Thank you for visiting nature.com. You are using a browser version with limited support for CSS. To obtain the best experience, we recommend you use a more up to date browser (or turn off compatibility mode in Internet Explorer). In the meantime, to ensure continued support, we are displaying the site without styles and JavaScript.
- View all journals
- My Account Login
- Explore content
- About the journal
- Publish with us
- Sign up for alerts
- Open access
- Published: 23 June 2020

The high cost of prescription drugs: causes and solutions
- S. Vincent Rajkumar ORCID: orcid.org/0000-0002-5862-1833 1
Blood Cancer Journal volume 10 , Article number: 71 ( 2020 ) Cite this article
96k Accesses
62 Citations
273 Altmetric
Metrics details
- Cancer therapy
- Public health
Global spending on prescription drugs in 2020 is expected to be ~$1.3 trillion; the United States alone will spend ~$350 billion 1 . These high spending rates are expected to increase at a rate of 3–6% annually worldwide. The magnitude of increase is even more alarming for cancer treatments that account for a large proportion of prescription drug costs. In 2018, global spending on cancer treatments was approximately 150 billion, and has increased by >10% in each of the past 5 years 2 .
The high cost of prescription drugs threatens healthcare budgets, and limits funding available for other areas in which public investment is needed. In countries without universal healthcare, the high cost of prescription drugs poses an additional threat: unaffordable out-of-pocket costs for individual patients. Approximately 25% of Americans find it difficult to afford prescription drugs due to high out-of-pocket costs 3 . Drug companies cite high drug prices as being important for sustaining innovation. But the ability to charge high prices for every new drug possibly slows the pace of innovation. It is less risky to develop drugs that represent minor modifications of existing drugs (“me-too” drugs) and show incremental improvement in efficacy or safety, rather than investing in truly innovative drugs where there is a greater chance of failure.
Causes for the high cost of prescription drugs
The most important reason for the high cost of prescription drugs is the existence of monopoly 4 , 5 . For many new drugs, there are no other alternatives. In the case of cancer, even when there are multiple drugs to treat a specific malignancy, there is still no real competition based on price because most cancers are incurable, and each drug must be used in sequence for a given patient. Patients will need each effective drug at some point during the course of their disease. There is seldom a question of whether a new drug will be needed, but only when it will be needed. Even some old drugs can remain as virtual monopolies. For example, in the United States, three companies, NovoNordisk, Sanofi-Aventis, and Eli Lilly control most of the market for insulin, contributing to high prices and lack of competition 6 .
Ideally, monopolies will be temporary because eventually generic competition should emerge as patents expire. Unfortunately, in cancers and chronic life-threatening diseases, this often does not happen. By the time a drug runs out of patent life, it is already considered obsolete (planned obsolescence) and is no longer the standard of care 4 . A “new and improved version” with a fresh patent life and monopoly protection has already taken the stage. In the case of biologic drugs, cumbersome manufacturing and biosimilar approval processes are additional barriers that greatly limit the number of competitors that can enter the market.
Clearly, all monopolies need to be regulated in order to protect citizens, and therefore most of the developed world uses some form of regulations to cap the launch prices of new prescription drugs. Unregulated monopolies pose major problems. Unregulated monopoly over an essential product can lead to unaffordable prices that threaten the life of citizens. This is the case in the United States, where there are no regulations to control prescription drug prices and no enforceable mechanisms for value-based pricing.
Seriousness of the disease
High prescription drug prices are sustained by the fact that treatments for serious disease are not luxury items, but are needed by vulnerable patients who seek to improve the quality of life or to prolong life. A high price is not a barrier. For serious diseases, patients and their families are willing to pay any price in order to save or prolong life.
High cost of development
Drug development is a long and expensive endeavor: it takes about 12 years for a drug to move from preclinical testing to final approval. It is estimated that it costs approximately $3 billion to develop a new drug, taking into account the high failure rate, wherein only 10–20% of drugs tested are successful and reach the market 7 . Although the high cost of drug development is a major issue that needs to be addressed, some experts consider these estimates to be vastly inflated 8 , 9 . Further, the costs of development are inversely proportional to the incremental benefit provided by the new drug, since it takes trials with a larger sample size, and a greater number of trials to secure regulatory approval. More importantly, we cannot ignore the fact that a considerable amount of public funding goes into the science behind most new drugs, and the public therefore does have a legitimate right in making sure that life-saving drugs are priced fairly.
Lobbying power of pharmaceutical companies
Individual pharmaceutical companies and their trade organization spent approximately $220 million in lobbying in the United States in 2018 10 . Although nations recognize the major problems posed by high prescription drug prices, little has been accomplished in terms of regulatory or legislative reform because of the lobbying power of the pharmaceutical and healthcare industry.
Solutions: global policy changes
There are no easy solutions to the problem of high drug prices. The underlying reasons are complex; some are unique to the United States compared with the rest of the world (Table 1 ).
Patent reform
One of the main ways to limit the problem posed by monopoly is to limit the duration of patent protection. Current patent protections are too long, and companies apply for multiple new patents on the same drug in order to prolong monopoly. We need to reform the patent system to prevent overpatenting and patent abuse 11 . Stiff penalties are needed to prevent “pay-for-delay” schemes where generic competitors are paid money to delay market entry 12 . Patent life should be fixed, and not exceed 7–10 years from the date of first entry into the market (one-and-done approach) 13 . These measures will greatly stimulate generic and biosimilar competition.
Faster approval of generics and biosimilars
The approval process for generics and biosimilars must be simplified. A reciprocal regulatory approval process among Western European countries, the United States, Canada, and possibly other developed countries, can greatly reduce the redundancies 14 . In such a system, prescription drugs approved in one member country can automatically be granted regulatory approval in the others, greatly simplifying the regulatory process. This requires the type of trust, shared standards, and cooperation that we currently have with visa-free travel and trusted traveler programs 6 .
For complex biologic products, such as insulin, it is impossible to make the identical product 15 . The term “biosimilars” is used (instead of “generics”) for products that are almost identical in composition, pharmacologic properties, and clinical effects. Biosimilar approval process is more cumbersome, and unlike generics requires clinical trials prior to approval. Further impediments to the adoption of biosimilars include reluctance on the part of providers to trust a biosimilar, incentives offered by the manufacturer of the original biologic, and lawsuits to prevent market entry. It is important to educate providers on the safety of biosimilars. A comprehensive strategy to facilitate the timely entry of cost-effective biosimilars can also help lower cost. In the United States, the FDA has approved 23 biosimilars. Success is mixed due to payer arrangements, but when optimized, these can be very successful. For example, in the case of filgrastim, there is over 60% adoption of the biosimilar, with a cost discount of approximately 30–40% 16 .
Nonprofit generic companies
One way of lowering the cost of prescription drugs and to reduce drug shortages is nonprofit generic manufacturing. This can be set up and run by governments, or by nonprofit or philanthropic foundations. A recent example of such an endeavor is Civica Rx, a nonprofit generic company that has been set up in the United States.
Compulsory licensing
Developed countries should be more willing to use compulsory licensing to lower the cost of specific prescription drugs when negotiations with drug manufacturers on reasonable pricing fail or encounter unacceptable delays. This process permitted under the Doha declaration of 2001, allows countries to override patent protection and issue a license to manufacture and distribute a given prescription drug at low cost in the interest of public health.
Solutions: additional policy changes needed in the United States
The cost of prescription drugs in the United States is much higher than in other developed countries. The reasons for these are unique to the United States, and require specific policy changes.
Value-based pricing
Unlike other developed countries, the United States does not negotiate over the price of a new drug based on the value it provides. This is a fundamental problem that allows drugs to be priced at high levels, regardless of the value that they provide. Thus, almost every new cancer drug introduced in the last 3 years has been priced at more than $100,000 per year, with a median price of approximately $150,000 in 2018. The lack of value-based pricing in the United States also has a direct adverse effect on the ability of other countries to negotiate prices with manufacturers . It greatly reduces leverage that individual countries have. Manufacturers can walk away from such negotiations, knowing fully well that they can price the drugs in the United States to compensate. A governmental or a nongovernmental agency, such as the Institute for Clinical and Economic Review (ICER), must be authorized in the United States by law, to set ceiling prices for new drugs based on incremental value, and monitor and approve future price increases. Until this is possible, the alternative solution is to cap prices of lifesaving drugs to an international reference price.
Medicare negotiation
In addition to not having a system for value-based pricing, the United States has specific legislation that actually prohibits the biggest purchaser of oral prescription drugs (Medicare) from directly negotiating with manufacturers. One study found that if Medicare were to negotiate prices to those secured by the Veterans Administration (VA) hospital system, there would be savings of $14.4 billion on just the top 50 dispensed oral drugs 17 .
Cap on price increases
The United States also has a peculiar problem that is not seen in other countries: marked price increases on existing drugs. For example, between 2012 and 2017, the United States spent $6.8 billion solely due to price increases on the existing brand name cancer drugs; in the same period, the rest of the world spent $1.7 billion less due to decreases in the prices of similar drugs 18 . But nothing illustrates this problem better than the price of insulin 19 . One vial of Humalog (insulin lispro), that costs $21 in 1999, is now priced at over $300. On January 1, 2020, drugmakers increased prices on over 250 drugs by approximately 5% 20 . The United States clearly needs state and/or federal legislation to prevent such unjustified price increases 21 .
Remove incentive for more expensive therapy
Doctors in the United States receive a proportionally higher reimbursement for parenteral drugs, including intravenous chemotherapy, for more expensive drugs. This creates a financial incentive to choosing a more expensive drug when there is a choice for a cheaper alternative. We need to reform physician reimbursement to a model where the amount paid for drug administration is fixed, and not proportional to the cost of the drug.
Other reforms
We need transparency on arrangements between middlemen, such as pharmacy-benefit managers (PBMs) and drug manufacturers, and ensure that rebates on drug prices secured by PBMS do not serve as profits, but are rather passed on to patients. Drug approvals should encourage true innovation, and approval of marginally effective drugs with statistically “significant” but clinically unimportant benefits should be discouraged. Importation of prescription drugs for personal use should be legalized. Finally, we need to end direct-to-patient advertising.
Solutions that can be implemented by physicians and physician organizations
Most of the changes discussed above require changes to existing laws and regulations, and physicians and physician organizations should be advocating for these changes. It is disappointing that there is limited advocacy in this regard for changes that can truly have an impact. The close financial relationships of physician and patient organizations with pharmaceutical companies may be preventing us from effective advocacy. We also need to generate specific treatment guidelines that take cost into account. Current guidelines often present a list of acceptable treatment options for a given condition, without clear recommendation that guides patients and physicians to choose the most cost=effective option. Prices of common prescription drugs can vary markedly in the United States, and physicians can help patients by directing them to the pharmacy with the lowest prices using resources such as goodrx.com 22 . Physicians must become more educated on drug prices, and discuss affordability with patients 23 .
IQVIA. The global use of medicine in 2019 and outlook to 2023. https://www.iqvia.com/insights/the-iqvia-institute/reports/the-global-use-of-medicine-in-2019-and-outlook-to-2023 (Accessed December 27, 2019).
IQVIA. Global oncology trends 2019. https://www.iqvia.com/insights/the-iqvia-institute/reports/global-oncology-trends-2019 (Accessed December 27, 2019).
Kamal, R., Cox, C. & McDermott, D. What are the recent and forecasted trends in prescription drug spending? https://www.healthsystemtracker.org/chart-collection/recent-forecasted-trends-prescription-drug-spending/#item-percent-of-total-rx-spending-by-oop-private-insurance-and-medicare_nhe-projections-2018-27 (Accessed December 31, 2019).
Siddiqui, M. & Rajkumar, S. V. The high cost of cancer drugs and what we can do about it. Mayo Clinic Proc. 87 , 935–943 (2012).
Article Google Scholar
Kantarjian, H. & Rajkumar, S. V. Why are cancer drugs so expensive in the United States, and what are the solutions? Mayo Clinic Proc. 90 , 500–504 (2015).
Rajkumar, S. V. The high cost of insulin in the united states: an urgent call to action. Mayo Clin. Proc. ; this issue (2020).
DiMasi, J. A., Grabowski, H. G. & Hansen, R. W. Innovation in the pharmaceutical industry: new estimates of R&D costs. J. Health Econ. 47 , 20–33 (2016).
Almashat, S. Pharmaceutical research costs: the myth of the $2.6 billion pill. https://www.citizen.org/news/pharmaceutical-research-costs-the-myth-of-the-2-6-billion-pill/ (Accessed December 31, 2019) (2017).
Prasad, V. & Mailankody, S. Research and development spending to bring a single cancer drug to market and revenues after approval. JAMA Intern. Med. 177 , 1569–1575 (2017).
Scutti, S. Big Pharma spends record millions on lobbying amid pressure to lower drug prices. https://www.cnn.com/2019/01/23/health/phrma-lobbying-costs-bn/index.html (Accessed December 31, 2019).
Amin, T. Patent abuse is driving up drug prices. https://www.statnews.com/2018/12/07/patent-abuse-rising-drug-prices-lantus/ (Accessed November 16, 2019).
Hancock, J. & Lupkin, S. Secretive ‘rebate trap’ keeps generic drugs for diabetes and other ills out of reach. https://khn.org/news/secretive-rebate-trap-keeps-generic-drugs-for-diabetes-and-other-ills-out-of-reach/ (Accessed November 16, 2019).
Feldman, R. ‘One-and-done’ for new drugs could cut patent thickets and boost generic competition. https://www.statnews.com/2019/02/11/drug-patent-protection-one-done/ (Accessed December 31, 2019).
Cohen, M. et al. Policy options for increasing generic drug competition through importation. Health Affairs Blog https://www.healthaffairs.org/do/10.1377/hblog20190103.333047/full/ (Accessed November 16, 2019).
Bennett, C. L. et al. Regulatory and clinical considerations for biosimilar oncology drugs. Lancet Oncol 15 , e594–e605 (2014).
Fein, A. J. We shouldn’t give up on biosimilars—and here are the data to prove it. https://www.drugchannels.net/2019/09/we-shouldnt-give-up-on-biosimilarsand.html (Accessed December 31, 2019).
Venker, B., Stephenson, K. B. & Gellad, W. F. Assessment of spending in medicare part D if medication prices from the department of veterans affairs were used. JAMA Intern. Med. 179 , 431–433 (2019).
IQVIA. Global oncology trends 2018. https://www.iqvia.com/insights/the-iqvia-institute/reports/global-oncology-trends-2018 (Accessed January 2, 2018).
Prasad, R. The human cost of insulin in America. https://www.bbc.com/news/world-us-canada-47491964 (Accessed November 16, 2019).
Erman, M. More drugmakers hike U.S. prices as new year begins. https://www.reuters.com/article/us-usa-healthcare-drugpricing/more-drugmakers-hike-u-s-prices-as-new-year-begins-idUSKBN1Z01X9 (Accessed January 3, 2020).
Anderson, G. F. It’s time to limit drug price increases. Health Affairs Blog. https://www.healthaffairs.org/do/10.1377/hblog20190715.557473/full/ (Accessed November 16, 2019).
Gill, L. Shop around for lower drug prices. Consumer Reports 2018. https://www.consumerreports.org/drug-prices/shop-around-for-better-drug-prices/ (Accessed November 16, 2019).
Warsame, R. et al. Conversations about financial issues in routine oncology practices: a multicenter study. J. Oncol. Pract. 15 , e690–e703 (2019).
Download references
Author information
Authors and affiliations.
The Division of Hematology, Mayo Clinic, Rochester, MN, USA
S. Vincent Rajkumar
You can also search for this author in PubMed Google Scholar
Corresponding author
Correspondence to S. Vincent Rajkumar .
Ethics declarations
Conflict of interest.
The author declares that he has no conflict of interest.
Additional information
Publisher’s note Springer Nature remains neutral with regard to jurisdictional claims in published maps and institutional affiliations.
Supported in part by grants CA 107476, CA 168762, and CA186781 from the National Cancer Institute, Rockville, MD, USA.
Rights and permissions
Open Access This article is licensed under a Creative Commons Attribution 4.0 International License, which permits use, sharing, adaptation, distribution and reproduction in any medium or format, as long as you give appropriate credit to the original author(s) and the source, provide a link to the Creative Commons license, and indicate if changes were made. The images or other third party material in this article are included in the article’s Creative Commons license, unless indicated otherwise in a credit line to the material. If material is not included in the article’s Creative Commons license and your intended use is not permitted by statutory regulation or exceeds the permitted use, you will need to obtain permission directly from the copyright holder. To view a copy of this license, visit http://creativecommons.org/licenses/by/4.0/ .
Reprints and permissions
About this article
Cite this article.
Vincent Rajkumar, S. The high cost of prescription drugs: causes and solutions. Blood Cancer J. 10 , 71 (2020). https://doi.org/10.1038/s41408-020-0338-x
Download citation
Received : 23 April 2020
Revised : 08 June 2020
Accepted : 10 June 2020
Published : 23 June 2020
DOI : https://doi.org/10.1038/s41408-020-0338-x
Share this article
Anyone you share the following link with will be able to read this content:
Sorry, a shareable link is not currently available for this article.
Provided by the Springer Nature SharedIt content-sharing initiative
This article is cited by
Oral contraceptive pills shortage in lebanon amidst the economic collapse: a nationwide exploratory study.
- Rania Itani
- Hani MJ Khojah
- Abdalla El-Lakany
BMC Health Services Research (2023)
K-means clustering of outpatient prescription claims for health insureds in Iran
- Shekoofeh Sadat Momahhed
- Sara Emamgholipour Sefiddashti
- Zahra Shahali
BMC Public Health (2023)
An Industry Survey on Unmet Needs in South Korea’s New Drug Listing System
- Ji Yeon Lee
- Jong Hyuk Lee
Therapeutic Innovation & Regulatory Science (2023)
Voice of a caregiver: call for action for multidisciplinary teams in the care for children with atypical hemolytic uremic syndrome
- Linda Burke
- Sidharth Kumar Sethi
- Rupesh Raina
Pediatric Nephrology (2023)
Analysis of new treatments proposed for malignant pleural mesothelioma raises concerns about the conduction of clinical trials in oncology
- Tomer Meirson
- Valerio Nardone
- Luciano Mutti
Journal of Translational Medicine (2022)
Quick links
- Explore articles by subject
- Guide to authors
- Editorial policies

Life Course Patterns of Prescription Drug Use in the United States

- Standard View
- Article contents
- Figures & tables
- Supplementary Data
- Peer Review
- Open the PDF for in another window
- Permissions
- Cite Icon Cite
- Search Site
Jessica Y. Ho; Life Course Patterns of Prescription Drug Use in the United States. Demography 1 October 2023; 60 (5): 1549–1579. doi: https://doi.org/10.1215/00703370-10965990
Download citation file:
- Reference Manager
Prescription drug use has reached historic highs in the United States—a trend linked to increases in medicalization, institutional factors relating to the health care and pharmaceutical industries, and population aging and growing burdens of chronic disease. Despite the high and rising prevalence of use, no estimates exist of the total number of years Americans can expect to spend taking prescription drugs over their lifetimes. This study provides the first estimates of life course patterns of prescription drug use using data from the 1996–2019 Medical Expenditure Panel Surveys, the Human Mortality Database, and the National Center for Health Statistics. Newborns in 2019 could be expected to take prescription drugs for roughly half their lives: 47.54 years for women and 36.84 years for men. The number of years individuals can expect to take five or more drugs increased substantially. Americans also experienced particularly dramatic increases in years spent taking statins, antihypertensives, and antidepressants. There are also important differences in prescription drug use by race and ethnicity: non-Hispanic Whites take the most, Hispanics take the least, and non-Hispanic Blacks fall in between these extremes. Americans are taking drugs over a wide and expanding swathe of the life course, a testament to the centrality of prescription drugs in Americans' lives today.
- Introduction
Prescription drug use in the United States has reached record levels, rising to 6.3 billion prescriptions—approximately 19 prescriptions for every American—filled in 2020 alone ( IQVIA Institute for Human Data Science 2021 ). Now “the most common therapeutic intervention” ( World Health Organization [WHO] 2019 ), the modern prescription drug only emerged around the mid-twentieth century ( Tomes 2005 ). With few exceptions, most commonly used drugs today were developed after 1950. Beta-blockers were discovered in the mid-1960s, and statins and calcium channel blockers were not introduced until the late 1980s ( Chang and Lauderdale 2009 ; Moser 1997 ). Our most frequently prescribed drugs are relatively new medical innovations that have quickly become widespread over a short time period.
Prescription drugs have become accepted as an integral part of life in the United States—just under half (45.8%) of all Americans and 85.0% of older adults (aged 60+) interviewed in 2015–2016 reported having taken prescription drugs in the previous month ( Martin et al. 2019 ). However, we lack a full understanding of how important a role they play over the life course. This study answers the question, How many years can Americans now expect to spend taking prescription drugs? Understanding life course patterns of prescription drug use is particularly important given the high and rising rates of use. Although prescription drugs have contributed to tremendous improvements in health and life expectancy, they are not all equally beneficial or safe. As use has increased, concerns about overutilization, increasing costs, and the potential for negative health impacts have grown.
Drivers of Rising Drug Use
Several factors contribute to high and increasing levels of prescription drug use in the United States, including: population aging and rising burdens of chronic disease, medicalization (when aspects of normal life become viewed as medical conditions to be treated by drugs and other therapies; Conrad 2007 ), the ascendance of drugs to first-line treatment for an increasing array of medical conditions, the development of new drugs or new uses for existing drugs, and other institutional factors relating to the health care and pharmaceutical industries. Antibiotics, such as penicillin and sulfa drugs, were among the earliest drugs to be developed. As mortality from infectious diseases declined and the burden of chronic diseases rose over the course of the epidemiological transition ( Horiuchi 1999 ; Omran 1971 ), efforts to treat and prevent chronic diseases, such as cardiovascular disease and cancer, and other conditions (e.g., chronic pain) spurred drug development during the twentieth century ( Zajacova et al. 2021 ; Zimmer and Zajacova 2020 ).
Increasing medicalization has been the predominant trend in the United States ( Conrad 2005 ). Numerous aspects of daily life are increasingly regarded as conditions requiring medical diagnoses and treatment, especially using prescription drugs. Medicalized phenomena include obesity, substance use, hyperactivity, loneliness, childbirth, pain, and aging ( Armstrong 2003 ; Conrad and Schneider 1992 ; Owens 2020 ; Zajacova et al. 2021 ; Zimmer and Zajacova 2020 ). Society has come to expect and demand “a pill for every ill” ( Busfield 2010 :934). Prescription drugs thus moved from the periphery to the core of medicine. Drugs have become the go-to treatment, a shift reinforced by factors relating to the culture of medicine, changing institutional constraints in health care, and health policy. Increased prescribing is spurred by physicians' curative orientation and factors that became increasingly salient during the managed care era, including increased time constraints, the tying of physician pay to patient satisfaction, and uncertainty in clinical practice ( Busfield 2010 ; Ho 2017 , 2019 ; Tomes 2005 ). Insurance providers' reimbursement policies also constrain treatment options. Prescription drugs, and often specific drug classes, must frequently be tried before or have higher insurance coverage than nondrug therapies. For example, insurers had more generous coverage of opioids and fewer or no requirements for approval relative to non-opioid painkillers for pain-related conditions ( Thomas and Ornstein 2017 ).
Pharmaceutical companies contributed to increased prescribing through physician payments, sponsorship of medical conferences and educational seminars, and direct-to-consumer advertising. Between 1997 and 2016, medical marketing increased from $17.7 to $29.9 billion ( Schwartz and Woloshin 2019 ). Prescription drug advertising was a key driver of this expansion, with 4.6 million advertisements (including 663,000 TV commercials) amounting to $6 billion spent in 2016 alone ( Schwartz and Woloshin 2019 ). Growth of the generic drug market and policies such as Medicare Part D have also increased access to prescription drugs and their affordability ( Carr 2017 ; Catlin et al. 2008 ; Qato et al. 2008 ).
The Changing Nature of Drug Use
Individuals increasingly take large quantities of several prescription drugs simultaneously, for long durations, and for a widening array of conditions. Polypharmacy—the concurrent use of multiple prescription drugs—is high and increasing among adults and children ( Hales et al. 2018 ; Kantor et al. 2015 ; Qato et al. 2018 ). Older adults have the highest levels of polypharmacy: among adults aged 65+, 42% took five or more prescription drugs in 2012 ( IMS Institute for Healthcare Informatics 2013 ). Contributing factors include high burdens of chronic disease and multimorbidity, the intensification of treatment for chronic diseases, a growing propensity to treat earlier disease stages (e.g., prediabetes), fragmentation in the health care system, and increasing use of drugs to counter side effects of other drugs.
People are taking more drugs for longer durations. The earliest drugs—antibiotics—were typically taken for short periods to treat acute infections. With the rise of chronic diseases, people began to take drugs for extended periods—often from initial diagnosis through the remainder of their lifetimes. For example, individuals with hypertension are expected to take antihypertensive medications for the rest of their lives ( Lemelin 1989 ; van der Wardt et al. 2017 ). Similarly, the United Kingdom's National Health Service states, “You usually have to continue taking statins for life because if you stop taking them, your cholesterol will return to a high level within a few weeks” ( National Health Service 2018 ). Medicalization and expansions in prescription drug use mean that people are being prescribed drugs for more conditions. Antidepressant and other psychotherapeutic drug use has been rising since the 1950s, and hormone therapy for menopause began increasing in the early 1980s ( Goldman 2010 ; López-Muñoz and Alamo 2009 ).
Although transitioning people off prescription drugs is not presently a routine part of medical care, efforts involving deprescribing—a patient-centered approach to reduce or stop the use of drugs entirely—have intensified ( Hill et al. 2020 ; Rochon et al. 2021 ). Deprescribing often aims to address polypharmacy among older adults and decrease the use of drugs that are unnecessary, cause adverse effects, or have potential harms outweighing expected benefits ( Scott et al. 2015 ). Evidence indicates that deprescribing interventions have successfully reduced inappropriate medication usage ( Bloomfield et al. 2020 ).
Racial and Ethnic Differences in Drug Use
Racial and ethnic disparities in health care are a long-standing concern. Several studies have documented that racial and ethnic minorities have greater difficulty accessing care, receive lower quality of care, and are less likely to receive routine medical care ( Centers for Disease Control and Prevention [CDC] 2004a ; Kirby and Kaneda 2010 ; Schulman et al. 1999 ; Smedley et al. 2003 ). These differences persist even after accounting for socioeconomic characteristics and health status, and they contribute to racial and ethnic disparities in health and mortality ( CDC 2004b ; Williams and Jackson 2005 ). Prescription drug use is considerably lower among Blacks and Hispanics than non-Hispanic Whites ( Smedley et al. 2003 ). This pattern applies to children and older adults, with one study finding that Black and Hispanic Medicare beneficiaries used 10% to 40% fewer drugs than White beneficiaries with the same chronic conditions ( Briesacher et al. 2003 ; Gaskin et al. 2006 ; Hahn 1995 ; Mahmoudi and Jensen 2014 ). Several studies have documented racial and ethnic disparities in pain treatment and opioid prescribing ( Anderson et al. 2009 ; Burgess et al. 2014 ; Pletcher et al. 2008 ). Blacks and Hispanics are less likely to be prescribed painkillers for acute and chronic pain in many settings, including emergency room, ambulatory care, and palliative care settings, and they also receive lower dosages ( Meghani et al. 2012 ; Pletcher et al. 2008 ). Patterns of psychotropic medication use are mixed. Some studies have found that White children and adults were more likely to be prescribed such drugs than Blacks and Hispanics ( Akincigil et al. 2011 ; Cook et al. 2016 ; Zito et al. 1998 ), but others have found that Blacks were more likely to be prescribed antipsychotics ( Aggarwal et al. 2012 ; Segal et al. 1996 ). Although some studies were based on nationally representative data, others were restricted to small samples from single states, and most did not examine differences over the entire age range. One of this study's goals is to examine the implications of these patterns for racial and ethnic differences in prescription drug use over the life course.
Although Americans are taking drugs in record quantities, no estimates exist of the total number of years individuals can expect to take prescription drugs over their lifetimes. It is critical to understand a phenomenon that occurs across so much of the life course and whose importance shows no sign of lessening, only increasing. This study provides the first estimates characterizing life course patterns of prescription drug use in the United States between 1996 and 2019. These estimates allow us to identify how much of their lives Americans now spend taking prescription drugs and how these measures have changed over time, with particular attention to differences by gender, race, and ethnicity.
- Data and Methods
Data on prescription drug use come from the Medical Expenditure Panel Survey (MEPS), a long-running, annual survey that is nationally representative of the U.S. civilian noninstitutionalized population ( Agency for Healthcare Research and Quality 2021 ). Its sampling frame draws from a subsample of households interviewed in the prior year's National Health Interview Survey. The MEPS uses a panel design consisting of five interviews covering two calendar years, with a new panel of approximately 15,000 sample households selected each year. A single household respondent provides information about each household member. Multiple panels are used to capture the household's prescription drug use during each calendar year, but the panel structure is not used for other purposes in this study. The MEPS is the primary source of national estimates of health care use, expenditures, insurance coverage, sources of payment, and access to care ( Hill et al. 2014 ).
The MEPS Prescribed Medicines files provide information on all prescriptions purchased by household members. The drug names and therapeutic classes are supplied by the Multum Lexicon database, which is also used by other surveys (e.g., the National Health and Nutrition Examination Survey and the Health and Retirement Study). The MEPS prescription drug information is based on household reports and, if respondents grant permission, is verified by contacting pharmacies. These reports include all medications prescribed during any health care encounter, including outpatient visits, emergency room visits, and hospital stays ( Agency for Healthcare Research and Quality 2019 ; Hill et al. 2014 ). I link the Prescribed Medicines files to the MEPS Full-Year Consolidated Data files ( Blewett et al. 2019 ), which provide key demographic characteristics, including sex, birth year, race, and ethnicity.
Advantages of the MEPS include that it is nationally representative, has a large sample size, covers the entire age range, and has high response rates. Several MEPS features give us confidence in the quality of its prescription data. First, households are interviewed in person five times within two years, resulting in an average recall period of just five months ( Hill et al. 2011 ). This is a relatively short period compared with other surveys, which are typically fielded at least one and often multiple years apart. Shorter recall periods are associated with greater reporting accuracy ( Boudreau et al. 2004 ). Accuracy is generally greatest for drugs used to treat chronic or serious conditions ( Hill et al. 2011 ). The MEPS prompts respondents with lists of drugs they reported taking in prior interviews, asks them about both new prescriptions and refills, and asks about prescriptions phoned in by a doctor to a pharmacy ( Hill et al. 2011 ). Respondents are also asked whether medicines were prescribed during specific medical encounters, including emergency room visits, dentist visits, and inpatient stays. The MEPS verifies respondent-reported information using a follow-back survey of pharmacies ( Wang et al. 2008 ). In 2011, 69.7% of households permitted the MEPS to contact their pharmacies ( Hill et al. 2014 ). A validation study of the MEPS data (performed by matching to a sample of Medicare claims) found that the MEPS was highly accurate, with high levels of concordance on prescription drug use (an agreement rate of .97), average number of fills (37.4 in the MEPS vs. 38.2 in the Medicare sample), and mean annual expenditures ($2,426 in the MEPS vs. $2,331 in the Medicare sample) ( Hill et al. 2011 ). The MEPS prescription data are much more accurate than other commonly used surveys, such as the Medicare Current Beneficiary Survey and Consumer Expenditure Surveys, which have higher underreporting rates ( Poisal 2003 ; U.S. Bureau of Labor Statistics 2023 ).
For each year in 1996–2019, I combine the MEPS data with the corresponding sex-specific period U.S. life tables from the Human Mortality Database (HMD; 2021) . For the analyses by race and ethnicity, I combine the MEPS data with the corresponding race-ethnicity-sex-specific period life tables from the National Center for Health Statistics (NCHS) for each year in 2006 (the earliest year they are available) through 2019 ( NCHS 2022a ).
The analysis examines three dimensions of prescription drug use: (1) any use versus no use, (2) the number of drugs, and (3) the class of drugs. I start with two descriptive measures: the percentage of people at each age taking any drugs and the age-standardized percentages of people taking zero, one, two, three, four, or five or more drugs. The age standard is the 2010 U.S. population ( NCHS 2022b ).
Estimates of the number of years individuals can be expected to take prescription drugs are produced using Sullivan's method, the most widely used method for calculating health expectancies ( Sullivan 1971 ). Sullivan's method has been used to study many phenomena, including disability, loneliness, and health insurance coverage ( Jagger and Robine 2011 ; Kirby and Kaneda 2010 ; Raymo and Wang 2022 ). I combine the MEPS data with life tables from the HMD and NCHS to apportion the total number of years lived into years expected to be spent in different states (e.g., taking any prescription drugs, a certain number of drugs, or various classes of drugs). Sullivan's estimator is consistent and unbiased, and no additional assumptions are required beyond stationarity, the standard life table assumption ( Imai and Soneji 2007 ). In this application, the method takes into account mortality differentials between individuals taking drugs and those not taking drugs and between individuals taking different classes or numbers of drugs. The estimates are independent of population age structure, such that changes in the population age distribution will not drive estimated trends in drug use ( Jagger et al. 2014 ; Mathers 1991 ).
where x = 0, 1, 5, . . . , 85, n π a is the proportion of individuals in age group a to a + n taking prescription drugs (from the MEPS), n L a is the number of person-years lived in the age interval a to a + n (from the HMD or NCHS life tables), and l x is the number of survivors to exact age x (from the HMD or NCHS life tables). The interpretation of YLPD 0 in 2019 is the number of years a newborn in 2019 could expect to spend taking prescription drugs during their lifetime if, at each age, they experienced the prescription drug use prevalence and mortality rates observed in 2019.
Together, YLPD x and PDFLE x sum to life expectancy at age x .
where e x is life expectancy at age x .
and the expected number of years taking zero, one, two, three, four, and five or more drugs sum to overall life expectancy at age x .
To investigate the number of years individuals can expect to spend taking different types of drugs, I calculate n π a D C proportions specific to drug (sub)classes specified by the Multum Lexicon therapeutic classification system. Each drug is assigned to a primary therapeutic class. A consistent set of therapeutic classes is used to account for changes over time in the classification scheme. I specify 22 mutually exclusive and exhaustive classes of drugs: anti-infectives, antineoplastics, biologicals and immunologic agents, cardiovascular agents (excluding antihypertensives), antihypertensives, central nervous system (CNS) agents (excluding analgesics and opioids), analgesics (excluding opioids), opioids, coagulation modifiers, gastrointestinal agents, genitourinary tract agents, hormones and hormone modifiers, respiratory agents, psychotherapeutic agents (excluding antidepressants and antipsychotics), antidepressants, antipsychotics, metabolic agents (excluding statins), statins, topical agents, alternative medicines, nutritional products, and miscellaneous agents. 1 These drug classes include the most commonly used drugs ( Kantor et al. 2015 ), the top five classes in terms of spending ( Moeller et al. 2004 ), and the ones most commonly implicated in adverse drug events ( CDC 2023 ; Shehab et al. 2016 ). I also examine a subset of drugs referred to as “key prescription drugs” expected to be used over the longer term or for more severe or chronic conditions: antineoplastics, biologicals and immunologic agents, cardiovascular agents (excluding antihypertensives), antihypertensives, CNS agents (excluding analgesics and opioids), non-opioid analgesics, opioids, coagulation modifiers, gastrointestinal agents, genitourinary tract agents, hormones and hormone modifiers, respiratory agents, psychotherapeutic agents (excluding antidepressants and antipsychotics), antidepressants, antipsychotics, metabolic agents (excluding statins), and statins. In these analyses, I use an approach similar to the one described previously, using the proportion of individuals in each age group taking each class of drugs.
I conducted all analyses using Stata version 16.1 and used MEPS-provided weights to account for complex survey design.
Expected Years and Share of the Life Course Spent Taking Drugs
Prescription drug use has reached very high levels, with more than half of all men (52.2%) and women (62.0%) taking prescription drugs in 2019 (percentages are age-standardized). For the subset of key drugs, these figures remain fairly high, at 43.8% and 53.0% for men and women, respectively. Use is strongly patterned by age and sex (panel a, Figure 1 ). Among men, use decreases between childhood and young adulthood (between ages 1–4 and 20–24) and increases from age 25 onward. In contrast, use increases nearly continuously among women from age 5 onward. At all ages above 40, more than half of men take prescription drugs. Women cross the 50% threshold much earlier: at all ages above 15, the majority of women take prescription drugs. 2 These gender differences are partly related to women's 12% to 24% greater use at reproductive ages. Appendix Figure A1 (shown in the online appendix, along with all other figures and tables designated with an “A”) shows that if hormonal contraceptives were excluded, gender differences would be smaller but would not disappear; at reproductive ages, the reduction in the gender gap would be roughly one third. Other important contributors to gender differences include other hormones, analgesics, and psychotherapeutics.
Large differences across racial and ethnic groups exist (panel b of Figure 1 ), with use ramping up at much earlier ages for non-Hispanic Whites than for non-Hispanic Blacks and Hispanics. The age above which the majority of men take prescription drugs is 40 for Whites, 45 for Blacks, and 55 for Hispanics. For women, these disparities are even greater. More than half of all White women older than 15 take drugs, whereas Black and Hispanic women do not reach this rate until they are much older (40 and 45, respectively). Large gaps in use occur between Whites and the two other groups at young and prime adult ages. The percentage of White men taking drugs is an average of 11% to 12% higher at ages 10–44 and 10–84 than for Black and Hispanic men, respectively. The pattern for women is similar, although the differences are larger (at 16% to 18%, on average), and convergence occurs at age 50 for Black women and 65 for Hispanic women.
The percentages shown in Figure 1 translate to a considerable number of years that individuals can be expected to take drugs ( Table 1 ). For example, life expectancy for a newborn boy in 2019 was 76.59 years. Of those years, he could expect to take prescription drugs for 36.84 years (48% of his life) and not to take drugs for the remaining 39.75 years (52% of his life). A newborn girl in 2019, with a life expectancy of 81.72 years, could expect to take prescription drugs for many more years (47.54 years, nearly 60% of her life). The number of years U.S. men and women can expect to spend taking drugs has generally increased at every age during 1996–2019 (the exceptions are for women at ages 0 and 1).
The share of remaining life expectancy spent taking drugs also increased with age. For example, 25-year-old men and women in 2019 could expect to take prescription drugs for, respectively, 59.4% and 71.1% of their remaining years. For 65-year-old men and women, this share approached 90%. Between 1996 and 2019, the share of remaining life expectancy spent taking drugs increased at every age among men. Among women, it decreased slightly at ages below 50 and increased at ages above 50.
Compared with Whites, Blacks and Hispanics can expect to take prescription drugs for far fewer years (appendix Table A2 ). For example, in 2019, newborn boys in these groups could expect to take drugs for 39.01, 30.83, and 33.99 years, respectively. The corresponding figures for newborn girls were 50.99, 41.34, and 43.80 years. These differences partly reflect differences in life expectancy. Comparing the proportion of remaining life expectancy spent taking drugs helps account for those differences. Using this metric, I find that Black and Hispanic newborn boys could be expected to spend 43% of their lives taking drugs compared with 51% for White boys in 2019. In 2019, newborn Black and Hispanic girls could be expected to spend roughly 10% less of their lives (53% and 52%, respectively) taking drugs than newborn White girls (63%). These patterns hold through much of the age range, with convergence occurring at roughly age 65 for women and 80 for men.
Trends in the Use of Multiple Drugs
Figure 2 shows trends in the age-standardized percentages of people taking zero, one, two, three, four, and five or more prescription drugs. Most of the change has occurred at the extremes. Declines in the percentage of Americans taking only one drug have been matched by concomitant rises in the percentage taking five or more drugs (panel a, Figure 2 ). In the mid-1990s, among people taking any drugs, taking only one drug was the modal category. Within a decade, the percentages of people taking one and five or more prescription drugs had converged and remained fairly similar for both men and women through 2019, when they reached, respectively, 14% and 13% for men and 15% and 16% for women. In contrast, the percentages for the intermediate categories (i.e., taking two, three, or four drugs) were fairly stable over time.
These trends translate into large expansions in the share of life people take large numbers of prescription drugs. Figure 3 shows the years that individuals could expect to take zero, one, two, three, four, and five or more drugs at various ages in 1996 and 2019. With increasing age, many fewer years of remaining life are expected to be spent taking no drugs. The most dramatic change between 1996 and 2019 was the substantial increase in years of taking five or more drugs (red bars) that have occurred at each age. By 2019, the share of Americans' lives spent taking large numbers of drugs was nontrivial. A newborn boy in 2019 could expect to take five or more drugs for 11.56 years (or 15% of his life), compared with 16.29 years (a fifth of her life) for a newborn girl in 2019. These figures were considerably lower (9% and 16%, respectively) in 1996. Among 85-year-olds in 2019, men and women could expect to take five or more drugs for more than half (52% and 58%, respectively) of their remaining lives, up from 28% and 35%, respectively, in 1996.
There are important differences in the number of drugs taken across racial and ethnic groups (panel b of Figure 2 ). Between 2006 and 2019, the percentage taking no prescription drugs was consistently highest among Hispanics, intermediate for Blacks, and lowest for Whites. Correspondingly, the inverse of this pattern was generally replicated across each of the other categories (i.e., Whites had the highest percentages taking one, two, three, and four drugs, followed by Blacks and then Hispanics). Levels of polypharmacy (five or more drugs) were lowest for Hispanics but were very similar for Blacks and Whites. Over this period, the share of Black and White men taking five or more drugs was 14%, compared with 11% for Hispanic men. The corresponding figures for women were 18% and 14%, respectively.
These patterns are reflected in racial and ethnic differences in the proportions of remaining life expectancy expected to be spent taking prescription drugs (appendix Figure A2 ). Whites could expect not to take drugs for the smallest shares of their lives, whereas Blacks and Hispanics experienced similarly larger shares. For example, among newborns in 2019, White boys could expect not to take drugs for roughly half (49%) their lives, compared with 57% for Black and Hispanic boys. These differences were even larger for women. White newborn girls could expect not to take drugs for only a third (34%) of their lives, compared with 47% and 48% for Black and Hispanic girls, respectively. Similar patterns are observed at age 65, although the magnitudes of the differences are smaller given the racial and ethnic convergence in drug use with age. Interestingly, the distribution across the number of drugs among those taking any drugs is highly similar across racial and ethnic groups. In other words, much of difference across racial and ethnic groups is driven by the larger proportions of Blacks and Hispanics taking no drugs.
Trends in Types of Drugs Used
Figure 4 (panels a and b) shows trends in the number of years expected to be spent taking eight common classes of drugs (see also Table 2 and appendix Figures A4–A6 ). Among these classes, newborns could expect to be taking antihypertensives for the most years, followed by analgesics (through the early 2000s) or statins for men and CNS agents (post-2003) for women. Among 65-year-olds, antihypertensives are taken the most years, followed by statins (since the mid-2000s). Among men, antihypertensive drug use increased tremendously over the period studied. Newborn boys in 1996 could expect to take antihypertensives for 10.14 years; by 2019, this figure reached 17.21 years. Among men aged 65, this figure nearly doubled from 6.67 to 12.18 years. Women experienced large increases in antidepressant use. A newborn girl in 1996 could expect to take antidepressants for 5.55 years; by 2019, this figure more than doubled to 12.52 years. Antidepressant use among older women also grew substantially, from 1.89 to 5.13 years. Men could expect to spend many fewer years taking antidepressants than women—5.42 years for newborn boys (vs. 12.52 years for girls) and 2.29 years for men aged 65 (vs. 5.13 years for women aged 65) in 2019. Use of statins, CNS agents, and other metabolic agents also increased substantially over time. In contrast, years taking other cardiovascular agents remained fairly stable over time among men and decreased among women.
Disaggregating patterns by race and ethnicity reveals some similarities but also key differences ( Figure 4 , panels c and d). Members of all racial and ethnic groups could expect to spend the most years taking antihypertensives. In 2019, years taking antihypertensives were similar among men (with large increases occurring among Hispanics between 2006 and 2019), but Black and Hispanic women actually took antihypertensives for more years than White women. Among men, Whites took cardiovascular agents, CNS agents, and antidepressants for many more years than Blacks and Hispanics. Among women, Whites took cardiovascular agents, CNS agents, antidepressants, and opioids for more years. The discrepancies in antidepressant use are particularly large, amounting to roughly 10-year differences. In 2019, Black and Hispanic women could expect to take antidepressants for 4.83 and 6.76 years, respectively, compared with 15.94 years among White women. Black men and women took statins for the fewest years, but years of statin use were similar for White and Hispanic men and women in 2019.
Trends in opioid use are of interest, given the ongoing drug overdose epidemic and recommendations to reduce opioid prescribing. Although attention surrounding drug overdose increased in the 2000s, culminating in restrictions on opioid prescribing and a shift from prescribed to illicit drugs ( Ho 2019 ; Rudd et al. 2016 ), the expected number of years spent taking opioids continued to rise through 2015 ( Figure 4 ). Despite subsequent declines in years of life expected to be taking opioids among newborns between 1996 and 2019, expected years of taking opioids remained higher for men and women aged 65 in 2019 than in 1996. Years taking opioids declined from their peak for all racial and ethnic groups. Among men, declines were largest for Hispanics, followed by Whites and then Blacks. Among women, Blacks experienced the largest declines, followed by Hispanics and then Whites.
Figure 5 shows the share of remaining life expectancy at ages 0 and 65 spent taking specific classes of drugs for the total population. (Appendix Figure A7 shows the corresponding information by race and ethnicity, with patterns highly similar to those described here.) These classes account for the largest shares of drug use over individuals' lifetimes (see Table 2 for all drug classes). Individuals can expect to take cardiometabolic drugs, anti-infectives, and psychotherapeutics for the largest shares of their lives. For most of these drugs, the share of remaining life expectancy taking these drugs increases with age. Among men, shares of remaining life expected to be spent taking cardiometabolic drugs, psychotherapeutics, gastrointestinal agents, and coagulation modifiers increased between 1996 and 2019. The share of remaining life expected to be spent taking anti-infectives, topical agents, respiratory agents, and opioids decreased. Similar patterns are observed for women. The share of remaining years taking hormones and hormone modifiers increased for men but decreased for women at ages 0 and 65. Compared with men, women took several types of drugs for considerably greater shares of their lives. For example, women aged 65 and 85 in 2019 could expect to take antidepressants for, respectively, 24% and 22% of their remaining lives. Men could expect to take antidepressants for many fewer years than women: the shares of their lives spent taking antidepressants were roughly half those of women at ages below 85 in 2019. In 1996, a woman aged 85 could expect to take cardiometabolic drugs for 78% of her remaining years, compared with only 58% for a man aged 85. In 2019, a woman aged 45 could expect to take psychotherapeutics for 39% of her remaining lifetime, compared with only 25% for her male counterpart.
Prescription drug use has become a highly central, prevalent, and expanding part of the life course in the United States. However, for a phenomenon that occurs across so much of the life course and has enormous potential to affect health, well-being, and other outcomes, it remains surprisingly understudied. This study finds that newborn girls and boys in 2019 can now expect to spend more than or close to half their lives—47.54 and 36.84 years, respectively—taking prescription drugs. In other words, a newborn girl in 2019 can be expected not to take drugs for only approximately 40% of her life. Even as life expectancy has increased—albeit much more slowly since 2010 than in earlier decades ( Ho and Hendi 2018 )—the share of Americans' lives spent taking drugs increased at all ages for men and at all ages above 50 for women between 1996 and 2019. The years Americans can expect to spend taking drugs increased at most ages (except below age 5 for women); the number of years expected to be spent not taking any drugs also increased in some of the younger age groups. With future life expectancy increases, it will be interesting to observe whether those additional years are spent taking or not taking prescription drugs.
High levels of use begin early in the life course. The majority of women older than 15 take prescription drugs; the corresponding age for men is 40. This study also finds that the nature of drug use has shifted considerably over time. The share of Americans' lives spent simultaneously taking large numbers of drugs is substantial and expanded dramatically over time. In 1996, no men at any age could expect to take five or more drugs for more than a third of their remaining life expectancy. By 2019, men in all age groups above 50 could expect to take five or more drugs for 36% to 53% of their remaining life expectancy. Among women, the maximum share of remaining life taking five or more drugs was 38% in 1996. By 2019, women in all age groups above 50 could expect to take five or more drugs for 40% to 58% of their remaining years.
Americans are taking statins, antihypertensives, and antidepressants for large and growing portions of their lives. In 2019, newborns could be expected to take antihypertensives for nearly a quarter of their lives, compared with two thirds for 65-year-olds. The share of remaining years Americans could be expected to take statins increased more than fourfold at every age, compared with 30% to 68% for antihypertensives. These trends are likely related to several factors, including the growing burden of obesity, longer survival with chronic conditions, and the intensification of treatment of high blood pressure and cholesterol. The rising and potentially unnecessary use of psychotherapeutics, particularly among adolescents, is of growing concern ( Richtel 2022 ). This study finds that the share of newborns' lives expected to be spent taking antidepressants roughly doubled between 1996 and 2019.
This article documents striking gender differences. At every age, women have much higher rates of prescription drug use than men: women can expect to take drugs for more years and for a greater share of their remaining lifetimes and to take five or more drugs for a greater proportion of their lives. Men and women also take different types of drugs. Large gender differences in the number of years newborns in 2019 could expect to take drugs were observed for psychotherapeutics (8.45 years), particularly antidepressants (7.11 years); hormones and hormone modifiers (6.72 years); anti-infectives (6.09 years); and painkillers (3.73 years) (appendix Table A5 ). Gender differences in drug use are multifaceted and related to many factors, including the fact that contraceptives remain largely targeted toward women. These patterns also reflect physicians' long-standing tendencies to prescribe psychotherapeutics (particularly tranquilizers) to women since the late 1800s and early 1900s, Valium in the 1960s and 1970s, and benzodiazepines in recent decades ( Courtwright 1982 ; Herzberg 2006 ; Olfson et al. 2015 ). Other factors include gender differences in health care access and utilization, norms relating to care seeking and symptom presentation (especially related to pain relief), and morbidity (e.g., women experience a higher burden of pain-related chronic conditions, such as rheumatoid arthritis, migraines, and fibromyalgia) ( Case and Paxson 2005 ; Ho 2020 ; Roe et al. 2002 ). However, there is one class of drugs women take for fewer years of their lives than men: drugs to treat cardiovascular disease. Interestingly, these differences widened, rather than narrowed, over time (appendix Table A5 ). This widening is concerning in light of prior assessments that gender differences in prescribing may not be evidence-based and studies finding that women are more likely to be prescribed drugs for the management of depression, migraines, thyroid disorders, and other chronic conditions but less likely to be prescribed drugs for cardiovascular disease prevention ( Rochon et al. 2021 ).
This study also reveals complex patterns of racial and ethnic differences in prescription drug use. Use starts much earlier among Whites than among Blacks and Hispanics, with particularly large gaps at the young and middle adult ages among women before convergence at the older ages. As a result, for most of the age range (below ages 65 and 80 for women and men, respectively), Blacks and Hispanics can expect to take no prescription drugs for higher proportions of their remaining lifetimes than Whites. This finding is unlikely to be due to better health among Blacks and Hispanics, given that both groups experience higher mortality than Whites at these ages ( Cunningham et al. 2017 ; Fenelon et al. 2017 ). Instead, this difference likely reflects inequality across racial and ethnic groups in access to care or receipt of prescriptions. Some phenomena are shared across racial and ethnic groups, including greater levels of use among women than men, high polypharmacy levels, and high rates of antihypertensive use. However, we also observe key racial and ethnic differences in the proportions of life people can expect to spend taking certain classes of drugs (appendix Tables A3 and A4 ). Use of cardiometabolic drugs generally converged among women but remained lower for Black and Hispanic men than White men, particularly at young and middle adult ages. Black men can be expected to take statins for 4% to 14% less of their lives than White men, which is concerning given Black men's high rates of cardiovascular disease mortality and that these gaps did not narrow appreciably between 2006 and 2019. Opioid use was similar among Black and White men but lower among Hispanic men. Among women, who take opioids for greater shares of their lives than men, Blacks and Hispanics can generally be expected to take opioids for lower proportions of their lives than Whites. The most sizable differences are observed for psychotherapeutics. For example, newborn White girls in 2019 could be expected to take such drugs for nearly a third (32%) of their lives, compared with 18% and 20% for Black and Hispanic girls, respectively. The magnitudes of these differences are fairly stable over the life course. The patterns for newborn boys in 2019 are similar, although the differences are slightly smaller (e.g., 8% vs. 12–14%).
The study results have implications for health care spending. Prescription drug expenditures are high, reaching $335.0 billion—roughly a tenth of national health expenditures—in 2018 ( Centers for Medicare & Medicaid Services 2020 ; Hartman et al. 2020 ). Per capita drug spending is higher in the United States than in all other high-income countries ( Kesselheim et al. 2016 ; OECD 2023 ; Sarnak et al. 2017 ). Trends in drug use may have outsized impacts on specific programs. Medicare and Medicaid are among the largest payers for prescriptions, which accounted for nearly a fifth of Medicare spending in 2016 ( Kaiser Family Foundation 2019 ). The finding that men and women aged 65 in 2019 could expect to take prescription drugs for an additional 16.46 and 18.88 years—up from 12.74 and 16.00 years in 1996, respectively—is particularly salient for Medicare. For more than half of those 16.46 and 18.88 years, men and women can be expected to take five or more drugs. Out-of-pocket expenditures are also significant, accounting for 14% of drug spending. Not only are current levels of drug spending high, but they are projected to be the fastest-growing category of health care spending in the coming decade ( Centers for Medicare & Medicaid Services 2020 ; Cuckler et al. 2018 ; Hartman et al. 2020 ). By 2026, prescription spending is projected to increase to $875 billion, or 15.4% of national health expenditures ( Roehrig 2018 ).
In addition to their fiscal impacts, trends in prescription drug use exert complex influences on health and mortality. On the one hand, prescription drugs are a cornerstone of disease management and contributed to health and life expectancy improvements over the past several decades. On the other hand, the opioid epidemic, which has resulted in over 1 million (1,082,050) drug overdose deaths as of 2021, constitutes one of the starkest manifestations of the unintended consequences of use ( CDC 2021a , 2021b ). The risks of drug–drug interactions and adverse drug events increase with the number of drugs taken. Each year, adverse drug events result in approximately 1.3 million emergency department visits, with blood thinners, diabetes medications, heart medications, seizure medications, and opioid painkillers most commonly implicated in these events ( CDC 2023 ). Rises in polypharmacy are particularly impactful for older adults, who are at the greatest risk of experiencing negative effects due to their greater likelihood of taking more drugs; metabolic changes associated with aging, including decreased renal and hepatic function; body composition (e.g., lower lean body mass); and higher prevalence of impaired cognition and mobility ( IMS Institute for Healthcare Informatics 2013 ; Masnoon et al. 2017 ). Polypharmacy increases the risk of falls, hospitalization, cognitive impairment, lower quality of life, and mortality ( Maher et al. 2014 ; Masnoon et al. 2017 ; WHO 2019 ). Deprescribing, as mentioned earlier, is an approach that seeks to reduce polypharmacy and inappropriate prescribing. Both domestic (e.g., U.S. Deprescribing Research Network) and international (e.g., International Group for Reducing Inappropriate Medication Use & Polypharmacy) networks provide information and coordinate efforts relating to deprescribing. Several frameworks or decision algorithms have been developed to identify inappropriate medication use and implement deprescribing ( Curtin et al. 2021 ; Farrell et al. 2016 ; Rochon et al. 2021 ; Scott et al. 2015 ). As such efforts become more widespread and accepted, adverse impacts associated with drug use might be reduced ( Reeve et al. 2017 ). Given the high prevalence and initiation of use at younger ages, extending deprescribing efforts beyond older adults may be beneficial.
The environmental impacts of prescription drug use pose additional concerns. The first reports documenting that heart medications, analgesics, contraceptives, and other pharmaceuticals were present in wastewater and other water resources in the United States date from the 1970s ( Caban and Stepnowski 2021 ). One study estimated that 80% of 139 streams sampled in 30 states contained pharmaceuticals in 1999–2000 ( Kolpin et al. 2002 ). Pharmaceutical ingredients have been detected in water supplies worldwide ( WHO 2012 ; Wilkinson et al. 2022 ). Prescription drugs can make their way into the environment through manufacturing, sewage and wastewater systems from residential areas and health care facilities, and improper disposal of unused or expired medicines ( Boxall 2004 ; WHO 2012 ). Because wastewater treatment plants do not entirely eliminate pharmaceuticals, people can be exposed to residues in drinking water and food ( OECD 2019 ). Growing antibiotic resistance is also a concern. Studies indicate that the levels present in the environment are below the thresholds that would pose health risks to humans ( Boxall 2004 ; WHO 2012 ). However, these studies generally study one substance at a time rather than capturing joint or interactive impacts of exposure, are typically of short duration, and rarely examine the effects of long-term, low-level exposure. Furthermore, several commonly used drugs (e.g., beta-blockers, antidepressants, analgesics, and anti-inflammatory drugs) have been found to negatively impact aquatic species and ecosystems ( Boxall 2004 ; Kusturica et al. 2022 ).
The present study's limitations include that the MEPS sample is restricted to the noninstitutionalized population and the potential for underreporting in the survey. Because the institutionalized population likely has higher levels of drug use, the estimates produced in these analyses should be conservative. The MEPS attempts to address potential underreporting of prescription drug use by following up with pharmacies, using multiple prompts to elicit information about prescription drug use (including asking respondents to bring medication containers to the interview), and using multiple rounds within each survey wave to enable shorter recall periods that improve reporting accuracy ( Boudreau et al. 2004 ; Hill et al. 2011 ; Nahin et al. 2019 ). The MEPS also prompts respondents by asking whether medicines are prescribed during various health care events, including emergency room visits, dentist visits, and inpatient stays ( Hill et al. 2014 ). A validation study of the MEPS based on matching the MEPS to Medicare claims data found the MEPS data to be highly accurate ( Hill et al. 2011 ). Furthermore, accuracy was highest for maintenance drugs and for drugs for serious conditions, which are the categories this study is most interested in capturing. One limitation of Sullivan's method is that it may underestimate or overestimate health expectancies when transition rates between health states are changing rapidly. Because drug use has been increasing over time, the estimates produced in these analyses are expected to be conservative ( Mathers and Robine 1997 ). Another potential concern is that short-term drug use may inflate estimates of lifetime drug use. However, this concern is somewhat mitigated because most prescription drug use is expected to be permanent rather than transient ( Lemelin 1989 ; National Health Service 2018 ; van der Wardt et al. 2017 ). Finally, this article focuses exclusively on prescription drugs, even though over-the-counter medications are also an important feature of medication use.
Over the past several decades, prescription drug use has become a highly central, prevalent, and expanding part of the life course in the United States. Americans today can expect to spend more than or close to half their lives taking prescription drugs. This is more than the share of their lives expected to be spent completing formal education, in a first marriage ( Hendi 2019 ), and perhaps even in the labor force. We are just beginning to be able to observe cohorts of older adults who experienced high, sustained levels of prescription drug use over several decades of their lives, but these conditions are likely to characterize all subsequent cohorts going forward. It will therefore be important to gain a full understanding of how sustained use of high levels of prescription drugs over decades of people's lives impacts health and well-being over the long run.
- Acknowledgments
Research reported in this publication was supported by the National Institute on Aging of the National Institutes of Health under Award Number R03AG073081. The content is solely the responsibility of the author and does not necessarily represent the official views of the National Institutes of Health.
Some common examples include amoxicillin (anti-infective), used to treat bacterial infections like pneumonia; digoxin (cardiovascular agent), used to regulate heart rhythms; amlodipine (antihypertensive), used to treat high blood pressure; diazepam (e.g., brand name Valium) and alprazolam (Xanax), which are CNS agents used to treat anxiety, seizures, and panic disorders; naproxen (e.g., Aleve), a non-opioid analgesic, and oxycodone (e.g., OxyContin), an opioid analgesic, used for pain relief; warfarin (coagulation modifier), used to treat and prevent blood clots; omeprazole (e.g., Prilosec), a gastrointestinal agent used for heartburn; oxybutynin (e.g., Oxytrol), a genitourinary tract agent used for overactive bladder; contraceptives containing estrogen or progesterone and corticosteroids, examples of hormones; albuterol (respiratory agent), a bronchodilator used to treat asthma, bronchitis, and chronic obstructive pulmonary lung disease; sertraline (e.g., Zoloft), an antidepressant used for depression, posttraumatic stress disorder, and panic attacks; metformin (metabolic agent), used to treat type 2 diabetes; and atorvastatin (e.g., Lipitor), a statin used for high cholesterol.
This finding holds for the subset of key drugs (panel b of Figure 1 ). The age at which the majority of the population takes drugs is slightly older (at 45–49 for men and 25–29 for women) but still encompasses a substantial swathe of the life course.
Supplementary data
Data & figures.

Percentage of Americans taking (a) any prescription drugs by age and sex and (b) key prescription drugs by age, sex, race, and ethnicity in 2019. The key prescription drugs are antineoplastics, biologicals and immunologic agents, cardiovascular agents, CNS agents, coagulation modifiers, gastrointestinal agents, genitourinary tract agents, hormones and hormone modifiers, respiratory agents, psychotherapeutic agents, and metabolic agents. The dotted horizontal line indicates the point at which more than 50% of the population in that age group is taking prescription drugs. Source : Author's analysis of data from the MEPS, 2019.

Age-standardized percentage of U.S. men and women taking zero, one, two, three, four, and five or more key prescription drugs (a) for the total population in 1996–2019 and (b) by race and ethnicity in 2006–2019. The key prescription drugs are antineoplastics, biologicals and immunologic agents, cardiovascular agents, CNS agents, coagulation modifiers, gastrointestinal agents, genitourinary tract agents, hormones and hormone modifiers, respiratory agents, psychotherapeutic agents, and metabolic agents. Source : Author's analysis of data from the MEPS, 1996–2019.

Expected years taking zero, one, two, three, four, and five or more key prescription drugs at selected ages by sex for the total population in 1996 and 2019. The key prescription drugs are antineoplastics, biologicals and immunologic agents, cardiovascular agents, CNS agents, coagulation modifiers, gastrointestinal agents, genitourinary tract agents, hormones and hormone modifiers, respiratory agents, psychotherapeutic agents, and metabolic agents. Source : Author's analysis of data from the MEPS and the HMD, 1996 and 2019.

Expected years spent taking common classes of prescription drugs at age 0 by sex (a and b) for the total population between 1996 and 2019 and (c and d) by race and ethnicity between 2006 and 2019. Source : Author's analysis of data from the MEPS, the HMD, and the NCHS, 1996–2019.

Share of remaining life expectancy spent taking specific classes of prescription drugs at ages 0 and 65 for men and women in 1996 and 2019. Source : Author's analysis of data from the MEPS and the HMD, 1996 and 2019.
Expected years and percentage of remaining life expectancy taking key prescription drugs a for survivors to each age, by sex and year
Source: Data are from the author's calculations based on the MEPS and the HMD, 1996 and 2019.
Key prescription drugs are antineoplastics, biologicals and immunologic agents, cardiovascular agents, CNS agents, coagulation modifiers, gastrointestinal agents, genitourinary tract agents, hormones and hormone modifiers, respiratory agents, psychotherapeutic agents, and metabolic agents.
Change is calculated as expected years (or percentage of remaining life expectancy) taking prescription drugs in 2019 – expected years (or percentage of remaining life expectancy) taking prescription drugs in 1996.
Share of remaining life expectancy taking specific classes of prescription drugs at ages 0, 25, 45, 65, and 85 for men and women in 1996 and 2019: Percentages
Note: Main (nonindented) categories are mutually exclusive and exhaustive.

- Previous Issue
- Previous Article
- Next Article
Advertisement
Supplements
Citing articles via, email alerts, related articles, related topics, related book chapters, affiliations.
- About Demography
- Editorial Board
- For Authors
- Rights and Permissions Inquiry
- Online ISSN 1533-7790
- Print ISSN 0070-3370
- Copyright © 2024
- Duke University Press
- 905 W. Main St. Ste. 18-B
- Durham, NC 27701
- (888) 651-0122
- International
- +1 (919) 688-5134
- Information For
- Advertisers
- Book Authors
- Booksellers/Media
- Journal Authors/Editors
- Journal Subscribers
- Prospective Journals
- Licensing and Subsidiary Rights
- View Open Positions
- email Join our Mailing List
- catalog Current Catalog
- Accessibility
- Get Adobe Reader
This Feature Is Available To Subscribers Only
Sign In or Create an Account
Research Reports
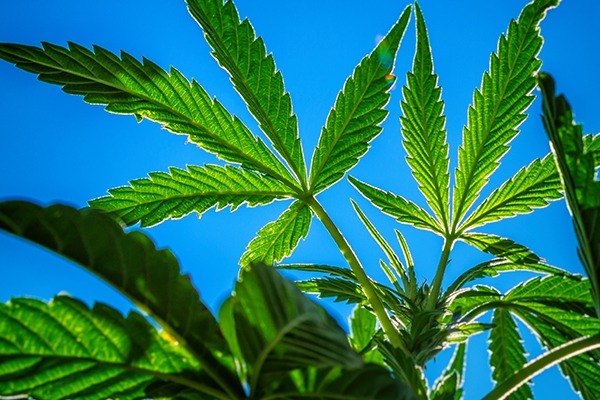
Cannabis (Marijuana) Research Report
Common comorbidities with substance use disorders research report.
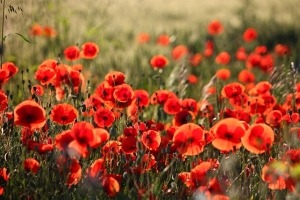
Heroin Research Report
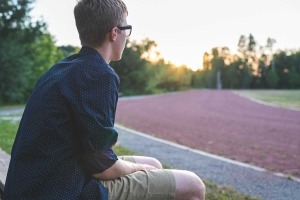
Inhalants Research Report
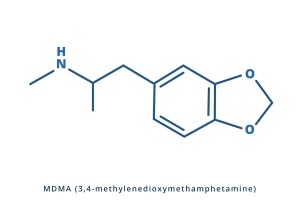
MDMA (Ecstasy) Abuse Research Report
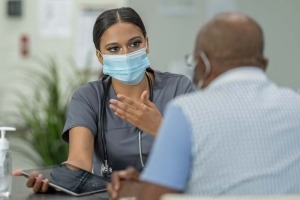
Medications to Treat Opioid Use Disorder Research Report
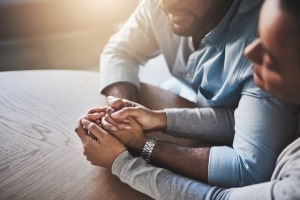
Methamphetamine Research Report
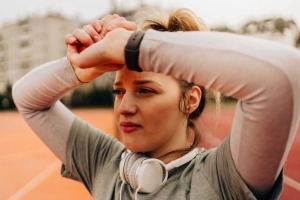
Misuse of Prescription Drugs Research Report
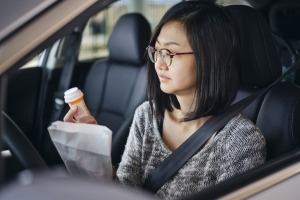
Prescription Opioids and Heroin Research Report
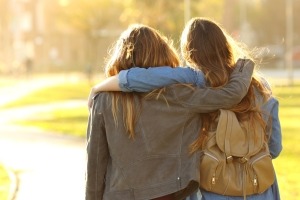
Substance Use in Women Research Report
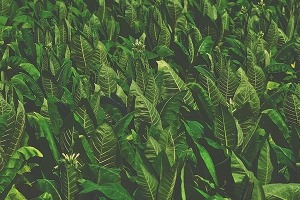
Tobacco, Nicotine, and E-Cigarettes Research Report
- Introduction
- Article Information
ADHD indicates attention-deficit/hyperactivity disorder.
eTable 1. School-Level and Individual-Level Correlates of Past-Month Nonmedical Use of Prescription Stimulants
eTable 2. School-Level and Individual-Level Correlates of Nonmedical Use of Prescription Stimulants (NUPS) Controlling for School-Level NUPS
eTable 3. School-Level and Individual-Level Correlates of Nonmedical Use of Prescription Stimulants Among Those Without Stimulant Therapy
eTable 4. School-Level and Individual-Level Correlates of Nonmedical Use of Prescription Stimulants With Continuous School-Level Correlates (With Additional Characteristics)
eTable 5. School-Level and Individual-Level Correlates of Nonmedical Use of Prescription Stimulants With Continuous School-Level Correlates
eTable 6. Bivariate Correlations Between Stimulant Therapy for ADHD and Nonmedical Use of Prescription Stimulants by Secondary School-Level Characteristics, 2005–2020
eTable 7. Demographics for School-Level Characteristics
eTable 8. Demographics for Individual-Level Characteristics
Data Sharing Statement
See More About
Sign up for emails based on your interests, select your interests.
Customize your JAMA Network experience by selecting one or more topics from the list below.
- Academic Medicine
- Acid Base, Electrolytes, Fluids
- Allergy and Clinical Immunology
- American Indian or Alaska Natives
- Anesthesiology
- Anticoagulation
- Art and Images in Psychiatry
- Artificial Intelligence
- Assisted Reproduction
- Bleeding and Transfusion
- Caring for the Critically Ill Patient
- Challenges in Clinical Electrocardiography
- Climate and Health
- Climate Change
- Clinical Challenge
- Clinical Decision Support
- Clinical Implications of Basic Neuroscience
- Clinical Pharmacy and Pharmacology
- Complementary and Alternative Medicine
- Consensus Statements
- Coronavirus (COVID-19)
- Critical Care Medicine
- Cultural Competency
- Dental Medicine
- Dermatology
- Diabetes and Endocrinology
- Diagnostic Test Interpretation
- Drug Development
- Electronic Health Records
- Emergency Medicine
- End of Life, Hospice, Palliative Care
- Environmental Health
- Equity, Diversity, and Inclusion
- Facial Plastic Surgery
- Gastroenterology and Hepatology
- Genetics and Genomics
- Genomics and Precision Health
- Global Health
- Guide to Statistics and Methods
- Hair Disorders
- Health Care Delivery Models
- Health Care Economics, Insurance, Payment
- Health Care Quality
- Health Care Reform
- Health Care Safety
- Health Care Workforce
- Health Disparities
- Health Inequities
- Health Policy
- Health Systems Science
- History of Medicine
- Hypertension
- Images in Neurology
- Implementation Science
- Infectious Diseases
- Innovations in Health Care Delivery
- JAMA Infographic
- Law and Medicine
- Leading Change
- Less is More
- LGBTQIA Medicine
- Lifestyle Behaviors
- Medical Coding
- Medical Devices and Equipment
- Medical Education
- Medical Education and Training
- Medical Journals and Publishing
- Mobile Health and Telemedicine
- Narrative Medicine
- Neuroscience and Psychiatry
- Notable Notes
- Nutrition, Obesity, Exercise
- Obstetrics and Gynecology
- Occupational Health
- Ophthalmology
- Orthopedics
- Otolaryngology
- Pain Medicine
- Palliative Care
- Pathology and Laboratory Medicine
- Patient Care
- Patient Information
- Performance Improvement
- Performance Measures
- Perioperative Care and Consultation
- Pharmacoeconomics
- Pharmacoepidemiology
- Pharmacogenetics
- Pharmacy and Clinical Pharmacology
- Physical Medicine and Rehabilitation
- Physical Therapy
- Physician Leadership
- Population Health
- Primary Care
- Professional Well-being
- Professionalism
- Psychiatry and Behavioral Health
- Public Health
- Pulmonary Medicine
- Regulatory Agencies
- Reproductive Health
- Research, Methods, Statistics
- Resuscitation
- Rheumatology
- Risk Management
- Scientific Discovery and the Future of Medicine
- Shared Decision Making and Communication
- Sleep Medicine
- Sports Medicine
- Stem Cell Transplantation
- Substance Use and Addiction Medicine
- Surgical Innovation
- Surgical Pearls
- Teachable Moment
- Technology and Finance
- The Art of JAMA
- The Arts and Medicine
- The Rational Clinical Examination
- Tobacco and e-Cigarettes
- Translational Medicine
- Trauma and Injury
- Treatment Adherence
- Ultrasonography
- Users' Guide to the Medical Literature
- Vaccination
- Venous Thromboembolism
- Veterans Health
- Women's Health
- Workflow and Process
- Wound Care, Infection, Healing
Get the latest research based on your areas of interest.
Others also liked.
- Download PDF
- X Facebook More LinkedIn
McCabe SE , Schulenberg JE , Wilens TE , Schepis TS , McCabe VV , Veliz PT. Prescription Stimulant Medical and Nonmedical Use Among US Secondary School Students, 2005 to 2020. JAMA Netw Open. 2023;6(4):e238707. doi:10.1001/jamanetworkopen.2023.8707
Manage citations:
© 2024
- Permissions
Prescription Stimulant Medical and Nonmedical Use Among US Secondary School Students, 2005 to 2020
- 1 Center for the Study of Drugs, Alcohol, Smoking and Health, School of Nursing, University of Michigan, Ann Arbor
- 2 Institute for Research on Women and Gender, University of Michigan, Ann Arbor
- 3 Institute for Healthcare Policy and Innovation, University of Michigan, Ann Arbor
- 4 Injury Prevention Center, University of Michigan, Ann Arbor
- 5 Institute for Social Research, University of Michigan, Ann Arbor
- 6 Department of Psychology, University of Michigan, Ann Arbor
- 7 Department of Psychiatry, School of Medicine, Harvard University, Boston, Massachusetts
- 8 Department of Psychology, Texas State University, San Marcos
- 9 Department of Psychiatry, Medical School, University of Michigan, Ann Arbor
Question What is the association between school-level stimulant therapy for attention-deficit/hyperactivity disorder (ADHD) and prescription stimulant nonmedical use?
Findings In this cross-sectional study of 231 141 students in 3284 secondary schools, school-level past-year prescription stimulant nonmedical use ranged from 0% to more than 25% across US schools. Students attending schools with the highest rates of stimulant therapy for ADHD had 36% increased odds of nonmedical prescription stimulant use compared with students attending schools with the lowest rates.
Meaning This study’s results suggest potential targets for strategies to reduce school-level prescription stimulant nonmedical use.
Importance Recent information on the prevalence of prescription stimulant therapy for attention-deficit/hyperactivity disorder (ADHD) and nonmedical use of prescription stimulants (NUPS) at the school-level among US secondary school students is limited.
Objective To investigate the school-level prevalence of and association between stimulant therapy for ADHD and NUPS among US secondary school students.
Design, Setting, and Participants This cross-sectional study used survey data collected between 2005 and 2020 as part of the Monitoring the Future study (data collected annually via self-administered survey in schools from independent cohorts). Participants were from a nationally representative sample of 3284 US secondary schools. The mean (SD) response rates were 89.5% (1.3%) for 8th-grade students, 87.4% (1.1%) for 10th-grade students, and 81.5% (1.8%) for 12th-grade students. Statistical analysis was performed from July to September 2022.
Main Outcome and Measure Past-year NUPS.
Results The 3284 schools contained 231 141 US 8th-, 10th-, and 12th-grade students (111 864 [50.8%, weighted] female; 27 234 [11.8%, weighted] Black, 37 400 [16.2%, weighted] Hispanic, 122 661 [53.1%, weighted] White, 43 846 [19.0%, weighted] other race and ethnicity). Across US secondary schools, the past-year prevalence of NUPS ranged from 0% to more than 25%. The adjusted odds of an individual engaging in past-year NUPS were higher at secondary schools with higher proportions of students who reported stimulant therapy for ADHD, after controlling for other individual-level and school-level covariates. Students attending schools with the highest rates of prescription stimulant therapy for ADHD had approximately 36% increased odds of past-year NUPS compared with students attending schools with no medical use of prescription stimulants (adjusted odds ratio, 1.36; 95% CI, 1.20-1.55). Other significant school-level risk factors included schools in more recent cohorts (2015-2020), schools with higher proportions of parents with higher levels of education, schools located in non-Northeastern regions, schools located in suburban areas, schools with higher proportion of White students, and schools with medium levels of binge drinking.
Conclusions and Relevance In this cross-sectional study of US secondary schools, the prevalence of past-year NUPS varied widely, highlighting the need for schools to assess their own students rather than relying solely on regional, state, or national results. The study offered new evidence of an association between a greater proportion of the student body that uses stimulant therapy and a greater risk for NUPS in schools. The association between greater school-level stimulant therapy for ADHD and other school-level risk factors suggests valuable targets for monitoring, risk-reduction strategies, and preventive efforts to reduce NUPS.
The diagnosis of attention-deficit/hyperactivity disorder (ADHD) and prescribing of stimulant therapy for ADHD have increased substantially in the US over the past 2 decades with recent estimates indicating one in every nine 12th graders report lifetime stimulant therapy for ADHD. 1 - 6 Although prescription stimulants are efficacious when used appropriately, there is growing concern regarding the acute and long-term adverse health effects (eg, cardiovascular events, depressed mood, overdoses, psychosis, seizures, and stimulant use disorder) associated with nonmedical use of prescription stimulants (NUPS). 7 - 11 The leading medication source for NUPS by US adolescents remains same-age peers, 10 , 12 - 15 and a regional Canadian study 16 found that the number of secondary school students prescribed stimulants who divert the medication in the classroom is linked to the prevalence of NUPS in that classroom. Thus, the challenge of reducing NUPS while providing necessary stimulant therapy continues to perplex the public health and clinical fields. 17 - 21
Despite a call for more attention to school-level contextual influences in adolescent substance use research, 22 - 26 little is known about whether school-level stimulant therapy for ADHD is associated with NUPS among US secondary school students. Previous studies have largely neglected school-level factors associated with NUPS among US secondary school students, including school size, school geographical location, school-level racial composition, school-level rates of substance use (eg, binge drinking), and school-level stimulant therapy for ADHD. Research addressing these gaps is needed to identify important sociocontextual influences and intervention targets for NUPS.
To address existing knowledge gaps, this study used data from the Monitoring the Future (MTF) study, a multicohort survey of US nationally representative samples of secondary school students (8th, 10th, and 12th grade) to improve understanding of school-level prevalence and risk factors of NUPS. The main objectives of this study were to examine school-level prevalence rates of NUPS within a large national sample of US secondary school students and determine the school-level characteristics (eg, percentage of students in school prescribed stimulant therapy) and individual-level characteristics (eg, demographic characteristics) associated with NUPS.
The MTF study is an annual self-administered survey conducted with nationally representative samples of 8th-, 10th-, and 12th-grade students in US secondary schools. 15 The 12th-grade sample has been collected since 1975, and the 8th- and 10th-grade samples have been collected since 1991. 15 Approximately 350 students in each school (for the targeted grade) are selected to participate (either by randomly sampling entire classrooms or by some other unbiased, random method). In schools with smaller numbers of students, the usual procedure is to select all students (for the targeted grade) for participation. The mean (SD) response rates were 89.5% (1.3%) for 8th graders, 87.4% (1.1%) for 10th graders, and 81.5% (1.8%) for 12th graders. The MTF project design, protocol, and sampling methods are described in greater detail elsewhere. 15
This cross-sectional study used the 2005 to 2020 MTF samples; questions regarding stimulant therapy for ADHD were introduced in 2005. The 2005 to 2020 samples were composed of 231 141 students in 3284 unique public and private schools. The breakdown of schools was 1232 8th-grade schools, 1009 10th-grade schools, and 1043 12th-grade schools. The analytic sample of students consisted of 80 746 8th graders, 78 361 10th graders, and 72 034 12th graders. Schools typically participated for 2 consecutive years in the MTF sample, with 740 participating once, 2462 participating twice, and 82 participating 3 or more times (because they were selected more than once across the 16-year period), yielding 5963 observations at the school-level. There was a mean (SD) of 170.2 (149.7) students per 8th grade school, 249.7 (200.7) students per 10th grade school, and 212.8 (179.3) students per 12th grade school. The questions assessing stimulant therapy for ADHD were asked on only 1 of 4 randomly assigned survey forms for 8th and 10th graders, and 2 of 6 randomly assigned survey forms for 12th graders. Across the years, paper or tablet self-administered surveys have been administered in schools. Informed consent (active or passive, per school policy) was obtained from parents of students younger than 18 years and from students aged 18 years or older, and adolescent assent was obtained. The University of Michigan institutional review board deemed this study exempt because data were recorded at another time by the original investigators and in such a manner that respondents cannot be identified. This study followed the Strengthening the Reporting of Observational Studies in Epidemiology ( STROBE ) reporting guideline.
Current and previous medical use of prescription stimulants was measured at the individual level with an item that assesses medical use of stimulant medications to treat ADHD. Respondents were told stimulant medications are prescribed for people with ADHD who have problems concentrating on one task at a time, or with being too active or too disruptive (hyperactive), or both. Respondents were given a list of generic and brand name stimulant medications (eg, amphetamine, methylphenidate, Ritalin, Adderall, Concerta, Metadate, Dexedrine, Focalin, Vyvanse). Respondents were instructed not to count drugs that are not stimulant-type medications such as Strattera, Wellbutrin, Provigil, Tenex, Intuniv, or Catapres. Respondents were asked if they had ever taken stimulant medications for ADHD under a physician’s or health professional’s supervision. At the individual level, the variable was treated as a 3-category variable: (1) no; (2) yes, in the past, but not now; (3) yes, current use. At the school level, both response options for in the past and current use were combined and aggregated to the school level to reflect the percentage of the study body who used prescription stimulants for ADHD. For sensitivity analysis, we also examined current use at the school level.
Individual-level sociodemographic characteristics included several self-reported measures at the student level, such as sex, race and ethnicity, parental education, and grade point average. Race and ethnicity options were defined by the MTF study team and included Hispanic, non-Hispanic Black, non-Hispanic White, and other (which included American Indian, Asian, and those who selected multiple races and ethnicities). Race and ethnicity were assessed and included based on the well-established race differences in stimulant therapy for ADHD and NUPS. Individual-level substance use included binge drinking, cigarette smoking, and marijuana use.
School-level characteristics were assessed with a set of questions aimed at both the school level and aggregated from measures at the student level consistent with previous work. 25 - 28 The school-level characteristics included school type (public or private), urbanicity (rural, suburban, or urban), US Census region (Northeast, Midwest, South, or West), grade level sampled at school (8th, 10th, or 12th), school size, percentage of the student body that was female, percentage of the student body that had at least 1 parent with a college degree or higher, percentage of the student body that was White, and cohort year (2005 to 2009, 2010 to 2014, 2015 to 2020).
School-level substance use was estimated from student-level variables. It included the percentage of the student body that engaged in past-2-week binge drinking, percentage of the student body that reported past-month cigarette smoking, and the percentage of the student body that reported past-month marijuana use.
NUPS was measured by asking respondents the following item: “On how many occasions (if any) have you taken amphetamines or other prescription stimulant drugs on your own—that is, without a doctor telling you to take them... in your lifetime?...during the last 12 months?...during the last 30 days?” Respondents were given several details about prescription stimulants and informed these medications are prescribed by physicians or health professionals for people who have ADHD and have trouble paying attention, are hyperactive, or both.
All analyses were design-based using the svyset command in Stata 17.0 (StataCorp), fully accounting for the MTF sampling weights and complex sampling design when estimating parameters for the target MTF individual-level population. The school was treated as the primary sampling unit and was used to correct for the clustering of students within schools. First, we performed descriptive statistics assessing the associations between key school-level characteristics ( Table 1 ) and both past-year NUPS and current and previous medical use of prescription stimulants at the school level. School-level rates of NUPS and medical use were treated as continuous measures for this descriptive analysis. The school-level bivariate analysis did not use the individual-level weights provided by the MTF given that these weights only reflected the 8th-, 10th-, and 12th-grade population in the US and not schools (the MTF does not provide school-level weights). Accordingly, the MTF weights were only used at the individual level.
Second, multivariable logistic regression models were fitted to estimate the association between past-year individual-level NUPS and the individual- and school-level characteristics. Accordingly, 3 sets of models were estimated: one assessed the association between individual-level NUPS and individual-level characteristics, a second assessed the association between individual-level NUPS and school-level characteristics, and a third included both the individual- and school-level characteristics to estimate individual-level NUPS. Finally, an additional set of models was estimated to assess the association between past-year individual-level NUPS and current and previous use of stimulants to treat ADHD at both the individual level and school level. No data were missing for school-level characteristics, and items missing rates for individual-level characteristics ranged from 0.0% for race and ethnicity to 9.4% for parental education; all analyses used listwise deletion. Models using full information maximum likelihood estimation found similar results.
Statistical analysis was performed from July to September 2022. Analyses included 95% CIs to determine statistical significance at a .05 α level or lower.
As shown in the Figure , there was wide variation across 3284 US secondary schools in the prevalence rates of school-level medical use of prescription stimulants (231 141 US 8th-, 10th-, and 12th-grade students; 111 864 [50.8%, weighted] female; 27 234 [11.8%, weighted] Black, 37 400 [16.2%, weighted] Hispanic, 122 661 [53.1%, weighted] White, 43 846 [19.0%, weighted] other race and ethnicity). The percentage of the student body that reported medical use of prescription stimulants ranged from 1266 schools that had 0% to 116 schools that had 25% or higher. The mean (SD) of medical use of prescription stimulants was 7.9% (7.1%) at the school level, and the mean (SE) at the individual level was 8.0% (0.001%). Additional school-level information: mean (SD) percentage female: 50.9% (0.1%); mean (SD) percentage White: 55.8% (0.3%); mean (SD) percentage of the student body that has at least 1 parent with a college degree or higher: 56.0% (0.2%); mean (SD) percentage with low grades: 19.8% (0.1%); mean (SD) percentage binge drinking: 13.4% (0.1%); mean (SD) percentage cigarette smoking: 10.2% (0.1%); mean (SD) percentage marijuana use: 13.9% (0.1%); mean (SD) percentage nonmedical use of prescription stimulants: 5.7% (0.1%); mean (SD) percentage stimulant therapy for ADHD: 7.9% (0.1%).
As depicted in the Figure , similar to medical use, there was a wide variation across US secondary schools in the past-year prevalence rates of school-level NUPS. Across schools, the percentage of the student body that engaged in past-year NUPS ranged from 1706 schools that had 0% to 58 schools with 25% or higher, including a maximum of 75%. The mean (SD) of past-year NUPS was 5.7% (6.1%) at the school level and mean (SE) of 6.0% (0.001%) at the individual level.
As illustrated in Table 2 , the school-level prevalence of past-year NUPS differed significantly as a function of each school-level characteristic. Most notably, schools with a high percentage of the student body using stimulant therapy for ADHD had a higher percentage of the student body engaged in past-year NUPS when compared with schools where none of the students reported medical use of prescription stimulants for ADHD (7.6% [95% CI, 7.3%-8.0%] vs 3.6% [95% CI, 3.2%-3.9%], respectively).
As illustrated in Table 3 , multivariable regression analyses revealed that all individual-level characteristics were associated with past-year NUPS when adjusting for both individual- and school-level factors (ie, model 3). For instance, adolescents who engaged in past 30-day marijuana use had approximately 4 times greater odds of past-year NUPS when compared with adolescents who did not use marijuana (adjusted odds ratio [aOR], 3.93 [95% CI, 3.69-4.19]). With respect to the school-level characteristics in the fully adjusted model (ie, both individual- and school-level factors; model 3), several characteristics increased the odds of NUPS at the individual level: suburban school location (vs urban), Midwest, South, and West US census region (vs Northeast), higher proportions of 1 or more parents with a college degree, greater percentage of the student body that is White, and higher prevalence of student binge drinking.
Moreover, in Table 3 (models 2 and 3), 12th graders had lower odds of past-year NUPS when compared with 8th graders (the opposite was true in the bivariate analyses in Table 2 ). Finally, of central importance and similar to what was found in the bivariate analyses, adolescents that attended schools with a higher percentage of the student body who indicated using stimulant therapy for ADHD had higher odds of past-year NUPS. For instance, the odds of an adolescent who attended a school with a high percentage of the student body who indicated using stimulant therapy for ADHD had approximately one-and-a-half times greater odds of NUPS when compared with adolescents who attended a school where no students used stimulant therapy for ADHD (aOR, 1.64 [95% CI, 1.45-1.86]). Supplemental analyses found this association was significant for past-month NUPS (see eTable 1 in Supplement 1 ) and after controlling for the percentage of students engaging in NUPS at the school-level (see eTable 2 in Supplement 1 ). Table 4 shows that both the individual-level and school-level factors assessing stimulant therapy for ADHD were associated with past-year NUPS. For instance, model 2 in Table 4 shows that adolescents who indicated either current (aOR, 2.34 [95% CI, 2.09-2.61]) or past (aOR, 2.44 [95% CI, 2.22-2.67]) stimulant therapy for ADHD had approximately two-and-one-half times greater odds of past-year NUPS when compared with their peers who never used stimulants to treat ADHD. Moreover, adolescents who attended schools with a higher percentage of the student body who used stimulant therapy for ADHD had higher odds of NUPS when also controlling for medical use at the individual level. For instance, the adjusted odds of an adolescent who attended a school with a high percentage of the student body who reported stimulant therapy for ADHD had approximately 36% greater odds of NUPS when compared with adolescents who attended a school where no students used stimulant therapy for ADHD (aOR, 1.36 [95% CI, 1.20-1.55]). Stratified analyses showed that these findings remained robust for students who did not currently or had not previously used stimulant therapy for ADHD (aOR, 1.33 [95% CI, 1.16-1.52]) (eTable 3 in Supplement 1 ). Finally, sensitivity analyses found similar results for both current and previous use as well as for continuous school-level factors (see eTables 4, 5, 6, 7, and 8 in Supplement 1 ).
To our knowledge, this is the first national study to examine school-level prevalence and risk factors associated with NUPS among US secondary school students. Past-year NUPS prevalence across US secondary schools varied considerably, with a range of 0% to 25% or higher of the student body engaging in NUPS. The present study extends previous research that found similar variation in past-year NUPS prevalence across US colleges (0%-25%). 29 The wide variation found between US secondary schools in the present study serves as notice that individual schools are strongly encouraged to assess their own student body to guide their prevention efforts. Of central importance, based on controlled analyses, the present study found that school-level NUPS was varied significantly with school-level stimulant therapy for ADHD, after adjusting for individual- and school-level risk factors including other school-level substance use.
This is, to our knowledge, the first study to provide detailed national estimates of the association between school-level stimulant therapy for ADHD as an environmental risk factor and school-level NUPS. School-level stimulant therapy had a significant association with individual- and school-level NUPS, indicating a valuable potential target for monitoring, risk-reduction strategies, and prevention. This is especially important given that peers are a leading source for NUPS among adolescents. 10 , 14 The study is consistent with previous evidence from a regional Canadian study that found significant associations between the number of students receiving prescription stimulants who share medications in a secondary school classroom and NUPS prevalence in each classroom. 16
There is growing evidence that the majority of NUPS among older adolescents is primarily motivated by a desire to enhance academics and/or cognition and most often involves obtaining stimulants from friends at the same school. 7 , 12 , 16 , 30 - 33 NUPS is associated with an increased risk of substance use disorder, neuropsychological dysfunction, polysubstance use, depressed mood, and lower graduation rates. 11 , 34 - 38 The findings from this study indicate a need for more research to examine whether closely monitoring medical availability of prescription stimulants and reducing stimulant diversion among same-age peers could have a significant influence on reducing NUPS. However, exerting medication management control and monitoring measures at both the individual- and school-level becomes more challenging after high school when adolescents often become more responsible for their own medication management. Adolescents who are prescribed stimulant therapy for ADHD should be educated and prepared that nearly a quarter (24%) will be approached to divert their stimulant medications by their peers before the completion of high school (and more than half [54%] during college). 37 , 38
We sought to better understand what was linked with NUPS with a multilevel approach to examine the association between individual- and school-level risk factors and NUPS during adolescence. In these controlled analyses, it was found that the adjusted odds of past-year NUPS were higher at schools in more recent cohorts (2015 to 2020), schools located in non-Northeastern regions, schools located in suburban areas, schools that had proportionally more White students, and schools that had higher proportions of parents with more education. The higher odds of NUPS in more recent cohorts could be driven by recent increases in stimulant therapy. Clearly, the school context, including the attitudes and behaviors of fellow students regarding the stimulant therapy for ADHD, NUPS, and other substances in general, sets the stage for schoolwide NUPS. Secondary schools vary in terms of how much they are “academic-focused” (ie, high academics, low substance use, and high social integration) and “party-focused” (ie, low academics, high substance use, and low social integration). 39 The present study found schools that were high on NUPS were also higher on other substance use; nonetheless, when school-level other substance use was controlled for, the association between stimulant therapy for ADHD and NUPS remained strong. We also controlled for numerous individual- and school-level sociodemographics, and together, the association between stimulant therapy and NUPS remained significant.
This study had strengths and limitations. The main strength of the present study is the nationally representative sample of 8th-, 10th-, and 12th-grade students attending US public and private schools with large enough samples to derive school-level estimates of stimulant therapy for ADHD and NUPS. It is also worth noting that large national studies, such as MTF, also carry limitations, such as not including students who were home-schooled, dropped out, or were absent on the day of data collection which can all affect estimates. Although the MTF study measures have been reported to be reliable and valid, there is some evidence that adolescents may misclassify or underreport sensitive substance use behaviors. 15 , 40 - 42
This cross-sectional study found that there is a wide variation in the prevalence of NUPS between US secondary schools (0% to more than 25%), highlighting the need for individual schools to assess their own student body rather than relying solely on regional, state, or national results. To our knowledge, this is the first national study to identify school-level risk factors associated with NUPS among US secondary school students. These findings suggest that school-level stimulant therapy for ADHD and other school-level risk factors were significantly associated with NUPS and should be accounted for in risk-reduction strategies and prevention efforts.
Accepted for Publication: March 4, 2023.
Published: April 18, 2023. doi:10.1001/jamanetworkopen.2023.8707
Open Access: This is an open access article distributed under the terms of the CC-BY License . © 2023 McCabe SE et al. JAMA Network Open .
Corresponding Author: Sean Esteban McCabe, PhD, Center for the Study of Drugs, Alcohol, Smoking and Health, School of Nursing, University of Michigan, 400 N Ingalls St, Ann Arbor, MI 48109 ( [email protected] ).
Author Contributions: Drs S.E. McCabe and Veliz had full access to all of the data in the study and take responsibility for the integrity of the data and the accuracy of the data analysis.
Concept and design: S.E. McCabe, Schepis, V.V. McCabe, Veliz.
Acquisition, analysis, or interpretation of data: S.E. McCabe, Schulenberg, Wilens, V.V. McCabe, Veliz.
Drafting of the manuscript: S.E. McCabe, Wilens, Schepis, Veliz.
Critical revision of the manuscript for important intellectual content: S.E. McCabe, Schulenberg, Schepis, V.V. McCabe, Veliz.
Statistical analysis: S.E. McCabe, Wilens, Veliz.
Obtained funding: S.E. McCabe, Schulenberg, Schepis.
Administrative, technical, or material support: V.V. McCabe.
Supervision: S.E. McCabe.
Conflict of Interest Disclosures: Dr Wilens reported receiving personal fees from Massachusetts General Hospital (employee), Gavin Foundation (clinical consulting), Bay Cove Human Services (clinical consulting), US Minor/Major League Baseball (clinical consulting); other from White Rhino / 3D (consultant, shared intellectual property); and nonfinancial support from Elsevier Psychiatric Clinics of North America (ADHD coeditor, no personal income) outside the submitted work; in addition, Dr Wilens had a licensing agreement with Ironshore with royalties paid (licensing agreement), royalties paid from Guilford Press and Cambridge University Press (coedited textbook). No other disclosures were reported.
Funding/Support: The development of this article was supported by a research award 75F40121C00148 from the US Food and Drug Administration and research grants R01DA001411, R01DA031160, R01DA036541, and R01DA043691 from the National Institute on Drug Abuse, National Institutes of Health.
Role of the Funder/Sponsor: The US Food and Drug Administration reviewed this study. The funders had no role in the design and conduct of the study; collection, management, analysis, and interpretation of the data; preparation, or approval of the manuscript; and decision to submit the manuscript for publication.
Disclaimer: The content is solely the responsibility of the authors and does not necessarily represent the official views of the US Food and Drug Administration, National Institute on Drug Abuse, the National Institutes of Health, or the US Government.
Data Sharing Statement: See Supplement 2 .
Additional Contributions: The authors would like to thank Caroline J. Huang, PhD; Jana McAninch, MD, MPH, MS; Rose Radin, PhD; and Zimri S. Yaseen, MD, from the US Food and Drug Administration for their input on an earlier version of this article. The authors would also like to thank Ms Kathryn Lundquist, ABA, from the Center for the Study of Drugs, Alcohol, Smoking and Health, University of Michigan School of Nursing, for her assistance in editing and formatting the article. All contributors provided written permission to be included in this section. In addition, the authors thank the respondents, school personnel, and research staff for their participation in the study. No one received compensation for their efforts regarding this article.
Additional Information: Coauthor John E. Schulenberg, PhD, died unexpectedly during the revision of this manuscript. He was a loving father, an influential scientist, a generous mentor, and a wonderful friend. He is deeply missed, and the other coauthors dedicate this article to his memory.
- Register for email alerts with links to free full-text articles
- Access PDFs of free articles
- Manage your interests
- Save searches and receive search alerts
Misuse of Prescription Drugs Research Report
About this resource:.
Source: National Institute on Drug Abuse
The last reviewed date indicates when the evidence for this resource last underwent a comprehensive review.
Workgroups: Older Adults Workgroup , Substance Use Workgroup
This report defines misuse of prescription drugs and describes the extent of prescription drug misuse in the United States. It also provides information about the safety of using prescription drugs in combination with other medicines, describes ways to prevent and treat prescription drug misuse and addiction, and lists resources that provide more information. In addition, the report includes information about prescription drug misuse in specific populations, like adolescents and older adults.
Objectives related to this resource (2)
Suggested citation.
National Institutes of Health, National Institute on Drug Abuse. (2020). Misuse of Prescription Drugs Research Report. Retrieved from https://www.drugabuse.gov/publications/research-reports/misuse-prescription-drugs
The Office of Disease Prevention and Health Promotion (ODPHP) cannot attest to the accuracy of a non-federal website.
Linking to a non-federal website does not constitute an endorsement by ODPHP or any of its employees of the sponsors or the information and products presented on the website.
You will be subject to the destination website's privacy policy when you follow the link.
This paper is in the following e-collection/theme issue:
Published on 16.4.2024 in Vol 26 (2024)
Adverse Event Signal Detection Using Patients’ Concerns in Pharmaceutical Care Records: Evaluation of Deep Learning Models
Authors of this article:

Original Paper
- Satoshi Nishioka 1 , PhD ;
- Satoshi Watabe 1 , BSc ;
- Yuki Yanagisawa 1 , PhD ;
- Kyoko Sayama 1 , MSc ;
- Hayato Kizaki 1 , MSc ;
- Shungo Imai 1 , PhD ;
- Mitsuhiro Someya 2 , BSc ;
- Ryoo Taniguchi 2 , PhD ;
- Shuntaro Yada 3 , PhD ;
- Eiji Aramaki 3 , PhD ;
- Satoko Hori 1 , PhD
1 Division of Drug Informatics, Keio University Faculty of Pharmacy, Tokyo, Japan
2 Nakajima Pharmacy, Hokkaido, Japan
3 Nara Institute of Science and Technology, Nara, Japan
Corresponding Author:
Satoko Hori, PhD
Division of Drug Informatics
Keio University Faculty of Pharmacy
1-5-30 Shibakoen
Tokyo, 105-8512
Phone: 81 3 5400 2650
Email: [email protected]
Background: Early detection of adverse events and their management are crucial to improving anticancer treatment outcomes, and listening to patients’ subjective opinions (patients’ voices) can make a major contribution to improving safety management. Recent progress in deep learning technologies has enabled various new approaches for the evaluation of safety-related events based on patient-generated text data, but few studies have focused on the improvement of real-time safety monitoring for individual patients. In addition, no study has yet been performed to validate deep learning models for screening patients’ narratives for clinically important adverse event signals that require medical intervention. In our previous work, novel deep learning models have been developed to detect adverse event signals for hand-foot syndrome or adverse events limiting patients’ daily lives from the authored narratives of patients with cancer, aiming ultimately to use them as safety monitoring support tools for individual patients.
Objective: This study was designed to evaluate whether our deep learning models can screen clinically important adverse event signals that require intervention by health care professionals. The applicability of our deep learning models to data on patients’ concerns at pharmacies was also assessed.
Methods: Pharmaceutical care records at community pharmacies were used for the evaluation of our deep learning models. The records followed the SOAP format, consisting of subjective (S), objective (O), assessment (A), and plan (P) columns. Because of the unique combination of patients’ concerns in the S column and the professional records of the pharmacists, this was considered a suitable data for the present purpose. Our deep learning models were applied to the S records of patients with cancer, and the extracted adverse event signals were assessed in relation to medical actions and prescribed drugs.
Results: From 30,784 S records of 2479 patients with at least 1 prescription of anticancer drugs, our deep learning models extracted true adverse event signals with more than 80% accuracy for both hand-foot syndrome (n=152, 91%) and adverse events limiting patients’ daily lives (n=157, 80.1%). The deep learning models were also able to screen adverse event signals that require medical intervention by health care providers. The extracted adverse event signals could reflect the side effects of anticancer drugs used by the patients based on analysis of prescribed anticancer drugs. “Pain or numbness” (n=57, 36.3%), “fever” (n=46, 29.3%), and “nausea” (n=40, 25.5%) were common symptoms out of the true adverse event signals identified by the model for adverse events limiting patients’ daily lives.
Conclusions: Our deep learning models were able to screen clinically important adverse event signals that require intervention for symptoms. It was also confirmed that these deep learning models could be applied to patients’ subjective information recorded in pharmaceutical care records accumulated during pharmacists’ daily work.
Introduction
Increasing numbers of people are expected to develop cancers in our aging society [ 1 - 3 ]. Thus, there is increasing interest in how to detect and manage the side effects of anticancer therapies in order to improve treatment regimens and patients’ quality of life [ 4 - 8 ]. The primary approaches for side effect management are “early signal detection and early intervention” [ 9 - 11 ]. Thus, more efficient approaches for this purpose are needed.
It has been recognized that patients’ voices concerning adverse events represent an important source of information. Several studies have indicated that the number, severity, and time of occurrence of adverse events might be underevaluated by physicians [ 12 - 15 ]. Thus, patient-reported outcomes (PROs) have recently received more attention in the drug evaluation process, reflecting patients’ real voices. Various kinds of PRO measures have been developed and investigated in different disease populations [ 16 , 17 ]. Health care authorities have also encouraged the pharmaceutical industry to use PROs for drug evaluation [ 18 , 19 ], and it is becoming more common to take PRO assessment results into consideration for drug marketing approval [ 20 , 21 ]. Similar trends can be seen in the clinical management of individual patients. Thus, health care professionals have an interest in understanding how to appropriately gather patients’ concerns in order to improve safety management and clinical decisions [ 22 - 24 ].
The applications of deep learning for natural language processing have expanded dramatically in recent years [ 25 ]. Since the development of a high-performance deep learning model in 2018 [ 26 ], attempts to apply cutting-edge deep learning models to various kinds of patient-generated text data for the evaluation of safety events or the analysis of unscalable subjective information from patients have been accelerating [ 27 - 31 ]. Most studies have been conducted to use patients’ narrative data for pharmacovigilance [ 27 , 32 - 35 ], while few have been aimed at improvement of real-time safety monitoring for individual patients. In addition, there have been some studies on adverse event severity grading based on health care records [ 36 - 39 ], but none has yet aimed to extract clinically important adverse event signals that require medical intervention from patients’ narratives. It is important to know whether deep learning models could contribute to the detection of such important adverse event signals from concern texts generated by individual patients.
To address this question, we have developed deep learning models to detect adverse event signals from individual patients with cancer based on patients’ blog articles in online communities, following other types of natural language processing–related previous work [ 40 , 41 ]. One deep learning model focused on the specific symptom of hand-foot syndrome (HFS), which is one of the typical side effects of anticancer treatments [ 42 ], and another focused on a broad range of adverse events that impact patients’ activities of daily living [ 43 ]. We showed that our models can provide good performance scores in targeting adverse event signals. However, the evaluation relied on patients’ narratives from the patients’ blog data used for deep learning model training, so further evaluation is needed to ensure the validity and applicability of the models to other texts regarding patients’ concerns. In addition, the blog data source did not contain medical information, so it was not feasible to assess whether the models could contribute to the extraction of clinically important adverse event signals.
To address these challenges, we focused on pharmaceutical care records written by pharmacists at community pharmacies. The gold standard format for pharmaceutical care records in Japan is the SOAP (subjective, objective, assessment, plan)-based document that follows the “problem-oriented system” concept proposed by Weed [ 44 ] in 1968. Pharmacists track patients’ subjective concerns in the S column, provide objective information or observations in the O column, give their assessment from the pharmacist perspective in the A column, and suggest a plan for moving forward in the P column [ 45 , 46 ]. We considered that SOAP-based pharmaceutical care records could be a unique data source suitable for further evaluation of our deep learning models because they contain both patients’ concerns and professional health care records by pharmacists, including the medication prescription history with time stamps. Therefore, this study was designed to assess whether our deep learning models could extract clinically important adverse event signals that require intervention by medical professionals from these records. We also aimed to evaluate the characteristics of the models when applied to patients’ subjective information noted in the pharmaceutical care records, as there have been only a few studies on the application of deep learning models to patients’ concerns recorded during pharmacists’ daily work [ 47 - 49 ].
Here, we report the results of applying our deep learning models to patients’ concern text data in pharmaceutical care records, focusing on patients receiving anticancer treatment.
Data Source
The original data source was 2,276,494 pharmaceutical care records for 303,179 patients, created from April 2020 to December 2021 at community pharmacies belonging to the Nakajima Pharmacy Group in Japan [ 50 ]. To focus on patients with cancer, records of patients with at least 1 prescription for an anticancer drug were retrieved by sorting individual drug codes (YJ codes) used in Japan (YJ codes starting with 42 refer to anticancer drugs). Records in the S column (ie, S records) were collected from the patients with cancer as the text data of patients’ concerns for deep learning model analysis.
Deep Learning Models
The deep learning models used for this research were those that we constructed based on patients’ narratives in blog articles posted in an online community and that showed the best performance score in each task in our previous work (ie, a Bidirectional Encoder Representations From Transformers [BERT]–based model for HFS signal extraction [ 42 ] and a T5-based model for adverse event signal extraction [ 43 ]). BERT [ 26 ] and T5 [ 51 ] both belong to a type of deep learning model that has recently shown high performance in several studies [ 29 , 52 ]. Hereafter, we refer to the deep learning model for HFS signals as the HFS model, the model for any adverse event signals as All AE (ie, all or any adverse events) model, and the model for adverse event signals limited to patients’ activities of daily living as the AE-L (adverse events limiting patients’ daily lives) model. It was also confirmed that these deep learning models showed similar or higher performance scores for the HFS, All AE, or AE-L identification tasks using 1000 S records randomly extracted from the data source of this study compared to the values obtained in our previous work [ 42 , 43 ] (the performance scores of sentence-level tasks from our previous work are comparable, as the mean number of words in the sentences in the data source in our previous work was 32.7 [SD 33.9], which is close to that of the S records used in this study, 38.8 [SD 29.4]). The method and results of the performance-level check are described in detail in Multimedia Appendix 1 [ 42 , 43 ]. We applied the deep learning models to all text data in this study without any adjustment in setting parameters from those used in constructing them based on patient-authored texts in our previous work [ 42 , 43 ].
Evaluation of Extracted S Records by the Deep Learning Models
In this study, we focused on the evaluation of S records that our deep learning models extracted as HFS or AE-L positive. Each positive S record was assessed as if it was a true adverse event signal, a sort of adverse event symptom, whether or not an intervention was made by health care professionals. We also investigated the kind of anticancer treatment prescription in connection with each adverse event signal identified in S records.
To assess whether an extracted positive S record was a true adverse event signal, we used the same annotation guidelines as in our previous work [ 43 ]. In brief, each S record was treated as an “adverse event signal” if any untoward medical occurrence happened to the patient, regardless of the cause. For the AE-L model only, if a positive S record was confirmed as an adverse event signal, it was further categorized into 1 or more of the following adverse event symptoms: “fatigue,” “nausea,” “vomiting,” “diarrhea,” “constipation,” “appetite loss,” “pain or numbness,” “rash or itchy,” “hair loss,” “menstrual irregularity,” “fever,” “taste disorder,” “dizziness,” “sleep disorder,” “edema,” or “others.”
For the assessment of interventions by health care professionals and anticancer treatment prescriptions, information from the O, A, and P columns and drug prescription history in the data source were investigated for the extracted positive S records. The interventions by health care professionals were categorized in any of the following: “adding symptomatic treatment for the adverse event signal,” “dose reduction or discontinuation of causative anticancer treatment,” “consultation with physician,” “others,” or “no intervention (ie, just following up the adverse event signal).” The actions categorized in “others” were further evaluated individually. For this assessment, we also randomly extracted 200 S records and evaluated them in the same way for comparison with the results from the deep learning model. Prescription history of anticancer treatment was analyzed by primary category of mechanism of action (MoA) with subcategories if applicable (eg, target molecule for kinase inhibitors).
Applicability Check to Other Text Data Including Patients’ Concerns
To check the applicability of our deep learning models to data from a different source, interview transcripts from patients with cancer were also evaluated. The interview transcripts were created by the Database of Individual Patient Experiences-Japan (DIPEx-Japan) [ 53 ]. DIPEx-Japan divides the interview transcripts into sections for each topic, such as “onset of disease” and “treatment,” and posts the processed texts on its website. Processing is conducted by accredited researchers based on qualitative research methods established by the University of Oxford [ 54 ]. In this study, interview text data created from interviews with 52 patients with breast cancer conducted from January 2008 to October 2018 were used to assess whether our deep learning models can extract adverse event signals from this source. In total, 508 interview transcripts were included with the approval of DIPEx-Japan.
Ethical Considerations
This study was conducted with anonymized data following approval by the ethics committee of the Keio University Faculty of Pharmacy (210914-1 and 230217-1) and in accordance with relevant guidelines and regulations and the Declaration of Helsinki. Informed consent specific to this study was waived due to the retrospective observational design of the study with the approval of the ethics committee of the Keio University Faculty of Pharmacy. To respect the will of each individual stakeholder, however, we provided patients and pharmacists of the pharmacy group with an opportunity to refuse the sharing of their pharmaceutical care records by posting an overview of this study at each pharmacy store or on their web page regarding the analysis using pharmaceutical care records. Interview transcripts from DIPEx-Japan were provided through a data sharing arrangement for using narrative data for research and education. Consent for interview transcription and its sharing from DIPEx-Japan was obtained from the participants when the interviews were recorded.
From the original data source of 2,180,902 pharmaceutical care records for 291,150 patients, S records written by pharmacists for patients with a history of at least 1 prescription of an anticancer drug were extracted. This yielded 30,784 S records for 2479 patients with cancer ( Table 1 ). The mean and median number of words in the S records were 38.8 (SD 29.4) and 32 (IQR 20-50), respectively. We applied our deep learning models, HFS, All AE, and AE-L, to these 30,784 S records for the evaluation of the deep learning models for adverse event signal detection.
For interview transcripts created by DIPEx-Japan, the mean and median number of words were 428.9 (SD 160.9) and 416 (IQR 308-526), respectively, in the 508 transcripts for 52 patients with breast cancer.
a SOAP: subjective, objective, assessment, plan.
b S: subjective.
Application of the HFS Model
First, we applied the HFS model to the S records for patients with cancer. The BERT-based model was used for this research as it showed the best performance score in our previous work [ 42 ].
S Records Extracted as HFS Positive
The S records extracted as HFS positive by the HFS model ( Table 2 ) amounted to 167 (0.5%) records for 119 (4.8%) patients. A majority of the patients had 1 HFS-positive record in their S records (n=91, 76.5%), while 2 patients had as many as 6 (1.7%) HFS-positive records. When we examined whether the extracted S records were true adverse event signals or not, 152 records were confirmed to be adverse event signals, while the other 15 records were false-positives. All the false-positive S records were descriptions about the absence of symptoms or confirmation of improving condition (eg, “no diarrhea, mouth ulcers, or limb pain so far” or “the skin on the soles of my feet has calmed down a lot with this ointment”). Some examples of S records that were predicted as HFS positive by the model are shown in Table S1 in Multimedia Appendix 2 .
The same examination was conducted with interview transcripts from DIPEx-Japan. Only 1 (0.2%) transcript was extracted as HFS positive by the HFS model, and it was a true adverse event signal (100%). The actual transcript extracted as HFS positive is shown in Table S2 in Multimedia Appendix 2 .
a S: subjective.
b HFS: hand-foot syndrome.
c All false-positive S records were denial of symptoms or confirmation of improving condition.
Interventions by Health Care Professionals
The 167 S records extracted as HFS positive as well as 200 randomly selected records were checked for interventions by health care professionals ( Figure 1 ). The proportion showing any action by health care professionals was 64.1% for 167 HFS-positive S records compared to 13% for the 200 random S records. Among the actions taken for HFS positives, “adding symptomatic treatment” was the most common, accounting for around half (n=79, 47.3%), followed by “other” (n=18, 10.8%). Most “other” actions were educational guidance from pharmacists, such as instructions on moisturizing, nail care, or application of ointment and advice on daily living (eg, “avoid tight socks”).

Anticancer Drugs Prescribed
The types of anticancer drugs prescribed for HFS-positive patients are summarized based on the prescription histories in Table 3 . For the 152 adverse event signals identified by the HFS model in the previous section, the most common MoA class of anticancer drugs used for the patients was antimetabolite (n=62, 40.8%), specifically fluoropyrimidines (n=59, 38.8%). Kinase inhibitors were next (n=49, 32.2%), with epidermal growth factor receptor (EGFR) inhibitors and multikinase inhibitors as major subgroups (n=28, 18.4% and n=14, 9.2%, respectively). The third and fourth most common MoAs were aromatase inhibitors (n=24, 15.8%) and antiandrogen or estrogen drugs (n=7, 4.6% each) for hormone therapy.
a EGFR: epidermal growth factor receptor.
b VEGF: vascular endothelial growth factor.
c HER2: human epidermal growth factor receptor-2.
d CDK4/6: cyclin-dependent kinase 4/6.
Application of the All AE or AE-L model
The All AE and AE-L models were also applied to the same S records for patients with cancer. The T5-based model was used for this research as it gave the best performance score in our previous work [ 43 ].
S Records Extracted as All AE or AE-L positive
The numbers of S records extracted as positive were 7604 (24.7%) for 1797 patients and 196 (0.6%) for 142 patients for All AE and AE-L, respectively. In the case of All AE, patients tended to have multiple adverse event positives in their S records (n=1315, 73.2% of patients had at least 2 positives). In the case of AE-L, most patients had only 1 AE-L positive (n=104, 73.2%), and the largest number of AE-L positives for 1 patient was 4 (2.8%; Table 4 ).
We focused on AE-L evaluation due to its greater importance from a medical viewpoint and lower workload for manual assessment, considering the number of positive S records. Of the 197 AE-L–positive S records, it was confirmed that 157 (80.1%) records accurately extracted adverse event signals, while 39 (19.9%) records were false-positives that did not include any adverse event signals ( Table 4 ). The contents of the 39 false-positives were all descriptions about the absence of symptoms or confirmation of improving condition, showing a similar tendency to the HFS false-positives (eg, “The diarrhea has calmed down so far. Symptoms in hands and feet are currently fine” and “No symptoms for the following: upset in stomach, diarrhea, nausea, abdominal pain, abdominal pain or stomach cramps, constipation”). Examples of S records that were predicted as AE-L positive are shown in Table S3 in Multimedia Appendix 2 .
The deep learning models were also applied to interview transcripts from DIPEx-Japan in the same manner. The deep learning models identified 84 (16.5%) and 18 (3.5%) transcripts as All AE or AE-L positive, respectively. Of the 84 All AE–positive transcripts, 73 (86.9%) were true adverse event signals. The false-positives of All AE (n=11, 13.1%) were categorized into any of the following 3 types: explanations about the disease or its prognosis, stories when their cancer was discovered, or emotional changes that did not include clear adverse event mentions. With regard to AE-L, all the 18 (100%) positives were true adverse event signals (Table S4 in Multimedia Appendix 2 ). Examples of actual transcripts extracted as All AE or AE-L positive are shown in Table S5 in Multimedia Appendix 2 .
b All AE: all (or any of) adverse event.
c AE-L: adverse events limiting patients’ daily lives.
d All false-positive S records were denial of symptoms or confirmation of improving condition.
Whether or not interventions were made by health care professionals was investigated for the 196 AE-L–positive S records. As in the HFS model evaluation, data from 200 randomly selected S records were used for comparison ( Figure 2 ). In total, 91 (46.4%) records in the 196 AE-L–positive records were accompanied by an intervention, while the corresponding figure in the 200 random records was 26 (13%) records. The most common action in response to adverse event signals identified by the AE-L model was “adding symptomatic treatment” (n=71, 36.2%), followed by “other” (n=11, 5.6%). “Other” included educational guidance from pharmacists, inquiries from pharmacists to physicians, or recommendations for patients to visit a doctor.

The types of anticancer drugs prescribed for patients with adverse event signals identified by the AE-L model were summarized based on the prescription histories ( Table 5 ). In connection with the 157 adverse event signals, the most common MoA of the prescribed anticancer drug was antimetabolite (n=62, 39.5%) and fluoropyrimidine (n=53, 33.8%), which accounted for the majority. Kinase inhibitor (n=31, 19.7%) was the next largest category with multikinase inhibitor (n=14, 8.9%) as the major subgroup. These were followed by antiandrogen (n=27, 17.2%), antiestrogen (n=10, 6.4%), and aromatase inhibitor (n=10, 6.4%) for hormone therapy.
b JAK: janus kinase.
c VEGF: vascular endothelial growth factor.
d BTK: bruton tyrosine kinase.
e FLT3: FMS-like tyrosine kinase-3.
f PARP: poly-ADP ribose polymerase.
g CDK4/6: cyclin-dependent kinase 4/6.
h CD20: cluster of differentiation 20.
Adverse Event Symptoms
For the 157 adverse event signals identified by the AE-L model, the symptoms were categorized according to the predefined guideline in our previous work [ 43 ]. “Pain or numbness” (n=57, 36.3%) accounted for the largest proportion followed by “fever” (n=46, 29.3%) and “nausea” (n=40, 25.5%; Table 6 ). Symptoms classified as “others” included chills, tinnitus, running tears, dry or peeling skin, and frequent urination. When comparing the proportion of the symptoms associated with or without interventions by health care professionals, a trend toward a greater proportion of interventions was observed in “fever,” “nausea,” “diarrhea,” “constipation,” “vomiting,” and “edema” ( Figure 3 , black boxes). On the other hand, a smaller proportion was observed in “pain or numbness,” “fatigue,” “appetite loss,” “rash or itchy,” “taste disorder,” and “dizziness” ( Figure 3 , gray boxes).

This study was designed to evaluate our deep learning models, previously constructed based on patient-authored texts posted in an online community, by applying them to pharmaceutical care records that contain both patients’ subjective concerns and medical information created by pharmacists. Based on the results, we discuss whether these deep learning models can extract clinically important adverse event signals that require medical intervention, and what characteristics they show when applied to data on patients’ concerns in pharmaceutical care records.
Performance for Adverse Event Signal Extraction
The first requirement for the deep learning models is to extract adverse event signals from patients’ narratives precisely. In this study, we evaluated the proportion of true adverse event signals in positive S records extracted by the HFS or AE-L model. True adverse event signals amounted to 152 (91%) and 157 (80.1%) for the HFS and AE-L models, respectively ( Tables 2 and 4 ). Given that the proportion of true adverse event signals in 200 randomly extracted S records without deep learning models was 54 (27%; categories other than “no adverse event” in Figures 1 and 2 ), the HFS and AE-L models were able to concentrate S records with adverse event mentions. Although 15 (9%) for the HFS model and 39 (19.9%) for the AE-L model were false-positives, it was confirmed all of the false-positive records described a lack of symptoms or confirmation of improving condition. We considered that such false-positives are due to the unique feature of pharmaceutical care records, where pharmacists might proactively interview patients about potential side effects of their medications. As the data set of blog articles we used to construct the deep learning models included few such cases (especially comments on lack of symptoms), our models seemed unable to exclude them correctly. Even though we confirmed that the proportion of true “adverse event” signals extracted from the S records by the HFS or AE-L model was more than 80%, the performance scores to extract true “HFS” or “AE-L” signals were not so high based on the performance check using 1000 randomly extracted S records ( F 1 -scores were 0.50 and 0.22 for true HFS and AE-L signals, respectively; Table S1 in Multimedia Appendix 1 ). It is considered that the performance to extract true HFS and AE-L signals was relatively low due to the short length of texts in the S records, providing less context to judge the impact on patients’ daily lives, especially for the AE-L model (the mean word number of the S records was 38.8 [SD 29.4; Table 1 ], similar to the sentence-level tasks in our previous work [ 42 , 43 ]). However, we consider a true adverse event signal proportion of more than 80% in this study represents a promising outcome, as this is the first attempt to apply our deep learning models to a different source of patients’ concern data, and the extracted positive cases would be worthy of evaluation by a medical professional, as the potential adverse events could be caused by drugs taken by the patients.
When the deep learning models were applied to DIPEx-Japan interview transcripts, including patients’ concerns, the proportion of true adverse event signals was also more than 80% (for All AE: n=73, 86.9% and for HFS and AE-L: n=18, 100%). The difference in the results between pharmaceutical care S records and DIPEx-Japan interview transcripts was the features of false-positives, descriptions about lack of symptoms or confirmation of improving condition in S records versus explanations about disease or its prognosis, stories about when their cancer was discovered, or emotional changes in interview transcripts. This is considered due to the difference in the nature of the data source; the pharmaceutical care records were generated in a real-time manner by pharmacists through their daily work, where adverse event signals are proactively monitored, while the interview transcripts were purely based on patients’ retrospective memories. Our deep learning models were able to extract true adverse event signals with an accuracy of more than 80% from both text data sources in spite of the difference in their nature. When looking at future implementation of the deep learning models in society (discussed in the Potential for Deep Learning Model Implementation in Society section), it may be desirable to further adjust deep learning models to reduce false-positives depending upon the features of the data source.

Identification of Important Adverse Events Requiring Medical Intervention
To assess whether the models could extract clinically important adverse event signals, we investigated interventions by health care professionals connected with the adverse event signals that are identified by our deep learning models. In the 200 randomly extracted S records, only 26 (13%) consisted of adverse event signals, leading to any intervention by health care professionals. On the other hand, the proportion of signals associated with interventions was increased to 107 (64.1%) and 91 (46.4%) in the S records extracted as positive by the HFS and AE-L models, respectively ( Figures 1 and 2 ). These results suggest that both deep learning models can screen clinically important adverse event signals that require intervention from health care professionals. The performance level in screening adverse event signals requiring medical intervention was higher in the HFS model than in the AE-L model (n=107, 64.1% vs n=91, 46.4%; Figures 1 and 2 ). Since the target events were specific and adverse event signals of HFS were narrowly defined, which is one of the typical side effects of some anticancer drugs, we consider that health care providers paid special attention to HFS-related signals and took action proactively. In both deep learning models, similar trends were observed in actions taken by health care professionals in response to extracted adverse event signals; common actions were attempts to manage adverse event symptoms by symptomatic treatment or other mild interventions, including educational guidance from pharmacists or recommendations for patients to visit a doctor. More direct interventions focused on the causative drugs (ie, “dose reduction or discontinuation of anticancer treatment”) amounted to less than 5%; 7 (4.2%) for the HFS model and 6 (3.1%) for the AE-L model ( Figures 1 and 2 ). Thus, it appears that our deep learning models can contribute to screening mild to moderate adverse event signals that require preventive actions such as symptomatic treatments or professional advice from health care providers, especially for patients with less sensitivity to adverse event signals or who have few opportunities to visit clinics and pharmacies.
Ability to Catch Real Side Effect Signals of Anticancer Drugs
Based on the drug prescription history associated with S records extracted as HFS or AE-L positive, the type and duration of anticancer drugs taken by patients experiencing the adverse event signals were investigated. For the HFS model, the most common MoA of anticancer drug was antimetabolite (fluoropyrimidine: n=59, 38.8%), followed by kinase inhibitors (n=49, 32.2%, of which EGFR inhibitors and multikinase inhibitors accounted for n=28, 18.4% and n=14, 9.2%, respectively) and aromatase inhibitors (n=24, 15.8%; Table 3 ). It is known that fluoropyrimidine and multikinase inhibitors are typical HFS-inducing drugs [ 55 - 58 ], suggesting that the HFS model accurately extracted HFS side effect signals derived from these drugs. Note that symptoms such as acneiform rash, xerosis, eczema, paronychia, changes in the nails, arthralgia, or stiffness of limb joints, which are common side effects of EGFR inhibitors or aromatase inhibitors [ 59 , 60 ], might be extracted as closely related expressions to those of HFS signals. When looking at the MoA of anticancer drugs for patients with adverse event signals identified by the AE-L model, antimetabolite (fluoropyrimidine) was the most common one (n=53, 33.8%), as in the case of those identified by the HFS model, followed by kinase inhibitors (n=31, 19.7%) and antiandrogens (n=27, 17.2%; Table 5 ). Since the AE-L model targets a broad range of adverse event symptoms, it is difficult to rationalize the relationship between the adverse event signals and types of anticancer drugs. However, the type of anticancer drugs would presumably closely correspond to the standard treatments of the cancer types of the patients. Based on the prescribed anticancer drugs, we can infer that a large percentage of the patients had breast or lung cancer, indicating that our study results were based on data from such a population. Thus, a possible direction for the expansion of this research would be adjusting the deep learning models by additional training with expressions for typical side effects associated with standard treatments of other cancer types. To interpret these results correctly, it should be noted that we could not investigate anticancer treatments conducted outside of the pharmacies (eg, the time-course relationship with intravenously administered drugs would be missed, as the administration will be done at hospitals). To further evaluate how useful this model is in side effect signal monitoring for patients with cancer, comprehensive medical information for the eligible patients would be required.
Suitability of the Deep Learning Models for Specific Adverse Event Symptoms
Among the adverse event signals identified by the AE-L model, the type of symptom was categorized according to a predefined annotation guideline that we previously developed [ 43 ]. The most frequently recorded adverse event signals identified by the AE-L model were “pain or numbness” (n=57, 36.3%), “fever” (n=46, 29.3%), and “nausea” (n=40, 25.5%; Table 6 ). Since the pharmaceutical care records had information about interventions by health care professionals, the frequency of the presence or absence of the interventions for each symptom was examined. A trend toward a greater proportion of interventions was observed in “fever,” “nausea,” “diarrhea,” “constipation,” “vomiting,” and “edema” ( Figure 3 , black boxes). There seem to be 2 possible explanations for this: these symptoms are of high importance and require early medical intervention or effective symptomatic treatments are available for these symptoms in clinical practice so that medical intervention is an easy option. On the other hand, a trend for a smaller proportion of adverse event signals to result in interventions was observed for “pain or numbness,” “fatigue,” “appetite loss,” “rash or itchy,” “taste disorder,” and “dizziness” ( Figure 3 , gray boxes). The reason for this may be the lack of effective symptomatic treatments or the difficulty of judging whether the severity of these symptoms justifies medical intervention by health care providers. In either case, there may be room for improvement in the quality of medical care for these symptoms. We expect that our research will contribute to a quality improvement in safety monitoring in clinical practice by supporting adverse event signal detection in a cost-effective manner.
Potential for Deep Learning Model Implementation in Society
Although we evaluated our deep learning models using pharmaceutical care records in this study, the main target of future implementation of our deep learning models in society would be narrative texts that patients directly write to record their daily experiences. For example, the application of these deep learning models to electronic media where patients record their daily experiences in their lives with disease (eg, health care–related e-communities and disease diary applications) could enable information about adverse event signal onset that patients experience to be provided to health care providers in a timely manner. Adverse event signals can automatically be identified and shared with health care providers based on the concern texts that patients post to any platform. This system will have the advantage that health care providers can efficiently grasp safety-related events that patients experience outside of clinic visits so that they can conduct more focused or personalized interactions with patients at their clinic visits. However, consideration should be given to avoid an excessive burden on health care providers. For instance, limiting the sharing of adverse event signals to those of high severity or summarizing adverse event signals over a week rather than sharing each one in a real-time manner may be reasonable approaches for medical staff. We also need to think about how to encourage patients to record their daily experiences using electronic tools. Not only technical progress and support but also the establishment of an ecosystem where both patients and medical staff can feel benefit will be required. Prospective studies with deep learning models to follow up patients in the long term and evaluate outcomes will be needed. We primarily looked at patient-authored texts as targets of implementation, but our deep learning models may also be worth using medical data including patients’ subjective concerns, such as pharmaceutical care S records. As this study confirmed that our deep learning models are applicable to patients’ concern texts tracked by pharmacists, it should be possible to use them to analyze other “patient voice-like” medical text data that have not been actively investigated so far.
Limitations
First, the major limitation of this study was that we were not able to collect complete medical information of the patients. Although we designed this study to analyze patients’ concerns extracted by the deep learning models and their relationship with medical information contained in the pharmaceutical care records, some information could not be tracked (eg, missing history of medical interventions or anticancer treatment at hospitals as well as diagnosis of patients’ primary cancers). Second, there might be a data creation bias in S records for patients’ concerns by pharmacists. For example, symptoms that have little impact on intervention decisions might less likely be recorded by them. It should be also noted that the characteristics of S records may not be consistent at different community pharmacies.
Conclusions
Our deep learning models were able to screen clinically important adverse event signals that require intervention by health care professionals from patients’ concerns in pharmaceutical care records. Thus, these models have the potential to support real-time adverse event monitoring of individual patients taking anticancer treatments in an efficient manner. We also confirmed that these deep learning models constructed based on patient-authored texts could be applied to patients’ subjective information recorded by pharmacists through their daily work. Further research may help to expand the applicability of the deep learning models for implementation in society or for analysis of data on patients’ concerns accumulated in professional records at pharmacies or hospitals.
Acknowledgments
This work was supported by Japan Society for the Promotion of Science, Grants-in-Aid for Scientific Research (KAKENHI; grant 21H03170) and Japan Science and Technology Agency, Core Research for Evolutional Science and Technology (CREST; grant JPMJCR22N1), Japan. Mr Yuki Yokokawa and Ms Sakura Yokoyama at our laboratory advised SN about the structure of pharmaceutical care records. This study would not have been feasible without the high quality of pharmaceutical care records created by many individual pharmacists at Nakajima Pharmacy Group through their daily work.
Data Availability
The data sets generated and analyzed during this study are available from the corresponding author on reasonable request.
Authors' Contributions
SN and SH designed the study. SN retrieved the subjective records of patients with cancer from the data source for the application of deep learning models and organized other data for subsequent evaluations. SN ran the deep learning models with the support of SW. SN, YY, and KS checked the adverse event signals for each subjective record that was extracted as positive by the models for hand-foot syndrome or adverse events limiting patients’ daily lives and evaluated the adverse event signal symptoms, details of interventions taken by health care professionals, and types of anticancer drugs prescribed for patients based on available data from the data source. HK and SI advised on the study concept and process. MS and RT provided pharmaceutical records at their community pharmacies along with advice on how to use and interpret them. SY and EA supervised the natural language processing research as specialists. SH supervised the study overall. SN drafted and finalized the paper. All authors reviewed and approved the paper.
Conflicts of Interest
SN is an employee of Daiichi Sankyo Co, Ltd. All other authors declare no conflicts of interest.
Performance evaluation of deep learning models.
Examples of S records and sample interview transcripts.
- Global cancer observatory: cancer over time. World Health Organization. URL: https://gco.iarc.fr/overtime/en [accessed 2023-07-02]
- Mattiuzzi C, Lippi G. Current cancer epidemiology. J Epidemiol Glob Health. 2019;9(4):217-222. [ FREE Full text ] [ CrossRef ] [ Medline ]
- Montazeri F, Komaki H, Mohebi F, Mohajer B, Mansournia MA, Shahraz S, et al. Editorial: disparities in cancer prevention and epidemiology. Front Oncol. 2022;12:872051. [ FREE Full text ] [ CrossRef ] [ Medline ]
- Lasala R, Santoleri F. Association between adherence to oral therapies in cancer patients and clinical outcome: a systematic review of the literature. Br J Clin Pharmacol. 2022;88(5):1999-2018. [ FREE Full text ] [ CrossRef ] [ Medline ]
- Pudkasam S, Polman R, Pitcher M, Fisher M, Chinlumprasert N, Stojanovska L, et al. Physical activity and breast cancer survivors: importance of adherence, motivational interviewing and psychological health. Maturitas. 2018;116:66-72. [ FREE Full text ] [ CrossRef ] [ Medline ]
- Markman M. Chemotherapy-associated neurotoxicity: an important side effect-impacting on quality, rather than quantity, of life. J Cancer Res Clin Oncol. 1996;122(9):511-512. [ CrossRef ] [ Medline ]
- Jitender S, Mahajan R, Rathore V, Choudhary R. Quality of life of cancer patients. J Exp Ther Oncol. 2018;12(3):217-221. [ Medline ]
- Di Nardo P, Lisanti C, Garutti M, Buriolla S, Alberti M, Mazzeo R, et al. Chemotherapy in patients with early breast cancer: clinical overview and management of long-term side effects. Expert Opin Drug Saf. 2022;21(11):1341-1355. [ FREE Full text ] [ CrossRef ] [ Medline ]
- Cuomo RE. Improving cancer patient outcomes and cost-effectiveness: a Markov simulation of improved early detection, side effect management, and palliative care. Cancer Invest. 2023;41(10):858-862. [ CrossRef ] [ Medline ]
- Pulito C, Cristaudo A, La Porta C, Zapperi S, Blandino G, Morrone A, et al. Oral mucositis: the hidden side of cancer therapy. J Exp Clin Cancer Res. 2020;39(1):210. [ FREE Full text ] [ CrossRef ] [ Medline ]
- Bartal A, Mátrai Z, Szûcs A, Liszkay G. Main treatment and preventive measures for hand-foot syndrome, a dermatologic side effect of cancer therapy. Magy Onkol. 2011;55(2):91-98. [ FREE Full text ] [ Medline ]
- Basch E, Jia X, Heller G, Barz A, Sit L, Fruscione M, et al. Adverse symptom event reporting by patients vs clinicians: relationships with clinical outcomes. J Natl Cancer Inst. 2009;101(23):1624-1632. [ FREE Full text ] [ CrossRef ] [ Medline ]
- Basch E. The missing voice of patients in drug-safety reporting. N Engl J Med. 2010;362(10):865-869. [ FREE Full text ] [ CrossRef ] [ Medline ]
- Fromme EK, Eilers KM, Mori M, Hsieh YC, Beer TM. How accurate is clinician reporting of chemotherapy adverse effects? A comparison with patient-reported symptoms from the Quality-of-Life Questionnaire C30. J Clin Oncol. 2004;22(17):3485-3490. [ FREE Full text ] [ CrossRef ] [ Medline ]
- Liu L, Suo T, Shen Y, Geng C, Song Z, Liu F, et al. Clinicians versus patients subjective adverse events assessment: based on patient-reported outcomes version of the common terminology criteria for adverse events (PRO-CTCAE). Qual Life Res. 2020;29(11):3009-3015. [ CrossRef ] [ Medline ]
- Churruca K, Pomare C, Ellis LA, Long JC, Henderson SB, Murphy LED, et al. Patient-reported outcome measures (PROMs): a review of generic and condition-specific measures and a discussion of trends and issues. Health Expect. 2021;24(4):1015-1024. [ FREE Full text ] [ CrossRef ] [ Medline ]
- Pérez-Alfonso KE, Sánchez-Martínez V. Electronic patient-reported outcome measures evaluating cancer symptoms: a systematic review. Semin Oncol Nurs. 2021;37(2):151145. [ FREE Full text ] [ CrossRef ] [ Medline ]
- Patient-reported outcome measures: use in medical product development to support labeling claims. U.S. Food & Drug Administration. 2009. URL: https://www.fda.gov/regulatory-information/search-fda-guidance-documents/patient-reported-outcome-measures-use-medical-product-development-support-labeling-claims [accessed 2023-11-26]
- Appendix 2 to the guideline on the evaluation of anticancer medicinal products in man—the use of patient-reported outcome (PRO) measures in oncology studies—scientific guideline. European Medicines Agency. 2016. URL: https://www.ema.europa.eu/en/appendix-2-guideline-evaluation-anticancer-medicinal-products-man-use-patient-reported-outcome-pro [accessed 2023-11-26]
- Weber SC. The evolution and use of patient-reported outcomes in regulatory decision making. RF Q. 2023;3(1):4-9. [ FREE Full text ]
- Teixeira MM, Borges FC, Ferreira PS, Rocha J, Sepodes B, Torre C. A review of patient-reported outcomes used for regulatory approval of oncology medicinal products in the European Union between 2017 and 2020. Front Med (Lausanne). 2022;9:968272. [ FREE Full text ] [ CrossRef ] [ Medline ]
- Newell S, Jordan Z. The patient experience of patient-centered communication with nurses in the hospital setting: a qualitative systematic review protocol. JBI Database System Rev Implement Rep. 2015;13(1):76-87. [ FREE Full text ] [ CrossRef ] [ Medline ]
- Yagasaki K, Takahashi H, Ouchi T, Yamagami J, Hamamoto Y, Amagai M, et al. Patient voice on management of facial dermatological adverse events with targeted therapies: a qualitative study. J Patient Rep Outcomes. 2019;3(1):27. [ FREE Full text ] [ CrossRef ] [ Medline ]
- Giardina TD, Korukonda S, Shahid U, Vaghani V, Upadhyay DK, Burke GF, et al. Use of patient complaints to identify diagnosis-related safety concerns: a mixed-method evaluation. BMJ Qual Saf. 2021;30(12):996-1001. [ FREE Full text ] [ CrossRef ] [ Medline ]
- Lauriola I, Lavelli A, Aiolli F. An introduction to deep learning in natural language processing: models, techniques, and tools. Neurocomputing. 2022;470:443-456. [ CrossRef ]
- Devlin J, Chang MW, Lee K, Toutanova K. BERT: pre-training of deep bidirectional transformers for language understanding. 2019. Presented at: 2019 Annual Conference of the North American Chapter of the Association for Computational Linguistics: Human Language Technologies; June 2-7, 2019;4171-4186; Minneapolis, MN, USA.
- Dreisbach C, Koleck TA, Bourne PE, Bakken S. A systematic review of natural language processing and text mining of symptoms from electronic patient-authored text data. Int J Med Inform. 2019;125:37-46. [ FREE Full text ] [ CrossRef ] [ Medline ]
- Sim JA, Huang X, Horan MR, Stewart CM, Robison LL, Hudson MM, et al. Natural language processing with machine learning methods to analyze unstructured patient-reported outcomes derived from electronic health records: a systematic review. Artif Intell Med. 2023;146:102701. [ CrossRef ] [ Medline ]
- Weissenbacher D, Banda JM, Davydova V, Estrada-Zavala D, Gascó Sánchez L, Ge Y, et al. Overview of the seventh social media mining for health applications (#SMM4H) shared tasks at COLING 2022. 2022. Presented at: Proceedings of the Seventh Workshop on Social Media Mining for Health Applications, Workshop & Shared Task; October 12-17, 2022;221-241; Gyeongju, Republic of Korea. URL: https://aclanthology.org/2022.smm4h-1.54/
- Matsuda S, Ohtomo T, Okuyama M, Miyake H, Aoki K. Estimating patient satisfaction through a language processing model: model development and evaluation. JMIR Form Res. 2023;7(1):e48534. [ FREE Full text ] [ CrossRef ] [ Medline ]
- Yu D, Vydiswaran VGV. An assessment of mentions of adverse drug events on social media with natural language processing: model development and analysis. JMIR Med Inform. 2022;10(9):e38140. [ FREE Full text ] [ CrossRef ] [ Medline ]
- Liu X, Chen H. A research framework for pharmacovigilance in health social media: identification and evaluation of patient adverse drug event reports. J Biomed Inform. 2015;58:268-279. [ FREE Full text ] [ CrossRef ] [ Medline ]
- Nikfarjam A, Sarker A, O'Connor K, Ginn R, Gonzalez G. Pharmacovigilance from social media: mining adverse drug reaction mentions using sequence labeling with word embedding cluster features. J Am Med Inform Assoc. 2015;22(3):671-681. [ FREE Full text ] [ CrossRef ] [ Medline ]
- Kakalou C, Dimitsaki S, Dimitriadis VK, Natsiavas P. Exploiting social media for active pharmacovigilance: the PVClinical social media workspace. Stud Health Technol Inform. 2022;290:739-743. [ CrossRef ] [ Medline ]
- Bousquet C, Dahamna B, Guillemin-Lanne S, Darmoni SJ, Faviez C, Huot C, et al. The adverse drug reactions from patient reports in social media project: five major challenges to overcome to operationalize analysis and efficiently support pharmacovigilance process. JMIR Res Protoc. 2017;6(9):e179. [ FREE Full text ] [ CrossRef ] [ Medline ]
- Young IJB, Luz S, Lone N. A systematic review of natural language processing for classification tasks in the field of incident reporting and adverse event analysis. Int J Med Inform. 2019;132:103971. [ CrossRef ] [ Medline ]
- Jacobsson R, Bergvall T, Sandberg L, Ellenius J. Extraction of adverse event severity information from clinical narratives using natural language processing. Pharmacoepidemiol Drug Saf. 2017;26(S2):37. [ FREE Full text ]
- Liang C, Gong Y. Predicting harm scores from patient safety event reports. Stud Health Technol Inform. 2017;245:1075-1079. [ CrossRef ] [ Medline ]
- Jiang G, Wang L, Liu H, Solbrig HR, Chute CG. Building a knowledge base of severe adverse drug events based on AERS reporting data using semantic web technologies. Stud Health Technol Inform. 2013;192(1-2):496-500. [ CrossRef ] [ Medline ]
- Usui M, Aramaki E, Iwao T, Wakamiya S, Sakamoto T, Mochizuki M. Extraction and standardization of patient complaints from electronic medication histories for pharmacovigilance: natural language processing analysis in Japanese. JMIR Med Inform. 2018;6(3):e11021. [ FREE Full text ] [ CrossRef ] [ Medline ]
- Watanabe T, Yada S, Aramaki E, Yajima H, Kizaki H, Hori S. Extracting multiple worries from breast cancer patient blogs using multilabel classification with the natural language processing model bidirectional encoder representations from transformers: infodemiology study of blogs. JMIR Cancer. 2022;8(2):e37840. [ FREE Full text ] [ CrossRef ] [ Medline ]
- Nishioka S, Watanabe T, Asano M, Yamamoto T, Kawakami K, Yada S, et al. Identification of hand-foot syndrome from cancer patients' blog posts: BERT-based deep-learning approach to detect potential adverse drug reaction symptoms. PLoS One. 2022;17(5):e0267901. [ FREE Full text ] [ CrossRef ] [ Medline ]
- Nishioka S, Asano M, Yada S, Aramaki E, Yajima H, Yanagisawa Y, et al. Adverse event signal extraction from cancer patients' narratives focusing on impact on their daily-life activities. Sci Rep. 2023;13(1):15516. [ FREE Full text ] [ CrossRef ] [ Medline ]
- Weed LL. Medical records that guide and teach. N Engl J Med. 1968;278(11):593-600. [ CrossRef ] [ Medline ]
- Podder V, Lew V, Ghassemzadeh S. SOAP Notes. Treasure Island, FL. StatPearls Publishing; 2023.
- Shenavar Masooleh I, Ramezanzadeh E, Yaseri M, Sahere Mortazavi Khatibani S, Sadat Fayazi H, Ali Balou H, et al. The effectiveness of training on daily progress note writing by medical interns. J Adv Med Educ Prof. 2021;9(3):168-175. [ FREE Full text ] [ CrossRef ] [ Medline ]
- Grothen AE, Tennant B, Wang C, Torres A, Sheppard BB, Abastillas G, et al. Application of artificial intelligence methods to pharmacy data for cancer surveillance and epidemiology research: a systematic review. JCO Clin Cancer Inform. 2020;4:1051-1058. [ FREE Full text ] [ CrossRef ] [ Medline ]
- Ohno Y, Kato R, Ishikawa H, Nishiyama T, Isawa M, Mochizuki M, et al. Using the natural language processing system MedNER-J to analyze pharmaceutical care records. medRxiv. Preprint posted online on October 2, 2023. [ CrossRef ]
- Ranchon F, Chanoine S, Lambert-Lacroix S, Bosson JL, Moreau-Gaudry A, Bedouch P. Development of artificial intelligence powered apps and tools for clinical pharmacy services: a systematic review. Int J Med Inform. 2023;172:104983. [ CrossRef ] [ Medline ]
- Nakajima Pharmacy. URL: https://www.nakajima-phar.co.jp/ [accessed 2023-12-07]
- Raffel C, Shazeer N, Roberts A, Lee K, Narang S, Matena M, et al. Exploring the limits of transfer learning with a unified text-to-text transformer. J Mach Learn Res. 2020;21(140):1-67. [ FREE Full text ]
- Pathak A. Comparative analysis of transformer based language models. Comput Sci Inf Technol. 2021.:165-176. [ FREE Full text ] [ CrossRef ]
- DIPEx Japan. URL: https://www.dipex-j.org/ [accessed 2024-02-04]
- Herxheimer A, McPherson A, Miller R, Shepperd S, Yaphe J, Ziebland S. Database of patients' experiences (DIPEx): a multi-media approach to sharing experiences and information. Lancet. 2000;355(9214):1540-1543. [ CrossRef ] [ Medline ]
- Lara PE, Muiño CB, de Spéville BD, Reyes JJ. Hand-foot skin reaction to regorafenib. Actas Dermosifiliogr. 2016;107(1):71-73. [ CrossRef ]
- Zaiem A, Hammamia SB, Aouinti I, Charfi O, Ladhari W, Kastalli S, et al. Hand-foot syndrome induced by chemotherapy drug: case series study and literature review. Indian J Pharmacol. 2022;54(3):208-215. [ FREE Full text ] [ CrossRef ] [ Medline ]
- McLellan B, Ciardiello F, Lacouture ME, Segaert S, Van Cutsem E. Regorafenib-associated hand-foot skin reaction: practical advice on diagnosis, prevention, and management. Ann Oncol. 2015;26(10):2017-2026. [ FREE Full text ] [ CrossRef ] [ Medline ]
- Ai L, Xu Z, Yang B, He Q, Luo P. Sorafenib-associated hand-foot skin reaction: practical advice on diagnosis, mechanism, prevention, and management. Expert Rev Clin Pharmacol. 2019;12(12):1121-1127. [ CrossRef ] [ Medline ]
- Tenti S, Correale P, Cheleschi S, Fioravanti A, Pirtoli L. Aromatase inhibitors-induced musculoskeletal disorders: current knowledge on clinical and molecular aspects. Int J Mol Sci. 2020;21(16):5625. [ FREE Full text ] [ CrossRef ] [ Medline ]
- Lacouture ME, Melosky BL. Cutaneous reactions to anticancer agents targeting the epidermal growth factor receptor: a dermatology-oncology perspective. Skin Therapy Lett. 2007;12(6):1-5. [ FREE Full text ] [ Medline ]
Abbreviations
Edited by G Eysenbach; submitted 25.12.23; peer-reviewed by CY Wang, L Guo; comments to author 24.01.24; revised version received 14.02.24; accepted 09.03.24; published 16.04.24.
©Satoshi Nishioka, Satoshi Watabe, Yuki Yanagisawa, Kyoko Sayama, Hayato Kizaki, Shungo Imai, Mitsuhiro Someya, Ryoo Taniguchi, Shuntaro Yada, Eiji Aramaki, Satoko Hori. Originally published in the Journal of Medical Internet Research (https://www.jmir.org), 16.04.2024.
This is an open-access article distributed under the terms of the Creative Commons Attribution License (https://creativecommons.org/licenses/by/4.0/), which permits unrestricted use, distribution, and reproduction in any medium, provided the original work, first published in the Journal of Medical Internet Research, is properly cited. The complete bibliographic information, a link to the original publication on https://www.jmir.org/, as well as this copyright and license information must be included.
Advertisement
Drug-drug interactions between psychiatric medications and MDMA or psilocybin: a systematic review
- Published: 07 March 2022
- Volume 239 , pages 1945–1976, ( 2022 )
Cite this article
- Aryan Sarparast 1 ,
- Kelan Thomas 2 ,
- Benjamin Malcolm 3 &
- Christopher S. Stauffer 1 , 4
8260 Accesses
24 Citations
129 Altmetric
16 Mentions
Explore all metrics
Rationale & objectives
± 3,4-Methylenedioxymethamphetamine (MDMA) and psilocybin are currently moving through the US Food and Drug Administration’s phased drug development process for psychiatric treatment indications: posttraumatic stress disorder and depression, respectively. The current standard of care for these disorders involves treatment with psychiatric medications (e.g., selective serotonin reuptake inhibitors), so it will be important to understand drug-drug interactions between MDMA or psilocybin and psychiatric medications.
In accordance with Preferred Reporting Items for Systematic Reviews and Meta-Analyses (PRISMA) guidelines, we queried the MEDLINE database via PubMed for publications of human studies in English spanning between the first synthesis of psilocybin (1958) and December 2020. We used 163 search terms containing 22 psychiatric medication classes, 135 specific psychiatric medications, and 6 terms describing MDMA or psilocybin.
Forty publications were included in our systematic review: 26 reporting outcomes from randomized controlled studies with healthy adults, 3 epidemiologic studies, and 11 case reports. Publications of studies describe interactions between MDMA ( N = 24) or psilocybin ( N = 5) and medications from several psychiatric drug classes: adrenergic agents, antipsychotics, anxiolytics, mood stabilizers, NMDA antagonists, psychostimulants, and several classes of antidepressants. We focus our results on pharmacodynamic, physiological, and subjective outcomes of drug-drug interactions.
Conclusions
As MDMA and psilocybin continue to move through the FDA drug development process, this systematic review offers a compilation of existing research on psychiatric drug-drug interactions with MDMA or psilocybin.
This is a preview of subscription content, log in via an institution to check access.
Access this article
Price includes VAT (Russian Federation)
Instant access to the full article PDF.
Rent this article via DeepDyve
Institutional subscriptions
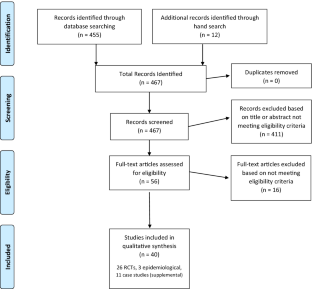
Similar content being viewed by others
Dosing Psychedelics and MDMA
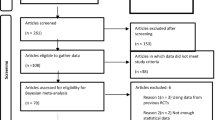
Selective serotonin reuptake inhibitors in the treatment of depression, anxiety, and post-traumatic stress disorder in substance use disorders: a Bayesian meta-analysis
Dimy Fluyau, Paroma Mitra, … Christopher G. Pierre
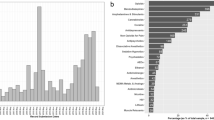
Concomitant drugs associated with increased mortality for MDMA users reported in a drug safety surveillance database
Isaac V. Cohen, Tigran Makunts, … Kelan Thomas
Abraham TT, Barnes AJ, Lowe RH, Spargo EAK, Milman G, Pirnay SO, Gorelick DA, Goodwin RS, Huestis MA (2009) Urinary MDMA, MDA, HMMA, and HMA Excretion Following Controlled MDMA Administration to Humans. J Anal Toxicol 33(8):439–446
Article CAS Google Scholar
Akhondzadeh S, Hampa AD (2005) Topiramate prevents ecstasy consumption: A case report. Fundam Clin Pharmacol 19(5):601–602. https://doi.org/10.1111/j.1472-8206.2005.00355.x
Article CAS PubMed Google Scholar
Artin H, Zisook S, Ramanathan D (2021) How do serotonergic psychedelics treat depression: The potential role of neuroplasticity. World J Psychiatr 11(6):201–214. https://doi.org/10.5498/wjp.v11.i6.201
Article PubMed PubMed Central Google Scholar
Baggott MJ, Kirkpatrick MG, Bedi G, de Wit H (2015) Intimate insight: MDMA changes how people talk about significant others. J Psychopharmacol 29(6):669–677. https://doi.org/10.1177/0269881115581962
Article CAS PubMed PubMed Central Google Scholar
Barrett FS, Bradstreet MP, Leoutsakos JMS, Johnson MW, Griffiths RR (2016) The Challenging Experience Questionnaire: Characterization of challenging experiences with psilocybin mushrooms. J Psychopharmacol 30(12):1279–1295
Article Google Scholar
Barrett FS, Griffiths RR (2018) Classic Hallucinogens and Mystical Experiences: Phenomenology and Neural Correlates. Curr Top Behav Neurosci 36:393–430. https://doi.org/10.1007/7854_2017_474
Battaglia G, Brooks BP, Kulsakdinun C, De Souza EB (1988) Pharmacologic profile of MDMA (3,4-methylenedioxymethamphetamine) at various brain recognition sites. Eur J Pharmacol 149(1–2):159–163. https://doi.org/10.1016/0014-2999(88)90056-8
Baylen CA, Rosenberg H (2006) A review of the acute subjective effects of MDMA/ecstasy. Addiction 101(7):933–947. https://doi.org/10.1111/j.1360-0443.2006.01423.x
Article PubMed Google Scholar
Becker AM, Holze F, Grandinetti T, Klaiber A, Toedtli VE, Kolaczynska KE, Duthaler U, Varghese N, Eckert A, Grünblatt E, Liechti ME (2021) Acute Effects of Psilocybin After Escitalopram or Placebo Pretreatment in a Randomized, Double-Blind, Placebo-Controlled, Crossover Study in Healthy Subjects. Clin Pharmacol Ther n/a(n/a). https://doi.org/10.1002/cpt.2487
Bertilsson L, Dahl M-L, Dalén P, Al-Shurbaji A (2002) Molecular genetics of CYP2D6: Clinical relevance with focus on psychotropic drugs: Molecular genetics of CYP2D6 . Br J Clin Pharmacol 53(2):111–122. https://doi.org/10.1046/j.0306-5251.2001.01548.x
Bigler MB, Egli SB, Hysek CM, Hoenger G, Schmied L, Baldin FS, Marquardsen FA, Recher M, Liechti ME, Hess C, Berger CT (2015) Stress-Induced In Vivo Recruitment of Human Cytotoxic Natural Killer Cells Favors Subsets with Distinct Receptor Profiles and Associates with Increased Epinephrine Levels. PLoS ONE 10(12):e0145635. https://doi.org/10.1371/journal.pone.0145635
Bingham C (1998) Necrotizing renal vasculopathy resulting in chronic renal failure after ingestion of methamphetamine and 3,4-methylenedioxymethamphetamine ('ecstasy’). Nephrol Dial Transplant 13(10):2654–2655. https://doi.org/10.1093/ndt/13.10.2654
Blier P, Ward HE, Tremblay P, Laberge L, Hébert C, Bergeron R (2010) Combination of Antidepressant Medications From Treatment Initiation for Major Depressive Disorder: A Double-Blind Randomized Study. Am J Psychiatry 167(3):281–288. https://doi.org/10.1176/appi.ajp.2009.09020186
Bogen IL, Haug KH, Myhre O, Fonnum F (2003) Short- and long-term effects of MDMA (“ecstasy”) on synaptosomal and vesicular uptake of neurotransmitters in vitro and ex vivo. Neurochem Int 43(4–5):393–400. https://doi.org/10.1016/S0197-0186(03)00027-5
Bogenschutz MP, Forcehimes AA, Pommy JA, Wilcox CE, Barbosa P, Strassman RJ (2015) Psilocybin-assisted treatment for alcohol dependence: A proof-of-concept study. J Psychopharmacol 29(3):289–299. https://doi.org/10.1177/0269881114565144
Bonhaus DW, Weinhardt KK, Taylor M, Desouza A, Mcneeley PM, Szczepanski K, Fontana DJ, Trinh J, Rocha CL, Dawson MW, Flippin LA, Eglen RM (1997) RS-102221: A Novel High Affinity and Selective, 5-HT 2C Receptor Antagonist. Neuropharmacology 36(4–5):621–629. https://doi.org/10.1016/S0028-3908(97)00049-X
Bonson K (1996) Chronic Administration of Serotonergic Antidepressants Attenuates the Subjective Effects of LSD in Humans. Neuropsychopharmacology 14(6):425–436. https://doi.org/10.1016/0893-133X(95)00145-4
Borowiak KS, Ciechanowski K, Waloszczyk P (1998) Psilocybin mushroom (Psilocybe semilanceata) intoxication with myocardial infarction. J Toxicol Clin Toxicol 36(1–2):47–49. https://doi.org/10.3109/15563659809162584
Brown RT, Nicholas CR, Cozzi NV, Gassman MC, Cooper KM, Muller D, Thomas CD, Hetzel SJ, Henriquez KM, Ribaudo AS, Hutson PR (2017) Pharmacokinetics of Escalating Doses of Oral Psilocybin in Healthy Adults. Clin Pharmacokinet 56(12):1543–1554. https://doi.org/10.1007/s40262-017-0540-6
Carhart-Harris R, Giribaldi B, Watts R, Baker-Jones M, Murphy-Beiner A, Murphy R, Martell J, Blemings A, Erritzoe D, Nutt DJ (2021) Trial of Psilocybin versus Escitalopram for Depression. N Engl J Med 384(15):1402–1411. https://doi.org/10.1056/NEJMoa2032994
Carhart-Harris R, Leech R, Hellyer PJ, Shanahan M, Feilding A, Tagliazucchi E, Chialvo DR, Nutt D (2014) The entropic brain: A theory of conscious states informed by neuroimaging research with psychedelic drugs. Front Hum Neurosci 8. https://doi.org/10.3389/fnhum.2014.00020
Carhart-Harris R, Nutt D (2017) Serotonin and brain function: A tale of two receptors. J Psychopharmacol 31(9):1091–1120. https://doi.org/10.1177/0269881117725915
Carpenter LL, Yasmin S, Price LH (2002) A double-blind, placebo-controlled study of antidepressant augementation with mirtazapine. Biol Psychiat 51(2):183–188. https://doi.org/10.1016/S0006-3223(01)01262-8
Castro Santos H, Gama Marques J (2021) What is the clinical evidence on psilocybin for the treatment of psychiatric disorders? A Systematic Review Porto Biomedical Journal 6(1):e128. https://doi.org/10.1097/j.pbj.0000000000000128
Center for Behavioral Health Statistics and Quality (2020) National Survey on Drug Use and Health—2019. Substance Abuse and Mental Health Services Administration. https://www.samhsa.gov/data/
Cho S, Ning M, Zhang Y, Rubin LH, Jeong H (2016) 17β-Estradiol up-regulates UDP-glucuronosyltransferase 1A9 expression via estrogen receptor α. Acta Pharmaceutica Sinica B 6(5):504–509. https://doi.org/10.1016/j.apsb.2016.04.005
Cohen IV, Makunts T, Abagyan R, Thomas K (2021) Concomitant drugs associated with increased mortality for MDMA users reported in a drug safety surveillance database. Sci Rep 11(1):5997. https://doi.org/10.1038/s41598-021-85389-x
Coleman JA, Green EM, Gouaux E (2016) X-ray structures and mechanism of the human serotonin transporter. Nature 532(7599):334–339. https://doi.org/10.1038/nature17629
Copeland J, Dillon P, Gascoigne M (2006) Ecstasy and the concomitant use of pharmaceuticals. Addict Behav 31(2):367–370. https://doi.org/10.1016/j.addbeh.2005.05.025
Danforth AL, Grob CS, Struble C, Feduccia AA, Walker N, Jerome L, Yazar-Klosinski B, Emerson A (2018) Reduction in social anxiety after MDMA-assisted psychotherapy with autistic adults: A randomized, double-blind, placebo-controlled pilot study. Psychopharmacology 235(11):3137–3148. https://doi.org/10.1007/s00213-018-5010-9
Davis AK, Barrett FS, May DG, Cosimano MP, Sepeda ND, Johnson MW, Finan PH, Griffiths RR (2021) Effects of Psilocybin-Assisted Therapy on Major Depressive Disorder: A Randomized Clinical Trial. JAMA Psychiat 78(5):481–489. https://doi.org/10.1001/jamapsychiatry.2020.3285
De Jong J, Wauben P, Huijbrechts I, Oolders H, Haffmans J (2010) Doxazosin treatment for posttraumatic stress disorder. J Clin Psychopharmacol 30(1):84–85. https://doi.org/10.1097/JCP.0b013e3181c827ae
Perna DSF, E. B., Theunissen, E. L., Kuypers, K. P., Heckman, P., de la Torre, R., Farre, M., & Ramaekers, J. G. (2014) Memory and mood during MDMA intoxication, with and without memantine pretreatment. Neuropharmacology 87:198–205. https://doi.org/10.1016/j.neuropharm.2014.03.008
Delgado JH, Caruso MJ, Waksman JC, Honigman B, Stillman D (2004) Acute, transient urinary retention from combined ecstasy and methamphetamine use. J Emerg Med 26(2):173–175. https://doi.org/10.1016/j.jemermed.2003.05.007
Dinis-Oliveira RJ (2017) Metabolism of psilocybin and psilocin: Clinical and forensic toxicological relevance. Drug Metab Rev 49(1):84–91. https://doi.org/10.1080/03602532.2016.1278228
Dobry Y, Rice T, Sher L (2013) Ecstasy use and serotonin syndrome: A neglected danger to adolescents and young adults prescribed selective serotonin reuptake inhibitors. Int J Adolesc Med Health 25(3):193–199. https://doi.org/10.1515/ijamh-2013-0052
Dore J, Turnipseed B, Dwyer S, Turnipseed A, Andries J, Ascani G, Monnette C, Huidekoper A, Strauss N, Wolfson P (2019) Ketamine Assisted Psychotherapy (KAP): Patient Demographics, Clinical Data and Outcomes in Three Large Practices Administering Ketamine with Psychotherapy. J Psychoactive Drugs 51(2):189–198. https://doi.org/10.1080/02791072.2019.1587556
English BA, Dortch M, Ereshefsky L, Jhee S (2012) Clinically Significant Psychotropic Drug-Drug Interactions in the Primary Care Setting. Curr Psychiatry Rep 14(4):376–390. https://doi.org/10.1007/s11920-012-0284-9
Farré M, de la Torre R, Mathúna BO, Roset PN, Peiró AM, Torrens M, Ortuño J, Pujadas M, Camí J (2004) Repeated doses administration of MDMA in humans: pharmacological effects and pharmacokinetics. Psychopharmacology (Berl) 173(3–4):364–75
Farré M, Abanades S, Roset PN, Peiró AM, Torrens M, O’Mathúna B, Segura M, de la Torre R (2007) Pharmacological Interaction between 3,4-Methylenedioxymethamphetamine (Ecstasy) and Paroxetine: Pharmacological Effects and Pharmacokinetics. J Pharmacol Exp Ther 323(3):954–962. https://doi.org/10.1124/jpet.107.129056
Feduccia AA, Jerome L, Mithoefer MC, Holland J (2020) Discontinuation of medications classified as reuptake inhibitors affects treatment response of MDMA-assisted psychotherapy. Psychopharmacology. https://doi.org/10.1007/s00213-020-05710-w
Feduccia AA, Mithoefer MC (2018) MDMA-assisted psychotherapy for PTSD: Are memory reconsolidation and fear extinction underlying mechanisms? Prog Neuropsychopharmacol Biol Psychiatry 84:221–228. https://doi.org/10.1016/j.pnpbp.2018.03.003
Fleckenstein AE, Hanson GR (2003) Impact of psychostimulants on vesicular monoamine transporter function. Eur J Pharmacol 479(1):283–289. https://doi.org/10.1016/j.ejphar.2003.08.077
Fogg C, Michaels TI, de la Salle S, Jahn ZW, Williams MT (2021) Ethnoracial health disparities and the ethnopsychopharmacology of psychedelic-assisted psychotherapies. Exp Clin Psychopharmacol. https://doi.org/10.1037/pha0000490
Gable RS (2004) Comparison of acute lethal toxicity of commonly abused psychoactive substances. Addiction 99(6):686–696. https://doi.org/10.1111/j.1360-0443.2004.00744.x
Gorman I, Belser AB, Jerome L, Hennigan C, Shechet B, Hamilton S, Yazar-Klosinski B, Emerson A, Feduccia AA (2020) Posttraumatic Growth After MDMA-Assisted Psychotherapy for Posttraumatic Stress Disorder. J Trauma Stress 33(2):161–170. https://doi.org/10.1002/jts.22479
Griffiths RR, Richards WA, Johnson MW, McCann UD, Jesse R (2008) Mystical-type experiences occasioned by psilocybin mediate the attribution of personal meaning and spiritual significance 14 months later. Journal of Psychopharmacology (oxford, England) 22(6):621–632. https://doi.org/10.1177/0269881108094300
Griffiths, R. R., Richards, W. A., McCann, U., & Jesse, R. (2006). Psilocybin can occasion mystical-type experiences having substantial and sustained personal meaning and spiritual significance. Psychopharmacology , 187 (3), 268–283 284–292. https://doi.org/10.1007/s00213-006-0457-5
Grob, C. S., & Grigsby, J. (Eds.). (2021). Handbook of medical hallucinogens . The Guilford Press.
Grob CS, Poland RE, Chang L, Ernst T (1995) Psychobiologic effects of 3,4-methylenedioxymethamphetamine in humans: Methodological considerations and preliminary observations. Behav Brain Res 73(1):103–107. https://doi.org/10.1016/0166-4328(96)00078-2
Harmer CJ, Duman RS, Cowen PJ (2017) How do antidepressants work? New perspectives for refining future treatment approaches. The Lancet Psychiatry 4(5):409–418. https://doi.org/10.1016/S2215-0366(17)30015-9
Hasler F, Grimberg U, Benz MA, Huber T, Vollenweider FX (2004) Acute psychological and physiological effects of psilocybin in healthy humans: a double-blind, placebo-controlled dose-effect study. Psychopharmacology (Berl) 172(2):145–56
Hasler F, Studerus E, Lindner K, Ludewig S, Vollenweider F (2009) Investigation of serotonin-1A receptor function in the human psychopharmacology of MDMA. J Psychopharmacol 23(8):923–935. https://doi.org/10.1177/0269881108094650
Hilber B, Scholze P, Dorostkar MM, Sandtner W, Holy M, Boehm S, Singer EA, Sitte HH (2005) Serotonin-transporter mediated efflux: A pharmacological analysis of amphetamines and non-amphetamines. Neuropharmacology 49(6):811–819. https://doi.org/10.1016/j.neuropharm.2005.08.008
Hofmann A, Frey A, Ott H, Petrzilka Th, Troxler F (1958) Konstitutionsaufklärung und Synthese von Psilocybin. Experientia 14(11):397–399. https://doi.org/10.1007/BF02160424
Hysek CM, Brugger R, Simmler LD, Bruggisser M, Donzelli M, Grouzmann E, Hoener MC, Liechti ME (2012a) Effects of the α 2 -Adrenergic Agonist Clonidine on the Pharmacodynamics and Pharmacokinetics of 3,4-Methylenedioxymethamphetamine in Healthy Volunteers. J Pharmacol Exp Ther 340(2):286–294. https://doi.org/10.1124/jpet.111.188425
Hysek CM, Domes G, Liechti ME (2012b) MDMA enhances “mind reading” of positive emotions and impairs “mind reading” of negative emotions. Psychopharmacology 222(2):293–302. https://doi.org/10.1007/s00213-012-2645-9
Hysek CM, Fink AE, Simmler LD, Donzelli M, Grouzmann E, Liechti ME (2013) Α 1 -Adrenergic receptors contribute to the acute effects of 3,4-methylenedioxymethamphetamine in humans. J Clin Psychopharmacol 33(5):658–666. https://doi.org/10.1097/JCP.0b013e3182979d32
Hysek CM, Liechti ME (2012) Effects of MDMA alone and after pretreatment with reboxetine, duloxetine, clonidine, carvedilol, and doxazosin on pupillary light reflex. Psychopharmacology 224(3):363–376. https://doi.org/10.1007/s00213-012-2761-6
Hysek CM, Schmid Y, Rickli A, Simmler L, Donzelli M, Grouzmann E, Liechti ME (2012c) Carvedilol inhibits the cardiostimulant and thermogenic effects of MDMA in humans. Br J Pharmacol 166(8):2277–2288. https://doi.org/10.1111/j.1476-5381.2012.01936.x
Hysek CM, Schmid Y, Simmler LD, Domes G, Heinrichs M, Eisenegger C, Preller KH, Quednow BB, Liechti ME (2014a) MDMA enhances emotional empathy and prosocial behavior. Social Cognitive and Affective Neuroscience 9(11):1645–1652. https://doi.org/10.1093/scan/nst161
Hysek CM, Simmler LD, Ineichen M, Grouzmann E, Hoener MC, Brenneisen R, Huwyler J, Liechti ME (2011) The Norepinephrine Transporter Inhibitor Reboxetine Reduces Stimulant Effects of MDMA (“Ecstasy”) in Humans. Clin Pharmacol Ther 90(2):246–255. https://doi.org/10.1038/clpt.2011.78
Hysek CM, Simmler LD, Nicola VG, Vischer N, Donzelli M, Krähenbühl S, Grouzmann E, Huwyler J, Hoener MC, Liechti ME (2012d) Duloxetine Inhibits Effects of MDMA (“Ecstasy") In Vitro and in Humans in a Randomized Placebo-Controlled Laboratory Study. PLoS ONE 7(5):e36476. https://doi.org/10.1371/journal.pone.0036476
Hysek CM, Simmler LD, Schillinger N, Meyer N, Schmid Y, Donzelli M, Grouzmann E, Liechti ME (2014b) Pharmacokinetic and pharmacodynamic effects of methylphenidate and MDMA administered alone or in combination. Int J Neuropsychopharmacol 17(03):371–381. https://doi.org/10.1017/S1461145713001132
Hysek CM, Vollenweider FX, Liechti ME (2010) Effects of a beta-blocker on the cardiovascular response to MDMA (Ecstasy). Emergency Medicine Journal : EMJ 27(8):586–589. https://doi.org/10.1136/emj.2009.079905
Jerome L, Feduccia AA, Wang JB, Hamilton S, Yazar-Klosinski B, Emerson A, Mithoefer MC, Doblin R (2020) Long-term follow-up outcomes of MDMA-assisted psychotherapy for treatment of PTSD: A longitudinal pooled analysis of six phase 2 trials. Psychopharmacology 237(8):2485–2497. https://doi.org/10.1007/s00213-020-05548-2
Johnson MW, Garcia-Romeu A, Cosimano MP, Griffiths RR (2014) Pilot study of the 5-HT2AR agonist psilocybin in the treatment of tobacco addiction. J Psychopharmacol 28(11):983–992. https://doi.org/10.1177/0269881114548296
Johnson MW, Griffiths RR, Hendricks PS, Henningfield JE (2018) The abuse potential of medical psilocybin according to the 8 factors of the Controlled Substances Act. Neuropharmacology 142:143–166. https://doi.org/10.1016/j.neuropharm.2018.05.012
Johnson MW, Hendricks PS, Barrett FS, Griffiths RR (2019) Classic psychedelics: An integrative review of epidemiology, therapeutics, mystical experience, and brain network function. Pharmacol Ther 197:83–102. https://doi.org/10.1016/j.pharmthera.2018.11.010
Johnson MW, Richards WA, Griffiths RR (2008) Human Hallucinogen Research: Guidelines for Safety. Journal of Psychopharmacology (oxford, England) 22(6):603–620. https://doi.org/10.1177/0269881108093587
Kaskey G (1992) Possible interaction between an MAOI and “ecstasy.” Am J Psychiatry 149(3):411a–4412. https://doi.org/10.1176/ajp.149.3.411a
Keeler, M. H. (1967). Chlorpromazine Antagonism of Psilocybin Effect. International Journal of Neuropsychiatry , 3 , 66–71. https://bibliography.maps.org/bibliography/default/citation/1972
Khachatryan D, Groll D, Booij L, Sepehry AA, Schütz CG (2016) Prazosin for treating sleep disturbances in adults with posttraumatic stress disorder: A systematic review and meta-analysis of randomized controlled trials. Gen Hosp Psychiatry 39:46–52. https://doi.org/10.1016/j.genhosppsych.2015.10.007
Kinzie JD, Leung P (1989) Clonidine in Cambodian patients with posttraumatic stress disorder. J Nerv Ment Dis 177(9):546–550. https://doi.org/10.1097/00005053-198909000-00005
Kirkpatrick MG, de Wit H (2015) MDMA: A social drug in a social context. Psychopharmacology 232(6):1155–1163. https://doi.org/10.1007/s00213-014-3752-6
Kolbrich EA, Goodwin RS, Gorelick DA, Hayes RJ, Stein EA, Huestis MA (2008) Plasma pharmacokinetics of 3,4-methylenedioxymethamphetamine after controlled oral administration to young adults. Ther Drug Monit 30(3):320–332. https://doi.org/10.1097/FTD.0b013e3181684fa0
Krystal, J. H., Rosenheck, R. A., Cramer, J. A., Vessicchio, J. C., Jones, K. M., Vertrees, J. E., Horney, R. A., Huang, G. D., Stock, C., & Veterans Affairs Cooperative Study No. 504 Group (2011) Adjunctive risperidone treatment for antidepressant-resistant symptoms of chronic military service-related PTSD: A randomized trial. JAMA 306(5):493–502. https://doi.org/10.1001/jama.2011.1080
Lauerma H, Wuorela M, Halme M (1998) Interaction of serotonin reuptake inhibitor and 3,4-methylenedioxymethamphetamine? Biol Psychiat 43(12):929
Leonard JB, Anderson B, Klein-Schwartz W (2018) Does getting high hurt? Characterization of cases of LSD and psilocybin-containing mushroom exposures to national poison centers between 2000 and 2016. Journal of Psychopharmacology (oxford, England) 32(12):1286–1294. https://doi.org/10.1177/0269881118793086
Liechti ME (2000) Acute Psychological Effects of 3,4-Methylenedioxymethamphetamine (MDMA, “Ecstasy”) are Attenuated by the Serotonin Uptake Inhibitor Citalopram. Neuropsychopharmacology 22(5):513–521. https://doi.org/10.1016/S0893-133X(99)00148-7
Liechti, M. E., Geyer, M. A., Hell, D., & Vollenweider, F. X. (2001). Effects of MDMA (ecstasy) on prepulse inhibition and habituation of startle in humans after pretreatment with citalopram, haloperidol, or ketanserin. Neuropsychopharmacology: Official Publication of the American College of Neuropsychopharmacology , 24 (3), 240–252. https://doi.org/10.1016/S0893-133X(00)00199-8
Liechti ME, Vollenweider FX (2000a) The serotonin uptake inhibitor citalopram reduces acute cardiovascular and vegetative effects of 3, 4-methylenedioxymethamphetamine (‘Ecstasy’) in healthy volunteers. J Psychopharmacol 14(3):269–274. https://doi.org/10.1177/026988110001400313
Liechti ME, Vollenweider FX (2000b) Acute psychological and physiological effects of MDMA (“Ecstasy”) after haloperidol pretreatment in healthy humans. Eur Neuropsychopharmacol 10(4):289–295. https://doi.org/10.1016/S0924-977X(00)00086-9
Liu Y, She M, Wu Z, Dai R (2011) The inhibition study of human UDP-glucuronosyltransferases with cytochrome P450 selective substrates and inhibitors. J Enzyme Inhib Med Chem 26(3):386–393. https://doi.org/10.3109/14756366.2010.518965
Lyon RA, Glennon RA, Titeler M (1986) 3,4-Methylenedioxymethamphetamine (MDMA): Stereoselective interactions at brain 5-HT1 and 5-HT2 receptors. Psychopharmacology 88(4):525–526. https://doi.org/10.1007/BF00178519
Malcolm B, Thomas K (2021) Serotonin toxicity of serotonergic psychedelics. Psychopharmacology. https://doi.org/10.1007/s00213-021-05876-x
Manevski N, Kurkela M, Höglund C, Mauriala T, Court MH, Yli-Kauhaluoma J, Finel M (2010) Glucuronidation of psilocin and 4-hydroxyindole by the human UDP-glucuronosyltransferases. Drug Metabolism and Disposition: the Biological Fate of Chemicals 38(3):386–395. https://doi.org/10.1124/dmd.109.031138
Mattusek, N., & Halbach, A. (1964). Über den Einfluss von Ergotamin auf die LSD-"Psychose" beim Menschen. [On the Influence of Ergotamine on LSD-"Psychosis" in Man]. In Psychopharmacologia (Vol. 5, pp. 158–160). https://doi.org/10.1007/BF00413052
McCann UD, Ricaurte GA (1993) Reinforcing subjective effects of (+/-) 3,4-methylenedioxymethamphetamine (“ecstasy”) may be separable from its neurotoxic actions: Clinical evidence. J Clin Psychopharmacol 13(3):214–217
Mitchell JM, Bogenschutz M, Lilienstein A, Harrison C, Kleiman S, Parker-Guilbert K, G, M. O., Garas, W., Paleos, C., Gorman, I., Nicholas, C., Mithoefer, M., Carlin, S., Poulter, B., Mithoefer, A., Quevedo, S., Wells, G., Klaire, S. S., van der Kolk, B., … Doblin, R. (2021) MDMA-assisted therapy for severe PTSD: A randomized, double-blind, placebo-controlled phase 3 study. Nat Med 27(6):1025–1033. https://doi.org/10.1038/s41591-021-01336-3
Mithoefer MC, Grob CS, Brewerton TD (2016) Novel psychopharmacological therapies for psychiatric disorders: Psilocybin and MDMA. The Lancet Psychiatry 3(5):481–488. https://doi.org/10.1016/S2215-0366(15)00576-3
Mithoefer MC, Wagner MT, Mithoefer AT, Jerome L, Doblin R (2011) The safety and efficacy of ±3,4-methylenedioxymethamphetamine-assisted psychotherapy in subjects with chronic, treatment-resistant posttraumatic stress disorder: The first randomized controlled pilot study. Journal of Psychopharmacology (oxford, England) 25(4):439–452. https://doi.org/10.1177/0269881110378371
Morefield KM, Keane M, Felgate P, White JM, Irvine RJ (2011) Pill content, dose and resulting plasma concentrations of 3,4-methylendioxymethamphetamine (MDMA) in recreational ‘ecstasy’ users. Addiction 106(7):1293–1300. https://doi.org/10.1111/j.1360-0443.2011.03399.x
Moreno FA, Wiegand CB, Taitano EK, Delgado PL (2006) Safety, tolerability, and efficacy of psilocybin in 9 patients with obsessive-compulsive disorder. J Clin Psychiatry 67(11):1735–1740. https://doi.org/10.4088/jcp.v67n1110
Müller F, Holze F, Dolder P, Ley L, Vizeli P, Soltermann A, Liechti ME, Borgwardt S (2021) MDMA-induced changes in within-network connectivity contradict the specificity of these alterations for the effects of serotonergic hallucinogens. Neuropsychopharmacology 46(3):545–553. https://doi.org/10.1038/s41386-020-00906-2
Multidisciplinary Association for Psychedelic Studies. (2021). MAPS MDMA Investigator’s Brochure .
Nardou R, Lewis EM, Rothhaas R, Xu R, Yang A, Boyden E, Dölen G (2019) Oxytocin-dependent reopening of a social reward learning critical period with MDMA. Nature 569(7754):116–120. https://doi.org/10.1038/s41586-019-1075-9
Nayak, S., Gukasyan, N., Barrett, F. S., Erowid, E., Erowid, F., & Griffiths, R. R. (2021). Classic psychedelic coadministration with lithium, but not lamotrigine, is associated with seizures: An analysis of online psychedelic experience reports . PsyArXiv. https://doi.org/10.31234/osf.io/r726d
Nichols DE (2020) Psilocybin: From ancient magic to modern medicine. J Antibiot 73(10):679–686. https://doi.org/10.1038/s41429-020-0311-8
Oeri HE (2021) Beyond ecstasy: Alternative entactogens to 3,4-methylenedioxymethamphetamine with potential applications in psychotherapy. J Psychopharmacol 35(5):512–536. https://doi.org/10.1177/0269881120920420
O’Mathúna B, Farré M, Rostami-Hodjegan A, Yang J, Cuyàs E, Torrens M, Pardo R, Abanades S, Maluf S, Tucker GT, Torre R (2008) The consequences of 3,4-methylenedioxymethamphetamine induced CYP2D6 inhibition in humans. J Clin Psychopharmacol 28(5):523–529. https://doi.org/10.1097/JCP.0b013e318184ff6e
Pacifici R, Pichini S, Zuccaro P, Farré M, Segura M, Ortuño J, Di Carlo S, Bacosi A, Roset PN, Segura J, de la Torre R (2004) Paroxetine Inhibits Acute Effects of 3,4-Methylenedioxymethamphetamine on the Immune System in Humans. J Pharmacol Exp Ther 309(1):285–292. https://doi.org/10.1124/jpet.103.061374
Papaseit E, Pérez-Mañá C, Torrens M, Farré A, Poyatos L, Hladun O, Sanvisens A, Muga R, Farré M (2020) MDMA interactions with pharmaceuticals and drugs of abuse. Expert Opin Drug Metab Toxicol 16(5):357–369. https://doi.org/10.1080/17425255.2020.1749262
Papaseit E, Vázquez A, Pérez-Mañá C, Pujadas M, de la Torre R, Farré M, Nolla J (2012) Surviving life-threatening MDMA (3,4-methylenedioxymethamphetamine, ecstasy) toxicity caused by ritonavir (RTV). Intensive Care Med 38(7):1239–1240. https://doi.org/10.1007/s00134-012-2537-9
Partilla JS, Dempsey AG, Nagpal AS, Blough BE, Baumann MH, Rothman RB (2006) Interaction of Amphetamines and Related Compounds at the Vesicular Monoamine Transporter. J Pharmacol Exp Ther 319(1):237–246. https://doi.org/10.1124/jpet.106.103622
Passie T (2018) The early use of MDMA (‘Ecstasy’) in psychotherapy (1977–1985). Drug Science, Policy and Law 4:2050324518767442. https://doi.org/10.1177/2050324518767442
Penmatsa A, Wang KH, Gouaux E (2013) X-ray structure of dopamine transporter elucidates antidepressant mechanism. Nature 503(7474):85–90. https://doi.org/10.1038/nature12533
Penmatsa A, Wang KH, Gouaux E (2015) X-ray structures of Drosophila dopamine transporter in complex with nisoxetine and reboxetine. Nat Struct Mol Biol 22(6):506–508. https://doi.org/10.1038/nsmb.3029
Pilgrim JL, Gerostamoulos D, Woodford N, Drummer OH (2012) Serotonin toxicity involving MDMA (ecstasy) and moclobemide. Forensic Sci Int 215(1–3):184–188. https://doi.org/10.1016/j.forsciint.2011.04.008
Pokorny T, Preller KH, Kraehenmann R, Vollenweider FX (2016) Modulatory effect of the 5-HT1A agonist buspirone and the mixed non-hallucinogenic 5-HT1A/2A agonist ergotamine on psilocybin-induced psychedelic experience. Eur Neuropsychopharmacol 26(4):756–766. https://doi.org/10.1016/j.euroneuro.2016.01.005
Ramcharan S, Meenhorst P, Otten J, Koks C, de Boer D, Maes R, Beijnen J (1998) Survival After Massive Ecstasy Overdose. J Toxicol Clin Toxicol 36(7):727–731. https://doi.org/10.3109/15563659809162623
Richards JR, Hollander JE, Ramoska EA, Fareed FN, Sand IC, Gómez MMI, Lange RA (2017) β-Blockers, Cocaine, and the Unopposed α-Stimulation Phenomenon. J Cardiovasc Pharmacol Ther 22(3):239–249. https://doi.org/10.1177/1074248416681644
Rickli A, Moning OD, Hoener MC, Liechti ME (2016) Receptor interaction profiles of novel psychoactive tryptamines compared with classic hallucinogens. Eur Neuropsychopharmacol 26(8):1327–1337. https://doi.org/10.1016/j.euroneuro.2016.05.001
Rigg KK, Sharp A (2018) Deaths related to MDMA (ecstasy/molly): Prevalence, root causes, and harm reduction interventions. Journal of Substance Use 23(4):345–352. https://doi.org/10.1080/14659891.2018.1436607
Roseman L, Leech R, Feilding A, Nutt DJ, Carhart-Harris RL (2014) The effects of psilocybin and MDMA on between-network resting state functional connectivity in healthy volunteers. Front Hum Neurosci 8. https://doi.org/10.3389/fnhum.2014.00204
Rudnick G, Wall SC (1992) The molecular mechanism of “ecstasy” [3,4-methylenedioxy-methamphetamine (MDMA)]: Serotonin transporters are targets for MDMA-induced serotonin release. Proc Natl Acad Sci 89(5):1817–1821. https://doi.org/10.1073/pnas.89.5.1817
Saleemi S, Pennybaker SJ, Wooldridge M, Johnson MW (2017) Who is “Molly”? MDMA adulterants by product name and the impact of harm-reduction services at raves. Journal of Psychopharmacology (oxford, England) 31(8):1056–1060. https://doi.org/10.1177/0269881117715596
Schatzberg, A. F., & Nemeroff, C. B. (2017). The American Psychiatric Association Publishing Textbook Of Psychopharmacology . American Psychiatric Pub.
Scherrer, J. F., Salas, J., Cohen, B. E., Schnurr, P. P., Schneider, F. D., Chard, K. M., Tuerk, P., Friedman, M. J., Norman, S. B., van den Berk‐Clark, C., & Lustman, P. J. (2019). Comorbid Conditions Explain the Association Between Posttraumatic Stress Disorder and Incident Cardiovascular Disease. Journal of the American Heart Association: Cardiovascular and Cerebrovascular Disease , 8 (4). https://doi.org/10.1161/JAHA.118.011133
Schmid Y, Rickli A, Schaffner A, Duthaler U, Grouzmann E, Hysek CM, Liechti ME (2015) Interactions between Bupropion and 3,4-Methylenedioxymethamphetamine in Healthy Subjects. J Pharmacol Exp Ther 353(1):102–111. https://doi.org/10.1124/jpet.114.222356
Schmid Y, Vizeli P, Hysek CM, Prestin K, Meyer zu Schwabedissen, H. E., & Liechti, M. E. (2016) CYP2D6 function moderates the pharmacokinetics and pharmacodynamics of 3,4-methylene-dioxymethamphetamine in a controlled study in healthy individuals. Pharmacogenet Genomics 26(8):397–401. https://doi.org/10.1097/FPC.0000000000000231
Scriba, G. K. E. (2011). Sean C. Sweetman (Ed.): Martindale: The Complete Drug Reference. Chromatographia , 74 (7), 647–648. https://doi.org/10.1007/s10337-011-2101-8
Segura M, Farre M, Pichini S, Peiro AM, Roset PN, Ramirez A, Ortuno J, Pacifici R, Zuccaro P, Segura J, de la Torre R (2005) Contribution of Cytochrome P450 2D6 to 3,4-Methylenedioxymethamphetamine Disposition in Humans: Use of Paroxetine as a Metabolic Inhibitor Probe. Clin Pharmacokinet 44(6):649–660. https://doi.org/10.2165/00003088-200544060-00006
Sessa B (2017) MDMA and PTSD treatment: “PTSD: From novel pathophysiology to innovative therapeutics.” Neurosci Lett 649:176–180. https://doi.org/10.1016/j.neulet.2016.07.004
Sessa B, Higbed L, O’Brien S, Durant C, Sakal C, Titheradge D, Williams TM, Rose-Morris A, Brew-Girard E, Burrows S, Wiseman C, Wilson S, Rickard J, Nutt DJ (2021) First study of safety and tolerability of 3,4-methylenedioxymethamphetamine-assisted psychotherapy in patients with alcohol use disorder. J Psychopharmacol 35(4):375–383. https://doi.org/10.1177/0269881121991792
Shulgin AT (1986) The background and chemistry of MDMA. J Psychoactive Drugs 18(4):291–304. https://doi.org/10.1080/02791072.1986.10472361
Silins E, Copeland J, Dillon P (2007) Qualitative Review of Serotonin Syndrome, Ecstasy (MDMA) and the use of Other Serotonergic Substances: Hierarchy of Risk. Aust N Z J Psychiatry 41(8):649–655. https://doi.org/10.1080/00048670701449237
Simmler LD, Hysek CM, Liechti ME (2011) Sex Differences in the Effects of MDMA (Ecstasy) on Plasma Copeptin in Healthy Subjects. J Clin Endocrinol Metab 96(9):2844–2850. https://doi.org/10.1210/jc.2011-1143
Smilkstein MJ, Smolinske SC, Rumack BH (1987) A Case of Mao Inhibitor/MDMA Interaction: Agony After Ecstasy. J Toxicol Clin Toxicol 25(1–2):149–159. https://doi.org/10.3109/15563658708992620
Stein, D. J., & Rink, J. (1999). Effects of “Ecstasy” Blocked by Serotonin Reuptake Inhibitors. The Journal of Clinical Psychiatry , 60 (7), 485–485. https://www.psychiatrist.com/JCP/article/Pages/effects-ecstasy-blocked-serotonin-reuptake-inhibitors.aspx
Steuer AE, Schmidhauser C, Tingelhoff EH, Schmid Y, Rickli A, Kraemer T, Liechti ME (2016) Impact of Cytochrome P450 2D6 Function on the Chiral Blood Plasma Pharmacokinetics of 3,4-Methylenedioxymethamphetamine (MDMA) and Its Phase I and II Metabolites in Humans. PLoS ONE 11(3):e0150955. https://doi.org/10.1371/journal.pone.0150955
Strassman, R. (1992). Human Hallucinogen Interactions with Drugs Affecting Serotonergic. Neuropsychopharmacol , 7 (3).
Studerus, E., Gamma, A., & Vollenweider, F. X. (2010). Psychometric Evaluation of the Altered States of Consciousness Rating Scale (OAV). PLoS ONE , 5 (8). https://doi.org/10.1371/journal.pone.0012412
Studerus E, Vizeli P, Harder S, Ley L, Liechti ME (2021) Prediction of MDMA response in healthy humans: A pooled analysis of placebo-controlled studies. J Psychopharmacol 0269881121998322. https://doi.org/10.1177/0269881121998322
Tancer M, Johanson C-E (2006) The effects of fluoxetine on the subjective and physiological effects of 3,4-methylenedioxymethamphetamine (MDMA) in humans. Psychopharmacology 189(4):565–573. https://doi.org/10.1007/s00213-006-0576-z
Thomas K, Malcolm B, Lastra D (2017) Psilocybin-Assisted Therapy: A Review of a Novel Treatment for Psychiatric Disorders. J Psychoactive Drugs 49(5):446–455. https://doi.org/10.1080/02791072.2017.1320734
Togni LR, Lanaro R, Resende RR, Costa JL (2015) The variability of ecstasy tablets composition in Brazil. J Forensic Sci 60(1):147–151. https://doi.org/10.1111/1556-4029.12584
Usona Institute. (2021). Usona Institute Psilocybin Investigator’s Brochure (Vol. 4).
VA/DOD Clinical Practice Guideline for the Management of Posttraumatic Stress Disorder and Acute Stress Disorder. (2017) 200
Valeriani G, Corazza O, Bersani FS, Melcore C, Metastasio A, Bersani G, Schifano F (2015) Olanzapine as the ideal “trip terminator”? Analysis of online reports relating to antipsychotics’ use and misuse following occurrence of novel psychoactive substance-related psychotic symptoms. Hum Psychopharmacol 30(4):249–254. https://doi.org/10.1002/hup.2431
Vargas AS, Luís Â, Barroso M, Gallardo E, Pereira L (2020) Psilocybin as a New Approach to Treat Depression and Anxiety in the Context of Life-Threatening Diseases—A Systematic Review and Meta-Analysis of Clinical Trials. Biomedicines 8(9):331. https://doi.org/10.3390/biomedicines8090331
Article CAS PubMed Central Google Scholar
Verrico CD, Miller GM, Madras BK (2007) MDMA (Ecstasy) and human dopamine, norepinephrine, and serotonin transporters: Implications for MDMA-induced neurotoxicity and treatment. Psychopharmacology 189(4):489–503. https://doi.org/10.1007/s00213-005-0174-5
Vizeli P, Schmid Y, Prestin K, Schwabedissen MZ, H. E., & Liechti, M. E. (2017) Pharmacogenetics of ecstasy: CYP1A2, CYP2C19, and CYP2B6 polymorphisms moderate pharmacokinetics of MDMA in healthy subjects. European Neuropsychopharmacology: the Journal of the European College of Neuropsychopharmacology 27(3):232–238. https://doi.org/10.1016/j.euroneuro.2017.01.008
Vollenweider, F. X., Vollenweider-Scherpenhuyzen, M. F. I., Bäbler, A., Vogel, H., & Hell, D. (1998). Psilocybin induces schizophrenia-like psychosis in humans via a serotonin-2 agonist action: NeuroReport , 9 (17), 3897–3902. https://doi.org/10.1097/00001756-199812010-00024
Vuori E, Henry JA, Ojanperä I, Nieminen R, Savolainen T, Wahlsten P, Jäntti M (2003) Death following ingestion of MDMA (ecstasy) and moclobemide: Fatal MDMA-moclobemide interaction. Addiction 98(3):365–368. https://doi.org/10.1046/j.1360-0443.2003.00292.x
Wagner MT, Mithoefer MC, Mithoefer AT, MacAulay RK, Jerome L, Yazar-Klosinski B, Doblin R (2017) Therapeutic effect of increased openness: Investigating mechanism of action in MDMA-assisted psychotherapy. Journal of Psychopharmacology (oxford, England) 31(8):967–974. https://doi.org/10.1177/0269881117711712
White TL, Justice AJH, de Wit H (2002) Differential subjective effects of D-amphetamine by gender, hormone levels and menstrual cycle phase. Pharmacol Biochem Behav 73(4):729–741. https://doi.org/10.1016/s0091-3057(02)00818-3
Wood DM, Stribley V, Dargan PI, Davies S, Holt DW, Ramsey J (2011) Variability in the 3,4-methylenedioxymethamphetamine content of “ecstasy” tablets in the UK. Emergency Medicine Journal: EMJ 28(9):764–765. https://doi.org/10.1136/emj.2010.092270
Yockey A, King K (2021) Use of psilocybin (“mushrooms”) among US adults: 2015–2018. Journal of Psychedelic Studies 5(1):17–21. https://doi.org/10.1556/2054.2020.00159
Download references
Acknowledgements
The authors thank Paul Bonney and Jason Jarria for assisting in abstract review. Salary support for CSS provided by the Department of Veterans Affairs, Clinical Science Research & Development, Federal Award Identification Number IK2CX001495.
Author information
Authors and affiliations.
Department of Psychiatry, Oregon Health & Science University, Portland, OR, 97239, USA
Aryan Sarparast & Christopher S. Stauffer
College of Pharmacy, Touro University California, Vallejo, CA, 94592, USA
Kelan Thomas
Awake Movement LLC, Chino Hills, CA, USA
Benjamin Malcolm
Department of Mental Health, VA Portland Health Care System, Portland, OR, 97239, USA
Christopher S. Stauffer
You can also search for this author in PubMed Google Scholar
Corresponding author
Correspondence to Christopher S. Stauffer .
Ethics declarations
Conflict of interest.
CSS has received payment from the MAPS Public Benefit Corporation for Training clinicians in MAP. BM runs www.spiritpharmacist.com , which offers a monthly membership service for access to drug interactions guides, courses, and consultation services. AS and KT have no conflicts of interest to report.
Additional information
Publisher’s note.
Springer Nature remains neutral with regard to jurisdictional claims in published maps and institutional affiliations.
This article belongs to a Special Issue on Psychopharmacology on Psychedelic Drugs
Supplementary Information
Below is the link to the electronic supplementary material.
Supplementary file1 (DOCX 557 kb)
Rights and permissions.
Reprints and permissions
About this article
Sarparast, A., Thomas, K., Malcolm, B. et al. Drug-drug interactions between psychiatric medications and MDMA or psilocybin: a systematic review. Psychopharmacology 239 , 1945–1976 (2022). https://doi.org/10.1007/s00213-022-06083-y
Download citation
Received : 04 October 2021
Accepted : 03 February 2022
Published : 07 March 2022
Issue Date : June 2022
DOI : https://doi.org/10.1007/s00213-022-06083-y
Share this article
Anyone you share the following link with will be able to read this content:
Sorry, a shareable link is not currently available for this article.
Provided by the Springer Nature SharedIt content-sharing initiative
- N-Methyl-3,4-methylenedioxyamphetamine
- Hallucinogens
- Drug interactions
- Stress disorders, Post-traumatic
- Serotonin uptake inhibitors
- Psychopharmacology
- Find a journal
- Publish with us
- Track your research
Opinion Why prescription drugs cost so much
In their April 9 op-ed, “ Big Pharma’s high prices don’t drive innovation ,” Avik Roy and Gregg Girvan argued that Medicare’s new authority to demand lower prices from drug manufacturers will not result in the development and approval of fewer new drugs. Because most biotech innovation comes from smaller firms, they wrote, reducing revenue to Big Pharma will have minimal impact on drug creation.
No one contests that most pharmaceutical innovation begins with start-ups, often working on a single promising compound. But the ability of these start-ups to attract essential investment is inseparably tied to the lifetime revenue a successful new medication will generate. The business model of most start-up biotech companies is not to become the next Pfizer. Rather, it’s to develop a new drug to the point at which a major player in the market deems an acquisition or partnership with the developer worthwhile.
Start-ups have no expertise in shepherding a drug through what Mr. Roy and Mr. Girvan acknowledged are extensive clinical trials and a complex Food and Drug Administration approval process — or in manufacturing and marketing. Big firms do, and their expertise in navigating those processes matters. The potential revenue stream from a new drug, which price controls would diminish, determines the value of a start-up in an acquisition or partnership. That potential value, in turn, drives the calculations of venture investors — without whom innovation cannot proceed.
In reaching their sanguine conclusion, Mr. Roy and Mr. Girvan underemphasized these essential steps and the benefits that come from an ecosystem that includes both small, nimble start-ups and larger firms that can navigate the process of making sure drugs are safe and effective before bringing them to market.
Indeed, the evidence-based economic analysis I led with my University of Chicago colleagues — which predicted the loss of 135 drugs over the next two decades and an additional cut in 109 post-approval FDA indications — now appears to have been too conservative. Dozens of publicly traded biopharmaceutical firms have announced pipeline impacts on earning calls, blaming the Inflation Reduction Act. Such earning call announcements face criminal penalties if they turn out to be false.
Tomas J. Philipson , Chicago
The writer is Daniel Levin professor of public policy studies emeritus at the University of Chicago and a former member and acting chairman of the White House Council of Economic Advisers.
Avik Roy and Gregg Girvan’s op-ed explained why Big Pharma’s excessive drug prices are not a result of their investment in the development of new drugs. What the piece neglected to mention, and what similar articles also ignore, are the pharmaceutical industry’s huge expenditures on advertising, which do help explain those prices. In 2023, pharmaceutical companies spent more money on advertising than any other industry except for packaged consumer goods, more even than the tech companies that previously occupied second place. That annual spending rose $17 billion in the past decade — from $1 billion annually in 2013 to $18 billion last year . The United States and New Zealand are the only industrialized countries that allow companies to advertise specific prescription drugs directly to consumers. Why? Is there any public health gain to be made from being told over and over that we should ask our doctor to prescribe something with an exorbitant price tag that we might or might not need? I suspect that drug prices, as well as demand, would decrease significantly if our government joined other countries with much lower drug prices in prohibiting this barrage of advertising.
Barbara E. Taylor , Arlington
When discussing prescription drug pricing, it’s worth looking at how Albert Bourla, the head of Pfizer, described the process in his book about the development of the coronavirus vaccine, “Moonshot.” He wrote that the algorithm used to put a cost on drugs determines the cost savings in medical expenses to the government and society from the benefits of the drug. For example, if drug trials reveal that the average result of taking the drug is a patient avoiding a lengthy hospital stay, then the price of the drug should be equivalent to the cost of that stay. Mr. Bourla wrote that maintaining control of intellectual property and reaping its benefits are key to encouraging continued innovation. Because U.S. medical costs have risen rather than fallen, so, too, have the prices of drugs during those initial patent periods. This approach should be subject to more scrutiny if we, as a society, want to address drug pricing.
John Durning , Bowie
I enjoyed Avik Roy and Gregg Girvan’s op-ed on drug prices and innovation with a focus on small companies that are the force driving research and development of new drugs.
But I think two of their assumptions about the relationship between prices and innovation are mistaken. The first regards how investment decisions are made. Investors make the decision as to which drugs to bring forward based on a present value calculation, which takes into account market size, market price and other factors — such as project risk based on similar classes of drugs that have been tested in the past. If market prices are negotiated downward, present values fall and drugs that are on the bubble might not be funded. It is heartbreaking when a good drug idea fails because there is no business in developing and manufacturing it.
The larger problem with Mr. Roy and Mr. Girvan’s piece is that they imply that drug prices are unfairly high. Yes, there are individual cases of price gouging — the 2015 decision by a drug company to charge $750 per pill for pyrimethamine, an old, generic drug used to treat toxoplasmosis and malaria, is an excellent example. But prescription drug prices have made up a fairly stable share of health care spending for years.
This is not news to politicians who have been leery of lowering drug prices because they know that killing the goose that creates lifesaving drugs is not a winning idea at the polls. Patient interest groups vote, and they remember who helped bring treatments to them and who did not. And so do investors.
Stuart Gallant , Belmont, Mass.
Focus on patients, not profits
Regarding the April 6 front-page article “ Algorithm impairs care, senior-home staffers say ”:
Thank you for your excellent article about how for-profit health care affects health-care quality. The incentives are aligned toward profit and not toward quality of care.
Ten years ago, my husband was admitted to a memory care unit in Columbia, Md. The cost was $7,000 per month. He had been there 10 days when I found urine burns and a sacral bedsore. I brought him home and cared for him myself. He lived for six more months and died without bedsores or urine burns.
If the stockholders are winning, the patients are losing.
Pam Foster , Ellicott City
Not only do the nursing home algorithms such as the ones described in The Post on April 6 fail to provide adequate staffing for discrete resident care tasks; but they also fail to consider the importance of allowing time for the staff to chat with the residents and treat them as actual people. No wonder people are reluctant to move into assisted-living facilities. It’s self-defeating to chase efficiency at the expense of a larger business or of human decency.
Cathy Winer , Washington
The Medicaid purge hits immigrant families
Many thanks to Post columnist Catherine Rampell for a candid look at the brutal reality of Medicaid “unwinding” in her April 6 op-ed “ The Great Medicaid Purge was even worse than expected. ” From a health equity perspective, the news is catastrophic.
Last year, 18 percent of lawfully present immigrants in the United States and 6 percent of naturalized citizens lacked health insurance ; 8 percent of U.S.-born citizens were uninsured. Immigrant families are more likely to be uninsured and have unmet health-care needs than all-citizen families. And as states resumed assessing the eligibility of Medicaid recipients after a pause during the pandemic, those immigrant families faced additional hurdles to staying on the rolls, ranging from worries about translation to concerns about future immigration consequences.
The research conducted by our coalition shows those warnings were largely ignored by the states, with serious consequences for the insurance rates of immigrants. Advocates reported that most states failed to clarify that the verification process would not endanger future immigration applications and to make reenrollment processes available in the languages immigrant families read and speak. Unsurprisingly, a survey of Spanish speakers in immigrant families found two-thirds of them knew little or nothing about reenrollment requirements, most had heard misinformation about Medicaid, and many had persistent immigration-related questions that would deter them from applying.
We’re not talking about undocumented immigrants here, though we strongly believe everyone should be able to get the care they need. Millions of people in immigrant families qualify for Medicaid under federal law, as U.S. citizens with immigrant family members or as lawfully present immigrants themselves.
But because of the poor policy choices Ms. Rampell detailed, as well as the additional failures specific to immigrant families, millions of eligible people — largely families of color — lost Medicaid. Our national and state leaders must not tolerate this abject failure. And if they do, we must find new leaders.
Adriana Cadena , El Paso
The writer is director of Protecting Immigrant Families.
About letters to the editor
The Post welcomes letters to the editor on any subject, especially those that expand upon the ideas raised by published pieces and those that raise valuable questions about The Post’s practices and choices. Letters should run no more than 400 words, be submitted only to the Post and must be published under your real name. Submit a letter .
- Opinion | The world’s best hope for survival? Saving us from ourselves. April 17, 2024 Opinion | The world’s best hope for survival? Saving us from ourselves. April 17, 2024
- Opinion | Tradwives, meet trad guys April 16, 2024 Opinion | Tradwives, meet trad guys April 16, 2024
- Opinion | Did Oregon’s drug decriminalization experiment have to end this way? April 15, 2024 Opinion | Did Oregon’s drug decriminalization experiment have to end this way? April 15, 2024

The independent source for health policy research, polling, and news.
Medicare Part D in 2024: A First Look at Prescription Drug Plan Availability, Premiums, and Cost Sharing
Juliette Cubanski and Anthony Damico Published: Nov 08, 2023
During the Medicare open enrollment period from October 15 to December 7 each year, people with Medicare can enroll in a plan that provides Part D prescription drug coverage , either a stand-alone prescription drug plan (PDP) for people in traditional Medicare, or a Medicare Advantage plan that covers all Medicare benefits, including prescription drugs (MA-PD). In 2023, 50.5 million of the 66 million people covered by Medicare are enrolled in Part D plans, with more than half (56%) enrolled in MA-PDs and 44% in PDPs. This issue brief provides an overview of Part D plan availability and premiums in 2024 and key trends over time. (An overview of the 2024 Medicare Advantage market is also available.) ( See Methods box for details on the analysis).
Part D Highlights for 2024
- The average Medicare beneficiary has a choice of close to 60 Medicare plans with Part D drug coverage in 2024 , including 21 Medicare stand-alone drug plans and 36 Medicare Advantage drug plans. While the market for Part D coverage overall remains robust, the number of PDP options for 2024 is lower and the number of MA-PD options is higher than in any other year since Part D started. The total number of PDPs (709) and firms offering these plans (11) have decreased from 2023 .
- Medicare beneficiaries who receive Part D Low-Income Subsidies (LIS) will have access to fewer so-called “benchmark” PDPs in 2024 than in any year since Part D started, with three benchmark plans available out of the average 21 PDPs available overall for 2024. Benchmark plans are PDPs available to LIS enrollees for no monthly premium. The reduction in the number of benchmark plans for 2024 is largely the result of PDPs offered by Cigna, Humana, and CVS Health qualifying as benchmark plans in far fewer regions in 2024 compared to 2023. An estimated 2.4 million LIS enrollees – half of all LIS enrollees in PDPs – need to switch plans during the 2023 open enrollment period if they want to be enrolled in a benchmark plan in 2024.
- Although the Inflation Reduction Act included a premium stabilization provision that capped annual growth in the Part D base beneficiary premium at 6%, the law did not apply this 6% cap to individual plan premiums that enrollees pay. The Part D base beneficiary premium of $34.70 for 2024 is based on standardized bids submitted by PDPs and MA-PDs to cover basic Part D benefits in 2024, while actual Part D plan premiums vary across plans and may be higher or lower than the base beneficiary premium, depending on several factors. The estimated average enrollment-weighted monthly premium for Medicare Part D stand-alone drug plans is projected to be $48 in 2024, based on current enrollment, up 21% from $40 in 2023 . This increase is driven by higher expected plan costs to provide the Part D benefit in 2024, including a new cap on enrollees’ out-of-pocket spending above the catastrophic threshold rather than requiring them to pay 5% coinsurance, as in prior years. After accounting for enrollment choices by new enrollees and plan changes by current enrollees, the actual average weighted PDP premium for 2024 is likely to be lower than the estimated weighted average of $48.
- Monthly premiums for drug coverage are substantially higher for PDPs compared to MA-PDs – five times higher, on average, in 2024 (based on unweighted amounts). While the average premium is projected to increase between 2023 and 2024 for PDPs, it is expected to remain stable (and low, or even zero) for MA-PDs. MA-PD sponsors can use rebate dollars from Medicare payments to lower or eliminate their Part D premiums , but there is no equivalent rebate system for PDPs.
- Average monthly premiums for the 14 national PDPs are projected to range from under $1 to $108 in 2024 . Premium variation across plans is in part related to whether plans offer basic or enhanced benefits and the value of benefits offered, as well as variation in the underlying costs that plans incur for their enrollees. Among the national PDPs, average monthly premiums are increasing for 12 PDPs, including 4 PDPs with increases greater than $20 and 3 with increases between $10 and $20.
- Most PDP enrollees will face much higher cost sharing for brands than for generic drugs in 2024, as in prior years, including coinsurance for non-preferred drugs of 50% (the maximum coinsurance rate allowed for the non-preferred drug tier) in 6 of the 14 national PDPs . PDP enrollees in 9 of the 14 national PDPs will also face coinsurance, rather than copays, for preferred brands, ranging from 15% to 25%, and coinsurance for specialty tier drugs ranging from 25% in 7 of the national PDPs to 33% in 2 national PDPs. Coinsurance can mean less predictable out-of-pocket costs than copayments. In a change from prior years, however, beneficiaries in 2024 will no longer be required to pay 5% coinsurance once they qualify for catastrophic coverage, due to a provision in the Inflation Reduction Act that eliminated this cost-sharing requirement.
Part D Plan Availability
For 2024, the average medicare beneficiary has fewer stand-alone drug plan options than in prior years but more medicare advantage drug plan options.
The Part D market for 2024 offers the average Medicare beneficiary fewer choices for drug coverage from stand-alone prescription drug plans than in prior years but more choices for coverage from Medicare Advantage drug plans. The average Medicare beneficiary has a choice of close to 60 options for Part D coverage in 2024, including 21 PDPs and 36 MA-PDs (Figure 1). Since 2020, the number of PDPs available to the average beneficiary has decreased by 25% while the number of MA-PDs has increased by 57%.
Of the 21 PDPs available to the average beneficiary in 2024, 14 are national PDPs – that is, available in all 34 PDP regions nationwide ( Appendix Table 1). This is a reduction of two national PDPs from 2023, the result of one plan sponsor (Elixir Insurance) pulling out of the Part D market entirely and an AARP-branded PDP sponsored by UnitedHealthcare no longer being offered in all 34 regions in 2024. The 270,000 enrollees in Elixir’s PDPs (as of March 2023) will need to select a new Part D plan from a different plan sponsor during the 2023 open enrollment period if they want their Part D coverage to continue in 2024.
A Total of 709 Medicare Part D Stand-Alone Prescription Drug Plans Will Be Offered by 11 Firms in 2024, the Lowest Number of PDPs and Firms Offering These Plans Since Part D Started
In 2024, a total of 709 PDPs will be offered by 11 firms in the 34 PDP regions (plus another 10 PDPs in the territories), a decrease of 92 PDPs (-11%) from 2023, and the lowest number of PDPs available in any year since Part D started in 2006 (Figure 2). The number of firms sponsoring stand-alone drug plans is decreasing from 15 firms in 2023 to 11 firms in 2024, the smallest number since the Medicare benefit was launched in 2006.
Despite the reduction in PDP availability overall, beneficiaries in each state will have a choice of multiple PDPs, ranging from 15 PDPs in New York to 24 PDPs in Alabama and Tennessee, plus multiple MA-PDs offered at the local level (Figure 3, Appendix Table 2).
Although the Inflation Reduction Act included a premium stabilization provision that capped annual growth in the Part D base beneficiary premium at 6% beginning in 2024, the base beneficiary premium is not the same as the amount that Part D enrollees pay for coverage, and the law did not cap the growth in individual plan premiums to 6%. The Part D base beneficiary premium of $34.70 for 2024 is based on standardized bids submitted by PDPs and MA-PDs to cover basic Part D benefits in 2024. (Absent the premium stabilization provision, the 2024 base beneficiary premium would have increased by 20% to $39.35, reflecting a higher average plan bid for offering Part D coverage in 2024 compared to 2023 .) Actual Part D plan premiums for 2024 vary across plans, may be higher or lower than the base beneficiary premium, and may be increasing by more or less than 6% (or even decreasing).
The estimated national average monthly PDP premium is projected to be $48 in 2024, a 21% increase from $40 in 2023, weighted by June 2023 enrollment. This higher average projected premium is driven by higher expected plan costs to provide the Part D benefit in 2024, including a new cap on enrollees’ out-of-pocket spending above the catastrophic threshold rather than requiring them to pay 5% coinsurance, as in prior years. This change is based on a provision in the Inflation Reduction Act.
Comparing monthly premiums for Part D coverage between stand-alone PDPs and MA-PDs shows the competitive advantage that MA-PDs have over PDPs when it comes to the premiums that enrollees pay for drug coverage. On an unweighted basis, monthly premiums for drug coverage are substantially higher for PDPs compared to MA-PDs – five times higher, on average in 2024 ($60 vs. $12) (Figure 4). Moreover, between 2023 and 2024, the unweighted average premium is increasing for PDPs, while remaining stable for MA-PDs. MA-PD sponsors can use rebate dollars from Medicare payments to lower or eliminate their Part D premiums and/or offer other extra benefits, but there is no equivalent rebate system for PDPs. According to MedPAC, Medicare Advantage monthly rebates per enrollee have more than doubled over the last five years, from $95 in 2018 to $196 in 2023 .
It is likely that, after accounting for enrollment choices by new enrollees and plan changes by current enrollees, the actual average weighted PDP premium for 2024 will be lower than the estimated weighted average of $48 but well above the average MA-PD premium. In 2023, the enrollment-weighted average monthly portion of the premium for drug coverage in MA-PDs is $10, compared to $40 for PDPs.
Average Monthly Premiums for the 14 National PDPs Are Projected to Range from Less Than $1 to $108 in 2024
PDP premiums will vary widely across plans in 2024, as in previous years. Among the 14 national PDPs, there is a difference of more than $1,200 in average annual premiums between the highest-premium PDP and the lowest-premium PDP. At the high end, the monthly premium for Humana Premier Rx Plan (the 10 th largest plan by overall enrollment) will be $108, totaling nearly $1,300 annually. At the low end, the monthly premium for Wellcare Value Script (the second largest plan) will average $0.40, or $5 annually (Figure 5). In addition to Humana Premier Rx Plan, two other national PDPs will charge monthly premiums of more than $100 in 2024: AARP Medicare Rx Preferred, the fourth largest plan ($106), and CVS Health’s SilverScript Plus, the 12 th largest plan ($103).
Two-Thirds of Part D Stand-alone Drug Plan Enrollees Without Low-income Subsidies Will Pay Higher Premiums in 2024 If They Stay in Their Current Plan
Two-thirds of Part D stand-alone plan enrollees (66%) – 8.6 million of the 12.9 million Part D PDP enrollees who are responsible for paying the entire premium (which excludes Low-Income Subsidy (LIS) recipients) – will see their monthly premium increase in 2024 if they stay in their current plan, while 4.4 million (34%) will see a premium reduction if they stay in their current plan (Figure 6).
Compared to 2023, more Medicare Part D stand-alone drug plan enrollees will see their monthly premium either increase or decrease by $10 or more if they stay in their same plan in 2024 (Figure 7). For 2024, 4.8 million non-LIS PDP enrollees (37%) will see a premium increase of $10 or more per month – or at least $120 more annually if they remain in their current plan – compared to 2.1 million enrollees (16%) in 2023.
Among the 14 national PDPs, average monthly premiums are increasing for 12 PDPs, including 7 PDPs (having a combined 3.9 million non-LIS enrollees) with increases exceeding $10:
- AARP Medicare Rx Walgreens (+$30, from $30 to $60)
- SilverScript Plus (+$29, from $74 to $103)
- Humana Premier Rx (+$25, from $83 to $108)
- Cigna Extra Rx (+$22, from $63 to $85)
- SilverScript Choice (+$16, from $33 to $49)
- Cigna Secure Rx (+$14, from $33 to $47)
- Humana Basic Rx Plan (+$14, from $37 to $51)
Another 1.6 million non-LIS PDP enrollees (12%) will see a monthly premium reduction of $10 or more for 2024, compared to under 150,000 (1%) for 2023. This largely reflects a premium reduction for the second largest PDP, Wellcare Value Script, where the average monthly premium will decrease by $9, from $10 in 2023 to less than $1 in 2024. These amounts are averaged over the 34 PDP regions; enrollees in this plan in 16 of the 34 regions will see a premium reduction of $10 or more.
Over half (56%) of non-LIS enrollees (7.3 million) are projected to pay monthly premiums of at least $40 if they stay in their current plans, or nearly $500 annually, including 2.1 million (16% of non-LIS enrollees) projected to pay monthly premiums of at least $100, or at least $1,200 annually. These estimates are higher than the comparable estimates for 2023, when 40% of non-LIS PDP enrollees (5.3 million) were projected to pay at least $40 per month, including 1.6 million paying $100 or more, if they stayed in their plans.
- Cost Sharing
Part D Enrollees Pay Much Higher Cost Sharing for Brands and Non-preferred Drugs Than for Generic-Tier Drugs, and a Mix of Copays and Coinsurance for Different Formulary Tiers
In 2024, as in prior years, Part D enrollees will face much higher cost-sharing amounts for brands and non-preferred drugs (which can include both brands and generics) than for drugs on a generic tier, and a mix of copayments and coinsurance for different formulary tiers. The typical five-tier formulary design in Part D includes tiers for preferred generics, generics, preferred brands, non-preferred drugs, and specialty drugs.
Among all PDPs, median standard cost sharing in 2024 for different types of drugs is (Figure 8):
- Generics : $0 for preferred generics and $5 for other generics
- Preferred brands : a copayment of $47 or coinsurance of 21% for preferred brands (up from $44/17% in 2023)
- Non-preferred drugs : 46% coinsurance for non-preferred drugs, which can include both brands and generics (an increase from 45% in 2023; the maximum allowed is 50%)
- Specialty drugs : 25% coinsurance for specialty drugs (the same as in 2023; the maximum allowed is 33%)
Among the 14 national PDPs, 9 PDPs will charge $0 for preferred generics in 2024, but copays of $40 to $47 or coinsurance of 16% to 25% for preferred brands, and coinsurance ranging from 39% to 50% for non-preferred drugs; 6 out of the 14 national PDPs are charging the maximum 50% coinsurance for non-preferred drugs. Coinsurance for specialty tier drugs ranges from 25% to 33% in these plans, with 7 of the 14 national PDPs charging 25% and 2 charging 33%. (Plans that charge the full deductible amount cannot charge more than 25% for specialty tier drugs.)
Low-Income Subsidy Plan Availability
In 2024, a smaller number of part d stand-alone drug plans will be premium-free to enrollees receiving the low-income subsidy than in any year since part d started.
Through the Part D LIS program, enrollees with low incomes and modest assets are eligible for assistance with Part D plan premiums and cost sharing. More than 13 million Part D enrollees are receiving LIS, including 8.3 million (62%) in MA-PDs and 5.2 million (38%) in PDPs.
In 2024, a smaller number of PDPs will be premium-free benchmark plans – that is, PDPs available for no monthly premium to Medicare Part D enrollees receiving the Low-Income Subsidy (LIS) – than in any year since Part D started, with 126 premium-free benchmark plans, or less than 20% of all PDPs in 2024 (Figure 9). The number of benchmark plans available in 2024 will vary by region, from two to seven ( Appendix Table 2).
PDPs offering basic benefits qualify to be benchmark plans if they have premiums below the benchmark amount in a given region. The benchmark is calculated as a weighted average of the beneficiary premiums for basic drug coverage offered by both PDPs and MA-PDs in a given region (calculated before taking MA rebates into account). (MA-PD premiums are included in this calculation even though MA-PDs do not qualify as benchmark plans.)
The reduction in the number of benchmark plans for 2024 is largely the result of PDPs offered by Cigna, Humana, and CVS Health qualifying as benchmark plans in far fewer regions in 2024 compared to 2023: Cigna Secure Rx (from 34 down to 16 regions); Humana Basic Rx Plan (from 27 down to 11 regions); and CVS Health’s SilverScript Choice (from 34 down to 11 regions).
On average (weighted by 2023 Medicare enrollment), LIS beneficiaries have three benchmark plans available to them out of the average 21 PDPs available overall for 2024 – the lowest average number of benchmark plan options in any year since Part D started. All LIS enrollees can select any plan offered in their area, but if they enroll in a non-benchmark plan, they must pay some portion of their chosen plan’s monthly premium.
In 2024, half (49%) of all LIS PDP enrollees who are eligible for premium-free Part D coverage (2.4 million LIS enrollees) will pay Part D premiums averaging $15 per month unless they switch or are reassigned by CMS to premium-free plans. Among this group are the 1.9 million LIS enrollees in Cigna Secure Rx, Humana Basic Rx Plan, or CVS Health’s SilverScript Choice in the regions where these PDPs will no longer qualify as benchmark plans. These enrollees will need to switch plans for 2024 if they want to remain in a benchmark (premium-free) plan.
The overall market for Part D coverage remains robust based on the overall number of plan options, but recent years have seen a growing divide in the Part D plan market between stand-alone PDPs, where the number of plans has generally been trending downward over time in conjunction with a reduction in PDP enrollment, and MA-PDs, where plan availability and enrollment have experienced steady growth.
The average weighted monthly premium for PDPs in 2024 will increase substantially over the 2023 amount (based on current enrollment), while premiums for drug coverage offered by MA-PDs are likely to remain stable (and low or zero). Provisions in the Inflation Reduction Act to make the Part D benefit more generous – such as the elimination of the 5% coinsurance requirement for catastrophic coverage taking effect in 2024 – will help lower out-of-pocket costs for enrollees, but with these changes, it could become harder for some Part D plan sponsors to offer low-priced coverage, particularly sponsors of stand-alone drug plans. MA-PD sponsors have a competitive advantage in this regard because they can use rebate dollars from Medicare payments to lower or eliminate their Part D premiums . The premium imbalance between PDPs and MA-PDs could be exacerbated as plans assume greater liability for high drug costs above the catastrophic threshold in 2025 with a $2,000 cap on out-of-pocket spending. To keep Part D premiums low in the face of rising costs for the basic Part D benefit, Medicare Advantage plans may end up using more of their rebate dollars to buy down the Part D premium. This could mean less rebate money available for other benefit enhancements or lower profits, depending on the amount of rebates plans receive in the future.
The increasing availability of low- or zero-premium MA-PDs, combined with the aggressive marketing of Medicare Advantage plans and the appeal of other features of these plans, such as supplemental benefits, could tilt enrollment even more towards Medicare Advantage plans in the future. Monitoring trends in Part D plan availability and enrollment could inform policymakers in considering whether or how to ensure continued availability of competitively priced stand-alone Part D drug plans for the millions of Medicare beneficiaries in traditional Medicare.
Juliette Cubanski is with KFF. Anthony Damico is an independent contractor.
Appendix Tables
- Medicare Part D
- Medicare Advantage
- Prescription Drugs
news release
- With Medicare Open Enrollment Underway, Beneficiaries Typically Will Have a Choice of 43 Medicare Advantage Plans for 2024, Consistent with 2023 But More than Double The Number From 2018
Also of Interest
- An Overview of the Medicare Part D Prescription Drug Benefit
- Changes to Medicare Part D in 2024 and 2025 Under the Inflation Reduction Act and How Enrollees Will Benefit
- Key Facts About Medicare Part D Enrollment and Costs in 2023
- Medicare Advantage in 2023: Enrollment Update and Key Trends

An official website of the United States government
The .gov means it’s official. Federal government websites often end in .gov or .mil. Before sharing sensitive information, make sure you’re on a federal government site.
The site is secure. The https:// ensures that you are connecting to the official website and that any information you provide is encrypted and transmitted securely.
- Publications
- Account settings
Preview improvements coming to the PMC website in October 2024. Learn More or Try it out now .
- Advanced Search
- Journal List
- HHS Author Manuscripts

The Changing Opioid Crisis: development, challenges and opportunities
Nora d. volkow.
1 National Institute on Drug Abuse, Bethesda, MD 20892
Carlos Blanco
The current opioid epidemic is one of the most severe public health crisis in US history. Responding to it has been difficult due to its rapidly changing nature and the severity of its associated outcomes. This review examines the origin and evolution of the crisis, the pharmacological properties of opioids, the neurobiology of opioid use and opioid use disorder (OUD), medications for opioid use disorder (MOUD), and existing and promising approaches to prevention. The results of the review indicate that the opioid epidemic is a complex, evolving phenomenon that involves neurobiological vulnerabilities and social determinants of health. Successfully addressing the epidemic will require advances in basic science, development of more acceptable and effective treatments, and implementation of public health approaches, including prevention. The advances achieved in addressing the current crisis should also serve to advance the science and treatment of other substance use disorders.
Origins and Evolution of the Crisis
The current opioid epidemic is one of the most severe public health crisis in US history. Providing an effective response has been difficult because of its changing nature, geographic and demographic diversity, multiplicity of its causes, and the severity of adverse outcomes associated with opioid use and opioid use disorder (OUD). Furthermore, opioid analgesics, which fueled the origins of the opioid epidemic, are therapeutically beneficial when used properly. This, compounds the difficulties of regulating their availability because they cannot be banned, in contrast with illicit drugs. While earlier phases (i.e., first wave) of the crisis were predominantly driven by non-medical use and addiction to prescription opioid analgesics, heroin (second wave) and subsequently illicit synthetic opioids (third wave) have become progressively important as the crisis progressed and more recently there is emerging evidence of increasing fatalities associated with the combination of psychostimulant drugs with opioids (fourth wave). These changes explain why despite the decreases in the number of opioid prescription dispensed, the number of opioid fatalities has continued to rise unabated. 1 Although the opioid crisis is often seen through the prism of fatal overdoses, its most dramatic manifestation, the misuse of opioids and OUD also lead to death, disease and suffering from many other causes with devastating medical, social and economic consequences. 2 - 5
Like most complex problems, the opioid crisis has multiple roots, 5 including changing social and economic conditions, limited availability of safe and effective analgesics, insufficient treatment capacity for OUD and legal approaches that criminalize OUD rather than fostering treatment. The two major driving factors of the crisis, however, were the steady increase in the rate of opioid prescriptions, particularly in the early stages of the crisis, and the decrease in price and the increase in availability of heroin and synthetic opioids.
Increases in the rate of opioid prescribing followed the identification of undertreatment of pain in the 1990s as an important clinical problem 6 and the mistaken belief, based on anecdotal evidence, that patients in pain were not at risk for OUD. 7 Although less than 10% of individuals to whom opioids are prescribed develop OUD, 8 large increases in the rates of opioid prescriptions inevitably led to more individuals being exposed to opioids and subsequent increases in OUD prevalence. More importantly, increased availability of opioids generated an enormous surplus of medication that was diverted for non-medical use. 9 From 1991 to 2013, the prevalence of non-medical use of prescription opioids in the US more than doubled, from 1.5% to 4.1%, and the prevalence of prescription OUD tripled, from 0.3% to 0.9%. 10 , 11 At the same time, the severity of nonmedical use, as measured by the frequency of use, also increased among nonmedical users. 12
A second major factor in the crisis was the increased accessibility and purity of heroin coupled with reduced price partly due to increases in the efficiency of its distribution channels, which led to increases in heroin use and heroin use disorder. From 2001-2002 and 2012-2013 the prevalence of lifetime heroin use in the US increased from 0.33% to 1.6% and the lifetime prevalence of heroin use disorder increased from 0.21% to 0.69%. 13 , 14 There is controversy regarding whether efforts to decrease use of prescription opioids led to increases in heroin use and use disorder. Although individuals who use prescription opioids are more likely than those who don’t to use heroin, only 3%-5% of individuals who used prescription drugs non-medically in the previous year also reported using heroin during the same year. 15 Furthermore, increases in heroin use among nonmedical prescription opioids users preceded the development of policies to address misuse of prescription opioids. Thus, it may be the case that efforts to improve prescription of opioids have played a more limited role in the increases in heroin use than that ascribed to them. 16
A more recent entrant has been fentanyl and other very potent synthetic fentanyl analogues. From 2010 to 2017, deaths from fentanyl and other synthetic opioids increased nearly ten -fold, from around 3,000 (14.3% of opioid-related deaths) to over 28,466 (59.8%). 17 , 18 Synthetic opioids are now almost twice as commonly involved in overdose deaths as prescription opioids or heroin. 17 The low production costs of fentanyl and its potency (50- fold compared to heroin) make it an attractive option to mix (“lace”) with heroin and illicit manufactured prescription opioids. 19 At present, it is not known how many users actively seek fentanyl, but regardless of intent, heroin users are being exposed to fentanyl or other analogues without realizing it, increasing their risk of overdosing. Overdoses from fentanyl by itself or combined with heroin appear to be harder to reverse with naloxone than overdoses due to prescription opioids or pure heroin, contributing to the lethality of fentanyl or drugs laced with it. The reasons for the decreased efficacy of naloxone for reversing fentanyl overdoses are unclear and might reflect, its very high potency at the mu opioid receptor (MOR), its very fast pharmacokinetics (entering the brain very rapidly minimizing time for intervention), longer duration of its respiratory depressing effects (that might explain re-narcotization after a temporary reversal of an overdose by naloxone) and/or an additive effects when fentanyl is combined with other drugs including heroin. 20
Pharmacological properties of opioids
In addition to their effect on MOR, opioid drugs also bind to kappa- (KOR) and delta-opioid (DOR) receptors, although their affinity, intrinsic efficacy, pharmacokinetics and bioavailability vary by drug. In particular opioid drugs with fast uptake into the brain and full agonist effects at MOR such as heroin and fentanyl are particularly rewarding. 20 A strategy for developing opioid medications with lower abuse liability entails opioids formulations with slower entry into the brain and/or formulations that cannot be injected, since this is the route of administration that results in the faster rate of drug uptake in brain. The intrinsic efficacy of full agonist drugs such as heroin and fentanyl leads to greater rewarding effects than for partial agonists such as buprenorphine. Additionally, the rate of clearance of opioid drugs from the brain determines their duration of action and the severity of withdrawal symptoms upon their discontinuation. For that reason, heroin is associated with a much more severe withdrawal than a drug such as buprenorphine, which clears the brain more slowly. Opioids drugs with longer half-lives, slower clearance rates and slower brain uptake are favored for the treatment of OUD. By binding to MOR they decrease craving and prevent the emergence of withdrawal symptoms. Methadone enters the brain rapidly, which is why it is given orally when used for OUD treatment, for this will slow its entry into the brain. Also while it is a full MOR agonist, it has agonist effects at galanin receptors, which are co-expressed with MOR in brain reward regions antagonizing them, and thus reducing methadone’s rewarding effects. 31
The positive (increase reward) and negative (avoid pain) reinforcing effects of opioids, trigger learned associations between the receipt of the drug and these experiences resulting in conditioning. In parallel, the repeated administration of opioids triggers physiological adaptations that result in tolerance and in physical dependence. Tolerance necessitates increasing opioid doses in order to achieve the same levels of analgesia and when misusing them increasing the doses or shifting to more potent opioids such as fentanyl in order to experience their rewarding effects. The development of tolerance does not occur at the same rate for the various pharmacological effects of opioids. In general, tolerance to the analgesic and hedonic effects develops faster than to respiratory depression, whereas tolerance to constipation might never develop. 32 This explains why increases in dose to maintain analgesia (or reward) or doses injected to get high can markedly increase the risk of overdose.
Physical dependence to opioids also occurs rapidly and is responsible for the emergence of withdrawal symptoms when opioids are abruptly discontinued, which creates a negative reinforcement mechanism that can contribute to the maintenance of opioid use. The severity of withdrawal symptoms varies as a function of the opioid drug used; greater for higher potency short acting opioids than for longer lasting opioids as well as the chronicity of exposure. The symptoms from acute withdrawal usually resolve within days and are rarely lethal, but are extremely uncomfortable and are a powerful trigger for relapse in those with an OUD and for continued opioid use among those being treated with opioid analgesics. The risk of withdrawal is minimized or prevented by tapering opioids gradually.
Repeated use of opioids often results in physical dependence as a result of multiple neuroadaptations including desensitization and internalization of the MOR, impaired MOR signaling with intracellular effectors, and adaptations in glial signaling and in neuropeptide systems that interact with MOR-sensitive neurons, among others 33 , 34 . In contrast to physical dependence, OUD develops only in a minority of individuals exposed to opioids. It is characterized by a pattern of maladaptive opioid use that leads to clinically significant impairment or distress and is manifested as intense craving for opioids, erosion of inhibitory control over efforts to refrain from using them, persistent thinking about procuring the drug, and impaired control over opioid use. Because of their repeated opioid use, those with OUD also suffer from physical dependence and tolerance, unless they have undergone supervised medical withdrawal (formerly known as detoxification) in which case they can have OUD without having physical dependence or tolerance. This is clinically relevant in that patients undergoing medically supervised withdrawal without subsequent treatment for OUD are at an extremely high risk of relapse and of overdosing since they crave the drug as intensely as prior to their withdrawal but have lost their tolerance to the opioids. The behavioral manifestations of OUD are associated with structural and functional changes in the brain’s reward, executive control, emotion and interoceptive circuits. 58 , 59
Biological factors contributing to OUD
In addition to the environmental contributions to the crisis, multiple biological factors modulate an individuals’ vulnerability for opioid use, non-medical use and OUD, including genetic predisposition, brain development, mental illness and social factors.
Studies of genetic epidemiology indicate that genes contribute about 50% of the vulnerability to SUD, including OUD. Yet identifying specific genetic variants for increased OUD risk has been difficult, which is likely to reflect in part the fact that OUD, like other psychiatric disorders is a polygenic disease. Genes influence brain development and function of brain circuits and neurotransmitter systems that mediate the reactivity to the environment including drug responses. Furthermore, genes can intervene at different stages of OUD development, including propensity to use (i.e. genes that modulate personality traits) risk of transition from use to OUD (i.e. gene involved in conditioning and neuroplasticity), and vulnerability to relapse (i.e. genes that modulate severity of withdrawal symptoms, sensitivity to stress or other potential triggers). Because OUD often co-occurs with other psychiatric disorders, genes that increase the risk for those co-occurring disorders can also indirectly increase OUD risk. The effects of genes are also moderated by environmental influences.
Despite these complexities, studies have been able to identify genes that appear to contribute to OUD risk ( Table 1 ). For example, OPRM1 the gene that encodes for MOR has been implicated in increased vulnerability to OUD. 35 Similarly, converging evidence of genome-wide association, neuroimaging and rodent studies support a role in OUD for CNIH3, a gene that encodes for the Cornichon Family AMPA Receptor Auxiliary Protein that regulates trafficking and gating properties of AMPA receptors. 36 Preliminary results also suggest that the BDNF Val(66) Met genotype, which has been associated with neurobehavioral deficits, may promote drug-seeking in individuals with OUD. 37
Genes associated with increased vulnerability to opioid use disorder
Other genes, though not directly linked to OUD, relate to risk factors, such as personality traits, or brain regions implicated in the circuitry of addiction. For example, polymorphisms associated with low activity in MAOA , the gene for monoamine oxidase A, have been linked to a predisposition to externalizing behaviors and disorders that is moderated by environmental exposures. 38 , 39 Similarly, the catecholamine-O-methyltransferase (COMT) gene variant V(108/158)M, leads to greater dopamine degradation and impaired modulation of prefrontal cortex 40 , and has been associated with increased amygdalar reactivity 41 and with disrupted modulation of cortical and striatal activation during anticipation or receipt of a reward. 42 , 43 Numerous animal and human studies have also demonstrated the role of dopamine receptors in reward related behaviors, 44 - 46 but to date, no study has directly linked any dopamine-related gene to the risk of OUD.
Genes whose product influence synaptic plasticity can also contribute to OUD risk, such as Homer proteins, which regulate the level and activity of glutamate receptors, 47 and matrix metalloproteinase 9 (MMP-9), which in animal models increases motivation for drug-seeking. 48 , 49 Genes that influence response to stress by modulating the glucocorticoid receptor’s affinity for cortisol such as the FKBP5 chaperone protein 50 may also increase risk of OUD.
Brain Development
Drug experimentation is commonly initiated during adolescence and the risk to addiction is increased with early drug use. The greater vulnerability of adolescents to drug use and experimentation is driven by multiple factors including genetics that are associated with the developmental trajectories of the human brain. At the same time the social environments during childhood will influence brain development in ways that can increase its vulnerability to drugs or provide with resilience. Notably, the development of the human brain has a greater sensitivity to environmental factor during the first than second decades of life whereas genes influence brain development throughout the first and second decades. 51 This explain why social stressors are particularly harmful during early childhood. However while it is heuristically useful to distinguish environmental from genetic factors, it is likely that it is their interactions that ultimately determine how the brain will develop.
A better characterization of human neurodevelopment has allowed us to start to understand the role of the environment at critical moments of brain development and how differences in the rate of development of neuronal circuits influence vulnerability for drug use. For example, during adolescence reward and emotion circuits develop faster than those related to executive function, creating an imbalance between systems that favor experimentation, reward-seeking and drug use and systems underpinning self-regulation. Early exposure to drugs of abuse may further impair the development of the prefrontal cortex, decreasing self-regulating capabilities and increasing the long-term risk for SUD. 52
Several brain imaging studies have started or will soon start to generate data to inform the development of the brain and the influence of substance use in this development. The Adolescent Brain Cognitive Development Study (ABCD) is a longitudinal study of children 9-10 years old who will be assessed with brain imaging, genotyping, and deep phenotyping and followed for 10 years. It recently completed the baseline assessment of 11,875 children and has started to provide valuable data on normal variability in brain development and its influence by environmental factors. 53 The Baby Connectome Project is a four-year study of children from birth through five years of age, intended to provide a better understanding of how the brain develops from infancy through early childhood and the factors that contribute to healthy brain development. 54 It will help characterize human brain connectivity and map patterns of structural and functional connectivity to important behavioral skills. Additional biological (e.g., genetic markers) and environmental measures (e.g., family demographics) will be collected and examined to provide a more comprehensive picture of the factors that affect brain development. Finally, the planned HEALthy Brain and Child Development (HBCD) study aims to prospectively follow 7,500 infants through childhood (e.g., age 9-10) to assess structural and functional brain development as well as cognitive, behavioral, social, and emotional development and the long-term impacts of pre/postnatal drug (expected oversampling for opioid prenatal exposures) and adverse environmental exposures on brain and behavioral health and risk for substance use and mental disorders.
Social determinants
Epigenetics are implicated in the persistent neuroplastic changes associated with exposure to environmental factors that increase vulnerability to addiction such as social stressors or drug exposures. 55 For example, the environment’s ability to shape the circuits of emotion, particularly those impacted during critical periods of prenatal, postnatal, and adolescent brain development, 56 taps heavily on epigenetic mechanisms. 46 , 57 Studies have also started to assess the effects of social stressors on the development of the human brain, and these studies are relevant for understanding why social stressors increase the risk for SUD and other psychiatric disorders. Studies evaluating the effects of social deprivation during infancy and early childhood have reported delayed maturation that results in impaired brain connectivity, which could underlie increased impulsivity in these children. 58 Fortunately, preliminary studies suggest that interventions that provide social support may help reverse some of these impairments. 59 Nevertheless, stressful events at any age can increase vulnerability to opioid use and OUD.
Though the developing brain is the most sensitive to adverse social environments, these can also negatively influence the adult brain in ways that increase the vulnerability to drugs use and addiction. This is apparent in the current opioid epidemic and the other “deaths of despair” (overdoses, suicide and alcohol-driven cirrhosis) that have prominently affected adult white Americans from economically impoverished environments. There is a scarcity of research on the effects of adverse social environments on adult brain function. 60 , 61
Neurocircuitry of addiction
Reward circuitry.
Although much remains to be learned, our growing knowledge of the brain’s reward circuitry (originating in the dopamine neurons in the ventral tegmental area projecting to the nucleus accumbens, ventral prefrontal cortex and amygdala) and its changes have been fundamental for understanding drug taking and addiction. Reward (more precisely, reinforcement) can be defined as any event that increases the probability of repeating the action. Animal 62 , 63 and human studies consistently indicate that drugs release dopamine (DA) in the nucleus accumbens (NAc), which is fundamental to their rewarding effects. Additionally, increases in endogenous opioids and cannabinoids are also associated with the rewarding effects of various drugs. 64 , 65 It is thought that the rapid release of DA and its binding to D 1 receptors (D1R) in the ventral (location of NAc) and dorsal striatum, which stimulates cyclic AMP (cAMP) signaling is associated with euphoria and pleasure (so-called “high”) and triggers conditioning (learned association between the drug effects and situation where it occurred). By contrast, stimulation of D 2 receptors (D2R), which inhibit cAMP signaling, does not appear to be associated with rewarding effects per se but blocks the aversive effects when D2R-expressing medium spiny neurons (D2R-MSN) are not inhibited by DA. 66 Individuals with SUD often have decreased baseline DA release in the striatum (including in nucleus accumbens) and experience an attenuated DA increase and less intense reward from drug use (i.e., tolerance), which is interpreted to reflect a hypofunction of their reward circuit. It is unknown to what extent this hypofunction reflects a predisposition of the individual versus consequences from chronic drug exposures.
With repeated drug use, conditioning strengthens and drives the motivation to procure the drug reinforcers. Exposure to conditioned stimuli (referred to as cues) by themselves triggers firing of DA neurons and DA release, energizing the motivation to obtain the drug. When previously neutral stimuli become conditioned to the drug they acquire incentive salience, becoming desirable 67 . Conditioning and the associated DA increases in the striatum are hypothesized to underlie the intense desire for drugs that addicted individuals experience upon exposure to environments or situations where they have taken drugs that frequently leads them to relapse.
The drug-induced stimulation of D1R signaling involved with conditioning triggers synaptic changes in N-methyl-D-aspartate (NMDA) and α-amino-3-hydroxy-5-methyl-4-isoxazolepropionic acid (AMPA) receptors that enhance glutamatergic signaling in the affected synapses. 68 , 69 At the circuit levels these neuroplastic changes strengthen striatal- pallidal-thalamo-cortical loops that include the ventral and the dorsal striatum, resulting in habit formation 70 and compulsive response for drugs. As SUD progresses, a prominent hypothesis is that these circuits become increasingly sensitized to drug-cues, environmental stressors or negative emotional states, which can then more readily trigger compulsive drug consumption. In more advanced stages, substance use behaviors are more driven by a growing importance of dorsal striatum and habits and a decreasing role of positive drug reward. In parallel, as drug-procuring and taking becomes increasingly salient in the addicted individual, non-drug related activities become less motivating and rewarding. 71 , 72
Although DA and glutamate remain central to our understanding of the reward circuits, these circuits are also modulated by γ-aminobutyric acid (GABA), serotonin, acetylcholine, and the endogenous opioid and cannabinoid systems. Similarly, it is important to realize that, like any other, the reward circuit (as well as the executive control circuit) interact and overlap with circuitry involved in the perception of internal bodily states or interoception (including primary sensory cortex, insula, anterior cingulate cortex and precuneus), homeostasis (hypothalamus), stress (amygdala, hypothalamus, and habenula), salience attribution (orbitofrontal cortex) and learning and memory (amygdala, hippocampus and dorsal striatum) and that the interplay with these circuits modulates the responses to rewards and to conditioned cues ( Figure 1 ). 73

The circuitry of opioid use disorder (reprinted with permission from: George O, Koob GF. Control of craving by the prefrontal cortex. Proc Natl Acad Sci U S A. 2013 Mar 12;110(11):4165-4166
Emotion Circuitry
Although positive reinforcers play a major role in the initial phases of drug taking and the development of OUD, in more advance stages negative emotional experiences such as withdrawal symptoms, craving and enhanced sensitivity to stress become increasingly important and drive drug taking. The increased role of negative reinforcement in drug taking (as a means to escape the negative emotional state) is a considerable barrier to abstinence and a formidable obstacle to successful treatment.
The negative emotional state can be understood as enhancement of processes that are the inverse of those involved in reward (i.e., “anti-reward processes”) 74 that are derived from neuroadaptions to drug-induced stimulation. 62 In the case of opioids most of the physical manifestations of withdrawal, which emerge with drug discontinuation are caused by decreased MOR-triggered intracellular signaling and by enhanced autonomic reactivity. 75 Early signs of withdrawal, appearing generally in the first 8-36 hours of heroin discontinuation include mydriasis, piloerection, muscle twitching, lacrimation, rhinorrhea, diaphoresis, yawning, restlessness, myalgia, abdominal pain, nausea/vomiting, tremor and insomnia. Individuals with fully developed opioid withdrawal can also experience tachycardia, tachypnea, hypertension or hypotension, dehydration, hyperglycemia, fever, anorexia and diarrhea.
In addition to these physiological manifestations, opioid withdrawal is often associated with neuropsychological symptoms that include, irritability, emotional pain, dysphoria, insomnia and subjective distress that can last several months. The subjective aspects of the negative emotional state are associated with disrupted dopaminergic, serotonergic, noradrenergic, glutamatergic, GABAergic neurotransmission in reward and emotion networks (including NAc). 67 Stress-related neurotransmitters, such as CRF, norepinephrine, and dynorphin, are also recruited in the extended amygdala and contribute to the distress and increased irritability often present in withdrawal and protracted abstinence. 76 The lateral habenula, which decreases DA neuronal firing in VTA when expected rewards do not materialize 77 - 79 and is a target of serotonin neurons from the dorsal raphe that modulate mood, also plays a crucial role in triggering and maintaining negative emotional states. 80 Similarly, the insula, which is reciprocally connected to several limbic regions and to the default mode network (DMN) has an interoceptive function that integrates autonomic and visceral information with emotion and motivation 81 , plays a key role in the self-awareness of negative emotional states and of craving. 82 - 84
Executive Control Circuitry
Impairments in executive function (including self-regulation), which can precede as a vulnerability factor for SUD or develop secondary to chronic drug exposures, are important contributors to impulsive and compulsive drug taking. 67
During withdrawal the emergence of the preoccupation/anticipation phase along with mounting craving for the drug results from the interplay of two opposing systems: a Go and a Stop system. The Go system includes most of the circuitry described in the two previous sections, which underlies the hedonic, habitual and emotional aversive aspects that leads to continued drug taking. 85 , 86 The Stop system seeks to control the incentive value of choices, regulate habitual behavior, maintain goal-directed behavior and suppress affective responses to negative emotional signals. 87 - 89 In this framework, the Stop system seeks to inhibit signals generated by the Go system.
The Stop system involves a widely distributed and complex prefrontal cortex–subcortical circuitry. It is mediated through glutamatergic projections from prefrontal cortex to the caudate and ventral striatum to modulate the striatal-pallidal-thalamocortical direct (D 1 receptor-mediated) and indirect (D 2 receptor-mediated) pathways, and to modulate the mesocortical dopamine neurons in the ventral tegmental area, which exert control over DA release in the prefrontal cortex. 73 Deficits in prefrontal cortex in individuals with SUD are associated in impairments in executive function that can interfere with decision making, self-regulation, inhibitory control, and working memory. 90 Reduced activity in the prefrontal cortex (including dorsolateral prefrontal cortex, anterior cingulate gyrus, and medial orbitofrontal cortex) is also associated with downregulation of D2R in the striatum, 91 - 93 and disrupted GABAergic activity in striatum and prefrontal cortex. 94 , 95
The orbital frontal cortex, anterior cingulate cortex and dorsolateral prefrontal cortex are involved with incentive salience, emotional regulation, and decision making, respectively. Deficits in striatal D2R-mediated DA signaling, which modulates them, may underlie the enhanced motivational value of drugs and the loss of control over drug intake in SUD. 96 Furthermore, because dysfunction of the orbital frontal cortex and the anterior cingulate cortex are associated with compulsive behaviors and impulsivity, 97 DA’s impaired modulation of these regions may contribute to compulsive and impulsive drug taking in SUD. 96
Several pathways can encode signals that increase the risk of relapse with exposure to cues, which can activate the ventral prefrontal cortex, including ventral anterior cingulate, and medial and lateral orbitofrontal cortices. 98 - 101 Cue-induced reinstatement involves glutamatergic projections from the prefrontal cortex, basolateral amygdala, and ventral subiculum to the NAc, and DA projections to the basolateral amygdala and dorsal striatum. 102 - 104
In contrast to cue-induced relapse, stress-induced reinstatement depends on the activation of both CRF and norepinephrine in parts of the extended amygdala (i.e., central nucleus of the amygdala and bed nucleus of the stria terminalis) and the VTA. 105 Protracted abstinence, mostly described for alcohol, appears to involve overactive glutamatergic and CRF systems. 106 , 107 A third pathway to relapse is through interoceptive stimuli related to the insula 82 - 84 and its activation during craving has been associated with relapse. 108
Pharmacological treatment of opioids
A range of pharmacological treatments exists to treat different components of OUD. Information on these medications was recently reviewed by the U.S. Substance Abuse and Mental Health Administration 109 and by the National Academy of Medicine. 110
Medically supervised withdrawal (formerly referred to as detoxification) is the gradual taper of opioid agonist medications (methadone or buprenorphine) guided by a clinician to alleviate withdrawal symptoms. The use of α 2 -agonists such as lofexidine (recently approved by the FDA for the treatment of opioid withdrawal) or clonidine can also help attenuate symptoms of withdrawal, 111 though subsequent initiation of medications for OUD (MOUD) is required to prevent relapse into drug taking. Indeed, medically supervised withdrawal is not recommended as an isolated strategy, as most patients without subsequent MOUD initiation relapse shortly thereafter 112 - 114 and are at increased risk for overdosing due to the loss of tolerance. 115 Medically supervised withdrawal is required, though, for patients starting naltrexone, as described in the next section.
Maintenance
Ongoing outpatient treatment with MOUD leads to better retention and outcomes for OUD 116 and reduced risk of HIV and HCV infection and overdose death. 117 - 121 At present, MOUD constitutes the standard of care for most patients with OUD. There are three FDA-approved MOUD: methadone, buprenorphine and naltrexone. In deciding on a specific medication the clinician should evaluate the patient’s responses to prior MOUD treatment, tolerance to opioids and patient preferences. Patients, in turn, should be informed of the efficacy, risks, benefits and relative advantages of each of these medications.
Methadone has been available the longest and has the largest evidence of efficacy. 119 , 121 Higher doses of methadone are associated with better retention in treatment, less heroin use during treatment and lower withdrawal symptoms, until around 100 mg/day, after which the reliability of evidence is lower. 122 Because methadone is a full MOR agonist, it has no ceiling effect. It can lead to overdoses if it is used at doses above the patient’s tolerance or combined with other central nervous depressants such as alcohol, benzodiazepines or other opioids. To minimize the risk of intoxication or overdose, methadone should be started at low doses and increased gradually with daily monitoring over several weeks. An important barrier to the use of methadone, particularly in rural settings is that, with a few exceptions, in the US methadone has to be administered in a licensed opioid treatment programs (methadone clinics) and cannot be prescribed by office-based clinicians.
The use of buprenorphine 123 , 124 has certain advantages over methadone, which explains its growing use for OUD treatment. First, although it can still be lethal when combined with other central nervous depressant substances, as a partial agonist, buprenorphine has lower lethality than methadone. 124 Second, its antagonist effects at the KOR may contribute to its efficacy for OUD. 125 , 126 Its KOR antagonist properties may also have antidepressant effects, which could help improve the depressive symptoms that are common in OUD. Buprenorphine is also an agonist at the nociceptin receptor, which is also implicated on its therapeutic benefits. To deter the injection of buprenorphine, which would enhance its rewarding effects, it is often prescribed in a formulation that includes naloxone, a short-acting opioid antagonist that has poor bioavailability when sublingually administered, but blocks buprenorphine effects if injected. Currently, in the US, prescribers of buprenorphine for the treatment of OUD need to obtain a waiver from the Drug Enforcement Administration (DEA) after completing an 8-hour training.
Extended-release (XR) formulations of buprenorphine have been developed to improve patient adherence. Although, 6-months buprenorphine implants were shown to be as effective as low-dose sublingual buprenorphine, 127 its benefits are restricted to patients who respond to low doses of buprenorphine (8mg). In 2017, the FDA approved a 1-month XR buprenorphine injection for patients with OUD who have been treated with sublingual buprenorphine for at least one week. Another 1-month and a 1-week XR buprenorphine are currently under FDA review. It would be important to know whether those new formulations can increase treatment retention.
The third FDA-approved MOUD is naltrexone, which is a MOR antagonist that can be prescribed by clinicians in outpatient practice. The efficacy of its immediate release formulation was limited by poor adherence, but the development of a monthly extended release formulation (XR-NTX) significantly improved outcomes. 128 - 131 It has been particularly useful in justice system settings reluctant to use agonist therapies, 130 although there is need to assess whether it would be superior to XR-buprenorphine in those settings. Naltrexone is also a KOR antagonist, and while its affinity is lower than that for MOR, a recent brain imaging study reported KOR occupancies > 80% in patients with alcohol use disorder treated with naltrexone. 132 Antagonism of KOR by naltrexone could contribute to the mood improvements reported in OUD patients treated with naltrexone. 133 A potential barrier for the use of XR-NTX is the need for medically supervised withdrawal. Patients cannot use short-acting opioids for at least 7 days and long-acting opioids for 10-14 days preceding induction onto naltrexone to avoid triggering a withdrawal syndrome. A rapid taper consisting of a single day of buprenorphine followed by ascending doses of oral naltrexone along with clonidine and other adjunctive medications (e.g., clonazepam and prochlorperazine) may allow for faster induction protocols for XR-NTX, 131 but requires further study before it can be recommended as standard strategy.
At present, limited data are available regarding the comparative effectiveness of MOUD or about which patients will respond better to each medication. 109 A Cochrane review concluded that flexible-dose methadone leads to greater retention than sublingual buprenorphine, 119 but whether the same results would hold when compared with XR-buprenorphine is unknown. There are currently no published Cochrane reviews of XR-NTX versus buprenorphine, but two studies 134 , 135 have suggested that patients who can be inducted onto XR-NTX have similar outcomes to those treated with sublingual buprenorphine. However, in one of those studies 134 a substantial proportion of patients were unable to complete XR-NTX induction, mostly due to early relapse, leading to superior outcomes for the buprenorphine group in the intent-to-treat analysis.
As knowledge about MOUDs continues to grow, three priority areas need to be addressed. First, studies suggest that longer time in treatment is associated with better outcomes and that the risk of relapse greatly increases after medication discontinuation, yet rates of MOUD discontinuation in the first 6 months of treatment remain very high. Thus, there is a need to improve retention in MOUD treatment. It is also important to know which patients, when and under what circumstances can safely discontinue MOUD.
Second, current evidence indicates that counseling or psychotherapy do not increase retention in buprenorphine treatment or improve abstinence rates 116 , 136 - 139 and that methadone treatment 140 and buprenorphine without concomitant counseling is vastly superior to no treatment. 141 It is important to determine whether the potential benefits of concurrent psychotherapy outweigh the barrier to treatment created by requiring provision of psychotherapy when delivering buprenorphine treatment (such counseling is at present not required for XR-NTX). This was highlighted in the recent report of the National Academy of Medicine , which emphasized that lack of behavioral treatment support is not a reason for withholding MOUD. 110 Nevertheless, evidence-based behavioral interventions can be useful in engaging some patients with OUD in treatment, retaining them in treatment, improving outcomes, and helping them achieve recovery . 142 More research is needed to determine which behavioral interventions provided in conjunction with MOUD are most helpful for which patients, including evidence on the effectiveness peer support. There is also a need to develop models of care that better attract and retain patients in care and can overcome the barriers posed by the limited availability of well-trained clinicians. The use of technology-based approaches (i.e Telehealth, mHealth) may be a promising avenue to achieve these goals.
Third, research is needed to evaluate whether residential or inpatient treatment is superior to outpatient treatment for initiating MOUD. This question is important to evaluate the optimal allocation of resources for the treatment of OUD.
Preventing Opioid-Related Overdoses
Despite national and local efforts, the number of opioid-related overdoses continue to increase, highlighting the need of clinicians to educate patients and their families about the risk of overdose and how to respond to it. Several medication- and patient-related factors increase the risk of overdose. 143 Patient-related factors include: 1) Age above 65 years; 2) respiratory problems; 3) long-term opioid use; 4) substance use disorder (including alcohol use disorder); 5) comorbid mood disorders and/or suicidality; 6) use after a period of abstinence (e.g. following medically supervised withdrawal or incarceration); 7) prior overdose. Medication-related factors include: 1) Daily dose above 100 morphine milligram equivalents; 2) use of higher doses than prescribed (or than usually consumed, in the case of illicit opioids); 3) combination with fentanyl, other high-potency opioids or other substances, such as alcohol or benzodiazepines.
The acute treatment of overdose is administration of naloxone. For many years naloxone, which required injection, could only be administered by health care providers, but the availability of an auto-injectable naloxone device and a naloxone spray now allow laypersons to administer naloxone to revert overdoses. 144 , 145 Nevertheless, increasing the availability of naloxone to ensure that it can reach those who need it on short notice remains a challenge, as there is considerable variability in the availability of naloxone by locality. 146 A 2019 analysis concluded that making naloxone available without prescription (“over the counter”) would substantially increase its availability. 147
In most cases a single dose of naloxone is sufficient to reverse the overdose. However, when high doses or high potency opioids such as fentanyl are used more than one dose is necessary to restore or maintain spontaneous breathing. 148 Furthermore, the fast pharmacokinetics of fentanyl can result in abrupt respiratory depression, which in some instances does not provide sufficient time to administer naloxone. It is believed that the duration of the respiratory depression from fentanyl or other analogues is longer than the duration of its reversal from naloxone. Moreover, thoracic rigidity associated with serotonergic effects from some of the opioids drugs might also interfere with overdose reversals. Naloxone can also fail to reverse overdoses in which opioids are combined with other CNS depressants, such as alcohol or benzodiazepines. First-responders to an overdose should stay with patients until emergency medical services arrive and transport them to an emergency room for a more systematic evaluation. Reversal of the overdose generally triggers an acute withdrawal syndrome, 111 which can lead patients to leave medical supervision to seek opioids for relieving withdrawal. Upon reversal of an overdose OUD patients should be linked to OUD treatment otherwise their risk of overdosing again is very high. The emergency room offers unique opportunities to start patients on MOUD if they can be linked with ongoing services. 149 Nevertheless, more research is needed to improve the management of patients immediately after reversal of an overdose, to retain them under care and to initiate effective MOUD.
Because of the urgency of the crisis, most efforts to date have emphasized treatment approaches. There is growing recognition, however, of the need to develop effective preventive interventions for OUD. 1 , 5 , 150 Initial preventive approaches in the US focused on improving prescription practices for opioid analgesics and increasing the availability of naloxone to prevent overdoses. The growing role of heroin, fentanyl and other synthetic opioids in the opioid crisis, 1 , 16 , 151 has required broadening the scope of preventive interventions. There are no evidence-based primary and secondary prevention for OUD for adults or for youth transitioning into adulthood, but several approaches appear as promising directions.
One approach would be to adapt existing interventions that have been successful for youth. Evidence-supported prevention interventions delivered in community or school settings have shown effectiveness at reducing substance use and other related problem behaviors, including middle-school interventions that have specifically demonstrated an impact on reducing prescription opioid misuse. 152 However, whether those interventions would work in adults is unknown. An obvious difficulty in adapting these interventions is the lack of a setting similar to school where adults could be easily reached.
A second direction would be the development of conceptual frameworks that articulate the relationship among risk factors for OUD to help guide which interventions might be most effective given the prevalence of the risk factors, how they relate to other relevant risk factors and how modifiable the risk factors are. These models could help examine how risk factors present at birth (e.g., family history of substance use disorders) or childhood (e.g., adverse childhood events) can increase the likelihood of risk factors in adolescence (e.g., early onset of psychiatric disorders, low educational achievement), which in turn increase the risk of OUD in adulthood. 153 Simulations could help identify which interventions might be most promising at the full population level or for subgroups with specific constellations of risk factors, as well as to identify potential unintended consequences of those interventions.
A third approach would be to increase the focus on populations at risk that can be accessed for screening and treatment. Those include, for example, individuals who receive regular health care and those in the justice system. Improved management of opioid prescriptions and of treatment of OUD for pregnant women is also a high priority, as it could benefit the mother and simultaneously decrease the risk of neonatal abstinence syndrome (NAS) in newborns by decreasing their in-utero exposure to opioids.
Even with wider use of non-opioid analgesics or use of non-pharmacological approaches, opioids will continue to be necessary for the treatment of many patients with severe pain. Efforts to prevent the development of opioid misuse and OUD can be started in clinical settings. This includes assessment of risk of OUD before opioids are prescribed, periodic assessment of the need for opioid use, and use of urine testing to rule out illicit use of other substances. There is evidence that prescribing lower doses/fewer pills in the emergency room/post-surgery is associated with lower rates of long-term use and possibly OUD. There is also a need to assess each patient for licit and illicit use of other substances, particularly benzodiazepines and alcohol, which can increase the lethality of opioids by potentiating their depressing respiratory effects. Individuals who misuse opioids or develop OUD should be treated by their primary physicians if they have the necessary expertise and support or otherwise be referred to an addiction specialist.
Prevention approaches should also consider supply approaches. The most common sources of diverted opioid analgesics are friends or relatives who were legitimately prescribed opioids. 154 As with any other medication, it is important to educate patients who receive legitimate prescriptions about the health hazards they create for others when they give them their medications. Similarly, it is important to educate patients about the health risks they incur when they take medications (including opioids) that were not prescribed to them. Use of Prescription Drug Monitoring Programs (PDMPs) can help reduce doctor shopping and overdoses, but their use to date is inconsistent. This may be due in part to the voluntary nature of the programs in many states, delays in updating the information, restrictions in data sharing across jurisdictions and the need to access them through a separate computer from the one used to access electronic health records. 150 Approaches that could help decreased the availability of heroin and fentanyl, traditionally in the purview of law enforcement primarily, would also advance prevention, but their development and implementation are challenging in the face of the evolving nature of the epidemic.
Research Gaps and Translational Opportunities
Basic science.
In addition to making progress in clinical and public health approaches, there is a need to advance fundamental science that can provide the foundations of more effective interventions for the current crisis and provide the basis to combat future ones. For example, there is a need to better understand the genes implicated in individual differences in vulnerability to the stages and sequelae along the OUD trajectory, including the development of tolerance, physical dependence, addiction, hyperalgesia, and respiratory depression for they could help identify new medication targets and in the future could serve as biomarkers to predict risk for side effects. Animal models could be used to identify the functions of the relevant genetic variants, epigenetic modifications, and gene networks.
Similarly, technological advances, such as those emerging from the BRAIN initiative could be leveraged to gain a deeper knowledge of endogenous opioids and to identify the consequences of exogenous opioid administration on the endogenous opioid systems and the cells and circuits they modulate across a trajectory of opioid use, ranging from acute administration, tolerance, physical dependence, addiction, and recovery to relapse. This could include studying profile changes in the transcriptome and methylome of opioid-synthesizing neurons, imaging the intracellular signaling cascades following opioid receptor activation (and how those cascades change across the trajectory of opioid use) and using quantitative brain-wide optical imaging combined with computational pipelines for cellular registration to provide unbiased documentation of the stereotyped distributions of cellular activity and cell-types engaged by opioids under different conditions. Post-mortem brains could be used to identify human-specific genes and their proteins, as has been done in the study of other psychiatric disorders. Use of post-mortem brains would enable the discovery and integration of genomic, epigenomic, proteomic, metabolomic, and non-coding RNA features across many brain regions and cell types that distinguish individuals with vulnerabilities to OUD and opioid-induced respiratory depression. This knowledge could be combined to validate findings in animal models (i.e., does the animal model recapitulate the human brain biology?) and to reverse translate from human to test a hypothesis in rodents.
A systematic investigation of developmental trajectories of the human brain is relevant for our understanding of the mechanism through which adverse child experiences as well as drug exposures during fetal development increase risk for drug use and other mental illnesses. To address this question, the HEALthy Brain and Child Development (HBCD) Study, which is part of the HEAL Initiative from the National Institutes of Health, 155 will establish a large cohort of pregnant women from regions of the country significantly affected by the opioid crisis, and follow them and their children for at least 10 years. The study will help better understand typical brain development, beginning in the prenatal period through early childhood, including variability in development and how it contributes to cognitive, behavioral, social, and emotional function.
New Medications
Despite the existence of effective medications for the treatment of OUD, current rates of engagement and retention in treatment suggest that new medications and devices are needed. The introduction of more acceptable and effective medications is crucial to improve the outcomes of individuals with OUD. In turn, improved outcomes could lead to changes in societal attitudes towards OUD, with broader acceptance of OUD as a treatable brain disorder and lower stigmatization of those suffering from it. Because the circuitry and neurotransmitters of the different phases of OUD only partially overlap, several complementary approaches may be necessary to treat the whole spectrum of severity from sporadic to chronic use, withdrawal and relapse.
To help speed the development of novel pharmacotherapies (i.e., excluding already known mechanisms) NIDA recently created a list of medication development priorities based on data from published literature and internal studies with the most direct relevance to OUD. 156 This list, which is not exclusive did not prioritize any of the 10 proposed mechanisms and included: 1) orexin-1 or 1/2 antagonists; 2) Kappa opioid antagonists; 3) GABA-B agonists; 4) Muscarinic M 5 antagonists; 5) AMPA antagonists; 6) nociception opioid peptide receptors/opioid receptor like agonists or antagonists; 7) mGluR2/3 agonists; 8) Ghrelin antagonists; 9) Dopamine D 3 partial agonists; and, 10) Cannabinoid CB-1 antagonists.
In addition to medications that target specific receptors, the development of allosteric modulators of those receptors is also a high priority. Negative allosteric modulators (NAMs) and positive allosteric modulators (PAMs) may provide more physiologically relevant effects compared with agonists and antagonists acting on the same receptor, which may ultimately result in improved clinical outcomes. 157 The development of opioids that selectively activate MOR G-protein intracellular pathways (“biased agonists”) may lead to medication with potent analgesic properties but with lower risk of respiratory depression and addictive liability, which would contribute to decrease the incidence and prevalence of OUD. 158 Other potential directions could include epigenetic, micro RNA and neuroimmune targets.
Better training of health professionals
Despite the severity of the dual crises of chronic pain and OUD, very few medical schools offer adequate training in pain management, and still fewer offer even one course in addiction. Furthermore, surprisingly little is known about how best to train physicians and other health professionals on the management of OUD including the use of medications. 159 There is also evidence that many individuals trained to provide MOUD do not provide that treatment. Some national organizations offer a combination of didactics, supervision and mentoring to provide training beyond residency, 159 and the new subspecialty of addiction medicine is likely to further increase the availability of well-trained professionals that can treat individuals with OUD. However, broader changes, including combating stigma, enhancing institutional support and increasing reimbursement rates may be necessary to increase rates of treatment among those trained to provide it. Increasing the number of addiction treatment and prevention programs, particularly in low-resource communities is also essential.
The opioid crisis is a complex, evolving phenomenon. It involves neurobiological vulnerabilities and social determinants of health. Successfully addressing the crisis will require advances in basic science, development of more effective treatments, and public health approaches to implement current and emerging knowledge. We hope that this review will alert clinicians and researchers about the current approaches to the crisis and suggest opportunities for future research and for practice improvement. The advances achieved in addressing the current crisis should also serve to expand the science and treatment of other substance use disorders.
Acknowledgments:
The views and opinions expressed in this report are those of the authors and should not be construed to represent the views of any of the sponsoring organizations, agencies or the US government.
Conflict of Interest : The authors have no competing financial interests in relation to the work described in this manuscript.
An Intelligent Disease Prediction and Drug Recommendation Prototype by Using Multiple Approaches of Machine Learning Algorithms
Ieee account.
- Change Username/Password
- Update Address
Purchase Details
- Payment Options
- Order History
- View Purchased Documents
Profile Information
- Communications Preferences
- Profession and Education
- Technical Interests
- US & Canada: +1 800 678 4333
- Worldwide: +1 732 981 0060
- Contact & Support
- About IEEE Xplore
- Accessibility
- Terms of Use
- Nondiscrimination Policy
- Privacy & Opting Out of Cookies
A not-for-profit organization, IEEE is the world's largest technical professional organization dedicated to advancing technology for the benefit of humanity. © Copyright 2024 IEEE - All rights reserved. Use of this web site signifies your agreement to the terms and conditions.
Top Drugs With Greater Health Risks for People Over 65
W e all know even the best of medications come with side-effects , but there’s a large portion of the population that’s even more vulnerable to the health risks of drugs than everyone else. If you’re 65 and over, there are many medications your body does not process like it used to; unfortunately, at times, this can be overlooked by your doctor, and the responsibility for being mindful of these risks lies in your own hands. With most adults middle-aged and overtaking an average of four prescription medications every day (1), things can get complicated. However, there are a few experts in older adult health that have come forward with helpful advice.
Why Older Adults Have A Greater Health Risk From Prescription Medications
So, why does age matter? Over time, your liver , which is the primary organ for metabolizing drugs can become less and less efficient. However, it’s important to note that everyone has their own natural metabolism speed- and it can be difficult for doctors to pinpoint a 100% safe dosage of a prescription drug. Someone with a fast drug metabolism may process their medications so quickly that they aren’t as effective as they are expected to be; someone with a slow drug metabolism may absorb too much of a medication before it’s excreted from the body: even toxic levels ( 2 ).
Read More: 6 Types of Medications That Can Potentially Harm Your Kidneys
Kirby Lee, a pharmacist and associate professor of clinical pharmacy at the University of California at San Francisco explains:
" As your body ages, it absorbs medications differently. They can be metabolized differently by your liver and excreted differently by your kidneys, so you may be more sensitive to some medications. Prescribing medications for people 65 and older can be more challenging because some drugs can be more toxic or cause more side effects than when you were younger." Kirby Lee, Pharmacist – via NextAvenue.org
To make matters even more complicated
Changes to your diet can impact your drug metabolism in unexpected ways; doctors can’t predict how your body might respond to a particular medication dosage, and unfortunately, specialists in the field are few and far between. According to Patricia Corrigan, a journalist with NextAvenue , “Only about 7,500 physicians in the U.S. specialize in the care of older adults, according to the American Geriatrics Society. With 46 million Americans age 65 and older today, that works out to about one geriatrician per 6,100 patients.”
Which Medications Are More Dangerous for People Over 65?
In 1991, American geriatrician, Mark Beers, M.D. developed his signature list of medication types and the health risks of drugs which posed a unique risk to seniors. This list is now known as the Beers Criteria , and is widely circulated and updated by American geriatricians as newer prescription drugs are created. The criteria is meant to help both older patients and their doctors have increased awareness about which medications may have greater health risks than their intended benefits, thanks to their physiological makeup and their effect on the aging metabolic system. While it isn’t meant to overrule a physician’s recommendations, it’s important to be aware of your body’s unique needs and to ask questions and raise concerns for your medical care provider and pharmacist.
Read More: 13 Medication and Supplement Combinations That Should Avoided
The Beers Criteria: Download Printable List
- Benzodiazepines such as diazepam (Valium), lorazepam (Ativan), alpraxolam (Xanax) and chlordiazepoxide (Librium). Side effects include confusion and risk of falling.
- Non-Benzodiazepines such as Zolpidem (Ambien), zaleplon (Sonata) and eszopiclone (Lunesta). Side effects of chronic use include delirium, increased risk of falling and fracture
- Anticholinergics such as Diphenhydramine (Benadryl), acetaminophen with diphenhydramine (Tylenol PM) and muscle relaxants containing diphenhydramine. Some side effects include confusion, constipation, and dry mouth.
- NSAIDs such as Aspirin, naproxen, and ibuprofen. Side effects include increased risk of kidney damage and stomach damage.
- Blood Pressure medications including alpha-blockers: Doxazosin, Prazosin, Terazosin. Side effects include risk of falling and fatigue.
Antipsychotics sometimes prescribed for anxiety or depression. Side effects include increased risk of stroke in persons with dementia.
The above is not a complete list. For an up-to-date, downloadable list from the American Geriatrics Society, click here .
Patricia Corrigan’s Story
Patricia Corrigan is a journalist and the author of 19 books. She recently shared her personal encounter with a bad reaction to a prescription medication. Patricia’s story highlights the importance of taking your health into your own hands and learning to be aware of your body’s changing behavior. Here’s how to learn the easy way and not the hard way.
“ One recent weekend, I experienced a relapse while on doxycycline, an antibiotic prescribed for a bacterial sinus infection. The doctor on call I spoke with prescribed a stronger antibiotic for me, levofloxacin (Levaquin), one I'd taken successfully a decade ago. After four days on the new drug, a throbbing Achilles tendon awakened me in the wee hours. I hobbled to the computer and learned the drug is not recommended for people 65 and older. I am 68. Later that morning, I called my internist, who advised me to stop taking it and start helping the tendon to heal - no easy task, and one with no quick fix. I can't be angry with the physician who neglected to take my age into consideration, because I share in the blame. When I picked up the prescription, I waved off a consultation with the pharmacist, saying I'd taken this antibiotic before. Then, after reading just a sentence or two of the lengthy list provided to me of possible side effects, I tossed the paper into a recycling bin. “ Patricia Corrigan, Journalist – NextAvenue.org
Read More: 5 Easy Ways to Help Calm Anxiety Without Medications
How to Protect Yourself From The Health Risks of Drugs
You and your loved ones can develop a few small habits that can help to protect your health from harmful effects from your medications.
Be Open With Your Doctor
Try to develop the habit of making yourself comfortable with your medical care provider. Ask questions until you feel comfortable understanding what they’re telling you about your health. You can ask your doctor if they’re comfortable with being recorded so you can refer back to your conversations should you have any follow-up questions. If not, bring a small notebook and pen to your appointments. It’s also imperative not to exclude small details such as medications or multivitamins you’re taking or specific health conditions you have. This will inform your doctor’s recommendations.
Ask Your Pharmacist
Pharmacists are the real experts in your medications. Feel free to ask your pharmacist about the potential side effects of each prescription drug you’re taking, as well as whether there is a risk of interactions between medications. Your pharmacist can also provide recommendations for alternatives.
Read More: Don't Ever Use Turmeric If You're On Any of the Following Medications
Take Your Body Seriously
Even if your pharmacist and doctor are vouching for a particular medication, if your body isn’t feeling its best while you’re taking it, listen to it! Pay attention to red flags like upset stomach, dizziness, changes in disposition, pain, or discomfort. Any prescription medication you’re taking should work for you; if it doesn’t, make an appointment with your doctor to find an alternative. (It’s important to call your doctor first with your concerns before removing yourself from any prescription medication.)
Do Your Research
Save a copy of the Beers Criteria on your computer or print it off and keep it in a place you’ll remember to check. Make good use of online resources to find what possible side effects or downsides come with each prescription medication. You should also take into account added risks of long-term conditions such as asthma or diabetes.
Read More: 10 Medications Linked to Dementia
- “ Prescription Drug Use Among Midlife and Older Americans .” Assets . January 2005
- “ Drug Metabolism .” Merck Manuals . Jennifer Le, PharmD, MAS, BCPS-ID, FIDSA, FCCP, FCSHP. 2022
“The Top Drugs for Older Adults to Avoid” NextAvenue . November 22, 2016
Disclaimer: This information is not intended to be a substitute for professional medical advice, diagnosis or treatment and is for information only. Always seek the advice of your physician or another qualified health provider with any questions about your medical condition and/or current medication. Do not disregard professional medical advice or delay seeking advice or treatment because of something you have read here.
This article was originally published on November 3, 2017, and has since been updated.
The post Top Drugs With Greater Health Risks for People Over 65 appeared first on The Hearty Soul .

IMAGES
VIDEO
COMMENTS
Global spending on prescription drugs in 2020 is expected to be ~$1.3 trillion; the United States alone will spend ~$350 billion 1. These high spending rates are expected to increase at a rate of ...
Nonfederal hospitals accounted for $39.6 billion in prescription expenditures in 2020, an increase of 8.4% in 2021 after a historic decrease in 2020, whereas in clinics spending grew 7.7% to $105.0 billion. For 2022, we expect overall prescription drug spending to rise by 4.0% to 6.0%, whereas in clinics and hospitals we anticipate increases of ...
The papers in this special issue include a sampling of the latest research on the epidemiology, clinical correlates, treatment, and public policy considerations of prescription drug abuse. Although much has been learned about prescription drug abuse in recent years, this research remains in early stages, particularly with respect to ...
Introduction. Prescription drug use in the United States has reached record levels, rising to 6.3 billion prescriptions—approximately 19 prescriptions for every American—filled in 2020 alone (IQVIA Institute for Human Data Science 2021).Now "the most common therapeutic intervention" (World Health Organization [WHO] 2019), the modern prescription drug only emerged around the mid ...
Most research designed to answer the "why" of the prescription opioid epidemic has relied on structured interviews, which rigidly attempt to capture the complex reasons people use opioids. In contrast this systematic literature review focuses on peer-reviewed studies that have used a qualitative approach to examine the development of an ...
Prescription drug monitoring programs (PDMPs) are used to mitigate harms from high-risk medicines including misuse, prescription shopping, overdoses, and death. ... Observational research designs • Papers with quantitative data or measures association between PDMP and prescription opioid related outcome.
Overview. Misuse of prescription drugs means taking a medication in a manner or dose other than prescribed; taking someone else's prescription, even if for a legitimate medical complaint such as pain; or taking a medication to feel euphoria (i.e., to get high). The term nonmedical use of prescription drugs also refers to these categories of ...
Prescription drug misuse (PDM), or medication use without a prescription or in ways not intended by the prescriber, is a notable public health concern, especially in the United States. Accumulating research has characterized PDM prevalence and processes, but age-based or lifespan changes in PDM are understudied.
R&D Spending. R&D spending in the pharmaceutical industry covers a variety of activities, including the following: • Invention, or research and discovery of new drugs; • Development, or clinical testing, preparation and submission of applications for FDA approval, and design of production processes for new drugs;
Misuse of Prescription Drugs Research Report | En español. June 2020 | Offers the latest research findings on prescription drug misuse ... Explores the relationship between prescription opioid abuse and heroin use, including prescription opioid use as a risk factor for heroin use, reasons why people progress from using ...
Prescription drug prices in the US are more than 2.5 times as high as those in other similar high-income nations and are a leading health care concern among US residents. Given these factors, and in response to President Biden's executive order promoting competition, the US Department of Health and Human Services (HHS) released a comprehensive plan to address drug prices in September 2021. 1 ...
Objectives. : This systematic. review was conducted to improve the body of knowledge on three main aspects of online pharmacies: (1) type and characteristics of the online pharmacies selling drugs ...
manufacturing sources of generic prescription drugs between 2017, based on 2013 and previously non-public data provided us by the FDA. 10. Here, we report updated findings through . 5. Rena M. Conti and Ernst R. Berndt, "Four Facts Concerning Competition in U.S. Generic Prescription Drug Markets", International Journal o fthe Economics o ...
The validity of self-reported drug use in survey research: an overview and critique of research methods. ... and 2 of 6 randomly assigned survey forms for 12th graders. Across the years, paper or tablet self-administered surveys have been administered in schools. ... Wilens TE, McCabe SE. Prescription drug misuse: sources of controlled ...
This report defines misuse of prescription drugs and describes the extent of prescription drug misuse in the United States. It also provides information about the safety of using prescription drugs in combination with other medicines, describes ways to prevent and treat prescription drug misuse and addiction, and lists resources that provide more information.
Putting the U.S. generic drug industry into context, in Table 1 we report descriptive statistics of our sample of brand and generic manufacturers and molecule markets by year. Approximately 500-650 manufacturers are in our data between 2004 through 2016. The count of manufacturers increases roughly linearly over time.
The drug development process requires investments, estimated at between $60 million to $2.6 billion,[6;67;68;77] though most estimations are close to $800 million from bench research to prescription medicine.[33;66;72;84;85] The wide range of cost estimates is due to the lack of clear data and various methods of calculation, and depends on the ...
Several states in the U.S. have piloted a type of subscription model for prescription drugs, under which a state pays a fixed amount to a pharmaceutical manufacturer for a targeted population (e.g., inmates) over a contract horizon. ... (May 19, 2021). Johns Hopkins Carey Business School Research Paper No. 20-11, Available at SSRN: https://ssrn ...
Results: From 30,784 S records of 2479 patients with at least 1 prescription of anticancer drugs, our deep learning models extracted true adverse event signals with more than 80% accuracy for both hand-foot syndrome (n=152, 91%) and adverse events limiting patients' daily lives (n=157, 80.1%).
Rationale & objectives ± 3,4-Methylenedioxymethamphetamine (MDMA) and psilocybin are currently moving through the US Food and Drug Administration's phased drug development process for psychiatric treatment indications: posttraumatic stress disorder and depression, respectively. The current standard of care for these disorders involves treatment with psychiatric medications (e.g., selective ...
GENERIC PRESCRIPTION IN INDIA - A REVIEW. VIGNESH M, GANESH GNK*. Department of Pharmaceutics, JSS College of Pharmacy, JSS Academy of Higher Education and Research, Ooty, T amil Nadu, India ...
Oral prescription drugs often disturb the gut microbiome, killing off some species or changing the balance in a way that impacts patient health. In other combinations, bacteria get the upper hand ...
smoking of cigarettes and gradually drowns the person into the trap of drug abuse. Stress, anxiety, peer pressure, poverty are some of the main causes of drug abuse.As is well said -"it is ...
The Centers for Disease Control and Prevention has reported that four in 10 Americans aged 65 or over had been taking at least five prescription drugs as recently as 2018. Half or more of the drugs would be metabolized by the same enzyme, Tao noted. One drug may interfere with another drug through affecting the enzyme's activity.
When discussing prescription drug ... and Gregg Girvan's op-ed on drug prices and innovation with a focus on small companies that are the force driving research and development of new drugs.
Most PDP enrollees will face much higher cost sharing for brands than for generic drugs in 2024, as in prior years, including coinsurance for non-preferred drugs of 50% (the maximum coinsurance ...
Abstract. The current opioid epidemic is one of the most severe public health crisis in US history. Responding to it has been difficult due to its rapidly changing nature and the severity of its associated outcomes. This review examines the origin and evolution of the crisis, the pharmacological properties of opioids, the neurobiology of opioid ...
Large blocks of data must be analyzed and explored by utilizing the data mining procedures in order to uncover significant patterns and trends. Medical databases are one area where the data mining procedures can be utilized. Many people all over the world are struggling with their health and medical diagnoses. Massive amounts of data are produced by hospital information systems (HIS), yet it ...
The fully revised and expanded Global Pharma Handbook provides pharmaceutical industry decision-makers with a unique source of accurate, up-to-date information and analysis on prescription drug ...
W e all know even the best of medications come with side-effects, but there's a large portion of the population that's even more vulnerable to the health risks of drugs than everyone else. If ...