

What Is A Research (Scientific) Hypothesis? A plain-language explainer + examples
By: Derek Jansen (MBA) | Reviewed By: Dr Eunice Rautenbach | June 2020
If you’re new to the world of research, or it’s your first time writing a dissertation or thesis, you’re probably noticing that the words “research hypothesis” and “scientific hypothesis” are used quite a bit, and you’re wondering what they mean in a research context .
“Hypothesis” is one of those words that people use loosely, thinking they understand what it means. However, it has a very specific meaning within academic research. So, it’s important to understand the exact meaning before you start hypothesizing.
Research Hypothesis 101
- What is a hypothesis ?
- What is a research hypothesis (scientific hypothesis)?
- Requirements for a research hypothesis
- Definition of a research hypothesis
- The null hypothesis
What is a hypothesis?
Let’s start with the general definition of a hypothesis (not a research hypothesis or scientific hypothesis), according to the Cambridge Dictionary:
Hypothesis: an idea or explanation for something that is based on known facts but has not yet been proved.
In other words, it’s a statement that provides an explanation for why or how something works, based on facts (or some reasonable assumptions), but that has not yet been specifically tested . For example, a hypothesis might look something like this:
Hypothesis: sleep impacts academic performance.
This statement predicts that academic performance will be influenced by the amount and/or quality of sleep a student engages in – sounds reasonable, right? It’s based on reasonable assumptions , underpinned by what we currently know about sleep and health (from the existing literature). So, loosely speaking, we could call it a hypothesis, at least by the dictionary definition.
But that’s not good enough…
Unfortunately, that’s not quite sophisticated enough to describe a research hypothesis (also sometimes called a scientific hypothesis), and it wouldn’t be acceptable in a dissertation, thesis or research paper . In the world of academic research, a statement needs a few more criteria to constitute a true research hypothesis .
What is a research hypothesis?
A research hypothesis (also called a scientific hypothesis) is a statement about the expected outcome of a study (for example, a dissertation or thesis). To constitute a quality hypothesis, the statement needs to have three attributes – specificity , clarity and testability .
Let’s take a look at these more closely.
Need a helping hand?
Hypothesis Essential #1: Specificity & Clarity
A good research hypothesis needs to be extremely clear and articulate about both what’ s being assessed (who or what variables are involved ) and the expected outcome (for example, a difference between groups, a relationship between variables, etc.).
Let’s stick with our sleepy students example and look at how this statement could be more specific and clear.
Hypothesis: Students who sleep at least 8 hours per night will, on average, achieve higher grades in standardised tests than students who sleep less than 8 hours a night.
As you can see, the statement is very specific as it identifies the variables involved (sleep hours and test grades), the parties involved (two groups of students), as well as the predicted relationship type (a positive relationship). There’s no ambiguity or uncertainty about who or what is involved in the statement, and the expected outcome is clear.
Contrast that to the original hypothesis we looked at – “Sleep impacts academic performance” – and you can see the difference. “Sleep” and “academic performance” are both comparatively vague , and there’s no indication of what the expected relationship direction is (more sleep or less sleep). As you can see, specificity and clarity are key.
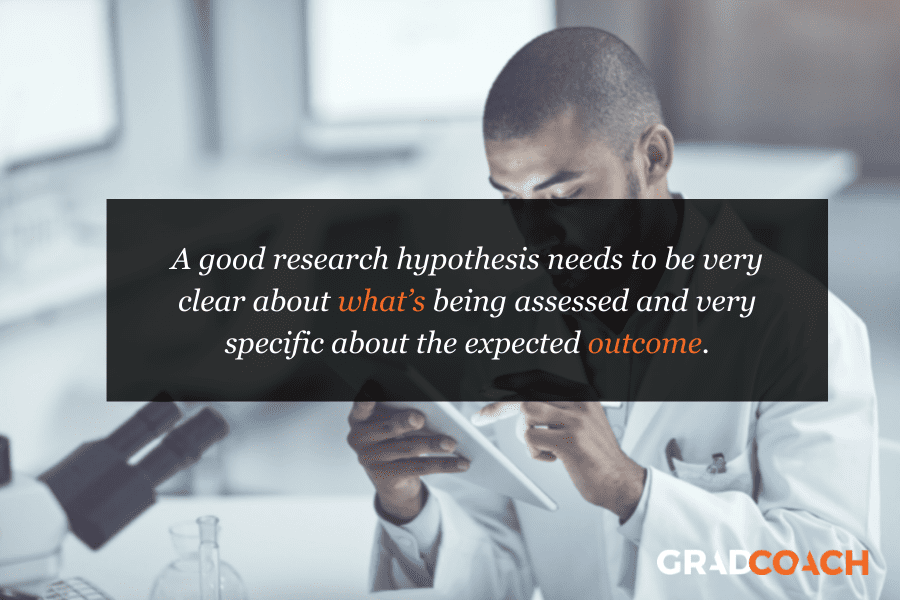
Hypothesis Essential #2: Testability (Provability)
A statement must be testable to qualify as a research hypothesis. In other words, there needs to be a way to prove (or disprove) the statement. If it’s not testable, it’s not a hypothesis – simple as that.
For example, consider the hypothesis we mentioned earlier:
Hypothesis: Students who sleep at least 8 hours per night will, on average, achieve higher grades in standardised tests than students who sleep less than 8 hours a night.
We could test this statement by undertaking a quantitative study involving two groups of students, one that gets 8 or more hours of sleep per night for a fixed period, and one that gets less. We could then compare the standardised test results for both groups to see if there’s a statistically significant difference.
Again, if you compare this to the original hypothesis we looked at – “Sleep impacts academic performance” – you can see that it would be quite difficult to test that statement, primarily because it isn’t specific enough. How much sleep? By who? What type of academic performance?
So, remember the mantra – if you can’t test it, it’s not a hypothesis 🙂
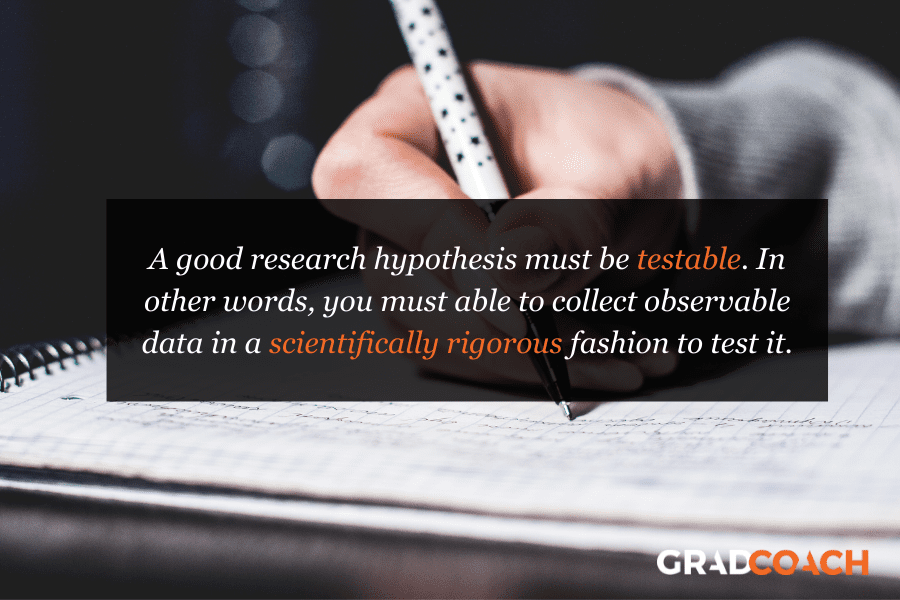
Defining A Research Hypothesis
You’re still with us? Great! Let’s recap and pin down a clear definition of a hypothesis.
A research hypothesis (or scientific hypothesis) is a statement about an expected relationship between variables, or explanation of an occurrence, that is clear, specific and testable.
So, when you write up hypotheses for your dissertation or thesis, make sure that they meet all these criteria. If you do, you’ll not only have rock-solid hypotheses but you’ll also ensure a clear focus for your entire research project.
What about the null hypothesis?
You may have also heard the terms null hypothesis , alternative hypothesis, or H-zero thrown around. At a simple level, the null hypothesis is the counter-proposal to the original hypothesis.
For example, if the hypothesis predicts that there is a relationship between two variables (for example, sleep and academic performance), the null hypothesis would predict that there is no relationship between those variables.
At a more technical level, the null hypothesis proposes that no statistical significance exists in a set of given observations and that any differences are due to chance alone.
And there you have it – hypotheses in a nutshell.
If you have any questions, be sure to leave a comment below and we’ll do our best to help you. If you need hands-on help developing and testing your hypotheses, consider our private coaching service , where we hold your hand through the research journey.
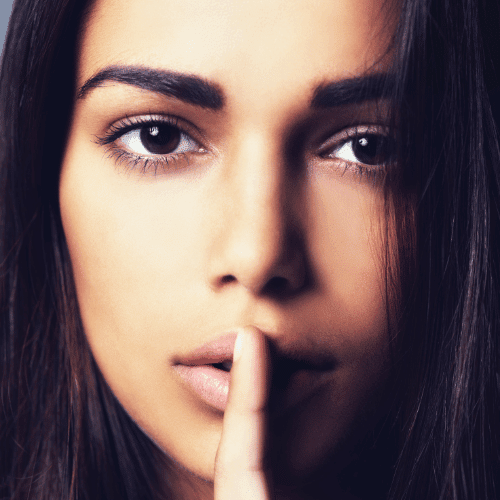
Psst... there’s more!
This post was based on one of our popular Research Bootcamps . If you're working on a research project, you'll definitely want to check this out ...
You Might Also Like:
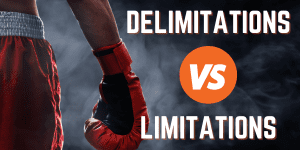
16 Comments
Very useful information. I benefit more from getting more information in this regard.
Very great insight,educative and informative. Please give meet deep critics on many research data of public international Law like human rights, environment, natural resources, law of the sea etc
In a book I read a distinction is made between null, research, and alternative hypothesis. As far as I understand, alternative and research hypotheses are the same. Can you please elaborate? Best Afshin
This is a self explanatory, easy going site. I will recommend this to my friends and colleagues.
Very good definition. How can I cite your definition in my thesis? Thank you. Is nul hypothesis compulsory in a research?
It’s a counter-proposal to be proven as a rejection
Please what is the difference between alternate hypothesis and research hypothesis?
It is a very good explanation. However, it limits hypotheses to statistically tasteable ideas. What about for qualitative researches or other researches that involve quantitative data that don’t need statistical tests?
In qualitative research, one typically uses propositions, not hypotheses.
could you please elaborate it more
I’ve benefited greatly from these notes, thank you.
This is very helpful
well articulated ideas are presented here, thank you for being reliable sources of information
Excellent. Thanks for being clear and sound about the research methodology and hypothesis (quantitative research)
I have only a simple question regarding the null hypothesis. – Is the null hypothesis (Ho) known as the reversible hypothesis of the alternative hypothesis (H1? – How to test it in academic research?
this is very important note help me much more
Trackbacks/Pingbacks
- What Is Research Methodology? Simple Definition (With Examples) - Grad Coach - […] Contrasted to this, a quantitative methodology is typically used when the research aims and objectives are confirmatory in nature. For example,…
Submit a Comment Cancel reply
Your email address will not be published. Required fields are marked *
Save my name, email, and website in this browser for the next time I comment.
- Print Friendly
- Privacy Policy

Home » What is a Hypothesis – Types, Examples and Writing Guide
What is a Hypothesis – Types, Examples and Writing Guide
Table of Contents

Definition:
Hypothesis is an educated guess or proposed explanation for a phenomenon, based on some initial observations or data. It is a tentative statement that can be tested and potentially proven or disproven through further investigation and experimentation.
Hypothesis is often used in scientific research to guide the design of experiments and the collection and analysis of data. It is an essential element of the scientific method, as it allows researchers to make predictions about the outcome of their experiments and to test those predictions to determine their accuracy.
Types of Hypothesis
Types of Hypothesis are as follows:
Research Hypothesis
A research hypothesis is a statement that predicts a relationship between variables. It is usually formulated as a specific statement that can be tested through research, and it is often used in scientific research to guide the design of experiments.
Null Hypothesis
The null hypothesis is a statement that assumes there is no significant difference or relationship between variables. It is often used as a starting point for testing the research hypothesis, and if the results of the study reject the null hypothesis, it suggests that there is a significant difference or relationship between variables.
Alternative Hypothesis
An alternative hypothesis is a statement that assumes there is a significant difference or relationship between variables. It is often used as an alternative to the null hypothesis and is tested against the null hypothesis to determine which statement is more accurate.
Directional Hypothesis
A directional hypothesis is a statement that predicts the direction of the relationship between variables. For example, a researcher might predict that increasing the amount of exercise will result in a decrease in body weight.
Non-directional Hypothesis
A non-directional hypothesis is a statement that predicts the relationship between variables but does not specify the direction. For example, a researcher might predict that there is a relationship between the amount of exercise and body weight, but they do not specify whether increasing or decreasing exercise will affect body weight.
Statistical Hypothesis
A statistical hypothesis is a statement that assumes a particular statistical model or distribution for the data. It is often used in statistical analysis to test the significance of a particular result.
Composite Hypothesis
A composite hypothesis is a statement that assumes more than one condition or outcome. It can be divided into several sub-hypotheses, each of which represents a different possible outcome.
Empirical Hypothesis
An empirical hypothesis is a statement that is based on observed phenomena or data. It is often used in scientific research to develop theories or models that explain the observed phenomena.
Simple Hypothesis
A simple hypothesis is a statement that assumes only one outcome or condition. It is often used in scientific research to test a single variable or factor.
Complex Hypothesis
A complex hypothesis is a statement that assumes multiple outcomes or conditions. It is often used in scientific research to test the effects of multiple variables or factors on a particular outcome.
Applications of Hypothesis
Hypotheses are used in various fields to guide research and make predictions about the outcomes of experiments or observations. Here are some examples of how hypotheses are applied in different fields:
- Science : In scientific research, hypotheses are used to test the validity of theories and models that explain natural phenomena. For example, a hypothesis might be formulated to test the effects of a particular variable on a natural system, such as the effects of climate change on an ecosystem.
- Medicine : In medical research, hypotheses are used to test the effectiveness of treatments and therapies for specific conditions. For example, a hypothesis might be formulated to test the effects of a new drug on a particular disease.
- Psychology : In psychology, hypotheses are used to test theories and models of human behavior and cognition. For example, a hypothesis might be formulated to test the effects of a particular stimulus on the brain or behavior.
- Sociology : In sociology, hypotheses are used to test theories and models of social phenomena, such as the effects of social structures or institutions on human behavior. For example, a hypothesis might be formulated to test the effects of income inequality on crime rates.
- Business : In business research, hypotheses are used to test the validity of theories and models that explain business phenomena, such as consumer behavior or market trends. For example, a hypothesis might be formulated to test the effects of a new marketing campaign on consumer buying behavior.
- Engineering : In engineering, hypotheses are used to test the effectiveness of new technologies or designs. For example, a hypothesis might be formulated to test the efficiency of a new solar panel design.
How to write a Hypothesis
Here are the steps to follow when writing a hypothesis:
Identify the Research Question
The first step is to identify the research question that you want to answer through your study. This question should be clear, specific, and focused. It should be something that can be investigated empirically and that has some relevance or significance in the field.
Conduct a Literature Review
Before writing your hypothesis, it’s essential to conduct a thorough literature review to understand what is already known about the topic. This will help you to identify the research gap and formulate a hypothesis that builds on existing knowledge.
Determine the Variables
The next step is to identify the variables involved in the research question. A variable is any characteristic or factor that can vary or change. There are two types of variables: independent and dependent. The independent variable is the one that is manipulated or changed by the researcher, while the dependent variable is the one that is measured or observed as a result of the independent variable.
Formulate the Hypothesis
Based on the research question and the variables involved, you can now formulate your hypothesis. A hypothesis should be a clear and concise statement that predicts the relationship between the variables. It should be testable through empirical research and based on existing theory or evidence.
Write the Null Hypothesis
The null hypothesis is the opposite of the alternative hypothesis, which is the hypothesis that you are testing. The null hypothesis states that there is no significant difference or relationship between the variables. It is important to write the null hypothesis because it allows you to compare your results with what would be expected by chance.
Refine the Hypothesis
After formulating the hypothesis, it’s important to refine it and make it more precise. This may involve clarifying the variables, specifying the direction of the relationship, or making the hypothesis more testable.
Examples of Hypothesis
Here are a few examples of hypotheses in different fields:
- Psychology : “Increased exposure to violent video games leads to increased aggressive behavior in adolescents.”
- Biology : “Higher levels of carbon dioxide in the atmosphere will lead to increased plant growth.”
- Sociology : “Individuals who grow up in households with higher socioeconomic status will have higher levels of education and income as adults.”
- Education : “Implementing a new teaching method will result in higher student achievement scores.”
- Marketing : “Customers who receive a personalized email will be more likely to make a purchase than those who receive a generic email.”
- Physics : “An increase in temperature will cause an increase in the volume of a gas, assuming all other variables remain constant.”
- Medicine : “Consuming a diet high in saturated fats will increase the risk of developing heart disease.”
Purpose of Hypothesis
The purpose of a hypothesis is to provide a testable explanation for an observed phenomenon or a prediction of a future outcome based on existing knowledge or theories. A hypothesis is an essential part of the scientific method and helps to guide the research process by providing a clear focus for investigation. It enables scientists to design experiments or studies to gather evidence and data that can support or refute the proposed explanation or prediction.
The formulation of a hypothesis is based on existing knowledge, observations, and theories, and it should be specific, testable, and falsifiable. A specific hypothesis helps to define the research question, which is important in the research process as it guides the selection of an appropriate research design and methodology. Testability of the hypothesis means that it can be proven or disproven through empirical data collection and analysis. Falsifiability means that the hypothesis should be formulated in such a way that it can be proven wrong if it is incorrect.
In addition to guiding the research process, the testing of hypotheses can lead to new discoveries and advancements in scientific knowledge. When a hypothesis is supported by the data, it can be used to develop new theories or models to explain the observed phenomenon. When a hypothesis is not supported by the data, it can help to refine existing theories or prompt the development of new hypotheses to explain the phenomenon.
When to use Hypothesis
Here are some common situations in which hypotheses are used:
- In scientific research , hypotheses are used to guide the design of experiments and to help researchers make predictions about the outcomes of those experiments.
- In social science research , hypotheses are used to test theories about human behavior, social relationships, and other phenomena.
- I n business , hypotheses can be used to guide decisions about marketing, product development, and other areas. For example, a hypothesis might be that a new product will sell well in a particular market, and this hypothesis can be tested through market research.
Characteristics of Hypothesis
Here are some common characteristics of a hypothesis:
- Testable : A hypothesis must be able to be tested through observation or experimentation. This means that it must be possible to collect data that will either support or refute the hypothesis.
- Falsifiable : A hypothesis must be able to be proven false if it is not supported by the data. If a hypothesis cannot be falsified, then it is not a scientific hypothesis.
- Clear and concise : A hypothesis should be stated in a clear and concise manner so that it can be easily understood and tested.
- Based on existing knowledge : A hypothesis should be based on existing knowledge and research in the field. It should not be based on personal beliefs or opinions.
- Specific : A hypothesis should be specific in terms of the variables being tested and the predicted outcome. This will help to ensure that the research is focused and well-designed.
- Tentative: A hypothesis is a tentative statement or assumption that requires further testing and evidence to be confirmed or refuted. It is not a final conclusion or assertion.
- Relevant : A hypothesis should be relevant to the research question or problem being studied. It should address a gap in knowledge or provide a new perspective on the issue.
Advantages of Hypothesis
Hypotheses have several advantages in scientific research and experimentation:
- Guides research: A hypothesis provides a clear and specific direction for research. It helps to focus the research question, select appropriate methods and variables, and interpret the results.
- Predictive powe r: A hypothesis makes predictions about the outcome of research, which can be tested through experimentation. This allows researchers to evaluate the validity of the hypothesis and make new discoveries.
- Facilitates communication: A hypothesis provides a common language and framework for scientists to communicate with one another about their research. This helps to facilitate the exchange of ideas and promotes collaboration.
- Efficient use of resources: A hypothesis helps researchers to use their time, resources, and funding efficiently by directing them towards specific research questions and methods that are most likely to yield results.
- Provides a basis for further research: A hypothesis that is supported by data provides a basis for further research and exploration. It can lead to new hypotheses, theories, and discoveries.
- Increases objectivity: A hypothesis can help to increase objectivity in research by providing a clear and specific framework for testing and interpreting results. This can reduce bias and increase the reliability of research findings.
Limitations of Hypothesis
Some Limitations of the Hypothesis are as follows:
- Limited to observable phenomena: Hypotheses are limited to observable phenomena and cannot account for unobservable or intangible factors. This means that some research questions may not be amenable to hypothesis testing.
- May be inaccurate or incomplete: Hypotheses are based on existing knowledge and research, which may be incomplete or inaccurate. This can lead to flawed hypotheses and erroneous conclusions.
- May be biased: Hypotheses may be biased by the researcher’s own beliefs, values, or assumptions. This can lead to selective interpretation of data and a lack of objectivity in research.
- Cannot prove causation: A hypothesis can only show a correlation between variables, but it cannot prove causation. This requires further experimentation and analysis.
- Limited to specific contexts: Hypotheses are limited to specific contexts and may not be generalizable to other situations or populations. This means that results may not be applicable in other contexts or may require further testing.
- May be affected by chance : Hypotheses may be affected by chance or random variation, which can obscure or distort the true relationship between variables.
About the author
Muhammad Hassan
Researcher, Academic Writer, Web developer
You may also like

Data Collection – Methods Types and Examples

Delimitations in Research – Types, Examples and...

Research Process – Steps, Examples and Tips

Research Design – Types, Methods and Examples

Institutional Review Board – Application Sample...

Evaluating Research – Process, Examples and...
- Resources Home 🏠
- Try SciSpace Copilot
- Search research papers
- Add Copilot Extension
- Try AI Detector
- Try Paraphraser
- Try Citation Generator
- April Papers
- June Papers
- July Papers

The Craft of Writing a Strong Hypothesis

Table of Contents
Writing a hypothesis is one of the essential elements of a scientific research paper. It needs to be to the point, clearly communicating what your research is trying to accomplish. A blurry, drawn-out, or complexly-structured hypothesis can confuse your readers. Or worse, the editor and peer reviewers.
A captivating hypothesis is not too intricate. This blog will take you through the process so that, by the end of it, you have a better idea of how to convey your research paper's intent in just one sentence.
What is a Hypothesis?
The first step in your scientific endeavor, a hypothesis, is a strong, concise statement that forms the basis of your research. It is not the same as a thesis statement , which is a brief summary of your research paper .
The sole purpose of a hypothesis is to predict your paper's findings, data, and conclusion. It comes from a place of curiosity and intuition . When you write a hypothesis, you're essentially making an educated guess based on scientific prejudices and evidence, which is further proven or disproven through the scientific method.
The reason for undertaking research is to observe a specific phenomenon. A hypothesis, therefore, lays out what the said phenomenon is. And it does so through two variables, an independent and dependent variable.
The independent variable is the cause behind the observation, while the dependent variable is the effect of the cause. A good example of this is “mixing red and blue forms purple.” In this hypothesis, mixing red and blue is the independent variable as you're combining the two colors at your own will. The formation of purple is the dependent variable as, in this case, it is conditional to the independent variable.
Different Types of Hypotheses

Types of hypotheses
Some would stand by the notion that there are only two types of hypotheses: a Null hypothesis and an Alternative hypothesis. While that may have some truth to it, it would be better to fully distinguish the most common forms as these terms come up so often, which might leave you out of context.
Apart from Null and Alternative, there are Complex, Simple, Directional, Non-Directional, Statistical, and Associative and casual hypotheses. They don't necessarily have to be exclusive, as one hypothesis can tick many boxes, but knowing the distinctions between them will make it easier for you to construct your own.
1. Null hypothesis
A null hypothesis proposes no relationship between two variables. Denoted by H 0 , it is a negative statement like “Attending physiotherapy sessions does not affect athletes' on-field performance.” Here, the author claims physiotherapy sessions have no effect on on-field performances. Even if there is, it's only a coincidence.
2. Alternative hypothesis
Considered to be the opposite of a null hypothesis, an alternative hypothesis is donated as H1 or Ha. It explicitly states that the dependent variable affects the independent variable. A good alternative hypothesis example is “Attending physiotherapy sessions improves athletes' on-field performance.” or “Water evaporates at 100 °C. ” The alternative hypothesis further branches into directional and non-directional.
- Directional hypothesis: A hypothesis that states the result would be either positive or negative is called directional hypothesis. It accompanies H1 with either the ‘<' or ‘>' sign.
- Non-directional hypothesis: A non-directional hypothesis only claims an effect on the dependent variable. It does not clarify whether the result would be positive or negative. The sign for a non-directional hypothesis is ‘≠.'
3. Simple hypothesis
A simple hypothesis is a statement made to reflect the relation between exactly two variables. One independent and one dependent. Consider the example, “Smoking is a prominent cause of lung cancer." The dependent variable, lung cancer, is dependent on the independent variable, smoking.
4. Complex hypothesis
In contrast to a simple hypothesis, a complex hypothesis implies the relationship between multiple independent and dependent variables. For instance, “Individuals who eat more fruits tend to have higher immunity, lesser cholesterol, and high metabolism.” The independent variable is eating more fruits, while the dependent variables are higher immunity, lesser cholesterol, and high metabolism.
5. Associative and casual hypothesis
Associative and casual hypotheses don't exhibit how many variables there will be. They define the relationship between the variables. In an associative hypothesis, changing any one variable, dependent or independent, affects others. In a casual hypothesis, the independent variable directly affects the dependent.
6. Empirical hypothesis
Also referred to as the working hypothesis, an empirical hypothesis claims a theory's validation via experiments and observation. This way, the statement appears justifiable and different from a wild guess.
Say, the hypothesis is “Women who take iron tablets face a lesser risk of anemia than those who take vitamin B12.” This is an example of an empirical hypothesis where the researcher the statement after assessing a group of women who take iron tablets and charting the findings.
7. Statistical hypothesis
The point of a statistical hypothesis is to test an already existing hypothesis by studying a population sample. Hypothesis like “44% of the Indian population belong in the age group of 22-27.” leverage evidence to prove or disprove a particular statement.
Characteristics of a Good Hypothesis
Writing a hypothesis is essential as it can make or break your research for you. That includes your chances of getting published in a journal. So when you're designing one, keep an eye out for these pointers:
- A research hypothesis has to be simple yet clear to look justifiable enough.
- It has to be testable — your research would be rendered pointless if too far-fetched into reality or limited by technology.
- It has to be precise about the results —what you are trying to do and achieve through it should come out in your hypothesis.
- A research hypothesis should be self-explanatory, leaving no doubt in the reader's mind.
- If you are developing a relational hypothesis, you need to include the variables and establish an appropriate relationship among them.
- A hypothesis must keep and reflect the scope for further investigations and experiments.
Separating a Hypothesis from a Prediction
Outside of academia, hypothesis and prediction are often used interchangeably. In research writing, this is not only confusing but also incorrect. And although a hypothesis and prediction are guesses at their core, there are many differences between them.
A hypothesis is an educated guess or even a testable prediction validated through research. It aims to analyze the gathered evidence and facts to define a relationship between variables and put forth a logical explanation behind the nature of events.
Predictions are assumptions or expected outcomes made without any backing evidence. They are more fictionally inclined regardless of where they originate from.
For this reason, a hypothesis holds much more weight than a prediction. It sticks to the scientific method rather than pure guesswork. "Planets revolve around the Sun." is an example of a hypothesis as it is previous knowledge and observed trends. Additionally, we can test it through the scientific method.
Whereas "COVID-19 will be eradicated by 2030." is a prediction. Even though it results from past trends, we can't prove or disprove it. So, the only way this gets validated is to wait and watch if COVID-19 cases end by 2030.
Finally, How to Write a Hypothesis
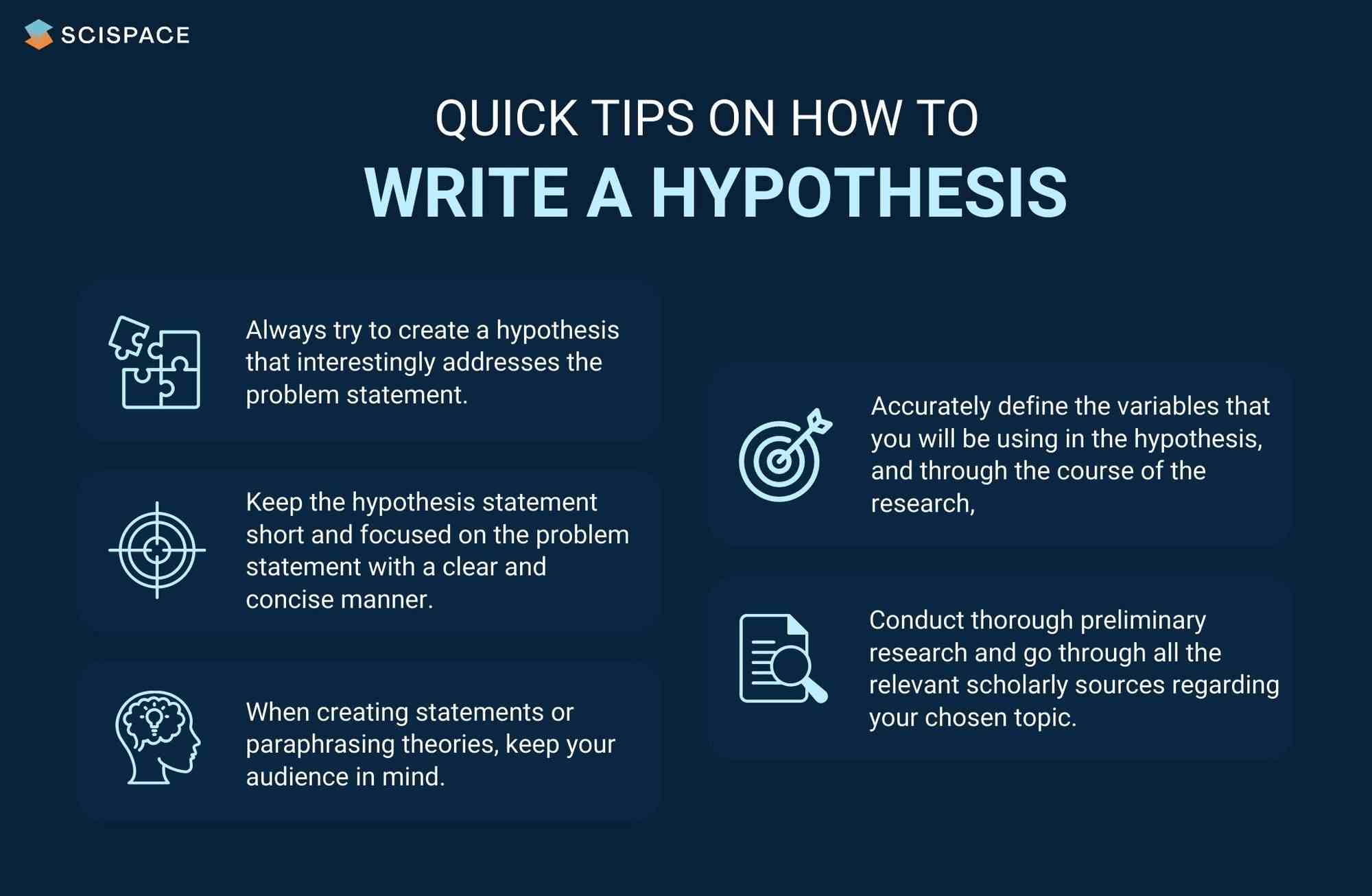
Quick tips on writing a hypothesis
1. Be clear about your research question
A hypothesis should instantly address the research question or the problem statement. To do so, you need to ask a question. Understand the constraints of your undertaken research topic and then formulate a simple and topic-centric problem. Only after that can you develop a hypothesis and further test for evidence.
2. Carry out a recce
Once you have your research's foundation laid out, it would be best to conduct preliminary research. Go through previous theories, academic papers, data, and experiments before you start curating your research hypothesis. It will give you an idea of your hypothesis's viability or originality.
Making use of references from relevant research papers helps draft a good research hypothesis. SciSpace Discover offers a repository of over 270 million research papers to browse through and gain a deeper understanding of related studies on a particular topic. Additionally, you can use SciSpace Copilot , your AI research assistant, for reading any lengthy research paper and getting a more summarized context of it. A hypothesis can be formed after evaluating many such summarized research papers. Copilot also offers explanations for theories and equations, explains paper in simplified version, allows you to highlight any text in the paper or clip math equations and tables and provides a deeper, clear understanding of what is being said. This can improve the hypothesis by helping you identify potential research gaps.
3. Create a 3-dimensional hypothesis
Variables are an essential part of any reasonable hypothesis. So, identify your independent and dependent variable(s) and form a correlation between them. The ideal way to do this is to write the hypothetical assumption in the ‘if-then' form. If you use this form, make sure that you state the predefined relationship between the variables.
In another way, you can choose to present your hypothesis as a comparison between two variables. Here, you must specify the difference you expect to observe in the results.
4. Write the first draft
Now that everything is in place, it's time to write your hypothesis. For starters, create the first draft. In this version, write what you expect to find from your research.
Clearly separate your independent and dependent variables and the link between them. Don't fixate on syntax at this stage. The goal is to ensure your hypothesis addresses the issue.
5. Proof your hypothesis
After preparing the first draft of your hypothesis, you need to inspect it thoroughly. It should tick all the boxes, like being concise, straightforward, relevant, and accurate. Your final hypothesis has to be well-structured as well.
Research projects are an exciting and crucial part of being a scholar. And once you have your research question, you need a great hypothesis to begin conducting research. Thus, knowing how to write a hypothesis is very important.
Now that you have a firmer grasp on what a good hypothesis constitutes, the different kinds there are, and what process to follow, you will find it much easier to write your hypothesis, which ultimately helps your research.
Now it's easier than ever to streamline your research workflow with SciSpace Discover . Its integrated, comprehensive end-to-end platform for research allows scholars to easily discover, write and publish their research and fosters collaboration.
It includes everything you need, including a repository of over 270 million research papers across disciplines, SEO-optimized summaries and public profiles to show your expertise and experience.
If you found these tips on writing a research hypothesis useful, head over to our blog on Statistical Hypothesis Testing to learn about the top researchers, papers, and institutions in this domain.
Frequently Asked Questions (FAQs)
1. what is the definition of hypothesis.
According to the Oxford dictionary, a hypothesis is defined as “An idea or explanation of something that is based on a few known facts, but that has not yet been proved to be true or correct”.
2. What is an example of hypothesis?
The hypothesis is a statement that proposes a relationship between two or more variables. An example: "If we increase the number of new users who join our platform by 25%, then we will see an increase in revenue."
3. What is an example of null hypothesis?
A null hypothesis is a statement that there is no relationship between two variables. The null hypothesis is written as H0. The null hypothesis states that there is no effect. For example, if you're studying whether or not a particular type of exercise increases strength, your null hypothesis will be "there is no difference in strength between people who exercise and people who don't."
4. What are the types of research?
• Fundamental research
• Applied research
• Qualitative research
• Quantitative research
• Mixed research
• Exploratory research
• Longitudinal research
• Cross-sectional research
• Field research
• Laboratory research
• Fixed research
• Flexible research
• Action research
• Policy research
• Classification research
• Comparative research
• Causal research
• Inductive research
• Deductive research
5. How to write a hypothesis?
• Your hypothesis should be able to predict the relationship and outcome.
• Avoid wordiness by keeping it simple and brief.
• Your hypothesis should contain observable and testable outcomes.
• Your hypothesis should be relevant to the research question.
6. What are the 2 types of hypothesis?
• Null hypotheses are used to test the claim that "there is no difference between two groups of data".
• Alternative hypotheses test the claim that "there is a difference between two data groups".
7. Difference between research question and research hypothesis?
A research question is a broad, open-ended question you will try to answer through your research. A hypothesis is a statement based on prior research or theory that you expect to be true due to your study. Example - Research question: What are the factors that influence the adoption of the new technology? Research hypothesis: There is a positive relationship between age, education and income level with the adoption of the new technology.
8. What is plural for hypothesis?
The plural of hypothesis is hypotheses. Here's an example of how it would be used in a statement, "Numerous well-considered hypotheses are presented in this part, and they are supported by tables and figures that are well-illustrated."
9. What is the red queen hypothesis?
The red queen hypothesis in evolutionary biology states that species must constantly evolve to avoid extinction because if they don't, they will be outcompeted by other species that are evolving. Leigh Van Valen first proposed it in 1973; since then, it has been tested and substantiated many times.
10. Who is known as the father of null hypothesis?
The father of the null hypothesis is Sir Ronald Fisher. He published a paper in 1925 that introduced the concept of null hypothesis testing, and he was also the first to use the term itself.
11. When to reject null hypothesis?
You need to find a significant difference between your two populations to reject the null hypothesis. You can determine that by running statistical tests such as an independent sample t-test or a dependent sample t-test. You should reject the null hypothesis if the p-value is less than 0.05.

You might also like

Consensus GPT vs. SciSpace GPT: Choose the Best GPT for Research
Literature Review and Theoretical Framework: Understanding the Differences

Types of Essays in Academic Writing - Quick Guide (2024)
- Bipolar Disorder
- Therapy Center
- When To See a Therapist
- Types of Therapy
- Best Online Therapy
- Best Couples Therapy
- Best Family Therapy
- Managing Stress
- Sleep and Dreaming
- Understanding Emotions
- Self-Improvement
- Healthy Relationships
- Student Resources
- Personality Types
- Guided Meditations
- Verywell Mind Insights
- 2024 Verywell Mind 25
- Mental Health in the Classroom
- Editorial Process
- Meet Our Review Board
- Crisis Support
How to Write a Great Hypothesis
Hypothesis Definition, Format, Examples, and Tips
Kendra Cherry, MS, is a psychosocial rehabilitation specialist, psychology educator, and author of the "Everything Psychology Book."
:max_bytes(150000):strip_icc():format(webp)/IMG_9791-89504ab694d54b66bbd72cb84ffb860e.jpg)
Amy Morin, LCSW, is a psychotherapist and international bestselling author. Her books, including "13 Things Mentally Strong People Don't Do," have been translated into more than 40 languages. Her TEDx talk, "The Secret of Becoming Mentally Strong," is one of the most viewed talks of all time.
:max_bytes(150000):strip_icc():format(webp)/VW-MIND-Amy-2b338105f1ee493f94d7e333e410fa76.jpg)
Verywell / Alex Dos Diaz
- The Scientific Method
Hypothesis Format
Falsifiability of a hypothesis.
- Operationalization
Hypothesis Types
Hypotheses examples.
- Collecting Data
A hypothesis is a tentative statement about the relationship between two or more variables. It is a specific, testable prediction about what you expect to happen in a study. It is a preliminary answer to your question that helps guide the research process.
Consider a study designed to examine the relationship between sleep deprivation and test performance. The hypothesis might be: "This study is designed to assess the hypothesis that sleep-deprived people will perform worse on a test than individuals who are not sleep-deprived."
At a Glance
A hypothesis is crucial to scientific research because it offers a clear direction for what the researchers are looking to find. This allows them to design experiments to test their predictions and add to our scientific knowledge about the world. This article explores how a hypothesis is used in psychology research, how to write a good hypothesis, and the different types of hypotheses you might use.
The Hypothesis in the Scientific Method
In the scientific method , whether it involves research in psychology, biology, or some other area, a hypothesis represents what the researchers think will happen in an experiment. The scientific method involves the following steps:
- Forming a question
- Performing background research
- Creating a hypothesis
- Designing an experiment
- Collecting data
- Analyzing the results
- Drawing conclusions
- Communicating the results
The hypothesis is a prediction, but it involves more than a guess. Most of the time, the hypothesis begins with a question which is then explored through background research. At this point, researchers then begin to develop a testable hypothesis.
Unless you are creating an exploratory study, your hypothesis should always explain what you expect to happen.
In a study exploring the effects of a particular drug, the hypothesis might be that researchers expect the drug to have some type of effect on the symptoms of a specific illness. In psychology, the hypothesis might focus on how a certain aspect of the environment might influence a particular behavior.
Remember, a hypothesis does not have to be correct. While the hypothesis predicts what the researchers expect to see, the goal of the research is to determine whether this guess is right or wrong. When conducting an experiment, researchers might explore numerous factors to determine which ones might contribute to the ultimate outcome.
In many cases, researchers may find that the results of an experiment do not support the original hypothesis. When writing up these results, the researchers might suggest other options that should be explored in future studies.
In many cases, researchers might draw a hypothesis from a specific theory or build on previous research. For example, prior research has shown that stress can impact the immune system. So a researcher might hypothesize: "People with high-stress levels will be more likely to contract a common cold after being exposed to the virus than people who have low-stress levels."
In other instances, researchers might look at commonly held beliefs or folk wisdom. "Birds of a feather flock together" is one example of folk adage that a psychologist might try to investigate. The researcher might pose a specific hypothesis that "People tend to select romantic partners who are similar to them in interests and educational level."
Elements of a Good Hypothesis
So how do you write a good hypothesis? When trying to come up with a hypothesis for your research or experiments, ask yourself the following questions:
- Is your hypothesis based on your research on a topic?
- Can your hypothesis be tested?
- Does your hypothesis include independent and dependent variables?
Before you come up with a specific hypothesis, spend some time doing background research. Once you have completed a literature review, start thinking about potential questions you still have. Pay attention to the discussion section in the journal articles you read . Many authors will suggest questions that still need to be explored.
How to Formulate a Good Hypothesis
To form a hypothesis, you should take these steps:
- Collect as many observations about a topic or problem as you can.
- Evaluate these observations and look for possible causes of the problem.
- Create a list of possible explanations that you might want to explore.
- After you have developed some possible hypotheses, think of ways that you could confirm or disprove each hypothesis through experimentation. This is known as falsifiability.
In the scientific method , falsifiability is an important part of any valid hypothesis. In order to test a claim scientifically, it must be possible that the claim could be proven false.
Students sometimes confuse the idea of falsifiability with the idea that it means that something is false, which is not the case. What falsifiability means is that if something was false, then it is possible to demonstrate that it is false.
One of the hallmarks of pseudoscience is that it makes claims that cannot be refuted or proven false.
The Importance of Operational Definitions
A variable is a factor or element that can be changed and manipulated in ways that are observable and measurable. However, the researcher must also define how the variable will be manipulated and measured in the study.
Operational definitions are specific definitions for all relevant factors in a study. This process helps make vague or ambiguous concepts detailed and measurable.
For example, a researcher might operationally define the variable " test anxiety " as the results of a self-report measure of anxiety experienced during an exam. A "study habits" variable might be defined by the amount of studying that actually occurs as measured by time.
These precise descriptions are important because many things can be measured in various ways. Clearly defining these variables and how they are measured helps ensure that other researchers can replicate your results.
Replicability
One of the basic principles of any type of scientific research is that the results must be replicable.
Replication means repeating an experiment in the same way to produce the same results. By clearly detailing the specifics of how the variables were measured and manipulated, other researchers can better understand the results and repeat the study if needed.
Some variables are more difficult than others to define. For example, how would you operationally define a variable such as aggression ? For obvious ethical reasons, researchers cannot create a situation in which a person behaves aggressively toward others.
To measure this variable, the researcher must devise a measurement that assesses aggressive behavior without harming others. The researcher might utilize a simulated task to measure aggressiveness in this situation.
Hypothesis Checklist
- Does your hypothesis focus on something that you can actually test?
- Does your hypothesis include both an independent and dependent variable?
- Can you manipulate the variables?
- Can your hypothesis be tested without violating ethical standards?
The hypothesis you use will depend on what you are investigating and hoping to find. Some of the main types of hypotheses that you might use include:
- Simple hypothesis : This type of hypothesis suggests there is a relationship between one independent variable and one dependent variable.
- Complex hypothesis : This type suggests a relationship between three or more variables, such as two independent and dependent variables.
- Null hypothesis : This hypothesis suggests no relationship exists between two or more variables.
- Alternative hypothesis : This hypothesis states the opposite of the null hypothesis.
- Statistical hypothesis : This hypothesis uses statistical analysis to evaluate a representative population sample and then generalizes the findings to the larger group.
- Logical hypothesis : This hypothesis assumes a relationship between variables without collecting data or evidence.
A hypothesis often follows a basic format of "If {this happens} then {this will happen}." One way to structure your hypothesis is to describe what will happen to the dependent variable if you change the independent variable .
The basic format might be: "If {these changes are made to a certain independent variable}, then we will observe {a change in a specific dependent variable}."
A few examples of simple hypotheses:
- "Students who eat breakfast will perform better on a math exam than students who do not eat breakfast."
- "Students who experience test anxiety before an English exam will get lower scores than students who do not experience test anxiety."
- "Motorists who talk on the phone while driving will be more likely to make errors on a driving course than those who do not talk on the phone."
- "Children who receive a new reading intervention will have higher reading scores than students who do not receive the intervention."
Examples of a complex hypothesis include:
- "People with high-sugar diets and sedentary activity levels are more likely to develop depression."
- "Younger people who are regularly exposed to green, outdoor areas have better subjective well-being than older adults who have limited exposure to green spaces."
Examples of a null hypothesis include:
- "There is no difference in anxiety levels between people who take St. John's wort supplements and those who do not."
- "There is no difference in scores on a memory recall task between children and adults."
- "There is no difference in aggression levels between children who play first-person shooter games and those who do not."
Examples of an alternative hypothesis:
- "People who take St. John's wort supplements will have less anxiety than those who do not."
- "Adults will perform better on a memory task than children."
- "Children who play first-person shooter games will show higher levels of aggression than children who do not."
Collecting Data on Your Hypothesis
Once a researcher has formed a testable hypothesis, the next step is to select a research design and start collecting data. The research method depends largely on exactly what they are studying. There are two basic types of research methods: descriptive research and experimental research.
Descriptive Research Methods
Descriptive research such as case studies , naturalistic observations , and surveys are often used when conducting an experiment is difficult or impossible. These methods are best used to describe different aspects of a behavior or psychological phenomenon.
Once a researcher has collected data using descriptive methods, a correlational study can examine how the variables are related. This research method might be used to investigate a hypothesis that is difficult to test experimentally.
Experimental Research Methods
Experimental methods are used to demonstrate causal relationships between variables. In an experiment, the researcher systematically manipulates a variable of interest (known as the independent variable) and measures the effect on another variable (known as the dependent variable).
Unlike correlational studies, which can only be used to determine if there is a relationship between two variables, experimental methods can be used to determine the actual nature of the relationship—whether changes in one variable actually cause another to change.
The hypothesis is a critical part of any scientific exploration. It represents what researchers expect to find in a study or experiment. In situations where the hypothesis is unsupported by the research, the research still has value. Such research helps us better understand how different aspects of the natural world relate to one another. It also helps us develop new hypotheses that can then be tested in the future.
Thompson WH, Skau S. On the scope of scientific hypotheses . R Soc Open Sci . 2023;10(8):230607. doi:10.1098/rsos.230607
Taran S, Adhikari NKJ, Fan E. Falsifiability in medicine: what clinicians can learn from Karl Popper [published correction appears in Intensive Care Med. 2021 Jun 17;:]. Intensive Care Med . 2021;47(9):1054-1056. doi:10.1007/s00134-021-06432-z
Eyler AA. Research Methods for Public Health . 1st ed. Springer Publishing Company; 2020. doi:10.1891/9780826182067.0004
Nosek BA, Errington TM. What is replication ? PLoS Biol . 2020;18(3):e3000691. doi:10.1371/journal.pbio.3000691
Aggarwal R, Ranganathan P. Study designs: Part 2 - Descriptive studies . Perspect Clin Res . 2019;10(1):34-36. doi:10.4103/picr.PICR_154_18
Nevid J. Psychology: Concepts and Applications. Wadworth, 2013.
By Kendra Cherry, MSEd Kendra Cherry, MS, is a psychosocial rehabilitation specialist, psychology educator, and author of the "Everything Psychology Book."

Research Hypothesis In Psychology: Types, & Examples
Saul Mcleod, PhD
Editor-in-Chief for Simply Psychology
BSc (Hons) Psychology, MRes, PhD, University of Manchester
Saul Mcleod, PhD., is a qualified psychology teacher with over 18 years of experience in further and higher education. He has been published in peer-reviewed journals, including the Journal of Clinical Psychology.
Learn about our Editorial Process
Olivia Guy-Evans, MSc
Associate Editor for Simply Psychology
BSc (Hons) Psychology, MSc Psychology of Education
Olivia Guy-Evans is a writer and associate editor for Simply Psychology. She has previously worked in healthcare and educational sectors.
On This Page:
A research hypothesis, in its plural form “hypotheses,” is a specific, testable prediction about the anticipated results of a study, established at its outset. It is a key component of the scientific method .
Hypotheses connect theory to data and guide the research process towards expanding scientific understanding
Some key points about hypotheses:
- A hypothesis expresses an expected pattern or relationship. It connects the variables under investigation.
- It is stated in clear, precise terms before any data collection or analysis occurs. This makes the hypothesis testable.
- A hypothesis must be falsifiable. It should be possible, even if unlikely in practice, to collect data that disconfirms rather than supports the hypothesis.
- Hypotheses guide research. Scientists design studies to explicitly evaluate hypotheses about how nature works.
- For a hypothesis to be valid, it must be testable against empirical evidence. The evidence can then confirm or disprove the testable predictions.
- Hypotheses are informed by background knowledge and observation, but go beyond what is already known to propose an explanation of how or why something occurs.
Predictions typically arise from a thorough knowledge of the research literature, curiosity about real-world problems or implications, and integrating this to advance theory. They build on existing literature while providing new insight.
Types of Research Hypotheses
Alternative hypothesis.
The research hypothesis is often called the alternative or experimental hypothesis in experimental research.
It typically suggests a potential relationship between two key variables: the independent variable, which the researcher manipulates, and the dependent variable, which is measured based on those changes.
The alternative hypothesis states a relationship exists between the two variables being studied (one variable affects the other).
A hypothesis is a testable statement or prediction about the relationship between two or more variables. It is a key component of the scientific method. Some key points about hypotheses:
- Important hypotheses lead to predictions that can be tested empirically. The evidence can then confirm or disprove the testable predictions.
In summary, a hypothesis is a precise, testable statement of what researchers expect to happen in a study and why. Hypotheses connect theory to data and guide the research process towards expanding scientific understanding.
An experimental hypothesis predicts what change(s) will occur in the dependent variable when the independent variable is manipulated.
It states that the results are not due to chance and are significant in supporting the theory being investigated.
The alternative hypothesis can be directional, indicating a specific direction of the effect, or non-directional, suggesting a difference without specifying its nature. It’s what researchers aim to support or demonstrate through their study.
Null Hypothesis
The null hypothesis states no relationship exists between the two variables being studied (one variable does not affect the other). There will be no changes in the dependent variable due to manipulating the independent variable.
It states results are due to chance and are not significant in supporting the idea being investigated.
The null hypothesis, positing no effect or relationship, is a foundational contrast to the research hypothesis in scientific inquiry. It establishes a baseline for statistical testing, promoting objectivity by initiating research from a neutral stance.
Many statistical methods are tailored to test the null hypothesis, determining the likelihood of observed results if no true effect exists.
This dual-hypothesis approach provides clarity, ensuring that research intentions are explicit, and fosters consistency across scientific studies, enhancing the standardization and interpretability of research outcomes.
Nondirectional Hypothesis
A non-directional hypothesis, also known as a two-tailed hypothesis, predicts that there is a difference or relationship between two variables but does not specify the direction of this relationship.
It merely indicates that a change or effect will occur without predicting which group will have higher or lower values.
For example, “There is a difference in performance between Group A and Group B” is a non-directional hypothesis.
Directional Hypothesis
A directional (one-tailed) hypothesis predicts the nature of the effect of the independent variable on the dependent variable. It predicts in which direction the change will take place. (i.e., greater, smaller, less, more)
It specifies whether one variable is greater, lesser, or different from another, rather than just indicating that there’s a difference without specifying its nature.
For example, “Exercise increases weight loss” is a directional hypothesis.
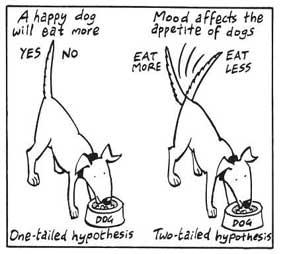
Falsifiability
The Falsification Principle, proposed by Karl Popper , is a way of demarcating science from non-science. It suggests that for a theory or hypothesis to be considered scientific, it must be testable and irrefutable.
Falsifiability emphasizes that scientific claims shouldn’t just be confirmable but should also have the potential to be proven wrong.
It means that there should exist some potential evidence or experiment that could prove the proposition false.
However many confirming instances exist for a theory, it only takes one counter observation to falsify it. For example, the hypothesis that “all swans are white,” can be falsified by observing a black swan.
For Popper, science should attempt to disprove a theory rather than attempt to continually provide evidence to support a research hypothesis.
Can a Hypothesis be Proven?
Hypotheses make probabilistic predictions. They state the expected outcome if a particular relationship exists. However, a study result supporting a hypothesis does not definitively prove it is true.
All studies have limitations. There may be unknown confounding factors or issues that limit the certainty of conclusions. Additional studies may yield different results.
In science, hypotheses can realistically only be supported with some degree of confidence, not proven. The process of science is to incrementally accumulate evidence for and against hypothesized relationships in an ongoing pursuit of better models and explanations that best fit the empirical data. But hypotheses remain open to revision and rejection if that is where the evidence leads.
- Disproving a hypothesis is definitive. Solid disconfirmatory evidence will falsify a hypothesis and require altering or discarding it based on the evidence.
- However, confirming evidence is always open to revision. Other explanations may account for the same results, and additional or contradictory evidence may emerge over time.
We can never 100% prove the alternative hypothesis. Instead, we see if we can disprove, or reject the null hypothesis.
If we reject the null hypothesis, this doesn’t mean that our alternative hypothesis is correct but does support the alternative/experimental hypothesis.
Upon analysis of the results, an alternative hypothesis can be rejected or supported, but it can never be proven to be correct. We must avoid any reference to results proving a theory as this implies 100% certainty, and there is always a chance that evidence may exist which could refute a theory.
How to Write a Hypothesis
- Identify variables . The researcher manipulates the independent variable and the dependent variable is the measured outcome.
- Operationalized the variables being investigated . Operationalization of a hypothesis refers to the process of making the variables physically measurable or testable, e.g. if you are about to study aggression, you might count the number of punches given by participants.
- Decide on a direction for your prediction . If there is evidence in the literature to support a specific effect of the independent variable on the dependent variable, write a directional (one-tailed) hypothesis. If there are limited or ambiguous findings in the literature regarding the effect of the independent variable on the dependent variable, write a non-directional (two-tailed) hypothesis.
- Make it Testable : Ensure your hypothesis can be tested through experimentation or observation. It should be possible to prove it false (principle of falsifiability).
- Clear & concise language . A strong hypothesis is concise (typically one to two sentences long), and formulated using clear and straightforward language, ensuring it’s easily understood and testable.
Consider a hypothesis many teachers might subscribe to: students work better on Monday morning than on Friday afternoon (IV=Day, DV= Standard of work).
Now, if we decide to study this by giving the same group of students a lesson on a Monday morning and a Friday afternoon and then measuring their immediate recall of the material covered in each session, we would end up with the following:
- The alternative hypothesis states that students will recall significantly more information on a Monday morning than on a Friday afternoon.
- The null hypothesis states that there will be no significant difference in the amount recalled on a Monday morning compared to a Friday afternoon. Any difference will be due to chance or confounding factors.
More Examples
- Memory : Participants exposed to classical music during study sessions will recall more items from a list than those who studied in silence.
- Social Psychology : Individuals who frequently engage in social media use will report higher levels of perceived social isolation compared to those who use it infrequently.
- Developmental Psychology : Children who engage in regular imaginative play have better problem-solving skills than those who don’t.
- Clinical Psychology : Cognitive-behavioral therapy will be more effective in reducing symptoms of anxiety over a 6-month period compared to traditional talk therapy.
- Cognitive Psychology : Individuals who multitask between various electronic devices will have shorter attention spans on focused tasks than those who single-task.
- Health Psychology : Patients who practice mindfulness meditation will experience lower levels of chronic pain compared to those who don’t meditate.
- Organizational Psychology : Employees in open-plan offices will report higher levels of stress than those in private offices.
- Behavioral Psychology : Rats rewarded with food after pressing a lever will press it more frequently than rats who receive no reward.

Frequently asked questions
What is the definition of a hypothesis.
A hypothesis states your predictions about what your research will find. It is a tentative answer to your research question that has not yet been tested. For some research projects, you might have to write several hypotheses that address different aspects of your research question.
A hypothesis is not just a guess. It should be based on existing theories and knowledge. It also has to be testable, which means you can support or refute it through scientific research methods (such as experiments, observations, and statistical analysis of data).
Frequently asked questions: Methodology
Quantitative observations involve measuring or counting something and expressing the result in numerical form, while qualitative observations involve describing something in non-numerical terms, such as its appearance, texture, or color.
To make quantitative observations , you need to use instruments that are capable of measuring the quantity you want to observe. For example, you might use a ruler to measure the length of an object or a thermometer to measure its temperature.
Scope of research is determined at the beginning of your research process , prior to the data collection stage. Sometimes called “scope of study,” your scope delineates what will and will not be covered in your project. It helps you focus your work and your time, ensuring that you’ll be able to achieve your goals and outcomes.
Defining a scope can be very useful in any research project, from a research proposal to a thesis or dissertation . A scope is needed for all types of research: quantitative , qualitative , and mixed methods .
To define your scope of research, consider the following:
- Budget constraints or any specifics of grant funding
- Your proposed timeline and duration
- Specifics about your population of study, your proposed sample size , and the research methodology you’ll pursue
- Any inclusion and exclusion criteria
- Any anticipated control , extraneous , or confounding variables that could bias your research if not accounted for properly.
Inclusion and exclusion criteria are predominantly used in non-probability sampling . In purposive sampling and snowball sampling , restrictions apply as to who can be included in the sample .
Inclusion and exclusion criteria are typically presented and discussed in the methodology section of your thesis or dissertation .
The purpose of theory-testing mode is to find evidence in order to disprove, refine, or support a theory. As such, generalisability is not the aim of theory-testing mode.
Due to this, the priority of researchers in theory-testing mode is to eliminate alternative causes for relationships between variables . In other words, they prioritise internal validity over external validity , including ecological validity .
Convergent validity shows how much a measure of one construct aligns with other measures of the same or related constructs .
On the other hand, concurrent validity is about how a measure matches up to some known criterion or gold standard, which can be another measure.
Although both types of validity are established by calculating the association or correlation between a test score and another variable , they represent distinct validation methods.
Validity tells you how accurately a method measures what it was designed to measure. There are 4 main types of validity :
- Construct validity : Does the test measure the construct it was designed to measure?
- Face validity : Does the test appear to be suitable for its objectives ?
- Content validity : Does the test cover all relevant parts of the construct it aims to measure.
- Criterion validity : Do the results accurately measure the concrete outcome they are designed to measure?
Criterion validity evaluates how well a test measures the outcome it was designed to measure. An outcome can be, for example, the onset of a disease.
Criterion validity consists of two subtypes depending on the time at which the two measures (the criterion and your test) are obtained:
- Concurrent validity is a validation strategy where the the scores of a test and the criterion are obtained at the same time
- Predictive validity is a validation strategy where the criterion variables are measured after the scores of the test
Attrition refers to participants leaving a study. It always happens to some extent – for example, in randomised control trials for medical research.
Differential attrition occurs when attrition or dropout rates differ systematically between the intervention and the control group . As a result, the characteristics of the participants who drop out differ from the characteristics of those who stay in the study. Because of this, study results may be biased .
Criterion validity and construct validity are both types of measurement validity . In other words, they both show you how accurately a method measures something.
While construct validity is the degree to which a test or other measurement method measures what it claims to measure, criterion validity is the degree to which a test can predictively (in the future) or concurrently (in the present) measure something.
Construct validity is often considered the overarching type of measurement validity . You need to have face validity , content validity , and criterion validity in order to achieve construct validity.
Convergent validity and discriminant validity are both subtypes of construct validity . Together, they help you evaluate whether a test measures the concept it was designed to measure.
- Convergent validity indicates whether a test that is designed to measure a particular construct correlates with other tests that assess the same or similar construct.
- Discriminant validity indicates whether two tests that should not be highly related to each other are indeed not related. This type of validity is also called divergent validity .
You need to assess both in order to demonstrate construct validity. Neither one alone is sufficient for establishing construct validity.
Face validity and content validity are similar in that they both evaluate how suitable the content of a test is. The difference is that face validity is subjective, and assesses content at surface level.
When a test has strong face validity, anyone would agree that the test’s questions appear to measure what they are intended to measure.
For example, looking at a 4th grade math test consisting of problems in which students have to add and multiply, most people would agree that it has strong face validity (i.e., it looks like a math test).
On the other hand, content validity evaluates how well a test represents all the aspects of a topic. Assessing content validity is more systematic and relies on expert evaluation. of each question, analysing whether each one covers the aspects that the test was designed to cover.
A 4th grade math test would have high content validity if it covered all the skills taught in that grade. Experts(in this case, math teachers), would have to evaluate the content validity by comparing the test to the learning objectives.
Content validity shows you how accurately a test or other measurement method taps into the various aspects of the specific construct you are researching.
In other words, it helps you answer the question: “does the test measure all aspects of the construct I want to measure?” If it does, then the test has high content validity.
The higher the content validity, the more accurate the measurement of the construct.
If the test fails to include parts of the construct, or irrelevant parts are included, the validity of the instrument is threatened, which brings your results into question.
Construct validity refers to how well a test measures the concept (or construct) it was designed to measure. Assessing construct validity is especially important when you’re researching concepts that can’t be quantified and/or are intangible, like introversion. To ensure construct validity your test should be based on known indicators of introversion ( operationalisation ).
On the other hand, content validity assesses how well the test represents all aspects of the construct. If some aspects are missing or irrelevant parts are included, the test has low content validity.
- Discriminant validity indicates whether two tests that should not be highly related to each other are indeed not related
Construct validity has convergent and discriminant subtypes. They assist determine if a test measures the intended notion.
The reproducibility and replicability of a study can be ensured by writing a transparent, detailed method section and using clear, unambiguous language.
Reproducibility and replicability are related terms.
- A successful reproduction shows that the data analyses were conducted in a fair and honest manner.
- A successful replication shows that the reliability of the results is high.
- Reproducing research entails reanalysing the existing data in the same manner.
- Replicating (or repeating ) the research entails reconducting the entire analysis, including the collection of new data .
Snowball sampling is a non-probability sampling method . Unlike probability sampling (which involves some form of random selection ), the initial individuals selected to be studied are the ones who recruit new participants.
Because not every member of the target population has an equal chance of being recruited into the sample, selection in snowball sampling is non-random.
Snowball sampling is a non-probability sampling method , where there is not an equal chance for every member of the population to be included in the sample .
This means that you cannot use inferential statistics and make generalisations – often the goal of quantitative research . As such, a snowball sample is not representative of the target population, and is usually a better fit for qualitative research .
Snowball sampling relies on the use of referrals. Here, the researcher recruits one or more initial participants, who then recruit the next ones.
Participants share similar characteristics and/or know each other. Because of this, not every member of the population has an equal chance of being included in the sample, giving rise to sampling bias .
Snowball sampling is best used in the following cases:
- If there is no sampling frame available (e.g., people with a rare disease)
- If the population of interest is hard to access or locate (e.g., people experiencing homelessness)
- If the research focuses on a sensitive topic (e.g., extra-marital affairs)
Stratified sampling and quota sampling both involve dividing the population into subgroups and selecting units from each subgroup. The purpose in both cases is to select a representative sample and/or to allow comparisons between subgroups.
The main difference is that in stratified sampling, you draw a random sample from each subgroup ( probability sampling ). In quota sampling you select a predetermined number or proportion of units, in a non-random manner ( non-probability sampling ).
Random sampling or probability sampling is based on random selection. This means that each unit has an equal chance (i.e., equal probability) of being included in the sample.
On the other hand, convenience sampling involves stopping people at random, which means that not everyone has an equal chance of being selected depending on the place, time, or day you are collecting your data.
Convenience sampling and quota sampling are both non-probability sampling methods. They both use non-random criteria like availability, geographical proximity, or expert knowledge to recruit study participants.
However, in convenience sampling, you continue to sample units or cases until you reach the required sample size.
In quota sampling, you first need to divide your population of interest into subgroups (strata) and estimate their proportions (quota) in the population. Then you can start your data collection , using convenience sampling to recruit participants, until the proportions in each subgroup coincide with the estimated proportions in the population.
A sampling frame is a list of every member in the entire population . It is important that the sampling frame is as complete as possible, so that your sample accurately reflects your population.
Stratified and cluster sampling may look similar, but bear in mind that groups created in cluster sampling are heterogeneous , so the individual characteristics in the cluster vary. In contrast, groups created in stratified sampling are homogeneous , as units share characteristics.
Relatedly, in cluster sampling you randomly select entire groups and include all units of each group in your sample. However, in stratified sampling, you select some units of all groups and include them in your sample. In this way, both methods can ensure that your sample is representative of the target population .
When your population is large in size, geographically dispersed, or difficult to contact, it’s necessary to use a sampling method .
This allows you to gather information from a smaller part of the population, i.e. the sample, and make accurate statements by using statistical analysis. A few sampling methods include simple random sampling , convenience sampling , and snowball sampling .
The two main types of social desirability bias are:
- Self-deceptive enhancement (self-deception): The tendency to see oneself in a favorable light without realizing it.
- Impression managemen t (other-deception): The tendency to inflate one’s abilities or achievement in order to make a good impression on other people.
Response bias refers to conditions or factors that take place during the process of responding to surveys, affecting the responses. One type of response bias is social desirability bias .
Demand characteristics are aspects of experiments that may give away the research objective to participants. Social desirability bias occurs when participants automatically try to respond in ways that make them seem likeable in a study, even if it means misrepresenting how they truly feel.
Participants may use demand characteristics to infer social norms or experimenter expectancies and act in socially desirable ways, so you should try to control for demand characteristics wherever possible.
A systematic review is secondary research because it uses existing research. You don’t collect new data yourself.
Ethical considerations in research are a set of principles that guide your research designs and practices. These principles include voluntary participation, informed consent, anonymity, confidentiality, potential for harm, and results communication.
Scientists and researchers must always adhere to a certain code of conduct when collecting data from others .
These considerations protect the rights of research participants, enhance research validity , and maintain scientific integrity.
Research ethics matter for scientific integrity, human rights and dignity, and collaboration between science and society. These principles make sure that participation in studies is voluntary, informed, and safe.
Research misconduct means making up or falsifying data, manipulating data analyses, or misrepresenting results in research reports. It’s a form of academic fraud.
These actions are committed intentionally and can have serious consequences; research misconduct is not a simple mistake or a point of disagreement but a serious ethical failure.
Anonymity means you don’t know who the participants are, while confidentiality means you know who they are but remove identifying information from your research report. Both are important ethical considerations .
You can only guarantee anonymity by not collecting any personally identifying information – for example, names, phone numbers, email addresses, IP addresses, physical characteristics, photos, or videos.
You can keep data confidential by using aggregate information in your research report, so that you only refer to groups of participants rather than individuals.
Peer review is a process of evaluating submissions to an academic journal. Utilising rigorous criteria, a panel of reviewers in the same subject area decide whether to accept each submission for publication.
For this reason, academic journals are often considered among the most credible sources you can use in a research project – provided that the journal itself is trustworthy and well regarded.
In general, the peer review process follows the following steps:
- First, the author submits the manuscript to the editor.
- Reject the manuscript and send it back to author, or
- Send it onward to the selected peer reviewer(s)
- Next, the peer review process occurs. The reviewer provides feedback, addressing any major or minor issues with the manuscript, and gives their advice regarding what edits should be made.
- Lastly, the edited manuscript is sent back to the author. They input the edits, and resubmit it to the editor for publication.
Peer review can stop obviously problematic, falsified, or otherwise untrustworthy research from being published. It also represents an excellent opportunity to get feedback from renowned experts in your field.
It acts as a first defence, helping you ensure your argument is clear and that there are no gaps, vague terms, or unanswered questions for readers who weren’t involved in the research process.
Peer-reviewed articles are considered a highly credible source due to this stringent process they go through before publication.
Many academic fields use peer review , largely to determine whether a manuscript is suitable for publication. Peer review enhances the credibility of the published manuscript.
However, peer review is also common in non-academic settings. The United Nations, the European Union, and many individual nations use peer review to evaluate grant applications. It is also widely used in medical and health-related fields as a teaching or quality-of-care measure.
Peer assessment is often used in the classroom as a pedagogical tool. Both receiving feedback and providing it are thought to enhance the learning process, helping students think critically and collaboratively.
- In a single-blind study , only the participants are blinded.
- In a double-blind study , both participants and experimenters are blinded.
- In a triple-blind study , the assignment is hidden not only from participants and experimenters, but also from the researchers analysing the data.
Blinding is important to reduce bias (e.g., observer bias , demand characteristics ) and ensure a study’s internal validity .
If participants know whether they are in a control or treatment group , they may adjust their behaviour in ways that affect the outcome that researchers are trying to measure. If the people administering the treatment are aware of group assignment, they may treat participants differently and thus directly or indirectly influence the final results.
Blinding means hiding who is assigned to the treatment group and who is assigned to the control group in an experiment .
Explanatory research is a research method used to investigate how or why something occurs when only a small amount of information is available pertaining to that topic. It can help you increase your understanding of a given topic.
Explanatory research is used to investigate how or why a phenomenon occurs. Therefore, this type of research is often one of the first stages in the research process , serving as a jumping-off point for future research.
Exploratory research is a methodology approach that explores research questions that have not previously been studied in depth. It is often used when the issue you’re studying is new, or the data collection process is challenging in some way.
Exploratory research is often used when the issue you’re studying is new or when the data collection process is challenging for some reason.
You can use exploratory research if you have a general idea or a specific question that you want to study but there is no preexisting knowledge or paradigm with which to study it.
To implement random assignment , assign a unique number to every member of your study’s sample .
Then, you can use a random number generator or a lottery method to randomly assign each number to a control or experimental group. You can also do so manually, by flipping a coin or rolling a die to randomly assign participants to groups.
Random selection, or random sampling , is a way of selecting members of a population for your study’s sample.
In contrast, random assignment is a way of sorting the sample into control and experimental groups.
Random sampling enhances the external validity or generalisability of your results, while random assignment improves the internal validity of your study.
Random assignment is used in experiments with a between-groups or independent measures design. In this research design, there’s usually a control group and one or more experimental groups. Random assignment helps ensure that the groups are comparable.
In general, you should always use random assignment in this type of experimental design when it is ethically possible and makes sense for your study topic.
Clean data are valid, accurate, complete, consistent, unique, and uniform. Dirty data include inconsistencies and errors.
Dirty data can come from any part of the research process, including poor research design , inappropriate measurement materials, or flawed data entry.
Data cleaning takes place between data collection and data analyses. But you can use some methods even before collecting data.
For clean data, you should start by designing measures that collect valid data. Data validation at the time of data entry or collection helps you minimize the amount of data cleaning you’ll need to do.
After data collection, you can use data standardisation and data transformation to clean your data. You’ll also deal with any missing values, outliers, and duplicate values.
Data cleaning involves spotting and resolving potential data inconsistencies or errors to improve your data quality. An error is any value (e.g., recorded weight) that doesn’t reflect the true value (e.g., actual weight) of something that’s being measured.
In this process, you review, analyse, detect, modify, or remove ‘dirty’ data to make your dataset ‘clean’. Data cleaning is also called data cleansing or data scrubbing.
Data cleaning is necessary for valid and appropriate analyses. Dirty data contain inconsistencies or errors , but cleaning your data helps you minimise or resolve these.
Without data cleaning, you could end up with a Type I or II error in your conclusion. These types of erroneous conclusions can be practically significant with important consequences, because they lead to misplaced investments or missed opportunities.
Observer bias occurs when a researcher’s expectations, opinions, or prejudices influence what they perceive or record in a study. It usually affects studies when observers are aware of the research aims or hypotheses. This type of research bias is also called detection bias or ascertainment bias .
The observer-expectancy effect occurs when researchers influence the results of their own study through interactions with participants.
Researchers’ own beliefs and expectations about the study results may unintentionally influence participants through demand characteristics .
You can use several tactics to minimise observer bias .
- Use masking (blinding) to hide the purpose of your study from all observers.
- Triangulate your data with different data collection methods or sources.
- Use multiple observers and ensure inter-rater reliability.
- Train your observers to make sure data is consistently recorded between them.
- Standardise your observation procedures to make sure they are structured and clear.
Naturalistic observation is a valuable tool because of its flexibility, external validity , and suitability for topics that can’t be studied in a lab setting.
The downsides of naturalistic observation include its lack of scientific control , ethical considerations , and potential for bias from observers and subjects.
Naturalistic observation is a qualitative research method where you record the behaviours of your research subjects in real-world settings. You avoid interfering or influencing anything in a naturalistic observation.
You can think of naturalistic observation as ‘people watching’ with a purpose.
Closed-ended, or restricted-choice, questions offer respondents a fixed set of choices to select from. These questions are easier to answer quickly.
Open-ended or long-form questions allow respondents to answer in their own words. Because there are no restrictions on their choices, respondents can answer in ways that researchers may not have otherwise considered.
You can organise the questions logically, with a clear progression from simple to complex, or randomly between respondents. A logical flow helps respondents process the questionnaire easier and quicker, but it may lead to bias. Randomisation can minimise the bias from order effects.
Questionnaires can be self-administered or researcher-administered.
Self-administered questionnaires can be delivered online or in paper-and-pen formats, in person or by post. All questions are standardised so that all respondents receive the same questions with identical wording.
Researcher-administered questionnaires are interviews that take place by phone, in person, or online between researchers and respondents. You can gain deeper insights by clarifying questions for respondents or asking follow-up questions.
In a controlled experiment , all extraneous variables are held constant so that they can’t influence the results. Controlled experiments require:
- A control group that receives a standard treatment, a fake treatment, or no treatment
- Random assignment of participants to ensure the groups are equivalent
Depending on your study topic, there are various other methods of controlling variables .
An experimental group, also known as a treatment group, receives the treatment whose effect researchers wish to study, whereas a control group does not. They should be identical in all other ways.
A true experiment (aka a controlled experiment) always includes at least one control group that doesn’t receive the experimental treatment.
However, some experiments use a within-subjects design to test treatments without a control group. In these designs, you usually compare one group’s outcomes before and after a treatment (instead of comparing outcomes between different groups).
For strong internal validity , it’s usually best to include a control group if possible. Without a control group, it’s harder to be certain that the outcome was caused by the experimental treatment and not by other variables.
A questionnaire is a data collection tool or instrument, while a survey is an overarching research method that involves collecting and analysing data from people using questionnaires.
A Likert scale is a rating scale that quantitatively assesses opinions, attitudes, or behaviours. It is made up of four or more questions that measure a single attitude or trait when response scores are combined.
To use a Likert scale in a survey , you present participants with Likert-type questions or statements, and a continuum of items, usually with five or seven possible responses, to capture their degree of agreement.
Individual Likert-type questions are generally considered ordinal data , because the items have clear rank order, but don’t have an even distribution.
Overall Likert scale scores are sometimes treated as interval data. These scores are considered to have directionality and even spacing between them.
The type of data determines what statistical tests you should use to analyse your data.
A research hypothesis is your proposed answer to your research question. The research hypothesis usually includes an explanation (‘ x affects y because …’).
A statistical hypothesis, on the other hand, is a mathematical statement about a population parameter. Statistical hypotheses always come in pairs: the null and alternative hypotheses. In a well-designed study , the statistical hypotheses correspond logically to the research hypothesis.
Cross-sectional studies are less expensive and time-consuming than many other types of study. They can provide useful insights into a population’s characteristics and identify correlations for further research.
Sometimes only cross-sectional data are available for analysis; other times your research question may only require a cross-sectional study to answer it.
Cross-sectional studies cannot establish a cause-and-effect relationship or analyse behaviour over a period of time. To investigate cause and effect, you need to do a longitudinal study or an experimental study .
Longitudinal studies and cross-sectional studies are two different types of research design . In a cross-sectional study you collect data from a population at a specific point in time; in a longitudinal study you repeatedly collect data from the same sample over an extended period of time.
Longitudinal studies are better to establish the correct sequence of events, identify changes over time, and provide insight into cause-and-effect relationships, but they also tend to be more expensive and time-consuming than other types of studies.
The 1970 British Cohort Study , which has collected data on the lives of 17,000 Brits since their births in 1970, is one well-known example of a longitudinal study .
Longitudinal studies can last anywhere from weeks to decades, although they tend to be at least a year long.
A correlation reflects the strength and/or direction of the association between two or more variables.
- A positive correlation means that both variables change in the same direction.
- A negative correlation means that the variables change in opposite directions.
- A zero correlation means there’s no relationship between the variables.
A correlational research design investigates relationships between two variables (or more) without the researcher controlling or manipulating any of them. It’s a non-experimental type of quantitative research .
A correlation coefficient is a single number that describes the strength and direction of the relationship between your variables.
Different types of correlation coefficients might be appropriate for your data based on their levels of measurement and distributions . The Pearson product-moment correlation coefficient (Pearson’s r ) is commonly used to assess a linear relationship between two quantitative variables.
Controlled experiments establish causality, whereas correlational studies only show associations between variables.
- In an experimental design , you manipulate an independent variable and measure its effect on a dependent variable. Other variables are controlled so they can’t impact the results.
- In a correlational design , you measure variables without manipulating any of them. You can test whether your variables change together, but you can’t be sure that one variable caused a change in another.
In general, correlational research is high in external validity while experimental research is high in internal validity .
The third variable and directionality problems are two main reasons why correlation isn’t causation .
The third variable problem means that a confounding variable affects both variables to make them seem causally related when they are not.
The directionality problem is when two variables correlate and might actually have a causal relationship, but it’s impossible to conclude which variable causes changes in the other.
As a rule of thumb, questions related to thoughts, beliefs, and feelings work well in focus groups . Take your time formulating strong questions, paying special attention to phrasing. Be careful to avoid leading questions , which can bias your responses.
Overall, your focus group questions should be:
- Open-ended and flexible
- Impossible to answer with ‘yes’ or ‘no’ (questions that start with ‘why’ or ‘how’ are often best)
- Unambiguous, getting straight to the point while still stimulating discussion
- Unbiased and neutral
Social desirability bias is the tendency for interview participants to give responses that will be viewed favourably by the interviewer or other participants. It occurs in all types of interviews and surveys , but is most common in semi-structured interviews , unstructured interviews , and focus groups .
Social desirability bias can be mitigated by ensuring participants feel at ease and comfortable sharing their views. Make sure to pay attention to your own body language and any physical or verbal cues, such as nodding or widening your eyes.
This type of bias in research can also occur in observations if the participants know they’re being observed. They might alter their behaviour accordingly.
A focus group is a research method that brings together a small group of people to answer questions in a moderated setting. The group is chosen due to predefined demographic traits, and the questions are designed to shed light on a topic of interest. It is one of four types of interviews .
The four most common types of interviews are:
- Structured interviews : The questions are predetermined in both topic and order.
- Semi-structured interviews : A few questions are predetermined, but other questions aren’t planned.
- Unstructured interviews : None of the questions are predetermined.
- Focus group interviews : The questions are presented to a group instead of one individual.
An unstructured interview is the most flexible type of interview, but it is not always the best fit for your research topic.
Unstructured interviews are best used when:
- You are an experienced interviewer and have a very strong background in your research topic, since it is challenging to ask spontaneous, colloquial questions
- Your research question is exploratory in nature. While you may have developed hypotheses, you are open to discovering new or shifting viewpoints through the interview process.
- You are seeking descriptive data, and are ready to ask questions that will deepen and contextualise your initial thoughts and hypotheses
- Your research depends on forming connections with your participants and making them feel comfortable revealing deeper emotions, lived experiences, or thoughts
A semi-structured interview is a blend of structured and unstructured types of interviews. Semi-structured interviews are best used when:
- You have prior interview experience. Spontaneous questions are deceptively challenging, and it’s easy to accidentally ask a leading question or make a participant uncomfortable.
- Your research question is exploratory in nature. Participant answers can guide future research questions and help you develop a more robust knowledge base for future research.
The interviewer effect is a type of bias that emerges when a characteristic of an interviewer (race, age, gender identity, etc.) influences the responses given by the interviewee.
There is a risk of an interviewer effect in all types of interviews , but it can be mitigated by writing really high-quality interview questions.
A structured interview is a data collection method that relies on asking questions in a set order to collect data on a topic. They are often quantitative in nature. Structured interviews are best used when:
- You already have a very clear understanding of your topic. Perhaps significant research has already been conducted, or you have done some prior research yourself, but you already possess a baseline for designing strong structured questions.
- You are constrained in terms of time or resources and need to analyse your data quickly and efficiently
- Your research question depends on strong parity between participants, with environmental conditions held constant
More flexible interview options include semi-structured interviews , unstructured interviews , and focus groups .
When conducting research, collecting original data has significant advantages:
- You can tailor data collection to your specific research aims (e.g., understanding the needs of your consumers or user testing your website).
- You can control and standardise the process for high reliability and validity (e.g., choosing appropriate measurements and sampling methods ).
However, there are also some drawbacks: data collection can be time-consuming, labour-intensive, and expensive. In some cases, it’s more efficient to use secondary data that has already been collected by someone else, but the data might be less reliable.
Data collection is the systematic process by which observations or measurements are gathered in research. It is used in many different contexts by academics, governments, businesses, and other organisations.
A mediator variable explains the process through which two variables are related, while a moderator variable affects the strength and direction of that relationship.
A confounder is a third variable that affects variables of interest and makes them seem related when they are not. In contrast, a mediator is the mechanism of a relationship between two variables: it explains the process by which they are related.
If something is a mediating variable :
- It’s caused by the independent variable
- It influences the dependent variable
- When it’s taken into account, the statistical correlation between the independent and dependent variables is higher than when it isn’t considered
Including mediators and moderators in your research helps you go beyond studying a simple relationship between two variables for a fuller picture of the real world. They are important to consider when studying complex correlational or causal relationships.
Mediators are part of the causal pathway of an effect, and they tell you how or why an effect takes place. Moderators usually help you judge the external validity of your study by identifying the limitations of when the relationship between variables holds.
You can think of independent and dependent variables in terms of cause and effect: an independent variable is the variable you think is the cause , while a dependent variable is the effect .
In an experiment, you manipulate the independent variable and measure the outcome in the dependent variable. For example, in an experiment about the effect of nutrients on crop growth:
- The independent variable is the amount of nutrients added to the crop field.
- The dependent variable is the biomass of the crops at harvest time.
Defining your variables, and deciding how you will manipulate and measure them, is an important part of experimental design .
Discrete and continuous variables are two types of quantitative variables :
- Discrete variables represent counts (e.g., the number of objects in a collection).
- Continuous variables represent measurable amounts (e.g., water volume or weight).
Quantitative variables are any variables where the data represent amounts (e.g. height, weight, or age).
Categorical variables are any variables where the data represent groups. This includes rankings (e.g. finishing places in a race), classifications (e.g. brands of cereal), and binary outcomes (e.g. coin flips).
You need to know what type of variables you are working with to choose the right statistical test for your data and interpret your results .
Determining cause and effect is one of the most important parts of scientific research. It’s essential to know which is the cause – the independent variable – and which is the effect – the dependent variable.
You want to find out how blood sugar levels are affected by drinking diet cola and regular cola, so you conduct an experiment .
- The type of cola – diet or regular – is the independent variable .
- The level of blood sugar that you measure is the dependent variable – it changes depending on the type of cola.
No. The value of a dependent variable depends on an independent variable, so a variable cannot be both independent and dependent at the same time. It must be either the cause or the effect, not both.
Yes, but including more than one of either type requires multiple research questions .
For example, if you are interested in the effect of a diet on health, you can use multiple measures of health: blood sugar, blood pressure, weight, pulse, and many more. Each of these is its own dependent variable with its own research question.
You could also choose to look at the effect of exercise levels as well as diet, or even the additional effect of the two combined. Each of these is a separate independent variable .
To ensure the internal validity of an experiment , you should only change one independent variable at a time.
To ensure the internal validity of your research, you must consider the impact of confounding variables. If you fail to account for them, you might over- or underestimate the causal relationship between your independent and dependent variables , or even find a causal relationship where none exists.
A confounding variable is closely related to both the independent and dependent variables in a study. An independent variable represents the supposed cause , while the dependent variable is the supposed effect . A confounding variable is a third variable that influences both the independent and dependent variables.
Failing to account for confounding variables can cause you to wrongly estimate the relationship between your independent and dependent variables.
There are several methods you can use to decrease the impact of confounding variables on your research: restriction, matching, statistical control, and randomisation.
In restriction , you restrict your sample by only including certain subjects that have the same values of potential confounding variables.
In matching , you match each of the subjects in your treatment group with a counterpart in the comparison group. The matched subjects have the same values on any potential confounding variables, and only differ in the independent variable .
In statistical control , you include potential confounders as variables in your regression .
In randomisation , you randomly assign the treatment (or independent variable) in your study to a sufficiently large number of subjects, which allows you to control for all potential confounding variables.
In scientific research, concepts are the abstract ideas or phenomena that are being studied (e.g., educational achievement). Variables are properties or characteristics of the concept (e.g., performance at school), while indicators are ways of measuring or quantifying variables (e.g., yearly grade reports).
The process of turning abstract concepts into measurable variables and indicators is called operationalisation .
In statistics, ordinal and nominal variables are both considered categorical variables .
Even though ordinal data can sometimes be numerical, not all mathematical operations can be performed on them.
A control variable is any variable that’s held constant in a research study. It’s not a variable of interest in the study, but it’s controlled because it could influence the outcomes.
Control variables help you establish a correlational or causal relationship between variables by enhancing internal validity .
If you don’t control relevant extraneous variables , they may influence the outcomes of your study, and you may not be able to demonstrate that your results are really an effect of your independent variable .
‘Controlling for a variable’ means measuring extraneous variables and accounting for them statistically to remove their effects on other variables.
Researchers often model control variable data along with independent and dependent variable data in regression analyses and ANCOVAs . That way, you can isolate the control variable’s effects from the relationship between the variables of interest.
An extraneous variable is any variable that you’re not investigating that can potentially affect the dependent variable of your research study.
A confounding variable is a type of extraneous variable that not only affects the dependent variable, but is also related to the independent variable.
There are 4 main types of extraneous variables :
- Demand characteristics : Environmental cues that encourage participants to conform to researchers’ expectations
- Experimenter effects : Unintentional actions by researchers that influence study outcomes
- Situational variables : Eenvironmental variables that alter participants’ behaviours
- Participant variables : Any characteristic or aspect of a participant’s background that could affect study results
The difference between explanatory and response variables is simple:
- An explanatory variable is the expected cause, and it explains the results.
- A response variable is the expected effect, and it responds to other variables.
The term ‘ explanatory variable ‘ is sometimes preferred over ‘ independent variable ‘ because, in real-world contexts, independent variables are often influenced by other variables. This means they aren’t totally independent.
Multiple independent variables may also be correlated with each other, so ‘explanatory variables’ is a more appropriate term.
On graphs, the explanatory variable is conventionally placed on the x -axis, while the response variable is placed on the y -axis.
- If you have quantitative variables , use a scatterplot or a line graph.
- If your response variable is categorical, use a scatterplot or a line graph.
- If your explanatory variable is categorical, use a bar graph.
A correlation is usually tested for two variables at a time, but you can test correlations between three or more variables.
An independent variable is the variable you manipulate, control, or vary in an experimental study to explore its effects. It’s called ‘independent’ because it’s not influenced by any other variables in the study.
Independent variables are also called:
- Explanatory variables (they explain an event or outcome)
- Predictor variables (they can be used to predict the value of a dependent variable)
- Right-hand-side variables (they appear on the right-hand side of a regression equation)
A dependent variable is what changes as a result of the independent variable manipulation in experiments . It’s what you’re interested in measuring, and it ‘depends’ on your independent variable.
In statistics, dependent variables are also called:
- Response variables (they respond to a change in another variable)
- Outcome variables (they represent the outcome you want to measure)
- Left-hand-side variables (they appear on the left-hand side of a regression equation)
Deductive reasoning is commonly used in scientific research, and it’s especially associated with quantitative research .
In research, you might have come across something called the hypothetico-deductive method . It’s the scientific method of testing hypotheses to check whether your predictions are substantiated by real-world data.
Deductive reasoning is a logical approach where you progress from general ideas to specific conclusions. It’s often contrasted with inductive reasoning , where you start with specific observations and form general conclusions.
Deductive reasoning is also called deductive logic.
Inductive reasoning is a method of drawing conclusions by going from the specific to the general. It’s usually contrasted with deductive reasoning, where you proceed from general information to specific conclusions.
Inductive reasoning is also called inductive logic or bottom-up reasoning.
In inductive research , you start by making observations or gathering data. Then, you take a broad scan of your data and search for patterns. Finally, you make general conclusions that you might incorporate into theories.
Inductive reasoning is a bottom-up approach, while deductive reasoning is top-down.
Inductive reasoning takes you from the specific to the general, while in deductive reasoning, you make inferences by going from general premises to specific conclusions.
There are many different types of inductive reasoning that people use formally or informally.
Here are a few common types:
- Inductive generalisation : You use observations about a sample to come to a conclusion about the population it came from.
- Statistical generalisation: You use specific numbers about samples to make statements about populations.
- Causal reasoning: You make cause-and-effect links between different things.
- Sign reasoning: You make a conclusion about a correlational relationship between different things.
- Analogical reasoning: You make a conclusion about something based on its similarities to something else.
It’s often best to ask a variety of people to review your measurements. You can ask experts, such as other researchers, or laypeople, such as potential participants, to judge the face validity of tests.
While experts have a deep understanding of research methods , the people you’re studying can provide you with valuable insights you may have missed otherwise.
Face validity is important because it’s a simple first step to measuring the overall validity of a test or technique. It’s a relatively intuitive, quick, and easy way to start checking whether a new measure seems useful at first glance.
Good face validity means that anyone who reviews your measure says that it seems to be measuring what it’s supposed to. With poor face validity, someone reviewing your measure may be left confused about what you’re measuring and why you’re using this method.
Face validity is about whether a test appears to measure what it’s supposed to measure. This type of validity is concerned with whether a measure seems relevant and appropriate for what it’s assessing only on the surface.
Statistical analyses are often applied to test validity with data from your measures. You test convergent validity and discriminant validity with correlations to see if results from your test are positively or negatively related to those of other established tests.
You can also use regression analyses to assess whether your measure is actually predictive of outcomes that you expect it to predict theoretically. A regression analysis that supports your expectations strengthens your claim of construct validity .
When designing or evaluating a measure, construct validity helps you ensure you’re actually measuring the construct you’re interested in. If you don’t have construct validity, you may inadvertently measure unrelated or distinct constructs and lose precision in your research.
Construct validity is often considered the overarching type of measurement validity , because it covers all of the other types. You need to have face validity , content validity, and criterion validity to achieve construct validity.
Construct validity is about how well a test measures the concept it was designed to evaluate. It’s one of four types of measurement validity , which includes construct validity, face validity , and criterion validity.
There are two subtypes of construct validity.
- Convergent validity : The extent to which your measure corresponds to measures of related constructs
- Discriminant validity: The extent to which your measure is unrelated or negatively related to measures of distinct constructs
Attrition bias can skew your sample so that your final sample differs significantly from your original sample. Your sample is biased because some groups from your population are underrepresented.
With a biased final sample, you may not be able to generalise your findings to the original population that you sampled from, so your external validity is compromised.
There are seven threats to external validity : selection bias , history, experimenter effect, Hawthorne effect , testing effect, aptitude-treatment, and situation effect.
The two types of external validity are population validity (whether you can generalise to other groups of people) and ecological validity (whether you can generalise to other situations and settings).
The external validity of a study is the extent to which you can generalise your findings to different groups of people, situations, and measures.
Attrition bias is a threat to internal validity . In experiments, differential rates of attrition between treatment and control groups can skew results.
This bias can affect the relationship between your independent and dependent variables . It can make variables appear to be correlated when they are not, or vice versa.
Internal validity is the extent to which you can be confident that a cause-and-effect relationship established in a study cannot be explained by other factors.
There are eight threats to internal validity : history, maturation, instrumentation, testing, selection bias , regression to the mean, social interaction, and attrition .
A sampling error is the difference between a population parameter and a sample statistic .
A statistic refers to measures about the sample , while a parameter refers to measures about the population .
Populations are used when a research question requires data from every member of the population. This is usually only feasible when the population is small and easily accessible.
Systematic sampling is a probability sampling method where researchers select members of the population at a regular interval – for example, by selecting every 15th person on a list of the population. If the population is in a random order, this can imitate the benefits of simple random sampling .
There are three key steps in systematic sampling :
- Define and list your population , ensuring that it is not ordered in a cyclical or periodic order.
- Decide on your sample size and calculate your interval, k , by dividing your population by your target sample size.
- Choose every k th member of the population as your sample.
Yes, you can create a stratified sample using multiple characteristics, but you must ensure that every participant in your study belongs to one and only one subgroup. In this case, you multiply the numbers of subgroups for each characteristic to get the total number of groups.
For example, if you were stratifying by location with three subgroups (urban, rural, or suburban) and marital status with five subgroups (single, divorced, widowed, married, or partnered), you would have 3 × 5 = 15 subgroups.
You should use stratified sampling when your sample can be divided into mutually exclusive and exhaustive subgroups that you believe will take on different mean values for the variable that you’re studying.
Using stratified sampling will allow you to obtain more precise (with lower variance ) statistical estimates of whatever you are trying to measure.
For example, say you want to investigate how income differs based on educational attainment, but you know that this relationship can vary based on race. Using stratified sampling, you can ensure you obtain a large enough sample from each racial group, allowing you to draw more precise conclusions.
In stratified sampling , researchers divide subjects into subgroups called strata based on characteristics that they share (e.g., race, gender, educational attainment).
Once divided, each subgroup is randomly sampled using another probability sampling method .
Multistage sampling can simplify data collection when you have large, geographically spread samples, and you can obtain a probability sample without a complete sampling frame.
But multistage sampling may not lead to a representative sample, and larger samples are needed for multistage samples to achieve the statistical properties of simple random samples .
In multistage sampling , you can use probability or non-probability sampling methods.
For a probability sample, you have to probability sampling at every stage. You can mix it up by using simple random sampling , systematic sampling , or stratified sampling to select units at different stages, depending on what is applicable and relevant to your study.
Cluster sampling is a probability sampling method in which you divide a population into clusters, such as districts or schools, and then randomly select some of these clusters as your sample.
The clusters should ideally each be mini-representations of the population as a whole.
There are three types of cluster sampling : single-stage, double-stage and multi-stage clustering. In all three types, you first divide the population into clusters, then randomly select clusters for use in your sample.
- In single-stage sampling , you collect data from every unit within the selected clusters.
- In double-stage sampling , you select a random sample of units from within the clusters.
- In multi-stage sampling , you repeat the procedure of randomly sampling elements from within the clusters until you have reached a manageable sample.
Cluster sampling is more time- and cost-efficient than other probability sampling methods , particularly when it comes to large samples spread across a wide geographical area.
However, it provides less statistical certainty than other methods, such as simple random sampling , because it is difficult to ensure that your clusters properly represent the population as a whole.
If properly implemented, simple random sampling is usually the best sampling method for ensuring both internal and external validity . However, it can sometimes be impractical and expensive to implement, depending on the size of the population to be studied,
If you have a list of every member of the population and the ability to reach whichever members are selected, you can use simple random sampling.
The American Community Survey is an example of simple random sampling . In order to collect detailed data on the population of the US, the Census Bureau officials randomly select 3.5 million households per year and use a variety of methods to convince them to fill out the survey.
Simple random sampling is a type of probability sampling in which the researcher randomly selects a subset of participants from a population . Each member of the population has an equal chance of being selected. Data are then collected from as large a percentage as possible of this random subset.
Sampling bias occurs when some members of a population are systematically more likely to be selected in a sample than others.
In multistage sampling , or multistage cluster sampling, you draw a sample from a population using smaller and smaller groups at each stage.
This method is often used to collect data from a large, geographically spread group of people in national surveys, for example. You take advantage of hierarchical groupings (e.g., from county to city to neighbourhood) to create a sample that’s less expensive and time-consuming to collect data from.
In non-probability sampling , the sample is selected based on non-random criteria, and not every member of the population has a chance of being included.
Common non-probability sampling methods include convenience sampling , voluntary response sampling, purposive sampling , snowball sampling , and quota sampling .
Probability sampling means that every member of the target population has a known chance of being included in the sample.
Probability sampling methods include simple random sampling , systematic sampling , stratified sampling , and cluster sampling .
Samples are used to make inferences about populations . Samples are easier to collect data from because they are practical, cost-effective, convenient, and manageable.
While a between-subjects design has fewer threats to internal validity , it also requires more participants for high statistical power than a within-subjects design .
Advantages:
- Prevents carryover effects of learning and fatigue.
- Shorter study duration.
Disadvantages:
- Needs larger samples for high power.
- Uses more resources to recruit participants, administer sessions, cover costs, etc.
- Individual differences may be an alternative explanation for results.
In a factorial design, multiple independent variables are tested.
If you test two variables, each level of one independent variable is combined with each level of the other independent variable to create different conditions.
Yes. Between-subjects and within-subjects designs can be combined in a single study when you have two or more independent variables (a factorial design). In a mixed factorial design, one variable is altered between subjects and another is altered within subjects.
Within-subjects designs have many potential threats to internal validity , but they are also very statistically powerful .
- Only requires small samples
- Statistically powerful
- Removes the effects of individual differences on the outcomes
- Internal validity threats reduce the likelihood of establishing a direct relationship between variables
- Time-related effects, such as growth, can influence the outcomes
- Carryover effects mean that the specific order of different treatments affect the outcomes
Quasi-experimental design is most useful in situations where it would be unethical or impractical to run a true experiment .
Quasi-experiments have lower internal validity than true experiments, but they often have higher external validity as they can use real-world interventions instead of artificial laboratory settings.
In experimental research, random assignment is a way of placing participants from your sample into different groups using randomisation. With this method, every member of the sample has a known or equal chance of being placed in a control group or an experimental group.
A quasi-experiment is a type of research design that attempts to establish a cause-and-effect relationship. The main difference between this and a true experiment is that the groups are not randomly assigned.
In a between-subjects design , every participant experiences only one condition, and researchers assess group differences between participants in various conditions.
In a within-subjects design , each participant experiences all conditions, and researchers test the same participants repeatedly for differences between conditions.
The word ‘between’ means that you’re comparing different conditions between groups, while the word ‘within’ means you’re comparing different conditions within the same group.
A confounding variable , also called a confounder or confounding factor, is a third variable in a study examining a potential cause-and-effect relationship.
A confounding variable is related to both the supposed cause and the supposed effect of the study. It can be difficult to separate the true effect of the independent variable from the effect of the confounding variable.
In your research design , it’s important to identify potential confounding variables and plan how you will reduce their impact.
Triangulation can help:
- Reduce bias that comes from using a single method, theory, or investigator
- Enhance validity by approaching the same topic with different tools
- Establish credibility by giving you a complete picture of the research problem
But triangulation can also pose problems:
- It’s time-consuming and labour-intensive, often involving an interdisciplinary team.
- Your results may be inconsistent or even contradictory.
There are four main types of triangulation :
- Data triangulation : Using data from different times, spaces, and people
- Investigator triangulation : Involving multiple researchers in collecting or analysing data
- Theory triangulation : Using varying theoretical perspectives in your research
- Methodological triangulation : Using different methodologies to approach the same topic
Experimental designs are a set of procedures that you plan in order to examine the relationship between variables that interest you.
To design a successful experiment, first identify:
- A testable hypothesis
- One or more independent variables that you will manipulate
- One or more dependent variables that you will measure
When designing the experiment, first decide:
- How your variable(s) will be manipulated
- How you will control for any potential confounding or lurking variables
- How many subjects you will include
- How you will assign treatments to your subjects
Exploratory research explores the main aspects of a new or barely researched question.
Explanatory research explains the causes and effects of an already widely researched question.
The key difference between observational studies and experiments is that, done correctly, an observational study will never influence the responses or behaviours of participants. Experimental designs will have a treatment condition applied to at least a portion of participants.
An observational study could be a good fit for your research if your research question is based on things you observe. If you have ethical, logistical, or practical concerns that make an experimental design challenging, consider an observational study. Remember that in an observational study, it is critical that there be no interference or manipulation of the research subjects. Since it’s not an experiment, there are no control or treatment groups either.
These are four of the most common mixed methods designs :
- Convergent parallel: Quantitative and qualitative data are collected at the same time and analysed separately. After both analyses are complete, compare your results to draw overall conclusions.
- Embedded: Quantitative and qualitative data are collected at the same time, but within a larger quantitative or qualitative design. One type of data is secondary to the other.
- Explanatory sequential: Quantitative data is collected and analysed first, followed by qualitative data. You can use this design if you think your qualitative data will explain and contextualise your quantitative findings.
- Exploratory sequential: Qualitative data is collected and analysed first, followed by quantitative data. You can use this design if you think the quantitative data will confirm or validate your qualitative findings.
Triangulation in research means using multiple datasets, methods, theories and/or investigators to address a research question. It’s a research strategy that can help you enhance the validity and credibility of your findings.
Triangulation is mainly used in qualitative research , but it’s also commonly applied in quantitative research . Mixed methods research always uses triangulation.
Operationalisation means turning abstract conceptual ideas into measurable observations.
For example, the concept of social anxiety isn’t directly observable, but it can be operationally defined in terms of self-rating scores, behavioural avoidance of crowded places, or physical anxiety symptoms in social situations.
Before collecting data , it’s important to consider how you will operationalise the variables that you want to measure.
Hypothesis testing is a formal procedure for investigating our ideas about the world using statistics. It is used by scientists to test specific predictions, called hypotheses , by calculating how likely it is that a pattern or relationship between variables could have arisen by chance.
There are five common approaches to qualitative research :
- Grounded theory involves collecting data in order to develop new theories.
- Ethnography involves immersing yourself in a group or organisation to understand its culture.
- Narrative research involves interpreting stories to understand how people make sense of their experiences and perceptions.
- Phenomenological research involves investigating phenomena through people’s lived experiences.
- Action research links theory and practice in several cycles to drive innovative changes.
There are various approaches to qualitative data analysis , but they all share five steps in common:
- Prepare and organise your data.
- Review and explore your data.
- Develop a data coding system.
- Assign codes to the data.
- Identify recurring themes.
The specifics of each step depend on the focus of the analysis. Some common approaches include textual analysis , thematic analysis , and discourse analysis .
In mixed methods research , you use both qualitative and quantitative data collection and analysis methods to answer your research question .
Methodology refers to the overarching strategy and rationale of your research project . It involves studying the methods used in your field and the theories or principles behind them, in order to develop an approach that matches your objectives.
Methods are the specific tools and procedures you use to collect and analyse data (e.g. experiments, surveys , and statistical tests ).
In shorter scientific papers, where the aim is to report the findings of a specific study, you might simply describe what you did in a methods section .
In a longer or more complex research project, such as a thesis or dissertation , you will probably include a methodology section , where you explain your approach to answering the research questions and cite relevant sources to support your choice of methods.
The research methods you use depend on the type of data you need to answer your research question .
- If you want to measure something or test a hypothesis , use quantitative methods . If you want to explore ideas, thoughts, and meanings, use qualitative methods .
- If you want to analyse a large amount of readily available data, use secondary data. If you want data specific to your purposes with control over how they are generated, collect primary data.
- If you want to establish cause-and-effect relationships between variables , use experimental methods. If you want to understand the characteristics of a research subject, use descriptive methods.
Ask our team
Want to contact us directly? No problem. We are always here for you.
- Chat with us
- Email [email protected]
- Call +44 (0)20 3917 4242
- WhatsApp +31 20 261 6040
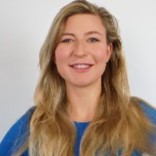
Our support team is here to help you daily via chat, WhatsApp, email, or phone between 9:00 a.m. to 11:00 p.m. CET.
Our APA experts default to APA 7 for editing and formatting. For the Citation Editing Service you are able to choose between APA 6 and 7.
Yes, if your document is longer than 20,000 words, you will get a sample of approximately 2,000 words. This sample edit gives you a first impression of the editor’s editing style and a chance to ask questions and give feedback.
How does the sample edit work?
You will receive the sample edit within 24 hours after placing your order. You then have 24 hours to let us know if you’re happy with the sample or if there’s something you would like the editor to do differently.
Read more about how the sample edit works
Yes, you can upload your document in sections.
We try our best to ensure that the same editor checks all the different sections of your document. When you upload a new file, our system recognizes you as a returning customer, and we immediately contact the editor who helped you before.
However, we cannot guarantee that the same editor will be available. Your chances are higher if
- You send us your text as soon as possible and
- You can be flexible about the deadline.
Please note that the shorter your deadline is, the lower the chance that your previous editor is not available.
If your previous editor isn’t available, then we will inform you immediately and look for another qualified editor. Fear not! Every Scribbr editor follows the Scribbr Improvement Model and will deliver high-quality work.
Yes, our editors also work during the weekends and holidays.
Because we have many editors available, we can check your document 24 hours per day and 7 days per week, all year round.
If you choose a 72 hour deadline and upload your document on a Thursday evening, you’ll have your thesis back by Sunday evening!
Yes! Our editors are all native speakers, and they have lots of experience editing texts written by ESL students. They will make sure your grammar is perfect and point out any sentences that are difficult to understand. They’ll also notice your most common mistakes, and give you personal feedback to improve your writing in English.
Every Scribbr order comes with our award-winning Proofreading & Editing service , which combines two important stages of the revision process.
For a more comprehensive edit, you can add a Structure Check or Clarity Check to your order. With these building blocks, you can customize the kind of feedback you receive.
You might be familiar with a different set of editing terms. To help you understand what you can expect at Scribbr, we created this table:
View an example
When you place an order, you can specify your field of study and we’ll match you with an editor who has familiarity with this area.
However, our editors are language specialists, not academic experts in your field. Your editor’s job is not to comment on the content of your dissertation, but to improve your language and help you express your ideas as clearly and fluently as possible.
This means that your editor will understand your text well enough to give feedback on its clarity, logic and structure, but not on the accuracy or originality of its content.
Good academic writing should be understandable to a non-expert reader, and we believe that academic editing is a discipline in itself. The research, ideas and arguments are all yours – we’re here to make sure they shine!
After your document has been edited, you will receive an email with a link to download the document.
The editor has made changes to your document using ‘Track Changes’ in Word. This means that you only have to accept or ignore the changes that are made in the text one by one.
It is also possible to accept all changes at once. However, we strongly advise you not to do so for the following reasons:
- You can learn a lot by looking at the mistakes you made.
- The editors don’t only change the text – they also place comments when sentences or sometimes even entire paragraphs are unclear. You should read through these comments and take into account your editor’s tips and suggestions.
- With a final read-through, you can make sure you’re 100% happy with your text before you submit!
You choose the turnaround time when ordering. We can return your dissertation within 24 hours , 3 days or 1 week . These timescales include weekends and holidays. As soon as you’ve paid, the deadline is set, and we guarantee to meet it! We’ll notify you by text and email when your editor has completed the job.
Very large orders might not be possible to complete in 24 hours. On average, our editors can complete around 13,000 words in a day while maintaining our high quality standards. If your order is longer than this and urgent, contact us to discuss possibilities.
Always leave yourself enough time to check through the document and accept the changes before your submission deadline.
Scribbr is specialised in editing study related documents. We check:
- Graduation projects
- Dissertations
- Admissions essays
- College essays
- Application essays
- Personal statements
- Process reports
- Reflections
- Internship reports
- Academic papers
- Research proposals
- Prospectuses
Calculate the costs
The fastest turnaround time is 24 hours.
You can upload your document at any time and choose between three deadlines:
At Scribbr, we promise to make every customer 100% happy with the service we offer. Our philosophy: Your complaint is always justified – no denial, no doubts.
Our customer support team is here to find the solution that helps you the most, whether that’s a free new edit or a refund for the service.
Yes, in the order process you can indicate your preference for American, British, or Australian English .
If you don’t choose one, your editor will follow the style of English you currently use. If your editor has any questions about this, we will contact you.
Definition of a Hypothesis
What it is and how it's used in sociology
- Key Concepts
- Major Sociologists
- News & Issues
- Research, Samples, and Statistics
- Recommended Reading
- Archaeology
A hypothesis is a prediction of what will be found at the outcome of a research project and is typically focused on the relationship between two different variables studied in the research. It is usually based on both theoretical expectations about how things work and already existing scientific evidence.
Within social science, a hypothesis can take two forms. It can predict that there is no relationship between two variables, in which case it is a null hypothesis . Or, it can predict the existence of a relationship between variables, which is known as an alternative hypothesis.
In either case, the variable that is thought to either affect or not affect the outcome is known as the independent variable, and the variable that is thought to either be affected or not is the dependent variable.
Researchers seek to determine whether or not their hypothesis, or hypotheses if they have more than one, will prove true. Sometimes they do, and sometimes they do not. Either way, the research is considered successful if one can conclude whether or not a hypothesis is true.
Null Hypothesis
A researcher has a null hypothesis when she or he believes, based on theory and existing scientific evidence, that there will not be a relationship between two variables. For example, when examining what factors influence a person's highest level of education within the U.S., a researcher might expect that place of birth, number of siblings, and religion would not have an impact on the level of education. This would mean the researcher has stated three null hypotheses.
Alternative Hypothesis
Taking the same example, a researcher might expect that the economic class and educational attainment of one's parents, and the race of the person in question are likely to have an effect on one's educational attainment. Existing evidence and social theories that recognize the connections between wealth and cultural resources , and how race affects access to rights and resources in the U.S. , would suggest that both economic class and educational attainment of the one's parents would have a positive effect on educational attainment. In this case, economic class and educational attainment of one's parents are independent variables, and one's educational attainment is the dependent variable—it is hypothesized to be dependent on the other two.
Conversely, an informed researcher would expect that being a race other than white in the U.S. is likely to have a negative impact on a person's educational attainment. This would be characterized as a negative relationship, wherein being a person of color has a negative effect on one's educational attainment. In reality, this hypothesis proves true, with the exception of Asian Americans , who go to college at a higher rate than whites do. However, Blacks and Hispanics and Latinos are far less likely than whites and Asian Americans to go to college.
Formulating a Hypothesis
Formulating a hypothesis can take place at the very beginning of a research project , or after a bit of research has already been done. Sometimes a researcher knows right from the start which variables she is interested in studying, and she may already have a hunch about their relationships. Other times, a researcher may have an interest in a particular topic, trend, or phenomenon, but he may not know enough about it to identify variables or formulate a hypothesis.
Whenever a hypothesis is formulated, the most important thing is to be precise about what one's variables are, what the nature of the relationship between them might be, and how one can go about conducting a study of them.
Updated by Nicki Lisa Cole, Ph.D
- What Is a Hypothesis? (Science)
- Understanding Path Analysis
- Null Hypothesis Examples
- What Are the Elements of a Good Hypothesis?
- What It Means When a Variable Is Spurious
- What 'Fail to Reject' Means in a Hypothesis Test
- How Intervening Variables Work in Sociology
- Null Hypothesis Definition and Examples
- Understanding Simple vs Controlled Experiments
- Scientific Method Vocabulary Terms
- Null Hypothesis and Alternative Hypothesis
- Six Steps of the Scientific Method
- What Are Examples of a Hypothesis?
- Structural Equation Modeling
- Scientific Method Flow Chart
- Lambda and Gamma as Defined in Sociology
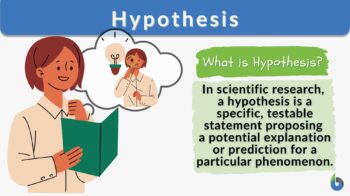
Hypothesis n., plural: hypotheses [/haɪˈpɑːθəsɪs/] Definition: Testable scientific prediction
Table of Contents
What Is Hypothesis?
A scientific hypothesis is a foundational element of the scientific method . It’s a testable statement proposing a potential explanation for natural phenomena. The term hypothesis means “little theory” . A hypothesis is a short statement that can be tested and gives a possible reason for a phenomenon or a possible link between two variables . In the setting of scientific research, a hypothesis is a tentative explanation or statement that can be proven wrong and is used to guide experiments and empirical research.
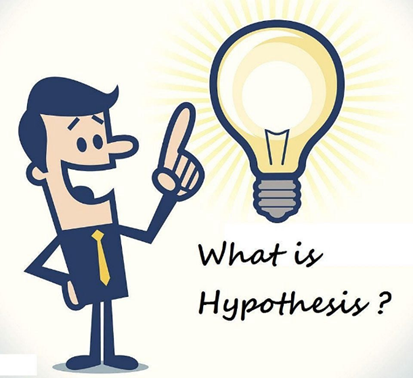
It is an important part of the scientific method because it gives a basis for planning tests, gathering data, and judging evidence to see if it is true and could help us understand how natural things work. Several hypotheses can be tested in the real world, and the results of careful and systematic observation and analysis can be used to support, reject, or improve them.
Researchers and scientists often use the word hypothesis to refer to this educated guess . These hypotheses are firmly established based on scientific principles and the rigorous testing of new technology and experiments .
For example, in astrophysics, the Big Bang Theory is a working hypothesis that explains the origins of the universe and considers it as a natural phenomenon. It is among the most prominent scientific hypotheses in the field.
“The scientific method: steps, terms, and examples” by Scishow:
Biology definition: A hypothesis is a supposition or tentative explanation for (a group of) phenomena, (a set of) facts, or a scientific inquiry that may be tested, verified or answered by further investigation or methodological experiment. It is like a scientific guess . It’s an idea or prediction that scientists make before they do experiments. They use it to guess what might happen and then test it to see if they were right. It’s like a smart guess that helps them learn new things. A scientific hypothesis that has been verified through scientific experiment and research may well be considered a scientific theory .
Etymology: The word “hypothesis” comes from the Greek word “hupothesis,” which means “a basis” or “a supposition.” It combines “hupo” (under) and “thesis” (placing). Synonym: proposition; assumption; conjecture; postulate Compare: theory See also: null hypothesis
Characteristics Of Hypothesis
A useful hypothesis must have the following qualities:
- It should never be written as a question.
- You should be able to test it in the real world to see if it’s right or wrong.
- It needs to be clear and exact.
- It should list the factors that will be used to figure out the relationship.
- It should only talk about one thing. You can make a theory in either a descriptive or form of relationship.
- It shouldn’t go against any natural rule that everyone knows is true. Verification will be done well with the tools and methods that are available.
- It should be written in as simple a way as possible so that everyone can understand it.
- It must explain what happened to make an answer necessary.
- It should be testable in a fair amount of time.
- It shouldn’t say different things.
Sources Of Hypothesis
Sources of hypothesis are:
- Patterns of similarity between the phenomenon under investigation and existing hypotheses.
- Insights derived from prior research, concurrent observations, and insights from opposing perspectives.
- The formulations are derived from accepted scientific theories and proposed by researchers.
- In research, it’s essential to consider hypothesis as different subject areas may require various hypotheses (plural form of hypothesis). Researchers also establish a significance level to determine the strength of evidence supporting a hypothesis.
- Individual cognitive processes also contribute to the formation of hypotheses.
One hypothesis is a tentative explanation for an observation or phenomenon. It is based on prior knowledge and understanding of the world, and it can be tested by gathering and analyzing data. Observed facts are the data that are collected to test a hypothesis. They can support or refute the hypothesis.
For example, the hypothesis that “eating more fruits and vegetables will improve your health” can be tested by gathering data on the health of people who eat different amounts of fruits and vegetables. If the people who eat more fruits and vegetables are healthier than those who eat less fruits and vegetables, then the hypothesis is supported.
Hypotheses are essential for scientific inquiry. They help scientists to focus their research, to design experiments, and to interpret their results. They are also essential for the development of scientific theories.
Types Of Hypothesis
In research, you typically encounter two types of hypothesis: the alternative hypothesis (which proposes a relationship between variables) and the null hypothesis (which suggests no relationship).
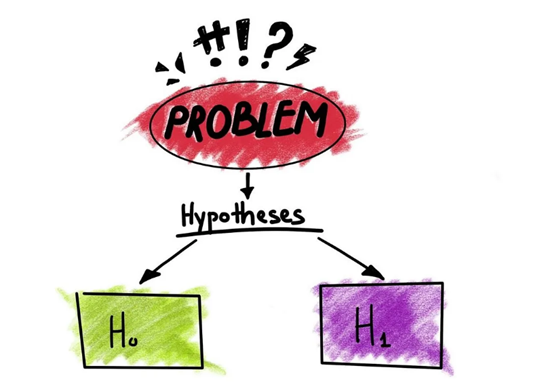
Simple Hypothesis
It illustrates the association between one dependent variable and one independent variable. For instance, if you consume more vegetables, you will lose weight more quickly. Here, increasing vegetable consumption is the independent variable, while weight loss is the dependent variable.
Complex Hypothesis
It exhibits the relationship between at least two dependent variables and at least two independent variables. Eating more vegetables and fruits results in weight loss, radiant skin, and a decreased risk of numerous diseases, including heart disease.
Directional Hypothesis
It shows that a researcher wants to reach a certain goal. The way the factors are related can also tell us about their nature. For example, four-year-old children who eat well over a time of five years have a higher IQ than children who don’t eat well. This shows what happened and how it happened.
Non-directional Hypothesis
When there is no theory involved, it is used. It is a statement that there is a connection between two variables, but it doesn’t say what that relationship is or which way it goes.
Null Hypothesis
It says something that goes against the theory. It’s a statement that says something is not true, and there is no link between the independent and dependent factors. “H 0 ” represents the null hypothesis.
Associative and Causal Hypothesis
When a change in one variable causes a change in the other variable, this is called the associative hypothesis . The causal hypothesis, on the other hand, says that there is a cause-and-effect relationship between two or more factors.
Examples Of Hypothesis
Examples of simple hypotheses:
- Students who consume breakfast before taking a math test will have a better overall performance than students who do not consume breakfast.
- Students who experience test anxiety before an English examination will get lower scores than students who do not experience test anxiety.
- Motorists who talk on the phone while driving will be more likely to make errors on a driving course than those who do not talk on the phone, is a statement that suggests that drivers who talk on the phone while driving are more likely to make mistakes.
Examples of a complex hypothesis:
- Individuals who consume a lot of sugar and don’t get much exercise are at an increased risk of developing depression.
- Younger people who are routinely exposed to green, outdoor areas have better subjective well-being than older adults who have limited exposure to green spaces, according to a new study.
- Increased levels of air pollution led to higher rates of respiratory illnesses, which in turn resulted in increased costs for healthcare for the affected communities.
Examples of Directional Hypothesis:
- The crop yield will go up a lot if the amount of fertilizer is increased.
- Patients who have surgery and are exposed to more stress will need more time to get better.
- Increasing the frequency of brand advertising on social media will lead to a significant increase in brand awareness among the target audience.
Examples of Non-Directional Hypothesis (or Two-Tailed Hypothesis):
- The test scores of two groups of students are very different from each other.
- There is a link between gender and being happy at work.
- There is a correlation between the amount of caffeine an individual consumes and the speed with which they react.
Examples of a null hypothesis:
- Children who receive a new reading intervention will have scores that are different than students who do not receive the intervention.
- The results of a memory recall test will not reveal any significant gap in performance between children and adults.
- There is not a significant relationship between the number of hours spent playing video games and academic performance.
Examples of Associative Hypothesis:
- There is a link between how many hours you spend studying and how well you do in school.
- Drinking sugary drinks is bad for your health as a whole.
- There is an association between socioeconomic status and access to quality healthcare services in urban neighborhoods.
Functions Of Hypothesis
The research issue can be understood better with the help of a hypothesis, which is why developing one is crucial. The following are some of the specific roles that a hypothesis plays: (Rashid, Apr 20, 2022)
- A hypothesis gives a study a point of concentration. It enlightens us as to the specific characteristics of a study subject we need to look into.
- It instructs us on what data to acquire as well as what data we should not collect, giving the study a focal point .
- The development of a hypothesis improves objectivity since it enables the establishment of a focal point.
- A hypothesis makes it possible for us to contribute to the development of the theory. Because of this, we are in a position to definitively determine what is true and what is untrue .
How will Hypothesis help in the Scientific Method?
- The scientific method begins with observation and inquiry about the natural world when formulating research questions. Researchers can refine their observations and queries into specific, testable research questions with the aid of hypothesis. They provide an investigation with a focused starting point.
- Hypothesis generate specific predictions regarding the expected outcomes of experiments or observations. These forecasts are founded on the researcher’s current knowledge of the subject. They elucidate what researchers anticipate observing if the hypothesis is true.
- Hypothesis direct the design of experiments and data collection techniques. Researchers can use them to determine which variables to measure or manipulate, which data to obtain, and how to conduct systematic and controlled research.
- Following the formulation of a hypothesis and the design of an experiment, researchers collect data through observation, measurement, or experimentation. The collected data is used to verify the hypothesis’s predictions.
- Hypothesis establish the criteria for evaluating experiment results. The observed data are compared to the predictions generated by the hypothesis. This analysis helps determine whether empirical evidence supports or refutes the hypothesis.
- The results of experiments or observations are used to derive conclusions regarding the hypothesis. If the data support the predictions, then the hypothesis is supported. If this is not the case, the hypothesis may be revised or rejected, leading to the formulation of new queries and hypothesis.
- The scientific approach is iterative, resulting in new hypothesis and research issues from previous trials. This cycle of hypothesis generation, testing, and refining drives scientific progress.
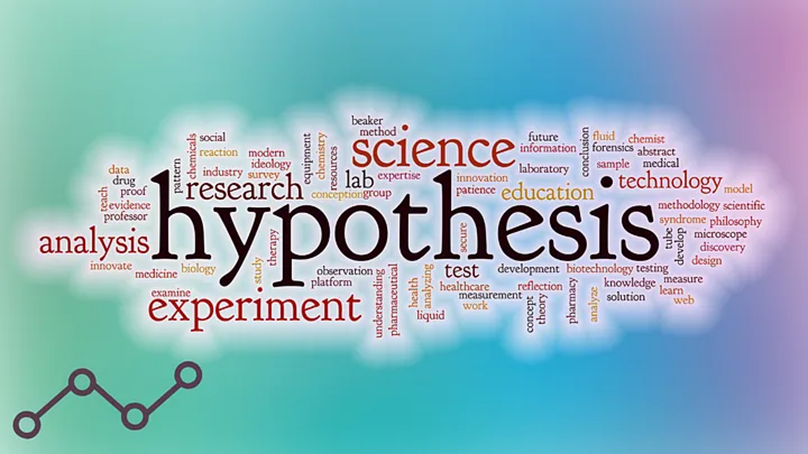
Importance Of Hypothesis
- Hypothesis are testable statements that enable scientists to determine if their predictions are accurate. This assessment is essential to the scientific method, which is based on empirical evidence.
- Hypothesis serve as the foundation for designing experiments or data collection techniques. They can be used by researchers to develop protocols and procedures that will produce meaningful results.
- Hypothesis hold scientists accountable for their assertions. They establish expectations for what the research should reveal and enable others to assess the validity of the findings.
- Hypothesis aid in identifying the most important variables of a study. The variables can then be measured, manipulated, or analyzed to determine their relationships.
- Hypothesis assist researchers in allocating their resources efficiently. They ensure that time, money, and effort are spent investigating specific concerns, as opposed to exploring random concepts.
- Testing hypothesis contribute to the scientific body of knowledge. Whether or not a hypothesis is supported, the results contribute to our understanding of a phenomenon.
- Hypothesis can result in the creation of theories. When supported by substantive evidence, hypothesis can serve as the foundation for larger theoretical frameworks that explain complex phenomena.
- Beyond scientific research, hypothesis play a role in the solution of problems in a variety of domains. They enable professionals to make educated assumptions about the causes of problems and to devise solutions.
Research Hypotheses: Did you know that a hypothesis refers to an educated guess or prediction about the outcome of a research study?
It’s like a roadmap guiding researchers towards their destination of knowledge. Just like a compass points north, a well-crafted hypothesis points the way to valuable discoveries in the world of science and inquiry.
Choose the best answer.
Send Your Results (Optional)
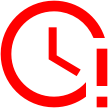
Further Reading
- RNA-DNA World Hypothesis
- BYJU’S. (2023). Hypothesis. Retrieved 01 Septermber 2023, from https://byjus.com/physics/hypothesis/#sources-of-hypothesis
- Collegedunia. (2023). Hypothesis. Retrieved 1 September 2023, from https://collegedunia.com/exams/hypothesis-science-articleid-7026#d
- Hussain, D. J. (2022). Hypothesis. Retrieved 01 September 2023, from https://mmhapu.ac.in/doc/eContent/Management/JamesHusain/Research%20Hypothesis%20-Meaning,%20Nature%20&%20Importance-Characteristics%20of%20Good%20%20Hypothesis%20Sem2.pdf
- Media, D. (2023). Hypothesis in the Scientific Method. Retrieved 01 September 2023, from https://www.verywellmind.com/what-is-a-hypothesis-2795239#toc-hypotheses-examples
- Rashid, M. H. A. (Apr 20, 2022). Research Methodology. Retrieved 01 September 2023, from https://limbd.org/hypothesis-definitions-functions-characteristics-types-errors-the-process-of-testing-a-hypothesis-hypotheses-in-qualitative-research/#:~:text=Functions%20of%20a%20Hypothesis%3A&text=Specifically%2C%20a%20hypothesis%20serves%20the,providing%20focus%20to%20the%20study.
©BiologyOnline.com. Content provided and moderated by Biology Online Editors.
Last updated on September 8th, 2023
You will also like...
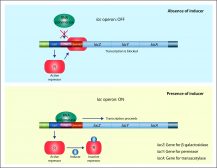
Gene Action – Operon Hypothesis
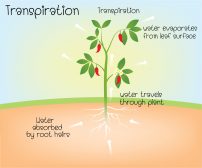
Water in Plants
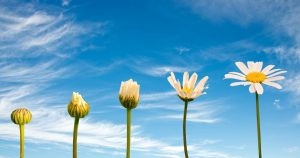
Growth and Plant Hormones
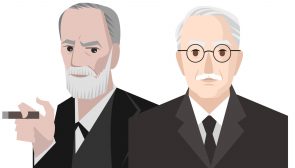
Sigmund Freud and Carl Gustav Jung
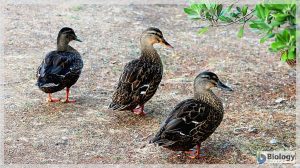
Population Growth and Survivorship
Related articles....
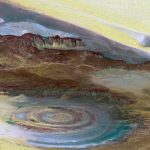
RNA-DNA World Hypothesis?
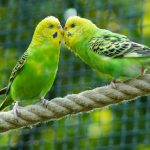
On Mate Selection Evolution: Are intelligent males more attractive?
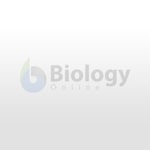
Actions of Caffeine in the Brain with Special Reference to Factors That Contribute to Its Widespread Use
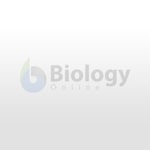
Dead Man Walking
Get science-backed answers as you write with Paperpal's Research feature
How to Write a Hypothesis? Types and Examples
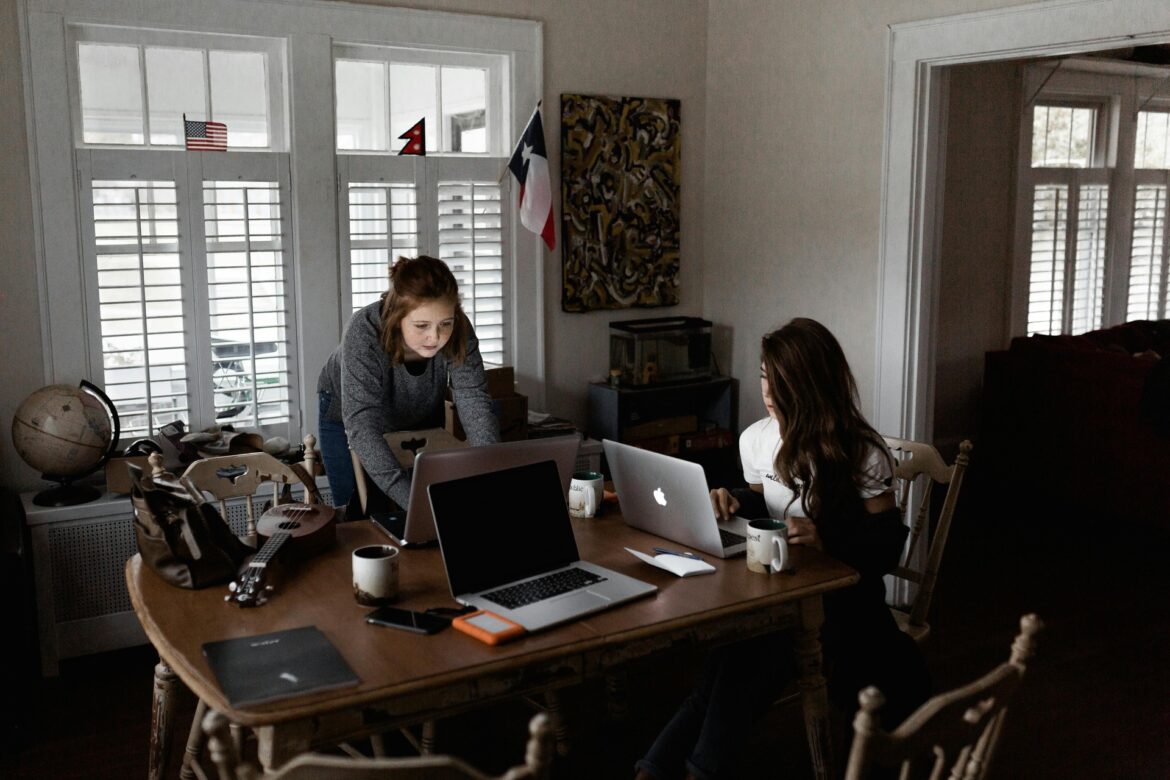
All research studies involve the use of the scientific method, which is a mathematical and experimental technique used to conduct experiments by developing and testing a hypothesis or a prediction about an outcome. Simply put, a hypothesis is a suggested solution to a problem. It includes elements that are expressed in terms of relationships with each other to explain a condition or an assumption that hasn’t been verified using facts. 1 The typical steps in a scientific method include developing such a hypothesis, testing it through various methods, and then modifying it based on the outcomes of the experiments.
A research hypothesis can be defined as a specific, testable prediction about the anticipated results of a study. 2 Hypotheses help guide the research process and supplement the aim of the study. After several rounds of testing, hypotheses can help develop scientific theories. 3 Hypotheses are often written as if-then statements.
Here are two hypothesis examples:
Dandelions growing in nitrogen-rich soils for two weeks develop larger leaves than those in nitrogen-poor soils because nitrogen stimulates vegetative growth. 4
If a company offers flexible work hours, then their employees will be happier at work. 5
Table of Contents
- What is a hypothesis?
- Types of hypotheses
- Characteristics of a hypothesis
- Functions of a hypothesis
- How to write a hypothesis
- Hypothesis examples
- Frequently asked questions
What is a hypothesis?
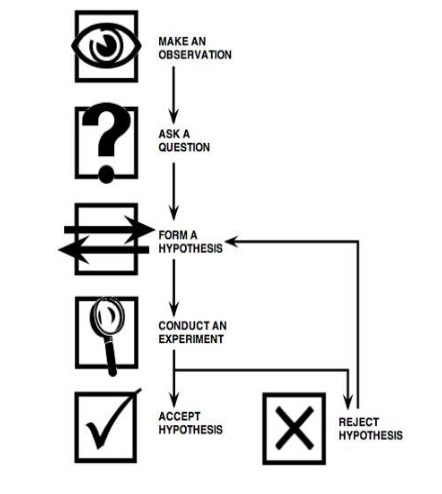
A hypothesis expresses an expected relationship between variables in a study and is developed before conducting any research. Hypotheses are not opinions but rather are expected relationships based on facts and observations. They help support scientific research and expand existing knowledge. An incorrectly formulated hypothesis can affect the entire experiment leading to errors in the results so it’s important to know how to formulate a hypothesis and develop it carefully.
A few sources of a hypothesis include observations from prior studies, current research and experiences, competitors, scientific theories, and general conditions that can influence people. Figure 1 depicts the different steps in a research design and shows where exactly in the process a hypothesis is developed. 4
There are seven different types of hypotheses—simple, complex, directional, nondirectional, associative and causal, null, and alternative.
Types of hypotheses
The seven types of hypotheses are listed below: 5 , 6,7
- Simple : Predicts the relationship between a single dependent variable and a single independent variable.
Example: Exercising in the morning every day will increase your productivity.
- Complex : Predicts the relationship between two or more variables.
Example: Spending three hours or more on social media daily will negatively affect children’s mental health and productivity, more than that of adults.
- Directional : Specifies the expected direction to be followed and uses terms like increase, decrease, positive, negative, more, or less.
Example: The inclusion of intervention X decreases infant mortality compared to the original treatment.
- Non-directional : Does not predict the exact direction, nature, or magnitude of the relationship between two variables but rather states the existence of a relationship. This hypothesis may be used when there is no underlying theory or if findings contradict prior research.
Example: Cats and dogs differ in the amount of affection they express.
- Associative and causal : An associative hypothesis suggests an interdependency between variables, that is, how a change in one variable changes the other.
Example: There is a positive association between physical activity levels and overall health.
A causal hypothesis, on the other hand, expresses a cause-and-effect association between variables.
Example: Long-term alcohol use causes liver damage.
- Null : Claims that the original hypothesis is false by showing that there is no relationship between the variables.
Example: Sleep duration does not have any effect on productivity.
- Alternative : States the opposite of the null hypothesis, that is, a relationship exists between two variables.
Example: Sleep duration affects productivity.
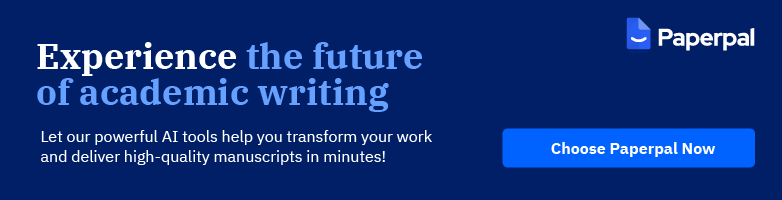
Characteristics of a hypothesis
So, what makes a good hypothesis? Here are some important characteristics of a hypothesis. 8,9
- Testable : You must be able to test the hypothesis using scientific methods to either accept or reject the prediction.
- Falsifiable : It should be possible to collect data that reject rather than support the hypothesis.
- Logical : Hypotheses shouldn’t be a random guess but rather should be based on previous theories, observations, prior research, and logical reasoning.
- Positive : The hypothesis statement about the existence of an association should be positive, that is, it should not suggest that an association does not exist. Therefore, the language used and knowing how to phrase a hypothesis is very important.
- Clear and accurate : The language used should be easily comprehensible and use correct terminology.
- Relevant : The hypothesis should be relevant and specific to the research question.
- Structure : Should include all the elements that make a good hypothesis: variables, relationship, and outcome.
Functions of a hypothesis
The following list mentions some important functions of a hypothesis: 1
- Maintains the direction and progress of the research.
- Expresses the important assumptions underlying the proposition in a single statement.
- Establishes a suitable context for researchers to begin their investigation and for readers who are referring to the final report.
- Provides an explanation for the occurrence of a specific phenomenon.
- Ensures selection of appropriate and accurate facts necessary and relevant to the research subject.
To summarize, a hypothesis provides the conceptual elements that complete the known data, conceptual relationships that systematize unordered elements, and conceptual meanings and interpretations that explain the unknown phenomena. 1
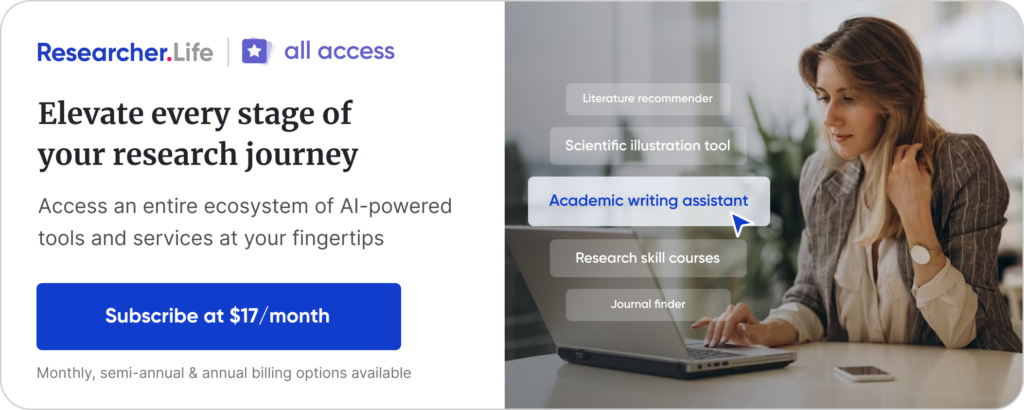
How to write a hypothesis
Listed below are the main steps explaining how to write a hypothesis. 2,4,5
- Make an observation and identify variables : Observe the subject in question and try to recognize a pattern or a relationship between the variables involved. This step provides essential background information to begin your research.
For example, if you notice that an office’s vending machine frequently runs out of a specific snack, you may predict that more people in the office choose that snack over another.
- Identify the main research question : After identifying a subject and recognizing a pattern, the next step is to ask a question that your hypothesis will answer.
For example, after observing employees’ break times at work, you could ask “why do more employees take breaks in the morning rather than in the afternoon?”
- Conduct some preliminary research to ensure originality and novelty : Your initial answer, which is your hypothesis, to the question is based on some pre-existing information about the subject. However, to ensure that your hypothesis has not been asked before or that it has been asked but rejected by other researchers you would need to gather additional information.
For example, based on your observations you might state a hypothesis that employees work more efficiently when the air conditioning in the office is set at a lower temperature. However, during your preliminary research you find that this hypothesis was proven incorrect by a prior study.
- Develop a general statement : After your preliminary research has confirmed the originality of your proposed answer, draft a general statement that includes all variables, subjects, and predicted outcome. The statement could be if/then or declarative.
- Finalize the hypothesis statement : Use the PICOT model, which clarifies how to word a hypothesis effectively, when finalizing the statement. This model lists the important components required to write a hypothesis.
P opulation: The specific group or individual who is the main subject of the research
I nterest: The main concern of the study/research question
C omparison: The main alternative group
O utcome: The expected results
T ime: Duration of the experiment
Once you’ve finalized your hypothesis statement you would need to conduct experiments to test whether the hypothesis is true or false.
Hypothesis examples
The following table provides examples of different types of hypotheses. 10 ,11
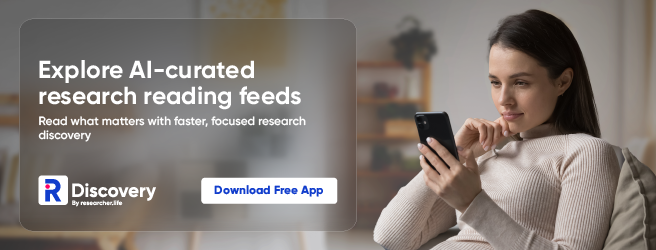
Key takeaways
Here’s a summary of all the key points discussed in this article about how to write a hypothesis.
- A hypothesis is an assumption about an association between variables made based on limited evidence, which should be tested.
- A hypothesis has four parts—the research question, independent variable, dependent variable, and the proposed relationship between the variables.
- The statement should be clear, concise, testable, logical, and falsifiable.
- There are seven types of hypotheses—simple, complex, directional, non-directional, associative and causal, null, and alternative.
- A hypothesis provides a focus and direction for the research to progress.
- A hypothesis plays an important role in the scientific method by helping to create an appropriate experimental design.
Frequently asked questions
Hypotheses and research questions have different objectives and structure. The following table lists some major differences between the two. 9
Here are a few examples to differentiate between a research question and hypothesis.
Yes, here’s a simple checklist to help you gauge the effectiveness of your hypothesis. 9 1. When writing a hypothesis statement, check if it: 2. Predicts the relationship between the stated variables and the expected outcome. 3. Uses simple and concise language and is not wordy. 4. Does not assume readers’ knowledge about the subject. 5. Has observable, falsifiable, and testable results.
As mentioned earlier in this article, a hypothesis is an assumption or prediction about an association between variables based on observations and simple evidence. These statements are usually generic. Research objectives, on the other hand, are more specific and dictated by hypotheses. The same hypothesis can be tested using different methods and the research objectives could be different in each case. For example, Louis Pasteur observed that food lasts longer at higher altitudes, reasoned that it could be because the air at higher altitudes is cleaner (with fewer or no germs), and tested the hypothesis by exposing food to air cleaned in the laboratory. 12 Thus, a hypothesis is predictive—if the reasoning is correct, X will lead to Y—and research objectives are developed to test these predictions.
Null hypothesis testing is a method to decide between two assumptions or predictions between variables (null and alternative hypotheses) in a statistical relationship in a sample. The null hypothesis, denoted as H 0 , claims that no relationship exists between variables in a population and any relationship in the sample reflects a sampling error or occurrence by chance. The alternative hypothesis, denoted as H 1 , claims that there is a relationship in the population. In every study, researchers need to decide whether the relationship in a sample occurred by chance or reflects a relationship in the population. This is done by hypothesis testing using the following steps: 13 1. Assume that the null hypothesis is true. 2. Determine how likely the sample relationship would be if the null hypothesis were true. This probability is called the p value. 3. If the sample relationship would be extremely unlikely, reject the null hypothesis and accept the alternative hypothesis. If the relationship would not be unlikely, accept the null hypothesis.
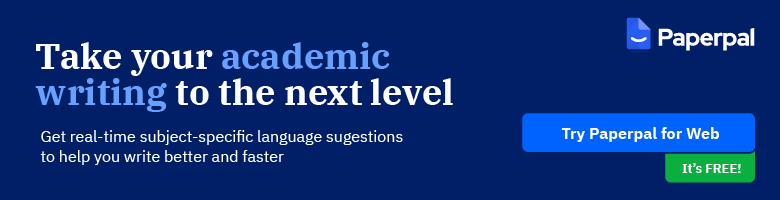
To summarize, researchers should know how to write a good hypothesis to ensure that their research progresses in the required direction. A hypothesis is a testable prediction about any behavior or relationship between variables, usually based on facts and observation, and states an expected outcome.
We hope this article has provided you with essential insight into the different types of hypotheses and their functions so that you can use them appropriately in your next research project.
References
- Dalen, DVV. The function of hypotheses in research. Proquest website. Accessed April 8, 2024. https://www.proquest.com/docview/1437933010?pq-origsite=gscholar&fromopenview=true&sourcetype=Scholarly%20Journals&imgSeq=1
- McLeod S. Research hypothesis in psychology: Types & examples. SimplyPsychology website. Updated December 13, 2023. Accessed April 9, 2024. https://www.simplypsychology.org/what-is-a-hypotheses.html
- Scientific method. Britannica website. Updated March 14, 2024. Accessed April 9, 2024. https://www.britannica.com/science/scientific-method
- The hypothesis in science writing. Accessed April 10, 2024. https://berks.psu.edu/sites/berks/files/campus/HypothesisHandout_Final.pdf
- How to develop a hypothesis (with elements, types, and examples). Indeed.com website. Updated February 3, 2023. Accessed April 10, 2024. https://www.indeed.com/career-advice/career-development/how-to-write-a-hypothesis
- Types of research hypotheses. Excelsior online writing lab. Accessed April 11, 2024. https://owl.excelsior.edu/research/research-hypotheses/types-of-research-hypotheses/
- What is a research hypothesis: how to write it, types, and examples. Researcher.life website. Published February 8, 2023. Accessed April 11, 2024. https://researcher.life/blog/article/how-to-write-a-research-hypothesis-definition-types-examples/
- Developing a hypothesis. Pressbooks website. Accessed April 12, 2024. https://opentext.wsu.edu/carriecuttler/chapter/developing-a-hypothesis/
- What is and how to write a good hypothesis in research. Elsevier author services website. Accessed April 12, 2024. https://scientific-publishing.webshop.elsevier.com/manuscript-preparation/what-how-write-good-hypothesis-research/
- How to write a great hypothesis. Verywellmind website. Updated March 12, 2023. Accessed April 13, 2024. https://www.verywellmind.com/what-is-a-hypothesis-2795239
- 15 Hypothesis examples. Helpfulprofessor.com Published September 8, 2023. Accessed March 14, 2024. https://helpfulprofessor.com/hypothesis-examples/
- Editage insights. What is the interconnectivity between research objectives and hypothesis? Published February 24, 2021. Accessed April 13, 2024. https://www.editage.com/insights/what-is-the-interconnectivity-between-research-objectives-and-hypothesis
- Understanding null hypothesis testing. BCCampus open publishing. Accessed April 16, 2024. https://opentextbc.ca/researchmethods/chapter/understanding-null-hypothesis-testing/#:~:text=In%20null%20hypothesis%20testing%2C%20this,said%20to%20be%20statistically%20significant
Paperpal is a comprehensive AI writing toolkit that helps students and researchers achieve 2x the writing in half the time. It leverages 21+ years of STM experience and insights from millions of research articles to provide in-depth academic writing, language editing, and submission readiness support to help you write better, faster.
Get accurate academic translations, rewriting support, grammar checks, vocabulary suggestions, and generative AI assistance that delivers human precision at machine speed. Try for free or upgrade to Paperpal Prime starting at US$19 a month to access premium features, including consistency, plagiarism, and 30+ submission readiness checks to help you succeed.
Experience the future of academic writing – Sign up to Paperpal and start writing for free!
Related Reads:
- Empirical Research: A Comprehensive Guide for Academics
- How to Write a Scientific Paper in 10 Steps
- What is a Literature Review? How to Write It (with Examples)
- What are Journal Guidelines on Using Generative AI Tools
Measuring Academic Success: Definition & Strategies for Excellence
What are scholarly sources and where can you find them , you may also like, 4 ways paperpal encourages responsible writing with ai, what are scholarly sources and where can you..., what is academic writing: tips for students, why traditional editorial process needs an upgrade, paperpal’s new ai research finder empowers authors to..., what is hedging in academic writing , how to use ai to enhance your college..., ai + human expertise – a paradigm shift..., how to use paperpal to generate emails &....
- More from M-W
- To save this word, you'll need to log in. Log In
Definition of hypothesis
Did you know.
The Difference Between Hypothesis and Theory
A hypothesis is an assumption, an idea that is proposed for the sake of argument so that it can be tested to see if it might be true.
In the scientific method, the hypothesis is constructed before any applicable research has been done, apart from a basic background review. You ask a question, read up on what has been studied before, and then form a hypothesis.
A hypothesis is usually tentative; it's an assumption or suggestion made strictly for the objective of being tested.
A theory , in contrast, is a principle that has been formed as an attempt to explain things that have already been substantiated by data. It is used in the names of a number of principles accepted in the scientific community, such as the Big Bang Theory . Because of the rigors of experimentation and control, it is understood to be more likely to be true than a hypothesis is.
In non-scientific use, however, hypothesis and theory are often used interchangeably to mean simply an idea, speculation, or hunch, with theory being the more common choice.
Since this casual use does away with the distinctions upheld by the scientific community, hypothesis and theory are prone to being wrongly interpreted even when they are encountered in scientific contexts—or at least, contexts that allude to scientific study without making the critical distinction that scientists employ when weighing hypotheses and theories.
The most common occurrence is when theory is interpreted—and sometimes even gleefully seized upon—to mean something having less truth value than other scientific principles. (The word law applies to principles so firmly established that they are almost never questioned, such as the law of gravity.)
This mistake is one of projection: since we use theory in general to mean something lightly speculated, then it's implied that scientists must be talking about the same level of uncertainty when they use theory to refer to their well-tested and reasoned principles.
The distinction has come to the forefront particularly on occasions when the content of science curricula in schools has been challenged—notably, when a school board in Georgia put stickers on textbooks stating that evolution was "a theory, not a fact, regarding the origin of living things." As Kenneth R. Miller, a cell biologist at Brown University, has said , a theory "doesn’t mean a hunch or a guess. A theory is a system of explanations that ties together a whole bunch of facts. It not only explains those facts, but predicts what you ought to find from other observations and experiments.”
While theories are never completely infallible, they form the basis of scientific reasoning because, as Miller said "to the best of our ability, we’ve tested them, and they’ve held up."
- proposition
- supposition
hypothesis , theory , law mean a formula derived by inference from scientific data that explains a principle operating in nature.
hypothesis implies insufficient evidence to provide more than a tentative explanation.
theory implies a greater range of evidence and greater likelihood of truth.
law implies a statement of order and relation in nature that has been found to be invariable under the same conditions.
Examples of hypothesis in a Sentence
These examples are programmatically compiled from various online sources to illustrate current usage of the word 'hypothesis.' Any opinions expressed in the examples do not represent those of Merriam-Webster or its editors. Send us feedback about these examples.
Word History
Greek, from hypotithenai to put under, suppose, from hypo- + tithenai to put — more at do
1641, in the meaning defined at sense 1a
Phrases Containing hypothesis
- counter - hypothesis
- nebular hypothesis
- null hypothesis
- planetesimal hypothesis
- Whorfian hypothesis
Articles Related to hypothesis
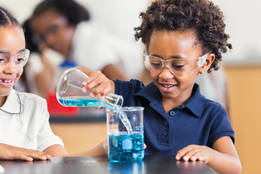
This is the Difference Between a...
This is the Difference Between a Hypothesis and a Theory
In scientific reasoning, they're two completely different things
Dictionary Entries Near hypothesis
hypothermia
hypothesize
Cite this Entry
“Hypothesis.” Merriam-Webster.com Dictionary , Merriam-Webster, https://www.merriam-webster.com/dictionary/hypothesis. Accessed 6 May. 2024.
Kids Definition
Kids definition of hypothesis, medical definition, medical definition of hypothesis, more from merriam-webster on hypothesis.
Nglish: Translation of hypothesis for Spanish Speakers
Britannica English: Translation of hypothesis for Arabic Speakers
Britannica.com: Encyclopedia article about hypothesis
Subscribe to America's largest dictionary and get thousands more definitions and advanced search—ad free!
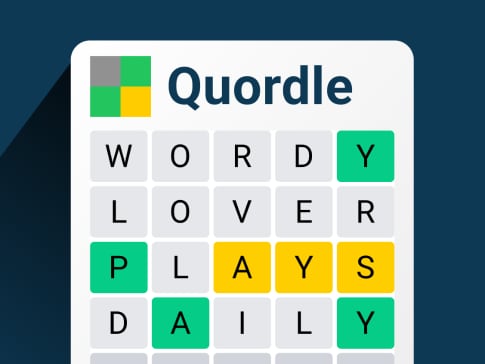
Can you solve 4 words at once?
Word of the day.
See Definitions and Examples »
Get Word of the Day daily email!
Popular in Grammar & Usage
More commonly misspelled words, your vs. you're: how to use them correctly, every letter is silent, sometimes: a-z list of examples, more commonly mispronounced words, how to use em dashes (—), en dashes (–) , and hyphens (-), popular in wordplay, 12 star wars words, the words of the week - may 3, a great big list of bread words, 10 scrabble words without any vowels, 8 uncommon words related to love, games & quizzes.
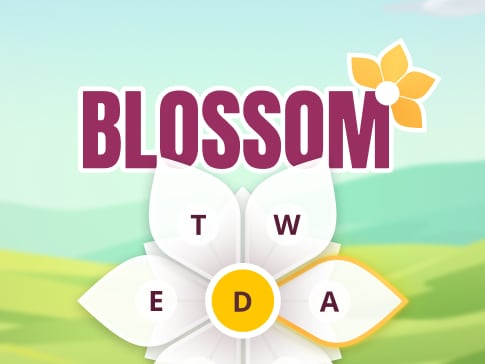
How to Improve the Explanatory Power of an Intelligent Textbook: a Case Study in Legal Writing
- Open access
- Published: 06 May 2024
Cite this article
You have full access to this open access article
- Francesco Sovrano ORCID: orcid.org/0000-0002-6285-1041 1 , 2 ,
- Kevin Ashley 3 ,
- Peter Leonid Brusilovsky 4 &
- Fabio Vitali 1
Explanatory processes are at the core of scientific investigation, legal reasoning, and education. However, effectively explaining complex or large amounts of information, such as that contained in a textbook or library, in an intuitive, user-centered way is still an open challenge. Indeed, different people may search for and request different types of information, even though texts typically have a predefined exposition and content. With this paper, we investigate how explanatory AI can better exploit the full potential of the vast and rich content library at our disposal. Based on a recent theory of explanations from Ordinary Language Philosophy, which frames the explanation process as illocutionary question-answering, we have developed a new type of interactive and adaptive textbook. Using the latest question-answering technology, our e-book software (YAI4Edu, for short) generates on-demand, expandable explanations that can help readers effectively explore teaching materials in a pedagogically productive way. It does this by extracting a specialized knowledge graph from a collection of books or other resources that helps identify the most relevant questions to be answered for a satisfactory explanation. We tested our technology with excerpts from a textbook that teaches how to write legal memoranda in the U.S. legal system. Then, to see whether YAI4Edu-enhanced textbooks are better than random and existing, general-purpose explanatory tools, we conducted a within-subjects user study with more than 100 English-speaking students. The students rated YAI4Edu’s explanations the highest. According to the students, the explanatory content generated by YAI4Edu is, on average, statistically better than two baseline alternatives ( P values below .005).
Avoid common mistakes on your manuscript.
Introduction
As pointed out by UNESCO, the United Nations specialized agency for education, in one of its recent publications (Miao et al., 2021 ), the opportunities and challenges that Artificial Intelligence (AI) offers for education in the AI era are yet to be fully understood. Nonetheless, the expectations are high given the potential of AI to foster our ability to acquire and convey knowledge. Indeed, since the invention of writing, we have collected a vast amount of written content that now forms the basis of our collective wisdom and industriousness.
However, fully harnessing the potential of such written knowledge by automatically explaining it in an intuitive and user-centered way is still an open problem . For instance, different explainees Footnote 1 may search for and request different types of information, even though, usually, the exposition and content of an explanatory document (e.g., a book, an article, a web page, or technical documentation) are predetermined and static.
Different books on the same subject expound the same knowledge in different ways and with different levels of detail. Additionally, this type of static representation is sub-optimal and time-consuming in the most generic scenario because helpful information may be sparse and scattered over hundreds or thousands of pages. As a result, explaining to a person can be an extremely challenging task, regardless of whether the explanandum Footnote 2 is from technical documentation, a scientific article, a regulation or a textbook. Additionally, the complexity of this task is increased by the elusiveness of the notion of explanation.
To address the problem of automatically explaining, we study how to automatically enhance static educational books by making them more interactive. We do this by reducing the sparsity of relevant information, thereby increasing the explanatory power of the medium while also linking it to a knowledge graph extracted from a collection of supplementary materials.
The approach is based on a recent theory from Ordinary Language Philosophy (Sovrano & Vitali, 2022a , explained in section “ Explanations According to Ordinary Language Philosophy ”), which views explaining as more than just providing answers to questions. It emphasizes that explaining involves answering unspoken or implicit questions in a meaningful and relevant way to the individual seeking understanding.
For instance, if you ask “How are you doing?” and the response is “I am fine”, this is not an explanation. However, if the response is “I am okay because I was worried I could have tested positive for COVID-19, but I am not, and [...]”, it becomes an explanation. The reason: this response intends to create an understanding of the person’s state of being.
This concept of responding to implicit questions is called illocution (Sovrano & Vitali, 2022a ). It makes the explanation process more focused on the individual seeking understanding. By anticipating these implicit questions, both the person explaining and the person seeking understanding can communicate more efficiently, resulting in a reduction of steps required to explain something Footnote 3 .
Hence, assuming that the goal of an educational e-book is to explain something to the reader and consistent with the abovementioned definition of explanations (Sovrano & Vitali, 2022a , b ), we built an explanatory process capable, through question-answering, of organizing a textbook’s explanatory space Footnote 4 to enable a reader to more efficiently and effectively retrieve helpful information. Specifically, our proposed solution consists of a pipeline of Explanatory AI (YAI) algorithms and heuristics to build, on top of textbooks, intelligent interfaces for:
answering explicit questions asked by a user;
automatically identifying the topics that can be explained by the contents of a textbook (or any collection of texts);
generating explanations by anticipating a set of useful (implicit) questions a user might have about the textbook.
Assuming that the content of a textbook (or a collection of texts integrating it) properly explains a given explanandum, our work relies on the following hypothesis:
Hypothesis 1
The most useful implicit questions a user may have about a collection of texts are those best answered in the collection as a whole. These questions are neither too detailed (because they would otherwise only be answered in a minor part of the collection) nor too general (because they would be answered inaccurately in the more detailed textual content).
If the assumption mentioned above holds, we believe that the hypothesis is correct for the following reasons:
any question falling outside the scope of the collection of documents could not be answered, thus not being useful;
whoever wrote the textbook tried to explain as well as possible (for her/his narrative purposes) the most important topics at hand, thus (according to the adopted definition of explanation) implicitly identifying the most important questions whose answers provide a good overview of the topics.
Consequently, our main contribution is a novel pipeline of YAI software for enhancing the explanatory power of a textbook based on an algorithm (called Intelligent Explanation Generator) for identifying the questions best answered by a collection of texts. Indeed, according to theory, what makes a YAI good at explaining is its ability to identify implicit and relevant questions to answer, somehow anticipating the needs of the explainee.
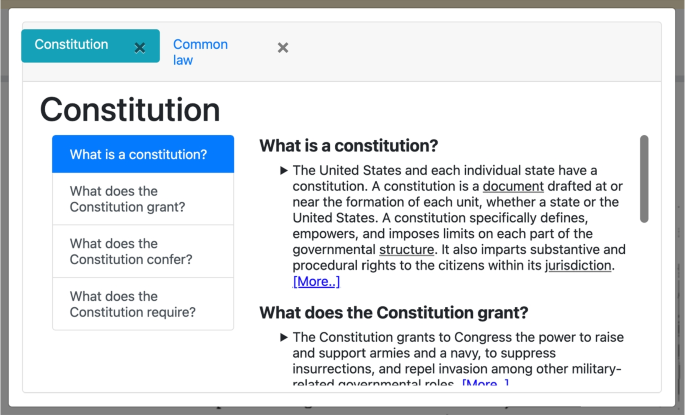
Example of intelligent explanatory overview generated by YAI4Edu. This figure contains an example of interactive overview in the form of a scrollspy showing relevant questions and answers as explanations. The reader can select a new topic to overview by clicking on any underlined word
As a case study for our proposed YAI for education (YAI4Edu, for short), we considered a teaching scenario where the excerpts of a textbook, “United States Legal Language and Culture: An Introduction to the U.S. Common Law System” (Brostoff & Sinsheimer, 2013 ), together with the encyclopedia of the Legal Information Institute of the Cornell Law School and thousands of cases of the Board of Veterans’ Appeals (BVA) Footnote 5 are used for teaching how to write a legal memorandum Footnote 6 in support of a disability claim for Post-Traumatic Stress Disorder (PTSD) according to the U.S. legal system.
The complexity of legal jargon and intricate language in the field of law offers a substantial test for YAI4Edu, making our case study an ideal showcase of its abilities. We utilize over 16,000 diverse resources for this study, including a textbook actively used in a University of Pittsburgh course. This enhances the study’s real-world practicality and educational relevance. When coupled with the important societal context of veterans’ PTSD disability claims, our case study highlights the potential of AI applications in education, particularly in complex fields such as law. For an example of an intelligent explanation generated by YAI4Edu in this scenario, see Fig. 1 .
As an example to clarify what Hypothesis 1 means in this case, let us suppose we want to explain what a legal memorandum is. The textbook (Brostoff & Sinsheimer, 2013 ) does it by describing what a memo is in a legal sense, what it is for, what the proper form of a legal memorandum is, and what sections it should include. The textbook also provides secondary details , explaining each step of drafting a memorandum, why writing a memo is difficult, what the heading of a memorandum contains, and so on. Hence, in this case, Hypothesis 1 implies that the most useful implicit questions to ask are not those whose answers are only secondary details. This is because they are too specific to represent the whole textbook’s explanatory content adequately. Instead, the best choices are the main questions such as “what is the proper form of a legal memorandum”, “what is a memo in a legal sense”, because they best represent the content of the textbook.
To evaluate YAI4Edu and verify Hypothesis 1 , we conducted a within-subjects user study , comprising more than 100 students. This was done to study how different strategies to identify helpful implicit questions impact the quality of the resulting explanations. In particular, during the study, different explanations (an example is shown in Fig. 5 ) were given to English-speaking students about the task of writing a legal memorandum . For more details about the explanations used in the experiment, see Table 2 .
We compared the explanations generated by the Intelligent Explanation Generator (relying on Hypothesis 1 ) with those of the following two baseline algorithms :
a random explanations generator : an algorithm that organizes explanations by randomly selecting implicit questions from those answered by the corpus of considered texts;
a generic explanations generator : an algorithm that uses very generic questions (e.g., why , how , what ) instead of those extracted from the textbook, under the assumption that all possible (implicit) questions are instances of such generic questions.
The results supported Hypothesis 1 . They showed that our Intelligent Explanation Generator outperforms the baselines mentioned above in all the considered explanations, selecting questions that are not too specific or generic. The differences in performance were statistically significant ( P values below .005). Notably, the results also show that the explanations generated from generic questions are significantly better than those generated from random questions, giving further evidence in support of the validity of the hypothesis.
Synopsis. In section “ Related Work ”, we discuss existing literature on interactive e-books for education, focusing on AI-based solutions. In section “ Background ”, we provide sufficient background to understand the theory behind the algorithms presented in section “ YAI4Edu: a YAI for Improving the Explanatory Power of an Intelligent Textbook ”. Then, in section “ Case Study: A Textbook for Teaching How to Write Legal Memoranda ”, we present our case study on legal writing, and in section “ Experiment ”, we introduce the experiment for verifying Hypothesis 1 . Finally, in section “ Discussion: Results and Limitations ” and section “ Limitations and Future Work ”, we discuss the experimental results and the limitations of the proposed approach, while also highlighting potential avenues for future work.
Related Work
Some studies suggest that using intelligent textbooks Footnote 7 and interactive e-books leads to an increase in use, motivation and learning gains versus static e-books (Ericson, 2019 ). Of the several streams of work on the topic of interactive e-books and intelligent textbooks, most focus on the cognitive process of the reader, studying how to enhance the pedagogical productivity of textbooks through expert systems or sophisticated interfaces. They usually accomplish this by:
showing personal progress through open learner models (Kay & Kummerfeld, 2019 , 2021 );
specializing on ad hoc tasks through some domain modeling (Beier & Rau, 2022 ; Deligiannis et al., 2019 ; Chacon et al., 2021 ; Matsuda & Shimmei, 2019 );
modeling a student through questions, in order to identify and suggest personalized contents (Thaker et al., 2019 ; Mohammed & Shaffer, 2021 ; Matsuda & Shimmei, 2019 );
associating pedagogically valuable quizzes and exercises to portions of the e-book (Wang et al., 2022 ; Shimmei & Matsuda, 2022 ; Campenhout et al., 2021a , b );
providing tools for manually creating new interactive e-books (Wang et al., 2021 ; Pursel et al., 2019 ; Kluga et al., 2019 ).
The use of AI for the automatic generation of interactive e-books seems to be under-explored. In one such project, (Barria-Pineda et al., 2022 ) propose to automatically augment the sections of existing books with related YouTube videos by directly annotating the PDF, thus without breaking the structure of these textbooks.
Unlike the previous literature examples, our approach attempts to fully automatically convert an existing e-book into an interactive version by exploiting theories of explanations, intelligent interfaces, and YAI. In particular, we are not interested in the task of generating verbatim Footnote 8 questions for quizzes or exercises as in Wang et al. ( 2022 ); Shimmei and Matsuda ( 2022 ); Campenhout et al. ( 2021a , 2021b ). Instead we pursue the idea that questions (even non-verbatim ones) can be a practical criterion to organize and categorize the content of explanations. Moreover, instead of considering any question as a suitable candidate for this task, we empirically show that some questions are more useful than others and that the best questions for explanatory overviews are neither too generic nor too specific.
This section aims to provide enough background information for the reader to understand what an explanation is according to Ordinary Language Philosophy and the consequent theory of YAI.
Explanations According to Ordinary Language Philosophy
The concept of explanation in philosophy Footnote 9 began to have a more precise role in the 20th century with the growth and development of the philosophy of science. The publication by Hempel and Oppenheim ( 1948 ) of their “Studies in the Logic of Explanation” gave rise to what is considered the first theory of explanations: the deductive-nomological model. Sometime later, this first model came to be considered fatally flawed (Bromberger, 1966 ; Salmon, 1984 ). Indeed, Hempel’s epistemic theory of explanations is not empiricist: it is concerned (mistakenly) only with logical form, so an explanation can be such regardless of the actual processes and entities conceptually required to understand it.
Several more modern and competing theories of explanation have resulted from this criticism (Mayes, 2001 ). Some of these theories, for instance, those proposed by Salmon ( 1984 ) and van Fraassen ( 1980 ), take a scientific approach, framing explanations purely as answers to why questions. These theories represent a theoretical and largely scientific interpretation of the process of explanation.
On the other hand, other theorists have taken a more grounded approach to the idea of explanations. These are grounded in the practicalities of how people perform explanations in everyday life (Mayes, 2001 ). An exemplar of this approach is the theory proposed by Achinstein ( 1983 ). His theory, which is firmly rooted in Ordinary Language Philosophy, underscores the communicative or linguistic aspect of an explanation. It highlights the importance of an explanation’s role in fostering understanding between individuals by answering questions.
In particular, according to Achinstein’s theory, explaining is an illocutionary act born of a clear intention to produce new understandings in an explainee by providing a correct content-giving answer to an open-ended question. According to this view, answering by “filling in the blanks” of a pre-defined answer template, as with most one-size-fits-all approaches, prevents answering from being explanatory since it lacks this illocutionary purpose.
Despite this definition, illocution seems too abstract to implement in an actual software application. Nonetheless, recent efforts towards the automated generation of explanations (Sovrano & Vitali, 2021 , 2022b ), have shown that it may be possible to define illocution in a more “computer-friendly” way. Indeed, as stated by Sovrano and Vitali ( 2021 ), illocution in explaining involves informed and pertinent answers not just to the main question but also to other questions that are implicitly relevant to the explanations and the explainee. These questions can be understood as instances of archetypes such as why , why not , how , what for , what if , what , who , when , where , how much , etc.
Definition 1
(Illocution in Explaining) Explaining is an illocutionary act that provides answers to an explicit question on some topic along with answers to several other implicit or unformulated questions deemed necessary for the explainee to understand the topic properly. Sometimes these implicit questions can be inferred through a thorough analysis of the explainee’s background knowledge, history, and objectives, also considering Frequently Asked Questions (FAQs). However, in the most generic case, no assumption can be made about the explainee’s knowledge and objectives. The only implicit questions that can then be exploited for illocution are the most generic ones, called archetypal questions .
For example, if someone asks “How are you doing?”, an answer like “I am good” would not be considered an explanation. By contrast, a different answer, such as “I am happy because I just got a paper accepted at this important venue, and [...]” would generally be considered an explanation because it answers other archetypal questions along with the main question.
Definition 2
(Archetypal Question) An archetypal question is an archetype applied on a specific aspect of the explanandum. Examples of archetypes are the interrogative particles (e.g., why , how , what , who , when , where ), or their derivatives (e.g., why not , what for , what if , how much ), or also more complex interrogative formulas (e.g., what reason , what cause , what effect ). Accordingly, the same archetypal question may be rewritten in several different ways, as “why” can be rewritten in “what is the reason” or “what is the cause”.
Archetypal Questions in Linguistic Theories
Casting the semantic annotations of individual propositions as narrating an archetypal question-answer pair recently gained increasing attention in computational linguistics (He et al., 2015 ; FitzGerald et al., 2018 ; Michael et al., 2018 ; Pyatkin et al., 2020 ). In particular, the main archetypes coming from Abstract Meaning Representation theory (Michael et al., 2018 ) are: what , who , how , where , when , which , whose , why . We refer to these archetypes as the primary ones because they consist only of interrogative particles.
On the other hand, the main archetypes coming from PDTB-style discourse theory (Pyatkin et al., 2020 ) (also called secondary archetypes because they make use of the primary archetypes ) are: in what manner , what is the reason , what is the result , what is an example , after what , while what , in what case , despite what , what is contrasted with , before what , since when , what is similar , until when , instead of what , what is an alternative , except when , unless what .
Although many more archetypes could be devised (e.g., where to or who by ), we believe that the list of questions we provided earlier is already rich enough to be generally representative, whereas more specific questions can always be framed by using the interrogative particles we considered (e.g., why , what ). Primary archetypes can be used to represent any fact and abstract meaning (Bos, 2016 ). In contrast, the secondary archetypes can cover all the discourse relations between them (at least according to the PDTB theory).
How to Measure the Degree of Explainability
Degree of Explainability (DoX) is a model-agnostic approach and metric, proposed by Sovrano and Vitali ( 2022c ), to objectively evaluate explainability. It builds on Achinstein’s theory of explanations and the interpretation of illocution given by Sovrano and Vitali ( 2022b ). DoX can quantify the degree of explainability of a corpus of texts by estimating how adequately that corpus could be used to answer in an illocutionary way an arbitrary set of archetypal questions about the explanandum.
In other words, Sovrano and Vitali ( 2022c ) show how the degree of explainability of information depends on the number of archetypal questions to which it can adequately answer. In practice, DoX scores are computed by measuring and aggregating the pertinence with which a snippet of text can answer a (pre-defined) set of archetypal questions. Specifically, pertinence scores are obtained employing pre-trained deep language models for general-purpose answer retrieval (Karpukhin et al., 2020 ; Bowman et al., 2015 ) applied to a particular graph of triplets automatically extracted from text to facilitate this type of information retrieval.
Explanatory Artificial Intelligence
An Explanatory AI (YAI) is an artificial intelligence program designed to generate user-centered, interactive explanations out of (possibly extensive) collections of explainable information (Sovrano & Vitali, 2022a ). An example of YAI based on Achinstein’s theory of explanation is YAI4Hu Footnote 10 (Sovrano & Vitali, 2021 , 2022b ).
YAI4Hu is a fully automatic explanatory tool that explains (pre-existing) documentation about an AI-based system. In particular, the textual content of such documentation is algorithmically reorganized and represented as a special hypergraph where information can be either explored through overviewing or searched via open-ended questioning . On the one hand, open-ended questioning can be performed by asking open-ended questions in English through a search box that uses the knowledge graph for efficient answer retrieval.
On the other hand, overviewing can be performed iteratively from an initial explanation by clicking on automatically annotated words for which more information is needed. In particular, annotated words are visible because they have a unique format that makes them easy to recognize. After clicking on an annotation, a modal window opens (see Fig. 1 ), showing a navigation bar of tabs containing explanatory overviews of the clicked annotated words. The information shown in the overview is as follows:
A short description of the explained word (if available).
The list of other words that are taxonomically connected.
A list of pre-defined archetypal questions (e.g., why is this aspect/concept important, what is this aspect/concept, etc.) and their respective answers ordered by estimated pertinence (see section “ Intelligent Explanation Generator: An Algorithm for the Identification of Pedagogically Useful Questions from Textbooks ” for a definition of pertinence).
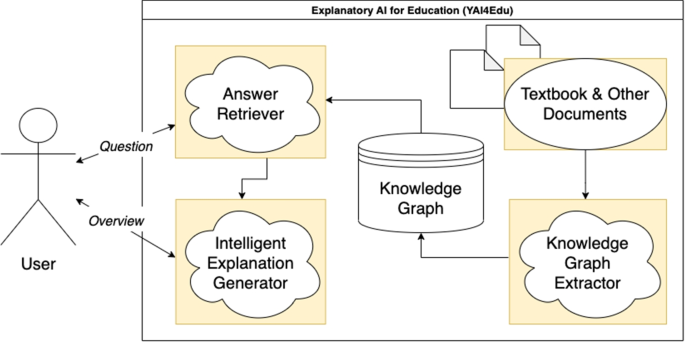
Simplified Flow Diagram of YAI4Edu. This diagram shows the main components of YAI4Edu. As in YAI4Hu, the user can ask questions and get overviews. However, differently, YAI4Edu uses a new component (i.e., the “Intelligent Explanation Generator”) for generating overviews, as described in section “ Intelligent Explanation Generator: An Algorithm for the Identification of Pedagogically Useful Questions from Textbooks ”
YAI4Edu: a YAI for Improving the Explanatory Power of an Intelligent Textbook
The benefit of answering questions for learning has been shown in many studies (Rivers, 2021 ; Pan & Rickard, 2018 ), further supporting the assertion that explaining is akin to question-answering and that organizing contents on a question-answer base might be beneficial for the explainee. However, creating questions with proper detail that effectively helps students’ learning usually requires experience and extensive efforts (Shimmei & Matsuda, 2022 ).
For this reason, with the present work, we propose YAI4Edu (pipeline shown in Fig. 2 ), an extension of YAI4Hu (see section “ Explanatory Artificial Intelligence ”) to automatically transform static educative e-books (in PDF, XML or HTML format) into interactive intelligent textbooks by increasing their explanatory power. Similarly to YAI4Hu, YAI4Edu uses open-ended questioning and overviewing as main mechanisms for producing explanations. More specifically, interaction is given by: i) word glosses that can be clicked to open an overview ; ii) a special kind of search box that allows the reader to get answers to any open-ended English question.
In particular, we exploit Achinstein’s theory of explanations to reorganize, on-demand, the contents of a textbook and connect it with external integrative resources (e.g., an encyclopedia) to provide the user with more useful explanations. We build a novel heuristic to identify and anticipate the most useful implicit questions an explainee might have about a textbook, organizing information accordingly. Indeed, according to theory (see section “ Explanations According to Ordinary Language Philosophy ”), what makes an Explanatory AI good at explaining is its ability to identify implicit and relevant questions to answer, i.e., its illocutionary power .
We developed an algorithm capable of automatically extracting questions from a textbook and identifying those that are neither too detailed (because they would otherwise only be answered in a minor part of the textbook) nor too general (because they would be answered inaccurately in the more detailed textual content). We did it by starting from the hypothesis that the most useful implicit questions a user may have about a collection of texts are those best answered by the whole collection.
Suppose it is possible to quantify the explanatory power of a collection of explainable texts (i.e., through DoX; see section “ How to Measure the Degree of Explainability ”) and organize it accordingly by prioritizing the most explanatory contents (i.e., those with the highest DoX scores) over the others. In that case, it is also possible to identify which texts are the most explained within a corpus of documents and, therefore, the most useful questions to ask about the textbook. More specifically, for YAI4Edu, we developed several AI-based mechanisms that improve over the baseline YAI4Hu algorithm producing more pedagogically useful explanations via:
intelligent overviewing (an example of overview is shown in Fig. 3 ), by automatically extracting the most useful questions that the textbook (or the support material) is answering;
smart annotation generation, through the automatic identification of a glossary of words explained by the textbook’s contents.
In the following subsections, we will describe the technical details behind these new mechanisms and briefly summarize how the answer retrieval algorithm works. We also release the source code of YAI4Edu Footnote 11 and the anonymized data collected to evaluate it (see section “ Experiment ”) under MIT license at https://github.com/Francesco-Sovrano/YAI4Edu .
Automated Question Extraction for Intelligent Overviewing
According to theory, illocution in explaining involves informed and pertinent answering questions, implicitly relevant to the explanations and the explainee, understood as instances of archetypes. In section “ Archetypal Questions in Linguistic Theories ”, we presented different types of these archetypal questions identified by foundational linguistic theories.
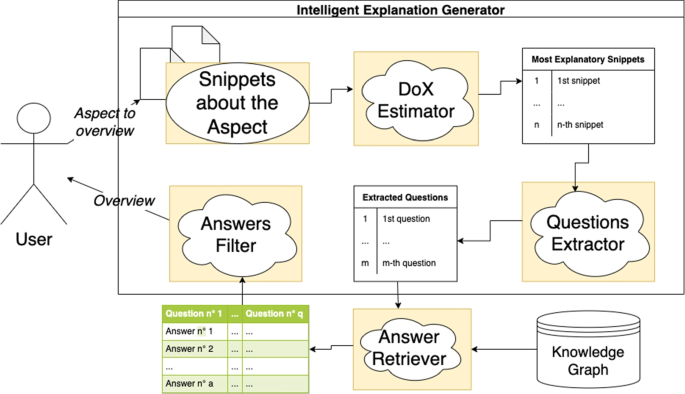
Flow Diagram of the Intelligent Explanation Generator. This diagram shows how an explainee can obtain an intelligent overview. First, the user decides which aspect of the explanandum to overview (i.e., by clicking on an annotated word available on the screen). Then the system extracts an explanation from the textbook or any other collection of texts (e.g., other textbooks, an encyclopedia) by using an AI for question extraction and an AI for answer retrieval as described in section “ Answer Retrieval ”
Specifically, the secondary archetypes coming from discourse theory (Pyatkin et al., 2020 ) are meant to capture how Elementary Discourse Units (EDUs) Footnote 12 are connected. In contrast, the primary archetypes coming from Abstract Meaning Representation theory Footnote 13 (Michael et al., 2018 ) are meant to capture the informative components within the EDUs by possibly supporting answering basic questions such as “ who did what to whom , when or where ”. For example, from the sentence, “ The existence and validity of a contract, or any term of a contract, shall be determined by the law which would govern it under this regulation if the contract or term were valid, ” it is possible to extract the following discourse relation Footnote 14 about contingency: “In what case would the law govern under this regulation? If the contract or term were valid,” and the following Abstract Meaning Representation (AMR) question-answer: “By what is the existence and validity of a contract determined? By the law that would govern it under this regulation if the contract or clause were valid.”
In particular, the discourse-based and AMR-based archetypal questions we need for YAI4Edu are extracted from sentences and paragraphs through a deep language model based on T5 Footnote 15 (Raffel et al., 2020 ), pre-trained on a multi-task mixture of unsupervised and supervised tasks.
Since vanilla T5 is not trained to extract discourse-based and AMR-based questions, we had to fine-tune T5 on some public datasets designed for this task. These datasets are QAMR (Michael et al., 2018 ) for extracting AMR-based questions and QADiscourse (Pyatkin et al., 2020 ) for discourse-based questions.
Most importantly, the QAMR and QADiscourse datasets are unrelated to any of the textbooks we will consider (see section “ Case Study: A Textbook for Teaching How to Write Legal Memoranda ”) for evaluating YAI4Edu. In particular, they do not contain any legal document or snippet of text written in legalese. In other words, we do not refine T5 on legal texts but instead train it on spoken English, given the high data availability. Therefore, training T5 the way we do does not imply any legal fine-tuning. Legal fine-tuning would require the costly extraction of a dataset of AMR- and discourse-based questions from legal texts, as well as ad-hoc adaptations of the theories of discourse and abstract meaning representation to legalese.
In particular, the QAMR dataset is made of 107,880 different questions (and answers), while the QADiscourse dataset is made of 16,613 different questions (and answers), as described in section “ Archetypal Questions in Linguistic Theories ”. The two considered datasets are tuples of \(<s, q, a>\) , where s is a source sentence, q is a question implicitly expressed in s , and a is an answer expressed in s . T5 is fine-tuned to tackle at once the following four tasks per dataset:
extract a given s and q ,
extract q given s and a ,
extract all the possible q given s ,
extract all the possible a given s .
Specifically, we fine-tuned the T5 model on QAMR and QADiscourse for five epochs. Footnote 16 The objective of the fine-tuning was to minimize a loss function measuring the difference between the expected output and the output given by T5. A mathematical definition of such a loss function is given by Raffel et al. ( 2020 ).
At the end of the training, the average loss was 0.4098, meaning that our fine-tuned T5 model cannot perfectly extract AMRs or EDUs from the text composing the training set. On the one hand, this is a good thing because it is likely that the model did not over-fit the training set. On the other hand, this clearly indicates that the questions extracted by our T5 model can be imperfect, containing errors that could propagate to the Intelligent Explanation Generator.
Intelligent Explanation Generator: An Algorithm for the Identification of Pedagogically Useful Questions from Textbooks
Assuming that the goal of a textbook is to explain something to the reader, and based on the theoretical understandings expressed in section “ Explanations According to Ordinary Language Philosophy ”, our YAI4Edu is designed around the idea that organizing the explanatory space (i.e., the space of all possible bits of explanation) as clusters of archetypal questions and answers is beneficial for an explainee. In particular, YAI4Edu uses the following predefined interactions inherited from YAI4Hu to allow the user to explore this explanatory space:
Open-ended Question-Answering: the user writes a question and receives one or more relevant answers.
Aspect Overviewing: the user selects an aspect of the explanandum (i.e., contained in an answer) and receives as an explanation a set of relevant archetypal answers involving different aspects that can be explored as well. Archetypal answers can also be expanded, increasing the level of detail.
As hypothesized (cf. Hypothesis 1 ), archetypal questions that are too generic are unlikely to represent the explanatory goals of a sufficiently complex and elaborated collection of texts. The archetypal questions originally used by YAI4Hu for overviewing are too generic and predefined, frequently not adhering to the explanatory requirements of the overview. Therefore, considering the need for YAI4Edu to be pedagogically helpful, we designed a novel theoretically grounded AI algorithm to quantify how much an archetypal question is likely to represent the explanatory goals of a collection of texts. We called this algorithm the Intelligent Explanation Generator.
More specifically, instead of using predefined generic archetypal questions for our Intelligent Explanation Generator, we also consider more domain-specific ones automatically extracted from the knowledge graph through the AI for question-answer extraction. In particular, once an explanandum is chosen, the workflow of the Intelligent Explanation Generator consists of the following steps (also shown in Fig. 3 ).
Step 1: the algorithm computes the DoX (see section “ How to Measure the Degree of Explainability ”) of all snippets of text about a given explanandum (i.e., an aspect to overview), finding the top k snippets with the highest DoX and also finding the archetypal questions extracted from them by the algorithm described in section “ Automated Question Extraction for Intelligent Overviewing ”;
Step 2: for each question selected in the previous step, the algorithm identifies a set of pertinent answers within the text snippets. An answer is said to be pertinent to a question when its pertinence score Footnote 17 is greater than a given pertinence threshold p ;
Step 3: the algorithm filters the pertinent answers, keeping the best q questions and a answers by executing the following sub-steps: i) questions that are too long are removed, i.e., questions whose length (without considering the length of the explanandum label) exceeds a threshold L ; ii) if a question has some grammatical error, it is automatically corrected via Gramformer Footnote 18 , a deep neural network; iii) questions that are too similar are removed, Footnote 19 prioritizing the questions extracted from the most explanatory snippets (i.e., those with the highest DoX) and the shortest questions; iv) answers that are too short or too long are removed; v) the questions with no valid answers are removed; vi) the answers that could be assigned to several questions are given to the question with the highest estimated pertinence; vii) for each question, only the a answers with the highest pertinence score are kept; viii) the questions are sorted by the decreasing pertinence of the first answer, and only the top q questions are kept.
Importantly, step 1 is performed before step 2 to reduce the asymptotic time complexity of step 2. Selecting the questions best answered by the corpus (step 2) has an asymptotic complexity \(O(\vert Q_c \vert \cdot \vert S \vert )\) that grows with the number of questions extracted from the snippets of text, where Q is the set of questions about an aspect c to be explained and S is the set of snippets of text. Therefore, this complexity in the worst-case scenario (without step 1) can be quadratic in the size of the textbook or collection of texts, i.e., \(O(\vert S \vert ^2)\) .
Rather than having a quadratic complexity, a computationally simpler approach can perform an initial filtering procedure to consider only those questions coming from the paragraphs with the highest DoX (as step 1 does), thus converting \(\vert Q_c \vert \) into a constant number independent from \(\vert S \vert \) . Hence, considering that computing the DoX of \(\vert S \vert \) snippets of text has an asymptotic time complexity equal to \(O(\vert S \vert )\) , it follows that step 1 reduces the complexity of the Intelligent Explanation Generator to \(O(\vert S \vert )\) .
If Hypothesis 1 is true, then the Intelligent Explanation Generator will be able to produce better and more satisfying explanation overviews (than the baseline YAI4Hu). This is because it will be able to anticipate, in a sense, implicit questions the user may have.
Not all words, though, require an overview. That is because, in practice, only a tiny fraction of the words in a text are helpful to explain. Indeed, many words have common-sense meanings (e.g., the words: “and”, “first”, “figure”) and, therefore, should not be explained. Otherwise, the explainee might be overwhelmed by largely redundant and pointless information that would only hinder the usability of the YAI.
To intelligently avoid jotting down unnecessary words, our smart annotation mechanism only annotates those concepts and words that can be explained by (the knowledge graph extracted from) the textbook and other supplementary texts. More specifically, to understand whether a word should be annotated, the algorithm executes the following instructions:
It checks whether the word is a stop word (i.e., a commonly used word such as “and” or “or”). If so, the word is not annotated.
If the word is not a stop word, the algorithm generates its overview through the Intelligent Explanation Generator. Then, it computes the cumulative pertinence score of the answers composing the overview; if greater than a given threshold, it annotates the word.
This annotation mechanism is intended to remove noisy annotations and distractors so that the reader can focus only on the most central and well-explained concepts. Moreover, the cumulative pertinence score, used to understand whether a word should be annotated, can also be used to understand the topics in the corpus of documents that are better explained.
Consequently, the smart annotation mechanism and the Intelligent Explanation Generator are integral components of the YAI4Edu system. Notably, the Intelligent Explanation Generator tackles the challenge of selecting questions that balance between being too general or overly specific through the abovementioned filtering and refinement steps. By incorporating these steps, the Intelligent Explanation Generator prioritizes questions derived from the most informative excerpts, favors concise questions, and filters out excessively similar ones. This process aims to identify questions that align well with the explanatory objectives of the text collection, as discussed in section “ Discussion: Results and Limitations ”.
Answer Retrieval
Similarly to YAI4Hu, YAI4Edu uses a pipeline of AI algorithms to extract a particular graph of knowledge from a collection of texts that an information retrieval system can exploit to answer a given question. In particular, a dependency parser detects all the possible grammatical clauses Footnote 20 within the collection of texts. Each such clause stands for an edge of the knowledge graph.
In practice, these clauses are represented as combinations of subjects, templates, and objects/nominal modifiers. Footnote 21 We refer to these as template-triplets. Specifically, templates consist of an ordered token sequence connecting subjects and objects/modifiers in a clause. In these templates, the subjects and the objects/modifiers are represented by the placeholders \(``\{subj\}''\) and \(``\{obj\}''\) , respectively. The resulting template-triplets are a sort of function where the predicate is the body, and the object/modifier and the subject are the parameters.
Obtaining a natural language representation of these template-triplets involves replacing the instances of the parameters in the body. This natural language representation is then used as a possible answer for retrieval by measuring the similarity between its embedding and the embedding of a question. An example (taken from the case study discussed in section “ Case Study: A Textbook for Teaching How to Write Legal Memoranda ”) of template-triple is:
Subject: “A constitution”
Template: \(``\{subj\}''\) specifically defines, empowers, and imposes \(\{obj\}\) on each part of the governmental structure
Object: “limits”
The resulting knowledge graph is imperfect because of the adopted extraction procedure. It may contain mistakes caused by wrongly identified grammatical dependencies or other issues. However, the likelihood of such errors occurring is relatively low. This is primarily attributed to the high accuracy of the model we used for part-of-speech tagging. Footnote 22
To increase the interoperability of the extracted knowledge graph with external resources, we formatted it as an RDF Footnote 23 graph. In particular, RDF has features that facilitate data merging even if the underlying schemas differ. To format a graph of template triplets in an RDF graph, we performed the following steps:
We assigned a URI Footnote 24 to every node (i.e., subject and object) and edge (i.e., template) of the graph by lemmatizing the associated text. We assigned an RDFS Footnote 25 label to each URI corresponding to the associated text.
We added special triplets to keep track of the sources from which the template-triplets were extracted so that each node and edge can refer to its source document or paragraph.
We added sub-class relations between composite concepts (syntagms) and the simplest concepts (if any) composing the syntagm. For instance, “ contractual obligation ” is a sub-class of “ obligation ”. Therefore, we employ the RDFS predicate \(``\{subj\}rdfs:subClassOf ~\{obj\}''\) as a template for the triplet that signifies this sub-class relationship.
For more technical details about how we performed all the steps mentioned above to convert the template-triplets into an RDF graph, please refer to Sovrano et al. ( 2020 ) or the source code of YAI4Edu.
Finally, the algorithm to retrieve answers from the extracted knowledge graph is based on the following steps. Let C be the set of concepts in a question q , \(m=<s,t,o>\) be a template-triplet, \(u=t(s,o)\) be the natural language representation of m , also called an information unit , and z be its source paragraph. DiscoLQA retrieves answers by finding the most similar concepts to C within the knowledge graph, retrieving all their related template-triplets m (including those of the sub-classes), and selecting, among the natural language representations u of the retrieved template-triplets, those that are likely to answer q . The likelihood of u answering q is estimated by computing the cosine similarity between its contextualized embedding \(<u,z>\) and the embedding of q . If \(<u,z>\) is similar enough to q , then z is said to answer q .
The embeddings of \(<u,z>\) and q are obtained through a deep language model specialized in answer retrieval pre-trained in ordinary English to associate similar vectorial representations to a question and its correct answers. The pre-trained deep language model we employed in implementing YAI4Edu is a variation of MiniLM (Wang et al., 2021 ) published by Reimers and Gurevych ( 2019 ).
Case Study: A Textbook for Teaching How to Write Legal Memoranda
To showcase and evaluate YAI4Edu, we considered a case study in the intersection between AI and law. In particular, we applied YAI4Edu to the following material explaining, among other things, how to write a legal memorandum in a U.S. legal context for a veteran’s PTSD disability claim:
22 pages excerpted from the textbook “ United States Legal Language and Culture: An Introduction to the U.S. Common Law System ” (Brostoff & Sinsheimer, 2013 pp. 47-60, 93-96, 101-103, 131-132). Footnote 26
5,407 open access web pages about concepts related to the U.S. legal system coming from the encyclopedia of the Legal Information Institute of the Cornell Law School Footnote 27 (5,406 web pages) and Wikipedia Footnote 28 (1 web page).
11,198 legal cases on PTSD disability claims taken from the official website of the Board of Veterans’ Appeals (BVA). Footnote 29
Altogether, the included material, comprising more than 16,000 documents, complements the primary teaching material on which YAI4Edu focuses, i.e., the excerpts of the selected textbook. In particular, the textbook is used in “Applied Legal Analytics and AI,” Footnote 30 an interdisciplinary course at the University of Pittsburgh, co-taught by instructors from the University of Pittsburgh School of Law and Carnegie Mellon University’s Language Technologies Institute. It provides “a hands-on practical introduction to the fields of artificial intelligence, machine learning, and natural language processing as they are being applied to support the work of legal professionals, researchers, and administrators.”
Teaching how to write a legal memorandum for the U.S. legal system is a course objective, in part, because in a common law system, such as the American one, the use of AI assists practitioners in efficiently retrieving legal cases for constructing arguments (Phelps & Ashley, 2022 ). A legal memorandum is an organized document that summarizes relevant laws to support a conclusion on a particular legal issue. Writing it can require legal practitioners to navigate through large databases of cases, i.e., to retrieve the definitions of technical and specific concepts or to understand which argumentation patterns are most common in a particular context. Indeed, some of the distinguishing features of legal writing are:
Authority: The writer must back up assertions and statements with citations of authority (i.e., precedents and other decided cases).
Argument re-use: A more effective memorandum may reuse existing documents as templates or argumentation patterns.
Formality: The written legal document should be properly formatted according to existing standards.
Hence, legal practitioners may now be required to learn how to efficiently and effectively interact with existing AI-based technological solutions for information retrieval to speed up legal writing and to learn the complexities of legal writing. Given the task’s complexity and the course’s goals, we envisaged that it might be of utmost relevance and utility to design and create a tool such as YAI4Edu that could ease the acquisition of the necessary knowledge for a student to learn legal writing.
Specifically, YAI4Edu should help students understand, from real examples, how to write a legal memorandum comprising legal arguments to defend or attack a claim. In particular, students have to understand how to use statutes, read and summarize cases, synthesize cases, draft a legal memorandum, and use legal concepts in writing. This may involve learning legal concepts as well as skills of making arguments with concrete cases selected using legal information retrieval tools, as is typical in the U.S. legal context.
In particular, applying YAI4Edu to the collection of documents mentioned above, we extracted a knowledge graph of 52,987,778 RDF triplets from the BVA cases and a knowledge graph of 2,059,145 RDF triplets from the textbook excerpts and the other web pages; other statistics are shown in Table 1 .
Thanks to the compositional nature of RDF graphs, we can combine smaller individual graphs into larger ones without altering the original meaning or semantics. Taking advantage of this feature, we enriched the graph with about ten manually added RDF triplets to fill identified knowledge gaps, such as “memo” being a synonym for “memorandum”. These gaps surfaced during software testing.
However, we have not thoroughly analyzed the whole extracted RDF graph. As a result, other knowledge gaps may yet emerge. Despite our incomplete analysis, we believe this property of compositionality of the knowledge graph used by YAI4Edu is of utmost importance. Indeed, it enables manually correcting any error produced during the graph extraction and easily integrating it with additional or missing knowledge.
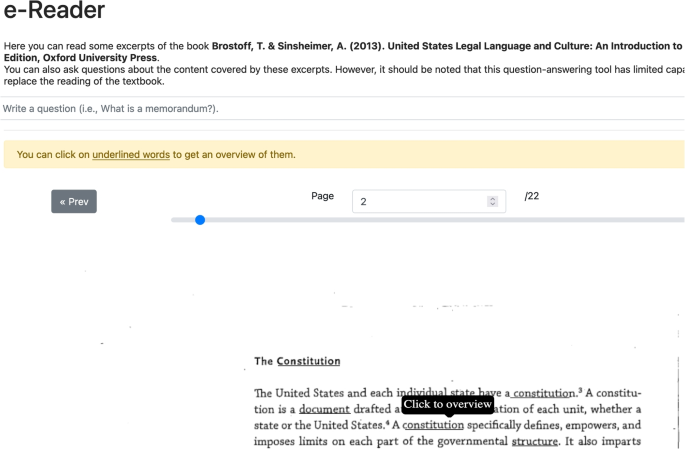
Landing page of YAI4Edu applied to the case study. This figure contains a screenshot of the annotated textbook (Brostoff & Sinsheimer, 2013 ) and the input for open-ended questioning . Clicking on underlined words opens an explanatory overview , an example of which is shown in Fig. 1
This knowledge graph helped to build an interactive and intelligent version of the textbook, as described in section “ YAI4Edu: a YAI for Improving the Explanatory Power of an Intelligent Textbook ” and shown in Fig. 2 . There, an input box for open-ended questioning and annotated (i.e., underlined) words for overviewing (shown in Figs. 4 and 1 ) provide the user with interactive elements to obtain intelligent explanations without breaking the structure of the textbook.
The choice of hyper-parameters (i.e., the pertinence threshold p or the question similarity threshold s ; cf. section “ Intelligent Explanation Generator: An Algorithm for the Identification of Pedagogically Useful Questions from Textbooks ”) of the Intelligent Explanation Generator is focused on generating concise and compact explanations. In particular, the hyper-parameters chosen for this instance of YAI4Edu are the following: i) number of snippets with the highest DoX to consider \(k=10\) ; ii) answer pertinence threshold \(p=.57\) ; iii) maximum overview question length \(L=50\) ; iv) question similarity threshold \(s=.95\) ; v) minimum and maximum answer length equal to 150 and 1000; vi) maximum number of questions per overview \(q=4\) ; vii) maximum number of answers per overview question \(a=2\) .
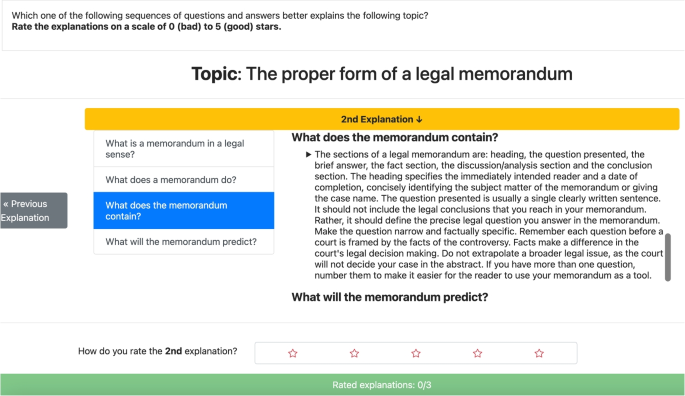
Screenshot of the web application used during the experiment. This figure shows what the participants in the user study see during the experiment
Explanations and explanatory tools may be complex artifacts whose quality depends on a wide range of different factors (Sovrano et al., 2020 ), including:
the quality of the explainable information;
the logic used for presenting this information, namely the set of rules employed to present the information in a meaningful and coherent manner, which we refer to as the presentation logic ;
the quality of the interface.
In particular, with this experiment, we are interested in evaluating the presentation logic used by YAI4Edu for selecting and reorganizing questions and answers into explanations.
Sovrano and Vitali ( 2022a ) have already demonstrated, with several examples and experiments, a user-centered YAI is better than one-size-fits-all and static explanations. In this experiment, instead of evaluating the whole interactive e-book with a rather time-consuming test, we focused on evaluating the one feature of YAI4Edu that should be responsible for improving the explanatory power of the e-book: the Intelligent Explanation Generator. According to theory (see section “ Explanations According to Ordinary Language Philosophy ”), what makes a YAI good at explaining is its ability to identify implicit and relevant questions to answer, i.e., its illocutionary power.
Therefore, in this experiment (which is a within-subjects user study), we directly ask real students to rate explanations (on a scale of 0 to 5 stars) for how well they adequately explain a given topic, as shown in Fig. 5 . We do it to understand the extent to which the explanations generated by our Intelligent Explanation Generator are satisfactory and whether they are better than baseline explanatory strategies, as stated by Hypothesis 1 . More details about the experiment can be found in Appendix A .
The two baselines against which the Intelligent Explanation Generator is compared are variants of the same. They use the same sequence of steps to generate their explanations, apart from the step responsible for selecting the explanatory questions, which is different. These two baselines are:
An explainer that uses randomly chosen questions to organize the contents of an overview . This explainer randomly selects \(q=4\) questions, setting the maximum question length to \(L=\infty \) and using a lower answer pertinence threshold \(p=.3\) (and not \(p=.57\) as in the Intelligent Explanation Generator). This prevents the number of questions from diminishing too much due to not finding sufficiently relevant answers.
YAI4Hu’s generic overview generator, which instead employs pre-defined and very generic archetypal questions, using the same four questions (i.e., what is it, how is it, where is it and why ) for each topic.
Given the case study at hand (see section “ Case Study: A Textbook for Teaching How to Write Legal Memoranda ”), the main objective of the explanatory contents is to explain how to write a legal memorandum appropriate for the U.S. legal system and a veteran’s PTSD disability claim. The excerpts of the considered textbook are about legal writing, while the collection of legal cases of the BVA are about PTSD disability claims. Thus, we can say that some of the goals of the YAI for this case study are to explain: i) what is the proper form of a legal memorandum; ii) what sections should be included in a legal memorandum; iii) what legal standard does a veteran need to satisfy for a disability claim; iv) what are the elements of the legal standard a veteran needs to satisfy for a disability claim; v) what issues do the required elements of a disability claim raise; and vi) what kinds of legal arguments are appropriate for resolving such issues.
Considering that we need an experiment lasting a maximum of 10 minutes (in order to minimize costs: each participant cannot be paid less than 6 \(\pounds \) per hour on Prolific), we chose the following three topics for evaluating the explainers:
Topic 1: The proper form of a legal memorandum.
Topic 2: The effects of a disability.
Topic 3: The elements of the legal standard a veteran needs to satisfy for a PTSD disability claim.
In particular, the explanations for the first two topics are extracted from the textbook and web pages (the first is better explained by the textbook, the second by the web pages). In contrast, the explanations for the third topic are extracted from legal cases. For more details about the explanations used in the experiment, see Table 2 .
Discussion: Results and Limitations
Through Prolific, we gathered 130 participants, all students aged between 19 and 38. 28 participants were discarded for the reasons stated in Appendix A . Eventually, 102 valid submissions were collected.
The results (shown in Fig. 6 ) indicate that the intelligent explainer received the highest rates, followed by the generic one; the worst was the random explainer. To further validate the results and verify that the improvements of the intelligent explainer over the baselines are statistically significant, we performed one-sided Mann-Whitney U-tests (a non-parametric version of the t-test for independent samples) whose results are summarized in Fig. 6 . Results clearly show that, assuming \(P < .05\) is enough to assert statistical significance, the intelligent explainer is superior to the baselines regarding perceived explanatory power in all three chosen topics. For more details on statistical tests, see Appendix B .
Interestingly, looking at the topics separately, we also have statistical evidence showing that the intelligent explainer is better than the generic and the random explainer for the first two topics, but not enough statistical evidence for the last one. This may be because the variance of the cumulative pertinence of the explanations about the third topic (see Table 2 ) is too low. Alternatively (and more likely), this may also be because the explanations about the last topic were extracted from a corpus of legal cases rather than textbooks or other educational contents as the other two, thus being harder to explain. In particular, this intuition is corroborated by the statistics of Table 1 , where one can see that the legal cases have a ratio of explained concepts close to 0.32%. In contrast, the textbook and web pages have 2.96% (10 times greater). This difference in explainability between the two documents corpora may impact the quality of extracted explanations. Indeed, as pointed out by some qualitative feedback, the explanations extracted from the BVA cases contain too much (unexplained) technical jargon and too long sentences (e.g., “the first topic was easy to understand also the second one, the problem with the last one it was too long and was not straight to the point.”).
This shows that the most useful implicit questions a user may have about a collection of texts will likely be those best answered by the whole collection. Furthermore, even if the random explanations have a cumulative pertinence score greater than generic explanations (at least for the first two topics, as shown in Table 2 ), they are evaluated as worse explanations nonetheless. This evidence further supports Hypothesis 1 , showing that too specific archetypal questions may be less effective than generic ones at explaining and that intelligently balancing between generality and specificity is needed, as also suggested by some qualitative feedback:
“All of the [random] explanations were OK, but improvement is needed. They lack a sense of direction. It’s like they go around mountains to prove one single point. All [generic] explanations were easy to decode and were straight to the point. All [intelligent] explanations were a mixture of first and second explanations.”
“The [random explanations] proved unsatisfactory for all three topics: the explanations do not follow a logical order, are incomplete, and often contain incorrect or irrelevant elements. The [generic and intelligent explanations] are quite complete. The [generic explanations] seem to fit more practical questions, while [intelligent explanations] fit more theoretical ones. I believe [intelligent explanations] are preferable for the topic at hand.”
Limitations and Future Work
One limitation of our study pertains to its scope, encompassing both the duration of the study and the number of participants involved. Specifically, the study was conducted over a brief 10-minute period and comprised a relatively small group of 102 individuals. This restricted scope raises concerns regarding the generalizability of our findings. Furthermore, our research primarily concentrated on participant ratings and qualitative feedback, without directly measuring changes in learning outcomes.
As a result, while our results offer initial insights into the potential applications of educational tools like YAI4Edu, they should be approached with caution. In order to validate and expand upon these preliminary findings, it is necessary to conduct more comprehensive studies over longer time-frames, involving larger and more diverse participant groups. However, previous research (Sovrano and Vitali, 2022a ) provides compelling evidence supporting the notion that user-centered illocution significantly enhances the effectiveness of YAI software, leading to more efficient explanations and improved learning outcomes, as suggested also by qualitative feedback.
It is worth noting that even though qualitative feedback was optional, a notable 81% of users provided feedback. Interestingly, 19 participants left highly positive feedback (e.g., “the explanations were superb and of good quality”) without suggesting any improvement or explaining their rates, unlike the remaining 64 users. Overall, we identified 6 major suggestions for improvement :
Avoid long and redundant explanations : suggested by 32 participants;
Avoid or explain legal jargon : 24;
Avoid generic or incomplete information : 18;
Use simpler questions : 9;
Provide examples when explaining : 7;
Provide better organized and compartmentalized contents : 5.
The complaints were primarily about too-long explanations, unexplained legal jargon, or generic/incomplete information. Some qualitative feedback comments ask for more conciseness, and others for less. Some participants preferred generic explanations over intelligent ones. Interestingly, one could turn this into a feature if the system could offer users a choice of generic or intelligent explanations.
The qualitative feedback was extremely helpful in identifying the main problems and limitations of YAI4Edu and the baselines, pointing to future work. In particular, we believe YAI4Edu’s smart annotation mechanism can partially mitigate the jargon problem by explaining it. However, we could not verify this with the experiment because it was set to take 10 minutes, so intelligent annotations were excluded. See Appendix A for more details on qualitative feedback.
Feedback has also indicated that YAI4Edu could significantly improve in terms of efficiently integrating retrieved information into a coherent structure. As it stands, explanations often tend to be lengthy, repetitive, and disjointed. The introduction of generative AI like ChatGPT might serve as a valuable solution to these issues, re-elaborating the information retrieved by YAI4Edu. Indeed, ChatGPT has the potential to produce content that is not only concise and coherent but also well-organized. It can adeptly avoid or interpret complex (legal) jargon, presenting content in a well-structured and compartmentalized manner. These features could address at least three out of the six primary improvement areas identified in the qualitative feedback. As a result, the integration of such AI technology could significantly enhance the effectiveness of YAI4Edu, as shown in Sovrano et al. ( 2023 ).
It should be noted, however, that while ChatGPT can complement YAI4Edu, it cannot fully replace it. There are unique challenges associated with using large language models like ChatGPT to generate explanations from input documents, such as textbooks. These challenges include memory limitations and the risk of generating inaccurate or copyrighted content. Unlike ChatGPT, YAI4Edu primarily relies on information retrieval technologies, eliminating the need to input the entire context of the explanation into the language model. This is particularly important also for standard-sized textbooks, where memory constraints can easily become an issue, as discussed in the study by Sovrano et al. ( 2023 ).
Future studies also need to focus on several factors to secure more robust results. First, we need further investigation into the effectiveness of our random explanations generator as a baseline. Given the feedback regarding redundant explanations and the need for better-organized content, exploring sophisticated alternatives like ChatGPT might provide a stronger baseline for comparison with YAI4Edu.
Second, our research did not integrate commonsense and domain-general knowledge into the YAI pipeline, making the system highly dependent on well-written, logically coherent texts. This dependency sets a high standard for the source text, potentially limiting the extensibility of our work. Addressing this concern might be possible with the help of large language models, such as ChatGPT, which could potentially excel in tasks similar to YAI4Edu. This holds particularly true for unstructured texts and those not professionally written, offering promising avenues for future research.
In this paper, we presented YAI4Edu, an Explanatory AI for the automatic generation of interactive and adaptive e-books for education. To create YAI4Edu, we enhanced the YAI proposed by Sovrano and Vitali ( 2022b ), making it more explanatory and more intelligent through a novel AI, called Intelligent Explanation Generator, for extracting explanations that are neither too specific nor too generic.
The idea behind our work is that explaining is akin to question answering, so the more questions an intelligent textbook can answer, the more it can explain. In other words, the explanatory power of a book can be improved by reorganizing it interactively, firstly to help readers identify the most critical questions to be answered about the textbook, but also to get answers to their questions. In particular, YAI4Edu uses the Intelligent Explanation Generator, an efficient pipeline for automatic (archetypal) question extraction and answer retrieval, to select these important questions and use them to reorganize the contents of a textbook. This is done following Hypothesis 1 for which the most useful questions a user may have about a collection of texts (e.g., a textbook) are best answered in the collection as a whole.
We applied YAI4Edu to a textbook for teaching how to write legal memoranda appropriate for the U.S. legal system and a PTSD disability claim. This was integrated with 5,406 external web pages from a legal encyclopedia and 11,198 legal cases from the Board of Veterans’ Appeals. Then, to show that our novel strategy for generating explanations is more effective than baseline approaches, we devised a within-subjects user study involving more than 100 English-speaking students who evaluated explanations about three different and relevant topics. The final results were in many cases statistically significant and always in favor of our Intelligent Explanation Generator, suggesting that Hypothesis 1 is true.
The impact of our YAI software pipeline extends to various sectors, including education, professional training, and industry. Within the education sector, our tool enhances the use of textbooks by seamlessly integrating external content, facilitating efficient exploration and retrieval of information through answer retrieval mechanisms. In professional training, it has the potential to elucidate complex concepts and procedures, thereby expediting knowledge acquisition, particularly in highly technical domains. In the industrial context, our tool can simplify technical documentation and improve customer support, as suggested by Sovrano and Vitali ( 2022b ); Sovrano et al. ( 2023 ).
Looking ahead, we foresee a notable shift in education with interactive e-books and AI-generated content supplementing or replacing traditional textbooks. These tools promise personalized, up-to-date learning experiences. Rather than replacing educators, these AI tools could augment their capabilities by providing personalized instruction at scale. They could explain basic concepts and answer common questions, thus allowing educators to focus on more complex topics, critical thinking, creativity, and personalized guidance.
An explainee is a person receiving an explanation.
The word explanandum means “what has to be explained” in Latin.
This theory has been supported by various experiments and studies involving over 190 participants (Sovrano & Vitali, 2022a , b ).
The explanatory space is the space of all possible bits of explanation about an explanandum. A more formal definition is given by Sovrano and Vitali ( 2022a ).
The BVA is an administrative tribunal within the United States Department of Veterans Affairs that determines whether U.S. military veterans are entitled to claimed veterans’ benefits and services.
A legal memorandum is an organized document that summarizes relevant laws to support a conclusion on a particular legal issue.
Intelligent textbooks extend regular textbooks by integrating machine-manipulable knowledge (Wang et al., 2021 ).
The verbatim question is a question for which an answer can be identified in a related instructional text (i.e., source text) (Shimmei & Matsuda, 2022 ).
One of the first known philosophers to work on the concept of explanation was Aristotle with his theory of causation (Stein, 2011 ).
YAI4Hu stands for YAI for Humans. Examples of YAI for reinforcement learning agents are discussed by Sovrano et al. ( 2022 ).
We cannot release the textbook excerpts (Brostoff & Sinsheimer, 2013 ) because they are copyrighted.
The smallest unit of coherent text that expresses a complete or partial discourse relation.
A semantic formalism that represents the meaning of natural language sentences as rooted, directed, and labeled graphs.
A discourse relation identifies two EDUs: the first one is encoded in the question, the second one in the answer.
T5 is an encoder-decoder model based on the assumption that all Natural Language Processing problems can be converted in a text-to-text problem.
An epoch is one complete cycle through the entire training dataset.
Pertinence scores are numbers in [0, 1] computed by measuring the cosine similarity between vectorial representations of question and answer obtained through deep neural networks specialized for answer retrieval.
https://github.com/PrithivirajDamodaran/Gramformer
A question is said to be similar to another when its similarity score is above a given threshold s . Similarity scores are calculated like pertinence scores.
A typical clause consists of a subject and a syntactic predicate, the latter typically a verb phrase composed of a verb with any objects and other modifiers.
A nominal modifier is a noun (or noun phrase) functioning as a non-core (oblique) argument or adjunct.
Known as the “en_core_web_md” model, it boasts an accuracy score of 0.97. More details about this model can be found at https://spacy.io/models/en .
RDF is a standard model for data interchange on the Web (Allemang and Hendler, 2011 ).
A URI, or Uniform Resource Identifier, is a string of characters that provides a simple and extensible means for identifying a resource on the Internet.
RDFS, or RDF Schema, is a language used for creating vocabularies in the semantic web, allowing for the description of classes, properties, and their interrelationships.
We received explicit consent from the copyright holder to use excerpts of this textbook for our experiments and the related scientific publications.
https://www.law.cornell.edu
https://en.wikipedia.org/wiki/Law_of_the_United_States
https://search.usa.gov/search?affiliate=bvadecisions &sort_by= &query=PTSD &commit=Search
https://www.law.pitt.edu/academics/courses/catalog/5719
https://www.prolific.co
Achinstein, P. (1983). The nature of explanation. Oxford University Press. Retrieved from https://books.google.it/books?id=0XI8DwAAQBAJ
Allemang, D., & Hendler, J. A. (2011). Semantic web for the working ontologist - effective modeling in RDFS and owl, second edition. Morgan Kaufmann. Retrieved from http://www.elsevierdirect.com/product.jsp?isbn=9780123859655
Armstrong, R. A. (2014). When to use the bonferroni correction. Ophthalmic and Physiological Optics, 34 (5), 502–508.
Article Google Scholar
Barria-Pineda, J., Narayanan, A. B. L., & Brusilovsky, P. (2022). Augmenting digital textbooks with reusable smart learning content: Solutions and challenges. S. A. Sosnovsky, P. Brusilovsky, & A. S. Lan (Eds.), Proceedings of the fourth international workshop on intelligent textbooks 2022 co-located with 23d international conference on artificial intelligence in education (AIED 2022), durham, uk, july 27, 2022 (Vol. 3192, pp. 77–91). CEUR-WS.org. Retrieved from http://ceur-ws.org/Vol-3192/itb22_p8_full9434.pdf
Beier, J. P., & Rau, M. A. (2022). Embodied learning with physical and virtual manipulatives in an intelligent tutor for chemistry. M.M.T. Rodrigo, N. Matsuda, A.I. Cristea, & V. Dimitrova (Eds.), Artificial intelligence in education - 23rd international conference, AIED 2022, durham, uk, july 27-31, 2022, proceedings, part I (Vol. 13355, pp. 103–114). Springer. Retrieved from https://doi.org/10.1007/978-3-031-11644-5_9
Bos, J. (2016). Expressive power of abstract meaning representations. Comput. Linguistics, 42 (3), 527–535. Retrieved from https://doi.org/10.1162/COLI_a_00257
Bowman, S. R., Angeli, G., Potts, C., & Manning, C. D. (2015). A large annotated corpus for learning natural language inference. L. Márquez, C. Callison-Burch, J. Su, D. Pighin, & Y. Marton (Eds.), Proceedings of the 2015 conference on empirical methods in natural language processing, EMNLP 2015, lisbon, portugal, september 17-21, 2015 (pp. 632–642). The Association for Computational Linguistics. Retrieved from https://doi.org/10.18653/v1/d15-1075
Bromberger, S. (1966). Why-questions. R.G. Colodny (Ed.), Mind and cosmos - essays in contemporary science and philosophy (pp. 86–111). University of Pittsburgh Press
Brostoff, T.K., & Sinsheimer, A. (2013). United states legal language and culture: An introduction to the u.s. common law system. Oxford University Press USA. Retrieved from https://books.google.it/books?id=SVsGAQAAQBAJ
Campenhout, R. V., Brown, N., Jerome, B., Dittel, J. S., & Johnson, B. G. (2021a). Toward effective courseware at scale: Investigating automatically generated questions as formative practice. C. Meinel, M. Pérez-Sanagustín, M. Specht, & A. Ogan (Eds.), L@s’21: Eighth ACM conference on learning @ scale, virtual event, germany, june 22-25, 2021 (pp. 295–298). ACM. Retrieved from https://doi.org/10.1145/3430895.3460162
Campenhout, R. V., Dittel, J. S., Jerome, B., & Johnson, B. G. (2021b). Transforming textbooks into learning by doing environments: An evaluation of textbook-based automatic question generation. S.A. Sosnovsky, P. Brusilovsky, R.G. Baraniuk, & A.S. Lan (Eds.), Proceedings of the third international workshop on inteligent textbooks 2021 co-located with 22nd international conference on artificial intelligence in education (AIED 2021), online, june 15, 2021 (Vol. 2895, pp. 60–73). CEURWS. org. Retrieved from http://ceur-ws.org/Vol-2895/paper06.pdf
Chacon, I.A., Barria-Pineda, J., Akhuseyinoglu, K., Sosnovsky, S.A., & Brusilovsky, P. (2021). Integrating textbooks with smart interactive content for learning programming. S.A. Sosnovsky, P. Brusilovsky, R.G. Baraniuk, & A.S. Lan (Eds.), Proceedings of the third international workshop on inteligent textbooks 2021 co-located with 22nd international conference on artificial intelligence in education (AIED 2021), online, june 15, 2021 (Vol. 2895, pp. 4–18). CEUR-WS.org. Retrieved from http://ceur-ws.org/Vol-2895/paper11.pdf
Deligiannis, N., Panagiotopoulos, D., Patsilinakos, P., Raftopoulou, C. N., & Symvonis, A. (2019). Interactive and personalized activity ebooks for learning to read: The iread case. S.A. Sosnovsky, P. Brusilovsky, R.G. Baraniuk, R. Agrawal, & A.S. Lan (Eds.), Proceedings of the first workshop on intelligent textbooks co-located with 20th international conference on artificial intelligence in education (AIED 2019), chicago, il, usa, june 25, 2019 (Vol. 2384, pp. 57–69). CEUR-WS.org. Retrieved from http://ceur-ws.org/Vol-2384/paper06.pdf
Ericson, B. (2019). An analysis of interactive feature use in two ebooks. S. A. Sosnovsky, P. Brusilovsky, R. G. Baraniuk, R. Agrawal, & A. S. Lan (Eds.), Proceedings of the first workshop on intelligent textbooks colocated with 20th international conference on artificial intelligence in education (AIED 2019), chicago, il, usa, june 25, 2019 (Vol. 2384, pp. 4–17). CEUR-WS.org. Retrieved from http://ceur-ws.org/Vol-2384/paper01.pdf
FitzGerald, N., Michael, J., He, L., & Zettlemoyer, L. (2018). Large-scale QASRL parsing. I. Gurevych & Y. Miyao (Eds.), Proceedings of the 56th annual meeting of the association for computational linguistics, ACL 2018, melbourne, australia, july 15-20, 2018, volume 1: Long papers (pp. 2051–2060). Association for Computational Linguistics. Retrieved from https://aclanthology.org/P18-1191/
He, L., Lewis, M., & Zettlemoyer, L. (2015). Question-answer driven semantic role labeling: Using natural language to annotate natural language. L. Márquez, C. Callison-Burch, J. Su, D. Pighin, & Y. Marton (Eds.), Proceedings of the 2015 conference on empirical methods in natural language processing, EMNLP 2015, lisbon, portugal, september 17-21, 2015 (pp. 643–653). The Association for Computational Linguistics. Retrieved from https://doi.org/10.18653/v1/d15-1076
Hempel, C. G., & Oppenheim, P. (1948). Studies in the logic of explanation. Philosophy of Science, 15 (2), 135–175.
Karpukhin, V., Oguz, B., Min, S., Lewis, P.S.H., Wu, L., Edunov, S., . . . & Yih, W. (2020). Dense passage retrieval for open-domain question answering. B. Webber, T. Cohn, Y. He, & Y. Liu (Eds.), Proceedings of the 2020 conference on empirical methods in natural language processing, EMNLP 2020, online, november 16-20, 2020 (pp. 6769–6781). Association for Computational Linguistics. Retrieved from https://doi.org/10.18653/v1/2020.emnlp-main.550
Kay, J., & Kummerfeld, B. (2019). Scaffolded, scrutable open learner model (SOLM) as a foundation for personalised e-textbooks. S.A. Sosnovsky, P. Brusilovsky, R.G. Baraniuk, R. Agrawal, & A.S. Lan (Eds.), Proceedings of the first workshop on intelligent textbooks co-located with 20th international conference on artificial intelligence in education (AIED 2019), chicago, il, usa, june 25, 2019 (Vol. 2384, pp. 38–43). CEURWS. org. Retrieved from http://ceur-ws.org/Vol-2384/paper04.pdf
Kay, J., & Kummerfeld, B. (2021). PUMPT: an e-textbook platform based on a personal user model for learning (short paper). S. A. Sosnovsky, P. Brusilovsky, R. G. Baraniuk, & A.S. Lan (Eds.), Proceedings of the third international workshop on inteligent textbooks 2021 co-located with 22nd international conference on artificial intelligence in education (AIED 2021), online, june 15, 2021 (Vol. 2895, pp. 27–34). CEURWS. org. Retrieved from http://ceur-ws.org/Vol-2895/paper12.pdf
Kluga, B., Jasti, M.S., Naples, V., & Freedman, R. (2019). Adding intelligence to a textbook for human anatomy with a causal concept map based ITS. S. A. Sosnovsky, P. Brusilovsky, R. G. Baraniuk, R. Agrawal, & A. S. Lan (Eds.), Proceedings of the first workshop on intelligent textbooks co-located with 20th international conference on artificial intelligence in education (AIED 2019), chicago, il, usa, june 25, 2019 (Vol. 2384, pp. 124–134). CEUR-WS.org. Retrieved from http://ceur-ws.org/Vol-2384/paper13.pdf
Matsuda, N., & Shimmei, M. (2019). PASTEL: evidence-based learning engineering method to create intelligent online textbook at scale. S.A. Sosnovsky, P. Brusilovsky, R.G. Baraniuk, R. Agrawal, & A. S. Lan (Eds.), Proceedings of the first workshop on intelligent textbooks colocated with 20th international conference on artificial intelligence in education (AIED 2019), chicago, il, usa, june 25, 2019 (Vol. 2384, pp. 70–80). CEUR-WS.org. Retrieved from http://ceur-ws.org/Vol-2384/paper07.pdf
Mayes, G.R. (2001). Theories of explanation. Retrieved from https://iep.utm.edu/explanat/
Miao, F., Holmes, W., Huang, R., & Zhang, H. (2021). Ai and education: A guidance for policymakers. UNESCO Publishing
Michael, J., Stanovsky, G., He, L., Dagan, I., & Zettlemoyer, L. (2018). Crowdsourcing question-answer meaning representations. M.A. Walker, H. Ji, & A. Stent (Eds.), Proceedings of the 2018 conference of the north american chapter of the association for computational linguistics: Human language technologies, naacl-hlt, new orleans, louisiana, usa, june 1-6, 2018, volume 2 (short papers) (pp. 560–568). Association for Computational Linguistics. Retrieved from https://doi.org/10.18653/v1/n18-2089
Mohammed, M., & Shaffer, C. A. (2021). Increasing student interaction with an etextbook using programmed instruction (short paper). S.A. Sosnovsky, P. Brusilovsky, R. G. Baraniuk, & A. S. Lan (Eds.), Proceedings of the third international workshop on inteligent textbooks 2021 co-located with 22nd international conference on artificial intelligence in education (AIED 2021), online, june 15, 2021 (Vol. 2895, pp. 40–44). CEURWS. org. Retrieved from http://ceur-ws.org/Vol-2895/paper14.pdf
Pan, S. C., & Rickard, T. C. (2018). Transfer of test-enhanced learning: Metaanalytic review and synthesis. Psychological Bulletin, 144 (7), 710.
Phelps, T., & Ashley, K. (2022). “alexa, write a memo”: The promise and challenges of ai and legal writing. Legal Writing: J. Legal Writing Inst., 26 , 329.
Pursel, B., Ramsay, C.M., Dave, N., Liang, C., & Giles, C.L. (2019). Bbookx: Creating semi-automated textbooks to support student learning and decrease student costs. S. A. Sosnovsky, P. Brusilovsky, R. G. Baraniuk, R. Agrawal, & A. S. Lan (Eds.), Proceedings of the first workshop on intelligent textbooks co-located with 20th international conference on artificial intelligence in education (AIED 2019), chicago, il, usa, june 25, 2019 (Vol. 2384, pp. 81–86). CEUR-WS.org. Retrieved from http://ceur-ws.org/Vol-2384/paper08.pdf
Pyatkin, V., Klein, A., Tsarfaty, R., & Dagan, I. (2020). Qadiscourse - discourse relations as QA pairs: Representation, crowdsourcing and baselines. B. Webber, T. Cohn, Y. He, & Y. Liu (Eds.), Proceedings of the 2020 conference on empirical methods in natural language processing, EMNLP 2020, online, november 16-20, 2020 (pp. 2804–2819). Association for Computational Linguistics. Retrieved from https://doi.org/10.18653/v1/2020.emnlp-main.224
Raffel, C., Shazeer, N., Roberts, A., Lee, K., Narang, S., Matena, M., . . . & Liu, P. J. (2020). Exploring the limits of transfer learning with a unified textto- text transformer. Journal of Machine Learning Research, 21 (140), 1–140:67. Retrieved from http://jmlr.org/papers/v21/20-074.html
Reimers, N., & Gurevych, I. (2019). Sentence-bert: Sentence embeddings using siamese bert-networks. K. Inui, J. Jiang, V. Ng, & X. Wan (Eds.), Proceedings of the 2019 conference on empirical methods in natural language processing and the 9th international joint conference on natural language processing, EMNLP-IJCNLP 2019, hong kong, china, november 3-7, 2019 (pp. 3980–3990). Association for Computational Linguistics. Retrieved from https://doi.org/10.18653/v1/D19-1410
Rivers, M.L. (2021). Metacognition about practice testing: a review of learners’beliefs, monitoring, and control of test-enhanced learning. Educational Psychology Review, 33 (3), 823–862. Retrieved from https://doi.org/10.1007/s10648-020-09578-2
Salmon, W. (1984). Scientific explanation and the causal structure of the world. Princeton University Press. Retrieved from https://books.google.it/books?id=2ug9DwAAQBAJ
Shimmei, M., & Matsuda, N. (2022). Automatic question generation for evidence-based online courseware engineering. S.A. Sosnovsky, P. Brusilovsky, & A.S. Lan (Eds.), Proceedings of the fourth international workshop on intelligent textbooks 2022 co-located with 23d international conference on artificial intelligence in education (AIED 2022), durham, uk, july 27, 2022 (Vol. 3192, pp. 18–25). CEUR-WS.org. Retrieved from http://ceur-ws.org/Vol-3192/itb22_p2_short1338.pdf
Sovrano, F., Ashley, K., & Bacchelli, A. (2023). Toward eliminating hallucinations: Gpt-based explanatory ai for intelligent textbooks and documentation. S. A. Sosnovsky, P. Brusilovsky, & A. S. Lan (Eds.), Proceedings of the fifth international workshop on intelligent textbooks 2023 co-located with 24th international conference on artificial intelligence in education (AIED 2023), tokyo, japan, july 3-7, 2023. CEUR-WS.org
Sovrano, F., Palmirani, M., & Vitali, F. (2020). Legal knowledge extraction for knowledge graph based question-answering. S. Villata, J. Harasta, & P. Kremen (Eds.), Legal knowledge and information systems - JURIX 2020: The thirty-third annual conference, brno, czech republic, december 9-11, 2020 (Vol. 334, pp. 143–153). IOS Press. Retrieved from https://doi.org/10.3233/FAIA200858
Sovrano, F., Raymond, A., & Prorok, A. (2022). Explanation-aware experience replay in rule-dense environments. IEEE Robotics and Automation Letters, 7 (2), 898–905.
Sovrano, F., & Vitali, F. (2021). From philosophy to interfaces: an explanatory method and a tool inspired by achinstein’s theory of explanation. T. Hammond, K. Verbert, D. Parra, B. P. Knijnenburg, J. O’Donovan, & P. Teale (Eds.), IUI ’21: 26th international conference on intelligent user interfaces, college station, tx, usa, april 13-17, 2021 (pp. 81–91). ACM. Retrieved from https://doi.org/10.1145/3397481.3450655
Sovrano, F., & Vitali, F. (2022). Explanatory artificial intelligence (yai): human-centered explanations of explainable ai and complex data . Data Mining and Knowledge Discovery: Retrieved from. https://doi.org/10.1007/s10618-022-00872-x
Book Google Scholar
Sovrano, F., & Vitali, F. (2022b, nov). Generating user-centred explanations via illocutionary question answering: From philosophy to interfaces. ACM Transactions on Interactive Intelligent Systems, 12 , (4). Retrieved from https://doi.org/10.1145/3519265
Sovrano, F., & Vitali, F. (2022c). How to quantify the degree of explainability: Experiments and practical implications. 31th IEEE international conference on fuzzy systems, FUZZ-IEEE 2022, padova, july 18-23, 2022 (pp. 1–9). IEEE
Sovrano, F., Vitali, F., & Palmirani, M. (2020). Making things explainable vs explaining: Requirements and challenges under the GDPR. V. Rodríguez-Doncel, M. Palmirani, M. Araszkiewicz, P. Casanovas, U. Pagallo, & G. Sartor (Eds.), AI approaches to the complexity of legal systems XI-XII - AICOL international workshops 2018 and 2020: Aicol-xi@jurix 2018, aicol-xii@jurix 2020, xaila@jurix 2020, revised selected papers (Vol. 13048, pp. 169–182). Springer. Retrieved from https://doi.org/10.1007/978-3-030-89811-3_12
Stein, N. (2011). Causation and explanation in aristotle. Philosophy Compass, 6 (10), 699-707. Retrieved from https://compass.onlinelibrary.wiley.com/doi/abs/10.1111/j.1747-9991.2011.00436.x https://arxiv.org/abs/https://compass.onlinelibrary.wiley.com/doi/pdf/10.1111/j.1747-9991.2011.00436.x
Thaker, K., Brusilovsky, P., & He, D. (2019). Student modeling with automatic knowledge component extraction for adaptive textbooks. S.A. Sosnovsky, P. Brusilovsky, R.G. Baraniuk, R. Agrawal, & A. S. Lan (Eds.), Proceedings of the first workshop on intelligent textbooks co-located with 20th international conference on artificial intelligence in education (AIED 2019), chicago, il, usa, june 25, 2019 (Vol. 2384, pp. 95–102). CEURWS. org. Retrieved from http://ceur-ws.org/Vol-2384/paper10.pdf
van Fraassen, B.C. (1980). The scientific image. Clarendon Press. Retrieved from https://books.google.it/books?id=VLz2F1zMr9QC
Wang, M., Chau, H., Thaker, K., Brusilovsky, P., & He, D. (2021). Knowledge annotation for intelligent textbooks . Technology, Knowledge and Learning: Retrieved from. https://doi.org/10.1007/s10758-021-09544-z
Wang, W., Bao, H., Huang, S., Dong, L., & Wei, F. (2021). Minilmv2: Multi-head self-attention relation distillation for compressing pretrained transformers. C. Zong, F. Xia, W. Li, & R. Navigli (Eds.), Findings of the association for computational linguistics: ACL/IJCNLP 2021, online event, august 1-6, 2021 (Vol. ACL/IJCNLP 2021, pp. 2140–2151). Association for Computational Linguistics. Retrieved from https://doi.org/10.18653/v1/2021.findings-acl.188
Wang, Z., Valdez, J., Mallick, D.B., & Baraniuk, R.G. (2022). Towards human-like educational question generation with large language models. M.M.T. Rodrigo, N. Matsuda, A.I. Cristea, & V. Dimitrova (Eds.), Artificial intelligence in education - 23rd international conference, AIED 2022, durham, uk, july 27-31, 2022, proceedings, part I (Vol. 13355, pp. 153–166). Springer. Retrieved from https://doi.org/10.1007/978-3-031-11644-5_13
Download references
Acknowledgements
We thank the copyright holders of (Brostoff and Sinsheimer, 2013 ) for allowing us to use (parts of) the book to conduct the experiments, carry out the case study and present this article.
Open access funding provided by University of Zurich. This work was partially supported by the European Union’s Horizon 2020 research and innovation programme under the MSCA grant agreement No 777822 “GHAIA: Geometric and Harmonic Analysis with Interdisciplinary Applications”. F. Sovrano gratefully acknowledge the support of the Swiss National Science Foundation through the SNF Project 200021_197227.
Author information
Authors and affiliations.
Department of Informatics, University of Zurich, Rämistrasse 71, 8006, Zürich, Switzerland
Francesco Sovrano & Fabio Vitali
Department of Computer Science and Engineering, University of Bologna, Bologna, Italy
Francesco Sovrano
School of Law, University of Pittsburgh, Pittsburgh, PA, USA
Kevin Ashley
School of Computing and Information, University of Pittsburgh, Pittsburgh, PA, USA
Peter Leonid Brusilovsky
You can also search for this author in PubMed Google Scholar
Contributions
F. Sovrano : conceptualization, methodology, software, data curation, original draft preparation, visualization, investigation, validation, formal analysis. K. Ashley : conceptualization, review, editing and supervision. P. L. Brusilovsky : supervision. F. Vitali : supervision.
Corresponding author
Correspondence to Francesco Sovrano .
Ethics declarations
Conflicts of interest.
The authors have no conflicts of interest to declare which are relevant to the content of this article.
Additional information
Publisher's note.
Springer Nature remains neutral with regard to jurisdictional claims in published maps and institutional affiliations.
Appendix A Experiment Details
The experiment consisted of a 10-minute within-subjects user study where the explanations generated by two baseline explainers and YAI4Edu were evaluated by English-speaking students assembled with Prolific Footnote 31 , an online platform that helps recruit paid participants for online research.
Through Prolific, we gathered 130 participants, all students aged between 19 and 38, each paid \(\pounds \) 1. 28 of the participants were discarded for the following reasons:
26 participants were discarded because they were too quick (i.e., they spent less than 4 minutes completing the evaluation of all topics) or they skipped at least one topic (i.e., they spent less than 35 seconds on it without being a legal expert);
2 participants were rejected because they reported poor knowledge of written English (i.e., A1 or A2) or wrote non-grammatical qualitative feedback.
Eventually, 102 valid submissions were collected.
Of these 102 participants:
46 were males, 52 females, 1 identified itself as other/non-binary, and three preferred not to say;
81% stated that their written English proficiency level is C1 or C2, while 16% indicated a B2 level;
86% rated their knowledge of the U.S. legal system with a score lower than or equal to 3;
86 said they are not legal experts;
68 reported that they have low or no experience with legal writing, 29 wrote they have some experience, and five that their experience with legal writing is high.
Participants were required to be fluent in English, be resident in English-speaking countries (i.e., USA, UK, Ireland, Australia, Canada, New Zealand, South Africa), use a device with a large screen (e.g., a laptop, a desktop computer, a tablet in landscape mode), be at least 18 years old, and possess a student status and a minimum approval rating of 75% on Prolific.
Moreover, at the beginning of the study, they were asked to provide the following information anonymously: age; gender; whether they are legal experts; their proficiency in written English, on a scale of A1 (very low) to C2 (very high); their experience in legal writing (from none to high ); how they would rate their knowledge of the U.S. legal system on a scale of 0 (bad) to 5 (good) stars.
It is important to note that during the experiment, for each topic, each participant could compare the explanations generated by all three explainers, provided in random order to avoid unwanted biases that might be caused by some explanations being shown before or after others. Furthermore, using a predefined random seed, the explanations generated by the random explainer were the same for all participants.
In the experiment, participants were not informed about the origin of the explanations they evaluated, specifically whether they came from random, generic, or intelligent generators, nor were they made aware of the existence of these three different generators. Each explanation was to be evaluated on a 0 to 5-star scale, with 0 representing “bad” and 5 indicating “good”, as depicted in Fig. 5 . Once the testing was completed, participants were also invited to share their qualitative feedback in an open-ended format.
Some of the most interesting feedback examples are the following:
“The more lengthy explanations offer more details and give the reader a greater understanding but can feel a bit harder to read rather than the [generic explanations].”
“Dividing the topic into sections is good if the [questions] are relevant and make sense. Relatively simple explanations supported by evidence are better, in my opinion.”
“The longer explanations were more detailed and more understandable. The shorter definitions were also understandable and compact, but the law should be detailed. ”
“I particularly liked the layout that included ’what, why, how’, as it made the explanations easy to follow. [...] The headings with long sentences lost me before I began, and I found it hard to decipher the explanations.”
“I would make [explanations] shorter.”
“The answers should be less vague and focus more on details.”
“The explanations with very long or involved subheadings were difficult to follow. Often, when there are large blocks of text, my mind tends to get overwhelmed - simply making shorter subheadings and adding more paragraph breaks to the explanations helps with this.”
“The answers to the questions could have been more concise, for instance, in the first topic on what a memorandum is, though I found [intelligent] and [generic explanations] to be similar in their verbiage. I found [generic explanations] always easier to understand across the board. Because of its simplistic presentation, I did not spend time focusing on unnecessary details”
“The explanations are a bit difficult to follow as they are long, so as a reader, you get lost in the middle of the explanation and forget what you just read on top.”
“They were pretty straightforward and easy to understand, especially because descriptions relating to the law or topics that are difficult to understand are always filled with difficult jargon, but this simplified version made it easy to understand.”
“Generally, the explanations were full but a bit difficult to digest. There were cases in which not enough was explained to fully understand what a specific legal term meant and encompassed. The explanations that had a short and quick explanation of some legal terms followed by a more prolonged and detailed explanation were, for me, the easiest to grasp.”
Appendix B Statistical Tests
Complementing Fig. 6 , Table 3 provides the extended results of Mann-Whitney U-tests conducted on three different comparisons: Random vs Generic, Random vs Intelligent, and Generic vs Intelligent. We carried out these tests both on the aggregate data across all three topics (memorandum, disability, elements of legal standard), and on each topic individually. For each comparison, the results include the U statistic, the p-value, the Common Language Effect Size (CLES), and the Rank-biserial Correlation.
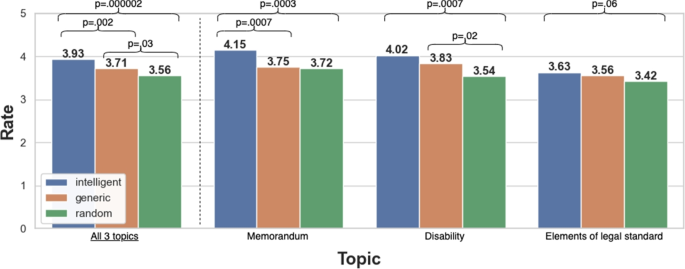
Experiment results. This figure contains bar charts showing the average scores and the statistically significant P values (indicated above the curly brackets) for each topic and explainer. For a list of all P values, see Table 3 . On the left side of the figure, one can see the results obtained by aggregating the scores for all three topics. In particular, the bar plot labeled as “memorandum” refers to the first topic, “disability” to the second topic, and “elements of legal standard” to the third topic
The interpretation of the results across all three topics reveals a consistent pattern. On the whole, we find a significant difference favoring the Generic over the Random group, and the Intelligent group over the Random group. The effect sizes of these differences range from small to medium, as indicated by the Common Language Effect Size and Rank-biserial Correlation. The differences between the Generic and Intelligent groups are also significant, albeit smaller.
When it comes to the specific topic of “Memorandum”, there is no notable difference between the Random and Generic groups. However, both the Random and Generic groups score significantly lower than the Intelligent group, with small to medium effect sizes.
In the case of “Disability”, the Random group performs significantly worse than both the Generic and Intelligent groups, with small to medium effect sizes. However, the Generic and Intelligent groups do not significantly differ.
For the “Elements of legal standard” topic, no significant difference is found between the groups, but the Random group does trend lower than the Generic and Intelligent groups, with small effect sizes. The Generic and Intelligent groups are also very similar in performance.
In all, while we see some variation by topic, the overarching pattern is that the Intelligent group consistently outperforms the Random group, and the Generic group falls somewhere in between.
Since we are performing multiple comparisons with Mann-Whitney U-tests, the chances of a false comparison increase. Some statistical tools that are used in this case to reduce the chance of a type I error (false positive) are: the Bonferroni correction, the Holm-Bonferroni method, or the Dunn-Šidák correction. These tools, however, are known to increase false negatives (Armstrong, 2014 ). Regardless, if we would use a Dunn-Šidák correction to adjust for 3 multiple comparisons per topic, then the minimum P value for claiming a statistically significant result would not be .05 but instead something close to .017.
Nevertheless, the collected findings still support Hypothesis 1 . As shown in Fig. 6 and Table 3 , many P values are much less than .017.
Rights and permissions
Open Access This article is licensed under a Creative Commons Attribution 4.0 International License, which permits use, sharing, adaptation, distribution and reproduction in any medium or format, as long as you give appropriate credit to the original author(s) and the source, provide a link to the Creative Commons licence, and indicate if changes were made. The images or other third party material in this article are included in the article’s Creative Commons licence, unless indicated otherwise in a credit line to the material. If material is not included in the article’s Creative Commons licence and your intended use is not permitted by statutory regulation or exceeds the permitted use, you will need to obtain permission directly from the copyright holder. To view a copy of this licence, visit http://creativecommons.org/licenses/by/4.0/ .
Reprints and permissions
About this article
Sovrano, F., Ashley, K., Brusilovsky, P.L. et al. How to Improve the Explanatory Power of an Intelligent Textbook: a Case Study in Legal Writing. Int J Artif Intell Educ (2024). https://doi.org/10.1007/s40593-024-00399-w
Download citation
Accepted : 29 February 2024
Published : 06 May 2024
DOI : https://doi.org/10.1007/s40593-024-00399-w
Share this article
Anyone you share the following link with will be able to read this content:
Sorry, a shareable link is not currently available for this article.
Provided by the Springer Nature SharedIt content-sharing initiative
- Intelligent textbooks
- Explanatory artificial intelligence
- Answer retrieval
- Automated question extraction
Advertisement
- Find a journal
- Publish with us
- Track your research
- Skip to main content
- Keyboard shortcuts for audio player

- Your Health
- Treatments & Tests
- Health Inc.
- Public Health
How to Thrive as You Age
Elevator or stairs your choice could boost longevity, study finds.

Allison Aubrey

Climbing stairs is a good way to get quick bursts of aerobic exercise, says cardiologist Dr. Carlin Long. lingqi xie/Getty Images hide caption
Climbing stairs is a good way to get quick bursts of aerobic exercise, says cardiologist Dr. Carlin Long.
At a time when less than half of adults in the U.S. get the recommended amount of exercise, there is new evidence that climbing stairs can reduce the risk of heart disease and help people live longer.
A new meta-analysis presented at a European Society of Cardiology conference finds that people in the habit of climbing stairs had about a 39% lower likelihood of death from heart disease, compared to those who didn't climb stairs. They also had a lower risk of heart attacks and strokes.
"I was surprised that such a simple form of exercise can reduce all-cause mortality," says study author Dr. Sophie Paddock, of the University of East Anglia and Norfolk and Norwich University Hospital Foundation Trust in the UK.
She and her colleagues looked at data from about 480,000 participants, ranging in age from mid-30s to mid-80s – about half were women. Paddock says the findings fit with a body of evidence pointing to the benefits of moderate-intensity exercise.

Shots - Health News
A cheap drug may slow down aging. a study will determine if it works.
The moment you start climbing steps, your body responds. "Your heart rate goes up, your cardiac output goes up, and your circulatory status improves," explains Dr. Manish Parikh , chief of cardiology at NewYork-Presbyterian Brooklyn Methodist Hospital. "And all of those we know have positive impacts," he says.
So, how much stair climbing is enough? One study found climbing six to ten flights a day was linked to a reduced risk of premature death. And another study found climbing more than five flights a day lowered the risk of cardiovascular disease by 20%.
To calculate this, researchers analyzed participants' risk of heart disease based on factors including blood pressure, cholesterol, smoking history, family history and genetic risk factors. Participants filled out questionnaires about their lifestyle and exercise habits including stair climbing. Over the course of 12 years, the stair climbers fared better at fending off heart disease. Notably, people who stopped climbing stairs during this time saw their risk rise. It's a reminder that in order to benefit from exercise, you've got to keep doing it.
The benefits can kick in pretty quickly. A review published earlier this year found a minimum of four to eight weeks are needed to start improving your cardiometabolic risk. The study found regular stair climbing can improve body composition, blood pressure, insulin sensitivity and blood pressure.
If you're trying to incorporate more movement into your day, adding stair climbing is a good way to get quick bursts of aerobic exercise, says Dr. Carlin Long , a cardiologist at University of California, San Francisco. "I think if people are able to achieve six to ten flights of exercise on stairs a day, that that would be a good target," but this will depend on your personal level of fitness, Long says.

Women who do strength training live longer. How much is enough?
"Exercise is one of the best approaches to cardiovascular fitness," Long says, and stair climbing can be convenient. "It doesn't require a gym membership," he says and many people can climb stairs at home or at work. Long says there's also value in more sustained exercise, such as a longer bike ride, a walk or jog, or a treadmill session, to meet the recommended 30 minutes a day of exercise.
Taking the stairs can also help build muscle. "Climbing stairs can be a wonderful mix of both aerobic exercise and resistance training," says Dr. Tamara Horwich , a cardiologist at UCLA who focuses on women's heart health.
You raise your heart rate and work your muscles at the same time. "You are building up those leg muscles by having to pull your weight up to the next stair," she says. And this is a key benefit given that only 24% of adults in the U.S. meet the recommended targets for both aerobic and muscle-strengthening exercise.
If you're not in the habit of stair climbing, you may have to start slowly.
If you track steps on their FitBit or Apple, these devices can be used to track climbing, too. "So instead of just looking at steps, [you] can also look at the number of stairs climbed and try to increase that," she says. It's a good way to gauge progress.

Millions of women are 'under-muscled.' These foods help build strength
This story was edited by Jane Greenhalgh
- heart disease
Help | Advanced Search
Electrical Engineering and Systems Science > Image and Video Processing
Title: pre-training on high definition x-ray images: an experimental study.
Abstract: Existing X-ray based pre-trained vision models are usually conducted on a relatively small-scale dataset (less than 500k samples) with limited resolution (e.g., 224 $\times$ 224). However, the key to the success of self-supervised pre-training large models lies in massive training data, and maintaining high resolution in the field of X-ray images is the guarantee of effective solutions to difficult miscellaneous diseases. In this paper, we address these issues by proposing the first high-definition (1280 $\times$ 1280) X-ray based pre-trained foundation vision model on our newly collected large-scale dataset which contains more than 1 million X-ray images. Our model follows the masked auto-encoder framework which takes the tokens after mask processing (with a high rate) is used as input, and the masked image patches are reconstructed by the Transformer encoder-decoder network. More importantly, we introduce a novel context-aware masking strategy that utilizes the chest contour as a boundary for adaptive masking operations. We validate the effectiveness of our model on two downstream tasks, including X-ray report generation and disease recognition. Extensive experiments demonstrate that our pre-trained medical foundation vision model achieves comparable or even new state-of-the-art performance on downstream benchmark datasets. The source code and pre-trained models of this paper will be released on this https URL .
Submission history
Access paper:.
- HTML (experimental)
- Other Formats
References & Citations
- Google Scholar
- Semantic Scholar
BibTeX formatted citation

Bibliographic and Citation Tools
Code, data and media associated with this article, recommenders and search tools.
- Institution
arXivLabs: experimental projects with community collaborators
arXivLabs is a framework that allows collaborators to develop and share new arXiv features directly on our website.
Both individuals and organizations that work with arXivLabs have embraced and accepted our values of openness, community, excellence, and user data privacy. arXiv is committed to these values and only works with partners that adhere to them.
Have an idea for a project that will add value for arXiv's community? Learn more about arXivLabs .

IMAGES
VIDEO
COMMENTS
A research hypothesis (also called a scientific hypothesis) is a statement about the expected outcome of a study (for example, a dissertation or thesis). To constitute a quality hypothesis, the statement needs to have three attributes - specificity, clarity and testability. Let's take a look at these more closely.
Definition: Hypothesis is an educated guess or proposed explanation for a phenomenon, based on some initial observations or data. It is a tentative statement that can be tested and potentially proven or disproven through further investigation and experimentation. Hypothesis is often used in scientific research to guide the design of experiments ...
Simple hypothesis. A simple hypothesis is a statement made to reflect the relation between exactly two variables. One independent and one dependent. Consider the example, "Smoking is a prominent cause of lung cancer." The dependent variable, lung cancer, is dependent on the independent variable, smoking. 4.
A research hypothesis is a statement that proposes a possible explanation for an observable phenomenon or pattern. It guides the direction of a study and predicts the outcome of the investigation. A research hypothesis is testable, i.e., it can be supported or disproven through experimentation or observation. Characteristics of a good hypothesis
Developing a hypothesis (with example) Step 1. Ask a question. Writing a hypothesis begins with a research question that you want to answer. The question should be focused, specific, and researchable within the constraints of your project. Example: Research question.
A hypothesis is a tentative statement about the relationship between two or more variables. It is a specific, testable prediction about what you expect to happen in a study. It is a preliminary answer to your question that helps guide the research process. Consider a study designed to examine the relationship between sleep deprivation and test ...
A research hypothesis, in its plural form "hypotheses," is a specific, testable prediction about the anticipated results of a study, established at its outset. It is a key component of the scientific method. Hypotheses connect theory to data and guide the research process towards expanding scientific understanding.
The Royal Society - On the scope of scientific hypotheses (Apr. 24, 2024) scientific hypothesis, an idea that proposes a tentative explanation about a phenomenon or a narrow set of phenomena observed in the natural world. The two primary features of a scientific hypothesis are falsifiability and testability, which are reflected in an "If ...
A research hypothesis is your proposed answer to your research question. The research hypothesis usually includes an explanation ('x affects y because …'). A statistical hypothesis, on the other hand, is a mathematical statement about a population parameter. Statistical hypotheses always come in pairs: the null and alternative hypotheses.
The hypothesis of Andreas Cellarius, showing the planetary motions in eccentric and epicyclical orbits.. A hypothesis (pl.: hypotheses) is a proposed explanation for a phenomenon.For a hypothesis to be a scientific hypothesis, the scientific method requires that one can test it. Scientists generally base scientific hypotheses on previous observations that cannot satisfactorily be explained ...
INTRODUCTION. Scientific research is usually initiated by posing evidenced-based research questions which are then explicitly restated as hypotheses.1,2 The hypotheses provide directions to guide the study, solutions, explanations, and expected results.3,4 Both research questions and hypotheses are essentially formulated based on conventional theories and real-world processes, which allow the ...
A hypothesis (plural hypotheses) is a proposed explanation for an observation. The definition depends on the subject. In science, a hypothesis is part of the scientific method. It is a prediction or explanation that is tested by an experiment. Observations and experiments may disprove a scientific hypothesis, but can never entirely prove one.
A hypothesis is a prediction of what will be found at the outcome of a research project and is typically focused on the relationship between two different variables studied in the research. It is usually based on both theoretical expectations about how things work and already existing scientific evidence. Within social science, a hypothesis can ...
Scientific hypotheses writing promoting and predicting is a skill that can help researchers to formulate and test their ideas about schizophrenia and its symptoms. This article provides a guide on how to write clear, testable, and falsifiable hypotheses, and how to use them to generate predictions and implications for future research.
HYPOTHESIS TESTING. A clinical trial begins with an assumption or belief, and then proceeds to either prove or disprove this assumption. In statistical terms, this belief or assumption is known as a hypothesis. Counterintuitively, what the researcher believes in (or is trying to prove) is called the "alternate" hypothesis, and the opposite ...
hypothesis, something supposed or taken for granted, with the object of following out its consequences (Greek hypothesis, "a putting under," the Latin equivalent being suppositio ). Discussion with Kara Rogers of how the scientific model is used to test a hypothesis or represent a theory. Kara Rogers, senior biomedical sciences editor of ...
A hypothesis is a short statement that can be tested and gives a possible reason for a phenomenon or a possible link between two variables. In the setting of scientific research, a hypothesis is a tentative explanation or statement that can be proven wrong and is used to guide experiments and empirical research. Figure 1: What is Hypothesis.
There are 5 main steps in hypothesis testing: State your research hypothesis as a null hypothesis and alternate hypothesis (H o) and (H a or H 1 ). Collect data in a way designed to test the hypothesis. Perform an appropriate statistical test. Decide whether to reject or fail to reject your null hypothesis. Present the findings in your results ...
A hypothesis states your predictions about what your research will find. It is a tentative answer to your research question that has not yet been tested. For some research projects, you might have to write several hypotheses that address different aspects of your research question. A hypothesis is not just a guess — it should be based on ...
A research hypothesis can be defined as a specific, testable prediction about the anticipated results of a study. 2 Hypotheses help guide the research process and supplement the aim of the study. After several rounds of testing, hypotheses can help develop scientific theories. 3 Hypotheses are often written as if-then statements.
hypothesis: [noun] an assumption or concession made for the sake of argument. an interpretation of a practical situation or condition taken as the ground for action.
A hypothesis is often called an "educated guess," but this is an oversimplification. An example of a hypothesis would be: "If snake species A and B compete for the same resources, and if we ...
hypothesis: 1 n a tentative insight into the natural world; a concept that is not yet verified but that if true would explain certain facts or phenomena "a scientific hypothesis that survives experimental testing becomes a scientific theory" Synonyms: possibility , theory Types: show 17 types... hide 17 types... hypothetical a hypothetical ...
The development of the research question, including a supportive hypothesis and objectives, is a necessary key step in producing clinically relevant results to be used in evidence-based practice. A well-defined and specific research question is more likely to help guide us in making decisions about study design and population and subsequently ...
As a case study for our proposed YAI for education (YAI4Edu, for short), we considered a teaching scenario where the excerpts of a textbook, "United States Legal Language and Culture: An Introduction to the U.S. Common Law System" (Brostoff & Sinsheimer, 2013), together with the encyclopedia of the Legal Information Institute of the Cornell Law School and thousands of cases of the Board of ...
Definition. The Riemann zeta function, denoted by , is defined as: (1) where is a complex variable with a real part greater than 1, i.e. . For it reduces to the harmonic series, which diverges. The Riemann zeta function converges absolutely for all complex numbers with a real part greater than 1.
A new study shows people who are in the habit of climbing stairs are less likely to die from heart disease compared to those who don't. Stair climbers also had a slight boost in longevity.
Existing X-ray based pre-trained vision models are usually conducted on a relatively small-scale dataset (less than 500k samples) with limited resolution (e.g., 224 $\\times$ 224). However, the key to the success of self-supervised pre-training large models lies in massive training data, and maintaining high resolution in the field of X-ray images is the guarantee of effective solutions to ...