference on Sustainable Computing in Science, Technology and Management (SUSCOM), Amity University Rajasthan, Jaipur - India, February 26-28, 2019
Kosta, S., Aucinas, A., Hui, P., Mortier, R., Zhang, X.: ThinkAir: dynamic resource allocation and parallel execution in the cloud for mobile code offloading. Proc. IEEE INFOCOM 2012 , 945–953 (2012)
Gai, K., Qiu, M., Zhao, H., Tao, L., Zong, Z.: Dynamic energy-aware cloudlet-based mobile cloud computing model for green computing. J. Netw. Comput. Appl. 59 , 46–54 (2016)
Banerjee, X., Chen, J.E., Gopalakrishnan, V., Lee, S., Van Der Merwe, J.: MOCA. In: Proceedings of the Eighth ACM International Workshop on Mobility in the Evolving Internet architecture, pp. 11–16, ACM, Miami, FL, USA (2013)
Lin, Y., Kämäräinen, T., Di Francesco, M., Ylä-Jääski, A.: Performance evaluation of remote display access for mobile cloud computing. Comput. Commun. 72 , 17–25 (2015). https://doi.org/10.1016/j.comcom.2015.05.006
Khan, A.N., Kiah, M.L., Ali, M., Shamshirband, S.: A cloud-manager-based re-encryption scheme for mobile users in cloud environment: a hybrid approach. J. Grid Comput. 13 (4), 651–675 (2015)
Kaur, S., Sohal, H.S.: Hybrid application partitioning and process offloading method for the mobile cloud computing. Proc. First Int. Conf. Intell. Comput. Commun. 87 , 95 (2016). https://doi.org/10.1007/978-981-10-2035-3_10
Gu, Y., March, V., Sung Lee, B.: GMoCA: Workshop on Green and Sustainable Software GREENS), Zurich, 3–3 June 2012, pp 15–20, Print ISBN: 978-1-4673-1833-4. https://doi.org/10.1109/GREENS.2012.6224265
Cao, J., Yu, B., Dong, F., Zhu, X., Xu, S.: Entropy-based denial-of-service attack detection in cloud data center. Concurrency Computat. Pract Exper. 27 , 5623–5639 (2015). https://doi.org/10.1002/cpe.3590
Tong, Z., Zhu, Z., Wang, Z., Wang, L., Zhang, Y., Liu, Y.: Cache side-channel attacks detection based on machine learning. In: 2020 IEEE 19th International Conference on Trust, Security and Privacy in Computing and Communications (TrustCom), pp. 919–926 (2020). https://doi.org/10.1109/TrustCom50675.2020.00123
Donald, A.C., Arockiam, L., Kalaimani, S.: ORBUA: An effective data access model for MobiCloud environment. Int. J. Pure Appl. Math. 118 , 79–84 (2018)
Bhatia, T., Verma, A.K., Sharma, G.: Towards a secure incremental proxy re-encryption for e-healthcare data sharing in mobile cloud computing. Concurr. Computat. Pract. Exper. 32 , e5520 (2020). https://doi.org/10.1002/cpe.5520
Irshad, A., Chaudhry, S.A., Shafiq, M., Usman, M., Asif, M., Ghani, A.: A provable and secure mobile user authentication scheme for mobile cloud computing services. Int. J. Commun. Syst. 32 , e3980 (2019). https://doi.org/10.1002/dac.3980
Yadav, H., Dave, M.: Secure data storage operations with verifiable outsourced decryption for mobile cloud computing. In: International Conference on Recent Advances and Innovations in Engineering (ICRAIE-2014), pp. 1–5 (2014). https://doi.org/10.1109/ICRAIE.2014.6909236
Rashidi, O., Azzahra Mohd Zaifuddin, F., Mohd Hassan, Z.: Carotenoid biosynthesis regulatory mechanisms in plants. J. Oleo Sci. 63 (8), 753–760 (2014). Released July 29, 2014, [Advance publication] Released July 14, 2014, Online ISSN 1347-3352, Print ISSN 1345-8957 https://doi.org/10.5650/jos.ess13183
Mathew, S.M.: Implementation of cloud computing in education - a revolution. Int. J. Comput. Theory Eng. 4 , 473–475 (2012). https://doi.org/10.7763/IJCTE.2012.V4.511
Agrawal, S.: A survey on recent applications of cloud computing in education: COVID-19 perspective. J. Phys. Conf. Ser. 1828 (1), 012076 (2021). https://doi.org/10.1088/1742-6596/1828/1/012076
Dadhich, P., Kavita, Dr.: Cloud Computing Impact on Healthcare Services During COVID-19 Pandemic (2021)
Popadina, O., Salah, A.-M., Jalal, K.: Voice analysis framework for asthma-COVID-19 early diagnosis and prediction: AI-based mobile cloud computing application. IEEE Conf. Russian Young Res. Electr. Electron. Eng. (ElConRus) 2021 , 1803–1807 (2021). https://doi.org/10.1109/ElConRus51938.2021.9396367
Sharma, A., Bahl, S., Bagha, A.K., Javaid, M., Shukla, D.K., Haleem, A.: Blockchain technology and its applications to combat COVID-19 pandemic. Res. Biomed. Eng. 1 , 8 (2020). https://doi.org/10.1007/s42600-020-00106-3
Download references
Acknowledgement
We thank our college, Vellore Institute of Technology, Chennai for their constant support, encouragement and support. The authors have properly acknowledged and cited the scholars whose articles or content were consulted for this manuscript. The authors extend their gratitude to authors/editors/publishers of all those articles, journals and books. All the diagrams/figures/illustrations used and added in the paper are made using Open-Source software, ensuring no copyright issues.
Author information
Authors and affiliations.
School of Computer Science and Engineering, Vellore Institute of Technology, Chennai, Tamil Nadu, 600127, India
Hariket Sukesh Kumar Sheth
Centre for Advanced Data Science, Vellore Institute of Technology, Chennai, Tamil Nadu, 600127, India
Amit Kumar Tyagi
You can also search for this author in PubMed Google Scholar
Contributions
All persons who meet authorship criteria are listed as authors and took the public responsibility for the content, including participation in the concept, analysis, writing, and revision of the manuscript. All authors revised and gave final approval for the version submitted.
Corresponding author
Correspondence to Hariket Sukesh Kumar Sheth .
Editor information
Editors and affiliations.
Scientific Network for Innovation and Research Excellence, Machine Intelligence Research Labs (MIR Labs), Auburn, WA, USA
Ajith Abraham
Niketa Gandhi
Institut für Wirtschaftsinformatik, Fachhochschule Nordwestschweiz, Olten, Switzerland
Thomas Hanne
Department of Computer Science and information Engineering, National University of Kaohsiung, Kaohsiung, Taiwan
Tzung-Pei Hong
Federal University of Bahia, Ondina, Brazil
Tatiane Nogueira Rios
Nantong University, Nantong Shi, Jiangsu, China
Weiping Ding
Rights and permissions
Reprints and permissions
Copyright information
© 2022 The Author(s), under exclusive license to Springer Nature Switzerland AG
About this paper
Cite this paper.
Sheth, H.S.K., Tyagi, A.K. (2022). Mobile Cloud Computing: Issues, Applications and Scope in COVID-19. In: Abraham, A., Gandhi, N., Hanne, T., Hong, TP., Nogueira Rios, T., Ding, W. (eds) Intelligent Systems Design and Applications. ISDA 2021. Lecture Notes in Networks and Systems, vol 418. Springer, Cham. https://doi.org/10.1007/978-3-030-96308-8_55
Download citation
DOI : https://doi.org/10.1007/978-3-030-96308-8_55
Published : 27 March 2022
Publisher Name : Springer, Cham
Print ISBN : 978-3-030-96307-1
Online ISBN : 978-3-030-96308-8
eBook Packages : Intelligent Technologies and Robotics Intelligent Technologies and Robotics (R0)
Share this paper
Anyone you share the following link with will be able to read this content:
Sorry, a shareable link is not currently available for this article.
Provided by the Springer Nature SharedIt content-sharing initiative
- Publish with us
Policies and ethics
- Find a journal
- Track your research
Mobile Systems
Mobile devices are the prevalent computing device in many parts of the world, and over the coming years it is expected that mobile Internet usage will outpace desktop usage worldwide. Google is committed to realizing the potential of the mobile web to transform how people interact with computing technology. Google engineers and researchers work on a wide range of problems in mobile computing and networking, including new operating systems and programming platforms (such as Android and ChromeOS); new interaction paradigms between people and devices; advanced wireless communications; and optimizing the web for mobile settings. In addition, many of Google’s core product teams, such as Search, Gmail, and Maps, have groups focused on optimizing the mobile experience, making it faster and more seamless. We take a cross-layer approach to research in mobile systems and networking, cutting across applications, networks, operating systems, and hardware. The tremendous scale of Google’s products and the Android and Chrome platforms make this a very exciting place to work on these problems.
Some representative projects include mobile web performance optimization, new features in Android to greatly reduce network data usage and energy consumption; new platforms for developing high performance web applications on mobile devices; wireless communication protocols that will yield vastly greater performance over today’s standards; and multi-device interaction based on Android, which is now available on a wide variety of consumer electronics.
Recent Publications
Some of our teams.
We're always looking for more talented, passionate people.
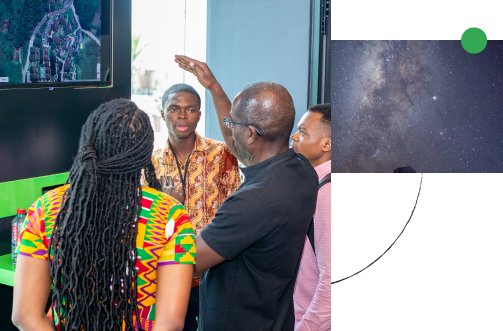
IEEE Account
- Change Username/Password
- Update Address
Purchase Details
- Payment Options
- Order History
- View Purchased Documents
Profile Information
- Communications Preferences
- Profession and Education
- Technical Interests
- US & Canada: +1 800 678 4333
- Worldwide: +1 732 981 0060
- Contact & Support
- About IEEE Xplore
- Accessibility
- Terms of Use
- Nondiscrimination Policy
- Privacy & Opting Out of Cookies
A not-for-profit organization, IEEE is the world's largest technical professional organization dedicated to advancing technology for the benefit of humanity. © Copyright 2024 IEEE - All rights reserved. Use of this web site signifies your agreement to the terms and conditions.
mobile computing Recently Published Documents
Total documents.
- Latest Documents
- Most Cited Documents
- Contributed Authors
- Related Sources
- Related Keywords

Location-Based Internationalization and Localization With Mobile Computing
The Android system operates on many smartphones in many locales. Websites and web tools have their own requirements in day-to-day life. To reach the maximum users, the app and website should handle all the resources such as text strings, functions, layouts, graphics, and any other static data that the app/website needs. It requires internationalization and localization of the website and app to support multiple languages. The basic idea of this chapter is to present an approach for localizing the Android application according to the location data that the app received from the device, but many users do not allow the “access location” feature so this approach will be a dead end in this case. The authors have proposed some other techniques to achieve this feature of localization and internationalization by implementing the “choose language” service so that the app can itself optimize its content and translate it into the user's native language.
Why Does Privacy Paradox Exist?
In this exploratory study, the main aim was to find, ‘why do people disclose information when they are concerned about their privacy?’. The reasons that provide a plausible explanation to the privacy paradox have been conjectural. From the analysis of the eighteen in-depth interviews using grounded theory, themes were then conceptualized. We found rational and irrational explanations in terms of cognitive biases and heuristics that explain the privacy paradox among mobile users. We figured out some reasons in this context of mobile computing which were not emphasized earlier in the privacy paradox literature such as Peanut Effect, Fear of Missing Out- FoMo, Learned Helplessness, and Neophiliac Personality. These results add to the privacy paradox discourse and provide implications for smartphone users for making privacy-related decisions more consciously rather than inconsiderately disclosing information. Also, the results would help marketers and policymakers design nudges and choice architectures that consider privacy decision-making hurdles.
Database Recovery Technique for Mobile Computing: A Game Theory Approach
Intelligent multilevel node authentication in mobile computing using clone node, 2021 index ieee transactions on mobile computing vol. 20, mobile computing and sustainable informatics, computer mathematical modeling based on the improved genetic algorithm and mobile computing.
In order to change the problem of data redundancy in a genetic algorithm, this paper proposes a computer mathematical model based on the combination of an improved genetic algorithm and mobile computing. Combined with the least square method, MATLAB software is used to solve the equations, determine the range of parameters, and solve the estimation parameter range and identification problems. The improved genetic algorithm combined with mobile computing and least square method to establish a mathematical model greatly increased the individual search space and increased the operation rate of 90% compared to the basic genetic algorithm or mobile computing. The results show that the improved genetic algorithm and mobile computing have a certain ability to identify the optimal solution and greatly improve the work efficiency.
Integrated Fan‐Out ( InFO ) for Mobile Computing
Mobile computing as a support to teach the concept of function.
We make a proposal to teach the concept of function using mobile computing. This proposal is based on research that has support in education theories such as Constructivism and Problem Solving Learning. In the first part we show the difficulties that undergraduate Computer Science students have in the first semester of Engineering while working with that mathematical concept. And application to be used by the students anywhere and anytime in mobile devices is designed that process data and is intended to show them the concept of function in a problem solving situation. The proposed activities are part of the education methodology used in the research and within this paper we show the diagnostic questionnaire as one of the methodological tools and its results supporting the designed activities and its application to mobile devices
Prediction of Mineralization Prospects Based on Geological Semantic Model and Mobile Computer Machine Learning
Mineral resources are indispensable in the development of human society and are the foundation of national economic development. As the prospecting target shifts from outcrop ore to concealed ore, from shallow to deep, the difficulty of prospecting becomes more and more difficult. Therefore, the prediction of mineralization prospects is of great significance. This paper is aimed at completing the prediction of mineralization prospects by constructing geological semantic models and using mobile computer learning to improve the accuracy of prediction of mineralization prospects and expanding the application of semantic mobile computing. We use five different semantic relations to build a semantic knowledge library, realize semantic retrieval, complete information extraction of geological text data, and study mineral profiles. Through the distributed database of mobile computing, the association rules and random forest algorithm are used to describe the characteristics of minerals and the ore-controlling elements, find the association rules, and finally combine the geological and mineral data of the area and use the random forest algorithm to realize the prospect of mineralization district forecast. The geological semantic model constructed in the article uses the knowledge library for associative search to achieve an accuracy rate of 87.9% and a recall rate of 96.5%. The retrieval effect is much higher than that of traditional keyword retrieval methods. The maximum value of the posterior result of the mineralization prospect is 0.9027, the average value is 0.0421, and the standard deviation is 0.1069. The picture is brighter, and the probability of mineralization is higher.
Export Citation Format
Share document.
- DOI: 10.1007/s11227-024-06395-0
- Corpus ID: 271774713
Multiple heterogeneous cluster-head-based secure data collection in mobile crowdsensing environment
- R. Sahoo , S. Pradhan , +1 author S. Udgata
- Published in Journal of Supercomputing 7 August 2024
- Computer Science, Engineering, Environmental Science
5 References
Arabertopic: a neural topic modeling approach for news extraction from arabic facebook pages using pre-trained bert transformer model, security analysis of mobile crowd sensing applications, sensecrypt: a security framework for mobile crowd sensing applications, privacy protection in mobile crowd sensing: a survey, mobile crowd sensing and computing, related papers.
Showing 1 through 3 of 0 Related Papers
The Impact of Cloud Computing and AI on Industry Dynamics and Concentration
We examine the rise of cloud computing and AI in China and their impacts on industry dynamics after the shock to the cost of Internet-based computing power and services. We find that cloud computing is associated with an increase in firm entry, exit and the likelihood of M&A in industries that depend more on cloud infrastructure. Conversely, AI adoption has no impact on entry but reduces the likelihood of exit and M&A. Firm size plays a crucial role in these dynamics: cloud computing increases exit rates across all firms, while larger firms benefit from AI, experiencing reduced exit rates. Cloud computing decreases industry concentration but AI increases concentration. On the financing side, firms exposed to cloud computing increase equity and venture capital financing, while only large firms increase equity financing when exposed to AI.
We thank Heitor Almeida, Tanya Babina, Jianwei Xing, Keer Yang, and Michael Ewens and seminar participants at the Australia Banking and Finance Conference, the Finance and Organizations (FOM) Conference, the 2023 China Financial Research Conference, the 2024 Midwest Finance Association Conference, Tel Aviv University, and Tsinghua University for their helpful comments. The views expressed herein are those of the authors and do not necessarily reflect the views of the National Bureau of Economic Research.
MARC RIS BibTeΧ
Download Citation Data
Working Groups
More from nber.
In addition to working papers , the NBER disseminates affiliates’ latest findings through a range of free periodicals — the NBER Reporter , the NBER Digest , the Bulletin on Retirement and Disability , the Bulletin on Health , and the Bulletin on Entrepreneurship — as well as online conference reports , video lectures , and interviews .
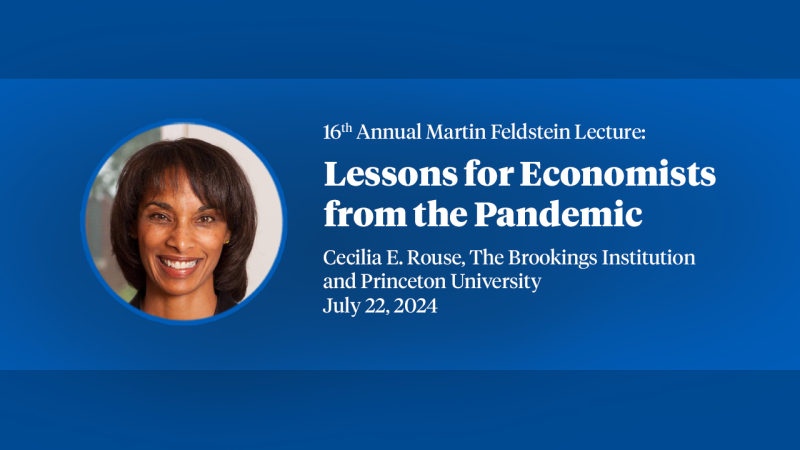
Information
- Author Services
Initiatives
You are accessing a machine-readable page. In order to be human-readable, please install an RSS reader.
All articles published by MDPI are made immediately available worldwide under an open access license. No special permission is required to reuse all or part of the article published by MDPI, including figures and tables. For articles published under an open access Creative Common CC BY license, any part of the article may be reused without permission provided that the original article is clearly cited. For more information, please refer to https://www.mdpi.com/openaccess .
Feature papers represent the most advanced research with significant potential for high impact in the field. A Feature Paper should be a substantial original Article that involves several techniques or approaches, provides an outlook for future research directions and describes possible research applications.
Feature papers are submitted upon individual invitation or recommendation by the scientific editors and must receive positive feedback from the reviewers.
Editor’s Choice articles are based on recommendations by the scientific editors of MDPI journals from around the world. Editors select a small number of articles recently published in the journal that they believe will be particularly interesting to readers, or important in the respective research area. The aim is to provide a snapshot of some of the most exciting work published in the various research areas of the journal.
Original Submission Date Received: .
- Active Journals
- Find a Journal
- Proceedings Series
- For Authors
- For Reviewers
- For Editors
- For Librarians
- For Publishers
- For Societies
- For Conference Organizers
- Open Access Policy
- Institutional Open Access Program
- Special Issues Guidelines
- Editorial Process
- Research and Publication Ethics
- Article Processing Charges
- Testimonials
- Preprints.org
- SciProfiles
- Encyclopedia
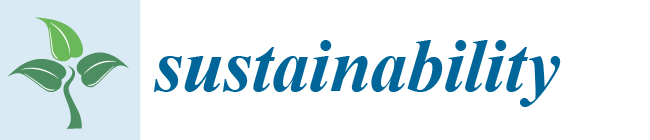
Article Menu

- Subscribe SciFeed
- Recommended Articles
- Author Biographies
- Google Scholar
- on Google Scholar
- Table of Contents
Find support for a specific problem in the support section of our website.
Please let us know what you think of our products and services.
Visit our dedicated information section to learn more about MDPI.
JSmol Viewer
The role of 6g technologies in advancing smart city applications: opportunities and challenges.
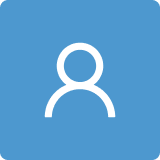
1. Introduction
- Literature Survey and Review Process
- Step-1: Literature Retrieval
- Step-2: Literature Filtering
- Step-3: Classification
- Through this article, we have extensively covered in detail the potential 6G technologies in terms of requirements, architecture, visions, and usage, which are anticipated to be integrated in futuristic 6G-enabled smart cities.
- Secondly, we have discussed prominent smart city applications with underlying 6G technologies. This includes smart waste management, smart healthcare, smart grids, and more.
- Thirdly, potential challenges are also highlighted along with discussion on each technology and application. Also, at the end of this survey paper, challenges and suggestions for possible future research directions are also highlighted for the 6G-enabled smart city paradigm.
- Research Objectives
- Structure of Paper
2. Potential 6G Enabling Technologies
2.1. role of ai in 6g and smart city arena, 2.1.1. applications, 2.1.2. challenges, 2.2. role of integrated sensing and communication (isac) in smart city concept, 2.2.1. applications, 2.2.2. challenges, 2.3. iot for smart cities with 6g, 2.3.1. characteristics of 6g-iot, 2.3.2. classification of iot, 2.4. blockchain (bc) and 6g-enabled smart cities, 2.5. terahertz (thz) communication, 2.5.1. applications/use cases, 2.5.2. challenges, 2.6. quantum communication (qc), 2.6.1. applications, 2.6.2. challenges, 2.7. immersive communication (ic), 2.7.1. types of immersive communication, 2.7.2. use cases for immersive communication, 2.8. visible light communication (vlc), 2.8.1. free-space optics (fso), 2.8.2. fiber-wireless system (fiwi), 2.8.3. power over fiber (pof), 2.8.4. challenges, 2.9. mobile edge computing (mec), applications, 2.10. reconfigurable intelligent surfaces (riss), 2.11. non-terrestrial networks (ntns), 2.11.1. airborne base stations (abs), uavs, and drones uses in a 6g smart city, applications/benefits, 2.11.2. satellite communication, 3. applications of 6g in smart cities, 3.1. industrial automation and smart manufacturing, 3.2. vehicle-to-everything (v2x) technology in smart cities, use cases of v2x, 3.3. smart healthcare, 3.4. smart grid, 3.5. smart waste management, 4. conclusions, open challenges and possible future research, conflicts of interest.
- Xin, B.; Qu, Y. Effects of smart city policies on green total factor productivity: Evidence from a quasi-natural experiment in China. Int. J. Environ. Res. Public Health 2019 , 16 , 2396. [ Google Scholar ] [ CrossRef ] [ PubMed ]
- Antwi-Afari, P.; Owusu-Manu, D.G.; Simons, B.; Debrah, C. Ato Ghansah Sustainability guidelines to attaining smart sustainable cities in developing countries: A ghanaian context Sustain. Futures 2021 , 3 , 100044. [ Google Scholar ]
- UN [United Nations]. The 2030 Agenda for Sustainable Development ; UN [United Nations]: San Francisco, CA, USA, 2015. [ Google Scholar ]
- Cartalis, C. Toward resilient cities—A review of definitions, challenges and prospects. Adv. Build. Energy Res. 2014 , 8 , 259–266. [ Google Scholar ] [ CrossRef ]
- Voytenko, Y.; Mccormick, K.; Evans, J.; Schliwa, G. Urban living labs for sustainability and low carbon cities in Europe: Towards a research agenda. J. Clean. Prod. 2016 , 123 , 45–54. [ Google Scholar ] [ CrossRef ]
- Sharifi, A. A typology of smart city assessment tools and indicator sets. Sustain. Cities Soc. 2020 , 53 , 101936. [ Google Scholar ] [ CrossRef ]
- Weber-Lewerenz, B.; Traverso, M. Navigating Applied Artificial Intelligence (AI) in the Digital Era: How Smart Buildings and Smart Cities Become the Key to Sustainability. J. Artif. Intell. Appl. (AIA) 2023 , 1 , 230–243. [ Google Scholar ] [ CrossRef ]
- Blasi, S.; Ganzaroli, A.; De Noni, I. Smartening Sustainable Development in Cities: Strengthening the Theoretical Linkage between Smart Cities and SDGs. Sustain. Cities Soc. 2022 , 80 , 103793. [ Google Scholar ] [ CrossRef ]
- Smart Cities Market—Global Industry Analysis, Size, Share, Growth, Trends, Regional Outlook, and Forecast 2023–2033. Available online: https://www.precedenceresearch.com/smart-cities-market (accessed on 27 July 2024).
- Ishteyaq, I.; Muzaffar, K.; Shafi, N.; Alathbah, M.A. Unleashing the Power of Tomorrow: Exploration of Next Frontier with 6G Networks and Cutting Edge Technologies. IEEE Access 2024 , 12 , 29445–29463. [ Google Scholar ] [ CrossRef ]
- Tariq, F.; Khandaker, M.R.; Wong, K.-K.; Imran, M.A.; Bennis, M.; Debbah, M. A speculative study on 6G. IEEE Wirel. Commun. 2020 , 27 , 118–125. [ Google Scholar ] [ CrossRef ]
- IMT Traffic Estimates for the Years 2020 to 2030, Document ITU 0-2370. 2015. Available online: https://www.itu.int/dms_pub/itu-r/opb/rep/R-REP-M.2370-2015-PDF-E.pdf (accessed on 27 July 2024).
- ITU. IMT towards 2030 and Beyond. 2023. Available online: https://www.itu.int/en/ITU-R/study-groups/rsg5/rwp5d/imt-2030/Pages/default.aspx (accessed on 27 July 2024).
- Dang, S.; Amin, O.; Shihada, B.; Alouini, M.-S. What should 6G be? Nat. Electron. 2020 , 3 , 20–29. [ Google Scholar ] [ CrossRef ]
- Zhang, Z.; Xiao, Y.; Ma, Z.; Xiao, M.; Ding, Z.; Lei, X.; Karagiannidis, G.K.; Fan, P. 6G wireless networks: Vision, requirements, architecture, and key technologies. IEEE Veh. Technol. Mag. 2019 , 14 , 28–41. [ Google Scholar ] [ CrossRef ]
- Kugley, S.; Wade, A.; Thomas, J.; Mahood, Q.; Anne-Marie Klint, J.; Hammerstrøm, K.; Sathe, N. Searching for studies: A guide to information retrieval for Campbell systematic reviews. Campbell Syst. Rev. 2017 , 13 , 1–73. [ Google Scholar ] [ CrossRef ]
- Fong, B.; Kim, H.; Fong, A.C.M.; Hong, G.Y.; Tsang, K.F. Reliability Optimization in the Design and Implementation of 6G Vehicle-to-Infrastructure Systems for Emergency Management in a Smart City Environment. IEEE Commun. Mag. 2023 , 61 , 148–153. [ Google Scholar ] [ CrossRef ]
- Mishra, P.; Singh, G. 6G-IoT Framework for Sustainable Smart City: Vision and Challenges. IEEE Consum. Electron. Mag. 2024 , 13 , 93–103. [ Google Scholar ] [ CrossRef ]
- Parvaresh, N.; Kantarci, B. A Continuous Actor–Critic Deep Q-Learning-Enabled Deployment of UAV Base Stations: Toward 6G Small Cells in the Skies of Smart Cities. IEEE Open J. Commun. Soc. 2023 , 4 , 700–712. [ Google Scholar ] [ CrossRef ]
- Yang, Z.; Hu, D.; Guo, Q.; Zuo, L.; Ji, W. Visual E2C: AI-Driven Visual End-Edge-Cloud Architecture for 6G in Low-Carbon Smart Cities. IEEE Wirel. Commun. 2023 , 30 , 204–210. [ Google Scholar ] [ CrossRef ]
- Sehito, N.; Shouyi, Y.; Alshahrani, H.M.; Alamgeer, M.; Dutta, A.K.; Alsubai, S.; Nkenyereye, L.; Dhanaraj, R.K. Optimizing User Association, Power Control and Beamforming for 6G Multi-IRS Multi-UAV NOMA Communications in Smart Cities. IEEE Trans. Consum. Electron. 2024 . [ Google Scholar ] [ CrossRef ]
- Singh, P.R.; Singh, V.K.; Yadav, R.; Chaurasia, S.N. 6G networks for artificial intelligence-enabled smart cities applications: A scoping review. Telemat. Inform. Rep. 2023 , 9 , 100044. [ Google Scholar ] [ CrossRef ]
- Murroni, M.; Anedda, M.; Fadda, M.; Ruiu, P.; Popescu, V.; Zaharia, C.; Giusto, D. 6G—Enabling the New Smart City: A Survey. Sensors 2023 , 23 , 7528. [ Google Scholar ] [ CrossRef ]
- Kamruzzaman, M.M. Key Technologies, Applications and Trends of Internet of Things for Energy-Efficient 6G Wireless Communication in Smart Cities. Energies 2022 , 15 , 5608. [ Google Scholar ] [ CrossRef ]
- Kim, N.; Kim, G.; Shim, S.; Jang, S.; Song, J.; Lee, B. Key Technologies for 6G-Enabled Smart Sustainable City. Electronics 2024 , 13 , 268. [ Google Scholar ] [ CrossRef ]
- Ismail, L.; Buyya, R. Artificial Intelligence Applications and Self-Learning 6G Networks for Smart Cities Digital Ecosystems: Taxonomy, Challenges, and Future Directions. Sensors 2022 , 22 , 5750. [ Google Scholar ] [ CrossRef ]
- Qadir, Z.; Le, K.N.; Saeed, N.; Munawar, H.S. Towards 6G Internet of Things: Recent advances, use cases, and open challenges. ICT Express 2023 , 9 , 296–312. [ Google Scholar ] [ CrossRef ]
- Shafi, M.; Jha, R.K.; Jain, S. 6G: Technology Evolution in Future Wireless Networks. IEEE Access 2024 , 12 , 57548–57573. [ Google Scholar ] [ CrossRef ]
- Lombardi, P.; Giordano, S.; Farouh, H.; Yousef, W. Modelling the smart city performance. Innovation 2012 , 25 , 137–149. [ Google Scholar ] [ CrossRef ]
- Talebkhah, M.; Sali, A.; Marjani, M.; Gordan, M.; Hashim, S.J.; Rokhani, F.Z. IoT and Big Data Applications in Smart Cities: Recent Advances, Challenges, and Critical Issues. IEEE Access 2021 , 9 , 55465–55484. [ Google Scholar ] [ CrossRef ]
- Singh, T.; Solanki, A.; Sharma, S.K.; Nayyar, A.; Paul, A. A Decade Review on Smart Cities: Paradigms, Challenges and Opportunities. IEEE Access 2022 , 10 , 68319–68364. [ Google Scholar ] [ CrossRef ]
- Farooq, M.S.; Nadir, R.M.; Rustam, F.; Hur, S.; Park, Y.; Ashraf, I. Nested Bee Hive: A Conceptual Multilayer Architecture for 6G in Futuristic Sustainable Smart Cities. Sensors 2022 , 22 , 5950. [ Google Scholar ] [ CrossRef ] [ PubMed ]
- Zhu, Y.; Mao, B.; Kato, N. Intelligent Reflecting Surface in 6G Vehicular Communications: A Survey. IEEE Open J. Veh. Technol. 2022 , 3 , 266–277. [ Google Scholar ] [ CrossRef ]
- Hijji, M.; Iqbal, R.; Pandey, A.K.; Doctor, F.; Karyotis, C.; Rajeh, W.; Alshehri, A.; Aradah, F. 6G Connected Vehicle Framework to Support Intelligent Road Maintenance Using Deep Learning Data Fusion. IEEE Trans. Intell. Transp. Syst. 2023 , 24 , 7726–7735. [ Google Scholar ] [ CrossRef ]
- Kamruzzaman, M.; Hossin, A.; Alruwaili, O.; Alanazi, S.; Alruwaili, M.; Alshammari, N.; Alaerjan, A.; Zaman, R. IoT-Oriented 6G Wireless Network System for Smart Cities. Comput. Intell. Neurosci. 2022 , 2022 , 4436. [ Google Scholar ] [ CrossRef ] [ PubMed ]
- Bekkali, A.E.; Essaaidi, M.; Boulmalf, M. A Blockchain-Based Architecture and Framework for Cybersecure Smart Cities. IEEE Access 2023 , 11 , 76359–76370. [ Google Scholar ] [ CrossRef ]
- Samanta, S.; Sarkar, A.; Bulo, Y. Secure 6G Communication in Smart City Using Blockchain. In Proceedings of the Emerging Technologies in Data Mining and Information Security, Singapore, 4–10 May 2023; Dutta, P., Chakrabarti, S., Bhattacharya, A., Dutta, S., Piuri, V., Eds.; Springer Nature: Singapore, 2023; pp. 487–496. [ Google Scholar ]
- Tekale, S.; Rajagopal, R.; Bhoopathy, V. 6G: Transformation of Smart Cities with Blockchain and AI. In Challenges and Risks Involved in Deploying 6G and NextGen Networks ; IGI Global: Hershey, PA, USA, 2022; pp. 220–228. [ Google Scholar ]
- Yadav, M.; Agarwal, U.; Rishiwal, V.; Tanwar, S.; Kumar, S.; Alqahtani, F.; Tolba, A. Exploring Synergy of Blockchain and 6G Network for Industrial Automation. IEEE Access 2023 , 11 , 137163–137187. [ Google Scholar ] [ CrossRef ]
- Mohammed, N.J. Quantum cryptography in Convolution neural network approach in Smart cities. J. Surv. Fish. Sci. 2023 , 10 , 2043–2056. [ Google Scholar ]
- Muheidat, F.; Dajani, K.; Tawalbeh, L.A. Security Concerns for 5G/6G Mobile Network Technology and Quantum Communication. Procedia Comput. Sci. 2022 , 203 , 32–40. [ Google Scholar ] [ CrossRef ]
- Ali, M.Z.; Abohmra, A.; Usman, M.; Zahid, A.; Heidari, H.; Imran, M.A.; Abbasi, Q.H. Quantum for 6G communication: A perspective. IET Quantum Commun. 2023 , 10 , 12391. [ Google Scholar ] [ CrossRef ]
- Manzalini, A. Quantum communications in future networks and services. Quantum Rep. 2020 , 2 , 221–232. [ Google Scholar ] [ CrossRef ]
- Jahid, A.; Alsharif, M.H.; Hall, T.J. The convergence of Blockchain, IoT and 6G: Potential, opportunities, challenges and research roadmap. J. Netw. Comput. Appl. 2023 , 217 , 103677. [ Google Scholar ] [ CrossRef ]
- Pajooh, H.H.; Demidenko, S.; Aslam, S.; Harris, M. Blockchain and 6G-Enabled IoT. Inventions 2022 , 7 , 109. [ Google Scholar ] [ CrossRef ]
- Mukherjee, A.; De, D.; Dey, N.; Crespo, R.G.; Herrera-Viedma, E. DisastDrone: A Disaster Aware Consumer Internet of Drone Things System in Ultra-Low Latent 6G Network. IEEE Trans. Consum. Electron. 2023 , 69 , 38–48. [ Google Scholar ] [ CrossRef ]
- Lucic, M.C.; Bouhamed, O.; Ghazzai, H.; Khanfor, A.; Massoud, Y. Leveraging UAVs to Enable Dynamic and Smart Aerial Infrastructure for ITS and Smart Cities: An Overview. Drones 2023 , 7 , 79. [ Google Scholar ] [ CrossRef ]
- Wei, X.; Yang, H.; Huang, W. Low-delay Routing Scheme for UAV Communications in Smart Cities. IEEE Internet Things J. 2023 , 32 , 67131. [ Google Scholar ] [ CrossRef ]
- Saini, H.K.; Jain, K.L. A New Way of Improving Network by Smart IoE with UAV. In Proceedings of the 2023 IEEE International Conference on Computational Intelligence, Communication Technology and Networking (CICTN), Berlin, Germany, 4–8 May 2023; pp. 485–489. [ Google Scholar ]
- Alawadhi, A.; Almogahed, A.; Azrag, E. Towards Edge Computing for 6G Internet of Everything: Challenges and Opportunities. In Proceedings of the 2023 1st International Conference on Advanced Innovations in Smart Cities (ICAISC), Jeddah, Saudi Arabia, 23–25 January 2023; pp. 1–6. [ Google Scholar ]
- Konhäuser, W. Digitalization in Buildings and Smart Cities on the Way to 6G. Wirel. Pers. Commun. 2021 , 121 , 1289–1302. [ Google Scholar ] [ CrossRef ]
- Cheng, C.; Lv, H.; Lv, Z. Sensing fusion in vehicular network digital twins for 6G smart city. ITU J. Future Evol. Technol. 2022 , 3 , 342–358. [ Google Scholar ] [ CrossRef ]
- Du, J.; Jiang, C.; Wang, J.; Ren, Y.; Debbah, M. Machine learning for 6G wireless networks: Carrying forward enhanced bandwidth, massive access, and ultrareliable/low-latency service. IEEE Veh. Technol. Mag. 2020 , 15 , 122–134. [ Google Scholar ] [ CrossRef ]
- Mikalef, P.; Lemmer, K.; Schaefer, C.; Ylinen, M.; Fjørtoft, S.O.; Torvatn, H.Y.; Gupta, M.; Niehaves, B. Enabling AI capabilities in government agencies: A study of determinants for European municipalities. Gov. Inf. Q. 2022 , 39 , 101596. [ Google Scholar ] [ CrossRef ]
- Yigitcanlar, T.; Desouza, K.; Butler, L.; Roozkhosh, F.; Yigitcanlar, T.; Desouza, K.; Butler, L.; Roozkhosh, F. Contributions and risks of artificial intelligence (AI) in building smarter cities: Insights from a systematic review of the literature. Energies 2020 , 13 , 1473. [ Google Scholar ] [ CrossRef ]
- Shaw, S.; Rowland, Z.; Machova, V. Internet of Things smart devices sustainable industrial big data and artificial intelligence-based decision-making algorithms in cyber-physical system-based manufacturing. Econ. Manag. Financ. Mark. 2021 , 16 , 106–116. [ Google Scholar ]
- Bandaragoda, T.; Adikari, A.; Nawaratne, R.; Nallaperuma, D.; Luhach, A.K.; Kempitiya, T.; Nguyen, S.; Alahakoon, D.; De Silva, D.; Chilamkurti, N. Artificial intelligence based commuter behaviour profiling framework using Internet of Things for real-time decision-making. Neural Comput. Appl. 2020 , 32 , 16057–16071. [ Google Scholar ] [ CrossRef ]
- Zhang, S.; Zhu, D. Towards artificial intelligence enabled 6G: State of the art, challenges, and opportunities. Comput. Netw. 2020 , 183 , 107556. [ Google Scholar ] [ CrossRef ]
- Ma, X.; Gao, Z. Data-driven deep learning to design pilot and channel estimator for massive MIMO. IEEE Trans. Veh. Technol. 2020 , 69 , 5677–5682. [ Google Scholar ] [ CrossRef ]
- Wang, C.X.; di Renzo, M.; Stańczak, S.; Wang, S.; Larsson, E.G. Artificial intelligence enabled wireless networking for 5G and beyond: Recent advances and future challenges. IEEE Wirel. Commun. 2020 , 27 , 16–23. [ Google Scholar ] [ CrossRef ]
- Tataria, H.; Shafi, M.; Molisch, A.F.; Dohler, M.; Sjoland, H.; Tufvesson, F. 6G wireless systems: Vision, requirements, challenges, insights, and opportunities. Proc. IEEE 2021 , 109 , 1166–1199. [ Google Scholar ] [ CrossRef ]
- Rekkas, V.P.; Sotiroudis, S.; Sarigiannidis, P.; Wan, S.; Karagiannidis, G.K.; Goudos, S.K. Machine learning in beyond 5g/6g networks—State-of-the-art and future trends. Electronics 2021 , 10 , 2786. [ Google Scholar ] [ CrossRef ]
- Kato, N.; Mao, B.; Tang, F.; Kawamoto, Y.; Liu, J. Ten challenges in advancing machine learning technologies toward 6G. IEEE Wirel. Commun. 2020 , 27 , 96–103. [ Google Scholar ] [ CrossRef ]
- Khan, N.A.; Schmid, S. AI-RAN in 6G Networks: State-of-the-Art and Challenges. IEEE Open J. Commun. Soc. 2023 , 5 , 294–311. [ Google Scholar ] [ CrossRef ]
- Letaief, K.B.; Chen, W.; Shi, Y.; Zhang, J.; Zhang, Y.-J.A. The roadmap to 6G: AI empowered wireless networks. IEEE Commun. Mag. 2019 , 57 , 84–90. [ Google Scholar ] [ CrossRef ]
- Narottama, B.; Mohamed, Z.; Aïssa, S. Quantum Machine Learning for Next-G Wireless Communications: Fundamentals and the Path Ahead. IEEE Open J. Commun. Soc. 2023 , 4 , 2204–2224. [ Google Scholar ] [ CrossRef ]
- Shvetsov, A.V.; Alsamhi, S.H.; Hawbani, A.; Kumar, S.; Srivastava, S.; Agarwal, S.; Rajput, N.S.; Alammari, A.A.; Nashwan, F.M.A. Federated Learning Meets Intelligence Reflection Surface in Drones for Enabling 6G Networks: Challenges and Opportunities. IEEE Access 2023 , 11 , 130860–130887. [ Google Scholar ] [ CrossRef ]
- Klaine, P.V.; Nadas, J.P.B.; Souza, R.D.; Imran, M.A. Distributed drone base station positioning for emergency cellular networks using reinforcement learning. Cogn. Comput. 2018 , 10 , 790–804. [ Google Scholar ] [ CrossRef ]
- Gao, H.M.; Zhong, C.; Li, G.Y.; Zhang, Z. An attention-aided deep learning framework for massive MIMO channel estimation. IEEE Trans. Wirel. Commun. 2021 , 21 , 1823–1835. [ Google Scholar ] [ CrossRef ]
- O’Shea, T.; Hoydis, J. An Introduction to Deep Learning for the Physical Layer. IEEE Trans. Cogn. Commun. Netw. 2017 , 3 , 563–575. [ Google Scholar ] [ CrossRef ]
- Xu, W.; Yang, Z.; Ng, D.W.K.; Levorato, M.; Eldar, Y.C.; Debbah, M. Edge learning for B5G networks with distributed signal processing: Semantic communication, edge computing, and wireless sensing. IEEE J. Sel. Top. Signal Process. 2023 , 17 , 9–39. [ Google Scholar ] [ CrossRef ]
- Schiller, J.H.; Wesley, A. Mobile Communications , 2nd ed.; Addison-Wesley: Boston, MA, USA, 2003. [ Google Scholar ]
- Kairouz, P.; McMahan, H.B.; Avent, B.; Bellet, A.; Bennis, M.; Bhagoji, A.N.; Bonawitz, K.; Charles, Z.; Cormode, G.; Cummings, R.; et al. Advances and Open Problems in Federated Learning. Found. Trends Mach. Learn. 2021 , 14 , 1–210. [ Google Scholar ] [ CrossRef ]
- Zhang, C.; Xie, Y.; Bai, H.; Yu, B.; Li, W.; Gao, Y. A survey on federated learning. Knowl. -Based Syst. 2021 , 216 , 106775. [ Google Scholar ] [ CrossRef ]
- Yang, C.; He, Z.; Peng, Y.; Wang, Y.; Yang, J. Deep learning aided method for automatic modulation recognition. IEEE Access 2019 , 7 , 109063–109068. [ Google Scholar ] [ CrossRef ]
- Vinayakumar, R.; Soman, K.; Poornachandran, P. Applying deep learning approaches for network traffic prediction. In Proceedings of the 2017 International Conference on Advances in Computing, Communications and Informatics (ICACCI), Manipal, India, 13–16 September 2017; pp. 2353–2358. [ Google Scholar ]
- Zhang, H.; Zhang, H.; Long, K.; Karagiannidis, G.K. Deep learning based radio resource management in NOMA networks: User association, subchannel and power allocation. IEEE Trans. Netw. Sci. Eng. 2020 , 7 , 2406–2415. [ Google Scholar ] [ CrossRef ]
- Zhao, Z.; Karimzadeh, M.; Pacheco, L.; Santos, H.; Rosário, D.; Braun, T.; Cerqueira, E. Mobility management with transferable reinforcement learning trajectory prediction. IEEE Trans. Netw. Serv. Manag. 2020 , 17 , 2102–2116. [ Google Scholar ] [ CrossRef ]
- Ozpoyraz, B.; Dogukan, A.T.; Gevez, Y.; Altun, U.; Basar, E. Deep Learning-Aided 6G Wireless Networks: A Comprehensive Survey of Revolutionary PHY Architectures. IEEE Open J. Commun. Soc. 2022 , 3 , 1749–1809. [ Google Scholar ] [ CrossRef ]
- Samuel, N.; Diskin, T.; Ami, W. Deep MIMO detection. In Proceedings of the IEEE 18th International Workshop on Signal Processing Advances in Wireless Communications (SPAWC), Sapporo, Japan, 3–6 July 2014; pp. 1–5. [ Google Scholar ]
- Samuel, N.; Diskin, T.; Wiesel, A. Learning to detect. IEEE Trans. Signal Process. 2019 , 67 , 2554–2564. [ Google Scholar ] [ CrossRef ]
- He, H.; Wen, C.-K.; Jin, S.; Li, G.Y. A model-driven deep learning network for MIMO detection. In Proceedings of the IEEE Global Conference on Signal and Information Processing (GlobalSIP), Anaheim, CA, USA, 26–28 November 2018; pp. 584–588. [ Google Scholar ]
- Al-Baidhani, A.; Fan, H.H. Learning for detection: A deep learning wireless communication receiver over rayleigh fading channels. In Proceedings of the 2019 International Conference on Computing, Networking and Communications (ICNC), Honolulu, HI, USA, 18–21 February 2019; pp. 6–10. [ Google Scholar ]
- Shamasundar, B.; Chockalingam, A. A DNN architecture for the detection of generalized spatial modulation Signalsieee. Commun. Lett. 2020 , 24 , 2770–2774. [ Google Scholar ]
- Albinsaid, H.; Singh, K.; Biswas, S.; Li, C.-P.; Alouini, M.-S. Block deep neural network-based signal detector for generalized spatial modulation. IEEE Commun. Lett. 2020 , 24 , 2775–2779. [ Google Scholar ] [ CrossRef ]
- Xiang, L.; Liu, Y.; Van Luong, T.; Maunder, R.G.; Yang, L.-L.; Hanzo, L. Deep-learning-aided joint channel estimation and data detection for spatial modulation. IEEE Access 2020 , 8 , 191910–191919. [ Google Scholar ] [ CrossRef ]
- He, H.; Wen, C.-K.; Jin, S.; Li, G.Y. Model-driven deep learning for MIMO detection. IEEE Trans. Signal Process. 2020 , 68 , 1702–1715. [ Google Scholar ] [ CrossRef ]
- Wang, X.; Hua, H.; Xu, Y. Pilot-assisted channel estimation and signal detection in uplink multi-user MIMO systems with deep learning. IEEE Access 2020 , 8 , 44936–44946. [ Google Scholar ] [ CrossRef ]
- Sohrabi, F.; Cheng, H.V.; Yu, W. Robust symbol-level precoding via autoencoder-based deep learning. In Proceedings of the ICASSP 2020-2020 IEEE International Conference on Acoustics, Speech and Signal Processing (ICASSP), Virtual, 4–9 May 2020; pp. 8951–8955. [ Google Scholar ]
- Bo, Z.; Liu, R.; Li, M.; Liu, Q. Deep learning based efficient symbol-level precoding design for MU-MISO systems. IEEE Trans. Veh. Technol. 2021 , 70 , 8309–8313. [ Google Scholar ] [ CrossRef ]
- Sheraz, M.; Ahmed, M.; Hou, X.; Li, Y.; Jin, D.; Han, Z.; Jiang, T. Artificial Intelligence for Wireless Caching: Schemes, Performance, and Challenges. IEEE Commun. Surv. Tutor. 2020 , 23 , 631–661. [ Google Scholar ] [ CrossRef ]
- Huang, X.; Zhang, K.; Wu, F.; Leng, S. Collaborative Machine Learning for Energy-Efficient Edge Networks in 6G. IEEE Netw. 2021 , 35 , 12–19. [ Google Scholar ] [ CrossRef ]
- Navarathna, P.J.; Malagi, V.P. Artificial intelligence in smart city analysis. In Proceedings of the 2018 International Conference on Smart Systems and Inventive Technology (ICSSIT), Tirunelveli, India, 13–14 December 2018; pp. 44–47. [ Google Scholar ]
- Sharma, V.; Kumar, S. Role of artificial intelligence (AI) to enhance the security and privacy of data in smart cities. In Proceedings of the 2023 3rd International Conference on Advance Computing and Innovative Technologies in Engineering (ICACITE), Greater Noida, India, 12–13 May 2023; pp. 596–599. [ Google Scholar ]
- Ilyas, M. IoT applications in smart cities. In Proceedings of the 2021 International Conference on Electronic Communications, Internet of Things and Big Data (ICEIB), Yilan, Taiwan, 10–12 December 2021; pp. 44–47. [ Google Scholar ]
- Liu, F.; Cui, Y.; Masouros, C.; Xu, J.; Han, T.X.; Eldar, Y.C.; Buzzi, S. Integrated sensing and communications: Towards dual-functional wireless networks for 6G and beyond. IEEE J. Sel. Areas Commun. 2022 , 40 , 1728–1767. [ Google Scholar ] [ CrossRef ]
- Liu, F.; Zheng, L.; Cui, Y.; Masouros, C.; Petropulu, A.P.; Griffiths, H.; Eldar, Y.C. Seventy Years of Radar and Communications: The road from separation to integration. IEEE Signal Process. Mag. 2023 , 40 , 106–121. [ Google Scholar ] [ CrossRef ]
- Cui, Y.; Liu, F.; Jing, X.; Mu, J. Integrating sensing and communications for ubiquitous IoT: Applications, trends, and challenges. IEEE Netw. 2021 , 35 , 158–167. [ Google Scholar ] [ CrossRef ]
- Chiriyath, A.R.; Paul, B.; Bliss, D.W. Radar-communications convergence: Coexistence cooperation and co-Des. Trans. Cogn. Commun. Netw. 2017 , 3 , 1–12. [ Google Scholar ] [ CrossRef ]
- Zhang, J.A.; Liu, F.; Masouros, C.; Heath, R.W.; Feng, Z.; Zheng, L.; Petropulu, A. An overview of signal processing techniques for joint communication and radar sensing. IEEE J. Sel. Top. Signal Process. 2021 , 15 , 1295–1315. [ Google Scholar ] [ CrossRef ]
- Mishra, K.V.; Shankar, M.B.; Koivunen, V.; Ottersten, B.; Vorobyov, S.A. Toward millimeter-wave joint radar communications: A signal processing perspective. IEEE Signal Process. Mag. 2019 , 36 , 100–114. [ Google Scholar ] [ CrossRef ]
- Althobaiti, T.; Khalil, R.A.; Saeed, N. Robust ISAC Localization in Smart Cities: A Hybrid Network Approach for NLOS Challenges with Uncertain Parameters. J. Sens. Actuator Netw. 2024 , 13 , 2. [ Google Scholar ] [ CrossRef ]
- Khalil, R.A.; Saeed, N.; Almutiry, M. UAVs-assisted passive source localization using robust TDOA ranging for search and rescue. ICT Express 2022 , 9 , 677–682. [ Google Scholar ] [ CrossRef ]
- Huang, S.; Wang, B.; Zhao, Y.; Luan, M. Near-field RSS-based localization algorithms using reconfigurable intelligent surface. IEEE Sens. J. 2022 , 22 , 3493–3505. [ Google Scholar ] [ CrossRef ]
- Liu, J.; Liu, H.; Chen, Y.; Wang, Y.; Wang, C. Wireless sensing for human activity: A survey. IEEE Commun. Surv. Tutor. 2020 , 22 , 1629–1645. [ Google Scholar ] [ CrossRef ]
- Chu, N.H.; Nguyen, D.N.; Hoang, D.T.; Pham, Q.-V.; Hwang, W.-J.; Dutkiewicz, E. Ai-empowered joint communication and radar systems with adaptive waveform for autonomous vehicles. arXiv 2022 , arXiv:2202.11508. [ Google Scholar ]
- Demirhan, U.; Alkhateeb, A. Integrated sensing and communication for 6g: Ten key machine learning roles. IEEE Commun. Mag. 2023 , 61 , 113–119. [ Google Scholar ] [ CrossRef ]
- Lu, S.; Liu, F.; Li, Y.; Zhang, K.; Huang, H.; Zou, J.; Li, X.; Dong, Y.; Dong, F.; Zhu, J.; et al. Integrated Sensing and Communications: Recent Advances and Ten Open Challenges. IEEE Internet Things J. 2024 , 11 , 19094–19120. [ Google Scholar ] [ CrossRef ]
- Saeed, N.; Al-Naffouri, T.Y.; Alouini, M.-S. Around the world of IoT/climate monitoring using internet of X-things. IEEE Internet Things Mag. 2020 , 3 , 82–83. [ Google Scholar ] [ CrossRef ]
- Gurgen, L.; Gunalp, O.; Benazzouz, Y.; Gallissot, M. Self-aware cyber-physical systems and applications in smart buildings and cities. In Proceedings of the 2013 Design, Automation & Test in Europe Conference & Exhibition (DATE), Grenoble, France, 18–22 March 2013; pp. 1149–1154. [ Google Scholar ]
- Kato, T.; Fukumoto, N.; Sasaki, C.; Tagami, A.; Nakao, A. Challenges of CPS/IoT Network Architecture in 6G Era. IEEE Access 2024 , 12 , 62804–62817. [ Google Scholar ] [ CrossRef ]
- Guo, F.; Yu, F.R.; Zhang, H.; Li, X.; Ji, H.; Leung, V.C.M. Enabling Massive IoT Toward 6G: A Comprehensive Survey. IEEE Internet Things J. 2021 , 8 , 11891–11915. [ Google Scholar ] [ CrossRef ]
- Mahmood, N.H.; Berardinelli, G.; Khatib, E.J.; Hashemi, R.; Morais de Lima, C.; Latva-aho, M. A Functional Architecture for 6G Special Purpose Industrial IoT Networks. IEEE Trans. Ind. Inform. 2022 , 19 , 2530–2540. [ Google Scholar ] [ CrossRef ]
- Eldrandaly, K.A.; Abdel-Basset, M.; Shawky, L.A. Internet of Spatial Things: A New Reference Model with Insight Analysis. IEEE Access 2019 , 7 , 19653–19669. [ Google Scholar ] [ CrossRef ]
- Banafaa, M.; Shayea, I.; Din, J.; Azmi, M.H.; Alashbi, A.; Daradkeh, Y.I.; Alhammadi, A. 6G Mobile Communication Technology: Requirements, Targets, Applications, Challenges, Advantages, and Opportunities. Alex. Eng. J. 2023 , 64 , 245–274. [ Google Scholar ] [ CrossRef ]
- Gupta, R.; Reebadiya, D.; Tanwar, S. 6G-enabled edge intelligence for ultra -reliable low latency applications: Vision and mission. Comput. Stand. Interfaces 2021 , 77 , 103521. [ Google Scholar ] [ CrossRef ]
- Alwis, C.D.; Kalla, A.; Pham, Q.-V.; Kumar, P.; Dev, K.; Hwang, W.-J.; Liyanage, M. Survey on 6G frontiers: Trends, applications, requirements, technologies and future research. IEEE Open J. Commun. Soc. 2021 , 2 , 836–886. [ Google Scholar ] [ CrossRef ]
- Kua, J.; Loke, S.W.; Arora, C.; Fernando, N.; Ranaweera, C. Internet of Things in Space: A Review of Opportunities and Challenges from Satellite-Aided Computing to Digitally-Enhanced Space Living. Sensors 2021 , 21 , 8117. [ Google Scholar ] [ CrossRef ]
- Alabdulatif, A.; Thilakarathne, N.N.; Lawal, Z.K.; Fahim, K.E.; Zakari, R.Y. Internet of Nano-Things (IoNT): A Comprehensive Review from Architecture to Security and Privacy Challenges. Sensors 2023 , 23 , 2807. [ Google Scholar ] [ CrossRef ] [ PubMed ]
- Ullah, Z.; Naeem, M.; Coronato, A.; Ribino, P.; De Pietro, G. Applications in Sustainable Smart Cities. Sustain. Cities Soc. 2023 , 97 , 104697. [ Google Scholar ] [ CrossRef ]
- Khan, A.H.; Hassan, N.U.; Yuen, C.; Zhao, J.; Niyato, D.; Zhang, Y.; Poor, H.V. Blockchain and 6G: The Future of Secure and Ubiquitous Communication. IEEE Wirel. Commun. 2021 , 29 , 194–201. [ Google Scholar ] [ CrossRef ]
- Kang, J.; Xiong, Z.; Niyato, D.; Ye, D.; Kim, D.I.; Zhao, J. Toward secure blockchain-enabled internet of vehicles: Optimizing consensus management using reputation and contract theory. IEEE Trans. Veh. Technol. 2019 , 68 , 2906–2920. [ Google Scholar ] [ CrossRef ]
- Kim, M.; Oh, I.; Yim, K.; Sahlabadi, M.; Shukur, Z. Security of 6G-Enabled Vehicle-to-Everything Communication in Emerging Federated Learning and Blockchain Technologies. IEEE Access 2023 , 12 , 33972–34001. [ Google Scholar ] [ CrossRef ]
- Hassan, N.U.; Yuen, C.; Niyato, D. Blockchain technologies for smart energy systems: Fundamentals, challenges, and solutions. IEEE Ind. Electron. Mag. 2019 , 13 , 106–118. [ Google Scholar ] [ CrossRef ]
- Leng, J.; Ye, S.; Zhou, M.; Zhao, J.L.; Liu, Q.; Guo, W.; Cao, W.; Fu, L. Blockchain-Secured Smart Manufacturing in Industry 4.0: A Survey. IEEE Trans. Syst. Man Cybern. Syst. 2021 , 51 , 237–252. [ Google Scholar ] [ CrossRef ]
- Balandina, E.; Balandin, S.; Mouromtsev, D. IoT use cases in healthcare and tourism. In Proceedings of the 2015 IEEE 17th Conference on Business Informatics (CBI), Lisbon, Portugal, 13–16 July 2015; pp. 37–44. [ Google Scholar ]
- Chaudjary, S.; Kakkar, R.; Gupta, R.; Tanwar, S.; Agrawal, S.; Sharma, R. Blockchain and federated learning-based security solutions for telesurgery system: A comprehensive review. Turk. J. Electr. Eng. Comput. Sci. 2022 , 30 , 2446–2488. [ Google Scholar ] [ CrossRef ]
- Bhushan, B.; Khamparia, A.; Sagayam, K.M.; Sharma, S.K.; Ahad, M.A.; Debnath, N.C. Blockchain for smart cities: A review of architectures integration trends and future research directions. Sustain. Cities Soc. 2020 , 61 , 102360. [ Google Scholar ] [ CrossRef ]
- Chen, Y.; Qiu, Y.; Tang, Z.; Long, S.; Zhao, L.; Tang, Z. Exploring the Synergy of Blockchain, IoT, and Edge Computing in Smart Traffic Management across Urban Landscapes. J. Grid Comput. 2024 , 22 , 45. [ Google Scholar ] [ CrossRef ]
- Ahmad, A.Y.A.; Verma, N.; Sarhan, N.M.; Awwad, E.M.; Arora, A.; Nyangaresi, V.O. An IoT and Blockchain-Based Secure and Transparent Supply Chain Management Framework in Smart Cities Using Optimal Queue Model. IEEE Access 2024 , 12 , 51752–51771. [ Google Scholar ] [ CrossRef ]
- Sun, X.; Dou, H.; Chen, S.; Zhao, H. A novel block-chain based secure cross-domain interaction approach for intelligent transportation systems. Phys. Commun. 2024 , 63 , 102223. [ Google Scholar ] [ CrossRef ]
- Raja, G.; Senthivel, S.G.; Balaganesh, S.; Rajakumar, B.R.; Ravichandran, V.; Guizani, M. MLB-IoD: Multi Layered Blockchain Assisted 6G Internet of Drones Ecosystem. IEEE Trans. Veh. Technol. 2023 , 72 , 2511–2520. [ Google Scholar ] [ CrossRef ]
- Qian, K.; Liu, Y.; Shu, C.; Sun, Y.; Wang, K. Fine-Grained Benchmarking and Targeted Optimization: Enabling Green IoT-Oriented Blockchain in the 6G Era. IEEE Trans. Green Commun. Netw. 2022 , 7 , 1036–1051. [ Google Scholar ] [ CrossRef ]
- Shah, K.; Chadotra, S.; Tanwar, S.; Gupta, R.; Kumar, N. Blockchain for IoV in 6G environment: Review solutions and challenges. Clust. Comput. 2022 , 25 , 1927–1955. [ Google Scholar ] [ CrossRef ]
- Kaur, A.; Singh, G.; Kukreja, V.; Sharma, S.; Singh, S.; Yoon, B. Adaptation of IoT with Blockchain in Food Supply Chain Management: An Analysis-Based Review in Development, Benefits and Potential Applications. Sensors 2022 , 22 , 8174. [ Google Scholar ] [ CrossRef ]
- Akyildiz, I.F.; Kak, A.; Nie, S. 6G and beyond: The future of wireless communications systems. IEEE Access 2020 , 8 , 133995–134030. [ Google Scholar ] [ CrossRef ]
- Bariah, L.; Mohjazi, L.; Muhaidat, S.; Sofotasios, P.C.; Kurt, G.K.; Yanikomeroglu, H.; Dobre, O.A. A prospective look: Key enabling technologies applications and open research topics in 6G networks. arXiv 2020 , arXiv:2004.06049. [ Google Scholar ] [ CrossRef ]
- Jiang, W.; Zhou, Q.; He, J.; Habibi, M.A.; Melnyk, S.; El-Absi, M.; Han, B.; Di Renzo, M.; Schotten, H.D.; Luo, F.-L.; et al. Terahertz Communications and Sensing for 6G and Beyond: A Comprehensive View. TechRxiv 2023 . [ Google Scholar ] [ CrossRef ]
- Elayan, H.; Amin, O.; Shihada, B.; Shubair, R.M.; Alouini, M.-S. Terahertz band: The last piece of RF spectrum puzzle for communication systems. IEEE Open J. Commun. Soc. 2019 , 1 , 1–32. [ Google Scholar ] [ CrossRef ]
- Saad, W.; Bennis, M.; Chen, M. A vision of 6G wireless systems: Applications trends technologies and open research problems. IEEE Netw. 2020 , 34 , 134–142. [ Google Scholar ] [ CrossRef ]
- Lin, C.; Li, G.Y. Terahertz communications: An array-of-subarrays solution. IEEE Commun. Mag. 2016 , 54 , 124–131. [ Google Scholar ] [ CrossRef ]
- Pan, C.; Ren, H.; Wang, K.; Kolb, J.F.; Elkashlan, M.; Chen, M.; Di Renzo, M.; Hao, Y.; Wang, J.; Swindlehurst, A.L.; et al. Reconfigurable intelligent surfaces for 6G systems: Principles applications and research directions. arXiv 2020 , arXiv:2011.04300. [ Google Scholar ] [ CrossRef ]
- Basharat, S.; Hassan, S.A.; Pervaiz, H.; Mahmood, A.; Ding, Z.; Gidlund, M. Reconfigurable Intelligent Surfaces: Potentials, Applications, and Challenges for 6G Wireless Networks. IEEE Wirel. Commun. 2021 , 28 , 184–191. [ Google Scholar ] [ CrossRef ]
- Rappaport, T.S.; Xing, Y.; Kanhere, O.; Ju, S.; Madanayake, A.; Mandal, S.; Trichopoulos, G.C. Wireless communications andapplications above 100 GHz: Opportunities and challenges for 6G andbeyond. IEEE Access 2019 , 7 , 78729–78757. [ Google Scholar ] [ CrossRef ]
- Lemic, F.; Abadal, S.; Tavernier, W.; Stroobant, P.; Colle, D.; Alarcón, E.; Marquez-Barja, J.M.; Famaey, J. Survey on terahertz nanocommunication and networking: A top-down perspective. IEEE J. Sel. Areas Commun. 2021 , 39 , 1506–1543. [ Google Scholar ] [ CrossRef ]
- Akyildiz, I.F.; Jornet, J.M. The Internet of nano-things. IEEE Wirel. Commun. 2010 , 17 , 58–63. [ Google Scholar ] [ CrossRef ]
- Guan, K.; He, D.; Ai, B.; Chen, Y.; Han, C.; Peng, B.; Zhong, Z.; Kuerner, T. Channel Characterization and Capacity Analysis for THz Communication Enabled Smart Rail Mobility. IEEE Trans. Veh. Technol. 2021 , 70 , 4065–4080. [ Google Scholar ] [ CrossRef ]
- Gezimati, M.; Singh, G. Terahertz Imaging and Sensing for Healthcare: Current Status and Future Perspectives. IEEE Access 2023 , 11 , 18590–18619. [ Google Scholar ] [ CrossRef ]
- Bandyopadhyay, A.; Sengupta, A. A review of the concept applications and implementation issues of terahertz spectral imaging technique. IETE Tech. Rev. 2020 , 39 , 471–489. [ Google Scholar ] [ CrossRef ]
- Park, H.; Son, J.-H. Machine learning techniques for thz imaging and time-domain spectroscopy. Sensors 2021 , 21 , 1186. [ Google Scholar ] [ CrossRef ]
- Wang, J.; Wang, C.-X.; Huang, J.; Chen, Y. 6G THz Propagation Channel Characteristics and Modeling: Recent Developments and Future Challenges. IEEE Commun. Mag. 2024 , 62 , 56–62. [ Google Scholar ] [ CrossRef ]
- Song, H.-J.; Lee, N. Terahertz Communications: Challenges in the Next Decade. IEEE Trans. Terahertz Sci. Technol. 2021 , 12 , 105–117. [ Google Scholar ] [ CrossRef ]
- Nawaz, S.J.; Sharma, S.K.; Wyne, S.; Patwary, M.N.; Asaduzzaman, M. Quantum machine learning for 6G communication networks: State-of-the-art and vision for the future. IEEE Access 2019 , 7 , 46317–46350. [ Google Scholar ] [ CrossRef ]
- Botsinis, P.; Alanis, D.; Babar, Z.; Nguyen, H.V.; Chandra, D.; Ng, S.X.; Hanzo, L. Quantum search algorithms for wireless communications. IEEE Commun. Surv. Tutor. 2018 , 21 , 1209–1242. [ Google Scholar ] [ CrossRef ]
- Tarantino, S.; Da Lio, B.; Cozzolino, D.; Bacco, D. Feasibility of quantum communications in aquatic scenarios. Optik 2020 , 216 , 164639. [ Google Scholar ] [ CrossRef ]
- Gyongyosi, L.; Imre, S.; Nguyen, H.V. A survey on quantum channel capacities. IEEE Commun. Surv. Tutor. 2018 , 20 , 1149–1205. [ Google Scholar ] [ CrossRef ]
- Bennett, C.H.; Brassard, G.; Crépeau, C.; Jozsa, R.; Peres, A.; Wootters, W.K. Teleporting an unknown quantum state via dual classical and einstein-podolsky-rosen channels. Phys. Rev. Lett. 1993 , 70 , 1895–1899. [ Google Scholar ] [ CrossRef ] [ PubMed ]
- Sharma, V.; Banerjee, S. Analysis of quantum key distribution based satellite communication. In Proceedings of the 2018 9th International Conference on Computing, Communication and Networking Technologies (ICCCNT), Bengaluru, India, 11–12 July 2018; pp. 1–5. [ Google Scholar ]
- Inoue, K. Quantum key distribution technologies. IEEE J. Sel. Top. Quantum Electron. 2006 , 12 , 888–896. [ Google Scholar ] [ CrossRef ]
- Bennett, C.H.; Wiesner, S.J. Communication via one- and two-particle operators on Einstein-Podolsky-Rosen states. Phys. Rev. Lett. 1992 , 69 , 2881–2884. [ Google Scholar ] [ CrossRef ]
- Rozenman, G.G.; Kundu, N.K.; Liu, R.; Zhang, L.; Maslennikov, A.; Reches, Y.; Youm, H.Y. The quantum internet: A synergy of quantum information technologies and 6G networks. IET Quantum Commun. 2023 , 4 , 147–166. [ Google Scholar ] [ CrossRef ]
- Duong, T.Q.; Nguyen, L.D.; Narottama, B.; Ansere, J.A.; Huynh, D.V.; Shin, H. Quantum-Inspired Real-Time Optimization for 6G Networks: Opportunities, Challenges, and the Road Ahead. IEEE Open J. Commun. Soc. 2022 , 3 , 1347–1359. [ Google Scholar ] [ CrossRef ]
- Kim, M.; Venturelli, D.; Jamieson, K. Leveraging quantum annealing for large MIMO processing in centralized radio access networks. In Proceedings of the ACM Special Interest Group on Data Communication, Beijing, China, 19–23 August 2019; pp. 241–255. [ Google Scholar ]
- Singh, A.K.; Jamieson, K.; McMahon, P.L.; Venturelli, D. Ising machines’ dynamics and regularization for near-optimal MIMO detection. IEEE Trans. Wirel. Commun. 2022 , 21 , 11080–11094. [ Google Scholar ] [ CrossRef ]
- Hurvitz, I.; Karnieli, A.; Arie, A. Frequency-domain engineering of bright squeezed vacuum for continuous-variable quantum information. Opt. Express 2023 , 31 , 20387–20397. [ Google Scholar ] [ CrossRef ]
- Wang, C.; Rahman, A. Quantum-enabled 6G wireless networks: Opportunities and challenges. IEEE Wirel. Commun. 2022 , 29 , 58–69. [ Google Scholar ] [ CrossRef ]
- Edwards, M.; Mashatan, A.; Ghose, S. A review of quantum and hybrid quantum/classical blockchain. Protoc. Inf. Process. 2020 , 19 , 184. [ Google Scholar ] [ CrossRef ]
- Fernandez-Carames, T.M.; Fraga-Lamas, P. Towards post-quantum blockchain: A review on blockchain cryptography resistant to quantum computing attacks. IEEE Access 2020 , 8 , 21091–21116. [ Google Scholar ] [ CrossRef ]
- Wootters, W.K.; Zurek, W.H. A single quantum cannot be cloned. Nature 1982 , 299 , 802–803. [ Google Scholar ] [ CrossRef ]
- Bennett, C.H.; Shor, P.W.; Smolin, J.A.; Thapliyal, A.V. Entanglement-assisted capacity of a quantum channel and the reverse Shannon theorem. IEEE Trans. Inf. Theory 2002 , 48 , 2637–2655. [ Google Scholar ] [ CrossRef ]
- Devetak, I. The private classical capacity and quantum capacity of a quantum channel. IEEE Trans. Inf. Theory 2005 , 51 , 44–55. [ Google Scholar ] [ CrossRef ]
- Wang, D.; Ohnishi, K.; Xu, W. Multimodal haptic display for virtual reality: A survey. IEEE Trans. Ind. Electron. 2019 , 67 , 610–623. [ Google Scholar ] [ CrossRef ]
- Microsoft HoloLens 2. Available online: https://www.microsoft.com/en-us/hololens/ (accessed on 27 July 2024).
- Clemm, A.; Vega, M.T.; Ravuri, H.K.; Wauters, T.; De Turck, F. Toward truly immersive holographic-type communication: Challenges and solutions. IEEE Commun. Mag. 2020 , 58 , 93–99. [ Google Scholar ] [ CrossRef ]
- Strinati, E.C.; Barbarossa, S.; Gonzalez-Jimenez, J.L.; Ktenas, D.; Cassiau, N.; Maret, L.; Dehos, C. 6G: The next frontier: From holographic messaging to artificial intelligence using subterahertz and visible light communication. IEEE Veh. Technol. Mag. 2019 , 14 , 42–50. [ Google Scholar ] [ CrossRef ]
- Kumar, A.; Kumar, S.; Kaushik, A.; Kumar, A.; Saini, J. Real time estimation and suppression of hand tremor for surgical robotic applications. Microsyst. Technol. 2020 , 28 , 305–311. [ Google Scholar ] [ CrossRef ]
- Diana, M.; Marescaux, J. Robotic surgery. J. Br. Surg. 2015 , 102 , e15–e28. [ Google Scholar ] [ CrossRef ] [ PubMed ]
- Liu, X.; Kang, S.; Plishker, W.; Zaki, G.; Kane, T.D.; Shekhar, R. Laparoscopic stereoscopic augmented reality: Toward a clinically viable electromagnetic tracking solution. J. Med. Imaging 2016 , 3 , 045001. [ Google Scholar ] [ CrossRef ]
- Siemonsma, S.; Bell, T. HoloKinect: Holographic 3D video conferencing. Sensors 2022 , 22 , 8118. [ Google Scholar ] [ CrossRef ] [ PubMed ]
- Bastug, E.; Bennis, M.; Medard, M.; Debbah, M. Toward interconnected virtual reality: Opportunities, challenges, and enablers. IEEE Commun. Mag. 2017 , 55 , 110–117. [ Google Scholar ] [ CrossRef ]
- Suzuki, S.-N.; Kanematsu, H.; Barry, D.M.; Ogawa, N.; Yajima, K.; Nakahira, K.T.; Shirai, T.; Kawaguchi, M.; Kobayashi, T.; Yoshitake, M. Virtual Experiments in metaverse and their applications to collaborative projects: The framework and its significance. Procedia Comput. Sci. 2020 , 176 , 2125–2132. [ Google Scholar ] [ CrossRef ]
- Pathak, P.H.; Feng, X.; Hu, P.; Mohapatra, P. Visible light communication networking and sensing: A survey potential and challenges. IEEE Commun. Surv. Tutor. 2015 , 17 , 2047–2077. [ Google Scholar ] [ CrossRef ]
- Filgueiras, H.R.D.; Lima, E.S.; Cunha, M.S.B.; Lopes, C.H.D.S.; De Souza, L.C.; Borges, R.M.; Pereira, L.A.M.; Brandao, T.H.; Andrade, T.P.V.; Alexandre, L.C.; et al. Wireless and Optical Convergent Access Technologies Toward 6G. IEEE Access 2023 , 11 , 9232–9259. [ Google Scholar ] [ CrossRef ]
- Hussein, A.T.; Alresheedi, M.T.; Elmirghani, J.M.H. 20 Gb/s mobile indoor visible light communication system employing beam steering and computer generated holograms. J. Light. Technol. 2015 , 33 , 5242–5260. [ Google Scholar ] [ CrossRef ]
- Chi, N.; Zhou, Y.; Wei, Y.; Hu, F. Visible light communication in 6G: Advances challenges and prospects. IEEE Veh. Technol. Mag. 2020 , 15 , 93–102. [ Google Scholar ] [ CrossRef ]
- Katz, M.; Ahmed, I. Opportunities and challenges for visible light communications in 6G. In Proceedings of the 2020 2nd 6G Wireless Summit (6G SUMMIT), Levi, Finland, 17–20 March 2020; pp. 1–5. [ Google Scholar ]
- Bian, R.; Tavakkolnia, I.; Haas, H. 15.73 Gb/s visible light communication with off-the-shelf leds. J. Light. Technol. 2019 , 37 , 2418–2424. [ Google Scholar ] [ CrossRef ]
- Na, Z.; Wang, Y.; Xiong, M.; Liu, X.; Xia, J. Modeling and throughput analysis of an ADO-OFDM based relay-assisted VLC system for 5G networks. IEEE Access 2018 , 6 , 17586–17594. [ Google Scholar ] [ CrossRef ]
- Chou, H.-H.; Tsai, C.-Y. Demonstration of micro-projection enabled short-range communications for 5G. In Proceedings of the 2016 21st OptoElectronics and Communications Conference (OECC) Held Jointly with 2016 International Conference on Photonics in Switching (PS), Niigata, Japan, 3–7 July 2016; pp. 1–3. [ Google Scholar ]
- Grubor, J.; Randel, S.; Langer, K.-D.; Walewski, J.W. Broadband information broadcasting using LED-based interior lighting. J. Light. Technol. 2009 , 26 , 3883–3892. [ Google Scholar ] [ CrossRef ]
- Wei, L.-Y.; Liu, Y.; Chow, C.-W.; Chen, G.-H.; Peng, C.-W.; Guo, P.-C.; Tsai, J.-F.; Yeh, C.-H. 6.915-Gbit/s white-light phosphor laser diode-based DCO-OFDM visible light communication (VLC) system with functional transmission distance. Electron. Lett. 2020 , 56 , 945–947. [ Google Scholar ] [ CrossRef ]
- Khalighi, M.A.; Uysal, M. Survey on free space optical communication: A communication theory perspective. IEEE Commun. Surv. Tutor. 2014 , 16 , 2231–2258. [ Google Scholar ] [ CrossRef ]
- Jahid, A.; Alsharif, M.H.; Hall, T.J. A contemporary survey on free space optical communication: Potentials technical challenges recent advances and research direction. J. Netw. Comput. Appl. 2022 , 200 , 103311. [ Google Scholar ] [ CrossRef ]
- Liu, X. Free-space optics optimization models for building sway and atmospheric interference using variable Wavelengthieee. Trans. Commun. 2009 , 57 , 492–498. [ Google Scholar ]
- Tzanakaki, A.; Anastasopoulos, M.; Berberana, I.; Syrivelis, D.; Flegkas, P.; Korakis, T.; Mur, D.C.; Demirkol, I.; Gutierrez, J.; Grass, E.; et al. Wireless-optical network convergence: Enabling the 5G architecture to support operational and end-user services. IEEE Commun. Mag. 2017 , 55 , 184–192. [ Google Scholar ] [ CrossRef ]
- Chih-Lin, I.; Li, H.; Korhonen, J.; Huang, J.; Han, L. RAN revolution with NGFI (xHaul) for 5G. J. Light. Technol. 2017 , 36 , 541–550. [ Google Scholar ]
- Matsuura, M.; Tajima, N.; Nomoto, H.; Kamiyama, D. 150-W power-over-fiber using double-clad fibers. J. Light. Technol. 2019 , 38 , 401–408. [ Google Scholar ] [ CrossRef ]
- Ishtiaq, M.; Saeed, N.; Khan, M. Edge Computing in IoT: A 6G Perspective. arXiv 2021 , arXiv:2111.08943. [ Google Scholar ]
- Alalewi, A.; Dayoub, I.; Cherkaoui, S. On 5G-V2X Use Cases and Enabling Technologies: A Comprehensive Survey. IEEE Access 2021 , 9 , 107710–107737. [ Google Scholar ] [ CrossRef ]
- Saeed, N.; Almorad, H.; Dahrouj, H.; Al-Naffouri, T.Y.; Shamma, J.S.; Alouini, M.-S. Point-to-Point communication in Integrated Satellite-Aerial 6G Networks: State-of-the-art and future challenges. IEEE Open J. Commun. Soc. 2021 , 2 , 1505–1525. [ Google Scholar ] [ CrossRef ]
- Di Renzo, M.; Zappone, A.; Debbah, M.; Alouini, M.-S.; Yuen, C.; de Rosny, J.; Tretyakov, S. Smart Radio Environments Empowered by Reconfigurable Intelligent Surfaces: How It Works, State of Research, and The Road Ahead. IEEE J. Sel. Areas Commun. 2020 , 38 , 2450–2525. [ Google Scholar ] [ CrossRef ]
- Wu, Q.; Zhang, R. Intelligent reflecting surface enhanced wireless network via joint active and passive beamforming. IEEE Trans. Wirel. Commun. 2019 , 18 , 5394–5409. [ Google Scholar ] [ CrossRef ]
- Hu, J.; Zhang, H.; Di, B.; Li, L.; Bian, K.; Song, L.; Li, Y.; Han, Z.; Poor, H.V. Reconfigurable intelligent surface based RF sensing: Design, optimization, and implementation. IEEE J. Sel. Areas Commun. 2020 , 38 , 2700–2716. [ Google Scholar ] [ CrossRef ]
- Wymeersch, H.; He, J.; Denis, B.; Clemente, A.; Juntti, M. Radio localization and mapping with reconfigurable intelligent surfaces: Challenges, opportunities, and research directions. IEEE Veh. Technol. Mag. 2020 , 15 , 52–61. [ Google Scholar ] [ CrossRef ]
- Chen, R.; Liu, M.; Hui, Y.; Cheng, N.; Li, J. Reconfigurable Intelligent Surfaces for 6G IoT Wireless Positioning: A Contemporary Survey. IEEE Internet Things J. 2022 , 9 , 23570–23582. [ Google Scholar ] [ CrossRef ]
- Lin, Z.; Niu, H.; An, K.; Wang, Y.; Zheng, G.; Chatzinotas, S.; Hu, Y. Refracting RIS-Aided Hybrid Satellite-Terrestrial Relay Networks: Joint Beamforming Design and Optimization. IEEE Trans. Aerosp. Electron. Syst. 2022 , 58 , 3717–3724. [ Google Scholar ] [ CrossRef ]
- Xu, J.; Liu, Y.; Mu, X.; Dobre, O.A. STAR-RISs: Simultaneous transmitting and reflecting reconfigurable intelligent surfaces. IEEE Comm. Lett. 2021 , 25 , 3134–3138. [ Google Scholar ] [ CrossRef ]
- Hu, S.; Rusek, F.; Edfors, O. Beyond massive MIMO: The potential of data transmission with large intelligent surfaces. IEEE Trans. Signal Process. 2018 , 66 , 2746–2758. [ Google Scholar ] [ CrossRef ]
- Wu, Q.; Zhang, R. Weighted sum power maximization for intelligent reflecting surface aided SWIPT. IEEE Wirel. Commun. Lett. 2020 , 9 , 586–590. [ Google Scholar ] [ CrossRef ]
- Niu, H.; Chu, Z.; Zhou, F.; Zhu, Z.; Zhang, M.; Wong, K.-K. Weighted sum secrecy rate maximization using intelligent reflecting surface. IEEE Trans. Commun. 2021 , 69 , 6170–6184. [ Google Scholar ] [ CrossRef ]
- Niu, H.; Chu, Z.; Zhou, F.; Zhu, Z.; Zhen, L.; Wong, K.-K. Robust Design for Intelligent Reflecting Surface-Assisted Secrecy SWIPT Network. IEEE Trans. Wirel. Commun. 2021 , 21 , 4133–4149. [ Google Scholar ] [ CrossRef ]
- Zhang, Z.; Dai, L. Reconfigurable Intelligent Surfaces for 6G: Nine Fundamental Issues and One Critical Problem. Tsinghua Sci. Technol. 2023 , 28 , 929–939. [ Google Scholar ] [ CrossRef ]
- Hayajneh, A.M.; Zaidi, S.A.R.; McLernon, D.C.; Ghogho, M. Drone empowered small cellular disaster recovery networks for resilient smart cities. In Proceedings of the 2016 IEEE International Conference on Sensing, Communication and Networking (SECON Workshops), London, UK, 27 June 2016; pp. 1–6. [ Google Scholar ]
- Zhao, S.; Ota, K.; Dong, M. UAV base station trajectory optimization based on reinforcement learning in post-disaster search and rescue operations. arXiv 2022 , arXiv:2202.10338. [ Google Scholar ]
- Angjo, J.; Shayea, I.; Ergen, M.; Mohamad, H.; Alhammadi, A.; Daradkeh, Y.I. Handover management of drones in future mobile networks: 6G technologies. IEEE Access 2021 , 9 , 12803–12823. [ Google Scholar ] [ CrossRef ]
- Friderikos, V. Airborne Urban Microcells with Grasping End Effectors: A Game Changer for 6G Networks? In Proceedings of the 2021 IEEE International Mediterranean Conference on Communications and Networking (MeditCom), Athens, Greece, 7–10 September 2021. [ Google Scholar ] [ CrossRef ]
- Liu, S.; Gao, Z.; Wu, Y.; Ng, D.W.K.; Gao, X.; Wong, K.; Chatzinotas, S.; Ottersten, B. LEO satellite constellations for 5G and beyond: How will they reshape vertical domains? IEEE Commun. Mag. 2021 , 59 , 30–36. [ Google Scholar ] [ CrossRef ]
- Hadani, R.; Rakib, S.; Tsatsanis, M.; Monk, A.; Goldsmith, A.J.; Molisch, A.F.; Calderbank, R. Orthogonal time frequency space modulation. In Proceedings of the 2017 IEEE Wireless Communications and Networking Conference (WCNC), San Francisco, CA, USA, 19–22 March 2017; pp. 1–6. [ Google Scholar ]
- Tang, G.; Zhu, L. Integrated Signal Design of Communication and Navigation Based on LEO Satellite ; University of Electronic Science and Technology of China: Chengdu, China, 2022. [ Google Scholar ]
- Zhou, C.; Wu, W.; He, H.; Yang, P.; Lyu, F.; Cheng, N.; Shen, X. Deep Reinforcement Learning for Delay-Oriented IoT Task Scheduling in SAGIN. IEEE Trans. Wirel. Commun. 2020 , 20 , 911–925. [ Google Scholar ] [ CrossRef ]
- Mondal, S.; Al-Rubaye, S.; Tsourdos, A. Handover prediction for aircraft dual connectivity using model predictive control. IEEE Access 2021 , 9 , 44463–44475. [ Google Scholar ] [ CrossRef ]
- Warrier, A.; Aljaburi, L.; Whitworth, H.; Al-Rubaye, S.; Tsourdos, A. Future 6G Communications Powering Vertical Handover in Non-Terrestrial Networks. IEEE Access 2024 , 12 , 33016–33034. [ Google Scholar ] [ CrossRef ]
- Ghildiyal, Y.; Singh, R.; Alkhayyat, A.; Gehlot, A.; Malik, P.; Sharma, R.; Akram, S.V.; Alkwai, L.M. An imperative role of 6G communication with perspective of industry 4.0: Challenges and research directions, Sustainable Energy Technologies and Assessments. Sustain. Energy Technol. Assess. 2023 , 56 , 103047. [ Google Scholar ] [ CrossRef ]
- The New High Tech Strategy Innovations for Germany. 2014. [Online]. Available online: https://ec.europa.eu/futurium/en/system/files/ged/hts_broschuere_engl_bf_1.pdf (accessed on 27 July 2024).
- Kusiak, A. Smart manufacturing. Int. J. Prod. Res. 2018 , 56 , 508–517. [ Google Scholar ] [ CrossRef ]
- Grau, A.; Indri, M.; Bello, L.; Sauter, T.O. Robots in industry. IEEE Ind. Electron. 2021 , 15 , 50–61. [ Google Scholar ] [ CrossRef ]
- Aracil, C.; Aziebig, G.; Korondi, P.; Oh, S.; Tan, Z.; Ruderman, M.; He, W.; Ding, L.; Luo, H.; Yin, S.; et al. Toward smart systems. IEEE Ind. Electron. 2021 , 15 , 104–114. [ Google Scholar ] [ CrossRef ]
- Chen, Z.; Chen, K.-C.; Dong, C.; Nie, Z. 6G Mobile Communications for Multi-Robot Smart Factory. J. ICT Stand. 2021 , 9 , 371–404. [ Google Scholar ] [ CrossRef ]
- Han, B.; Habibi, M.A.; Richerzhagen, B.; Schindhelm, K.; Zeiger, F.; Lamberti, F.; Pratticò, F.G.; Upadhya, K.; Korovesis, C.; Belikaidis, I.-P.; et al. Digital Twins for Industry 4.0 in the 6G Era. IEEE Open J. Veh. Technol. 2023 , 4 , 820–835. [ Google Scholar ] [ CrossRef ]
- Kuruvatti, N.P.; Habibi, M.A.; Partani, S.; Han, B.; Fellan, A.; Schotten, H.D. Empowering 6G communication systems with Digital Twin technology: A comprehensive survey of key concepts potential use cases standardization activities and future research directions. IEEE Access 2022 , 10 , 112158–112186. [ Google Scholar ] [ CrossRef ]
- United Nations. Sustainable Development Goals. 2015. [Online]. Available online: https://www.un.org/sustainabledevelopment/ (accessed on 3 February 2023).
- Mihalj, T.; Li, H.; Babić, D.; Lex, C.; Jeudy, M.; Zovak, G.; Babić, D.; Eichberger, A. Road Infrastructure Challenges Faced by Automated Driving: A Review. Appl. Sci. 2022 , 12 , 3477. [ Google Scholar ] [ CrossRef ]
- Noor-A-Rahim, M.; Liu, Z.; Lee, H.; Khyam, M.O.; He, J.; Pesch, D.; Moessner, K.; Saad, W.; Poor, H.V. 6G for Vehicle-to-Everything (V2X) Communications: Enabling Technologies, Challenges, and Opportunities. Proc. IEEE 2022 , 110 , 712–734. [ Google Scholar ] [ CrossRef ]
- Kumar, V.; Ahmad, M.; Mishra, D.; Kumari, S.; Khan, M.K. RSEAP: RFID Based Secure and Efficient Authentication Protocol for Vehicular Cloud Computing. Veh. Commun. 2020 , 22 , 100213. [ Google Scholar ] [ CrossRef ]
- Chen, C.H.; Lee, C.R.; Lu, W.C.H. Smart In-Car Camera System Using Mobile Cloud Computing Framework for Deep Learning. Veh. Commun. 2017 , 10 , 84–90. [ Google Scholar ] [ CrossRef ]
- Ma, Y.; Wang, Z.; Yang, H.; Yang, L. Artificial intelligence applications in the development of autonomous vehicles: A survey. IEEE CAA J. Autom. Sin. 2020 , 7 , 315–329. [ Google Scholar ] [ CrossRef ]
- Reddy, P.P. Driverless Car-Design of a Parallel and Self-Organizing System ; EasyChair: Manchester, UK, 2019. [ Google Scholar ]
- Mendez, J.; Bierzynski, K.; Cuéllar, M.; Morales, D.P. Edge Intelligence: Concepts, architectures, applications and future directions. ACM Trans. Embed. Comput. Syst. TECS 2022 , 21 , 1–41. [ Google Scholar ] [ CrossRef ]
- Rout, R.R.; Vemireddy, S.; Raul, S.K.; Somayajulu, D. Fuzzy logic-based emergency vehicle routing: An IoT system development for smart city applications. Comput. Elect. Eng. 2020 , 88 , 106839. [ Google Scholar ] [ CrossRef ]
- Charoniti, E.; Klunder, G.; Schackmann, P.-P.; Schreuder, M.; de Souza Schwartz, R.; Spruijtenburg, D.; Stelwagen, U.; Wilmink, I. Environmental Benefits of C-V2X for 5GAA-5G Automotive. TNO, TNO Rep. TNO 2020 R11817. 2020. Available online: https://5gaa.org/content/uploads/2020/11/Environmental-Benefits-of-C-V2X.pdf (accessed on 27 July 2024).
- Lee, G.; Guo, J.; Kim, K.J.; Orlik, P.; Ahn, H.; Di Cairano, S.; Saad, W. Edge computing for interconnected intersections in internet of vehicles. In Proceedings of the 2020 IEEE Intelligent Vehicles Symposium (IV), Las Vegas, NV, USA, 19 October–13 November 2020; pp. 480–486. [ Google Scholar ]
- Ageing and Health. 1 October 2022. Available online: https://www.who.int/news-room/fact-sheets/detail/ageing-and-health (accessed on 27 July 2024).
- Shah, T.; Yavari, A.; Mitra, K.; Saguna, S.; Jayaraman, P.P.; Rabhi, F.; Ranjan, R. Remote health care cyber-physical system: Quality of service (QoS) challenges and opportunities. IET Cyber-Phys. Syst. Theory Appl. 2016 , 1 , 40–48. [ Google Scholar ] [ CrossRef ]
- Khullar, V.; Singh, H.P.; Miro, Y.; Anand, D.; Mohamed, H.G.; Gupta, D.; Kumar, N.; Goyal, N. IoT Fog-Enabled Multi-Node Centralized Ecosystem for Real Time Screening and Monitoring of Health Information. Appl. Sci. 2022 , 12 , 9845. [ Google Scholar ] [ CrossRef ]
- Kiourti, A.; Nikita, K.S. A review of in-body biotelemetry devices: Implantables, ingestibles, and injectables. IEEE Trans. Biomed. Eng. 2017 , 64 , 1422–1430. [ Google Scholar ] [ CrossRef ] [ PubMed ]
- Abbasi, Q.H.; Ur-Rehman, M.; Qaraqe, K.; Alomainy, A. Advances in Body-CentricWireless Communication: Applications and State-of-the-Art ; no. 65; Institution of Engineering and Technology: London, UK, 2016. [ Google Scholar ]
- Movassaghi, S.; Abolhasan, M.; Lipman, J.; Smith, D.; Jamalipour, A. Wireless Body Area Networks: A Survey. IEEE Commun. Surv. Tutor. 2014 , 16 , 1658–1686. [ Google Scholar ] [ CrossRef ]
- Ahmed, A.; Xi, R.; Hou, M.; Shah, S.A.; Hameed, S. Harnessing Big Data Analytics for Healthcare: A Comprehensive Review of Frameworks, Implications, Applications, and Impacts. IEEE Access 2023 , 11 , 112891–112928. [ Google Scholar ] [ CrossRef ]
- Lee, C.; Koo, B.-H.; Chae, C.-B.; Schober, R. The Internet of bio-nano things in blood vessels: System design and Prototypesj. Commun. Netw. 2023 , 25 , 222–231. [ Google Scholar ] [ CrossRef ]
- Azmi, K.H.M.; Radzi, N.A.M.; Azhar, N.A.; Samidi, F.S.; Zulkifli, I.T.; Zainal, A.M. Active electric distribution network: Applications, challenges, and opportunities. IEEE Access 2022 , 10 , 134655–134689. [ Google Scholar ] [ CrossRef ]
- Khosrojerdi, F.; Akhigbe, O.; Gagnon, S.; Ramirez, A.; Richards, G. Integrating artificial intelligence and analytics in smart grids: A systematic literature review. Int. J. Energy Sect. Manag. 2022 , 16 , 318–338. [ Google Scholar ] [ CrossRef ]
- Abdullah, A.A.; Hassan, T.M. Smart grid (SG) properties and challenges: An overview. Discov. Energy 2022 , 2 , 8. [ Google Scholar ] [ CrossRef ]
- Halle, P.D.; Shiyamala, S. Secure advance metering infrastructure protocol for smart grid power system enabled by the Internet of Things. Microprocess. Microsyst. 2022 , 95 , 104708. [ Google Scholar ] [ CrossRef ]
- Goudarzi, A.; Ghayoor, F.; Waseem, M.; Fahad, S. Traore A survey on iot-enabled smart grids: Emerging, applications, challenges, and outlook. Energies 2022 , 15 , 6984. [ Google Scholar ] [ CrossRef ]
- Mohammed, H. Alsharif, Abu Jahid, Raju Kannadasan, Mun-Kyeom Kim, Unleashing the potential of sixth generation (6G) wireless networks in smart energy grid management: A comprehensive review. Energy Rep. 2024 , 11 , 1376–1398. [ Google Scholar ] [ CrossRef ]
- Judge, M.A.; Khan, A.; Manzoor, A.; Khattak, H.A. Overview of smart grid implementation: Frameworks, impact, performance and challenges. J. Energy Storage 2022 , 49 , 104056. [ Google Scholar ] [ CrossRef ]
- Aggarwal, S.; Kumar, N.; Tanwar, S.; Alazab, M. A survey on energy trading in the smart grid: Taxonomy, research challenges and solutions. IEEE Access 2021 , 9 , 116231–116253. [ Google Scholar ] [ CrossRef ]
- Arcas, G.I.; Cioara, T.; Anghel, I.; Lazea, D.; Hangan, A. Edge Offloading in Smart Grid. Smart Cities 2024 , 7 , 680–711. [ Google Scholar ] [ CrossRef ]
- Afzal, M.; Li, J.; Amin, W.; Huang, Q.; Umer, K.; Ahmad, S.A.; Ahmad, F.; Raza, A. Role of blockchain technology in transactive energy market: A review. Sustain. Energy Technol. Assess. 2022 , 53 , 102646. [ Google Scholar ] [ CrossRef ]
- Tariq, M.; Ali, M.; Naeem, F.; Poor, H.V. Vulnerability Assessment of 6G-Enabled Smart Grid Cyber–Physical Systems. IEEE Internet Things J. 2020 , 8 , 5468–5475. [ Google Scholar ] [ CrossRef ]
- Gao, J.; Asamoah, K.O.; Xia, Q.; Sifah, E.B.; Amankona, O.I.; Xia, H. A Blockchain Peer-to-Peer Energy Trading System for Microgrids. IEEE Trans. Smart Grid 2023 , 14 , 3944–3960. [ Google Scholar ] [ CrossRef ]
- Vergara, S.E.; Tchobanoglous, G. Municipal solid waste and the environment: A global perspective. Annu. Rev. Environ. Resour. 2012 , 37 , 277–309. [ Google Scholar ] [ CrossRef ]
- Anagnostopoulos, T.; Zaslavsky, A.; Sosunova, I.; Fedchenkov, P.; Medvedev, A.; Ntalianis, K.; Skourlas, C.; Rybin, A.; Khoruznikov, S. A stochastic multi-agent system for Internet of Things-enabled waste management in smart cities. Waste Manag. Res. J. Sustain. Circ. Econ. 2018 , 36 , 1113–1121. [ Google Scholar ] [ CrossRef ]
- Henaien, A.; Ben Elhadj, H.; Fourati, L.C. A sustainable smart IoT-based solid waste management system. Futur. Gener. Comput. Syst. 2024 , 157 , 587–602. [ Google Scholar ] [ CrossRef ]
- Ahmed, M.M.; Hassanien, E.; Hassanien, A.E. IoT-based intelligent waste management system. Neural Comput. Appl. 2023 , 35 , 23551–23579. [ Google Scholar ] [ CrossRef ]
- Maoliang, L.; Lin, X. Incentivizing household recycling crowds out public support for other waste management policies: A long-term quasi-experimental study. J. Environ. Manag. 2021 , 299 , 1–9. [ Google Scholar ] [ CrossRef ]
- Belsare, K.; Singh, M.; Gandam, A.; Malik, P.K.; Agarwal, R.; Gehlot, A. An integrated approach of IoT and WSN using wavelet transform and machine learning for the solid waste image classification in smart cities. Trans. Emerg. Telecommun. Technol. 2023 , 35 , e4857. [ Google Scholar ] [ CrossRef ]
- Bourougaa-Tria, S.; Mokhati, F.; Tria, H.; Bouziane, O. SPubBin: Smart Public Bin Based on Deep Learning Waste Classification: An IOT system for Smart Environment in Algeria. Informatica 2022 , 46 , 41. [ Google Scholar ] [ CrossRef ]
- Mookkaiah, S.S.; Thangavelu, G.; Hebbar, R.; Haldar, N.; Singh, H. Design and development of smart Internet of Things–based solid waste management system using computer vision. Environ. Sci. Pollut. Res. 2022 , 29 , 64871–64885. [ Google Scholar ] [ CrossRef ]
- Cao, B.; Chen, X.; Lv, Z.; Li, R.; Fan, S. Optimization of Classified Municipal Waste Collection Based on the Internet of Connected Vehicles. IEEE Trans. Intell. Transp. Syst. 2020 , 22 , 5364–5373. [ Google Scholar ] [ CrossRef ]
- Rahmanifar, G.; Mohammadi, M.; Sherafat, A.; Hajiaghaei-Keshteli, M.; Fusco, G.; Colombaroni, C. Heuristic approaches to address vehicle routing problem in the Iot-based waste management system. Expert Syst. Appl. 2023 , 220 , 119708. [ Google Scholar ] [ CrossRef ]
- Kona, V.V.S.; Subramoniam, M. A smart Iot-based waste management system using vehicle shortest path routing and trashcan visiting decision making based ondeep convolutional neural network. Peer-Peer Netw. Appl. 2024 , 17 , 1051–1074. [ Google Scholar ] [ CrossRef ]
- Salehi-Amiri, A.; Akbapour, N.; Hajiaghaei-Keshteli, M.; Gajpal, Y.; Jabbarzadeh, A. Designing an effective two-stage, sustainable, and IoT based waste management system. Renew. Sustain. Energy Rev. 2022 , 157 , 112031. [ Google Scholar ] [ CrossRef ]
- Qi, Q.; Chen, X.; Zhong, C.; Zhang, Z. Integration of energy, computation and communication in 6g cellular internet of things. IEEE Commun. Lett. 2020 , 24 , 1333–1337. [ Google Scholar ] [ CrossRef ]
- Tasci, R.A.; Kilinc, F.; Basar, E.; Alexandropoulos, G.C. A New RIS Architecture with a Single Power Amplifier: Energy Efficiency and Error Performance Analysis. IEEE Access 2022 , 10 , 44804–44815. [ Google Scholar ] [ CrossRef ]
- Malik, U.M.; Javed, M.A.; Zeadally, S.; Islam, S.U. Energy-Efficient Fog Computing for 6G-Enabled Massive IoT: Recent Trends and Future Opportunities. IEEE Internet Things J. 2021 , 9 , 14572–14594. [ Google Scholar ] [ CrossRef ]
- Hossain, M.A.; Hossain, A.R.; Ansari, N. AI in 6G: Energy-Efficient Distributed Machine Learning for Multilayer Heterogeneous Networks. IEEE Netw. 2022 , 36 , 84–91. [ Google Scholar ] [ CrossRef ]
- Shen, S.; Yu, C.; Zhang, K.; Ni, J.; Ci, S. Adaptive and Dynamic Security in AI-Empowered 6G: From an Energy Efficiency Perspective. IEEE Commun. Stand. Mag. 2021 , 5 , 80–88. [ Google Scholar ] [ CrossRef ]
- Xie, H.; Zhan, Y.; Zeng, G.; Pan, X. LEO Mega-Constellations for 6G Global Coverage: Challenges and Opportunities. IEEE Access 2021 , 9 , 164223–164244. [ Google Scholar ] [ CrossRef ]
- José, R.; Rodrigues, H. A Review on Key Innovation Challenges for Smart City Initiatives. Smart Cities 2024 , 7 , 141–162. [ Google Scholar ] [ CrossRef ]
- Yoo, Y.; Boland, R.J.; Lyytinen, K.; Majchrzak, A. Organizing for innovation in the digitized world. Organ. Sci. 2012 , 23 , 1398–1408. [ Google Scholar ] [ CrossRef ]
- Zittrain, J.L. The Generative Internet ; The Harvard Law Review Association: Cambridge, MA, USA, 2006. [ Google Scholar ]
- Goumopoulos, C. Smart City Middleware: A Survey and a Conceptual Framework. IEEE Access 2024 , 12 , 4015–4047. [ Google Scholar ] [ CrossRef ]
- Chang, K.-C.; Chu, K.-C.; Wang, H.-C.; Lin, Y.-C.; Pan, J.-S. Agent-based middleware framework using distributed CPS for improving resource utilization in smart city. Futur. Gener. Comput. Syst. 2020 , 108 , 445–453. [ Google Scholar ] [ CrossRef ]
- Puiu, D.; Barnaghi, P.; Tonjes, R.; Kumper, D.; Ali, M.I.; Mileo, A.; Parreira, J.X.; Fischer, M.; Kolozali, S.; Farajidavar, N.; et al. CityPulse: Large scale data analytics framework for smart cities. IEEE Access 2016 , 4 , 1086–1108. [ Google Scholar ] [ CrossRef ]
- Cirillo, F.; Solmaz, G.; Berz, E.L.; Bauer, M.; Cheng, B.; Kovacs, E. A standard-based open source IoT platform: FIWARE. IEEE Internet Things Mag. 2019 , 2 , 12–18. [ Google Scholar ] [ CrossRef ]
- Pliatsios, A.; Lymperis, D.; Goumopoulos, C. S2NetM: A semantic social network of things middleware for developing smart and collaborative IoT-based solutions. Future Internet 2023 , 15 , 207. [ Google Scholar ] [ CrossRef ]
Click here to enlarge figure
Parameter | 5G | 6G |
---|---|---|
Data Rate, Band | ~20 Gbps, sub-6 GHz, Crowded | ~1 TBPS, ultra-fast (THz) |
Services | Limited capability to support new communication | Holographic communication, augmented reality, immersive gaming, etc. |
Latency | Low latency | Ultra-low latency and high reliability |
Architecture | Massive MIMO | Cell-free massive MIMO, intelligent surfaces |
Coverage | Infrastructure-based | Ubiquitous connectivity (space–air–ground–sea) |
Security | Security issues | Blockchain and quantum communication. |
AI Integration | Partial | Full |
Satellite Integration | No | Full |
Source Databases | IEEE Xplore, Web of Science (WoS), Taylor and Francis, ASCE Library, Scopus, and Springer |
---|---|
Search String | (“Artificial Intelligence” OR “THz” OR “ISAC” OR “Block Chain” OR “UAV”) AND (“6G”) AND (“Smart Cities”) |
Time period | 2019–2024 |
Article Type | Journal, Review, Letter, Book Chapter, Short Survey, Article |
Language Restriction | English |
Included Subject Area | Computer Science, Engineering, Energy, Business, Management and Accounting, Mathematics, Environmental Science, Decision Sciences |
Excluded Subject Area | Chemical Engineering, Arts and Humanities, Health Professions, Agricultural and Biological Sciences, Neuroscience, Multidisciplinary, Psychology, Pharmacology, Toxicology and Pharmaceutics, Immunology and Microbiology, Nursing, Social Sciences, Economics Econometrics and Finance, Physics and Astronomy, Materials Science, Medicine, Biochemistry, Genetics and Molecular Biology, Chemistry, Earth and Planetary Sciences |
Ref. | Authors | Year of Public. | Research Area | Major Contribution |
---|---|---|---|---|
[ ] | Fong, B et al. | 2023 | Vehicular | Investigates technical issues regarding the design and implementation of vehicle-to-infrastructure (V2I) systems to enhance reliability in a smart city with 6G as backbone. |
[ ] | P Mishra et al. | 2023 | IoT, Vision | Proposes framework, architecture and requirements for 6G IoT network. Discusses emerging technologies for 6G concerning artificial intelligence/machine learning, sensing networks, spectrum bands, and security. |
[ ] | Nahid Parvaresh, Burak Kantarci, | 2023 | UAV base station | Network performance of UAV-BS is improved by use of proposed continuous actor-critic deep reinforcement learning method to address the 3D location optimization issue of UAV-BSs in smart cities. |
[ ] | Z. Yang et al. | 2023 | Edge cloud, Energy efficiency | Paper analyzes challenges in developing a low-carbon smart city in 6G-enabled smart cities. Also proposes a visual end-edge-cloud architecture (E C) that is AI-driven for attaining low carbon emission in smart cities. |
[ ] | N. Sehito et al. | 2024 | IRS, UAV, NOMA, Spectral efficiency | Paper introduces a new optimization scheme by utilizing IRSs in NOMA multi-UAV networks in 6G-enabled smart cities, resulting in significant performance enhancement in terms of spectral efficiency. |
[ ] | Prabhat Ranjan Singh et al. | 2023 | AI, Technology evolution, Smart city applications | Paper covers evolution of network technology, AI approaches for 6G systems, importance of AI in advanced network model development in 6G-enabled smart city applications. |
[ ] | Murroni, M et al. | 2023 | Vision, Enabling technologies | Paper furnishes an update on the smart city arena with the use of 6G. Paper describes the role of enabling technologies and their specific employment plans. |
[ ] | Kamruzzaman | 2022 | IoT, Energy efficiency, Use cases | Presents key technologies, their applications, and IoT technologies trends for energy-efficient 6G-enabled smart city. Also, identifies and discusses key enabling technologies. |
[ ] | Kim, N et al. | 2024 | Standardization and key enabling technologies | Paper provides key features and recent trends in standardization of smart city concept. Paper highlights potential key technologies of 6G that can be used in various urban use cases in 6G-enabled smart cities. |
[ ] | Ismail, L.; Buyya, R | 2022 | AI-enabled 6G smart cities | Discusses evolution of wireless-technology generations, AI implementation in 6G and its self-learning models in smart city applications. |
[ ] | Zakria Qadir et al. | 2023 | Survey, IoT | Emerging 6G connectivity solutions and their applications in IoT to serve smart cities are surveyed in this paper. |
[ ] | Misbah Shafi et al. | 2024 | 6G technologies | The framework of 6G network is presented with its key technologies that have substantial effect on the key performance indicators of a wireless communication network. |
Natural Resources and Energy | Mobility and Transport | Living and Environment | People and Economy | Government |
---|---|---|---|---|
Smart Grid. | People Mobility. | Pollution Control. | Education and School. | e-Governance. |
Public Lighting. | City Logistics. | Public Safety. | Entertainment and Culture. | Transparency. |
Waste Management. | Health Care. | Entrepreneurship and Innovation. | ||
Water Management | Public Spaces | |||
Welfare Services. | ||||
Smart Homes. |
Ref. | THz | AI | BC | QC | NTN (UAV) | MEC | RIS | ISAC | HC | VLC |
---|---|---|---|---|---|---|---|---|---|---|
[ ] | √ | √ | ||||||||
[ ] | √ | √ | √ | √ | √ | |||||
[ ] | √ | |||||||||
[ ] | √ | |||||||||
[ ] | ||||||||||
[ ] | √ | √ | ||||||||
[ ] | √ | √ | ||||||||
[ ] | √ | √ | ||||||||
[ ] | √ | |||||||||
[ ] | √ | √ | ||||||||
[ ] | √ | |||||||||
[ ] | √ | |||||||||
[ ] | √ | √ | √ | |||||||
[ ] | √ | √ | ||||||||
[ ] | √ | √ | ||||||||
[ ] | √ | |||||||||
[ ] | √ | |||||||||
[ ] | √ | |||||||||
[ ] | √ | √ | ||||||||
[ ] | √ | |||||||||
[ ] | √ | |||||||||
[ ] | √ | |||||||||
[ ] | √ | |||||||||
[ ] | √ | |||||||||
[ ] | √ | |||||||||
[ ] | √ | √ | √ | √ | √ | |||||
[ ] | √ | √ | √ | |||||||
[ ] | √ | √ | √ | √ | √ | √ | ||||
This Paper | √ | √ | √ | √ | √ | √ | √ | √ | √ | √ |
Potential 6G Technology | Brief Description |
---|---|
Artificial Intelligence (AI) | AI can be used to analyze, manage and optimize resources and to efficiently support 6G networks. AI can be used for tasks like efficient channel estimation, energy efficiency, modulation recognition, data caching, traffic prediction, radio resource management, mobility management, etc. |
Terahertz Communication (THz) | Uses frequency band 0.1 to 10 THz. Ability to attain ultra-high (up to 1 Tbps) data rates and wide bandwidth. |
Blockchain (BC) | A type of distributed ledger technology to ensure safety, privacy, scalability and reliability in this complex heterogeneous architecture. |
Quantum Computing (QC) | Based on quantum no-cloning theorem and the principle of uncertainty, absolute randomness is introduced by the use of the quantum nature of information, which provides security and enhanced channel capacity. |
Non Terrestrial networks (NTN) | Includes drones and satellites and is used to extend coverage footprint of terrestrial base stations, provide additional capacity in dense urban hotspots. Used in disaster recovery and remote or rural areas. |
Mobile Edge Communication (MEC) | By placing computing resources closer to end user, it reduces delays and latency and enhances processing speed and on-premise security |
Integrated Sensing and Communication (ISAC) | Optimizes the allocation of scarce resources and contributes to better decision-making processes by combining both sensing and communication tasks, which enhances efficiency. |
Reconfigurable Intelligent Surfaces (RISs) | A planar surface with array of passive elements whose characteristics can be altered dynamically. Used in 6G-THz to improve coverage, NLOS scenarios. |
Holographic Communication (HC) | HC is an application used in transmitting human-sized immersive and interactive holograms consisting of 3D videos and images that require extremely high data rates with ultra-low latency. |
Visible Light Communication (VLC) | VLC offers numerous advantages, such as, energy efficiency, cost-effectiveness, un-licensed spectrum, no electromagnetic interference, secure access technology, and large bandwidth. |
Ref. | Year | Application Domain of Smart Cities | Technologies Used | Areas/Topics Covered |
---|---|---|---|---|
[ ] | 2024 | V2X | 6G, Blockchain, Federated learning, Fog Computing | Comprehensive V2X security analysis. Future research direction for privacy in XR, secure SDN, physical layer security in THz. |
[ ] | 2024 | Smart Traffic Management | Edge Computing, Blockchain, Reinforced learning | Traffic optimization is achieved by decentralized integration of IoT sensors on vehicles and traffic signals and edge devices and the use of BC rules for real-time decisions. |
[ ] | 2024 | Supply Chain Management | Blockchain, IoT, Edge Computing | A Blockchain-based and IoT-enabled transparent and secure supply chain management framework is proposed for public emergency services in smart cities. |
[ ] | 2023 | Intelligent Transport System (ITS) | Blockchain | An ITS cross-domain data interaction framework between devices and agencies is proposed to achieve secure and efficient cross-chain communication. |
[ ] | 2023 | IoT | Blockchain, Big Data, AI | Framework and architecture based on Blockchain, AI and Big Data. |
[ ] | 2023 | Industrial Applications | 6G, Blockchain, IoT | Case study of smart supply chain. Benefits and challenges of BT and 6G-IoT |
[ ] | 2023 | IoD (Internet of Drones) | 6G, Blockchain | Analysis of multilayered Blockchain-IoD novel Global Compliance System (GCoS) and Swarm Security (Sse) system |
[ ] | 2023 | IoT-Blockchain efficiency | 6G, IoT-oriented Blockchain | Improves Blockchain-IoT performance by targeted optimization to improve low power efficiency and slow ledger synchronization. |
[ ] | 2022 | IoV | 6G, Blockchain | A survey paper for BC in IoVs sharing underlying 6G technology. Explores how privacy and security issues in IoVs can be tackled using BC technology. |
[ ] | 2022 | Food Supply Chain Management | IoT, Blockchain | Blockchain enables traceability of food supply from factories/fields to the customer’s table. IoT devices probe food condition. |
Use Case | Description |
---|---|
Remote Surgery | ]. ]. ]. |
Holographic Teleconferencing | ]. ]. ]. |
Immersive Gaming | ]. |
Metaverse | ]. |
Tech. | Applications/Benefits | Challenges |
---|---|---|
AI | ]. , ]. , , , ]. ]. ] ]. ]. , , , , , , , , , , , ]. ]. ]. | , , ]. |
ISAC | , ]. ]. | ]. ]. ]. |
THz | , ]. , ]. ] | ]. ]. ]. |
BC | , ]. ]. ]. ] | |
QC | , , ]. , ]. , ]. , , ] | ]. , ]. |
NTN | , ]. | |
MEC | , ]. ]. | |
RIS | ]. ] ] and high-precision positioning [ , ]. ] | |
IC | , , ]. , ]. ]. ]. | |
VLC |
Application (Use Case) | Benefits | Devices/Tech Used |
---|---|---|
Smart Routing | Avoidance of traffic congestion. Useful for emergency vehicles. Traffic balancing on roads. Reduction in emissions [ ] Reduce delays. | IOT sensors. Vehicle ad-hoc networks. AI real-time routing algorithms [ ]. Cloud and edge computing for data processing and analysis. |
Smart Parking | Contribution to sustainability. Optimal utilization of parking spaces. Reduced time for drivers to search for parking spaces. | V2V and V2I communication. Use of sensors for indicating parking status. AI and cloud computing. |
Speed Harmonization | Reduces frequent need for acceleration and deceleration. Continuous traffic flow. Reduces emissions. Safe travel. | AI and cloudification. Green-light coordination. |
Green Driving | Reduction of fuel consumption. Reduction of pollution near critical areas like hospitals. | Collection of pollution data by roadside sensors. Data transfer to centralized cloud. Traffic management decision based on AI algorithm. On-road displays for flashing traffic management decisions. |
Coordinated Maneuvers | Smooth traffic flow. Emission reduction. | V2I information exchange among vehicles and RSU [ ]. Low-latency, low-delay transmission. Advanced AI implemented at edge for delay-free decisions. |
The statements, opinions and data contained in all publications are solely those of the individual author(s) and contributor(s) and not of MDPI and/or the editor(s). MDPI and/or the editor(s) disclaim responsibility for any injury to people or property resulting from any ideas, methods, instructions or products referred to in the content. |
Share and Cite
Sharma, S.; Popli, R.; Singh, S.; Chhabra, G.; Saini, G.S.; Singh, M.; Sandhu, A.; Sharma, A.; Kumar, R. The Role of 6G Technologies in Advancing Smart City Applications: Opportunities and Challenges. Sustainability 2024 , 16 , 7039. https://doi.org/10.3390/su16167039
Sharma S, Popli R, Singh S, Chhabra G, Saini GS, Singh M, Sandhu A, Sharma A, Kumar R. The Role of 6G Technologies in Advancing Smart City Applications: Opportunities and Challenges. Sustainability . 2024; 16(16):7039. https://doi.org/10.3390/su16167039
Sharma, Sanjeev, Renu Popli, Sajjan Singh, Gunjan Chhabra, Gurpreet Singh Saini, Maninder Singh, Archana Sandhu, Ashutosh Sharma, and Rajeev Kumar. 2024. "The Role of 6G Technologies in Advancing Smart City Applications: Opportunities and Challenges" Sustainability 16, no. 16: 7039. https://doi.org/10.3390/su16167039
Article Metrics
Article access statistics, further information, mdpi initiatives, follow mdpi.
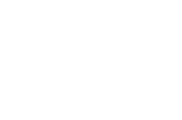
Subscribe to receive issue release notifications and newsletters from MDPI journals
Mobile computing
- October 1993
- Computer 26(9):81 - 82
- 26(9):81 - 82
- IEEE Xplore

- Carnegie Mellon University
Discover the world's research
- 25+ million members
- 160+ million publication pages
- 2.3+ billion citations
- J. Michael Barton
- Yajing Leng
- Jiwei Huang
- Ana Juan Ferrer
- Mahadev Satyanarayanan

- Brian Barritt

- Grace A. Lewis
- Brandon Lucia

- Hanan Elazhary
- J Venkata giri
- A S R Murty

- Senthil Kumar

- James J. Kistler

- David C. Steere
- M Satyanarayanan
- J J Kistler
- M E Okasaki
- Recruit researchers
- Join for free
- Login Email Tip: Most researchers use their institutional email address as their ResearchGate login Password Forgot password? Keep me logged in Log in or Continue with Google Welcome back! Please log in. Email · Hint Tip: Most researchers use their institutional email address as their ResearchGate login Password Forgot password? Keep me logged in Log in or Continue with Google No account? Sign up
IBM, NUS to set up new AI research and innovation centre
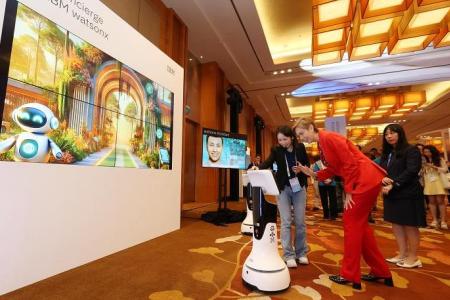
- Share on Twitter
- Share on WhatsApp
- E-mail this article
- 0 Engagements
Green computing and artificial intelligence safety will be among the focus areas of a new AI research and innovation centre, which is expected to be set up at the NUS School of Computing by 2025.
The proposed centre, a collaboration between IBM and NUS, aims to accelerate scientific research here by tapping the American tech giant’s full-stack AI infrastructure.
Minister for Digital Development and Information Josephine Teo announced the new centre at the IBM Think 2024 event held at the Sands Expo and Convention Centre on Aug 15.
Mrs Teo described IBM as a longstanding partner of the Republic in the area of AI, with many of the firm’s efforts serving the public good.
This will mark the first time IBM’s full-stack AI infrastructure system – the entire spectrum of software and technology required to build, test and deploy an application – is installed on a university campus in the Asia-Pacific region.
The AI-optimised computing infrastructure will operate on watsonx, a data and AI platform developed by IBM, as well as the company’s Red Hat hybrid cloud platform.

Tougher laws for those who misuse SIM cards for scams
Related stories, s’pore to upgrade national broadband network to 10gbps, mobile phone users to be given option to block overseas calls as part of new anti-scam measures, government has right to terminate funding for sph media if wrongdoings are found: josephine teo.
Government agencies and companies, as well as academic and research institutions, will be able to take advantage of the new centre to conduct cutting-edge AI research that can benefit people, NUS and IBM said in a statement.
“The proposed collaboration would leverage NUS’ expertise to drive technological progress in AI, enabling more powerful, efficient and versatile AI systems that can tackle increasingly complex tasks,” they said.
The centre will take a sustainability-focused open innovation approach to developing AI technologies – incorporating ideas from both external and internal sources – which has greater potential to improve the quality and pace of adoption of new AI technologies, NUS and IBM added.
They hope to work together to develop tools and methodologies to help build trust in AI.
“IBM and NUS share a common goal to enable innovations in AI and sustainable computing, and we look forward to furthering this collaboration,” said IBM Research hybrid cloud and AI platform vice-president Priya Nagpurkar.
Professor Liu Bin, NUS’ deputy president for research and technology, said the university was “very excited” about the opportunity to collaborate with IBM.
“Building on the new NUS AI Research Institute announced earlier this year and the university’s commitment to green computing and sustainability, we aim to be the leading force in addressing rising industry demand for AI intelligence, cultivating a robust talent pool and contributing to Singapore’s decarbonisation efforts,” she said.
As part of efforts to grow local deep-tech start-ups, the collaboration will also allow the NUS Graduate Research Innovation Programme (Grip), together with local start-ups as well as small and medium-sized enterprises, to access technologies such as IBM’s watsonx platform and Red Hat OpenShift AI.
Grip is an initiative to help launch start-ups stemming from university research.
IBM and NUS did not say how much money was being invested in the new centre.
It is separate from the NUS AI Institute, launched in March, which conducts research on addressing ethical concerns associated with AI as well as the technology’s application across areas such as education and healthcare.
IBM was one of the university’s collaborators in the NUS AI Institute.
NUS said that while the two initiatives are separate, it expects “a lot of synergy” in the research efforts of the NUS AI Institute and the new centre.
Mrs Teo, who is also Minister-in-charge of Smart Nation and Cybersecurity, described the project as an example of how Singapore’s AI ecosystem is “steadily building up”.
The Government appreciates the potential for AI to serve the public good, she added.
She pointed to Readliao, an AI-enabled tool developed by Open Government Products – an independent division of the Government Technology Agency – which provides the elderly with simplified summaries of letters from the authorities, so they can better understand them.
“We will continue to bring together industry, government and academia for meaningful partnerships that we can all benefit from,” she said.
Get The New Paper on your phone with the free TNP app. Download from the Apple App Store or Google Play Store now
- Share on Facebook
Pardon Our Interruption
As you were browsing something about your browser made us think you were a bot. There are a few reasons this might happen:
- You've disabled JavaScript in your web browser.
- You're a power user moving through this website with super-human speed.
- You've disabled cookies in your web browser.
- A third-party browser plugin, such as Ghostery or NoScript, is preventing JavaScript from running. Additional information is available in this support article .
To regain access, please make sure that cookies and JavaScript are enabled before reloading the page.

IMAGES
COMMENTS
Explore the latest full-text research PDFs, articles, conference papers, preprints and more on MOBILE COMPUTING. Find methods information, sources, references or conduct a literature review on ...
Finally, we highlight several research issues and future directions relevant to our analysis in the area of mobile data science and intelligent apps. Overall, this paper aims to serve as a reference point and guidelines for the mobile application developers as well as the researchers in this domain, particularly from the technical point of view.
Mobile cloud computing promises several benefits such as extra battery life and storage, scalability, and reliability. However, there are still challenges that must be addressed in order to enable the ubiquitous deployment and adoption of mobile cloud computing. Some of these challenges include security, privacy and trust, bandwidth and data ...
Definition of Mobile Computing. Mobile computing refers to the computing that happens when the user interacts while the computer or parts of the computer are in motion during the use. Hardware components (like computing silicon, various sensors, and input/output devices), software components (like programs that communicates with underlying ...
Mobile Cloud Computing broadly focuses on offloading the two vital processes of data processing and data storage. The issues are specifically in energy, QoS (Quality of Service), application, and Security. Because of the numerous advancements in MCC, industries and a wide range of sectors are resorting to MCC.
This paper introduces the conceptual overview of mobile computing, its achievements, challenges and opportunities. The current status and ongoing research projects in mobile computing worldwide are detailed. This paper also discusses the two Australian workshops on mobile computing, databases and applications held in 1996 and 1997.
Together with an explosive growth of the mobile applications and emerging of cloud computing concept, mobile cloud computing (MCC) has been introduced to be a potential technology for mobile services.
The rest of the paper analyses the challenges of mobile cloud computing, summary of some research projects related to this area, and points out promising future research directions.
A main thrust of MEC research is to seamlessly merge the two disciplines of wireless communications and mobile computing, resulting in a wide-range of new designs ranging from techniques for compu-tation offloading to network architectures.
With the explosion of mobile applications and the support of CC for a variety of services for mobile users, mobile cloud computing (MCC) is introduced as an inte-gration of CC into the mobile environment. MCC brings new types of services and facilities mobile users to take full advantages of CC. This paper presents a comprehensive survey on MCC.
Issues and challenges of mobile cloud computing. The author in [1 8 ] presents different issues and challenges in. the field of MCC and the issues are related to the different. factors like end ...
Google engineers and researchers work on a wide range of problems in mobile computing and networking, including new operating systems and programming platforms (such as Android and ChromeOS); new interaction paradigms between people and devices; advanced wireless communications; and optimizing the web for mobile settings.
This vision p ses new challenging problems to We have categorized research challenges idata the database commlmity. Howis the mobility of management for mobile wireless computing into users going toaffect da distribution, a query pro- roughly four o thogonal categories: cessing and transaction processing?
The rapid advance of mobile computing technology and wireless networking, there is a significant increase of mobile subscriptions. This drives a strong demand for mobile cloud applications and services for mobile device users. This brings out a great business and research opportunity in mobile cloud computing (MCC). This paper first discusses the market trend and related business driving ...
Mobile cloud computing promises several benefits such as extra battery life and storage, scalability, and reliability. However, there are still challenges that must be addressed in order to enable the ubiquitous deployment and adoption of mobile cloud computing. Some of these challenges include security, privacy and trust, bandwidth and data ...
Mobile Cloud Computing: Challe nges and Future Research Directions. Samaher Al-Janabi. Department of Computer Science, Faculty of Science for Women (SCIW), University of Babylon, Babylon, Iraq ...
An increasing number of research works are exploring the interplay between mobile computing and IoT to enable the facilitation and development of more fine-grained, personalised, and enriched services. While mobile computing for IoT is of enormous potential, it also faces several challenges.
It then analyses the features and infrastructure of mobile cloud computing. The rest of the paper analyses the challenges of mobile cloud computing, summary of some research projects related to this area, and points out promising future research directions.
Find the latest published documents for mobile computing, Related hot topics, top authors, the most cited documents, and related journals
The analysis of literature review suggests filling a research gap to present formal models for estimating mobile application considering specific characteristics of mobile software. Previousarticlein issue Nextarticlein issue Keywords
Mobile Edge Computing: A Survey. Nasir Abbas, Yan Zhang, Senior Member, IEEE, Amir Taherkordi, Member, IEEE, and Tor Skeie. Abstract—Mobile edge computing (MEC) is an emergent architecture where cloud computing services are extended to the edge of networks leveraging mobile base stations. As a promising edge technology, it can be applied to ...
This paper proposes "SenseCrypt", a framework that automatically annotates and signcrypts sensitive location information of mobile crowd sensing users and incorporates spatial coding as the data compression technique and message query telemetry transport as the messaging protocol.
We examine the rise of cloud computing and AI in China and their impacts on industry dynamics after the shock to the cost of Internet-based computing power and services. We find that cloud computing is associated with an increase in firm entry, exit and the likelihood of M&A in industries that depend more on cloud infrastructure.
Feature papers represent the most advanced research with significant potential for high impact in the field. A Feature Paper should be a substantial original Article that involves several techniques or approaches, provides an outlook for future research directions and describes possible research applications.
PDF | The problems, limitations, and potential advantages of mobile computing systems are discussed. It is suggested that the constraints violate many... | Find, read and cite all the research you ...
Cloud computing is essential for transformation. Discover how embracing cloud technology can drive innovation and prevent your organization from falling behind. Learn more today.
The selected papers were divided into three categories such as research studies relevant to the hardware component, software and communication component related to mobile computing were separated.
Your official source for the latest T-Mobile news and updates, along with the newest devices, offers, and stories from the world of T-Mobile.
Green computing and artificial intelligence safety will be among the focus areas of a new AI research and innovation centre, which is expected to be set up at the NUS School of Computing by 2025. The proposed centre, a collaboration between IBM and NUS, aims to accelerate...
This assessment requires students to read and understand a high-quality research paper (from a list we provide) in the area of Mobile and Distributed Computing, and then critically analyse and evaluate the paper and present its key points in a 10-minutes presentation.