- ASME Foundation
- Sections & Divisions
- Back to Main Menu
- Access Benefits
- Communication Preferences
- Digital Downloads
- Purchase History
- Committee History
- Sign In/Create Account
- Publications & Submissions
- Find Journal

Journal of Mechanical Design
This Standard was last reviewed and reaffirmed in {{activeProduct.ReaffirmationYear}}. Therefore this version remains in effect.
{{activeProduct.DisplayTitle}}
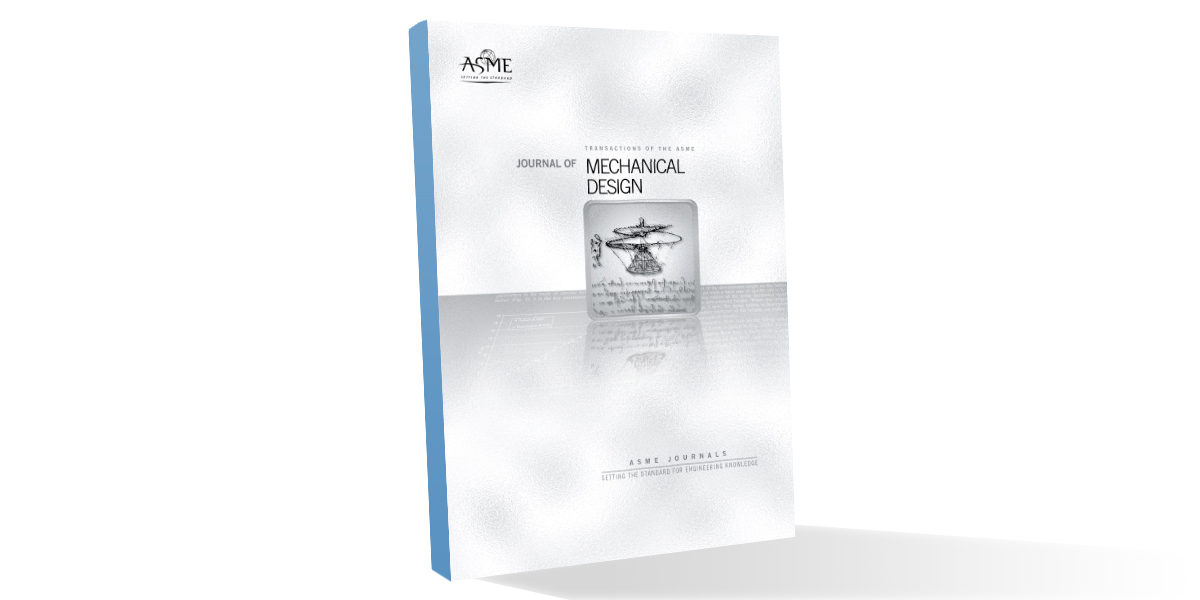
Digital products are restricted to one per purchase.
{{activeProduct.CurrencySymbol}}{{ formatPrice(activeProduct.ListPrice) }} activeProduct.ListPrice"> was {{activeProduct.CurrencySymbol}}{{ formatPrice(originalPrice) }}
{{activeProduct.CurrencySymbol}}{{ formatPrice(activeProduct.ListPriceSale) }} activeProduct.ListPriceSale"> was {{activeProduct.CurrencySymbol}}{{ formatPrice(activeProduct.ListPrice) }}
{{activeProduct.CurrencySymbol}}{{ formatPrice(activeProduct.ListPriceSale) }} activeProduct.ListPriceSale"> was {{activeProduct.CurrencySymbol}}{{ formatPrice(originalPrice) }}
0}"> {{activeProduct.CurrencySymbol}}{{ formatPrice(activeProduct.MemberPrice) }} activeProduct.MemberPrice"> was {{activeProduct.CurrencySymbol}}{{ formatPrice(originalPrice) }}
0"> {{activeProduct.CurrencySymbol}}{{ formatPrice(activeProduct.MemberPriceSale) }} activeProduct.MemberPriceSale"> was {{activeProduct.CurrencySymbol}}{{ formatPrice(originalPrice) }}
Become a member
*Excluding Lite Members
Final invoices will include applicable sales and use tax.
Print or Share
Journal options.
- Format Availability Order No. Price List Price Member Price
- Print Journal Ships in 3-5 Days MD List $1343 Member $135 $1343 $135 Select Selected
- Online Journal Immediately MDOL List $1000 Member $99 $1000 $99 Select Selected
The Journal of Mechanical Design publishes technical papers concerned with design automation, including design representation, virtual reality, geometric design, design evaluation, design optimization, risk and reliability-based optimization, design sensitivity analysis, system design integration, ergonomic and aesthetic considerations, and design for market systems; design of direct contact systems, including cams, gears, and power transmission systems; design education; design of energy, fluid, and power handing systems; design innovation and devices, including design of smart products and materials; design for manufacturing and the life cycle, including design for the environment, DFX, and sustainable design; design of mechanisms and robotic systems, including design of macro-, micro- and nano-scaled mechanical systems, machine component, and machine system design; design theory and methodology, including creativity in design, decision analysis, design cognition, and design synthesis.
Published: Monthly
Subscriptions currently include the years 2000 - present. Access to archived content prior to the year 2000 can be obtained with an institutional subscription or an ASME Member Article Pack.
Please allow three to five days for electronic subscription activation.
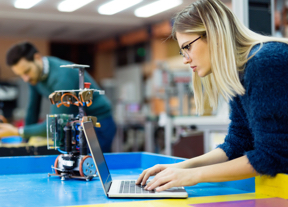
Publisher: STM Journals, an imprint of CELNET (Consortium e-Learning Network Pvt. Ltd.)
Address: A-118, 1st Floor, Sector-63, Noida, Uttar Pradesh-201301, India
Phone no.: 0120-478-1242/ Email: [email protected]
Vol 3, No 2 (2016)
Table of contents.
MACHINE DESIGN
PUBLISHED BY: University of Novi Sad Faculty of Technical Sciences ISSN 1821-1259 Print e-ISSN 2406-0666 Online
- General Information
- Editorial Policy
- Editors and Editorial Board
- Submission and Review process
- Current Issue (Volume 12, Number 4)
- Author Guidelines
- Volume 12 (2020)
- Volume 12, Number 1
- Volume 12, Number 2
- Volume 12, Number 3
- Volume 12, Number 4
- Volume 11 (2019)
- Volume 11, Number 1
- Volume 11, Number 2
- Volume 11, Number 3
- Volume 11, Number 4
- Volume 10 (2018)
- Volume 10, Number 1
- Volume 10, Number 2
- Volume 10, Number 3
- Volume 10, Number 4
- Volume 9 (2017)
- Volume 9, Number 1
- Volume 9, Number 2
- Volume 9, Number 3
- Volume 9, Number 4
- Volume 8 (2016)
- Volume 8, Number 1
- Volume 8, Number 2
- Volume 8, Number 3
- Volume 8, Number 4
- Volume 7 (2015)
- Volume 7, Number 1
- Volume 7, Number 2
- Volume 7, Number 3
- Volume 7, Number 4
- Volume 6 (2014)
- Volume 6, Number 1
- Volume 6, Number 2
- Volume 6, Number 3
- Volume 6, Number 4
- Volume 5 (2013)
- Volume 5, Number 1
- Volume 5, Number 2
- Volume 5, Number 3
- Volume 5, Number 4
- Volume 4 (2012)
- Volume 4, Number 1
- Volume 4, Number 2
- Volume 4, Number 3
- Volume 4, Number 4
- Volume 3 (2011)
- Volume 3, Number 1
- Volume 3, Number 2
- Volume 3, Number 3
- Volume 3, Number 4
- Volume 2 (2010)
- Volume 2, Number 1
- Volume 1 (2009)
- Volume 1, Number 1
GENERAL INFORMATION
Dear Colleagues, the journal Machine Design publishes fundamental research about mechanical engineering and design including machineelements, design fundamentals, computer aided design, product forms, shapes and performances, manufacturing processes and technologies, theory of materials, its structures and capabilities, product design management, technology management, communication and cognitive science. The journal Machine Design is published by the Faculty of Technical Sciences in Novi Sad four issues per year . Publishing this journal we would like to make mechanical engineering more interesting and to promote it as an important branch of engineering in the light of modern techniques and new technologies. The journal is a good opportunity to show and present the results of our recent work and researching. Also, it is a chance for leader researchers and scientists in the field of machine design from abroad to represent their researching results. In such way, we would like to obtain insight in the present situation of mechanical engineering in the region, to know and learn about researching in other institutions, to compare results and find out new solutions, as well as to make new contacts and find out mutual interests for international cooperation and researching on a project or some topic. The journal Machine Design is on the Index Copernicus international journals master list and on DOAJ – Directory of Open Access Journals . Its editorial board will try further to develop this publication in order to achieve and maintain a high quality of publications, so we can receive an Impact factor. Our goals are to be referred in international publication databases, to provide an international medium for scientific contribution and participation to mechanical engineers and to create a platform for the communication between science and industry in the field of technical sciences. Also, we would like to promote and to encourage international cooperation, mutual researching, projects and publishing papers between foreign partners’ institutions. Thus, we want to help better understanding and knowing about work and researching of colleagues from all over the world. I hope You will recognize the interest to publish Your paper in the journal Machine Design ; so, with a great pleasure, I call You to send further Your papers for this journal.
With deep respect and gratitude, Editors, Siniša Kuzmanović and Milan Rackov
Machine Design is currently indexed by
CONTACT INFO
Address: Faculty of Technical Sciences Trg Dositeja Obradovića 6 21000 Novi Sad Serbia
Telephone numbers: +381 21 485 2358 +381 64 153 22 67 +381 64 190 31 04 Fax number: +381 21 6350 592
Email: [email protected]
Web address: www.mdesign.ftn.uns.ac.rs
PUBLISHER: University of Novi Sad, Faculty of Technical Sciences, Trg Dositeja Obradovića 6, 21000 Novi Sad, Serbia SUPPORTED BY: ADEKO, Association for Design, Elements and Constructions CEEPUS III RS0304; CEEPUS III PL0033; CEEPUS III BG0703 EDITORS: Siniša KUZMANOVIĆ Milan RACKOV ISSN 1821-1259 Print e-ISSN 2406-0666 Online
Academia.edu no longer supports Internet Explorer.
To browse Academia.edu and the wider internet faster and more securely, please take a few seconds to upgrade your browser .
- We're Hiring!
- Help Center
Machine Design
- Most Cited Papers
- Most Downloaded Papers
- Newest Papers
- Save to Library
- Last »
- Engineering Design Follow Following
- Wind Energy Follow Following
- Wind Energy (Engineering) Follow Following
- Applied Dynamics Follow Following
- Mechanical Engineering Follow Following
- Engineering Follow Following
- Design of Machine Elements Follow Following
- Control Engineering Follow Following
- Mechanical Vibration and Machinery System Dynamics Follow Following
- Vibrations Follow Following
Enter the email address you signed up with and we'll email you a reset link.
- Academia.edu Publishing
- We're Hiring!
- Help Center
- Find new research papers in:
- Health Sciences
- Earth Sciences
- Cognitive Science
- Mathematics
- Computer Science
- Academia ©2024
Advertisement
Design of experiments and machine learning with application to industrial experiments
- Regular Article
- Open access
- Published: 26 March 2023
- Volume 64 , pages 1251–1274, ( 2023 )
Cite this article
You have full access to this open access article
- Roberto Fontana ORCID: orcid.org/0000-0002-3989-4887 1 ,
- Alberto Molena 2 ,
- Luca Pegoraro 2 &
- Luigi Salmaso 2
6276 Accesses
3 Citations
Explore all metrics
In the context of product innovation, there is an emerging trend to use Machine Learning (ML) models with the support of Design Of Experiments (DOE). The paper aims firstly to review the most suitable designs and ML models to use jointly in an Active Learning (AL) approach; it then reviews ALPERC, a novel AL approach, and proves the validity of this method through a case study on amorphous metallic alloys, where this algorithm is used in combination with a Random Forest model.
Similar content being viewed by others
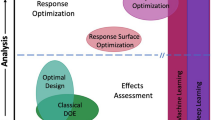
Prospective on methods of design of experiments for limited data scenarios in materials design and engineering
Emily Ryan, Athar Roshandelpoor, … Pirooz Vakili
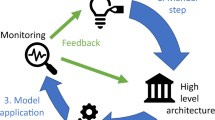
MLOps Challenges in Industry 4.0
Leonhard Faubel, Klaus Schmid & Holger Eichelberger
On the Use of Process Mining and Machine Learning to Support Decision Making in Systems Design
Avoid common mistakes on your manuscript.
1 Introduction
In the context of product innovation, there is an emerging trend to use Machine Learning (ML) models with the support of Design Of Experiments (DOE). In this work DOE, often refers both to methods for experimental design generation and to regression models, like polynomial models. These two topics have very different backgrounds. DOE can be perceived as a classic technique, because there are industrial applications involving this topic that date back to the 1950 s and earlier Bisgaard ( 1992 ), while use of ML in industry can be considered quite recent. Moreover, DOE has a precise and organised approach that leans on a vast and established body of literature, while ML is still mainly application-oriented. Another relevant difference between these two disciplines is the fact that while DOE tends to favour inference over predictions, allowing the experimenter to understand the existing relationships between input factors and output responses, ML models tend to behave as black boxes. Especially when the underlying phenomenon has a non-linear behaviour, the predictive performances of ML models are not always met by the traditional approaches used in DOE. What makes ML models very interesting is their ability to continuously learn and improve as more data are supplied: this characteristic matches with the principle of sequential experimentation in DOE. In ML literature, Active Learning (AL) is a kind of supervised learning technique devoted to the iterative collection of the most informative data points, with the aim of maximising information gain Olsson ( 2009 ).
From the analysis of the literature, it is possible to affirm that if we consider DOE and ML individually, their respective research areas are broad and have been intensively investigated; but things change if we consider these two topics jointly. Two different works Arboretti et al. ( 2022 ) and Freiesleben et al. ( 2020 ) state that there are few papers that consider DOE and ML jointly. Nevertheless, it is possible to identify Arboretti et al. ( 2022 ) two main currents: one concerns the utilization of ML techniques in order to analyse data that have been collected according to a DOE, and the other regards the use of DOE to optimize the training process of ML algorithms. As regards the first, in recent years the application of DOE and ML has begun to take hold, with several applications in many fields. We will delve into the analysis of this category the next section. The second current contains various contributions on the use of DOE as a method for choosing the best combination of hyperparameters for ML models: the contributions of Lujan-Moreno et al. ( 2018 ) and Staelin ( 2003 ) represent two examples of this. A systematic literature review on ML and DOE for product innovation performed by Arboretti et al. ( 2022c ) showed that in recent years the interest on DOE+ML has grown and, in 2019 and 2020 there was a spike in the publication of papers about this topic. Moreover, Arboretti et al. ( 2022c ) has shown that the typical application of the DOE+ML framework is non-sequential; only 8 out of the 82 analysed papers included the use of some features of the ML model to suggest the choice of the next experimental configurations.
The aim of this paper is firstly to provide a solid review of some contributions in the field of AL: to this purpose, Sect. 2 reviews a contribution about the choice of the best design and ML method for a joint application in the context of the prediction of a phenomenon of interest in physical experiments. Section 3 describes ALPERC, a recently-developed AL approach suitable for physical experiments. It is compared with other AL approaches in Sect. 4 . The main novelty element of the paper is discussed in Sect. 5 , that presents a real case study, in which this new AL approach is jointly used with a Random Forest (RF) model. Conclusions are in Sect. 6 .
2 Experimental designs and machine learning models
In this section, the connection between experimental designs and ML models is investigated. The aim of this part is to carry out a review to understand which experimental design is more suitable for a joint application with ML models when the global focus is on the prediction of a phenomenon of interest. In the following sections, we will analyse a study by Arboretti et al. ( 2022b ), that considers 12 experimental designs, which will be presented in Sect. 2.1 and 7 different ML models, presented in Sect. 2.2 . These will be tested considering 7 test functions (each test function is a computer simulator which models a physical phenomenon) under 8 different noise settings, including both homoschedastic and heteroschedastic noise.
2.1 Experimental designs
Table 1 , contains a summary of the different DOEs settings which have been studied. It is possible to distinguish three main categories of experimental designs: “classical designs”, “optimal designs” and “space-filling designs”. There are six factors, with three levels each. The same number of runs (52) was allocated to each DOE, in order to provide a fair comparison between the different designs.
The data collected through the different experimental designs were used to predict the behaviour of different test functions, that are deterministic functions, mainly simulating some physical processes, described in Table 2 . These test functions are detailed in the supplemental material of Arboretti et al. ( 2022b ). The dependent variables were standardized:
where \(y_n^{\textrm{std}}\) is the standardized value corresponding to \(y_n\) (the observed value for the n -th observation), \(\overline{y}\) and \(s_y\) are the mean and standard deviation of y respectively. 100 random Latin Hypercube Designs (LHDs) with 500, 000 observations each are used for computing \(\overline{y}\) and \(s_y\) .
The classical designs category includes Central Composite Designs (CCDs), Box-Behnken Designs (BBDs) and Full Factorial Designs (FFDs), which are among the most used designs when it comes to data collection in ML studies. The optimal design category includes D-optimal and I-optimal designs. It is worth noting that
both FFD and D_opt are 52-run D-optimal designs which have been generated using a \(6^6\) full factorial design as candidate set. The difference lies in the algorithms used for their construction;
both D_opt and I_opt have been generated without adapting their criteria functions to take into account heteroschedasticity.
Space-filling designs, namely Random Latin Hypercube Designs (LHD_rand) and MaxPro space-filling designs (MAXPRO), are almost exclusively used in computer experiments because they have too many levels for the factors. This makes the experimentation too costly or unfeasible when it comes to physical experiments; however, these designs were included in the analysis to provide a benchmark for researchers working in computer experiments. Lastly, it is crucial to underline that in this analysis also a “hybrid” design, derived from the space-filling literature but with characteristics that enable its applications also on physical experiment, was considered. This hybrid design is the MaxPro discrete numeric design (MAXPRO_dis) Joseph et al. ( 2020 ). Also, the role of replication was investigated in the simulation study: additional D-Optimal, I-Optimal, MAXPRO_Dis designs with 50% level of replication and a MAXPRO_Dis with 25% level of replication were included. The 50% level of replication of D_opt, I_opt, and MAXPRO_dis (D_opt_50%repl, I_opt_50%repl, and MAXPRO_Dis_50%repl, respectively) have been obtained generating optimal 26-run designs and replicating them twice. This type of procedure is often performed in DOE studies concerning physical experiments. The 25% level of replication (MAXPRO_dis_25%repl) has been obtained generating a 39-run MAXPRO discrete design and randomly choosing 13 runs out of the 39 to be replicated once.
2.2 Machine learning models
As regards machine learning models, from some evidence in the literature review conducted by Arboretti et al. ( 2022c ) it has emerged that Artificial Neural Networks (ANNs) are the most used models for the analysis of DOE when the focus is on prediction. Two different types of ANNs were used: ANN shallow (ANN_sh), which is an ANN with one hidden layer and a number of neurons chosen in the range \([3-12]\) and ANN deep (ANN_dp), which is an ANN with multiple (2 to 4) hidden layers, and either 6 or 12 neurons per layer. Several other models were considered, including Support Vector Regression models (SVRs), Gaussian Processes (GPs), which are the most used when it comes to computer simulation, Linear Models (LMs) based on quadratic regression with interactions, Random Forests (RFs) and other models contained within the Automated Machine Learning (aml) platform offered by H2O LeDell and Poirier ( 2020 ). Both homoscedastic and heteroscedastic noise situations were considered (Table 3 ). Let \(s_y\) , \(\min y\) , and \(\max y\) be the standard deviation, the minimum, and the maximum of y computed using the previously mentioned 100 random LHDs, respectively. The homoschedatic case assumes noise components in the form \(\epsilon \sim \mathcal {N}(0,\sigma _{hom}^2)\) where \(\sigma _{hom}=k s_y\) with \(k=0\%, 5\%, 12.5\%, 20\%, 50\%\) ; \(k=0\%\) corresponds to the deterministic case. The heteroschedatic case assumes noise components in the form \(\epsilon \sim \mathcal {N}(0,\sigma _{het}^2)\) where \(\sigma _{het}\) increases linearly with the value of \(y=f(\textbf{x})\) . More specifically, at a given value \(\textbf{x}\) of the input \(\sigma _{het}=0.05 s_y+ a(f(\textbf{x})-\min y)\) where \(a=(m-0.05)s_y/(\max y - \min y)\) , \(m=50\%,100\%,500\%\) . The minimum value of \(\sigma _{het}\) is \(0.05 s_y\) for all cases and the maximum values of \(\sigma _{het}\) are \(0.5 s_y\) , \(s_y\) , and \(5 s_y\) . Each model has been implemented after a careful tuning of the hyperparameters with the objective of minimizing the Root Mean Square Error (RMSE).
2.3 Results and discussion
In the paper by Arboretti et al. ( 2022b ) a methodology based on nonparametric permutation tests was used to evaluate the different designs and models. This approach is also described in Arboretti et al. ( 2014 ).
2.3.1 Ranking of DOEs
Tables 4 and 5 report the final rankings of the experimental designs. The rankings are based on RMSE. Table 4 reports the final ranks of the designs in the homoscedastic noise settings, while Table 5 reports the final ranks of the designs in the heteroscedastic noise settings. These tables should be read column-wise because the relative ranks are computed for each noise setting. Then, by adding up all the different positions obtained by each design for all noise settings we obtained the overall ranking. For example, considering Table 4 , by adding up all the positions in the different noise settings for FFD we get 9 (it is the sum of the values in the first row); this value is the lowest obtained among all designs; that’s why the position of FFD in the ranking is the first one. It is possible to observe that the choice of the experimental design has an impact on the quality of the outcome of the analysis. Focusing on the homoscedastic case, the three best ranked designs are FFD, MAXPRO_dis and MAXPRO, closely followed by the I-optimal design. The presence of replicates makes the predictions worse; D_opt_50%repl, I_opt_50%repl, MAXPRO_dis_50%repl and MAXPRO_dis_25%repl are among the worst performers. The situation is different when it comes to the heteroscedastic noise setting. The best performer is MAXPRO_dis, ranking first for the intermediate and severe noise situations, and second in the case of moderate heteroscedasticity. In this situation the two worst performers are the CCD and the D_opt_50%repl. If we jointly consider the homoscedastic and the heteroscedastic noise settings, it is possible to state that the best overall performer is the MAXPRO_dis. For this reason, we can affirm that even if a heteroscedastic noise, not expected or initially detected, appears, the best choice would be the MAXPRO_dis design, as it results among the best methods in the homoscedastic case (as it may be observed in Table 4 ) and the best method in the heteroscedastic case (Table 5 ). The results obtained by MAXPRO_dis may be justified by the fact that it uses the space-filling criterion which leads to a combination of the factor settings that maximises the ability of several different predictive models to capture the non-linearity of the underlying functions. At the same time the limited number of factors levels makes the design robust to the presence of noise and applicable for physical experiments. This design performs better than all the other designs with the same number of factor levels, FFD, D_opt and I_opt, particularly in the heteroschedastic setting. A possible explanation to this phenomenon is represented in Fig. 1 , which visually compares the ability of different designs to appropriately fill the design space, while sharing the same characteristics for factor levels and runs. The space-filling criterion at the basis of the MAXPRO_dis enables a better filling of the design space favours flexible non-linear predictive models in capturing the behaviour of the underlying function across the whole experimental region.
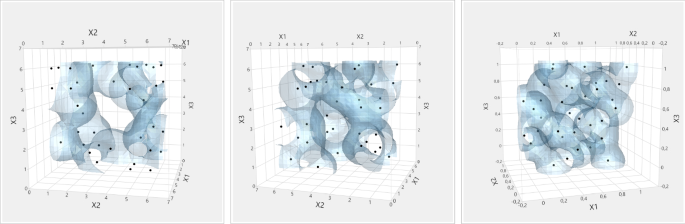
Visualization of the space-filling capability of D_opt (left figure), I_opt (center figure) and MAXPRO_dis (right figure)
It is worth underlining the difference, in the performances, between two classical designs: BBDs and CCDs. From this simulation study it has emerged that the BBDs performs better than the CCDs, especially in settings influenced by large noise. If we analyse the performances of the replicated design, it is possible to observe that these kinds of designs show some advantages only as the noise becomes larger and especially in the input dependent noise case. A possible explanation for this phenomenon is that the exploration of a smaller number of unique input configurations, in the replicated designs, weakens the ability of the predictive model to learn the behaviour of the underlying test functions; it seems that replicated design should only be preferred if the underlying phenomenon is severely affected by heteroscedasticity.
2.3.2 Predictive models
The strategy used in order to rank the different predictive models is equivalent to the one used to rank the designs, with the only difference that, in this second case, the groups are dependent since for each experimental design the same data were used in order to train the model.
From the results of the simulation, shown in Tables 6 and 7 , it is evident that the choice of a specific prediction model widely impacts the results of the analysis. In the situation of homoscedastic noise, represented in Table 6 , the best model is the Gaussian Process, since it ranked first in all the five cases. The performances of this model are also excellent in the situation of presence of heteroscedastic noise. This model ranks first in the low and medium noise settings and third in the high noise setting. It is also possible to state that LM is the second-best option when it comes to situation affected by homoscedastic noise, while SVM and ANN_sh are respectively the third and the fourth options in this specific situation. RF, ANN_dp and aml behave in an unsatisfactory manner in this situation. In the case of presence of heteroscedastic noise, represented in Table 7 , it is possible to observe that the SVM performs very well, indeed it is the best performer in the case of low uncertainty (together with the GP) and high uncertainty. The results obtained by LM, RF and ANN_sh can be considered as acceptable, while aml and ANN_dp perform in an unsatisfactory manner also in this situation. Lastly it is important to underline that in the simulation the focus was only on the predictive performance, and that other fundamental aspects, such as the quantification of uncertainty and the model interpretability weren’t considered, even if these are two crucial factors to consider in order to obtain robust and trustworthy results that may support decision making in real industrial applications. More details about this simulation study can be found in Arboretti et al. ( 2022b ).
3 The ALPERC method
In this section the aim is to firstly introduce some notions about AL, then to present and review the theoretical aspects of ALPERC, an iterative approach based on non parametric ranking and clustering suitable for physical experiments, recently proposed by Arboretti et al. ( 2022a ).
3.1 Active Learning
The general framework of the AL technique requires three core ingredients: (1) an initial dataset \({\Phi }_0=[\textbf{A}_0 \ \textbf{Y}_0]\) where \(\textbf{A}_0\) is the \(n_0 \times d\) matrix whose rows are the \(n_0\) input configurations \(\textbf{x}_i=(x_{1i},\ldots ,x_{di}), i=1,\ldots ,n_0\) , and \(\textbf{Y}_0\) is the \(n_0 \times c\) response matrix whose rows are the vectors \(\textbf{y}_i = (y_{1i},\ldots ,y_{ci})\) of the c dependent variables corresponding to the input vector \(\textbf{x}_i, i=1,\ldots ,n_0\) . (2) c predictive models, developed on the dataset \({\Phi }_0\) and lastly (3) a criterion that uses some features of the model to propose which experimental configurations should be added to the dataset at the subsequent iterations. When this configuration is defined and added to the dataset, the above described steps are iterated until a stopping condition is reached.
The idea underlying this process is that by collecting data on the most informative input configurations it is possible to achieve the goal of the study more efficiently in terms of time and required resources.
Among the first algorithms proposed for the emulation of complex functions by sequential data acquisition there are the active learning MacKay (ALM) and the active learning Cohn (ALC). ALM, Yue et al. ( 2021 ), adds the input configurations which are characterised by the highest predictive uncertainty, maximising the expected information gain; two important features of this algorithm favoured its wide diffusion: it is intuitive and easy to be implemented. On the other hand, ALC, Gramacy and Lee ( 2009 ) proposes for inclusion those data points that minimize the expected integrated variance over the entirety of the input space. This results in the selection of those \(\mathbf {x'}\) points that maximise the expected reduction in predictive uncertainty in the input space as in the formula:
where \(\sigma ^2(\textbf{x})\) represents the estimated variance in \(\textbf{x}\) given the currently available observations and \(\sigma ^2_{\mathbf {x'}}(\textbf{x})\) is the expected predictive variance in \(\textbf{x}\) when the configuration \(\mathbf {x'}\) is included. Other approaches are proposed in the literature, like the one in Binois et al. ( 2019 ). One common characteristic between all the proposed AL criteria is that they all exploit a quantification of the predictions of the uncertainty to select subsequent experimental configurations. Another common trait between all the analysed criteria is that they deal with the analysis of computer experiments, while in this article the interest is on physical experiments.
Arboretti et al. ( 2022a ) propose ALPERC, an AL approach which is based on nonparametric Ranking and Clustering and is suitable in Physical Experiments. ALPERC can be implemented for sequential data collection when three or more response variables are investigated in the same experiment in noisy settings. This algorithm is based on the combination of different building blocks:
an experimental design for collection of data at the first iteration and a set of candidate points from which it is possible to choose new input configurations in the subsequent iterations;
a predictive model developed on the available data which provides a quantification of the uncertainty of candidate points;
a variable importance technique;
a ranking procedure to obtain an inferential rank of candidate configurations concerning predictive uncertainty;
a clustering procedure that groups candidate configurations with respect to continguity in the design space.
The underlying idea is to propose a model-agnostic AL methodology, with the only strict condition that the predictive models must provide an appropriate quantification of uncertainty of predictions. In Arboretti et al. ( 2022a ) the focus was on Gaussian Process models, as they are the most common in the AL literature and they can directly provide a quantification of uncertainty, but other models can also be used, as we will see in the case study. As regards the variable importance, the choice of the appropriate technique depends on the predictive model selected. In case Gaussian Process models are selected, an appropriate strategy consists of the use of the Sobol’ indices. These consider independent input variables and quantify the relative importance of one input dimension as the partial variance of model output explained by this variableWei et al. ( 2015 ).
Let’s consider an objective function \(f(\textbf{x})\) , that is assumed to be square-integrable; Sobol’s method considers the functional decomposition of \(f(\textbf{x})\) :
where \(f_0\) is a costant that represents the mean value of \(f(\textbf{x})\) , \(f_i(x_i)\) is the main effects of \(x_i\) , \(f_{ij}(x_i,x_j)\) is the interaction effect between two different factors \(x_i\) and \(x_j\) , and \(f_{i_1 \ldots i_k}(x_{i_1},\ldots ,x_{i_k})\) is the interaction effect among the factors \(x_{i_1}, \ldots x_{i_k}\) , \(k>2\) , and \(i_1<\ldots <i_k\) .
Sobol demonstrates that if the input variables are independent and \(f(\cdot )\) is square integrable, from Eq.( 2 ) the variance associated to the model response Y can be written as:
where \(V_i = V(\mathbb {E}(Y|x_i))\) , \(V_{ij} = V(\mathbb {E}(Y|x_i,x_j)) - V_i - V_j \) and so on.
The first-order sensitivity indices \(S_i\) are expressed as:
The index \(S_i\) measures the proportion of variability in the response that is attributable to the i -th input variable. Another relevant indicator is the total partial variance \(V_{T_i}\) associated to the i -th input variable, as it considers not only the main effect of the i -th input, but also its interaction effects with all the other \(d-1\) input variables \(\textbf{x}_{\sim i}=(x_1,\dots ,x_{i-1},x_{i+1},\dots ,x_d)\) . From Eq.( 3 ), \(V_{T_i}=V(Y)-V(\mathbb {E}(Y|\textbf{x}_{\sim i}))\) is obtained. The total sensitivity index \(S_i\) can be computed as:
In practical applications Sobol’ indices can be obtained by Monte Carlo simulations. Sobol ( 2001 )
The problem of clustering, which is an unsupervised classification task, is related to the grouping of objects based on some measure of similarity. In Arboretti et al. ( 2022a ) a hierarchical agglomerative clustering algorithm based on weighted Euclidean distance was proposed. The choice of this criterion was made because this algorithm results more stable than other common choices, such as k-means clustering, as it is insensitive to the initial seed selection, ensuring replicable results. A centroid-linkage was considered and in order to estimate the similarity between two vectors \(\textbf{x}\) and \(\mathbf {x'}\) the weighted Euclidean distance was used:
where w=( \(w_1\) ,..., \(w_d\) ) represent the vector of the weights assigned to the d dimensions. To identify the best number of clusters the Silhouette index was chosen, as it has been shown to be the best methods in most situations. Arbelaitz et al. ( 2013 )

3.3 How ALPERC works
The algorithm begins by considering an initial dataset \({\Phi }_0=[\textbf{A}_0 \ \textbf{Y}_0]\) , where \(\textbf{A}_0\) is often chosen in the class of Maximum Projection design with discrete numeric factors and \(\textbf{Y}_0\) , is the matrix of responses, assumed to be independent. Then an additional design \(\textbf{A}_{cand}\) that includes \(n_{cand}\) candidate configurations is built. The number of \(n_{cand}\) have to guarantee an appropriate coverage of the design space, however it should be remembered that the computational effort increases with the size of \(\textbf{A}_{cand}\) , thus a valid option may consist of the temporary augmentation of \(\textbf{A}_0\) at each AL iteration by the selection from \(\textbf{A}_{cand}\) of a limited number of combinations which minimize the Maximum Projection criterion, and to use this subset as a candidate set at that specific AL iteration. A commonly used value for the number of candidate points at each iteration is 100.
The next steps of the procedure are the construction of c predictive models \(f_1(\cdot ),\dots , f_c(\cdot )\) that are trained on \({\Phi }_0\) and the quantification of the importance of each feature \(x_i, \; i=1,\ldots ,d\) . As already mentioned, in Arboretti et al. ( 2022a ), a GP model was considered and the total Sobol’ indices were used in order to obtain a quantification of the uncertainty for the candidates in \(\textbf{A}_{cand}\) .
Then, the ranking procedure introduced by Arboretti et al. ( 2014 ), is employed, where for ALPERC the groups G considered in the paper are the rows of \(\textbf{A}_{cand}\) and the dependent variables are the predictive uncertainty observed on the c responses. The permutation tests use the difference in means as test statistics and consider c observations in each group G . Therefore, a value \(c \ge 3\) is recommended to guarantee a minimal sample size for the permutation tests. As a result, the procedure provides a synthetic rank of candidates with respect to the uncertainty associated to all the responses. In comparison to the application of ALM method, the main advantage of ALPERC is that the ranks assign different positions only to those candidates whose global predictive uncertainty is significantly different after the execution of permutation tests. It may happen that some area of the design space is characterized by the highest uncertainty, so the candidates from that region will rank high. If the predictive uncertainty is the only indicator driving AL acquisition, the proposed points would all be close together and located in that specific region of the design space, but in general, one prefers to explore several areas of the experimental domain, in order to increase the global accuracy. For this reason, a clustering method is employed to group together candidates that are close together in the design space. The weighted Euclidean distance is used as a similarity measure for the generation of clusters, where the weights are given by the rescaled relative importances of each variable. The adoption of this strategy increases the possibility to put two candidates that differ with respect to "irrelevant" dimensions in the same cluster, and in an opposite way two configurations that are spatially near but differ with respect to a few decisive factors tend to be assigned to different clusters. At this point, a batch of experimental configurations must be selected from the candidate set for inclusion in the next AL iteration. First, the size of the batch \(n_{add}\) has to be set, and this is usually application-specific. A general guideline is to set \(n_{cand}\) at least one order of magnitude larger than \(n_{add}\) , in order to provide a reasonably large set of candidates at each AL iteration. Two different rules to guide sequential candidate selection can now be chosen, one favoring the exploration of the design space, and the other, more conservative one, that favors exploitation of the current knowledge.
Let’s consider a situation in which two candidate experimental configurations both share the highest rank position and are in the same cluster. In the case of exploration of the design space, only one of these candidate configurations is selected (the one with highest mean uncertainty) and then the rank is descended until a new candidate is found in another cluster and/or has a different position in the rank. In the case of exploitation , the idea is to perform some replicates of the experimental configuration characterized by the highest mean uncertainty, while sharing the same ranking position and cluster with others. The number of required replicates should be equal to the number of candidates which share the same position in the ranking and cluster. In practice, the exploration strategy is preferable in most situations, but in presence of severe heteroscedasticity the predictive models greatly benefit from the execution of replicates, as a separation of noise from signal can be achieved more easily. In the end, \(n_{add}\) configurations are selected from \(\textbf{A}_{cand}\) in accordance to one of the principles already explained, and the new runs can be executed. Once the new responses are collected, the new dataset \({\Phi }_{add,0}\) is concatenated to \({\Phi }_{0}\) and the procedure can be iterated \(n_{iter}\) times, i.e. until a certain accuracy threshold is reached or until the company has exhausted the resources allocated for the project.
4 Simulation study: ALPERC vs competitors
In this section the aim is to review a simulation study about different AL algorithms, including ALPERC and a non-active-learning (non-AL) approach, in order to understand the validity of the AL approaches and more specifically of ALPERC. In order to achieve this goal, we have analysed the simulation study conducted in Arboretti et al. ( 2022a ) that compares the predictive accuracy of ALPERC against some competitors from the literature. This simulation is based on several test functions, that are those already presented in Table 2 , together with multiple noise settings, considering both homoscedastic and heteroscedastic situations, and two different sparsity levels: 0% sparsity and 25% sparsity, where sparsity is the ratio of the number of inactive factors with the number of factors which are considered in the experiment. As regards ALPERC, the exploration strategy was preferred in all noise situations, except the one with the highest heteroscedasticity. The others sequential data acquisition techniques which have been analysed are:
a variation of ALPERC (ALPERC_unw), that considered all the weights equal 1, w=1 , so the attribution of each candidate configuration to a cluster wasn’t adjusted by variable importance;
a selection based on optimisation of the Maximum Projection criteria (MaxPro_aug);
an augmentation based on D-optimality (D_opt);
an iterative data acquisition based on the principle of maximum variance (ALM);
a sequential sampling based on the expected variance reduction throughout the design space (ALC);
a non-active-learning approach in which the models are retrained at each iteration on a new design of suitable size (non-AL). A new Maximum Projection design with a limited number of levels was built at each iteration and its size that matched the other AL counterparts. This is the reference approach that shows the performance of non-sequential methods, which are, as previously seen, the most employed in the literature on DOE+ML.
From the simulation it emerges that for the homoscedastic noise setting ALPERC performs as the best method in three out of four situations, and as the second best method in the remaining one, as it is possible to observe in Table 8 . In the remaining case, the one with the highest levels of uncertainty and sparsity, the best method results the ALPERC_unw. It is also important to underline that the performances of the MaxPro_aug are equal to the ones of ALPERC, when the level of sparsity is low, while, if the level of sparsity increases the perfomances of this method decreases.
As regards the heteroscedastic noise settings ALPERC always ranks first, regardless of the sparsity and noise levels, as it is possible to observe from Table 9 .The unweighted version of ALPERC always ranks second, except in the case of high sparsity level and high heteroscedastic noise, when it matches the results of ALPERC. ALPERC was the only strategy including replicates at the most severe level of heteroscedasticity, because of the exploitation approach. In Arboretti et al. ( 2022a ) it is underlined that even if at each AL step, the experimental configuration selected by the closest competitors had been replicated three times, to match the level of replication of ALPERC, this strategy would perform worse than ALPERC. This demonstrates that at the highest level of uncertainty, the benefits provided by the ALPERC methodology don’t exclusively depend on the presence of replicates, but are a result of the essential principles of the methodology. ALPERC is a sequential algorithm that allows to reassess the situation at each iteration, so if a heteroscedastic noise not initially expected or detected appears, the most obvious choice would be to favour the exploitation strategy. Lastly, it is possible to state that the results of the non-active-learning approach (non-AL) can be considered as unsatisfactory, because this methodology ranks last in all noise settings.
5 Case study: amorphous metallic alloys
5.1 overview.
This section presents a case study about the costruction and refinement of a multi-response emulator to estimate three critical temperatures in some innovative metallic alloys. To achieve the desired goal, data from real experiments are used, along with ALPERC, which results useful in the sequential data collection for iteratively refining the predictive algorithms.
As already mentioned, the case study is about innovative metallic alloys: the amorphous metals. These materials mantain, even at solid state, the typical disordered structure of the liquid state, so they don’t have a cristalline structure, and for this reason they are also known as metallic glasses. The particular structure of these materials results in some very interesting properties, like high strength and wear resistance Jafary-Zadeh et al. ( 2018 ), high hardness and elasticity Chan and Sort ( 2015 ), high magnetic permeability Khan et al. ( 2018 ) and high corrosion resistance Nair and Priyadarshini ( 2016 ). Moreover, the unique characteristics of these metallic glasses make them interesting for different applications in various industries such as sporting good, advanced aerospace applications and medical and electronic devices Chan and Sort ( 2015 ).
A limit to the pratical application of these materials is caused by the fact that it is difficult to obtain amorphous alloys with a thickness greater than 1 mm. Another limit is represented by the high number of elements that are necessary to achieve an appropriate alloy structure; this also makes the size of the combinatorial space prohibitive.
The process of solidification results critical in obtaining the desired structural features of the material and the cooling process is governed by some critical transformation temperatures (CTTs):
the glass transition temperature \(T_g\) ;
the onset of crystalizzation temperature \(T_x\) ;
the liquidus temperature \(T_l\) .
A rapid and precise prediction of the CTTs of candidate material is required to improve the properties of amorphous metallic alloys. That’s why this case study regards the construction and the refinement of predictive models to emulate the three CTTs given both the alloy elements and the composition.
5.2 Dataset and ALPERC implementation
In the case study the data collected by Xiong et al. ( 2020 ) are employed. After the cleaning phase, the dataset consists of 555 measurements from differential thermal analysis or differential scanning calorimetry at a constant heating rate. The alloys investigated in the dataset include 44 elements, as highlighted in Fig. 2 . All the observations were rescaled to \([0-1]\) , using
where \(z_n\) is the rescaled value corresponding to \(y_n\) (the observed value for the n -th observation), \(y_{min}\) represents the minimum value in the dataset, while \(y_{max}\) represents the maximum value in the dataset.
This rescaling was performed to allow a fair comparison between variables that may have different orders of magnitude. Both the responses ( \(T_g\) , \(T_x\) , and \(T_l\) ) and the 44 explanatory variables have been rescaled.
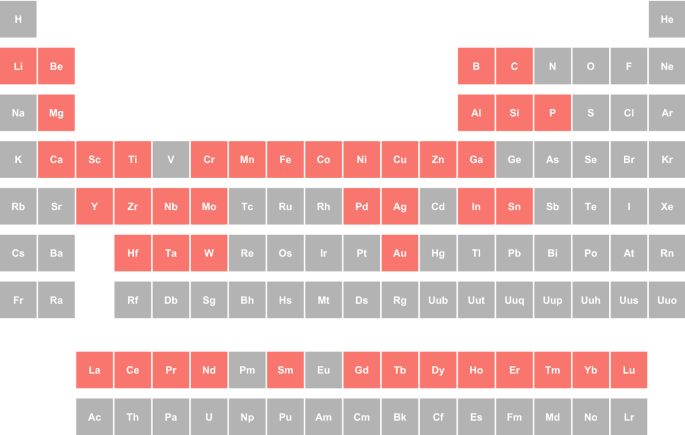
The elements forming the alloys in the metallic glasses dataset
It is important to underline that the data used in this case study are unstructured.
A random partitioning of the experimental data into training and test sets (80% and 20% of the data respectively) is operated, and repeated 10 times for robustness. ALPERC is applied to each initial dataset, with the following starting conditions: \(n_0=40\) , \(n_{cand}=100\) , \(n_{add}=10\) , \(n_{iter}=20\) and the exploration strategy. \({\textbf {A}}_{0}\) is composed by observations randomly selected from the training data. A random design \({\textbf {A}}_{0}\) has been chosen to focus on the behaviour of ALPERC in the active learning phase when the starting design is non-optimal, i.e. potentially worse than it could be. At each AL iteration, \({\textbf {A}}_{cand}\) is constructed including the \(n_{cand}\) data configurations that optimize the Maximum Projection criterion given the experimental trials already included in the design. Considering the large number of predictors, a RF model was chosen and it has been trained using 5-fold Cross Validation Gareth et al. ( 2013 ). The uncertainty quantification associated with this ML model used in the case study follows the methodology of Wager et al. ( 2014 ). Lastly the estimation of the variable importance is performed with the permutation method Breiman ( 2001 ).
5.3 Results and discussion
The evolution of the mean test error obtained with ALPERC is represented in Fig. 3 . From the comparison with the baseline approach, it appears that ALPERC is preferable to the random sampling of candidate configurations. The median test error obtained when using 85% more data than ALPERC at iteration 20 is represented in Fig. 3 by the black dotted line: this is another proof of the good predictive accuracy obtained by the models using ALPERC.
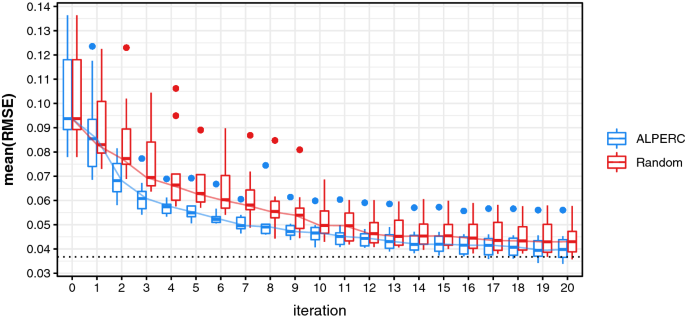
Results of the average MSE at each AL iteraction. The black dotted line corresponds to the median mean(RMSE) when all training data are used for training the models
As it is possible to observe from the three scatterplots in Fig. 4 , which represent the observed vs predicted values, the accuracy on the test data is very high for all the three responses.
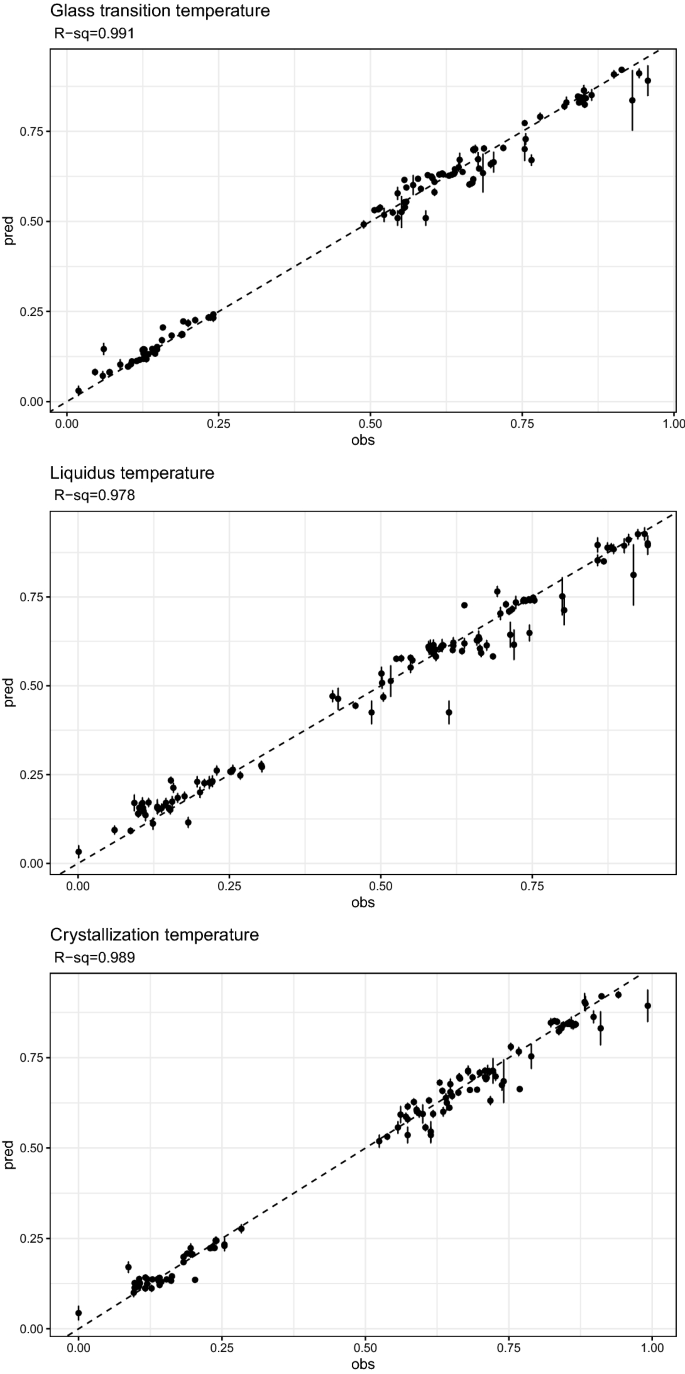
Scatterplot of observed vs predicted values on the test data, considering the training-test partion that leads to the best results at iteration=20 for ALPERC
In Fig. 5 the evolution of the variable importance of the \(T_g\) through ALPERC iterations is represented. To improve the visual impact, those variables for which the median variable importance always results smaller than 10% in each of the AL iterations and for all the CTTs are displayed in light grey. From this plot emerges that 29 out of the 44 predictors are barely important for all the responses or, equivalently, that only 15 predictors should be taken into account. This is a very useful information, because it allows to understand which elements need the most investigation.
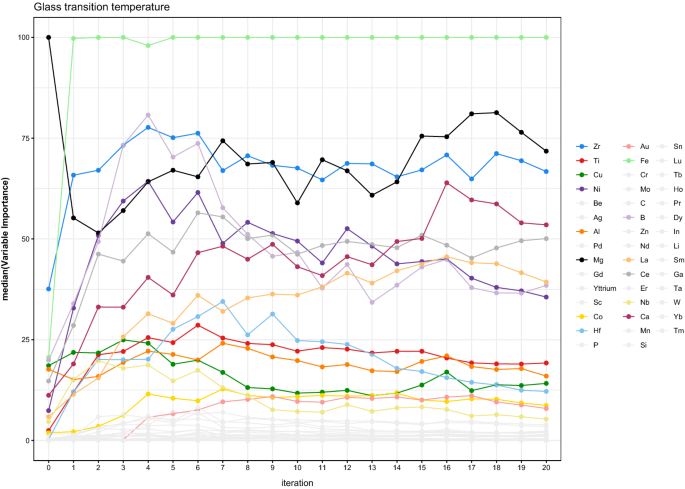
Median variable importance over the ALPERC iterations for \(T_g\)
To better comprehend the RF model employed, the SHAP (SHapley Additive exPlanations) techniqueLundberg et al. ( 2018 ) can be used. The SHAP values are inspired from the Shapley indices of game theory literature Shapley ( 2016 ), and provide an explanation of individual predictions.
By calculating the contribution of each feature to the prediction, SHAP aims to explain the prediction of a single instance \(\textbf{x}\) : this can be achieved by assuming an additive model form and analysing, by using the Shapley values, how much each variable affects the prediction for the instance \(\textbf{x}\) in relation to the overall mean prediction calculated on a given dataset.
Let’s consider the response \(T_g\) as an example (Fig. 6 ): the plot indicates that the average test data prediction is 0.496 (for each response the values have been rescaled to \([0-1]\) ). Then, the value of the predictor Co \(=0.4\) adds 0.061 to the mean prediction, while the content of Zirconium, Zr \(=0\) , subtracts 0.054 to the mean prediction, and so on. Considering all the input variable values, the final prediction for the selected test instance is of 0.509, corresponding to 626.63K. To aid in visualization, just the contribution of the five most relevant predictors is shown for the given test instance, whereas the rest is collapsed in the "other variables" category. For the other responses this approach leads to a final prediction of \(T_x=682.72\) K and \(T_l=996.65\) K. This visualization offers a precise explanation of how each predictor contributes to the prediction of a certain data configuration, providing also insights on the rationale underlying the ML model.
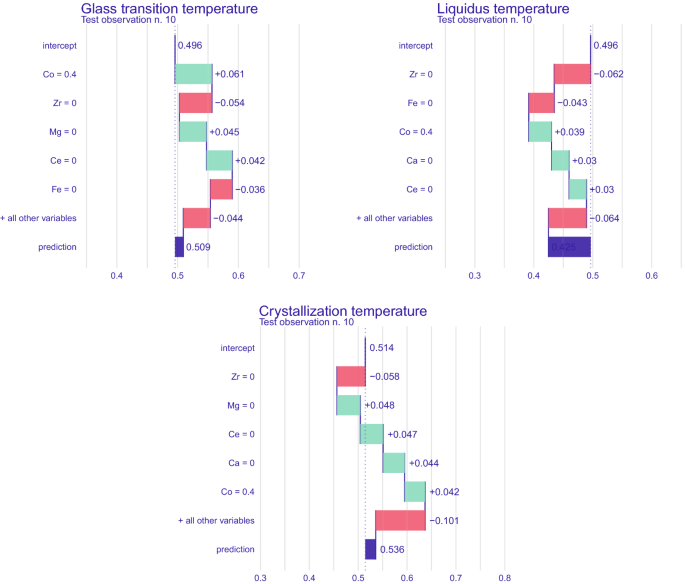
SHAP break-down values for one observation obtained via ALPERC considering the training-test partition that leads to the best results at \(iteration=20\)
By plotting the feature value associated with each test instance on the horizontal axis and the related SHAP value Molnar et al. ( 2020 ) on the vertical axis it is possible to obtain a global view of the SHAP values for each predictor, considering all the test data. An example of these plots is provided for \(T_g\) , the Glass transition temperature, represented in Fig. 7 , considering the 15 most relevant predictors identified in Fig. 5 ; if we consider the case of Copper (Cu) it is possible to observe that, if \(Cu=0.25\) , \(T_g\) descreases by almost 0.025, while, if \(Cu=0.8\) , the increase in \(T_g\) is equal to 0.025. So, using this partial dependence plot it is possible to observe how the various levels of some elements affect the different temperatures.
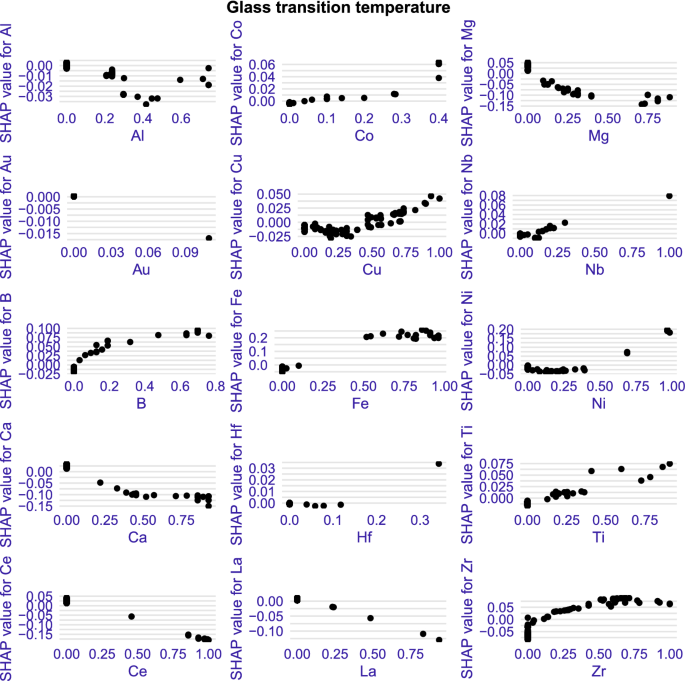
Partial dependence plots on the test data for \(T_g\) considering the 15 most relevant predictors
Moreover, from this plot it is possible to observe how these relationships are non-linear and also rather complex. A limitation of this kind of visualization is represented by the fact that these plots only provide information on the effect of the input variables taken individually. However it is possible to compute SHAP interaction values, which quantify the impact of the interactions after removing the impacts of the individual effects Molnar et al. ( 2020 ).
In Fig. 8 , obtained using the treeshap R Package Komisarczyk et al. ( 2023 ), an example that displays a relevant interaction effect between Zirconium (Zr) and Copper (Cu) for the response \(T_l\) is represented.
In this example, it is important to point out that since the dataset is not a result of a designed experiment some confounding effects may arise while interpreting the interactions.
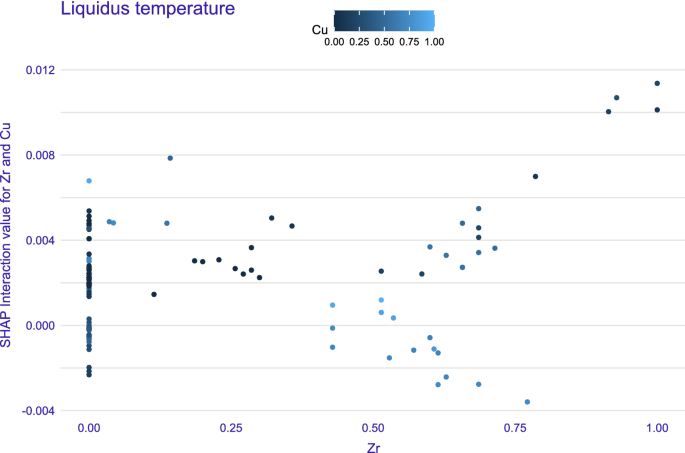
Interaction plot of the variables Zr and Cu for the response \(T_l\)
6 Results, interpretations and conclusions
In the first part of this paper, we provided a review of some contributions in the field of AL: firstly we reviewed a simulation study Arboretti et al. ( 2022b ), in which the aim was to investigate which experimental design and ML model result most suitable for a joint application in physical experiments. From this simulation study, performed on 7 different test functions, it emerged that the best experimental design is MAXPRO_dis. The fact that this design is the best in the majority of situations may be explained by the fact that it is based on a space-filling criterion. This may favor flexible non-linear predictive models in capturing the behavior of the underlying function and, it just needs a limited number of levels, so it is also feasible for physical experiments. As regards the ML models, it emerged that the best choice is the Gaussian process, which resulted as the best choice in the vast majority of the different noise settings analyzed. This simulation study may represent the base for future research where the loss functions of the D-opt and I-Opt criteria are adapted to include heteroscedasticity. In Sect. 3 there is a review of ALPERC, a recently developed AL algorithm suitable for physical experiments when three or more responses are investigated. In Sect. 4 a simulation study compares ALPERC with other AL algorithms and also with a non-AL approach. From this review, it emerged that ALPERC provided a lower prediction error in comparison to the competitors, and also that AL algorithms performed better in almost all the analyzed situations, in contrast to a non-AL approach. Section 5 introduces the main novelty element of this paper, a case study, about amorphous metallic alloys, in which ALPERC is used together with an RF, in order to train and refine predictive models for emulating three different CTTs. In this case study, ALPERC proved to be more efficient than the non-AL strategy (Fig. 3 ) and this is a confirmation of the goodness of the algorithm. Moreover, also thanks to the adoption of the SHAP technique, the obtained model results could be easily interpreted by the analyst. To conclude, we can sum up the findings of this novel case study by saying that not only does ALPERC have a high potential for reducing predictive errors, but it also provides researchers with a more intuitive interpretation of the results.
Data availability
The data used in the case study is taken from the work of Xiong et al. ( 2020 ). A R package including ALPERC functions is available at https://github.com/PegoraroL/ALPERC .
Arbelaitz O, Gurrutxaga I, Muguerza J, Pérez JM, Perona I (2013) An extensive comparative study of cluster validity indices. Pattern Recogn 46(1):243–256. https://doi.org/10.1016/j.patcog.2012.07.021
Article Google Scholar
Arboretti R, Bonnini S, Corain L, Salmaso L (2014) A permutation approach for ranking of multivariate populations. J Multivar Anal 132:39–57. https://doi.org/10.1016/j.jmva.2014.07.009
Article MathSciNet MATH Google Scholar
Arboretti R, Ceccato R, Pegoraro L, Salmaso L (2022) Active learning for noisy physical experiments with more than two responses. Chemom Intell Lab Syst 226:104595. https://doi.org/10.1016/j.chemolab.2022.104595
Arboretti R, Ceccato R, Pegoraro L, Salmaso L (2022) Design choice and machine learning model performances. Qual Reliabil Eng Int 38(7):3357–3378. https://doi.org/10.1002/qre.3123
Arboretti R, Ceccato R, Pegoraro L, Salmaso L (2022) Design of experiments and machine learning for product innovation: a systematic literature review. Qual Reliabil Eng Int 38(2):1131–1156. https://doi.org/10.1002/qre.3025
Arboretti R, Ceccato R, Pegoraro L, Salmaso L, Housmekerides C, Spadoni L, Pierangelo E, Quaggia S, Tveit C, Vianello S (2022) Machine learning and design of experiments with an application to product innovation in the chemical industry. J Appl Stat 49(10):2674–2699. https://doi.org/10.1080/02664763.2021.1907840
Binois M, Huang J, Gramacy RB, Ludkovski M (2019) Replication or exploration? sequential design for stochastic simulation experiments. Technometrics 61(1):7–23. https://doi.org/10.1080/00401706.2018.1469433
Article MathSciNet Google Scholar
Bisgaard S (1992) Industrial use of statistically designed experiments: case study references and some historical anecdotes. Qual Eng 4(4):547–562. https://doi.org/10.1080/08982119208918936
Breiman L (2001) Random forests. Mach Learn 45(1):5–32. https://doi.org/10.1023/A:1010933404324
Article MATH Google Scholar
Chan K, Sort J (2015) Metallic glasses. Metals 5:2397–2400. https://doi.org/10.3390/met5042397
Freiesleben J, Keim J, Grutsch M (2020) Machine learning and design of experiments: alternative approaches or complementary methodologies for quality improvement? Qual Reliabil Eng Int 36(6):1837–1848. https://doi.org/10.1002/qre.2579
Gareth J, Daniela W, Trevor H, Robert T (2013) An introduction to statistical learning: with applications in R. Springer, Berlin
MATH Google Scholar
Gramacy RB, Lee HKH (2009) Adaptive design and analysis of supercomputer experiments. Technometrics 51(2):130–145. https://doi.org/10.1198/TECH.2009.0015
Jafary-Zadeh M, Praveen Kumar G, Branicio PS, Seifi M, Lewandowski JJ, Cui F (2018) A critical review on metallic glasses as structural materials for cardiovascular stent applications. J Funct Biomater 9(1):10019. https://doi.org/10.3390/jfb9010019
Joseph VR, Gul E, Ba S (2020) Designing computer experiments with multiple types of factors: the maxpro approach. J Qual Technol 52(4):343–354. https://doi.org/10.1080/00224065.2019.1611351
Khan MM, Nemati A, Rahman ZU, Shah UH, Asgar H, Haider W (2018) Recent advancements in bulk metallic glasses and their applications: a review. Crit Rev Solid State Mater Sci 43(3):233–268. https://doi.org/10.1080/10408436.2017.1358149
Komisarczyk K, Kozminski P, Maksymiuk S, Biecek P (2023) treeshap: fast SHAP values computation for tree ensemble models. r package version 0.1.1. https://github.com/ModelOriented/treeshap
LeDell E, Poirier S (2020) H2o automl: scalable automatic machine learning. Proc AutoML Workshop ICML 2020:1–16
Google Scholar
Lujan-Moreno GA, Howard PR, Rojas OG, Montgomery DC (2018) Design of experiments and response surface methodology to tune machine learning hyperparameters, with a random forest case-study. Expert Syst Appl 109:195–205. https://doi.org/10.1016/j.eswa.2018.05.024
Lundberg SM, Erion GG, Lee SI (2018) Consistent individualized feature attribution for tree ensembles. arXiv:1802.03888
Molnar C, Casalicchio G, Bischl B (2020) Interpretable machine learning: a brief history, state-of-the-art and challenges. In: Koprinska I, Kamp M, Appice A, Loglisci C, Antonie L, Zimmermann A, Guidotti R, Özgöbek Ö, Ribeiro RP, Gavaldà R, Gama J, Adilova L, Krishnamurthy Y, Ferreira PM, Malerba D, Medeiros I, Ceci M, Manco G, Masciari E, Ras ZW, Christen P, Ntoutsi E, Schubert E, Zimek A, Monreale A, Biecek P, Rinzivillo S, Kille B, Lommatzsch A, Gulla JA (eds) ECML PKDD 2020 Workshops. Springer International Publishing, Cham, pp 417–431
Chapter Google Scholar
Nair B, Priyadarshini BG (2016) Process, structure, property and applications of metallic glasses. AIMS Mater Sci 3(3):1022–1053. https://doi.org/10.3934/matersci.2016.3.1022
Olsson F (2009) A literature survey of active machine learning in the context of natural language processing. Tech. Rep. T2009:06, Swedish Institute of Computer Science. https://www.diva-portal.org/smash/get/diva2:1042586/FULLTEXT01.pdf
Shapley LS (2016) A value for n-person games. Princeton University Press, Princeton, pp 307–318
Sobol I (2001) Global sensitivity indices for nonlinear mathematical models and their Monte Carlo estimates. Math Comput Simul 55(1):271–280. https://doi.org/10.1016/S0378-4754(00)00270-6
Staelin C (2003) Parameter selection for support vector machines
Wager S, Hastie T, Efron B (2014) Confidence intervals for random forests: the jackknife and the infinitesimal jackknife. J Mach Learn Res 15(48):1625–1651
MathSciNet MATH Google Scholar
Wei P, Lu Z, Song J (2015) Variable importance analysis: a comprehensive review. Reliabil Eng Syst Saf 142:399–432. https://doi.org/10.1016/j.ress.2015.05.018
Xiong J, Shi SQ, Zhang TY (2020) A machine-learning approach to predicting and understanding the properties of amorphous metallic alloys. Mater Des 187:108378. https://doi.org/10.1016/j.matdes.2019.108378
Yue X, Wen Y, Hunt JH, Shi J (2021) Active learning for gaussian process considering uncertainties with application to shape control of composite fuselage. IEEE Trans Autom Sci Eng 18(1):36–46. https://doi.org/10.1109/TASE.2020.2990401
Download references
Open access funding provided by Politecnico di Torino within the CRUI-CARE Agreement.
Author information
Authors and affiliations.
Department of Mathematical Sciences, Politecnico di Torino, Turin, Italy
Roberto Fontana
Department of Management and Engineering, University of Padova, Padua, Italy
Alberto Molena, Luca Pegoraro & Luigi Salmaso
You can also search for this author in PubMed Google Scholar
Corresponding author
Correspondence to Roberto Fontana .
Additional information
Publisher's note.
Springer Nature remains neutral with regard to jurisdictional claims in published maps and institutional affiliations.
Rights and permissions
Open Access This article is licensed under a Creative Commons Attribution 4.0 International License, which permits use, sharing, adaptation, distribution and reproduction in any medium or format, as long as you give appropriate credit to the original author(s) and the source, provide a link to the Creative Commons licence, and indicate if changes were made. The images or other third party material in this article are included in the article’s Creative Commons licence, unless indicated otherwise in a credit line to the material. If material is not included in the article’s Creative Commons licence and your intended use is not permitted by statutory regulation or exceeds the permitted use, you will need to obtain permission directly from the copyright holder. To view a copy of this licence, visit http://creativecommons.org/licenses/by/4.0/ .
Reprints and permissions
About this article
Fontana, R., Molena, A., Pegoraro, L. et al. Design of experiments and machine learning with application to industrial experiments. Stat Papers 64 , 1251–1274 (2023). https://doi.org/10.1007/s00362-023-01437-w
Download citation
Received : 29 December 2022
Revised : 28 February 2023
Published : 26 March 2023
Issue Date : August 2023
DOI : https://doi.org/10.1007/s00362-023-01437-w
Share this article
Anyone you share the following link with will be able to read this content:
Sorry, a shareable link is not currently available for this article.
Provided by the Springer Nature SharedIt content-sharing initiative
- Design of Experiments
- Machine learning
- Active learning
- Industrial statistics
- Find a journal
- Publish with us
- Track your research
machine design research papers
Machine learning research papers, protein interaction in machine learning, bearing fault finding and solution, ieee projects 2022, seminar reports, free ieee projects ieee papers.
Subscribe to the PwC Newsletter
Join the community, trending research, visual autoregressive modeling: scalable image generation via next-scale prediction.

We present Visual AutoRegressive modeling (VAR), a new generation paradigm that redefines the autoregressive learning on images as coarse-to-fine "next-scale prediction" or "next-resolution prediction", diverging from the standard raster-scan "next-token prediction".

InstantStyle: Free Lunch towards Style-Preserving in Text-to-Image Generation
Tuning-free diffusion-based models have demonstrated significant potential in the realm of image personalization and customization.

ReFT: Representation Finetuning for Language Models
LoReFT is a drop-in replacement for existing PEFTs and learns interventions that are 10x-50x more parameter-efficient than prior state-of-the-art PEFTs.
AIOS: LLM Agent Operating System
agiresearch/aios • 25 Mar 2024
Inspired by these challenges, this paper presents AIOS, an LLM agent operating system, which embeds large language model into operating systems (OS) as the brain of the OS, enabling an operating system "with soul" -- an important step towards AGI.
VoiceCraft: Zero-Shot Speech Editing and Text-to-Speech in the Wild
We introduce VoiceCraft, a token infilling neural codec language model, that achieves state-of-the-art performance on both speech editing and zero-shot text-to-speech (TTS) on audiobooks, internet videos, and podcasts.
AutoWebGLM: Bootstrap And Reinforce A Large Language Model-based Web Navigating Agent
thudm/autowebglm • 4 Apr 2024
Large language models (LLMs) have fueled many intelligent agent tasks, such as web navigation -- but most existing agents perform far from satisfying in real-world webpages due to three factors: (1) the versatility of actions on webpages, (2) HTML text exceeding model processing capacity, and (3) the complexity of decision-making due to the open-domain nature of web.
CameraCtrl: Enabling Camera Control for Text-to-Video Generation
Controllability plays a crucial role in video generation since it allows users to create desired content.
AniPortrait: Audio-Driven Synthesis of Photorealistic Portrait Animation
In this study, we propose AniPortrait, a novel framework for generating high-quality animation driven by audio and a reference portrait image.

Cross-Attention Makes Inference Cumbersome in Text-to-Image Diffusion Models
This study explores the role of cross-attention during inference in text-conditional diffusion models.
Champ: Controllable and Consistent Human Image Animation with 3D Parametric Guidance
In this study, we introduce a methodology for human image animation by leveraging a 3D human parametric model within a latent diffusion framework to enhance shape alignment and motion guidance in curernt human generative techniques.
‘ICML 2023 Topological Deep Learning Challenge: Design and Results’

“This paper presents the computational challenge on topological deep learning that was hosted within the ICML 2023 Workshop on Topology and Geometry in Machine Learning. The competition asked participants to provide open-source implementations of topological neural networks from the literature by contributing to the python packages TopoNetX (data processing) and TopoModelX (deep learning). The challenge attracted twenty-eight qualifying submissions in its two month duration. This paper describes the design of the challenge and summarizes its main findings.”
Find the paper and full list of authors at Proceedings of Machine Learning Research.
‘Hierarchical RL-Guided Large-Scale Navigation of a Snake Robot’
‘bergeron: combating adversarial attacks through a conscience-based alignment framework’, ‘more samples or more prompt inputs exploring effective in-context sampling for llm few-shot prompt engineering’, ‘multi-instance randomness extraction and security against bounded-storage mass surveillance’, ‘is a seat at the table enough engaging teachers and students in dataset specification for ml in education’, ‘”the wallpaper is ugly”: indoor localization using vision and language’, ‘human still wins over llm: an empirical study of active learning on domain-specific annotation tasks’, ‘beyond labels: empowering human annotators with natural language explanations through a novel active-learning architecture’, ‘automatic collation for diversifying corpora: commonly copied texts as distant supervision for handwritten text recognition’.

IMAGES
VIDEO
COMMENTS
Arne Bilberg. Saule Rakhimova. Dec 2023. Adhan Efendi. Jiawang Chen. Explore the latest full-text research PDFs, articles, conference papers, preprints and more on MACHINE DESIGN. Find methods ...
This is to help the novel ways of manufacturing process to move forward, where, the Machine Design will feature and compile the newest product line with an inventive technology to keep modernized techniques at the top of mind for our OEMs, end-users, integrators, and the entire supply community. This research paper will explore how the ...
A Feature Paper should be a substantial original Article that involves several techniques or approaches, provides an outlook for future research directions and describes possible research applications. Feature papers are submitted upon individual invitation or recommendation by the scientific editors and must receive positive feedback from the ...
The Journal of Mechanical Design publishes technical papers concerned with design automation, including design representation, virtual reality, geometric design, design evaluation, design optimization, risk and reliability-based optimization, design sensitivity analysis, system design integration, ergonomic and aesthetic considerations, and design for market systems; design of direct contact ...
Further research directions on the use of machine learning and neural networks in the fields of mechanical design and optimization are discussed. ... Feature papers represent the most advanced research with significant potential for high impact in the field. ... CAD files, and other design-related data used in computer-aided design applications ...
Design Methods for Mechanical and Industrial Innovation. Special Issue Editors. Special Issue Information. Published Papers. A special issue of Machines (ISSN 2075-1702). This special issue belongs to the section "Machines Testing and Maintenance". Deadline for manuscript submissions: 30 June 2024 | Viewed by 12821.
Trends in Machine Design (TMD) eISSN: 2455-3352. Journal DOI: 10.37591/TMD. Scientific Journal Impact Factor (SJIF Value): 6.003. Click here for complete Editorial Board. Trends in Machine Design (TMD) is a print and e-journal focused towards the rapid publication of fundamental research papers on all areas concerning manufacturing and machine ...
community. This research paper will explore how the simulation derived model of Mechatronic could manage the most complex scheme of the machinery profile with a systematic approach by understanding the concept with precise machine design actions, dynamic behavior, and effective interaction with the various components of the machine.
The journal Machine Design is published by the Faculty of Technical Sciences in Novi Sad four issues per year. Publishing this journal we would like to make mechanical engineering more interesting and to promote it as an important branch of engineering in the light of modern techniques and new technologies. The journal is a good opportunity to ...
Fundamentals of Machine Design Volume I Machine design is a part of Engineering Design. Fundamentals of Machine Design is compiled in two volumes. Vol. I provides extensive coverage and comprehensive discussion on the fundamental concepts and processes of machine design. Unit 1 of this volume starts by giving a background to the subject and
DESIGN AND IMPLEMENTATION OF UNIVERSAL MOTOR CONTROL USING IR REMOTE AND ARDUINO. The main objective types of machine drives. In industry research paper shows the methodology to interface stepper, servo and DC motor on a single platform. IR (remote control) is implemented to control all motors.
State of the art review papers. Review papers related to machine learning applications in the manufacturing domain in this special issue bring together quantitative and qualitative components and provide new conceptual frameworks, synthesise diverse results, and give the broader research community a 'state-of-the-art' snapshot of essential ...
The second category is conceptual papers, in which there is a degree of discussion of the DOE + ML approach adopted and not simply a straight application of the method. Less relevant are the reviews and simulation studies. For papers adopting a mixed research methodology, the same paper was counted in each pertinent category (Figure 8).
To discuss the applicability of machine learning-based solutions in various real-world application domains. To highlight and summarize the potential research directions within the scope of our study for intelligent data analysis and services. The rest of the paper is organized as follows.
In the context of product innovation, there is an emerging trend to use Machine Learning (ML) models with the support of Design Of Experiments (DOE). The paper aims firstly to review the most suitable designs and ML models to use jointly in an Active Learning (AL) approach; it then reviews ALPERC, a novel AL approach, and proves the validity of this method through a case study on amorphous ...
Analyze the state-of-the-art in engineering machine learning systems. for the purpose of exploration and analysis. ... " An example of a design-related obstacle is ... Fig. 7 displays the research methods used in the primary studies split across the SE knowledge areas addressed in the papers. The dominant research method, i.e., experiment, is ...
Hydraulic switching actuators are high-efficiency, fast response, and low-cost solutions for hydraulic control systems. One of the challenging problems is throttling losses during valve transitions. Previously, the authors proposed a zero-flowrate switching method to reduce the throttling energy loss of the switching valve, where a hydraulic resonator is applied to make the flowrates through ...
This review paper focused on the dispensing machines in the medical field particularly the design development of the automated dispensing machines which can be considered as one of the important technologies in pharmacy. This paper also briefly describes the main criteria for fabricating the dispensing machine.
FABSTRACT This paper presents how to analytically design a high-torque three-phase flux- switching permanent magnet machine with 12 stator poles and 14 rotor poles. Firstly, the machine design parameters are studied addressing on high output torque and its flux. Precision machine design. free download.
justimyhxu/grm • • 21 Mar 2024. We introduce GRM, a large-scale reconstructor capable of recovering a 3D asset from sparse-view images in around 0. 1s. 3D Reconstruction Image to 3D +1. 362. 0.77 stars / hour. Paper. Code. Papers With Code highlights trending Machine Learning research and the code to implement it.
Memristor-based design has gained significant attention in recent years due to its potential to revolutionize various fields such as artificial intelligence, neuromorphic computing, non-volatile memory, signal processing, filtering, and radio frequency design. These emerging devices offer unique advantages such as non-volatile memory, low power consumption, and a high integration density ...
Noah Lloyd. April 5, 2024. "This paper presents the computational challenge on topological deep learning that was hosted within the ICML 2023 Workshop on Topology and Geometry in Machine Learning. The competition asked participants to provide open-source implementations of topological neural networks from the literature by contributing to the ...
Coordination between the construction of transport infrastructure and the development and protection of territorial space is an important factor in promoting sustainable regional development, but there is still a lack of systematic research on the impact of transport on territorial space worldwide. Following the logic of "development trend revealing—theoretical and technological summary ...