- Skip to main content
- Skip to primary sidebar
- Skip to footer
- QuestionPro

- Solutions Industries Gaming Automotive Sports and events Education Government Travel & Hospitality Financial Services Healthcare Cannabis Technology Use Case NPS+ Communities Audience Contactless surveys Mobile LivePolls Member Experience GDPR Positive People Science 360 Feedback Surveys
- Resources Blog eBooks Survey Templates Case Studies Training Help center

Home Market Research

Data Analysis in Research: Types & Methods
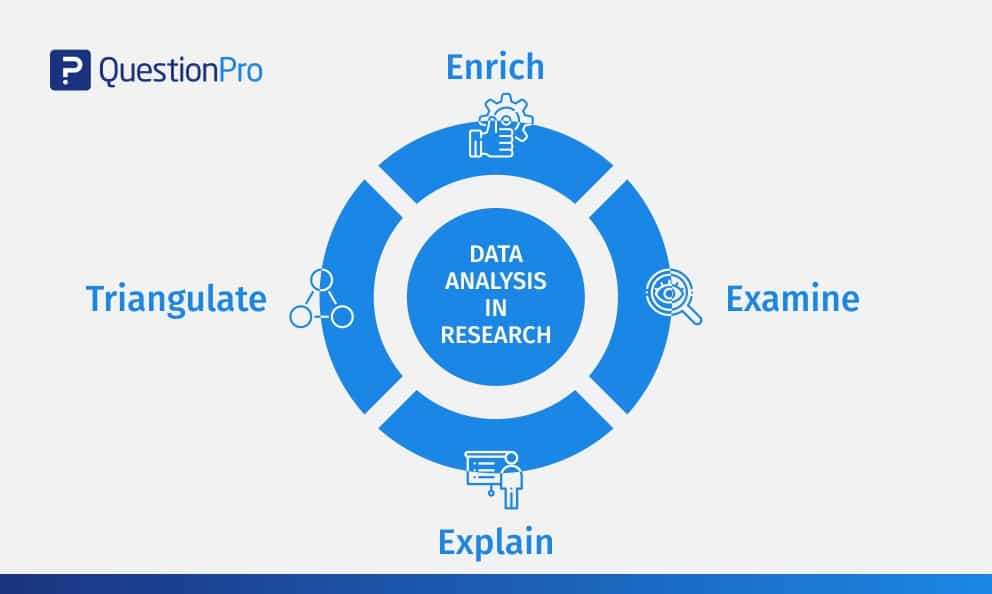
Content Index
Why analyze data in research?
Types of data in research, finding patterns in the qualitative data, methods used for data analysis in qualitative research, preparing data for analysis, methods used for data analysis in quantitative research, considerations in research data analysis, what is data analysis in research.
Definition of research in data analysis: According to LeCompte and Schensul, research data analysis is a process used by researchers to reduce data to a story and interpret it to derive insights. The data analysis process helps reduce a large chunk of data into smaller fragments, which makes sense.
Three essential things occur during the data analysis process — the first is data organization . Summarization and categorization together contribute to becoming the second known method used for data reduction. It helps find patterns and themes in the data for easy identification and linking. The third and last way is data analysis – researchers do it in both top-down and bottom-up fashion.
LEARN ABOUT: Research Process Steps
On the other hand, Marshall and Rossman describe data analysis as a messy, ambiguous, and time-consuming but creative and fascinating process through which a mass of collected data is brought to order, structure and meaning.
We can say that “the data analysis and data interpretation is a process representing the application of deductive and inductive logic to the research and data analysis.”
Researchers rely heavily on data as they have a story to tell or research problems to solve. It starts with a question, and data is nothing but an answer to that question. But, what if there is no question to ask? Well! It is possible to explore data even without a problem – we call it ‘Data Mining’, which often reveals some interesting patterns within the data that are worth exploring.
Irrelevant to the type of data researchers explore, their mission and audiences’ vision guide them to find the patterns to shape the story they want to tell. One of the essential things expected from researchers while analyzing data is to stay open and remain unbiased toward unexpected patterns, expressions, and results. Remember, sometimes, data analysis tells the most unforeseen yet exciting stories that were not expected when initiating data analysis. Therefore, rely on the data you have at hand and enjoy the journey of exploratory research.
Create a Free Account
Every kind of data has a rare quality of describing things after assigning a specific value to it. For analysis, you need to organize these values, processed and presented in a given context, to make it useful. Data can be in different forms; here are the primary data types.
- Qualitative data: When the data presented has words and descriptions, then we call it qualitative data . Although you can observe this data, it is subjective and harder to analyze data in research, especially for comparison. Example: Quality data represents everything describing taste, experience, texture, or an opinion that is considered quality data. This type of data is usually collected through focus groups, personal qualitative interviews , qualitative observation or using open-ended questions in surveys.
- Quantitative data: Any data expressed in numbers of numerical figures are called quantitative data . This type of data can be distinguished into categories, grouped, measured, calculated, or ranked. Example: questions such as age, rank, cost, length, weight, scores, etc. everything comes under this type of data. You can present such data in graphical format, charts, or apply statistical analysis methods to this data. The (Outcomes Measurement Systems) OMS questionnaires in surveys are a significant source of collecting numeric data.
- Categorical data: It is data presented in groups. However, an item included in the categorical data cannot belong to more than one group. Example: A person responding to a survey by telling his living style, marital status, smoking habit, or drinking habit comes under the categorical data. A chi-square test is a standard method used to analyze this data.
Learn More : Examples of Qualitative Data in Education
Data analysis in qualitative research
Data analysis and qualitative data research work a little differently from the numerical data as the quality data is made up of words, descriptions, images, objects, and sometimes symbols. Getting insight from such complicated information is a complicated process. Hence it is typically used for exploratory research and data analysis .
Although there are several ways to find patterns in the textual information, a word-based method is the most relied and widely used global technique for research and data analysis. Notably, the data analysis process in qualitative research is manual. Here the researchers usually read the available data and find repetitive or commonly used words.
For example, while studying data collected from African countries to understand the most pressing issues people face, researchers might find “food” and “hunger” are the most commonly used words and will highlight them for further analysis.
LEARN ABOUT: Level of Analysis
The keyword context is another widely used word-based technique. In this method, the researcher tries to understand the concept by analyzing the context in which the participants use a particular keyword.
For example , researchers conducting research and data analysis for studying the concept of ‘diabetes’ amongst respondents might analyze the context of when and how the respondent has used or referred to the word ‘diabetes.’
The scrutiny-based technique is also one of the highly recommended text analysis methods used to identify a quality data pattern. Compare and contrast is the widely used method under this technique to differentiate how a specific text is similar or different from each other.
For example: To find out the “importance of resident doctor in a company,” the collected data is divided into people who think it is necessary to hire a resident doctor and those who think it is unnecessary. Compare and contrast is the best method that can be used to analyze the polls having single-answer questions types .
Metaphors can be used to reduce the data pile and find patterns in it so that it becomes easier to connect data with theory.
Variable Partitioning is another technique used to split variables so that researchers can find more coherent descriptions and explanations from the enormous data.
LEARN ABOUT: Qualitative Research Questions and Questionnaires
There are several techniques to analyze the data in qualitative research, but here are some commonly used methods,
- Content Analysis: It is widely accepted and the most frequently employed technique for data analysis in research methodology. It can be used to analyze the documented information from text, images, and sometimes from the physical items. It depends on the research questions to predict when and where to use this method.
- Narrative Analysis: This method is used to analyze content gathered from various sources such as personal interviews, field observation, and surveys . The majority of times, stories, or opinions shared by people are focused on finding answers to the research questions.
- Discourse Analysis: Similar to narrative analysis, discourse analysis is used to analyze the interactions with people. Nevertheless, this particular method considers the social context under which or within which the communication between the researcher and respondent takes place. In addition to that, discourse analysis also focuses on the lifestyle and day-to-day environment while deriving any conclusion.
- Grounded Theory: When you want to explain why a particular phenomenon happened, then using grounded theory for analyzing quality data is the best resort. Grounded theory is applied to study data about the host of similar cases occurring in different settings. When researchers are using this method, they might alter explanations or produce new ones until they arrive at some conclusion.
LEARN ABOUT: 12 Best Tools for Researchers
Data analysis in quantitative research
The first stage in research and data analysis is to make it for the analysis so that the nominal data can be converted into something meaningful. Data preparation consists of the below phases.
Phase I: Data Validation
Data validation is done to understand if the collected data sample is per the pre-set standards, or it is a biased data sample again divided into four different stages
- Fraud: To ensure an actual human being records each response to the survey or the questionnaire
- Screening: To make sure each participant or respondent is selected or chosen in compliance with the research criteria
- Procedure: To ensure ethical standards were maintained while collecting the data sample
- Completeness: To ensure that the respondent has answered all the questions in an online survey. Else, the interviewer had asked all the questions devised in the questionnaire.
Phase II: Data Editing
More often, an extensive research data sample comes loaded with errors. Respondents sometimes fill in some fields incorrectly or sometimes skip them accidentally. Data editing is a process wherein the researchers have to confirm that the provided data is free of such errors. They need to conduct necessary checks and outlier checks to edit the raw edit and make it ready for analysis.
Phase III: Data Coding
Out of all three, this is the most critical phase of data preparation associated with grouping and assigning values to the survey responses . If a survey is completed with a 1000 sample size, the researcher will create an age bracket to distinguish the respondents based on their age. Thus, it becomes easier to analyze small data buckets rather than deal with the massive data pile.
LEARN ABOUT: Steps in Qualitative Research
After the data is prepared for analysis, researchers are open to using different research and data analysis methods to derive meaningful insights. For sure, statistical analysis plans are the most favored to analyze numerical data. In statistical analysis, distinguishing between categorical data and numerical data is essential, as categorical data involves distinct categories or labels, while numerical data consists of measurable quantities. The method is again classified into two groups. First, ‘Descriptive Statistics’ used to describe data. Second, ‘Inferential statistics’ that helps in comparing the data .
Descriptive statistics
This method is used to describe the basic features of versatile types of data in research. It presents the data in such a meaningful way that pattern in the data starts making sense. Nevertheless, the descriptive analysis does not go beyond making conclusions. The conclusions are again based on the hypothesis researchers have formulated so far. Here are a few major types of descriptive analysis methods.
Measures of Frequency
- Count, Percent, Frequency
- It is used to denote home often a particular event occurs.
- Researchers use it when they want to showcase how often a response is given.
Measures of Central Tendency
- Mean, Median, Mode
- The method is widely used to demonstrate distribution by various points.
- Researchers use this method when they want to showcase the most commonly or averagely indicated response.
Measures of Dispersion or Variation
- Range, Variance, Standard deviation
- Here the field equals high/low points.
- Variance standard deviation = difference between the observed score and mean
- It is used to identify the spread of scores by stating intervals.
- Researchers use this method to showcase data spread out. It helps them identify the depth until which the data is spread out that it directly affects the mean.
Measures of Position
- Percentile ranks, Quartile ranks
- It relies on standardized scores helping researchers to identify the relationship between different scores.
- It is often used when researchers want to compare scores with the average count.
For quantitative research use of descriptive analysis often give absolute numbers, but the in-depth analysis is never sufficient to demonstrate the rationale behind those numbers. Nevertheless, it is necessary to think of the best method for research and data analysis suiting your survey questionnaire and what story researchers want to tell. For example, the mean is the best way to demonstrate the students’ average scores in schools. It is better to rely on the descriptive statistics when the researchers intend to keep the research or outcome limited to the provided sample without generalizing it. For example, when you want to compare average voting done in two different cities, differential statistics are enough.
Descriptive analysis is also called a ‘univariate analysis’ since it is commonly used to analyze a single variable.
Inferential statistics
Inferential statistics are used to make predictions about a larger population after research and data analysis of the representing population’s collected sample. For example, you can ask some odd 100 audiences at a movie theater if they like the movie they are watching. Researchers then use inferential statistics on the collected sample to reason that about 80-90% of people like the movie.
Here are two significant areas of inferential statistics.
- Estimating parameters: It takes statistics from the sample research data and demonstrates something about the population parameter.
- Hypothesis test: I t’s about sampling research data to answer the survey research questions. For example, researchers might be interested to understand if the new shade of lipstick recently launched is good or not, or if the multivitamin capsules help children to perform better at games.
These are sophisticated analysis methods used to showcase the relationship between different variables instead of describing a single variable. It is often used when researchers want something beyond absolute numbers to understand the relationship between variables.
Here are some of the commonly used methods for data analysis in research.
- Correlation: When researchers are not conducting experimental research or quasi-experimental research wherein the researchers are interested to understand the relationship between two or more variables, they opt for correlational research methods.
- Cross-tabulation: Also called contingency tables, cross-tabulation is used to analyze the relationship between multiple variables. Suppose provided data has age and gender categories presented in rows and columns. A two-dimensional cross-tabulation helps for seamless data analysis and research by showing the number of males and females in each age category.
- Regression analysis: For understanding the strong relationship between two variables, researchers do not look beyond the primary and commonly used regression analysis method, which is also a type of predictive analysis used. In this method, you have an essential factor called the dependent variable. You also have multiple independent variables in regression analysis. You undertake efforts to find out the impact of independent variables on the dependent variable. The values of both independent and dependent variables are assumed as being ascertained in an error-free random manner.
- Frequency tables: The statistical procedure is used for testing the degree to which two or more vary or differ in an experiment. A considerable degree of variation means research findings were significant. In many contexts, ANOVA testing and variance analysis are similar.
- Analysis of variance: The statistical procedure is used for testing the degree to which two or more vary or differ in an experiment. A considerable degree of variation means research findings were significant. In many contexts, ANOVA testing and variance analysis are similar.
- Researchers must have the necessary research skills to analyze and manipulation the data , Getting trained to demonstrate a high standard of research practice. Ideally, researchers must possess more than a basic understanding of the rationale of selecting one statistical method over the other to obtain better data insights.
- Usually, research and data analytics projects differ by scientific discipline; therefore, getting statistical advice at the beginning of analysis helps design a survey questionnaire, select data collection methods, and choose samples.
LEARN ABOUT: Best Data Collection Tools
- The primary aim of data research and analysis is to derive ultimate insights that are unbiased. Any mistake in or keeping a biased mind to collect data, selecting an analysis method, or choosing audience sample il to draw a biased inference.
- Irrelevant to the sophistication used in research data and analysis is enough to rectify the poorly defined objective outcome measurements. It does not matter if the design is at fault or intentions are not clear, but lack of clarity might mislead readers, so avoid the practice.
- The motive behind data analysis in research is to present accurate and reliable data. As far as possible, avoid statistical errors, and find a way to deal with everyday challenges like outliers, missing data, data altering, data mining , or developing graphical representation.
LEARN MORE: Descriptive Research vs Correlational Research The sheer amount of data generated daily is frightening. Especially when data analysis has taken center stage. in 2018. In last year, the total data supply amounted to 2.8 trillion gigabytes. Hence, it is clear that the enterprises willing to survive in the hypercompetitive world must possess an excellent capability to analyze complex research data, derive actionable insights, and adapt to the new market needs.
LEARN ABOUT: Average Order Value
QuestionPro is an online survey platform that empowers organizations in data analysis and research and provides them a medium to collect data by creating appealing surveys.
MORE LIKE THIS
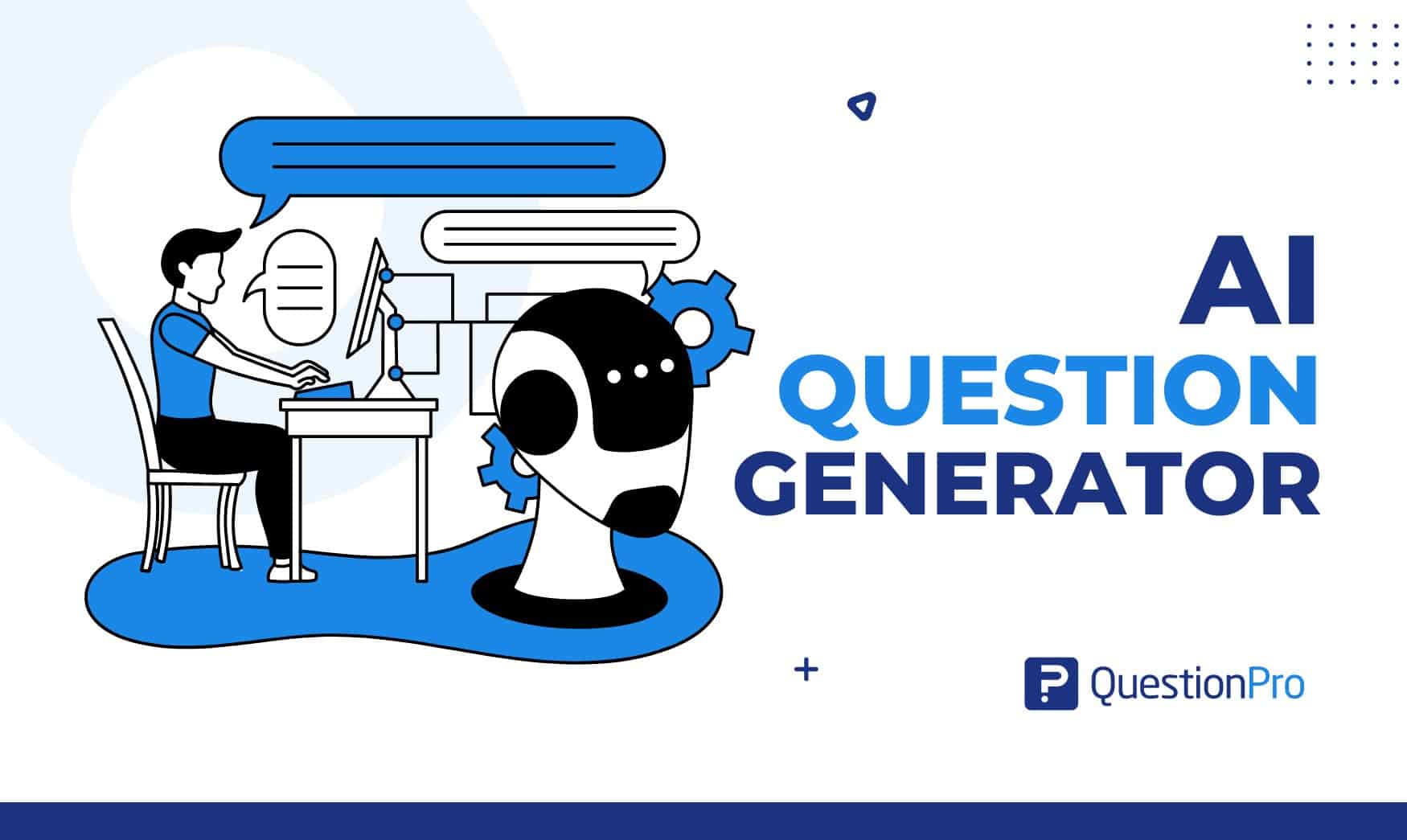
AI Question Generator: Create Easy + Accurate Tests and Surveys
Apr 6, 2024
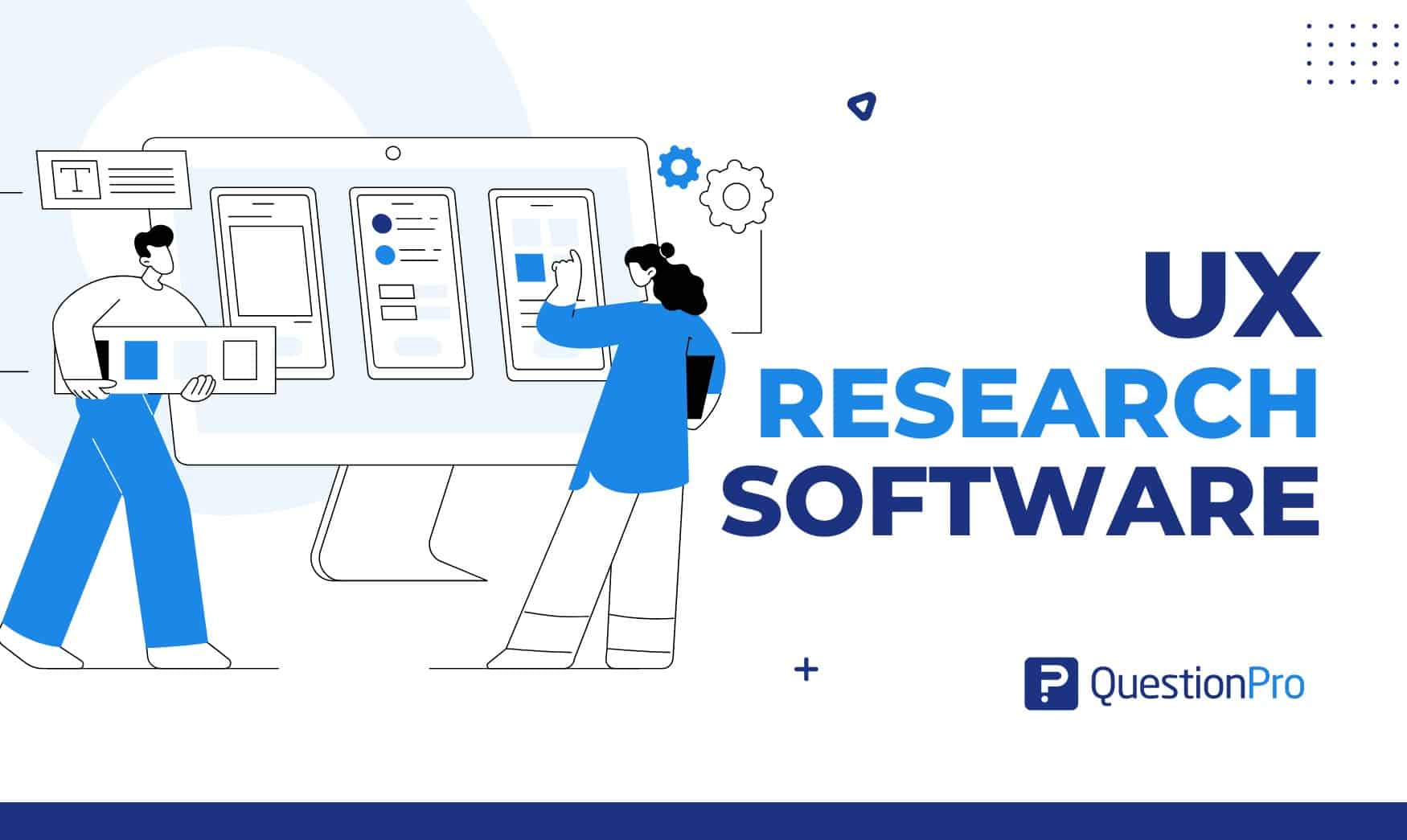
Top 17 UX Research Software for UX Design in 2024
Apr 5, 2024
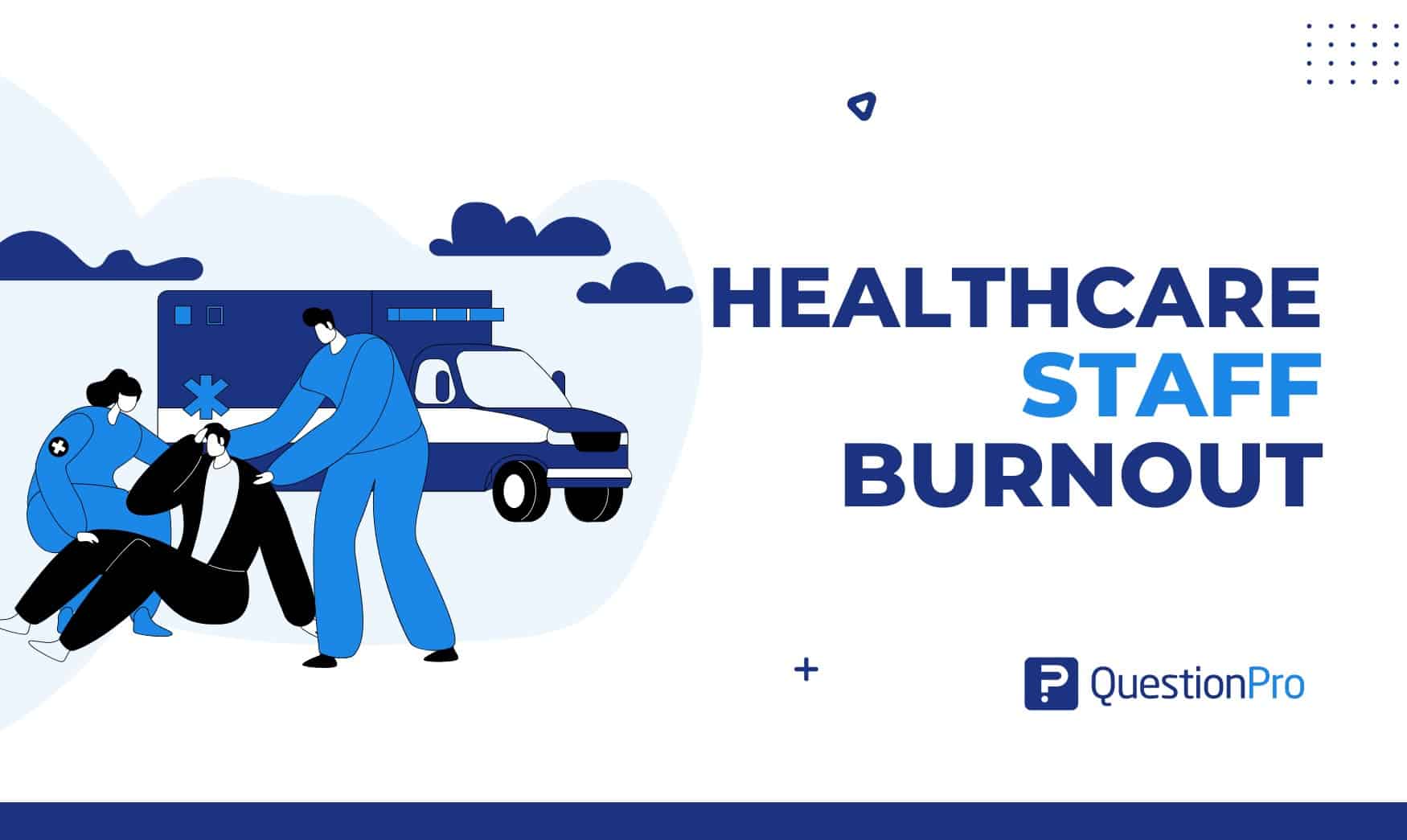
Healthcare Staff Burnout: What it Is + How To Manage It
Apr 4, 2024
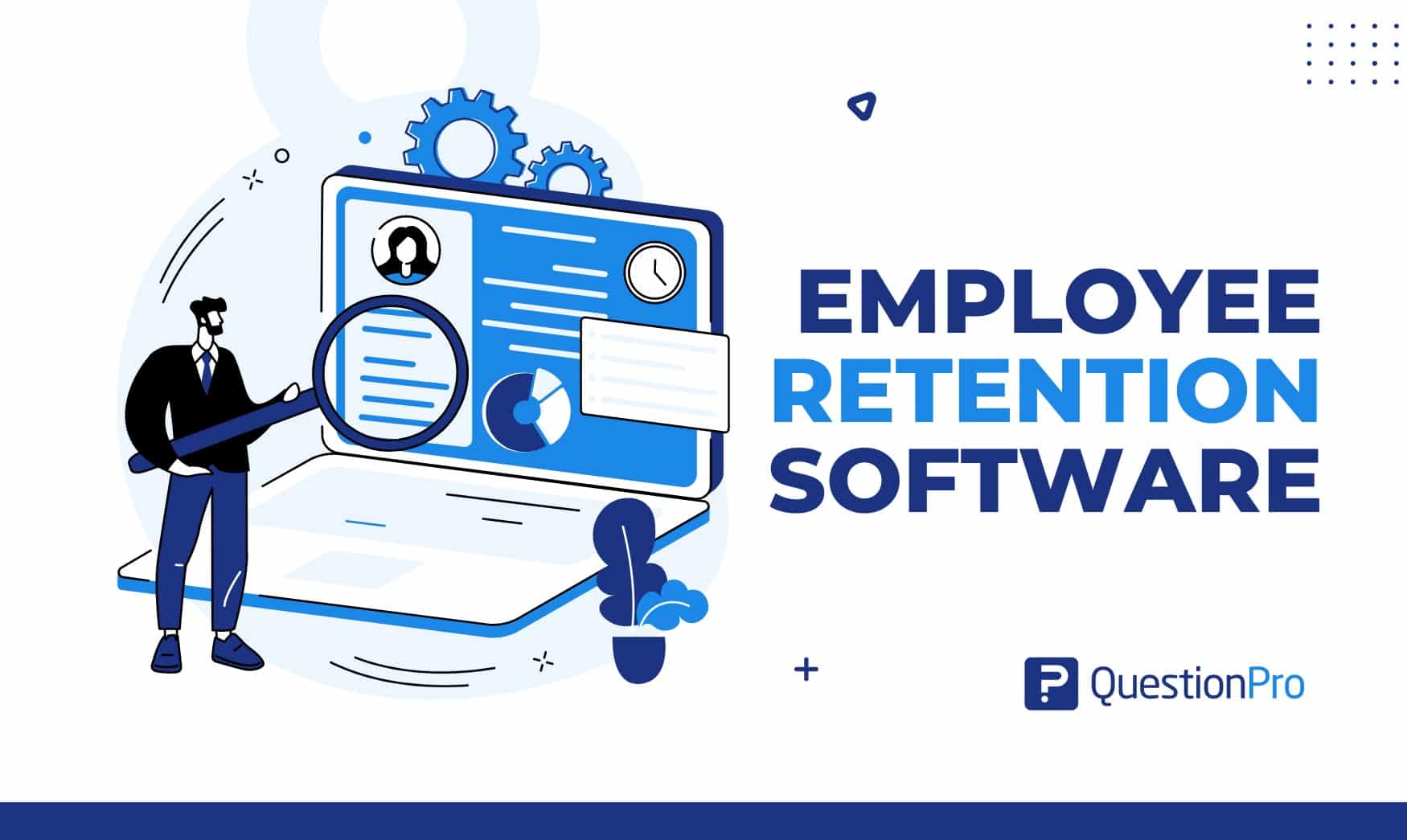
Top 15 Employee Retention Software in 2024
Other categories.
- Academic Research
- Artificial Intelligence
- Assessments
- Brand Awareness
- Case Studies
- Communities
- Consumer Insights
- Customer effort score
- Customer Engagement
- Customer Experience
- Customer Loyalty
- Customer Research
- Customer Satisfaction
- Employee Benefits
- Employee Engagement
- Employee Retention
- Friday Five
- General Data Protection Regulation
- Insights Hub
- Life@QuestionPro
- Market Research
- Mobile diaries
- Mobile Surveys
- New Features
- Online Communities
- Question Types
- Questionnaire
- QuestionPro Products
- Release Notes
- Research Tools and Apps
- Revenue at Risk
- Survey Templates
- Training Tips
- Uncategorized
- Video Learning Series
- What’s Coming Up
- Workforce Intelligence
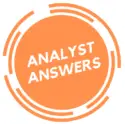
Data & Finance for Work & Life

Data Analysis: Types, Methods & Techniques (a Complete List)
( Updated Version )
While the term sounds intimidating, “data analysis” is nothing more than making sense of information in a table. It consists of filtering, sorting, grouping, and manipulating data tables with basic algebra and statistics.
In fact, you don’t need experience to understand the basics. You have already worked with data extensively in your life, and “analysis” is nothing more than a fancy word for good sense and basic logic.
Over time, people have intuitively categorized the best logical practices for treating data. These categories are what we call today types , methods , and techniques .
This article provides a comprehensive list of types, methods, and techniques, and explains the difference between them.
For a practical intro to data analysis (including types, methods, & techniques), check out our Intro to Data Analysis eBook for free.
Descriptive, Diagnostic, Predictive, & Prescriptive Analysis
If you Google “types of data analysis,” the first few results will explore descriptive , diagnostic , predictive , and prescriptive analysis. Why? Because these names are easy to understand and are used a lot in “the real world.”
Descriptive analysis is an informational method, diagnostic analysis explains “why” a phenomenon occurs, predictive analysis seeks to forecast the result of an action, and prescriptive analysis identifies solutions to a specific problem.
That said, these are only four branches of a larger analytical tree.
Good data analysts know how to position these four types within other analytical methods and tactics, allowing them to leverage strengths and weaknesses in each to uproot the most valuable insights.
Let’s explore the full analytical tree to understand how to appropriately assess and apply these four traditional types.
Tree diagram of Data Analysis Types, Methods, and Techniques
Here’s a picture to visualize the structure and hierarchy of data analysis types, methods, and techniques.
If it’s too small you can view the picture in a new tab . Open it to follow along!

Note: basic descriptive statistics such as mean , median , and mode , as well as standard deviation , are not shown because most people are already familiar with them. In the diagram, they would fall under the “descriptive” analysis type.
Tree Diagram Explained
The highest-level classification of data analysis is quantitative vs qualitative . Quantitative implies numbers while qualitative implies information other than numbers.
Quantitative data analysis then splits into mathematical analysis and artificial intelligence (AI) analysis . Mathematical types then branch into descriptive , diagnostic , predictive , and prescriptive .
Methods falling under mathematical analysis include clustering , classification , forecasting , and optimization . Qualitative data analysis methods include content analysis , narrative analysis , discourse analysis , framework analysis , and/or grounded theory .
Moreover, mathematical techniques include regression , Nïave Bayes , Simple Exponential Smoothing , cohorts , factors , linear discriminants , and more, whereas techniques falling under the AI type include artificial neural networks , decision trees , evolutionary programming , and fuzzy logic . Techniques under qualitative analysis include text analysis , coding , idea pattern analysis , and word frequency .
It’s a lot to remember! Don’t worry, once you understand the relationship and motive behind all these terms, it’ll be like riding a bike.
We’ll move down the list from top to bottom and I encourage you to open the tree diagram above in a new tab so you can follow along .
But first, let’s just address the elephant in the room: what’s the difference between methods and techniques anyway?
Difference between methods and techniques
Though often used interchangeably, methods ands techniques are not the same. By definition, methods are the process by which techniques are applied, and techniques are the practical application of those methods.
For example, consider driving. Methods include staying in your lane, stopping at a red light, and parking in a spot. Techniques include turning the steering wheel, braking, and pushing the gas pedal.
Data sets: observations and fields
It’s important to understand the basic structure of data tables to comprehend the rest of the article. A data set consists of one far-left column containing observations, then a series of columns containing the fields (aka “traits” or “characteristics”) that describe each observations. For example, imagine we want a data table for fruit. It might look like this:
Now let’s turn to types, methods, and techniques. Each heading below consists of a description, relative importance, the nature of data it explores, and the motivation for using it.
Quantitative Analysis
- It accounts for more than 50% of all data analysis and is by far the most widespread and well-known type of data analysis.
- As you have seen, it holds descriptive, diagnostic, predictive, and prescriptive methods, which in turn hold some of the most important techniques available today, such as clustering and forecasting.
- It can be broken down into mathematical and AI analysis.
- Importance : Very high . Quantitative analysis is a must for anyone interesting in becoming or improving as a data analyst.
- Nature of Data: data treated under quantitative analysis is, quite simply, quantitative. It encompasses all numeric data.
- Motive: to extract insights. (Note: we’re at the top of the pyramid, this gets more insightful as we move down.)
Qualitative Analysis
- It accounts for less than 30% of all data analysis and is common in social sciences .
- It can refer to the simple recognition of qualitative elements, which is not analytic in any way, but most often refers to methods that assign numeric values to non-numeric data for analysis.
- Because of this, some argue that it’s ultimately a quantitative type.
- Importance: Medium. In general, knowing qualitative data analysis is not common or even necessary for corporate roles. However, for researchers working in social sciences, its importance is very high .
- Nature of Data: data treated under qualitative analysis is non-numeric. However, as part of the analysis, analysts turn non-numeric data into numbers, at which point many argue it is no longer qualitative analysis.
- Motive: to extract insights. (This will be more important as we move down the pyramid.)
Mathematical Analysis
- Description: mathematical data analysis is a subtype of qualitative data analysis that designates methods and techniques based on statistics, algebra, and logical reasoning to extract insights. It stands in opposition to artificial intelligence analysis.
- Importance: Very High. The most widespread methods and techniques fall under mathematical analysis. In fact, it’s so common that many people use “quantitative” and “mathematical” analysis interchangeably.
- Nature of Data: numeric. By definition, all data under mathematical analysis are numbers.
- Motive: to extract measurable insights that can be used to act upon.
Artificial Intelligence & Machine Learning Analysis
- Description: artificial intelligence and machine learning analyses designate techniques based on the titular skills. They are not traditionally mathematical, but they are quantitative since they use numbers. Applications of AI & ML analysis techniques are developing, but they’re not yet mainstream enough to show promise across the field.
- Importance: Medium . As of today (September 2020), you don’t need to be fluent in AI & ML data analysis to be a great analyst. BUT, if it’s a field that interests you, learn it. Many believe that in 10 year’s time its importance will be very high .
- Nature of Data: numeric.
- Motive: to create calculations that build on themselves in order and extract insights without direct input from a human.
Descriptive Analysis
- Description: descriptive analysis is a subtype of mathematical data analysis that uses methods and techniques to provide information about the size, dispersion, groupings, and behavior of data sets. This may sounds complicated, but just think about mean, median, and mode: all three are types of descriptive analysis. They provide information about the data set. We’ll look at specific techniques below.
- Importance: Very high. Descriptive analysis is among the most commonly used data analyses in both corporations and research today.
- Nature of Data: the nature of data under descriptive statistics is sets. A set is simply a collection of numbers that behaves in predictable ways. Data reflects real life, and there are patterns everywhere to be found. Descriptive analysis describes those patterns.
- Motive: the motive behind descriptive analysis is to understand how numbers in a set group together, how far apart they are from each other, and how often they occur. As with most statistical analysis, the more data points there are, the easier it is to describe the set.
Diagnostic Analysis
- Description: diagnostic analysis answers the question “why did it happen?” It is an advanced type of mathematical data analysis that manipulates multiple techniques, but does not own any single one. Analysts engage in diagnostic analysis when they try to explain why.
- Importance: Very high. Diagnostics are probably the most important type of data analysis for people who don’t do analysis because they’re valuable to anyone who’s curious. They’re most common in corporations, as managers often only want to know the “why.”
- Nature of Data : data under diagnostic analysis are data sets. These sets in themselves are not enough under diagnostic analysis. Instead, the analyst must know what’s behind the numbers in order to explain “why.” That’s what makes diagnostics so challenging yet so valuable.
- Motive: the motive behind diagnostics is to diagnose — to understand why.
Predictive Analysis
- Description: predictive analysis uses past data to project future data. It’s very often one of the first kinds of analysis new researchers and corporate analysts use because it is intuitive. It is a subtype of the mathematical type of data analysis, and its three notable techniques are regression, moving average, and exponential smoothing.
- Importance: Very high. Predictive analysis is critical for any data analyst working in a corporate environment. Companies always want to know what the future will hold — especially for their revenue.
- Nature of Data: Because past and future imply time, predictive data always includes an element of time. Whether it’s minutes, hours, days, months, or years, we call this time series data . In fact, this data is so important that I’ll mention it twice so you don’t forget: predictive analysis uses time series data .
- Motive: the motive for investigating time series data with predictive analysis is to predict the future in the most analytical way possible.
Prescriptive Analysis
- Description: prescriptive analysis is a subtype of mathematical analysis that answers the question “what will happen if we do X?” It’s largely underestimated in the data analysis world because it requires diagnostic and descriptive analyses to be done before it even starts. More than simple predictive analysis, prescriptive analysis builds entire data models to show how a simple change could impact the ensemble.
- Importance: High. Prescriptive analysis is most common under the finance function in many companies. Financial analysts use it to build a financial model of the financial statements that show how that data will change given alternative inputs.
- Nature of Data: the nature of data in prescriptive analysis is data sets. These data sets contain patterns that respond differently to various inputs. Data that is useful for prescriptive analysis contains correlations between different variables. It’s through these correlations that we establish patterns and prescribe action on this basis. This analysis cannot be performed on data that exists in a vacuum — it must be viewed on the backdrop of the tangibles behind it.
- Motive: the motive for prescriptive analysis is to establish, with an acceptable degree of certainty, what results we can expect given a certain action. As you might expect, this necessitates that the analyst or researcher be aware of the world behind the data, not just the data itself.
Clustering Method
- Description: the clustering method groups data points together based on their relativeness closeness to further explore and treat them based on these groupings. There are two ways to group clusters: intuitively and statistically (or K-means).
- Importance: Very high. Though most corporate roles group clusters intuitively based on management criteria, a solid understanding of how to group them mathematically is an excellent descriptive and diagnostic approach to allow for prescriptive analysis thereafter.
- Nature of Data : the nature of data useful for clustering is sets with 1 or more data fields. While most people are used to looking at only two dimensions (x and y), clustering becomes more accurate the more fields there are.
- Motive: the motive for clustering is to understand how data sets group and to explore them further based on those groups.
- Here’s an example set:

Classification Method
- Description: the classification method aims to separate and group data points based on common characteristics . This can be done intuitively or statistically.
- Importance: High. While simple on the surface, classification can become quite complex. It’s very valuable in corporate and research environments, but can feel like its not worth the work. A good analyst can execute it quickly to deliver results.
- Nature of Data: the nature of data useful for classification is data sets. As we will see, it can be used on qualitative data as well as quantitative. This method requires knowledge of the substance behind the data, not just the numbers themselves.
- Motive: the motive for classification is group data not based on mathematical relationships (which would be clustering), but by predetermined outputs. This is why it’s less useful for diagnostic analysis, and more useful for prescriptive analysis.
Forecasting Method
- Description: the forecasting method uses time past series data to forecast the future.
- Importance: Very high. Forecasting falls under predictive analysis and is arguably the most common and most important method in the corporate world. It is less useful in research, which prefers to understand the known rather than speculate about the future.
- Nature of Data: data useful for forecasting is time series data, which, as we’ve noted, always includes a variable of time.
- Motive: the motive for the forecasting method is the same as that of prescriptive analysis: the confidently estimate future values.
Optimization Method
- Description: the optimization method maximized or minimizes values in a set given a set of criteria. It is arguably most common in prescriptive analysis. In mathematical terms, it is maximizing or minimizing a function given certain constraints.
- Importance: Very high. The idea of optimization applies to more analysis types than any other method. In fact, some argue that it is the fundamental driver behind data analysis. You would use it everywhere in research and in a corporation.
- Nature of Data: the nature of optimizable data is a data set of at least two points.
- Motive: the motive behind optimization is to achieve the best result possible given certain conditions.
Content Analysis Method
- Description: content analysis is a method of qualitative analysis that quantifies textual data to track themes across a document. It’s most common in academic fields and in social sciences, where written content is the subject of inquiry.
- Importance: High. In a corporate setting, content analysis as such is less common. If anything Nïave Bayes (a technique we’ll look at below) is the closest corporations come to text. However, it is of the utmost importance for researchers. If you’re a researcher, check out this article on content analysis .
- Nature of Data: data useful for content analysis is textual data.
- Motive: the motive behind content analysis is to understand themes expressed in a large text
Narrative Analysis Method
- Description: narrative analysis is a method of qualitative analysis that quantifies stories to trace themes in them. It’s differs from content analysis because it focuses on stories rather than research documents, and the techniques used are slightly different from those in content analysis (very nuances and outside the scope of this article).
- Importance: Low. Unless you are highly specialized in working with stories, narrative analysis rare.
- Nature of Data: the nature of the data useful for the narrative analysis method is narrative text.
- Motive: the motive for narrative analysis is to uncover hidden patterns in narrative text.
Discourse Analysis Method
- Description: the discourse analysis method falls under qualitative analysis and uses thematic coding to trace patterns in real-life discourse. That said, real-life discourse is oral, so it must first be transcribed into text.
- Importance: Low. Unless you are focused on understand real-world idea sharing in a research setting, this kind of analysis is less common than the others on this list.
- Nature of Data: the nature of data useful in discourse analysis is first audio files, then transcriptions of those audio files.
- Motive: the motive behind discourse analysis is to trace patterns of real-world discussions. (As a spooky sidenote, have you ever felt like your phone microphone was listening to you and making reading suggestions? If it was, the method was discourse analysis.)
Framework Analysis Method
- Description: the framework analysis method falls under qualitative analysis and uses similar thematic coding techniques to content analysis. However, where content analysis aims to discover themes, framework analysis starts with a framework and only considers elements that fall in its purview.
- Importance: Low. As with the other textual analysis methods, framework analysis is less common in corporate settings. Even in the world of research, only some use it. Strangely, it’s very common for legislative and political research.
- Nature of Data: the nature of data useful for framework analysis is textual.
- Motive: the motive behind framework analysis is to understand what themes and parts of a text match your search criteria.
Grounded Theory Method
- Description: the grounded theory method falls under qualitative analysis and uses thematic coding to build theories around those themes.
- Importance: Low. Like other qualitative analysis techniques, grounded theory is less common in the corporate world. Even among researchers, you would be hard pressed to find many using it. Though powerful, it’s simply too rare to spend time learning.
- Nature of Data: the nature of data useful in the grounded theory method is textual.
- Motive: the motive of grounded theory method is to establish a series of theories based on themes uncovered from a text.
Clustering Technique: K-Means
- Description: k-means is a clustering technique in which data points are grouped in clusters that have the closest means. Though not considered AI or ML, it inherently requires the use of supervised learning to reevaluate clusters as data points are added. Clustering techniques can be used in diagnostic, descriptive, & prescriptive data analyses.
- Importance: Very important. If you only take 3 things from this article, k-means clustering should be part of it. It is useful in any situation where n observations have multiple characteristics and we want to put them in groups.
- Nature of Data: the nature of data is at least one characteristic per observation, but the more the merrier.
- Motive: the motive for clustering techniques such as k-means is to group observations together and either understand or react to them.
Regression Technique
- Description: simple and multivariable regressions use either one independent variable or combination of multiple independent variables to calculate a correlation to a single dependent variable using constants. Regressions are almost synonymous with correlation today.
- Importance: Very high. Along with clustering, if you only take 3 things from this article, regression techniques should be part of it. They’re everywhere in corporate and research fields alike.
- Nature of Data: the nature of data used is regressions is data sets with “n” number of observations and as many variables as are reasonable. It’s important, however, to distinguish between time series data and regression data. You cannot use regressions or time series data without accounting for time. The easier way is to use techniques under the forecasting method.
- Motive: The motive behind regression techniques is to understand correlations between independent variable(s) and a dependent one.
Nïave Bayes Technique
- Description: Nïave Bayes is a classification technique that uses simple probability to classify items based previous classifications. In plain English, the formula would be “the chance that thing with trait x belongs to class c depends on (=) the overall chance of trait x belonging to class c, multiplied by the overall chance of class c, divided by the overall chance of getting trait x.” As a formula, it’s P(c|x) = P(x|c) * P(c) / P(x).
- Importance: High. Nïave Bayes is a very common, simplistic classification techniques because it’s effective with large data sets and it can be applied to any instant in which there is a class. Google, for example, might use it to group webpages into groups for certain search engine queries.
- Nature of Data: the nature of data for Nïave Bayes is at least one class and at least two traits in a data set.
- Motive: the motive behind Nïave Bayes is to classify observations based on previous data. It’s thus considered part of predictive analysis.
Cohorts Technique
- Description: cohorts technique is a type of clustering method used in behavioral sciences to separate users by common traits. As with clustering, it can be done intuitively or mathematically, the latter of which would simply be k-means.
- Importance: Very high. With regard to resembles k-means, the cohort technique is more of a high-level counterpart. In fact, most people are familiar with it as a part of Google Analytics. It’s most common in marketing departments in corporations, rather than in research.
- Nature of Data: the nature of cohort data is data sets in which users are the observation and other fields are used as defining traits for each cohort.
- Motive: the motive for cohort analysis techniques is to group similar users and analyze how you retain them and how the churn.
Factor Technique
- Description: the factor analysis technique is a way of grouping many traits into a single factor to expedite analysis. For example, factors can be used as traits for Nïave Bayes classifications instead of more general fields.
- Importance: High. While not commonly employed in corporations, factor analysis is hugely valuable. Good data analysts use it to simplify their projects and communicate them more clearly.
- Nature of Data: the nature of data useful in factor analysis techniques is data sets with a large number of fields on its observations.
- Motive: the motive for using factor analysis techniques is to reduce the number of fields in order to more quickly analyze and communicate findings.
Linear Discriminants Technique
- Description: linear discriminant analysis techniques are similar to regressions in that they use one or more independent variable to determine a dependent variable; however, the linear discriminant technique falls under a classifier method since it uses traits as independent variables and class as a dependent variable. In this way, it becomes a classifying method AND a predictive method.
- Importance: High. Though the analyst world speaks of and uses linear discriminants less commonly, it’s a highly valuable technique to keep in mind as you progress in data analysis.
- Nature of Data: the nature of data useful for the linear discriminant technique is data sets with many fields.
- Motive: the motive for using linear discriminants is to classify observations that would be otherwise too complex for simple techniques like Nïave Bayes.
Exponential Smoothing Technique
- Description: exponential smoothing is a technique falling under the forecasting method that uses a smoothing factor on prior data in order to predict future values. It can be linear or adjusted for seasonality. The basic principle behind exponential smoothing is to use a percent weight (value between 0 and 1 called alpha) on more recent values in a series and a smaller percent weight on less recent values. The formula is f(x) = current period value * alpha + previous period value * 1-alpha.
- Importance: High. Most analysts still use the moving average technique (covered next) for forecasting, though it is less efficient than exponential moving, because it’s easy to understand. However, good analysts will have exponential smoothing techniques in their pocket to increase the value of their forecasts.
- Nature of Data: the nature of data useful for exponential smoothing is time series data . Time series data has time as part of its fields .
- Motive: the motive for exponential smoothing is to forecast future values with a smoothing variable.
Moving Average Technique
- Description: the moving average technique falls under the forecasting method and uses an average of recent values to predict future ones. For example, to predict rainfall in April, you would take the average of rainfall from January to March. It’s simple, yet highly effective.
- Importance: Very high. While I’m personally not a huge fan of moving averages due to their simplistic nature and lack of consideration for seasonality, they’re the most common forecasting technique and therefore very important.
- Nature of Data: the nature of data useful for moving averages is time series data .
- Motive: the motive for moving averages is to predict future values is a simple, easy-to-communicate way.
Neural Networks Technique
- Description: neural networks are a highly complex artificial intelligence technique that replicate a human’s neural analysis through a series of hyper-rapid computations and comparisons that evolve in real time. This technique is so complex that an analyst must use computer programs to perform it.
- Importance: Medium. While the potential for neural networks is theoretically unlimited, it’s still little understood and therefore uncommon. You do not need to know it by any means in order to be a data analyst.
- Nature of Data: the nature of data useful for neural networks is data sets of astronomical size, meaning with 100s of 1000s of fields and the same number of row at a minimum .
- Motive: the motive for neural networks is to understand wildly complex phenomenon and data to thereafter act on it.
Decision Tree Technique
- Description: the decision tree technique uses artificial intelligence algorithms to rapidly calculate possible decision pathways and their outcomes on a real-time basis. It’s so complex that computer programs are needed to perform it.
- Importance: Medium. As with neural networks, decision trees with AI are too little understood and are therefore uncommon in corporate and research settings alike.
- Nature of Data: the nature of data useful for the decision tree technique is hierarchical data sets that show multiple optional fields for each preceding field.
- Motive: the motive for decision tree techniques is to compute the optimal choices to make in order to achieve a desired result.
Evolutionary Programming Technique
- Description: the evolutionary programming technique uses a series of neural networks, sees how well each one fits a desired outcome, and selects only the best to test and retest. It’s called evolutionary because is resembles the process of natural selection by weeding out weaker options.
- Importance: Medium. As with the other AI techniques, evolutionary programming just isn’t well-understood enough to be usable in many cases. It’s complexity also makes it hard to explain in corporate settings and difficult to defend in research settings.
- Nature of Data: the nature of data in evolutionary programming is data sets of neural networks, or data sets of data sets.
- Motive: the motive for using evolutionary programming is similar to decision trees: understanding the best possible option from complex data.
- Video example :
Fuzzy Logic Technique
- Description: fuzzy logic is a type of computing based on “approximate truths” rather than simple truths such as “true” and “false.” It is essentially two tiers of classification. For example, to say whether “Apples are good,” you need to first classify that “Good is x, y, z.” Only then can you say apples are good. Another way to see it helping a computer see truth like humans do: “definitely true, probably true, maybe true, probably false, definitely false.”
- Importance: Medium. Like the other AI techniques, fuzzy logic is uncommon in both research and corporate settings, which means it’s less important in today’s world.
- Nature of Data: the nature of fuzzy logic data is huge data tables that include other huge data tables with a hierarchy including multiple subfields for each preceding field.
- Motive: the motive of fuzzy logic to replicate human truth valuations in a computer is to model human decisions based on past data. The obvious possible application is marketing.
Text Analysis Technique
- Description: text analysis techniques fall under the qualitative data analysis type and use text to extract insights.
- Importance: Medium. Text analysis techniques, like all the qualitative analysis type, are most valuable for researchers.
- Nature of Data: the nature of data useful in text analysis is words.
- Motive: the motive for text analysis is to trace themes in a text across sets of very long documents, such as books.
Coding Technique
- Description: the coding technique is used in textual analysis to turn ideas into uniform phrases and analyze the number of times and the ways in which those ideas appear. For this reason, some consider it a quantitative technique as well. You can learn more about coding and the other qualitative techniques here .
- Importance: Very high. If you’re a researcher working in social sciences, coding is THE analysis techniques, and for good reason. It’s a great way to add rigor to analysis. That said, it’s less common in corporate settings.
- Nature of Data: the nature of data useful for coding is long text documents.
- Motive: the motive for coding is to make tracing ideas on paper more than an exercise of the mind by quantifying it and understanding is through descriptive methods.
Idea Pattern Technique
- Description: the idea pattern analysis technique fits into coding as the second step of the process. Once themes and ideas are coded, simple descriptive analysis tests may be run. Some people even cluster the ideas!
- Importance: Very high. If you’re a researcher, idea pattern analysis is as important as the coding itself.
- Nature of Data: the nature of data useful for idea pattern analysis is already coded themes.
- Motive: the motive for the idea pattern technique is to trace ideas in otherwise unmanageably-large documents.
Word Frequency Technique
- Description: word frequency is a qualitative technique that stands in opposition to coding and uses an inductive approach to locate specific words in a document in order to understand its relevance. Word frequency is essentially the descriptive analysis of qualitative data because it uses stats like mean, median, and mode to gather insights.
- Importance: High. As with the other qualitative approaches, word frequency is very important in social science research, but less so in corporate settings.
- Nature of Data: the nature of data useful for word frequency is long, informative documents.
- Motive: the motive for word frequency is to locate target words to determine the relevance of a document in question.
Types of data analysis in research
Types of data analysis in research methodology include every item discussed in this article. As a list, they are:
- Quantitative
- Qualitative
- Mathematical
- Machine Learning and AI
- Descriptive
- Prescriptive
- Classification
- Forecasting
- Optimization
- Grounded theory
- Artificial Neural Networks
- Decision Trees
- Evolutionary Programming
- Fuzzy Logic
- Text analysis
- Idea Pattern Analysis
- Word Frequency Analysis
- Nïave Bayes
- Exponential smoothing
- Moving average
- Linear discriminant
Types of data analysis in qualitative research
As a list, the types of data analysis in qualitative research are the following methods:
Types of data analysis in quantitative research
As a list, the types of data analysis in quantitative research are:
Data analysis methods
As a list, data analysis methods are:
- Content (qualitative)
- Narrative (qualitative)
- Discourse (qualitative)
- Framework (qualitative)
- Grounded theory (qualitative)
Quantitative data analysis methods
As a list, quantitative data analysis methods are:
Tabular View of Data Analysis Types, Methods, and Techniques
About the author.
Noah is the founder & Editor-in-Chief at AnalystAnswers. He is a transatlantic professional and entrepreneur with 5+ years of corporate finance and data analytics experience, as well as 3+ years in consumer financial products and business software. He started AnalystAnswers to provide aspiring professionals with accessible explanations of otherwise dense finance and data concepts. Noah believes everyone can benefit from an analytical mindset in growing digital world. When he's not busy at work, Noah likes to explore new European cities, exercise, and spend time with friends and family.
File available immediately.
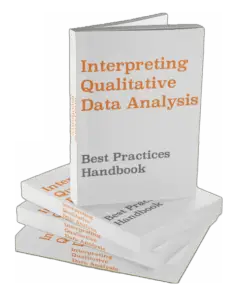
Notice: JavaScript is required for this content.
8 Types of Data Analysis

Data analysis is an aspect of data science and data analytics that is all about analyzing data for different kinds of purposes. The data analysis process involves inspecting, cleaning, transforming and modeling data to draw useful insights from it.
What Are the Different Types of Data Analysis?
- Descriptive analysis
- Diagnostic analysis
- Exploratory analysis
- Inferential analysis
- Predictive analysis
- Causal analysis
- Mechanistic analysis
- Prescriptive analysis
With its multiple facets, methodologies and techniques, data analysis is used in a variety of fields, including business, science and social science, among others. As businesses thrive under the influence of technological advancements in data analytics, data analysis plays a huge role in decision-making , providing a better, faster and more efficacious system that minimizes risks and reduces human biases .
That said, there are different kinds of data analysis catered with different goals. We’ll examine each one below.
Two Camps of Data Analysis
Data analysis can be divided into two camps, according to the book R for Data Science :
- Hypothesis Generation — This involves looking deeply at the data and combining your domain knowledge to generate hypotheses about why the data behaves the way it does.
- Hypothesis Confirmation — This involves using a precise mathematical model to generate falsifiable predictions with statistical sophistication to confirm your prior hypotheses.
Types of Data Analysis
Data analysis can be separated and organized into types, arranged in an increasing order of complexity.
1. Descriptive Analysis
The goal of descriptive analysis is to describe or summarize a set of data. Here’s what you need to know:
- Descriptive analysis is the very first analysis performed in the data analysis process.
- It generates simple summaries about samples and measurements.
- It involves common, descriptive statistics like measures of central tendency, variability, frequency and position.
Descriptive Analysis Example
Take the Covid-19 statistics page on Google, for example. The line graph is a pure summary of the cases/deaths, a presentation and description of the population of a particular country infected by the virus.
Descriptive analysis is the first step in analysis where you summarize and describe the data you have using descriptive statistics, and the result is a simple presentation of your data.
More on Data Analysis: Data Analyst vs. Data Scientist: Similarities and Differences Explained
2. Diagnostic Analysis
Diagnostic analysis seeks to answer the question “Why did this happen?” by taking a more in-depth look at data to uncover subtle patterns. Here’s what you need to know:
- Diagnostic analysis typically comes after descriptive analysis, taking initial findings and investigating why certain patterns in data happen.
- Diagnostic analysis may involve analyzing other related data sources, including past data, to reveal more insights into current data trends.
- Diagnostic analysis is ideal for further exploring patterns in data to explain anomalies.
Diagnostic Analysis Example
A footwear store wants to review its website traffic levels over the previous 12 months. Upon compiling and assessing the data, the company’s marketing team finds that June experienced above-average levels of traffic while July and August witnessed slightly lower levels of traffic.
To find out why this difference occurred, the marketing team takes a deeper look. Team members break down the data to focus on specific categories of footwear. In the month of June, they discovered that pages featuring sandals and other beach-related footwear received a high number of views while these numbers dropped in July and August.
Marketers may also review other factors like seasonal changes and company sales events to see if other variables could have contributed to this trend.
3. Exploratory Analysis (EDA)
Exploratory analysis involves examining or exploring data and finding relationships between variables that were previously unknown. Here’s what you need to know:
- EDA helps you discover relationships between measures in your data, which are not evidence for the existence of the correlation, as denoted by the phrase, “ Correlation doesn’t imply causation .”
- It’s useful for discovering new connections and forming hypotheses. It drives design planning and data collection.
Exploratory Analysis Example
Climate change is an increasingly important topic as the global temperature has gradually risen over the years. One example of an exploratory data analysis on climate change involves taking the rise in temperature over the years from 1950 to 2020 and the increase of human activities and industrialization to find relationships from the data. For example, you may increase the number of factories, cars on the road and airplane flights to see how that correlates with the rise in temperature.
Exploratory analysis explores data to find relationships between measures without identifying the cause. It’s most useful when formulating hypotheses.
4. Inferential Analysis
Inferential analysis involves using a small sample of data to infer information about a larger population of data.
The goal of statistical modeling itself is all about using a small amount of information to extrapolate and generalize information to a larger group. Here’s what you need to know:
- Inferential analysis involves using estimated data that is representative of a population and gives a measure of uncertainty or standard deviation to your estimation.
- The accuracy of inference depends heavily on your sampling scheme. If the sample isn’t representative of the population, the generalization will be inaccurate. This is known as the central limit theorem .
Inferential Analysis Example
The idea of drawing an inference about the population at large with a smaller sample size is intuitive. Many statistics you see on the media and the internet are inferential; a prediction of an event based on a small sample. For example, a psychological study on the benefits of sleep might have a total of 500 people involved. When they followed up with the candidates, the candidates reported to have better overall attention spans and well-being with seven-to-nine hours of sleep, while those with less sleep and more sleep than the given range suffered from reduced attention spans and energy. This study drawn from 500 people was just a tiny portion of the 7 billion people in the world, and is thus an inference of the larger population.
Inferential analysis extrapolates and generalizes the information of the larger group with a smaller sample to generate analysis and predictions.
5. Predictive Analysis
Predictive analysis involves using historical or current data to find patterns and make predictions about the future. Here’s what you need to know:
- The accuracy of the predictions depends on the input variables.
- Accuracy also depends on the types of models. A linear model might work well in some cases, and in other cases it might not.
- Using a variable to predict another one doesn’t denote a causal relationship.
Predictive Analysis Example
The 2020 US election is a popular topic and many prediction models are built to predict the winning candidate. FiveThirtyEight did this to forecast the 2016 and 2020 elections. Prediction analysis for an election would require input variables such as historical polling data, trends and current polling data in order to return a good prediction. Something as large as an election wouldn’t just be using a linear model, but a complex model with certain tunings to best serve its purpose.
Predictive analysis takes data from the past and present to make predictions about the future.
More on Data: Explaining the Empirical for Normal Distribution
6. Causal Analysis
Causal analysis looks at the cause and effect of relationships between variables and is focused on finding the cause of a correlation. Here’s what you need to know:
- To find the cause, you have to question whether the observed correlations driving your conclusion are valid. Just looking at the surface data won’t help you discover the hidden mechanisms underlying the correlations.
- Causal analysis is applied in randomized studies focused on identifying causation.
- Causal analysis is the gold standard in data analysis and scientific studies where the cause of phenomenon is to be extracted and singled out, like separating wheat from chaff.
- Good data is hard to find and requires expensive research and studies. These studies are analyzed in aggregate (multiple groups), and the observed relationships are just average effects (mean) of the whole population. This means the results might not apply to everyone.
Causal Analysis Example
Say you want to test out whether a new drug improves human strength and focus. To do that, you perform randomized control trials for the drug to test its effect. You compare the sample of candidates for your new drug against the candidates receiving a mock control drug through a few tests focused on strength and overall focus and attention. This will allow you to observe how the drug affects the outcome.
Causal analysis is about finding out the causal relationship between variables, and examining how a change in one variable affects another.
7. Mechanistic Analysis
Mechanistic analysis is used to understand exact changes in variables that lead to other changes in other variables. Here’s what you need to know:
- It’s applied in physical or engineering sciences, situations that require high precision and little room for error, only noise in data is measurement error.
- It’s designed to understand a biological or behavioral process, the pathophysiology of a disease or the mechanism of action of an intervention.
Mechanistic Analysis Example
Many graduate-level research and complex topics are suitable examples, but to put it in simple terms, let’s say an experiment is done to simulate safe and effective nuclear fusion to power the world. A mechanistic analysis of the study would entail a precise balance of controlling and manipulating variables with highly accurate measures of both variables and the desired outcomes. It’s this intricate and meticulous modus operandi toward these big topics that allows for scientific breakthroughs and advancement of society.
Mechanistic analysis is in some ways a predictive analysis, but modified to tackle studies that require high precision and meticulous methodologies for physical or engineering science .
8. Prescriptive Analysis
Prescriptive analysis compiles insights from other previous data analyses and determines actions that teams or companies can take to prepare for predicted trends. Here’s what you need to know:
- Prescriptive analysis may come right after predictive analysis, but it may involve combining many different data analyses.
- Companies need advanced technology and plenty of resources to conduct prescriptive analysis. AI systems that process data and adjust automated tasks are an example of the technology required to perform prescriptive analysis.
Prescriptive Analysis Example
Prescriptive analysis is pervasive in everyday life, driving the curated content users consume on social media. On platforms like TikTok and Instagram, algorithms can apply prescriptive analysis to review past content a user has engaged with and the kinds of behaviors they exhibited with specific posts. Based on these factors, an algorithm seeks out similar content that is likely to elicit the same response and recommends it on a user’s personal feed.
When to Use the Different Types of Data Analysis
- Descriptive analysis summarizes the data at hand and presents your data in a comprehensible way.
- Diagnostic analysis takes a more detailed look at data to reveal why certain patterns occur, making it a good method for explaining anomalies.
- Exploratory data analysis helps you discover correlations and relationships between variables in your data.
- Inferential analysis is for generalizing the larger population with a smaller sample size of data.
- Predictive analysis helps you make predictions about the future with data.
- Causal analysis emphasizes finding the cause of a correlation between variables.
- Mechanistic analysis is for measuring the exact changes in variables that lead to other changes in other variables.
- Prescriptive analysis combines insights from different data analyses to develop a course of action teams and companies can take to capitalize on predicted outcomes.
A few important tips to remember about data analysis include:
- Correlation doesn’t imply causation.
- EDA helps discover new connections and form hypotheses.
- Accuracy of inference depends on the sampling scheme.
- A good prediction depends on the right input variables.
- A simple linear model with enough data usually does the trick.
- Using a variable to predict another doesn’t denote causal relationships.
- Good data is hard to find, and to produce it requires expensive research.
- Results from studies are done in aggregate and are average effects and might not apply to everyone.
Built In’s expert contributor network publishes thoughtful, solutions-oriented stories written by innovative tech professionals. It is the tech industry’s definitive destination for sharing compelling, first-person accounts of problem-solving on the road to innovation.
Great Companies Need Great People. That's Where We Come In.
Your Modern Business Guide To Data Analysis Methods And Techniques

Table of Contents
1) What Is Data Analysis?
2) Why Is Data Analysis Important?
3) What Is The Data Analysis Process?
4) Types Of Data Analysis Methods
5) Top Data Analysis Techniques To Apply
6) Quality Criteria For Data Analysis
7) Data Analysis Limitations & Barriers
8) Data Analysis Skills
9) Data Analysis In The Big Data Environment
In our data-rich age, understanding how to analyze and extract true meaning from our business’s digital insights is one of the primary drivers of success.
Despite the colossal volume of data we create every day, a mere 0.5% is actually analyzed and used for data discovery , improvement, and intelligence. While that may not seem like much, considering the amount of digital information we have at our fingertips, half a percent still accounts for a vast amount of data.
With so much data and so little time, knowing how to collect, curate, organize, and make sense of all of this potentially business-boosting information can be a minefield – but online data analysis is the solution.
In science, data analysis uses a more complex approach with advanced techniques to explore and experiment with data. On the other hand, in a business context, data is used to make data-driven decisions that will enable the company to improve its overall performance. In this post, we will cover the analysis of data from an organizational point of view while still going through the scientific and statistical foundations that are fundamental to understanding the basics of data analysis.
To put all of that into perspective, we will answer a host of important analytical questions, explore analytical methods and techniques, while demonstrating how to perform analysis in the real world with a 17-step blueprint for success.
What Is Data Analysis?
Data analysis is the process of collecting, modeling, and analyzing data using various statistical and logical methods and techniques. Businesses rely on analytics processes and tools to extract insights that support strategic and operational decision-making.
All these various methods are largely based on two core areas: quantitative and qualitative research.
To explain the key differences between qualitative and quantitative research, here’s a video for your viewing pleasure:
Gaining a better understanding of different techniques and methods in quantitative research as well as qualitative insights will give your analyzing efforts a more clearly defined direction, so it’s worth taking the time to allow this particular knowledge to sink in. Additionally, you will be able to create a comprehensive analytical report that will skyrocket your analysis.
Apart from qualitative and quantitative categories, there are also other types of data that you should be aware of before dividing into complex data analysis processes. These categories include:
- Big data: Refers to massive data sets that need to be analyzed using advanced software to reveal patterns and trends. It is considered to be one of the best analytical assets as it provides larger volumes of data at a faster rate.
- Metadata: Putting it simply, metadata is data that provides insights about other data. It summarizes key information about specific data that makes it easier to find and reuse for later purposes.
- Real time data: As its name suggests, real time data is presented as soon as it is acquired. From an organizational perspective, this is the most valuable data as it can help you make important decisions based on the latest developments. Our guide on real time analytics will tell you more about the topic.
- Machine data: This is more complex data that is generated solely by a machine such as phones, computers, or even websites and embedded systems, without previous human interaction.
Why Is Data Analysis Important?
Before we go into detail about the categories of analysis along with its methods and techniques, you must understand the potential that analyzing data can bring to your organization.
- Informed decision-making : From a management perspective, you can benefit from analyzing your data as it helps you make decisions based on facts and not simple intuition. For instance, you can understand where to invest your capital, detect growth opportunities, predict your income, or tackle uncommon situations before they become problems. Through this, you can extract relevant insights from all areas in your organization, and with the help of dashboard software , present the data in a professional and interactive way to different stakeholders.
- Reduce costs : Another great benefit is to reduce costs. With the help of advanced technologies such as predictive analytics, businesses can spot improvement opportunities, trends, and patterns in their data and plan their strategies accordingly. In time, this will help you save money and resources on implementing the wrong strategies. And not just that, by predicting different scenarios such as sales and demand you can also anticipate production and supply.
- Target customers better : Customers are arguably the most crucial element in any business. By using analytics to get a 360° vision of all aspects related to your customers, you can understand which channels they use to communicate with you, their demographics, interests, habits, purchasing behaviors, and more. In the long run, it will drive success to your marketing strategies, allow you to identify new potential customers, and avoid wasting resources on targeting the wrong people or sending the wrong message. You can also track customer satisfaction by analyzing your client’s reviews or your customer service department’s performance.
What Is The Data Analysis Process?

When we talk about analyzing data there is an order to follow in order to extract the needed conclusions. The analysis process consists of 5 key stages. We will cover each of them more in detail later in the post, but to start providing the needed context to understand what is coming next, here is a rundown of the 5 essential steps of data analysis.
- Identify: Before you get your hands dirty with data, you first need to identify why you need it in the first place. The identification is the stage in which you establish the questions you will need to answer. For example, what is the customer's perception of our brand? Or what type of packaging is more engaging to our potential customers? Once the questions are outlined you are ready for the next step.
- Collect: As its name suggests, this is the stage where you start collecting the needed data. Here, you define which sources of data you will use and how you will use them. The collection of data can come in different forms such as internal or external sources, surveys, interviews, questionnaires, and focus groups, among others. An important note here is that the way you collect the data will be different in a quantitative and qualitative scenario.
- Clean: Once you have the necessary data it is time to clean it and leave it ready for analysis. Not all the data you collect will be useful, when collecting big amounts of data in different formats it is very likely that you will find yourself with duplicate or badly formatted data. To avoid this, before you start working with your data you need to make sure to erase any white spaces, duplicate records, or formatting errors. This way you avoid hurting your analysis with bad-quality data.
- Analyze : With the help of various techniques such as statistical analysis, regressions, neural networks, text analysis, and more, you can start analyzing and manipulating your data to extract relevant conclusions. At this stage, you find trends, correlations, variations, and patterns that can help you answer the questions you first thought of in the identify stage. Various technologies in the market assist researchers and average users with the management of their data. Some of them include business intelligence and visualization software, predictive analytics, and data mining, among others.
- Interpret: Last but not least you have one of the most important steps: it is time to interpret your results. This stage is where the researcher comes up with courses of action based on the findings. For example, here you would understand if your clients prefer packaging that is red or green, plastic or paper, etc. Additionally, at this stage, you can also find some limitations and work on them.
Now that you have a basic understanding of the key data analysis steps, let’s look at the top 17 essential methods.
17 Essential Types Of Data Analysis Methods
Before diving into the 17 essential types of methods, it is important that we go over really fast through the main analysis categories. Starting with the category of descriptive up to prescriptive analysis, the complexity and effort of data evaluation increases, but also the added value for the company.
a) Descriptive analysis - What happened.
The descriptive analysis method is the starting point for any analytic reflection, and it aims to answer the question of what happened? It does this by ordering, manipulating, and interpreting raw data from various sources to turn it into valuable insights for your organization.
Performing descriptive analysis is essential, as it enables us to present our insights in a meaningful way. Although it is relevant to mention that this analysis on its own will not allow you to predict future outcomes or tell you the answer to questions like why something happened, it will leave your data organized and ready to conduct further investigations.
b) Exploratory analysis - How to explore data relationships.
As its name suggests, the main aim of the exploratory analysis is to explore. Prior to it, there is still no notion of the relationship between the data and the variables. Once the data is investigated, exploratory analysis helps you to find connections and generate hypotheses and solutions for specific problems. A typical area of application for it is data mining.
c) Diagnostic analysis - Why it happened.
Diagnostic data analytics empowers analysts and executives by helping them gain a firm contextual understanding of why something happened. If you know why something happened as well as how it happened, you will be able to pinpoint the exact ways of tackling the issue or challenge.
Designed to provide direct and actionable answers to specific questions, this is one of the world’s most important methods in research, among its other key organizational functions such as retail analytics , e.g.
c) Predictive analysis - What will happen.
The predictive method allows you to look into the future to answer the question: what will happen? In order to do this, it uses the results of the previously mentioned descriptive, exploratory, and diagnostic analysis, in addition to machine learning (ML) and artificial intelligence (AI). Through this, you can uncover future trends, potential problems or inefficiencies, connections, and casualties in your data.
With predictive analysis, you can unfold and develop initiatives that will not only enhance your various operational processes but also help you gain an all-important edge over the competition. If you understand why a trend, pattern, or event happened through data, you will be able to develop an informed projection of how things may unfold in particular areas of the business.
e) Prescriptive analysis - How will it happen.
Another of the most effective types of analysis methods in research. Prescriptive data techniques cross over from predictive analysis in the way that it revolves around using patterns or trends to develop responsive, practical business strategies.
By drilling down into prescriptive analysis, you will play an active role in the data consumption process by taking well-arranged sets of visual data and using it as a powerful fix to emerging issues in a number of key areas, including marketing, sales, customer experience, HR, fulfillment, finance, logistics analytics , and others.

As mentioned at the beginning of the post, data analysis methods can be divided into two big categories: quantitative and qualitative. Each of these categories holds a powerful analytical value that changes depending on the scenario and type of data you are working with. Below, we will discuss 17 methods that are divided into qualitative and quantitative approaches.
Without further ado, here are the 17 essential types of data analysis methods with some use cases in the business world:
A. Quantitative Methods
To put it simply, quantitative analysis refers to all methods that use numerical data or data that can be turned into numbers (e.g. category variables like gender, age, etc.) to extract valuable insights. It is used to extract valuable conclusions about relationships, differences, and test hypotheses. Below we discuss some of the key quantitative methods.
1. Cluster analysis
The action of grouping a set of data elements in a way that said elements are more similar (in a particular sense) to each other than to those in other groups – hence the term ‘cluster.’ Since there is no target variable when clustering, the method is often used to find hidden patterns in the data. The approach is also used to provide additional context to a trend or dataset.
Let's look at it from an organizational perspective. In a perfect world, marketers would be able to analyze each customer separately and give them the best-personalized service, but let's face it, with a large customer base, it is timely impossible to do that. That's where clustering comes in. By grouping customers into clusters based on demographics, purchasing behaviors, monetary value, or any other factor that might be relevant for your company, you will be able to immediately optimize your efforts and give your customers the best experience based on their needs.
2. Cohort analysis
This type of data analysis approach uses historical data to examine and compare a determined segment of users' behavior, which can then be grouped with others with similar characteristics. By using this methodology, it's possible to gain a wealth of insight into consumer needs or a firm understanding of a broader target group.
Cohort analysis can be really useful for performing analysis in marketing as it will allow you to understand the impact of your campaigns on specific groups of customers. To exemplify, imagine you send an email campaign encouraging customers to sign up for your site. For this, you create two versions of the campaign with different designs, CTAs, and ad content. Later on, you can use cohort analysis to track the performance of the campaign for a longer period of time and understand which type of content is driving your customers to sign up, repurchase, or engage in other ways.
A useful tool to start performing cohort analysis method is Google Analytics. You can learn more about the benefits and limitations of using cohorts in GA in this useful guide . In the bottom image, you see an example of how you visualize a cohort in this tool. The segments (devices traffic) are divided into date cohorts (usage of devices) and then analyzed week by week to extract insights into performance.
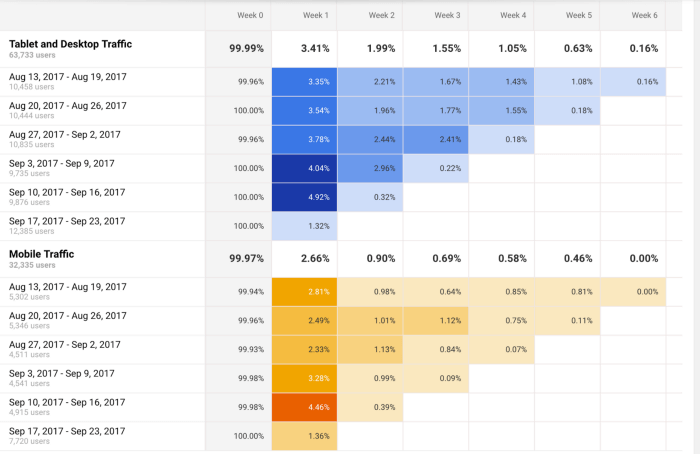
3. Regression analysis
Regression uses historical data to understand how a dependent variable's value is affected when one (linear regression) or more independent variables (multiple regression) change or stay the same. By understanding each variable's relationship and how it developed in the past, you can anticipate possible outcomes and make better decisions in the future.
Let's bring it down with an example. Imagine you did a regression analysis of your sales in 2019 and discovered that variables like product quality, store design, customer service, marketing campaigns, and sales channels affected the overall result. Now you want to use regression to analyze which of these variables changed or if any new ones appeared during 2020. For example, you couldn’t sell as much in your physical store due to COVID lockdowns. Therefore, your sales could’ve either dropped in general or increased in your online channels. Through this, you can understand which independent variables affected the overall performance of your dependent variable, annual sales.
If you want to go deeper into this type of analysis, check out this article and learn more about how you can benefit from regression.
4. Neural networks
The neural network forms the basis for the intelligent algorithms of machine learning. It is a form of analytics that attempts, with minimal intervention, to understand how the human brain would generate insights and predict values. Neural networks learn from each and every data transaction, meaning that they evolve and advance over time.
A typical area of application for neural networks is predictive analytics. There are BI reporting tools that have this feature implemented within them, such as the Predictive Analytics Tool from datapine. This tool enables users to quickly and easily generate all kinds of predictions. All you have to do is select the data to be processed based on your KPIs, and the software automatically calculates forecasts based on historical and current data. Thanks to its user-friendly interface, anyone in your organization can manage it; there’s no need to be an advanced scientist.
Here is an example of how you can use the predictive analysis tool from datapine:

**click to enlarge**
5. Factor analysis
The factor analysis also called “dimension reduction” is a type of data analysis used to describe variability among observed, correlated variables in terms of a potentially lower number of unobserved variables called factors. The aim here is to uncover independent latent variables, an ideal method for streamlining specific segments.
A good way to understand this data analysis method is a customer evaluation of a product. The initial assessment is based on different variables like color, shape, wearability, current trends, materials, comfort, the place where they bought the product, and frequency of usage. Like this, the list can be endless, depending on what you want to track. In this case, factor analysis comes into the picture by summarizing all of these variables into homogenous groups, for example, by grouping the variables color, materials, quality, and trends into a brother latent variable of design.
If you want to start analyzing data using factor analysis we recommend you take a look at this practical guide from UCLA.
6. Data mining
A method of data analysis that is the umbrella term for engineering metrics and insights for additional value, direction, and context. By using exploratory statistical evaluation, data mining aims to identify dependencies, relations, patterns, and trends to generate advanced knowledge. When considering how to analyze data, adopting a data mining mindset is essential to success - as such, it’s an area that is worth exploring in greater detail.
An excellent use case of data mining is datapine intelligent data alerts . With the help of artificial intelligence and machine learning, they provide automated signals based on particular commands or occurrences within a dataset. For example, if you’re monitoring supply chain KPIs , you could set an intelligent alarm to trigger when invalid or low-quality data appears. By doing so, you will be able to drill down deep into the issue and fix it swiftly and effectively.
In the following picture, you can see how the intelligent alarms from datapine work. By setting up ranges on daily orders, sessions, and revenues, the alarms will notify you if the goal was not completed or if it exceeded expectations.
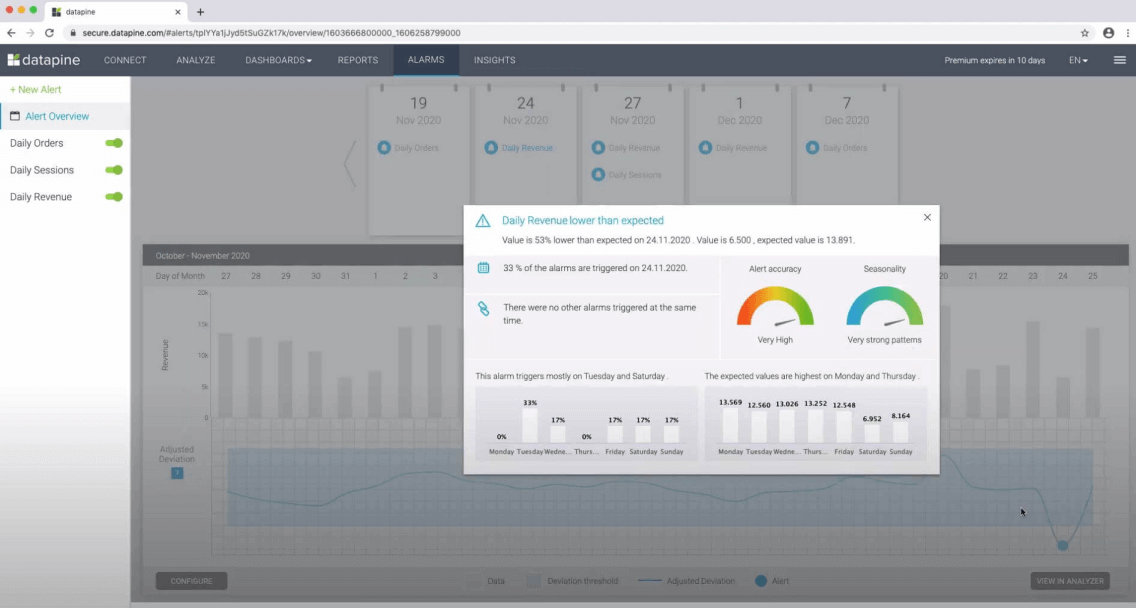
7. Time series analysis
As its name suggests, time series analysis is used to analyze a set of data points collected over a specified period of time. Although analysts use this method to monitor the data points in a specific interval of time rather than just monitoring them intermittently, the time series analysis is not uniquely used for the purpose of collecting data over time. Instead, it allows researchers to understand if variables changed during the duration of the study, how the different variables are dependent, and how did it reach the end result.
In a business context, this method is used to understand the causes of different trends and patterns to extract valuable insights. Another way of using this method is with the help of time series forecasting. Powered by predictive technologies, businesses can analyze various data sets over a period of time and forecast different future events.
A great use case to put time series analysis into perspective is seasonality effects on sales. By using time series forecasting to analyze sales data of a specific product over time, you can understand if sales rise over a specific period of time (e.g. swimwear during summertime, or candy during Halloween). These insights allow you to predict demand and prepare production accordingly.
8. Decision Trees
The decision tree analysis aims to act as a support tool to make smart and strategic decisions. By visually displaying potential outcomes, consequences, and costs in a tree-like model, researchers and company users can easily evaluate all factors involved and choose the best course of action. Decision trees are helpful to analyze quantitative data and they allow for an improved decision-making process by helping you spot improvement opportunities, reduce costs, and enhance operational efficiency and production.
But how does a decision tree actually works? This method works like a flowchart that starts with the main decision that you need to make and branches out based on the different outcomes and consequences of each decision. Each outcome will outline its own consequences, costs, and gains and, at the end of the analysis, you can compare each of them and make the smartest decision.
Businesses can use them to understand which project is more cost-effective and will bring more earnings in the long run. For example, imagine you need to decide if you want to update your software app or build a new app entirely. Here you would compare the total costs, the time needed to be invested, potential revenue, and any other factor that might affect your decision. In the end, you would be able to see which of these two options is more realistic and attainable for your company or research.
9. Conjoint analysis
Last but not least, we have the conjoint analysis. This approach is usually used in surveys to understand how individuals value different attributes of a product or service and it is one of the most effective methods to extract consumer preferences. When it comes to purchasing, some clients might be more price-focused, others more features-focused, and others might have a sustainable focus. Whatever your customer's preferences are, you can find them with conjoint analysis. Through this, companies can define pricing strategies, packaging options, subscription packages, and more.
A great example of conjoint analysis is in marketing and sales. For instance, a cupcake brand might use conjoint analysis and find that its clients prefer gluten-free options and cupcakes with healthier toppings over super sugary ones. Thus, the cupcake brand can turn these insights into advertisements and promotions to increase sales of this particular type of product. And not just that, conjoint analysis can also help businesses segment their customers based on their interests. This allows them to send different messaging that will bring value to each of the segments.
10. Correspondence Analysis
Also known as reciprocal averaging, correspondence analysis is a method used to analyze the relationship between categorical variables presented within a contingency table. A contingency table is a table that displays two (simple correspondence analysis) or more (multiple correspondence analysis) categorical variables across rows and columns that show the distribution of the data, which is usually answers to a survey or questionnaire on a specific topic.
This method starts by calculating an “expected value” which is done by multiplying row and column averages and dividing it by the overall original value of the specific table cell. The “expected value” is then subtracted from the original value resulting in a “residual number” which is what allows you to extract conclusions about relationships and distribution. The results of this analysis are later displayed using a map that represents the relationship between the different values. The closest two values are in the map, the bigger the relationship. Let’s put it into perspective with an example.
Imagine you are carrying out a market research analysis about outdoor clothing brands and how they are perceived by the public. For this analysis, you ask a group of people to match each brand with a certain attribute which can be durability, innovation, quality materials, etc. When calculating the residual numbers, you can see that brand A has a positive residual for innovation but a negative one for durability. This means that brand A is not positioned as a durable brand in the market, something that competitors could take advantage of.
11. Multidimensional Scaling (MDS)
MDS is a method used to observe the similarities or disparities between objects which can be colors, brands, people, geographical coordinates, and more. The objects are plotted using an “MDS map” that positions similar objects together and disparate ones far apart. The (dis) similarities between objects are represented using one or more dimensions that can be observed using a numerical scale. For example, if you want to know how people feel about the COVID-19 vaccine, you can use 1 for “don’t believe in the vaccine at all” and 10 for “firmly believe in the vaccine” and a scale of 2 to 9 for in between responses. When analyzing an MDS map the only thing that matters is the distance between the objects, the orientation of the dimensions is arbitrary and has no meaning at all.
Multidimensional scaling is a valuable technique for market research, especially when it comes to evaluating product or brand positioning. For instance, if a cupcake brand wants to know how they are positioned compared to competitors, it can define 2-3 dimensions such as taste, ingredients, shopping experience, or more, and do a multidimensional scaling analysis to find improvement opportunities as well as areas in which competitors are currently leading.
Another business example is in procurement when deciding on different suppliers. Decision makers can generate an MDS map to see how the different prices, delivery times, technical services, and more of the different suppliers differ and pick the one that suits their needs the best.
A final example proposed by a research paper on "An Improved Study of Multilevel Semantic Network Visualization for Analyzing Sentiment Word of Movie Review Data". Researchers picked a two-dimensional MDS map to display the distances and relationships between different sentiments in movie reviews. They used 36 sentiment words and distributed them based on their emotional distance as we can see in the image below where the words "outraged" and "sweet" are on opposite sides of the map, marking the distance between the two emotions very clearly.

Aside from being a valuable technique to analyze dissimilarities, MDS also serves as a dimension-reduction technique for large dimensional data.
B. Qualitative Methods
Qualitative data analysis methods are defined as the observation of non-numerical data that is gathered and produced using methods of observation such as interviews, focus groups, questionnaires, and more. As opposed to quantitative methods, qualitative data is more subjective and highly valuable in analyzing customer retention and product development.
12. Text analysis
Text analysis, also known in the industry as text mining, works by taking large sets of textual data and arranging them in a way that makes it easier to manage. By working through this cleansing process in stringent detail, you will be able to extract the data that is truly relevant to your organization and use it to develop actionable insights that will propel you forward.
Modern software accelerate the application of text analytics. Thanks to the combination of machine learning and intelligent algorithms, you can perform advanced analytical processes such as sentiment analysis. This technique allows you to understand the intentions and emotions of a text, for example, if it's positive, negative, or neutral, and then give it a score depending on certain factors and categories that are relevant to your brand. Sentiment analysis is often used to monitor brand and product reputation and to understand how successful your customer experience is. To learn more about the topic check out this insightful article .
By analyzing data from various word-based sources, including product reviews, articles, social media communications, and survey responses, you will gain invaluable insights into your audience, as well as their needs, preferences, and pain points. This will allow you to create campaigns, services, and communications that meet your prospects’ needs on a personal level, growing your audience while boosting customer retention. There are various other “sub-methods” that are an extension of text analysis. Each of them serves a more specific purpose and we will look at them in detail next.
13. Content Analysis
This is a straightforward and very popular method that examines the presence and frequency of certain words, concepts, and subjects in different content formats such as text, image, audio, or video. For example, the number of times the name of a celebrity is mentioned on social media or online tabloids. It does this by coding text data that is later categorized and tabulated in a way that can provide valuable insights, making it the perfect mix of quantitative and qualitative analysis.
There are two types of content analysis. The first one is the conceptual analysis which focuses on explicit data, for instance, the number of times a concept or word is mentioned in a piece of content. The second one is relational analysis, which focuses on the relationship between different concepts or words and how they are connected within a specific context.
Content analysis is often used by marketers to measure brand reputation and customer behavior. For example, by analyzing customer reviews. It can also be used to analyze customer interviews and find directions for new product development. It is also important to note, that in order to extract the maximum potential out of this analysis method, it is necessary to have a clearly defined research question.
14. Thematic Analysis
Very similar to content analysis, thematic analysis also helps in identifying and interpreting patterns in qualitative data with the main difference being that the first one can also be applied to quantitative analysis. The thematic method analyzes large pieces of text data such as focus group transcripts or interviews and groups them into themes or categories that come up frequently within the text. It is a great method when trying to figure out peoples view’s and opinions about a certain topic. For example, if you are a brand that cares about sustainability, you can do a survey of your customers to analyze their views and opinions about sustainability and how they apply it to their lives. You can also analyze customer service calls transcripts to find common issues and improve your service.
Thematic analysis is a very subjective technique that relies on the researcher’s judgment. Therefore, to avoid biases, it has 6 steps that include familiarization, coding, generating themes, reviewing themes, defining and naming themes, and writing up. It is also important to note that, because it is a flexible approach, the data can be interpreted in multiple ways and it can be hard to select what data is more important to emphasize.
15. Narrative Analysis
A bit more complex in nature than the two previous ones, narrative analysis is used to explore the meaning behind the stories that people tell and most importantly, how they tell them. By looking into the words that people use to describe a situation you can extract valuable conclusions about their perspective on a specific topic. Common sources for narrative data include autobiographies, family stories, opinion pieces, and testimonials, among others.
From a business perspective, narrative analysis can be useful to analyze customer behaviors and feelings towards a specific product, service, feature, or others. It provides unique and deep insights that can be extremely valuable. However, it has some drawbacks.
The biggest weakness of this method is that the sample sizes are usually very small due to the complexity and time-consuming nature of the collection of narrative data. Plus, the way a subject tells a story will be significantly influenced by his or her specific experiences, making it very hard to replicate in a subsequent study.
16. Discourse Analysis
Discourse analysis is used to understand the meaning behind any type of written, verbal, or symbolic discourse based on its political, social, or cultural context. It mixes the analysis of languages and situations together. This means that the way the content is constructed and the meaning behind it is significantly influenced by the culture and society it takes place in. For example, if you are analyzing political speeches you need to consider different context elements such as the politician's background, the current political context of the country, the audience to which the speech is directed, and so on.
From a business point of view, discourse analysis is a great market research tool. It allows marketers to understand how the norms and ideas of the specific market work and how their customers relate to those ideas. It can be very useful to build a brand mission or develop a unique tone of voice.
17. Grounded Theory Analysis
Traditionally, researchers decide on a method and hypothesis and start to collect the data to prove that hypothesis. The grounded theory is the only method that doesn’t require an initial research question or hypothesis as its value lies in the generation of new theories. With the grounded theory method, you can go into the analysis process with an open mind and explore the data to generate new theories through tests and revisions. In fact, it is not necessary to collect the data and then start to analyze it. Researchers usually start to find valuable insights as they are gathering the data.
All of these elements make grounded theory a very valuable method as theories are fully backed by data instead of initial assumptions. It is a great technique to analyze poorly researched topics or find the causes behind specific company outcomes. For example, product managers and marketers might use the grounded theory to find the causes of high levels of customer churn and look into customer surveys and reviews to develop new theories about the causes.
How To Analyze Data? Top 17 Data Analysis Techniques To Apply

Now that we’ve answered the questions “what is data analysis’”, why is it important, and covered the different data analysis types, it’s time to dig deeper into how to perform your analysis by working through these 17 essential techniques.
1. Collaborate your needs
Before you begin analyzing or drilling down into any techniques, it’s crucial to sit down collaboratively with all key stakeholders within your organization, decide on your primary campaign or strategic goals, and gain a fundamental understanding of the types of insights that will best benefit your progress or provide you with the level of vision you need to evolve your organization.
2. Establish your questions
Once you’ve outlined your core objectives, you should consider which questions will need answering to help you achieve your mission. This is one of the most important techniques as it will shape the very foundations of your success.
To help you ask the right things and ensure your data works for you, you have to ask the right data analysis questions .
3. Data democratization
After giving your data analytics methodology some real direction, and knowing which questions need answering to extract optimum value from the information available to your organization, you should continue with democratization.
Data democratization is an action that aims to connect data from various sources efficiently and quickly so that anyone in your organization can access it at any given moment. You can extract data in text, images, videos, numbers, or any other format. And then perform cross-database analysis to achieve more advanced insights to share with the rest of the company interactively.
Once you have decided on your most valuable sources, you need to take all of this into a structured format to start collecting your insights. For this purpose, datapine offers an easy all-in-one data connectors feature to integrate all your internal and external sources and manage them at your will. Additionally, datapine’s end-to-end solution automatically updates your data, allowing you to save time and focus on performing the right analysis to grow your company.
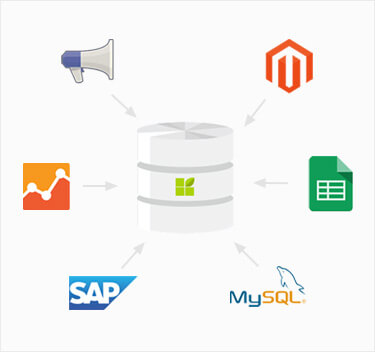
4. Think of governance
When collecting data in a business or research context you always need to think about security and privacy. With data breaches becoming a topic of concern for businesses, the need to protect your client's or subject’s sensitive information becomes critical.
To ensure that all this is taken care of, you need to think of a data governance strategy. According to Gartner , this concept refers to “ the specification of decision rights and an accountability framework to ensure the appropriate behavior in the valuation, creation, consumption, and control of data and analytics .” In simpler words, data governance is a collection of processes, roles, and policies, that ensure the efficient use of data while still achieving the main company goals. It ensures that clear roles are in place for who can access the information and how they can access it. In time, this not only ensures that sensitive information is protected but also allows for an efficient analysis as a whole.
5. Clean your data
After harvesting from so many sources you will be left with a vast amount of information that can be overwhelming to deal with. At the same time, you can be faced with incorrect data that can be misleading to your analysis. The smartest thing you can do to avoid dealing with this in the future is to clean the data. This is fundamental before visualizing it, as it will ensure that the insights you extract from it are correct.
There are many things that you need to look for in the cleaning process. The most important one is to eliminate any duplicate observations; this usually appears when using multiple internal and external sources of information. You can also add any missing codes, fix empty fields, and eliminate incorrectly formatted data.
Another usual form of cleaning is done with text data. As we mentioned earlier, most companies today analyze customer reviews, social media comments, questionnaires, and several other text inputs. In order for algorithms to detect patterns, text data needs to be revised to avoid invalid characters or any syntax or spelling errors.
Most importantly, the aim of cleaning is to prevent you from arriving at false conclusions that can damage your company in the long run. By using clean data, you will also help BI solutions to interact better with your information and create better reports for your organization.
6. Set your KPIs
Once you’ve set your sources, cleaned your data, and established clear-cut questions you want your insights to answer, you need to set a host of key performance indicators (KPIs) that will help you track, measure, and shape your progress in a number of key areas.
KPIs are critical to both qualitative and quantitative analysis research. This is one of the primary methods of data analysis you certainly shouldn’t overlook.
To help you set the best possible KPIs for your initiatives and activities, here is an example of a relevant logistics KPI : transportation-related costs. If you want to see more go explore our collection of key performance indicator examples .

7. Omit useless data
Having bestowed your data analysis tools and techniques with true purpose and defined your mission, you should explore the raw data you’ve collected from all sources and use your KPIs as a reference for chopping out any information you deem to be useless.
Trimming the informational fat is one of the most crucial methods of analysis as it will allow you to focus your analytical efforts and squeeze every drop of value from the remaining ‘lean’ information.
Any stats, facts, figures, or metrics that don’t align with your business goals or fit with your KPI management strategies should be eliminated from the equation.
8. Build a data management roadmap
While, at this point, this particular step is optional (you will have already gained a wealth of insight and formed a fairly sound strategy by now), creating a data governance roadmap will help your data analysis methods and techniques become successful on a more sustainable basis. These roadmaps, if developed properly, are also built so they can be tweaked and scaled over time.
Invest ample time in developing a roadmap that will help you store, manage, and handle your data internally, and you will make your analysis techniques all the more fluid and functional – one of the most powerful types of data analysis methods available today.
9. Integrate technology
There are many ways to analyze data, but one of the most vital aspects of analytical success in a business context is integrating the right decision support software and technology.
Robust analysis platforms will not only allow you to pull critical data from your most valuable sources while working with dynamic KPIs that will offer you actionable insights; it will also present them in a digestible, visual, interactive format from one central, live dashboard . A data methodology you can count on.
By integrating the right technology within your data analysis methodology, you’ll avoid fragmenting your insights, saving you time and effort while allowing you to enjoy the maximum value from your business’s most valuable insights.
For a look at the power of software for the purpose of analysis and to enhance your methods of analyzing, glance over our selection of dashboard examples .
10. Answer your questions
By considering each of the above efforts, working with the right technology, and fostering a cohesive internal culture where everyone buys into the different ways to analyze data as well as the power of digital intelligence, you will swiftly start to answer your most burning business questions. Arguably, the best way to make your data concepts accessible across the organization is through data visualization.
11. Visualize your data
Online data visualization is a powerful tool as it lets you tell a story with your metrics, allowing users across the organization to extract meaningful insights that aid business evolution – and it covers all the different ways to analyze data.
The purpose of analyzing is to make your entire organization more informed and intelligent, and with the right platform or dashboard, this is simpler than you think, as demonstrated by our marketing dashboard .

This visual, dynamic, and interactive online dashboard is a data analysis example designed to give Chief Marketing Officers (CMO) an overview of relevant metrics to help them understand if they achieved their monthly goals.
In detail, this example generated with a modern dashboard creator displays interactive charts for monthly revenues, costs, net income, and net income per customer; all of them are compared with the previous month so that you can understand how the data fluctuated. In addition, it shows a detailed summary of the number of users, customers, SQLs, and MQLs per month to visualize the whole picture and extract relevant insights or trends for your marketing reports .
The CMO dashboard is perfect for c-level management as it can help them monitor the strategic outcome of their marketing efforts and make data-driven decisions that can benefit the company exponentially.
12. Be careful with the interpretation
We already dedicated an entire post to data interpretation as it is a fundamental part of the process of data analysis. It gives meaning to the analytical information and aims to drive a concise conclusion from the analysis results. Since most of the time companies are dealing with data from many different sources, the interpretation stage needs to be done carefully and properly in order to avoid misinterpretations.
To help you through the process, here we list three common practices that you need to avoid at all costs when looking at your data:
- Correlation vs. causation: The human brain is formatted to find patterns. This behavior leads to one of the most common mistakes when performing interpretation: confusing correlation with causation. Although these two aspects can exist simultaneously, it is not correct to assume that because two things happened together, one provoked the other. A piece of advice to avoid falling into this mistake is never to trust just intuition, trust the data. If there is no objective evidence of causation, then always stick to correlation.
- Confirmation bias: This phenomenon describes the tendency to select and interpret only the data necessary to prove one hypothesis, often ignoring the elements that might disprove it. Even if it's not done on purpose, confirmation bias can represent a real problem, as excluding relevant information can lead to false conclusions and, therefore, bad business decisions. To avoid it, always try to disprove your hypothesis instead of proving it, share your analysis with other team members, and avoid drawing any conclusions before the entire analytical project is finalized.
- Statistical significance: To put it in short words, statistical significance helps analysts understand if a result is actually accurate or if it happened because of a sampling error or pure chance. The level of statistical significance needed might depend on the sample size and the industry being analyzed. In any case, ignoring the significance of a result when it might influence decision-making can be a huge mistake.
13. Build a narrative
Now, we’re going to look at how you can bring all of these elements together in a way that will benefit your business - starting with a little something called data storytelling.
The human brain responds incredibly well to strong stories or narratives. Once you’ve cleansed, shaped, and visualized your most invaluable data using various BI dashboard tools , you should strive to tell a story - one with a clear-cut beginning, middle, and end.
By doing so, you will make your analytical efforts more accessible, digestible, and universal, empowering more people within your organization to use your discoveries to their actionable advantage.
14. Consider autonomous technology
Autonomous technologies, such as artificial intelligence (AI) and machine learning (ML), play a significant role in the advancement of understanding how to analyze data more effectively.
Gartner predicts that by the end of this year, 80% of emerging technologies will be developed with AI foundations. This is a testament to the ever-growing power and value of autonomous technologies.
At the moment, these technologies are revolutionizing the analysis industry. Some examples that we mentioned earlier are neural networks, intelligent alarms, and sentiment analysis.
15. Share the load
If you work with the right tools and dashboards, you will be able to present your metrics in a digestible, value-driven format, allowing almost everyone in the organization to connect with and use relevant data to their advantage.
Modern dashboards consolidate data from various sources, providing access to a wealth of insights in one centralized location, no matter if you need to monitor recruitment metrics or generate reports that need to be sent across numerous departments. Moreover, these cutting-edge tools offer access to dashboards from a multitude of devices, meaning that everyone within the business can connect with practical insights remotely - and share the load.
Once everyone is able to work with a data-driven mindset, you will catalyze the success of your business in ways you never thought possible. And when it comes to knowing how to analyze data, this kind of collaborative approach is essential.
16. Data analysis tools
In order to perform high-quality analysis of data, it is fundamental to use tools and software that will ensure the best results. Here we leave you a small summary of four fundamental categories of data analysis tools for your organization.
- Business Intelligence: BI tools allow you to process significant amounts of data from several sources in any format. Through this, you can not only analyze and monitor your data to extract relevant insights but also create interactive reports and dashboards to visualize your KPIs and use them for your company's good. datapine is an amazing online BI software that is focused on delivering powerful online analysis features that are accessible to beginner and advanced users. Like this, it offers a full-service solution that includes cutting-edge analysis of data, KPIs visualization, live dashboards, reporting, and artificial intelligence technologies to predict trends and minimize risk.
- Statistical analysis: These tools are usually designed for scientists, statisticians, market researchers, and mathematicians, as they allow them to perform complex statistical analyses with methods like regression analysis, predictive analysis, and statistical modeling. A good tool to perform this type of analysis is R-Studio as it offers a powerful data modeling and hypothesis testing feature that can cover both academic and general data analysis. This tool is one of the favorite ones in the industry, due to its capability for data cleaning, data reduction, and performing advanced analysis with several statistical methods. Another relevant tool to mention is SPSS from IBM. The software offers advanced statistical analysis for users of all skill levels. Thanks to a vast library of machine learning algorithms, text analysis, and a hypothesis testing approach it can help your company find relevant insights to drive better decisions. SPSS also works as a cloud service that enables you to run it anywhere.
- SQL Consoles: SQL is a programming language often used to handle structured data in relational databases. Tools like these are popular among data scientists as they are extremely effective in unlocking these databases' value. Undoubtedly, one of the most used SQL software in the market is MySQL Workbench . This tool offers several features such as a visual tool for database modeling and monitoring, complete SQL optimization, administration tools, and visual performance dashboards to keep track of KPIs.
- Data Visualization: These tools are used to represent your data through charts, graphs, and maps that allow you to find patterns and trends in the data. datapine's already mentioned BI platform also offers a wealth of powerful online data visualization tools with several benefits. Some of them include: delivering compelling data-driven presentations to share with your entire company, the ability to see your data online with any device wherever you are, an interactive dashboard design feature that enables you to showcase your results in an interactive and understandable way, and to perform online self-service reports that can be used simultaneously with several other people to enhance team productivity.
17. Refine your process constantly
Last is a step that might seem obvious to some people, but it can be easily ignored if you think you are done. Once you have extracted the needed results, you should always take a retrospective look at your project and think about what you can improve. As you saw throughout this long list of techniques, data analysis is a complex process that requires constant refinement. For this reason, you should always go one step further and keep improving.
Quality Criteria For Data Analysis
So far we’ve covered a list of methods and techniques that should help you perform efficient data analysis. But how do you measure the quality and validity of your results? This is done with the help of some science quality criteria. Here we will go into a more theoretical area that is critical to understanding the fundamentals of statistical analysis in science. However, you should also be aware of these steps in a business context, as they will allow you to assess the quality of your results in the correct way. Let’s dig in.
- Internal validity: The results of a survey are internally valid if they measure what they are supposed to measure and thus provide credible results. In other words , internal validity measures the trustworthiness of the results and how they can be affected by factors such as the research design, operational definitions, how the variables are measured, and more. For instance, imagine you are doing an interview to ask people if they brush their teeth two times a day. While most of them will answer yes, you can still notice that their answers correspond to what is socially acceptable, which is to brush your teeth at least twice a day. In this case, you can’t be 100% sure if respondents actually brush their teeth twice a day or if they just say that they do, therefore, the internal validity of this interview is very low.
- External validity: Essentially, external validity refers to the extent to which the results of your research can be applied to a broader context. It basically aims to prove that the findings of a study can be applied in the real world. If the research can be applied to other settings, individuals, and times, then the external validity is high.
- Reliability : If your research is reliable, it means that it can be reproduced. If your measurement were repeated under the same conditions, it would produce similar results. This means that your measuring instrument consistently produces reliable results. For example, imagine a doctor building a symptoms questionnaire to detect a specific disease in a patient. Then, various other doctors use this questionnaire but end up diagnosing the same patient with a different condition. This means the questionnaire is not reliable in detecting the initial disease. Another important note here is that in order for your research to be reliable, it also needs to be objective. If the results of a study are the same, independent of who assesses them or interprets them, the study can be considered reliable. Let’s see the objectivity criteria in more detail now.
- Objectivity: In data science, objectivity means that the researcher needs to stay fully objective when it comes to its analysis. The results of a study need to be affected by objective criteria and not by the beliefs, personality, or values of the researcher. Objectivity needs to be ensured when you are gathering the data, for example, when interviewing individuals, the questions need to be asked in a way that doesn't influence the results. Paired with this, objectivity also needs to be thought of when interpreting the data. If different researchers reach the same conclusions, then the study is objective. For this last point, you can set predefined criteria to interpret the results to ensure all researchers follow the same steps.
The discussed quality criteria cover mostly potential influences in a quantitative context. Analysis in qualitative research has by default additional subjective influences that must be controlled in a different way. Therefore, there are other quality criteria for this kind of research such as credibility, transferability, dependability, and confirmability. You can see each of them more in detail on this resource .
Data Analysis Limitations & Barriers
Analyzing data is not an easy task. As you’ve seen throughout this post, there are many steps and techniques that you need to apply in order to extract useful information from your research. While a well-performed analysis can bring various benefits to your organization it doesn't come without limitations. In this section, we will discuss some of the main barriers you might encounter when conducting an analysis. Let’s see them more in detail.
- Lack of clear goals: No matter how good your data or analysis might be if you don’t have clear goals or a hypothesis the process might be worthless. While we mentioned some methods that don’t require a predefined hypothesis, it is always better to enter the analytical process with some clear guidelines of what you are expecting to get out of it, especially in a business context in which data is utilized to support important strategic decisions.
- Objectivity: Arguably one of the biggest barriers when it comes to data analysis in research is to stay objective. When trying to prove a hypothesis, researchers might find themselves, intentionally or unintentionally, directing the results toward an outcome that they want. To avoid this, always question your assumptions and avoid confusing facts with opinions. You can also show your findings to a research partner or external person to confirm that your results are objective.
- Data representation: A fundamental part of the analytical procedure is the way you represent your data. You can use various graphs and charts to represent your findings, but not all of them will work for all purposes. Choosing the wrong visual can not only damage your analysis but can mislead your audience, therefore, it is important to understand when to use each type of data depending on your analytical goals. Our complete guide on the types of graphs and charts lists 20 different visuals with examples of when to use them.
- Flawed correlation : Misleading statistics can significantly damage your research. We’ve already pointed out a few interpretation issues previously in the post, but it is an important barrier that we can't avoid addressing here as well. Flawed correlations occur when two variables appear related to each other but they are not. Confusing correlations with causation can lead to a wrong interpretation of results which can lead to building wrong strategies and loss of resources, therefore, it is very important to identify the different interpretation mistakes and avoid them.
- Sample size: A very common barrier to a reliable and efficient analysis process is the sample size. In order for the results to be trustworthy, the sample size should be representative of what you are analyzing. For example, imagine you have a company of 1000 employees and you ask the question “do you like working here?” to 50 employees of which 49 say yes, which means 95%. Now, imagine you ask the same question to the 1000 employees and 950 say yes, which also means 95%. Saying that 95% of employees like working in the company when the sample size was only 50 is not a representative or trustworthy conclusion. The significance of the results is way more accurate when surveying a bigger sample size.
- Privacy concerns: In some cases, data collection can be subjected to privacy regulations. Businesses gather all kinds of information from their customers from purchasing behaviors to addresses and phone numbers. If this falls into the wrong hands due to a breach, it can affect the security and confidentiality of your clients. To avoid this issue, you need to collect only the data that is needed for your research and, if you are using sensitive facts, make it anonymous so customers are protected. The misuse of customer data can severely damage a business's reputation, so it is important to keep an eye on privacy.
- Lack of communication between teams : When it comes to performing data analysis on a business level, it is very likely that each department and team will have different goals and strategies. However, they are all working for the same common goal of helping the business run smoothly and keep growing. When teams are not connected and communicating with each other, it can directly affect the way general strategies are built. To avoid these issues, tools such as data dashboards enable teams to stay connected through data in a visually appealing way.
- Innumeracy : Businesses are working with data more and more every day. While there are many BI tools available to perform effective analysis, data literacy is still a constant barrier. Not all employees know how to apply analysis techniques or extract insights from them. To prevent this from happening, you can implement different training opportunities that will prepare every relevant user to deal with data.
Key Data Analysis Skills
As you've learned throughout this lengthy guide, analyzing data is a complex task that requires a lot of knowledge and skills. That said, thanks to the rise of self-service tools the process is way more accessible and agile than it once was. Regardless, there are still some key skills that are valuable to have when working with data, we list the most important ones below.
- Critical and statistical thinking: To successfully analyze data you need to be creative and think out of the box. Yes, that might sound like a weird statement considering that data is often tight to facts. However, a great level of critical thinking is required to uncover connections, come up with a valuable hypothesis, and extract conclusions that go a step further from the surface. This, of course, needs to be complemented by statistical thinking and an understanding of numbers.
- Data cleaning: Anyone who has ever worked with data before will tell you that the cleaning and preparation process accounts for 80% of a data analyst's work, therefore, the skill is fundamental. But not just that, not cleaning the data adequately can also significantly damage the analysis which can lead to poor decision-making in a business scenario. While there are multiple tools that automate the cleaning process and eliminate the possibility of human error, it is still a valuable skill to dominate.
- Data visualization: Visuals make the information easier to understand and analyze, not only for professional users but especially for non-technical ones. Having the necessary skills to not only choose the right chart type but know when to apply it correctly is key. This also means being able to design visually compelling charts that make the data exploration process more efficient.
- SQL: The Structured Query Language or SQL is a programming language used to communicate with databases. It is fundamental knowledge as it enables you to update, manipulate, and organize data from relational databases which are the most common databases used by companies. It is fairly easy to learn and one of the most valuable skills when it comes to data analysis.
- Communication skills: This is a skill that is especially valuable in a business environment. Being able to clearly communicate analytical outcomes to colleagues is incredibly important, especially when the information you are trying to convey is complex for non-technical people. This applies to in-person communication as well as written format, for example, when generating a dashboard or report. While this might be considered a “soft” skill compared to the other ones we mentioned, it should not be ignored as you most likely will need to share analytical findings with others no matter the context.
Data Analysis In The Big Data Environment
Big data is invaluable to today’s businesses, and by using different methods for data analysis, it’s possible to view your data in a way that can help you turn insight into positive action.
To inspire your efforts and put the importance of big data into context, here are some insights that you should know:
- By 2026 the industry of big data is expected to be worth approximately $273.4 billion.
- 94% of enterprises say that analyzing data is important for their growth and digital transformation.
- Companies that exploit the full potential of their data can increase their operating margins by 60% .
- We already told you the benefits of Artificial Intelligence through this article. This industry's financial impact is expected to grow up to $40 billion by 2025.
Data analysis concepts may come in many forms, but fundamentally, any solid methodology will help to make your business more streamlined, cohesive, insightful, and successful than ever before.
Key Takeaways From Data Analysis
As we reach the end of our data analysis journey, we leave a small summary of the main methods and techniques to perform excellent analysis and grow your business.
17 Essential Types of Data Analysis Methods:
- Cluster analysis
- Cohort analysis
- Regression analysis
- Factor analysis
- Neural Networks
- Data Mining
- Text analysis
- Time series analysis
- Decision trees
- Conjoint analysis
- Correspondence Analysis
- Multidimensional Scaling
- Content analysis
- Thematic analysis
- Narrative analysis
- Grounded theory analysis
- Discourse analysis
Top 17 Data Analysis Techniques:
- Collaborate your needs
- Establish your questions
- Data democratization
- Think of data governance
- Clean your data
- Set your KPIs
- Omit useless data
- Build a data management roadmap
- Integrate technology
- Answer your questions
- Visualize your data
- Interpretation of data
- Consider autonomous technology
- Build a narrative
- Share the load
- Data Analysis tools
- Refine your process constantly
We’ve pondered the data analysis definition and drilled down into the practical applications of data-centric analytics, and one thing is clear: by taking measures to arrange your data and making your metrics work for you, it’s possible to transform raw information into action - the kind of that will push your business to the next level.
Yes, good data analytics techniques result in enhanced business intelligence (BI). To help you understand this notion in more detail, read our exploration of business intelligence reporting .
And, if you’re ready to perform your own analysis, drill down into your facts and figures while interacting with your data on astonishing visuals, you can try our software for a free, 14-day trial .
The 4 Types of Data Analysis [Ultimate Guide]
The most successful businesses and organizations are those that constantly learn and adapt.
No matter what industry you’re operating in, it’s essential to understand what has happened in the past, what’s going on now, and to anticipate what might happen in the future. So how do companies do that?
The answer lies in data analytics . Most companies are collecting data all the time—but, in its raw form, this data doesn’t really mean anything. It’s what you do with the data that counts. Data analytics is the process of analyzing raw data in order to draw out patterns, trends, and insights that can tell you something meaningful about a particular area of the business. These insights are then used to make smart, data-driven decisions.
The kinds of insights you get from your data depends on the type of analysis you perform. In data analytics and data science, there are four main types of data analysis: Descriptive , diagnostic , predictive , and prescriptive .
In this post, we’ll explain each of the four and consider why they’re useful. If you’re interested in a particular type of analysis, jump straight to the relevant section using the clickable menu below.
- Types of data analysis: Descriptive
- Types of data analysis: Diagnostic
- Types of data analysis: Predictive
- Types of data analysis: Prescriptive
- Key takeaways and further reading
So, what are the four main types of data analysis? Let’s find out.
1. Types of data analysis: Descriptive (What happened?)
Descriptive analytics looks at what has happened in the past.
As the name suggests, the purpose of descriptive analytics is to simply describe what has happened; it doesn’t try to explain why this might have happened or to establish cause-and-effect relationships. The aim is solely to provide an easily digestible snapshot.
Google Analytics is a good example of descriptive analytics in action; it provides a simple overview of what’s been going on with your website, showing you how many people visited in a given time period, for example, or where your visitors came from. Similarly, tools like HubSpot will show you how many people opened a particular email or engaged with a certain campaign.
There are two main techniques used in descriptive analytics: Data aggregation and data mining.
Data aggregation
Data aggregation is the process of gathering data and presenting it in a summarized format.
Let’s imagine an ecommerce company collects all kinds of data relating to their customers and people who visit their website. The aggregate data, or summarized data, would provide an overview of this wider dataset—such as the average customer age, for example, or the average number of purchases made.
Data mining
Data mining is the analysis part . This is when the analyst explores the data in order to uncover any patterns or trends. The outcome of descriptive analysis is a visual representation of the data—as a bar graph, for example, or a pie chart.
So: Descriptive analytics condenses large volumes of data into a clear, simple overview of what has happened. This is often the starting point for more in-depth analysis, as we’ll now explore.
2. Types of data analysis: Diagnostic (Why did it happen?)
Diagnostic analytics seeks to delve deeper in order to understand why something happened. The main purpose of diagnostic analytics is to identify and respond to anomalies within your data . For example: If your descriptive analysis shows that there was a 20% drop in sales for the month of March, you’ll want to find out why. The next logical step is to perform a diagnostic analysis.
In order to get to the root cause, the analyst will start by identifying any additional data sources that might offer further insight into why the drop in sales occurred. They might drill down to find that, despite a healthy volume of website visitors and a good number of “add to cart” actions, very few customers proceeded to actually check out and make a purchase.
Upon further inspection, it comes to light that the majority of customers abandoned ship at the point of filling out their delivery address. Now we’re getting somewhere! It’s starting to look like there was a problem with the address form; perhaps it wasn’t loading properly on mobile, or was simply too long and frustrating. With a little bit of digging, you’re closer to finding an explanation for your data anomaly.
Diagnostic analytics isn’t just about fixing problems, though; you can also use it to see what’s driving positive results. Perhaps the data tells you that website traffic was through the roof in October—a whopping 60% increase compared to the previous month! When you drill down, it seems that this spike in traffic corresponds to a celebrity mentioning one of your skincare products in their Instagram story.
This opens your eyes to the power of influencer marketing , giving you something to think about for your future marketing strategy.
When running diagnostic analytics, there are a number of different techniques that you might employ, such as probability theory, regression analysis, filtering, and time-series analysis. You can learn more about each of these techniques in our introduction to data analytics .
So: While descriptive analytics looks at what happened, diagnostic analytics explores why it happened.
3. Types of data analysis: Predictive (What is likely to happen in the future?)
Predictive analytics seeks to predict what is likely to happen in the future. Based on past patterns and trends, data analysts can devise predictive models which estimate the likelihood of a future event or outcome. This is especially useful as it enables businesses to plan ahead.
Predictive models use the relationship between a set of variables to make predictions; for example, you might use the correlation between seasonality and sales figures to predict when sales are likely to drop. If your predictive model tells you that sales are likely to go down in summer, you might use this information to come up with a summer-related promotional campaign, or to decrease expenditure elsewhere to make up for the seasonal dip.
Perhaps you own a restaurant and want to predict how many takeaway orders you’re likely to get on a typical Saturday night. Based on what your predictive model tells you, you might decide to get an extra delivery driver on hand.
In addition to forecasting, predictive analytics is also used for classification. A commonly used classification algorithm is logistic regression, which is used to predict a binary outcome based on a set of independent variables. For example: A credit card company might use a predictive model, and specifically logistic regression, to predict whether or not a given customer will default on their payments—in other words, to classify them in one of two categories: “will default” or “will not default”.
Based on these predictions of what category the customer will fall into, the company can quickly assess who might be a good candidate for a credit card. You can learn more about logistic regression and other types of regression analysis .
Machine learning (ML)
Machine learning is a branch of predictive analytics. Just as humans use predictive analytics to devise models and forecast future outcomes, machine learning models are designed to recognize patterns in the data and automatically evolve in order to make accurate predictions. If you’re interested in learning more, there are some useful guides to the similarities and differences between (human-led) predictive analytics and machine learning .
Learn more in our full guide to machine learning .
As you can see, predictive analytics is used to forecast all sorts of future outcomes, and while it can never be one hundred percent accurate, it does eliminate much of the guesswork. This is crucial when it comes to making business decisions and determining the most appropriate course of action.
So: Predictive analytics builds on what happened in the past and why to predict what is likely to happen in the future.
4. Types of data analysis: Prescriptive (What’s the best course of action?)
Prescriptive analytics looks at what has happened, why it happened, and what might happen in order to determine what should be done next.
In other words, prescriptive analytics shows you how you can best take advantage of the future outcomes that have been predicted. What steps can you take to avoid a future problem? What can you do to capitalize on an emerging trend?
Prescriptive analytics is, without doubt, the most complex type of analysis, involving algorithms, machine learning, statistical methods, and computational modeling procedures. Essentially, a prescriptive model considers all the possible decision patterns or pathways a company might take, and their likely outcomes.
This enables you to see how each combination of conditions and decisions might impact the future, and allows you to measure the impact a certain decision might have. Based on all the possible scenarios and potential outcomes, the company can decide what is the best “route” or action to take.
An oft-cited example of prescriptive analytics in action is maps and traffic apps. When figuring out the best way to get you from A to B, Google Maps will consider all the possible modes of transport (e.g. bus, walking, or driving), the current traffic conditions and possible roadworks in order to calculate the best route. In much the same way, prescriptive models are used to calculate all the possible “routes” a company might take to reach their goals in order to determine the best possible option.
Knowing what actions to take for the best chances of success is a major advantage for any type of organization, so it’s no wonder that prescriptive analytics has a huge role to play in business.
So: Prescriptive analytics looks at what has happened, why it happened, and what might happen in order to determine the best course of action for the future.
5. Key takeaways and further reading
In some ways, data analytics is a bit like a treasure hunt; based on clues and insights from the past, you can work out what your next move should be.
With the right type of analysis, all kinds of businesses and organizations can use their data to make smarter decisions, invest more wisely, improve internal processes, and ultimately increase their chances of success. To summarize, there are four main types of data analysis to be aware of:
- Descriptive analytics: What happened?
- Diagnostic analytics: Why did it happen?
- Predictive analytics: What is likely to happen in the future?
- Prescriptive analytics: What is the best course of action to take?
Now you’re familiar with the different types of data analysis, you can start to explore specific analysis techniques, such as time series analysis, cohort analysis, and regression—to name just a few! We explore some of the most useful data analysis techniques in this guide .
If you’re not already familiar, it’s also worth learning about the different levels of measurement (nominal, ordinal, interval, and ratio) for data .
Ready for a hands-on introduction to the field? Give this free, five-day data analytics short course a go! And, if you’d like to learn more, check out some of these excellent free courses for beginners . Then, to see what it takes to start a career in the field, check out the following:
- How to become a data analyst: Your five-step plan
- What are the key skills every data analyst needs?
- What’s it actually like to work as a data analyst?
Types of Data Analysis
Analysis of data is a vital part of running a successful business. When data is used effectively, it leads to better understanding of a business’s previous performance and better decision-making for its future activities. There are many ways that data can be utilized, at all levels of a company’s operations.
There are four types of data analysis that are in use across all industries. While we separate these into categories, they are all linked together and build upon each other. As you begin moving from the simplest type of analytics to more complex, the degree of difficulty and resources required increases. At the same time, the level of added insight and value also increases.
Four Types of Data Analysis
The four types of data analysis are:
Descriptive Analysis
Diagnostic analysis, predictive analysis, prescriptive analysis.
Below, we will introduce each type and give examples of how they are utilized in business.
The first type of data analysis is descriptive analysis. It is at the foundation of all data insight. It is the simplest and most common use of data in business today. Descriptive analysis answers the “what happened” by summarizing past data, usually in the form of dashboards.
The biggest use of descriptive analysis in business is to track Key Performance Indicators (KPIs). KPIs describe how a business is performing based on chosen benchmarks.
Business applications of descriptive analysis include:
- KPI dashboards
- Monthly revenue reports
- Sales leads overview
After asking the main question of “what happened”, the next step is to dive deeper and ask why did it happen? This is where diagnostic analysis comes in.
Diagnostic analysis takes the insights found from descriptive analytics and drills down to find the causes of those outcomes. Organizations make use of this type of analytics as it creates more connections between data and identifies patterns of behavior.
A critical aspect of diagnostic analysis is creating detailed information. When new problems arise, it is possible you have already collected certain data pertaining to the issue. By already having the data at your disposal, it ends having to repeat work and makes all problems interconnected.
Business applications of diagnostic analysis include:
- A freight company investigating the cause of slow shipments in a certain region
- A SaaS company drilling down to determine which marketing activities increased trials
Predictive analysis attempts to answer the question “what is likely to happen”. This type of analytics utilizes previous data to make predictions about future outcomes.
This type of analysis is another step up from the descriptive and diagnostic analyses. Predictive analysis uses the data we have summarized to make logical predictions of the outcomes of events. This analysis relies on statistical modeling, which requires added technology and manpower to forecast. It is also important to understand that forecasting is only an estimate; the accuracy of predictions relies on quality and detailed data.
While descriptive and diagnostic analysis are common practices in business, predictive analysis is where many organizations begin show signs of difficulty. Some companies do not have the manpower to implement predictive analysis in every place they desire. Others are not yet willing to invest in analysis teams across every department or not prepared to educate current teams.
Business applications of predictive analysis include:
- Risk Assessment
- Sales Forecasting
- Using customer segmentation to determine which leads have the best chance of converting
- Predictive analytics in customer success teams
The final type of data analysis is the most sought after, but few organizations are truly equipped to perform it. Prescriptive analysis is the frontier of data analysis, combining the insight from all previous analyses to determine the course of action to take in a current problem or decision.
Prescriptive analysis utilizes state of the art technology and data practices. It is a huge organizational commitment and companies must be sure that they are ready and willing to put forth the effort and resources.
Artificial Intelligence (AI) is a perfect example of prescriptive analytics. AI systems consume a large amount of data to continuously learn and use this information to make informed decisions. Well-designed AI systems are capable of communicating these decisions and even putting those decisions into action. Business processes can be performed and optimized daily without a human doing anything with artificial intelligence.
Currently, most of the big data-driven companies (Apple, Facebook, Netflix, etc.) are utilizing prescriptive analytics and AI to improve decision making. For other organizations, the jump to predictive and prescriptive analytics can be insurmountable. As technology continues to improve and more professionals are educated in data, we will see more companies entering the data-driven realm.
As we have shown, each of these types of data analysis are connected and rely on each other to a certain degree. They each serve a different purpose and provide varying insights. Moving from descriptive analysis towards predictive and prescriptive analysis requires much more technical ability, but also unlocks more insight for your organization.
- Journal of Accountancy – The next frontier in data analytics
- ScienceSoft – 4 Types of Data Analytics to Improve Decision-Making
- Ingram Micro – Four Types of Big Data Analytics and Examples of Their Use
similar articles
What is ad hoc analysis and how does it work.
Ad hoc analysis (aka ad hoc reporting) is the process of using business data to find specific answers to in-the-moment, often one-off, questions. It introduces flexibility and spontaneity to the traditionally rigid process of BI reporting (occasionally at the expense of accuracy).
Where to Find Free Datasets & How to Know if They're Good Quality
There is a lot of free data out there, ready for you to use for school projects, for market research, or just for fun. Before you get too crazy, though, you need to be aware of the quality of the data you find. Here are a few great sources for free data and a few ways to determine their quality.
Distinguishing Data Roles: Engineers, Analysts, and Scientists
Learn about the responsibilities that data engineers, analysts, scientists, and other related 'data' roles have on a data team.
Data Analysis
- Introduction to Data Analysis
- Quantitative Analysis Tools
- Qualitative Analysis Tools
- Mixed Methods Analysis
- Geospatial Analysis
- Further Reading
What is Data Analysis?
According to the federal government, data analysis is "the process of systematically applying statistical and/or logical techniques to describe and illustrate, condense and recap, and evaluate data" ( Responsible Conduct in Data Management ). Important components of data analysis include searching for patterns, remaining unbiased in drawing inference from data, practicing responsible data management , and maintaining "honest and accurate analysis" ( Responsible Conduct in Data Management ).
In order to understand data analysis further, it can be helpful to take a step back and understand the question "What is data?". Many of us associate data with spreadsheets of numbers and values, however, data can encompass much more than that. According to the federal government, data is "The recorded factual material commonly accepted in the scientific community as necessary to validate research findings" ( OMB Circular 110 ). This broad definition can include information in many formats.
Some examples of types of data are as follows:
- Photographs
- Hand-written notes from field observation
- Machine learning training data sets
- Ethnographic interview transcripts
- Sheet music
- Scripts for plays and musicals
- Observations from laboratory experiments ( CMU Data 101 )
Thus, data analysis includes the processing and manipulation of these data sources in order to gain additional insight from data, answer a research question, or confirm a research hypothesis.
Data analysis falls within the larger research data lifecycle, as seen below.
( University of Virginia )
Why Analyze Data?
Through data analysis, a researcher can gain additional insight from data and draw conclusions to address the research question or hypothesis. Use of data analysis tools helps researchers understand and interpret data.
What are the Types of Data Analysis?
Data analysis can be quantitative, qualitative, or mixed methods.
Quantitative research typically involves numbers and "close-ended questions and responses" ( Creswell & Creswell, 2018 , p. 3). Quantitative research tests variables against objective theories, usually measured and collected on instruments and analyzed using statistical procedures ( Creswell & Creswell, 2018 , p. 4). Quantitative analysis usually uses deductive reasoning.
Qualitative research typically involves words and "open-ended questions and responses" ( Creswell & Creswell, 2018 , p. 3). According to Creswell & Creswell, "qualitative research is an approach for exploring and understanding the meaning individuals or groups ascribe to a social or human problem" ( 2018 , p. 4). Thus, qualitative analysis usually invokes inductive reasoning.
Mixed methods research uses methods from both quantitative and qualitative research approaches. Mixed methods research works under the "core assumption... that the integration of qualitative and quantitative data yields additional insight beyond the information provided by either the quantitative or qualitative data alone" ( Creswell & Creswell, 2018 , p. 4).
- Next: Planning >>
- Last Updated: Apr 2, 2024 3:53 PM
- URL: https://guides.library.georgetown.edu/data-analysis

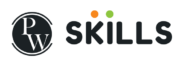
Data Analysis Techniques in Research – Methods, Tools & Examples
By Varun Saharawat | January 22, 2024
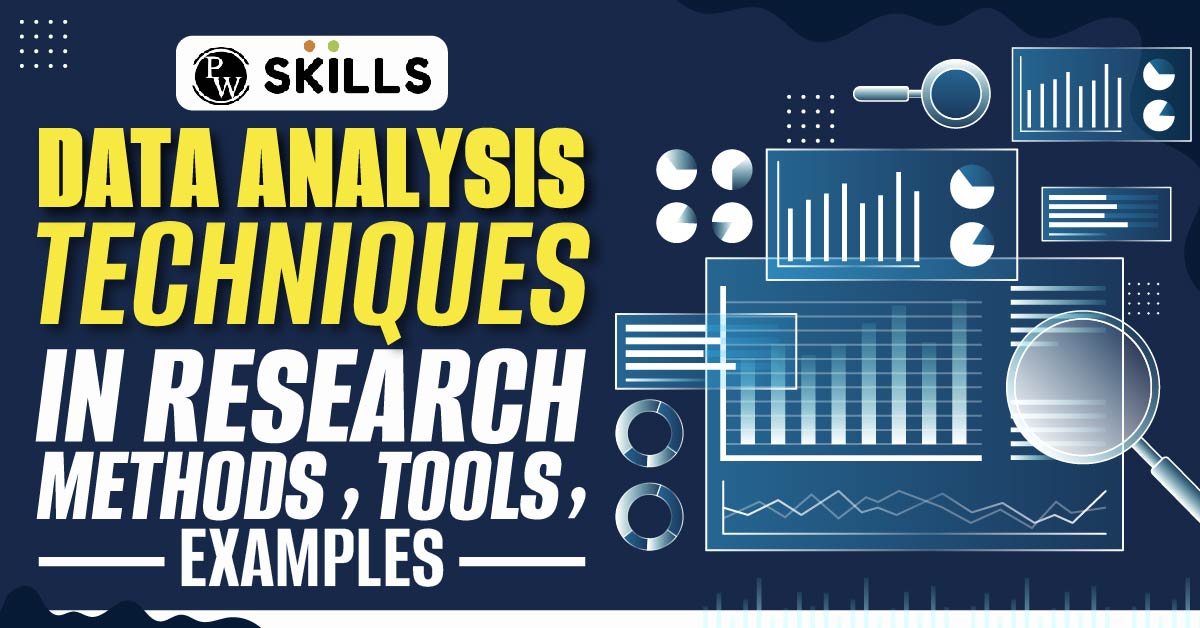
Data analysis techniques in research are essential because they allow researchers to derive meaningful insights from data sets to support their hypotheses or research objectives.
Data Analysis Techniques in Research : While various groups, institutions, and professionals may have diverse approaches to data analysis, a universal definition captures its essence. Data analysis involves refining, transforming, and interpreting raw data to derive actionable insights that guide informed decision-making for businesses.
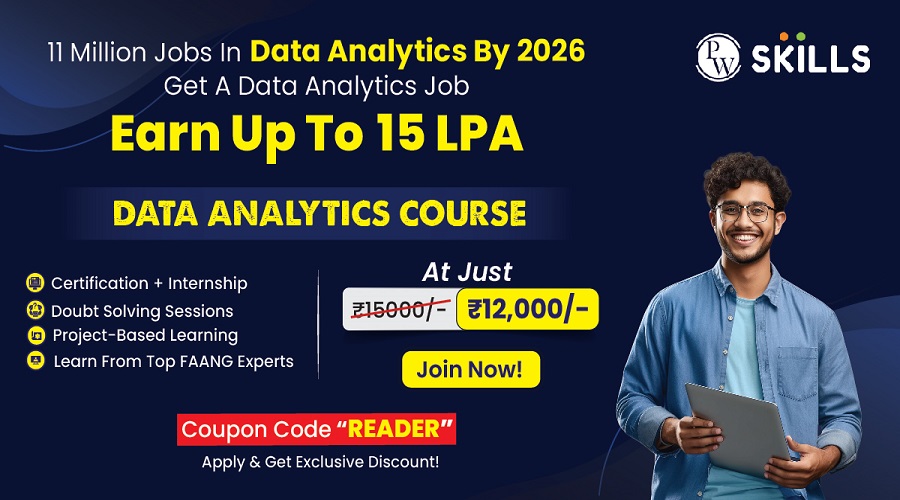
A straightforward illustration of data analysis emerges when we make everyday decisions, basing our choices on past experiences or predictions of potential outcomes.
If you want to learn more about this topic and acquire valuable skills that will set you apart in today’s data-driven world, we highly recommend enrolling in the Data Analytics Course by Physics Wallah . And as a special offer for our readers, use the coupon code “READER” to get a discount on this course.
Table of Contents
What is Data Analysis?
Data analysis is the systematic process of inspecting, cleaning, transforming, and interpreting data with the objective of discovering valuable insights and drawing meaningful conclusions. This process involves several steps:
- Inspecting : Initial examination of data to understand its structure, quality, and completeness.
- Cleaning : Removing errors, inconsistencies, or irrelevant information to ensure accurate analysis.
- Transforming : Converting data into a format suitable for analysis, such as normalization or aggregation.
- Interpreting : Analyzing the transformed data to identify patterns, trends, and relationships.
Types of Data Analysis Techniques in Research
Data analysis techniques in research are categorized into qualitative and quantitative methods, each with its specific approaches and tools. These techniques are instrumental in extracting meaningful insights, patterns, and relationships from data to support informed decision-making, validate hypotheses, and derive actionable recommendations. Below is an in-depth exploration of the various types of data analysis techniques commonly employed in research:
1) Qualitative Analysis:
Definition: Qualitative analysis focuses on understanding non-numerical data, such as opinions, concepts, or experiences, to derive insights into human behavior, attitudes, and perceptions.
- Content Analysis: Examines textual data, such as interview transcripts, articles, or open-ended survey responses, to identify themes, patterns, or trends.
- Narrative Analysis: Analyzes personal stories or narratives to understand individuals’ experiences, emotions, or perspectives.
- Ethnographic Studies: Involves observing and analyzing cultural practices, behaviors, and norms within specific communities or settings.
2) Quantitative Analysis:
Quantitative analysis emphasizes numerical data and employs statistical methods to explore relationships, patterns, and trends. It encompasses several approaches:
Descriptive Analysis:
- Frequency Distribution: Represents the number of occurrences of distinct values within a dataset.
- Central Tendency: Measures such as mean, median, and mode provide insights into the central values of a dataset.
- Dispersion: Techniques like variance and standard deviation indicate the spread or variability of data.
Diagnostic Analysis:
- Regression Analysis: Assesses the relationship between dependent and independent variables, enabling prediction or understanding causality.
- ANOVA (Analysis of Variance): Examines differences between groups to identify significant variations or effects.
Predictive Analysis:
- Time Series Forecasting: Uses historical data points to predict future trends or outcomes.
- Machine Learning Algorithms: Techniques like decision trees, random forests, and neural networks predict outcomes based on patterns in data.
Prescriptive Analysis:
- Optimization Models: Utilizes linear programming, integer programming, or other optimization techniques to identify the best solutions or strategies.
- Simulation: Mimics real-world scenarios to evaluate various strategies or decisions and determine optimal outcomes.
Specific Techniques:
- Monte Carlo Simulation: Models probabilistic outcomes to assess risk and uncertainty.
- Factor Analysis: Reduces the dimensionality of data by identifying underlying factors or components.
- Cohort Analysis: Studies specific groups or cohorts over time to understand trends, behaviors, or patterns within these groups.
- Cluster Analysis: Classifies objects or individuals into homogeneous groups or clusters based on similarities or attributes.
- Sentiment Analysis: Uses natural language processing and machine learning techniques to determine sentiment, emotions, or opinions from textual data.
Also Read: AI and Predictive Analytics: Examples, Tools, Uses, Ai Vs Predictive Analytics
Data Analysis Techniques in Research Examples
To provide a clearer understanding of how data analysis techniques are applied in research, let’s consider a hypothetical research study focused on evaluating the impact of online learning platforms on students’ academic performance.
Research Objective:
Determine if students using online learning platforms achieve higher academic performance compared to those relying solely on traditional classroom instruction.
Data Collection:
- Quantitative Data: Academic scores (grades) of students using online platforms and those using traditional classroom methods.
- Qualitative Data: Feedback from students regarding their learning experiences, challenges faced, and preferences.
Data Analysis Techniques Applied:
1) Descriptive Analysis:
- Calculate the mean, median, and mode of academic scores for both groups.
- Create frequency distributions to represent the distribution of grades in each group.
2) Diagnostic Analysis:
- Conduct an Analysis of Variance (ANOVA) to determine if there’s a statistically significant difference in academic scores between the two groups.
- Perform Regression Analysis to assess the relationship between the time spent on online platforms and academic performance.
3) Predictive Analysis:
- Utilize Time Series Forecasting to predict future academic performance trends based on historical data.
- Implement Machine Learning algorithms to develop a predictive model that identifies factors contributing to academic success on online platforms.
4) Prescriptive Analysis:
- Apply Optimization Models to identify the optimal combination of online learning resources (e.g., video lectures, interactive quizzes) that maximize academic performance.
- Use Simulation Techniques to evaluate different scenarios, such as varying student engagement levels with online resources, to determine the most effective strategies for improving learning outcomes.
5) Specific Techniques:
- Conduct Factor Analysis on qualitative feedback to identify common themes or factors influencing students’ perceptions and experiences with online learning.
- Perform Cluster Analysis to segment students based on their engagement levels, preferences, or academic outcomes, enabling targeted interventions or personalized learning strategies.
- Apply Sentiment Analysis on textual feedback to categorize students’ sentiments as positive, negative, or neutral regarding online learning experiences.
By applying a combination of qualitative and quantitative data analysis techniques, this research example aims to provide comprehensive insights into the effectiveness of online learning platforms.
Also Read: Learning Path to Become a Data Analyst in 2024
Data Analysis Techniques in Quantitative Research
Quantitative research involves collecting numerical data to examine relationships, test hypotheses, and make predictions. Various data analysis techniques are employed to interpret and draw conclusions from quantitative data. Here are some key data analysis techniques commonly used in quantitative research:
1) Descriptive Statistics:
- Description: Descriptive statistics are used to summarize and describe the main aspects of a dataset, such as central tendency (mean, median, mode), variability (range, variance, standard deviation), and distribution (skewness, kurtosis).
- Applications: Summarizing data, identifying patterns, and providing initial insights into the dataset.
2) Inferential Statistics:
- Description: Inferential statistics involve making predictions or inferences about a population based on a sample of data. This technique includes hypothesis testing, confidence intervals, t-tests, chi-square tests, analysis of variance (ANOVA), regression analysis, and correlation analysis.
- Applications: Testing hypotheses, making predictions, and generalizing findings from a sample to a larger population.
3) Regression Analysis:
- Description: Regression analysis is a statistical technique used to model and examine the relationship between a dependent variable and one or more independent variables. Linear regression, multiple regression, logistic regression, and nonlinear regression are common types of regression analysis .
- Applications: Predicting outcomes, identifying relationships between variables, and understanding the impact of independent variables on the dependent variable.
4) Correlation Analysis:
- Description: Correlation analysis is used to measure and assess the strength and direction of the relationship between two or more variables. The Pearson correlation coefficient, Spearman rank correlation coefficient, and Kendall’s tau are commonly used measures of correlation.
- Applications: Identifying associations between variables and assessing the degree and nature of the relationship.
5) Factor Analysis:
- Description: Factor analysis is a multivariate statistical technique used to identify and analyze underlying relationships or factors among a set of observed variables. It helps in reducing the dimensionality of data and identifying latent variables or constructs.
- Applications: Identifying underlying factors or constructs, simplifying data structures, and understanding the underlying relationships among variables.
6) Time Series Analysis:
- Description: Time series analysis involves analyzing data collected or recorded over a specific period at regular intervals to identify patterns, trends, and seasonality. Techniques such as moving averages, exponential smoothing, autoregressive integrated moving average (ARIMA), and Fourier analysis are used.
- Applications: Forecasting future trends, analyzing seasonal patterns, and understanding time-dependent relationships in data.
7) ANOVA (Analysis of Variance):
- Description: Analysis of variance (ANOVA) is a statistical technique used to analyze and compare the means of two or more groups or treatments to determine if they are statistically different from each other. One-way ANOVA, two-way ANOVA, and MANOVA (Multivariate Analysis of Variance) are common types of ANOVA.
- Applications: Comparing group means, testing hypotheses, and determining the effects of categorical independent variables on a continuous dependent variable.
8) Chi-Square Tests:
- Description: Chi-square tests are non-parametric statistical tests used to assess the association between categorical variables in a contingency table. The Chi-square test of independence, goodness-of-fit test, and test of homogeneity are common chi-square tests.
- Applications: Testing relationships between categorical variables, assessing goodness-of-fit, and evaluating independence.
These quantitative data analysis techniques provide researchers with valuable tools and methods to analyze, interpret, and derive meaningful insights from numerical data. The selection of a specific technique often depends on the research objectives, the nature of the data, and the underlying assumptions of the statistical methods being used.
Also Read: Analysis vs. Analytics: How Are They Different?
Data Analysis Methods
Data analysis methods refer to the techniques and procedures used to analyze, interpret, and draw conclusions from data. These methods are essential for transforming raw data into meaningful insights, facilitating decision-making processes, and driving strategies across various fields. Here are some common data analysis methods:
- Description: Descriptive statistics summarize and organize data to provide a clear and concise overview of the dataset. Measures such as mean, median, mode, range, variance, and standard deviation are commonly used.
- Description: Inferential statistics involve making predictions or inferences about a population based on a sample of data. Techniques such as hypothesis testing, confidence intervals, and regression analysis are used.
3) Exploratory Data Analysis (EDA):
- Description: EDA techniques involve visually exploring and analyzing data to discover patterns, relationships, anomalies, and insights. Methods such as scatter plots, histograms, box plots, and correlation matrices are utilized.
- Applications: Identifying trends, patterns, outliers, and relationships within the dataset.
4) Predictive Analytics:
- Description: Predictive analytics use statistical algorithms and machine learning techniques to analyze historical data and make predictions about future events or outcomes. Techniques such as regression analysis, time series forecasting, and machine learning algorithms (e.g., decision trees, random forests, neural networks) are employed.
- Applications: Forecasting future trends, predicting outcomes, and identifying potential risks or opportunities.
5) Prescriptive Analytics:
- Description: Prescriptive analytics involve analyzing data to recommend actions or strategies that optimize specific objectives or outcomes. Optimization techniques, simulation models, and decision-making algorithms are utilized.
- Applications: Recommending optimal strategies, decision-making support, and resource allocation.
6) Qualitative Data Analysis:
- Description: Qualitative data analysis involves analyzing non-numerical data, such as text, images, videos, or audio, to identify themes, patterns, and insights. Methods such as content analysis, thematic analysis, and narrative analysis are used.
- Applications: Understanding human behavior, attitudes, perceptions, and experiences.
7) Big Data Analytics:
- Description: Big data analytics methods are designed to analyze large volumes of structured and unstructured data to extract valuable insights. Technologies such as Hadoop, Spark, and NoSQL databases are used to process and analyze big data.
- Applications: Analyzing large datasets, identifying trends, patterns, and insights from big data sources.
8) Text Analytics:
- Description: Text analytics methods involve analyzing textual data, such as customer reviews, social media posts, emails, and documents, to extract meaningful information and insights. Techniques such as sentiment analysis, text mining, and natural language processing (NLP) are used.
- Applications: Analyzing customer feedback, monitoring brand reputation, and extracting insights from textual data sources.
These data analysis methods are instrumental in transforming data into actionable insights, informing decision-making processes, and driving organizational success across various sectors, including business, healthcare, finance, marketing, and research. The selection of a specific method often depends on the nature of the data, the research objectives, and the analytical requirements of the project or organization.
Also Read: Quantitative Data Analysis: Types, Analysis & Examples
Data Analysis Tools
Data analysis tools are essential instruments that facilitate the process of examining, cleaning, transforming, and modeling data to uncover useful information, make informed decisions, and drive strategies. Here are some prominent data analysis tools widely used across various industries:
1) Microsoft Excel:
- Description: A spreadsheet software that offers basic to advanced data analysis features, including pivot tables, data visualization tools, and statistical functions.
- Applications: Data cleaning, basic statistical analysis, visualization, and reporting.
2) R Programming Language:
- Description: An open-source programming language specifically designed for statistical computing and data visualization.
- Applications: Advanced statistical analysis, data manipulation, visualization, and machine learning.
3) Python (with Libraries like Pandas, NumPy, Matplotlib, and Seaborn):
- Description: A versatile programming language with libraries that support data manipulation, analysis, and visualization.
- Applications: Data cleaning, statistical analysis, machine learning, and data visualization.
4) SPSS (Statistical Package for the Social Sciences):
- Description: A comprehensive statistical software suite used for data analysis, data mining, and predictive analytics.
- Applications: Descriptive statistics, hypothesis testing, regression analysis, and advanced analytics.
5) SAS (Statistical Analysis System):
- Description: A software suite used for advanced analytics, multivariate analysis, and predictive modeling.
- Applications: Data management, statistical analysis, predictive modeling, and business intelligence.
6) Tableau:
- Description: A data visualization tool that allows users to create interactive and shareable dashboards and reports.
- Applications: Data visualization , business intelligence , and interactive dashboard creation.
7) Power BI:
- Description: A business analytics tool developed by Microsoft that provides interactive visualizations and business intelligence capabilities.
- Applications: Data visualization, business intelligence, reporting, and dashboard creation.
8) SQL (Structured Query Language) Databases (e.g., MySQL, PostgreSQL, Microsoft SQL Server):
- Description: Database management systems that support data storage, retrieval, and manipulation using SQL queries.
- Applications: Data retrieval, data cleaning, data transformation, and database management.
9) Apache Spark:
- Description: A fast and general-purpose distributed computing system designed for big data processing and analytics.
- Applications: Big data processing, machine learning, data streaming, and real-time analytics.
10) IBM SPSS Modeler:
- Description: A data mining software application used for building predictive models and conducting advanced analytics.
- Applications: Predictive modeling, data mining, statistical analysis, and decision optimization.
These tools serve various purposes and cater to different data analysis needs, from basic statistical analysis and data visualization to advanced analytics, machine learning, and big data processing. The choice of a specific tool often depends on the nature of the data, the complexity of the analysis, and the specific requirements of the project or organization.
Also Read: How to Analyze Survey Data: Methods & Examples
Importance of Data Analysis in Research
The importance of data analysis in research cannot be overstated; it serves as the backbone of any scientific investigation or study. Here are several key reasons why data analysis is crucial in the research process:
- Data analysis helps ensure that the results obtained are valid and reliable. By systematically examining the data, researchers can identify any inconsistencies or anomalies that may affect the credibility of the findings.
- Effective data analysis provides researchers with the necessary information to make informed decisions. By interpreting the collected data, researchers can draw conclusions, make predictions, or formulate recommendations based on evidence rather than intuition or guesswork.
- Data analysis allows researchers to identify patterns, trends, and relationships within the data. This can lead to a deeper understanding of the research topic, enabling researchers to uncover insights that may not be immediately apparent.
- In empirical research, data analysis plays a critical role in testing hypotheses. Researchers collect data to either support or refute their hypotheses, and data analysis provides the tools and techniques to evaluate these hypotheses rigorously.
- Transparent and well-executed data analysis enhances the credibility of research findings. By clearly documenting the data analysis methods and procedures, researchers allow others to replicate the study, thereby contributing to the reproducibility of research findings.
- In fields such as business or healthcare, data analysis helps organizations allocate resources more efficiently. By analyzing data on consumer behavior, market trends, or patient outcomes, organizations can make strategic decisions about resource allocation, budgeting, and planning.
- In public policy and social sciences, data analysis is instrumental in developing and evaluating policies and interventions. By analyzing data on social, economic, or environmental factors, policymakers can assess the effectiveness of existing policies and inform the development of new ones.
- Data analysis allows for continuous improvement in research methods and practices. By analyzing past research projects, identifying areas for improvement, and implementing changes based on data-driven insights, researchers can refine their approaches and enhance the quality of future research endeavors.
However, it is important to remember that mastering these techniques requires practice and continuous learning. That’s why we highly recommend the Data Analytics Course by Physics Wallah . Not only does it cover all the fundamentals of data analysis, but it also provides hands-on experience with various tools such as Excel, Python, and Tableau. Plus, if you use the “ READER ” coupon code at checkout, you can get a special discount on the course.
For Latest Tech Related Information, Join Our Official Free Telegram Group : PW Skills Telegram Group
Data Analysis Techniques in Research FAQs
What are the 5 techniques for data analysis.
The five techniques for data analysis include: Descriptive Analysis Diagnostic Analysis Predictive Analysis Prescriptive Analysis Qualitative Analysis
What are techniques of data analysis in research?
Techniques of data analysis in research encompass both qualitative and quantitative methods. These techniques involve processes like summarizing raw data, investigating causes of events, forecasting future outcomes, offering recommendations based on predictions, and examining non-numerical data to understand concepts or experiences.
What are the 3 methods of data analysis?
The three primary methods of data analysis are: Qualitative Analysis Quantitative Analysis Mixed-Methods Analysis
What are the four types of data analysis techniques?
The four types of data analysis techniques are: Descriptive Analysis Diagnostic Analysis Predictive Analysis Prescriptive Analysis
- The 11 Best Analytical Tools For Data Analysis in 2024
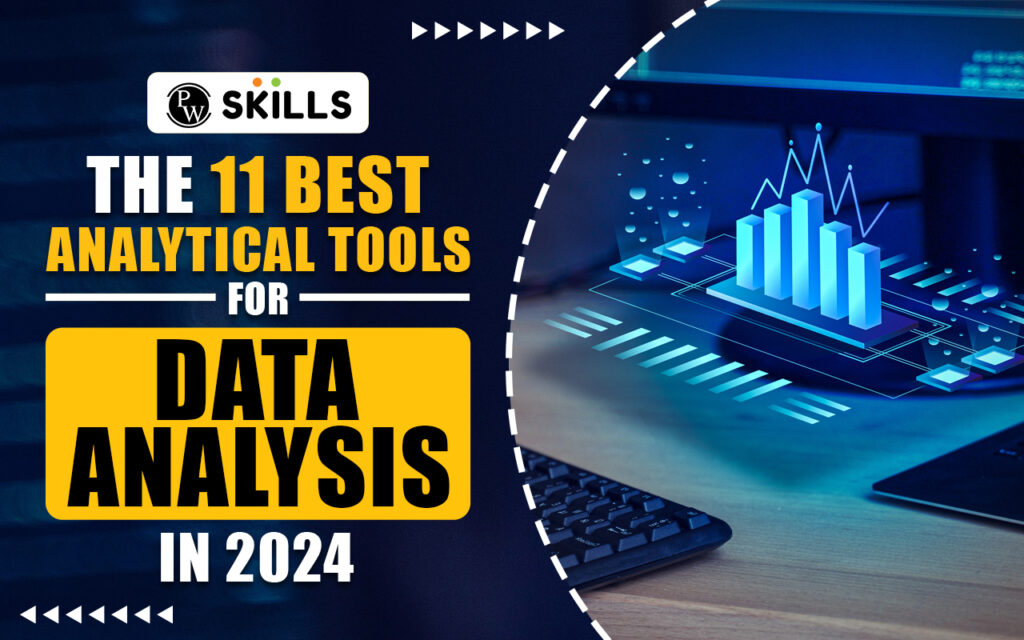
Data Analytical tools help to extract important insights from raw and unstructured data. Read this article to get a list…
- 10 Most Popular Big Data Analytics Tools
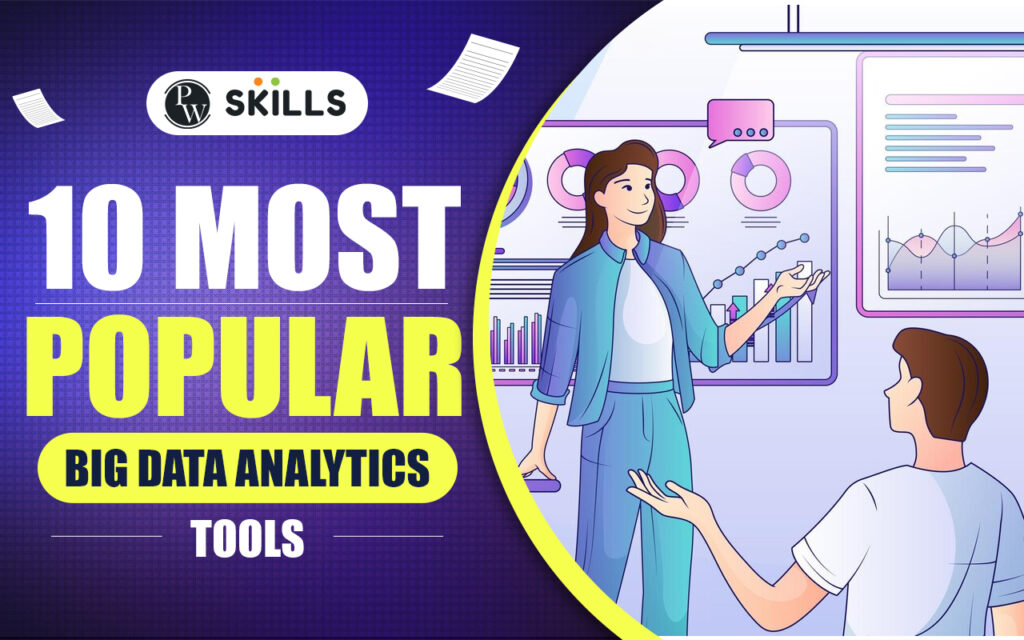
The world of big data analytics tools is diverse, with each tool offering a unique set of skills. Choose your…
- Top 20 Big Data Tools Used By Professionals
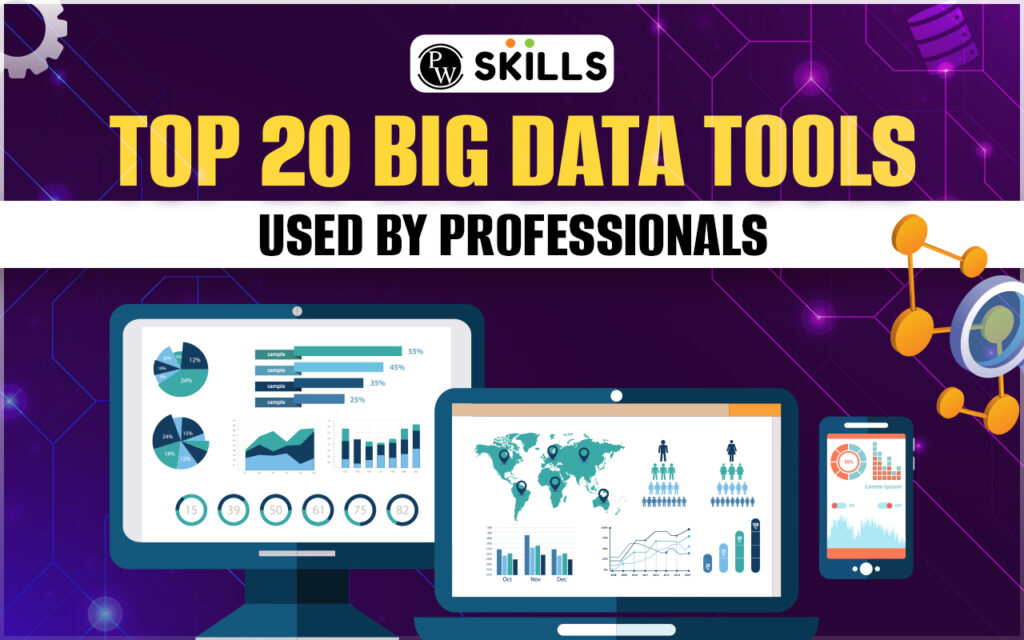
There are plenty of big data tools available online for free. However, some of the handpicked big data tools used…
Related Articles
- Top Best Big Data Analytics Classes 2024
- Best Courses For Data Analytics: Top 10 Courses For Your Career in Trend
- Big Data and Analytics – Definition, Benefits, and More
- Big Data Defined: Examples and Benefits
- Best 5 Unique Strategies to Use Artificial Intelligence Data Analytics
- Best BI Tool: Top 15 Business Intelligence Tools (BI Tools)
- Applications of Big Data
Quantitative Data Analysis: A Comprehensive Guide
By: Ofem Eteng Published: May 18, 2022
Related Articles
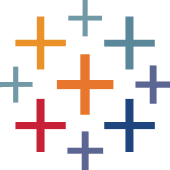
A healthcare giant successfully introduces the most effective drug dosage through rigorous statistical modeling, saving countless lives. A marketing team predicts consumer trends with uncanny accuracy, tailoring campaigns for maximum impact.
Table of Contents
These trends and dosages are not just any numbers but are a result of meticulous quantitative data analysis. Quantitative data analysis offers a robust framework for understanding complex phenomena, evaluating hypotheses, and predicting future outcomes.
In this blog, we’ll walk through the concept of quantitative data analysis, the steps required, its advantages, and the methods and techniques that are used in this analysis. Read on!
What is Quantitative Data Analysis?
Quantitative data analysis is a systematic process of examining, interpreting, and drawing meaningful conclusions from numerical data. It involves the application of statistical methods, mathematical models, and computational techniques to understand patterns, relationships, and trends within datasets.
Quantitative data analysis methods typically work with algorithms, mathematical analysis tools, and software to gain insights from the data, answering questions such as how many, how often, and how much. Data for quantitative data analysis is usually collected from close-ended surveys, questionnaires, polls, etc. The data can also be obtained from sales figures, email click-through rates, number of website visitors, and percentage revenue increase.
Quantitative Data Analysis vs Qualitative Data Analysis
When we talk about data, we directly think about the pattern, the relationship, and the connection between the datasets – analyzing the data in short. Therefore when it comes to data analysis, there are broadly two types – Quantitative Data Analysis and Qualitative Data Analysis.
Quantitative data analysis revolves around numerical data and statistics, which are suitable for functions that can be counted or measured. In contrast, qualitative data analysis includes description and subjective information – for things that can be observed but not measured.
Let us differentiate between Quantitative Data Analysis and Quantitative Data Analysis for a better understanding.

Data Preparation Steps for Quantitative Data Analysis
Quantitative data has to be gathered and cleaned before proceeding to the stage of analyzing it. Below are the steps to prepare a data before quantitative research analysis:
- Step 1: Data Collection
Before beginning the analysis process, you need data. Data can be collected through rigorous quantitative research, which includes methods such as interviews, focus groups, surveys, and questionnaires.
- Step 2: Data Cleaning
Once the data is collected, begin the data cleaning process by scanning through the entire data for duplicates, errors, and omissions. Keep a close eye for outliers (data points that are significantly different from the majority of the dataset) because they can skew your analysis results if they are not removed.
This data-cleaning process ensures data accuracy, consistency and relevancy before analysis.
- Step 3: Data Analysis and Interpretation
Now that you have collected and cleaned your data, it is now time to carry out the quantitative analysis. There are two methods of quantitative data analysis, which we will discuss in the next section.
However, if you have data from multiple sources, collecting and cleaning it can be a cumbersome task. This is where Hevo Data steps in. With Hevo, extracting, transforming, and loading data from source to destination becomes a seamless task, eliminating the need for manual coding. This not only saves valuable time but also enhances the overall efficiency of data analysis and visualization, empowering users to derive insights quickly and with precision
Hevo is the only real-time ELT No-code Data Pipeline platform that cost-effectively automates data pipelines that are flexible to your needs. With integration with 150+ Data Sources (40+ free sources), we help you not only export data from sources & load data to the destinations but also transform & enrich your data, & make it analysis-ready.
Start for free now!
Now that you are familiar with what quantitative data analysis is and how to prepare your data for analysis, the focus will shift to the purpose of this article, which is to describe the methods and techniques of quantitative data analysis.
Methods and Techniques of Quantitative Data Analysis
Quantitative data analysis employs two techniques to extract meaningful insights from datasets, broadly. The first method is descriptive statistics, which summarizes and portrays essential features of a dataset, such as mean, median, and standard deviation.
Inferential statistics, the second method, extrapolates insights and predictions from a sample dataset to make broader inferences about an entire population, such as hypothesis testing and regression analysis.
An in-depth explanation of both the methods is provided below:
- Descriptive Statistics
- Inferential Statistics
1) Descriptive Statistics
Descriptive statistics as the name implies is used to describe a dataset. It helps understand the details of your data by summarizing it and finding patterns from the specific data sample. They provide absolute numbers obtained from a sample but do not necessarily explain the rationale behind the numbers and are mostly used for analyzing single variables. The methods used in descriptive statistics include:
- Mean: This calculates the numerical average of a set of values.
- Median: This is used to get the midpoint of a set of values when the numbers are arranged in numerical order.
- Mode: This is used to find the most commonly occurring value in a dataset.
- Percentage: This is used to express how a value or group of respondents within the data relates to a larger group of respondents.
- Frequency: This indicates the number of times a value is found.
- Range: This shows the highest and lowest values in a dataset.
- Standard Deviation: This is used to indicate how dispersed a range of numbers is, meaning, it shows how close all the numbers are to the mean.
- Skewness: It indicates how symmetrical a range of numbers is, showing if they cluster into a smooth bell curve shape in the middle of the graph or if they skew towards the left or right.
2) Inferential Statistics
In quantitative analysis, the expectation is to turn raw numbers into meaningful insight using numerical values, and descriptive statistics is all about explaining details of a specific dataset using numbers, but it does not explain the motives behind the numbers; hence, a need for further analysis using inferential statistics.
Inferential statistics aim to make predictions or highlight possible outcomes from the analyzed data obtained from descriptive statistics. They are used to generalize results and make predictions between groups, show relationships that exist between multiple variables, and are used for hypothesis testing that predicts changes or differences.
There are various statistical analysis methods used within inferential statistics; a few are discussed below.
- Cross Tabulations: Cross tabulation or crosstab is used to show the relationship that exists between two variables and is often used to compare results by demographic groups. It uses a basic tabular form to draw inferences between different data sets and contains data that is mutually exclusive or has some connection with each other. Crosstabs help understand the nuances of a dataset and factors that may influence a data point.
- Regression Analysis: Regression analysis estimates the relationship between a set of variables. It shows the correlation between a dependent variable (the variable or outcome you want to measure or predict) and any number of independent variables (factors that may impact the dependent variable). Therefore, the purpose of the regression analysis is to estimate how one or more variables might affect a dependent variable to identify trends and patterns to make predictions and forecast possible future trends. There are many types of regression analysis, and the model you choose will be determined by the type of data you have for the dependent variable. The types of regression analysis include linear regression, non-linear regression, binary logistic regression, etc.
- Monte Carlo Simulation: Monte Carlo simulation, also known as the Monte Carlo method, is a computerized technique of generating models of possible outcomes and showing their probability distributions. It considers a range of possible outcomes and then tries to calculate how likely each outcome will occur. Data analysts use it to perform advanced risk analyses to help forecast future events and make decisions accordingly.
- Analysis of Variance (ANOVA): This is used to test the extent to which two or more groups differ from each other. It compares the mean of various groups and allows the analysis of multiple groups.
- Factor Analysis: A large number of variables can be reduced into a smaller number of factors using the factor analysis technique. It works on the principle that multiple separate observable variables correlate with each other because they are all associated with an underlying construct. It helps in reducing large datasets into smaller, more manageable samples.
- Cohort Analysis: Cohort analysis can be defined as a subset of behavioral analytics that operates from data taken from a given dataset. Rather than looking at all users as one unit, cohort analysis breaks down data into related groups for analysis, where these groups or cohorts usually have common characteristics or similarities within a defined period.
- MaxDiff Analysis: This is a quantitative data analysis method that is used to gauge customers’ preferences for purchase and what parameters rank higher than the others in the process.
- Cluster Analysis: Cluster analysis is a technique used to identify structures within a dataset. Cluster analysis aims to be able to sort different data points into groups that are internally similar and externally different; that is, data points within a cluster will look like each other and different from data points in other clusters.
- Time Series Analysis: This is a statistical analytic technique used to identify trends and cycles over time. It is simply the measurement of the same variables at different times, like weekly and monthly email sign-ups, to uncover trends, seasonality, and cyclic patterns. By doing this, the data analyst can forecast how variables of interest may fluctuate in the future.
- SWOT analysis: This is a quantitative data analysis method that assigns numerical values to indicate strengths, weaknesses, opportunities, and threats of an organization, product, or service to show a clearer picture of competition to foster better business strategies
How to Choose the Right Method for your Analysis?
Choosing between Descriptive Statistics or Inferential Statistics can be often confusing. You should consider the following factors before choosing the right method for your quantitative data analysis:
1. Type of Data
The first consideration in data analysis is understanding the type of data you have. Different statistical methods have specific requirements based on these data types, and using the wrong method can render results meaningless. The choice of statistical method should align with the nature and distribution of your data to ensure meaningful and accurate analysis.
2. Your Research Questions
When deciding on statistical methods, it’s crucial to align them with your specific research questions and hypotheses. The nature of your questions will influence whether descriptive statistics alone, which reveal sample attributes, are sufficient or if you need both descriptive and inferential statistics to understand group differences or relationships between variables and make population inferences.
Pros and Cons of Quantitative Data Analysis
1. Objectivity and Generalizability:
- Quantitative data analysis offers objective, numerical measurements, minimizing bias and personal interpretation.
- Results can often be generalized to larger populations, making them applicable to broader contexts.
Example: A study using quantitative data analysis to measure student test scores can objectively compare performance across different schools and demographics, leading to generalizable insights about educational strategies.
2. Precision and Efficiency:
- Statistical methods provide precise numerical results, allowing for accurate comparisons and prediction.
- Large datasets can be analyzed efficiently with the help of computer software, saving time and resources.
Example: A marketing team can use quantitative data analysis to precisely track click-through rates and conversion rates on different ad campaigns, quickly identifying the most effective strategies for maximizing customer engagement.
3. Identification of Patterns and Relationships:
- Statistical techniques reveal hidden patterns and relationships between variables that might not be apparent through observation alone.
- This can lead to new insights and understanding of complex phenomena.
Example: A medical researcher can use quantitative analysis to pinpoint correlations between lifestyle factors and disease risk, aiding in the development of prevention strategies.
1. Limited Scope:
- Quantitative analysis focuses on quantifiable aspects of a phenomenon , potentially overlooking important qualitative nuances, such as emotions, motivations, or cultural contexts.
Example: A survey measuring customer satisfaction with numerical ratings might miss key insights about the underlying reasons for their satisfaction or dissatisfaction, which could be better captured through open-ended feedback.
2. Oversimplification:
- Reducing complex phenomena to numerical data can lead to oversimplification and a loss of richness in understanding.
Example: Analyzing employee productivity solely through quantitative metrics like hours worked or tasks completed might not account for factors like creativity, collaboration, or problem-solving skills, which are crucial for overall performance.
3. Potential for Misinterpretation:
- Statistical results can be misinterpreted if not analyzed carefully and with appropriate expertise.
- The choice of statistical methods and assumptions can significantly influence results.
This blog discusses the steps, methods, and techniques of quantitative data analysis. It also gives insights into the methods of data collection, the type of data one should work with, and the pros and cons of such analysis.
Gain a better understanding of data analysis with these essential reads:
- Data Analysis and Modeling: 4 Critical Differences
- Exploratory Data Analysis Simplified 101
- 25 Best Data Analysis Tools in 2024
Carrying out successful data analysis requires prepping the data and making it analysis-ready. That is where Hevo steps in.
Want to give Hevo a try? Sign Up for a 14-day free trial and experience the feature-rich Hevo suite first hand. You may also have a look at the amazing Hevo price , which will assist you in selecting the best plan for your requirements.
Share your experience of understanding Quantitative Data Analysis in the comment section below! We would love to hear your thoughts.
Ofem is a freelance writer specializing in data-related topics, who has expertise in translating complex concepts. With a focus on data science, analytics, and emerging technologies.
No-code Data Pipeline for your Data Warehouse
- Data Analysis
- Data Warehouse
- Quantitative Data Analysis
Continue Reading
Sarad Mohanan
Best Data Reconciliation Tools: Complete Guide
Satyam Agrawal
What is Data Reconciliation? Everything to Know
Sarthak Bhardwaj
Data Observability vs Data Quality: Difference and Relationships Explored
I want to read this e-book.

Qualitative Data Analysis Methods 101:
The “big 6” methods + examples.
By: Kerryn Warren (PhD) | Reviewed By: Eunice Rautenbach (D.Tech) | May 2020 (Updated April 2023)
Qualitative data analysis methods. Wow, that’s a mouthful.
If you’re new to the world of research, qualitative data analysis can look rather intimidating. So much bulky terminology and so many abstract, fluffy concepts. It certainly can be a minefield!
Don’t worry – in this post, we’ll unpack the most popular analysis methods , one at a time, so that you can approach your analysis with confidence and competence – whether that’s for a dissertation, thesis or really any kind of research project.
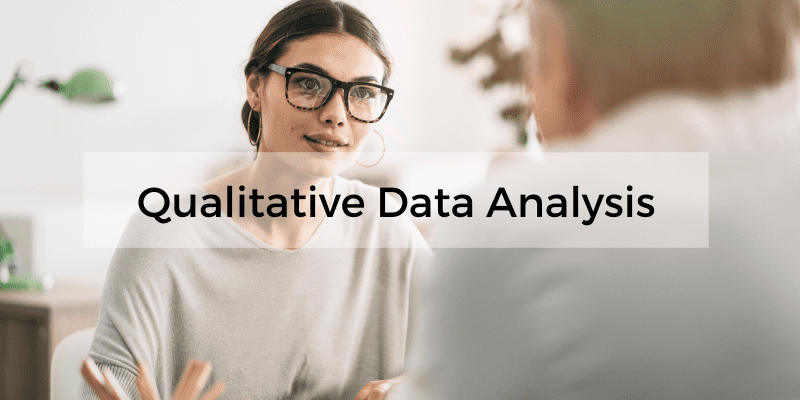
What (exactly) is qualitative data analysis?
To understand qualitative data analysis, we need to first understand qualitative data – so let’s step back and ask the question, “what exactly is qualitative data?”.
Qualitative data refers to pretty much any data that’s “not numbers” . In other words, it’s not the stuff you measure using a fixed scale or complex equipment, nor do you analyse it using complex statistics or mathematics.
So, if it’s not numbers, what is it?
Words, you guessed? Well… sometimes , yes. Qualitative data can, and often does, take the form of interview transcripts, documents and open-ended survey responses – but it can also involve the interpretation of images and videos. In other words, qualitative isn’t just limited to text-based data.
So, how’s that different from quantitative data, you ask?
Simply put, qualitative research focuses on words, descriptions, concepts or ideas – while quantitative research focuses on numbers and statistics . Qualitative research investigates the “softer side” of things to explore and describe , while quantitative research focuses on the “hard numbers”, to measure differences between variables and the relationships between them. If you’re keen to learn more about the differences between qual and quant, we’ve got a detailed post over here .
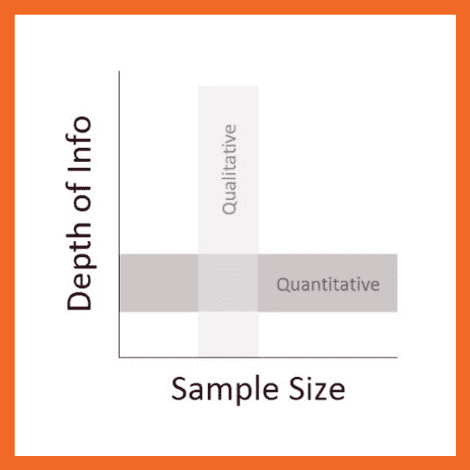
So, qualitative analysis is easier than quantitative, right?
Not quite. In many ways, qualitative data can be challenging and time-consuming to analyse and interpret. At the end of your data collection phase (which itself takes a lot of time), you’ll likely have many pages of text-based data or hours upon hours of audio to work through. You might also have subtle nuances of interactions or discussions that have danced around in your mind, or that you scribbled down in messy field notes. All of this needs to work its way into your analysis.
Making sense of all of this is no small task and you shouldn’t underestimate it. Long story short – qualitative analysis can be a lot of work! Of course, quantitative analysis is no piece of cake either, but it’s important to recognise that qualitative analysis still requires a significant investment in terms of time and effort.
Need a helping hand?
In this post, we’ll explore qualitative data analysis by looking at some of the most common analysis methods we encounter. We’re not going to cover every possible qualitative method and we’re not going to go into heavy detail – we’re just going to give you the big picture. That said, we will of course includes links to loads of extra resources so that you can learn more about whichever analysis method interests you.
Without further delay, let’s get into it.
The “Big 6” Qualitative Analysis Methods
There are many different types of qualitative data analysis, all of which serve different purposes and have unique strengths and weaknesses . We’ll start by outlining the analysis methods and then we’ll dive into the details for each.
The 6 most popular methods (or at least the ones we see at Grad Coach) are:
- Content analysis
- Narrative analysis
- Discourse analysis
- Thematic analysis
- Grounded theory (GT)
- Interpretive phenomenological analysis (IPA)
Let’s take a look at each of them…
QDA Method #1: Qualitative Content Analysis
Content analysis is possibly the most common and straightforward QDA method. At the simplest level, content analysis is used to evaluate patterns within a piece of content (for example, words, phrases or images) or across multiple pieces of content or sources of communication. For example, a collection of newspaper articles or political speeches.
With content analysis, you could, for instance, identify the frequency with which an idea is shared or spoken about – like the number of times a Kardashian is mentioned on Twitter. Or you could identify patterns of deeper underlying interpretations – for instance, by identifying phrases or words in tourist pamphlets that highlight India as an ancient country.
Because content analysis can be used in such a wide variety of ways, it’s important to go into your analysis with a very specific question and goal, or you’ll get lost in the fog. With content analysis, you’ll group large amounts of text into codes , summarise these into categories, and possibly even tabulate the data to calculate the frequency of certain concepts or variables. Because of this, content analysis provides a small splash of quantitative thinking within a qualitative method.
Naturally, while content analysis is widely useful, it’s not without its drawbacks . One of the main issues with content analysis is that it can be very time-consuming , as it requires lots of reading and re-reading of the texts. Also, because of its multidimensional focus on both qualitative and quantitative aspects, it is sometimes accused of losing important nuances in communication.
Content analysis also tends to concentrate on a very specific timeline and doesn’t take into account what happened before or after that timeline. This isn’t necessarily a bad thing though – just something to be aware of. So, keep these factors in mind if you’re considering content analysis. Every analysis method has its limitations , so don’t be put off by these – just be aware of them ! If you’re interested in learning more about content analysis, the video below provides a good starting point.
QDA Method #2: Narrative Analysis
As the name suggests, narrative analysis is all about listening to people telling stories and analysing what that means . Since stories serve a functional purpose of helping us make sense of the world, we can gain insights into the ways that people deal with and make sense of reality by analysing their stories and the ways they’re told.
You could, for example, use narrative analysis to explore whether how something is being said is important. For instance, the narrative of a prisoner trying to justify their crime could provide insight into their view of the world and the justice system. Similarly, analysing the ways entrepreneurs talk about the struggles in their careers or cancer patients telling stories of hope could provide powerful insights into their mindsets and perspectives . Simply put, narrative analysis is about paying attention to the stories that people tell – and more importantly, the way they tell them.
Of course, the narrative approach has its weaknesses , too. Sample sizes are generally quite small due to the time-consuming process of capturing narratives. Because of this, along with the multitude of social and lifestyle factors which can influence a subject, narrative analysis can be quite difficult to reproduce in subsequent research. This means that it’s difficult to test the findings of some of this research.
Similarly, researcher bias can have a strong influence on the results here, so you need to be particularly careful about the potential biases you can bring into your analysis when using this method. Nevertheless, narrative analysis is still a very useful qualitative analysis method – just keep these limitations in mind and be careful not to draw broad conclusions . If you’re keen to learn more about narrative analysis, the video below provides a great introduction to this qualitative analysis method.
QDA Method #3: Discourse Analysis
Discourse is simply a fancy word for written or spoken language or debate . So, discourse analysis is all about analysing language within its social context. In other words, analysing language – such as a conversation, a speech, etc – within the culture and society it takes place. For example, you could analyse how a janitor speaks to a CEO, or how politicians speak about terrorism.
To truly understand these conversations or speeches, the culture and history of those involved in the communication are important factors to consider. For example, a janitor might speak more casually with a CEO in a company that emphasises equality among workers. Similarly, a politician might speak more about terrorism if there was a recent terrorist incident in the country.
So, as you can see, by using discourse analysis, you can identify how culture , history or power dynamics (to name a few) have an effect on the way concepts are spoken about. So, if your research aims and objectives involve understanding culture or power dynamics, discourse analysis can be a powerful method.
Because there are many social influences in terms of how we speak to each other, the potential use of discourse analysis is vast . Of course, this also means it’s important to have a very specific research question (or questions) in mind when analysing your data and looking for patterns and themes, or you might land up going down a winding rabbit hole.
Discourse analysis can also be very time-consuming as you need to sample the data to the point of saturation – in other words, until no new information and insights emerge. But this is, of course, part of what makes discourse analysis such a powerful technique. So, keep these factors in mind when considering this QDA method. Again, if you’re keen to learn more, the video below presents a good starting point.
QDA Method #4: Thematic Analysis
Thematic analysis looks at patterns of meaning in a data set – for example, a set of interviews or focus group transcripts. But what exactly does that… mean? Well, a thematic analysis takes bodies of data (which are often quite large) and groups them according to similarities – in other words, themes . These themes help us make sense of the content and derive meaning from it.
Let’s take a look at an example.
With thematic analysis, you could analyse 100 online reviews of a popular sushi restaurant to find out what patrons think about the place. By reviewing the data, you would then identify the themes that crop up repeatedly within the data – for example, “fresh ingredients” or “friendly wait staff”.
So, as you can see, thematic analysis can be pretty useful for finding out about people’s experiences , views, and opinions . Therefore, if your research aims and objectives involve understanding people’s experience or view of something, thematic analysis can be a great choice.
Since thematic analysis is a bit of an exploratory process, it’s not unusual for your research questions to develop , or even change as you progress through the analysis. While this is somewhat natural in exploratory research, it can also be seen as a disadvantage as it means that data needs to be re-reviewed each time a research question is adjusted. In other words, thematic analysis can be quite time-consuming – but for a good reason. So, keep this in mind if you choose to use thematic analysis for your project and budget extra time for unexpected adjustments.
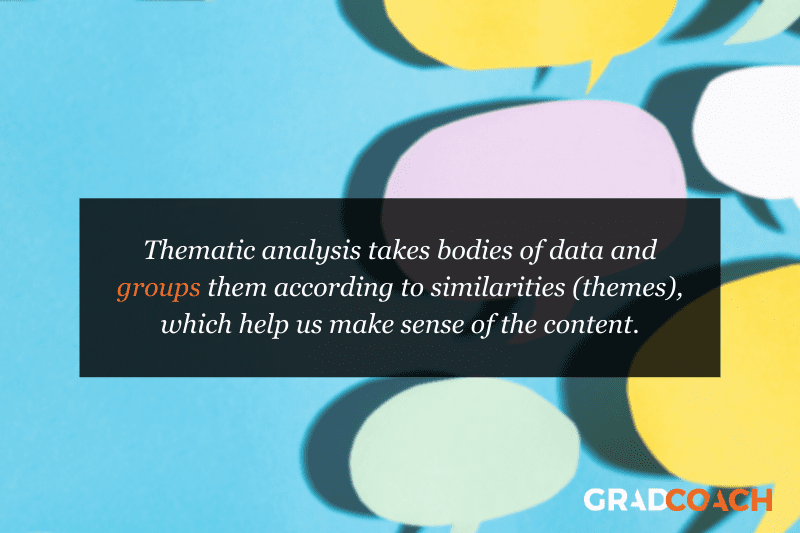
QDA Method #5: Grounded theory (GT)
Grounded theory is a powerful qualitative analysis method where the intention is to create a new theory (or theories) using the data at hand, through a series of “ tests ” and “ revisions ”. Strictly speaking, GT is more a research design type than an analysis method, but we’ve included it here as it’s often referred to as a method.
What’s most important with grounded theory is that you go into the analysis with an open mind and let the data speak for itself – rather than dragging existing hypotheses or theories into your analysis. In other words, your analysis must develop from the ground up (hence the name).
Let’s look at an example of GT in action.
Assume you’re interested in developing a theory about what factors influence students to watch a YouTube video about qualitative analysis. Using Grounded theory , you’d start with this general overarching question about the given population (i.e., graduate students). First, you’d approach a small sample – for example, five graduate students in a department at a university. Ideally, this sample would be reasonably representative of the broader population. You’d interview these students to identify what factors lead them to watch the video.
After analysing the interview data, a general pattern could emerge. For example, you might notice that graduate students are more likely to read a post about qualitative methods if they are just starting on their dissertation journey, or if they have an upcoming test about research methods.
From here, you’ll look for another small sample – for example, five more graduate students in a different department – and see whether this pattern holds true for them. If not, you’ll look for commonalities and adapt your theory accordingly. As this process continues, the theory would develop . As we mentioned earlier, what’s important with grounded theory is that the theory develops from the data – not from some preconceived idea.
So, what are the drawbacks of grounded theory? Well, some argue that there’s a tricky circularity to grounded theory. For it to work, in principle, you should know as little as possible regarding the research question and population, so that you reduce the bias in your interpretation. However, in many circumstances, it’s also thought to be unwise to approach a research question without knowledge of the current literature . In other words, it’s a bit of a “chicken or the egg” situation.
Regardless, grounded theory remains a popular (and powerful) option. Naturally, it’s a very useful method when you’re researching a topic that is completely new or has very little existing research about it, as it allows you to start from scratch and work your way from the ground up .
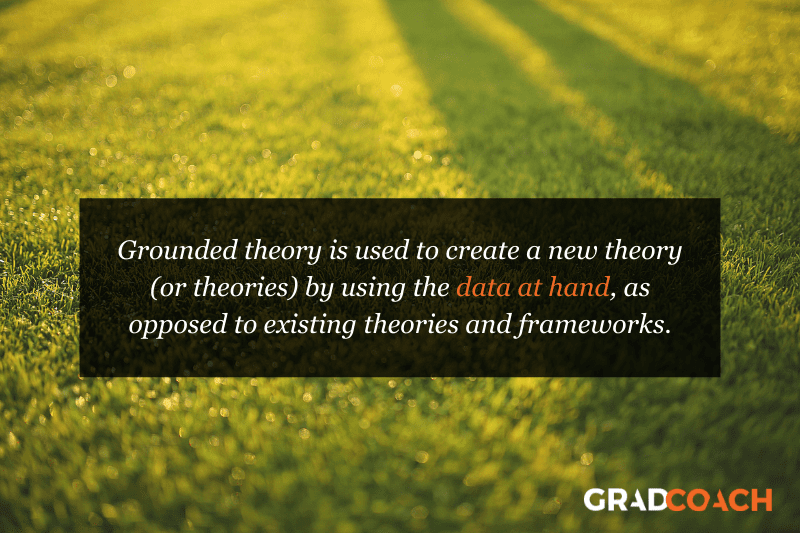
QDA Method #6: Interpretive Phenomenological Analysis (IPA)
Interpretive. Phenomenological. Analysis. IPA . Try saying that three times fast…
Let’s just stick with IPA, okay?
IPA is designed to help you understand the personal experiences of a subject (for example, a person or group of people) concerning a major life event, an experience or a situation . This event or experience is the “phenomenon” that makes up the “P” in IPA. Such phenomena may range from relatively common events – such as motherhood, or being involved in a car accident – to those which are extremely rare – for example, someone’s personal experience in a refugee camp. So, IPA is a great choice if your research involves analysing people’s personal experiences of something that happened to them.
It’s important to remember that IPA is subject – centred . In other words, it’s focused on the experiencer . This means that, while you’ll likely use a coding system to identify commonalities, it’s important not to lose the depth of experience or meaning by trying to reduce everything to codes. Also, keep in mind that since your sample size will generally be very small with IPA, you often won’t be able to draw broad conclusions about the generalisability of your findings. But that’s okay as long as it aligns with your research aims and objectives.
Another thing to be aware of with IPA is personal bias . While researcher bias can creep into all forms of research, self-awareness is critically important with IPA, as it can have a major impact on the results. For example, a researcher who was a victim of a crime himself could insert his own feelings of frustration and anger into the way he interprets the experience of someone who was kidnapped. So, if you’re going to undertake IPA, you need to be very self-aware or you could muddy the analysis.
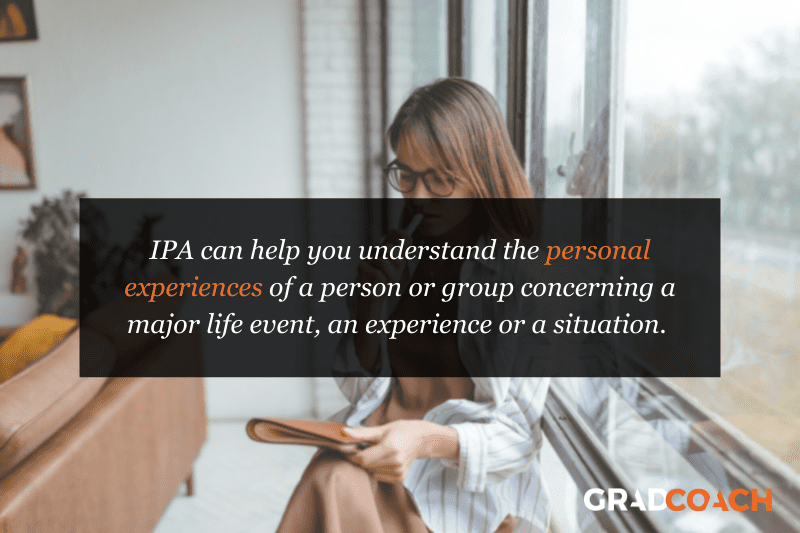
How to choose the right analysis method
In light of all of the qualitative analysis methods we’ve covered so far, you’re probably asking yourself the question, “ How do I choose the right one? ”
Much like all the other methodological decisions you’ll need to make, selecting the right qualitative analysis method largely depends on your research aims, objectives and questions . In other words, the best tool for the job depends on what you’re trying to build. For example:
- Perhaps your research aims to analyse the use of words and what they reveal about the intention of the storyteller and the cultural context of the time.
- Perhaps your research aims to develop an understanding of the unique personal experiences of people that have experienced a certain event, or
- Perhaps your research aims to develop insight regarding the influence of a certain culture on its members.
As you can probably see, each of these research aims are distinctly different , and therefore different analysis methods would be suitable for each one. For example, narrative analysis would likely be a good option for the first aim, while grounded theory wouldn’t be as relevant.
It’s also important to remember that each method has its own set of strengths, weaknesses and general limitations. No single analysis method is perfect . So, depending on the nature of your research, it may make sense to adopt more than one method (this is called triangulation ). Keep in mind though that this will of course be quite time-consuming.
As we’ve seen, all of the qualitative analysis methods we’ve discussed make use of coding and theme-generating techniques, but the intent and approach of each analysis method differ quite substantially. So, it’s very important to come into your research with a clear intention before you decide which analysis method (or methods) to use.
Start by reviewing your research aims , objectives and research questions to assess what exactly you’re trying to find out – then select a qualitative analysis method that fits. Never pick a method just because you like it or have experience using it – your analysis method (or methods) must align with your broader research aims and objectives.
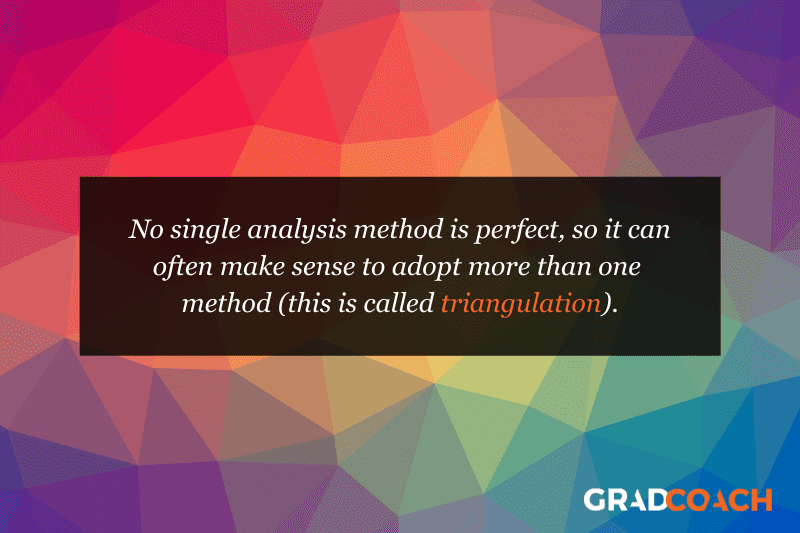
Let’s recap on QDA methods…
In this post, we looked at six popular qualitative data analysis methods:
- First, we looked at content analysis , a straightforward method that blends a little bit of quant into a primarily qualitative analysis.
- Then we looked at narrative analysis , which is about analysing how stories are told.
- Next up was discourse analysis – which is about analysing conversations and interactions.
- Then we moved on to thematic analysis – which is about identifying themes and patterns.
- From there, we went south with grounded theory – which is about starting from scratch with a specific question and using the data alone to build a theory in response to that question.
- And finally, we looked at IPA – which is about understanding people’s unique experiences of a phenomenon.
Of course, these aren’t the only options when it comes to qualitative data analysis, but they’re a great starting point if you’re dipping your toes into qualitative research for the first time.
If you’re still feeling a bit confused, consider our private coaching service , where we hold your hand through the research process to help you develop your best work.
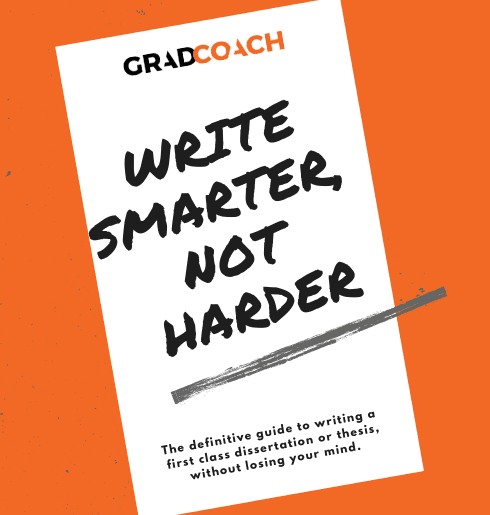
Psst… there’s more (for free)
This post is part of our dissertation mini-course, which covers everything you need to get started with your dissertation, thesis or research project.
You Might Also Like:
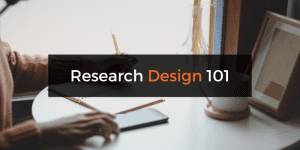
84 Comments
This has been very helpful. Thank you.
Thank you madam,
Thank you so much for this information
I wonder it so clear for understand and good for me. can I ask additional query?
Very insightful and useful
Good work done with clear explanations. Thank you.
Thanks so much for the write-up, it’s really good.
Thanks madam . It is very important .
thank you very good
This has been very well explained in simple language . It is useful even for a new researcher.
Great to hear that. Good luck with your qualitative data analysis, Pramod!
This is very useful information. And it was very a clear language structured presentation. Thanks a lot.
Thank you so much.
very informative sequential presentation
Precise explanation of method.
Hi, may we use 2 data analysis methods in our qualitative research?
Thanks for your comment. Most commonly, one would use one type of analysis method, but it depends on your research aims and objectives.
You explained it in very simple language, everyone can understand it. Thanks so much.
Thank you very much, this is very helpful. It has been explained in a very simple manner that even a layman understands
Thank nicely explained can I ask is Qualitative content analysis the same as thematic analysis?
Thanks for your comment. No, QCA and thematic are two different types of analysis. This article might help clarify – https://onlinelibrary.wiley.com/doi/10.1111/nhs.12048
This is my first time to come across a well explained data analysis. so helpful.
I have thoroughly enjoyed your explanation of the six qualitative analysis methods. This is very helpful. Thank you!
Thank you very much, this is well explained and useful
i need a citation of your book.
Thanks a lot , remarkable indeed, enlighting to the best
Hi Derek, What other theories/methods would you recommend when the data is a whole speech?
Keep writing useful artikel.
It is important concept about QDA and also the way to express is easily understandable, so thanks for all.
Thank you, this is well explained and very useful.
Very helpful .Thanks.
Hi there! Very well explained. Simple but very useful style of writing. Please provide the citation of the text. warm regards
The session was very helpful and insightful. Thank you
This was very helpful and insightful. Easy to read and understand
As a professional academic writer, this has been so informative and educative. Keep up the good work Grad Coach you are unmatched with quality content for sure.
Keep up the good work Grad Coach you are unmatched with quality content for sure.
Its Great and help me the most. A Million Thanks you Dr.
It is a very nice work
Very insightful. Please, which of this approach could be used for a research that one is trying to elicit students’ misconceptions in a particular concept ?
This is Amazing and well explained, thanks
great overview
What do we call a research data analysis method that one use to advise or determining the best accounting tool or techniques that should be adopted in a company.
Informative video, explained in a clear and simple way. Kudos
Waoo! I have chosen method wrong for my data analysis. But I can revise my work according to this guide. Thank you so much for this helpful lecture.
This has been very helpful. It gave me a good view of my research objectives and how to choose the best method. Thematic analysis it is.
Very helpful indeed. Thanku so much for the insight.
This was incredibly helpful.
Very helpful.
very educative
Nicely written especially for novice academic researchers like me! Thank you.
choosing a right method for a paper is always a hard job for a student, this is a useful information, but it would be more useful personally for me, if the author provide me with a little bit more information about the data analysis techniques in type of explanatory research. Can we use qualitative content analysis technique for explanatory research ? or what is the suitable data analysis method for explanatory research in social studies?
that was very helpful for me. because these details are so important to my research. thank you very much
I learnt a lot. Thank you
Relevant and Informative, thanks !
Well-planned and organized, thanks much! 🙂
I have reviewed qualitative data analysis in a simplest way possible. The content will highly be useful for developing my book on qualitative data analysis methods. Cheers!
Clear explanation on qualitative and how about Case study
This was helpful. Thank you
This was really of great assistance, it was just the right information needed. Explanation very clear and follow.
Wow, Thanks for making my life easy
This was helpful thanks .
Very helpful…. clear and written in an easily understandable manner. Thank you.
This was so helpful as it was easy to understand. I’m a new to research thank you so much.
so educative…. but Ijust want to know which method is coding of the qualitative or tallying done?
Thank you for the great content, I have learnt a lot. So helpful
precise and clear presentation with simple language and thank you for that.
very informative content, thank you.
You guys are amazing on YouTube on this platform. Your teachings are great, educative, and informative. kudos!
Brilliant Delivery. You made a complex subject seem so easy. Well done.
Beautifully explained.
Thanks a lot
Is there a video the captures the practical process of coding using automated applications?
Thanks for the comment. We don’t recommend using automated applications for coding, as they are not sufficiently accurate in our experience.
content analysis can be qualitative research?
THANK YOU VERY MUCH.
Thank you very much for such a wonderful content
do you have any material on Data collection
What a powerful explanation of the QDA methods. Thank you.
Great explanation both written and Video. i have been using of it on a day to day working of my thesis project in accounting and finance. Thank you very much for your support.
very helpful, thank you so much
Submit a Comment Cancel reply
Your email address will not be published. Required fields are marked *
Save my name, email, and website in this browser for the next time I comment.
- Print Friendly
Table of Contents
What is data analysis, why is data analysis important, what is the data analysis process, data analysis methods, applications of data analysis, top data analysis techniques to analyze data, what is the importance of data analysis in research, future trends in data analysis, choose the right program, what is data analysis: a comprehensive guide.
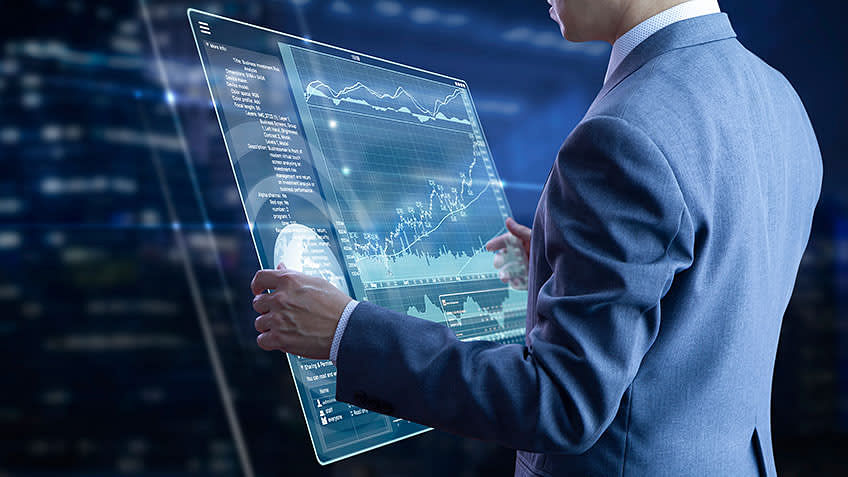
In the contemporary business landscape, gaining a competitive edge is imperative, given the challenges such as rapidly evolving markets, economic unpredictability, fluctuating political environments, capricious consumer sentiments, and even global health crises. These challenges have reduced the room for error in business operations. For companies striving not only to survive but also to thrive in this demanding environment, the key lies in embracing the concept of data analysis . This involves strategically accumulating valuable, actionable information, which is leveraged to enhance decision-making processes.
If you're interested in forging a career in data analysis and wish to discover the top data analysis courses in 2024, we invite you to explore our informative video. It will provide insights into the opportunities to develop your expertise in this crucial field.
Data analysis inspects, cleans, transforms, and models data to extract insights and support decision-making. As a data analyst , your role involves dissecting vast datasets, unearthing hidden patterns, and translating numbers into actionable information.
Data analysis plays a pivotal role in today's data-driven world. It helps organizations harness the power of data, enabling them to make decisions, optimize processes, and gain a competitive edge. By turning raw data into meaningful insights, data analysis empowers businesses to identify opportunities, mitigate risks, and enhance their overall performance.
1. Informed Decision-Making
Data analysis is the compass that guides decision-makers through a sea of information. It enables organizations to base their choices on concrete evidence rather than intuition or guesswork. In business, this means making decisions more likely to lead to success, whether choosing the right marketing strategy, optimizing supply chains, or launching new products. By analyzing data, decision-makers can assess various options' potential risks and rewards, leading to better choices.
2. Improved Understanding
Data analysis provides a deeper understanding of processes, behaviors, and trends. It allows organizations to gain insights into customer preferences, market dynamics, and operational efficiency .
3. Competitive Advantage
Organizations can identify opportunities and threats by analyzing market trends, consumer behavior , and competitor performance. They can pivot their strategies to respond effectively, staying one step ahead of the competition. This ability to adapt and innovate based on data insights can lead to a significant competitive advantage.
Become a Data Science & Business Analytics Professional
- 11.5 M Expected New Jobs For Data Science And Analytics
- 28% Annual Job Growth By 2026
- $46K-$100K Average Annual Salary
Post Graduate Program in Data Analytics
- Post Graduate Program certificate and Alumni Association membership
- Exclusive hackathons and Ask me Anything sessions by IBM
Data Analyst
- Industry-recognized Data Analyst Master’s certificate from Simplilearn
- Dedicated live sessions by faculty of industry experts
Here's what learners are saying regarding our programs:
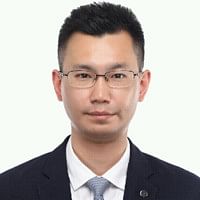
Felix Chong
Project manage , codethink.
After completing this course, I landed a new job & a salary hike of 30%. I now work with Zuhlke Group as a Project Manager.

Gayathri Ramesh
Associate data engineer , publicis sapient.
The course was well structured and curated. The live classes were extremely helpful. They made learning more productive and interactive. The program helped me change my domain from a data analyst to an Associate Data Engineer.
4. Risk Mitigation
Data analysis is a valuable tool for risk assessment and management. Organizations can assess potential issues and take preventive measures by analyzing historical data. For instance, data analysis detects fraudulent activities in the finance industry by identifying unusual transaction patterns. This not only helps minimize financial losses but also safeguards the reputation and trust of customers.
5. Efficient Resource Allocation
Data analysis helps organizations optimize resource allocation. Whether it's allocating budgets, human resources, or manufacturing capacities, data-driven insights can ensure that resources are utilized efficiently. For example, data analysis can help hospitals allocate staff and resources to the areas with the highest patient demand, ensuring that patient care remains efficient and effective.
6. Continuous Improvement
Data analysis is a catalyst for continuous improvement. It allows organizations to monitor performance metrics, track progress, and identify areas for enhancement. This iterative process of analyzing data, implementing changes, and analyzing again leads to ongoing refinement and excellence in processes and products.
The data analysis process is a structured sequence of steps that lead from raw data to actionable insights. Here are the answers to what is data analysis:
- Data Collection: Gather relevant data from various sources, ensuring data quality and integrity.
- Data Cleaning: Identify and rectify errors, missing values, and inconsistencies in the dataset. Clean data is crucial for accurate analysis.
- Exploratory Data Analysis (EDA): Conduct preliminary analysis to understand the data's characteristics, distributions, and relationships. Visualization techniques are often used here.
- Data Transformation: Prepare the data for analysis by encoding categorical variables, scaling features, and handling outliers, if necessary.
- Model Building: Depending on the objectives, apply appropriate data analysis methods, such as regression, clustering, or deep learning.
- Model Evaluation: Depending on the problem type, assess the models' performance using metrics like Mean Absolute Error, Root Mean Squared Error , or others.
- Interpretation and Visualization: Translate the model's results into actionable insights. Visualizations, tables, and summary statistics help in conveying findings effectively.
- Deployment: Implement the insights into real-world solutions or strategies, ensuring that the data-driven recommendations are implemented.
1. Regression Analysis
Regression analysis is a powerful method for understanding the relationship between a dependent and one or more independent variables. It is applied in economics, finance, and social sciences. By fitting a regression model, you can make predictions, analyze cause-and-effect relationships, and uncover trends within your data.
2. Statistical Analysis
Statistical analysis encompasses a broad range of techniques for summarizing and interpreting data. It involves descriptive statistics (mean, median, standard deviation), inferential statistics (hypothesis testing, confidence intervals), and multivariate analysis. Statistical methods help make inferences about populations from sample data, draw conclusions, and assess the significance of results.
3. Cohort Analysis
Cohort analysis focuses on understanding the behavior of specific groups or cohorts over time. It can reveal patterns, retention rates, and customer lifetime value, helping businesses tailor their strategies.
4. Content Analysis
It is a qualitative data analysis method used to study the content of textual, visual, or multimedia data. Social sciences, journalism, and marketing often employ it to analyze themes, sentiments, or patterns within documents or media. Content analysis can help researchers gain insights from large volumes of unstructured data.
5. Factor Analysis
Factor analysis is a technique for uncovering underlying latent factors that explain the variance in observed variables. It is commonly used in psychology and the social sciences to reduce the dimensionality of data and identify underlying constructs. Factor analysis can simplify complex datasets, making them easier to interpret and analyze.
6. Monte Carlo Method
This method is a simulation technique that uses random sampling to solve complex problems and make probabilistic predictions. Monte Carlo simulations allow analysts to model uncertainty and risk, making it a valuable tool for decision-making.
7. Text Analysis
Also known as text mining , this method involves extracting insights from textual data. It analyzes large volumes of text, such as social media posts, customer reviews, or documents. Text analysis can uncover sentiment, topics, and trends, enabling organizations to understand public opinion, customer feedback, and emerging issues.
8. Time Series Analysis
Time series analysis deals with data collected at regular intervals over time. It is essential for forecasting, trend analysis, and understanding temporal patterns. Time series methods include moving averages, exponential smoothing, and autoregressive integrated moving average (ARIMA) models. They are widely used in finance for stock price prediction, meteorology for weather forecasting, and economics for economic modeling.
9. Descriptive Analysis
Descriptive analysis involves summarizing and describing the main features of a dataset. It focuses on organizing and presenting the data in a meaningful way, often using measures such as mean, median, mode, and standard deviation. It provides an overview of the data and helps identify patterns or trends.
10. Inferential Analysis
Inferential analysis aims to make inferences or predictions about a larger population based on sample data. It involves applying statistical techniques such as hypothesis testing, confidence intervals, and regression analysis. It helps generalize findings from a sample to a larger population.
11. Exploratory Data Analysis (EDA)
EDA focuses on exploring and understanding the data without preconceived hypotheses. It involves visualizations, summary statistics, and data profiling techniques to uncover patterns, relationships, and interesting features. It helps generate hypotheses for further analysis.
12. Diagnostic Analysis
Diagnostic analysis aims to understand the cause-and-effect relationships within the data. It investigates the factors or variables that contribute to specific outcomes or behaviors. Techniques such as regression analysis, ANOVA (Analysis of Variance), or correlation analysis are commonly used in diagnostic analysis.
13. Predictive Analysis
Predictive analysis involves using historical data to make predictions or forecasts about future outcomes. It utilizes statistical modeling techniques, machine learning algorithms, and time series analysis to identify patterns and build predictive models. It is often used for forecasting sales, predicting customer behavior, or estimating risk.
14. Prescriptive Analysis
Prescriptive analysis goes beyond predictive analysis by recommending actions or decisions based on the predictions. It combines historical data, optimization algorithms, and business rules to provide actionable insights and optimize outcomes. It helps in decision-making and resource allocation.
Our Data Analyst Master's Program will help you learn analytics tools and techniques to become a Data Analyst expert! It's the pefect course for you to jumpstart your career. Enroll now!
Data analysis is a versatile and indispensable tool that finds applications across various industries and domains. Its ability to extract actionable insights from data has made it a fundamental component of decision-making and problem-solving. Let's explore some of the key applications of data analysis:
1. Business and Marketing
- Market Research: Data analysis helps businesses understand market trends, consumer preferences, and competitive landscapes. It aids in identifying opportunities for product development, pricing strategies, and market expansion.
- Sales Forecasting: Data analysis models can predict future sales based on historical data, seasonality, and external factors. This helps businesses optimize inventory management and resource allocation.
2. Healthcare and Life Sciences
- Disease Diagnosis: Data analysis is vital in medical diagnostics, from interpreting medical images (e.g., MRI, X-rays) to analyzing patient records. Machine learning models can assist in early disease detection.
- Drug Discovery: Pharmaceutical companies use data analysis to identify potential drug candidates, predict their efficacy, and optimize clinical trials.
- Genomics and Personalized Medicine: Genomic data analysis enables personalized treatment plans by identifying genetic markers that influence disease susceptibility and response to therapies.
- Risk Management: Financial institutions use data analysis to assess credit risk, detect fraudulent activities, and model market risks.
- Algorithmic Trading: Data analysis is integral to developing trading algorithms that analyze market data and execute trades automatically based on predefined strategies.
- Fraud Detection: Credit card companies and banks employ data analysis to identify unusual transaction patterns and detect fraudulent activities in real time.
4. Manufacturing and Supply Chain
- Quality Control: Data analysis monitors and controls product quality on manufacturing lines. It helps detect defects and ensure consistency in production processes.
- Inventory Optimization: By analyzing demand patterns and supply chain data, businesses can optimize inventory levels, reduce carrying costs, and ensure timely deliveries.
5. Social Sciences and Academia
- Social Research: Researchers in social sciences analyze survey data, interviews, and textual data to study human behavior, attitudes, and trends. It helps in policy development and understanding societal issues.
- Academic Research: Data analysis is crucial to scientific physics, biology, and environmental science research. It assists in interpreting experimental results and drawing conclusions.
6. Internet and Technology
- Search Engines: Google uses complex data analysis algorithms to retrieve and rank search results based on user behavior and relevance.
- Recommendation Systems: Services like Netflix and Amazon leverage data analysis to recommend content and products to users based on their past preferences and behaviors.
7. Environmental Science
- Climate Modeling: Data analysis is essential in climate science. It analyzes temperature, precipitation, and other environmental data. It helps in understanding climate patterns and predicting future trends.
- Environmental Monitoring: Remote sensing data analysis monitors ecological changes, including deforestation, water quality, and air pollution.
1. Descriptive Statistics
Descriptive statistics provide a snapshot of a dataset's central tendencies and variability. These techniques help summarize and understand the data's basic characteristics.
2. Inferential Statistics
Inferential statistics involve making predictions or inferences based on a sample of data. Techniques include hypothesis testing, confidence intervals, and regression analysis. These methods are crucial for drawing conclusions from data and assessing the significance of findings.
3. Regression Analysis
It explores the relationship between one or more independent variables and a dependent variable. It is widely used for prediction and understanding causal links. Linear, logistic, and multiple regression are common in various fields.
4. Clustering Analysis
It is an unsupervised learning method that groups similar data points. K-means clustering and hierarchical clustering are examples. This technique is used for customer segmentation, anomaly detection, and pattern recognition.
5. Classification Analysis
Classification analysis assigns data points to predefined categories or classes. It's often used in applications like spam email detection, image recognition, and sentiment analysis. Popular algorithms include decision trees, support vector machines, and neural networks.
6. Time Series Analysis
Time series analysis deals with data collected over time, making it suitable for forecasting and trend analysis. Techniques like moving averages, autoregressive integrated moving averages (ARIMA), and exponential smoothing are applied in fields like finance, economics, and weather forecasting.
7. Text Analysis (Natural Language Processing - NLP)
Text analysis techniques, part of NLP , enable extracting insights from textual data. These methods include sentiment analysis, topic modeling, and named entity recognition. Text analysis is widely used for analyzing customer reviews, social media content, and news articles.
8. Principal Component Analysis
It is a dimensionality reduction technique that simplifies complex datasets while retaining important information. It transforms correlated variables into a set of linearly uncorrelated variables, making it easier to analyze and visualize high-dimensional data.
9. Anomaly Detection
Anomaly detection identifies unusual patterns or outliers in data. It's critical in fraud detection, network security, and quality control. Techniques like statistical methods, clustering-based approaches, and machine learning algorithms are employed for anomaly detection.
10. Data Mining
Data mining involves the automated discovery of patterns, associations, and relationships within large datasets. Techniques like association rule mining, frequent pattern analysis, and decision tree mining extract valuable knowledge from data.
11. Machine Learning and Deep Learning
ML and deep learning algorithms are applied for predictive modeling, classification, and regression tasks. Techniques like random forests, support vector machines, and convolutional neural networks (CNNs) have revolutionized various industries, including healthcare, finance, and image recognition.
12. Geographic Information Systems (GIS) Analysis
GIS analysis combines geographical data with spatial analysis techniques to solve location-based problems. It's widely used in urban planning, environmental management, and disaster response.
- Uncovering Patterns and Trends: Data analysis allows researchers to identify patterns, trends, and relationships within the data. By examining these patterns, researchers can better understand the phenomena under investigation. For example, in epidemiological research, data analysis can reveal the trends and patterns of disease outbreaks, helping public health officials take proactive measures.
- Testing Hypotheses: Research often involves formulating hypotheses and testing them. Data analysis provides the means to evaluate hypotheses rigorously. Through statistical tests and inferential analysis, researchers can determine whether the observed patterns in the data are statistically significant or simply due to chance.
- Making Informed Conclusions: Data analysis helps researchers draw meaningful and evidence-based conclusions from their research findings. It provides a quantitative basis for making claims and recommendations. In academic research, these conclusions form the basis for scholarly publications and contribute to the body of knowledge in a particular field.
- Enhancing Data Quality: Data analysis includes data cleaning and validation processes that improve the quality and reliability of the dataset. Identifying and addressing errors, missing values, and outliers ensures that the research results accurately reflect the phenomena being studied.
- Supporting Decision-Making: In applied research, data analysis assists decision-makers in various sectors, such as business, government, and healthcare. Policy decisions, marketing strategies, and resource allocations are often based on research findings.
- Identifying Outliers and Anomalies: Outliers and anomalies in data can hold valuable information or indicate errors. Data analysis techniques can help identify these exceptional cases, whether medical diagnoses, financial fraud detection, or product quality control.
- Revealing Insights: Research data often contain hidden insights that are not immediately apparent. Data analysis techniques, such as clustering or text analysis, can uncover these insights. For example, social media data sentiment analysis can reveal public sentiment and trends on various topics in social sciences.
- Forecasting and Prediction: Data analysis allows for the development of predictive models. Researchers can use historical data to build models forecasting future trends or outcomes. This is valuable in fields like finance for stock price predictions, meteorology for weather forecasting, and epidemiology for disease spread projections.
- Optimizing Resources: Research often involves resource allocation. Data analysis helps researchers and organizations optimize resource use by identifying areas where improvements can be made, or costs can be reduced.
- Continuous Improvement: Data analysis supports the iterative nature of research. Researchers can analyze data, draw conclusions, and refine their hypotheses or research designs based on their findings. This cycle of analysis and refinement leads to continuous improvement in research methods and understanding.
Data analysis is an ever-evolving field driven by technological advancements. The future of data analysis promises exciting developments that will reshape how data is collected, processed, and utilized. Here are some of the key trends of data analysis:
1. Artificial Intelligence and Machine Learning Integration
Artificial intelligence (AI) and machine learning (ML) are expected to play a central role in data analysis. These technologies can automate complex data processing tasks, identify patterns at scale, and make highly accurate predictions. AI-driven analytics tools will become more accessible, enabling organizations to harness the power of ML without requiring extensive expertise.
2. Augmented Analytics
Augmented analytics combines AI and natural language processing (NLP) to assist data analysts in finding insights. These tools can automatically generate narratives, suggest visualizations, and highlight important trends within data. They enhance the speed and efficiency of data analysis, making it more accessible to a broader audience.
3. Data Privacy and Ethical Considerations
As data collection becomes more pervasive, privacy concerns and ethical considerations will gain prominence. Future data analysis trends will prioritize responsible data handling, transparency, and compliance with regulations like GDPR . Differential privacy techniques and data anonymization will be crucial in balancing data utility with privacy protection.
4. Real-time and Streaming Data Analysis
The demand for real-time insights will drive the adoption of real-time and streaming data analysis. Organizations will leverage technologies like Apache Kafka and Apache Flink to process and analyze data as it is generated. This trend is essential for fraud detection, IoT analytics, and monitoring systems.
5. Quantum Computing
It can potentially revolutionize data analysis by solving complex problems exponentially faster than classical computers. Although quantum computing is in its infancy, its impact on optimization, cryptography , and simulations will be significant once practical quantum computers become available.
6. Edge Analytics
With the proliferation of edge devices in the Internet of Things (IoT), data analysis is moving closer to the data source. Edge analytics allows for real-time processing and decision-making at the network's edge, reducing latency and bandwidth requirements.
7. Explainable AI (XAI)
Interpretable and explainable AI models will become crucial, especially in applications where trust and transparency are paramount. XAI techniques aim to make AI decisions more understandable and accountable, which is critical in healthcare and finance.
8. Data Democratization
The future of data analysis will see more democratization of data access and analysis tools. Non-technical users will have easier access to data and analytics through intuitive interfaces and self-service BI tools , reducing the reliance on data specialists.
9. Advanced Data Visualization
Data visualization tools will continue to evolve, offering more interactivity, 3D visualization, and augmented reality (AR) capabilities. Advanced visualizations will help users explore data in new and immersive ways.
10. Ethnographic Data Analysis
Ethnographic data analysis will gain importance as organizations seek to understand human behavior, cultural dynamics, and social trends. This qualitative data analysis approach and quantitative methods will provide a holistic understanding of complex issues.
11. Data Analytics Ethics and Bias Mitigation
Ethical considerations in data analysis will remain a key trend. Efforts to identify and mitigate bias in algorithms and models will become standard practice, ensuring fair and equitable outcomes.
Our Data Analytics courses have been meticulously crafted to equip you with the necessary skills and knowledge to thrive in this swiftly expanding industry. Our instructors will lead you through immersive, hands-on projects, real-world simulations, and illuminating case studies, ensuring you gain the practical expertise necessary for success. Through our courses, you will acquire the ability to dissect data, craft enlightening reports, and make data-driven choices that have the potential to steer businesses toward prosperity.
Having addressed the question of what is data analysis, if you're considering a career in data analytics, it's advisable to begin by researching the prerequisites for becoming a data analyst. You may also want to explore the Post Graduate Program in Data Analytics offered in collaboration with Purdue University. This program offers a practical learning experience through real-world case studies and projects aligned with industry needs. It provides comprehensive exposure to the essential technologies and skills currently employed in the field of data analytics.
Program Name Data Analyst Post Graduate Program In Data Analytics Data Analytics Bootcamp Geo All Geos All Geos US University Simplilearn Purdue Caltech Course Duration 11 Months 8 Months 6 Months Coding Experience Required No Basic No Skills You Will Learn 10+ skills including Python, MySQL, Tableau, NumPy and more Data Analytics, Statistical Analysis using Excel, Data Analysis Python and R, and more Data Visualization with Tableau, Linear and Logistic Regression, Data Manipulation and more Additional Benefits Applied Learning via Capstone and 20+ industry-relevant Data Analytics projects Purdue Alumni Association Membership Free IIMJobs Pro-Membership of 6 months Access to Integrated Practical Labs Caltech CTME Circle Membership Cost $$ $$$$ $$$$ Explore Program Explore Program Explore Program
1. What is the difference between data analysis and data science?
Data analysis primarily involves extracting meaningful insights from existing data using statistical techniques and visualization tools. Whereas, data science encompasses a broader spectrum, incorporating data analysis as a subset while involving machine learning, deep learning, and predictive modeling to build data-driven solutions and algorithms.
2. What are the common mistakes to avoid in data analysis?
Common mistakes to avoid in data analysis include neglecting data quality issues, failing to define clear objectives, overcomplicating visualizations, not considering algorithmic biases, and disregarding the importance of proper data preprocessing and cleaning. Additionally, avoiding making unwarranted assumptions and misinterpreting correlation as causation in your analysis is crucial.
Data Science & Business Analytics Courses Duration and Fees
Data Science & Business Analytics programs typically range from a few weeks to several months, with fees varying based on program and institution.
Learn from Industry Experts with free Masterclasses
Data science & business analytics.
How Can You Master the Art of Data Analysis: Uncover the Path to Career Advancement
Develop Your Career in Data Analytics with Purdue University Professional Certificate
Career Masterclass: How to Get Qualified for a Data Analytics Career
Recommended Reads
Big Data Career Guide: A Comprehensive Playbook to Becoming a Big Data Engineer
Why Python Is Essential for Data Analysis and Data Science?
The Best Spotify Data Analysis Project You Need to Know
The Rise of the Data-Driven Professional: 6 Non-Data Roles That Need Data Analytics Skills
Exploratory Data Analysis [EDA]: Techniques, Best Practices and Popular Applications
All the Ins and Outs of Exploratory Data Analysis
Get Affiliated Certifications with Live Class programs
- PMP, PMI, PMBOK, CAPM, PgMP, PfMP, ACP, PBA, RMP, SP, and OPM3 are registered marks of the Project Management Institute, Inc.
Have a language expert improve your writing
Run a free plagiarism check in 10 minutes, generate accurate citations for free.
- Knowledge Base
Methodology
Research Methods | Definitions, Types, Examples
Research methods are specific procedures for collecting and analyzing data. Developing your research methods is an integral part of your research design . When planning your methods, there are two key decisions you will make.
First, decide how you will collect data . Your methods depend on what type of data you need to answer your research question :
- Qualitative vs. quantitative : Will your data take the form of words or numbers?
- Primary vs. secondary : Will you collect original data yourself, or will you use data that has already been collected by someone else?
- Descriptive vs. experimental : Will you take measurements of something as it is, or will you perform an experiment?
Second, decide how you will analyze the data .
- For quantitative data, you can use statistical analysis methods to test relationships between variables.
- For qualitative data, you can use methods such as thematic analysis to interpret patterns and meanings in the data.
Table of contents
Methods for collecting data, examples of data collection methods, methods for analyzing data, examples of data analysis methods, other interesting articles, frequently asked questions about research methods.
Data is the information that you collect for the purposes of answering your research question . The type of data you need depends on the aims of your research.
Qualitative vs. quantitative data
Your choice of qualitative or quantitative data collection depends on the type of knowledge you want to develop.
For questions about ideas, experiences and meanings, or to study something that can’t be described numerically, collect qualitative data .
If you want to develop a more mechanistic understanding of a topic, or your research involves hypothesis testing , collect quantitative data .
You can also take a mixed methods approach , where you use both qualitative and quantitative research methods.
Primary vs. secondary research
Primary research is any original data that you collect yourself for the purposes of answering your research question (e.g. through surveys , observations and experiments ). Secondary research is data that has already been collected by other researchers (e.g. in a government census or previous scientific studies).
If you are exploring a novel research question, you’ll probably need to collect primary data . But if you want to synthesize existing knowledge, analyze historical trends, or identify patterns on a large scale, secondary data might be a better choice.
Descriptive vs. experimental data
In descriptive research , you collect data about your study subject without intervening. The validity of your research will depend on your sampling method .
In experimental research , you systematically intervene in a process and measure the outcome. The validity of your research will depend on your experimental design .
To conduct an experiment, you need to be able to vary your independent variable , precisely measure your dependent variable, and control for confounding variables . If it’s practically and ethically possible, this method is the best choice for answering questions about cause and effect.
Receive feedback on language, structure, and formatting
Professional editors proofread and edit your paper by focusing on:
- Academic style
- Vague sentences
- Style consistency
See an example
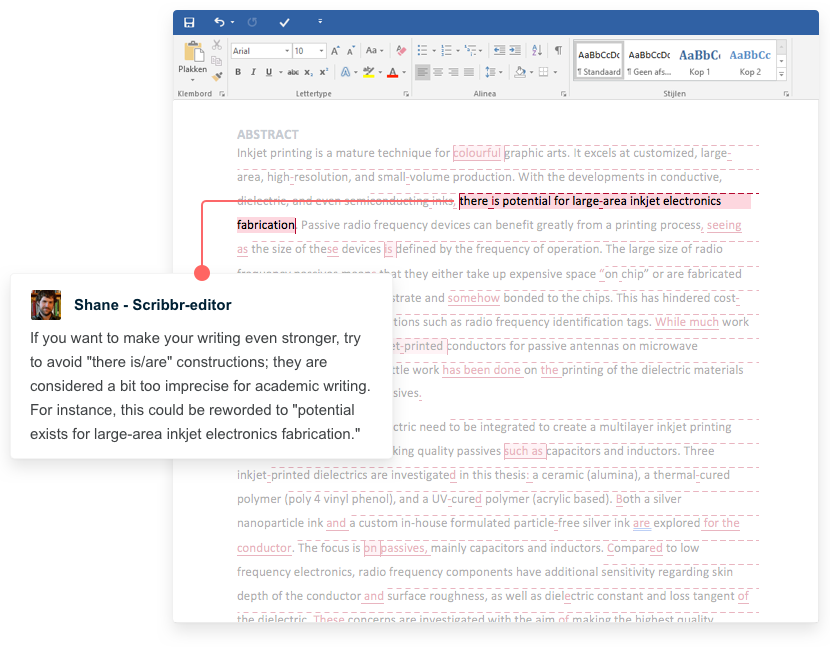
Your data analysis methods will depend on the type of data you collect and how you prepare it for analysis.
Data can often be analyzed both quantitatively and qualitatively. For example, survey responses could be analyzed qualitatively by studying the meanings of responses or quantitatively by studying the frequencies of responses.
Qualitative analysis methods
Qualitative analysis is used to understand words, ideas, and experiences. You can use it to interpret data that was collected:
- From open-ended surveys and interviews , literature reviews , case studies , ethnographies , and other sources that use text rather than numbers.
- Using non-probability sampling methods .
Qualitative analysis tends to be quite flexible and relies on the researcher’s judgement, so you have to reflect carefully on your choices and assumptions and be careful to avoid research bias .
Quantitative analysis methods
Quantitative analysis uses numbers and statistics to understand frequencies, averages and correlations (in descriptive studies) or cause-and-effect relationships (in experiments).
You can use quantitative analysis to interpret data that was collected either:
- During an experiment .
- Using probability sampling methods .
Because the data is collected and analyzed in a statistically valid way, the results of quantitative analysis can be easily standardized and shared among researchers.
Here's why students love Scribbr's proofreading services
Discover proofreading & editing
If you want to know more about statistics , methodology , or research bias , make sure to check out some of our other articles with explanations and examples.
- Chi square test of independence
- Statistical power
- Descriptive statistics
- Degrees of freedom
- Pearson correlation
- Null hypothesis
- Double-blind study
- Case-control study
- Research ethics
- Data collection
- Hypothesis testing
- Structured interviews
Research bias
- Hawthorne effect
- Unconscious bias
- Recall bias
- Halo effect
- Self-serving bias
- Information bias
Quantitative research deals with numbers and statistics, while qualitative research deals with words and meanings.
Quantitative methods allow you to systematically measure variables and test hypotheses . Qualitative methods allow you to explore concepts and experiences in more detail.
In mixed methods research , you use both qualitative and quantitative data collection and analysis methods to answer your research question .
A sample is a subset of individuals from a larger population . Sampling means selecting the group that you will actually collect data from in your research. For example, if you are researching the opinions of students in your university, you could survey a sample of 100 students.
In statistics, sampling allows you to test a hypothesis about the characteristics of a population.
The research methods you use depend on the type of data you need to answer your research question .
- If you want to measure something or test a hypothesis , use quantitative methods . If you want to explore ideas, thoughts and meanings, use qualitative methods .
- If you want to analyze a large amount of readily-available data, use secondary data. If you want data specific to your purposes with control over how it is generated, collect primary data.
- If you want to establish cause-and-effect relationships between variables , use experimental methods. If you want to understand the characteristics of a research subject, use descriptive methods.
Methodology refers to the overarching strategy and rationale of your research project . It involves studying the methods used in your field and the theories or principles behind them, in order to develop an approach that matches your objectives.
Methods are the specific tools and procedures you use to collect and analyze data (for example, experiments, surveys , and statistical tests ).
In shorter scientific papers, where the aim is to report the findings of a specific study, you might simply describe what you did in a methods section .
In a longer or more complex research project, such as a thesis or dissertation , you will probably include a methodology section , where you explain your approach to answering the research questions and cite relevant sources to support your choice of methods.
Is this article helpful?
Other students also liked, writing strong research questions | criteria & examples.
- What Is a Research Design | Types, Guide & Examples
- Data Collection | Definition, Methods & Examples
More interesting articles
- Between-Subjects Design | Examples, Pros, & Cons
- Cluster Sampling | A Simple Step-by-Step Guide with Examples
- Confounding Variables | Definition, Examples & Controls
- Construct Validity | Definition, Types, & Examples
- Content Analysis | Guide, Methods & Examples
- Control Groups and Treatment Groups | Uses & Examples
- Control Variables | What Are They & Why Do They Matter?
- Correlation vs. Causation | Difference, Designs & Examples
- Correlational Research | When & How to Use
- Critical Discourse Analysis | Definition, Guide & Examples
- Cross-Sectional Study | Definition, Uses & Examples
- Descriptive Research | Definition, Types, Methods & Examples
- Ethical Considerations in Research | Types & Examples
- Explanatory and Response Variables | Definitions & Examples
- Explanatory Research | Definition, Guide, & Examples
- Exploratory Research | Definition, Guide, & Examples
- External Validity | Definition, Types, Threats & Examples
- Extraneous Variables | Examples, Types & Controls
- Guide to Experimental Design | Overview, Steps, & Examples
- How Do You Incorporate an Interview into a Dissertation? | Tips
- How to Do Thematic Analysis | Step-by-Step Guide & Examples
- How to Write a Literature Review | Guide, Examples, & Templates
- How to Write a Strong Hypothesis | Steps & Examples
- Inclusion and Exclusion Criteria | Examples & Definition
- Independent vs. Dependent Variables | Definition & Examples
- Inductive Reasoning | Types, Examples, Explanation
- Inductive vs. Deductive Research Approach | Steps & Examples
- Internal Validity in Research | Definition, Threats, & Examples
- Internal vs. External Validity | Understanding Differences & Threats
- Longitudinal Study | Definition, Approaches & Examples
- Mediator vs. Moderator Variables | Differences & Examples
- Mixed Methods Research | Definition, Guide & Examples
- Multistage Sampling | Introductory Guide & Examples
- Naturalistic Observation | Definition, Guide & Examples
- Operationalization | A Guide with Examples, Pros & Cons
- Population vs. Sample | Definitions, Differences & Examples
- Primary Research | Definition, Types, & Examples
- Qualitative vs. Quantitative Research | Differences, Examples & Methods
- Quasi-Experimental Design | Definition, Types & Examples
- Questionnaire Design | Methods, Question Types & Examples
- Random Assignment in Experiments | Introduction & Examples
- Random vs. Systematic Error | Definition & Examples
- Reliability vs. Validity in Research | Difference, Types and Examples
- Reproducibility vs Replicability | Difference & Examples
- Reproducibility vs. Replicability | Difference & Examples
- Sampling Methods | Types, Techniques & Examples
- Semi-Structured Interview | Definition, Guide & Examples
- Simple Random Sampling | Definition, Steps & Examples
- Single, Double, & Triple Blind Study | Definition & Examples
- Stratified Sampling | Definition, Guide & Examples
- Structured Interview | Definition, Guide & Examples
- Survey Research | Definition, Examples & Methods
- Systematic Review | Definition, Example, & Guide
- Systematic Sampling | A Step-by-Step Guide with Examples
- Textual Analysis | Guide, 3 Approaches & Examples
- The 4 Types of Reliability in Research | Definitions & Examples
- The 4 Types of Validity in Research | Definitions & Examples
- Transcribing an Interview | 5 Steps & Transcription Software
- Triangulation in Research | Guide, Types, Examples
- Types of Interviews in Research | Guide & Examples
- Types of Research Designs Compared | Guide & Examples
- Types of Variables in Research & Statistics | Examples
- Unstructured Interview | Definition, Guide & Examples
- What Is a Case Study? | Definition, Examples & Methods
- What Is a Case-Control Study? | Definition & Examples
- What Is a Cohort Study? | Definition & Examples
- What Is a Conceptual Framework? | Tips & Examples
- What Is a Controlled Experiment? | Definitions & Examples
- What Is a Double-Barreled Question?
- What Is a Focus Group? | Step-by-Step Guide & Examples
- What Is a Likert Scale? | Guide & Examples
- What Is a Prospective Cohort Study? | Definition & Examples
- What Is a Retrospective Cohort Study? | Definition & Examples
- What Is Action Research? | Definition & Examples
- What Is an Observational Study? | Guide & Examples
- What Is Concurrent Validity? | Definition & Examples
- What Is Content Validity? | Definition & Examples
- What Is Convenience Sampling? | Definition & Examples
- What Is Convergent Validity? | Definition & Examples
- What Is Criterion Validity? | Definition & Examples
- What Is Data Cleansing? | Definition, Guide & Examples
- What Is Deductive Reasoning? | Explanation & Examples
- What Is Discriminant Validity? | Definition & Example
- What Is Ecological Validity? | Definition & Examples
- What Is Ethnography? | Definition, Guide & Examples
- What Is Face Validity? | Guide, Definition & Examples
- What Is Non-Probability Sampling? | Types & Examples
- What Is Participant Observation? | Definition & Examples
- What Is Peer Review? | Types & Examples
- What Is Predictive Validity? | Examples & Definition
- What Is Probability Sampling? | Types & Examples
- What Is Purposive Sampling? | Definition & Examples
- What Is Qualitative Observation? | Definition & Examples
- What Is Qualitative Research? | Methods & Examples
- What Is Quantitative Observation? | Definition & Examples
- What Is Quantitative Research? | Definition, Uses & Methods
What is your plagiarism score?

What is Data Analysis? Research, Types & Example
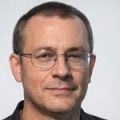
What is Data Analysis?
Data analysis is defined as a process of cleaning, transforming, and modeling data to discover useful information for business decision-making. The purpose of Data Analysis is to extract useful information from data and taking the decision based upon the data analysis.
A simple example of Data analysis is whenever we take any decision in our day-to-day life is by thinking about what happened last time or what will happen by choosing that particular decision. This is nothing but analyzing our past or future and making decisions based on it. For that, we gather memories of our past or dreams of our future. So that is nothing but data analysis. Now same thing analyst does for business purposes, is called Data Analysis.
In this Data Science Tutorial, you will learn:
Why Data Analysis?
To grow your business even to grow in your life, sometimes all you need to do is Analysis!
If your business is not growing, then you have to look back and acknowledge your mistakes and make a plan again without repeating those mistakes. And even if your business is growing, then you have to look forward to making the business to grow more. All you need to do is analyze your business data and business processes.
Data Analysis Tools
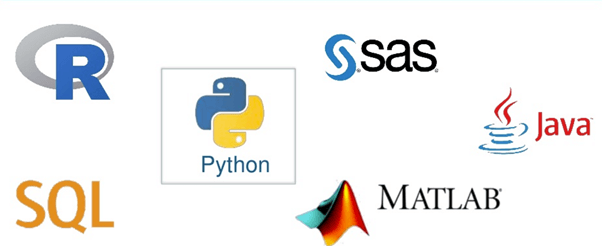
Data analysis tools make it easier for users to process and manipulate data, analyze the relationships and correlations between data sets, and it also helps to identify patterns and trends for interpretation. Here is a complete list of tools used for data analysis in research.
Types of Data Analysis: Techniques and Methods
There are several types of Data Analysis techniques that exist based on business and technology. However, the major Data Analysis methods are:
Text Analysis
Statistical analysis, diagnostic analysis, predictive analysis, prescriptive analysis.
Text Analysis is also referred to as Data Mining. It is one of the methods of data analysis to discover a pattern in large data sets using databases or data mining tools . It used to transform raw data into business information. Business Intelligence tools are present in the market which is used to take strategic business decisions. Overall it offers a way to extract and examine data and deriving patterns and finally interpretation of the data.
Statistical Analysis shows “What happen?” by using past data in the form of dashboards. Statistical Analysis includes collection, Analysis, interpretation, presentation, and modeling of data. It analyses a set of data or a sample of data. There are two categories of this type of Analysis – Descriptive Analysis and Inferential Analysis.
Descriptive Analysis
analyses complete data or a sample of summarized numerical data. It shows mean and deviation for continuous data whereas percentage and frequency for categorical data.
Inferential Analysis
analyses sample from complete data. In this type of Analysis, you can find different conclusions from the same data by selecting different samples.
Diagnostic Analysis shows “Why did it happen?” by finding the cause from the insight found in Statistical Analysis. This Analysis is useful to identify behavior patterns of data. If a new problem arrives in your business process, then you can look into this Analysis to find similar patterns of that problem. And it may have chances to use similar prescriptions for the new problems.
Predictive Analysis shows “what is likely to happen” by using previous data. The simplest data analysis example is like if last year I bought two dresses based on my savings and if this year my salary is increasing double then I can buy four dresses. But of course it’s not easy like this because you have to think about other circumstances like chances of prices of clothes is increased this year or maybe instead of dresses you want to buy a new bike, or you need to buy a house!
So here, this Analysis makes predictions about future outcomes based on current or past data. Forecasting is just an estimate. Its accuracy is based on how much detailed information you have and how much you dig in it.
Prescriptive Analysis combines the insight from all previous Analysis to determine which action to take in a current problem or decision. Most data-driven companies are utilizing Prescriptive Analysis because predictive and descriptive Analysis are not enough to improve data performance. Based on current situations and problems, they analyze the data and make decisions.
Data Analysis Process
The Data Analysis Process is nothing but gathering information by using a proper application or tool which allows you to explore the data and find a pattern in it. Based on that information and data, you can make decisions, or you can get ultimate conclusions.
Data Analysis consists of the following phases:
Data Requirement Gathering
Data collection, data cleaning, data analysis, data interpretation, data visualization.
First of all, you have to think about why do you want to do this data analysis? All you need to find out the purpose or aim of doing the Analysis of data. You have to decide which type of data analysis you wanted to do! In this phase, you have to decide what to analyze and how to measure it, you have to understand why you are investigating and what measures you have to use to do this Analysis.
After requirement gathering, you will get a clear idea about what things you have to measure and what should be your findings. Now it’s time to collect your data based on requirements. Once you collect your data, remember that the collected data must be processed or organized for Analysis. As you collected data from various sources, you must have to keep a log with a collection date and source of the data.
Now whatever data is collected may not be useful or irrelevant to your aim of Analysis, hence it should be cleaned. The data which is collected may contain duplicate records, white spaces or errors. The data should be cleaned and error free. This phase must be done before Analysis because based on data cleaning, your output of Analysis will be closer to your expected outcome.
Once the data is collected, cleaned, and processed, it is ready for Analysis. As you manipulate data, you may find you have the exact information you need, or you might need to collect more data. During this phase, you can use data analysis tools and software which will help you to understand, interpret, and derive conclusions based on the requirements.
After analyzing your data, it’s finally time to interpret your results. You can choose the way to express or communicate your data analysis either you can use simply in words or maybe a table or chart. Then use the results of your data analysis process to decide your best course of action.
Data visualization is very common in your day to day life; they often appear in the form of charts and graphs. In other words, data shown graphically so that it will be easier for the human brain to understand and process it. Data visualization often used to discover unknown facts and trends. By observing relationships and comparing datasets, you can find a way to find out meaningful information.
- Data analysis means a process of cleaning, transforming and modeling data to discover useful information for business decision-making
- Types of Data Analysis are Text, Statistical, Diagnostic, Predictive, Prescriptive Analysis
- Data Analysis consists of Data Requirement Gathering, Data Collection, Data Cleaning, Data Analysis, Data Interpretation, Data Visualization
- 40+ Best Data Science Courses Online with Certification in 2024
- SAS Tutorial for Beginners: What is & Programming Example
- What is Data Science? Introduction, Basic Concepts & Process
- Top 50 Data Science Interview Questions and Answers (PDF)
- 60+ Data Engineer Interview Questions and Answers in 2024
- Difference Between Data Science and Machine Learning
- 17 BEST Data Science Books (2024 Update)
- Data Science Tutorial for Beginners: Learn Basics in 3 Days

An official website of the United States government
The .gov means it’s official. Federal government websites often end in .gov or .mil. Before sharing sensitive information, make sure you’re on a federal government site.
The site is secure. The https:// ensures that you are connecting to the official website and that any information you provide is encrypted and transmitted securely.
- Publications
- Account settings
Preview improvements coming to the PMC website in October 2024. Learn More or Try it out now .
- Advanced Search
- Journal List
- Indian J Anaesth
- v.60(9); 2016 Sep
Basic statistical tools in research and data analysis
Zulfiqar ali.
Department of Anaesthesiology, Division of Neuroanaesthesiology, Sheri Kashmir Institute of Medical Sciences, Soura, Srinagar, Jammu and Kashmir, India
S Bala Bhaskar
1 Department of Anaesthesiology and Critical Care, Vijayanagar Institute of Medical Sciences, Bellary, Karnataka, India
Statistical methods involved in carrying out a study include planning, designing, collecting data, analysing, drawing meaningful interpretation and reporting of the research findings. The statistical analysis gives meaning to the meaningless numbers, thereby breathing life into a lifeless data. The results and inferences are precise only if proper statistical tests are used. This article will try to acquaint the reader with the basic research tools that are utilised while conducting various studies. The article covers a brief outline of the variables, an understanding of quantitative and qualitative variables and the measures of central tendency. An idea of the sample size estimation, power analysis and the statistical errors is given. Finally, there is a summary of parametric and non-parametric tests used for data analysis.
INTRODUCTION
Statistics is a branch of science that deals with the collection, organisation, analysis of data and drawing of inferences from the samples to the whole population.[ 1 ] This requires a proper design of the study, an appropriate selection of the study sample and choice of a suitable statistical test. An adequate knowledge of statistics is necessary for proper designing of an epidemiological study or a clinical trial. Improper statistical methods may result in erroneous conclusions which may lead to unethical practice.[ 2 ]
Variable is a characteristic that varies from one individual member of population to another individual.[ 3 ] Variables such as height and weight are measured by some type of scale, convey quantitative information and are called as quantitative variables. Sex and eye colour give qualitative information and are called as qualitative variables[ 3 ] [ Figure 1 ].

Classification of variables
Quantitative variables
Quantitative or numerical data are subdivided into discrete and continuous measurements. Discrete numerical data are recorded as a whole number such as 0, 1, 2, 3,… (integer), whereas continuous data can assume any value. Observations that can be counted constitute the discrete data and observations that can be measured constitute the continuous data. Examples of discrete data are number of episodes of respiratory arrests or the number of re-intubations in an intensive care unit. Similarly, examples of continuous data are the serial serum glucose levels, partial pressure of oxygen in arterial blood and the oesophageal temperature.
A hierarchical scale of increasing precision can be used for observing and recording the data which is based on categorical, ordinal, interval and ratio scales [ Figure 1 ].
Categorical or nominal variables are unordered. The data are merely classified into categories and cannot be arranged in any particular order. If only two categories exist (as in gender male and female), it is called as a dichotomous (or binary) data. The various causes of re-intubation in an intensive care unit due to upper airway obstruction, impaired clearance of secretions, hypoxemia, hypercapnia, pulmonary oedema and neurological impairment are examples of categorical variables.
Ordinal variables have a clear ordering between the variables. However, the ordered data may not have equal intervals. Examples are the American Society of Anesthesiologists status or Richmond agitation-sedation scale.
Interval variables are similar to an ordinal variable, except that the intervals between the values of the interval variable are equally spaced. A good example of an interval scale is the Fahrenheit degree scale used to measure temperature. With the Fahrenheit scale, the difference between 70° and 75° is equal to the difference between 80° and 85°: The units of measurement are equal throughout the full range of the scale.
Ratio scales are similar to interval scales, in that equal differences between scale values have equal quantitative meaning. However, ratio scales also have a true zero point, which gives them an additional property. For example, the system of centimetres is an example of a ratio scale. There is a true zero point and the value of 0 cm means a complete absence of length. The thyromental distance of 6 cm in an adult may be twice that of a child in whom it may be 3 cm.
STATISTICS: DESCRIPTIVE AND INFERENTIAL STATISTICS
Descriptive statistics[ 4 ] try to describe the relationship between variables in a sample or population. Descriptive statistics provide a summary of data in the form of mean, median and mode. Inferential statistics[ 4 ] use a random sample of data taken from a population to describe and make inferences about the whole population. It is valuable when it is not possible to examine each member of an entire population. The examples if descriptive and inferential statistics are illustrated in Table 1 .
Example of descriptive and inferential statistics

Descriptive statistics
The extent to which the observations cluster around a central location is described by the central tendency and the spread towards the extremes is described by the degree of dispersion.
Measures of central tendency
The measures of central tendency are mean, median and mode.[ 6 ] Mean (or the arithmetic average) is the sum of all the scores divided by the number of scores. Mean may be influenced profoundly by the extreme variables. For example, the average stay of organophosphorus poisoning patients in ICU may be influenced by a single patient who stays in ICU for around 5 months because of septicaemia. The extreme values are called outliers. The formula for the mean is

where x = each observation and n = number of observations. Median[ 6 ] is defined as the middle of a distribution in a ranked data (with half of the variables in the sample above and half below the median value) while mode is the most frequently occurring variable in a distribution. Range defines the spread, or variability, of a sample.[ 7 ] It is described by the minimum and maximum values of the variables. If we rank the data and after ranking, group the observations into percentiles, we can get better information of the pattern of spread of the variables. In percentiles, we rank the observations into 100 equal parts. We can then describe 25%, 50%, 75% or any other percentile amount. The median is the 50 th percentile. The interquartile range will be the observations in the middle 50% of the observations about the median (25 th -75 th percentile). Variance[ 7 ] is a measure of how spread out is the distribution. It gives an indication of how close an individual observation clusters about the mean value. The variance of a population is defined by the following formula:

where σ 2 is the population variance, X is the population mean, X i is the i th element from the population and N is the number of elements in the population. The variance of a sample is defined by slightly different formula:

where s 2 is the sample variance, x is the sample mean, x i is the i th element from the sample and n is the number of elements in the sample. The formula for the variance of a population has the value ‘ n ’ as the denominator. The expression ‘ n −1’ is known as the degrees of freedom and is one less than the number of parameters. Each observation is free to vary, except the last one which must be a defined value. The variance is measured in squared units. To make the interpretation of the data simple and to retain the basic unit of observation, the square root of variance is used. The square root of the variance is the standard deviation (SD).[ 8 ] The SD of a population is defined by the following formula:

where σ is the population SD, X is the population mean, X i is the i th element from the population and N is the number of elements in the population. The SD of a sample is defined by slightly different formula:

where s is the sample SD, x is the sample mean, x i is the i th element from the sample and n is the number of elements in the sample. An example for calculation of variation and SD is illustrated in Table 2 .
Example of mean, variance, standard deviation

Normal distribution or Gaussian distribution
Most of the biological variables usually cluster around a central value, with symmetrical positive and negative deviations about this point.[ 1 ] The standard normal distribution curve is a symmetrical bell-shaped. In a normal distribution curve, about 68% of the scores are within 1 SD of the mean. Around 95% of the scores are within 2 SDs of the mean and 99% within 3 SDs of the mean [ Figure 2 ].

Normal distribution curve
Skewed distribution
It is a distribution with an asymmetry of the variables about its mean. In a negatively skewed distribution [ Figure 3 ], the mass of the distribution is concentrated on the right of Figure 1 . In a positively skewed distribution [ Figure 3 ], the mass of the distribution is concentrated on the left of the figure leading to a longer right tail.

Curves showing negatively skewed and positively skewed distribution
Inferential statistics
In inferential statistics, data are analysed from a sample to make inferences in the larger collection of the population. The purpose is to answer or test the hypotheses. A hypothesis (plural hypotheses) is a proposed explanation for a phenomenon. Hypothesis tests are thus procedures for making rational decisions about the reality of observed effects.
Probability is the measure of the likelihood that an event will occur. Probability is quantified as a number between 0 and 1 (where 0 indicates impossibility and 1 indicates certainty).
In inferential statistics, the term ‘null hypothesis’ ( H 0 ‘ H-naught ,’ ‘ H-null ’) denotes that there is no relationship (difference) between the population variables in question.[ 9 ]
Alternative hypothesis ( H 1 and H a ) denotes that a statement between the variables is expected to be true.[ 9 ]
The P value (or the calculated probability) is the probability of the event occurring by chance if the null hypothesis is true. The P value is a numerical between 0 and 1 and is interpreted by researchers in deciding whether to reject or retain the null hypothesis [ Table 3 ].
P values with interpretation

If P value is less than the arbitrarily chosen value (known as α or the significance level), the null hypothesis (H0) is rejected [ Table 4 ]. However, if null hypotheses (H0) is incorrectly rejected, this is known as a Type I error.[ 11 ] Further details regarding alpha error, beta error and sample size calculation and factors influencing them are dealt with in another section of this issue by Das S et al .[ 12 ]
Illustration for null hypothesis

PARAMETRIC AND NON-PARAMETRIC TESTS
Numerical data (quantitative variables) that are normally distributed are analysed with parametric tests.[ 13 ]
Two most basic prerequisites for parametric statistical analysis are:
- The assumption of normality which specifies that the means of the sample group are normally distributed
- The assumption of equal variance which specifies that the variances of the samples and of their corresponding population are equal.
However, if the distribution of the sample is skewed towards one side or the distribution is unknown due to the small sample size, non-parametric[ 14 ] statistical techniques are used. Non-parametric tests are used to analyse ordinal and categorical data.
Parametric tests
The parametric tests assume that the data are on a quantitative (numerical) scale, with a normal distribution of the underlying population. The samples have the same variance (homogeneity of variances). The samples are randomly drawn from the population, and the observations within a group are independent of each other. The commonly used parametric tests are the Student's t -test, analysis of variance (ANOVA) and repeated measures ANOVA.
Student's t -test
Student's t -test is used to test the null hypothesis that there is no difference between the means of the two groups. It is used in three circumstances:

where X = sample mean, u = population mean and SE = standard error of mean

where X 1 − X 2 is the difference between the means of the two groups and SE denotes the standard error of the difference.
- To test if the population means estimated by two dependent samples differ significantly (the paired t -test). A usual setting for paired t -test is when measurements are made on the same subjects before and after a treatment.
The formula for paired t -test is:

where d is the mean difference and SE denotes the standard error of this difference.
The group variances can be compared using the F -test. The F -test is the ratio of variances (var l/var 2). If F differs significantly from 1.0, then it is concluded that the group variances differ significantly.
Analysis of variance
The Student's t -test cannot be used for comparison of three or more groups. The purpose of ANOVA is to test if there is any significant difference between the means of two or more groups.
In ANOVA, we study two variances – (a) between-group variability and (b) within-group variability. The within-group variability (error variance) is the variation that cannot be accounted for in the study design. It is based on random differences present in our samples.
However, the between-group (or effect variance) is the result of our treatment. These two estimates of variances are compared using the F-test.
A simplified formula for the F statistic is:

where MS b is the mean squares between the groups and MS w is the mean squares within groups.
Repeated measures analysis of variance
As with ANOVA, repeated measures ANOVA analyses the equality of means of three or more groups. However, a repeated measure ANOVA is used when all variables of a sample are measured under different conditions or at different points in time.
As the variables are measured from a sample at different points of time, the measurement of the dependent variable is repeated. Using a standard ANOVA in this case is not appropriate because it fails to model the correlation between the repeated measures: The data violate the ANOVA assumption of independence. Hence, in the measurement of repeated dependent variables, repeated measures ANOVA should be used.
Non-parametric tests
When the assumptions of normality are not met, and the sample means are not normally, distributed parametric tests can lead to erroneous results. Non-parametric tests (distribution-free test) are used in such situation as they do not require the normality assumption.[ 15 ] Non-parametric tests may fail to detect a significant difference when compared with a parametric test. That is, they usually have less power.
As is done for the parametric tests, the test statistic is compared with known values for the sampling distribution of that statistic and the null hypothesis is accepted or rejected. The types of non-parametric analysis techniques and the corresponding parametric analysis techniques are delineated in Table 5 .
Analogue of parametric and non-parametric tests

Median test for one sample: The sign test and Wilcoxon's signed rank test
The sign test and Wilcoxon's signed rank test are used for median tests of one sample. These tests examine whether one instance of sample data is greater or smaller than the median reference value.
This test examines the hypothesis about the median θ0 of a population. It tests the null hypothesis H0 = θ0. When the observed value (Xi) is greater than the reference value (θ0), it is marked as+. If the observed value is smaller than the reference value, it is marked as − sign. If the observed value is equal to the reference value (θ0), it is eliminated from the sample.
If the null hypothesis is true, there will be an equal number of + signs and − signs.
The sign test ignores the actual values of the data and only uses + or − signs. Therefore, it is useful when it is difficult to measure the values.
Wilcoxon's signed rank test
There is a major limitation of sign test as we lose the quantitative information of the given data and merely use the + or – signs. Wilcoxon's signed rank test not only examines the observed values in comparison with θ0 but also takes into consideration the relative sizes, adding more statistical power to the test. As in the sign test, if there is an observed value that is equal to the reference value θ0, this observed value is eliminated from the sample.
Wilcoxon's rank sum test ranks all data points in order, calculates the rank sum of each sample and compares the difference in the rank sums.
Mann-Whitney test
It is used to test the null hypothesis that two samples have the same median or, alternatively, whether observations in one sample tend to be larger than observations in the other.
Mann–Whitney test compares all data (xi) belonging to the X group and all data (yi) belonging to the Y group and calculates the probability of xi being greater than yi: P (xi > yi). The null hypothesis states that P (xi > yi) = P (xi < yi) =1/2 while the alternative hypothesis states that P (xi > yi) ≠1/2.
Kolmogorov-Smirnov test
The two-sample Kolmogorov-Smirnov (KS) test was designed as a generic method to test whether two random samples are drawn from the same distribution. The null hypothesis of the KS test is that both distributions are identical. The statistic of the KS test is a distance between the two empirical distributions, computed as the maximum absolute difference between their cumulative curves.
Kruskal-Wallis test
The Kruskal–Wallis test is a non-parametric test to analyse the variance.[ 14 ] It analyses if there is any difference in the median values of three or more independent samples. The data values are ranked in an increasing order, and the rank sums calculated followed by calculation of the test statistic.
Jonckheere test
In contrast to Kruskal–Wallis test, in Jonckheere test, there is an a priori ordering that gives it a more statistical power than the Kruskal–Wallis test.[ 14 ]
Friedman test
The Friedman test is a non-parametric test for testing the difference between several related samples. The Friedman test is an alternative for repeated measures ANOVAs which is used when the same parameter has been measured under different conditions on the same subjects.[ 13 ]
Tests to analyse the categorical data
Chi-square test, Fischer's exact test and McNemar's test are used to analyse the categorical or nominal variables. The Chi-square test compares the frequencies and tests whether the observed data differ significantly from that of the expected data if there were no differences between groups (i.e., the null hypothesis). It is calculated by the sum of the squared difference between observed ( O ) and the expected ( E ) data (or the deviation, d ) divided by the expected data by the following formula:

A Yates correction factor is used when the sample size is small. Fischer's exact test is used to determine if there are non-random associations between two categorical variables. It does not assume random sampling, and instead of referring a calculated statistic to a sampling distribution, it calculates an exact probability. McNemar's test is used for paired nominal data. It is applied to 2 × 2 table with paired-dependent samples. It is used to determine whether the row and column frequencies are equal (that is, whether there is ‘marginal homogeneity’). The null hypothesis is that the paired proportions are equal. The Mantel-Haenszel Chi-square test is a multivariate test as it analyses multiple grouping variables. It stratifies according to the nominated confounding variables and identifies any that affects the primary outcome variable. If the outcome variable is dichotomous, then logistic regression is used.
SOFTWARES AVAILABLE FOR STATISTICS, SAMPLE SIZE CALCULATION AND POWER ANALYSIS
Numerous statistical software systems are available currently. The commonly used software systems are Statistical Package for the Social Sciences (SPSS – manufactured by IBM corporation), Statistical Analysis System ((SAS – developed by SAS Institute North Carolina, United States of America), R (designed by Ross Ihaka and Robert Gentleman from R core team), Minitab (developed by Minitab Inc), Stata (developed by StataCorp) and the MS Excel (developed by Microsoft).
There are a number of web resources which are related to statistical power analyses. A few are:
- StatPages.net – provides links to a number of online power calculators
- G-Power – provides a downloadable power analysis program that runs under DOS
- Power analysis for ANOVA designs an interactive site that calculates power or sample size needed to attain a given power for one effect in a factorial ANOVA design
- SPSS makes a program called SamplePower. It gives an output of a complete report on the computer screen which can be cut and paste into another document.
It is important that a researcher knows the concepts of the basic statistical methods used for conduct of a research study. This will help to conduct an appropriately well-designed study leading to valid and reliable results. Inappropriate use of statistical techniques may lead to faulty conclusions, inducing errors and undermining the significance of the article. Bad statistics may lead to bad research, and bad research may lead to unethical practice. Hence, an adequate knowledge of statistics and the appropriate use of statistical tests are important. An appropriate knowledge about the basic statistical methods will go a long way in improving the research designs and producing quality medical research which can be utilised for formulating the evidence-based guidelines.
Financial support and sponsorship
Conflicts of interest.
There are no conflicts of interest.
- Privacy Policy
Buy Me a Coffee

Home » Research Data – Types Methods and Examples
Research Data – Types Methods and Examples
Table of Contents

Research Data
Research data refers to any information or evidence gathered through systematic investigation or experimentation to support or refute a hypothesis or answer a research question.
It includes both primary and secondary data, and can be in various formats such as numerical, textual, audiovisual, or visual. Research data plays a critical role in scientific inquiry and is often subject to rigorous analysis, interpretation, and dissemination to advance knowledge and inform decision-making.
Types of Research Data
There are generally four types of research data:
Quantitative Data
This type of data involves the collection and analysis of numerical data. It is often gathered through surveys, experiments, or other types of structured data collection methods. Quantitative data can be analyzed using statistical techniques to identify patterns or relationships in the data.
Qualitative Data
This type of data is non-numerical and often involves the collection and analysis of words, images, or sounds. It is often gathered through methods such as interviews, focus groups, or observation. Qualitative data can be analyzed using techniques such as content analysis, thematic analysis, or discourse analysis.
Primary Data
This type of data is collected by the researcher directly from the source. It can include data gathered through surveys, experiments, interviews, or observation. Primary data is often used to answer specific research questions or to test hypotheses.
Secondary Data
This type of data is collected by someone other than the researcher. It can include data from sources such as government reports, academic journals, or industry publications. Secondary data is often used to supplement or support primary data or to provide context for a research project.
Research Data Formates
There are several formats in which research data can be collected and stored. Some common formats include:
- Text : This format includes any type of written data, such as interview transcripts, survey responses, or open-ended questionnaire answers.
- Numeric : This format includes any data that can be expressed as numerical values, such as measurements or counts.
- Audio : This format includes any recorded data in an audio form, such as interviews or focus group discussions.
- Video : This format includes any recorded data in a video form, such as observations of behavior or experimental procedures.
- Images : This format includes any visual data, such as photographs, drawings, or scans of documents.
- Mixed media: This format includes any combination of the above formats, such as a survey response that includes both text and numeric data, or an observation study that includes both video and audio recordings.
- Sensor Data: This format includes data collected from various sensors or devices, such as GPS, accelerometers, or heart rate monitors.
- Social Media Data: This format includes data collected from social media platforms, such as tweets, posts, or comments.
- Geographic Information System (GIS) Data: This format includes data with a spatial component, such as maps or satellite imagery.
- Machine-Readable Data : This format includes data that can be read and processed by machines, such as data in XML or JSON format.
- Metadata: This format includes data that describes other data, such as information about the source, format, or content of a dataset.
Data Collection Methods
Some common research data collection methods include:
- Surveys : Surveys involve asking participants to answer a series of questions about a particular topic. Surveys can be conducted online, over the phone, or in person.
- Interviews : Interviews involve asking participants a series of open-ended questions in order to gather detailed information about their experiences or perspectives. Interviews can be conducted in person, over the phone, or via video conferencing.
- Focus groups: Focus groups involve bringing together a small group of participants to discuss a particular topic or issue in depth. The group is typically led by a moderator who asks questions and encourages discussion among the participants.
- Observations : Observations involve watching and recording behaviors or events as they naturally occur. Observations can be conducted in person or through the use of video or audio recordings.
- Experiments : Experiments involve manipulating one or more variables in order to measure the effect on an outcome of interest. Experiments can be conducted in a laboratory or in the field.
- Case studies: Case studies involve conducting an in-depth analysis of a particular individual, group, or organization. Case studies typically involve gathering data from multiple sources, including interviews, observations, and document analysis.
- Secondary data analysis: Secondary data analysis involves analyzing existing data that was collected for another purpose. Examples of secondary data sources include government records, academic research studies, and market research reports.
Analysis Methods
Some common research data analysis methods include:
- Descriptive statistics: Descriptive statistics involve summarizing and describing the main features of a dataset, such as the mean, median, and standard deviation. Descriptive statistics are often used to provide an initial overview of the data.
- Inferential statistics: Inferential statistics involve using statistical techniques to draw conclusions about a population based on a sample of data. Inferential statistics are often used to test hypotheses and determine the statistical significance of relationships between variables.
- Content analysis : Content analysis involves analyzing the content of text, audio, or video data to identify patterns, themes, or other meaningful features. Content analysis is often used in qualitative research to analyze open-ended survey responses, interviews, or other types of text data.
- Discourse analysis: Discourse analysis involves analyzing the language used in text, audio, or video data to understand how meaning is constructed and communicated. Discourse analysis is often used in qualitative research to analyze interviews, focus group discussions, or other types of text data.
- Grounded theory : Grounded theory involves developing a theory or model based on an analysis of qualitative data. Grounded theory is often used in exploratory research to generate new insights and hypotheses.
- Network analysis: Network analysis involves analyzing the relationships between entities, such as individuals or organizations, in a network. Network analysis is often used in social network analysis to understand the structure and dynamics of social networks.
- Structural equation modeling: Structural equation modeling involves using statistical techniques to test complex models that include multiple variables and relationships. Structural equation modeling is often used in social science research to test theories about the relationships between variables.
Purpose of Research Data
Research data serves several important purposes, including:
- Supporting scientific discoveries : Research data provides the basis for scientific discoveries and innovations. Researchers use data to test hypotheses, develop new theories, and advance scientific knowledge in their field.
- Validating research findings: Research data provides the evidence necessary to validate research findings. By analyzing and interpreting data, researchers can determine the statistical significance of relationships between variables and draw conclusions about the research question.
- Informing policy decisions: Research data can be used to inform policy decisions by providing evidence about the effectiveness of different policies or interventions. Policymakers can use data to make informed decisions about how to allocate resources and address social or economic challenges.
- Promoting transparency and accountability: Research data promotes transparency and accountability by allowing other researchers to verify and replicate research findings. Data sharing also promotes transparency by allowing others to examine the methods used to collect and analyze data.
- Supporting education and training: Research data can be used to support education and training by providing examples of research methods, data analysis techniques, and research findings. Students and researchers can use data to learn new research skills and to develop their own research projects.
Applications of Research Data
Research data has numerous applications across various fields, including social sciences, natural sciences, engineering, and health sciences. The applications of research data can be broadly classified into the following categories:
- Academic research: Research data is widely used in academic research to test hypotheses, develop new theories, and advance scientific knowledge. Researchers use data to explore complex relationships between variables, identify patterns, and make predictions.
- Business and industry: Research data is used in business and industry to make informed decisions about product development, marketing, and customer engagement. Data analysis techniques such as market research, customer analytics, and financial analysis are widely used to gain insights and inform strategic decision-making.
- Healthcare: Research data is used in healthcare to improve patient outcomes, develop new treatments, and identify health risks. Researchers use data to analyze health trends, track disease outbreaks, and develop evidence-based treatment protocols.
- Education : Research data is used in education to improve teaching and learning outcomes. Data analysis techniques such as assessments, surveys, and evaluations are used to measure student progress, evaluate program effectiveness, and inform policy decisions.
- Government and public policy: Research data is used in government and public policy to inform decision-making and policy development. Data analysis techniques such as demographic analysis, cost-benefit analysis, and impact evaluation are widely used to evaluate policy effectiveness, identify social or economic challenges, and develop evidence-based policy solutions.
- Environmental management: Research data is used in environmental management to monitor environmental conditions, track changes, and identify emerging threats. Data analysis techniques such as spatial analysis, remote sensing, and modeling are used to map environmental features, monitor ecosystem health, and inform policy decisions.
Advantages of Research Data
Research data has numerous advantages, including:
- Empirical evidence: Research data provides empirical evidence that can be used to support or refute theories, test hypotheses, and inform decision-making. This evidence-based approach helps to ensure that decisions are based on objective, measurable data rather than subjective opinions or assumptions.
- Accuracy and reliability : Research data is typically collected using rigorous scientific methods and protocols, which helps to ensure its accuracy and reliability. Data can be validated and verified using statistical methods, which further enhances its credibility.
- Replicability: Research data can be replicated and validated by other researchers, which helps to promote transparency and accountability in research. By making data available for others to analyze and interpret, researchers can ensure that their findings are robust and reliable.
- Insights and discoveries : Research data can provide insights into complex relationships between variables, identify patterns and trends, and reveal new discoveries. These insights can lead to the development of new theories, treatments, and interventions that can improve outcomes in various fields.
- Informed decision-making: Research data can inform decision-making in a range of fields, including healthcare, business, education, and public policy. Data analysis techniques can be used to identify trends, evaluate the effectiveness of interventions, and inform policy decisions.
- Efficiency and cost-effectiveness: Research data can help to improve efficiency and cost-effectiveness by identifying areas where resources can be directed most effectively. By using data to identify the most promising approaches or interventions, researchers can optimize the use of resources and improve outcomes.
Limitations of Research Data
Research data has several limitations that researchers should be aware of, including:
- Bias and subjectivity: Research data can be influenced by biases and subjectivity, which can affect the accuracy and reliability of the data. Researchers must take steps to minimize bias and subjectivity in data collection and analysis.
- Incomplete data : Research data can be incomplete or missing, which can affect the validity of the findings. Researchers must ensure that data is complete and representative to ensure that their findings are reliable.
- Limited scope: Research data may be limited in scope, which can limit the generalizability of the findings. Researchers must carefully consider the scope of their research and ensure that their findings are applicable to the broader population.
- Data quality: Research data can be affected by issues such as measurement error, data entry errors, and missing data, which can affect the quality of the data. Researchers must ensure that data is collected and analyzed using rigorous methods to minimize these issues.
- Ethical concerns: Research data can raise ethical concerns, particularly when it involves human subjects. Researchers must ensure that their research complies with ethical standards and protects the rights and privacy of human subjects.
- Data security: Research data must be protected to prevent unauthorized access or use. Researchers must ensure that data is stored and transmitted securely to protect the confidentiality and integrity of the data.
About the author
Muhammad Hassan
Researcher, Academic Writer, Web developer
You may also like

Primary Data – Types, Methods and Examples

Qualitative Data – Types, Methods and Examples

Quantitative Data – Types, Methods and Examples

Secondary Data – Types, Methods and Examples

Information in Research – Types and Examples
Advertisement
A global analysis of habitat fragmentation research in reptiles and amphibians: what have we done so far?
- Review Paper
- Open access
- Published: 08 January 2023
- Volume 32 , pages 439–468, ( 2023 )
Cite this article
You have full access to this open access article
- W. C. Tan ORCID: orcid.org/0000-0002-6067-3528 1 ,
- A. Herrel ORCID: orcid.org/0000-0003-0991-4434 2 , 3 , 4 &
- D. Rödder ORCID: orcid.org/0000-0002-6108-1639 1
7468 Accesses
10 Citations
27 Altmetric
Explore all metrics
Habitat change and fragmentation are the primary causes of biodiversity loss worldwide. Recent decades have seen a surge of funding, published papers and citations in the field as these threats to biodiversity continue to rise. However, how research directions and agenda are evolving in this field remains poorly understood. In this study, we examined the current state of research on habitat fragmentation (due to agriculture, logging, fragmentation, urbanisation and roads) pertaining to two of the most threatened vertebrate groups, reptiles and amphibians. We did so by conducting a global scale review of geographical and taxonomical trends on the habitat fragmentation types, associated sampling methods and response variables. Our analyses revealed a number of biases with existing research efforts being focused on three continents (e.g., North America, Europe and Australia) and a surplus of studies measuring species richness and abundance. However, we saw a shift in research agenda towards studies utilising technological advancements including genetic and spatial data analyses. Our findings suggest important associations between sampling methods and prevalent response variables but not with the types of habitat fragmentation. These research agendas are found homogeneously distributed across all continents. Increased research investment with appropriate sampling techniques is crucial in biodiversity hotpots such as the tropics where unprecedented threats to herpetofauna exist.
Similar content being viewed by others
Habitat conservation research for amphibians: methodological improvements and thematic shifts.
Gentile Francesco Ficetola
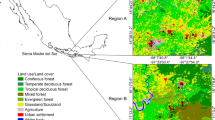
Effect of landscape composition and configuration on biodiversity at multiple scales: a case study with amphibians from Sierra Madre del Sur, Oaxaca, Mexico
Daniel G. Ramírez-Arce, Leticia M. Ochoa-Ochoa & Andrés Lira-Noriega
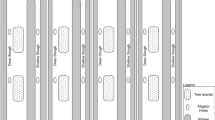
A dry future for the Everglades favors invasive herpetofauna
Hunter J. Howell, Giacomo L. Delgado, … Christopher A. Searcy
Avoid common mistakes on your manuscript.
Introduction
Habitat loss and fragmentation are the predominant causes underlying widespread biodiversity changes in terrestrial ecosystems (Fahrig 2003 ; Newbold et al. 2015 ). These processes may cause population declines by disrupting processes such as dispersal, gene flow, and survival. Over the past 30 years habitat loss and fragmentation have been suggested to have reduced biodiversity by up to 75% in different biomes around the world (Haddad et al. 2015 ). This is mainly due to the clearing of tropical forests, the expansion of agricultural landscapes, the intensification of farmland production, and the expansion of urban areas (FAO and UNEP 2020 ). The rate of deforestation and corresponding land conversions of natural habitats are happening rapidly and will continue to increase in the future at an accelerated rate, particularly in biodiversity hotspots (Deikumah et al. 2014 ; Habel et al. 2019 ; FAO and UNEP 2020 ).
For this reason, habitat fragmentation has been a central research focus for ecologists and conservationists over the past two decades (Fardila et al. 2017 ). However, habitat fragmentation consists of two different processes: loss of habitat and fragmentation of existing habitat (Fahrig 2003 ). The former simply means the removal of habitat, and latter is the transformation of continuous areas into discontinuous patches of a given habitat. In a radical review, Fahrig ( 2003 ) suggested that fragmentation per se, i.e., the breaking up of habitat after controlling for habitat loss, has a weaker or even no effect on biodiversity compared to habitat loss. She further recommended that the effects of these two components should be measured independently (Fahrig 2017 ). Despite being recognised as two different processes, researchers tend not to distinguish between their effects and commonly lump the combined consequences under a single umbrella term “habitat fragmentation” (Fahrig 2003 , 2017 ; Lindenmayer and Fischer 2007 ; Riva and Fahrig 2022 ). Nonetheless, fragmentation has been widely recognised in the literature and describes changes that occur in landscapes, including the loss of habitat (Hadley and Betts 2016 ). Hence, to avoid imprecise or inconsistent use of terminology and provide a holistic view of the effect of modified landscapes, we suggest the term “habitat fragmentation” to indicate any type of landscape change, both habitat loss and fragmentation throughout the current paper.
One main conundrum is that biodiversity decline does not occur homogeneously everywhere nor among all species (Blowes et al. 2019 ). Moreover, we should expect a global disparity in biodiversity responses to habitat fragmentation across different biomes (Newbold et al. 2020 ; Cordier et al. 2021 ). For example, tropical regions are predicted to have higher negative effects of habitat fragmentation than temperate regions. There are two possible reasons: a) higher intensification of land use change in the tropics (Barlow et al. 2018 ), and b) forest animals in the tropics are less likely to cross open areas (Lindell et al. 2007 ). Furthermore, individual species respond to landscape modification differently; some thrive whereas others decline (Fahrig 2003 ). Habitat specialists with broader habitat tolerance and wide-ranging distributions are most likely to benefit from increase landscape heterogeneity and more open and edge habitat (Hamer and McDonnell 2008 ; Newbold et al. 2014 ; Palmeirim et al. 2017 ). Therefore, appropriate response metrics should be used in measuring the effect of habitat fragmentation on biodiversity depending on the taxa group, biome and scale of study as patterns of richness can sometimes be masked by the abundance of generalist species (Riemann et al. 2015 ; Palmeirim et al. 2017 ).
Previous reviews have identified general patterns and responses of reptile and amphibian populations to habitat modification. They have been largely centred around specific types of habitat fragmentation: land use change (Newbold et al. 2020 ), logging (Sodhi et al. 2004 ), fragmentation per se (Fahrig 2017 ), urbanisation (Hamer and McDonnell 2008 ; McDonald et al. 2013 ), fire (Driscoll et al. 2021 ), and roads (Rytwinski and Fahrig 2012 ). Few reviews have, however, attempted a global synthesis of all types of land use changes and even fewer have addressed biases in geographical regions and taxonomical groups (but see Gardner et al. ( 2007 ) and Cordier et al. ( 2021 )). Gardner et al. ( 2007 ) synthesised the extant literature and focused on 112 papers on the consequences of habitat fragmentation on reptiles and amphibians published between 1945 and 2006. They found substantial biases across geographic regions, biomes, types of data collected as well as sampling design and effort. However, failure to report basic statistics by many studies prevented them from performing meta-analyses on research conclusions. More recently, a review by Cordier et al. ( 2021 ) conducted a meta-analysis based on 94 primary studies on the overall effects of land use changes through time and across the globe. Yet, there has been no comprehensive synthesis on the research patterns and agenda of published literature on habitat fragmentation associated with the recent advances of novel research tools and techniques. Therefore, our review may provide new insights of the evolution and biases in the field over the last decades and provide a basis for future research directions. Knowledge gaps caused by these biases could hamper the development of habitat fragmentation research and the implementation of effective strategies for conservation.
We aim to remedy this by examining research patterns for the two vertebrate classes Amphibia and Reptilia, at a global scale. We chose amphibians and reptiles for several reasons. First, habitat fragmentation research has been dominated by birds and mammals (Fardila et al. 2017 ). Reptiles and amphibians, on the other hand, are under-represented; together, they constitute only 10% of the studies (Fardila et al. 2017 ). Second, high proportions of amphibian and reptile species are threatened globally. To date, more than one third of amphibian (40%) and one in five reptile species (21%) are threatened with extinction (Stuart et al. 2004 ; Cox et al. 2022 ). Amphibians are known to be susceptible to land transformation as a result of their cryptic nature, habitat requirements, and reduced dispersal ability (Green 2003 ; Sodhi et al. 2008 ; Ofori‐Boateng et al. 2013 ; Nowakowski et al. 2017 ). Although poorly studied (with one in five species classified as data deficient) (Böhm et al. 2013 ), reptiles face the same threats as those impacting amphibians (Gibbons et al. 2000 ; Todd et al. 2010 ; Cox et al. 2022 ). Reptiles have small distributional ranges with high endemism compared to other vertebrates and as such are likely vulnerable to habitat fragmentation (Todd et al. 2010 ; Meiri et al. 2018 ). Third, both these groups are poikilotherms whose physiology makes them highly dependent on temperature and precipitation levels. Hence, they are very sensitive to changing thermal landscapes (Nowakowski et al. 2017 ).
Here, we first ask how is the published literature distributed across geographic regions and taxa? Is there a bias in the geographic distribution of species studied compared to known species? It is well known that conservation and research efforts are often concentrated in wealthy and English-speaking countries (Fazey et al. 2005 ), but has this bias improved over the years? Second, how are researchers conducting these studies? We assess whether certain sampling methods and response variables are associated to specific types of habitat fragmentation. Over the past decades new tools and techniques are constantly being discovered or developed. Combinations of methodologies are now shedding new light on biodiversity responses and consequences of habitat fragmentation. In particular, genetic techniques are useful in detecting changes in population structure, identifying isolated genetic clusters, and in estimating dispersal (Smith et al. 2016 ). Similarly, habitat occupancy and modelling can also provide powerful insights into dispersal (Driscoll et al. 2014 ). Remote sensing data are now used in analysing effects of area, edge, and isolation (Ray et al. 2002 ). Finally, how are these associations or research agendas distributed across space? We expect to find geographic structure of emerging agendas across the globe. For instance, we predict genetic studies to be located in North America and Europe but also in East Asian countries such as China and Japan as a result of their advancement in genetics (Forero et al. 2016 ). On the other hand, simple biodiversity response indicators which do not require extensive capacity building and application of advanced technologies are likely more used in developing regions of the world (Barber et al. 2014 ). These findings are valuable to evaluate and update the global status of our research on the effects of habitat fragmentation on herpetofauna and to suggest recommendations for conservation plans.
Materials and methods
Data collection.
We conducted the review according to the Preferred Reporting Items for Systematic Reviews and Meta-Analyses (PRISMA) guidelines (Fig. 1 ) (Moher et al. 2009 ). We conducted a comprehensive and exhaustive search using Web of Science to review published studies reporting the consequences of habitat fragmentation on amphibians and reptiles. We consulted the database in November 2019 by using two general search strings: (1) Habitat fragmentation AND (frog* OR amphib* OR salamander* OR tadpole*) (2) Habitat fragmentation AND (reptil* OR snake* OR lizard* OR turtle* OR crocodile*). This returned a total of 869 records from search (1) and 795 from search (2), with 1421 unique records remaining after duplicates were removed. We did not include “habitat loss” in our search term as it would only introduce unrelated articles focusing on biodiversity and conservation management instead of methodology and mechanistic approaches.
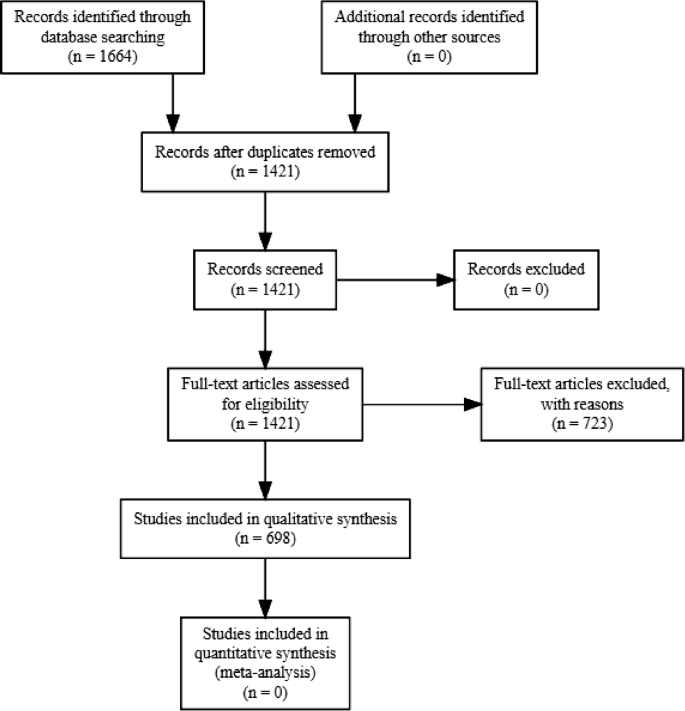
PRISMA flow-diagram of the study selection process
Throughout, we use the term papers to refer to individual journal article records. Out of the 1421 papers, we were unfortunately not able to locate seven papers from Acta Oecologica, Zoology: Analysis of Complex Systems, Israel Journal of Ecology and Evolution, Western North American Naturalist, Natural Areas Journal, Ecology, and the Herpetological Journal. We screened all articles from the title through the full text to determine whether they met our criteria for inclusion. To be included, studies needed to fulfil several criteria. First, papers needed to be peer-reviewed journal articles containing data collected on reptiles and/or amphibians at the species level (224 articles rejected because no species-specific data was available). Reviews and metastudies (n = 102) were excluded from the data analysis as they may represent duplicates as they are mainly based on data sets from other papers, but these form an integral part of our discussion. Furthermore, papers which do not provide data on contemporary time scales such as long-term (> 10, 000 years ago) changes on the paleo-spatial patterns (n = 59) were excluded. Because the effects of fragmentation per se have been measured inconsistently by many authors and have not been differentiated from habitat removal (Fahrig 2003 ), we consider any recent anthropogenic habitat degradation, and modification at patch or/and landscape scales during the Holocene as an effect of habitat fragmentation. Only papers which examined direct or indirect effects of habitat fragmentation were included in our analysis, regardless of the magnitude and direction. Papers which did not mention specific types of habitat fragmentation as the focus of their study (n = 338) were excluded.
Geographical and taxonomical distribution
Using the selected papers, we compiled a taxonomic and geographical database for each paper: (a) GPS or georeferenced location of the study site; (b) the focal group investigated (amphibian and/or reptile); (c) taxonomic groups (order, family, genus).
We listed the overall number of species studied covered by selected papers in each continent and compared them to the total number of currently described species. We obtained total described species of both reptiles and amphibians from the following sources: ReptileDatabase (Uetz et al. 2021 ) and AmphibiaWeb (AmphibiaWeb 2021 ). Then, we calculated the proportions of species covered by the selected papers compared to total number of described species for each continent. We did not update species nomenclature from selected papers as the mismatches from these potentially outdated taxonomies would be insignificant in our analyses.
Categorisation of papers
Each paper is classified into three main types of data collected: forms of habitat fragmentation, sampling methods, and response variables (Online Appendix 1). A paper can be classified into one or multiple categories in each type of data. The types of data and their following categories were:
Forms of habitat fragmentation
We recorded different types of habitat fragmentation from the selection of studies: (1) “Fragmentation” (includes patch isolation, edge and area effects); (2) “Agriculture” (includes any form of commercial and subsistence farming such as monocultures, plantations, and livestock farming); (3) “Logging” (e.g., agroforestry and silviculture); (4) “Mining” (presence of mining activities); (5) “Urbanisation” (includes presence of cities, towns or villages and parks created for recreational purposes); (6) “Road” (includes any vehicle roadway such as railways and highways) and (7) “Other types of habitat fragmentation” (e.g., fire, river dams, ditches, diseases, desertification etc.). Many studies deal with more than one type of habitat fragmentation. However, we made sure the selection for fragmentation forms is mainly based on the focus and wordings in the methodology section.
Sampling methods
We report trends in the design and sampling methods among the compiled studies over the last three decades. Due to the substantial variability in the level of sample design information reported by different studies, we narrowed them down into six general categories representing common sampling methods. Common methods used in estimating herpetofauna diversity (e.g., visual transect surveys, acoustic monitoring and trapping methods) were not included in the analyses due to their omnipresence in the data. The categories are:
(1) “Genetics” studies documented any use of codominant markers (i.e., allozymes and microsatellites), dominant markers [i.e., DNA sequences, random amplified polymorphic DNA (RAPDs) and amplified fragment length polymorphism (AFLPs)] to analyse genetic variability and gene diversity respectively. (2) “Direct tracking methods” studies measured potential dispersal distances or species movement patterns by means of radio telemetry, mark-recapture methods, or fluorescent powder tracking. (3) “Aerial photographs” studies reported the use of aerial photographs while (4) “GIS/Satellite image” studies described the use of satellite imagery and land cover data (i.e., Landsat) and GIS programs (e.g., QGIS and ArcGIS, etc.) in analysing spatial variables. (5) “Experimental” studies involved predictions tested through empirical studies, regardless if they occur naturally or artificially; in a natural or a captive environment. (6) “Prediction/simulation models” studies made use of techniques such as ecological niche models, habitat suitability (i.e., occurrence and occupancy models) and simulations for probability of survival and population connectivity.
Response variables
To further conceptualise how the effects of habitat fragmentation are measured, we assigned 12 biodiversity or ecological response variables. We recorded the type of data that was used in all selected studies: (1) “Species richness or diversity” which are measures of species richness, evenness or diversity (such as the Shannon–Wiener index) (Colwell 2009 ); (2) “Functional richness or species guilds” describes diversity indices based on functional traits (such as body size, reproductive modes, microhabitat association or taxonomic groups); (3) “Presence/absence” or species occupancy; (4) “Population” includes an estimation of population size or density (only when measured specifically in the paper). It includes genetic variation and divergence within and between populations; (5) “Abundance” or counts of individuals for comparison between habitat fragmentation type or species; (6) “Dispersal” takes into account any displacement or movement and can include indirect measurements of dispersal using genetic techniques; (7) “Breeding sites” which measures available breeding or reproduction sites; (8) “Fitness measure” are records of any physiological, ecological or behavioural changes; (9) “Interspecific interaction” depicts any interaction between species including competition and predation; (10) “Extinction or colonisation rate” counts the number of population extinctions or colonisations within a time period; (11) “Microhabitat preference” includes any direct observation made on an individual’s surrounding environmental features (substrate type, perch height, vegetation type, distance to cover etc.); (12) “Generalist or specialist comparison” involves any comparison made between generalist and specialist species. Generalists are able to thrive in various environments whereas specialists occupy a much narrower niche; (13) “Other response variables” can include road kill mortality counts, infection rate of diseases, injury, or any effect from introduced animals and a variety of other responses.
Data analysis
All statistical analyses were conducted in the open source statistical software package R 4.1.0 (R Core Team 2021 ). To gain a broad insight into our understanding of the complexity of habitat fragmentation we applied a Multiple Correspondence Analysis (MCA) (Roux and Rouanet 2004 ) and Hierarchical Clustering on Principle Components (HCPC) (Ward 1963 ) to investigate potential interactions between forms of habitat fragmentation, sampling methods and response variables. MCA is ideal for investigation of datasets with multiple categorical variables and exploration of unbiased relationships between these variables.
We first separate the dataset into papers concerning amphibians or reptiles. The MCA was performed using the MCA function from FactoMineR package of R version 3.1 (Lê et al. 2008 ). To identify subgroups (cluster) of similar papers within our dataset, we performed cluster analysis on our MCA results using HCPC. The cluster results are then visualised in factor map and dendrogram for easier interpretation using factoextra package. This allows us to identify categorical variables which have the highest effect within each cluster. Statistical analyses were considered significant at α = 0.05, while a p between 0.10 and 0.05 was considered as a tendency. The p-value is less than 5% when one category is significantly linked to other categories. The V tests show whether the category is over-expressed (positive values) or under-expressed (negative values) in the cluster (Lebart et al. 1995 ).
Results from the literature review were also analysed with VOSviewer, freeware for constructing and visualising bibliometric networks ( http://www.vosviewer.com/ ). The program uses clustering techniques to analyse co-authors, co-occurrence of keywords, citations, or co-citations (van Eck and Waltman 2014 ). First, we analyse co-authorships of countries to provide a geographical representation of groups of authors in various countries over the past 30 years. Each circle represents an author’s country and the size represents the collaboration frequency with other countries. The lines between the nodes represent the collaboration networks between the countries while the thickness of the lines indicates the collaboration intensities between them. Lastly, to complement the MCA and HCPC, we used VOSviewer to analyse a clustering solution of categories at an aggregate level. Aggregate clustering is a meta-clustering method to improve the robustness of clustering and does not require a priori information about the number of clusters. In this case, instead of author’s keywords, we used the co-occurrence of categories associated to each selected paper as input to run the software.
We identified a total of 698 papers published between January 1991 and November 2019 reporting consequences of habitat fragmentations corresponding to our selection criteria (Fig. 1 ). The complete list of studies included (hereafter termed “selected papers”) is available in Online Appendix 2. The distribution of these selected papers between focal groups and among continents was non-homogeneous (Fig. 2 ). Selected papers reviewed were predominantly studies which were conducted in North America 310 (44%) and Europe 131 (19%), but also from Oceania 104 (15%), South America 85 (12%), Asia 37 (5%) and Africa 31 (5%). For co-authorships between countries based on VOSviewer, the minimum document number of a country was set as 5 and a total of 21 and 14 countries met the threshold for amphibians and reptiles respectively (Fig. 3 ). For amphibians, countries in the American continent such as United States of America or USA (178 articles), Brazil (38 articles) and Canada (35 articles) have the largest research weight (Fig. 3 a). Authors from the USA have the largest international cooperation network, followed by Brazil. Australia and other European countries such as Germany, France and England also have high collaboration relationships with other countries. In contrast, reptile studies were mainly concentrated around two countries: the USA (139 articles) and Australia (86 articles) (Fig. 3 b). No other country from the rest of the world has more than 20 articles. While both the USA and Australia have the largest collaboration networks, Canada, Spain and Mexico are also highly cooperative with authors from other countries.
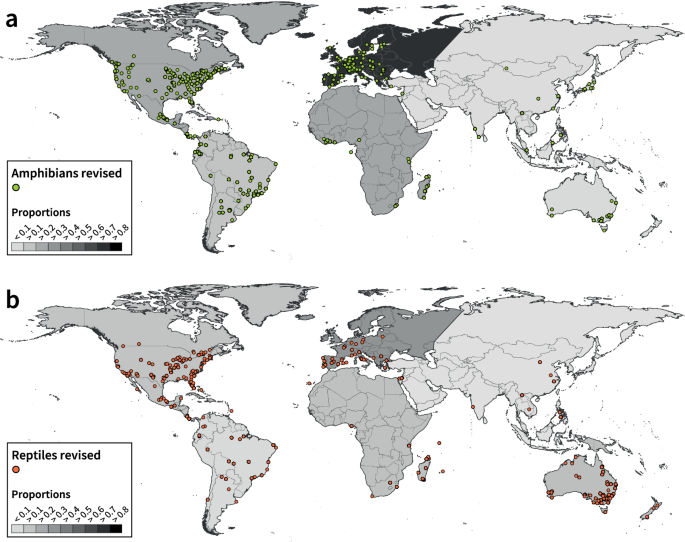
Map of study locations for a amphibians and b reptiles with each circle representing the study location of papers included in the review. The colour scale of the continents ranging from 0 – 0.9 indicates the proportions of amphibian and reptile species represented in the reviewed papers when compared to known species in the world (obtained from AmphibiaWeb and ReptileDatabase): a Europe (0.73), Africa (0.23), North America (0.23), South America (0.18), Oceania (0.07) and Asia (0.06) and b Europe (0.27), Oceania (0.18), Africa (0.12), North America (0.11), South America (0.09) and Asia (0.02)
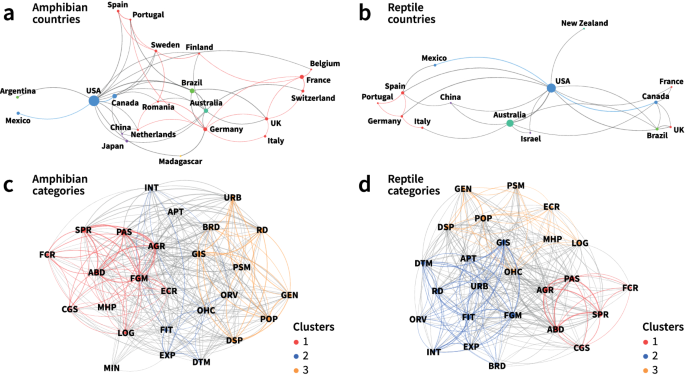
Co-authorship map of countries involved in habitat fragmentation research in a amphibians and b reptiles. The colours represent the continents countries belong to. Each circle represents an author’s country and the size represents the collaboration frequency with other countries. The lines between the nodes represent the collaboration networks between the countries while the thickness of the lines indicates the collaboration intensities between them. Category co-occurrence network maps for c amphibians and d reptiles. The colour represents the different cluster groups each category belongs to. Abbreviations for the categories in forms of habitat change: fragmentation (FGM), agriculture (AGR), Logging (LOG), Mining (MIN), Urbanisation (URB), road (RD), other habitat fragmentation (OHC); sampling methods: genetics (GEN), direct tracking method (DTM), aerial photographs (APT), GIS/ Satellite images (GIS), experimental (EXP), prediction/ simulation models (PSM) and response variables: species richness/ diversity (SPR), functional richness/ species guild (FCR), presence/ absence (PAS), population (POP), abundance (ABD), dispersal (DSP), breeding sites (BRD), fitness measure (FIT), interspecific interaction (INT),extinction/ colonisation rate (ECR), microhabitat preference (MHP), comparison between generalist and specialist (CGS), other response varialbes (ORV) (see also Online Appendix 1). Maps are created in VOSviewer
Overall, over half of all selected papers included only amphibians (376 papers; 54%), whilst 276 papers (39%) included only reptiles and 46 papers (7%) assessed both reptiles and amphibians. In relation to species richness, we identified 1490 amphibian species and 1199 reptile species across all papers; among which 141 taxa were not identified to species level but were still included in our analyses as taxonomic units analogous to species (Online Appendix 2). Among these species, more than half of the studied amphibians were found in South America (537; 38%) and North America (328; 23%), followed by Africa (297; 21%), Asia (137; 10%), Europe (77; 5%), and Oceania (51; 3%). Half of the reptile species studied were from North America (302; 25%) and Africa (278; 23%), with the other half consisting of species from Oceania (276; 23%), South America (200; 17%), Europe (76; 6%), and Asia (67; 6%).
When compared to the known species richness in the world, large portions of European species are studied while species from other continents were severely under-represented (Fig. 2 ). The proportions of amphibian species represented in papers were the highest in Europe (73%), while the proportions are much lower for Africa (23%), North America (23%), South America (18%), Oceania (7%) and Asia (6%) (Fig. 2 a). Among reptiles, Europe represents again the highest proportion of studied species (27%), followed by Oceania (18%), Africa (12%), North America (11%) and South America (8.9%) (Fig. 2 b). In contrast, of all Asian reptile species, only a mere 1.73% were included in the selected papers. The species coverage in our selected papers does not seem optimistic. Amphibians and reptiles each have only six families with more than half of the species covered (including three reptilian families containing one species in total). Meanwhile, 23 and 25 families remain fully neglected for amphibians and reptiles respectively (Figs. 4 – 5 ).
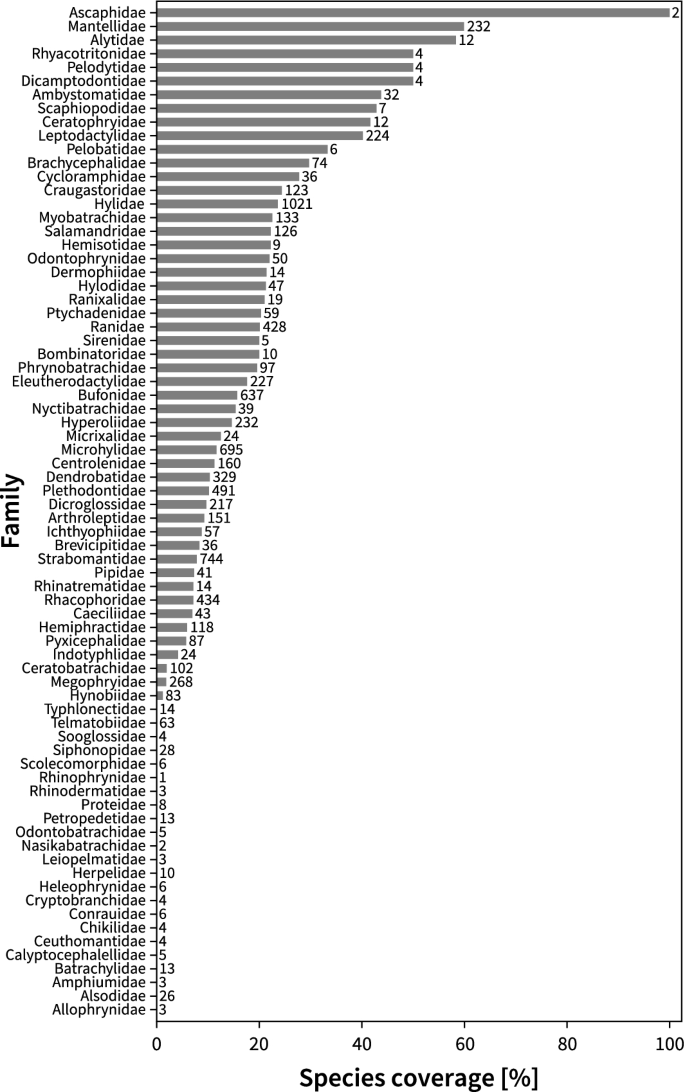
Species coverage for each taxonomic family in selected papers of amphibians. The numbers on each row indicate the total number of species known in its respective family (obtained from AmphibiaWeb 2021 )
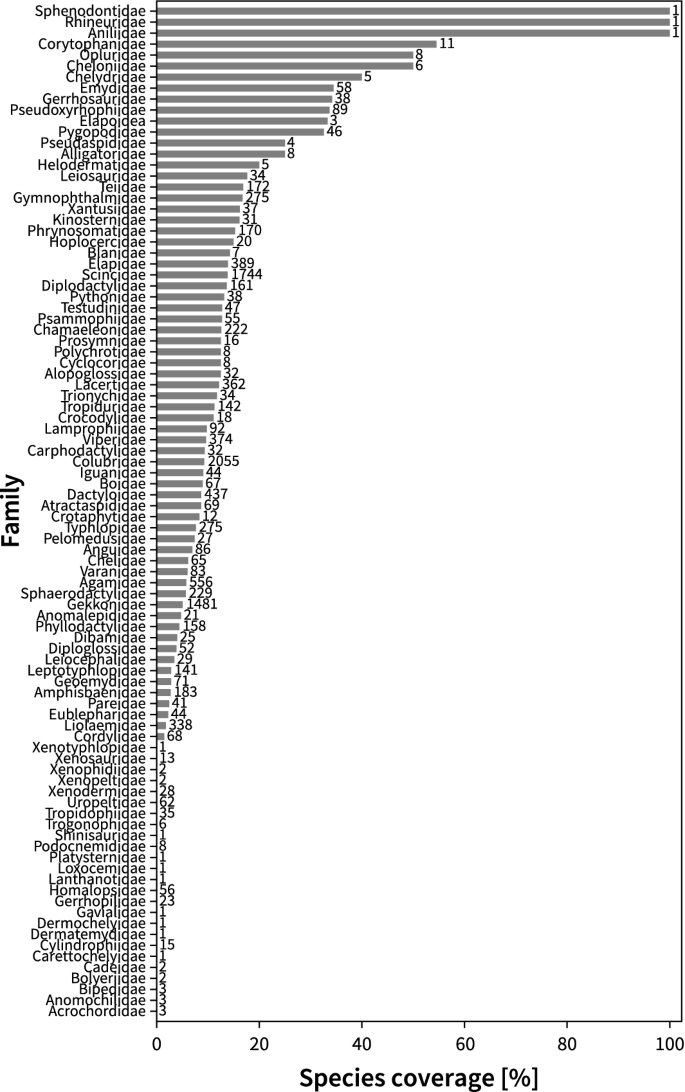
Species coverage for each taxonomic family in selected papers of reptiles. The numbers on each row indicate the total number of species known in its respective family (obtained from ReptileDatabase)
Multiple correspondence analysis provided important insights into underlying patterns in our data allowing us to visualise the relationship between forms of habitat fragmentation (Median = 1 [1–4]), sampling methods (Median = 1 [0–5]) and response variables (Median = 2 [1–6]). Percentage of variance (or eigenvalues) from MCA output represents the contribution of each dimension in explaining the observed patterns. The top ten new dimensions identified by MCA explained a total of 61.64% and 61.16% of the total variance for amphibians and reptiles respectively. The two dimensions with the highest variance percentages explained were found in the first (Dim1, 12.55%) and second (Dim2, 9.13%) dimensions in amphibians (Online Appendix 3–4). Genetics (sampling method; 13.73%) and population (response variable; 12.39%) contributed the most to Dim1, together with species richness (response variable;10.41%) and dispersal (response variable; 9.20%). For Dim2, experimental (sampling method; 14.38%) was the dominant variable, the rest was determined by GIS/Satellite images (sampling method; 9.71%), fitness measure (response variable; 9.12%) and urbanisation (form of fragmentation; 8.94%). For reptiles, the two dimensions explaining the most variation were the first (Dim1, 11.34%) and second (Dim2, 8.28%) dimensions (Online Appendix 3–4). The variables contributing the most to Dim1 were species richness (response variable; 15.51%), abundance (response variable; 10.11%), presence/absence (response variable; 6.97%) and genetics (sampling method; 6.39%). On the other hand, Dim2 was determined by interspecific interaction (response variable; 13.49%), genetics (12.79%), experimental methods (sampling method; 11.21%) and fitness measure (response variable; 10.94%). The contribution of each category to the definition of the dimensions is reported in Online Appendix 3. The categories identified in the MCA dimensions are subsequently used for building the distance matrix in the clustering analysis.
The HCPC analysis identified three clusters of variables for amphibians and reptiles (Online Appendix 5–6). The output of the HCPC analysis is reported in Online Appendix 7. V test represent the influence of variables in the cluster composition. In general, three clusters for both amphibians and reptiles appeared to be uniquely similar by definition of categories (Fig. 6 ). For amphibians, cluster 1 was defined by studies on species richness (p < 0.05, V test = 14.30) and presence/absence (p < 0.05, V test = 13.42), while cluster 2 was determined by experimental studies (p < 0.05, V test = 10.95) and fitness measures (p < 0.05, V test = 9.77). Cluster 3 was defined by genetics (p < 0.05, V test = 18.44) and population studies (p < 0.05, V test = 17.73) (Online Appendix 7). Abundance and functional richness were also unique to cluster 1; other response variables and direct tracking methods were important to cluster 2 and dispersal was present in cluster 3 even though these variables are less expressed (Fig. 6 a).
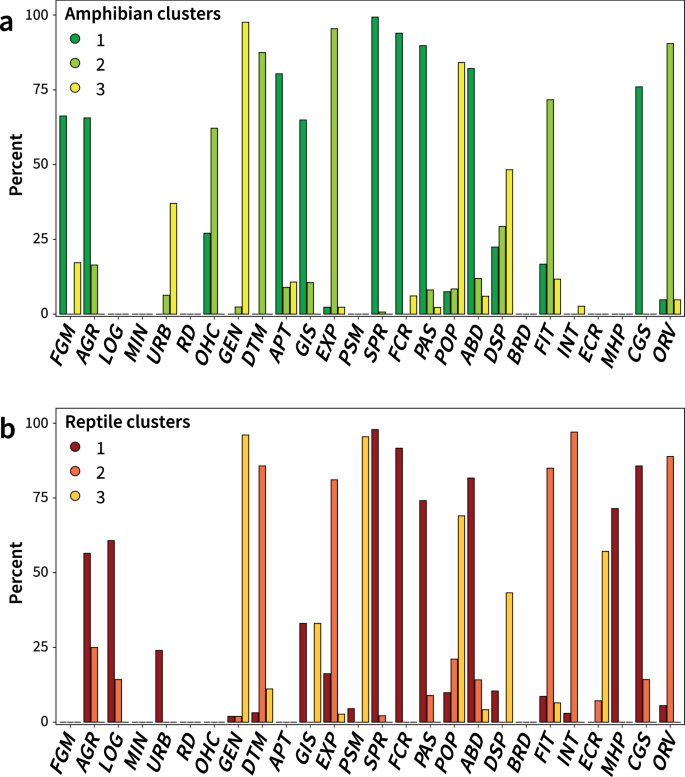
Percentage contribution of the categories contributing to the uniqueness of each cluster in amphibians (Dark green = 1, Bright green = 2, Bright yellow = 3) and reptiles (Dark red = 1, Orange = 2, Dark yellow = 3) based on the Cla/Mod results of HCPC (see Online Appendix 7). Abbreviations for the categories can be found in Fig. 3 and in Online Appendix 1
For reptiles, cluster 1 was represented by species richness (p < 0.05, V test = 14.26), abundance (p < 0.05, V test = 11.22) and presence absence (p < 0.05, V test = 8.55) papers, whereas cluster 2 was determined by papers on fitness measures (p < 0.05, V test = 10.99), direct tracking methods (p < 0.05, V test = 8.64) and interspecific interaction (p < 0.05, V test = 7.86), and cluster 3 was defined by genetics (p < 0.05, V test = 12.79), population (p < 0.05, V test = 9.95) and prediction/simulation models (p < 0.05, V test = 7.68) papers (Online Appendix 7). Although slightly less expressed in the clusters, papers using comparisons between generalist and specialist species and papers on functional richness were also unique to cluster 1; experimental methods and other response variables were heavily present in cluster 2, while dispersal studies were distinct to cluster 3 (Fig. 6 b).
Results from VOSviewer categories of both amphibians and reptiles appear to be similar to each other (Fig. 3 c, d). The clustering of the categories in the co-occurrence network maps confirms what we observed in the HCPC results (Fig. 6 ). In addition to geographical representation of study locations in (1), the corresponding clusters of selected papers are also mapped in Figs. 7 and 8 to investigate the spatial grouping patterns for the three clusters (see Online Appendix 8–9 for geographical representation for each category). We also plotted the temporal trend in Online Appendix 10 and 11. Overall, the three clusters are distributed homogeneously across the globe, but concentrated in the USA, Europe and south eastern Australia. Cluster 1 papers were found to be the most predominant cluster in amphibians (57% papers) across all continents (see Online Appendix 12; Fig. 7 ). When compared to other clusters, studies from this cluster are often conducted in Afrotropics, particularly Madagascar (100% papers), central (Costa Rica (60% papers) and Mexico (92% papers) and south America (80% papers) (Online Appendix 12, Figs. 7 , 8 ). On the other hand, cluster 2 papers appear to be more prevalent for reptile studies compared to amphibian studies, with a higher number of studies conducted across North America (65 to 51) and Australia (22 to 2) (Figs. 7 , 8 ). Lastly, a vast majority of cluster 3 papers were located in North America and Europe (both contributing to 79% of the papers) for amphibians and North America and Australia (both contributing to 84% of the papers) for reptiles (Online Appendix 12, Figs. 7 , 8 ). Publications from this cluster started to gain popularity from 2005 onwards, following similar increasing trends as cluster 2 (Online Appendix 10–11). Overall, except for cluster 1 in South America, most of the clusters in Asia and Africa appear to experience very little or no increase in publications over the years (Online Appendix 10–11).
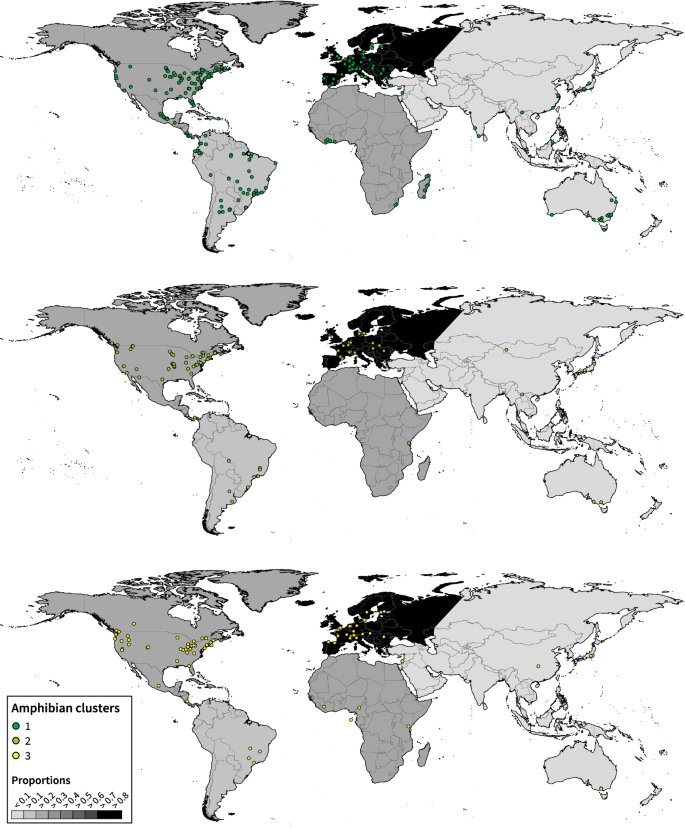
Map of the individual selected papers belonging to each cluster groups (Dark green = 1, Bright green = 2, Bright yellow = 3) for amphibians, with each circle representing the study location. The colour scale of the continents ranging from 0 to 0.9 indicates the proportions of amphibian species represented in the reviewed papers when compared to known species in the world (obtained from AmphibiaWeb)
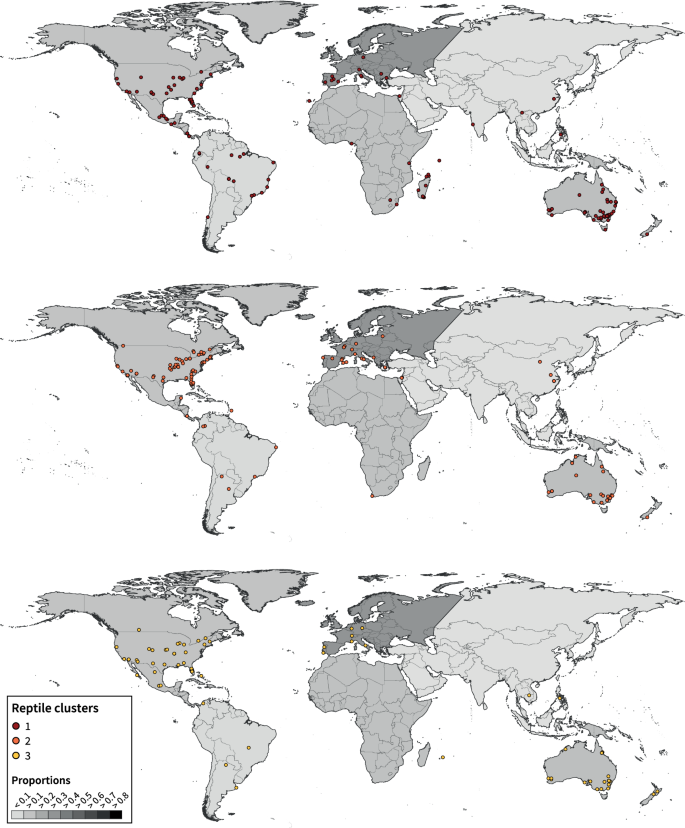
Map of the individual selected papers belonging to each cluster groups (Dark red=1, Orange=2, Dark yellow=3) for reptiles, with each circle representing the study location. The colour scale of the continents ranging from 0.0 – 0.9 indicates the proportions of reptile species represented in the reviewed papers when compared to known species in the world (obtained from ReptileDatabase).
Our review found no improvement in the geographical and taxonomic bias in habitat fragmentation studies for both reptiles and amphibians compared to earlier studies (Fardila et al. 2017 ). Yet, our study has made an effective contribution towards identifying major spatial gaps in habitat fragmentation studies over the past three decades (updating reviews such as Cushman 2006 ; Gardner et al. 2007 )). In particular, we found an overall increase in the number of studies measuring species richness and abundance throughout the years while population-level and genetics studies are still lacking in developing countries. Here, we discuss the issues of (1) biogeographical bias, (2) the extent and focus of habitat fragmentation research and (3) the limitations and knowledge gaps in habitat fragmentation research in herpetology and provide recommendations for future research.
Biogeographical bias
Geographic bias in research papers.
Given the research effort in relatively wealthy countries (Holmgren and Schnitzer 2004 ; Fazey et al. 2005 ) it is not surprising that more than half the papers concern North America and Europe, where there is strong prevalence of herpetological research. This pattern is also evident in other taxonomic groups and biological areas including invasion biology (Pyšek et al. 2008 ), biodiversity conservation (Trimble and Aarde 2012 ; Christie et al. 2020 ), and habitat fragmentation (Fardila et al. 2017 ). The USA alone contributed more than a third of the publications in terms of both authors and location of study (Fazey et al. 2005 ; Melles et al. 2019 ). English speaking countries including the USA, the United Kingdom, and Australia have dominated research output over the last 30 years (Melles et al. 2019 ). These patterns were reflected in the collaboration network maps generated by VOSviewer (Fig. 3 ). Similar hotspots found between who does the research (Fig. 3 ) and the study locations (Fig. 2 ) suggest that authors tend not to move much and only to study ecosystems near to where they are based (Meyer et al. 2015 ). One reason for this bias is the distance to field sites accentuated by the costs and time of travelling.
However, the near absence of studies from many parts of the world that are currently under extreme pressures of habitat loss and degradation are of great concern (Habel et al. 2019 ). We feel that the level of threat associated with habitat fragmentation in these continents is not proportional to the level of research attention required. Naturally biodiverse but less economically developed Southeast Asian and sub-Saharan countries will suffer greatest diversity losses in the next century (Newbold et al. 2015 ). If this persists at the current rate, biodiverse areas will likely disappear before new discoveries in those hotspots are made (Moura and Jetz 2021 ). Although conversely our study found that among other developing countries Brazil is currently conducting relatively more in-country amphibian studies and collaboration with other countries. However, how much of this information reaches decision makers and practitioners remains unknown. This is largely due to the lack of intermediary evidence bridges (Kadykalo et al. 2021 ). These intermediaries identify evidence summaries based on research and priorities and distribute them to practitioners, facilitating exchange of knowledge between and among researchers and practitioners (Holderegger et al. 2019 ; Kadykalo et al. 2021 ).
Geographic bias in focal groups
Congruent to results reported in Gardner et al. ( 2007 ), studies on amphibians were more abundant than studies on reptiles. Over the past years, there has been a strong focus on amphibian population declines. This was catalysed by the emergence of chytridiomycosis and global decline of amphibians (Fisher and Garner 2020 ). Amphibians, and predominantly frogs, are the principal focus of herpetological research, with the highest allocation of resources and the highest publication rates (Ferronato 2019 ). Another reason for this bias may be that amphibians serve as valuable indicators of environmental stress and degradation owing to their aquatic and terrestrial lifestyle and permeable skin (Green 2003 ). These attributes make them extremely sensitive to changes in temperature and precipitation as well as pollution (Sodhi et al. 2008 ). Lizards, also susceptible to temperature changes, however, are characterised by a high degree of endemism, restricted geographic ranges, late maturity, a long life-span and are thus very susceptible to population declines (Todd et al. 2010 ; Meiri et al. 2018 ). Certain groups of reptiles, such as worm lizards and blind snakes lead cryptic and solitary lives in contrast to the large breeding aggregations and choruses of, for example, frogs. Such characteristics make them difficult for researchers to study as they require large amount of search effort for little data (Thompson 2013 ).
- Taxonomic bias
We found a heightened geographical bias in the taxonomic coverage of studies. Given the sheer number of selected papers investigated, it is not surprising that the continents of North and South America cover more than half of the amphibian species studied whereas North America and Africa cover almost half of the reptile species studied. This trend broadly mirrors the geographic distribution pattern of the global described species in both these taxa (AmphibiaWeb 2021 ; Uetz et al. 2021 ). While a large proportion of the known European and North American families such as Alytidae and Ambystomatidae have been investigated (Fig. 4 ), species from other continents remain severely under-represented. Yet, the European continent represents only 2% of the described species globally. This high research intensity bias in low biodiverse regions of the world has been noted previously (Fazey et al. 2005 ). In general, reptiles and amphibians have been disproportionately poorly studied in the tropics and in developing areas despite that these areas show among the highest rates of deforestation and a corresponding rise in the number of threatened species (Böhm et al. 2013 ; Deikumah et al. 2014 ). These biodiverse areas largely consist of threatened species having restricted home ranges (Meiri et al. 2018 ). Even though we observed a great fraction of the species investigated in the Afrotropics (Vallan 2002 ; Hillers et al. 2008 ; Ofori‐Boateng et al. 2013 ; Riemann et al. 2015 ; Blumgart et al. 2017 ), especially Madagascar (see Mantellidae and Opluridae in Fig. 4 ), it seems insufficient when considering that an estimated 3.94 million hectares of forest area of the continent was cleared yearly over the last century (FAO and UNEP 2020 ). Further, biodiverse hotspots such as the neotropical regions and Indo-Malayan tropics have the highest chances of new species of amphibians and reptiles being discovered (Moura and Jetz 2021 ).
Being herpetofauna diversity hotspots, countries in South America and Asia are indeed understudied. Although Brazil has a high number of amphibian studies, less than one percent of known reptile species was studied in both continents (Fig. 2 ). A number of factors contribute to this lack of representation. First, there is an overwhelming number of new species being discovered every year in these hotspots (Moura et al. 2018 ; Moura and Jetz 2021 ). Furthermore, newly discovered species tend to belong to more secretive groups such as burrowing snakes, worm lizards and caecilians (Colli et al. 2016 ). Yet, these fossorial organisms are clearly neglected in fragmentation studies (see Fig. 4 – 5 ) with researchers focusing on well-known taxonomic groups (Böhm et al. 2013 ). On a positive note, despite having the country (Australia) with the highest reptile diversity (Uetz et al. 2021 ), Oceania represented a fair coverage of reptile diversity compared to other continents. Since 2001, there has been an increase of fragmentation studies in Australia (e.g., Brown 2001 ; Mac Nally and Brown 2001 ; Hazell et al. 2001 ) and there is a continuing increase in research output (Melles et al. 2019 ), contributing 85 out of 89 reviewed studies in Oceania over the last 30 years.
Extent and focus of research
Our findings showed important associations between methods and response metrics but not different forms of habitat fragmentation. This either suggests that researchers were not favouring any sampling method and response variable for evaluating the effects of certain habitat fragmentation or this pattern may occur due to a relatively even split of papers dealing with different forms or combinations of habitat fragmentation in the clusters. In general, species richness or diversity appears to explain most of the variation in our data ( see Online Appendix 4 ). While species richness remains a popular diversity metric employed in conservation biology (Online Appendix 12; also see Gardner et al. 2007 ), we also found an increasing trend in the use of genetic techniques for habitat fragmentation studies. More specifically in recent years, molecular genetics have become popular and are often studied together with population connectivity to capture species responses to habitat fragmentation ( see Online Appendix 4 ) (Keyghobadi 2007 ). The HCPC approach identified three main clusters of research fields which will be referred to as research agendas from here onwards. Contrary to our expectation, we did not find a global spatial pattern of research agendas, but instead a rather homogeneous distribution of papers, possibly due to the lack of selected studies which are found in developing countries outside USA, Europe and Australia (Figs. 7 , 8 ). This nevertheless indicates that different sampling methods are shared and used between leading herpetological experts from different countries and that there are continuing collaborations between countries, particularly in North America and Europe.
Below, we describe the research agendas and their corresponding categories (Fig. 6 ) that have contributed significantly to the study of habitat fragmentation for the past 30 years: (a) Agenda 1: Measures of direct individual species responses, (b) Agenda 2: Physiological and movement ecology, and (c) Agenda 3: Technology advancement in conservation research.
Agenda 1: Measures of direct individual species responses
We found that the majority of studies around the globe evaluated patterns of assemblage richness, species presence/absence, and abundance (Figs. 7 , 8 ). These simple patterns of richness, diversity and abundance are the most common responses measured because they provide a good indication of species response to habitat fragmentation and are easy to calculate (Colwell 2009 ). Although species richness does not consider abundance or biomass but treats each species as of equal importance to diversity, species evenness weighs each species by its relative abundance (Hill 1972). Further, composite measures like species diversity indices (e.g., Simpson’s 1/D or Shannon’s H) combine both richness and evenness in a single measure (Colwell 2009 ), preventing biases in results. However, directly measuring these species responses might not be ecologically relevant as they fail to account for patterns in species assemblage turnover. In fact, few selected papers (38 out of 697) in our study have attempted to categorise species into meaningful functional groups or guilds, despite that the categorisation of ecological functions such as habitat preference, taxonomic family, reproductive mode, and body size can be easily done (but see Knutson et al. 1999 ; Peltzer et al. 2006 ; Moreira and Maltchik 2014 ). Knutson et al.( 1999 ) was the first in our selected papers to group species with similar life-history characteristics into guilds and to examine their responses to landscape features. They observed negative associations between urban land use and anuran guilds. Analyses of guilds or functional groups can reveal contradictory results (but not always, see Moreira and Maltchik 2014 ). For example, the species richness of anurans in logged areas of West Africa is found to be as high as in primary habitat (Ernst et al. 2006 ). Yet, analyses of functional groups indicated significantly higher diversity in primary forest communities (Ernst et al. 2006 ). Similar differences were also observed for species with varying degrees of niche overlaps, habitat specialists, and for different continents (Ernst et al. 2006 ; Seshadri 2014 ). These results underline that species richness alone is a poor indicator of the functional value of species in the ecosystem as the relationships between functional diversity and species richness are inconsistent and can sometimes be redundant (functional diversity remains constant if assemblages are functionally similar; Riemann et al. 2017 ; Palmeirim et al. 2017 ; Silva et al. 2022 ). The results of some species richness studies may consequently provide misleading inferences regarding consequences of habitat fragmentation and conservation management (Gardner et al. 2007 ).
Although not substantially greater than the agendas 2 and 3, the measure of individual species responses has always been popular across the globe but also increasingly popular in the tropical and subtropical regions (e.g., South America and Africa; Online Appendix 10–11). For example, a research team led by Mark-Oliver Roedel from Germany has conducted numerous studies on Afrotropical amphibian communities (Hillers et al. 2008 ; Ofori‐Boateng et al. 2013 ; Riemann et al. 2017 ). Due to the higher biodiversity and species rarity in these regions compared to temperate areas, it is reasonable to expect a greater level of sampling effort in patterns of species richness, abundance, and guild assemblage to obtain comparisons of diversity with sufficient statistical power across different land use changes (Gardner et al. 2007 ). Access to highly specific expertise and most up to date methods and technology may not be available in these regions, and as such, study designs are limited to multispecies survey addressing simple patterns of diversity and species assemblages (Hetu et al. 2019 ). Unfortunately at the same time, these forest biomes holding the highest richness and abundance of amphibians and reptiles have showed consistent negative responses to land use changes (Cordier et al. 2021 ).
Agenda 2: physiological and movement ecology
We did not observe a strong association between occupancy and dispersal in our study. Perhaps this is because only a few papers investigated dispersal via habitat occupancy compared to the overwhelming proportions of papers examining the presence of species in response to habitat fragmentation in research agenda 1. Similarly, few studies measure dispersal with direct tracking methods, with the majority that discussed dispersal being based on indirect inferences, such as genetic divergence (see Fig. 3 c, d; Driscoll et al. 2014 ). Genetic approaches can be effective in situations where more direct approaches are not possible (Lowe and Allendorf 2010 ). For instance, using microsatellites and mitochondrial DNA, Buckland et al. ( 2014 ) found no migration occurring between isolated subpopulations of a forest day gecko ( Phelsuma guimbeaui ) in a fragmented forest and predicted a dramatic decrease in survival and allelic diversity in the next 50 years if no migration occurs (Buckland et al. 2014 ). In some cases, molecular markers also allow direct dispersal studies by assigning individuals to their parents or population of origin (Manel et al. 2005 ). However, there are limitations on when these techniques can be applied. Assignment tests require appropriate choices of molecular markers and sampling design to permit quantification of indices of dispersal (Broquet and Petit 2009 ; Lowe and Allendorf 2010 ). Parent–offspring analysis is constrained by the uncertainty in assessing whether offspring dispersal is completed at the time of sampling and sample size (Broquet and Petit 2009 ). Genetic tools may thus be best applied in combination with direct approaches because they contain complementary information (Lowe and Allendorf 2010 ; Safner et al. 2011 ; Smith et al. 2016 ).
Traditional approaches in habitat fragmentation research like radiotracking or capture-mark-recapture of animals can be effective in evaluating dispersal and ecological connectivity between populations. For example, based on mark-recapture data over a nine year period, facultative dispersal rates in an endangered amphibian ( Bombina variegata ) were found to be sex biased and relatively low from resulting patch loss (Cayuela et al. 2018 ). In our case, direct tracking methods are more commonly and effectively used in examining the impacts of habitat modification on changes in ecology directly relating to fitness (Fig. 6 ): home ranges (Price-Rees et al. 2013 ), foraging grounds (MacDonald et al. 2012 ) and survival rates (Breininger et al. 2012 ). Yet, such routine movements associated with resource exploitation do not reflect the biological reality and evolutionary consequences of how organisms change as landscape changes (Van Dyck and Baguette 2005 ). Instead, directed behavioural movements affecting dispersal processes (emigration, displacement or immigration) are crucial in determining the functional connectivity between populations in a fragmented landscape (Bonte et al. 2012 ). In one study, spotted salamanders Ambystoma maculatum tracked with fluorescent powder exhibited strong edge mediated behaviour when dispersing across borders between forest and field habitats and can perceive forest habitats from some distance (Pittman and Semlitsch 2013 ). Knowing such behaviour rules can improve predictions of the effects of habitat configuration on survival and dispersal. However, ongoing conversion of natural ecosystems to human modified land cover increases the need to consider various cover types that may be permeable to animal movements. As such, experimental approaches can be effective in examining the effect of matrix type on species movements as seen in our results (Fig. 6 ) (Rothermel and Semlitsch 2002 ; Mazerolle and Desrochers 2005 ). For example, researchers conducted experimental releases of post-metamorphic individuals of forest amphibians into different substrates and mapped the movements of paths and performance (Cline and Hunter Jr 2016 ). They showed that non-forest matrices with lower structural complexity influence the ability of frogs to travel across open cover and to orient themselves towards the forest from distances greater than 40–55 m. Therefore, it is inaccurate to assume matrix permeability to be uniform across all open-matrix types, particularly in amphibians (Cline and Hunter 2014 , 2016 ).
In addition, the ability to move and disperse is highly dependent on the range of external environments and internal physiological limits (Bonte et al. 2012 ), especially in reptiles and amphibians (Nowakowski et al. 2017 ). The study of physiological effects on movement was seen throughout our selected studies (Fig. 6 ). For example, higher temperatures and lower soil moisture in open habitats could increase evaporative water loss in salamanders (Rothermel and Semlitsch 2002 ). Other tests including interaction effects between landscape configuration and physiological constraints (e.g., dehydration rate Rothermel and Semlitsch 2002 ; Watling and Braga 2015 ); body size (Doherty et al. 2019 ) can be useful to better understand fitness and population persistence. We argue here that multidisciplinary projects examining movement physiology, behaviour and environmental constraints in addition to measuring distance moved are needed to progress this field.
Our results indicate a high bias of agenda 2 papers represented among developed countries, with a strong focus on reptiles compared to amphibians (Price-Rees et al. 2013 ; Doherty et al. 2019 ) (Online Appendix 12, Figs. 7 , 8 ). The adoption of direct tracking as well as genetic methods can be cost prohibitive in developing and poorer regions. However, cheaper and simpler methods to track individuals are increasing (Mennill et al. 2012 ; Cline and Hunter 2014 , 2016 ). Although existing application might not be ideal for reptiles and amphibians, new technologies for tagging and tracking small vertebrates are being developed including acoustic surveys and improved genetic methods (Broquet and Petit 2009 ; Mennill et al. 2012 ; Marques et al. 2013 ). While there are many improvements needed to obtain better quality dispersal data studies on movement ecology, reptiles and amphibians still only account for a mere 2.2% of the studies on dispersal when compared to plants and invertebrates which comprised over half of the studies based on a systematic review (Driscoll et al. 2014 ). Thus, we urge more studies to be conducted on these lesser-known taxa, especially in biodiverse regions. Given the limited dispersal in amphibians and reptiles, having a deeper understanding on their dispersal can be critical for the effective management and conservation of populations and metapopulations (Smith and Green 2005 ).
Agenda 3: technology advancement in conservation research
While community level approaches such as responses in species richness, occupancy, and abundance measure biodiversity response to habitat fragmentation, they are limited in inference because they do not reflect patterns of fitness across environmental gradients and landscape patterns. Instead, genetic structure at the population level can offer a higher resolution of species responses (Manel and Holderegger 2013 ). For instance, genetic erosion heavily affects the rate of species loss in many amphibian species (Allentoft and O’Brien 2010 ; Rivera‐Ortíz et al. 2015 ). Over the past decades we have seen a rapid increase in studies applying genetic analysis to assess the effects of habitat fragmentation (Keyghobadi 2007 ), reflecting the strength of these approaches. This growth is mostly evident in North America and Europe (but also Oceania for reptiles) ( Online Appendix 10–11). The availability of different genetic markers has been increasing, from microsatellites in the 1990s then shifting towards genotyping by sequencing (NGS) technologies that enable rapid genome-wide development (Allendorf et al. 2010 ; Monteiro et al. 2019 ). However, the study of population structure alone can lead to misleading results as environmental changes to species dynamics are not considered. The resistance imposed by landscape features on the dispersal of animals can ultimately shape gene flow and genetic structure (Bani et al. 2015 ; Pilliod et al. 2015 ; Monteiro et al. 2019 ).
To understand this, researchers combine genetic, land cover and climate variables to study the gene flow patterns across heterogeneous and fragmented landscapes (Manel and Holderegger 2013 ). Spatial analyses can be a powerful tool for monitoring biodiversity by quantifying environmental and landscape parameters. The growing interest in both landcover data and the rapid development of computer processing power prompted the development of new prediction methods, primarily in spatial models (Ray et al. 2002 ), ecological niche modelling (Urbina-Cardona and Loyola 2008 ; Tan et al. 2021 ), and landscape connectivity (Cushman et al. 2013 ; Ashrafzadeh et al. 2019 ). In some cases, niche models are useful in assessing the effectiveness of protected areas for endangered species (Urbina-Cardona and Loyola 2008 ; Tan et al. 2021 ).
The integration of genetic data in ecological niche models for recognising possible dispersal movements between populations were observed in our study (Fig. 3 c, d), especially in reptiles (Fig. 6b ). The hallmark of landscape genetics is the ability to estimate functional connectivity among populations and offer empirical approach of adaptive genetic variation in real landscapes to detect environmental factors driving evolutionary adaptation. The most common approach of landscape genetics is determining whether effective distances as determined by the presence of suitable habitat between populations, better predict genetic distances than do Euclidean distances (assuming spatially homogeneous landscape). However, straight-line geographic distance does not normally reflect true patterns of dispersal as landscape barriers or facilitators in a heterogeneous landscape could strongly affect gene flow (Emel and Storfer 2012 ; Fenderson et al. 2020 ). Therefore, in these cases, ecological distances or landscape resistance can often explain a greater deal of genetic variation between fragmented populations (Cushman 2006 ; Bani et al. 2015 ). Using a combination of habitat suitability modelling (e.g., Maxent, Phillips et al. 2017 ), multiple least-cost paths (LCPs) (Adriaensen et al. 2003 ) and the more recent circuit theory analysis (McRae et al. 2008 ) to investigate landscape resistance can be highly effective predicting potential pathways along which dispersal may occur, hence informing conservation management (Emel and Storfer 2012 ; Bani et al. 2015 ; Pilliod et al. 2015 ). To date, landscape genetics has been shown to be particularly useful in studying organisms with complex life histories (Emel and Storfer 2012 ; Shaffer et al. 2015 ). Yet, the applications of landscape genetics have been limited to contemporary patterns using modern genetic data. Few studies have benefitted from the inclusion of temporal genetic data (Fenderson et al. 2020 ). For example, historical DNA samples and heterochronous analyses could allow us to explore how anthropogenic impacts have affected past genetic diversity and population dynamics (Pacioni et al. 2015 ) and identify areas of future suitability of endangered animals in face of climate change (Nogués-Bravo et al. 2016 ). The possibility to investigate migration through spatiotemporal population connectivity can greatly improve the prediction of species responses under future landscape and climate change scenarios (Fenderson et al. 2020 ).
Population genetic and niche modelling studies for both taxa are rarely found in developing regions of the world, especially in Asia and Africa (Figs. 7 , 8 ). Even though conservation priorities are concentrated in these biodiverse regions, invaluable highly specific expertise such as conservation genetics and other contemporary methodologies might not be readily available due to lack of funding and infrastructure (Hetu et al. 2019 ). Thus, we encourage collaborations with the poorer countries initiated by foreign service providers from developed countries. Contrary to expectations, very few studies on conservation genetics were found in China and Japan despite their vast advances in genetic techniques. Fortunately, China has made substantial progress in the last 20 years in understanding human genetic history and interpreting genetic studies of human diseases (Forero et al. 2016 ) as well as biodiversity conservation (Wang et al. 2020 ), yet the same cannot be said for conservation genetics on reptiles and amphibians (Figs. 7 , 8 ), but see Fan et al. ( 2018 ) and Hu et al. ( 2021 ).
Limitations and knowledge gaps
The forms of habitat fragmentation which we categorised may not reflect the ecological impact in the real world as interactions between different habitat fragmentation forms were not accounted for. Although each of these forms of habitat fragmentation possesses serious environmental consequences, their combination could have severe synergistic impacts (Blaustein and Kiesecker 2002 ). For example, a fragmented landscape is not just reduced and isolated, but subject to other anthropogenic disturbances such as hunting, fire, invasive species, and pollution (Laurance and Useche 2009 ; Lazzari et al. 2022 ). Altered climatic conditions and emerging pathogens such as batrachochytrids can also interact with each other, and other threats (Fisher and Garner 2020 ). The use of habitat suitability models based on climatic scenarios, combined with hydrological and urbanisation models, are effective in detecting best to worst case scenarios and local extinctions, as shown for the spotted marsh frog ( Limnodynastes tasmaniensis ) (Wilson et al. 2013 ).
We acknowledge the bias of scientific research introduced from the limitation of search term to English-speaking literature on the geographic distribution of the papers we sampled (Konno et al. 2020 ; Angulo et al. 2021 ). In Latin American journals for example, we found a number of papers published in Spanish, but unfortunately, they did not fit the criteria of our selection (see Online Appendix 2). Conservation studies written in languages other than English are often published in local journals which do not normally go through international peer review.
The homogeneous distribution of the research agendas across geographical regions in our study may be explained by the lack of studies found in South America, Asia and Africa, preventing us to see a potentially dichotomous spatial pattern among the clusters. However, this reflects the current state of research and the challenges faced in less developed countries.
(4) Our study did not investigate whether habitat fragmentation has led to an improved or decreased biotic response. Predicting species response to habitat modification has been reviewed countless times (Rytwinski and Fahrig 2012 ; Driscoll et al. 2014 ; Doherty et al. 2020 ; Newbold et al. 2020 ; Cordier et al. 2021 ). Yet, these reviews often yield little or no general patterns (Doherty et al. 2020 ; Cordier et al. 2021 ). Response variables or traits measured are often found to be poor predictors of the impacts of habitat fragmentation. There are two possible explanations for this discrepancy. First, the strength and direction of the responses differs between species, ecophysiological groups (Rothermel and Semlitsch 2002 ), and phylogenetic or functional groups (Mazerolle and Desrochers 2005 ; Nowakowski et al. 2017 ). Second, responses in animals to different types of disturbance may be specific to the ecosystem where they live. Different biogeographic regions or biomes have different characteristics affecting local species (Lindell et al. 2007 ; Blowes et al. 2019 ; Newbold et al. 2020 ; Cordier et al. 2021 ).
Conclusions and recommendations
Our results underline promising research fields and geographic areas and may serve as a guideline or starting point for future habitat fragmentation studies. We suspect similar paradigms of geographic and thematic patterns to occur in other taxonomic groups.
Although studies dealing with habitat fragmentation impacts on mammals and birds are already widely recognised (Fardila et al. 2017 ), research on reptiles and amphibians has been lacking. We argue that amphibians and reptiles need more attention as they are equally or more threatened but highly neglected (Rytwinski and Fahrig 2012 ; Ferronato 2019 ; Cox et al. 2022 ).
Greater investment is required for studies in tropical and subtropical areas (Segovia et al. 2020 ), especially within the Asian continent. These areas are currently experiencing the highest rates of habitat loss (McDonald et al. 2013 ). Tropical specialists are further restricted to smaller geographic range sizes according to Rapoport’s rule which states that there is a positive latitudinal correlation with range size (Stevens 1989 ) (at least for amphibians in the Northern hemisphere where there is higher temperature and precipitation seasonality; Whitton et al. 2012 ). Having a small range size is often associated with negative responses to habitat modification (Doherty et al. 2020 ). Thus, more effort is needed in developing countries where the crisis is greatest and there is lack of funding and strong language barriers (Fazey et al. 2005 ). There is an urgent need to better integrate studies published in languages other than English with the broader international literature. Useful integration actions include training of local conservation biologists and promoting partnerships and research visits in these regions may have greater conservation consequences to understand global patterns of habitat modification (Meyer et al. 2015 ). Doing so will help remediate the sampling bias towards temperate generalists and will shed light on the fate of tropical specialists.
We encourage improved access to intermediary evidence-based conservation data (Kadykalo et al. 2021 ). Even when well-established genetic and genomic analyses have been proven to be promising area in herpetological conservation (Shaffer et al. 2015 ), there is a general lack of the transfer of knowledge between scientists and practitioners (Holderegger et al. 2019 ). As practitioners are generally interested in species monitoring and the evaluation of success of connectivity measures, an establishment of scientist-practitioner community to facilitate a platform for international exchange would help tremendously in future conservation planning and management (Holderegger et al. 2019 ).
Although different study designs and landscape measures have different strengths and limitations depending on the study objectives, we suggest reporting basic data to describe the effect of habitat fragmentation using standardised sampling methods, indices, and design (Holderegger et al. 2019 ). The results will allow future meta-analyses to be performed.
Incorporate remote sensing data, whenever possible, in studies involving habitat change and fragmentation. The use of niche modelling techniques combined with high resolution remote sensing has been instrumental in detecting potentially fragmented populations. With advances in landscape genomics, we are now able to examine the correlation between environmental factors and genomic data in natural populations (Manel and Holderegger 2013 ; Shaffer et al. 2015 ). Adopting such tools would be valuable in understanding how habitat amounts and configurations affect dispersal, survival, and population dynamics as well as the impacts of anthropogenic changes such as climate change (Shaffer et al. 2015 ).
Data availability
The datasets generated during the current study are available in Online Appendix 1. Codes used in the analyses are available from corresponding author on request.
Adriaensen F, Chardon JP, De Blust G et al (2003) The application of ‘least-cost’ modelling as a functional landscape model. Landsc Urban Plan 64:233–247. https://doi.org/10.1016/S0169-2046(02)00242-6
Article Google Scholar
Allendorf FW, Hohenlohe PA, Luikart G (2010) Genomics and the future of conservation genetics. Nat Rev Genet 11:697–709. https://doi.org/10.1038/nrg2844
Article CAS Google Scholar
Allentoft ME, O’Brien J (2010) Global amphibian declines, loss of genetic diversity and fitness: a review. Diversity 2:47–71. https://doi.org/10.3390/d2010047
AmphibiaWeb (2021) AmphibiaWeb. https://amphibiaweb.org/ . Accessed 22 Feb 2021
Angulo E, Diagne C, Ballesteros-Mejia L et al (2021) Non-English languages enrich scientific knowledge: the example of economic costs of biological invasions. Sci Total Environ 775:144441. https://doi.org/10.1016/j.scitotenv.2020.144441
Ashrafzadeh MR, Naghipour AA, Haidarian M et al (2019) Effects of climate change on habitat and connectivity for populations of a vulnerable, endemic salamander in Iran. Glob Ecol Conserv 19:e00637. https://doi.org/10.1016/j.gecco.2019.e00637
Bani L, Pisa G, Luppi M et al (2015) Ecological connectivity assessment in a strongly structured fire salamander ( Salamandra salamandra ) population. Ecol Evol 5:3472–3485. https://doi.org/10.1002/ece3.1617
Barber PH, Ablan-Lagman MCA, Ambariyanto, et al (2014) Advancing biodiversity research in developing countries: the need for changing paradigms. Bull Mar Sci 90:187–210. https://doi.org/10.5343/bms.2012.1108
Barlow J, França F, Gardner TA et al (2018) The future of hyperdiverse tropical ecosystems. Nature 559:517–526. https://doi.org/10.1038/s41586-018-0301-1
Blaustein AR, Kiesecker JM (2002) Complexity in conservation: lessons from the global decline of amphibian populations. Ecol Lett 5:597–608. https://doi.org/10.1046/j.1461-0248.2002.00352.x
Blowes SA, Supp SR, Antão LH et al (2019) The geography of biodiversity change in marine and terrestrial assemblages. Science 366:339–345. https://doi.org/10.1126/science.aaw1620
Blumgart D, Dolhem J, Raxworthy CJ (2017) Herpetological diversity across intact and modified habitats of Nosy Komba Island, Madagascar. J Nat Hist 51:625–642. https://doi.org/10.1080/00222933.2017.1287312
Böhm M, Collen B, Baillie JEM et al (2013) The conservation status of the world’s reptiles. Biol Conserv 157:372–385. https://doi.org/10.1016/j.biocon.2012.07.015
Bonte D, Van Dyck H, Bullock JM et al (2012) Costs of dispersal. Biol Rev 87:290–312. https://doi.org/10.1111/j.1469-185X.2011.00201.x
Breininger DR, Mazerolle MJ, Bolt MR et al (2012) Habitat fragmentation effects on annual survival of the federally protected eastern indigo snake: indigo snake survival. Anim Conserv 15:361–368. https://doi.org/10.1111/j.1469-1795.2012.00524.x
Broquet T, Petit EJ (2009) Molecular estimation of dispersal for ecology and population genetics. Annu Rev Ecol Evol Syst 40:193–216. https://doi.org/10.1146/annurev.ecolsys.110308.120324
Brown GW (2001) The influence of habitat disturbance on reptiles in a Box-Ironbark eucalypt forest of south-eastern Australia. Biodivers Conserv 10:161–176. https://doi.org/10.1023/A:1008919521638
Buckland S, Cole NC, Groombridge JJ et al (2014) High risks of losing genetic diversity in an endemic Mauritian gecko : implications for conservation. PLoS ONE 9:e93387. https://doi.org/10.1371/journal.pone.0093387
Cayuela H, Besnard A, Quay L et al (2018) Demographic response to patch destruction in a spatially structured amphibian population. J Appl Ecol 55:2204–2215. https://doi.org/10.1111/1365-2664.13198
Christie AP, Amano T, Martin PA et al (2020) The challenge of biased evidence in conservation. Conserv Biol 35:249–262. https://doi.org/10.1111/cobi.13577
Cline BB, Hunter ML Jr (2014) Different open-canopy vegetation types affect matrix permeability for a dispersing forest amphibian. J Appl Ecol 51:319–329. https://doi.org/10.1111/1365-2664.12197
Cline BB, Hunter ML Jr (2016) Movement in the matrix: substrates and distance-to-forest edge affect postmetamorphic movements of a forest amphibian. Ecosphere 7:e01202. https://doi.org/10.1002/ecs2.1202
Colli GR, Fenker J, Tedeschi LG et al (2016) In the depths of obscurity: Knowledge gaps and extinction risk of Brazilian worm lizards (Squamata, Amphisbaenidae). Biol Conserv 204:51–62. https://doi.org/10.1016/j.biocon.2016.07.033
Colwell R (2009) Biodiversity: concepts, patterns, and measurement. The Princeton guide to ecology. Princeton, Princeton University Press, pp 257–263
Chapter Google Scholar
Cordier JM, Aguilar R, Lescano JN et al (2021) A global assessment of amphibian and reptile responses to land-use changes. Biol Conserv 253:108863. https://doi.org/10.1016/j.biocon.2020.108863
Cox N, Young BE, Bowles P et al (2022) A global reptile assessment highlights shared conservation needs of tetrapods. Nature 605:285–290. https://doi.org/10.1038/s41586-022-04664-7
Cushman SA (2006) Effects of habitat loss and fragmentation on amphibians: a review and prospectus. Biol Conserv 128:231–240. https://doi.org/10.1016/j.biocon.2005.09.031
Cushman SA, Shirk AJ, Landguth EL (2013) Landscape genetics and limiting factors. Conserv Genet 14:263–274. https://doi.org/10.1007/s10592-012-0396-0
Deikumah JP, McAlpine CA, Maron M (2014) Biogeographical and taxonomic biases in tropical forest fragmentation research. Conserv Biol J Soc Conserv Biol 28:1522–1531. https://doi.org/10.1111/cobi.12348
Doherty TS, Fist CN, Driscoll DA (2019) Animal movement varies with resource availability, landscape configuration and body size: a conceptual model and empirical example. Landsc Ecol 34:603–614. https://doi.org/10.1007/s10980-019-00795-x
Doherty TS, Balouch S, Bell K et al (2020) Reptile responses to anthropogenic habitat modification: a global meta-analysis. Glob Ecol Biogeogr 29:1265–1279. https://doi.org/10.1111/geb.13091
Driscoll DA, Banks SC, Barton PS et al (2014) The trajectory of dispersal research in conservation biology. Syst Rev PLOS ONE 9:e95053. https://doi.org/10.1371/journal.pone.0095053
Driscoll DA, Armenteras D, Bennett AF et al (2021) How fire interacts with habitat loss and fragmentation. Biol Rev 96:976–998. https://doi.org/10.1111/brv.12687
Emel SL, Storfer A (2012) A decade of amphibian population genetic studies: synthesis and recommendations. Conserv Genet 13:1685–1689. https://doi.org/10.1007/s10592-012-0407-1
Ernst R, Linsenmair KE, Rödel M-O (2006) Diversity erosion beyond the species level: dramatic loss of functional diversity after selective logging in two tropical amphibian communities. Biol Conserv 133:143–155. https://doi.org/10.1016/j.biocon.2006.05.028
Fahrig L (2003) Effects of habitat fragmentation on biodiversity. Annu Rev Ecol Evol Syst 34:487–515. https://doi.org/10.1146/annurev.ecolsys.34.011802.132419
Fahrig L (2017) Ecological responses to habitat fragmentation per se. Annu Rev Ecol Evol Syst 48:1–23. https://doi.org/10.1146/annurev-ecolsys-110316-022612
Fan H, Hu Y, Wu Q et al (2018) Conservation genetics and genomics of threatened vertebrates in China. J Genet Genomics 45:593–601. https://doi.org/10.1016/j.jgg.2018.09.005
FAO and UNEP (2020) The State of the World’s Forests 2020: Forests, biodiversity and people. FAO and UNEP,
Fardila D, Kelly LT, Moore JL, McCarthy MA (2017) A systematic review reveals changes in where and how we have studied habitat loss and fragmentation over 20years. Biol Conserv 212:130–138. https://doi.org/10.1016/j.biocon.2017.04.031
Fazey I, Fischer J, Lindenmayer DB (2005) Who does all the research in conservation biology? Biodivers Conserv 14:917–934. https://doi.org/10.1007/s10531-004-7849-9
Fenderson LE, Kovach AI, Llamas B (2020) Spatiotemporal landscape genetics: investigating ecology and evolution through space and time. Mol Ecol 29:218–246. https://doi.org/10.1111/mec.15315
Ferronato B (2019) An assessment of funding and publication rates in herpetology. Herpetol J. https://doi.org/10.33256/hj29.4.264273
Fisher MC, Garner TWJ (2020) Chytrid fungi and global amphibian declines. Nat Rev Microbiol 18:332–343. https://doi.org/10.1038/s41579-020-0335-x
Forero DA, Wonkam A, Wang W et al (2016) Current needs for human and medical genomics research infrastructure in low and middle income countries. J Med Genet 53:438–440. https://doi.org/10.1136/jmedgenet-2015-103631
Gardner TA, Barlow J, Peres CA (2007) Paradox, presumption and pitfalls in conservation biology: the importance of habitat change for amphibians and reptiles. Biol Conserv 138:166–179. https://doi.org/10.1016/j.biocon.2007.04.017
Gibbons JW, Scott DE, Ryan TJ et al (2000) The Global Decline of Reptiles, Déjà Vu Amphibians: reptile species are declining on a global scale. Six significant threats to reptile populations are habitat loss and degradation, introduced invasive species, environmental pollution, disease, unsustainable use, and global climate change. Bioscience 50:653–666. https://doi.org/10.1641/0006-3568(2000)050[0653:TGDORD]2.0.CO;2
Green DM (2003) The ecology of extinction: population fluctuation and decline in amphibians. Biol Conserv 111:331–343. https://doi.org/10.1016/S0006-3207(02)00302-6
Habel JC, Rasche L, Schneider UA et al (2019) Final countdown for biodiversity hotspots. Conserv Lett 12:e12668. https://doi.org/10.1111/conl.12668
Haddad NM, Brudvig LA, Clobert J et al (2015) Habitat fragmentation and its lasting impact on Earth’s ecosystems. Sci Adv 1:e1500052. https://doi.org/10.1126/sciadv.1500052
Hadley AS, Betts MG (2016) Refocusing habitat fragmentation research using lessons from the last decade. Curr Landsc Ecol Rep 1:55–66. https://doi.org/10.1007/s40823-016-0007-8
Hamer AJ, McDonnell MJ (2008) Amphibian ecology and conservation in the urbanising world: a review. Biol Conserv 141:2432–2449. https://doi.org/10.1016/j.biocon.2008.07.020
Hazell D, Cunnningham R, Lindenmayer D et al (2001) Use of farm dams as frog habitat in an Australian agricultural landscape: factors affecting species richness and distribution. Biol Conserv 102:155–169. https://doi.org/10.1016/S0006-3207(01)00096-9
Hetu M, Koutouki K, Joly Y (2019) Genomics for All: International Open Science Genomics Projects and Capacity Building in the Developing World. Front Genet. https://doi.org/10.3389/fgene.2019.00095
Hillers A, Veith M, Rödel M-O (2008) Effects of forest fragmentation and habitat degradation on West African leaf-litter frogs. Conserv Biol 22:762–772. https://doi.org/10.1111/j.1523-1739.2008.00920.x
Holderegger R, Balkenhol N, Bolliger J et al (2019) Conservation genetics: linking science with practice. Mol Ecol 28:3848–3856. https://doi.org/10.1111/mec.15202
Holmgren M, Schnitzer SA (2004) Science on the rise in developing countries. PLOS Biol 2:e1. https://doi.org/10.1371/journal.pbio.0020001
Hu Y, Fan H, Chen Y et al (2021) Spatial patterns and conservation of genetic and phylogenetic diversity of wildlife in China. Sci Adv 7:eabd5725. https://doi.org/10.1126/sciadv.abd5725
Kadykalo AN, Buxton RT, Morrison P et al (2021) Bridging research and practice in conservation. Conserv Biol 35:1725–1737. https://doi.org/10.1111/cobi.13732
Keyghobadi NK (2007) The genetic implications of habitat fragmentation for animals. Can J Zool. https://doi.org/10.1139/Z07-095
Knutson MG, Sauer JR, Olsen DA et al (1999) Effects of Landscape Composition and Wetland Fragmentation on Frog and Toad Abundance and Species Richness in Iowa and Wisconsin, U.S.A. Conserv Biol 13:1437–1446. https://doi.org/10.1046/j.1523-1739.1999.98445.x
Konno K, Akasaka M, Koshida C et al (2020) Ignoring non-English-language studies may bias ecological meta-analyses. Ecol Evol 10:6373–6384. https://doi.org/10.1002/ece3.6368
Laurance WF, Useche DC (2009) Environmental synergisms and extinctions of tropical species. Conserv Biol 23:1427–1437. https://doi.org/10.1111/j.1523-1739.2009.01336.x
Lazzari J, Sato CF, Driscoll DA (2022) Traits influence reptile responses to fire in a fragmented agricultural landscape. Landsc Ecol 37:2363–2382. https://doi.org/10.1007/s10980-022-01417-9
Lê S, Josse J, Husson F (2008) FactoMineR: an R package for multivariate analysis. J Stat Softw 25:1–18. https://doi.org/10.18637/jss.v025.i01
Lebart L, Morineau A, Piron M (1995) Statistique exploratoire multidimensionnelle. Dunod Paris
Lindell CA, Riffell SK, Kaiser SA et al (2007) Edge responses of tropical and temperate birds. Wilson J Ornithol 119:205–220. https://doi.org/10.1676/05-133.1
Lindenmayer DB, Fischer J (2007) Tackling the habitat fragmentation panchreston. Trends Ecol Evol 22:127–132. https://doi.org/10.1016/j.tree.2006.11.006
Lowe WH, Allendorf FW (2010) What can genetics tell us about population connectivity? Mol Ecol 19:3038–3051. https://doi.org/10.1111/j.1365-294X.2010.04688.x
Mac Nally R, Brown GW (2001) Reptiles and habitat fragmentation in the box-ironbark forests of central Victoria, Australia: predictions, compositional change and faunal nestedness. Oecologia 128:116–125. https://doi.org/10.1007/s004420100632
MacDonald B, Lewison R, Madrak S et al (2012) Home ranges of East Pacific green turtles Chelonia mydas in a highly urbanized temperate foraging ground. Mar Ecol Prog Ser 461:211–221. https://doi.org/10.3354/meps09820
Manel S, Gaggiotti OE, Waples RS (2005) Assignment methods: matching biological questions with appropriate techniques. Trends Ecol Evol 20:136–142. https://doi.org/10.1016/j.tree.2004.12.004
Manel S, Holderegger R (2013) Ten years of landscape genetics. Trends Ecol Evol 28:614–621. https://doi.org/10.1016/j.tree.2013.05.012
Marques TA, Thomas L, Martin SW et al (2013) Estimating animal population density using passive acoustics. Biol Rev 88:287–309. https://doi.org/10.1111/brv.12001
Mazerolle MJ, Desrochers A (2005) Landscape resistance to frog movements. Can J Zool. https://doi.org/10.1139/z05-032
McDonald RI, Marcotullio PJ, Güneralp B (2013) Urbanization and Global Trends in Biodiversity and Ecosystem Services. In: Elmqvist T, Fragkias M, Goodness J et al (eds) Urbanization, Biodiversity and Ecosystem Services: Challenges and Opportunities: A Global Assessment. Springer, Dordrecht, pp 31–52
Google Scholar
McRae BH, Dickson BG, Keitt TH, Shah VB (2008) Using circuit theory to model connectivity in ecology, evolution, and conservation. Ecology 89:2712–2724. https://doi.org/10.1890/07-1861.1
Meiri S, Bauer AM, Allison A et al (2018) Extinct, obscure or imaginary: the lizard species with the smallest ranges. Divers Distrib 24:262–273. https://doi.org/10.1111/ddi.12678
Melles SJ, Scarpone C, Julien A et al (2019) Diversity of practitioners publishing in five leading international journals of applied ecology and conservation biology, 1987–2015 relative to global biodiversity hotspots. Écoscience 26:323–340. https://doi.org/10.1080/11956860.2019.1645565
Mennill DJ, Battiston M, Wilson DR et al (2012) Field test of an affordable, portable, wireless microphone array for spatial monitoring of animal ecology and behaviour. Methods Ecol Evol 3:704–712. https://doi.org/10.1111/j.2041-210X.2012.00209.x
Meyer C, Kreft H, Guralnick R, Jetz W (2015) Global priorities for an effective information basis of biodiversity distributions. Nat Commun 6:8221. https://doi.org/10.1038/ncomms9221
Moher D, Liberati A, Tetzlaff J et al (2009) Preferred reporting items for systematic reviews and meta-analyses: the PRISMA statement. PLoS Med 6:e1000097. https://doi.org/10.1371/journal.pmed.1000097
Monteiro WP, Veiga JC, Silva AR et al (2019) Everything you always wanted to know about gene flow in tropical landscapes (but were afraid to ask). PeerJ 7:e6446. https://doi.org/10.7717/peerj.6446
Moreira LFB, Maltchik L (2014) Does organic agriculture benefit anuran diversity in rice fields? Wetlands 34:725–733. https://doi.org/10.1007/s13157-014-0537-y
Moura MR, Jetz W (2021) Shortfalls and opportunities in terrestrial vertebrate species discovery. Nat Ecol Evol 5:631–639. https://doi.org/10.1038/s41559-021-01411-5
Moura MR, Costa HC, Peixoto MA et al (2018) Geographical and socioeconomic determinants of species discovery trends in a biodiversity hotspot. Biol Conserv 220:237–244. https://doi.org/10.1016/j.biocon.2018.01.024
Newbold T, Hudson LN, Phillips HRP et al (2014) A global model of the response of tropical and sub-tropical forest biodiversity to anthropogenic pressures. Proc R Soc B Biol Sci 281:20141371. https://doi.org/10.1098/rspb.2014.1371
Newbold T, Hudson LN, Hill SLL et al (2015) Global effects of land use on local terrestrial biodiversity. Nature 520:45–50. https://doi.org/10.1038/nature14324
Newbold T, Oppenheimer P, Etard A, Williams JJ (2020) Tropical and Mediterranean biodiversity is disproportionately sensitive to land-use and climate change. Nat Ecol Evol 4:1630–1638. https://doi.org/10.1038/s41559-020-01303-0
Nogués-Bravo D, Veloz S, Holt BG et al (2016) Amplified plant turnover in response to climate change forecast by Late Quaternary records. Nat Clim Change 6:1115–1119. https://doi.org/10.1038/nclimate3146
Nowakowski AJ, Watling JI, Whitfield SM et al (2017) Tropical amphibians in shifting thermal landscapes under land-use and climate change. Conserv Biol 31:96–105. https://doi.org/10.1111/cobi.12769
Ofori-Boateng C, Oduro W, Hillers A et al (2013) Differences in the Effects of Selective Logging on Amphibian Assemblages in Three West African Forest Types. Biotropica 45:94–101. https://doi.org/10.1111/j.1744-7429.2012.00887.x
Pacioni C, Hunt H, Allentoft ME et al (2015) Genetic diversity loss in a biodiversity hotspot: ancient DNA quantifies genetic decline and former connectivity in a critically endangered marsupial. Mol Ecol 24:5813–5828. https://doi.org/10.1111/mec.13430
Palmeirim AF, Vieira MV, Peres CA (2017) Herpetofaunal responses to anthropogenic forest habitat modification across the neotropics: insights from partitioning β-diversity. Biodivers Conserv 26:2877–2891. https://doi.org/10.1007/s10531-017-1394-9
Peltzer PM, Lajmanovich RC, Attademo AM, Beltzer AH (2006) Diversity of anurans across agricultural ponds in Argentina. In: Hawksworth DL, Bull AT (eds) Marine, Freshwater, and Wetlands Biodiversity Conservation. Springer, Dordrecht, pp 131–145
Phillips SJ, Anderson RP, Dudík M et al (2017) Opening the black box: an open-source release of Maxent. Ecography 40:887–893. https://doi.org/10.1111/ecog.03049
Pilliod DS, Arkle RS, Robertson JM et al (2015) Effects of changing climate on aquatic habitat and connectivity for remnant populations of a wide-ranging frog species in an arid landscape. Ecol Evol 5:3979–3994. https://doi.org/10.1002/ece3.1634
Pittman SE, Semlitsch RD (2013) Habitat type and distance to edge affect movement behavior of juvenile pond-breeding salamanders. J Zool 291:154–162. https://doi.org/10.1111/jzo.12055
Price-Rees SJ, Brown GP, Shine R (2013) Spatial ecology of bluetongue lizards ( Tiliqua spp.) in the Australian wet–dry tropics. Austral Ecol 38:493–503. https://doi.org/10.1111/j.1442-9993.2012.02439.x
Pyšek P, Richardson DM, Pergl J et al (2008) Geographical and taxonomic biases in invasion ecology. Trends Ecol Evol 23:237–244. https://doi.org/10.1016/j.tree.2008.02.002
R Core Team (2021) R: A language and environment for statistical computing
Ray N, Lehmann A, Joly P (2002) Modeling spatial distribution of amphibian populations: a GIS approach based on habitat matrix permeability. Biodivers Conserv 11:2143–2165. https://doi.org/10.1023/A:1021390527698
Riemann JC, Ndriantsoa SH, Raminosoa NR et al (2015) The value of forest fragments for maintaining amphibian diversity in Madagascar. Biol Conserv 191:707–715. https://doi.org/10.1016/j.biocon.2015.08.020
Riemann JC, Ndriantsoa SH, Rödel M-O, Glos J (2017) Functional diversity in a fragmented landscape — Habitat alterations affect functional trait composition of frog assemblages in Madagascar. Glob Ecol Conserv 10:173–183. https://doi.org/10.1016/j.gecco.2017.03.005
Riva F, Fahrig L (2022) Protecting many small patches will maximize biodiversity conservation for most taxa: the SS > SL principle. Preprints
Rivera-Ortíz FA, Aguilar R, Arizmendi MDC et al (2015) Habitat fragmentation and genetic variability of tetrapod populations. Anim Conserv 18:249–258. https://doi.org/10.1111/acv.12165
Rothermel BB, Semlitsch RD (2002) An Experimental investigation of landscape resistance of forest versus old-field habitats to emigrating juvenile amphibians. Conserv Biol 16:1324–1332. https://doi.org/10.1046/j.1523-1739.2002.01085.x
Roux BL, Rouanet H (2004) Geometric Data Analysis: From Correspondence Analysis to Structured Data Analysis. Springer Science, Dordrecht
Rytwinski T, Fahrig L (2012) Do species life history traits explain population responses to roads? A meta-analysis. Biol Conserv 147:87–98. https://doi.org/10.1016/j.biocon.2011.11.023
Safner T, Miaud C, Gaggiotti O et al (2011) Combining demography and genetic analysis to assess the population structure of an amphibian in a human-dominated landscape. Conserv Genet 12:161–173. https://doi.org/10.1007/s10592-010-0129-1
Segovia ALR, Romano D, Armsworth PR (2020) Who studies where? Boosting tropical conservation research where it is most needed. Front Ecol Environ 18:159–166. https://doi.org/10.1002/fee.2146
Seshadri KS (2014) Effects of Historical Selective Logging on Anuran Communities in a Wet Evergreen Forest, South India. Biotropica 46:615–623. https://doi.org/10.1111/btp.12141
Shaffer HB, Gidiş M, McCartney-Melstad E et al (2015) Conservation genetics and genomics of amphibians and reptiles. Annu Rev Anim Biosci 3:113–138. https://doi.org/10.1146/annurev-animal-022114-110920
Silva DJ, Palmeirim AF, Santos-Filho M et al (2022) Habitat Quality, Not Patch Size, Modulates Lizard Responses to Habitat Loss and Fragmentation in the Southwestern Amazon. J Herpetol 56:75–83. https://doi.org/10.1670/20-145
Smith MA, Green DM (2005) Dispersal and the metapopulation paradigm in amphibian ecology and conservation: are all amphibian populations metapopulations? Ecography 28:110–128. https://doi.org/10.1111/j.0906-7590.2005.04042.x
Smith AL, Landguth EL, Bull CM et al (2016) Dispersal responses override density effects on genetic diversity during post-disturbance succession. Proc R Soc B Biol Sci 283:20152934. https://doi.org/10.1098/rspb.2015.2934
Sodhi NS, Koh LP, Brook BW, Ng PKL (2004) Southeast Asian biodiversity: an impending disaster. Trends Ecol Evol 19:654–660. https://doi.org/10.1016/j.tree.2004.09.006
Sodhi NS, Bickford D, Diesmos AC et al (2008) Measuring the meltdown: drivers of global amphibian extinction and decline. PLoS ONE 3:e1636. https://doi.org/10.1371/journal.pone.0001636
Stevens GC (1989) The latitudinal gradient in geographical range: how so many species coexist in the tropics. Am Nat 133:240–256
Stuart SN, Chanson JS, Cox NA et al (2004) Status and trends of amphibian declines and extinctions worldwide. Science 306:1783–1786. https://doi.org/10.1126/science.1103538
Tan WC, Ginal P, Rhodin AGJ et al (2021) A present and future assessment of the effectiveness of existing reserves in preserving three critically endangered freshwater turtles in Southeast Asia and South Asia. Front Biogeogr. https://doi.org/10.21425/F5FBG50928
Thompson W (2013) Sampling Rare or Elusive Species: Concepts, Designs, and Techniques for Estimating Population Parameters. Island Press, Washington
Todd B, Willson J, Gibbons J (2010) The Global Status of Reptiles and Causes of Their Decline. Ecotoxicology of Amphibians and Reptiles. CRC Press, Boca Raton, pp 47–67
Trimble MJ, van Aarde RJ (2012) Geographical and taxonomic biases in research on biodiversity in human-modified landscapes. Ecosphere 3:art119. https://doi.org/10.1890/ES12-00299.1
Uetz P, Freed P, Aguilar R, Hošek J (2021) The Reptile Database. http://www.reptile-database.org/ . Accessed 6 Mar 2021
Urbina-Cardona JN, Loyola RD (2008) Applying niche-based models to predict endangered-hylid potential distributions: are neotropical protected areas effective enough? Trop Conserv Sci 1:417–445. https://doi.org/10.1177/194008290800100408
Vallan D (2002) Effects of anthropogenic environmental changes on amphibian diversity in the rain forests of Eastern Madagascar. J Trop Ecol 18:725–742
Van Dyck H, Baguette M (2005) Dispersal behaviour in fragmented landscapes: routine or special movements? Basic Appl Ecol 6:535–545. https://doi.org/10.1016/j.baae.2005.03.005
van Eck NJ, Waltman L (2014) Visualizing Bibliometric Networks. In: Ding Y, Rousseau R, Wolfram D (eds) Measuring Scholarly Impact: Methods and Practice. Springer International Publishing, Cham, pp 285–320
Wang W, Feng C, Liu F, Li J (2020) Biodiversity conservation in China: a review of recent studies and practices. Environ Sci Ecotechnology 2:100025. https://doi.org/10.1016/j.ese.2020.100025
Ward JH (1963) Hierarchical grouping to optimize an objective function. J Am Stat Assoc 58:236–244. https://doi.org/10.1080/01621459.1963.10500845
Watling JI, Braga L (2015) Desiccation resistance explains amphibian distributions in a fragmented tropical forest landscape. Landsc Ecol 30:1449–1459. https://doi.org/10.1007/s10980-015-0198-0
Whitton FJS, Purvis A, Orme CDL, Olalla-Tárraga MÁ (2012) Understanding global patterns in amphibian geographic range size: does Rapoport rule? Glob Ecol Biogeogr 21:179–190. https://doi.org/10.1111/j.1466-8238.2011.00660.x
Wilson JN, Bekessy S, Parris KM et al (2013) Impacts of climate change and urban development on the spotted marsh frog ( Limnodynastes tasmaniensis ). Austral Ecol 38:11–22. https://doi.org/10.1111/j.1442-9993.2012.02365.x
Download references
Acknowledgements
W.C. Tan was supported financially through a scholarship by the German Academic Exchange Service (DAAD). This work would not be possible without M. Flecks for his invaluable technical assistance with the figures.
Open Access funding enabled and organized by Projekt DEAL.
Author information
Authors and affiliations.
Herpetology Section, LIB, Museum Koenig, Bonn, Leibniz Institute for the Analysis of Biodiversity Change, Adenauerallee 127, 53113, Bonn, Germany
W. C. Tan & D. Rödder
UMR 7179 C.N.R.S/M.N.H.N., Département Adaptations du Vivant, Bâtiment d’Anatomie Comparée, 55 Rue Buffon, 75005, Paris, France
Department of Biology, Evolutionary Morphology of Vertebrates, Ghent University, K.L. Ledeganckstraat 35, 9000, Gent, Belgium
Department of Biology, University of Antwerp, Universiteitsplein 1, B-2610, Antwerpen, Belgium
You can also search for this author in PubMed Google Scholar
Contributions
WCT, AH, and DR contributed to the study idea and conception. Literature search and data collection were performed by WCT and data analysis by DR and WCT. The first draft of the manuscript was written by WCT and all authors critically revised on later versions. All authors read and approved the final manuscript.
Corresponding author
Correspondence to W. C. Tan .
Ethics declarations
Competing interests.
The authors declare no conflicts of interest.
Additional information
Communicated by Ricardo Correia.
Publisher's Note
Springer Nature remains neutral with regard to jurisdictional claims in published maps and institutional affiliations.
Online appendices
Below is the link to the electronic supplementary material.
Captions for appendices (PDF 288 kb)
Appendix 1(pdf 138 kb), appendix 2 (csv 3608 kb), appendix 3 (xlsx 47 kb), appendix 4 (pdf 113 kb), appendix 5 (pdf 293 kb), appendix 6 (pdf 80 kb), appendix 7 (xlsx 18 kb), appendix 8 (pdf 55343 kb), appendix 9 (pdf 55290 kb), appendix 10 (eps 5675 kb), appendix 11 (eps 5665 kb), appendix 12 (xlsx 13 kb), rights and permissions.
Open Access This article is licensed under a Creative Commons Attribution 4.0 International License, which permits use, sharing, adaptation, distribution and reproduction in any medium or format, as long as you give appropriate credit to the original author(s) and the source, provide a link to the Creative Commons licence, and indicate if changes were made. The images or other third party material in this article are included in the article's Creative Commons licence, unless indicated otherwise in a credit line to the material. If material is not included in the article's Creative Commons licence and your intended use is not permitted by statutory regulation or exceeds the permitted use, you will need to obtain permission directly from the copyright holder. To view a copy of this licence, visit http://creativecommons.org/licenses/by/4.0/ .
Reprints and permissions
About this article
Tan, W.C., Herrel, A. & Rödder, D. A global analysis of habitat fragmentation research in reptiles and amphibians: what have we done so far?. Biodivers Conserv 32 , 439–468 (2023). https://doi.org/10.1007/s10531-022-02530-6
Download citation
Received : 18 August 2022
Revised : 02 December 2022
Accepted : 09 December 2022
Published : 08 January 2023
Issue Date : February 2023
DOI : https://doi.org/10.1007/s10531-022-02530-6
Share this article
Anyone you share the following link with will be able to read this content:
Sorry, a shareable link is not currently available for this article.
Provided by the Springer Nature SharedIt content-sharing initiative
- Habitat change
- Herpetofauna
- Geographical bias
- Research agendas
- Systematic review
- Find a journal
- Publish with us
- Track your research
Thank you for visiting nature.com. You are using a browser version with limited support for CSS. To obtain the best experience, we recommend you use a more up to date browser (or turn off compatibility mode in Internet Explorer). In the meantime, to ensure continued support, we are displaying the site without styles and JavaScript.
- View all journals
- Explore content
- About the journal
- Publish with us
- Sign up for alerts
- Published: 08 April 2024
Tumor-selective activity of RAS-GTP inhibition in pancreatic cancer
- Urszula N. Wasko 1 , 2 na1 ,
- Jingjing Jiang 3 na1 ,
- Tanner C. Dalton 1 , 2 ,
- Alvaro Curiel-Garcia ORCID: orcid.org/0000-0001-6249-3267 1 , 2 ,
- A. Cole Edwards 4 ,
- Yingyun Wang 3 ,
- Bianca Lee 3 ,
- Margo Orlen ORCID: orcid.org/0000-0002-9834-6282 5 ,
- Sha Tian 6 ,
- Clint A. Stalnecker ORCID: orcid.org/0000-0002-0570-4416 7 , 8 ,
- Kristina Drizyte-Miller 7 ,
- Marie Menard 3 ,
- Julien Dilly ORCID: orcid.org/0000-0002-4006-5285 9 , 10 ,
- Stephen A. Sastra 1 , 2 ,
- Carmine F. Palermo 1 , 2 ,
- Marie C. Hasselluhn ORCID: orcid.org/0000-0001-9765-4075 1 , 2 ,
- Amanda R. Decker-Farrell 1 , 2 ,
- Stephanie Chang ORCID: orcid.org/0009-0000-2026-5215 3 ,
- Lingyan Jiang 3 ,
- Xing Wei 3 ,
- Yu C. Yang 3 ,
- Ciara Helland 3 ,
- Haley Courtney 3 ,
- Yevgeniy Gindin 3 ,
- Karl Muonio 3 ,
- Ruiping Zhao 3 ,
- Samantha B. Kemp 5 ,
- Cynthia Clendenin ORCID: orcid.org/0000-0003-4535-2088 11 ,
- Rina Sor ORCID: orcid.org/0000-0003-2042-5746 11 ,
- William P. Vostrejs ORCID: orcid.org/0000-0002-1659-0186 5 ,
- Priya S. Hibshman 4 ,
- Amber M. Amparo ORCID: orcid.org/0000-0003-3805-746X 7 ,
- Connor Hennessey 9 , 10 ,
- Matthew G. Rees ORCID: orcid.org/0000-0002-2987-7581 12 ,
- Melissa M. Ronan ORCID: orcid.org/0000-0003-4269-1404 12 ,
- Jennifer A. Roth ORCID: orcid.org/0000-0002-5117-5586 12 ,
- Jens Brodbeck 3 ,
- Lorenzo Tomassoni 2 , 13 ,
- Basil Bakir 1 , 2 ,
- Nicholas D. Socci 14 ,
- Laura E. Herring ORCID: orcid.org/0000-0003-4496-7312 15 ,
- Natalie K. Barker 15 ,
- Junning Wang 9 , 10 ,
- James M. Cleary 9 , 10 ,
- Brian M. Wolpin ORCID: orcid.org/0000-0002-0455-1032 9 , 10 ,
- John A. Chabot 16 ,
- Michael D. Kluger 16 ,
- Gulam A. Manji 1 , 2 ,
- Kenneth Y. Tsai ORCID: orcid.org/0000-0001-5325-212X 17 ,
- Miroslav Sekulic 18 ,
- Stephen M. Lagana 18 ,
- Andrea Califano 1 , 2 , 13 , 19 , 20 , 21 , 22 , 23 ,
- Elsa Quintana 3 ,
- Zhengping Wang 3 ,
- Jacqueline A. M. Smith ORCID: orcid.org/0000-0001-5028-8725 3 ,
- Matthew Holderfield 3 ,
- David Wildes ORCID: orcid.org/0009-0009-3855-7270 3 ,
- Scott W. Lowe ORCID: orcid.org/0000-0002-5284-9650 6 , 24 ,
- Michael A. Badgley 1 , 2 ,
- Andrew J. Aguirre ORCID: orcid.org/0000-0002-0701-6203 9 , 10 , 12 , 25 ,
- Robert H. Vonderheide ORCID: orcid.org/0000-0002-7252-954X 5 , 11 , 26 ,
- Ben Z. Stanger ORCID: orcid.org/0000-0003-0410-4037 5 , 11 ,
- Timour Baslan 27 ,
- Channing J. Der ORCID: orcid.org/0000-0002-7751-2747 7 , 8 ,
- Mallika Singh 3 &
- Kenneth P. Olive ORCID: orcid.org/0000-0002-3392-8994 1 , 2
Nature ( 2024 ) Cite this article
Metrics details
We are providing an unedited version of this manuscript to give early access to its findings. Before final publication, the manuscript will undergo further editing. Please note there may be errors present which affect the content, and all legal disclaimers apply.
- Pancreatic cancer
- Pharmacodynamics
Broad-spectrum RAS inhibition holds the potential to benefit roughly a quarter of human cancer patients whose tumors are driven by RAS mutations 1,2 . RMC-7977 is a highly selective inhibitor of the active GTP-bound forms of KRAS, HRAS, and NRAS, with affinity for both mutant and wild type (WT) variants (RAS(ON) multi-selective) 3 . As >90% of human pancreatic ductal adenocarcinoma (PDAC) cases are driven by activating mutations in KRAS 4 , we assessed the therapeutic potential of the RAS(ON) multi-selective inhibitor RMC-7977 in a comprehensive range of PDAC models. We observed broad and pronounced anti-tumor activity across models following direct RAS inhibition at exposures that were well-tolerated in vivo . Pharmacological analyses revealed divergent responses to RMC-7977 in tumor versus normal tissues. Treated tumors exhibited waves of apoptosis along with sustained proliferative arrest whereas normal tissues underwent only transient decreases in proliferation, with no evidence of apoptosis. In the autochthonous KPC model, RMC-7977 treatment resulted in a profound extension of survival followed by on-treatment relapse. Analysis of relapsed tumors identified Myc copy number gain as a prevalent candidate resistance mechanism, which could be overcome by combinatorial TEAD inhibition in vitro . Together, these data establish a strong preclinical rationale for the use of broad-spectrum RAS-GTP inhibition in the setting of PDAC and identify a promising candidate combination therapeutic regimen to overcome monotherapy resistance.
This is a preview of subscription content, access via your institution
Access options
Access Nature and 54 other Nature Portfolio journals
Get Nature+, our best-value online-access subscription
24,99 € / 30 days
cancel any time
Subscribe to this journal
Receive 51 print issues and online access
185,98 € per year
only 3,65 € per issue
Rent or buy this article
Prices vary by article type
Prices may be subject to local taxes which are calculated during checkout
Author information
These authors contributed equally: Urszula N. Wasko, Jingjing Jiang
Authors and Affiliations
Department of Medicine, Vagelos College of Physicians and Surgeons, Columbia University Irving Medical Center, New York, NY, USA
Urszula N. Wasko, Tanner C. Dalton, Alvaro Curiel-Garcia, Stephen A. Sastra, Carmine F. Palermo, Marie C. Hasselluhn, Amanda R. Decker-Farrell, Basil Bakir, Gulam A. Manji, Andrea Califano, Michael A. Badgley & Kenneth P. Olive
Herbert Irving Comprehensive Cancer Center, Columbia University Irving Medical Center, New York, NY, USA
Urszula N. Wasko, Tanner C. Dalton, Alvaro Curiel-Garcia, Stephen A. Sastra, Carmine F. Palermo, Marie C. Hasselluhn, Amanda R. Decker-Farrell, Lorenzo Tomassoni, Basil Bakir, Gulam A. Manji, Andrea Califano, Michael A. Badgley & Kenneth P. Olive
Revolution Medicines, Inc., Redwood City, CA, USA
Jingjing Jiang, Yingyun Wang, Bianca Lee, Marie Menard, Stephanie Chang, Lingyan Jiang, Xing Wei, Yu C. Yang, Ciara Helland, Haley Courtney, Yevgeniy Gindin, Karl Muonio, Ruiping Zhao, Jens Brodbeck, Elsa Quintana, Zhengping Wang, Jacqueline A. M. Smith, Matthew Holderfield, David Wildes & Mallika Singh
Department of Cell Biology and Physiology, University of North Carolina at Chapel Hill, Chapel Hill, NC, USA
A. Cole Edwards & Priya S. Hibshman
University of Pennsylvania Perelman School of Medicine, Department of Medicine, Philadelphia, PA, USA
Margo Orlen, Samantha B. Kemp, William P. Vostrejs, Robert H. Vonderheide & Ben Z. Stanger
Cancer Biology & Genetics Program, Sloan Kettering Institute, Memorial Sloan Kettering Cancer Center, New York, NY, USA
Sha Tian & Scott W. Lowe
Lineberger Comprehensive Cancer Center, University of North Carolina at Chapel Hill, Chapel Hill, NC, USA
Clint A. Stalnecker, Kristina Drizyte-Miller, Amber M. Amparo & Channing J. Der
Department of Pharmacology, University of North Carolina at Chapel Hill, Chapel Hill, NC, USA
Clint A. Stalnecker & Channing J. Der
Department of Medical Oncology, Dana-Farber Cancer Institute, Boston, MA, USA
Julien Dilly, Connor Hennessey, Junning Wang, James M. Cleary, Brian M. Wolpin & Andrew J. Aguirre
Harvard Medical School, Boston, MA, USA
University of Pennsylvania Perelman School of Medicine, Abramson Cancer Center, Philadelphia, PA, USA
Cynthia Clendenin, Rina Sor, Robert H. Vonderheide & Ben Z. Stanger
The Broad Institute of Harvard and MIT, Cambridge, MA, USA
Matthew G. Rees, Melissa M. Ronan, Jennifer A. Roth & Andrew J. Aguirre
Department of Systems Biology, Columbia University Irving Medical Center, New York, NY, USA
Lorenzo Tomassoni & Andrea Califano
Bioinformatics Core, Memorial Sloan Kettering Cancer Center, New York, NY, USA
Nicholas D. Socci
UNC Michael Hooker Proteomics Center, University of North Carolina at Chapel Hill, Chapel Hill, NC, USA
Laura E. Herring & Natalie K. Barker
Department of Surgery, Vagelos College of Physicians and Surgeons, Columbia University Irving Medical Center, New York, NY, USA
John A. Chabot & Michael D. Kluger
Departments of Pathology, Tumor Microenvironment and Metastasis; H. Lee Moffitt Cancer Center & Research Institute, Tampa, FL, USA
Kenneth Y. Tsai
Department of Pathology and Cell Biology, Columbia University Irving Medical Center, New York, NY, USA
Miroslav Sekulic & Stephen M. Lagana
Department of Oncology, The Johns Hopkins University School of Medicine, Baltimore, MD, USA
Andrea Califano
J.P. Sulzberger Columbia Genome Center, Columbia University, New York, NY, USA
Department of Biochemistry and Molecular Biophysics, Columbia University Irving Medical Center, New York, NY, USA
Department of Biomedical Informatics, Columbia University Irving Medical Center, New York, NY, USA
Chan Zuckerberg Biohub New York, New York, NY, USA
Howard Hughes Medical Institute, Chevy Chase, MD, USA
Scott W. Lowe
Department of Medicine, Brigham and Women’s Hospital, Boston, MA, USA
Andrew J. Aguirre
Parker Institute for Cancer Immunotherapy, San Francisco, CA, USA
Robert H. Vonderheide
Department of Biomedical Sciences, School of Veterinary Medicine, The University of Pennsylvania, Philadelphia, PA, USA
Timour Baslan
You can also search for this author in PubMed Google Scholar
Corresponding authors
Correspondence to Mallika Singh or Kenneth P. Olive .
Supplementary information
Supplementary figure 1.
uncropped Western Blot images with marked areas of interest, and target molecular weight.
Reporting Summary
Supplementary tables.
This file contains Supplementary Tables 1-10.
Rights and permissions
Reprints and permissions
About this article
Cite this article.
Wasko, U.N., Jiang, J., Dalton, T.C. et al. Tumor-selective activity of RAS-GTP inhibition in pancreatic cancer. Nature (2024). https://doi.org/10.1038/s41586-024-07379-z
Download citation
Received : 18 July 2023
Accepted : 02 April 2024
Published : 08 April 2024
DOI : https://doi.org/10.1038/s41586-024-07379-z
Share this article
Anyone you share the following link with will be able to read this content:
Sorry, a shareable link is not currently available for this article.
Provided by the Springer Nature SharedIt content-sharing initiative
By submitting a comment you agree to abide by our Terms and Community Guidelines . If you find something abusive or that does not comply with our terms or guidelines please flag it as inappropriate.
Quick links
- Explore articles by subject
- Guide to authors
- Editorial policies
Sign up for the Nature Briefing newsletter — what matters in science, free to your inbox daily.


- Facebook Icon
- Twitter Icon
- LinkedIn Icon
Linking Rapid Aging with Early-onset Cancer Risk
Abstract: An analysis of data from nearly 150,000 individuals has revealed that accelerated aging is more common in recent birth cohorts and correlates with an increased risk of several cancer types. These findings may help explain the recent troubling trend of rising cancer rates among younger adults.
Client Account
Advertisement
- Noted This Week Archive (2011-2023)
AACR Journals
- Blood Cancer Discovery
- Cancer Discovery
- Cancer Epidemiology, Biomarkers & Prevention
- Cancer Immunology Research
- Cancer Prevention Research
- Cancer Research
- Cancer Research Communications
- Clinical Cancer Research
- Molecular Cancer Research
- Molecular Cancer Therapeutics
- Info for Advertisers
- Information for Institutions/Librarians

- Privacy Policy
- Copyright © 2023 by the American Association for Cancer Research.
This Feature Is Available To Subscribers Only
Sign In or Create an Account

IMAGES
VIDEO
COMMENTS
Definition of research in data analysis: According to LeCompte and Schensul, research data analysis is a process used by researchers to reduce data to a story and interpret it to derive insights. The data analysis process helps reduce a large chunk of data into smaller fragments, which makes sense. Three essential things occur during the data ...
Quantitative data analysis then splits into mathematical analysis and artificial intelligence (AI) analysis. Mathematical types then branch into descriptive, diagnostic, predictive, and prescriptive. Methods falling under mathematical analysis include clustering, classification, forecasting, and optimization.
Exploratory analysis. Inferential analysis. Predictive analysis. Causal analysis. Mechanistic analysis. Prescriptive analysis. With its multiple facets, methodologies and techniques, data analysis is used in a variety of fields, including business, science and social science, among others. As businesses thrive under the influence of ...
Data Analysis. Definition: Data analysis refers to the process of inspecting, cleaning, transforming, and modeling data with the goal of discovering useful information, drawing conclusions, and supporting decision-making. It involves applying various statistical and computational techniques to interpret and derive insights from large datasets.
A method of data analysis that is the umbrella term for engineering metrics and insights for additional value, direction, and context. By using exploratory statistical evaluation, data mining aims to identify dependencies, relations, patterns, and trends to generate advanced knowledge.
data analysis, the process of systematically collecting, cleaning, transforming, describing, modeling, and interpreting data, generally employing statistical techniques. Data analysis is an important part of both scientific research and business, where demand has grown in recent years for data-driven decision making.Data analysis techniques are used to gain useful insights from datasets, which ...
What Is Data Analysis? (With Examples) Data analysis is the practice of working with data to glean useful information, which can then be used to make informed decisions. "It is a capital mistake to theorize before one has data. Insensibly one begins to twist facts to suit theories, instead of theories to suit facts," Sherlock Holme's proclaims ...
In data analytics and data science, there are four main types of data analysis: Descriptive, diagnostic, predictive, and prescriptive. In this post, we'll explain each of the four and consider why they're useful. If you're interested in a particular type of analysis, jump straight to the relevant section using the clickable menu below ...
The first type of data analysis is descriptive analysis. It is at the foundation of all data insight. It is the simplest and most common use of data in business today. ... There is a lot of free data out there, ready for you to use for school projects, for market research, or just for fun. Before you get too crazy, though, you need to be aware ...
Data analysis can be quantitative, qualitative, or mixed methods. Quantitative research typically involves numbers and "close-ended questions and responses" (Creswell & Creswell, 2018, p. 3).Quantitative research tests variables against objective theories, usually measured and collected on instruments and analyzed using statistical procedures (Creswell & Creswell, 2018, p. 4).
The type of quantitative data you have (specifically, level of measurement and the shape of the data). And, Your research questions and hypotheses; Let's take a closer look at each of these. Factor 1 - Data type. The first thing you need to consider is the type of data you've collected (or the type of data you will collect).
Data analysis is the process of cleaning, transforming, and interpreting data to uncover insights, patterns, and trends. It plays a crucial role in decision making, problem solving, and driving innovation across various domains. In addition to further exploring the role data analysis plays this blog post will discuss common data analysis ...
Types of Data Analysis Techniques in Research. Data analysis techniques in research are categorized into qualitative and quantitative methods, each with its specific approaches and tools. These techniques are instrumental in extracting meaningful insights, patterns, and relationships from data to support informed decision-making, validate ...
Quantitative data has to be gathered and cleaned before proceeding to the stage of analyzing it. Below are the steps to prepare a data before quantitative research analysis: Step 1: Data Collection. Before beginning the analysis process, you need data. Data can be collected through rigorous quantitative research, which includes methods such as ...
For many researchers unfamiliar with qualitative research, determining how to conduct qualitative analyses is often quite challenging. Part of this challenge is due to the seemingly limitless approaches that a qualitative researcher might leverage, as well as simply learning to think like a qualitative researcher when analyzing data. From framework analysis (Ritchie & Spencer, 1994) to content ...
QDA Method #1: Qualitative Content Analysis. Content analysis is possibly the most common and straightforward QDA method. At the simplest level, content analysis is used to evaluate patterns within a piece of content (for example, words, phrases or images) or across multiple pieces of content or sources of communication. For example, a collection of newspaper articles or political speeches.
Data analysis is a catalyst for continuous improvement. It allows organizations to monitor performance metrics, track progress, and identify areas for enhancement. This iterative process of analyzing data, implementing changes, and analyzing again leads to ongoing refinement and excellence in processes and products.
Data analysis is simply the process of converting the gathered data to meanin gf ul information. Different techniques such as modeling to reach trends, relatio nships, and therefore conclusions to ...
To analyze data collected in a statistically valid manner (e.g. from experiments, surveys, and observations). Meta-analysis. Quantitative. To statistically analyze the results of a large collection of studies. Can only be applied to studies that collected data in a statistically valid manner. Thematic analysis.
Data analysis tools make it easier for users to process and manipulate data, analyze the relationships and correlations between data sets, and it also helps to identify patterns and trends for interpretation. Here is a complete list of tools used for data analysis in research. Types of Data Analysis: Techniques and Methods
Abstract. Statistical methods involved in carrying out a study include planning, designing, collecting data, analysing, drawing meaningful interpretation and reporting of the research findings. The statistical analysis gives meaning to the meaningless numbers, thereby breathing life into a lifeless data. The results and inferences are precise ...
Analysis Methods. Some common research data analysis methods include: Descriptive statistics: Descriptive statistics involve summarizing and describing the main features of a dataset, such as the mean, median, and standard deviation. Descriptive statistics are often used to provide an initial overview of the data.
Data Preparation: The first step in quantitative data analysis is to prepare the data for analysis. This involves data validation, editing, and coding. Ensuring the accuracy and reliability of your data is crucial for obtaining meaningful results. Descriptive Analysis: Quantitative research often employs descriptive analysis, which yields ...
Yet incidence and mortality data of high quality remain sparse in many transitioning countries. Given the critical importance of building capacity for local data production, analysis, and dissemination within the countries themselves, the Global Initiative for Cancer Registry Development was launched by the IARC in 2012.
Each paper is classified into three main types of data collected: forms of habitat fragmentation, sampling methods, and response variables (Online Appendix 1). A paper can be classified into one or multiple categories in each type of data. The types of data and their following categories were: Forms of habitat fragmentation
Together, these data establish a strong preclinical rationale for the use of broad-spectrum RAS-GTP inhibition in the setting of PDAC and identify a promising candidate combination therapeutic ...
Abstract: An analysis of data from nearly 150,000 individuals has revealed that accelerated aging is more common in recent birth cohorts and correlates with an increased risk of several cancer types. These findings may help explain the recent troubling trend of rising cancer rates among younger adults.