IEEE Account
- Change Username/Password
- Update Address

Purchase Details
- Payment Options
- Order History
- View Purchased Documents
Profile Information
- Communications Preferences
- Profession and Education
- Technical Interests
- US & Canada: +1 800 678 4333
- Worldwide: +1 732 981 0060
- Contact & Support
- About IEEE Xplore
- Accessibility
- Terms of Use
- Nondiscrimination Policy
- Privacy & Opting Out of Cookies
A not-for-profit organization, IEEE is the world's largest technical professional organization dedicated to advancing technology for the benefit of humanity. © Copyright 2024 IEEE - All rights reserved. Use of this web site signifies your agreement to the terms and conditions.
Information
- Author Services
Initiatives
You are accessing a machine-readable page. In order to be human-readable, please install an RSS reader.
All articles published by MDPI are made immediately available worldwide under an open access license. No special permission is required to reuse all or part of the article published by MDPI, including figures and tables. For articles published under an open access Creative Common CC BY license, any part of the article may be reused without permission provided that the original article is clearly cited. For more information, please refer to https://www.mdpi.com/openaccess .
Feature papers represent the most advanced research with significant potential for high impact in the field. A Feature Paper should be a substantial original Article that involves several techniques or approaches, provides an outlook for future research directions and describes possible research applications.
Feature papers are submitted upon individual invitation or recommendation by the scientific editors and must receive positive feedback from the reviewers.
Editor’s Choice articles are based on recommendations by the scientific editors of MDPI journals from around the world. Editors select a small number of articles recently published in the journal that they believe will be particularly interesting to readers, or important in the respective research area. The aim is to provide a snapshot of some of the most exciting work published in the various research areas of the journal.
Original Submission Date Received: .
- Active Journals
- Find a Journal
- Proceedings Series
- For Authors
- For Reviewers
- For Editors
- For Librarians
- For Publishers
- For Societies
- For Conference Organizers
- Open Access Policy
- Institutional Open Access Program
- Special Issues Guidelines
- Editorial Process
- Research and Publication Ethics
- Article Processing Charges
- Testimonials
- Preprints.org
- SciProfiles
- Encyclopedia
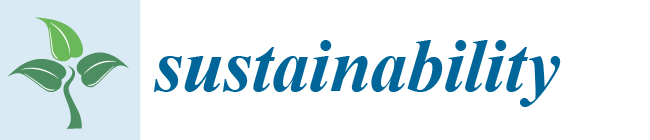
Journal Menu
- Sustainability Home
- Aims & Scope
- Editorial Board
- Reviewer Board
- Topical Advisory Panel
- Instructions for Authors
- Special Issues
- Sections & Collections
- Article Processing Charge
- Indexing & Archiving
- Editor’s Choice Articles
- Most Cited & Viewed
- Journal Statistics
- Journal History
- Journal Awards
- Society Collaborations
- Conferences
- Editorial Office
Journal Browser
- arrow_forward_ios Forthcoming issue arrow_forward_ios Current issue
- Vol. 16 (2024)
- Vol. 15 (2023)
- Vol. 14 (2022)
- Vol. 13 (2021)
- Vol. 12 (2020)
- Vol. 11 (2019)
- Vol. 10 (2018)
- Vol. 9 (2017)
- Vol. 8 (2016)
- Vol. 7 (2015)
- Vol. 6 (2014)
- Vol. 5 (2013)
- Vol. 4 (2012)
- Vol. 3 (2011)
- Vol. 2 (2010)
- Vol. 1 (2009)
Find support for a specific problem in the support section of our website.
Please let us know what you think of our products and services.
Visit our dedicated information section to learn more about MDPI.
Dynamic Traffic Assignment and Sustainable Transport Systems
- Print Special Issue Flyer
Special Issue Editors
Special issue information.
- Published Papers
A special issue of Sustainability (ISSN 2071-1050). This special issue belongs to the section " Sustainable Transportation ".
Deadline for manuscript submissions: closed (31 July 2023) | Viewed by 10182
Share This Special Issue

Dear Colleagues,
Dynamic traffic assignment (DTA) is one of the most important foundational theories in intelligent transportation systems (ITSs). DTA models and technologies could be used in the field of traffic planning, traffic control and management, transportation policy evaluation and online transportation systems. In recent years, technological advances have paved the way for the development of transportation systems, and have had a huge impact on the research of dynamic traffic assignment. Advanced technologies such as artificial intelligence, autonomous driving, wireless communication, and electric vehicles provide users with real-time information about traffic conditions and allow travelers to choose different travel modes, travel routes and real-time decisions. Such advanced technologies may have made the basis of DTA models’ change, such as the travel choice principle, travel demand evaluation, and traffic behaviors. Further, the application scenarios and effects of DTA models will also change greatly. All of this will have enormous potential for enhancing the sustainability of transportation systems.
In this Special Issue, we invite the submission of research papers that specifically address the potential related advanced technologies with dynamic traffic assignment models for enhancing the sustainability of transportation systems. The scope of this Special Issue is to cover DTA model and theory, DTA with autonomous driving, electric vehicles, shared traffic and other related advanced technologies. Topics of interest with a general focus on dynamic traffic assignment and sustainable transport systems include but are not limited to:
- Dynamic traffic assignment model and theory;
- Travel choice principle and traffic flow propagation models;
- Effective algorithms for solving DTA problems;
- Online DTA model and efficiency;
- Application of DTA models for traffic management and control;
- DTA model and application under autonomous driving environment;
- DTA model and application under electric vehicle environment;
- DTA model and application under shared traffic environment;
- DTA model and application under other advanced technologies environment;
- DTA and sustainable transport systems.
Dr. Zhiheng Li Dr. Jiyuan Tan Dr. Kai Zhang Dr. Yang Zhou Guest Editors
Manuscripts should be submitted online at www.mdpi.com by registering and logging in to this website . Once you are registered, click here to go to the submission form . Manuscripts can be submitted until the deadline. All submissions that pass pre-check are peer-reviewed. Accepted papers will be published continuously in the journal (as soon as accepted) and will be listed together on the special issue website. Research articles, review articles as well as short communications are invited. For planned papers, a title and short abstract (about 100 words) can be sent to the Editorial Office for announcement on this website.
Submitted manuscripts should not have been published previously, nor be under consideration for publication elsewhere (except conference proceedings papers). All manuscripts are thoroughly refereed through a single-blind peer-review process. A guide for authors and other relevant information for submission of manuscripts is available on the Instructions for Authors page. Sustainability is an international peer-reviewed open access semimonthly journal published by MDPI.
Please visit the Instructions for Authors page before submitting a manuscript. The Article Processing Charge (APC) for publication in this open access journal is 2400 CHF (Swiss Francs). Submitted papers should be well formatted and use good English. Authors may use MDPI's English editing service prior to publication or during author revisions.
- dynamic traffic assignment
- sustainable transport systems
- advanced technology
Published Papers (5 papers)
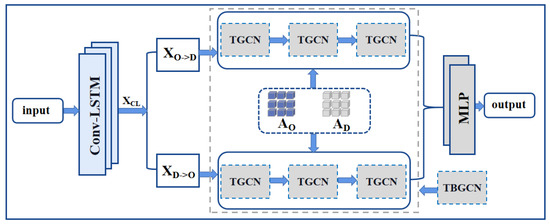
Further Information
Mdpi initiatives, follow mdpi.
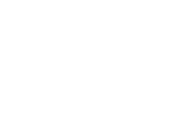
Subscribe to receive issue release notifications and newsletters from MDPI journals

Dynamic Traffic Assignment
Early Experiences
Current Practices
Research Needs
Page categories
Activity Based Models
Network Assignment
Topic Circles
More pages in this category:
(opens new window) is a hot topic in travel forecasting.
# Background
Traditional user equilibrium highway assignment models predict the effects of congestion and the routing changes of traffic as a result of that congestion. They neglect, however, many of the details of real-world traffic operations, such as queuing, shock waves, and signalization. Currently, it is common practice to feed the results of user equilibrium traffic assignments into dynamic network models as a mechanism for evaluating these policies. The simulation models themselves, however, do not predict the routing of traffic, and therefore are unable to account for re-routing owing to changes in congestion levels or policy, and can be inconsistent with the routes determined by the assignment. Dynamic network models overcome this dichotomy by combining a time-dependent shortest path algorithm with some type of simulation (often meso or macroscopic) of link travel times and delay. In doing so it allows added reality and consistency in the assignment step, as well as the ability to evaluate policies designed to improve traffic operations. These are some of the main benefits of dynamic network models .
DTA models can generally be classified by how they model link or intersection delay. Analytical DTA models treat it in the same manner as static equilibrium assignment models, with no explicit representation of signals. Link capacity functions, often similar or identical to those used in static assignment, are used to calculate link travel times. Analytical models have been widely used in research and for real-time control system applications. Simulation-based DTA models include explicit representation of traffic control devices. Such models require detailed signal parameters to include phasing, cycle length, and offsets for each signal in the network. Delay is calculated for each approach, with vehicles moving from one link to the next only if available downstream capacity is available. The underlying traffic model is often different, but at the network level such models behave in a similar fashion.
Demand is specified in the form of origin–destination matrices for short time intervals, typically 15 minutes each. Trips are typically randomly loaded onto the network during each time interval. As with traffic microsimulation models, adequate downstream capacity must be present to load the trips onto the network. The shortest paths through time and space are found for each origin–destination pair, and flows loaded to these paths. A generalized flowchart of the process is shown below.

As with static assignment models, the process shown above is iteratively solved until a stable solution is reached. The memory and computing requirements of DTA, however, are orders of magnitude larger than for static assignment, reducing the number of iterations and paths that can be kept in memory. Instead of a single time period, as with static assignment, DTA models must store data for each time interval as well. A three-hour static assignment would involve only one time interval. A DTA model of the same period, however, might require 12 intervals, each 15 minutes in duration. These are all in addition to the memory requirements imposed by the number of user classes and zones.
# Early Experiences
Research into DTA dates back several decades, but was largely limited to academics working on its formulation and theoretical aspects. DTA overcomes the limitations of static assignment models, although at the cost of increased data requirements and computational burden. Moreover, software platforms capable of solving the DTA problem for large urban systems and experience in their use are recent developments.
(opens new window) has been successfully applied to a large subarea of Calgary and to analyses of the Rue Notre-Dame in Montreal. Although user group presentations of both applications have been made, and reported very encouraging results, the work is currently unpublished and inaccessible except through contact with the developers.
(opens new window) . The network from the Atlanta Regional Commission (ARC) regional travel model formed the starting point for the DTA network. Intersections were coded, centroid connectors were re-defined, and network coding errors were corrected. A signal synthesizer derived locally optimal timing parameters for more than 2,200 signalized intersections in the network. Trip matrices from the ARC model were divided into 15-minute intervals for the specification of demand. Approximately 40 runs of the model were required to diagnose coding and software errors. Unfortunately, the execution time for the model was approximately one week per run. The resulting model eventually validated well to observed conditions; however, the length of time required to render it operational and the run time required prevented it from being used in studies as originally intended. Subsequent work by the developer has resulted in substantial reductions in run time, but this remains a significant issue that must be overcome before such models can be more widely used.
# Current Practices
# research needs.
A number of cities are currently testing DTA models, but are not far enough along in their work to share even preliminary results. At least a dozen such cases are known to be in varying stages of planning or execution, suggesting that the use of DTA models in planning applications is about to expand dramatically. However, in addition to the issue of long run times, a number of other issues must be addressed before such models are likely to be widely adopted:
- Criteria for the validation of such models have not been widely accepted. The paucity of traffic counts in most urban areas, and especially at 15, 30, or 60 minute intervals, is a significant barrier to definitive assessment of these models.
← Network assignment Land Use-Transport Modeling →
This site uses cookies to learn which topics interest our readers.
- View Record
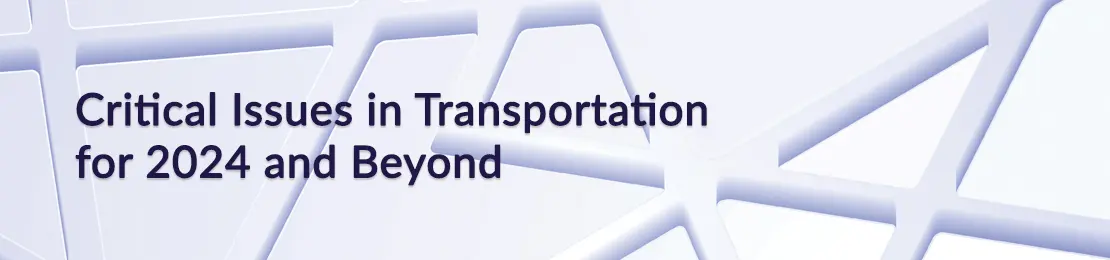
TRID the TRIS and ITRD database
Using the General Link Transmission Model in a Dynamic Traffic Assignment to Simulate Congestion on Urban Networks
This article presents two new models of Dynamic User Equilibrium that are particularly suited for intelligent transportation systems (ITS) applications, where the evolution of vehicle flows and travel times must be simulated on large road networks, possibly in real-time. The key feature of the proposed models is the detail representation of the main congestion phenomena occurring at nodes of urban networks, such as vehicle queues and their spillback, as well as flow conflicts in mergins and diversions. Compared to the simple word of static assignment, where only the congestion along the arc is typically reproduced through a separable relation between vehicle flow and travel time, this type of dynamic traffic assignment (DTA) models are much more complex, as the above relation becomes non-separable, both in time and space. Traffic simulation is here attained through a macroscopic flow model, that extends the theory of kinematic waves to urban networks and non-linear fundamental diagrams: the General Link Transmission Model (GLTM). The sub-models of the GLTM, namely the Node Intersection Model, the Forward Propagation Model of vehicles and the Backward Propagation Model of spaces, can be combined in two different ways to produce arc travel times starting from turn flows. The first approach is to consider short time intervals of a few seconds and process all nodes for each temporal layer in chronological order. The second approach allows to consider long time intervals of a few minutes and for each sub-model requires to process the whole temporal profile of involved variables. The two resulting DTA models are here analyzed and compared with the aim of identifying their possible use cases. A rigorous mathematical formulation is out of the scope of this paper, as well as a detailed explanation of the solution algorithm. The dynamic equilibrium is anyhow sought through a new method based on Gradient Projection, which is capable to solve both proposed models with any desired precision in a reasonable number of iterations. Its fast convergence is essential to show that the two proposed models for network congestion actually converge at equilibrium to nearly identical solutions in terms of arc flows and travel times, despite their two diametrical approaches wrt the dynamic nature of the problem, as shown in the numerical tests presented here.
- Record URL: https://doi.org/10.1016/j.trpro.2015.01.011
- Record URL: http://www.sciencedirect.com/science/article/pii/S2352146515000125
- Find a library where document is available. Order URL: http://worldcat.org/issn/23521465
- © 2013 Guido Gentile. Published by Elsevier B.V.
- Gentile, Guido
- Publication Date: 2015
- Media Type: Digital/other
- Features: Figures; References; Tables;
- Pagination: pp 66-81
- Transportation Research Procedia
- Issue Number: 0
- Publisher: Elsevier
- ISSN: 2352-1465
- Serial URL: http://www.sciencedirect.com/science/journal/23521465/
Open Access (libre)
Subject/Index Terms
- TRT Terms: Dynamic traffic assignment ; Intelligent transportation systems ; Macroscopic traffic flow ; Traffic assignment ; Traffic congestion ; Traffic forecasting ; Traffic simulation ; Travel time
- Uncontrolled Terms: Dynamic user equilibrium
- Subject Areas: Highways; Operations and Traffic Management; Planning and Forecasting; I72: Traffic and Transport Planning;
Filing Info
- Accession Number: 01554671
- Record Type: Publication
- Files: TRIS
- Created Date: Feb 26 2015 9:53AM
- DSpace@MIT Home
- MIT Open Access Articles
A dynamic traffic assignment model for highly congested urban networks

Publisher with Creative Commons License
Creative Commons Attribution
Terms of use
Date issued, collections.
Show Statistical Information

- Nov. 6-8, 2024 San Diego, CA
Popular Topics
- [Webinar] Fleet Editors' EV Market Roundtable
- Sandor Piszar Discusses New Role, GM Envolve for 2024 [Watch]
- Outlook 2024: Is Fleet Back to Normal?
- 2024 Work Truck Week in Photos
- Got Compliance? DOT GVWR Questions Answered

Connect with us
- Remarketing
- Global Fleet
- Green Fleet
- Maintenance
- Vehicle Research
- Fleet Forward
- Encyclopedia
- Whitepapers
- Contributed Content
- Subscription
- Terms and Conditions
- Cookie Settings
- Newsletter Sign Up
- Marketing Solutions
World's Worst Traffic In Moscow, TomTom Says
July 22, 2014 • Staff • Bookmark +

Photo of Moscow congestion from 2012 via Sergey Ivanov/Flickr.
Drivers in Moscow during the most congested periods will spend an average of 74 percent more time getting to their destination in a city TomTom has ranked as the most congested around the globe.
That would equate to an average delay of 76 minutes per hour during peak travel times. The finding came in the annual global traffic index from the Amsterdam-based navigation and traffic services company.
Commuters spend an average of eight days per year stuck in traffic jams. Traffic on secondary routes can be more congested, the study found.
The annual traffic index covers 180 global cities, and compares travel times during non-congested hours to those in peak hours. The index takes into consideration major highways and local roads.
Only one U.S. city, Los Angeles, made the list of the 10 most congested cities, according to TomTom. Here's the full list with the average percent increase in traffic times:
- Moscow, Russia: 74 percent
- Istanbul, Turkey: 62 percent
- Rio de Janeiro, Brazil: 55 percent
- Mexico City, Mexico: 54 percent
- São Paulo, Brazil: 46 percent
- Palermo, Italy: 39 percent
- Warsaw, Poland: 39 percent
- Rome, Italy: 37 percent
- Los Angeles, USA: 36 percent
- Dublin, Ireland: 35 percent
For the full report, click here .
Comments are moderated and may not appear for 24 hours or more. Comments that include personal attacks or slurs, hate speech, demonstrably false information, excessive profanity, or that are thinly veiled promotions for a product, will not be approved.
See all comments
We respect your data and privacy. By clicking the submit button below, you are agreeing with Bobit Business Media’s Privacy Policy and this outlined level of consent .
- View the latest:
- Fleet Trends & News
- Fleet Whitepapers
- Fleet Safety
Related Sites
- Auto Rental News
- Business Fleet
- Fleet Financials
- Government Fleet
- Heavy Duty Trucking
- School Bus Fleet
- Vehicle Remarketing
Create your free Bobit Connect account to bookmark content.
The secure and easy all-access connection to your content. Bookmarked content can then be accessed anytime on all of your logged in devices!
Already a member? Log In
We've detected unusual activity from your computer network
To continue, please click the box below to let us know you're not a robot.
Why did this happen?
Please make sure your browser supports JavaScript and cookies and that you are not blocking them from loading. For more information you can review our Terms of Service and Cookie Policy .
For inquiries related to this message please contact our support team and provide the reference ID below.
Data-Driven Traffic Assignment: A Novel Approach for Learning Traffic Flow Patterns Using Graph Convolutional Neural Network
- Published: 24 July 2023
- Volume 5 , article number 11 , ( 2023 )
Cite this article
- Rezaur Rahman 1 &
- Samiul Hasan 1
583 Accesses
5 Citations
Explore all metrics
We present a novel data-driven approach of learning traffic flow patterns of a transportation network given that many instances of origin to destination (OD) travel demand and link flows of the network are available. Instead of estimating traffic flow patterns assuming certain user behavior (e.g., user equilibrium or system optimal), here we explore the idea of learning those flow patterns directly from the data. To implement this idea, we have formulated the traditional traffic assignment problem (from the field of transportation science) as a data-driven learning problem and developed a neural network-based framework known as Graph Convolutional Neural Network (GCNN) to solve it. The proposed framework represents the transportation network and OD demand in an efficient way and utilizes the diffusion process of multiple OD demands from nodes to links. We validate the solutions of the model against analytical solutions generated from running static user equilibrium-based traffic assignments over Sioux Falls and East Massachusetts networks. The validation results show that the implemented GCNN model can learn the flow patterns very well with less than 2% mean absolute difference between the actual and estimated link flows for both networks under varying congested conditions. When the training of the model is complete, it can instantly determine the traffic flows of a large-scale network. Hence, this approach can overcome the challenges of deploying traffic assignment models over large-scale networks and open new directions of research in data-driven network modeling.
This is a preview of subscription content, log in via an institution to check access.
Access this article
Subscribe and save.
- Get 10 units per month
- Download Article/Chapter or Ebook
- 1 Unit = 1 Article or 1 Chapter
- Cancel anytime
Price includes VAT (Russian Federation)
Instant access to the full article PDF.
Rent this article via DeepDyve
Institutional subscriptions
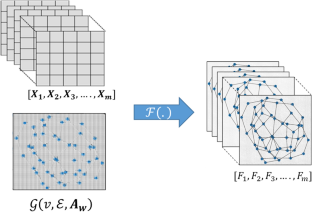
Similar content being viewed by others
Modeling Urban Traffic Data Through Graph-Based Neural Networks
Predicting Taxi Hotspots in Dynamic Conditions Using Graph Neural Network
MTGCN: A Multitask Deep Learning Model for Traffic Flow Prediction
Data availability.
The data that support the findings of this study are available from the corresponding author, [[email protected]], upon reasonable request.
Abdelfatah AS, Mahmassani HS (2002) A simulation-based signal optimization algorithm within a dynamic traffic assignment framework 428–433. https://doi.org/10.1109/itsc.2001.948695
Alexander L, Jiang S, Murga M, González MC (2015) Origin-destination trips by purpose and time of day inferred from mobile phone data. Transp Res Part C Emerg Technol 58:240–250. https://doi.org/10.1016/j.trc.2015.02.018
Article Google Scholar
Atwood J, Towsley D (2015) Diffusion-convolutional neural networks. Adv Neural Inf Process Syst. https://doi.org/10.5555/3157096.3157320
Ban XJ, Liu HX, Ferris MC, Ran B (2008) A link-node complementarity model and solution algorithm for dynamic user equilibria with exact flow propagations. Transp Res Part B Methodol 42:823–842. https://doi.org/10.1016/j.trb.2008.01.006
Bar-Gera H (2002) Origin-based algorithm for the traffic assignment problem. Transp Sci 36:398–417. https://doi.org/10.1287/trsc.36.4.398.549
Article MATH Google Scholar
Barthélemy J, Carletti T (2017) A dynamic behavioural traffic assignment model with strategic agents. Transp Res Part C Emerg Technol 85:23–46. https://doi.org/10.1016/j.trc.2017.09.004
Ben-Akiva ME, Gao S, Wei Z, Wen Y (2012) A dynamic traffic assignment model for highly congested urban networks. Transp Res Part C Emerg Technol 24:62–82. https://doi.org/10.1016/j.trc.2012.02.006
Billings D, Jiann-Shiou Y (2006) Application of the ARIMA Models to Urban Roadway Travel Time Prediction-A Case Study. Systems, Man and Cybernetics, 2006. SMC’06. IEEE International Conference on 2529–2534
Boyles S, Ukkusuri SV, Waller ST, Kockelman KM (2006) A comparison of static and dynamic traffic assignment under tolls: a study of the dallas-fort worth network. 85th Annual Meeting of 14
Brandes U (2001) A faster algorithm for betweenness centrality. J Math Sociol 25:163–177. https://doi.org/10.1080/0022250X.2001.9990249
Cai P, Wang Y, Lu G, Chen P, Ding C, Sun J (2016) A spatiotemporal correlative k-nearest neighbor model for short-term traffic multistep forecasting. Transp Res Part C Emerg Technol 62:21–34. https://doi.org/10.1016/j.trc.2015.11.002
Chien SI-J, Kuchipudi CM (2003) Dynamic travel time prediction with real-time and historic data. J Transp Eng 129:608–616. https://doi.org/10.1061/(ASCE)0733-947X(2003)129:6(608)
Cui Z, Henrickson K, Ke R, Wang Y (2018a) Traffic graph convolutional recurrent neural network: a deep learning framework for network-scale traffic learning and forecasting. IEEE Trans Intell Transp Syst. https://doi.org/10.1109/TITS.2019.2950416
Cui Z, Ke R, Pu Z, Wang Y (2018b) Deep bidirectional and unidirectional LSTM recurrent neural network for network-wide traffic speed prediction. International Workshop on Urban Computing (UrbComp) 2017
Cui Z, Lin L, Pu Z, Wang Y (2020) Graph Markov network for traffic forecasting with missing data. Transp Res Part C Emerg Technol 117:102671. https://doi.org/10.1016/j.trc.2020.102671
Defferrard M, Bresson X, Vandergheynst P (2016) Convolutional neural networks on graphs with fast localized spectral filtering. Adv Neural Inf Process Syst 3844–3852
Deshpande M, Bajaj PR (2016) Performance analysis of support vector machine for traffic flow prediction. 2016 International Conference on Global Trends in Signal Processing, Information Computing and Communication (ICGTSPICC) 126–129. https://doi.org/10.1109/ICGTSPICC.2016.7955283
Elhenawy M, Chen H, Rakha HA (2014) Dynamic travel time prediction using data clustering and genetic programming. Transp Res Part C Emerg Technol 42:82–98. https://doi.org/10.1016/j.trc.2014.02.016
Foytik P, Jordan C, Robinson RM (2017) Exploring simulation based dynamic traffic assignment with large scale microscopic traffic simulation model
Friesz TL, Luque J, Tobin RL, Wie BW (1989) Dynamic network traffic assignment considered as a continuous time optimal control problem. Oper Res 37:893–901. https://doi.org/10.2307/171471
Article MathSciNet MATH Google Scholar
Gundlegård D, Rydergren C, Breyer N, Rajna B (2016) Travel demand estimation and network assignment based on cellular network data. Comput Commun 95:29–42. https://doi.org/10.1016/j.comcom.2016.04.015
Guo S, Lin Y, Feng N, Song C, Wan H (2019) Attention based spatial-temporal graph convolutional networks for traffic flow forecasting. Proceed AAAI Conf Artif Intell 33:922–929. https://doi.org/10.1609/aaai.v33i01.3301922
Guo K, Hu Y, Qian Z, Sun Y, Gao J, Yin B (2020) Dynamic graph convolution network for traffic forecasting based on latent network of Laplace matrix estimation. IEEE Trans Intell Transp Syst 23:1009–1018. https://doi.org/10.1109/tits.2020.3019497
Guo K, Hu Y, Qian Z, Liu H, Zhang K, Sun Y, Gao J, Yin B (2021) Optimized graph convolution recurrent neural network for traffic prediction. IEEE Trans Intell Transp Syst 22:1138–1149. https://doi.org/10.1109/TITS.2019.2963722
Hammond DK, Vandergheynst P, Gribonval R (2011) Wavelets on graphs via spectral graph theory. Appl Comput Harmon Anal 30:129–150. https://doi.org/10.1016/j.acha.2010.04.005
He X, Guo X, Liu HX (2010) A link-based day-to-day traffic assignment model. Transp Res Part B 44:597–608. https://doi.org/10.1016/j.trb.2009.10.001
Huang Y, Kockelman KM (2019) Electric vehicle charging station locations: elastic demand, station congestion, and network equilibrium. Transp Res D Transp Environ. https://doi.org/10.1016/j.trd.2019.11.008
Innamaa S (2005) Short-term prediction of travel time using neural networks on an interurban highway. Transportation (amst) 32:649–669. https://doi.org/10.1007/s11116-005-0219-y
Jafari E, Pandey V, Boyles SD (2017) A decomposition approach to the static traffic assignment problem. Transp Res Part b Methodol 105:270–296. https://doi.org/10.1016/j.trb.2017.09.011
Janson BN (1989) Dynamic traffic assignment for urban road networks. Transp Res Part B Methodol 25:143–161
Ji X, Shao C, Wang B (2016) Stochastic dynamic traffic assignment model under emergent incidents. Procedia Eng 137:620–629. https://doi.org/10.1016/j.proeng.2016.01.299
Jiang Y, Wong SC, Ho HW, Zhang P, Liu R, Sumalee A (2011) A dynamic traffic assignment model for a continuum transportation system. Transp Res Part b Methodol 45:343–363. https://doi.org/10.1016/j.trb.2010.07.003
Kim H, Oh JS, Jayakrishnan R (2009) Effects of user equilibrium assumptions on network traffic pattern. KSCE J Civ Eng 13:117–127. https://doi.org/10.1007/s12205-009-0117-5
Kim TS, Lee WK, Sohn SY (2019) Graph convolutional network approach applied to predict hourly bike-sharing demands considering spatial, temporal, and global effects. PLoS ONE 14:e0220782. https://doi.org/10.1371/journal.pone.0220782
Kipf TN, Welling M (2016) Semi-supervised classification with graph convolutional networks. 5th International Conference on Learning Representations, ICLR 2017- Conference Track Proceedings
LeBlanc LJ, Morlok EK, Pierskalla WP (1975) An efficient approach to solving the road network equilibrium traffic assignment problem. Transp Res 9:309–318. https://doi.org/10.1016/0041-1647(75)90030-1
Lee YLY (2009) Freeway travel time forecast using artifical neural networks with cluster method. 2009 12th International Conference on Information Fusion 1331–1338
Leon-Garcia A, Tizghadam A (2009) A graph theoretical approach to traffic engineering and network control problem. 21st International Teletraffic Congress, ITC 21: Traffic and Performance Issues in Networks of the Future-Final Programme
Leurent F, Chandakas E, Poulhès A (2011) User and service equilibrium in a structural model of traffic assignment to a transit network. Procedia Soc Behav Sci 20:495–505. https://doi.org/10.1016/j.sbspro.2011.08.056
Li Y, Yu R, Shahabi C, Liu Y (2018) Diffusion convolutional recurrent neural network: Data-driven traffic forecasting. 6th International Conference on Learning Representations, ICLR 2018-Conference Track Proceedings 1–16
Li G, Knoop VL, van Lint H (2021) Multistep traffic forecasting by dynamic graph convolution: interpretations of real-time spatial correlations. Transp Res Part C Emerg Technol 128:103185. https://doi.org/10.1016/j.trc.2021.103185
Lin L, He Z, Peeta S (2018) Predicting station-level hourly demand in a large-scale bike-sharing network: a graph convolutional neural network approach. Transp Res Part C 97:258–276. https://doi.org/10.1016/j.trc.2018.10.011
Liu HX, Ban X, Ran B, Mirchandani P (2007) Analytical dynamic traffic assignment model with probabilistic travel times and perceptions. Transp Res Record 1783:125–133. https://doi.org/10.3141/1783-16
Liu Y, Zheng H, Feng X, Chen Z (2017) Short-term traffic flow prediction with Conv-LSTM. 2017 9th International Conference on Wireless Communications and Signal Processing, WCSP 2017-Proceedings. https://doi.org/10.1109/WCSP.2017.8171119
Lo HK, Szeto WY (2002) A cell-based variational inequality formulation of the dynamic user optimal assignment problem. Transp Res Part b Methodol 36:421–443. https://doi.org/10.1016/S0191-2615(01)00011-X
Luo X, Li D, Yang Y, Zhang S (2019) Spatiotemporal traffic flow prediction with KNN and LSTM. J Adv Transp. https://doi.org/10.1155/2019/4145353
Ma X, Tao Z, Wang Y, Yu H, Wang Y (2015) Long short-term memory neural network for traffic speed prediction using remote microwave sensor data. Transp Res Part C Emerg Technol 54:187–197. https://doi.org/10.1016/j.trc.2015.03.014
Ma X, Dai Z, He Z, Ma J, Wang Y, Wang Y (2017) Learning traffic as images: a deep convolutional neural network for large-scale transportation network speed prediction. Sensors 17:818. https://doi.org/10.3390/s17040818
Mahmassani HaniS (2001) Dynamic network traffic assignment and simulation methodology for advanced system management applications. Netw Spat Econ 1:267–292. https://doi.org/10.1023/A:1012831808926
Merchant DK, Nemhauser GL (1978) A model and an algorithm for the dynamic traffic assignment problems. Transp Sci 12:183–199. https://doi.org/10.1287/trsc.12.3.183
Mitradjieva M, Lindberg PO (2013) The stiff is moving—conjugate direction Frank-Wolfe methods with applications to traffic assignment * . Transp Sci 47:280–293. https://doi.org/10.1287/trsc.1120.0409
Myung J, Kim D-K, Kho S-Y, Park C-H (2011) Travel time prediction using k nearest neighbor method with combined data from vehicle detector system and automatic toll collection system. Transp Res Record 2256:51–59. https://doi.org/10.3141/2256-07
Nie YM, Zhang HM (2010) Solving the dynamic user optimal assignment problem considering queue spillback. Netw Spat Econ 10:49–71. https://doi.org/10.1007/s11067-007-9022-y
Peeta S, Mahmassani HS (1995) System optimal and user equilibrium time-dependent traffic assignment in congested networks. Ann Oper Res. https://doi.org/10.1007/BF02031941
Peeta S, Ziliaskopoulos AK (2001) Foundations of dynamic traffic assignment: the past, the present and the future. Netw Spat Econ 1:233–265
Peng H, Wang H, Du B, Bhuiyan MZA, Ma H, Liu J, Wang L, Yang Z, Du L, Wang S, Yu PS (2020) Spatial temporal incidence dynamic graph neural networks for traffic flow forecasting. Inf Sci (n y) 521:277–290. https://doi.org/10.1016/j.ins.2020.01.043
Peng H, Du B, Liu M, Liu M, Ji S, Wang S, Zhang X, He L (2021) Dynamic graph convolutional network for long-term traffic flow prediction with reinforcement learning. Inf Sci (n y) 578:401–416. https://doi.org/10.1016/j.ins.2021.07.007
Article MathSciNet Google Scholar
Polson NG, Sokolov VO (2017) Deep learning for short-term traffic flow prediction. Transp Res Part C Emerg Technol 79:1–17. https://doi.org/10.1016/j.trc.2017.02.024
Primer A (2011) Dynamic traffic assignment. Transportation Network Modeling Committee 1–39. https://doi.org/10.1016/j.trd.2016.06.003
PyTorch [WWW document], 2016. URL https://pytorch.org/ . Accessed 10 Jun 2020
Rahman R, Hasan S (2018). Short-term traffic speed prediction for freeways during hurricane evacuation: a deep learning approach 1291–1296. https://doi.org/10.1109/ITSC.2018.8569443
Ran BIN, Boyce DE, Leblanc LJ (1993) A new class of instantaneous dynamic user-optimal traffic assignment models. Oper Res 41:192–202
Sanchez-Gonzalez A, Godwin J, Pfaff T, Ying R, Leskovec J, Battaglia PW (2020) Learning to simulate complex physics with graph networks. 37th International Conference on Machine Learning, ICML 2020 PartF16814, 8428–8437
Shafiei S, Gu Z, Saberi M (2018) Calibration and validation of a simulation-based dynamic traffic assignment model for a large-scale congested network. Simul Model Pract Theory 86:169–186. https://doi.org/10.1016/j.simpat.2018.04.006
Sheffi Y (1985) Urban transportation networks. Prentice-Hall Inc., Englewood Cliffs. https://doi.org/10.1016/0191-2607(86)90023-3
Book Google Scholar
Tang C, Sun J, Sun Y, Peng M, Gan N (2020) A General traffic flow prediction approach based on spatial-temporal graph attention. IEEE Access 8:153731–153741. https://doi.org/10.1109/ACCESS.2020.3018452
Teng SH (2016) Scalable algorithms for data and network analysis. Found Trends Theor Comput Sci 12:1–274. https://doi.org/10.1561/0400000051
Tizghadam A, Leon-garcia A (2007) A robust routing plan to optimize throughput in core networks 117–128
Transportation Networks for Research Core Team (2016) Transportation Networks for Research [WWW Document]. 2016. URL https://github.com/bstabler/TransportationNetworks (Accessed 8 Jul 2018)
Ukkusuri SV, Han L, Doan K (2012) Dynamic user equilibrium with a path based cell transmission model for general traffic networks. Transp Res Part b Methodol 46:1657–1684. https://doi.org/10.1016/j.trb.2012.07.010
Waller ST, Fajardo D, Duell M, Dixit V (2013) Linear programming formulation for strategic dynamic traffic assignment. Netw Spat Econ 13:427–443. https://doi.org/10.1007/s11067-013-9187-5
Wang HW, Peng ZR, Wang D, Meng Y, Wu T, Sun W, Lu QC (2020) Evaluation and prediction of transportation resilience under extreme weather events: a diffusion graph convolutional approach. Transp Res Part C Emerg Technol 115:102619. https://doi.org/10.1016/j.trc.2020.102619
Wardrop J (1952) Some theoretical aspects of road traffic research. Proc Inst Civil Eng Part II 1:325–378. https://doi.org/10.1680/ipeds.1952.11362
Watling D, Hazelton ML (2003) The dynamics and equilibria of day-to-day assignment models. Netw Spat Econ. https://doi.org/10.1023/A:1025398302560
Wu CH, Ho JM, Lee DT (2004) Travel-time prediction with support vector regression. IEEE Trans Intell Transp Syst 5:276–281. https://doi.org/10.1109/TITS.2004.837813
Yasdi R (1999) Prediction of road traffic using a neural network approach. Neural Comput Appl 8:135–142. https://doi.org/10.1007/s005210050015
Yu B, Song X, Guan F, Yang Z, Yao B (2016) k-nearest neighbor model for multiple-time-step prediction of short-term traffic condition. J Transp Eng 142:4016018. https://doi.org/10.1061/(ASCE)TE.1943-5436.0000816
Yu B, Yin H, Zhu Z (2018) Spatio-temporal graph convolutional networks: a deep learning framework for traffic forecasting, in: Proceedings of the Twenty-Seventh International Joint Conference on Artificial Intelligence. International Joint Conferences on Artificial Intelligence Organization, California pp. 3634–3640. https://doi.org/10.24963/ijcai.2018/505
Zhang Y, Haghani A (2015) A gradient boosting method to improve travel time prediction. Transp Res Part C Emerg Technol 58:308–324. https://doi.org/10.1016/j.trc.2015.02.019
Zhang S, Tong H, Xu J, Maciejewski R (2019) Graph convolutional networks: a comprehensive review. Comput Soc Netw. https://doi.org/10.1186/s40649-019-0069-y
Zhao L, Song Y, Zhang C, Liu Y, Wang P, Lin T, Deng M, Li H (2020) T-GCN: a temporal graph convolutional network for traffic prediction. IEEE Trans Intell Transp Syst 21:3848–3858. https://doi.org/10.1109/TITS.2019.2935152
Zhou J, Cui G, Zhang Z, Yang C, Liu Z, Wang L, Li C, Sun M (2020) Graph neural networks: a review of methods and applications. AI Open. https://doi.org/10.1016/j.aiopen.2021.01.001
Download references
This study was supported by the U.S. National Science Foundation through the grant CMMI #1917019. However, the authors are solely responsible for the facts and accuracy of the information presented in the paper.
Author information
Authors and affiliations.
Department of Civil, Environmental, and Construction Engineering, University of Central Florida, Orlando, FL, USA
Rezaur Rahman & Samiul Hasan
You can also search for this author in PubMed Google Scholar
Contributions
The authors confirm their contribution to the paper as follows: study conception and design: RR, SH; analysis and interpretation of results: RR, SH; draft manuscript preparation: RR, SH. All authors reviewed the results and approved the final version of the manuscript.
Corresponding author
Correspondence to Rezaur Rahman .
Ethics declarations
Conflict of interest.
The authors declare that they have no conflict of interest.
Additional information
Publisher's note.
Springer Nature remains neutral with regard to jurisdictional claims in published maps and institutional affiliations.
Appendix A: modeling traffic flows using spectral graph convolution
In spectral graph convolution, a spectral convolutional filter is used to learn traffic flow patterns inside a transportation network in response to travel demand variations. The spectral filter is derived from spectrum of the Laplacian matrix, which consists of eigenvalues of the Laplacian matrix. So to construct the spectrum, we must calculate the eigenvalues of` the Laplacian matrix. For a symmetric graph, we can compute the eigenvalues using Eigen decomposition of the Laplacian matrix. In this problem, we consider the transportation network as a symmetric-directed graph, same number of links getting out and getting inside a node, which means the in-degree and out-degree matrices of the graph are similar. Thus, the Laplacian matrix of this graph is diagonalizable as follows using Eigen decomposition
where \(\boldsymbol{\Lambda }\) is a diagonal matrix with eigenvalues, \({\lambda }_{0},{\lambda }_{1},{\lambda }_{2}, . . . ,{\lambda }_{N}\) and \({\varvec{U}}\) indicates the eigen vectors, \({u}_{0},{u}_{1},{u}_{2}, . . . ,{u}_{N}\) . Eigen values represent characteristics of transportation network in terms of strength of a particular node based on its position, distance between adjacent nodes, and dimension of the network. The spectral graph convolution filter can be defined as follows:
where \(\theta\) is the parameter for the convolution filter shared by all the nodes of the network and \(K\) is the size of the convolution filter. Now the spectral graph convolution over the graph signal ( \({\varvec{X}})\) is defined as follows:
According to spectral graph theory, the shortest path distance i.e., minimum number of links connecting nodes \(i\) and \(j\) is longer than \(K\) , such that \({L}^{K}\left(i, j\right) = 0\) (Hammond et al. 2011 ). Consequently, for a given pair of origin ( \(i\) ) and destination ( \(j)\) nodes, a spectral graph filter of size K has access to all the nodes on the shortest path of the graph. It means that the spectral graph convolution filter of size \(K\) captures flow propagation through each node on the shortest path. So the spectral graph convolution operation can model the interdependency between a link and its \(i\) th order adjacent nodes on the shortest paths, given that 0 ≤ i ≤ K .
The computational complexity of calculating \({{\varvec{L}}}_{{\varvec{w}}}^{{\varvec{k}}}\) is high due to K times multiplication of \({L}_{w}\) . A way to overcome this challenge is to approximate the spectral filter \({g}_{\theta }\) with Chebyshev polynomials up to ( \(K-1\) )th order (Hammond et al. 2011 ). Defferrard et al. (Defferrard et al. 2016 ) applied this approach to build a K -localized ChebNet, where the convolution is defined as
in which \(\overline{{\varvec{L}} }=2{{\varvec{L}}}_{{\varvec{s}}{\varvec{y}}{\varvec{m}}}/{{\varvec{\uplambda}}}_{{\varvec{m}}{\varvec{a}}{\varvec{x}}}-{\varvec{I}}\) . \(\overline{{\varvec{L}} }\) represents a scaling of graph Laplacian that maps the eigenvalues from [0, \({\uplambda }_{max}\) ] to [-1,1]. \({{\varvec{L}}}_{{\varvec{s}}{\varvec{y}}{\varvec{m}}}\) is defined as symmetric normalization of the Laplacian matrix \({{{\varvec{D}}}_{{\varvec{w}}}}^{-1/2}{{\varvec{L}}}_{{\varvec{w}}}{{\boldsymbol{ }{\varvec{D}}}_{{\varvec{w}}}}^{-1/2}.\) \({T}_{k}\) and θ denote the Chebyshev polynomials and Chebyshev coefficients. The Chebyshev polynomials are defined recursively by \({T}_{k}\left(\overline{{\varvec{L}} }\right)=2x{T}_{k-1}\left(\overline{{\varvec{L}} }\right)-{T}_{k-2}\left(\overline{{\varvec{L}} }\right)\) with \({T}_{0}\left(\overline{{\varvec{L}} }\right)=1\) and \({T}_{1}\left(\overline{{\varvec{L}} }\right)=\overline{{\varvec{L}} }\) . These are the basis of Chebyshev polynomials. Kipf and Welling (Kipf and Welling 2016 ) simplified this model by approximating the largest eigenvalue \({\lambda }_{max}\) of \(\overline{L }\) as 2. In this way, the convolution becomes
where Chebyshev coefficient, \(\theta ={\theta }_{0}=-{\theta }_{1}\) , All the details about the assumptions and their implications of Chebyshev polynomial can be found in (Hammond et al. 2011 ). Now the simplified graph convolution can be written as follows:
Since \({\varvec{I}}+{{{\varvec{D}}}_{{\varvec{w}}}}^{-1/2}{{\varvec{A}}}_{{\varvec{w}}}{{\boldsymbol{ }{\varvec{D}}}_{{\varvec{w}}}}^{-1/2}\) has eigenvalues in the range [0, 2], it may lead to exploding or vanishing gradients when used in a deep neural network model. To alleviate this problem, Kipf et al. (Kipf and Welling 2016 ) use a renormalization trick by replacing the term \({\varvec{I}}+{{{\varvec{D}}}_{{\varvec{w}}}}^{-1/2}{{\varvec{A}}}_{{\varvec{w}}}{{\boldsymbol{ }{\varvec{D}}}_{{\varvec{w}}}}^{-1/2}\) with \({{\overline{{\varvec{D}}} }_{{\varvec{w}}}\boldsymbol{ }\boldsymbol{ }}^{-1/2}{\overline{{\varvec{A}}} }_{{\varvec{w}}}{{\boldsymbol{ }\overline{{\varvec{D}}} }_{{\varvec{w}}}}^{-1/2}\) , with \({\overline{{\varvec{A}}} }_{{\varvec{w}}}={{\varvec{A}}}_{{\varvec{w}}}+{\varvec{I}}\) , similar to adding a self-loop. Now, we can simplify the spectral graph convolution as follows:
where \({\varvec{\Theta}}\in {{\varvec{R}}}^{{\varvec{N}}\times {\varvec{N}}}\) indicates the parameters of the convolution filter to be learnt during training process. From Eq. 21 , we can observe that spectral graph convolution is a special case of diffusion convolution (Li et al. 2018 ), but the only difference is that in spectral convolution, we symmetrically normalized the adjacency matrix.
Rights and permissions
Springer Nature or its licensor (e.g. a society or other partner) holds exclusive rights to this article under a publishing agreement with the author(s) or other rightsholder(s); author self-archiving of the accepted manuscript version of this article is solely governed by the terms of such publishing agreement and applicable law.
Reprints and permissions
About this article
Rahman, R., Hasan, S. Data-Driven Traffic Assignment: A Novel Approach for Learning Traffic Flow Patterns Using Graph Convolutional Neural Network. Data Sci. Transp. 5 , 11 (2023). https://doi.org/10.1007/s42421-023-00073-y
Download citation
Received : 21 May 2023
Revised : 09 June 2023
Accepted : 13 June 2023
Published : 24 July 2023
DOI : https://doi.org/10.1007/s42421-023-00073-y
Share this article
Anyone you share the following link with will be able to read this content:
Sorry, a shareable link is not currently available for this article.
Provided by the Springer Nature SharedIt content-sharing initiative
- Traffic assignment problem
- Data-driven method
- Deep learning
- Graph convolutional neural network
- Find a journal
- Publish with us
- Track your research
if (!inwiki && isMobileDevice){ document.write(' (adsbygoogle = window.adsbygoogle || []).push({}); ');} Moskovsky Settlement (Moscow) | district, third-level administrative division
- Similar places
- Nearby places
- Nearby cities
- Municipality of Sosenskoye 0.9 km
- Yasenevo District 7.5 km
- Yuzhnoye Butovo District 8.8 km
- Ochakovo-Matveyevskoye District 12 km
- Ramenki District 14 km
- Khoroshyovo-Mnyovniki District 20 km
- Kuntsevo District 22 km
- Voronovskoye Settlement 35 km
- Pavlovsky Posad 78 km
- Kimovsk 193 km
- Bridge via river Setun 0.4 km
- The pond in the village Kartmazovo 0.5 km
- Missiles for A-135 Moscow ABM system 0.9 km
- derevnya Kartmazovo, 28 0.9 km
- Pond 1 km
- Alpine ski center "Novoperedelkino" 1.2 km
- Ulyanov Forest-Park (eastern part) 1.4 km
- Novo-Peredelkino District 3.4 km
- Solntsevo District 4.2 km
- Odintsovsky district 31 km
- 24 km
- 58 km
- 147 km
- 164 km
- 323 km
- 354 km
- 429 km
- 430 km
- 474 km
- 620 km
Post comment
or continue as guest

IMAGES
VIDEO
COMMENTS
To connect emissions to congestion more directly and provide appropriate suggestions on integrated implementations, in this review, the taxonomy used for traffic assignment models, namely static and dynamic modeling (Cappiello, 2002), is adopted for categorizing the commonly applied emission models.
1.. IntroductionDuring the last decade, the within-day Dynamic Traffic Assignment (DTA) has been one of the most active fields in transport modeling (for an extensive bibliography on this topic, see Carey and Ge, 2003).Indeed, the classical framework of static traffic assignment is often recognized to be an improper approach for the analysis of highly congested road networks, where the ...
Abstract. The management of severe congestion in complex urban networks calls for dynamic traffic assignment (DTA) models that can replicate real traffic situations with long queues and spillbacks. DynaMIT-P, a mesoscopic traffic simulation system, was enhanced and calibrated to capture the traffic characteristics in a sub-area of Beijing ...
Using the dynamic traffic assignment models, modeling of traffic flow dynamics explicitly ensures a direct link between travel time and congestion. In case the link outflow is lower than the link inflow, the link density increases (congestion) and the speed decreases, what is known as the fundamental speed-density relationship; therefore, link ...
Traffic congestion is widely distributed around network. Generally to analyze the traffic congestion static traffic capacity is adopted. But the dynamic characteristic must be studied because the congestion is a dynamic process. Dynamic traffic assignment modeling fundamental combined with urban congestion analysis method is studied in this paper. Three methods are erected on congestion ...
Dynamic Traffic Assignment Models 113 Link Volumes 'r Behavioral Model of Physical Model of Route Choice Link Performance j~ Link Travel-Times Fig. 7.1. The classical traffic assignment paradigm congestion formation are considered as are the impacts of time-varying travel costs on behavior.
Dynamic traffic assignment (DTA) is one of the most important foundational theories in intelligent transportation systems (ITSs). ... accurate short-term traffic congestion forecasting is critical for designing transport infrastructure and for the real-time optimization of traffic. The main objective of this paper was to devise a method to ...
As traffic congestion builds up within a transportation network, drivers may elect to either cancel their trip altogether, alter their travel departure time, change their mode of travel, or change their route of travel. ... The dynamic traffic assignment approach is summarized in Fig. 5 and involves three input variables, namely: dynamic link ...
Dynamic network assignment models (also referred to as dynamic traffic assignment models or DTA) capture the changes in network performance by detailed time-of-day, and can be used to generate time varying measures of this performance. ... Traditional user equilibrium highway assignment models predict the effects of congestion and the routing ...
This paper handles the findings of three recent dynamic traffic assignment models, namely; 1) A dynamic traffic assignment model for highly congested urban networks; 2) System-optimal dynamic traffic assignment with and without queue spillback: Its path-based formulation and solution via approximate path marginal cost; and 3) A dynamic traffic ...
Using the General Link Transmission Model in a Dynamic Traffic Assignment to Simulate Congestion on Urban Networks. This article presents two new models of Dynamic User Equilibrium that are particularly suited for intelligent transportation systems (ITS) applications, where the evolution of vehicle flows and travel times must be simulated on large road networks, possibly in real-time.
Spillback congestion in dynamic traffic assignment: a macroscopic flow model with time-varying bottlenecks. Transportation Research B 41, pp. 1114-1138. Gentile G., Meschini L., 2011. Using dynamic assignment models for real-time traffic forecast on large urban networks. In Proceedings of the 2nd International Conference on Models and ...
for traffic dynamics under congestion pricing are unclear. This research performs a systematic comparison of static traffic assignment with the VISTA model, a simulation-based dynamic traffic assignment approach, and with an approximation to DTA using an add-in for TransCAD software.
A discrete time model is presented for dynamic traffice assignment with a single destination. Congestion is treated explicitly in the flow equations. The model is a nonlinear and nonconvex mathematical programming problem. A piecewise linear version of the model, with additional assumptions on the objective function, can be solved for a global ...
The dynamic nature of traffic networks is manifested inboth temporal and spatial changes in traffic demand, roadwaycapacities, and traffic control settings. Typically, theunderlying network traffic demand builds up over time at theonset of a peak period, varies stochastically during thepeak period, and decays at the conclusion of the peak period.
If there is congestion on a link, BGP will continue to send packets down that link Normal traffic flow FASTER FORWARD ©2012 Akamai Web Content Web Users $ Infrastructure ... Resulting in traffic of: 13 Tbps peak traffic 100+ petabytes / day 1,468+ billion hits / day 560+ million unique clients IPs / day The Akamai EdgePlatform: 120,000+
Moskovsky is a town in Moskovsky Settlement of Moscow. The town of Moskovsky was formerly located in Leninsky District of Moscow Oblast, Russia, and since July 2012 it is a part of the federal city of Moscow. Photo: Stolbovsky, CC BY-SA 4.0. Ukraine is facing shortages in its brave fight to survive.
The dynamic traffic assignment problem has received much attention in the last decade, due to the advancement in intelligent transportation systems (ITS), in particular the route guidance systems. ... Dynamic model of peak period traffic congestion with elastic arrival rates. Transportation Science, 20 (1986), pp. 164-181. CrossRef View in ...
Abstract. The management of severe congestion in complex urban networks calls for dynamic traffic assignment (DTA) models that can replicate real traffic situations with long queues and spillbacks. DynaMIT-P, a mesoscopic traffic simulation system, was enhanced and calibrated to capture the traffic characteristics in a sub-area of Beijing, China.
World's Worst Traffic In Moscow, TomTom Says July 22, 2014 • Staff • Bookmark + Photo of Moscow congestion from 2012 via Sergey Ivanov/Flickr.
The management of severe congestion in complex urban networks calls for dynamic traffic assignment (DTA) models that can replicate real traffic situations with long queues and spillbacks. DynaMIT-P, a mesoscopic traffic simulation system, was enhanced and calibrated to capture the traffic characteristics in the city of Beijing, China.
The congestion that's been plaguing Singapore's port is showing signs of easing, with container ship arrivals falling to the lowest in more than a year last month, official data show.
The traffic assignment problem (TAP) is one of the key components of transportation planning and operations. It is used to determine the traffic flow of each link of a transportation network for a given travel demand based on modeling the interactions among traveler route choices and the congestion that results from their travel over the network (Sheffi 1985).
Settlement (both municipal and administrative unit in Moscow) in Novomoskovsky Administrative Okrug of Moscow. It was established in 2005 as Moskovsky urban settlement in Leninsky municipal raion of Moscow Oblast and now it consists of the town of Moskovsky and eight other inhabited localities of the abolished Moskovsky rural district. On July 1, 2012 Moskovsky Settlement was transferred to ...