Accessibility Links
- Skip to content
- Skip to search IOPscience
- Skip to Journals list
- Accessibility help
- Accessibility Help
Click here to close this panel.
Purpose-led Publishing is a coalition of three not-for-profit publishers in the field of physical sciences: AIP Publishing, the American Physical Society and IOP Publishing.
Together, as publishers that will always put purpose above profit, we have defined a set of industry standards that underpin high-quality, ethical scholarly communications.
We are proudly declaring that science is our only shareholder.

Ganga River: A Paradox of Purity and Pollution in India due to Unethical Practice
D C Jhariya 1 and Anoop Kumar Tiwari 2
Published under licence by IOP Publishing Ltd IOP Conference Series: Earth and Environmental Science , Volume 597 , National Conference on Challenges in Groundwater Development and Management 6-7 March 2020, NIT Raipur, India Citation D C Jhariya and Anoop Kumar Tiwari 2020 IOP Conf. Ser.: Earth Environ. Sci. 597 012023 DOI 10.1088/1755-1315/597/1/012023
Article metrics
1879 Total downloads
Share this article
Author e-mails.
Author affiliations
1 Assistant Professor, Department of Applied Geology, National Institute of Technology Raipur, Chhattisgarh-492010, India
2 Assistant Professor, Department of Humanities and Social Sciences, National Institute of Technology Raipur, Chhattisgarh-492010, India
Buy this article in print
In India, the river Ganga is believed as a goddess, and people worship it. Despite all the respect for the river, the river's condition is worsening, and we Indians are unable to maintain the purity of the river. The Ganga is a river of faith, devotion, and worship. Indians accept its water as "holy," which is known for its "curative" properties. The river is not limited to these beliefs but is also a significant water source, working as the life-supporting system for Indians since ancient times. The Ganga river and its tributaries come from cold, Himalayan-glacier-fed springs, which are pure and unpolluted. But when the river flows downgradient, it meets the highly populated cities before merging into the Bay of Bengal. From its origin to its fall, its water changes from crystal clear to trash-and sewage-infested sludge. Thousands of years passed since the river Ganga, and its tributaries provide substantial, divine, and cultural nourishment to millions of people living in the basin. Nowadays, with the increasing urbanization, the Ganges basin sustains more than 40 percent of the population. Due to the significant contribution of the growing population and rapid industrialization along its banks, river Ganga has reached an alarming pollution level.
Export citation and abstract BibTeX RIS
Content from this work may be used under the terms of the Creative Commons Attribution 3.0 licence . Any further distribution of this work must maintain attribution to the author(s) and the title of the work, journal citation and DOI.
Ganga water pollution: A potential health threat to inhabitants of Ganga basin
Affiliations.
- 1 Plant Ecology and Environmental Science Division, CSIR-National Botanical Research Institute, Rana Pratap Marg, Lucknow 226001, India.
- 2 Plant Ecology and Environmental Science Division, CSIR-National Botanical Research Institute, Rana Pratap Marg, Lucknow 226001, India. Electronic address: [email protected].
- PMID: 29783191
- DOI: 10.1016/j.envint.2018.05.015
Background: The water quality of Ganga, the largest river in Indian sub-continent and life line to hundreds of million people, has severely deteriorated. Studies have indicated the presence of high level of carcinogenic elements in Ganga water.
Objectives: We performed extensive review of sources and level of organic, inorganic pollution and microbial contamination in Ganga water to evaluate changes in the level of various pollutants in the recent decade in comparison to the past and potential health risk for the population through consumption of toxicant tainted fishes in Ganga basin.
Methods: A systematic search through databases, specific websites and reports of pollution regulatory agencies was conducted. The state wise level of contamination was tabulated along the Ganga river. We have discussed the major sources of various pollutants with particular focus on metal/metalloid and pesticide residues. Bioaccumulation of toxicants in fishes of Ganga water and potential health hazards to humans through consumption of tainted fishes was evaluated.
Results: The level of pesticides in Ganga water registered a drastic reduction in the last decade (i.e. after the establishment of National Ganga River Basin Authority (NGRBA) in 2009), still the levels of some organochlorines are beyond the permissible limits for drinking water. Conversely the inorganic pollutants, particularly carcinogenic elements have increased several folds. Microbial contamination has also significantly increased. Hazard quotient and hazard index indicated significant health risk due to metal/metalloid exposure through consumption of tainted fishes from Ganga. Target cancer risk assessment showed high carcinogenic risk from As, Cr, Ni and Pb as well as residues of DDT and HCHs.
Conclusion: Current data analysis showed that Ganga water quality is deteriorating day by day and at several places even in upper stretch of Ganga the water is not suitable for domestic uses. Although there is positive impact of ban on persistent pesticides with decreasing trend of pesticide residues in Ganga water, the increasing trend of trace and toxic elements is alarming and the prolong exposure to polluted Ganga water and/or consumption of Ganga water fishes may cause serious illness including cancer.
Copyright © 2018 Elsevier Ltd. All rights reserved.
Publication types
- Research Support, Non-U.S. Gov't
- Environmental Monitoring*
- Metals, Heavy / analysis
- Pesticide Residues / analysis
- Water Pollutants, Chemical / analysis*
- Water Pollution / analysis*
- Water Quality*
- Metals, Heavy
- Pesticide Residues
- Water Pollutants, Chemical

An official website of the United States government
The .gov means it’s official. Federal government websites often end in .gov or .mil. Before sharing sensitive information, make sure you’re on a federal government site.
The site is secure. The https:// ensures that you are connecting to the official website and that any information you provide is encrypted and transmitted securely.
- Publications
- Account settings
Preview improvements coming to the PMC website in October 2024. Learn More or Try it out now .
- Advanced Search
- Journal List
- Elsevier - PMC COVID-19 Collection

Cleaning the River Ganga: Impact of lockdown on water quality and future implications on river rejuvenation strategies
Associated data.
Clean rivers and healthy aquatic life symbolize that the ecosystem is functioning well. The Ganga River has shown signs of rejuvenation and a significant improvement on many parameters, following the eight-week nationwide lockdown due to coronavirus pandemic. Since industrial units and commercial establishments were closed, water was not being lifted by them with a negligible discharge of industrial wastewater. It was observed that during the lockdown period most of the districts falling under the Ganga basin observed 60% excess rainfall than the normal, which led to increased discharge in the river, further contributing towards the dilution of pollutants. Further, data analysis of live storages in the Ganga Basin revealed that the storage during the beginning of the third phase of lockdown was almost double than the storage during the same period the previous year. Analysis of the storage data of the last ten years revealed that the storage till May 6, 2020 was 82.83% more than the average of the previous ten years, which meant that more water was available for the river during the lockdown period. The impact could be seen in terms of increased dissolved oxygen (DO) and reduced biological oxygen demand (BOD), Faecal coliform, Total coliform and nitrate (NO 3 -) concentration. A declining trend in nitrate concentration was observed in most of the locations due to limited industrial activities and reduction in agricultural run-off due to harvesting season. The gradual transformation in the quality of the water has given a sign of optimism from the point of restoration. Yet, it is believed that this improvement in water quality is ‘short-lived’ and quality would deteriorate once the normal industrial activities are resumed, indicating a strong influence of untreated commercial–industrial wastewater. The paper concludes that the river can be rejuvenated if issues of wastewater and adequate flow releases are addressed.
Graphical abstract

1. Introduction
The Ganga alluvial plain is one of the most densely populated regions and the largest groundwater repositories on the earth ( Misra, 2011 ; Pal et al., 2020 ). About 43% of the population of India lives in the Ganga basin that stretches over 860,000 km 2 covering 26.3% of the country's total geographical area ( Trivedi, 2010 ; FAO, 2019 ). The basin extends over the states of Uttarakhand, Himachal Pradesh, Haryana, Delhi, Uttar Pradesh, Bihar, Jharkhand, Rajasthan, Madhya Pradesh, Chhattisgarh and West Bengal.
In 2008, Ganga River was declared as the ‘National River’ of India. There are over 29 cities, 97 towns and thousands of villages along the banks of the Ganga River ( Bhutiani et al., 2016 ). The bio-geomorphological functions of River Ganga have been significantly modified by various large-scale anthropogenic factors such as fragmentation of river habitats, dams and barrages, discharge of industrial and domestic wastewater and intensive agriculture relying on chemical fertilizers, pesticides and insecticides ( Bhardwaj et al., 2010 ; Sinha et al., 2017 ). The major contributors to pollution are tanneries in Kanpur, distilleries, paper mills and sugar mills in the Yamuna, Kosi, Ramganga and Kali river catchments.
The nationwide lockdown to contain the spread of the novel coronavirus (COVID-19) in India was announced on March 25 till April 14, 2020 (Lockdown 1.0). It was further extended by 19 days till May 3, 2020 (Lockdown 2.0). The lockdown was again extended until May 17, 2020 (Lockdown 3.0). While aerosol levels over the Indo-Gangetic Plains reported a 20-year-low during the lockdown as per the satellite data on optical depth measurements published by NASA due to restrictions imposed on industries, surface and air transport ( NASA, 2020 ); the impact on water quality in the Ganga River was arguable. Various news reports, as well as social media posts, indicated that ‘ life seemed to be returning to the river ’ ( India Today, 2020 ). It was reported that the lockdown had improved the health of River Ganga, which many projects of the government could not do during the past two decades. The water quality of Ganga River had witnessed visual improvement since enforcement of the nationwide lockdown started on March 24, 2020 that has led to a reduction in discharge of industrial effluents into it. The lockdown was extended for more than seven weeks, with its 1.3 billion people instructed to stay home in view of the coronavirus outbreak. With people staying indoors and industries shut during the lockdown period, it is crucial to assess if the water quality in the Ganga River has indeed seen a significant improvement. The paper analyses the impact of lockdown on water quality of Ganga River, and its major tributary Yamuna in Delhi, India. The paper also discusses issues and challenges to understand the magnitude of contamination and source relations and potential ways to improve the water quality. The paper finally provides important implications for future restoration strategies on Ganga River and approaches for designing appropriate control measures and action plans for river basin management.
2. About the study area
The Ganga River has been regarded as one of the holiest and sacred rivers of the world that witnesses high cultural and religious tourism on its banks, along with a heavy influx of tourists. The river has some of the most culturally significant stretches along with its courses, such as Rishikesh, Haridwar, and Allahabad where millions of people take holy dips during special days. The Bhagirathi river is the source stream of Ganga which originates from Gangotri glacier in the Himalaya in the Uttarkashi district of Uttarakhand State in India at an elevation of 3892 m (12,770 ft). Many small streams characterized by steep valleys and bedrock channels such as Alaknanda, Dhauliganga, Pindar, Mandakini and Bhilangana join together in the headwaters of Ganga. Alaknanda river joins Bhagirathi at Deoprayag, and the combined stream acquires the name of Ganga. It traverses 2525 km through a diverse climatic regime before flowing into the Bay of Bengal ( Fig. 1 ). The entire course of the Ganga River in India can be divided into three stretches ( Fig. 2 ): (i) upper stretch from the origin at Gomukh to Haridwar covering 294 km; (ii) middle stretch from Haridwar to Varanasi covering 1082 km, and (iii) lower stretch from Varanasi to its delta in Gangasagar covering 1134 km.

The course of Ganga River in India along with its major tributaries.

Three major segments of the Ganga Basin.
Major Himalayan tributaries of the Ganga are Yamuna, Ghagra, Gandak and Kosi which supply the majority of the water to the plains ( van der Vat et al., 2019 ). The river flow exhibits a marked seasonality with average monsoon season discharge 6 to 7 times higher than the average dry season discharge ( Singh and Pandey, 2019 ).
There has been a decline in fish catch along the river, suggesting a lack of supportive habitat and degradation of water quality. Destructive fishing, overfishing and the Farakka barrage were cited by fishers as the major causes of declines in fish catch from river–floodplain fisheries in Bihar ( Dey et al., 2020 ). Due to less discharge during the summer season, priority species like Gangetic dolphin and Gharial find difficulty in movement and are confined to few fragmented habitats. Lack of sufficient depth and flow of water during lean season become the most restraining factor as only 38.7% of the river stretch has a depth of 4 m or above ( WII, 2017 ).
3. Methodological approach
To have a better understanding of the transformation in the quality of water in Ganga, this paper analyses historical data on water quality and compares with quality observed during the lockdown period based upon the real-time water monitoring data of the Central Pollution Control Board (CPCB) and various state pollution control boards. Rainfall data obtained from Hydromet Division, India Meteorological Department New Delhi is analyzed to estimate the long-term departure from the normal in the Ganga Basin during the lockdown period and its possible contribution to the improvement of water quality. Basin storage data of the Central Water Commission (CWC) of the last ten years is analyzed and compared with the storage during the lockdown period. Minimum environmental -flow profile of river Ganga at Rishikesh is established following the flow norms prescribed by the Government of India and in view of water abstraction upstream. Summary of data points and significant parameters used in the study is provided in Table 1 .
Data points and major parameters used in the study.
4. Flow obstructions and water abstractions
There are many dams and barrages on the main stem and tributaries of the Ganga River that have affected the natural flow regime and fragmented the habitat of aquatic wildlife, including the Gangetic dolphins, otters, wetland birds, freshwater turtles and fishes ( Fig. 3 ). Tehri dam has been built on the Bhagirathi river for hydropower generation and regulates water discharge during the lean seasons. The hydroelectric potential of the Ganga basin has been assessed as 20,711 MW. Out of the 142 identified schemes in the basin, projects with a total installed capacity of 4987 MW are in operation and projects with an installed capacity of about 1751 MW are in various stages of construction.

Dams, barrages and hydro-electric plants on Ganga upstream of Kanpur.
At Haridwar, Ganga opens to the Gangetic Plains, where a barrage (Bhimgowda barrage) diverts a large quantity of its waters into the Upper Ganga Canal (UGC), to provide water for irrigation. It is estimated that the discharge of UGC is about 297.5 cumec (m 3 /s), which runs through 272 miles of the main canal and about 4000 miles of distribution canal irrigating over 900,000 ha of agricultural land of Uttarakhand and Uttar Pradesh ( Acharya et al., 2016 ).
Further, about 76 km downstream of Haridwar, at Bijnore, another barrage diverts water into the Madhya Ganga Canal during monsoon months. At Narora, about 155 km downstream of Bijnor barrage, there is a further diversion of water into the Lower Ganga Canal. From the barrage at Kanpur, Ganga water is being diverted to meet the drinking water requirements.
About 492 major and medium irrigation projects divert a significant portion of water to the canals for irrigation. The majority of these projects are in Uttar Pradesh which has a canal network of about 74,000 km for irrigation ( Shah and Rajan, 2019 ). The data of diversion schemes and water abstractions reveal that about 30 diversions on the main stem of the Ganga River and tributaries divert 40 to 60% of the annual flow of Ganga for canal irrigation. This leaves a minimal volume of water to flow in the river during the remaining eight dry months. The flow has been severely affected in the recent past due to the over-abstraction of groundwater in the basin, which has a marked effect on water quality of the river. If the dry season diversions from the main stem of Ganga is stopped, the base flows as the river enters Bihar would be at least 25% higher ( Khan et al., 2014 ).
5. Environmental flow (e-flow) requirements and the prescribed flow standards
Due to increased abstraction from the river and flow impoundments, very less amount of water is left in the river channel, which adversely affects the natural self-purification process of the river. The stretch between Haridwar and Allahabad has the problem of low flows, especially from December to May. The river gets less water for the dilution of pollutants coming from domestic and industrial sources, which ultimately make the water unfit for a healthy ecosystem. The sedimentation in the riverbed also increases. Recognizing the minimum ecological needs of the river, Clause 3.3 of India's National Water Policy 2012 specifies that “a portion of river flows should be kept aside to meet ecological needs ensuring that the low and high releases are proportional to the natural flow regime, including base flow contribution in the low flow season through regulated groundwater uses” ( NWP, 2012 ).
The National Green Tribunal (NGT) issued an order that requires all the riparian states to maintain a minimum 15% to 20% of the average lean season flow in all the rivers of the country (O.A. No.498/2015-Pushp Saini versus Ministry of Environment, Forests and Climate Change & Others dated 9.8.2017). However, the lean season flow is the lowest in the seasonal flow cycle of a river, and allocating only 15 to 20% will not be sufficient. The NGT has issued direction “ that as an interim measure , while diverting the water from Haridwar to the Ganga canal or even otherwise , the minimum e - flow in the main stem does not fall below 20 % of the average monthly lean season flow , which will be referred to the status of the river at Haridwar pre - diversion ” (NGT, order dated 13.7.2017 in the matter of O.A. 2000/2014-M.C. Mehta Vs. Union of Indian and Others).
Earlier, the expert appraisal committee for sanctioning of the river valley projects used to recommend 20% of average lean flow (average discharge of 4 leanest months) in 90% dependable year as environment flow in the river. The consortium of seven Indian Institute of Technology (IITs) recommended more than one-third of the average virgin flows of the river in the wet period and more than 40% of the dry period ( Consortium of 7 IITs, 2013 ). The Government of India (GoI) notified the minimum e-flows for Ganga River in October 2018 ( PIB, 2018 ) that are required to be maintained at various locations, which have been categorized into two parts – (i) e-flow for the upper stretch of the Ganga till Haridwar ( Table 2 ); and (ii) e-flow for the river from Haridwar to Unnao ( Table 3 ). Those projects which are not meeting the stipulated e-flows have to ensure the desired flow norms within three years.
Mandated e-flow notified for Upper Ganga River Basin starting from originating glaciers and through respective confluences finally meeting at Devprayag up to Haridwar.
Mandated e-flow notified for the main stem of Ganga River from Haridwar, Uttarakhand to Unnao, Uttar Pradesh.
For example, required e-flows during November 11–20 ten daily periods shall be 20% of average inflows observed during ten daily periods between November 1–10. According to CWC (2020) , at least four hydropower projects failed to comply with minimum e-flow requirements in the upper stretch of the Ganga river basin.
However, it is not clear on what basis the e-flow norms were calculated, as the flow volumes are too small for adequate river functions. The notification does not consider flow requirements to proliferate the aquatic biodiversity of the river. The standards for e-flows are too low to ensure a healthy river system ( Fig. 4 ). These arbitrary and generic e-flow interpretations lack the spatio-temporal resolution required to maintain a healthy aquatic life in the river. In developing the standards, location of the hot-spots, requirements of aquatic species, and river-specific studies have not been taken into account. The current standards are so low that minimum depth requirements of priority species such as endangered Gangetic dolphins will not be met on the main stem of the Ganga. The e-flow norms on the remaining sections of the Ganga downstream of Kanpur and for other tributaries have still not been defined. The recommended e-flow norms are just 5 to 8% of the mean annual run-off (MAR) of the Ganga River, whereas, at least 30 to 50% of the annual flow is recommended for healthy river functions ( Dutta et al., 2020 ).

Minimum e-flow profile of river Ganga at Rishikesh as per the CWC (2020) status report, the low flow allocation is ‘residual’ and will not support a healthy ecosystem.
6. Major hot spots of water pollution
Many cities and towns located in the catchment of Ganga generate vast quantities of wastewater, a major portion of which ultimately reaches the river untreated or partially treated through the natural drainage system. In the upper hilly stretches up to Rishikesh, the water quality is good throughout the year except for sediments. It is from Rishikesh onwards, disposal of sewage into Ganga begins. Downstream of Haridwar, the water quality starts declining due to the discharge of domestic and industrial wastewater; nevertheless, the river is intensively used in its entire stretch for holy dips or bathing by a large number of people.
There are many polluting industries such as paper and pulp, sugar, fertilizers, textiles, automobiles and distilleries along the tributaries of Ganga such as Yamuna, Kali, Hindon and Ramganga rivers that contribute to the pollution load in the Ganga. The number of Grossly Polluting Industries (GPIs) in April 2019 were 1072 (Namami Gange, 2020 ). The main water quality issues are organic pollution indicated by BOD and pathogens indicated by coliform count, which are recorded much above the critical limit prescribed for ‘outdoor bathing’ as the designated best use. The water quality in terms of Faecal coliform (FC) count has been poor virtually all along the river downstream of Haridwar due to the discharge of domestic sewage. According to Trivedi (2010) , the amount of industrial wastewater by volume is about 20% of the total volume of wastewater generated in the Ganga Basin, out of which nearly 55% comes from Uttar Pradesh. An earlier study indicated that the worst affected stretch is 350 km long between Kannauj and Allahabad in Uttar Pradesh ( Trivedi, 2010 ). Sewage from Kanpur coupled with untreated toxic waste discharge from about 400 industrial units, many of them tanneries, results in severe deterioration of water quality.
About 3250 million liters per day (MLD) of sewage is generated by the 97 towns situated on the main stem of the Ganga River, approximately 20% of this comes from industrial and commercial sources. Against this, 2074 MLD is treated and the remaining 1176 MLD is discharged without any treatment. National Mission for Clean Ganga has sanctioned projects for creation of an additional 1240 MLD which will increase the capacity to 3314 MLD, and these projects are at various stages of implementation ( Fig. 5 ).

Status of existing sewage generation and treatment capacity in 97 towns along the main stem of the Ganga River.
Due to high BOD and FC in the river, along the major cities of Kanpur, Allahabad and Varanasi, the river is not fit for its designated best use of ‘outdoor bathing’. The river along the urban segments receives a large amount of treated and untreated wastewater. Sah et al. (2020) observed the presence of 13 banned and restricted organochlorine pesticides (OCPs) in the surface water along the Ganga River with lower stretch most contaminated posing highest ecological risk. Lindane (γ-HCH) was found to be the most dominant and frequently detected pesticide, indicating continued use of this pesticide in agricultural practices even after banning. In the urban stretch of Varanasi, the concentration of heavy metals in the river sediment was found highest for Fe followed by Mn, Zn, Cr, Cu, Ni, Pb, and Cd as compared to upstream and downstream stretches suggesting greater contamination in anthropogenic impacted river stretches ( Pandey and Singh, 2017 ).
7. Impact of lockdown on water quality
The notable level of improvement in water quality was due to the absence of industrial pollutants and reduction in the amount of solid waste that spanned for eight weeks. While the discharge of domestic sewerage has not reduced in this period, industrial effluent has nearly ended, which provided temporary improvements to the water quality. Amid the nationwide lockdown to contain the spread of the Covid-19 outbreak, the water quality of river Ganga at Haridwar was classified as ‘fit for drinking’ as per the report of Uttarakhand Pollution Control Board. The sections of media described it as an unprecedented success that the ambitious schemes of the government could not do for years, even after spending a significant amount of money. Due to the lockdown, water in Har-ki-Pauri, Haridwar ranked as Class A for the first time in the last two decades. The water had always been placed in Class B since the state of Uttarakhand was formed in the year 2000. Earlier, the river water was not found to be suitable for bathing at most of the monitoring centers along the river, except the upper stretch till Haridwar ( Kamboj and Kamboj, 2019 ). The quality of water between Rishikesh to Haridwar in Uttarakhand was fit for drinking with conventional treatment (Class A) as DO, BOD and TC level were within the prescribed water quality criteria. Improvement in the water quality was also observed between Haridwar and Kanpur which was fit outdoor bathing (Class B). A noticeable improvement was observed during the lockdown phase along the entire stretch of the river, specially upstream of Kanpur ( Fig. 6 ).

Improvement in the water quality in the Ganga River upstream of Kanpur, stretch up to Haridwar was fit for dirking after disinfection (Class A) whereas water downstream of Haridwar was fit for outdoor bathing (Class B).
Since most of the factories and commercial establishments were closed due to the lockdown, the Ganga River had become comparatively cleaner. However, the assessment of data shows that there was not much improvement in the organic load in the river as domestic discharges witnessed no change after lockdown.
The DO concentrations remained above 5 mg/L at all the locations which met the bathing criteria ( Fig. 7 ). The impact is attributed to the combined effect of reduced release of industrial wastewater and increased freshwater inflows due to excessive rainfall observed during the lockdown. Water-intensive agriculture was not practiced in the northern plains of the Ganga basin during the lockdown, as the period was harvesting season. Therefore, huge extraction of water for irrigation was avoided both from the canal network and the groundwater aquifers. The additional water could also be the source of dilution. This increasing value of DO may also be attributed to a reduction in wastes from various non-point sources. The suspended solids and turbidity in the river increased immediately after the lockdown due to heavy rains.

Trend in the observed DO (mg/L) values in the main stem of the Ganga River during 2019 (pre-lockdown) and 2020 (lockdown), showing increase in DO during the lockdown period.
There was no major reduction in BOD at most of the monitoring stations during the first three weeks, though lower BOD values were observed during fourth week as compared to previous weeks ( Fig. 8 ). A comparative assessment of BOD (mg/L) of Ganga River during the three months-period in 2019 (pre-lockdown average of March to May) and 2020 (lockdown average of March to May), shows decrease in BOD during the lockdown period, except for two stations ( Fig. 9 ).

Trend in the observed BOD (mg/L) values in the main stem of the Ganga River.

Comparative assessment of BOD (mg/L) of Ganga River during 2019 (pre-lockdown) and 2020 (lockdown), showing decrease in BOD during the lockdown period, except two stations.
There has been marginal reduction in COD values during the first four weeks of the lockdown period ( Fig. 10 ), which can be attributed to continued wastewater discharge from municipal sources and secondly, due to longer time requirements for COD reduction as compared to BOD.

Trend in the observed COD (mg/L) values in the main stem of the Ganga River.
A declining trend in nitrate concentration was observed in most of the locations due to limited industrial activities and reduction in agricultural run-off during the lockdown ( Fig. 11 ). Nitrate level on an average varied from 0.69 to 2 mg/L during the beginning of third phase of lockdown.

Trend in the observed values of Nitrate (NO 3 -) in the main stem of the Ganga River.
All the locations except Bijnore have shown increasing trends in the observed values of Ammoniacal nitrogen in both weeks of the lockdown ( Fig. 12 ). The reason could be the increased discharge of the untreated and partially treated wastewater from the municipal sewage and slower rate of dilution during the first phase of the lockdown. However, during the beginning of the third phase of the lockdown, all the locations showed Ammoniacal nitrogen less than the prescribed criteria of 1.2 mg/L limit.

Trend in the observed values of Ammoniacal nitrogen (NH 3 −N) in the main stem of the Ganga River.
There was also improvement in the bacteriological quality of the Ganga River during the lockdown period. Most of the stations recorded large reductions in the Total coliform and Faecal coliform counts ( Table 4 ).
Comparative assessment of Total coliform (MPN/100 mL) and Faecal coliform (MPN/100 mL) in the main stem of the Ganga River during 2019 (pre-lockdown) and 2020 (lockdown period), (mean ± SD).
NA: data not available.
The improvement in the quality of water has also been observed in the most polluted stretch of Ganga's major tributary – Yamuna River in Delhi during April 2020 as compared to the previous year ( Fig. 13 ). Delhi covers only 0.2% of the Yamuna sub-basin in Ganga basin, but it impacts badly due to discharge of untreated municipal wastewater. BOD and COD values increased during the lockdown for first three sampling sites, the remaining sites showed decreasing trend. However, the values were still very high – rendering the water only suitable for propagation of wildlife and fisheries (Class D) and for irrigation and industrial cooling (Class E) ( Table 5 ).

BOD and COD values (mg/L) of River Yamuna in Delhi in April 2019 and 2020.
Average concentration of contaminants in the Yamuna river at different locations (January to April 2020) (mean ± SD).
Note: Nil means DO value is zero in all four months; data obtained from Delhi Pollution Control Committee, Delhi.
According to the data from real-time water monitoring of the CPCB, the water quality at 27 monitoring stations was suitable for bathing (Class B) and 9 stations suitable for propagation of wildlife and fisheries (Class C), out of the 36 monitoring stations placed on the main channel of the Ganga River. Earlier, except upper stretches in Uttarakhand and a couple of places in Uttar Pradesh, the water quality was found to be unfit for bathing for the remaining stretch till it merged into the Bay of Bengal. It is important to mention here that such speedy improvement spanning almost the entire stretch had not been witnessed in the past three to four decades. The data from various monitoring stations clearly show an increasing trend in DO values of the river at most of the locations during the second and third week since lockdown started ( Table 6 ).
Trends in observed quality of water during lockdown on the main stream of the River Ganga based on real time water quality data.
8. Reasons for the improvement of water quality in Ganga during the lockdown period
8.1. higher rainfall events.
The period also coincided with a high number of western disturbances, which brought excess rainfall in the basin, improving the flow in the river leading to dilution of the pollutants. Analysis of rainfall data obtained from Hydromet Division, India Meteorological Department New Delhi indicated that from March 1 to May 6, 2020 most of the districts falling under the Ganga basin observed 60% excess rainfall than the normal, which led to increased discharge in the river, further contributing towards the dilution of pollutants ( Fig. 14 ).

Rainfall in the Ganga Basin showing large excess (60% above the normal) which contributed to higher storage and discharge.
8.2. Increase in the surface storages in the basin
Snowmelt after April contributes a significant amount of water to the river. The last two winters of 2018–19 and 2019–20 have seen plentiful snowfall in Uttarakhand, in 2019 the state received six times more snowfall than it did in 2018. During the start of 2020, the state recorded almost 17 in. of snowfall ( Singh, 2020 ). During the last two winter seasons, significant snowfall events improved the snow cover on the glaciers. It was after 15 years that regions at an altitude of 2000 m witnessed snowfall. This increase in snowfall had a beneficial impact on reservoir storage and flow in the river. Storage on 6th May 2020 was 49.27%, which was almost double than the storage during the previous year (25.89%). Analyzing the data of the last ten years, the storage till May 6th, 2020 was 82.83% more than the average of previous 10 years ( Table 7 ).
Storage in the Ganga River Basin based on weekly storages and percentage departure with respect to last year and previous 10 years (data period 2 January, 2020 to 6 May, 2020).
8.3. Increase in baseflow due to harvesting season
In the Ganga Basin, the eight weeks of lockdown period coincided with the harvesting season; therefore, the agriculture sector was also not withdrawing much water. As the abstraction of groundwater in the Ganga Basin is very high, it affects the baseflow in the river ( Maheswaran et al., 2016 ). Due to unabated long term groundwater extraction, a sharp decrease in critical dry weather baseflow contributions has been observed ( MacDonald et al., 2016 ; de Graaf et al., 2019 ). The surge in groundwater extraction coincides with the demand for dry season irrigation for intensive agriculture. The baseflows are diverted for meeting the demand from irrigation ( Jain, 2015 ). It has been observed that when groundwater levels decline, discharges from groundwater to streams also decline ( Sharma and Dutta, 2020 ), which decreases streamflow, with potentially distressing effects on the health of the aquatic ecosystems. Apart from groundwater, over 40% of the annual flow of Ganga till Kanpur is diverted for canal irrigation. Since there was no sowing of crops or irrigation requirements, the amount of diversion also declined.
8.4. Reduction in the discharge of wastewater from industrial and commercial units
Almost zero industrial pollution due to complete lockdown increased the quality of water in the Ganga River. The water quality improved significantly at Kanpur, Varanasi and Allahabad since the enforcement of the lockdown, especially around the industrial clusters. This indicates that industrial effluents were not being adequately treated before being discharged into the river. The wastewater discharged into the Ganga basin ranged between 6500 and 6700 MLD in its middle and downstream stretch, of which around 20% was toxic load from industries. Therefore, there was a reduction of about 1300 to 1340 MLD of industrial wastewater during the lockdown period. When sewage is mixed with industrial effluents, it negatively affects the self-cleaning abilities of the stream. The organic pollution level from domestic sewage gets diluted in the river comparatively at a faster rate than the inorganic pollution ( Bhaskar et al., 2020 ). Still, it is the chemical pollution by industries (high COD) that destroys the river's self-cleaning properties in a big way due to complex nature of pollutants. The self-cleaning properties had improved due to which the water quality also improved.
8.5. Decrease in electricity demand
The daily requirement for electricity dipped by a minimum 15% across the globe based upon data from 30 countries on account of the shutting of industrial and commercial operations during lockdown ( IEA, 2020 ). In India, according to the Power System Operation Corporation, electricity production fell by 32.2% to 1.91 billion units (kilowatt-hours) per day, compared to the 2019 levels ( DTE, 2020 ). As per the statistics of all India installed capacity of power stations during and of March 2020, hydropower constituted about 23.01% of the total power production ( CEA, 2020 ). There are 39 hydro-electric projects in the Ganga basin in India, out of which 27 are major, and 12 are small hydro-electric projects. Due to a reduction in electricity demand, hydropower production may have dipped, allowing more water releases to the river. The water in Yamuna increased to 3900 cusec in April 2020 as compared to 1000 cusec in April 2019. This has allowed significant dilution to the pollutants coming from various drains.
8.6. Complete restriction on other activities such as tourism, fairs, bathing and cloth washing
As the public were not allowed to congregate for religious activities and fair, it reduced the local impacts of solid waste coming to the river. The activities near the ghats (river banks) were curtailed. River during lockdown was free from the problems of solid waste dumping and littering along its banks by visitors. The visual perception and aesthetics of river banks and accessible ghats along major cities improved due to the public not accessing the river for rituals and bathing.
9. Impact of infrastructure put up by national schemes and action plans
Significant improvement has been seen around Ganga in Kanpur, an industrial town, from where a large volume of industrial waste is generated and discharged into the rivers. In Kanpur, the Sisamau drain which used to discharge about 183.29 MLD of untreated water into the river was stopped in 2019 under the Namami Gange project ( Fig. 15 ). This has brought down the water pollution considerably during the lockdown pweriod. About 16 large drains used to discharge untreated wastewater in Kanpur, out of which 8 drains have been tapped and diverted to sewage treatment plant (STP) till December 2019.

Sisamau drain – the biggest source of untreated municipal wastewater (183.29 MLD discharge rate) in the Ganga River in the heavily polluted urban segment of Kanpur City, A: before lockdown (2019) B: during lockdown (2020).
A total of 254 projects worth Rs. 246,720 million have been sanctioned under Namami Gange programme for sewage infrastructure, river banks and crematoria development, riverfront development, river surface cleaning, biodiversity conservation, afforestation, rural sanitation, and public participation ( PIB, 2018a ). There are about 63 sewerage management projects which are under implementation, while 12 new sewerage projects were completed before the lockdown started. About 30 new STPs in Uttarakhand have been installed. Three STPs of total treatment capacity of 38.5 MLD started functioning at Rishikesh upstream of Haridwar from March 2020. A 14 MLD STP was also completed at Haridwar in July 2019. Till April 2019, 1930 MLD of sewerage treatment capacity in 97 towns has been developed, whereas the sewerage generation in these towns is 2953 MLD ( Dutta, 2020 ). In view of the current treatment capacity and wastewater generation, the gap in treatment capacity in the riparian states is very large which amounts to 6321 MLD ( Fig. 16 ). The various sanctioned projects would create additional 2205 MLD sewage treatment capacity and 4762 km of sewerage network in addition to rehabilitating older STPs of 564 MLD capacity.

Sewage generation, treatment capacity and shortfall in sewage treatment in the major riparian states of the Ganga Basin.
There have been several ambitious projects to clean the Ganga river, from Ganga Action Plan (GAP) – Phase I, and II to the Namami Gange project ( Table 8 ). The previous projects have yielded sub-optimal results, mainly due to (a) insufficient capacity of STPs as effluents from municipalities and industries flew untreated into the river, contaminating it and making the water unfit; (b) the amount of wastewater from non-point sources such as agricultural run-off is challenging to check. It is also due to the fact that, efforts of expanding sewage treatment infrastructure to achieve the target of cleaner Ganga were not adequate in comparison to the fast rate of urbanization. Water scarcity resulting from over-abstraction of surface and groundwater in the basin due to the rapid increase in water demand also exacerbates the problem.
River rejuvenation schemes to clean River Ganga and their shortcoming.
GAP was launched by Government of India in 1985 to assist the urban local bodies to install sewage treatment plants in the 27 priority towns along the Ganga River to restore its water quality. Under GAP, 1098.31 MLD sewage treatment capacities were created. In Phase-I of the GAP, a total treatment capacity of 870 MLD was created (Ministry of Environment and Forests, Government of India, 2009, http://www.envfor.nic.in/nrcd ). Subsequently, during Phase-II of the GAP, an additional capacity of 130 MLD was created in 48 smaller towns along the river. Similarly, a treatment capacity of 720 MLD was created under the Yamuna Action Plan, (YAP) and a capacity of 2330 MLD was created by Government of Delhi for the restoration of water quality in the Yamuna River. About 84 STPs having a combined treatment capacity of 1579 MLD were constructed under GAP – I and II, NGRBA, and state projects along the main stem of river Ganga. Various studies reported that many of these STPs are (a) underutilized as per design standards ; (b) non - functional and (c) do not meet the quality standards suggested by the regulatory bodies . Central Pollution Control Board (CPCB) initiated a project called Pollution Inventorization Assessment and Surveillance (PIAS) to assess the functioning and treatment efficiencies of 67 STPs located on the main stem of Ganga River. These STPs were having a total treatment capacity of 1209 MLD. As per their findings, only 32 STPs with a capacity of 675.7 MLD were observed to be functional.
Due to the paucity of sufficient financial resources to run the treatment plants created under GAP or YAP, and due to technical and operational disruptions, there has been no marked impact on improvement in water quality of the rivers ( Trivedi, 2010 ). India's National Green Tribunal (NGT) in its judgment order (O.A. No. 200/2014 in the matter of M.C. Mehta vs. Union of India for Segment B, Phase I dated 13th July 2017) observed that “ even after spending Rs . 7304.64 crore up to March 2017 , by the Central , State Government and local authorities of the State of UP , the status of river Ganga has not improved in terms of quality or otherwise and it continues to be a serious environmental issue ” ( Bhagirath, 2017 ).
After GAP Phase I and II, the GoI intensified its efforts for pollution abatement of river Ganga through various projects, mainly targeting at the treatment of municipal sewage, industrial effluents, river surface cleaning, rural sanitation, afforestation and biodiversity conservation. Realizing the shortcoming of GAP I and II, NMCG sanctioned projects for up-gradation and rehabilitation of 23 existing STPs. These projects are centered around cities located on the banks of the Ganga and heavily depend upon the construction of sewerage networks, interception and diversion projects and development of STPs.
10. Key lessons from the lockdown and future perspectives
The population in the Ganga basin will increase in the future with an increase in industries and urban settlements. This is expected to generate a huge demand for additional water. As the water in the main channel and its various tributaries are limited, the substantial increase in demand would further deteriorate the water quality. It is worthwhile to examine key lessons that the pandemic signaled for river management – most importantly, river can be rejuvenated if issues of industrial effluents and adequate flow releases are addressed. The six major recommendations from the lockdown period are outlined below as crucial lessons for developing future perspectives on river rejuvenation.
10.1. Requirement of more stringent regulatory controls
Strict quality regulation and enforcement are required to check the incompliance related to wastewater treatment and discharge. The state pollution control boards are not equipped to handle this and a new system may be devised. There is a need for third-party compliance verification against stipulated environmental norms for existing STPs and industries to bring in more efficiency and transparency.
10.2. Reducing the burden of water abstraction
It is clear from the flow profile that lean season flows in the basin will not be sufficient to meet the human demands and, at the same time, fulfill the ecological requirements. Even though the UGC and LGC are relatively large irrigation systems, irrigation in the Ganga basin today depends on tubewells far more than canals ( Shah and Rajan, 2019 ). A multipronged strategy is required to optimally manage the old canal network and storages in order to maximize water use efficiency and irrigation benefits. This would improve the dry season river flows.
10.3. Increasing the speed of project implementation
It has been observed that there is a slow implementation of projects sanctioned by NMCG, with many projects still in the conceptual and planning stage ( CAG, 2017 ). Monitoring and evaluation mechanisms of ongoing and completed projects have been far from adequate.
10.4. Redefining the standards for industries in view of the impact on water quality
The river entering a watershed boundary should leave with at least the same quality of water as it entered with. The wastewater must be treated up to freshwater levels before putting it back into the river. The volume-wise contribution of industrial pollution in Ganga is about 20%, but due to toxicity and high inorganic impurities, this has much more significant damage on the aquatic ecosystem. The industries are allowed based on meeting the required standards and compliances, but the level of pollution is high. In view of this, it is desirable to revisit the prescribed standards and make suitable amendments.
10.5. Moving to ecological flow regimes from the current conservative e-flow estimates
The current e-flow norms are treated as residual, which is insufficient to meet the requirements of the aquatic ecosystem. Several water abstraction, diversion, and storage projects have been designed on the Ganga River without looking at the needs of supporting its own ecosystem. Excessive water abstraction coupled with pollution ingress not only hampers aquatic life but also diminishes river's self-purification and dilution prospects. It appears that e-flow norms have been developed as a reference to water only; the other critical aspects such as sediment transport, biota and nutrients have been ignored. The holistic flow regime of adequate magnitude, timing, frequency and duration are required to sustain aquatic ecosystems. This would also sustainably ensure other supporting services by the river, such as sedimentation, flooding, river landscape and connected water bodies.
10.6. Designing different strategies for hot-spots and grossly polluting industries (GPIs)
The primary cause of water pollution in Kanpur and the catchment of Yamuna river is the discharge of untreated and partially treated toxic industrial waste mainly from tanneries, paper and pulp industries, electroplating and distilleries which is discharged into the river. As of April 2019, the number of GPI stood at 1072 (Namami Gange, 2020 ). Therefore, stringent actions are required for checking the pollution form these hot-spots and GPIs.
11. Conclusion
During the lockdown, all major polluting industries were closed; the toxic load was off the river. The improvement in water quality has been seen especially around the industrial clusters and urban areas, which used to witness huge pollution load due to the discharge of untreated and partially treated wastewater. The contributing factor of municipal sewage generation and treatment capacities remained the same since commissioned STPs were running as they used to run before the lockdown period. The lockdown period also witnessed large rainfall events resulting in more flow in the river with better prospects of dilution of pollutants. The way the quality of water has improved in the river during the lockdown, it is evident that the problem of water quality deterioration is largely anthropogenic – (i) stemming from discharge of untreated or partially treated effluents from industries, commercial establishments and municipalities; and (ii) reduced dilution prospects due to over-allocation to canals. The findings also reflected that domestic sewerage was not the only cause of concern; adequate flow is crucial for dilution of the pollutants. During the lockdown, industrial activities were stopped and the production of essential items was allowed after four weeks since lockdown began. There was definitely less effluent generation and discharge. However, it also indicated poor implementation of environmental regulation from various central and state regulatory bodies. In the past several elaborate plans with the allocation of budgets were made to clean the river. But there was no apparent improvement in the health of the river. The improvement in water quality is a temporary reprieve as major schemes to regulate the grossly polluting industries in the river's catchments are still awaited. There should be a rethink on the whole issue of river rejuvenation efforts—establishing the role of industrial discharges and the need for compliances of discharge standards. Various ambitious rejuvenation projects by multiple governments could not bring the desired results in such a short period. Keeping in mind the increasing rate of urbanization and pollution loading in the river, necessary measures should be taken to reduce future deterioration of water quality in the river. The challenge would be to keep the river in similar conditions post-lockdown, which can be possible with two times increases in the existing treatment capacity, stringent industrial pollution control measures and behavioral change to supplement infrastructure creation.
CRediT authorship contribution statement
Venkatesh Dutta: Conceptualization, Methodology, Writing - original draft. Divya Dubey: Data curation, Methodology. Saroj Kumar: Formal analysis, Visualization, Writing - review & editing.
Declaration of competing interest
The authors declare that they have no known competing financial interests or personal relationships that could have appeared to influence the work reported in this paper.
Appendix A Supplementary data to this article can be found online at https://doi.org/10.1016/j.scitotenv.2020.140756 .
Appendix A. Supplementary data
Variation in flow and organic load of priority drains during pre and post-monsoon in 2018 discharged into the Ganga River
Priority drains monitored during pre-monsoon, in 2018 discharged into the River Ganga
District-wise weekly departure in rainfall (cumulative) during March 1 to May 6, 2020
Storage in the Ganga River Basin based on weekly storages
Designated best uses of water quality criteria and Hydro projects under operation in the Upper Ganga Basin
Comparative assessment of water quality of Ganga River during 2019 (pre-lockdown) and 2020 (lockdown)
Water quality of Yamuna River in 2020 at nine locations
- Acharya S., Pandey A., Mishra S.K., Chaube U.C. GIS based graphical user interface for irrigation management. Water Sci. and Technol.: Water Supply. 2016; 16 :1536–1551. [ Google Scholar ]
- Bhagirath . vol.- LXIV (4) Central Water Commission; New Delhi: 2017. Water Resources in Parliament, Indian Water Resources Quarterly, October – December 2017. [ Google Scholar ]
- Bhardwaj V., Singh D.S., Singh A.K. Water quality of the Chhoti Gandak River using principal component analysis, Ganga Plain, India. J. Earth Syst. Sci. 2010; 119 :117–127. [ Google Scholar ]
- Bhaskar M., Dixit A.K., Ojha K.K., Dubey S., Singh A., Abhishek A. The impact of anthropogenic organic and inorganic pollutants on the Hasdeo River Water Quality in Korba Region, Chhattisgarh, India. Bioinformation. 2020; 16 (4):332–340. [ PMC free article ] [ PubMed ] [ Google Scholar ]
- Bhutiani R., Khanna D.R., Kulkarni D.B., Ruhela M. Assessment of Ganga river ecosystem at Haridwar, Uttarakhand, India with reference to water quality indices. Appl Water Sci. 2016; 6 :107–113. [ Google Scholar ]
- CAG . 2017. Rejuvenation of River Ganga (Namami Gange): Chapter 9, Monitoring and Evaluation, Comptroller and Auditor General of India. [ Google Scholar ]
- CEA All India Installed Capacity (in MW) of Power Stations. 2020. http://www.cea.nic.in/reports/monthly/installedcapacity/2020/installed_capacity-03.pdf available at.
- Consortium of 7 IITs . Indian Institute of Technology; Kanpur: 2013. Ganga River Basin Management Plan Interim Report, Report of the Consortium of 7 IITs. [ Google Scholar ]
- CWC . Upper Ganga Basin Organization, Central Water Commission; Government of India: 2020. Implementation of Minimum Environmental Flows in River Ganga, Status Report. [ Google Scholar ]
- de Graaf I.E., Gleeson T., van Beek L.R., Sutanudjaja E.H., Bierkens M.F. Environmental flow limits to global groundwater pumping. Nature. 2019; 574 :90–94. [ PubMed ] [ Google Scholar ]
- Dey S., Choudhary S.K., Dey S., Deshpande K., Kelkar N. Identifying potential causes of fish declines through local ecological knowledge of fishers in the Ganga River, eastern Bihar, India. Fisheries Manage. and Ecol. 2020; 27 :140–154. [ Google Scholar ]
- DTE COVID-19: daily electricity demand dips 15% globally, says report. 2020. https://www.downtoearth.org.in/news/energy/covid-19-daily-electricity-demand-dips-15-globally-says-report-70904 available at.
- Dutta V. Down to Earth, Centre for Science and Environment; 2020. 10 critical steps for Ganga revival. https://www.downtoearth.org.in/blog/water/10-critical-steps-for-ganga-revival-68482 available at.
- Dutta V., Sharma U., Kumar R. Environmental Concerns and Sustainable Development 2020. Springer; Singapore: 2020. Restoring environmental flows for managing river ecosystems: global scenario with special reference to India; pp. 163–183. [ Google Scholar ]
- FAO FAO Aquastat. 2019. http://www.fao.org/land-water/databases-and-software/aquastat/en/ Available online.
- Gange Namami. Industrial effluent monitoring. 2020. https://nmcg.nic.in/NamamiGanga.aspx available at.
- IEA . International Energy Agency; 2020. Global Energy Review 2020: The Impacts of the COVID-19 Crisis on Global Energy Demand and CO2 Emissions. (April 2020) [ Google Scholar ]
- India Today India Today; 2020. Lockdown impact: Ganga water in Haridwar becomes ‘fit to drink’ after decades. https://www.indiatoday.in/india/story/lockdown-impact-ganga-water-in-haridwar-becomes-fit-to-drink-after-decades-1669576-2020-04-22 April 22, 2020 available at.
- Jain S. Hydrologic inputs for Ganga rejuvenation, presentation at the Future Ganga Workshop. 2015. https://www.ceh.ac.uk/sites/default/files/Future%20Ganga%20Workshop%20-%20Sharad%20Jain%20-%20NIH.pdf available at.
- Kamboj N., Kamboj V. Water quality assessment using overall index of pollution in riverbed-mining area of Ganga-River Haridwar, India. Water Sci. 2019; 33 :65–74. [ Google Scholar ]
- Khan M.R., Voss C.I., Yu W., Michael H.A. Water resources management in the Ganges Basin: a comparison of three strategies for conjunctive use of groundwater and surface water. Water Res. Manage. 2014; 28 :1235–1250. [ Google Scholar ]
- MacDonald A.M., Bonsor H.C., Ahmed K.M., Burgess W.G., Basharat M., Calow R.C., Dixit A., Foster S.S., Gopal K., Lapworth D.J., Lark R.M. Groundwater quality and depletion in the Indo-Gangetic Basin mapped from in situ observations. Nat. Geosci. 2016; 9 :762–766. [ Google Scholar ]
- Maheswaran R., Khosa R., Gosain A.K., Lahari S., Sinha S.K., Chahar B.R., Dhanya C.T. Regional scale groundwater modelling study for Ganga River basin. J. Hydrol. 2016; 541 :727–741. [ Google Scholar ]
- Misra A.K. Impact of urbanization on the hydrology of Ganga Basin (India) Water Resour. Manag. 2011; 25 (2):705–719. [ Google Scholar ]
- NASA NASA, 2020. 2020. http://earthobservatory.nasa.gov/images
- NWP . Ministry of Water Resources; Government of India: 2012. National Water Policy. [ Google Scholar ]
- Pal S., Kundu S., Mahato S. Groundwater potential zones for sustainable management plans in a river basin of India and Bangladesh. J. Clean. Prod. 2020; 257 [ Google Scholar ]
- Pandey J., Singh R. Heavy metals in sediments of Ganga River: up-and downstream urban influences. Appl Water Sci. 2017; 7 :1669–1678. [ Google Scholar ]
- PIB Press Information Bureau, Government of India, Ministry of Water Resources; 2018. Nitin Gadkari says notification of E-flow for ganga is a significant step. https://pib.gov.in/newsite/PrintRelease.aspx?relid=184100 dated 10-October-2018 available at.
- PIB . Press Information Bureau; Government of India: 2018. Achievements of Ministry of Water Resources, River Development and Ganga Rejuvenation During 2018. https://pibindia.wordpress.com/2018/12/19/achievements-of-ministry-of-water-resources-river-development-and-ganga-rejuvenation-during-2018/#more-19520 available at. [ Google Scholar ]
- Sah R., Baroth A., Hussain S.A. First account of spatio-temporal analysis, historical trends, source apportionment and ecological risk assessment of banned organochlorine pesticides along the Ganga River. Environ. Pollut. 2020; 263 [ Google Scholar ]
- Shah T., Rajan A. Cleaning the ganga: rethinking irrigation is key. Econ. Polit. Wkly. 2019; 54 :57. [ Google Scholar ]
- Sharma U., Dutta V. Establishing environmental flows for intermittent tropical rivers: why hydrological methods are not adequate? Int. J. Environ. Sci. Technol. 2020; 17 :2949–2966. [ Google Scholar ]
- Singh V. 2020. Healthy Snowfall Will Help Uttarakhand’s Glaciers, Water Woes, Mongabay March 2020. [ Google Scholar ]
- Singh R., Pandey J. Non-point source-driven carbon and nutrient loading to Ganga River (India) Chem. Ecol. 2019; 35 (4):344–360. [ Google Scholar ]
- Sinha R., Mohanta H., Jain V., Tandon S.K. Geomorphic diversity as a river management tool and its application to the Ganga River, India. River Res. and Applica. 2017; 33 :1156–1176. [ Google Scholar ]
- Trivedi R.C. Water quality of the Ganga River—an overview. Aquatic Ecosystem Health & Management. 2010; 13 (4):347–351. [ Google Scholar ]
- van der Vat M., Boderie P., Bons K., Hegnauer M., Hendriksen G., van Oorschot M., Warren A. Participatory modelling of surface and groundwater to support strategic planning in the Ganga Basin in India. Water. 2019; 11 :2443. [ Google Scholar ]
- WII . Ganga Aqualife Conservation Monitoring Centre, Wildlife Institute of India; Dehradun: 2017. Aquatic Fauna of Ganga River: Status and Conservation. [ Google Scholar ]
Thank you for visiting nature.com. You are using a browser version with limited support for CSS. To obtain the best experience, we recommend you use a more up to date browser (or turn off compatibility mode in Internet Explorer). In the meantime, to ensure continued support, we are displaying the site without styles and JavaScript.
- View all journals
- My Account Login
- Explore content
- About the journal
- Publish with us
- Sign up for alerts
- Open access
- Published: 21 February 2022
Physicochemical and biological analysis of river Yamuna at Palla station from 2009 to 2019
- Pankaj Joshi 1 ,
- Akshansha Chauhan 2 ,
- Piyush Dua 3 ,
- Sudheer Malik 4 &
- Yuei-An Liou 2 , 5
Scientific Reports volume 12 , Article number: 2870 ( 2022 ) Cite this article
6857 Accesses
9 Citations
2 Altmetric
Metrics details
- Environmental sciences
Yamuna is one of the main tributaries of the river Ganga and passes through Delhi, the national capital of India. In the last few years, it is considered one of the most polluted rivers of India. We carried out the analysis for the physiochemical and biological conditions of the river Yamuna based on measurements acquired at Palla station, Delhi during 2009–19. For our analysis, we considered various physicochemical and biological parameters (Dissolved Oxygen (DO) Saturation, Biological Oxygen Demand (BOD), Chemical Oxygen Demand (COD), Total Alkalinity, Total Dissolved Solids (TDS), and Total Coliform. The water stats of river Yamuna at Palla station were matched with Water Standards of India, United Nations Economic Commission for Europe (UNECE), and World Health Organization (WHO). Maximum changes are observed in DO saturation and total coliform, while BOD and COD values are also seen higher than the upper limits. Total alkalinity rarely meets the minimum standards. TDS is found to be satisfactory as per the standard limit. The river quality falls under Class D or E (IS2296), Class III or IV (UNECE), and fails to fulfill WHO standards for water. After spending more than 130 million USD for the establishment of a large number of effluent treatment plants, sewage treatment plants, and common effluent treatment plants, increasing discharges of untreated sewage, partially treated industrial effluents and reduced discharge of freshwater from Hathnikund are causing deterioration in water quality and no major improvements are seen in water quality of river Yamuna.
Similar content being viewed by others
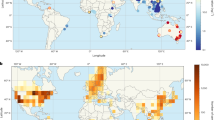
Underestimated burden of per- and polyfluoroalkyl substances in global surface waters and groundwaters
Diana Ackerman Grunfeld, Daniel Gilbert, … Denis M. O’Carroll
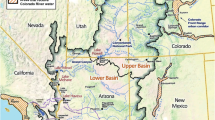
New water accounting reveals why the Colorado River no longer reaches the sea
Brian D. Richter, Gambhir Lamsal, … John C. Schmidt
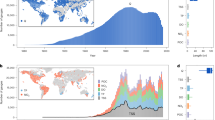
Deep learning for water quality
Wei Zhi, Alison P. Appling, … Li Li
Introduction
Water is the main need of human life. The majority of ancient civilizations were developed on the bank of major rivers across the world. Rivers fulfill the major demands of the freshwater supply from drinking to agriculture. In the northern parts of India, the Yamuna River basin is ranked the second largest basin after the Ganga River. It is the second-largest tributary of the river Ganga (the longest river of India) with a total catchment area of 345,848 km 2 and it originates at Yamunotri Glacier, Uttrakhand, India at a height of 6387 m 1 . It covers a total distance of 1376 km through four major states of India: Uttarakhand, Haryana, Delhi, and Uttar Pradesh, and finally confluences with river Ganga at Triveni Sangam, Prayagraj, Uttar Pradesh. Although it does not flow through Himachal Pradesh but receives water via the river Tons (which originates in Himachal Pradesh). The other tributaries of the river Yamuna are Chambal, Sindh, Betwa, and Ken 2 , 3 . The water abstracted from the river is mostly used for irrigation (about 94%), while 4% for domestic water supply and the remaining 2% for industrial and other uses 4 .
During the last few decades, Yamuna has been considered one of the most polluted rivers of India. Discharge from industries, partially or untreated sewage, and agricultural waste are the main sources for the river Yamuna degradation 5 , 6 , 7 . Almost 85% of the total pollution in the river Yamuna is due to domestic sources mainly from urban cities Sonipat, Panipat, Delhi, Ghaziabad, Mathura, Agra, Etawah, and Prayagraj. Industrial zones at various places like Yamunanagar, Panipat, Ghaziabad, Delhi, Noida, Faridabad, and Baghpat which are in the upper Yamuna basin (Fig. 1 ), comprise industries like Oil refineries, distilleries, pulp, pharmaceutical, chemical, electroplating, weaving, and sugar, and contribute to the degradation of Yamuna water quality significantly 8 , 9 . According to Kumar et al. 10 , Delhi leads the list of cities with 79% pollution load in river Yamuna followed by Agra and Mathura with a contribution of 9% and 4%, respectively, whereas a pollution load of 2% by Sonipat and Baghpat. The annual mixing of sewage from domestic and industrial sources in the Yamuna River basin is about 9.63 km 3 , 11 . In the last few decades, a sudden rise in the built-up and cropland areas is observed in the Yamuna River basin (Fig. 2 ). Kumar et al. 10 suggested a rise of 100% in the urbanization in Haryana and Rajasthan states and significant fall is observed in wetland, grassland, water bodies and forest areas of Yamuna River basin. The green revolution in India helped rise in the productivity of various crops, but the major water supply to the crop depends on the groundwater. The DO level of water in Delhi stretch shows a sudden fall due to high carbon level so that most of the time the river can not sustain fishery.
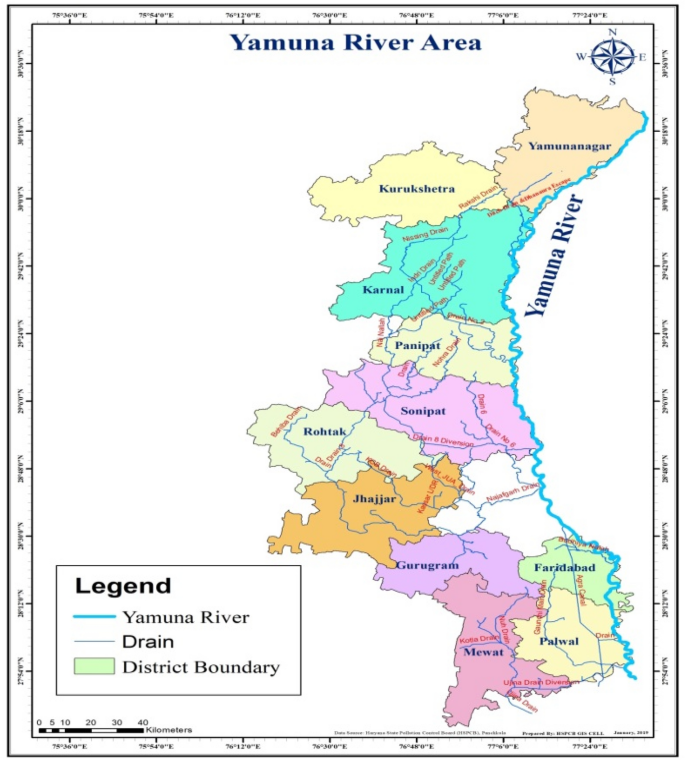
( Source : HSPCB).
River Yamuna in Haryana.
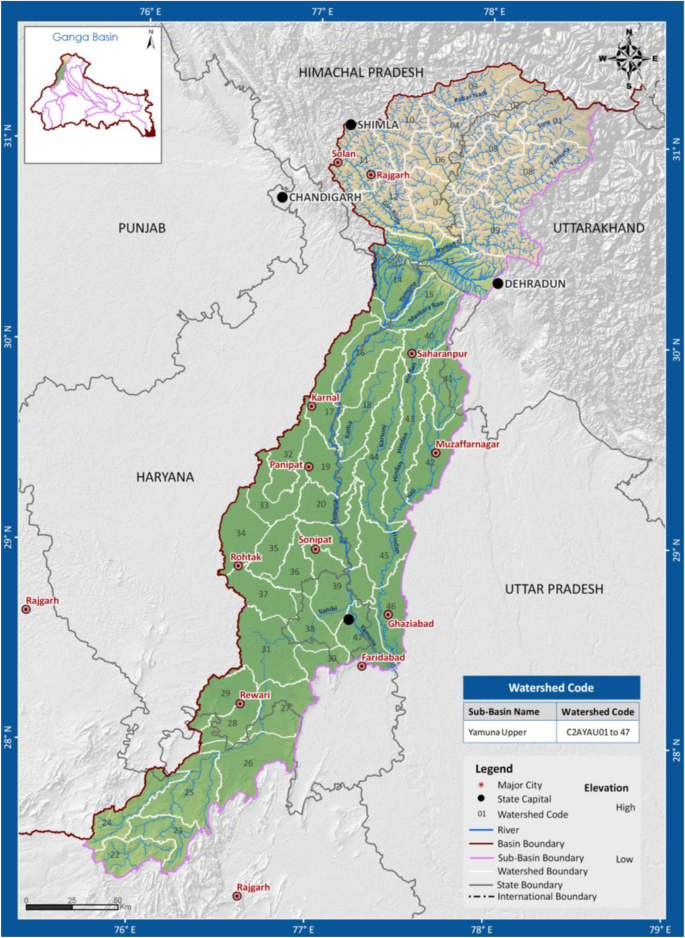
( Source : WRIS).
Upper river Yamuna basin area.
Parween et al. 12 showed the positive rise in the potassium and nitrate that affected the Yamuna River basin. Domestic waste consists of mainly organic matter and micro-organisms along with detergents, grease and total salts mixed in river Yamuna through various drainages in National Capital region. Lokhande and Tare 3 have shown rise in the flow rate of Yamuna during non-monsoon months due to the sewage water. Industrial effluents are the main source of heavy metal pollution like Cd, As, Cr, Fe and Zn with other inorganic and organic wastes adding to pollutant inventory 10 . According to National Capital Region Planning Board (NCRPB) report, sewage generation in Haryana was 374 MLD in 2001, and 599 MLD in 2011, whereas the sewage treatment capacity was 164 MLD in 2001 and 199 MLD in 2011. State monitoring committee appointed by National Green Tribunal (NGT) 2019 suggested that Haryana discharged 1140 MLD of untreated or partially treated sewage per day into river Yamuna, also 1268 industrial units discharged 138.75 MLD partially treated and another 827 units discharged 48.319 MLD of treated effluents per day in Yamuna River 13 .
Central Pollution Control Board (CPCB) is responsible for controlling the various sources of pollution in India and also monitoring water quality of the rivers with the State Pollution Control Board (SPCB) 4 , 5 , 14 , 15 . CPCB started national water quality monitoring in 1978 under Global Environmental Monitoring System (GEMS), followed by the Monitoring of Indian National Aquatic Resources (MINARS) in 1984, and helped reduce river pollution via National Water Quality Monitoring Programme (NWMP) 16 . Central Water Commission (CWE) is monitoring the water quality of all the major river basins in India through 519 water quality sites and 33 water sampling stations. According to CWC, the water quality of river Yamuna is monitored at 18 different stations of which 12 are manual and 6 are telemetry stations, starting from Naugaon (N-30.78, E-78.13) at Uttarakhand as the first river point station to the last river point station Pratappur (N-25.37, E-81.67) Uttar Pradesh.
Due to degradation in the water quality of the Yamuna River, Yamuna Action Plan (YAP-I) was launched in 1993 by the Ministry of Environment and Forests (MoEF), India to rejuvenate the Yamuna River especially in the Delhi segment having maximum pollution load. Haryana and Uttar Pradesh were also included along with Delhi in YAP-II in 2003. YAP-III, with an estimated cost of Rs.1656 crore, was launched in 2018 as an integrated component of the Namami Gange Mission 17 . The river Yamuna is analyzed monthly, seasonally, and yearly for its physical, chemical, and biological properties in previous research for various states and cities influenced by it or small stretches of river 18 , 19 , 20 , 21 . A sufficient amount of literature is available for the water quality of Yamuna and most of the analysis focused on the Delhi and stations lie after the Delhi’s. Kumar et al. 10 discussed the variability of various water quality parameters from 1999 to 2005 to investigate the relationship between environmental parameters and pollution sources. Kaur et al. 22 discussed the impact of industrial development and land use/land cover changes over the Yamuna water quality in Panipat, which is located between the Hathnikund and Baghpat stretch. The sampling was carried out during February, July, October, and December, 2018 to observe the variations in the quality of water and the impact of pollutants on the river Yamuna. Patel et al. 23 carried out the water quality analysis over the Yamuna River using satellite and remote sensing during the lockdown period and compared it with the water quality parameter before the lockdown. The analysis was carried out from January 2020 to April 2021. The impact of lockdown on the water quality was discussed assuming that the industrial waste and the other pollutants sources were reduced during the time of lockdown. Paliwal et al. 24 carried out the modeling analysis of the Yamuna water quality for the Delhi stretch. They emphasize the inflow of untreated water and effluents from various drains in the Yamuna in the Delhi stretch using QUAL2E-UNCAS before 2007. They also highlighted the need for common treatment plants and a rise in freshwater supply. Krishan et al. 25 conducted the groundwater study near the bank of the Yamuna in the Agra and Mathura districts. The study area is located downstream of Delhi and affected by severe falls in water quality. The change in the water quality at Agra and Mathura was discussed and the assessment of the treated water after the filtration was discussed. Kaur et al. 26 investigated the Yamuna water quality at the Delhi stretch and one site located near the Yamunotri. The analyses were implemented during March and October for 2017 and 2018. They have discussed the impact of the inflow of pollutants from the Delhi and NCR regions in the Yamuna. Jaiswal et al. 21 carried out the multivariate study of the Yamuna river water to study the river water quality across the whole stretch. The samples were collected from July to October and November to June for 2013 and 2014. The analysis suggested that the water quality of Palla was suitable for drinking during the study period. We found that these analyses were mostly carried out for a short period in recent years. Some long-term analyses were carried out before 2007. So, there is a dire need for long-term analysis in recent years. In recent times, Lokhande and Tare 3 performed the first long-term analysis of various water quality parameters of the river Yamuna and discussed the trends of various parameters. Due to classified data, Lokhande and Tare 3 were not able to quantify the monthly variations of various water parameters. Hence, these analyses, lack the long-term variability discussion and quantitative changes. In the current study, we conducted monthly and annual mean analysis of various physical and biological parameters, including Biological Oxygen Demand (BOD), Chemical Oxygen demand (COD), Dissolved Oxygen (DO) Saturation, Total Alkalinity, Total Dissolved Solids (TDS), Total Coliform and average rainfall from 2009 to 2019 at Palla station located at northwestern Delhi. We have shown the quantitative change in the physiochemical and biological parameters of the river Yamuna at the Palla station, which is mostly affected by the pollutants load of the watershed of Haryana. We compared the results with the water quality guidelines of national and international standards given in Table 1 to figure out the changes in water quality of river Yamuna at Palla station in the last 11 years. The current water quality at Palla is not suitable for drinking and sometimes not good for agricultural purposes due to the high influx of water pollutants.
The river Yamuna enters National Capital Territory (NCT) at approximately 1.5 km before village Palla, which is 23 km upstream of the Wazirabad barrage. Palla station (N-28.82 and E-77.22) is a manual type station with zero gauges at 206 m. Before entering NCT at Palla, the river Yamuna traveled about 393 km from its source and about 220 km from the Hatnikund barrage. According to Haryana State Pollution Control Board (HSPCB), the numbers of industries in Yamunanagar, Kernal, Panipat, and Sonipat are 142, 9, 346, and 503, generating effluent of 16,420.90, 26.00, 65,696.97, and 15,668.50 KLD, respectively, up to August 2019. As in Fig. 3 , several drains of Haryana state including 3 major drains at Dhanaura escape, Main Drain No.2, and Drain No. 8 also fallout in river Yamuna before reaching Palla 28 (Figs. 3 , 11 ).
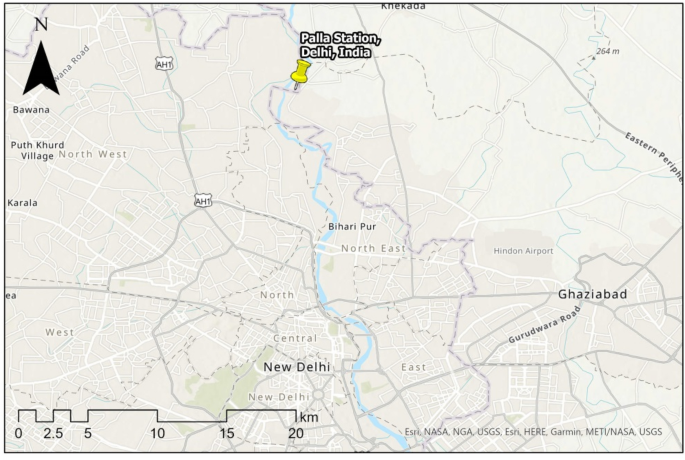
Location of Palla station. The base image is provided by ESRI and projection is done using ArcGIS Pro.
Data retrieval
Water quality data of Yamuna River at Palla station was obtained from Water Resources and Information System (WRIS), India, which is a centralized platform, acting as a database related to all water resources at the national or state level. It was initiated by CWC along with the Ministry of Water Resources, Ministry of Jal Shakti, and Indian Space Research Organization (ISRO) in 2008 to provide a single-window solution to all water resources data and information in a standardized national GIS framework 29 . Depending upon the availability of monthly average data, the parameters BOD, COD, DO saturation, total alkalinity, TDS, and total coliform at Palla station were analyzed during the period from January 2009 to December 2019. The rainfall data was procured from the Modern-Era Retrospective analysis for Research and Applications, Version 2 (MERRA-2) and available on the website http://www.soda-pro.com/web-services/meteo-data/merra . The spatial resolution of data is 0.50° × 0.625° and the time step ranges from 1 min to 1 month 30 , 31 . We also included the water discharge data in the current study of three locations Hathnikund Barrage, Baghpat, and Delhi Railway Bridge (DRB) stations. The freshwater in river Yamuna reached to the Palla station is controlled at the Hathnikund Barrage. Baghpat station is located just before the Palla station (no data is available at Palla station) and DRB is located after the Palla station. These stations are chosen based on the availability of data and the locations to show the water discharge in the Yamuna. The water discharge data of this location is taken from the Central Pollution Control Board of India ( https://yamuna-revival.nic.in/wp-content/uploads/2020/07/Final-Report-of-YMC-29.06.2020.pdf ).
Ethics approval and consent to participate
The paper did not involve any human participants.
Result and discussion
Analysis of various parameters related to the water quality of river Yamuna at Palla station was carried out.
The annual mean variation in rainfall at the Palla region (Fig. 4 a) suggested that the highest annual mean rainfall (95.8 mm) occurred during the year 2010. After 2010, the yearly average rainfall continuously declined every year till 2014 with a mean value of 17.9 mm. Although from 2014 to 2018, a rise in average rainfall was observed each year and during 2018, the average rainfall was calculated as 53.1 mm, but the linear trend illustrated a sharp decline in average rainfall between 2009 and 2019 as shown in Fig. 4 a.
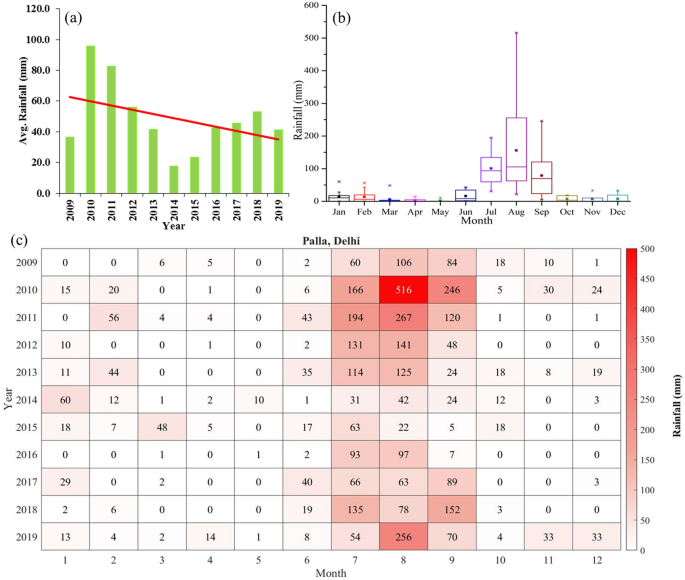
( a ) Yearly mean rainfall at Palla station and adjacent region from 2009 to 2019. The red line shows a linear trend of rainfall. ( b ) Rainfall monthly distribution at Palla station from 2009 to 2019. ( c ) Monthly mean variation of rainfall from 2009 to 2019.
The box plot in Fig. 4 b shows the rainfall data of each month during 2009–2019. The distribution suggested that the monthly mean rainfall variations were highest during August along with July and September. May was an almost driest month along with April and March as the rainfall was minimum during these months. The highest values of average rainfall for August, September, and July were 515.28, 233.45, 207.99 mm respectively, whereas these months’ minimum average rainfalls were 101.74, 61.95, and 72.76 mm, respectively, during 2009 and 2019. The median values of rainfall during March, April, October, and November were below 10 mm and, during January, February, June, and December, the median values were between 10 and 20 mm, while the median values for July, August, and September were above 60 mm.
The monthly mean variation of rainfall (2009–19) further elaborates the stats of rainfall in the Palla region. From 2009 to 2019 rainfall data showed various ups and downs in monthly rainfall (Fig. 4 c). In India, the onset of monsoon is observed during the start of June each year in the coastal part of India, which lasts approximately for a period of four months (June to September) each year. The northern parts of India receive major rainfall during this period, which is known as the monsoon season. During June, the average rainfall remained lower than the decadal mean during 2014–2019 except for 2017. During July, we observed the same deficit of rainfall during 2014–2019 except in 2018. The major deficit in rainfall was observed for August from 2012 to 2018 whereas, during August 2019, the rainfall remained higher than the decadal mean value. During September, the rainfall deficit was observed from 2012 to 2016. Hence, during 2012–2017, we observed a significant fall in the monsoon rainfall. The winter rainfall is also observed during January and February in northern parts of India. We also observed a significant fall in the winter rainfall for January and February since 2014. Yamuna River before Palla station receives runoff water from cities like Sonipat, Panipat, Karnal, and Yamunanagar. The rainfall statistics of Haryana analyzed by India Meteorological Department (IMD) between 1989 and 2018 showed annual rainfalls of 1053.5, 578.8, 573.9, and 495.3 mm at Yamunanagar, Sonipat, Karnal, and Panipat districts, respectively 32 . During the monsoon season, sudden changes in physicochemical and biological parameters were observed by various researchers. The addition of monsoon runoff in rivers dilutes the industrial effluent and sewage, causing the decline in parameters like BOD, COD, alkalinity, pH, and conductivity 33 , 34 , 35 , 36 . The catchment area of Yamuna is the smallest in Delhi. The size of the catchment area of the river is an important factor for the dilution of anthropogenic waste in river water. The small catchment area increases soil components in the river and makes difficult the dilution process of anthropogenic waste 37 . These conditions can further affect the river water quality. Hence, we further analyzed the variations in the various physical and biological parameters for each month from 2009 to 2019.
Dissolved oxygen (DO) saturation
Dissolved Oxygen (DO) saturation is a vibrant parameter for aquatic system health determination. The pollutants like sewage, soil, agricultural runoff, and other organic pollutants can reduce the DO saturation of water 38 and low DO saturation can impact the life of major aquatic organisms. The water having DO < 5% of saturation lies in an extremely severe pollution region; DO between 5 and 10% of saturation lies in severe pollution; DO in a range of 10–70% represents moderate pollution, whereas DO above 70% indicates slightly or no pollution condition. Heavy pollution load due to untreated sewage and industrial effluents are the main causes of decreasing DO concentration 39 . Value of DO saturation is also affected by the change in water salinity (chlorine), temperature, and air pressure.
Increasing pollution in the Yamuna River caused a decrease in DO saturation concentration along with an increase in temperature and salinity of water 3 . Figure 5 shows the yearly variation of DO saturation, and monthly distribution and mean variation of DO saturation at Palla station from 2009 to 2019. In recent years, the annual mean value of DO saturation during 2017–19 was found to be 4.81%. From 2009 to 2011, the mean DO saturation was found to be 69.49% with a maximum value (81.58%) in 2010. From 2012 to 2015, the yearly mean DO saturation was 86.48%. During this period, a small decrease was seen between 2013 and 2015. In recent years, the DO saturation reached critically low values to the average value before 2015 as demonstrated in Fig. 5 a. These conditions indicate the rise in pollutants in the river Yamuna.
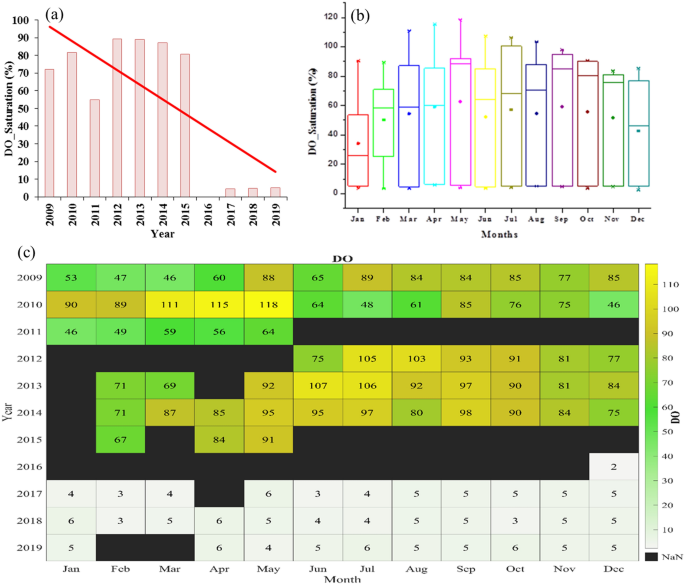
( a ) Yearly variation of DO saturation from 2009 to 2019. The red line shows a linear trend of DO saturation. ( b ) Monthly distribution of DO saturation at Palla station from 2009 to 2019. ( c ) Monthly mean variation of DO (%) saturation from 2009 to 2019.
The monthly distribution of DO saturation during 2009–2019 is shown in Fig. 5 b with boxes ranging from 25 to 75%. During May, the maximum median value was 88%, whereas in January minimum median value was about 25%. Similarly, the maximum monthly mean value was 62.7% during May, while the minimum mean value was 34.1% during January. Similarly, maximum DO saturation of 118% was perceived for May, while a minimum of 2.4% was observed for December. The monthly mean distribution suggested that the mean DO saturation plunged between 50 and 60% with a slight growth in trend from January to December. We further showed the monthly mean values of DO saturation in Fig. 5 c. From 2009 to 2015, the values suggested no major changes with monthly values well above 50, but just after 2017, a sudden fall was observed in the DO saturation each month. We observed 10 times fall in DO saturation just after 2017. During 2009–14, the DO saturation of Yamuna River at Palla station was Class I category of international standards for surface water of UNECE, but during 2016–19 its quality degraded to Class IV due to regular fall in the DO values of the river water. The lack of fresh water and rising carbon concentration have affected the DO concentration significantly.
Biological oxygen demand (BOD)
Biological Oxygen Demand (BOD) is one of the methods to assess the quality of water by calculating the oxygen requirement for decomposition of its organic matter. The yearly variations in BOD at Palla station during 2009–19 are shown in Fig. 6 a. The yearly mean BODs in 2009 and 2010 were estimated to be around 6 mg/l, while, during 2013 and 2012, the least values of BOD (1.4 and 2.7 mg/l, respectively) were found. The year 2015 showed the highest yearly mean BOD value of around 12 mg/l followed by years 2014 and 2016 with a value of about 9.5 mg/l. The decline in yearly mean BOD was observed from 2015 to 2019. In particular, a BOD of 3.5 mg/l was perceived for years 2018 and 2019. The monthly variation of BOD during 2009–19 was shown in Fig. 6 b, where the interquartile range for each month was between 25 and 75 percentiles. The first quartile for all months lay below the range between 0 and 5 mg/l, with outliers for months May, July, October, and December with BOD above 20 mg/l. January and February had the highest median value of BOD at 6.7 mg/l, while September had the least median value of 2.1 mg/l.
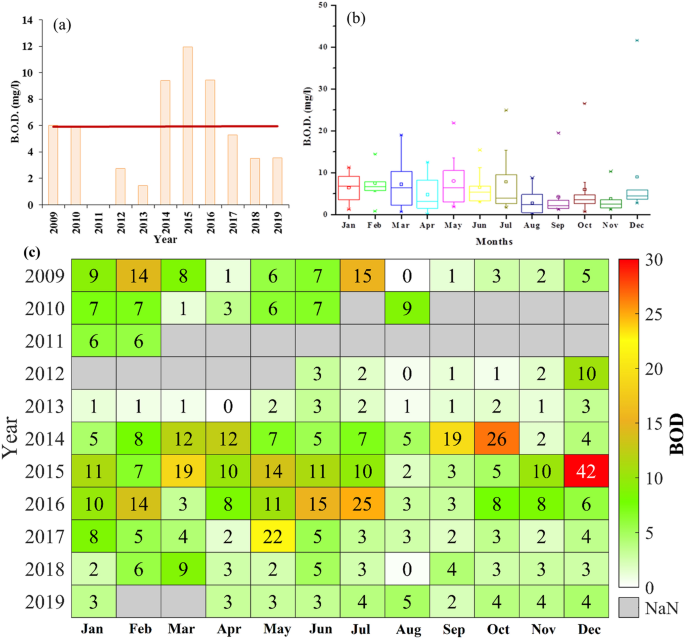
( a ) Temporal variation of yearly mean BOD from 2009 to 2019. The red line shows a linear trend of BOD. ( b ) Monthly distribution of BOD at Palla station from 2009 to 2019. ( c ) Monthly mean variation of BOD from 2009 to 2019.
The median value for April, June, July, October, and December lay between 3 and 5 mg/l. The monthly mean BOD during 2009–19 represented that BOD was maximum in December (8.9 mg/l) followed by the second highest mean value of 8 mg/l in May. The minimum mean value of 2.7 mg/l was found in August, while in April, September, October, and November, the mean BOD lay between 3 and 6 mg/l. Mean BOD from January to March and in July was in the range of 6–7.5 mg/l.
The fluctuation in BOD was observed during 2009–19 in Fig. 6 c. In January, February, and March, a decrease in BOD occurred during 2009–13, while in May, June, and July increase in BOD was seen during 2013–16. In August, September, and October, BOD mostly fell below 5 mg/l throughout the study period. The highest BOD values were measured in December 2015, October 2014, and July 2016 (41.6, 26.5, and 24.8 mg/l, respectively). The decreasing trend was observed for January, February, and July, with a rising trend for March, April, May, and November. From 2014 to 2016, the BOD values were found to be several times higher than the acceptable limits. In March and April, BOD values were less than 4, and else BOD was higher than 4 even in monsoon months. In years 2018–19, BOD is observed to be mostly ≤ 4 mg/l. However, the overall BOD values suggest that the water quality of Yamuna mostly lay beyond the C category (BIS) as the BOD values are mostly > 4 mg/l. To attain river quality standard it has to be ≤ 3 mg/l.
Chemical oxygen demand (COD)
Chemical Oxygen Demand (COD) determines the amount of oxygen required for the oxidation of organic matter present in water. We have shown the changes in COD at Palla station in Fig. 7 . Yearly mean COD varied indistinctly during 2009–19 even though the linear trend has moved upward with increasing year as shown in Fig. 7 a. The yearly mean COD increased from 15.9 to 30.8 mg/l during 2010–2012 and from 11.5 to 44.3 mg/l during 2013- 2015. Mean COD for the years 2018 and 2019 was 15.1 and 20.4 mg/l, respectively. The highest yearly mean COD was 44.33 mg/l for the year 2015 followed by the year 2012 with 30.8 mg/l, while the least mean COD value was observed in 2013 as 11.5 mg/l. A sharp decline in yearly mean COD was noted during the years 2012–13 and 2015–16. The monthly data of each month during 11 years period is shown in Fig. 7 b. The width between the first and third quartiles for January, April, and May indicated maximum variation in COD values. The September quartile indicated less variation in COD as compared with the other months. The CODs for January and May had the highest median values of 27 and 24.5 mg/l, respectively, whereas April had the least median value of 8 mg/l. The median CODs for March, August, September, October, and November lay in the range of 11–16 mg/l. We found that the monthly mean CODs of May and November had maximum and minimum values of 33.9 and 14.2 mg/l, respectively.
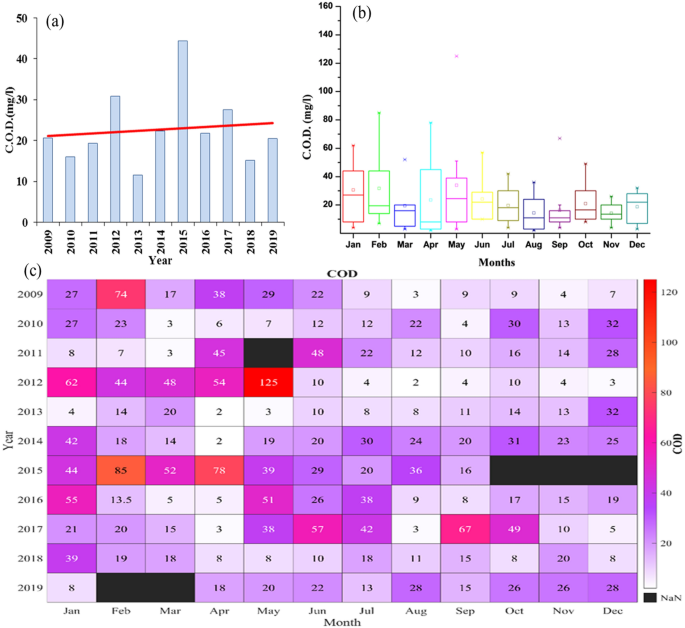
( a ) Yearly variation of COD from 2009 to 2019. The red line shows a linear trend of COD. ( b ) Monthly distribution of COD saturation at Palla station from 2009 to 2019. ( c ) Monthly mean variation of COD from 2009 to 2019.
The trend of COD followed a decreasing path moving from January to December. The monthly mean CODs of January, February, and May were found to be higher than 30 mg/l whereas for April, June, and October they lay between 20 and 30 mg/l, and for the remaining months mean COD was found to be lesser than 20 mg/l. A decline in the mean COD was observed from May to August, with a sharp increase in mean COD from March to May.
Figure 7 c shows changing COD for each month as the year preceding. From 2009 to 2012, the COD values for January, February, March, and April were higher than 30 mg/l, whereas, since 2017, the COD values were seen well below 30 mg/l during these months. In May, an exceptional high COD of 125 mg/l was observed and for the same month, a continuous increase in COD was noted from the years 2013 to 2016. Also, the rise in COD was seen for June, August, October, and December during 2012–15. Excluding May, February, April, and September had maximum CODs of 85, 78, and 67 mg/l, respectively. During 2009–19, January and June to November showed a growing linear trend, whereas the rest of the months had a declining trend. Compared with the international standards, the annual and monthly mean CODs exceeded the WHO guideline and were classified as Classes III-V of UNECE standards. Also, the values were found well above the threshold value. During the years 2009, 2012, and 2013, the monsoon period showed COD value in Class II, while, during the overall monsoon period, water quality lay in Class III. During the year 2015, Yamuna, in terms of COD, was in the worst condition as it lied in Class V. Monthly variation COD for 2018–19 rarely plunged under WHO standards and represented to be in Classes III and IV.
Total alkalinity
Total alkalinity is mostly due to calcium carbonate (CaCO 3 ) and also important for sustaining aquatic life. The yearly mean variation of total alkalinity during 2009–19 is shown in Fig. 8 a. Total alkalinity was found to be the highest during 2015 followed by 2011 with values of 187.37 and 164.6 mg/l, respectively, while, for the rest of the year, the annual means were in the range of 110–150 mg/l. The linear trend for the yearly mean for the entire period remained constant. With these values of total alkalinity, the quality of river water lay in category II (UNECE 1994).
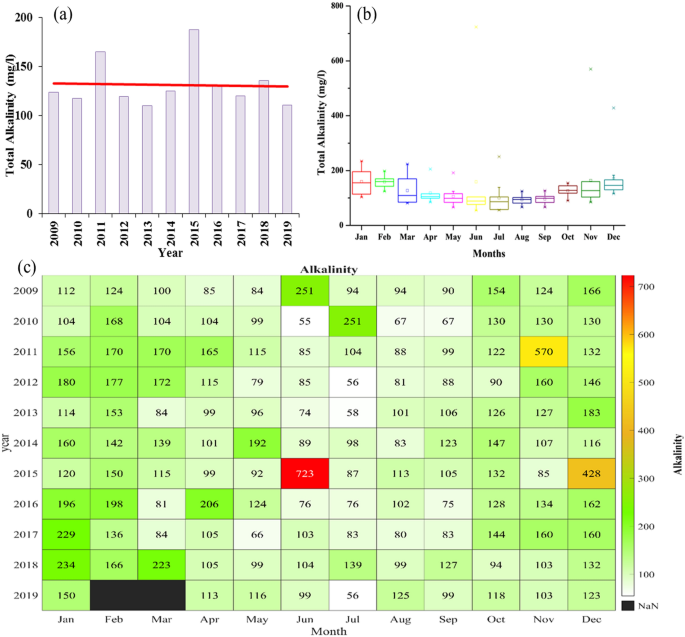
( a ) Yearly variation of total alkalinity from 2009 to 2019. The red line shows a linear trend of total alkalinity. ( b ) Monthly variation of total alkalinity from 2009 to 2019. ( c ) Monthly mean variation of total alkalinity from 2009 to 2019.
We have shown the monthly distribution of total alkalinity in Fig. 8 b with the third quartile of each month lying below the mark of 200 mg/l. The median values dropped from January to July and then raised from July to December. The monthly mean total alkalinities in February and January showed the first and second-highest median values of 159.8 and 155.5 mg/l, respectively, while July and June showed the lowest median values of 86.5 and 88.9 mg/l, respectively. Median values during May, August, and September lay in the range of 90–100 mg/l, while for March, October, November, and December they were between 100 and 150 mg/l. The linear trend for monthly mean total alkalinity also followed the same pattern as the yearly mean. The total alkalinity declined from January to May from 159.4 to 105.5 mg/l and, then from August to December, it raised from 93.8 to 170.7 mg/l. August and December showed the lowest and highest values of total alkalinity, respectively.
The monthly variation in total alkalinity for each year during 2009–19 is shown in Fig. 8 c. June (2015), November (2011), and December (2015) were the months with the highest total alkalinities of 723.3, 569.9, and 428.1 mg/l, respectively. During 2009–19, total alkalinities for February, March, May, July to September remained within 200 mg/l. The lowest total alkalinity of around 55 mg/l was noticed for June (2010) and July (2012). Although the linear trend for monthly total alkalinity showed almost the same slope except for January, July, and November. A wave pattern in total alkalinity for August, September, and October was noticed with an increasing trend during 2009–19. In 2019, the total alkalinity was below 150 mg/l throughout the year 2019. For maintaining WHO and BIS standards, the minimum total alkalinity must be 200 mg/l, while it was not possible to fulfill the standards for both monthly and yearly aspects in the study areas of concern. There were only a few months when total alkalinity was found to be higher than 200. Comparing with UNECE standard, 11 out of 12 monthly mean alkalinities lay in Class II and the remaining one month in Class III category. March 2018 was the last month since 2018 when Yamuna's total alkalinity was well above-mentioned the water standards.
Total dissolved solids (TDS)
Total Dissolved Solids (TDS) define the presence of inorganic compounds along with organic matter in small concentrations originated by naturally, household, and industrial sources. The data was available from 2013 onwards. The yearly mean TDS was found to be highest in 2015 (447 mg/l) followed by 2017 and 2018 with 421 mg/l (Fig. 9 a). The lowest yearly mean TDS was observed as 256 mg/l in 2010, while the recent value of 272 mg/l was observed in 2019. Although the linear trend for yearly TDS indicated the rise in overall TDS. A maximum drop in yearly TDS was observed with a fall of 36% during 2018–19.
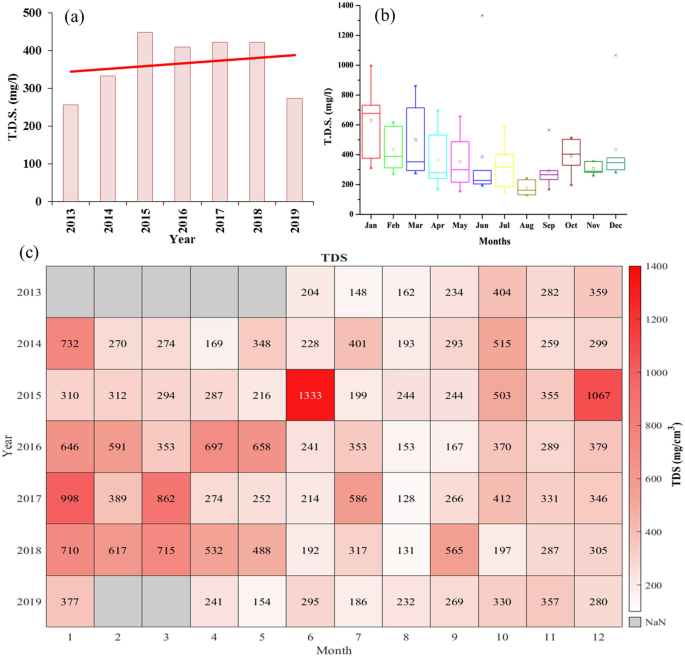
( a ) Yearly variation of TDS from 2013 to 2019. The Red line shows a linear trend of TDS. ( b ) Monthly variation of TDS from 2013 to 2019. ( c ) Monthly mean variation of TDS from 2013 to 2019.
The 25 to 75 percentiles of interquartile range of all twelve months for TDS are shown in Fig. 9 b. Although March had the maximum width of interquartile range, the maximum median value of TDS was 678 mg/l for January. Except for August and October, the median TDS values for the rest of the months lay in the range of 200–400 mg/l. August had the lowest median TDS of 162 mg/l and October had 404 mg/l. A sharp decline in the trend of monthly mean TDS was observed during 2013–19, but mean TDS fluctuated throughout the year. Similarly, with median TDS, the monthly mean TDS for January and August had maximum-minimum mean values of 628.3 and 177.57 mg/l, respectively. Except for August, the monthly mean values of TDS were above the mark of 200 mg/l. From April to July, they lay in the range of 300–400 mg/l, while in February, March, and December, they ranged between 400 and 500 mg/l.
In February and March, TDS had a higher magnitude of rising, while it appeared to be almost constant in August as shown in Fig. 9 c. In January, TDS was measured as 732 mg/l in 2014 and dropped to its lowest point of 310 mg/l in the next year, but then it reached 998 mg/l in 2017. The TDS values during June were well below the mark of 300 mg/l from 2009 to 2019, but during June 2015, the monthly mean TDS was found to be 1333 mg/l. This was the maximum value of TDS during the whole study period. For the same year, the second-highest TDS of 1067 mg/l was also observed in December. TDS remained mostly below 300 mg/l for August and September (mostly during monsoon months) with the lowest TDS of 128 mg/l in August 2017. In 2019, the TDS value mostly ranged between 200 and 300 mg/l. Yearly and monthly mean values of TDS were observed almost under WHO standards and in the Class A category of Indian standards. The TDS value was found below 500 mg/l during each monsoon season. During winter, summer, and post-monsoon months, the TDS of river water never exceeded the Class C category as per BIS standard and during January, the monthly mean average remained higher in comparison to other months.
Total coliform
Human and animal discharges are the main source of fecal coliform bacteria whose excessive presence in water degrades the water quality. During 2009–19, there was an exponential rise in total coliform as shown in Fig. 10 a. The yearly mean in 2009 was 177 MPN/100 ml, which in the decade reached 139,200 MPN/100 ml in 2019. The difference of yearly mean for two periods of 2009–13 and 2016–19 was more than 100 times the value at its starting period. The year 2018 was observed with the highest yearly mean of 490,818 MPN/100 ml, which was reduced in 2019, with the lowest total coliform count of 136.7 MPN/100 ml in 2010. Note that the years 2014–15 were excluded from comparison for this case due to less availability of data for the whole year.
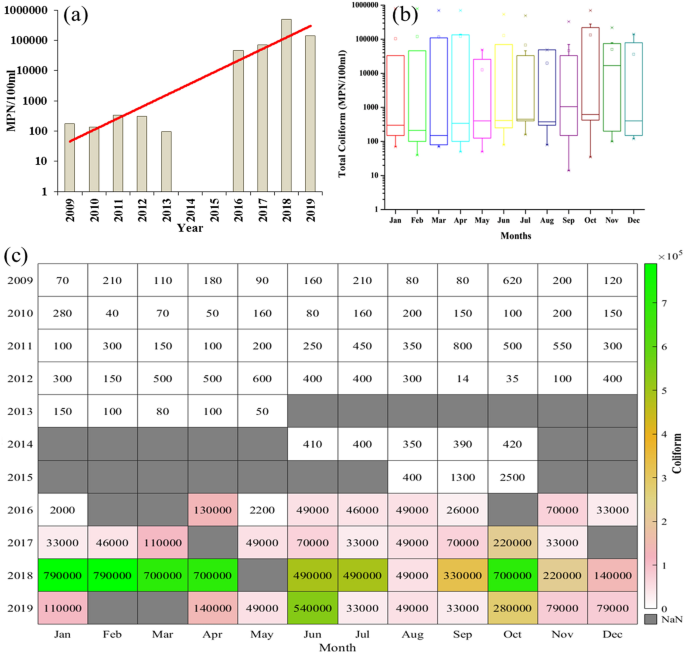
( a ) Yearly variation of total coliform from 2009 to 2019. Redline shows an exponential trend of total coliform. ( b ) Monthly variation of total coliform from 2009 to 2019. ( c ) Monthly mean variation of total coliform from 2009 to 2019.
Figure 10 b shows a large monthly variation in total coliform in each month during 2009–19. The median of most of the months was below 500 MPN/100 ml, whereas median values were 1050, 620, and 16,775 MPN/100 ml for September, October, and November, respectively. The monthly mean for January, February, March, April, June, and October was above 100,000 MPN/100 ml. Maximum and minimum monthly means were observed for October (133,797 MPN/100 ml) and May (12,663 MPN/100 ml), respectively.
Figure 10 c indicates the exponential rise in the trend of total coliform in each month since 2009. Total coliform counts during 2009–14 were well below the 500 MPN/100 ml mark, while some months also showed counts near 800 MPN/100 ml. However, from 2016 onwards, the counts crossed a sustainable mark of 5000 MPN/100 ml for every month of each year. From 2009 to 2013, the total coliform counts fell mostly in Class B but exceeded all limits of Indian water quality standards to a great extent during 2016–19. The monthly mean was not even close to the maximum total coliform limit of 5000 MPN/100 ml, which made water quality in Class D and E categories. WHO standards nullify the presence of fecal coliform in water, whereas the Yamuna River was found to be in its alarming situation for this particular parameter.
Water discharge
The freshwater supply and inflow of wastewater in the river may affect the water quality. To uncover the influence, we analyzed the water discharge in the river Yamuna from 2013 to 2018 (the data is available for this period only). In Fig. 11 , we show the major locations of water abstraction and confluence in the Yamuna river starting from Yamunotri to Faridabad stretch. Hathnikund barrage was constructed to regulate the Yamuna water supply to Haryana, Uttar Pradesh, and Delhi for agricultural and domestic purposes and it was also decided by the Government to maintain 10 cumes of water in the Yamuna downstream to maintain the aqua life in river. In Fig. 12 , we show the water discharge data of Hathnikund, Baghpat, and DRB. From 2013 to 2018, a significant fall in the water discharge is observed especially at Hathnikund, which allows the upstream water to reach Palla. The mean discharge in the river was found to be 123.7 cumes during the whole study period and the minimum was found much lower than 10 cumes (as suggested by the Government of India). Also, a significant fall is observed in recent years. The mean water discharge values at Baghpat are found to be 225 cumes and sometimes they reached far below than 5 cumes.
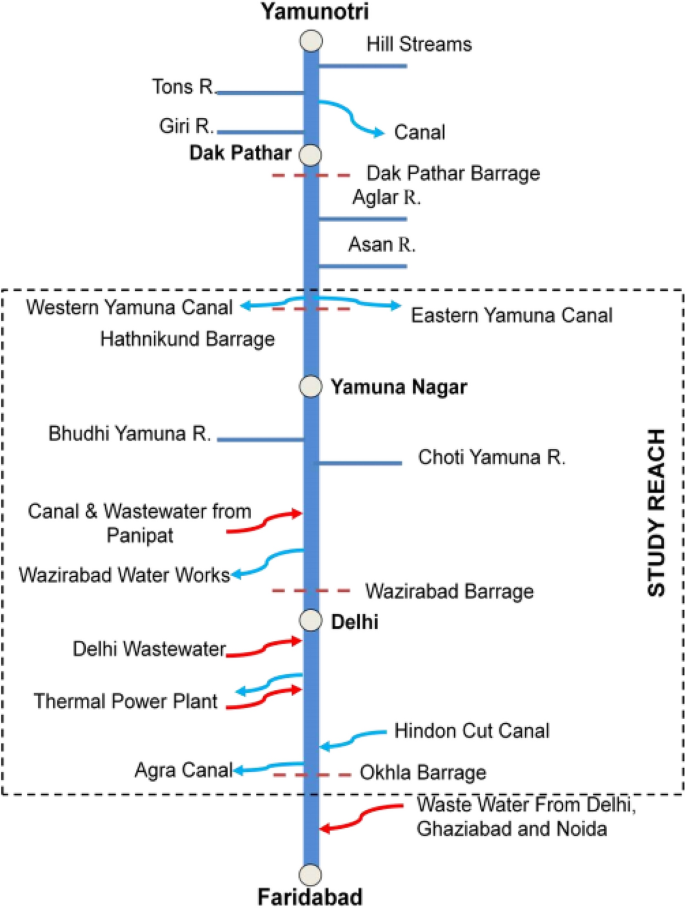
( Source : https://yamuna-revival.nic.in/wp-content/uploads/2020/07/Final-Report-of-YMC-29.06.2020.pdf ).
Points of water abstraction and additions in Yamuna river.
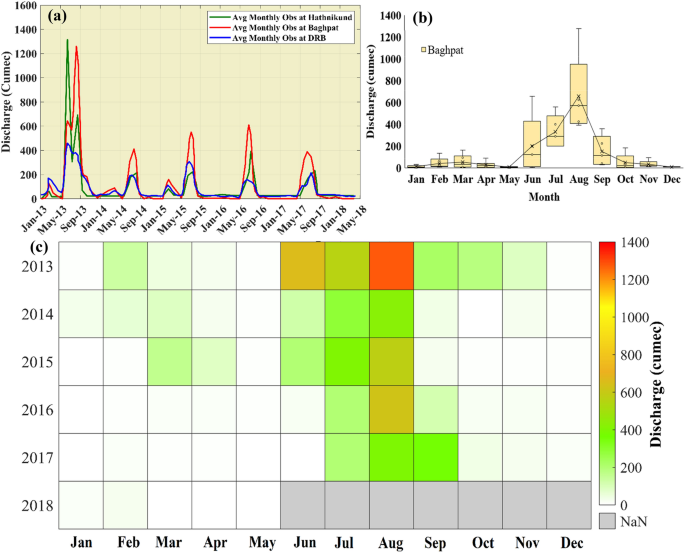
( a ) Temporal variation of water discharge at Hathnikund, Baghpat, and Delhi Railway Bridge (DRB) stations during Jan 2013 to May 2018. ( b ) The distribution of monthly mean water discharge during Jan 2013 to May 2018 at Baghpat. ( c ) Monthly mean variation of discharge from 2013 to 2018.
At DRB, the mean discharge value is found to be 107 cumes. During monsoon months, the water discharge at Baghpat is higher than that at the Hathnikund so that the watershed of the Yamuna also helps in the rise of the water discharge due to rainfall and also major drains confluence in the Yamuna. We can see that during August, the water discharge is the highest followed by July and September. During January, May, and December, the water discharge values reached far below the prescribed limit, and hence sometimes during these seasons, the Yamuna almost dried up and only the sewage and the industrial wastes water flow during these months (Fig. 12 c). After 2015, a significant fall in water discharge is observed during the summer and winter months. With the abstraction of fresh water at Hathnikund barrage and inflow of the drains, the water quality parameters are affected by a large extent in river Yamuna. Therefore, for further analysis of the impact of water discharge and rainfall, we also analyzed the relationship of monthly mean discharge, rainfall, DO saturation, BOD, and COD as shown by the polar plots (Figs. 13 , 14 ).
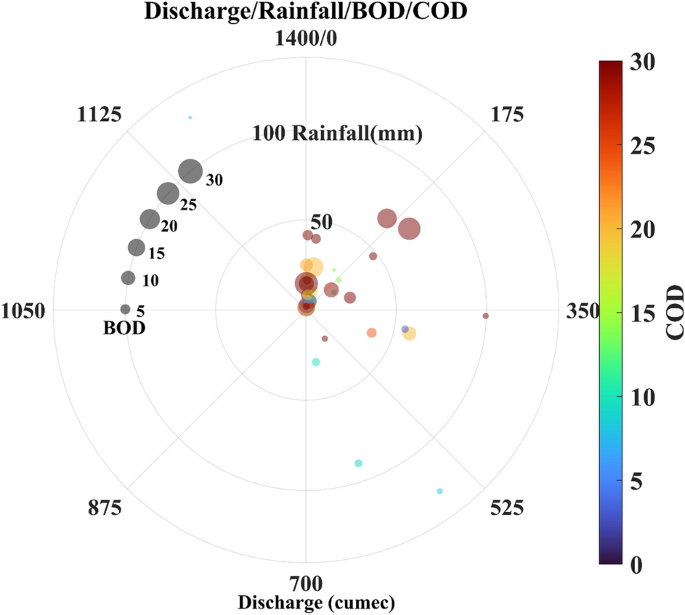
The relationship between the water discharge, Rainfall, BOD, and COD.
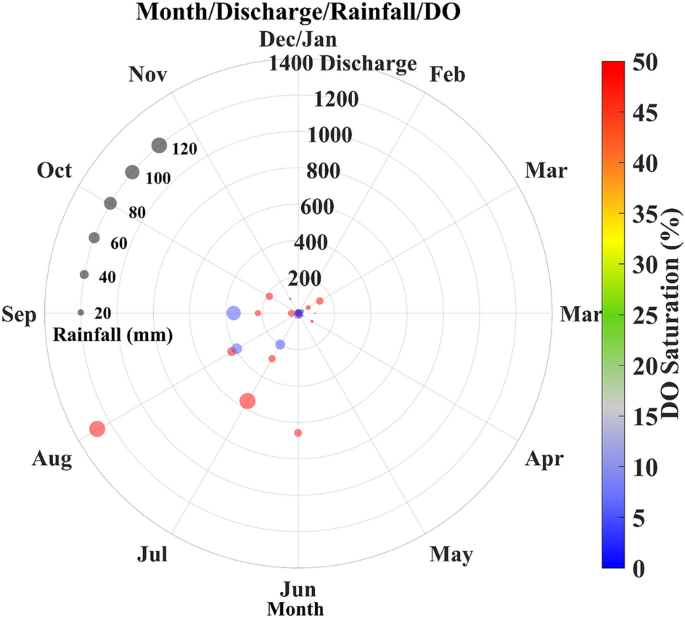
The monthly relationship between the water discharge, Rainfall and DO saturation.
Variability of DO, BOD, and COD
Water quality can be affected by various factors. Hence, we investigated the variability of the DO, BOD, and COD with water discharge and rainfall. In Fig. 13 , we plot the relationship of monthly mean water discharge at Baghpat, rainfall, BOD, and COD. The rainfall mostly affected the discharge values, but, sometimes, with low rainfall high discharge is observed. COD is mostly higher during the whole study period whereas BOD values have shown a fall in recent years. The impact of rainfall and high discharge is visible and low BOD and COD are observed. When the discharge is more than 525 cumes, the COD and BOD values are lower, and also more than 30 mm of rainfall is observed at that time. Further, we investigated the monthly relationship of the Discharge, rainfall, and DO saturation. Further analysis (Fig. 14 ) clearly shows that most rainfall occurred from June to September and caused a rise in the water discharge. During this time, the DO saturation values are more than 50%. The low DO saturation values are mostly observed during the time of low discharge and low rainfall (< 10%). We also found that during July to September with discharge between 200 to 400 cumes and rainfall more than 40 mm, the DO saturation remains lower than 10%. Further, Drain No. 6 having a catchment area of Samalkha Ganur and Sonipat, carries around 49 MLD of sewage in the year 2017 and rises to 210 MLD in the year 2018. Drain No. 8 crosses Drain No. 6 at Akbarpur, Sonipat, and meets river Yamuna just upstream of Palla village. There is a huge increase in the flow of Drain No. 8 between 2017 and 2018 from 196 to 2590 MLD. Drain No. 6 lined separately flow inside Drain No. 8 for 10 KM. Effluents from both drains mix with each other during the rainy season and due to accidental breach ( https://sandrp.in/2015/04/13/blow-by-blow-how-pollution-kills-the-yamuna-river-a-field-trip-report/ ). Similarly, sewage flow of Drain No. 2, which meets river Yamuna 100 km upstream of Delhi is increased from 62 to 2092 MLD between 2017 and 2018 by Central Pollution Control Board of India. As per Haryana State Pollution Control Board (HSPCB), the capacities of Sewage treatment plants (STP) for Drains No. 2, 6, and 8 were 72, 104.5, 125.3 MLD, respectively, till 2018. Also, the capacities of the common effluent treatment plant (CETP) for Drains No. 2, 6, and 8 were 21, 33.2, and 10 MLD, respectively. Since the total capacity for treating wastewater was far beyond sewage generation during 2017–18 and hence the untreated water mixed with the river water. This rise in wastewater generation from industrial and urban areas has caused a drastic decrease in DO saturation and an increase in Total Coliform. As per CPCB 2018 report ( https://yamuna-revival.nic.in/wp-content/uploads/2020/07/Final-Report-of-YMC-29.06.2020.pdf ), 7-day average discharge of the 10-year return period (7Q10) does not meet the habitat requirements of the indicator fish species. These conditions show the impact of rapid urbanization and industrialization along the river bank with high carbon concentration. With the recent development in industrial regions, change in land use/land cover and rapid urbanization in the Haryana, the watershed of Yamuna suffered a lot and hence the water quality of the river. One of the major causes of the sudden fall in the DO saturation in recent years is the fall in the freshwater discharge at the Hathnikund and also the fall in the rainfall in recent years. Also, the deteriorating water quality of Yamuna is a major concern for the Government and mostly this is affected in the stretch between Hathnikund and Palla due to rise in inflow of untreated drains water supply. In recent years, the National Green Tribunal (NGT) of India also requested the states Government to take necessary actions to combat present situation of river Yamuna by installation of more STEPs, ETPs and maintaining the treatment capacity of present treatment plants and channelizing the sewage network to reach treatment plants properly. However, due to lack of adequate fresh water supply and mixing of untreated sewage through regulated and unregulated drains, the quality of the Yamuna river lies in critical conditions.
Although National Capital Territory (NCT) is held responsible for most polluting river Yamuna, the study reveals that the quality of the river it receives is not admirable. The study of physiochemical and biological parameters shows variation in its monthly and yearly values during 2009–19. The effect of monsoon season can be easily seen on parameters like BOD, COD, total alkalinity, TDS and total coliform as their values declined, while DO saturation % showed a significant rise. DO saturation declined by more than 85% during this period. The BOD values improved during the last two years (2018–2019), but were still slightly higher than the permissible limit, while the COD value always remained quite higher than the permissible limits. In 2015, the worst condition was observed in terms of BOD and COD. Total alkalinity also remained low and below the prescribed standards, but TDS is the only parameter whose value was mostly in desired limits throughout the period. An exponential rise was observed in the total coliform count, which was 100–1000 times the maximum limit of IS:2296. Increasing discharge of partially treated industrial effluent and untreated sewage into the Yamuna in the past decade is considered to be the primary cause of the deterioration of water quality. Even after completion of YAP phases I and II, and ongoing phase III, the river still falls in the category of Class D or E under BIS specification, Class III or IV of UNECE standards, and does not fulfill the WHO guideline for water quality at Palla station.
Data availability
All the data used in the present study is freely available in the public domain and the web addresses are discussed in the manuscript, however, we will provide data to all the interested scientists.
Abbreviations
Bureau of Indian Standards
Biological oxygen demand
Common effluent treatment plants
Chemical oxygen demand
Central Pollution Control Board
Central Water Commission
Dissolved oxygen
Effluent treatment plant
Global Environmental Monitoring System
Haryana State Pollution Control Board
India Meteorological Department
Indian Space Research Organization
Kiloliters per day
Modern-Era Retrospective analysis for Research and Applications, Version 2
Monitoring of Indian National Aquatic Resources
Millions of litres per day
Ministry of Environment and Forests
Most probable number
National Capital Region Planning Board
National Capital Territory
National Green Tribunal
National Water Quality Monitoring Programme
State Pollution Control Board
Total dissolved solid
United Nations Economic Commission for Europe
World Health Organization
Water Resources and Information System
Yamuna Action Plan
CPCB 1980-81, The Ganga River Part I The Yamuna basin ADSORBS/22 . Central Pollution Control Board, Delhi, India (1981).
Jain, C. K. Metal fractionation study on bed sediments of River Yamuna, India. Water Res. 38 , 569–578. https://doi.org/10.1016/j.watres.2003.10.042 (2004).
Article CAS PubMed Google Scholar
Lokhande, S. & Tare, V. Spatio-temporal trends in the flow and water quality: Response of river Yamuna to urbanization. Environ. Monit. Assess. https://doi.org/10.1007/s10661-021-08873-x (2021).
Article PubMed Google Scholar
CPCB (2006) Water Quality Status Of Yamuna River (1999 – 2005). Central Pollution Control Board, Ministry Of Environment & Forests, Assessment and Development of River Basin Series: ADSORBS/41/2006-07.
CPCB (2003) Annual Report 2002–2003,Water Quality Status of River Yamuna in Delhi.
Misra, A. K. A river about to die: Yamuna. J. Water Resour. Prot. 02 , 489–500. https://doi.org/10.4236/jwarp.2010.25056 (2010).
Article CAS Google Scholar
Sharma, D., Kansal, A. & Pelletier, G. Water quality modeling for urban reach of Yamuna river, India (1999–2009), using QUAL2Kw. Appl. Water Sci. 7 , 1535–1559. https://doi.org/10.1007/s13201-015-0311-1 (2017).
Article ADS CAS Google Scholar
Manju, S. & Smita, C. Assessment of ground water quality in vicinity of industries and along Yamuna River in Yamuna Nagar, Haryana, India. Asian J. Sci. Technol. 4 , 54–61 (2013).
Google Scholar
Sharma, S. & Chhabra, M. S. Understanding the chemical metamorphosis of Yamuna River due to pollution load and human use. Int. Res. J. Environ. Sci. 4 , 2319–1414 (2015).
Kumar, B., Singh, U. K. & Ojha, S. N. Evaluation of geochemical data of Yamuna River using WQI and multivariate statistical analyses: A case study. Int. J. River Basin Manag. 17 , 143–155. https://doi.org/10.1080/15715124.2018.1437743 (2019).
Article Google Scholar
Pachamuthu Muthaiyah, N. Rejuvenating Yamuna river by wastewater treatment and management. Int. J. Energy Environ. Sci. 5 , 14. https://doi.org/10.11648/j.ijees.20200501.13 (2020).
Parween, M., Ramanathan, A. & Raju, N. J. Waste water management and water quality of river Yamuna in the megacity of Delhi. Int. J. Environ. Sci. Technol. 14 (10), 2109–2124. https://doi.org/10.1007/s13762-017-1280-8 (2017).
Arora, D. P. With most STPs out of order, Haryana continues to pollute the Yamuna [WWW Document]. Hindustan Times, Gurugram (2019). https://www.hindustantimes.com/gurugram/with-most-stps-out-of-order-haryana-continues-to-pollute-the-yamuna/story-4XTWZ9ErsWZrHvk17gWv0M.html . Accessed 16 Feb 2020.
CPCB 1982–1983 (1983) Assimilation capacity of point pollution load, CUPS/12
CPCB 1999–2000 (2000) Water quality status of Yamuna River, ADSORBS/32
Bhardwaj RM (2005) Water quality monitoring in India-achievements and constraints. IWG-Env International Work Session on Water Statistics 1–12
Sharma, A. Here We Go Again: Yamuna Action Plan Phase III [WWW Document]. Delhi Greens (2018). https://delhigreens.com/2018/12/29/here-we-go-again-yamuna-action-plan-phase-iii/ . Accessed 4 Oct 2020.
Anand, C., Akolkar, P. & Chakrabarti, R. Bacteriological water quality status of River Yamuna in Delhi. J. Environ. Biol. 27 , 97–101 (2006).
PubMed Google Scholar
Hassan, T., Parveen, S., Nabi, B. & Ahmad, U. Seasonal variations in water quality parameters of river Yamuna, India. Int. J. Curr. Microbiol. Appl. Sci 6 , 694–712. https://doi.org/10.20546/ijcmas.2017.605.079 (2017).
Mutiyar, P. K., Gupta, S. K. & Mittal, A. K. Fate of pharmaceutical active compounds (PhACs) from River Yamuna, India: An ecotoxicological risk assessment approach. Ecotoxicol. Environ. Saf. 15 (150), 297–304. https://doi.org/10.1016/j.ecoenv.2017.12.041 (2018).
Jaiswal, M., Hussain, J., Gupta, S. K., Nasr, M. & Nema, A. K. Comprehensive evaluation of water quality status for entire stretch of Yamuna River, India. Environ. Monit. Assess. 191 , 208. https://doi.org/10.1007/s10661-019-7312-8 (2019).
Kaur, L., Rishi, M. S. & Arora, N. K. Deciphering pollution vulnerability zones of River Yamuna in relation to existing land use land cover in Panipat, Haryana, India. Environ. Monit. Assess. 193 , 1–22. https://doi.org/10.1007/s10661-020-08832-y (2021).
Patel, P. P., Mondal, S. & Ghosh, K. G. Some respite for India’s dirtiest river? Examining the Yamuna’s water quality at Delhi during the COVID-19 lockdown period. Sci. Total Environ. 744 , 140851. https://doi.org/10.1016/j.scitotenv.2020.140851 (2020).
Article ADS CAS PubMed PubMed Central Google Scholar
Paliwal, R., Sharma, P. & Kansal, A. Water quality modelling of the river Yamuna (India) using QUAL2E-UNCAS. J. Environ. Manag. 83 , 131–144. https://doi.org/10.1016/j.jenvman.2006.02.003 (2007).
Krishan, G., Singh, S., Sharma, A., Sandhu, C., Grischek, T. et al. Assessment of river quality for river bank filtration along Yamuna River in Agra-Mathura districts of Uttar Pradesh. In Proceedings of National Conference on Monitoring and Management of Drinking Water Quality (MMDWQ) & XXVIII Annual Conference of National Environment Science Academy during 21–23 December, 2015 at UCOST, Dehradun (2015).
Kaur, H., Warren, A. & Kamra, K. Spatial variation in ciliate communities with respect to water quality in the Delhi NCR stretch of River Yamuna, India. Eur. J. Protistol. 79 , 125793. https://doi.org/10.1016/j.ejop.2021.125793 (2021).
UNECE (1994) Standard Statistical Classification of Surface Freshwater Quality for the Maintenance of Aquatic Life. In Readings in International Environment Statistics . United Nations Economic Commission for Europe, United Nations, New York and Geneva.
HSPCB (2018) Action Plan for River Yamuna November 2018
Satyanarayana, P. & Sharma, J. R. Web Enabled Water Resources Information System for India . ARC India News (2009).
Global Modeling and Assimilation Office (GMAO). MERRA-2 tavg1_2d_slv_Nx: 2d,1-Hourly,Time-Averaged,Single-Level,Assimilation,Single-Level Diagnostics V5.12.4, Greenbelt, MD, USA, Goddard Earth Sciences Data and Information Services Center (GES DISC) [WWW Document] (2015). https://doi.org/10.5067/VJAFPLI1CSIV . Accessed 8 Oct 2020.
Gelaro, R. et al. The modern-era retrospective analysis for research and applications, version 2 (MERRA-2). J. Clim. 30 , 5419–5454. https://doi.org/10.1175/JCLI-D-16-0758.1 (2017).
Article ADS PubMed Google Scholar
Guhathakurta, P., Narkhede, N., Menon, P., Prasad, A. K. & Sangwan, N. (2020) Observed Rainfall Variability and Changes over Haryana State. Report on ESSO/IMD/HS/Rainfall Variability/09(2020)/33.
Servais, P., Seidl, M. & Mouchel, J. M. Comparison of parameters characterizing organic matter in a combined sewer during rainfall events and dry weather. Water Environ. Res 71 , 408–417. https://doi.org/10.2175/106143097X122112 (1999).
Khan, M. Y. A., Gani, K. M. & Chakrapani, G. J. Spatial and temporal variations of physicochemical and heavy metal pollution in Ramganga River—A tributary of River Ganges, India. Environ. Earth Sci. 76 , 231. https://doi.org/10.1007/s12665-017-6547-3 (2017).
Kumar, V., Sharma, A., Thukral, A. K. & Bhardwaj, R. Water quality of River Beas, India. Curr. Sci. 112 , 1138–1157. https://doi.org/10.18520/cs/v112/i06/1138-1157 (2017).
Liu, J. et al. Spatial scale and seasonal dependence of land use impacts on riverine water quality in the Huai River basin, China. Environ. Sci. Pollut. Res. 24 , 20995–21010. https://doi.org/10.1007/s11356-017-9733-7 (2017).
Singh, K. P., Malik, A., Mohan, D. & Sinha, S. Multivariate statistical techniques for the evaluation of spatial and temporal variations in water quality of Gomti River (India)—A case study. Water Res. 38 , 3980–3992. https://doi.org/10.1016/j.watres.2004.06.011 (2004).
Prasad, B. S. R. V., Srinivasu, P. D. N., Varma, P. S., Raman, A. V. & Ray, S. Dynamics of dissolved oxygen in relation to saturation and health of an aquatic body: A case for Chilka Lagoon, India. J Ecosyst 2014 , 1–17. https://doi.org/10.1155/2014/526245 (2014).
Ravindra, K., Meenakshi, A., Rani, M. & Kaushik, A. Seasonal variations in physico-chemical characteristics of River Yamuna in Haryana and its ecological best-designated use. J. Environ. Monit. 5 , 419–426. https://doi.org/10.1039/b301723k (2003).
Download references

Acknowledgements
We would like to thank the National Water Informatics Centre (NWIC), a unit of the Ministry of Jal Shakti for providing updated data on water resources through a ‘Single Window’ source. We also thank research center O.I.E of Mines Paris Tech and ARMINES for providing meteorological data. The data used in the current study were freely available and their links are mentioned in their respective places. We express a great sense of gratitude towards the Central Pollution Control Board of India and other agencies for making data available.
This research was financially supported by the Ministry of Science and Technology (MOST) of Taiwan under the codes MOST 109-2923-E-008-004-MY2 and MOST 110-2111-M-008-008.
Author information
Authors and affiliations.
Department of Civil Engineering, SRM IST, Delhi-NCR Campus, Ghaziabad, India
Pankaj Joshi
Centre for Space and Remote Sensing Research, National Central University, Taoyuan City, Taiwan
Akshansha Chauhan & Yuei-An Liou
Department of Engineering, University of Technology and Applied Sciences, Suhar, 311, Sultanate of Oman
Department of Chemistry, SRM IST, Delhi-NCR Campus, Ghaziabad, India
Sudheer Malik
National Museum Marine Science and Technology (NMMST), No. 367, Beining Rd., Zhongzheng Dist., Keelung City, 202010, Taiwan
Yuei-An Liou
You can also search for this author in PubMed Google Scholar
Contributions
Conceptualization—A.C. and Y.-A.L., Data analysis: P.J. and A.C., Methodology: P.J., S.M., Y.-A.L., Writing—original draft by P.J., A.C., S.M., P.D.; Review and editing—A.C., P.D., and Y.-A.L.
Corresponding author
Correspondence to Yuei-An Liou .
Ethics declarations
Competing interests.
The authors declare no competing interests.
Additional information
Publisher's note.
Springer Nature remains neutral with regard to jurisdictional claims in published maps and institutional affiliations.
Rights and permissions
Open Access This article is licensed under a Creative Commons Attribution 4.0 International License, which permits use, sharing, adaptation, distribution and reproduction in any medium or format, as long as you give appropriate credit to the original author(s) and the source, provide a link to the Creative Commons licence, and indicate if changes were made. The images or other third party material in this article are included in the article's Creative Commons licence, unless indicated otherwise in a credit line to the material. If material is not included in the article's Creative Commons licence and your intended use is not permitted by statutory regulation or exceeds the permitted use, you will need to obtain permission directly from the copyright holder. To view a copy of this licence, visit http://creativecommons.org/licenses/by/4.0/ .
Reprints and permissions
About this article
Cite this article.
Joshi, P., Chauhan, A., Dua, P. et al. Physicochemical and biological analysis of river Yamuna at Palla station from 2009 to 2019. Sci Rep 12 , 2870 (2022). https://doi.org/10.1038/s41598-022-06900-6
Download citation
Received : 08 December 2021
Accepted : 07 February 2022
Published : 21 February 2022
DOI : https://doi.org/10.1038/s41598-022-06900-6
Share this article
Anyone you share the following link with will be able to read this content:
Sorry, a shareable link is not currently available for this article.
Provided by the Springer Nature SharedIt content-sharing initiative
This article is cited by
Water quality modeling-based assessment for the scope of wastewater treatment of the urban reach of river yamuna at delhi, india.
- Nibedita Verma
- Geeta Singh
- Naved Ahsan
Environmental Monitoring and Assessment (2024)
By submitting a comment you agree to abide by our Terms and Community Guidelines . If you find something abusive or that does not comply with our terms or guidelines please flag it as inappropriate.
Quick links
- Explore articles by subject
- Guide to authors
- Editorial policies
Sign up for the Nature Briefing newsletter — what matters in science, free to your inbox daily.

- Previous Article
- Next Article
INTRODUCTION
Materials and methodology, results and discussion, conclusions, acknowledgements, data availability statement, drinking water quality assessment of river ganga in west bengal, india through integrated statistical and gis techniques.

- Article contents
- Figures & tables
- Supplementary Data
- Open the PDF for in another window
- Guest Access
- Cite Icon Cite
- Permissions
- Search Site
Syed Yakub Ali , Sangeeta Sunar , Priti Saha , Pallavi Mukherjee , Sarmistha Saha , Suvanka Dutta; Drinking water quality assessment of river Ganga in West Bengal, India through integrated statistical and GIS techniques. Water Sci Technol 15 November 2021; 84 (10-11): 2997–3017. doi: https://doi.org/10.2166/wst.2021.293
Download citation file:
- Ris (Zotero)
- Reference Manager
An attempt has been made to assess the water quality status of the lower stretch of river Ganga flowing through West Bengal for drinking using integrated techniques. For this study, 11 parameters at 10 locations from Beharampur to Diamond Harbour over nine years (2011–2019) were considered. The eastern stretch of Ganga showed a variation of Water Quality Index (WQI) from 55 to 416 and Synthetic Pollution Index (SPI) from 0.59 to 3.68 in nine years. The result was endorsed through a fair correlation between WQI and SPI (r 2 > 0.95). The map interpolated through GIS revealed that the entire river stretch in the year 2011, 2012, and 2019 and location near to ocean during the entire period of nine years were severely polluted (WQI > 100 or SPI > 1). Turbidity and boron concentration mainly contribute to the high scores of indices. Further, the origin of these ions was estimated through multivariate statistical techniques. It was affirmed that the origin of boron is mainly attributed to seawater influx, that of fluoride to anthropogenic sources, and other parameters originated through geogenic as well as human activities. Based on the research, a few possible water treatment mechanisms are suggested to render the water fit for drinking.
The study provides a base line assessment of the water quality of river Ganga for drinking.
Water quality was marked as polluted and unfit for drinking.
The seawater influx, geogenic and anthropogenic activities were assessed as the major sources of pollution.
Water treatment technologies were suggested to render the water fit for drinking.
It will be helpful to formulate appropriate management strategies.
River Ganga, internationally known as The Ganges, is one of the major rivers in India and plays a pivotal role in sustaining the lives of millions of people both physically as well as spiritually. It was declared as the ‘National River of India’ and drains approximately one-fourth of the Indian territory. It flows through Uttarakhand, Uttar Pradesh, Bihar, Jharkhand and West Bengal, covering a length of 2,525 km, and further drains into the Bay of Bengal. The towns on the river banks support about 37% of the urban population.
Over a period of time, the quality of water of the river has deteriorated due to pollution from various point and non-point sources resulting from increasing population density, rapid industrialization, unplanned urbanization, and rising living standards. Untreated industrial effluents, enormous amounts of municipal sewage, agricultural runoff, open defecation, and other waste, including polythenes and dead bodies, are being released into the river indiscriminately, irrespective of its carrying capacity. Despite the alarming level of pollution, untreated water is still used for various purposes, which may impact human health. Therefore, water quality assessment is essential as the initial step towards generating public awareness and aiding the planners and government authorities to conserve and manage the water bodies.
Water quality assessment through quantification of various physico-chemical parameters is very difficult to understand in its primary form and barely represents the actual scenario. Therefore, an assessment tool is required to integrate all the parameters scientifically to represent water quality through a single numerical value. Among many assessment tools used for water quality analysis, the Water Quality Index (WQI) is the most popular indexing tool that integrates all the parameters while comparing with the standards recommended by the government authorities to safeguard human health ( Ewaid et al. 2020 ; Nong et al. 2020 ). It was developed by Horton (1965) , in which arithmetic weighting was used with multiplicative variables ( Horton 1965 ; Avvannavar & Shrihari 2008 ; Kumar & Dua 2009 ; Lkr et al. 2020 ). Further, many modifications were done according to requirement, and many new indices were developed, such as the National Sanitation Foundation-WQI (NSFWQI), Oregon Water Quality Index, Canadian Council of Ministers of the Environment-WQI (CCME-WQI), British Columbia Water Quality Index (BCWQI), Overall Index of Pollution (OIP), WQI by Bhargava, etc. ( Poonam et al. 2013 ). These indices were also applied to assess the water quality of the river systems in India, including various stretches of river Ganga ( Samantray et al. 2009 ; Sharma & Kansal 2011 ; Saha et al. 2012 ; Shah & Joshi 2017 ; Shukla et al. 2017 ; Mitra et al. 2018 ; Ghosh et al. 2019 ; Kamboj & Kamboj 2019 ; Lkr et al. 2020 ).
Another promising tool, the Synthetic Pollution Index (SPI), was also evaluated in this study to validate the pollution level assessed by WQI. It was developed by Ma et al. (2009) to assess the impact of pollutants on the water quality and was later adopted in various studies due to its simplicity ( Solangi et al. 2019 ; Hui et al. 2020 ; Sunar et al. 2020 ).
Although the indices depict water quality, the numbers do not make the general public visualize the water quality status. Therefore, integration of the data set with a geographic information system (GIS) can help in illustrating the water quality status of river Ganga to the general public, policy makers or stakeholders. This will make the research output more approachable and understandable to the policy makers and general public. Pollution source identification through various statistical techniques such as factor analysis (FA) and cluster analysis (CA) also helps the policy makers and government to control water pollution ( Bhatti et al. 2019 ; Saha & Paul 2019a ).
Since river Ganga is listed as the sixth most polluted river in the world ( Panigrahi & Pattnaik 2019 ), a number of studies have been carried out to asesss the water quality of river Ganga ( Tiwary et al. 2005 ; Mishra 2010 ; Bhutiani et al. 2016a , 2016b ; Shukla et al. 2017 ; Kamboj & Kamboj 2019 ; Matta et al. 2020 ). However, the use of WQI and SPI together for evaluation of the water quality of Ganga river is limited. Therefore, the present study aims to assess the water quality of river Ganga flowing in the state of West Bengal, India based on the monitoring data of the environmental regulatory board of West Bengal during 2011–2019 by using integrated techniques. The result was validated through a fair correlation between WQI and SPI (r 2 > 0.95), which authenticates the extent to which the water is suitable for drinking. This represents a pioneer study to assess the drinking water quality using these integrated techniques in the mentioned stretch of the river. Further, the pollution sources are identified and thereby water treatment techniques to safeguard the human health are proposed. This base line assessment of drinking water quality of lower stretch of river Ganga may assist the policy makers to formulate appropriate management strategies for pollution abatement of the river.
This study is conducted in the lower stretch of River Ganga, flowing in the state of West Bengal, India, between 87°55′24.315″ E, 24°48′20.227″N and 87°46′10.382″E, 21°41′28.733″N ( Figure 1 ). A total of ten sampling locations were selected for this study, which were strategically decided by Central Pollution Control Board (CPCB) for effective monitoring of the river ( Table 1 ). The river basin experiences subtropical humid climate with three distinct seasons, namely cold, hot dry season, and monsoon, with an average rainfall of about 1,500 mm. The geology comprises newer alluvium (Holocene sediments) as well as older alluvial (Middle-Upper Pleistocene sediments). Extensive pollution resulting from anthropogenic activities such as sewage as well as untreated industrial effluents discharge, large scale idol immersion, mass bathing during religious festivals, disposal of dead bodies etc. has caused massive deterioration in the water quality of the river. This stretch also receives more than 87 MLD (millions of litres per day) waste water from 22 grossly polluting industries, where the chemical industry discharges about 70% of the total waste water, followed by the pulp-paper industry with 20% discharge ( CPCB 2013 ). The remaining percentage includes other industries such as distillery; food, dairy and beverage; sugar; textile and bleaching and dyeing.
Details of monitoring station of river Ganga in the West Bengal Stretch ( CPCB 2013 )

Map showing study area and sampling locations.
In spite of these polluting activities, this stretch always remains as the primary source of drinking water in many cities of Murshidabad, Baharampur, Bardhaman, Nadia, Hooghly, North 24 Parganas, Kolkata, and Howrah districts. Moreover, Kolkata has three water works, namely Palta, Garden Reach and Baranagar, where a total of 1,136 million litres/day of river water is treated and supplied to the habitats ( UNU 2000 ). Similarly, Howrah has two water works, Padhmapukur and Serampore, supplying about 270 million litres of water/day ( UNU 2000 ). Therefore, water quality assessment and documentation of the management practices are required to safeguard human health.
Standards recommended by WHO and unit weightage of the parameters used for WQI and SPI
All parameters are units are in mg/L except pH, TH (mg/L as CaCO 3 ). TH: total hardness.
Categorization of WQI and SPI for estimation of water quality
The critical value of SPI was evaluated by assigning V o of the water quality parameter as the recommended standard, beyond which the water is unsuitable for drinking. By integrating all the water quality polluting parameters selected in the study with the unit weightage through Equation ( 4 ), the critical value estimated was 1. Further, the categorization was done based on four equal quartiles ( Table 3 ).
Geospatial assessment
Source identification.
The Kaiser Normalization (PC with eigen values >1) along with varimax rotation was followed in this study for better interpretability of results. Moreover, Bartlett's test of sphericity and Kaiser–Meyer–Olkin (KMO) test were also performed to test the significance of these multivariate statistics. PC > 0.5 showed most significant correlated variables, which helps in identification of anthropogenic or geogenic sources.
All statistical analyses were performed using software SPSS 21.0 version.
Physico-chemical analysis
In the present study, a detailed analysis of physico-chemical parameters is conducted for assessment of water quality of the mentioned stretch of river Ganga comprising ten locations as illustrated in box plot ( Figure 2 ) displaying the six-number summary (minimum, first quartile, mean, median, third quartile, and maximum) of the data set from the years 2011–2019. Apart from this, one way analysis of variance (ANOVA) was performed using Tukey test, with 95% level of confidence ( α :0.05) ( Table 4 ) to confirm the significant difference between the sampling sites. The descriptive statistics of all the parameters considered in this study for the year 2011–2019 are presented in Table 5 .
Test of ANOVA for the parameters within the years (2011–2019) and sampling locations
Digits in bold represent statistical significance.
Descriptive statistics of physico-chemical parameters of all the sampling locations in the lower stretch of River Ganga from 2011 to 2019
The concentrations of all parameters are in mg/L, except pH: unitless, turbidity: NTU, TH (total hardness): mg CaCO 3 /L.

Box plot of (a) ammonia (mg/L), (b) boron (mg/L), (c) chloride (mg/L), (d) pH, (e) fluoride (mg/L), (f) nitrate (mg/L), (g) Temperature (°C), (h) sodium (mg/L), (i) sulfate (mg/L), (j) TDS (mg/L), (k) total hardness (mg CaCO 3 /L), (l) Turbidity (NTU).
The pH of the river ranged from 7.31 to 8.15 ( Figure 2(d) ), which follows the drinking water quality standard recommended by WHO (7.0–8.5). The ANOVA ( Table 4 ) showed that there is no significant statistical difference of pH between the sampling locations, which may be due to low annual variation in free CO 2 ( Gupta et al. 2017 ). Moreover, the alkaline nature is due to the influx of domestic and industrial waste water into the river and photosynthetic algae activities that consume CO 2 dissolved in water ( Driche et al. 2008 ). This result is similar to the study of Sarkar et al. (2007) for Hoogly River (lower trench of Ganga River), which showed the pH variation from 7.2 to 8.9. The (TDS) in the study area ranged from 158 to 3,066 mg/L ( Figure 2(j) ), wherein the majority of sampling locations fit in the prescribed limit of WHO (600 mg/L) except Diamond Harbour. The high amount of TDS at Diamond Harbour is due to sea water intrusion during high tide, as it is located 70 km upstream from the sea. Similar result was reported by Sarkar et al. (2007) , where the maximum TDS concentration at Diamond Harbour was given as 2.55 ppt. The turbidity of the river varied from 43.6 to 350.82 Nephelometric Turbidity Units (NTU) ( Figure 2(l) ) and the highest value was found in Diamond Harbour, which showed a significant difference ( p < 0.05) from the other locations. Turbidity results from the presence of suspended particles such as clay, silt, organic matter, plankton and other microscopic organisms in the water ( Grobbelaar 2009 ). The clarity of water decreases due to the presence of these suspended particles that get deposited in the water. Total hardness (TH) ranged from 88 to 950 mg CaCO 3 /L ( Figure 2(k) ), where all the locations were within the drinking water standard limits of 200 mg CaCO 3 /L as recommended by WHO ( WHO 2017 ), except Diamond Harbour (950 mg CaCO 3 /L). Sodium concentration in the river water was significantly high ( p < 0.05) in Diamond Harbour, with a mean range from 310 to 992 mg/L ( Figure 2(h) ), whereas in other sampling locations it was within the drinking water standard limit of 200 mg/L, recommended by WHO ( WHO 2017 ). Hypertension and cardio-metabolic diseases may result from the intake of excessive sodium in drinking water along with normal dietary sodium consumption ( Nwankwo et al. 2020 ), although no prominent relation of the level of sodium to hypertension can be affirmed conclusively and therefore no health-based guideline is available in this regard ( WHO 2003 ). Moreover, major anions such as chloride and sulfate were also significantly high ( p < 0.05) in Diamond Harbour due to its vicinity to the estuary, with maximum concentration of 1,511.47 mg/L ( Figure 2(c) ) and 211.13 mg/L, respectively ( Figure 2(i) ). The occurrence of the major ions in river water is mainly from geogenic sources such as weathering of minerals ( Asare-Donkor et al. 2018 ). However, the mean ammoniacal nitrogen (NH 3 -N) showed a significantly high level at Serampore as compared to the other locations. It ranged from 0 to 0.53 mg/L ( Figure 2(a) ), which was below the permissible limit for drinking water standard recommended by WHO (1.5 mg/L). Ammonia enters into the aquatic environment via direct means such as municipal effluent discharge and excretion of nitrogenous waste from animals and indirect means such as nitrogen fixation, air deposition and runoff from agricultural lands ( EPA 2013 ). In addition, the nitrate in Ganges river mainly comes from domestic sewage, industrial wastewater, agriculture fertilizer and aquaculture ( Sarkar et al. 2007 ). Low values of nitrate nitrogen (mean ranging from 0.25 to 1.11 mg/L) were also observed in all the sites ( Figure 2(f) ), which may be due to utilization by phytoplankton and other primary producers. Boron concentration in the study area ranged from 0 to 0.39 mg/L ( Figure 2(b) ). The ANOVA reflected no significant difference ( p > 0.05) between the locations. However, maximum boron concentration was found in Dakhineswar followed by Garden Reach and Diamond Harbour. The significant increase in the boron content of surface water can be attributed to the input of waste water with washing agents containing borate compounds or leaching from sediment or rock containing borates or borosilicates ( WHO 1998 ). The fluoride concentration (mean ranged from 0.17 to 0.74 mg/L) ( Figure 2(e) ) showed no significant difference ( p > 0.05) among all the sampling locations. Although small concentrations of these ions are necessary for the human system, when present at high concentration in drinking water they may cause various acute or chronic diseases.
The values of these parameters cannot discretely conclude the extent of deviation of the river water quality from drinking standard. Therefore, this study was conducted to assess the status of the river water quality applying WQI and SPI, and the pollution sources were identified through multivariate statistical tools, thereby suggesting appropriate treatment measures required to render the water suitable for drinking.
Water quality assessment through WQI and SPI
WQI was evaluated for nine consecutive years (2011–2019) for the mentioned stretch of river Ganga in Eastern India. The results of WQI ( Figure 3 ) and SPI ( Figure 4 ) were interpolated through IDW geostatistics using ArcGIS for better interpretability of results. The WQI of the eastern stretch of Ganga from Beharampur to Diamond Harbour ranged from 107 to 416 in 2011, 117 to 289 in 2012, 85 to 346 in 2013, 115 to 259 in 2014, 80 to 258 in 2015, 55 to 378 in 2016, 67 to 182 in 2017, 57 to 427 in 2018 and 104 to 272 in 2019. The water quality over the mentioned time period was not at all found to be suitable for drinking without advanced treatment. A similar result was obtained for SPI, which ranged from 0.93 to 3.47 in 2011, 1.17 to 2.64 in 2012, 0.85 to 3.30 in 2013, 1.15 to 2.62 in 2014, 0.80 to 2.50 in 2015, 0.94 to 3.68 in 2016, 0.67 to 1.73 in 2017, 0.59 to 4.27 in 2018 and 1.04 to 2.62 in 2019. Figures 3 and 4 indicated that the entire river stretch was severely polluted (WQI > 100 or SPI > 1) in the years 2011, 2012 and 2019. However, the water quality improved in the stretches surrounding the sampling locations: S3 (Triveni) in 2013, S5 (Sreerampur); S6 (Dakhineswar) and S9 (Uluberia) in 2015, S2 (Nawadip); S3 (Triveni); S4 (Palta) and S5 (Sreerampur) in 2016, S2 (Nawadip); S5 (Sreerampur) and S7 (Shivpur) in 2017, S5 (Sreerampur) and S7 (Shivpur) in 2018, although it continued to remain polluted (WQI: 75–100 or SPI: 0.75–1), which signified that the usage can only be achieved with primary as well as secondary treatment. Moreover, the water quality improved in a few sampling locations across the stretches around S1 (Beharampur) in 2016, S6 (Dakhineswar) and S8 (Garden Reach) in 2017, S1 (Beharampur); S6 (Dakhineswar); S8 (Garden Reach) and S9 (Uluberia) in 2018, indicating the usage of water for drinking by primary treatment followed by disinfection. However, the water quality again deteriorated in 2019. The sampling site S10 (Diamond Harbour) showed the worst water quality throughout the entire period of nine years due to intrusion of sea water from Bay of Bengal during high tides. Since the river flows further downstream through numerous towns and cities to enter the state of West Bengal, it seemingly acquires more pollution load before draining into Bay of Bengal, as obtained in this study. Sharma et al. (2014) in their study demonstrated through the application of WQI that Ganges river at various locations in Allahabad stretch showed inferior water quality for drinking purpose.

Water quality index (WQI) of the river Ganga in eastern India for suitability assessment for drinking in subsequent years (2011–2019).

Synthetic pollution index (SPI) of the river Ganga in eastern India to validate the suitability for drinking in subsequent years (2011–2019).
The higher values of WQI or SPI in these stretches of Ganga were attributed to turbidity and boron concentration. Further, the relation between WQI and SPI was evaluated through regression model ( Table 6 ). The result confirmed the significant good relation between these two indexing tools, with r 2 > 0.9 in all the years.
Regression relation between WQI and SPI for the years 2011–2019
Pollution source identification
The WQI and SPI both affirmed the water quality of river Ganga as polluted, which requires treatment for drinking. However, the application of the treatment methodology is not feasible until the pollution sources are identified. Therefore, identification of pollution sources was done through multivariate statistics, FA supported by CA. The FA in this study follows Kaiser Normalization, where PCs greater than 1 were retained. It reduces the data set to four PCs, with a cumulative variance of 87.12 and KMO value of 0.792 > 0.5, affirming its statistical significance ( Table 7 ). The result of Principal Component Analysis (PCA) is presented as a component loading plot in Figure 5 . The components are indicated by colour code ( Figure 5 ). The first component (PC1) comprised Cl − , SO 4 2− , TDS, TH, Na + and turbidity, with an eigen value of 5.07 and maximum variance of 50.74. Around 35.5% of the samples have Na/Cl > 1, indicating silicate weathering from the alluvium deposits of Gangatic plains ( Frings et al. 2015 ), around 22.2% of samples have Na/Cl between 0.86 and 1, indicating seawater intrusion ( Shammi et al. 2017 ), while the rest originates from anthropogenic sources. The sulphates originate from the sedimentary sulphur containing minerals such as dolomite and gymsum as geogenic sources apart from other industrial and sewage effluents ( Sarin et al. 1989 ; Chakrapani & Veizer 2006 ; Dwivedi et al. 2018 ). TH comprises Ca 2+ , Mg 2+ , CO 3 2− and HCO 3 − , which dilutes in the water from the silicate, calcite and dolomite weathering as well as from the mass bathing and washing with detergents, discharge of industrial effluents and domestic sewage ( Sarin et al. 1989 ; Nath et al. 2017 ). The second component, PC2, is attributed to NH 3 -N and F − , with a variance of 13.66. The agricultural runoff, untreated industrial effluents, and domestic and municipal fresh sewage increase the level of fluoride and nitrate in the river water ( Khullar 2004 ; Mandal et al. 2010 ; Dubey & Ujjania 2013 ; Sankhla & Kumar 2018 ). The third component (PC3) comprised only boron (B), with a variance of 11.56. The origin of B may be attributed to the sea water intrusion during the tides, which can also be acknowledged by its high concentration in the location S10 (Diamond Harbour). The content of boron decreases in the stretch of river with increase in distance from the Bay of Bengal. Moreover, the content of boron is also evident in different parts of Bay of Bengal, which intrude in to fresh water during high tides ( Saxena et al. 2004 ; Gupta & Gupta 2015 ; Shammi et al. 2017 ; Danish et al. 2019 ). The fourth component (PC4) comprised NO 3 − -N only, with approximately the same variance as PC3, 11.15 and eigen value of 1.11. The nitrate is derived from the oxidation of ammonia in sewage, agricultural runoff, industrial effluents, etc. by autotrophic bacteria ( Indirani 2010 ; Deshmukh 2013 ; Dubey & Ujjania 2013 ; Mitra et al. 2018 ). Therefore, the presence of nitrate in water reveals that the water got polluted a long time ago. The result of PCA is also shown as a component loading plot, where the same colour indicates same component(s) ( Figure 5 ). This result is supported by cluster analysis, which was performed by wards linkage and euclidian distance measure ( Figure 6 ). The dendrogram shows close linkage between SO 4 2− , TDS, Cl − , Na + , TH and turbidity as the first group, which is attributed to geogenic and anthropogenic sources. On the other hand, NH 3 -N and F − are present in the second group, which might be attributed to anthropogenic activities. NO 3 − -N, which might be derived from ammoniacal sources, forms the third linkage, and boron as fourth linkage is attributed to sea water intrusion from Bay of Bengal.
Components of factor analysis to estimate the pollution sources
Extraction Method: Principal Component Analysis.
Rotation Method: Varimax with Kaiser Normalization.
Rotation converged in five iterations; digits in bold represent strong component loading.

Component loading plots of factor analysis (FA) to estimate the origin of pollutants.

Dendrogram for physio-chemical parameters of river Ganga (2011–2019).
Proposed water quality management practice
The water needs proper treatment such as screening/straining followed by primary sedimentation. Since the water showed high level of turbidity, coagulation-flocculation can be incorporated after primary sedimentation in the treatment plant as a vital practice. Here, aluminium-sulphate (alum), a cost effective and widely available coagulant, can be used to lower the turbidity. Higher amounts of boron can be treated using polymer coagulants such as polyvinyl alcohol, glucoheptanamide derivatives of poly(amidoamine) and poly(ethyleneimine), poly(glycidyl methacrylate) and poly-N,N′-diallyl morpholinium bromide modified with hydroxyethylaminoglycerol, hydroxyethylaminoglycerol functionalized to poly(glycidyl methacrylate) and poly(4-vinyl-1,3-dioxalan-2-one-co-vinyl acetate), alkyl monol, diol or triol containing polyethylenimines ( Wolska & Bryjak 2013 ). Apart from this, defluoridation of water can be done using most effective and conventional treatment with lime and alum (Nalgonda technique). This step should be followed by secondary sedimentation with optimum sedimentation time. Finally, before using the water for drinking, disinfection should be done through chlorination and filtration.
Apart from this, direct disposal of sewage in Ganga should be checked. The wastewater should at first be treated in Sewage Treatment Plants through necessary steps before discharging it into the river. Solid waste dumping should be prevented in the river to improve the water quality. Cremation as well as devotional activities such as idol immersion etc. should be checked in the river banks. The use of river water for domestic activities such as washing clothes, utensils etc. with detergents should be limited. The main source of pollution of river Ganga is the industries, which should be regularly monitored for zero discharge of wastewater and the efficiency of the effluent treatment plants. These measures can contribute towards ameliorating water pollution and restoring the water quality of the divine river Ganga.
This study may lead the way towards future research for validation of these treatment technologies for rendering Ganga river water potable. Apart from this, quantitative assessment of a few important parameters such as heavy metals, pesticides, etc. might be evaluated on a monthly basis for better assessment of the river water. This information can also be focused for further assessment of drinking water quality in the lower stretch of the river.
This constitutes a pioneer study for water quality assessment of the eastern stretch of one of the most polluted rivers in India, the Ganga (flowing through the State of West Bengal) by the application of WQI and SPI, and the data set is integrated with GIS for depicting the status of pollution in a better manner. The water quality assessed through these integrated techniques affirmed that the mentioned stretch of River Ganga is polluted and not suitable for drinking without appropriate treatment. A few parameters such as boron and turbidity were identified as critical polluting parameters responsible for deterioration in the water quality. The multivariate statistical techniques (FA and PCA) indicated that the presence of boron is attributed to sea water intrusion; whereas turbidity originates from silicate weathering from the alluvium deposits of Gangetic plains as well as anthropogenic activities (such as mass bathing and washing with detergents, discharge of industrial effluents and domestic sewage). Based on the water quality status and origin of the polluting factors, this study also suggested various treatment techniques. The outcome of this study and the suggested techniques can be used by the regulatory authority for documentation of management strategies and framing of effective water quality management plans to combat the pollution of the mighty river Ganga.
We would like to thank West Bengal Pollution Control Board for the online data for water quality of river Ganga. We would also like to thank Dr Supriyo Goswami (IEST Shivpur) for valuable contribution.
All relevant data are included in the paper or its Supplementary Information.
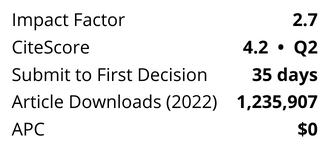
Affiliations
- ISSN 0273-1223 EISSN 1996-9732
- Open Access
- Collections
- Subscriptions
- Subscribe to Open
- Editorial Services
- Rights and Permissions
- Sign Up for Our Mailing List
- IWA Publishing
- Republic – Export Building, Units 1.04 & 1.05
- 1 Clove Crescent
- London, E14 2BA, UK
- Telephone: +44 208 054 8208
- Fax: +44 207 654 5555
- IWAPublishing.com
- IWA-network.org
- IWA-connect.org
- Cookie Policy
- Terms & Conditions
- Get Adobe Acrobat Reader
- ©Copyright 2021 IWA Publishing
This Feature Is Available To Subscribers Only
Sign In or Create an Account
Advertisement
Real-time assessment of the Ganga river during pandemic COVID-19 and predictive data modeling by machine learning
- Original Paper
- Published: 27 July 2022
- Volume 20 , pages 7887–7910, ( 2023 )
Cite this article
- J. Singh 1 ,
- S. Swaroop 1 ,
- P. Sharma 1 &
- V. Mishra ORCID: orcid.org/0000-0002-2877-7693 1
2121 Accesses
2 Citations
Explore all metrics
In this study, four water quality parameters were reviewed at 14 stations of river Ganga in pre-, during and post-lockdown and these parameters were modeled by using different machine learning algorithms. Various mathematical models were used for the computation of water quality parameters in pre-, during and post- lockdown period by using Central Pollution Control Board real-time data. Lockdown resulted in the reduction of Biochemical Oxygen Demand ranging from 55 to 92% with increased concentration of dissolved oxygen at few stations. pH was in range of 6.5–8.5 of during lockdown. Total coliform count declined during lockdown period at some stations. The modeling of oxygen saturation deficit showed supremacy of Thomas Mueller model ( R 2 = 0.75) during lockdown over Streeter Phelps ( R 2 = 0.57). Polynomial regression and Newton’s Divided Difference model predicted possible values of water quality parameters till 30th June, 2020 and 07th August, 2020, respectively. It was found that predicted and real values were close to each other. Genetic algorithm was used to optimize hyperparameters of algorithms like Support Vector Regression and Radical Basis Function Neural Network, which were then employed for prediction of all examined water quality metrics. Computed values from ANN model were found close to the experimental ones ( R 2 = 1). Support Vector Regression-Genetic Algorithm Hybrid proved to be very effective for accurate prediction of pH, Biochemical Oxygen Demand, Dissolved Oxygen and Total coliform count during lockdown.
Similar content being viewed by others
Water Quality Modelling and Parameter Assessment Using Machine Learning Algorithms: A Case Study of Ganga and Yamuna Rivers in Prayagraj, Uttar Pradesh, India
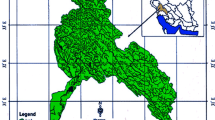
Prediction of the five-day biochemical oxygen demand and chemical oxygen demand in natural streams using machine learning methods
Mohammad Najafzadeh & Alireza Ghaemi
An Approach for Predicting River Water Quality Using Data Mining Technique
Avoid common mistakes on your manuscript.
Introduction
With the outbreak of the coronavirus pandemic, the life of people is adversely affected. COVID-19 came into light in December 2019 from Wuhan city in Hubei Province of China (Hasnain et al. 2020 ). It affects the respiratory tract and spreads from person to person through physical contact. As researchers are not sure about its source, having not discovered a vaccine to date, no specific treatment is known yet (Chakraborty and Maity 2020 ). The only options left with the public are social distancing, lockdown and personal hygiene. COVID-19 pandemic has severely affected countries like Italy, the USA, Pakistan, China, Germany and India etc. and their respective Government applied lockdown strictly (Paul et al. 2020 ).
As a consequence, people remained indoors and commercial activities were shut down (Wray 2020 ). India was also under lockdown in the wake of coronavirus pandemic. Restrictions on industrial activities during lockdown significantly lowered air and water pollution. This resulted in the substantial rejuvenation of rivers with a positive impact on stable marine life. During lockdown, the water quality of the Ganga river has improved significantly (Singh 2020 ). Lockdown has caused a reduction in the disposal of hazardous wastes not only in the Ganga but also in other rivers. The Ganga or Ganges is a 1,680 miles long river in India that originates from the Gangotri Glacier of the western Himalayas in Uttarakhand and the river flows from the northwest to the southeast, merges into the Bay of Bengal. In India, it covers states such as Uttarakhand, Uttar Pradesh, Bihar and West Bengal (Chaturvedi 2012 ). The Ganga is the lifeline of millions who live along the way. Approximately 43% of India's population lives in the Ganga basin, which is over 860,000 km 2 and covers 26.3% of the country's total geographical area (Trivedi 2010 ). It is a sacred river, worshipped as the goddess Ganga in the Hinduism, which witnesses high religious and cultural tourism on its banks. In 2008, the Ganga river declared was the ‘National River’ of India (Sati 2021 ). There are over 29 cities, 97 towns and thousands of villages on the banks of the Ganga River (Dutta et al. 2020 ).
It hosts about 140 species of fish and 90 species of amphibians. For most of its course, it is a wide and sluggish stream that flows through one of India's most fertile and densely populated regions. The major contributors of pollution are tanneries in Kanpur, distilleries, paper mills and sugar mills in the Yamuna, Ramganga, Kosi and Kali river catchments (Dutta et al. 2020 ). There has been a decrease in fish population along the river, indicating a lack of supportive habitat and water quality degradation. Fishermen report destructive fishing, overfishing and the construction of Farakka barrage as the significant reasons for the decline in fish population from the river-floodplain in Bihar (Dey et al. 2019 ). In 2017, the river Ganga was considered to be sixth most polluted river in the world (Paul 2017 ). Lots of steps have been taken to clean the river, but the desired results have not been achieved to date. Drew ( 2017 ) mentioned that there are numerous hydropower stations, dams and barrages in the main stem of the Ganga river and its tributaries that are harming and obstructing the flow of the river. Apart from this, construction and widening of roads and tunnels in the upper Ganga region affects the flow of water and leaves the river bed dry. The author termed this as “destructive model of development” and added that the continuous inflow of untreated wastewater in the Ganga, including untreated sewage and hazardous waste from the industry as well as agricultural runoff, is worsening the water quality of the river (Drew 2017 ).
The river Ganga passes through states that serve the various subsistence needs of people living in the surrounding areas, such as drinking, bathing, fishing and agriculture. Despite being one of the most functionally important rivers in the world, serving an estimated 500 million people, the Ganga is contaminated in large amounts by the discharge of untreated wastewater and untreated industrial waste (Postel and Richter 2012 ). High population density at the basin, several festive celebrations at the shore, garbage disposals and dumping of corpses directly into the river Ganga have contributed most to its pollution. The river also serves the agriculture in the surrounding region and therefore ends up with a vast amount of chemical fertilizers, pesticides and insecticides that worsen its quality (Chakraborty 2021 ). A non-point category source of pollution, that is, open defecation, is a significant and worrying cause of the disease-causing microorganisms that dwell in the river Ganga. In the river beyond Kanpur, fecal coliform levels have crossed the acceptable bathing standard (Srinivas et al. 2020 ). High pollution level increases the chances of obstructions, ultimately leads to stagnant water condition which breeds diseases such as dengue, malaria and chikungunya. These deadly diseases take millions of lives and cost the country colossal capital every year. The harmful microorganisms originating from fecal pollution are also suspected of having a pivotal role in antibiotic resistance (Lockwood 2016 ). The government has focused on pollution point source control policies (Srinivas et al. 2020 ), but no significant improvement has not yet been seen so far.
In this study, changes in water quality of the river Ganga have been evaluated during the lockdown phase and compared with pre-lockdown statistics. Bioinspired mathematical models such as Streeter Phelps, Thomas Mueller, Support Vector Regression with Genetic Algorithm (SVR-GA), Lasso regression, Artificial neural network (ANN), Newton’s divided difference (NDD) and Polynomial regression model have been used for the computation of water quality parameters in the river water under both pre-lockdown and during lockdown conditions. Streeter Phelps and Thomas Mueller model were utilized for predicting oxygen saturation deficit in the river Ganga . In addition to this, SVR-GA, Lasso regression and ANN were implemented to model levels of DO, BOD, pH and TC in the Ganga river. Finally, NDD and Polynomial regression models have been used to predict water quality parameters (DO, BOD, pH and TC) in the present condition and future changes in the water quality of the river Ganga such as after unlocking phase-I in India, i.e., 30th June 2020 based on the past trends. SVR-GA is a hybrid algorithm which uses a hyperparameter optimization algorithm (GA) along with a modeling algorithm (SVR) (Jiang et al. 2013 ). The ability of SVR marked by its margin approach is well suited for all kinds of data and has been successfully used for the modeling of pH and DO before. Lasso Regression model, which has a shrink or reject feature is advantageous when dealing with regression data. This model originates from Ridge regression and is a robust regression algorithm which was also used for lockdown data prediction.
ANN is an oversimplified version of the inter-neuron communication process that takes place in the brain. Their architecture depends on the number of hidden layers and the activation functions, thus leaving a room for improvisation and experimentation (Ahmed 2017 ). A highly interconnected neural network is very effective for accurate predictions. Still, it tends to over fit on the training data, that is why smaller and effective neural network models have been developed (Sarkar and Pandey 2015 ). One such model is the Radical Basis Function Neural Network (RBF-NN) is a simple one hidden layer ANN which uses a radical basis as its activation function. In the present study, the RBF-NN model, Levenberg–Marquardt algorithm (LMA) and a two hidden layer Multi-Layer Perceptron (MLP) model for prediction of water quality data have been applied. The RBF-NN model was used with GA as the optimizer of its hyperparameters. GA selects a random population based on the specified constraints and picks out the best possible pair of parameters which have the highest fitness. The GA fitness function has been represented with mean squared error (MSE) in the present work. The present study will be useful in developing technologies for reducing the pollution level in the river Ganga and other rivers, preventing it from returning to the previous state based on the data available from these models. This study is also helpful in formulating/revising the laws dealing with a permissible limit of discharge of industrial effluents in the river Ganga and other natural water resources. The entire analytical study of the Ganga river by using CPCB data was conducted at IIT (BHU) Varanasi (Co-ordinate 25° 15′ 30″ N 82° 59′ 39″ E) Varanasi, India.
Ganga river (literature survey before and during lockdown)
Before lockdown, the river Ganga was not suitable for bathing from Uttar Pradesh to West Bengal with the exception of certain places in Uttarakhand (Webdesk 2020 ). Figure 1 shows the sources of pollution in the river Ganga.
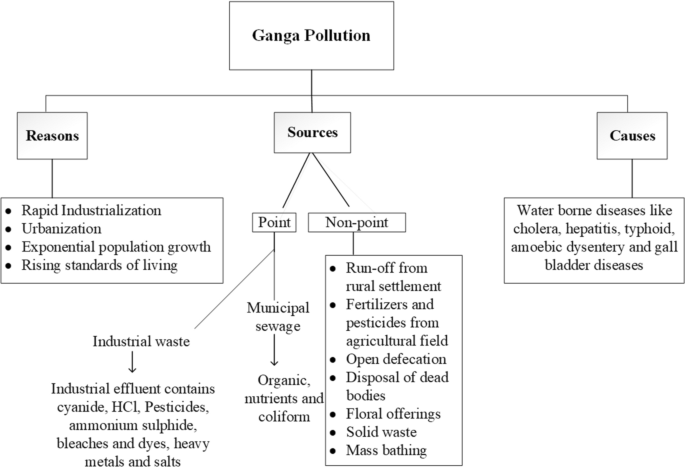
Ganga pollution overview
Over 500 water samples from April to June were analyzed for two consecutive years, i.e., 2017 and 2018 (Haider Naqvi 2020 ). The amount of DO decreased to less than 2 mg/L due to the hypoxic state of the river bed, which made the river unable to sustain aquatic life. The river Ganga has been used for dumping of industrial and domestic waste in industrial towns that contaminated the river. For instance, 400 tanning units contribute 50 MLD (million liters per day) of hazardous waste and 140 MLD of domestic waste in Kanpur (Haider Naqvi 2020 ). The water at Haridwar and Rishikesh was found unfit for drinking and bathing. The river water was in class B ever since the foundation of Uttarakhand was laid (Srivastava 2020 ).
It was reported that only 18 spots were fit while 62 spots were unfit for bathing and the river was almost unfit for drinking with a high level of coliform bacteria in the river. River water from 7 spots out of 86 monitoring stations was drinkable only after disinfection. The spots which were found suitable for drinking purpose after disinfection have been classified as ‘class A’ (Bhagirathi at Gangotri, Rudraprayag, Devprayag, Raiwala-Uttarakhand, Rishikesh, Bijnor and Diamond Harbor in West Bengal). Water at 78 monitoring stations was not suitable for drinking and bathing in Bhusaula in Bihar, Kanpur, Gola Ghat in Varanasi, Dalmau in Raebareli, Sangam in Allahabad, Ghazipur, Buxar, Patna, Bhagalpur, Howrah-Shivpur in West Bengal and many others. Thus, water available in pre-lockdown condition of the river Ganga was not suitable for drinking and bathing.
The industrial and commercial activities almost ceased during the lockdown, allowing the Ganga river to breathe again. In India, a total of four phases of lockdowns were observed for 68 days (Lockdown 1.0 (21 days)—25th March, 2020 to 14th April, 2020, Lockdown 2.0 (19 days)—14th April, 2020 to 3rd May, 2020, Lockdown 3.0 (14 days)—3rd May, 2020 to 17th May, 2020 and Lockdown 4.0 (14 days)—18th May, 2020 to 31st May, 2020).
Amid of lockdown, the CPCB, India reported on April 28, 2020 that the Ganga water has improved significantly for bathing purposes in most of the surveillance centers. Observations recorded during lockdown were as follows:
Rise in DO level from 22nd March, 2020 to15th April, 2020.
Level of BOD showed a significant decline. The lower range indicated the better health of the river.
A gradual rise in BOD level toward downstream stretches of the river Ganga.
Singh ( 2020 ) has made a remarkable observation that the level of DO increased from 25 to 30% at five ghats in Varanasi, while the level of BOD decreased up to 35%. Detailed information on changes in water quality parameters during lockdown is tabulated in Table S2 of supporting material.
Materials and methods
The total length of the Ganga river (measured along the Hooghly) from source to mouth is 2, 525 km. The Ganges originates near the Gangotri and travels about 350 km before entering into the village Balawali (district Bijnor) of Uttar Pradesh. It flows from Balawali approximately 1,150 km in Uttar Pradesh and enters the village Sitab Diara, Bihar. It flows 450 km from Sitab Diara and arrives into the West Bengal in Manikchak village (district Malda town). At the Farraka barrage, the Indian government controls water of the Ganga in distributaries namely Hooghly and Padma in the West Bengal and Bangladesh, respectively. It flows 550 km in West Bengal from village Manikchak to Haldia (near Calcutta) before merging into the Bay of Bengal. The 14 real-time stations from Anoopshahar, Uttar Pradesh to Howrah bridge, West Bengal have been considered in the present study for data modeling.
Water quality data set
The data sets of the pre-lockdown condition were collected from the system software ‘Suitability of river Ganga water’ designed by the Central Pollution Control Board (CPCB), India. This is a real-time water quality monitoring system established by CPCB, which helps in monitoring changes in the river at any given time. In India, CPCB has classified water into five classes (A to E), defining different treatment levels for the various purposes (Table S1 of supporting material shows the classes of water defined by CPCB). This classification helps managers and planners of the water quality monitoring system to set targets for water quality and to design appropriate rehabilitation programs for different water bodies. In India, water quality standards are established by CPCB in terms of the primary water quality criteria.
Water quality parameters
The parameters of water quality considered in the present study were pH, BOD, DO and TC. The pH is a measure of how acidic the water is and about 7.4 is considered as the optimum pH for the river water (Azad 2020 ). Wastewater from sewage treatment plants comprises of organic matter which is decomposed by the microorganisms and in return the dissolved oxygen is consumed. When more oxygen is consumed than produced, the concentration of DO decreases proportionately and possibly the population of a few susceptible organisms may move away, weaken or die. The DO level fluctuates in every 24 h and seasonally. It varies with the temperature of the water and altitude (APHA 1992 ). BOD influences the amount of DO in rivers and streams. Higher is the BOD value, faster is depletion of the oxygen in the stream, which means that there is less oxygen available for higher aquatic life forms. High level of BOD has similar effects as low DO concentration such as suffocation and death of aquatic organisms. A test for TC is the most basic measure for bacterial contamination of a water body. TC counts provide a general indication of a water supply's sanitary conditions. The risk of waterborne infection is increased when coliform bacteria are found in drinking water. Several types of malfunctions can cause TC contamination like seepage through the well casing, faulty well cap and well flooding. In order to cope with bacterial contamination, many long-term solutions are available such as inspection, repair of defective wells and installation of continuous disinfection equipment.
Mathematical models
Streeter phelps model.
Streeter and Phelps in 1925 developed a water quality model based on field data from the Ohio river, which was initially used by the US Public Health Service (Digvijay Kumar 2017 ).
In the present study, the Streeter Phelps model has been used to model DO in 14 real-time stations of the Ganga river.
Considering a mixed system (no in-/out flow) (Fig. 2 ) with the state variables Z and X ,
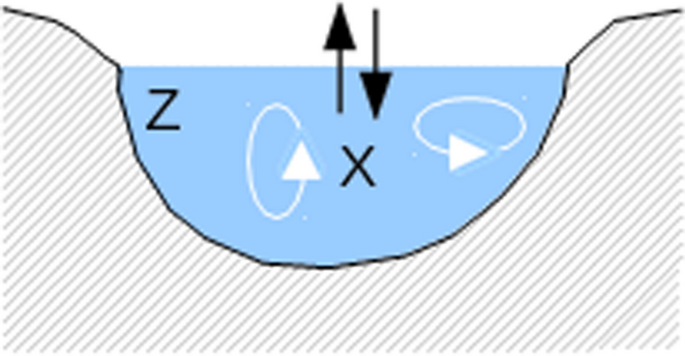
A mixed system with no inflow/outflow
where Z is degradable organic matter (mg/L) and X is the DO level (mg/L).
Aerobic decay of organic matter ‘ Z ’ by bacteria suspended in the water column (1st order kinetics)
Consumption of oxygen ‘ X ’ during mineralization of ‘ Z ’
Exchange of oxygen between water and atmosphere
Differential equations and parameters involved in the model are
where k d is decay rate (1/Time), k a is aeration rate (1/Time), s is a stoichiometric factor (Mass X /mass Z ) and X sat is O 2 saturation level (mg/L).
These equations are valid only when X > > 0.
Re-definition of state variables leads to simplified form at boundary conditions:
where L is BOD (biochemical oxygen demand) and Stoichiometric factor ‘s’ equals 1 → omitted.
Thus, Eqs. 1 and 2 can be rewritten as:
Equation 3 may be expanded by separation of variables for the initial condition L ( t = 0) = L o .
Integration of Eq. 3 yields Eq. 5 .
Substituting the value of L from Eq. 5 in Eq. 4 results in Eq. 6
Now, using the method of integrating factor, re-ordering of Eq. 6 yields
Multiplication with the factor “exp ( k a · t )” mimics Eq. 8
Applying the product rule, Eq. 9 was obtained as
Equation 10 was achieved after separation of variables and integration
Equation 10 is O 2 saturation deficit Streeter Phelps model.
Thomas and Mueller model
Thomas ( 1948 ) accounted for settle able BOD in the dissolved oxygen sag equation of Streeter Phelps model. Analytical solutions for simple initial and boundary conditions were developed by Thomann and Mueller ( 1987 ). The model includes changes in DO concentrations due to distributed sources (non-point sources) within the stream. Equation 11 illustrates the model of Thomas and Mueller (TM):
where L d = non-point source BOD (mg/L).
It is apparent from Eq. 11 that the soluble concentration of the DO generated in range by non-point sources was combined at the entry point with the attenuation phenomenon of the DO entering into the cell.
Polynomial interpolation determines a polynomial of order n that passes through n + 1 point. The NDD model is of interest due to its clarity and precision. This model shows where a function will go, based on its y -values at respective x -values (Das and Chakrabarty 2016 ). Newton’s polynomial possesses the permanence property, which means that new data values can be represented by ( n + 1)th degree polynomial and the term can be added to previously obtained n th degree polynomial. Accuracy of the polynomial interpolation depends on how close the interpolated point is to the middle of x -values used. It generates only one polynomial of least possible degree that passes through all the data points. Equation 19 depicts NDD model
Newton’s divided difference interpolation method has been used to generate the function depicting water quality of the Ganga river from pre-lockdown to lockdown period. After obtaining interpolating polynomial, it was extrapolated to predict water quality parameters (BOD, DO, pH and TC) till 7th August, 2020 (200th day from 20th January). In the present study, 20th January, 2020 has been marked as 0th day (pre-lockdown data). Using this model, polynomials were obtained for BOD, DO, pH and TC separately for each of the 14 stations and these were plotted to extrapolate values for upcoming months. This model was trained using python programming language.
Polynomial regression model
Polynomial regression determines nonlinear relationship between the value of ‘ x ’ and the corresponding conditional mean of ‘ y ’ (Ostertagová 2012 ). The expected value of ‘ y ’ can be modeled as n th degree polynomial, yielding a general polynomial regression model (Eq. 13 )
In this study, the polynomial regression model was used to model values of DO, BOD, pH and TC as a function of time to analyze and predict the Ganga water quality till 7th August, 2020. The model was trained to generate polynomials of degree 2, 3 and 4 for DO, BOD, pH and TC at real-time stations. Just to maintain consistency in results, this model was also trained using python programming language.
Radical basis function kernel support vector regression with genetic algorithm (SVR-GA)
Vapnik et al. ( 1997 ) developed an algorithm that used the earlier work of Support Vector Machines to address regression problems, which was then known as Support Vector Regression (SVR). The most powerful aspect of SVR is that it takes into account the error limit of epsilon, which means that an error between the predicted and the true value is allowed to lie within the range of [− \(\varepsilon ,\varepsilon\) ] and that no error greater than that is accepted. Using this rule, a function ‘ f ’ is generated that would be able to fulfill this condition. In linear form, function ‘ f ’ can be estimated as:
where \(\left\langle {w,x} \right\rangle\) is the dot product of w and x.
Flatness in Eq. 14 would mean to obtain a small value of w by minimizing the norm (Smola and Schölkopf 2004 ).
Usually, it is not always possible to search for a function ‘ f ’ which would produce data pairs which lie in the epsilon margin. Therefore, soft margin like approach is used, where slack variables \(\left( {\xi i,\xi i*} \right)\) representing the distance between the true values and the epsilon tunnel are introduced. This addition helps in making the optimization problem feasible. Thus, a risk function ‘ R ’ is defined by incorporating an epsilon insensitive loss function with a constant ‘ C ’. The regularized convex optimization problem (Smola and Schölkopf 2004 ) can be written as:
where C is a positive constant that plays a role in determining the extent to which a deviation from the error tunnel is tolerated.
This can be seen as a trade-off between the model flatness and empirical risk (Smola and Schölkopf 2004 ). Lagrange construction of the primary function gives a quadratic optimization problem that is solved for \(\left( {\alpha_{i} ,\alpha_{i} *} \right)\) (Vapnik and Vapnik 1998 ):
Here, ( \(\alpha_{i} ,\alpha_{i} *\) ) are Lagrange multipliers.
The vectors x i corresponding to non-zero Lagrange multipliers are then called as support vectors (Vapnik et al. 1997 ). After performing optimization, f ( x ) can be obtained as:
A kernel \(K\left( {x,x_{i} } \right)\) is defined for a nonlinear regression model. The kernel generates an inner product in some feature space and solves the corresponding dual optimization problem (Vapnik et al. 1997 ). Some examples of kernels are Polynomial, Gaussian, Radical basis function. In the present study, Radical basis function (RBF) kernel has been used. The kernel and the nonlinear objective function can then be written as:
The variables \(C,\varepsilon ,\gamma\) are user-defined while implementing SVR. Since these hyperparameters are crucial for the proper functioning of the algorithm, their right selection is of utmost importance. Genetic Algorithm (GA) was used to meet this requirement. It was first introduced by Holland ( 1992 ) and is a natural evolution-based technique that seeks inspiration from Darwin’s theory of survival of the fittest. The GAs are being applied successfully in a number of areas such as job shop problems (Falkenauer and Bouffouix 1991 ; Nakano and Yamada 1991 ), control system optimization (Krishnakumar and Goldberg 1992 ), pipeline optimization (Goldberg and Kuo 1987 ), molecular geometry optimization (Deaven and Ho 1995 ) and feature subset selection (Yang and Honavar 1998 ).
Goldberg ( 2006 ) has outlined the differences between GAs and other optimization techniques. Some of the advantages include the use of the coding of parameter set and not the parameters themselves, search from a population of points, using payoff information when binding to auxiliary information and the use of probabilistic transition rules over deterministic rules. These four advantages give GAs an edge over other commonly used traditional optimization techniques. GA can be broken down into four steps where the GA selects a population of individuals and computes the fitness function for each individual. Individuals with the highest fitness function are chosen to produce offsprings. The second and third steps involve crossovers and mutations between the selected individuals, which lead to the formation of a new generation. Finally, the fitness function for this new generation is calculated and the process repeats from step one unless the goal of the algorithm is reached.
The combination of SVR with a real-valued GA has been used as the optimization algorithm for SVRs hyperparameters ( \(C,\varepsilon ,\gamma\) ). Liu et al. 2013 used this hybrid model for water quality estimation (DO and temperature) and compared it with traditional SVR and BP neural network models. Their RGA-SVR model outperformed over the traditional models. Similarly, Wang et al. ( 2011 ) used SVR model with GA automated SVR parameter selection for the prediction of permanganate index (CODMn), ammonia–nitrogen (NH 3 –N) and chemical oxygen demand (COD) and found this superior to MLR algorithm.
Lasso regression
The lasso regression (LR) model was developed by Tibshirani ( 1996 ), which is built upon the robustness of ridge regression. It preserves the quality features of ridge regression and subset selection by shrinking some coefficients and setting others to zero. For data \(\left( {x_{i} ,y_{i} } \right)\) , i = 1, 2, … n . where, \(x_{i} = \left( {x_{i1} , \ldots x_{ik} } \right)\) are the predictor variables and y i are the responses.
The lasso optimization problem can be solved by minimizing Eq. ( 20 ).
An assumption is made that x ij are standardized to avoid any dependence on the measurement scale. Here, \(t \ge 0\) is a prespecified tuning parameter which controls the amount of shrinkage applied (Tibshirani 1996 ). Lasso regression has been previously used as a predictor algorithm for water quality estimates (Ahmed et al. 2019 ; Brooks et al. 2016 ).
Artificial neural network (ANN)
ANN is a very powerful algorithm whose architecture is inspired by the process of communication of neuronal cells. ANN can take many forms and in the present study the LMA, MLP and RBF-NN have been focused. ANN work immensely well with water quality data (El-Shafie et al. 2011 ). Authors compared the ANN model with the linear regression model and found that ANN has high accuracy as compared to the other models. Najah et al. ( 2013 ) performed a comparative study with different ANN models like RBF-NN, MLP-NN and Linear Regression model (LRM) for water quality estimation and found RBF-NN superior to MLP-NN and LRM. Authors showed that RBF-NN could be a reliable water quality predictor model. Both of these studies used a trial and error basis for determining the number of hidden layers and neuron units in the layers.
ANN with LMA
The chosen ANN for the pH, DO, BOD and TC models consisted of one input layer with fourteen input variables, one hidden layer and one output layer. In addition to this, TC consisted of a similar number of hidden and output layers except for 12 input variables. The designed ANN models (pH, DO, BOD and TC) were trained for utilizing LMA as it rapidly solves and tunes the model parameters in comparison with other algorithms (Singh et al. 2009 ). The model simulation has been done by ANN tool in MATLAB 2017a.
The MLP is a neural network with completely connected layers that are stacked against each other. Each layer is activated using a particular activation feature. In order to construct an MLP, two fully connected hidden dense layers were superimposed and activated by the function ‘rectified linear unit’ (RELU) from the python library ‘Keras.’ Data were then iterated over sufficient epochs until it converged to produce the lowest MSE (Gardner and Dorling 1998 ).
The RBF is a feedforward neural network with one hidden layer between the input and output layer. In an RBF-NN, all neurons from a layer are connected to all neurons in the next layer. Harpham et al. ( 2004 ) highlighted the advantages of applying GAs to RBF-NN, thus creating a hybrid. This addition eliminates the test and error approach since GA automatically produces an optimal solution for hyperparameters. In the present study, a GA-based search algorithm has been applied to find optimal hyperparameters for RBF-NN model.
Results and discussion
Statistics of the river ganga: pre-lockdown and during lockdown.
As shown in Table 1 , the parameters (pH, DO, BOD and TC) of the river Ganga varied in the lockdown period.
In the present study, 14 stations namely Anoopshahar; Farrukabad; Rajghat, Kannauj; Bithoor, Kanpur; Jajmau, Kanpur; Assi ghat, Varanasi; Malviya Bridge, Varanasi; Patna; Bhagalpur; Berhampore; Monipurghat, Nadia; Palta, Barrackpore; Serampore, Hooghly and Howrah bridge, West Bengal were analyzed. The changes in the parameters at these stations have been listed below.
At Anoopshahar, pH increased by 0.1, followed by an increment in BOD and DO with no detectable change in the values of TC. The increment was in the range as delineated by CPCB, India (shown in Table S1 of supporting material). Thus, this water quality at Anoopshahar permitted all the uses of water.
In the Farrukabad and Kannauj, there has been a decrease in pH, TC and DO with the simultaneous increase in BOD level. Though these changes were not positive yet the variation in pH, DO, TC and BOD were in the permissible range of CPCB (Table S1 of supplementary information).
In Bithoor and Jajmau Kanpur, there was a decrease in pH, DO and BOD and water at these stations were considered pollution-free which can be used for drinking, bathing, irrigation and other purposes. Considering TC, its level was increased in Bithoor but declined in Jajmau, Kanpur but it was in the range given by CPCB in Bithoor but not in Jajmau. Thus, the river ganga water can be used for all purpose in Bithoor but not in Jajmau, Kanpur.
In Assi ghat and Malaviya Bridge, Varanasi, a decrease in pH and DO level together with increase in BOD and TC was observed. These changes were not in an acceptable range of CPCB, India.
In Patna, the water quality was found unsuitable owing to a slight decrease in pH and DO and significant augmentation in BOD indicated a high level of pollution. But TC was found to decline here and it was within the acceptable range given by CPCB. At Bhagalpur, Bihar water sample was found unfit for drinking, bathing and irrigation.
In Berhampore, Monipurghat, Nadia; Palta, Barrackpore; Serampore, Hooghly and Howrah bridge, West Bengal a decrease in the pH, DO and BOD was observed with increase in TC and it was much higher than the acceptable range given by CPCB. The decrement in pH, DO and BOD was in the range of permissible limit demarcated by CPCB. Thus, these stations also possessed some positive changes similar to Anoopshahar, Farrukabad, Rajghat and Varanasi. The changes in pH, DO, BOD and TC during lockdown were studied and compared with pre-lockdown data as shown in Table 1 .
As shown in Table 1 , after lockdown pH in all stations was within an acceptable range of 6.5–8.5. Before lockdown, only two stations, namely Malviya Bridge, Varanasi and Serampore, Hooghly exceeded this range. But during the lockdown, these stations were within the standard range as depicted by CPCB. These changes replenished the Ganga river after a long gap.
It is appropriate to mention that there had been an insignificant change in water quality parameters during lockdown 3.0 and 4.0 as the time difference was of 14 days only.
Specifically, the health indicators of the Ganga's water improved significantly such as increased DO (in Anoopshahar), reduced BOD (in Bithoor, Kanpur; Jajmau, Kanpur; Malviya Bridge, Varanasi; Berhampore; Monipurghat, Nadia; Palta, Barrackpore; Serampore, Hooghly and Howrah bridge) and reduction in TC (Farrukabad, Rajghat, Jajmau, Patna and Palta, Barrackpore) during the lockdown.
Streeter–Phelps model
Streeter Phelps model equation was used to find O 2 saturation deficit ( D ) for 14 real-time stations of the river Ganga (Table 2 ). The value of ‘ D ’ was experimentally determined and compared with the theoretical value derived from the model (Fig. 3 ).
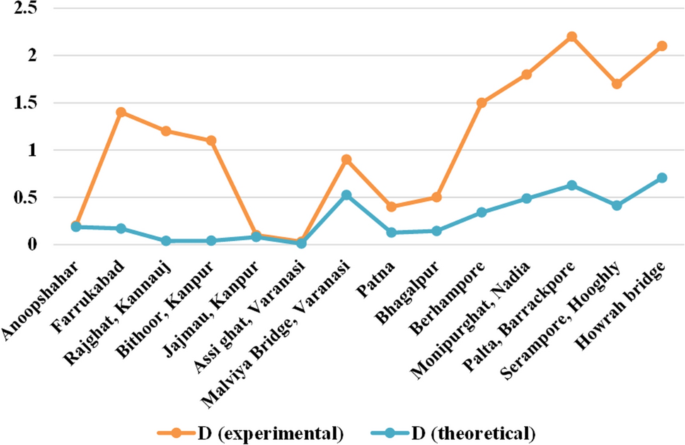
Comparison of experimental and theoretical values of D for 14 real-time stations with reference to Streeter Phelps model
It was observed from Table 2 that this model was not accurate for predicting the value of ‘ D ’ as it showed a very high percentage of error for each real-time station of the river Ganga together with a sluggish coefficient of regression ( R 2 = 0.57).
Bhargava ( 1986 ) revealed that Streeter Phelps models could not precisely predict DO sag of a stream instantly after sewage outfalls as model does not take bio-flocculation and sedimentation of the adjustable BOD into account. Jha et al. ( 2007 ) applied Streeter Phelps models for analyzing one of the most polluted rivers in India, i.e., the river Kali and showed the negative outcome with under and over-prediction. Kaushik et al. ( 2012 ) modified Streeter Phelps model by considering the settle able component of BOD and the effect of storage zones on river’s DO. Authors found that the modified model was able to predict parameters of rivers more accurately.
Thomas and Mueller model was used to find ‘ D ’ including non-point sources in the river water for 14 real-time stations. The theoretical results did not show a close agreement with the experimental values (Fig. 4 , Table 3 ). However, this model had a slightly better fit as compared to Streeter Phelps model based on the value of R 2 (= 0.75).
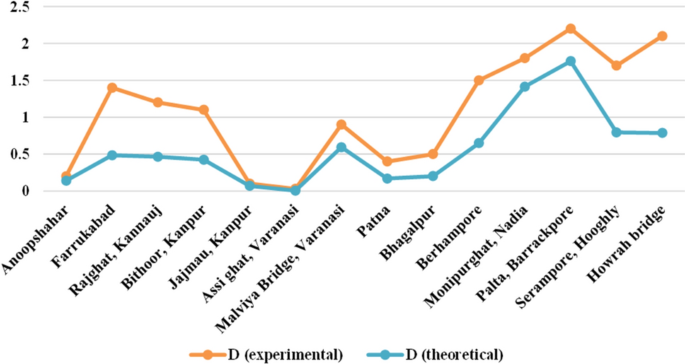
Comparison of experimental and theoretical of D for 14 real-time stations with reference to the Thomas and Mueller model
The water quality parameters were predicted for 7th August, 2020, i.e., the 200th day starting from 20th January, 2020. Table S3 of supporting material shows the value of predicted parameters on 7th August, 2020.
Assuming that the conditions do not return to original pre-lockdown conditions, this model analyzed the situation from pre-lockdown to lockdown and predicted the possible values for the near future. It also provided incorrect results for 3 stations, i.e., Rajghat, Patna and Bhagalpur, which do not seem to be possible. It was inferred from this model that the actual values were close to predicted values (pH, BOD, DO and TC) for 7th August, 2020.
Water quality parameters were predicted using 2, 3 and 4 degree polynomials on 30th June, 2020 (i.e., on day 162 starting on 20 January 2020) and these values are shown in Tables S4, S5, S6, S7 and S8 of the supporting material. For prediction, 30th June, 2020 was selected as it falls close to 31st May, 2020, and reduces the chance of error that could increase if one moves away from the 31st May, 2020 data values. Considering the range of values from these polynomials, it can be predicted that the water quality parameters (BOD, DO, pH and TC) will fall within the range of values that were predicted for 30th June, 2020.
The actual value of these parameters will depend on how the level of pollution goes back to the previous one. The values will more likely to fall in the ranges stated in Table S4, S5, S6, S7 and S8 of the supporting material.
This model analyses the situation from pre-lockdown to lockdown statistics and predicts somewhat possible values for near future. From the graphs, it was clinched that all values fall in acceptable range except BOD at Patna and Bhagalpur. Also, the DO levels at Rajghat, Patna and Bhagalpur show steep changes. The quality of the Ganga water appeared to be improved from pre-lockdown situation. Since the values and curves for polynomial second degree were the same as for NDD model, this implied that the NDD model was the reliable one.
The polynomial regression model was better than NDD as it provided the range (generated by 2nd- , 3rd- , and 4th-degree polynomial) in which the predicted parameters would lie. The polynomial regression model fitted better than NDD as most of the actual values lie in or near the predicted range. This is due to the fact that NDD is an interpolation method; however, in the present work it predicts the future values by extrapolating the curve. Also, NDD resulted in the second-degree polynomial, which does not correspond to the actual variation in the parameters in due course of the time.
The SVR model, a kernel-based regression model was used and its parameters, i.e., \(C,\varepsilon ,\gamma\) were optimized for each water quality parameter with the help of a simple GA. Here, GA was employed using a one-point crossover function having mutation with a root mean square as the fitness measure. The algorithm was performed on a population of 50 randomly selected individuals iterated upon 30 generations with a crossover probability of 0.5 and a mutation probability of 0.02. Upon running, the algorithm first randomly selects 50 individuals with their ranges being, C = [1, 100], \(\gamma = \left[ {0.1,1} \right]\) , \(\varepsilon = \left[ {0.001, 0.01} \right]\) . Each of these individuals undergoes crossover and mutation, after which the fitness of an individual is calculated. This process runs over a set of 30 generations with each generation producing a slightly better generation than itself. From the last generation, the individual with the highest fitness function is chosen as the best individual.
The model showed overfitting with zero MSE upon running. To solve this, fivefold cross-validation was used wherein the data were split into test and train set five times. This helped in solving overfitting. The model reported different MSE for pH, DO, BOD and TC in Table 4 .
The R 2 value for the pH, DO and TC approached unity signifying a perfect fit. BOD, however, showed a low R 2 value (Table 5 ).
These values show that out of the three parameters studied, the SVR—GA model works best for the pH, DO with R 2 value approaching unity (Table 6 , Fig. 5 ).
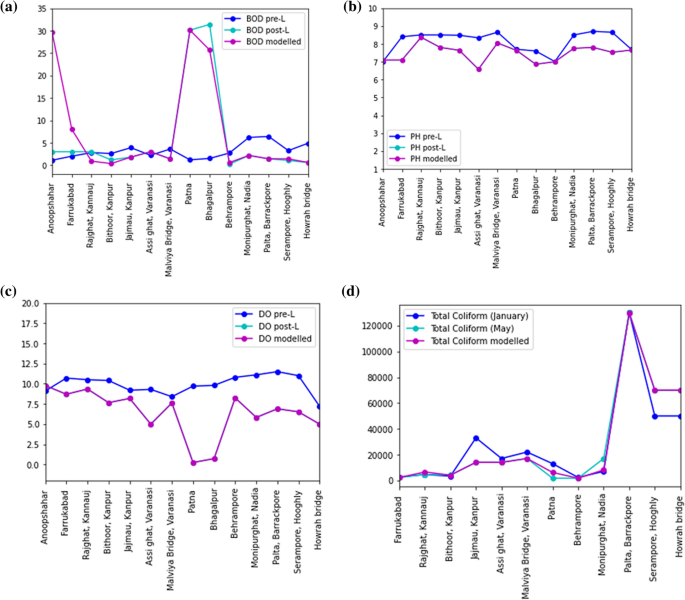
SVR-GA predicted values of BOD, pH, DO and TC
For TC analysis, data from January were paired with other parameters (pH, DO, BOD and TC). This was used as the input data set for the prediction of TC during the lockdown. SVR-GA gave an R 2 value of 0.99, pointing toward a high goodness of fit.
In this model, a ‘ t ’ value of 0.01 was used. Trial and error basis were used and alpha values have been modified and tested. The alpha value of 0.01 was finally selected. The model provided R 2 values leaning toward zero for pH, DO, BOD and TC and failed to predict the data correctly (Tables 4 , 5 , 7 , Fig. 6 ).
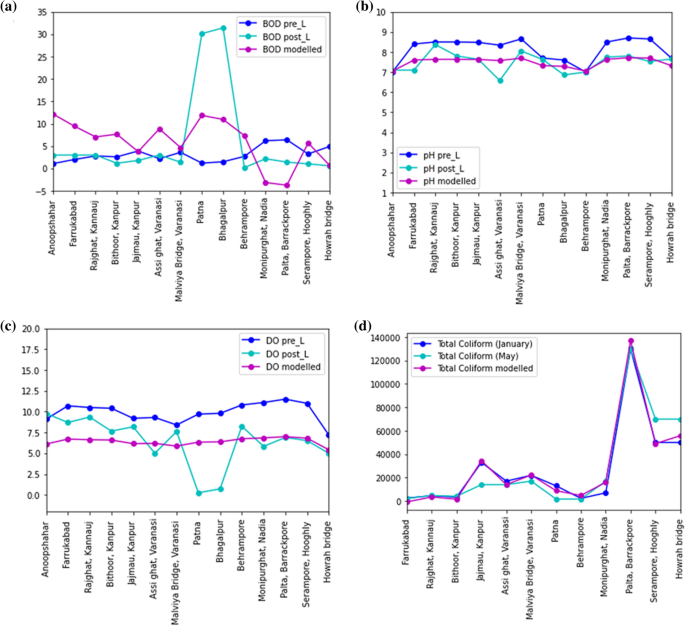
Lasso predicted values of BOD, pH, DO and TC
Apart from this, Lasso regression performed robustly for TC prediction and gave R 2 values of 0.93.
In the present study, a nonlinear transfer function (TANSIG) in the hidden layer was used for ANNs. The ANN predicted output and error in pH, DO, BOD and TC model for real-time stations of the river Ganga are shown in Table 8 .
The plots between experimental and theoretical values of pH, DO, BOD and TC values are shown in Fig. 7 .
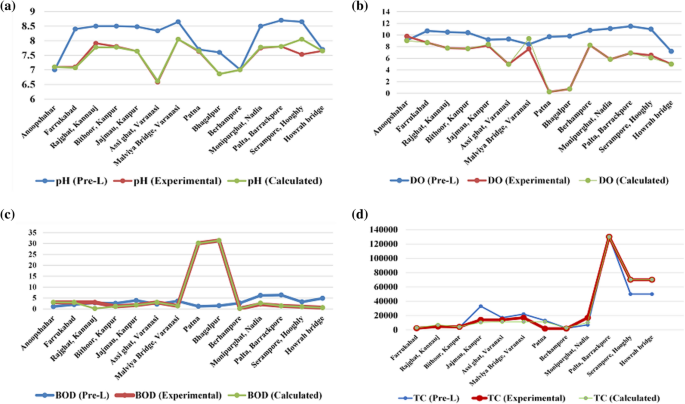
Comparison of the experimental and theoretical a pH, b DO, c BOD and d TC levels in the river Ganga
The best validation performance in ten neurons was 0.08877, 0.38177, 34.7517 and 16,371,716.42 at epoch 3, 3, 2 and 7 for pH, DO, BOD and TC, respectively, with the lowest MSE (Fig. 8 ).
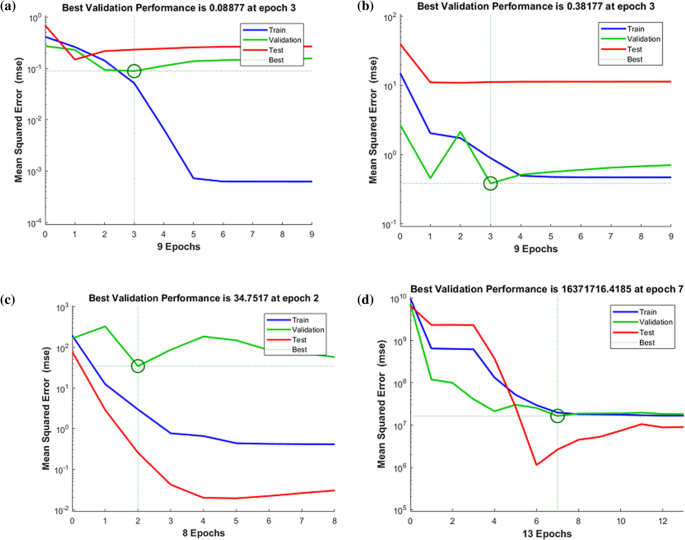
Performance plot for modeling of a pH, b DO, c BOD and d TC levels in the river Ganga
The linear R 2 values for training, validation and test data sets used for all the models (pH, DO, BOD and TC) are represented in Figure S1 of supporting material. The selected ANN generated the most trustworthy models for all three data sets. The experimental and theoretical values pH, DO, BOD and TC derived through these models were in close agreement ( R 2 = 0.92–1.0). This suggested that the model fitted well with the experimental data sets. ANNs have also been used to estimate and forecast the water quality variables like modeling of DO and BOD in the river water (Singh et al. 2009 ).
Similarly, Shamseldin ( 2010 ) used ANN for forecasting the flow of rivers in the developing countries. The chlorine concentration in the water distribution network has been assessed through ANN by Cordoba et al. ( 2014 ). ANN has been used for the prediction of water quality index (Bansal and Ganesan 2019 ; Gupta et al. 2019 ). The results of ANN-based modeling have shown significant accuracy over other traditional modeling techniques. Shakeri Abdolmaleki et al. ( 2013 ) applied ANN for predicting copper concentration in the drinking water reservoir of Iran. Authors found that predicted values were very close to the real concentration of copper. The BOD, DO and other water quality parameters were forecast by using ANN in the Karoon river (Emamgholizadeh et al. 2014 ). The predicted values were close to the real ones, which proved ANN, an effective modeling technique for predicting water quality variables in the river. Gomolka et al. ( 2018 ) used ANN to estimate the BOD level and for controlling rate of aeration in river.
Two RELU activated hidden layers were used and epochs were performed until full convergence of loss function was observed.
The MLP showed excellent results for pH, DO and BOD with R 2 values very close to one (Tables 4 , 5 , 9 , Fig. 9 ) but it's prediction for TC was not at par with its performance for the other indices.
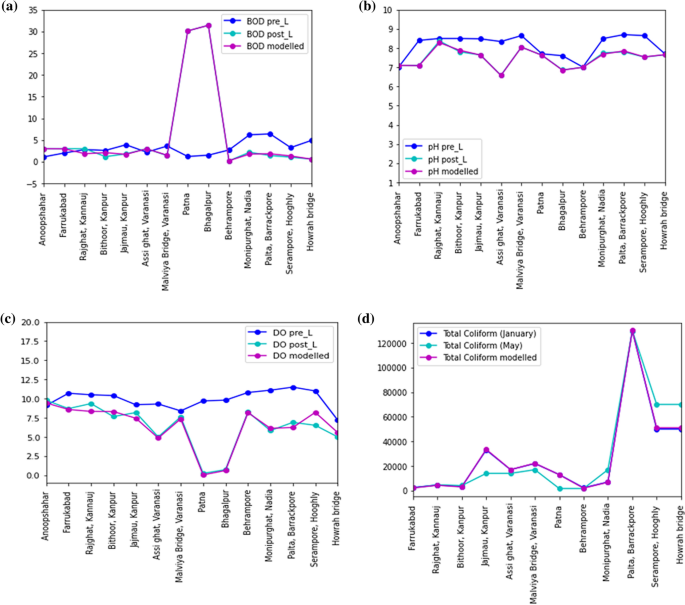
MLP predicted values of BOD, pH, DO and TC
An RBF-NN was applied with GA to optimize the hyperparameters like learning rate (lr) and several kernels (k). A multi-feature input algorithm was constructed which picked the hyperparameters using a GA where MSE was chosen as the fitness function. The initial population was picked out where the kernel number and learning rate constrained to a range of [1, 7] and [0.0001, 0.02], respectively. An initial population size of 50 was chosen. The algorithm was run for 30 generations with a crossover and a mutation probability of 0.7 and 0.02, respectively. The model ran for 100 epochs each time. The results of the model showed poor performance for BOD, DO and TC. The model’s goodness of fit for pH is better than Lasso regression but not SVR and MLP (Tables 4 , 5 , 10 , Fig. 10 ).
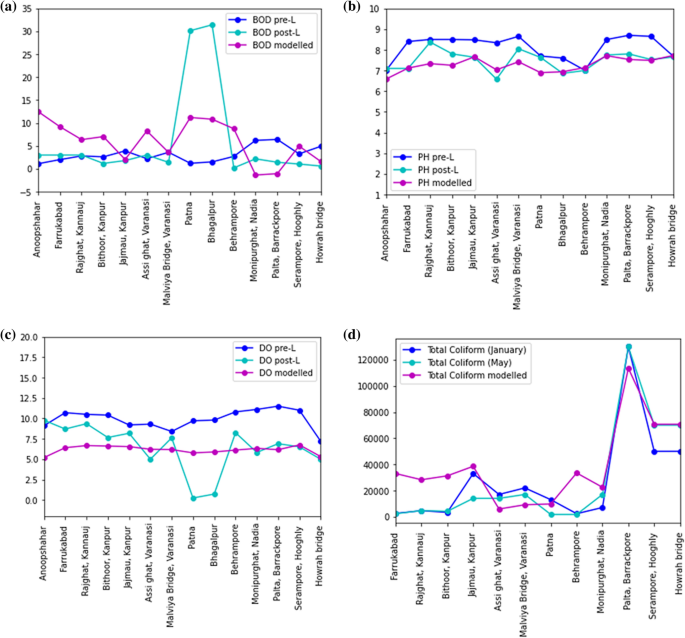
RBF-NN predicted values of BOD, pH, DO and TC
Comparative study
Several studies conducted by other researchers on the quality of the Ganga's water during lockdown have been discussed in detail in Table 11 . The outcomes of their work with the technique involved in the estimation of water quality parameters are included and have been compared with the present study.
In the present study, the water quality of the river Ganga has been evaluated during the lockdown and predicted for post lockdown conditions. It was found that the pH of all stations was within the standard range 6.5–8.5 in lockdown period. An increment in DO has been observed in Anoopshahar. Apart from that, all stations had DO > 5 mg/L except Patna and Bhagalpur. It was noted that Patna and Bhagalpur stations had very high BOD levels compared to other stations that signified a substantial level of pollution. During the lockdown, Anoopshahar, Farrukabad, Rajghat, Kannauj and Assi ghat, Varanasi had BOD exactly as 3 mg/L. The decrement in TC was observed in Farrukabad, Rajghat, Jajmau, Patna and Palta during the lockdown period. In the present study, bioengineered mathematical models, namely Streeter Phelps, Thomas Mueller, SVR-GA, Lasso Regression, ANN, NDD and Polynomial regression, were attempted to predict the water quality parameters. Polynomial regression and NDD model were able to predict pH, BOD, DO and TC levels from 20th January, 2020 to 30th June, 2020 and 07th August, 2020. Thus, NDD and polynomial regression models were used to predict the near future values of the water quality parameters (BOD, DO, pH and TC) of the river Ganga. But NDD model was not able to predict TC values. However, the NDD model is simply an interpolation method, which can be further extrapolated to predict the values. On the other hand, polynomials of 2, 3 and 4 degrees were generated in polynomial regression model to obtain the range of predicted values. The NDD model is verified by the polynomial degree 2 regression that appeared to be acceptable after comparison. Overall, polynomial regression model was better than NDD model. In ANN models using LMA, the best validation performance was observed with ten neurons as 0.08877, 0.38177, 34.7517 and 16,371,716.42 at epoch 3, 3, 2 and 7 for pH, DO, BOD and TC, respectively. Additionally, SVR-GA hybrid was superior compared to its counterparts such as Lasso Regression and RBF-NN in the prediction of real-time water quality data indices such as pH, DO of the river Ganga. It also produced the best results for TC forecast during the lockdown period. It was unable to predict the lockdown BOD values correctly. MLP was the second-best algorithm after SVR-GA, which showed accurate fits for three (pH, DO, BOD) of the indices but couldn’t accurately predict TC levels. SVR-GA and MLP showed a nearly perfect fit for the pH and TC data with significantly lesser MSE values. The R 2 value for pH modeled by SVR-GA ( R 2 = 0.99) and MLP ( R 2 = 0.99) was near unity, pointing to a perfect fit. Similarly, the R 2 value for TC modeled by SVR-GA is 0.99. The abnormal high deviations in BOD modeling in all the models except MLP ( R 2 = 0.99) can be due to the presence of outliers. It can, therefore, be stated that SVR and MLP are relatively quicker and better choices as the modeling techniques for predicting values of water quality parameters of the river Ganga. Thus, in the present study, SVR-GA, MLP and polynomial regression model were found superior to NDD for the prediction of water quality parameters in the long run. Moreover, as these models are fitted with the least error, there are numerous applications where their use is highly recommended. Like, SVR-GA algorithm can be effectively implemented to estimate parameters of water, MLP is capable of modeling a sequencing batch reactor that will treat municipal wastewater. The comparison of different models showed their applicability in predictive modeling of river flow and wastewater treatment.
Ahmed AAM (2017) Prediction of dissolved oxygen in Surma River by biochemical oxygen demand and chemical oxygen demand using the artificial neural networks (ANNs). J King Saud Univ Eng Sci 29:151–158. https://doi.org/10.1016/j.jksues.2014.05.001
Article Google Scholar
Ahmed U, Mumtaz R, Anwar H, Shah AA, Irfan R, García-Nieto J (2019) Efficient water quality prediction using supervised machine learning. Water 11:2210
Article CAS Google Scholar
APHA (1992) Standard methods for the examination of water and wastewater, 18th edn. American Public Health Association, Washington, DC
Google Scholar
Azad S (2020) For 1st time in decades, tests show Ganga water in Haridwar fit to drink
Bansal S, Ganesan G (2019) Advanced evaluation methodology for water quality assessment using artificial neural network approach. Water Resour Manag 33:3127–3141
Bhargava DS (1986) DO sag model for extremely fast river purification. J Environ Eng 112:572–585
Brooks W, Corsi S, Fienen M, Carvin R (2016) Predicting recreational water quality advisories: a comparison of statistical methods. Environ Model Softw 76:81–94
Chakraborty SK (2021) River pollution and perturbation: perspectives and processes. In: Chakraborty SK (ed) Riverine ecology, vol 2. Springer, Berlin, pp 443–530
Chapter Google Scholar
Chakraborty I, Maity P (2020) COVID-19 outbreak: migration, effects on society, global environment and prevention. Sci Total Environ 728:138882
Chaturvedi MC (2012) Ganga–Brahmaputra–Meghna waters: advances in development and management. CRC Press
Book Google Scholar
Cordoba GC, Tuhovčák L, Tauš M (2014) Using artificial neural network models to assess water quality in water distribution networks. Procedia Eng 70:399–408
Das B, Chakrabarty D (2016) Newton’s divided difference interpolation formula: representation of numerical data by a polynomial curve. Int J Math Trend Technol 35:26–32
Deaven DM, Ho K-M (1995) Molecular geometry optimization with a genetic algorithm. Phys Rev Lett 75:288
Dey S, Choudhary S, Dey S, Deshpande K, Kelkar N (2019) Identifying potential causes of fish declines through local ecological knowledge of fishers in the Ganga River, eastern Bihar, India. Fish Manag Ecol 27:140–154. https://doi.org/10.1111/fme.12390
Dhar I, Biswas S, Mitra A, Pramanick P, Mitra A (2020) COVID-19 Lockdown phase: a boon for the River Ganga water quality along the city of Kolkata. NUJS J Regul Stud Spec 53–57
DigvijayKumar TABAS (2017) D.O. modelling and water quality analysis of river Brahmaputra in Guwahati, Assam. J Civ Eng Environ Technol 4:64–67
Drew G (2017) River dialogues: Hindu faith and the political ecology of dams on the sacred Ganga. University of Arizona Press, Tucson
Dutta V, Dubey D, Kumar S (2020) Cleaning the River Ganga: impact of lockdown on water quality and future implications on river rejuvenation strategies. Sci Total Environ 743:140756
Duttagupta S et al (2021) Impact of Covid-19 lockdown on availability of drinking water in the arsenic-affected Ganges River Basin. Int J Environ Res Public Health 18:2832
El-Shafie A, Mukhlisin M, Najah AA, Taha MR (2011) Performance of artificial neural network and regression techniques for rainfall-runoff prediction. Int J Phys Sci 6:1997–2003
Emamgholizadeh S, Kashi H, Marofpoor I, Zalaghi E (2014) Prediction of water quality parameters of Karoon River (Iran) by artificial intelligence-based models. Int J Environ Sci Technol 11:645–656
Falkenauer E, Bouffouix S (1991) A genetic algorithm for job shop. In: ICRA. Citeseer, pp 824–829
Gardner MW, Dorling S (1998) Artificial neural networks (the multilayer perceptron)—a review of applications in the atmospheric sciences. Atmos Environ 32:2627–2636
Garg V, Aggarwal SP, Chauhan P (2020) Changes in turbidity along Ganga River using Sentinel-2 satellite data during lockdown associated with COVID-19 Geomatics. Nat Hazards Risk 11:1175–1195. https://doi.org/10.1080/19475705.2020.1782482
Goldberg DE (2006) Genetic algorithms. Pearson Education India, Delhi
Goldberg DE, Kuo CH (1987) Genetic algorithms in pipeline optimization. J Comput Civ Eng 1:128–141
Gomolka Z, Twarog B, Zeslawska E, Lewicki A, Kwater T (2018) Using artificial neural networks to solve the problem represented by BOD and DO indicators. Water 10:4
Gupta R, Singh A, Singhal A (2019) Application of ANN for water quality index. Int J Mach Learn Comput 9:688–693
Haider Naqvi SK (2020) Lockdown does what decades of schemes couldn’t: clean Ganga, Published on 4 April 2020 in Hindustan Times
Harpham C, Dawson CW, Brown MR (2004) A review of genetic algorithms applied to training radial basis function networks. Neural Comput Appl 13:193–201
Hasnain M, Pasha MF, Ghani I (2020) Combined measures to control the COVID-19 pandemic in Wuhan Hubei, China: a narrative review. J Biosaf Biosecur 2:51–57
Holland JH (1992) Adaptation in natural and artificial systems: an introductory analysis with applications to biology, control, and artificial intelligence. MIT Press, Cambridge
Jha R, Ojha C, Bhatia K (2007) Critical appraisal of BOD and DO models applied to a highly polluted river in India. Hydrol Sci J 52:362–375
Jiang M, Jiang S, Zhu L, Wang Y, Huang W, Zhang H (2013) Study on parameter optimization for support vector regression in solving the inverse ECG problem. Comput Math Methods Med 2013:158056. https://doi.org/10.1155/2013/158056
Kaushik N, Tyagi B, Jayaraman G (2012) Modeling of the dissolved oxygen in a river with storage zone on the banks. Appl Math 3:699–704
Krishnakumar K, Goldberg DE (1992) Control system optimization using genetic algorithms. J Guid Control Dyn 15:735–740. https://doi.org/10.2514/3.20898
Liu S, Tai H, Ding Q, Li D, Xu L, Wei Y (2013) A hybrid approach of support vector regression with genetic algorithm optimization for aquaculture water quality prediction. Math Comput Model 58:458–465
Lockwood AH (2016) Heat advisory: Protecting health on a warming planet. MIT Press, Cambridge
Muduli P et al (2021) Water quality assessment of the Ganges River during COVID-19 lockdown. Int J Environ Sci Technol 18:1645–1652
Mukherjee P, Pramanick P, Zaman S, Mitra A (2020) Eco-restoration of River Ganga water quality during COVID-19 lockdown period using Total Coliform (TC) as proxy. NUJS J Regul Stud Spec 69–74
Najah A, El-Shafie A, Karim O, El-Shafie AH (2013) Application of artificial neural networks for water quality prediction. Neural Comput Appl 22:187–201
Nakano R, Yamada T (1991) Conventional genetic algorithm for job shop problems. In: ICGA, pp 474–479
Ostertagová E (2012) Modelling using polynomial regression. Procedia Eng 48:500–506
Paul D (2017) Research on heavy metal pollution of river Ganga: a review. Ann Agrar Sci 15:278–286. https://doi.org/10.1016/j.aasci.2017.04.001
Paul A, Chatterjee S, Bairagi N (2020) Prediction on Covid-19 epidemic for different countries: focusing on South Asia under various precautionary measures. Medrxiv 395:689
Postel S, Richter B (2012) Rivers for life: managing water for people and nature. Island Press, Washington
Roy MB, Ghosh M, Roy PK (2021) Assessment of water quality of river ganga during covid-19 lockdown. Desalination Water Treat 223:26–33
Sarkar A, Pandey P (2015) River water quality modelling using artificial neural network technique. Aquat Procedia 4:1070–1077. https://doi.org/10.1016/j.aqpro.2015.02.135
Sati VP (2021) Ganges. Springer, Berlin
Shakeri Abdolmaleki A, Gholamalizadeh Ahangar A, Soltani J (2013) Artificial neural network (ANN) approach for predicting Cu concentration in drinking water of Chahnimeh1 reservoir in Sistan-Balochistan, Iran. Health Scope 2:31–38. https://doi.org/10.17795/jhealthscope-9828
Shamseldin A (2010) Artificial neural network model for river flow forecasting in a developing country. J Hydroinform 12:22–35. https://doi.org/10.2166/hydro.2010.027
Singh K, Jha R (2021) Critical appraisal of water quality model parameters for an urban city in lower Ganga basin during pre-and post-COVID19 Lockdown in India. Indian J Sci Technol 14:2439–2447
Singh K, Basant A, Malik A, Jain G (2009) Artificial neural network modeling of the river water quality—a case study. Ecol Model 220:888–895. https://doi.org/10.1016/j.ecolmodel.2009.01.004
Singh B (2020) Lockdown gift: gleaming Ganga lays new conservation roadmap. Times of India. https://m.timesofindia.com/city/varanasi/lockdown-gift-gleaming-ganga-lays-new-conservation-roadmap/amp_articleshow/75571128.cms . Accessed 06/05/2020
Smola AJ, Schölkopf B (2004) A tutorial on support vector regression. Stat Comput 14:199–222
Srinivas R, Singh AP, Shankar D (2020) Understanding the threats and challenges concerning Ganges River basin for effective policy recommendations towards sustainable development. Environ Dev Sustain 22:3655–3690
Srivastava NSaS (2020) Lockdown impact: Ganga water in Haridwar becomes 'fit to drink' after decades. India Today
Thomann RV, Mueller JA (1987) Principles of surface water quality modeling and control. Harper & Row Publishers, New York
Thomas H (1948) The pollution load capacity of streams, Water and sewage works GICAL survey Fohn Knox Rd-Suite F 240
Tibshirani R (1996) Regression shrinkage and selection via the Lasso. J R Stat Soc: Ser B (Methodol) 58:267–288
Trivedi R (2010) Water quality of the Ganga River—an overview. Aquat Ecosyst Health Manag 13:347–351. https://doi.org/10.1080/14634988.2010.528740
Vapnik V, Golowich SE, Smola AJ (1997) Support vector method for function approximation, regression estimation and signal processing. In: Advances in neural information processing systems, pp 281–287
Vapnik V, Vapnik V (1998) Statistical learning theory, vol 1. Wiley, New York, p 624
Wang X, Fu L, He C (2011) Applying support vector regression to water quality modelling by remote sensing data. Int J Remote Sens 32:8615–8627
Webdesk N (2020) Rivers heal during lockdown. National Herald India. https://www.nationalheraldindia.com/videos/rivers-heal-during-lockdown
Wray M ( 2020) Coronavirus lockdown eases pollution, Venice canal runs clear. Globalnews. https://globalnews.ca/news/6683226/climate-change-coronavirus/ . Accessed 16/03/2020
Yang J, Honavar V (1998) Feature subset selection using a genetic algorithm. In: Liu H, Motoda H (eds) Feature extraction, construction and selection. Springer, Berlin, pp 117–136
Download references
Acknowledgements
The authors are thankful to the School of Biochemical Engineering, IIT (BHU) Varanasi, Varanasi for financial and technical support of the present research work.
The authors did not receive support from any organization for the submitted work.
Author information
Authors and affiliations.
School of Biochemical Engineering, IIT (BHU) Varanasi, Uttar Pradesh, Varanasi, 221005, India
J. Singh, S. Swaroop, P. Sharma & V. Mishra
You can also search for this author in PubMed Google Scholar
Contributions
All authors contributed to the study conception and design. Material preparation, data collection and analysis were performed by JS, SS and PS. The final draft of the manuscript was reviewed by VM. All authors read and approved the final manuscript.
Corresponding author
Correspondence to V. Mishra .
Ethics declarations
Conflict of interest.
The authors have no conflicts of interest to declare that are relevant to the content of this article.
Additional information
Editorial responsibility: Samareh Mirkia.
Supplementary Information
Below is the link to the electronic supplementary material.
Supplementary file1 (DOCX 474 kb)
Rights and permissions.
Reprints and permissions
About this article
Singh, J., Swaroop, S., Sharma, P. et al. Real-time assessment of the Ganga river during pandemic COVID-19 and predictive data modeling by machine learning. Int. J. Environ. Sci. Technol. 20 , 7887–7910 (2023). https://doi.org/10.1007/s13762-022-04423-1
Download citation
Received : 19 May 2021
Revised : 10 March 2022
Accepted : 11 July 2022
Published : 27 July 2022
Issue Date : July 2023
DOI : https://doi.org/10.1007/s13762-022-04423-1
Share this article
Anyone you share the following link with will be able to read this content:
Sorry, a shareable link is not currently available for this article.
Provided by the Springer Nature SharedIt content-sharing initiative
- Artificial neural network
- Biochemical oxygen demand
- Dissolved oxygen
- Total Coliform Count
- Find a journal
- Publish with us
- Track your research
ORIGINAL RESEARCH article
The spatial spillover effect of green technology innovation on water pollution --evidence from 283 chinese cities provisionally accepted.
- 1 Fudan Development Institute, Fudan University, China
- 2 School of Customs and Public Economics, Shanghai Customs College, China
The final, formatted version of the article will be published soon.
While economic development brings serious environmental problems, technological advances can effectively reduce pollution, which helps to achieve the Sustainable Development Goals. Although the impact of green technology innovation on atmospheric pollutants and carbon emissions has been extensively studied, the effect of such innovation on pollutant reduction varies due to the diverse regional distribution characteristics of different pollutants. Thus, this paper contributes to the literature by examining the influence of green technology innovation on water pollution from a regional perspective, with a particular emphasis on the pronounced clustering of wastewater pollution in China's coastal areas. Our findings reveal a significant U-shaped relationship between technology innovation and water pollution, as measured by both industrial wastewater and the ratio of unprocessed sewage. Interestingly, this pollution reduction effect also exhibits a U-shaped spatial spillover. Given the rapid development of the digital economy, it can further amplify the spatial spillover effect of green innovation, especially in eastern regions. This study also provides recent empirical evidence from China to the Environmental Kuznets Curve.
Keywords: green technical innovation, Water polllution, Spatial spillover, Environmental kuznets curve, China
Received: 29 Feb 2024; Accepted: 17 Apr 2024.
Copyright: © 2024 Ruan and Zhang. This is an open-access article distributed under the terms of the Creative Commons Attribution License (CC BY) . The use, distribution or reproduction in other forums is permitted, provided the original author(s) or licensor are credited and that the original publication in this journal is cited, in accordance with accepted academic practice. No use, distribution or reproduction is permitted which does not comply with these terms.
* Correspondence: Dr. Anqi Zhang, Shanghai Customs College, School of Customs and Public Economics, pudong, China
People also looked at

IMAGES
VIDEO
COMMENTS
However, due to pollution, today the regular consumption of Ganga water or taking dip in it may cause serious health effects including cancer. Ganga covers as much as 8,61,404 km 2 of drainage basin within the country which is 15th in Asia and 29th in the world ( Joshi et al., 2009 ).
The tributaries of the Ganga account for 60% of the total water of the river. About 20% of Ganga water is fed by Ghaghara as against 16% by Yamuna [119]. The tributaries of Ganga have an important role in pollution of river Ganga because it transports considerable amounts of pollutants as well as heavy metals into the Ganga.
Polyethylene from discarded plastic bags, food-packaging films and milk bottles is increasingly gagging the Ganges river turning it into a toxic water body downstream, research shows. A survey of ...
Introduction. In recent decades, pollution of water bodies has become a matter of growing concern in the low- and middle-income countries. Rapid industrialization and population growth have increased the release of industrial and domestic effluents to surface water, jeopardizing aquatic ecosystems and compromising water quality (Paul, 2017).The Ganga basin, one of the most densely populated ...
Ganga river pollution is one of the most discussed topics on river water quality in the past few years. The uncontrolled discharge of domestic sewage without treatment, excessive pollutant ...
Pollution issues going on at the surface of the Ganges may also impact groundwater (Mariya et al., 2019), but as was the case in the previously discussed fieldwork and modeling sections of this paper, most groundwater pollution research from reviews are oriented around arsenic pollution. These recommendations normally involve behavioral changes ...
Abstract. In India, the river Ganga is believed as a goddess, and people worship it. Despite all the respect for the river, the river's condition is worsening, and we Indians are unable to maintain the purity of the river. The Ganga is a river of faith, devotion, and worship. Indians accept its water as "holy," which is known for its "curative ...
The municipal sewage is the major contributor to polluting the river Ganga followed by industrial effluents, agriculture runoff, pulp, and paper waste, pesticide, and fertilizer wastes (Das 2011).
The water quality data is also supplemented with new pollution levels reported in the literature. The analysis of available data in research reveals that the Ganga River water quality remains declined because of the presence of industrial waste, domestic waste, heavy metals, sewage, animal, and human skeletons.
The Ganga River is facing mounting environmental pressures due to rapidly increasing human population, urbanisation, industrialisation and agricultural intensification, resulting in worsening water quality, ecological status and impacts on human health. A combined inorganic chemical, algal and bacterial survey (using flow cytometry and 16S rRNA gene sequencing) along the upper and middle Ganga ...
Background: The water quality of Ganga, the largest river in Indian sub-continent and life line to hundreds of million people, has severely deteriorated. Studies have indicated the presence of high level of carcinogenic elements in Ganga water. Objectives: We performed extensive review of sources and level of organic, inorganic pollution and microbial contamination in Ganga water to evaluate ...
To have a better understanding of the transformation in the quality of water in Ganga, this paper analyses historical data on water quality and compares with quality observed during the lockdown period based upon the real-time water monitoring data of the Central Pollution Control Board (CPCB) and various state pollution control boards ...
Fig.1: Various sources of pollution in river Ganga water. (3.1) Sewage pollution: The sewage treatment system is still in need of development because big cities along the river create millions of
The water having DO < 5% of saturation lies in an extremely severe pollution region; DO between 5 and 10% of saturation lies in severe pollution; DO in a range of 10-70% represents moderate ...
This review paper presents experimental results of some researchers who have observed Ganga River Water from Gangotri to West Bengal in the past 21 years. An attempt has been made to understand the gradual degradation of Gangajal and its present pollution level...
The current review concluded that inorganic pollution in river Ganga water has increased several folds in last decade and conditions are even worst due to presence of carcinogenic elements (Cr, Cd, Pb, As). ... CSIR-National Botanical Research Institute, Lucknow for the facilities provided. ... Status Paper for River Ganga, Past Failures and ...
Logistic regression analysis revealed significant associations between water-borne/enteric disease occurrence and the use of the river for bathing, laundry, washing eating utensils, and brushing teeth, and possible risk factors for residents who live by and use the Ganges River in Varanasi. Expand. 124.
Results: The level of pesticides in Ganga water registered a drastic reduction in the last decade (i.e. after the establishment of National Ganga River Basin Authority (NGRBA) in 2009), still the ...
The study provides a base line assessment of the water quality of river Ganga for drinking.Water quality was marked as polluted and unfit for d. ... where the chemical industry discharges about 70% of the total waste water, followed by the pulp-paper industry with 20% discharge ... Environmental Science and Pollution Research. 24 (16),
The Ganga encounters about 15 tributaries during its journey from Gomukh to the Bay of Bengal, which contribute to 60% of its total water, making it the third largest river in the world in terms of the volume of water released (Zhang et al., 2019 ). In 2008, Ganga River was professed as the 'National River' of India.
First, we construct a metric that measures the climate change induced mean reduction in the natural capacity of the Ganges to absorb pollution in the time interval [0,t]. Second, we use this metric and determine the equilibrium production of leather by both tanneries in the benchmark case in which there is no pollution.
In this study, four water quality parameters were reviewed at 14 stations of river Ganga in pre-, during and post-lockdown and these parameters were modeled by using different machine learning algorithms. Various mathematical models were used for the computation of water quality parameters in pre-, during and post- lockdown period by using Central Pollution Control Board real-time data ...
While economic development brings serious environmental problems, technological advances can effectively reduce pollution, which helps to achieve the Sustainable Development Goals. Although the impact of green technology innovation on atmospheric pollutants and carbon emissions has been extensively studied, the effect of such innovation on pollutant reduction varies due to the diverse regional ...
Environmental Science and Pollution Research 28.36 (2021): ... "Ganga water pollution: ... This paper deals with the study on-"How environmental parameters impacts/affects the quality of river ...