Thank you for visiting nature.com. You are using a browser version with limited support for CSS. To obtain the best experience, we recommend you use a more up to date browser (or turn off compatibility mode in Internet Explorer). In the meantime, to ensure continued support, we are displaying the site without styles and JavaScript.
- View all journals
- Explore content
- About the journal
- Publish with us
- Sign up for alerts
- Review Article
- Open access
- Published: 13 September 2023

Ethics and discrimination in artificial intelligence-enabled recruitment practices
- Zhisheng Chen ORCID: orcid.org/0000-0002-0854-2547 1
Humanities and Social Sciences Communications volume 10 , Article number: 567 ( 2023 ) Cite this article
65k Accesses
29 Citations
241 Altmetric
Metrics details
- Business and management
- Science, technology and society
This study aims to address the research gap on algorithmic discrimination caused by AI-enabled recruitment and explore technical and managerial solutions. The primary research approach used is a literature review. The findings suggest that AI-enabled recruitment has the potential to enhance recruitment quality, increase efficiency, and reduce transactional work. However, algorithmic bias results in discriminatory hiring practices based on gender, race, color, and personality traits. The study indicates that algorithmic bias stems from limited raw data sets and biased algorithm designers. To mitigate this issue, it is recommended to implement technical measures, such as unbiased dataset frameworks and improved algorithmic transparency, as well as management measures like internal corporate ethical governance and external oversight. Employing Grounded Theory, the study conducted survey analysis to collect firsthand data on respondents’ experiences and perceptions of AI-driven recruitment applications and discrimination.
Similar content being viewed by others
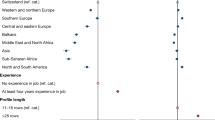
Monitoring hiring discrimination through online recruitment platforms
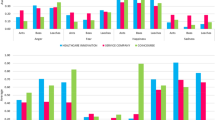
Measuring ethical behavior with AI and natural language processing to assess business success
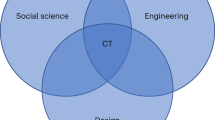
Introducing contextual transparency for automated decision systems
Introduction.
Technological innovation has revolutionized work across the first through fourth industrial revolutions. The fourth industrial revolution introduced disruptive technologies like big data and artificial intelligence (Zhang and Chen, 2023 ). The advancement of data processing and big data analytics, along with developments in artificial intelligence, has improved information processing capabilities, including problem-solving and decision-making (Raveendra et al., 2020 ). With the increasing normalization and timely usage of digital technologies, there is a potential for future higher-level implementation of AI systems (Beneduce, 2020 ).
AI can provide faster and more extensive data analysis than humans, achieving remarkable accuracy and establishing itself as a reliable tool (Chen, 2022 ). It can collect and evaluate large amounts of data that may exceed human analytical capacities, enabling AI to provide decision recommendations (Shaw, 2019 ).
Modern technologies, including artificial intelligence solutions, have revolutionized work and contributed to developing human resources management (HRM) for improved outcomes (Hmoud and Laszlo, 2019 ). One significant area where their impact is felt is in the recruitment process, where AI implementation can potentially provide a competitive advantage by enabling a better understanding of talent compared to competitors, thereby enhancing the company’s competitiveness (Johansson and Herranen, 2019 ).
AI receives commands and data input through algorithms. While AI developers believe their algorithmic procedures simplify hiring and mitigate bias, Miasato and Silva ( 2019 ) argue that algorithms cannot eliminate discrimination alone. The decisions made by AI are shaped by the initial data it receives. If the underlying data is unfair, the resulting algorithms can perpetuate bias, incompleteness, or discrimination, creating potential for widespread inequality (Bornstein, 2018 ). Many professionals assert that AI and algorithms reinforce socioeconomic divisions and expose disparities. To quote Immanuel Kant, “In the bentwood of these data sets, none of them is straight” (Raub, 2018 ). This undermines the principle of social justice, causing moral and economic harm to those affected by discrimination and reducing overall economic efficiency, leading to decreased production of goods and services.
AI recruitment tools have a concerning aspect that cannot be overlooked, highlighting the need to address these challenges through technical or managerial means (Raub, 2018 ). Increasing evidence suggests that AI is more impartial than commonly believed; however, algorithms and AI can result in unfair employment opportunities and the potential for discrimination without accountability. To harness the benefits of AI in recruiting, organizations should exercise careful selection of their programs, promote the adoption of accountable algorithms, and advocate for improvements in racial and gender diversity within high-tech companies.
The general construct of this study is, first, an extension of statistical discrimination theory in the context of the algorithmic economy; second, a synthesis of the current literature on the benefits of algorithmic hiring, the roots and classification of algorithmic discrimination; and third, initiatives to eliminate the existence of algorithmic hiring discrimination; fourth, based on the Grounded Theory, we conduct surveys with respondents and analyze primary data to support the study.
The contributions of this study are as follows:
First, discuss job market discrimination theories in the digital age context. When considering statistical discrimination theories, we should consider the current circumstances. It is necessary to apply these discrimination theories to evaluate the issues that arise from the use of technology in the digital age, particularly with the widespread adoption of artificial intelligence, big data, and blockchain across various industries.
Secondly, a literature review approach was employed to examine the factors contributing to discrimination in algorithmic hiring. Our goal with this analysis is to help managers and researchers better understand the limitations of AI algorithms in the hiring process. We conducted a thorough review of 49 papers published between 2007 and 2023 and found that there is currently a fragmented understanding of discrimination in algorithmic hiring. Building on this literature review, our study aims to offer a comprehensive and systematic examination of the sources, categorization, and possible solutions for discriminatory practices in algorithmic recruitment.
Thirdly, we take a comprehensive approach that considers technical and managerial aspects to tackle discrimination in algorithmic hiring. This study contends that resolving algorithmic discrimination in recruitment requires technical solutions and the implementation of internal ethical governance and external regulations.
The subsequent study is structured into five parts. The first section provides the theoretical background for this research. The following section outlines the research methodology employed in the literature review and identifies four key themes. The third section delves into a detailed discussion of these four themes: applications and benefits of AI-based recruitment, factors contributing to algorithmic recruitment discrimination, types of discrimination in algorithmic recruitment, and measures to mitigate algorithmic hiring discrimination. The fourth section involves conducting a survey among respondents and analyzing the primary data collected to support our study. The final section concludes by suggesting future directions for research.
Theory background
Discrimination theory.
Discrimination in the labor market is defined by the ILO’s Convention 111, which encompasses any unfavorable treatment based on race, ethnicity, color, and gender that undermines employment equality (Ruwanpura, 2008 ). Economist Samuelson ( 1952 ) offers a similar definition, indicating that discrimination involves differential treatment based on personal characteristics, such as ethnic origin, gender, skin color, and age.
Various perspectives on the causes and manifestations of discrimination can be broadly categorized into four theoretical groups. The first is the competitive market theory, which explains discriminatory practices within an equilibrium of perfect competition (Lundberg and Startz, 1983 ). This view attributes discrimination primarily to personal prejudice. The second is the monopoly model of discrimination, which posits that monopolistic power leads to discriminatory behavior (Cain, 1986 ). The third is the statistical theory of discrimination, which suggests that nonobjective variables, such as inadequate information, contribute to biased outcomes (Dickinson and Oaxaca, 2009 ). Lastly, we have the antecedent market discrimination hypothesis as the fourth category.
Statistical discrimination theory
Statistical discrimination refers to prejudice from assessment criteria that generalize group characteristics to individuals (Tilcsik, 2021 ). It arises due to limitations in employers’ research techniques or the cost constraint of obtaining information in the asymmetry between employers and job seekers. Even without monopolistic power, statistical discrimination can occur in the labor market due to information-gathering methods. Employers are primarily interested in assessing candidates’ competitiveness when making recruitment decisions. However, obtaining this information directly is challenging, so employers rely on various indirect techniques.
Discrimination carries both individual and societal economic costs. The social cost arises from the decrease in overall economic output caused by discrimination. However, this is still deemed efficient under imperfect information and aligns with the employer’s profit maximization goal. Therefore, it is likely that statistical discrimination in employment will persist.
Extension of statistical discrimination theory in the digital age
The digital economy has witnessed the application of various artificial intelligence technologies in the job market. Consequently, the issue of algorithmic hiring discrimination has emerged, shifting the focus of statistical discrimination theory from traditional hiring to intelligent hiring. The mechanisms that give rise to hiring discrimination problems remain similar, as both rely on historical data of specific populations to predict future hiring outcomes.
While AI recruiting offers numerous benefits, it is also susceptible to algorithmic bias. Algorithmic bias refers to the systematic and replicable errors in computer systems that lead to unequally and discrimination based on legally protected characteristics, such as race and gender (Jackson, 2021 ). When assessments consistently overestimate or underestimate a particular group’s scores, they produce “predictive bias” (Raghavan et al., 2020 ). Unfortunately, these discriminatory results are often overlooked or disregarded due to the misconception that AI processes are inherently “objective” and “neutral.”
Despite algorithms aiming for objectivity and clarity in their procedures, they can become biased when they receive partial input data from humans. Modern algorithms may appear neutral but can disproportionately harm protected class members, posing the risk of “agentic discrimination” (Prince and Schwarcz, 2019 ). If mishandled, algorithms can exacerbate inequalities and perpetuate discrimination against minority groups (Lloyd, 2018 ).
Within the recruitment process, algorithmic bias can manifest concerning gender, race, color, and personality.
Research methodology
The primary research strategy was a literature review approach. This review aimed to assess current research on recruitment supported by artificial intelligence algorithms. The systematic review process included gathering and evaluating the selected studies’ literature and topics. Driven by the direction of the research, studies focusing on algorithmic discrimination in recruitment over the past 10 years were included unless past literature was worth reviewing. This is because this is a relatively new phenomenon that has become prominent over the past 10 years. In defining the “algorithmic and hiring discrimination” literature, a fairly broad approach was taken based on article keywords rather than publication sources. Depending on the focus, keywords related to algorithms and hiring discrimination were included in the search string. The keyword search algorithm for this review is as follows. (“artificial intelligence” and “hiring discrimination”), (“algorithms” and “recruitment discrimination”), (artificial intelligence” and “recruitment discrimination”), and (“algorithms” and “hiring discrimination”). SCOPUS, Google Scholar, and Web of Science are three well-known search engines frequently used by the academic community and meet the criteria for technology-related topics in this review. WOS is used as a starting point for high-quality peer-reviewed scholarly articles. The study selected these three databases, used search engines, and maintained ten years. After applying an initial screening related to titles, keywords, or abstracts, the literature was selected based on its relevance to the research topic.
The obtained literature was studied in depth to reveal the surfaced themes. Several systematic research themes were identified, including AI-based recruitment applications and benefits, causes of algorithmic discrimination, which algorithmic recruitment discrimination exists, and algorithmic recruitment discrimination resolution.
The process applied for the reviews depicted in Fig. 1 . After excluding duplicates and less relevant and outdated literature, only 45 articles could be used as references for this study (referred to Table 1 ). The literature review shows that most of the research on algorithmic hiring discrimination has occurred in recent years. The research trend indicates that algorithmic hiring discrimination will be a hot research topic in the coming period.
The first theme is the application of various aspects of recruitment based on artificial intelligence support and its benefits. Bogen and Rieke ( 2018 ), Ahmed ( 2018 ), Hmoud and Laszlo ( 2019 ), Albert ( 2019 ), van Esch et al. ( 2019 ), Köchling et al. ( 2022 ), and Chen ( 2023 ) consider the recruitment process as a set of tasks that may be divided into four steps sourcing, screening, interviewing, and selection. Each step includes different activities, and AI algorithms can change how each stage is executed. Some studies point out that AI-supported recruitment has benefits. Beattie et al. ( 2012 ), Newell ( 2015 ), Raub ( 2018 ), Miasato and Silva ( 2019 ), Beneduce ( 2020 ), and Johnson et al. ( 2020 ) state that it can reduce costs; Hmoud and Laszlo ( 2019 ), Johansson and Herranen ( 2019 ), Raveendra et al. ( 2020 ), Black and van Esch ( 2020 ), and Allal-Chérif et al. ( 2021 ) suggest it saves time; Upadhyay and Khandelwal ( 2018 ) and Johansson and Herranen ( 2019 ) present it reducing transactional workload.
The second theme is the causes of algorithmic discrimination. McFarland and McFarland ( 2015 ), Mayson ( 2018 ), Raso et al. ( 2018 ), Raub ( 2018 ), Raghavan et al. ( 2020 ), Njoto ( 2020 ), Zixun ( 2020 ), and Jackson ( 2021 ) suggest that the reason for algorithmic discrimination is related to data selection. Data collection tends to prefer accessible, “mainstream” organizations unequally dispersed by race and gender. Inadequate data will screen out groups that have been historically underrepresented in the recruitment process. Predicting future hiring outcomes by observing historical data can amplify future hiring inequalities. Yarger et al. ( 2019 ), Miasato and Silva ( 2019 ), and Njoto ( 2020 ) propose that discrimination is due to the designer-induced selection of data features.
The third theme is which algorithmic recruitment discrimination exists. According to Correll et al. ( 2007 ), Kay et al. ( 2015 ), O’neil ( 2016 ), Raso et al. ( 2018 ), Miasato and Silva ( 2019 ), Langenkamp et al. ( 2019 ), Faragher ( 2019 ), Ong ( 2019 ), Fernández and Fernández ( 2019 ), Beneduce ( 2020 ), Jackson ( 2021 ), Yarger et al. ( 2023 ), and Avery et al. ( 2023 ), when partial human data is provided to a machine, so the algorithm is biased, it will eventually lead to the risk of “agent discrimination.” In recruitment, algorithmic bias can manifest in gender, race, skin color, and personality.
The fourth theme is algorithmic recruitment discrimination resolution. Kitchin and Lauriault ( 2015 ), Bornstein ( 2018 ), Raso et al. ( 2018 ), Xie et al. ( 2018 ), Raub ( 2018 ), Bornstein ( 2018 ), Grabovskyi and Martynovych ( 2019 ), Amini et al. ( 2019 ), Shin and Park ( 2019 ), Yarger et al. ( 2019 ), Gulzar et al. ( 2019 ), Kessing ( 2021 ) Jackson ( 2021 ), and Mishra ( 2022 ) argue that fair data sets need to be constructed and algorithmic transparency needs to be improved. Moreover, Smith and Shum ( 2018 ), Mitchell et al. ( 2019 ), Ong ( 2019 ), Zuiderveen Borgesius ( 2020 ), Peña et al. ( 2020 ), Kim et al. ( 2021 ), Yang et al. ( 2021 ), and Jackson ( 2021 ) propose that from a management perspective, data governance need be strengthened, including internal ethical governance and external ethical oversight.
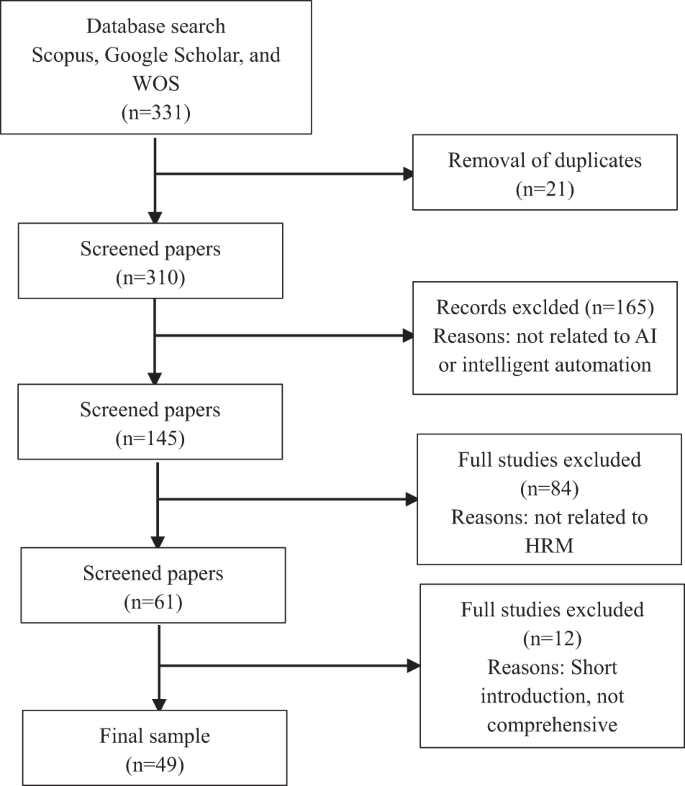
Procedures used in the literature review to reveal emerging themes.
Through this review, we have created an overarching conceptual framework to visualize how AI and AI-based technologies impact recruitment. This framework is illustrated in Fig. 2 and aligns with the four research themes identified.
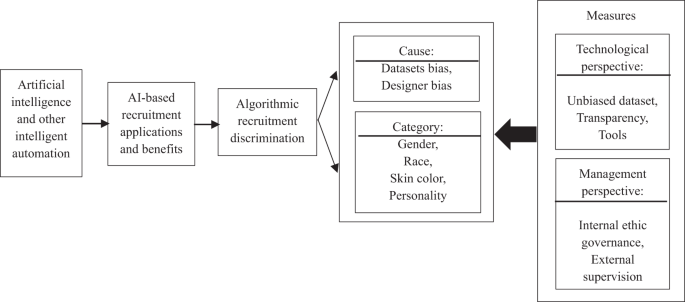
An overarching conceptual framework to visualize how AI and AI-based technologies can impact recruitment efforts.
Theme I. AI-based recruitment applications and benefits
Artificial intelligence and algorithm.
The idea of machine intelligence dates back to 1950, when Turing, the father of computer science, asked, “Can machines think?” (Ong, 2019 ). The term “artificial intelligence” was coined by John McCarthy. Although early scientists made outstanding contributions, artificial intelligence became an industry after the 1980s with hardware development. Initial applications of artificial intelligence were seen in the automation of repeated and complicated work assignments, like industrial robot production, that displaced human work in some plants. After the mid-1990s, artificial intelligence software saw significant advances. Until today’s digital economy, AI has been commonly used in various industries (Hmoud and Laszlo, 2019 ).
Artificial intelligence is defined as the ability of something like a machine to understand, learn, and interpret on its own in a human-like manner (Johansson and Herranen, 2019 ). Artificial intelligence aims “to understand and simulate human thought processes and to design machines that mimic this behavior.” It is designed to be a thinking machine with a level of human intelligence (Jackson, 2021 ). However, large amounts of data must be combined with fast and iterative intelligent algorithms to handle this process, allowing ML systems to learn from patterns or features in the data automatically.
A set of instructions or commands used to carry out a particular operation is known as an algorithm. This digital process makes decisions automatically depending on the data entered into the program (Miasato and Silva, 2019 ). The algorithm analyzes massive data patterns through data mining, searching, and using ways to predict, like our point of view encoded in the code. It explores the dataset using agents representing various traits, such as race, sexual orientation, and political opinions (Njoto, 2020 ). The algorithms frequently contain these biases due to the lengthy history of racial and gender prejudices, both intentional and unconscious. When biases exist in algorithmic data, AI may replicate these prejudices in its decision-making, a mistake known as algorithmic bias (Jackson, 2021 ).
AI-based recruitment stage
The recruitment procedure is a series of events that may be divided into four significant steps: searching, screening, interviewing, and selection (Bogen and Rieke, 2018 ). Each phase includes various activities, and artificial intelligence technology can influence the execution of each stage.
The searching phase aims at a system for searching web content. It screens passive job applicants online through social media and recruitment platforms, analyzing their profiles according to predefined job descriptions. The search engine recognizes the meaning of the searched content and performs a web-based search to match candidates’ profiles based on semantic annotations of job postings and profiles (Hmoud and Laszlo, 2019 ).
The screening phase includes evaluating and assessing the qualifications of candidates, where AI technology assists recruiters in scoring candidates and evaluating their competencies (Bogen and Rieke, 2018 ). The resumes are screened to match the job description better. The system can rank candidates according to the relevance of the qualification metrics.
The following phase is the interview. It is probably the most individual stage of the selection process and, thus, unlikely to be fully automated by artificial intelligence. However, some AI tools enable recruiters to conduct video interviews and research candidates’ reactions, voice tones, and facial expressions (Ahmed, 2018 ).
The final stage is the selection stage, which is the stage where the employer makes the final employment decision. In this stage, AI systems can calculate remuneration and benefits for companies and anticipate the risk that candidates would violate workplace rules. (Bogen and Rieke, 2018 ).
AI-based recruitment benefits
Recruitment quality.
Beattie et al. ( 2012 ) found that some large companies believe unconscious bias affects recruitment quality. Organizations may need to hire more qualified people to avoid financial losses (Newell, 2015 ). Artificial intelligence has become a part of the recruitment industry to automate the recruiting and selecting process, which can remove unconscious human bias that affects the hiring process (Raub, 2018 ). One of the ideas behind the development of AI in the selection of candidates is to bring higher standards to the selection process independent of the thoughts and beliefs of the interviewer (Miasato and Silva, 2019 ). Artificial intelligence tools can start with accurate job descriptions and targeted advertisements that match a candidate’s skills and abilities to job performance and create a profile of every candidate that indicates which candidate is best suited for the job (Johnson et al. 2020 ). In addition, automated resume screening systems allow recruiters to consider more candidates that would be overlooked (Beneduce, 2020 ). With advances in AI technology, candidate selection becomes impersonal based on data shared with the company and available on the Internet.
Recruitment efficiency
HR departments may receive many candidates for every position. Traditional screening and selection that depends on human intervention to evaluate candidate information is the most expensive and discouraging hiring process (Hmoud and Laszlo, 2019 ). Artificial intelligence can accelerate the hiring procedure, produce an outstanding candidate experience, and reduce costs (Johansson and Herranen, 2019 ). It can bring job information to applicants faster, allowing them to make informed decisions about their interests early in the hiring process. Artificial intelligence can also screen out many uninterested applicants and remove them from the applicant pool, thus reducing the number of applicants recruiters need to select later. It is even possible to source reticent candidates with the help of artificial intelligence and have more time to concentrate on the best match. Artificial intelligence can not only automate the evaluation of hundreds of resumes on a large scale in a short period, but it can also automatically classify candidates based on the job description provided. Moreover, The final results after the hiring decision can be more easily fed back to the candidate (Raveendra et al., 2020 ).
Transactional workload
The application of AI in recruitment can be described as a “new era in human resources” because artificial intelligence replaces the routine tasks performed by human recruiters, thus changing the traditional practices of the recruitment industry (Upadhyay and Khandelwal, 2018 ). Most professionals believe that AI is beneficial to recruiters in terms of reducing routine and administrative tasks (Johansson and Herranen, 2019 ). Recruiters will hand over time-consuming administrative tasks like recruiting, screening, and interviewing to AI, allowing more scope for recruiters to concentrate on strategic affairs (Upadhyay and Khandelwal, 2018 ).
Theme II. Why is there algorithmic recruitment discrimination
Algorithms are not inherently discriminatory, and engineers rarely intentionally introduce bias into algorithms. However, bias can still arise in algorithmic recruitment. This issue is closely linked to the fundamental technology behind AI and ML. The ML process can be simplified into several stages, each involving three key components contributing to algorithmic bias: dataset construction, the engineer’s target formulation, and feature selection (36KE, 2020 ). When the dataset lacks diverse representation from different companies, bias may be introduced during the development of algorithmic rules by engineers and when annotators handle unstructured data (Zixun, 2020 ).
Datasets: bias soil
Datasets serve as the foundation of machine learning (ML). If an algorithm’s data collection lacks quantity and quality, it will fail to represent reality objectively, leading to inevitable bias in algorithmic decisions. Researchers commonly use a 95% confidence level, which provides 95% certainty but still leaves a one in twenty chance of bias (Raub, 2018 ). Nearly every ML algorithm relies on biased databases.
One issue arises when datasets are skewed towards accessible and more “mainstream” groups due to the ease of data collection. Consequently, there is an imbalance in the distribution concerning gender and race dimensions (36KE, 2020 ). If the collected data inadequately represent a particular race or gender, the resulting system will inevitably overlook or mistreat them in its performance. In the hiring process, insufficient data may exclude historically underrepresented groups (Jackson, 2021 ). Assessing the success of potential employees based on existing employees perpetuates a bias toward candidates who resemble those already employed (Raghavan et al., 2020 ).
Without careful planning, most datasets consist of unstructured data acquired through observational measures, lacking rigorous methods in controlled environments (McFarland and McFarland, 2015 ). This can lead to significant issues with misreporting. When algorithms play a role in decision-making, underrepresented individuals are unequally positioned. Furthermore, as AI improves the algorithm, the model accommodates the lack of representation, reducing sensitivity to the underrepresented groups. The algorithm favors the represented group, operating less effectively for other groups (Njoto, 2020 ).
Existing social biases are introduced into the dataset. The raw data already reflects social prejudices, and the algorithm also incorporates biased relationships, leading to the “bias in and bias out” phenomenon (36KE, 2020 ). This phenomenon means that discrimination and disparities exist, just like in forecasting, where historical inequalities are projected into the future and may even be amplified (Mayson, 2018 ).
A research team at Princeton University discovered that algorithms lack access to the absolute truth. The machine corpus contains biases that closely resemble the implicit biases observed in the human brain. Artificial intelligence has the potential to perpetuate existing patterns of bias and discrimination because these systems are typically trained to replicate the outcomes achieved by human decision-makers (Raso et al. 2018 ). What is worse, the perception of objectivity surrounding high-tech systems obscures this fact.
In summary, if an algorithmic system is trained on biased and unrepresentative data, it runs the risk of replicating that bias.
Data feature selection: designer bias
The introduction of bias is sometimes not immediately apparent in model construction because computer scientists are often not trained to consider social issues in context. It is crucial to make them aware of attribute selection’s impact on the algorithm (Yarger et al., 2019 ).
The algorithm engineer plays a crucial role in the entire system, from setting goals for machine learning to selecting the appropriate model and determining data characteristics such as labels. If inappropriate goals are set, bias may be introduced from the outset (36KE, 2020 ).
An engineer is responsible for developing the algorithmic model. If they hold certain beliefs and preconceptions, those personal biases can be transmitted to the machine (Njoto, 2020 ). Although the device is responsible for selecting employee resumes, it operates based on underlying programming. The programmer guides the AI in making decisions about the best candidate, which can still result in discrimination (Miasato and Silva, 2019 ).
Furthermore, personal biases can manifest in the selection of data characteristics. For example, engineers may prioritize specific features or variables based on how they want the machine to behave (Miasato and Silva, 2019 )). The Amazon hiring case illustrates this, where engineers considered education, occupation, and gender when assigning labels to the algorithm. When gender is considered the crucial criterion, it influences how the algorithm responds to the data.
Theme III. Which algorithmic recruitment discrimination exists
In the recruitment process, algorithmic bias can be manifested in terms of gender, race, color, and personality.
Gender stereotypes have infiltrated the “lexical embedding framework” utilized in natural language processing (NLP) techniques and machine learning (ML). Munson’s research indicates that “occupational picture search outcomes slightly exaggerate gender stereotypes, portraying minority-gender occupations as less professional” ((Avery et al., 2023 ; Kay et al., 2015 ).
The impact of gender stereotypes on AI hiring poses genuine risks (Beneduce, 2020 ). In 2014, Amazon developed an ML-based hiring tool, but it exhibited gender bias. The system did not classify candidates neutrally for gender (Miasato and Silva, 2019 ). The bias stemmed from training the AI system on predominantly male employees’ CVs (Beneduce, 2020 ). Accordingly, the recruitment algorithm perceived this biased model as indicative of success, resulting in discrimination against female applicants (Langenkamp et al. 2019 ). The algorithm even downgraded applicants with keywords such as “female” (Faragher, 2019 ). These findings compelled Amazon to withdraw the tool and develop a new unbiased algorithm. However, this discrimination was inadvertent, revealing the flaws inherent in algorithmic bias that perpetuates existing gender inequalities and social biases (O’neil, 2016 ).
Microsoft’s chatbot Tay learned to produce sexist and racist remarks on Twitter. By interacting with users on the platform, Tay absorbed the natural form of human language, using human tweets as its training data. Unfortunately, the innocent chatbot quickly adopted hate speech targeting women and black individuals. As a result, Microsoft shut down Tay within hours of its release. Research has indicated that when machines passively absorb human biases, they can reflect subconscious biases (Fernández and Fernández, 2019 ; Ong, 2019 ). For instance, searches for names associated with Black individuals were more likely to be accompanied by advertisements featuring arrest records, even when no actual records existed. Conversely, searches for names associated with white individuals did not prompt such advertisements (Correll et al., 2007 ). A study on racial discrimination revealed that candidates with white names received 50% more interview offers than those with African-American names.
In 2015, Google’s photo application algorithm erroneously labeled a photo of two black people as gorillas (Jackson, 2021 ). The algorithm was insufficiently trained to recognize images with dark skin tones (Yarger et al., 2023 ). The company publicly apologized and committed to immediately preventing such errors. However, three years later, Google discontinued its facial identification service, citing the need to address significant technical and policy issues before resuming this service. Similarly, in 2017, an algorithm used for a contactless soap dispenser failed to correctly identify shades of skin color, resulting in the dispenser only responding to white hands and not detecting black and brown ones. These cases serve as examples of algorithmic bias (Jackson, 2021 ).
Personality
The algorithm assesses word choice, tone shifts, and facial expressions (using facial recognition) to determine the candidate’s “personality” and alignment with the company culture (Raso et al., 2018 ). Notable examples include correlating longer tenure in a specific job with “high creativity” and linking a stronger inclination towards curiosity to a higher likelihood of seeking other opportunities (O’neil, 2016 ). Additionally, sentiment analysis models are employed to gauge the level of positive or negative emotions conveyed in sentences.
Theme IV. How decreasing algorithmic recruitment discrimination
Changes should be made at the technical and regulatory levels to ensure that AI algorithms do not replicate existing biases or introduce new ones based on the provided data (Raub, 2018 ).
Building fair algorithms from a technical perspective
Constructing a more unbiased dataset.
Unfair datasets are the root cause of bias. Therefore, a direct approach to addressing algorithmic bias is reconfiguring unbalanced datasets. Using multiple data points can yield more accurate results while carefully eliminating data points that reflect past biases. However, this approach incurs significant costs (Bornstein, 2018 ).
Another method is to correct data imbalances by using more equitable data sources to ensure fair decision-making (36KE, 2020 ). Understanding the underlying structure of training data and adjusting the significance of specific data points during training based on known latent distributions makes it possible to uncover hidden biases and remove them automatically. For example, Microsoft revised their dataset for training the Face API, resulting in a 20-fold reduction in the recognition error ratio between men and women with darker skin tones and a 9-fold reduction for women by balancing factors such as skin color, age, and gender (Grabovskyi and Martynovych, 2019 ).
Integrating “small data” and “big data” can enhance accuracy (36KE, 2020 ). Data should not solely rely on extensive collections but also focus on precision. While big data analysis tends to emphasize correlations, which can lead to errors when inferring causation, small data, which is more user-specific, offers detailed information and helps avoid such mistakes. Combining the vastness of big data with the precision of small data can help somewhat mitigate hiring errors (Kitchin and Lauriault, 2015 ).
Biases in datasets can be identified through autonomous testing. The inaccuracies stemming from incomplete past data can be addressed through “oversampling” (Bornstein, 2018 ). Researchers from MIT demonstrated how an AI system called DB-VEA (unsupervised learning) can automatically reduce bias by re-sampling data. This approach allows the model to learn facial features such as skin color and gender while significantly reducing categorization biases related to race and gender (Amini et al., 2019 ).
Therefore, constructing a more unbiased dataset is one of the methods that can be employed to tackle algorithmic bias.
Enhancing algorithmic transparency
Engineers write algorithmic models, but they often need help understanding the processes that AI undergoes to produce a specific outcome. Many algorithmic biases are difficult to fully understand because their techniques and methods are not easily visible. This leaves many people unaware of why or how they are discriminated against and lacks public accountability (Jackson, 2021 ). There is an issue of “algorithmic black box” in ML. Therefore, transparency would facilitate remediation when deviant algorithms are discovered and solve the current “black box” dilemma (Shin and Park, 2019 ).
Technological tools against bias
Data blending process. Blendoor is inclusive recruiting and staffing analytics software that mitigates unconscious bias. It takes candidate profiles from existing online job boards and applicant tracking systems to reduce unconscious bias. Blendoor “blends” candidate profiles by removing names, photos, and dates (Yarger et al., 2019 ). Thus, Blendoor promotes design fairness by assisting underrepresented job seekers and encoding equal opportunity in the algorithm.
Decoupling technique. In resume screening, this technique allows the algorithm to identify the best candidates by considering variables optimized for other applicants based on specific categories like gender or race rather than the entire applicant pool (Raso et al., 2018 ). It means that the characteristics selected for minority or female applicants will be determined according to the trends of other minority or female applicants, which may differ from the features identified as successful representatives.
Word embedding. Microsoft researchers found that words exhibit distinct associations in news and web data. For instance, words like “fashion” and “knitting” are more closely related to females, while “hero” and “genius” are more closely related to males (36KE, 2020 ). Microsoft suggests a simple solution by removing the gender-specific measures in word embedding to reduce “presentation bias.”
Differential testing. Scientists at Columbia University developed Deep Xplore, a software that highlights vulnerabilities in algorithmic neural networks via “coaxing” the system to make mistakes (Xie et al., 2018 ). Deep Xplore utilizes discrepancy testing, which involves comparing several systems and observing their outputs’ differences. A model is considered vulnerable if all other models consistently predict a particular input while only one model predicts it differently (Gulzar et al., 2019 ).
Bias detection tool. In September 2018, Google introduced the innovative What-If tool for detecting bias (Mishra, 2022 ). It assists designers in identifying the causes of misclassification, determining decision boundaries, and detecting algorithmic fairness through interactive visual interfaces. Additionally, Facebook has developed Fairness Flow, an emerging tool for correcting algorithmic bias. Fairness Flow automatically notifies developers if an algorithm makes unfair judgments based on race, gender, or age (Kessing, 2021 ).
Improving the algorithm’s ethics from a management perspective
Internal ethics governance.
Several major technology companies have published AI principles addressing bias governance, signaling the start of self-regulation (36KE, 2020 ). Microsoft has formed an AI and ethical standards committee to enforce these principles, subjecting all future AI products to ethics scrutiny (Smith and Shum, 2018 ). Google has responded by introducing a Model Card function, similar to an algorithm manual, that explains the employed algorithm, highlights strengths and weaknesses, and even shares operational results from various datasets (Mitchell et al., 2019 ).
Algorithmic systems undergo audits to prevent unintended discrimination and make necessary adjustments to ensure fairness (Kim et al., 2021 ). Regular internal audits allow companies to monitor, identify, and correct biased algorithms. Increased involvement from multiple parties in the data collection process and continuous algorithm monitoring are essential to reduce or eliminate bias (Jackson, 2021 ). Some companies have introduced AI-HR audits, similar to traditional HR audits, to review employee selection and assess the reliability of AI algorithms and ML data (Yang et al., 2021 ). Companies should also stay updated on recruitment laws and regulations and ensure compliance with legal requirements.
Considering the programmers behind these algorithms, diversity in the high-tech industry is crucial. Algorithms often reflect the opinions of those who create them. The persistent underrepresentation of women, African-Americans, and Latino professionals in the IT workforce leads to biased algorithms. For instance, a study in 2019 found that only 2.5% of Google’s employees were black, while Microsoft and Facebook had only 4% representation. Another study revealed that 80% of AI professors in 2018 were male. Involving diverse individuals in data collection and training can regulate and eliminate human bias rooted in algorithms (Jackson, 2021 ).
Although self-regulation can help reduce discrimination and influence lawmakers, it has potential drawbacks. Self-regulation lacks binding power, necessitating external oversight through third-party testing and the development of AI principles, laws, and regulations by external agencies.
External supervision
To ensure transparency and accountability in recruitment, third-party certification and testing of AI products can help mitigate the negative impacts of unreliability. At the “Ethics and Artificial Intelligence” technical conference held at Carnegie Mellon University, the director of Microsoft Research Institute proposed a solution to ensure consistent standards and transparency in AI. Microsoft’s proposal, “Allowing third-party testing and comparison,” aims to uphold the integrity of AI technology in the market (Ong, 2019 ).
Various organizations have issued principles promoting equity, ethics, and responsibility in AI (Zuiderveen Borgesius, 2020 ). The Organization for Economic Cooperation and Development (OECD) has provided recommendations on AI, while the European Commission has drafted proposals regarding the influence of algorithmic systems on human rights. In 2019, the European Commission established a high-level expert group on AI, which proposed ethical guidelines and self-regulatory measures regarding AI and ethics.
Public organizations have played a role in establishing mechanisms to safeguard algorithmic fairness. The Algorithm Justice League (AJL) has outlined vital behaviors companies should follow in a signable agreement. Holding accountable those who design and deploy algorithms improves existing algorithms in practice (36KE, 2020 ). After evaluating IBM’s algorithm, AJL provided feedback, and IBM responded promptly, stating that they would address the identified issue. As a result, IBM significantly improved the accuracy of its algorithm in minority facial identification.
Data protection and non-discrimination laws safeguard against discriminatory practices in algorithmic decision-making. In the EU region, Article 14 of the European Convention on Human Rights (ECHR) guarantees the rights and freedoms outlined in the Convention, prohibiting direct and indirect discrimination (Zuiderveen Borgesius, 2020 ). Non-discrimination laws, particularly those about indirect discrimination, serve as a means to prevent various forms of algorithmic discrimination. The EU General Data Protection Regulation (GDPR), implemented in May 2018, addresses the impact of ML algorithms and offers a “right to explanation” (e.g., Articles 13–15) (Peña et al., 2020 ), enabling individuals to request explanations for algorithmic decisions and demand measures to avoid discriminatory influences when handling sensitive data. The GDPR mandates organizations to conduct a Data Protection Impact Assessment (DPIA), with each EU member state must maintain an independent data protection authority vested with investigative powers. Under the GDPR, a data protection authority can access an organization’s premises and computers using personal data (Zuiderveen Borgesius, 2020 ).
Investigation and analysis
Based on Grounded Theory, this section uses a qualitative research approach to explore AI-supported recruitment applications and discrimination.
Sources and methods
The study is based on Grounded Theory and qualitative analysis of interview data. Glaser and Strauss (1965,1968) proposed this theory. The basic idea is constructing a theory based on empirical data (Charmaz and Thornberg, 2021 ). Researchers generally do not make any theoretical assumptions before starting scientific research but start directly from a realistic point of view and summarize several empirical concepts in primary data, which are then raised to systematic theoretical knowledge. Grounded Theory must be supported by empirical evidence, but its most significant characteristic is not its practical nature but the extraction of new ideas from existing facts.
Grounded Theory is a qualitative approach to research that focuses on the importance of “primary sources” (Timmermans and Tavory, 2012 ). In the study of AI-driven hiring discrimination, the systematic collection and analysis of data are used to uncover intrinsic patterns, construct relevant concepts, and refine relevant theoretical models instead of adopting theoretical assumptions. The current research on the influence factors and measures of AI-driven recruitment discrimination is not intensive enough and lacks corresponding theoretical support. At the same time, Grounded Theory extracts from “primary data” and constructs a theoretical model to study AI-driven recruitment applications and discrimination.
Interviewees and content
Participants in the interview.
The interview period was June 2023, and the interview form was a face-to-face live/video/telephone interview. The interviewees were selected considering representativeness, authority, and operability, and ten people with experience in recruiting or interviewing with the help of intelligent tools were finally selected for the study. The basic information of the interviewees is shown in Table 2 . The study was conducted with the interviewees’ consent. Each interview lasted about 30 min, and notes were taken during the interview. The number of interviewees was determined based on the principle of information saturation.
Before conducting interviews, a large amount of data is collected to understand AI-driven hiring discrimination and propose appropriate improvement strategies. A study of AI-driven hiring discrimination was conducted using “dynamic sampling” and “information saturation” methods.
Interview outline
The interview outline was set in advance around the core objectives of this study, including the following six questions: “Do you know about AI-driven recruitment,” “How do you think about AI-driven recruitment discrimination,” “What do you think is the cause of AI-driven recruitment discrimination,” “Types of AI-driven hiring discrimination,” “Strategies to solve AI-driven hiring discrimination,” and “What other suggestions do you have.” Based on the predefined interview outline, appropriate adjustments were made as the interview progressed.
Interview ethics
The interview process is based on three main principles—the right-to-know principle. The interviewer fully understands the purpose, content, and use of the interview before being interviewed, the principle of objectivity. The researcher will guide the respondent to ask questions and answer what they cannot understand. Respondents make objective statements of their willingness that are not influenced by external factors, the principle of confidentiality. Interviews will be conducted anonymously, and the personal information of the interviewees will not be disclosed. The interviewee’s privacy is fully respected, and the original data is replaced by figures, which will be used only for the interviewer’s reference and analysis, appropriately kept by the interviewer, and used only for this study and no other purpose.
Interview tools
Nvivo 12.0 Plus qualitative analysis software was used as an auxiliary tool to clarify ideas and improve work efficiency.
Data organization after the interview
Within two working days after the completion of the interview, the analysis and organization of the interview data was completed. The Nvivo 12 plus software coded the interview data in three layers from the bottom up, with the content as the center.
The first layer was open coding. The interview data of 10 interviewees were imported and, using the software, parsed word by word to clarify the meaning of words and sentences, give an interpretation of the data, and obtain free nodes. The data from each section was summarized and inferred to organize the interviewees’ perceptions of AI-driven recruitment, and each node was given a name to derive the first-level nodes.
Next, the second-level spindles were coded. The researchers unfolded the induction of interrelated classes for the nodes formed by the open coding, constructed the relationship between concepts and classes, and coded a spindle concept, which, after the spindle coding, would form a second-level node.
The third part is the three-level core coding. Another coding core class genus is selected based on the secondary spindle, and a tertiary code is developed.
Interview quality control
In order to ensure the credibility of the interview results, the method adopted in this study is to use a uniform way of asking questions to different interviewees. Each round of interviews should be kept between 20 and 30 min. Too long an interval will reduce the effectiveness of feedback on the questions. Also, interviews should not span more than one month to ensure the timeliness of the information obtained.
Applying the Nvivo12 Plus qualitative analysis method, 182 free nodes were obtained by three-level coding, 31 primary nodes were formed after analogy, and 11 secondary nodes were deduced inductively. Finally, five core genera of tertiary nodes were identified (see Table 3 ).
Open level 1 coding
The interviews with 10 respondents resulted in 182 words and sentences related to AI-driven recruitment applications and discrimination, which were conceptualized and merged to form 31 open-ended Level 1 codes.
Main-axis second-level coding
The spindle codes were analyzed through cluster analysis to analyze the correlation and logical order among the primary open codes, forming more generalized categories. Eleven spindle codes were extracted and summarized.
Core-type tertiary coding
The Grounded Theory steps resulted in 31 open primary and 11 secondary spindle codes. Further categorization and analysis revealed that when “AI-driven recruitment applications and discrimination” is used as the core category, the five main categories are AI-driven recruitment applications, AI-driven recruitment effects, causes of AI-driven recruitment discrimination, types of AI-driven recruitment discrimination, and AI-driven recruitment discrimination measures.
The coding process described above was exemplified by an interview with a researcher, F2, who had taught information science at a university for 2 years and was now employed at an intelligent technology R&D company. After the interview, F1’s information was analyzed and explored in a three-level coding process.
Under the three-level node AI-driven recruitment application, F2 suggested that the AI tools currently developed could assist companies with simple recruitment tasks, including online profile retrieval, analysis, and evaluation. However, this technical engineer suggested that candidate assessment for high-level positions suits human-machine collaboration, although machines have an advantage in candidate profile searches.
Supported by three-level nodes of AI-driven recruiting effectiveness, F2 suggests that machine applications in recruiting can relieve human transactional workload, and chatbot Q&A services improve recruiting efficiency.
In the context of the causes of AI-driven hiring discrimination at the third level, F2 suggests that some job seekers are unfamiliar with the hiring interface and how to use it, leading to unfair interviews. She suggested the need for organizers to prepare usage guidelines or mock interview exercises. She argues that much of the data needed for intelligent machine learning comes from internal companies or external market supplies and that this data lacks fair scrutiny. It is even possible that the source data fed into the machines is compromised.
Under the tertiary node AI-driven hiring discrimination, F2 is concerned that the machines may misevaluate candidates due to individual differences, such as intelligence, or external characteristics, such as skin color. Moreover, some discriminatory judgments are difficult to resolve under current technology.
Under the tertiary node AI-driven hiring discrimination measures, F2 proposes utilizing technical tools, such as learning impartial historical data, or non-technical tools, such as anti-AI discrimination laws. She argues that in the future, humans use AI tools to solve more complex decisions, not just limited to hiring. Instead, humans need to embrace and accept the widespread use of machines.
Synthesizing the above analysis, the final overview of the AI-driven recruitment application and discrimination framework is obtained (see Fig. 3 ). After the conceptual model was constructed, the remaining original information was coded and comparatively analyzed, and no new codes were generated, indicating that this study was saturated.
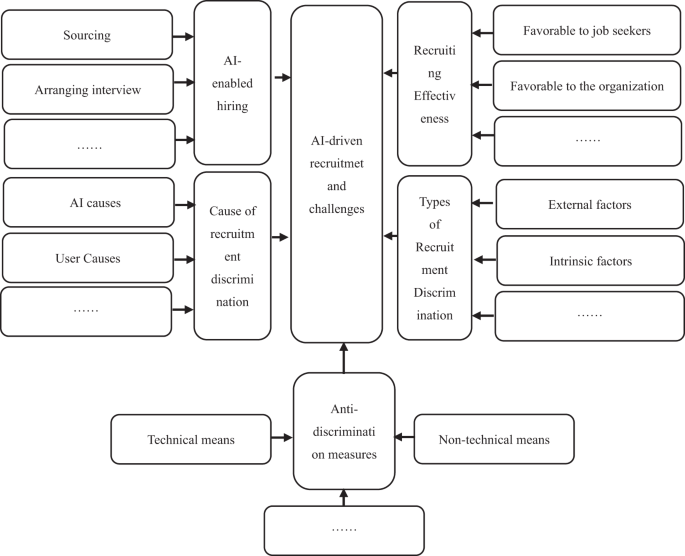
The comprehensive analysis produces an overview of AI-driven recruitment applications and recognition frameworks.
An analysis of interview results conducted using Grounded Theory indicates that AI-supported hiring discrimination should be approached from five perspectives. These perspectives align with the thematic directions identified through our literature review.
Firstly, AI-driven hiring applications impact various aspects, such as reviewing applicant profiles online, analyzing applicant information, scoring assessments based on hiring criteria, and generating preliminary rankings automatically.
Secondly, interviewers perceive benefits in AI-driven recruitment for job seekers. It eliminates subjective human bias, facilitates automated matchmaking between individuals and positions, and provides automated response services. Moreover, AI reduces the workload on humans and enhances efficiency.
Thirdly, concerns are raised regarding potential hiring discrimination perpetrated by machines. This can arise from AI tools, such as partial source data, or users unfamiliar with user interfaces and operations.
Fourthly, intrinsic factors like personality and IQ, as well as extrinsic factors like gender and nationality, have been observed to influence the accurate identification and judgment of AI systems concerning hiring discrimination.
Fifthly, respondents offer recommendations for combating discrimination by machines, including technical and non-technical approaches.
Recommendations for future studies
This study conducted a literature review to analyze algorithmic recruitment discrimination’s causes, types, and solutions. Future research on algorithmic recruitment discrimination could explore quantitative analysis or experimental methods across different countries and cultures. Additionally, future studies could examine the mechanics of algorithmic recruitment and the technical rules that impact the hiring process. It would be interesting to analyze the psychological effects of applying this algorithmic recruitment technique on various populations (gender, age, education level) from an organizational behavior perspective. While recent studies have primarily discussed discrimination theory in the traditional economy’s hiring market, future theoretical research should consider how advanced technology affects equity in hiring within the digital economy.
The study concludes that the fourth industrial revolution introduced technological innovations significantly affecting the recruitment industry. It extends the analysis of statistical discrimination theory in the digital age and adopts a literature review approach to explore four themes related to AI-based recruitment. The study argues that algorithmic bias remains an issue while AI recruitment tools offer benefits such as improved recruitment quality, cost reduction, and increased efficiency. Recruitment algorithms’ bias is evident in gender, race, color, and personality. The primary source of algorithmic bias lies in partial historical data. The personal preferences of algorithm engineers also contribute to algorithmic bias. Technical measures like constructing unbiased datasets and enhancing algorithm transparency can be implemented to tackle algorithmic hiring discrimination. However, strengthening management measures, such as corporate ethics and external oversight, is equally important.
Data availability
The study is still ongoing, and the results of subsequent analyses will continue to be applied to valuable and critical projects. Relevant data are currently available only to scholars conducting similar research, with the prerequisite of signing a confidentiality agreement. Corresponding author can be contacted for any requests.
36KE (2020) From sexism to recruitment injustice, how to make AI fair to treat. https://baijiahao.baidu.com/s?id=1663381718970013977&wfr=spider&for=pc
Ahmed O (2018) Artificial intelligence in HR. Int J Res Anal Rev 5(4):971–978
Google Scholar
Albert ET (2019) AI in talent acquisition: a review of AI-applications used in recruitment and selection. Strateg HR Rev 18(5):215–221. https://doi.org/10.1108/SHR-04-2019-0024
Article Google Scholar
Allal-Chérif O, Yela Aránega A, Castaño Sánchez R (2021) Intelligent recruitment: how to identify, select, and retain talents from around the world using artificial intelligence. Technol Forecast Soc Change 169:120822. https://doi.org/10.1016/j.techfore.2021.120822
Amini A, Soleimany AP, Schwarting W, Bhatia SN, Rus D (2019) Uncovering and mitigating algorithmic bias through learned latent structure. In: Conitzer V, Hadfield G, Vallor S (eds) Proceedings of the 2019 AAAI/ACM conference on AI, ethics, and society. Association for Computing Machinery
Avery M, Leibbrandt A, Vecci J (2023) Does artificial intelligence help or hurt gender diversity? In: Conitzer V, Hadfield G, Vallor SE (eds) vidence from two field experiments on recruitment in Tech, 14 February 2023. Association for Computing Machinery
Beattie G, Johnson PJPP, Education PiH (2012) Possible unconscious bias in recruitment and promotion and the need to promote equality. Perspect: Policy Pract High Educ 16(1), 7–13
Beneduce G (2020) Artificial intelligence in recruitment: just because it’s biased, does it mean it’s bad? NOVA—School of Business and Economics
Black JS, van Esch P (2020) AI-enabled recruiting: what is it and how should a manager use it? Bus Horiz 63(2):215–226. https://doi.org/10.1016/j.bushor.2019.12.001
Bogen M, Rieke A (2018) Help wanted: an examination of hiring algorithms, equity, and bias. Upturn
Bornstein S (2018) Antidiscriminatory algorithms. Alabama Law Rev 70:519
Cain GG (1986) The economic analysis of labor market discrimination: a survey. Handb Labor Econ 1:693–785
Charmaz K, Thornberg R (2021) The pursuit of quality in grounded theory. Qual Res Psychol 18(3):305–327. https://doi.org/10.1080/14780887.2020.1780357
Chen Z (2022) Artificial intelligence-virtual trainer: innovative didactics aimed at personalized training needs. J Knowl Econ. https://doi.org/10.1007/s13132-022-00985-0
Chen Z (2023) Collaboration among recruiters and artificial intelligence: removing human prejudices in employment. Cogn Technol Work 25(1):135–149
Article PubMed CAS Google Scholar
Correll SJ, Benard S, Paik I (2007) Getting a job: is there a motherhood penalty? Am J Sociol 112(5):1297–1338
Dickinson DL, Oaxaca RL (2009) Statistical discrimination in labor markets: an experimental analysis. South Econ J 76(1):16–31
Faragher JJEWSTBANHS (2019) Is AI the enemy of diversity? People Management
Fernández C, Fernández A (2019) Ethical and legal implications of AI recruiting software. Ercim News 116:22–23
Grabovskyi V, Martynovych O (2019) Facial recognition with using of the microsoft face API Service. Electron Inf Technol 12:36–48
Gulzar MA, Zhu Y, Han X (2019) Perception and practices of differential testing. In: Conitzer V, Hadfield G, Vallor S (eds) 2019 IEEE/ACM 41st International Conference on Software Engineering: Software Engineering in Practice (ICSE-SEIP). Association for Computing Machinery
Hmoud B, Laszlo V (2019) Will artificial intelligence take over human resources recruitment and selection? Netw Intell Stud 7(13):21–30
Jackson MC (2021) Artificial intelligence & algorithmic bias: the issues with technology reflecting history & humans. J Bus Technol Law 16:299
Johansson J, Herranen S (2019) The application of artificial intelligence (AI) in human resource management: current state of AI and its impact on the traditional recruitment process. Bachelor thesis, Jonkoping University
Johnson RD, Stone DL, Lukaszewski KM (2020) The benefits of eHRM and AI for talent acquisition. J Tour Futur 7(1):40–52
Kay M, Matuszek C, Munson SA (2015) Unequal representation and gender stereotypes in image search results for occupations. In: Conitzer V, Hadfield G, Vallor S (eds) Proceedings of the 33rd annual ACM conference on human factors in computing systems. Association for Computing Machinery
Kessing M (2021) Fairness in AI: discussion of a unified approach to ensure responsible AI development. Independent thesis Advanced level, KTH, School of Electrical Engineering and Computer Science (EECS)
Kim PT, Bodie MTJJOL, Law E (2021) Artificial intelligence and the challenges of workplace. Discrim Privy 35(2):289–315
Kitchin R, Lauriault TPJG (2015) Small data in the era of big data 80(4):463–475
Köchling A, Wehner MC, Warkocz J (2022) Can I show my skills? Affective responses to artificial intelligence in the recruitment process. Rev Manag Sci. https://doi.org/10.1007/s11846-021-00514-4
Langenkamp M, Costa A, Cheung C (2019) Hiring fairly in the age of algorithms. Available at: SSRN 3723046
Lloyd K (2018) Bias amplification in artificial intelligence systems. arXiv preprint arXiv07842
Lundberg SJ, Startz R (1983) Private discrimination and social intervention in competitive labor market. Am Econ Rev 73(3):340–347
Mayson SG (2018) Bias in, bias out. Yale Law J 128(8):2218–2300
McFarland DA, McFarland HR (2015) Big data and the danger of being precisely inaccurate. Big Data Soc Hum Resour Manag 2(2):2053951715602495
Miasato A, Silva FR (2019) Artificial intelligence as an instrument of discrimination in workforce recruitment. Acta Univ Sapientiae: Legal Stud 8(2):191–212
Mishra P (2022) AI model fairness using a what-if scenario. In: Practical explainable AI using Python. Springer, pp. 229–242
Mitchell M, Wu S, Zaldivar A, Barnes P, Vasserman L, Hutchinson B, … Gebru T (2019) Model cards for model reporting. In: Conitzer V, Hadfield G, Vallor S (eds) Proceedings of the conference on fairness, accountability, and transparency. Association for Computing Machinery
Newell S (2015) Recruitment and selection. Managing human resources: personnel management in transition. Blackwell Publishing, Oxford
Njoto S (2020) Research paper gendered bots? Bias in the use of artificial intelligence in recruitment
O’neil C (2016) Weapons of math destruction: how big data increases inequality and threatens democracy. Crown
Ong JH (2019) Ethics of artificial intelligence recruitment systems at the United States Secret Service. https://jscholarship.library.jhu.edu/handle/1774.2/61791
Peña A, Serna I, Morales A, Fierrez J (2020) Bias in multimodal AI: testbed for fair automatic recruitment. In: Conitzer V, Hadfield G, Vallor S (eds) Proceedings of the IEEE/CVF conference on computer vision and pattern recognition workshops. Association for Computing Machinery
Prince AE, Schwarcz D (2019) Proxy discrimination in the age of artificial intelligence and big data. Iowa Law Rev 105:1257
Raghavan M, Barocas S, Kleinberg J, Levy K (2020) Mitigating bias in algorithmic hiring: evaluating claims and practices. In: Conitzer V, Hadfield G, Vallor S (eds) Proceedings of the 2020 conference on fairness, accountability, and transparency. Association for Computing Machinery
Raso FA, Hilligoss H, Krishnamurthy V, Bavitz C, Kim L (2018). Artificial intelligence & human rights: opportunities & risks. Available at: SSRN3259344 (2018-6)
Raub M (2018) Bots, bias and big data: artificial intelligence, algorithmic bias and disparate impact liability in hiring practices. Ark Law Rev 71:529
Raveendra P, Satish Y, Singh P (2020) Changing landscape of recruitment industry: a study on the impact of artificial intelligence on eliminating hiring bias from recruitment and selection process. J Comput Theor Nanosci 17(9):4404–4407
Article CAS Google Scholar
Ruwanpura KN (2008) Multiple identities, multiple-discrimination: a critical review. Fem Econ 14(3):77–105
Samuelson PA (1952) Spatial price equilibrium and linear programming. Am Econ Rev 42(3):283–303
Shaw J (2019) Artificial intelligence and ethics. Perspect: Policy Pract High Educ 30, 1–11
Shin D, Park YJJCIHB (2019) Role of fairness, accountability, and transparency in algorithmic affordance. Perspect: Policy Pract High Educ 98, 277–284
Smith B, Shum H (2018). The future computed. Microsoft
Tilcsik A (2021) Statistical discrimination and the rationalization of stereotypes. Am Sociol Rev 86(1):93–122
Timmermans S, Tavory I (2012) Theory construction in qualitative research: from grounded theory to abductive analysis. Sociol Theory 30(3):167–186. https://doi.org/10.1177/0735275112457914
Upadhyay AK, Khandelwal K (2018) Applying artificial intelligence: implications for recruitment. Strateg HR Rev 17(5):255–258
van Esch P, Black JS, Ferolie J (2019) Marketing AI recruitment: the next phase in job application and selection. Comput Hum Behav 90:215–222. https://doi.org/10.1016/j.chb.2018.09.009
Xie X, Ma L, Juefei-Xu F, Chen H, Xue M, Li B, … See S (2018) Deephunter: Hunting deep neural network defects via coverage-guided fuzzing. Available at: arXiv preprint arXiv:.01266
Yang J, Im M, Choi S, Kim J, Ko DH (2021) Artificial intelligence-based hiring: an exploratory study of hiring market reactions. Japan Labor Issues 5(32):41–55
Yarger L, Payton FC, Neupane B (2019) Algorithmic equity in the hiring of underrepresented IT job candidates. Online Inf Rev 44(2):383–395
Yarger L, Smith C, Nedd A (2023) 11. We cannot build equitable artificial intelligence hiring systems without the inclusion of minoritized technology workers. In: Conitzer V, Hadfield G, Vallor S (eds) Handbook of gender and technology: environment, identity, individual. p. 200. Association for Computing Machinery
Zhang J, Chen Z (2023) Exploring human resource management digital transformation in the Digital Age. J Knowl Econ. https://doi.org/10.1007/s13132-023-01214-y
Zixun L (2020) From sexism to unfair hiring, how can AI treat people fairly? https://baijiahao.baidu.com/s?id=1662393382040525886&wfr=spider&for=pc
Zuiderveen Borgesius FJ (2020) Strengthening legal protection against discrimination by algorithms and artificial intelligence. Int J Hum Rights 24(10):1572–1593
Download references
Author information
Authors and affiliations.
College of Economics and Management, Nanjing University of Aeronautics and Astronautics, Nanjing, China
Zhisheng Chen
You can also search for this author in PubMed Google Scholar
Contributions
ZSC conceived, wrote, and approved the manuscript.
Corresponding author
Correspondence to Zhisheng Chen .
Ethics declarations
Competing interests.
The author declares no competing interests.
Ethical approval
Approval was obtained from the ethics committee of the University NUAA. The procedures used in this study adhere to the tenets of the Declaration of Helsinki.
Informed consent
Each participant in this study willingly provided informed permission after being fully told of the study’s purpose, its methods, its participants’ rights, and any possible dangers. They were therefore assured of their comprehension and consent.
Additional information
Publisher’s note Springer Nature remains neutral with regard to jurisdictional claims in published maps and institutional affiliations.
Supplementary information
Source of data and analysis, rights and permissions.
Open Access This article is licensed under a Creative Commons Attribution 4.0 International License, which permits use, sharing, adaptation, distribution and reproduction in any medium or format, as long as you give appropriate credit to the original author(s) and the source, provide a link to the Creative Commons license, and indicate if changes were made. The images or other third party material in this article are included in the article’s Creative Commons license, unless indicated otherwise in a credit line to the material. If material is not included in the article’s Creative Commons license and your intended use is not permitted by statutory regulation or exceeds the permitted use, you will need to obtain permission directly from the copyright holder. To view a copy of this license, visit http://creativecommons.org/licenses/by/4.0/ .
Reprints and permissions
About this article
Cite this article.
Chen, Z. Ethics and discrimination in artificial intelligence-enabled recruitment practices. Humanit Soc Sci Commun 10 , 567 (2023). https://doi.org/10.1057/s41599-023-02079-x
Download citation
Received : 18 February 2023
Accepted : 29 August 2023
Published : 13 September 2023
DOI : https://doi.org/10.1057/s41599-023-02079-x
Share this article
Anyone you share the following link with will be able to read this content:
Sorry, a shareable link is not currently available for this article.
Provided by the Springer Nature SharedIt content-sharing initiative
This article is cited by
Strong and weak alignment of large language models with human values.
- Mehdi Khamassi
- Marceau Nahon
- Raja Chatila
Scientific Reports (2024)
How AI hype impacts the LGBTQ + community
- Dawn McAra-Hunter
AI and Ethics (2024)
The Promise and Challenges of AI Integration in Ovarian Cancer Screenings
- Sierra Silverwood
- Margo Harrison
Reproductive Sciences (2024)
Quick links
- Explore articles by subject
- Guide to authors
- Editorial policies

- DOI: 10.48206/kceba.2023.7.2.137
- Corpus ID: 258086258
A Study on the Performances of AI Recruitment System: A Case Study on Domestic and Abroad Companies
- Seung-Cheul Son , Ju-Yong Oh
- Published in The Korean Career… 30 March 2023
- Business, Computer Science
- The Korean Career, Entrepreneurship & Business Association
2 Citations
Use of artificial intelligence in hr recruitment and selection in start-up companies, collaborative intelligence achieved from ai-enabled recruitment: a case study of posco in south korea, related papers.
Showing 1 through 3 of 0 Related Papers
Use of Artificial Intelligence (AI) in Recruitment and Selection
Proceedings of the Internatioal Conference on Applications of Machine Intelligence and Data Analytics (ICAMIDA 2022)
10 Pages Posted: 19 May 2023
Abhijeet Thakur
Sri Balaji University Pune; Christ University
Punamkumar Hinge
Christ University Banglore
Vikas Adhegaonkar
Date Written: May 19, 2023
The purpose of this paper is to examine the use of artificial intelligence (AI) in recruitment & selection. Multiple case studies of AI tools used for recruitment and selection have been used. Organizations can increase recruiting and selection efficiency and have access to a wider candidate pool by implementing AI in HRM. Subjective factors like nepotism and favouritism are less likely to be used in the hiring and selection of personnel as a result of the implementation of AI in HRM. The implementation of AI in HRM may also have a favourable effect on employee growth, retention, and effective use of time. AI is a developing field of study. With real-world experience, most HRM apps have not accumulated enough machine learning capabilities. Some of these are not supported by science. As a result, only a small part of the population is now impacted by AI in HRM. The research investigates how AI can broaden the pool of candidates. It also improves our knowledge of how AI-based HRM tools might lessen biases in candidate selection, which is very crucial. It also explores a number of ways in which AI aids in the training, retention, and efficient use of workers.
Keywords: AI, Augmented Intelligence, Autonomous AI, Chatbots
Suggested Citation: Suggested Citation
Abhijeet Thakur (Contact Author)
Sri balaji university pune ( email ).
Tathawade International Business Pune, 411057 India 09823583222 (Phone)
Christ University ( email )
Bangalore Pune India
Christ University Banglore ( email )
Do you have a job opening that you would like to promote on ssrn, paper statistics, related ejournals, artificial intelligence ejournal.
Subscribe to this fee journal for more curated articles on this topic
Libraries & Information Technology eJournal
Building an efficient and effective recruitment process is especially relevant in tight or competitive job markets. According to the U.S. Chamber of Commerce (link resides outside ibm.com), there were more than 10 million job openings in the spring of 2023, but only 5.7 million unemployed workers in the U.S. That puts workers at an advantage, allowing them to negotiate for higher pay, better benefits and conditions that create a more favorable work-life balance.
To achieve hiring goals, companies are reevaluating their recruitment processes—identifying inefficiencies and opportunities where artificial intelligence (AI) and automation can make the processes more attractive for candidates and employees.
Recruitment is the process of attracting and hiring qualified candidates to fill roles within an organization. This step-by-step workflow is comprehensive—from identifying needed skills to onboarding a new employee. The recruitment process is ultimately designed to make hiring more efficient, cost-effective, scalable and aligned with company goals and legal requirements.
In addition to filling roles and meeting labor needs, today’s recruitment strategies aim to provide a positive candidate experience. Human resources (HR) teams do this by highlighting the best of the company’s culture and using technology to make the application process more efficient. Within an environment that favors the job seeker, data and AI can give companies a competitive advantage by providing a better understanding of the type of workers they need and how to better attract them.
Building a better hiring process begins with defining a recruitment strategy. Here are some of the key stages businesses frequently include:
- Assess your hiring needs: The first step in an effective recruitment process is understanding what the organization needs. Companies will identify skill gaps, key vacancies and areas where understaffing is affecting service and performance. This includes reviewing all open positions, how existing employees are filling that need and how a new hire can best complement the team.
- Decide on a recruitment approach: Will you hire internally, use a recruiter or bring on a consulting team to steer hiring at your organization? When creating a recruitment plan, organizations often put together a hiring team of stakeholders that lead interactions with the job seekers.
- Responsibilities
- Minimum qualifications
- Whether it is onsite/hybrid/remote
- Post the job: At this stage, it’s important to let people, especially those within your industry, know about the opening. This could include posting the position internally, to a job board, via social media, etc. The goal here is to target the people most likely to be top candidates to increase the quality of the talent pool and more quickly identify top candidates.
- Review applications and resumes: Whether manually or using an applicant tracking system (ATS), your company will have to review applicants and whittle the pool down to the top candidates. Organizations often consider not just skills, but how various candidates align with company goals and diversity, equity and inclusion (DEI) metrics. Predictive AI tools can be used to create an ideal candidate profile you can compare to top candidates, further targeting those who are the best fit.
- Perform phone screening: Once top-tier candidates have been identified, schedule phone conversations to get a better sense of their experience and qualifications. This can be handled by HR staff or members of the recruitment team.
- Conduct interviews: After selecting the top two or three candidates, organizations can schedule in-person or video interviews to assess the right talent. Members of the recruitment team or those who will directly manage the hire should be present at the job interview.
- Select a candidate: The various stakeholders involved in the interview process should be involved in final candidate selection. This step can include vetting the job candidate by checking references and conducting a background check.
- Make a job offer: Next, send an offer letter, including essential details about the role, start date, conditions of employment, work hours and compensation.
- Begin onboarding: This includes training and providing tools and technology to do the job, as well as ensuring the new hire meets fellow staff. One-on-one onboarding can be time-consuming, so many companies are turning to automation and AI tools to more efficiently provision systems for employees and assist with training.
- Review, revise or repost: Using an ATS platform and analytics, review how long it takes to fill positions, the number of applicants and other data to adjust the process to make it more efficient. If at any point the top candidate refuses the offer, this can be where you understand what went wrong, make offers to other candidates, or adjust the job posting for another round of interviews.
Let’s rethink the recruiting process . If you were to design your recruiting process for a new company where would you apply AI and automation to find and get the best talent?
Whether the goal is to reduce the cost and time it takes to hire new employees or simply create a more diverse workplace, a well-designed recruitment process has many advantages, including the following:
- Lowering hiring costs: A successful talent acquisition strategy streamlines the hiring process, making it faster and more cost-effective. By targeting top talent in a proactive way, it increases the likelihood they will apply and the company will get the needed competencies to operate effectively.
- Attracting top talent: A 2021 survey by Robert Half (link resides outside ibm.com) found 62% of professionals lose interest in a job if they don’t hear back from the employer within two weeks. Having an efficient recruitment process improves the candidate’s experience and ensures you don’t leave top talent feeling they’re being strung along.
- Increasing HR productivity: Less time spent on the selection process gives the HR team more time to invest on training, employee development and company culture initiatives.
- Ensuring skills match business goals: Companies can improve the quality of the job description, increase the likelihood of selecting the right candidate and help ensure retention when they put in the work to fully understand the skills they need and business goals the position must work toward. Analytics and AI are valuable tools here, showing companies the skills gaps they may have missed.
- Meeting compliance and internal requirements: A recruitment strategy ensures best practices are being followed and regulatory requirements are being met—from protecting applicant data to equal opportunity and DEI initiatives.
- Addressing problems in the process faster: When you have a comprehensive approach to recruitment based on collecting and analyzing data, it’s easier to see successes and misses and eventually make better hiring decisions.
Developing and fine-tuning a recruitment process is an ongoing task, particularly in changing job markets. These are some ways organizations can improve their ability to efficiently attract talent:
- Leveraging brand reputation: Top talent is generally drawn to companies that invest in employee experience and maintain a positive employer brand. When talking to potential candidates, provide details about worker retention, employee satisfaction and community service initiatives, if appropriate, to highlight workplace benefits.
- Creating incentives: Consider what benefits can be offered above base salaries, such as signing bonuses. In addition, it’s a great idea to set up employee referral programs. Decide how and when these incentives should be used and whether HR or hiring managers own this decision.
- Outsourcing or using consultants: Decide whether hiring done internally is more effective than through third-party recruiters and consultants . This may be an organization-wide choice or situational, based on specific positions.
- Prioritizing internal or external candidates: Decide whether to prioritize hiring from within the organization or to look outside to bring in new perspectives.
- Targeting job postings: Conduct research into the best methods and platforms to use to reach the best candidates. This can include online channels like LinkedIn or industry-specific outlets.
- Using advanced tools: Determine the role technologies like automation and AI will play in recruitment, candidate selection and onboarding. Consider how these tools can help reduce bias, increase efficiency and improve employee experience.
Technology and data can help optimize the recruitment process by making key candidate information readily available during the hiring process and streamlining workflows. Commonly used tools include the following:
- An applicant tracking system (ATS): An ATS provides a wide range of capabilities for tracking candidate data and automating hands-on tasks, from posting job listings to filtering resumes to finding the most qualified applicants.
- Comprehensive data analytics: A data analytics system can show HR teams how many people applied for a job, how many people were interviewed and where the best candidates came from. Taking those insights and analyzing the process with each hire will help improve the overall recruitment process.
Other emerging tools are powered by AI to provide new capabilities and deeper insights. The following are some key tools:
- Machine learning (ML): The best way to improve a company’s recruitment process is to assess what is working and what’s not. However, many HR departments lack the time and resources to run analytics and assess historic hiring data. Here, machine learning can be invaluable, providing insights on recruitment data across applications to empower better decision-making.
- AI-based automation: Automating the onboarding process has saved HR departments countless hours of manual work in recent years, making the process more efficient. AI can help make the onboarding process more personalized. Incoming team members are guided through onboarding with tools powered by natural language processing and adaptive AI.
- Predictive AI: AI is being used today to not only create ideal candidate profiles and assess future talent needs but also to provide deeper insights into employee performance and better guide equitable, industry-based decisions on compensation.
Candidate recruiting involves endless back and forth with hiring managers, manual tracking and spreadsheets, and the use of multiple tools across different systems during the hiring process. You can streamline the process—reducing the number of steps and tools—by using IBM watsonx Orchestrate to intelligently automate tasks, such as creating and posting job requirements faster. Orchestrate integrates with the top tools you use every day, including candidate sourcing and application tracking software.
Get conversational access to the information and automations you need to create and post jobs faster
Academia.edu no longer supports Internet Explorer.
To browse Academia.edu and the wider internet faster and more securely, please take a few seconds to upgrade your browser .
Enter the email address you signed up with and we'll email you a reset link.
- We're Hiring!
- Help Center
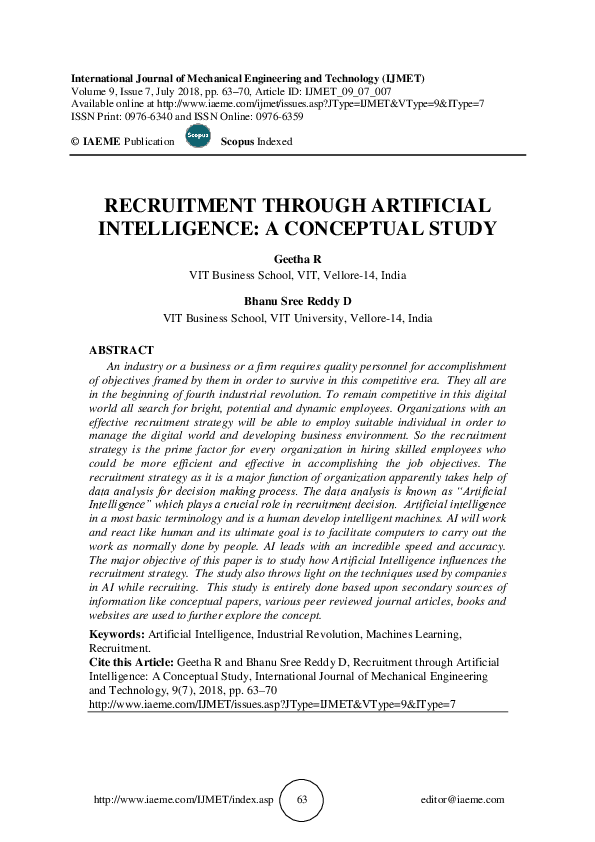
RECRUITMENT THROUGH ARTIFICIAL INTELLIGENCE: A CONCEPTUAL STUDY

An industry or a business or a firm requires quality personnel for accomplishment of objectives framed by them in order to survive in this competitive era. They all are in the beginning of fourth industrial revolution. To remain competitive in this digital world all search for bright, potential and dynamic employees. Organizations with an effective recruitment strategy will be able to employ suitable individual in order to manage the digital world and developing business environment. So the recruitment strategy is the prime factor for every organization in hiring skilled employees who could be more efficient and effective in accomplishing the job objectives. The recruitment strategy as it is a major function of organization apparently takes help of data analysis for decision making process. The data analysis is known as " Artificial Intelligence " which plays a crucial role in recruitment decision. Artificial intelligence in a most basic terminology and is a human develop intelligent machines. AI will work and react like human and its ultimate goal is to facilitate computers to carry out the work as normally done by people. AI leads with an incredible speed and accuracy. The major objective of this paper is to study how Artificial Intelligence influences the recruitment strategy. The study also throws light on the techniques used by companies in AI while recruiting. This study is entirely done based upon secondary sources of information like conceptual papers, various peer reviewed journal articles, books and websites are used to further explore the concept.
Related Papers
IAEME PUBLICATION
IAEME Publication
Recently applicant information services and interview-assistance services based on big data and AI technology are distributed rapidly worldwide to introduce an interview system that secures efficiency and fairness in the job interview market. Accordingly, this study presents an AI-based interview system developed based on deep-learning technology in which more than 100,000 evaluation data sets were derived from 400,000 interview image data sets. The resulting AI interview system has been applied to enterprises with a reliability of 0.88 Pearson score. Particularly, applying this system to 5 major public enterprises in Korea is presented in this paper. It turned out that the level of satisfaction with fairness and efficiency was as high as 85% in such aspects as evaluation processes, job fitness, and organization fitness. As the applicable range of AI-based solutions is expanding to the general area of personnel management with its time and cost efficiency, as well as reliability and fairness recognized, the deep learning-based job interview solution proposed by the present study needs to be applied widely to written examinations and personality and aptitude tests.
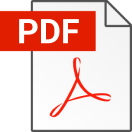
With the increase in global business activities, many companies expanding their business to overseas markets, Human Resource Management (HRM) is needed to make sure that they hire and retain well-performed employees. For a long time, companies/organizations have had big problems in getting accurate professionals to do the right work and training. The aim of this study is to design an automatic job satisfaction system using an optimized neural network approach. Initially, preprocessing is applied to the data to convert string data in terms of numeric data for fast computation purposes, and we change the name of "sales" by "Name of Department and "salary" by "low, medium, and high." The data analysis is performed based on three different factors, such as the number of employees in each department, the number of employees according to the salary range (low, medium, and high), and the number of employees according to the salary range and department. After this, we find out essential features (Satisfaction level and Last evaluation, Number of projects, Average monthly hours, Older employees with more than 10 years in the company), and then determine the correlation between these factors. Now, the Genetic Algorithm (GA) is applied as an optimization approach to enhance the quality of features. These optimal features are fed as input data to Artificial Neural Network (ANN), which is used for the prediction of employee’s satisfaction level. At last, to show the effectiveness of the proposed work, a comparison between proposed GA with the ANN approach with the traditional GA with the K-means approach has been presented.
IAEME PUBLCAITON
In the onset of 21st century, every day we witness change and up gradation of skills in masses. Human resources today lay stress on aligning human resource management with and organizations overall business goals and objectives. Human resources today combine itself with Artificial Intelligence to eliminate repetitive tasks, accelerate the search for talent, reduce employee attrition and improve employee engagement Human resource management is the most important component for a business or an organization to be successful. Human resource management role is to recruit, coordinate and train people in such a strategic way so as to get the maximum advantage from their work related activities. The human resource professionals are type of middleman in a business system who advises the managers and the management on issues related to assigning employees different responsibilities in an organization so that effective utilization of resources can be there. In an organization employees are often shifted from one department to another taking into consideration their skill factor. Over the past 3 decades there has been a tremendous change in Human Resource Management under the influence of new technology. HR processes have changed the way in which how companies collect, store, use and circulate information about employees. The process of recruitment has seen an exhaustive change , gone are the days when job boards and print media was used , electronic recruiting is the new trend in technology leading to qualified, diverse and motivated applicant job pool. With the help of e-recruitment HR gets more variety of employees to choose from. More than 90% of the large companies today are using more than one form of technology to advertise jobs. This current mentioned trend gives birth to a more advanced technology which helps us select the best suited applicant from the variety gathered with the help of e-recruitment i.e Artificial intelligence.
International Journal of Mechanical Engineering and Technology (IJMET)
Nishad Nawaz
Purpose-The aim of the paper to review the Robotic process automation in the recruitment process and its practical implications. This paper discussed possible applications for the recruitment industry caused due to the adoption of Robotic process automation in the recruitment process. Design/methodology/approach-This paper is structured by the independent academician, who have reviewed of the latest articles, research papers, reports and other relevant literature and synthesized their views in the process of presenting the paper. Findings-This paper offers technology development in the field of hiring industry. The RPA for managing the hiring process and also discussed possible applications and usage of RPA in the recruitment industry. Originality/value-The paper explains the role of technology and impact of RPA on the recruitment industry as well as clients in the Industry and the highlighted Inputs for successful integration and possible applications for recruitment industry. Article classification-Viewpoint.
karamath ateeq
Automation of industry and business practices termed as Industry 4.0(Fourth Industrial revolution) has led to the production of the smart machine. Information systems and applications used for business processes are now integrated with data analytics. The job description of employees is changing to suit the industry needs. Innovations in algorithms for decision making make AI (Artificial Intelligence) a useful recruitment tool for implementing Human resource management (HRM) strategy. The recruitment process has been a cognitive human ability. This has been taken over by machines which act as virtual assistants in providing recruitment solutions technically.HR personnel is now empowered to implement strategic business plans developed by the top management. In this paper, AI technologies, and the use of AI platforms to select employees with the required skill set to match management’s business strategy is focused on. The paper also highlights Industry 4.0 and technology trends that h...
Artificial intelligence can be used to efficiently automate diagnostics, analytics, logging, administering and decision making in the healthcare industry which is facing a human resource crunch in the present times. Artificial intelligence though offers very attractive solution for automation and cyber physical systems; but comes with technical, legal and most importantly ethical concerns. In this work, we discuss the challenges associated with incorporation of artificial intelligence in human resources management. We suggest a layered framework to adopt AI based tools and techniques for improving healthcare services. We also discuss the roadmap to evolve into a smart healthcare industry with clear separation of tasks between cyber physical systems and the human resource. We conclude this work by discussing the possible future directions in the area.
Journal of Critical Reviews
Dr. Ramar Veluchamy
To study the effectiveness of AI in eliminating the conscious and unconscious biases within the recruitment process. Artificial Intelligence (AI), the foremost debated and foretold technological advancement, has taken management over varied functions within the field of science, engineering, and business. HR departments have focused on a single, unsophisticated scheme for the last decade that would help end-to-end recruiting workflows, from applicant sourcing to onboarding a new employee. Will artificial intelligence be able to eliminate conscious and unconscious biases in the recruitment and selection process? The exact area of eliminating biases for choosing the eligible candidates during the recruitment process has not yet been sufficiently explored. The views are collected from HR managers and their colleagues to solve the research problem.
Talent acquisition and recruitment are the undergoing processes that also essential for the organization, but it also created challenges for the company to leverage the social network, aggressively market their employment brand and recruit employee every day. In order to ensure itself successfully, it is essential for the company to constantly attract the new talent and recruit the Talent that is already in the plan. In respect to this, the traditional staffing team replaced by the strategic talent acquisition function by making focus on building on employment brand, Sourcing people in a new place with the help of social media tool, creating an opportunity for the internal candidates and leveraging the use network of referral by making relationship within the company.
The mecanum wheel is a direction. circumference. Each roller have an axis of rotation at 45° to the plane of the wheel and at 45° to a line through the centre of the roller parallel to the axis of rotation of the wheel. A platform. By alternating wheels with left and right handed rollers, in such a way that each wheel applies force roughly at right angles to the wheelbase diagonally, the vehicle is st develop a mecanum wheel based robot platform for industrial applications which is equipped with the Infrared (IR) sensor to detect the obstacle around it and move accordingly and also to such type of robot platform is used in the warehouse automation. ABSTRACT The mecanum wheel is a direction. It is a conventional wheel with a series of rollers attached to its circumference. Each roller have an axis of rotation at 45° to the plane of the wheel and at 45° to a line through the centre of the roller parallel to the axis of rotation of the wheel. A typical configuration is the four platform. By alternating wheels with left and right handed rollers, in such a way that each wheel applies force roughly at right angles to the wheelbase diagonally, the vehicle is stable and can be made to move in any direction. The present work is to develop a mecanum wheel based robot platform for industrial applications which is equipped with the Infrared (IR) sensor to detect the obstacle around it and move accordingly and also to such type of robot platform is used in the warehouse automation. The mecanum wheel is a It is a conventional wheel with a series of rollers attached to its circumference. Each roller have an axis of rotation at 45° to the plane of the wheel and at 45° to a line through the centre of the roller parallel to the axis of rotation of typical configuration is the four platform. By alternating wheels with left and right handed rollers, in such a way that each wheel applies force roughly at right angles to the wheelbase diagonally, the able and can be made to move in any direction. The present work is to develop a mecanum wheel based robot platform for industrial applications which is equipped with the Infrared (IR) sensor to detect the obstacle around it and move accordingly and also to transfer the material from one place to another place. Mostly such type of robot platform is used in the warehouse automation. The mecanum wheel is a wheel which can move a vehicle or robot platform in any It is a conventional wheel with a series of rollers attached to its circumference. Each roller have an axis of rotation at 45° to the plane of the wheel and at 45° to a line through the centre of the roller parallel to the axis of rotation of typical configuration is the four platform. By alternating wheels with left and right handed rollers, in such a way that each wheel applies force roughly at right angles to the wheelbase diagonally, the able and can be made to move in any direction. The present work is to develop a mecanum wheel based robot platform for industrial applications which is equipped with the Infrared (IR) sensor to detect the obstacle around it and move transfer the material from one place to another place. Mostly such type of robot platform is used in the warehouse automation. which can move a vehicle or robot platform in any It is a conventional wheel with a series of rollers attached to its circumference. Each roller have an axis of rotation at 45° to the plane of the wheel and at 45° to a line through the centre of the roller parallel to the axis of rotation of typical configuration is the four-wheeled multi platform. By alternating wheels with left and right handed rollers, in such a way that each wheel applies force roughly at right angles to the wheelbase diagonally, the able and can be made to move in any direction. The present work is to develop a mecanum wheel based robot platform for industrial applications which is equipped with the Infrared (IR) sensor to detect the obstacle around it and move transfer the material from one place to another place. Mostly such type of robot platform is used in the warehouse automation. which can move a vehicle or robot platform in any It is a conventional wheel with a series of rollers attached to its circumference. Each roller have an axis of rotation at 45° to the plane of the wheel and at 45° to a line through the centre of the roller parallel to the axis of rotation of wheeled multi platform. By alternating wheels with left and right handed rollers, in such a way that each wheel applies force roughly at right angles to the wheelbase diagonally, the able and can be made to move in any direction. The present work is to develop a mecanum wheel based robot platform for industrial applications which is equipped with the Infrared (IR) sensor to detect the obstacle around it and move transfer the material from one place to another place. Mostly such type of robot platform is used in the warehouse automation. which can move a vehicle or robot platform in any It is a conventional wheel with a series of rollers attached to its circumference. Each roller have an axis of rotation at 45° to the plane of the wheel and at 45° to a line through the centre of the roller parallel to the axis of rotation of wheeled multi-directional mobile robot platform. By alternating wheels with left and right handed rollers, in such a way that each wheel applies force roughly at right angles to the wheelbase diagonally, the able and can be made to move in any direction. The present work is to develop a mecanum wheel based robot platform for industrial applications which is equipped with the Infrared (IR) sensor to detect the obstacle around it and move transfer the material from one place to another place. Mostly such type of robot platform is used in the warehouse automation. which can move a vehicle or robot platform in any It is a conventional wheel with a series of rollers attached to its circumference. Each roller have an axis of rotation at 45° to the plane of the wheel and at 45° to a line through the centre of the roller parallel to the axis of rotation of directional mobile robot platform. By alternating wheels with left and right handed rollers, in such a way that each wheel applies force roughly at right angles to the wheelbase diagonally, the able and can be made to move in any direction. The present work is to develop a mecanum wheel based robot platform for industrial applications which is equipped with the Infrared (IR) sensor to detect the obstacle around it and move transfer the material from one place to another place. Mostly which can move a vehicle or robot platform in any It is a conventional wheel with a series of rollers attached to its circumference. Each roller have an axis of rotation at 45° to the plane of the wheel and at 45° to a line through the centre of the roller parallel to the axis of rotation of directional mobile robot platform. By alternating wheels with left and right handed rollers, in such a way that each wheel applies force roughly at right angles to the wheelbase diagonally, the able and can be made to move in any direction. The present work is to develop a mecanum wheel based robot platform for industrial applications which is equipped with the Infrared (IR) sensor to detect the obstacle around it and move transfer the material from one place to another place. Mostly Mecanum wheel, mobile robot, robot platform, Warehouse, Automation.
krishna raj
Loading Preview
Sorry, preview is currently unavailable. You can download the paper by clicking the button above.
RELATED PAPERS
Iaeme Publication
International Journal of Management
Assist. Prof. (Dr.) Jafaar Fahad A.Rida A.Rida
Akram Marwan
IAEME Publication , HEZEKIAH FALOLA
IAEME Publications
RELATED TOPICS
- We're Hiring!
- Help Center
- Find new research papers in:
- Health Sciences
- Earth Sciences
- Cognitive Science
- Mathematics
- Computer Science
- Academia ©2024

How Artificial Intelligence Assists Recruiters

Recruit, retain and remember your people
Simplify your talent journey and make confident people-focused decisions with Xref. Find out why the organisations you trust, choose Xref.

Remember top talent with an Exit Survey
Reduce attrition, improve retention, build corporate memory to improve organisational metrics with an Xref Exit Survey.
Retain and engage your talent for positive change
Give your people a voice with a tailored Xref Engage survey.
Retain your people and make meaningful change
Increase retention and reduce turnover with quick employee feedback from an Xref Pulse Survey.
Try Xref Reference for free today
Get started with referencing in Xref today for free. No credit card required.
AI-driven recruitment solutions enhance the hiring process
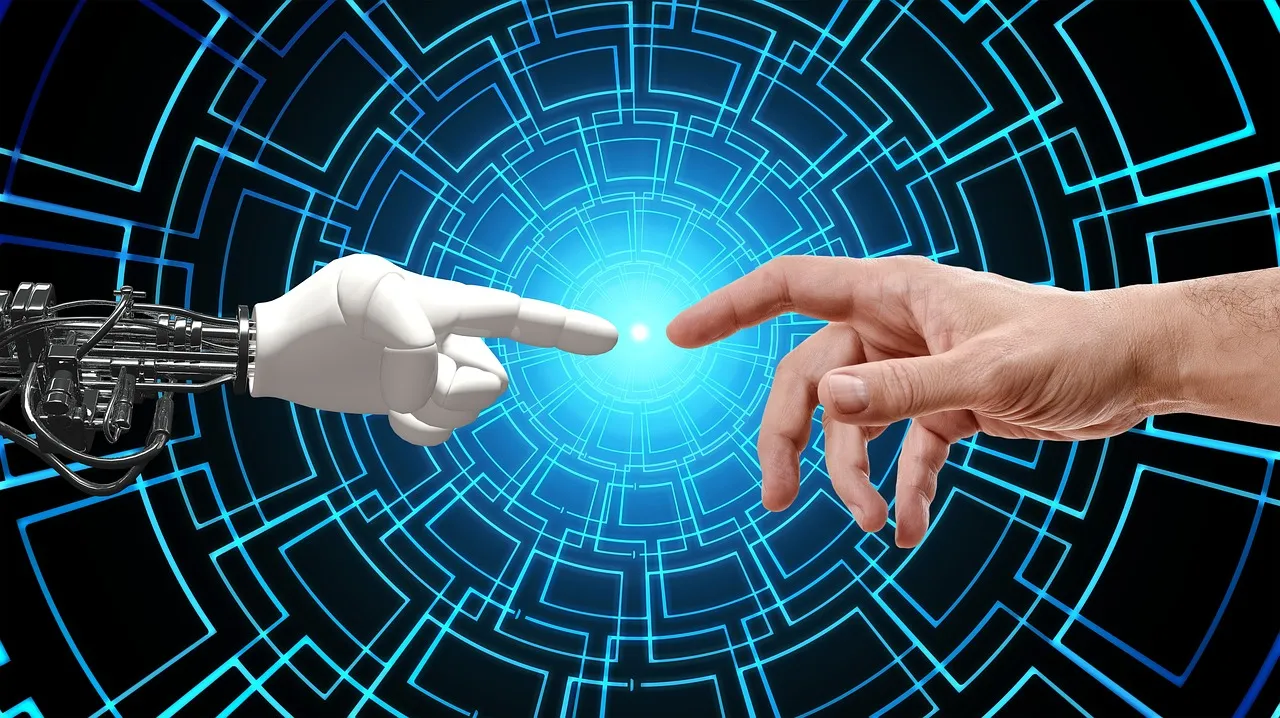
Ensuring the right talent ends up in the right role is challenging in today’s market.
For organisations, competition and candidate demands are high. Fast, confident decisions are needed but identifying a pool of appropriate candidates and taking them through the recruitment process can be slow, time-consuming and costly. Improving the efficiency of talent sourcing and selection is becoming increasingly critical.
The traditional hiring process can also be a difficult experience for candidates. A lack of transparency means it’s unclear whether it’s conducted fairly and consistently for all, or whether their chance of securing a role comes down to luck and timing.
Hired1st provides the data needed to help people make the right decisions about their career, and for companies to focus on the best suited talent for their jobs.
Rethinking Artificial Intelligence
All too often, AI is positioned as the technology that will threaten our jobs in the future. However, on the contrary, we believe it will ultimately help people perform better.
AI is a positive addition to the HR industry and can offer professionals the opportunity to improve the way they work and make their position even more valuable, as opposed to threatening it.
At the heart of the Hired1st concept was the drive to use technology to add efficiency and accuracy to the recruitment process, and ensure every candidate is treated fairly and equally. We wanted to deliver an AI tool that would help recruiters and anyone hiring talent to instantly identify the best candidates, and spend time focusing on the best suited first.
Four ways that Hired1st AI makes this possible
1. saving the recruiter time.
Hired1st is committed to streamlining the CV screening process by:
- Ranking candidates instantly in order of suitability, enabling the recruiter to spend more time on the right candidates.
- Offering objectivity and enabling recruiters to make a human decision based on the data presented.
- Providing an instant pool of talent so hirers can spend more time interviewing, testing and really getting to know candidates.
Our aim is to:
- Eliminate time spent reading through CV’s that are not relevant to the job.
- Give recruiter time back to personally engage with and assess the best talent first.
- Help ensure that suitable candidates are not missed with too many CV’s being received.
2. Treating every CV the same
Hired1st offers a fair and accurate assessment, by:
- Summarising each CV in terms of experience, periods of employment, titles and Jobs. It is not based on word count, but the quality of years performing at roles and learning skills.
- Analysing each CV against a job role in the same way and ranking them based on the candidates that objectively score the highest for the role.
Our aim is to ensure that:
- Each CV is given the same level of consideration, regardless of when the CV is submitted.
- There is no unconscious discrimination about a CV.
The AI-driven platform reads and understands CVs in the same way a recruiter would, and will continue to learn. It is more than a keyword search, understanding career progression, key qualifications, length and timing of experience, and industry focus.
3. Efficiently finding talent using an automated database
With Hired1st, users are given the opportunity to hire from a much larger talent pool, as:
- Every CV in the database is automatically matched against future jobs.
- Higher-ranking candidates (those that are added to the green pool) are automatically put forward for relevant roles on an ongoing basis.
- Recruiters can utilise candidates that have previously applied for jobs automatically, which saves time and money by not having to advertise for new candidates.
Often, recruiters don’t have the time to go through old candidate data, and they find it quicker to advertise for candidates. The Hired1st automated process brings old candidates forward, enabling talent to be better utilised.
Our aim is to ensure:
- Organisations can better utilise existing talent before advertising for external people
- Use candidates that have previously engaged with the business first, creating candidate loyalty
- Recruiters spend less time going through details of old candidates
4. Improving Candidate experience
Looking for a job can be a full-time job in itself and often it can be a stressful, repetitive and daunting experience for candidates.
In order to deliver an optimal experience and ensure candidates form the best first impression of an organisation (regardless of whether they secure the role), Hired1st offers simple but effective tools that improve the employer brand, such as:
- Automatically reply to candidates throughout the process and inform them if they have been unsuccessful
- Engaging candidates that have taken the time to apply previously
- Ensure that all candidates receive feedback when they apply for a role
- Assure candidates that if they send a CV into an organisation using Hired1st once, the platform will automatically consider them for any future job
We would encourage HR and recruitment professionals to explore the value provided by AI tools, such as Hired1st, to help people find the best jobs and companies to find the best people.
Recent articles
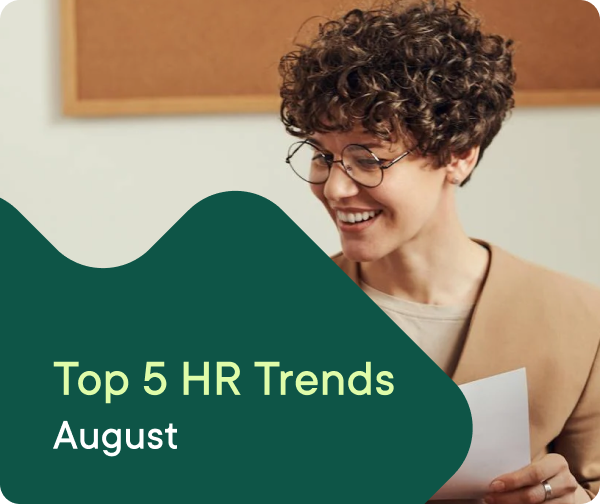
5 Trending HR and Recruitment Topics in August 2024
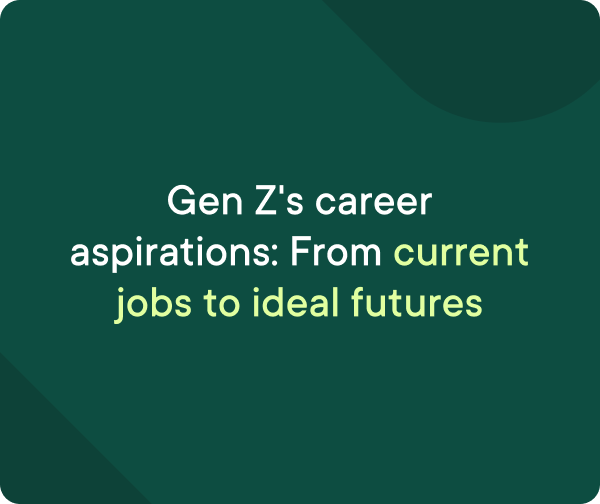
Navigating Gen Z's career aspirations in Australia: From current jobs to ideal futures
.jpg)
Our favourite HR topics from May to July 2024

How Xref achieved a 0% gender gap with more female leaders
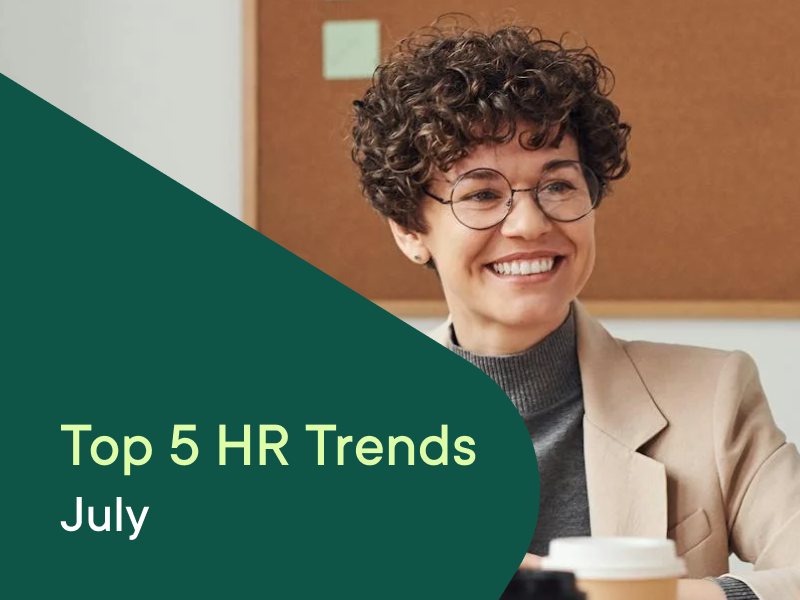
5 Trending HR and Recruitment Topics in July 2024
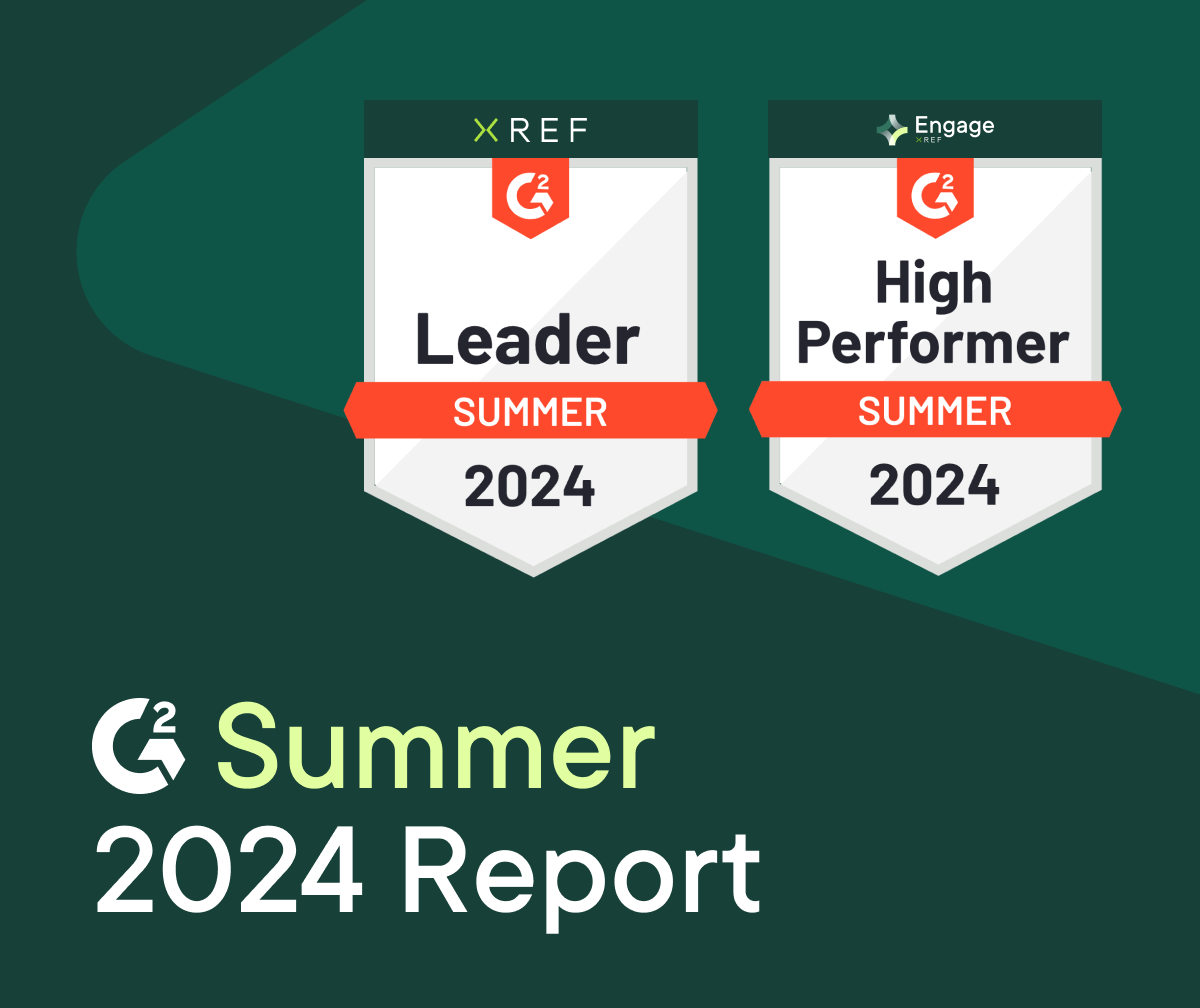
Xref and Xref Engage earn 19 badges in G2 Summer 2024 report
Recruit , retain and remember talent with xref.
Uncover data-driven, actionable insights with automated reference, pulse and exit surveys.
© 2024 Xref
L20, 135 King Street, Sydney NSW 2000

Book a free demo with one of our specialists
More From Forbes
Artificial intelligence in hiring: a tool for recruiters.
- Share to Facebook
- Share to Twitter
- Share to Linkedin
A philanthropist and the CEO of RChilli , Vinay Johar has been listed among TAtech's Top 100 HR Tech Thought Leaders.
A career in recruitment is the essence of finding a needle in a haystack. While there are plenty of reasons why this is true, a few include increased employee demand in particular segments, a company’s economic stats going haywire and a surplus of candidates hunting for the same job.
Lately, numerous talent acquisition professionals are reaping the benefits of AI-driven technologies. In fact, automated resume screening software has become an integral part of many recruiting systems globally.
According to the data from Predictive Hire, nearly 55% of companies are investing in recruitment automation and believe that it’ll enhance efficiency and enable data-driven judgments. For instance, a resume parser, a technology I work with extensively, helps screen resumes and extract candidate data. For the recruiters who are still in limbo about whether or not to go for augmented AI, I’ve lined up a few benefits that can be helpful as well as some best practices.
Carrying a passion for bringing innovative solutions to the HR Tech industry, I spend most of my time with enterprises, mentors and thought leaders to understand bottlenecks in the recruitment process. That’s why I understand how error-prone recruitment can be.
Understanding the vital role automation plays in the HR industry and how well it caters to the challenges, there are certain solutions that can make these automated tools more affordable and accurate.
Time-Saving
Recruiters are quite busy. Hence, the more the otherwise complicated tasks become automated, the better their productivity. AI-driven recruitment tools, for instance, help HR leaders fill open positions speedily by auto-filling and populating the resume data fields. Recruiters only have to upload and parse the resumes compared to the otherwise lengthy process when they had to scan each candidate’s information manually. This saves a lot of their valuable time. This time-saving aspect requires a strong foundation in how you set up the automated tool. Make sure everything is set up properly and to your specifications in order to save time in the long run.
Unbiased Recruitment
A study released by the economists at the University of Chicago and the University of California, Berkeley points to the rising discrimination among the teams in the large U.S.-based companies. Most recruiters have integrated resume parsers in their technology stack to negate biases while hiring. This has helped them infiltrate their workforce with diversity instead of homogeneity.
Ensuring Your Tool Helps In Unbiased Hiring
The AI tool is designed to oversee the resume layout and the type. Having the leverage to select essential parameters like skills, education, qualification and experience helps improve the quality of hire. When set up correctly, the knowledge and skills of the employee will matter rather than other personal attributes. To ensure unbiased hiring when using these tools, make sure to carefully select the fields of your parser and remove any data that could create bias. You can disable things like the name of a candidate or the name of their school from showing up. This can help your recruiters select the skills that they would otherwise overlook.
Ultimately, blind resume screening is led by automation in the backend. The parser, for instance, helps the hiring manager select essential fields over the non-essential ones, which further improves the quality of hires and leads to less bias.
An Improved Candidate Experience
A positive candidate experience is not just a cliché; the comfort and ease in applying to a job matter almost as much as the after-process, including interviewing and hiring. According to Talent Board’s 2021 candidate experience benchmark research report, conducted on nearly 200,000 candidates, the candidate resentment in North America rose from 8% in 2020 to 14% in 2021 .
Encouraging An Enhanced Candidate Experience
Recruitment has become a two-way road where candidates’ expectations are often just as important as that of the recruiters’. Automating the recruitment process not just helps the candidates upload their resumes in a single click, but they can also expect to be called for the next round within a day.
A candidate judges the company the moment they apply for the job. The single-click application functionality in the parser indeed speeds up the process.
You can judge whether a recruitment tool is helping by the decrease in the number of candidate drop-off rates and an overall strengthened brand image. When the call-back time decreases, hiring potential candidates becomes faster. Expediting the time to fill out the application and the time to hire the candidate increases the cost-saving metrics of a company.
A recruiter knows it best—a vacant post is a pricey proposition. To see your tool’s efficacy, compare the caliber of candidates you receive as well as the number who finish the process before and after employing the tool.
I have heard of many hiring managers losing potential candidates to their competitors because the latter shared their resumes on different email IDs. Catering to this dilemma, make sure to use a one-time configuration setup that can help HR personnel access candidate data and fetch information from multiple inboxes.
Automation can never replace human intelligence. But, there’s also no arguing that the accuracy and dexterity it brings to the process are unmatched. When synchronized with automation, augmentation only makes the talent search more efficient. Make sure to properly set up and utilize the tools available to you for optimal success.
Forbes Business Council is the foremost growth and networking organization for business owners and leaders. Do I qualify?
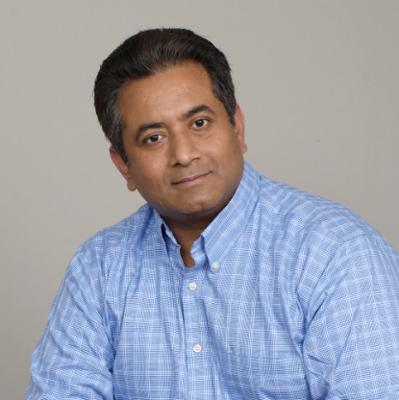
- Editorial Standards
- Reprints & Permissions
- Digital Marketing
- Facebook Marketing
- Instagram Marketing
- Ecommerce Marketing
- Content Marketing
- Data Science Certification
- Machine Learning
- Artificial Intelligence
- Data Analytics
- Graphic Design
- Adobe Illustrator
- Web Designing
- UX UI Design
- Interior Design
- Front End Development
- Back End Development Courses
- Business Analytics
- Entrepreneurship
- Supply Chain
- Financial Modeling
- Corporate Finance
- Project Finance
- Harvard University
- Stanford University
- Yale University
- Princeton University
- Duke University
- UC Berkeley
- Harvard University Executive Programs
- MIT Executive Programs
- Stanford University Executive Programs
- Oxford University Executive Programs
- Cambridge University Executive Programs
- Yale University Executive Programs
- Kellog Executive Programs
- CMU Executive Programs
- 45000+ Free Courses
- Free Certification Courses
- Free DigitalDefynd Certificate
- Free Harvard University Courses
- Free MIT Courses
- Free Excel Courses
- Free Google Courses
- Free Finance Courses
- Free Coding Courses
- Free Digital Marketing Courses
40 Detailed Artificial Intelligence Case Studies [2024]
In this dynamic era of technological advancements, Artificial Intelligence (AI) emerges as a pivotal force, reshaping the way industries operate and charting new courses for business innovation. This article presents an in-depth exploration of 40 diverse and compelling AI case studies from across the globe. Each case study offers a deep dive into the challenges faced by companies, the AI-driven solutions implemented, their substantial impacts, and the valuable lessons learned. From healthcare and finance to transportation and retail, these stories highlight AI’s transformative power in solving complex problems, optimizing processes, and driving growth, offering insightful glimpses into the potential and versatility of AI in shaping our world.
Related: How to Become an AI Thought Leader?
1. IBM Watson Health: Revolutionizing Patient Care with AI
Task/Conflict: The healthcare industry faces challenges in handling vast amounts of patient data, accurately diagnosing diseases, and creating effective treatment plans. IBM Watson Health aimed to address these issues by harnessing AI to process and analyze complex medical information, thus improving the accuracy and efficiency of patient care.
Solution: Utilizing the cognitive computing capabilities of IBM Watson, this solution involves analyzing large volumes of medical records, research papers, and clinical trial data. The system uses natural language processing to understand and process medical jargon, making sense of unstructured data to aid medical professionals in diagnosing and treating patients.
Overall Impact:
- Enhanced accuracy in patient diagnosis and treatment recommendations.
- Significant improvement in personalized healthcare services.
Key Learnings:
- AI can complement medical professionals’ expertise, leading to better healthcare outcomes.
- The integration of AI in healthcare can lead to significant advancements in personalized medicine.
2. Google DeepMind’s AlphaFold: Unraveling the Mysteries of Protein Folding
Task/Conflict: The scientific community has long grappled with the protein folding problem – understanding how a protein’s amino acid sequence determines its 3D structure. Solving this problem is crucial for drug discovery and understanding diseases at a molecular level, yet it remained a formidable challenge due to the complexity of biological structures.
Solution: AlphaFold, developed by Google DeepMind, is an AI model trained on vast datasets of known protein structures. It assesses the distances and angles between amino acids to predict how a protein folds, outperforming existing methods in terms of speed and accuracy. This breakthrough represents a major advancement in computational biology.
- Significant acceleration in drug discovery and disease understanding.
- Set a new benchmark for computational methods in biology.
- AI’s predictive power can solve complex biological problems.
- The application of AI in scientific research can lead to groundbreaking discoveries.
3. Amazon: Transforming Supply Chain Management through AI
Task/Conflict: Managing a global supply chain involves complex challenges like predicting product demand, optimizing inventory levels, and streamlining logistics. Amazon faced the task of efficiently managing its massive inventory while minimizing costs and meeting customer demands promptly.
Solution: Amazon employs sophisticated AI algorithms for predictive inventory management, which forecast product demand based on various factors like buying trends, seasonality, and market changes. This system allows for real-time adjustments, adapting swiftly to changing market dynamics.
- Reduced operational costs through efficient inventory management.
- Improved customer satisfaction with timely deliveries and availability.
- AI can significantly enhance supply chain efficiency and responsiveness.
- Predictive analytics in inventory management leads to reduced waste and cost savings.
4. Tesla’s Autonomous Vehicles: Driving the Future of Transportation
Task/Conflict: The development of autonomous vehicles represents a major technological and safety challenge. Tesla aimed to create self-driving cars that are not only reliable and safe but also capable of navigating complex traffic conditions without human intervention.
Solution: Tesla’s solution involves advanced AI and machine learning algorithms that process data from various sensors and cameras to understand and navigate the driving environment. Continuous learning from real-world driving data allows the system to improve over time, making autonomous driving safer and more efficient.
- Leadership in the autonomous vehicle sector, enhancing road safety.
- Continuous improvements in self-driving technology through AI-driven data analysis.
- Continuous data analysis is key to advancing autonomous driving technologies.
- AI can significantly improve road safety and driving efficiency.
Related: High-Paying AI Career Options
5. Zara: Fashioning the Future with AI in Retail
Task/Conflict: In the fast-paced fashion industry, predicting trends and managing inventory efficiently are critical for success. Zara faced the challenge of quickly adapting to changing fashion trends while avoiding overstock and meeting consumer demand.
Solution: Zara employs AI algorithms to analyze fashion trends, customer preferences, and sales data. The AI system also assists in managing inventory, ensuring that popular items are restocked promptly and that stores are not overburdened with unsold products. This approach optimizes both production and distribution.
- Increased sales and profitability through optimized inventory.
- Enhanced customer satisfaction by aligning products with current trends.
- AI can accurately predict consumer behavior and trends.
- Effective inventory management through AI can significantly impact business success.
6. Netflix: Personalizing Entertainment with AI
Task/Conflict: In the competitive streaming industry, providing a personalized user experience is key to retaining subscribers. Netflix needed to recommend relevant content to each user from its vast library, ensuring that users remained engaged and satisfied.
Solution: Netflix developed an advanced AI-driven recommendation engine that analyzes individual viewing habits, ratings, and preferences. This personalized approach keeps users engaged, as they are more likely to find content that interests them, enhancing their overall viewing experience.
- Increased viewer engagement and longer watch times.
- Higher subscription retention rates due to personalized content.
- Personalized recommendations significantly enhance user experience.
- AI-driven content curation is essential for success in digital entertainment.
7. Airbus: Elevating Aircraft Maintenance with AI
Task/Conflict: Aircraft maintenance is crucial for ensuring flight safety and operational efficiency. Airbus faced the challenge of predicting maintenance needs to prevent equipment failures and reduce downtime, which is critical in the aviation industry.
Solution: Airbus implemented AI algorithms for predictive maintenance, analyzing data from aircraft sensors to identify potential issues before they lead to failures. This system assesses the condition of various components, predicting when maintenance is needed. The solution not only enhances safety but also optimizes maintenance schedules, reducing unnecessary inspections and downtime.
- Decreased maintenance costs and reduced aircraft downtime.
- Improved safety with proactive maintenance measures.
- AI can predict and prevent potential equipment failures.
- Predictive maintenance is essential for operational efficiency and safety in aviation.
8. American Express: Securing Transactions with AI
Task/Conflict: Credit card fraud is a significant issue in the financial sector, leading to substantial losses and undermining customer trust. American Express needed an efficient way to detect and prevent fraudulent transactions in real-time.
Solution: American Express utilizes machine learning models to analyze transaction data. These models identify unusual patterns and behaviors indicative of fraud. By constant learning from refined data, the system becomes increasingly accurate in detecting fraudulent activities, providing real-time alerts and preventing unauthorized transactions.
- Minimized financial losses due to reduced fraudulent activities.
- Enhanced customer trust and security in financial transactions.
- Machine learning is highly effective in fraud detection.
- Real-time data analysis is crucial for preventing financial fraud.
Related: Is AI a Good Career Option for Women?
9. Stitch Fix: Tailoring the Future of Fashion Retail
Task/Conflict: In the competitive fashion retail industry, providing a personalized shopping experience is key to customer satisfaction and business growth. Stitch Fix aimed to offer customized clothing selections to each customer, based on their unique preferences and style.
Solution: Stitch Fix uses AI and algorithms analyze customer feedback, style preferences, and purchase history to recommend clothing items. This personalized approach is complemented by human stylists, ensuring that each customer receives a tailored selection that aligns with their individual style.
- Increased customer satisfaction through personalized styling services.
- Business growth driven by a unique, AI-enhanced shopping experience.
- AI combined with human judgment can create highly effective personalization.
- Tailoring customer experiences using AI leads to increased loyalty and business success.
10. Baidu: Breaking Language Barriers with Voice Recognition
Task/Conflict: Voice recognition technology faces the challenge of accurately understanding and processing speech in various languages and accents. Baidu aimed to enhance its voice recognition capabilities to provide more accurate and user-friendly interactions in multiple languages.
Solution: Baidu employs deep learning algorithms for voice and speech recognition, training its system on a diverse range of languages and dialects. This approach allows for more accurate recognition of speech patterns, enabling the technology to understand and respond to voice commands more effectively. The system continuously improves as it processes more voice data, making technology more accessible to users worldwide.
- Enhanced user interaction with technology in multiple languages.
- Reduced language barriers in voice-activated services and devices.
- AI can effectively bridge language gaps in technology.
- Continuous learning from diverse data sets is key to improving voice recognition.
11. JP Morgan: Revolutionizing Legal Document Analysis with AI
Task/Conflict: Analyzing legal documents, such as contracts, is a time-consuming and error-prone process. JP Morgan sought to streamline this process, reducing the time and effort required while increasing accuracy.
Solution: JP Morgan implemented an AI-powered tool, COIN (Contract Intelligence), to analyze legal documents quickly and accurately. COIN uses NLP to interpret and extract relevant information from contracts, significantly reducing the time required for document review.
- Dramatic reduction in time required for legal document analysis.
- Increased accuracy and reduced human error in contract interpretation.
- AI can efficiently handle large volumes of data, offering speed and accuracy.
- Automation in legal processes can significantly enhance operational efficiency.
12. Microsoft: AI for Accessibility
Task/Conflict: People with disabilities often face challenges in accessing technology. Microsoft aimed to create AI-driven tools to enhance accessibility, especially for individuals with visual, hearing, or cognitive impairments.
Solution: Microsoft developed a range of AI-powered tools including applications for voice recognition, visual assistance, and cognitive support, making technology more accessible and user-friendly. For instance, Seeing AI, an app developed by Microsoft, helps visually impaired users to understand their surroundings by describing people, texts, and objects.
- Improved accessibility and independence for people with disabilities.
- Creation of more inclusive technology solutions.
- AI can significantly contribute to making technology accessible for all.
- Developing inclusive technology is essential for societal progress.
Related: How to get an Internship in AI?
13. Alibaba’s City Brain: Revolutionizing Urban Traffic Management
Task/Conflict: Urban traffic congestion is a major challenge in many cities, leading to inefficiencies and environmental concerns. Alibaba’s City Brain project aimed to address this issue by using AI to optimize traffic flow and improve public transportation in urban areas.
Solution: City Brain uses AI to analyze real-time data from traffic cameras, sensors, and GPS systems. It processes this information to predict traffic patterns and optimize traffic light timing, reducing congestion. The system also provides data-driven insights for urban planning and emergency response coordination, enhancing overall city management.
- Significant reduction in traffic congestion and improved urban transportation.
- Enhanced efficiency in city management and emergency response.
- AI can effectively manage complex urban systems.
- Data-driven solutions are key to improving urban living conditions.
14. Deep 6 AI: Accelerating Clinical Trials with Artificial Intelligence
Task/Conflict: Recruiting suitable patients for clinical trials is often a slow and cumbersome process, hindering medical research. Deep 6 AI sought to accelerate this process by quickly identifying eligible participants from a vast pool of patient data.
Solution: Deep 6 AI employs AI to sift through extensive medical records, identifying potential trial participants based on specific criteria. The system analyzes structured and unstructured data, including doctor’s notes and diagnostic reports, to find matches for clinical trials. This approach significantly speeds up the recruitment process, enabling faster trial completions and advancements in medical research.
- Quicker recruitment for clinical trials, leading to faster research progress.
- Enhanced efficiency in medical research and development.
- AI can streamline the patient selection process for clinical trials.
- Efficient recruitment is crucial for the advancement of medical research.
15. NVIDIA: Revolutionizing Gaming Graphics with AI
Task/Conflict: Enhancing the realism and performance of gaming graphics is a continuous challenge in the gaming industry. NVIDIA aimed to revolutionize gaming visuals by leveraging AI to create more realistic and immersive gaming experiences.
Solution: NVIDIA’s AI-driven graphic processing technologies, such as ray tracing and deep learning super sampling (DLSS), provide highly realistic and detailed graphics. These technologies use AI to render images more efficiently, improving game performance without compromising on visual quality. This innovation sets new standards in gaming graphics, making games more lifelike and engaging.
- Elevated gaming experiences with state-of-the-art graphics.
- Set new industry standards for graphic realism and performance.
- AI can significantly enhance creative industries, like gaming.
- Balancing performance and visual quality is key to gaming innovation.
16. Palantir: Mastering Data Integration and Analysis with AI
Task/Conflict: Integrating and analyzing large-scale, diverse datasets is a complex task, essential for informed decision-making in various sectors. Palantir Technologies faced the challenge of making sense of vast amounts of data to provide actionable insights for businesses and governments.
Solution: Palantir developed AI-powered platforms that integrate data from multiple sources, providing a comprehensive view of complex systems. These platforms use machine learning to analyze data, uncover patterns, and predict outcomes, assisting in strategic decision-making. This solution enables users to make informed decisions in real-time, based on a holistic understanding of their data.
- Enhanced decision-making capabilities in complex environments.
- Greater insights and efficiency in data analysis across sectors.
- Effective data integration is crucial for comprehensive analysis.
- AI-driven insights are essential for strategic decision-making.
Related: Surprising AI Facts & Statistics
17. Blue River Technology: Sowing the Seeds of AI in Agriculture
Task/Conflict: The agriculture industry faces challenges in increasing efficiency and sustainability while minimizing environmental impact. Blue River Technology aimed to enhance agricultural practices by using AI to make farming more precise and efficient.
Solution: Blue River Technology developed AI-driven agricultural robots that perform tasks like precise planting and weed control. These robots use ML to identify plants and make real-time decisions, such as applying herbicides only to weeds. This targeted approach reduces chemical usage and promotes sustainable farming practices, leading to better crop yields and environmental conservation.
- Significant reduction in chemical usage in farming.
- Increased crop yields through precision agriculture.
- AI can contribute significantly to sustainable agricultural practices.
- Precision farming is key to balancing productivity and environmental conservation.
18. Salesforce: Enhancing Customer Relationship Management with AI
Task/Conflict: In the realm of customer relationship management (CRM), personalizing interactions and gaining insights into customer behavior are crucial for business success. Salesforce aimed to enhance CRM capabilities by integrating AI to provide personalized customer experiences and actionable insights.
Solution: Salesforce incorporates AI-powered tools into its CRM platform, enabling businesses to personalize customer interactions, automate responses, and predict customer needs. These tools analyze customer data, providing insights that help businesses tailor their strategies and communications. The AI integration not only improves customer engagement but also streamlines sales and marketing efforts.
- Improved customer engagement and satisfaction.
- Increased business growth through tailored marketing and sales strategies.
- AI-driven personalization is key to successful customer relationship management.
- Leveraging AI for data insights can significantly impact business growth.
19. OpenAI: Transforming Natural Language Processing
Task/Conflict: OpenAI aimed to advance NLP by developing models capable of generating coherent and contextually relevant text, opening new possibilities in AI-human interaction.
Solution: OpenAI developed the Generative Pre-trained Transformer (GPT) models, which use deep learning to generate text that closely mimics human language. These models are trained on vast datasets, enabling them to understand context and generate responses in a conversational and coherent manner.
- Pioneered advancements in natural language understanding and generation.
- Expanded the possibilities for AI applications in communication.
- AI’s ability to mimic human language has vast potential applications.
- Advancements in NLP are crucial for improving AI-human interactions.
20. Siemens: Pioneering Industrial Automation with AI
Task/Conflict: Industrial automation seeks to improve productivity and efficiency in manufacturing processes. Siemens faced the challenge of optimizing these processes using AI to reduce downtime and enhance output quality.
Solution: Siemens employs AI-driven solutions for predictive maintenance and process optimization to reduce downtime in industrial settings. Additionally, AI optimizes manufacturing processes, ensuring quality and efficiency.
- Increased productivity and reduced downtime in industrial operations.
- Enhanced quality and efficiency in manufacturing processes.
- AI is a key driver in the advancement of industrial automation.
- Predictive analytics are crucial for maintaining efficiency in manufacturing.
Related: Top Books for Learning AI
21. Ford: Driving Safety Innovation with AI
Task/Conflict: Enhancing automotive safety and providing effective driver assistance systems are critical challenges in the auto industry. Ford aimed to leverage AI to improve vehicle safety features and assist drivers in real-time decision-making.
Solution: Ford integrated AI into its advanced driver assistance systems (ADAS) to provide features like adaptive cruise control, lane-keeping assistance, and collision avoidance. These systems use sensors and cameras to gather data, which AI processes to make split-second decisions that enhance driver safety and vehicle performance.
- Improved safety features in vehicles, minimizing accidents and improving driver confidence.
- Enhanced driving experience with intelligent assistance features.
- AI can highly enhance safety in the automotive industry.
- Real-time data processing and decision-making are essential for effective driver assistance systems.
22. HSBC: Enhancing Banking Security with AI
Task/Conflict: As financial transactions increasingly move online, banks face heightened risks of fraud and cybersecurity threats. HSBC needed to bolster its protective measures to secure user data and prevent scam.
Solution: HSBC employed AI-driven security systems to observe transactions and identify suspicious activities. The AI models analyze patterns in customer behavior and flag anomalies that could indicate fraudulent actions, allowing for immediate intervention. This helps in minimizing the risk of financial losses and protects customer trust.
- Strengthened security measures and reduced incidence of fraud.
- Maintained high levels of customer trust and satisfaction.
- AI is critical in enhancing security in the banking sector.
- Proactive fraud detection can prevent significant financial losses.
23. Unilever: Optimizing Supply Chain with AI
Task/Conflict: Managing a global supply chain involves complexities related to logistics, demand forecasting, and sustainability practices. Unilever sought to enhance its supply chain efficiency while promoting sustainability.
Solution: Unilever implemented AI to optimize its supply chain operations, from raw material sourcing to distribution. AI algorithms analyze data to forecast demand, improve inventory levels, and minimize waste. Additionally, AI helps in selecting sustainable practices and suppliers, aligning with Unilever’s commitment to environmental responsibility.
- Enhanced efficiency and reduced costs in supply chain operations.
- Better sustainability practices, reducing environmental impact.
- AI can highly optimize supply chain management.
- Integrating AI with sustainability initiatives can lead to environmentally responsible operations.
24. Spotify: Personalizing Music Experience with AI
Task/Conflict: In the competitive music streaming industry, providing a personalized listening experience is crucial for user engagement and retention. Spotify needed to tailor music recommendations to individual tastes and preferences.
Solution: Spotify utilizes AI-driven algorithms to analyze user listening habits, preferences, and contextual data to recommend music tracks and playlists. This personalization ensures that users are continually engaged and discover new music that aligns with their tastes, enhancing their overall listening experience.
- Increased customer engagement and time spent on the platform.
- Higher user satisfaction and subscription retention rates.
- Personalized content delivery is key to user retention in digital entertainment.
- AI-driven recommendations significantly enhance user experience.
Related: How can AI be used in Instagram Marketing?
25. Walmart: Revolutionizing Retail with AI
Task/Conflict: Retail giants like Walmart face challenges in inventory management and providing a high-quality customer service experience. Walmart aimed to use AI to optimize these areas and enhance overall operational efficacy.
Solution: Walmart deployed AI technologies across its stores to manage inventory levels effectively and enhance customer service. AI systems predict product demand to optimize stock levels, while AI-driven robots assist in inventory management and customer service, such as guiding customers in stores and handling queries.
- Improved inventory management, reducing overstock and shortages.
- Enhanced customer service experience in stores.
- AI can streamline retail operations significantly.
- Enhanced customer service through AI leads to better customer satisfaction.
26. Roche: Innovating Drug Discovery with AI
Task/Conflict: The pharmaceutical industry faces significant challenges in drug discovery, requiring vast investments of time and resources. Roche aimed to utilize AI to streamline the drug development process and enhance the discovery of new therapeutics.
Solution: Roche implemented AI to analyze medical data and simulate drug interactions, speeding up the drug discovery process. AI models predict the effectiveness of compounds and identify potential candidates for further testing, significantly minimizing the time and cost related with traditional drug development procedures.
- Accelerated drug discovery processes, bringing new treatments to market faster.
- Reduced costs and increased efficiency in pharmaceutical research.
- AI can greatly accelerate the drug discovery process.
- Cost-effective and efficient drug development is possible with AI integration.
27. IKEA: Enhancing Customer Experience with AI
Task/Conflict: In the competitive home furnishings market, enhancing the customer shopping experience is crucial for success. IKEA aimed to use AI to provide innovative design tools and improve customer interaction.
Solution: IKEA introduced AI-powered tools such as virtual reality apps that allow consumers to visualize furniture before buying. These tools help customers make more informed decisions and enhance their shopping experience. Additionally, AI chatbots assist with customer service inquiries, providing timely and effective support.
- Improved customer decision-making and satisfaction with interactive tools.
- Enhanced efficiency in customer service.
- AI can transform the retail experience by providing innovative customer interaction tools.
- Effective customer support through AI can enhance brand loyalty and satisfaction.
28. General Electric: Optimizing Energy Production with AI
Task/Conflict: Managing energy production efficiently while predicting and mitigating potential issues is crucial for energy companies. General Electric (GE) aimed to improve the efficiency and reliability of its energy production facilities using AI.
Solution: GE integrated AI into its energy management systems to enhance power generation and distribution. AI algorithms predict maintenance needs and optimize energy production, ensuring efficient operation and reducing downtime. This predictive maintenance approach saves costs and enhances the reliability of energy production.
- Increased efficiency in energy production and distribution.
- Reduced operational costs and enhanced system reliability.
- Predictive maintenance is crucial for cost-effective and efficient energy management.
- AI can significantly improve the predictability and efficiency of energy production.
Related: Use of AI in Sales
29. L’Oréal: Transforming Beauty with AI
Task/Conflict: Personalization in the beauty industry enhances customer satisfaction and brand loyalty. L’Oréal aimed to personalize beauty products and experiences for its diverse customer base using AI.
Solution: L’Oréal leverages AI to assess consumer data and provide personalized product suggestions. AI-driven tools assess skin types and preferences to recommend the best skincare and makeup products. Additionally, virtual try-on apps powered by AI allow customers to see how products would look before making a purchase.
- Enhanced personalization of beauty products and experiences.
- Increased customer engagement and satisfaction.
- AI can provide highly personalized experiences in the beauty industry.
- Data-driven personalization enhances customer satisfaction and brand loyalty.
30. The Weather Company: AI-Predicting Weather Patterns
Task/Conflict: Accurate weather prediction is vital for planning and safety in various sectors. The Weather Company aimed to enhance the accuracy of weather forecasts and provide timely weather-related information using AI.
Solution: The Weather Company employs AI to analyze data from weather sensors, satellites, and historical weather patterns. AI models improve the accuracy of weather predictions by identifying trends and anomalies. These enhanced forecasts help in better planning and preparedness for weather events, benefiting industries like agriculture, transportation, and public safety.
- Improved accuracy in weather forecasting.
- Better preparedness and planning for adverse weather conditions.
- AI can enhance the precision of meteorological predictions.
- Accurate weather forecasting is crucial for safety and operational planning in multiple sectors.
31. Cisco: Securing Networks with AI
Task/Conflict: As cyber threats evolve and become more sophisticated, maintaining robust network security is crucial for businesses. Cisco aimed to leverage AI to enhance its cybersecurity measures, detecting and responding to threats more efficiently.
Solution: Cisco integrated AI into its cybersecurity framework to analyze network traffic and identify unusual patterns indicative of cyber threats. This AI-driven approach allows for real-time threat detection and automated responses, thus improving the speed and efficacy of security measures.
- Strengthened network security with faster threat detection.
- Reduced manual intervention by automating threat responses.
- AI is essential in modern cybersecurity for real-time threat detection.
- Automating responses can significantly enhance network security protocols.
32. Adidas: AI in Sports Apparel Manufacturing
Task/Conflict: To maintain competitive advantage in the fast-paced sports apparel market, Adidas sought to innovate its manufacturing processes by incorporating AI to improve efficiency and product quality.
Solution: Adidas employed AI-driven robotics and automation technologies in its factories to streamline the production process. These AI systems optimize manufacturing workflows, enhance quality control, and reduce waste by precisely cutting fabrics and assembling materials according to exact specifications.
- Increased production efficacy and reduced waste.
- Enhanced consistency and quality of sports apparel.
- AI-driven automation can revolutionize manufacturing processes.
- Precision and efficiency in production lead to higher product quality and sustainability.
Related: How can AI be used in Disaster Management?
33. KLM Royal Dutch Airlines: AI-Enhanced Customer Service
Task/Conflict: Enhancing the customer service experience in the airline industry is crucial for customer satisfaction and loyalty. KLM aimed to provide immediate and effective assistance to its customers by integrating AI into their service channels.
Solution: KLM introduced an AI-powered chatbot, which provides 24/7 customer service across multiple languages. The chatbot handles inquiries about flight statuses, bookings, and baggage policies, offering quick and accurate responses. This AI solution helps manage customer interactions efficiently, especially during high-volume periods.
- Improved customer service efficiency and responsiveness.
- Increased customer satisfaction through accessible and timely support.
- AI chatbots can highly improve user service in high-demand industries.
- Effective communication through AI leads to better customer engagement and loyalty.
34. Novartis: AI in Drug Formulation
Task/Conflict: The pharmaceutical industry requires rapid development and formulation of new drugs to address emerging health challenges. Novartis aimed to use AI to expedite the drug formulation process, making it faster and more efficient.
Solution: Novartis applied AI to simulate and predict how different formulations might behave, speeding up the lab testing phase. AI algorithms analyze vast amounts of data to predict the stability and efficacy of drug formulations, allowing researchers to focus on the most promising candidates.
- Accelerated drug formulation and reduced time to market.
- Improved efficacy and stability of pharmaceutical products.
- AI can significantly shorten the drug development lifecycle.
- Predictive analytics in pharmaceutical research can lead to more effective treatments.
35. Shell: Optimizing Energy Resources with AI
Task/Conflict: In the energy sector, optimizing exploration and production processes for efficiency and sustainability is crucial. Shell sought to harness AI to enhance its oil and gas operations, making them more efficient and less environmentally impactful.
Solution: Shell implemented AI to analyze geological data and predict drilling outcomes, optimizing resource extraction. AI algorithms also adjust production processes in real time, improving operational proficiency and minimizing waste.
- Improved efficiency and sustainability in energy production.
- Reduced environmental impact through optimized resource management.
- Automation can enhance the effectiveness and sustainability of energy production.
- Real-time data analysis is crucial for optimizing exploration and production.
36. Procter & Gamble: AI in Consumer Goods Production
Task/Conflict: Maintaining operational efficiency and innovating product development are key challenges in the consumer goods industry. Procter & Gamble (P&G) aimed to integrate AI into their operations to enhance these aspects.
Solution: P&G employs AI to optimize its manufacturing processes and predict market trends for product development. AI-driven data analysis helps in managing supply chains and production lines efficiently, while AI in market research informs new product development, aligning with consumer needs.
- Enhanced operational efficacy and minimized production charges.
- Improved product innovation based on consumer data analysis.
- AI is crucial for optimizing manufacturing and supply chain processes.
- Data-driven product development leads to more successful market introductions.
Related: Use of AI in the Navy
37. Disney: Creating Magical Experiences with AI
Task/Conflict: Enhancing visitor experiences in theme parks and resorts is a priority for Disney. They aimed to use AI to create personalized and magical experiences for guests, improving satisfaction and engagement.
Solution: Disney utilizes AI to manage park operations, personalize guest interactions, and enhance entertainment offerings. AI algorithms predict visitor traffic and optimize attractions and staff deployment. Personalized recommendations for rides, shows, and dining options enhance the guest experience by leveraging data from past visits and preferences.
- Enhanced guest satisfaction through personalized experiences.
- Improved operational efficiency in park management.
- AI can transform the entertainment and hospitality businesses by personalizing consumer experiences.
- Efficient management of operations using AI leads to improved customer satisfaction.
38. BMW: Reinventing Mobility with Autonomous Driving
Task/Conflict: The future of mobility heavily relies on the development of safe and efficient autonomous driving technologies. BMW aimed to dominate in this field by incorporating AI into their vehicles.
Solution: BMW is advancing its autonomous driving capabilities through AI, using sophisticated machine learning models to process data from vehicle sensors and external environments. This technology enables vehicles to make intelligent driving decisions, improving safety and passenger experiences.
- Pioneering advancements in autonomous vehicle technology.
- Enhanced safety and user experience in mobility.
- AI is crucial for the development of autonomous driving technologies.
- Safety and reliability are paramount in developing AI-driven vehicles.
39. Mastercard: Innovating Payment Solutions with AI
Task/Conflict: In the digital age, securing online transactions and enhancing payment processing efficiency are critical challenges. Mastercard aimed to leverage AI to address these issues, ensuring secure and seamless payment experiences for users.
Solution: Mastercard integrates AI to monitor transactions in real time, detect fraudulent activities, and enhance the efficiency of payment processing. AI algorithms analyze spending patterns and flag anomalies, while also optimizing authorization processes to reduce false declines and improve user satisfaction.
- Strengthened security and reduced fraud in transactions.
- Improved efficiency and user experience in payment processing.
- AI is necessary for securing and streamlining expense systems.
- Enhanced transaction processing efficiency leads to higher customer satisfaction.
40. AstraZeneca: Revolutionizing Oncology with AI
Task/Conflict: Advancing cancer research and developing effective treatments is a pressing challenge in healthcare. AstraZeneca aimed to utilize AI to revolutionize oncology research, enhancing the development and personalization of cancer treatments.
Solution: AstraZeneca employs AI to analyze genetic data and clinical trial results, identifying potential treatment pathways and personalizing therapies based on individual genetic profiles. This approach accelerates the development of targeted treatments and improves the efficacy of cancer therapies.
- Accelerated innovation and personalized treatment in oncology.
- Better survival chances for cancer patients.
- AI can significantly advance personalized medicine in oncology.
- Data-driven approaches in healthcare lead to better treatment outcomes and innovations.
Related: How can AI be used in Tennis?
Closing Thoughts
These 40 case studies illustrate the transformative power of AI across various industries. By addressing specific challenges and leveraging AI solutions, companies have achieved remarkable outcomes, from enhancing customer experiences to solving complex scientific problems. The key learnings from these cases underscore AI’s potential to revolutionize industries, improve efficiencies, and open up new possibilities for innovation and growth.
- 6 Ways to Pay for an Online Course [2024]
- How to Choose an Online Course? [An Ultimate Checklist] [2024]
Team DigitalDefynd
We help you find the best courses, certifications, and tutorials online. Hundreds of experts come together to handpick these recommendations based on decades of collective experience. So far we have served 4 Million+ satisfied learners and counting.
50 AI Engineer Interview Questions & Answers [2024]

AI in Product Development [5 Case Studies] [2024]

10 ways Netflix uses AI [2024]

7 Hobby Ideas for Artificial Intelligence Engineers [2024]

Career in AI vs IT: Which is Better? [2024]

Role of AI in Cybersecurity [2024]

IMAGES
VIDEO
COMMENTS
Purpose: The aim of this study is to contribute to the understanding of the power of artificial intelligence (AI) in recruitment and to highlight the opportunities and challenges associated with ...
A case study to reveal the strategic implications ... AI in recruitment: an exploratory study into the factors the impact its pace of adoption. Ayşegül Begüm Kerey and Enrico D'Alessandro Over the past few years, the adoption of AI in recruitment has ... Artificial intelligence is a modern technology that enables machines performing ...
This paper set out to consider artificial intelligence (AI) and the impact its growing influence is having within the recruitment industry. This research considered how AI is influencing employers and candidates, specifically throughout the initial stages of the recruitment process. With limited
In this article, we engage critically with the idea of promoting AI technologies 1 in recruitment as tools to eliminate discrimination 2 in decision-making. To this effect, we took a closer look at justifications for introducing AI technologies into organizations which attempt to reduce discrimination during the phase of recruitment called 'personnel selection'. 3 We analyzed a body of ...
A multiple case study is designed to analyze, compare, and combine several technologies dedicated to recruitment: (1) a social network with LinkedIn, (2) a MOOC with Udacity, (3) a serious game called Reveal from L'Oréal, (4) a chatbot called Ari from TextRecruit, and (5) a massive data analysis matching system with Randstad.tech. The ...
Abstract. The proliferation of Artificial intelligence (AI) technologies impacting entire business sectors is also transforming the field of human resources and recruitment. AI-based recruitment tools are changing the way recruitment processes are conducted. However, the perception of AI technology from the candidate's perspective has received ...
1. Introduction. Artificial intelligence (AI) is being used extensively by recruiters in a variety of industries and has now become one of the latest trends in the recruitment industry (Alam et al., 2020; Upadhyay & Khandelwal, 2018; Nugent & Scott-Parker, 2021). AI does not necessarily replace humans, but rather serves as a complement in ...
This study aims to address the research gap on algorithmic discrimination caused by AI-enabled recruitment and explore technical and managerial solutions. The primary research approach used is a ...
This study examines how AI technology is being used in the recruitment process through domestic and international case studies of AI recruitment systems such as Google, Softbank, Unilever, Amazon, Loreal, Samsung, Lotte, SK, KB Kookmin Bank, Hyundai and LG, and seeks to verify the introduction performance of AI recruitment systems, and presents matters to be considered when companies select AI ...
Abstract. This case describes how Unilever Plc, based in London, the United Kingdom, has used artificial intelligence and video-based recruitment to assess job candidates' suitability using AI machine learning algorithms. By 2022, half of job candidates hired at Unilever came through the AI-based recruitment system.
Using artificial intelligence for recruitment may also be challenged by poor data quality and restrictions over the data. This algorithm relies mainly on the data for which the data needs to be
Abstract — Artificial Intelligence (AI) can play a pivotal role in. the firm's recruiting process, fa cilitating excellence. This study. investigates the challenges AI faces in the h iring ...
The primary goal of this research is to investigate candidate attitudes toward artificial intelligence (AI)-supported chatbots as an alternative to traditional recruitment interviews, aiming to understand the potential impact of AI-assisted processes on the employee selection landscape.
AIR 12002613 - Free download as PDF File (.pdf), Text File (.txt) or read online for free. The document discusses an artificial intelligence recruitment solution called AIR. Large organizations that hire large numbers of candidates and have long hiring processes would benefit most from automating recruitment with AIR. The pricing structure would consider factors like the reliability, task ...
AI Recruiting in 2024: The Definitive Guide. Artificial intelligence (AI) is proving its worth to recruitment teams by providing benefits like efficiency, personalization, and data-informed decision making. Eighty-eight percent of companies globally already use AI in some way for HR, according to SHRM.
The purpose of this paper is to examine the use of artificial intelligence (AI) in recruitment & selection. Multiple case studies of AI tools used for recruitment and selection have been used. Organizations can increase recruiting and selection efficiency and have access to a wider candidate pool by implementing AI in HRM.
IBM, AI and the recruitment process. Candidate recruiting involves endless back and forth with hiring managers, manual tracking and spreadsheets, and the use of multiple tools across different systems during the hiring process. You can streamline the process—reducing the number of steps and tools—by using IBM watsonx Orchestrate to ...
The major objective of this paper is to study how Artificial Intelligence influences the recruitment strategy. The study also throws light on the techniques used by companies in AI while recruiting. ... p.91. Holm, A., 2010. The effect of e-recruitment on the recruitment process: Evidence from case studies of three Danish MNCs. In Proceedings ...
Rethinking Artificial Intelligence. All too often, AI is positioned as the technology that will threaten our jobs in the future. However, on the contrary, we believe it will ultimately help people perform better. AI is a positive addition to the HR industry and can offer professionals the opportunity to improve the way they work and make their ...
Unbiased Recruitment. A study released by the economists at the University of Chicago and the University of California, Berkeley points to the rising discrimination among the teams in the large U ...
Hevo Case Study - AIR.pdf - Free download as PDF File (.pdf), Text File (.txt) or read online for free. AI-Recruit is an artificial intelligence software that automates recruitment tasks like scheduling interviews and performing initial candidate screening. The software can schedule chat interviews between candidates and hiring managers based on their availabilities, send calendar invitations ...
In this dynamic era of technological advancements, Artificial Intelligence (AI) emerges as a pivotal force, reshaping the way industries operate and charting new courses for business innovation. This article presents an in-depth exploration of 40 diverse and compelling AI case studies from across the globe.