
- Curriculum and Instruction Master's
- TESOL Master's
- TESOL Certificate
- Educational Administration Master’s
- Educational Administration Certificate
- Autism Master's
- Autism Certificate
- Leadership in Special and Inclusive Education Certificate
- High Incidence Disabilities Master's
- Secondary Special Education and Transition Master's
- Secondary Special Education and Transition Certificate
- Virtual Learning Resources
- Frequently Asked Questions
- Video Gallery
- Financial Aid

Different Learning Styles—What Teachers Need To Know
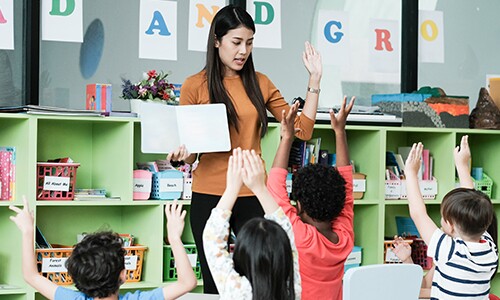
The concept of “learning styles” has been overwhelmingly embraced by educators in the U.S. and worldwide. Studies show that an estimated 89% of teachers believe in matching instruction to a student’s preferred learning style (Newton & Salvi, 2020). That’s a problem—because research tells us that this approach doesn’t work to improve learning.
What Do We Mean by “Learning Styles”?
It’s true that people have fairly stable strengths and weaknesses in their cognitive abilities, such as processing language or visual-spatial stimuli. People can also have preferences in the way they receive information—Joan may prefer to read an article while Jay may rather listen to a lecture.
The “learning styles” theory makes a big leap, suggesting that students will learn better if they are taught in a manner that conforms to their preferences. More than 70 different systems have been developed that use student questionnaires/self-reports to categorize their supposed learning preferences.
VARK Learning Styles
One of the most popular learning styles inventories used in schools is the VARK system (Cuevas, 2015). Students answer 25 multiple-choice questions that range from how they like their teachers to teach (discussions and guest speakers, textbooks and handouts, field trips and labs, or charts and diagrams) to how they would give directions to a neighbor’s house (draw a map, write out directions, say them aloud, or walk with the person) (VARK Learn Limited, 2021). Based on their responses, the system classifies them as Visual, Auditory, Read-write, and/or Kinesthetic learners and recommends specific learning strategies.
If only it were that simple. While this brief survey may provide some insights for teachers, we must be wary of overestimating the value of the results. By placing students in categories that reflect “preferred learning styles,” we run the risk of oversimplifying the complex nature of teaching and learning to the detriment of our students.
What Does the Science Say?
Study after study has shown that matching instructional mode to a student’s supposedly identified “learning style” does not produce better learning outcomes. In fact, a student’s “learning style” may not even predict the way they prefer to be taught or the way they actually choose to study on their own (Newton & Salvi, 2020).
Simply put, students’ learning preferences as identified via questionnaires do not predict the singular, best way to teach them. A single student may learn best with one approach in one subject and a different one in another. The best approach for them may even vary day-to-day. Most likely, students are best served when a variety of strategies are employed in a lesson.
As appealing as a framework like VARK is—relatively easy to conceptualize and quick to assess—everyone engages in different modes of learning in various ways. The brain processes information in very complex and nuanced ways that can’t be so simply generalized.
Fads are common in education. Having been embraced for several decades, though, “learning styles” has moved beyond fad to what experts refer to as “neuromyth,” one of many “commonly accepted, erroneous beliefs based on misunderstandings of neuroscience that contribute to pseudoscientific practice within education (Ruhaak & Cook, 2018). In fact, the idea that “students learn best when teaching styles are matched to their learning styles” earned a spot in 50 Great Myths of Popular Psychology (Lilienfeld, Lynn, & Beyerstein, 2009), alongside “Extrasensory perception is a well-established scientific phenomenon” and “Our handwriting reveals our personality traits.”
Unfortunately, the myth has become so prevalent that the majority of papers written about learning styles are based on the assumption that matching teaching style to learning style is desirable (Newton, 2015). It’s no surprise, then, that well-intentioned educators (and parents and caregivers) buy into the concept as well.
What Harm Does It Do?
When a student is pigeonholed as a particular “type” of learner, and their lessons are all prepared with that in mind, they could be missing out on other learning opportunities with a better chance of success.
Adapting instruction to individual students’ “learning styles” is no small task—and teachers who attempt to do so are clearly motivated to find the best way to help their students. They could put their time to better use, though.
Better Learning Style Approaches
Universal Design for Learning (UDL) is an evidence-driven framework for improving and optimizing learning for all students. When a learning opportunity provides for 1) multiple means of engagement, 2) multiple means of representation, and 3) multiple means of action and expression, different styles of learning are accounted for at the outset, reducing the need to personalize every activity. Nonprofit CAST.org, where KU Special Education Professor Jamie Basham is Senior Director for Learning & Innovation, offers free UDL Guidelines, with detailed information on how to optimize learning for all your students.
Operating within a UDL framework, teachers should use Evidence-based Practices (EBPs)—specific teaching techniques and interventions that have sufficient published, peer-reviewed studies that demonstrate their effectiveness in addressing specific issues with particular populations of students. (We discussed EBPs for autism spectrum disorder in a previous blog.) In addition, the Council for Exceptional Children recommends a core set of High Leverage Practices –basic, foundational practices that every special education teacher should know and perform fluently.
Evidence-based Learning Style Approaches at KU Special Education
Faculty in the University of Kansas Department of Special Education are world-renowned for their research in UDL and evidence-based special education practices. Students can be assured that our online master’s degrees and graduate certificates focus on research-based teaching and assessment methods—just one of the reasons we’ve been rated the #1 Best Online Master’s Degree in Special Education by U.S. News & World Report for two years in a row. 1
Explore our special education programs and consider how earning an online master’s from a Top 10 Best Education School (among public universities) can help you achieve your goals
CAST (2018). Universal Design for Learning Guidelines version 2.2. Retrieved March 4, 2021 from udlguidelines.cast.org.
Cuevas, J. (2015). Is learning styles-based instruction effective? A comprehensive analysis of recent research on learning styles. Theory & Research in Education. 13 (3), 308–333. doi.org/10.1177/1477878515606621
Lilienfeld, S., Lynn, J., Rucio, J., & Beyerstein, B. (2009) 50 great myths of popular psychology: Shattering widespread misconceptions about human behavior. Wiley-Blackwell. ISBN: 978-1405131117
Newton, P. M. (2015). The learning styles myth is thriving in higher education. Frontiers in Psychology, 6 , 1908. doi.org/10.3389/fpsyg.2015.01908
Newton, P. M. & Salvi, A. (2020). How common is belief in the learning styles neuromyth, and does it matter? A pragmatic systematic review. Frontiers in Education, 5 (602451), 1-14. doi.org/10.3389/feduc.2020.602451
Pashler, H., McDaniel, M., Rohrer, D., and Bjork, R. (2008). Learning styles: Concepts and evidence. Psychological Science in the Public Interest, 9 , 105–119. doi.org/10.1111/j.1539-6053.2009.01038.x
Ruhaak, A. E., & Cook, B. G. (2018). The prevalence of educational neuromythings among pre-service special education teachers. Mind, Brain, and Education. 12 (3) 155-161. doi.org/10.1111/mbe.12181
1 Retrieved on May 13, 2021, from usnews.com/best-graduate-schools/top-education-schools/university-of-kansas-06075 2 Retrieved on May 13, 2021, from usnews.com/best-graduate-schools/top-education-schools/edu-rankings
Return to Blog
IMPORTANT DATES
Stay connected.
Link to twitter Link to facebook Link to youtube Link to instagram
The University of Kansas has engaged Everspring , a leading provider of education and technology services, to support select aspects of program delivery.
The University of Kansas prohibits discrimination on the basis of race, color, ethnicity, religion, sex, national origin, age, ancestry, disability, status as a veteran, sexual orientation, marital status, parental status, retaliation, gender identity, gender expression and genetic information in the University's programs and activities. The following person has been designated to handle inquiries regarding the non-discrimination policies and is the University's Title IX Coordinator: the Executive Director of the Office of Institutional Opportunity and Access, [email protected] , 1246 W. Campus Road, Room 153A, Lawrence, KS, 66045, (785) 864-6414 , 711 TTY.
Learning Styles: Concepts and Evidence
- December 2008
- Psychological Science in the Public Interest 9(3):105-119
- 9(3):105-119
- This person is not on ResearchGate, or hasn't claimed this research yet.

- Washington University in St. Louis

- University of South Florida

- University of California, Los Angeles
Discover the world's research
- 25+ million members
- 160+ million publication pages
- 2.3+ billion citations

- Mishra Girish Lakhera

- Nethra Iyer
- Luke Landherr
- Megan Pontes
- James T. Todd

- Joshua Heyman
- Emilie M Bertsch

- Brittany Oletti
- L. L. Thurstone
- Jennifer C. Greene
- John R. Buri

- E. James Kehoe
- Penelope L. Peterson
- Richard E. Snow
- Psychol Monogr
- J.B. Rotter

- L.W. Porter
- D.T. Willingham
- EDUC LEADERSHIP
- Recruit researchers
- Join for free
- Login Email Tip: Most researchers use their institutional email address as their ResearchGate login Password Forgot password? Keep me logged in Log in or Continue with Google Welcome back! Please log in. Email · Hint Tip: Most researchers use their institutional email address as their ResearchGate login Password Forgot password? Keep me logged in Log in or Continue with Google No account? Sign up
- Our Mission & Impact
- Our Vision for a Well-Prepared Teacher
- Our Supporters
- News & Blog
- Programming
- For Teachers and Teacher-Educators
- For Educator-Preparation Leaders
- For Policymakers and Advocates
- Tools & Resources
- Work With Us
Phone: (512) 596-5417
2028 E. Ben White Blvd #240-5417 Austin, TX 78741
Posted on April 28, 2016
Learning styles: what does the research say?
Dylan Wiliam
Category: Cognitive Science
This post is the third in a periodic series exploring common misconceptions around how students learn. We first touched on these misconceptions in our September 2015 report, The Science of Learning , and will be exploring them in more depth over the next few months.
In today’s post, Dr. Dylan Wiliam explores what the research tells us about learning styles. Dylan Wiliam is Emeritus Professor of Educational Assessment at the Institute of Education, University College London. He served as dean and head of the School of Education (and later assistant principal) at King’s College London, senior research director at the Educational Testing Service in Princeton, New Jersey, and deputy director (provost) of the Institute of Education, University of London. Since 2010, he has devoted most of his time to research and teaching.
Since the beginning of Psychology as a field of study, psychologists have been categorizing people: as introverts and extroverts, in terms of their conscientiousness, their openness to experience, and so on. While many of these classification systems examine general personality, a number of classifications look specifically at the way people think—what is sometimes called their cognitive style. When solving problems, for example, some people like to focus on getting the evidence that is most likely to be relevant to the problem at hand, while others have a tendency to “think out of the box.”
More specifically still, many psychologists have moved from cognitive style—how people think—to the idea of learning style—how people learn (Adey, Fairbrother, Wiliam, Johnson, & Jones, 1999).
The basic idea is, of course, very attractive. We know that a particular piece of instruction might be effective for some students, and not for others, so it seems plausible that if the instruction was specifically designed to take into account a particular student’s preferred learning style, then it would be more effective for that student. This is what psychologists call the general learning-styles hypothesis—the idea that instruction students receive will be more (or less) effective if the instruction takes (or does not take) into account the student’s learning-style preferences.
Within education, a version of the learning-styles hypothesis, known by psychologists as the meshing hypothesis, has been of particular interest: the idea that students will learn more if they receive instruction that specifically matches their learning-style preferences. In other words, visual learners will learn better if they receive instruction that emphasizes visual ways of presenting information, and auditory learners will learn best by listening.
In their review of research on learning styles for the Association for Psychological Science, Pashler, McDaniel, Rohrer, and Bjork (2008) came to a stark conclusion: “If classification of students’ learning styles has practical utility, it remains to be demonstrated.” (p. 117)
Pashler et al pointed out that experiments designed to investigate the meshing hypothesis would have to satisfy three conditions:
- Based on some assessment of their presumed learning style, learners would be allocated to two or more groups (e.g., visual, auditory and kinesthetic learners).
- Learners within each of the learning-style groups would be randomly allocated to at least two different methods of instruction (e.g., visual and auditory based approaches).
- All students in the study would be given the same final test of achievement.
In such experiments, the meshing hypothesis would be supported if the results showed that the learning method that optimizes test performance of one learning-style group is different than the learning method that optimizes the test performance of a second learning-style group.
In their review, Pashler et al found only one study that gave even partial support to the meshing hypothesis, and two that clearly contradicted it.
Now, the fact that there is currently no evidence that knowing students’ learning styles helps us design more effective instruction does not mean that learning styles will never be useful in the future—absence of evidence is not the same as evidence of absence. Some psychologists are no doubt likely to continue to look for new ways to look at learning styles, even though there are at least 71 different learning-style classification systems already in existence (Coffield, Moseley, Hall, & Ecclestone, 2004). However, it could be that the whole idea of learning-styles research is misguided because its basic assumption—that the purpose of instructional design is to make learning easy—may just be incorrect.
Over the last 30 years, psychologists have found that performance on a learning task is a poor predictor of long-term retention. More precisely, when learners do well on a learning task, they are likely to forget things more quickly than if they do badly on the learning task; good instruction creates “desirable difficulties” (Bjork, 1994 p. 193) for the learner. In Daniel Willingham’s memorable phrase, “memory is the residue of thought” (Willingham, 2009). By trying to match our instruction to our students’ preferred learning style, we may, in fact, be reducing learning. If students do not have to work hard to make sense of what they are learning, then they are less likely to remember it in six weeks’ time.
Attempting to synthesize such a large and complex body of research is almost certainly a fool’s errand, but it seems to me that the important “takeaway” from the research on learning styles is that teachers need to know about learning styles if only to avoid the trap of teaching in the style they believe works best for them. As long as teachers are varying their teaching style, then it is likely that all students will get some experience of being in their comfort zone and some experience of being pushed beyond it. Ultimately, we have to remember that teaching is interesting because our students are so different, but only possible because they are so similar. Of course each of our students is a unique individual, but it is extraordinary how effective well-planned group instruction can be.
Adey, P. S., Fairbrother, R. W., Wiliam, D., Johnson, B., & Jones, C. (1999). A review of research related to learning styles and strategies . London, UK: King’s College London Centre for the Advancement of Thinking.
Bjork, R. A. (1994). Memory and metamemory considerations in the training of human beings. In J. Metcalfe & A. P. Shimamura (Eds.), Metacognition: Knowing about knowing (pp. 188-205). Cambridge, MA: MIT Press.
Coffield, F., Moseley, D., Hall, E., & Ecclestone, K. (2004). Learning styles and pedagogy in post-16 learning: A systematic and critical review . London, UK: Learning and Skills Development Agency.
Pashler, H., McDaniel, M., Rohrer, D., & Bjork, R. A. (2008). Learning styles: Concepts and evidence. Psychological Science in the Public Interest, 9 (3), 105-119.
Willingham, D. T. (2009). Why don’t students like school: A cognitive scientist answers questions about how the mind works and what it means for your classroom . San Francisco, CA: Jossey-Bass.
- Tutorial Review
- Open access
- Published: 24 January 2018
Teaching the science of learning
- Yana Weinstein ORCID: orcid.org/0000-0002-5144-968X 1 ,
- Christopher R. Madan 2 , 3 &
- Megan A. Sumeracki 4
Cognitive Research: Principles and Implications volume 3 , Article number: 2 ( 2018 ) Cite this article
272k Accesses
101 Citations
758 Altmetric
Metrics details
The science of learning has made a considerable contribution to our understanding of effective teaching and learning strategies. However, few instructors outside of the field are privy to this research. In this tutorial review, we focus on six specific cognitive strategies that have received robust support from decades of research: spaced practice, interleaving, retrieval practice, elaboration, concrete examples, and dual coding. We describe the basic research behind each strategy and relevant applied research, present examples of existing and suggested implementation, and make recommendations for further research that would broaden the reach of these strategies.
Significance
Education does not currently adhere to the medical model of evidence-based practice (Roediger, 2013 ). However, over the past few decades, our field has made significant advances in applying cognitive processes to education. From this work, specific recommendations can be made for students to maximize their learning efficiency (Dunlosky, Rawson, Marsh, Nathan, & Willingham, 2013 ; Roediger, Finn, & Weinstein, 2012 ). In particular, a review published 10 years ago identified a limited number of study techniques that have received solid evidence from multiple replications testing their effectiveness in and out of the classroom (Pashler et al., 2007 ). A recent textbook analysis (Pomerance, Greenberg, & Walsh, 2016 ) took the six key learning strategies from this report by Pashler and colleagues, and found that very few teacher-training textbooks cover any of these six principles – and none cover them all, suggesting that these strategies are not systematically making their way into the classroom. This is the case in spite of multiple recent academic (e.g., Dunlosky et al., 2013 ) and general audience (e.g., Dunlosky, 2013 ) publications about these strategies. In this tutorial review, we present the basic science behind each of these six key principles, along with more recent research on their effectiveness in live classrooms, and suggest ideas for pedagogical implementation. The target audience of this review is (a) educators who might be interested in integrating the strategies into their teaching practice, (b) science of learning researchers who are looking for open questions to help determine future research priorities, and (c) researchers in other subfields who are interested in the ways that principles from cognitive psychology have been applied to education.
While the typical teacher may not be exposed to this research during teacher training, a small cohort of teachers intensely interested in cognitive psychology has recently emerged. These teachers are mainly based in the UK, and, anecdotally (e.g., Dennis (2016), personal communication), appear to have taken an interest in the science of learning after reading Make it Stick (Brown, Roediger, & McDaniel, 2014 ; see Clark ( 2016 ) for an enthusiastic review of this book on a teacher’s blog, and “Learning Scientists” ( 2016c ) for a collection). In addition, a grassroots teacher movement has led to the creation of “researchED” – a series of conferences on evidence-based education (researchED, 2013 ). The teachers who form part of this network frequently discuss cognitive psychology techniques and their applications to education on social media (mainly Twitter; e.g., Fordham, 2016 ; Penfound, 2016 ) and on their blogs, such as Evidence Into Practice ( https://evidenceintopractice.wordpress.com/ ), My Learning Journey ( http://reflectionsofmyteaching.blogspot.com/ ), and The Effortful Educator ( https://theeffortfuleducator.com/ ). In general, the teachers who write about these issues pay careful attention to the relevant literature, often citing some of the work described in this review.
These informal writings, while allowing teachers to explore their approach to teaching practice (Luehmann, 2008 ), give us a unique window into the application of the science of learning to the classroom. By examining these blogs, we can not only observe how basic cognitive research is being applied in the classroom by teachers who are reading it, but also how it is being misapplied, and what questions teachers may be posing that have gone unaddressed in the scientific literature. Throughout this review, we illustrate each strategy with examples of how it can be implemented (see Table 1 and Figs. 1 , 2 , 3 , 4 , 5 , 6 and 7 ), as well as with relevant teacher blog posts that reflect on its application, and draw upon this work to pin-point fruitful avenues for further basic and applied research.
Spaced practice schedule for one week. This schedule is designed to represent a typical timetable of a high-school student. The schedule includes four one-hour study sessions, one longer study session on the weekend, and one rest day. Notice that each subject is studied one day after it is covered in school, to create spacing between classes and study sessions. Copyright note: this image was produced by the authors
a Blocked practice and interleaved practice with fraction problems. In the blocked version, students answer four multiplication problems consecutively. In the interleaved version, students answer a multiplication problem followed by a division problem and then an addition problem, before returning to multiplication. For an experiment with a similar setup, see Patel et al. ( 2016 ). Copyright note: this image was produced by the authors. b Illustration of interleaving and spacing. Each color represents a different homework topic. Interleaving involves alternating between topics, rather than blocking. Spacing involves distributing practice over time, rather than massing. Interleaving inherently involves spacing as other tasks naturally “fill” the spaces between interleaved sessions. Copyright note: this image was produced by the authors, adapted from Rohrer ( 2012 )
Concept map illustrating the process and resulting benefits of retrieval practice. Retrieval practice involves the process of withdrawing learned information from long-term memory into working memory, which requires effort. This produces direct benefits via the consolidation of learned information, making it easier to remember later and causing improvements in memory, transfer, and inferences. Retrieval practice also produces indirect benefits of feedback to students and teachers, which in turn can lead to more effective study and teaching practices, with a focus on information that was not accurately retrieved. Copyright note: this figure originally appeared in a blog post by the first and third authors ( http://www.learningscientists.org/blog/2016/4/1-1 )
Illustration of “how” and “why” questions (i.e., elaborative interrogation questions) students might ask while studying the physics of flight. To help figure out how physics explains flight, students might ask themselves the following questions: “How does a plane take off?”; “Why does a plane need an engine?”; “How does the upward force (lift) work?”; “Why do the wings have a curved upper surface and a flat lower surface?”; and “Why is there a downwash behind the wings?”. Copyright note: the image of the plane was downloaded from Pixabay.com and is free to use, modify, and share
Three examples of physics problems that would be categorized differently by novices and experts. The problems in ( a ) and ( c ) look similar on the surface, so novices would group them together into one category. Experts, however, will recognize that the problems in ( b ) and ( c ) both relate to the principle of energy conservation, and so will group those two problems into one category instead. Copyright note: the figure was produced by the authors, based on figures in Chi et al. ( 1981 )
Example of how to enhance learning through use of a visual example. Students might view this visual representation of neural communications with the words provided, or they could draw a similar visual representation themselves. Copyright note: this figure was produced by the authors
Example of word properties associated with visual, verbal, and motor coding for the word “SPOON”. A word can evoke multiple types of representation (“codes” in dual coding theory). Viewing a word will automatically evoke verbal representations related to its component letters and phonemes. Words representing objects (i.e., concrete nouns) will also evoke visual representations, including information about similar objects, component parts of the object, and information about where the object is typically found. In some cases, additional codes can also be evoked, such as motor-related properties of the represented object, where contextual information related to the object’s functional intention and manipulation action may also be processed automatically when reading the word. Copyright note: this figure was produced by the authors and is based on Aylwin ( 1990 ; Fig. 2 ) and Madan and Singhal ( 2012a , Fig. 3 )
Spaced practice
The benefits of spaced (or distributed) practice to learning are arguably one of the strongest contributions that cognitive psychology has made to education (Kang, 2016 ). The effect is simple: the same amount of repeated studying of the same information spaced out over time will lead to greater retention of that information in the long run, compared with repeated studying of the same information for the same amount of time in one study session. The benefits of distributed practice were first empirically demonstrated in the 19 th century. As part of his extensive investigation into his own memory, Ebbinghaus ( 1885/1913 ) found that when he spaced out repetitions across 3 days, he could almost halve the number of repetitions necessary to relearn a series of 12 syllables in one day (Chapter 8). He thus concluded that “a suitable distribution of [repetitions] over a space of time is decidedly more advantageous than the massing of them at a single time” (Section 34). For those who want to read more about Ebbinghaus’s contribution to memory research, Roediger ( 1985 ) provides an excellent summary.
Since then, hundreds of studies have examined spacing effects both in the laboratory and in the classroom (Kang, 2016 ). Spaced practice appears to be particularly useful at large retention intervals: in the meta-analysis by Cepeda, Pashler, Vul, Wixted, and Rohrer ( 2006 ), all studies with a retention interval longer than a month showed a clear benefit of distributed practice. The “new theory of disuse” (Bjork & Bjork, 1992 ) provides a helpful mechanistic explanation for the benefits of spacing to learning. This theory posits that memories have both retrieval strength and storage strength. Whereas retrieval strength is thought to measure the ease with which a memory can be recalled at a given moment, storage strength (which cannot be measured directly) represents the extent to which a memory is truly embedded in the mind. When studying is taking place, both retrieval strength and storage strength receive a boost. However, the extent to which storage strength is boosted depends upon retrieval strength, and the relationship is negative: the greater the current retrieval strength, the smaller the gains in storage strength. Thus, the information learned through “cramming” will be rapidly forgotten due to high retrieval strength and low storage strength (Bjork & Bjork, 2011 ), whereas spacing out learning increases storage strength by allowing retrieval strength to wane before restudy.
Teachers can introduce spacing to their students in two broad ways. One involves creating opportunities to revisit information throughout the semester, or even in future semesters. This does involve some up-front planning, and can be difficult to achieve, given time constraints and the need to cover a set curriculum. However, spacing can be achieved with no great costs if teachers set aside a few minutes per class to review information from previous lessons. The second method involves putting the onus to space on the students themselves. Of course, this would work best with older students – high school and above. Because spacing requires advance planning, it is crucial that the teacher helps students plan their studying. For example, teachers could suggest that students schedule study sessions on days that alternate with the days on which a particular class meets (e.g., schedule review sessions for Tuesday and Thursday when the class meets Monday and Wednesday; see Fig. 1 for a more complete weekly spaced practice schedule). It important to note that the spacing effect refers to information that is repeated multiple times, rather than the idea of studying different material in one long session versus spaced out in small study sessions over time. However, for teachers and particularly for students planning a study schedule, the subtle difference between the two situations (spacing out restudy opportunities, versus spacing out studying of different information over time) may be lost. Future research should address the effects of spacing out studying of different information over time, whether the same considerations apply in this situation as compared to spacing out restudy opportunities, and how important it is for teachers and students to understand the difference between these two types of spaced practice.
It is important to note that students may feel less confident when they space their learning (Bjork, 1999 ) than when they cram. This is because spaced learning is harder – but it is this “desirable difficulty” that helps learning in the long term (Bjork, 1994 ). Students tend to cram for exams rather than space out their learning. One explanation for this is that cramming does “work”, if the goal is only to pass an exam. In order to change students’ minds about how they schedule their studying, it might be important to emphasize the value of retaining information beyond a final exam in one course.
Ideas for how to apply spaced practice in teaching have appeared in numerous teacher blogs (e.g., Fawcett, 2013 ; Kraft, 2015 ; Picciotto, 2009 ). In England in particular, as of 2013, high-school students need to be able to remember content from up to 3 years back on cumulative exams (General Certificate of Secondary Education (GCSE) and A-level exams; see CIFE, 2012 ). A-levels in particular determine what subject students study in university and which programs they are accepted into, and thus shape the path of their academic career. A common approach for dealing with these exams has been to include a “revision” (i.e., studying or cramming) period of a few weeks leading up to the high-stakes cumulative exams. Now, teachers who follow cognitive psychology are advocating a shift of priorities to spacing learning over time across the 3 years, rather than teaching a topic once and then intensely reviewing it weeks before the exam (Cox, 2016a ; Wood, 2017 ). For example, some teachers have suggested using homework assignments as an opportunity for spaced practice by giving students homework on previous topics (Rose, 2014 ). However, questions remain, such as whether spaced practice can ever be effective enough to completely alleviate the need or utility of a cramming period (Cox, 2016b ), and how one can possibly figure out the optimal lag for spacing (Benney, 2016 ; Firth, 2016 ).
There has been considerable research on the question of optimal lag, and much of it is quite complex; two sessions neither too close together (i.e., cramming) nor too far apart are ideal for retention. In a large-scale study, Cepeda, Vul, Rohrer, Wixted, and Pashler ( 2008 ) examined the effects of the gap between study sessions and the interval between study and test across long periods, and found that the optimal gap between study sessions was contingent on the retention interval. Thus, it is not clear how teachers can apply the complex findings on lag to their own classrooms.
A useful avenue of research would be to simplify the research paradigms that are used to study optimal lag, with the goal of creating a flexible, spaced-practice framework that teachers could apply and tailor to their own teaching needs. For example, an Excel macro spreadsheet was recently produced to help teachers plan for lagged lessons (Weinstein-Jones & Weinstein, 2017 ; see Weinstein & Weinstein-Jones ( 2017 ) for a description of the algorithm used in the spreadsheet), and has been used by teachers to plan their lessons (Penfound, 2017 ). However, one teacher who found this tool helpful also wondered whether the more sophisticated plan was any better than his own method of manually selecting poorly understood material from previous classes for later review (Lovell, 2017 ). This direction is being actively explored within personalized online learning environments (Kornell & Finn, 2016 ; Lindsey, Shroyer, Pashler, & Mozer, 2014 ), but teachers in physical classrooms might need less technologically-driven solutions to teach cohorts of students.
It seems teachers would greatly appreciate a set of guidelines for how to implement spacing in the curriculum in the most effective, but also the most efficient manner. While the cognitive field has made great advances in terms of understanding the mechanisms behind spacing, what teachers need more of are concrete evidence-based tools and guidelines for direct implementation in the classroom. These could include more sophisticated and experimentally tested versions of the software described above (Weinstein-Jones & Weinstein, 2017 ), or adaptable templates of spaced curricula. Moreover, researchers need to evaluate the effectiveness of these tools in a real classroom environment, over a semester or academic year, in order to give pedagogically relevant evidence-based recommendations to teachers.
Interleaving
Another scheduling technique that has been shown to increase learning is interleaving. Interleaving occurs when different ideas or problem types are tackled in a sequence, as opposed to the more common method of attempting multiple versions of the same problem in a given study session (known as blocking). Interleaving as a principle can be applied in many different ways. One such way involves interleaving different types of problems during learning, which is particularly applicable to subjects such as math and physics (see Fig. 2 a for an example with fractions, based on a study by Patel, Liu, & Koedinger, 2016 ). For example, in a study with college students, Rohrer and Taylor ( 2007 ) found that shuffling math problems that involved calculating the volume of different shapes resulted in better test performance 1 week later than when students answered multiple problems about the same type of shape in a row. This pattern of results has also been replicated with younger students, for example 7 th grade students learning to solve graph and slope problems (Rohrer, Dedrick, & Stershic, 2015 ). The proposed explanation for the benefit of interleaving is that switching between different problem types allows students to acquire the ability to choose the right method for solving different types of problems rather than learning only the method itself, and not when to apply it.
Do the benefits of interleaving extend beyond problem solving? The answer appears to be yes. Interleaving can be helpful in other situations that require discrimination, such as inductive learning. Kornell and Bjork ( 2008 ) examined the effects of interleaving in a task that might be pertinent to a student of the history of art: the ability to match paintings to their respective painters. Students who studied different painters’ paintings interleaved at study were more successful on a later identification test than were participants who studied the paintings blocked by painter. Birnbaum, Kornell, Bjork, and Bjork ( 2013 ) proposed the discriminative-contrast hypothesis to explain that interleaving enhances learning by allowing the comparison between exemplars of different categories. They found support for this hypothesis in a set of experiments with bird categorization: participants benefited from interleaving and also from spacing, but not when the spacing interrupted side-by-side comparisons of birds from different categories.
Another type of interleaving involves the interleaving of study and test opportunities. This type of interleaving has been applied, once again, to problem solving, whereby students alternate between attempting a problem and viewing a worked example (Trafton & Reiser, 1993 ); this pattern appears to be superior to answering a string of problems in a row, at least with respect to the amount of time it takes to achieve mastery of a procedure (Corbett, Reed, Hoffmann, MacLaren, & Wagner, 2010 ). The benefits of interleaving study and test opportunities – rather than blocking study followed by attempting to answer problems or questions – might arise due to a process known as “test-potentiated learning”. That is, a study opportunity that immediately follows a retrieval attempt may be more fruitful than when that same studying was not preceded by retrieval (Arnold & McDermott, 2013 ).
For problem-based subjects, the interleaving technique is straightforward: simply mix questions on homework and quizzes with previous materials (which takes care of spacing as well); for languages, mix vocabulary themes rather than blocking by theme (Thomson & Mehring, 2016 ). But interleaving as an educational strategy ought to be presented to teachers with some caveats. Research has focused on interleaving material that is somewhat related (e.g., solving different mathematical equations, Rohrer et al., 2015 ), whereas students sometimes ask whether they should interleave material from different subjects – a practice that has not received empirical support (Hausman & Kornell, 2014 ). When advising students how to study independently, teachers should thus proceed with caution. Since it is easy for younger students to confuse this type of unhelpful interleaving with the more helpful interleaving of related information, it may be best for teachers of younger grades to create opportunities for interleaving in homework and quiz assignments rather than putting the onus on the students themselves to make use of the technique. Technology can be very helpful here, with apps such as Quizlet, Memrise, Anki, Synap, Quiz Champ, and many others (see also “Learning Scientists”, 2017 ) that not only allow instructor-created quizzes to be taken by students, but also provide built-in interleaving algorithms so that the burden does not fall on the teacher or the student to carefully plan which items are interleaved when.
An important point to consider is that in educational practice, the distinction between spacing and interleaving can be difficult to delineate. The gap between the scientific and classroom definitions of interleaving is demonstrated by teachers’ own writings about this technique. When they write about interleaving, teachers often extend the term to connote a curriculum that involves returning to topics multiple times throughout the year (e.g., Kirby, 2014 ; see “Learning Scientists” ( 2016a ) for a collection of similar blog posts by several other teachers). The “interleaving” of topics throughout the curriculum produces an effect that is more akin to what cognitive psychologists call “spacing” (see Fig. 2 b for a visual representation of the difference between interleaving and spacing). However, cognitive psychologists have not examined the effects of structuring the curriculum in this way, and open questions remain: does repeatedly circling back to previous topics throughout the semester interrupt the learning of new information? What are some effective techniques for interleaving old and new information within one class? And how does one determine the balance between old and new information?
Retrieval practice
While tests are most often used in educational settings for assessment, a lesser-known benefit of tests is that they actually improve memory of the tested information. If we think of our memories as libraries of information, then it may seem surprising that retrieval (which happens when we take a test) improves memory; however, we know from a century of research that retrieving knowledge actually strengthens it (see Karpicke, Lehman, & Aue, 2014 ). Testing was shown to strengthen memory as early as 100 years ago (Gates, 1917 ), and there has been a surge of research in the last decade on the mnemonic benefits of testing, or retrieval practice . Most of the research on the effectiveness of retrieval practice has been done with college students (see Roediger & Karpicke, 2006 ; Roediger, Putnam, & Smith, 2011 ), but retrieval-based learning has been shown to be effective at producing learning for a wide range of ages, including preschoolers (Fritz, Morris, Nolan, & Singleton, 2007 ), elementary-aged children (e.g., Karpicke, Blunt, & Smith, 2016 ; Karpicke, Blunt, Smith, & Karpicke, 2014 ; Lipko-Speed, Dunlosky, & Rawson, 2014 ; Marsh, Fazio, & Goswick, 2012 ; Ritchie, Della Sala, & McIntosh, 2013 ), middle-school students (e.g., McDaniel, Thomas, Agarwal, McDermott, & Roediger, 2013 ; McDermott, Agarwal, D’Antonio, Roediger, & McDaniel, 2014 ), and high-school students (e.g., McDermott et al., 2014 ). In addition, the effectiveness of retrieval-based learning has been extended beyond simple testing to other activities in which retrieval practice can be integrated, such as concept mapping (Blunt & Karpicke, 2014 ; Karpicke, Blunt, et al., 2014 ; Ritchie et al., 2013 ).
A debate is currently ongoing as to the effectiveness of retrieval practice for more complex materials (Karpicke & Aue, 2015 ; Roelle & Berthold, 2017 ; Van Gog & Sweller, 2015 ). Practicing retrieval has been shown to improve the application of knowledge to new situations (e.g., Butler, 2010 ; Dirkx, Kester, & Kirschner, 2014 ); McDaniel et al., 2013 ; Smith, Blunt, Whiffen, & Karpicke, 2016 ); but see Tran, Rohrer, and Pashler ( 2015 ) and Wooldridge, Bugg, McDaniel, and Liu ( 2014 ), for retrieval practice studies that showed limited or no increased transfer compared to restudy. Retrieval practice effects on higher-order learning may be more sensitive than fact learning to encoding factors, such as the way material is presented during study (Eglington & Kang, 2016 ). In addition, retrieval practice may be more beneficial for higher-order learning if it includes more scaffolding (Fiechter & Benjamin, 2017 ; but see Smith, Blunt, et al., 2016 ) and targeted practice with application questions (Son & Rivas, 2016 ).
How does retrieval practice help memory? Figure 3 illustrates both the direct and indirect benefits of retrieval practice identified by the literature. The act of retrieval itself is thought to strengthen memory (Karpicke, Blunt, et al., 2014 ; Roediger & Karpicke, 2006 ; Smith, Roediger, & Karpicke, 2013 ). For example, Smith et al. ( 2013 ) showed that if students brought information to mind without actually producing it (covert retrieval), they remembered the information just as well as if they overtly produced the retrieved information (overt retrieval). Importantly, both overt and covert retrieval practice improved memory over control groups without retrieval practice, even when feedback was not provided. The fact that bringing information to mind in the absence of feedback or restudy opportunities improves memory leads researchers to conclude that it is the act of retrieval – thinking back to bring information to mind – that improves memory of that information.
The benefit of retrieval practice depends to a certain extent on successful retrieval (see Karpicke, Lehman, et al., 2014 ). For example, in Experiment 4 of Smith et al. ( 2013 ), students successfully retrieved 72% of the information during retrieval practice. Of course, retrieving 72% of the information was compared to a restudy control group, during which students were re-exposed to 100% of the information, creating a bias in favor of the restudy condition. Yet retrieval led to superior memory later compared to the restudy control. However, if retrieval success is extremely low, then it is unlikely to improve memory (e.g., Karpicke, Blunt, et al., 2014 ), particularly in the absence of feedback. On the other hand, if retrieval-based learning situations are constructed in such a way that ensures high levels of success, the act of bringing the information to mind may be undermined, thus making it less beneficial. For example, if a student reads a sentence and then immediately covers the sentence and recites it out loud, they are likely not retrieving the information but rather just keeping the information in their working memory long enough to recite it again (see Smith, Blunt, et al., 2016 for a discussion of this point). Thus, it is important to balance success of retrieval with overall difficulty in retrieving the information (Smith & Karpicke, 2014 ; Weinstein, Nunes, & Karpicke, 2016 ). If initial retrieval success is low, then feedback can help improve the overall benefit of practicing retrieval (Kang, McDermott, & Roediger, 2007 ; Smith & Karpicke, 2014 ). Kornell, Klein, and Rawson ( 2015 ), however, found that it was the retrieval attempt and not the correct production of information that produced the retrieval practice benefit – as long as the correct answer was provided after an unsuccessful attempt, the benefit was the same as for a successful retrieval attempt in this set of studies. From a practical perspective, it would be helpful for teachers to know when retrieval attempts in the absence of success are helpful, and when they are not. There may also be additional reasons beyond retrieval benefits that would push teachers towards retrieval practice activities that produce some success amongst students; for example, teachers may hesitate to give students retrieval practice exercises that are too difficult, as this may negatively affect self-efficacy and confidence.
In addition to the fact that bringing information to mind directly improves memory for that information, engaging in retrieval practice can produce indirect benefits as well (see Roediger et al., 2011 ). For example, research by Weinstein, Gilmore, Szpunar, and McDermott ( 2014 ) demonstrated that when students expected to be tested, the increased test expectancy led to better-quality encoding of new information. Frequent testing can also serve to decrease mind-wandering – that is, thoughts that are unrelated to the material that students are supposed to be studying (Szpunar, Khan, & Schacter, 2013 ).
Practicing retrieval is a powerful way to improve meaningful learning of information, and it is relatively easy to implement in the classroom. For example, requiring students to practice retrieval can be as simple as asking students to put their class materials away and try to write out everything they know about a topic. Retrieval-based learning strategies are also flexible. Instructors can give students practice tests (e.g., short-answer or multiple-choice, see Smith & Karpicke, 2014 ), provide open-ended prompts for the students to recall information (e.g., Smith, Blunt, et al., 2016 ) or ask their students to create concept maps from memory (e.g., Blunt & Karpicke, 2014 ). In one study, Weinstein et al. ( 2016 ) looked at the effectiveness of inserting simple short-answer questions into online learning modules to see whether they improved student performance. Weinstein and colleagues also manipulated the placement of the questions. For some students, the questions were interspersed throughout the module, and for other students the questions were all presented at the end of the module. Initial success on the short-answer questions was higher when the questions were interspersed throughout the module. However, on a later test of learning from that module, the original placement of the questions in the module did not matter for performance. As with spaced practice, where the optimal gap between study sessions is contingent on the retention interval, the optimum difficulty and level of success during retrieval practice may also depend on the retention interval. Both groups of students who answered questions performed better on the delayed test compared to a control group without question opportunities during the module. Thus, the important thing is for instructors to provide opportunities for retrieval practice during learning. Based on previous research, any activity that promotes the successful retrieval of information should improve learning.
Retrieval practice has received a lot of attention in teacher blogs (see “Learning Scientists” ( 2016b ) for a collection). A common theme seems to be an emphasis on low-stakes (Young, 2016 ) and even no-stakes (Cox, 2015 ) testing, the goal of which is to increase learning rather than assess performance. In fact, one well-known charter school in the UK has an official homework policy grounded in retrieval practice: students are to test themselves on subject knowledge for 30 minutes every day in lieu of standard homework (Michaela Community School, 2014 ). The utility of homework, particularly for younger children, is often a hotly debated topic outside of academia (e.g., Shumaker, 2016 ; but see Jones ( 2016 ) for an opposing viewpoint and Cooper ( 1989 ) for the original research the blog posts were based on). Whereas some research shows clear links between homework and academic achievement (Valle et al., 2016 ), other researchers have questioned the effectiveness of homework (Dettmers, Trautwein, & Lüdtke, 2009 ). Perhaps amending homework to involve retrieval practice might make it more effective; this remains an open empirical question.
One final consideration is that of test anxiety. While retrieval practice can be very powerful at improving memory, some research shows that pressure during retrieval can undermine some of the learning benefit. For example, Hinze and Rapp ( 2014 ) manipulated pressure during quizzing to create high-pressure and low-pressure conditions. On the quizzes themselves, students performed equally well. However, those in the high-pressure condition did not perform as well on a criterion test later compared to the low-pressure group. Thus, test anxiety may reduce the learning benefit of retrieval practice. Eliminating all high-pressure tests is probably not possible, but instructors can provide a number of low-stakes retrieval opportunities for students to help increase learning. The use of low-stakes testing can serve to decrease test anxiety (Khanna, 2015 ), and has recently been shown to negate the detrimental impact of stress on learning (Smith, Floerke, & Thomas, 2016 ). This is a particularly important line of inquiry to pursue for future research, because many teachers who are not familiar with the effectiveness of retrieval practice may be put off by the implied pressure of “testing”, which evokes the much maligned high-stakes standardized tests (e.g., McHugh, 2013 ).
Elaboration
Elaboration involves connecting new information to pre-existing knowledge. Anderson ( 1983 , p.285) made the following claim about elaboration: “One of the most potent manipulations that can be performed in terms of increasing a subject’s memory for material is to have the subject elaborate on the to-be-remembered material.” Postman ( 1976 , p. 28) defined elaboration most parsimoniously as “additions to nominal input”, and Hirshman ( 2001 , p. 4369) provided an elaboration on this definition (pun intended!), defining elaboration as “A conscious, intentional process that associates to-be-remembered information with other information in memory.” However, in practice, elaboration could mean many different things. The common thread in all the definitions is that elaboration involves adding features to an existing memory.
One possible instantiation of elaboration is thinking about information on a deeper level. The levels (or “depth”) of processing framework, proposed by Craik and Lockhart ( 1972 ), predicts that information will be remembered better if it is processed more deeply in terms of meaning, rather than shallowly in terms of form. The leves of processing framework has, however, received a number of criticisms (Craik, 2002 ). One major problem with this framework is that it is difficult to measure “depth”. And if we are not able to actually measure depth, then the argument can become circular: is it that something was remembered better because it was studied more deeply, or do we conclude that it must have been studied more deeply because it is remembered better? (See Lockhart & Craik, 1990 , for further discussion of this issue).
Another mechanism by which elaboration can confer a benefit to learning is via improvement in organization (Bellezza, Cheesman, & Reddy, 1977 ; Mandler, 1979 ). By this view, elaboration involves making information more integrated and organized with existing knowledge structures. By connecting and integrating the to-be-learned information with other concepts in memory, students can increase the extent to which the ideas are organized in their minds, and this increased organization presumably facilitates the reconstruction of the past at the time of retrieval.
Elaboration is such a broad term and can include so many different techniques that it is hard to claim that elaboration will always help learning. There is, however, a specific technique under the umbrella of elaboration for which there is relatively strong evidence in terms of effectiveness (Dunlosky et al., 2013 ; Pashler et al., 2007 ). This technique is called elaborative interrogation, and involves students questioning the materials that they are studying (Pressley, McDaniel, Turnure, Wood, & Ahmad, 1987 ). More specifically, students using this technique would ask “how” and “why” questions about the concepts they are studying (see Fig. 4 for an example on the physics of flight). Then, crucially, students would try to answer these questions – either from their materials or, eventually, from memory (McDaniel & Donnelly, 1996 ). The process of figuring out the answer to the questions – with some amount of uncertainty (Overoye & Storm, 2015 ) – can help learning. When using this technique, however, it is important that students check their answers with their materials or with the teacher; when the content generated through elaborative interrogation is poor, it can actually hurt learning (Clinton, Alibali, & Nathan, 2016 ).
Students can also be encouraged to self-explain concepts to themselves while learning (Chi, De Leeuw, Chiu, & LaVancher, 1994 ). This might involve students simply saying out loud what steps they need to perform to solve an equation. Aleven and Koedinger ( 2002 ) conducted two classroom studies in which students were either prompted by a “cognitive tutor” to provide self-explanations during a problem-solving task or not, and found that the self-explanations led to improved performance. According to the authors, this approach could scale well to real classrooms. If possible and relevant, students could even perform actions alongside their self-explanations (Cohen, 1981 ; see also the enactment effect, Hainselin, Picard, Manolli, Vankerkore-Candas, & Bourdin, 2017 ). Instructors can scaffold students in these types of activities by providing self-explanation prompts throughout to-be-learned material (O’Neil et al., 2014 ). Ultimately, the greatest potential benefit of accurate self-explanation or elaboration is that the student will be able to transfer their knowledge to a new situation (Rittle-Johnson, 2006 ).
The technical term “elaborative interrogation” has not made it into the vernacular of educational bloggers (a search on https://educationechochamberuncut.wordpress.com , which consolidates over 3,000 UK-based teacher blogs, yielded zero results for that term). However, a few teachers have blogged about elaboration more generally (e.g., Hobbiss, 2016 ) and deep questioning specifically (e.g., Class Teaching, 2013 ), just without using the specific terminology. This strategy in particular may benefit from a more open dialog between researchers and teachers to facilitate the use of elaborative interrogation in the classroom and to address possible barriers to implementation. In terms of advancing the scientific understanding of elaborative interrogation in a classroom setting, it would be informative to conduct a larger-scale intervention to see whether having students elaborate during reading actually helps their understanding. It would also be useful to know whether the students really need to generate their own elaborative interrogation (“how” and “why”) questions, versus answering questions provided by others. How long should students persist to find the answers? When is the right time to have students engage in this task, given the levels of expertise required to do it well (Clinton et al., 2016 )? Without knowing the answers to these questions, it may be too early for us to instruct teachers to use this technique in their classes. Finally, elaborative interrogation takes a long time. Is this time efficiently spent? Or, would it be better to have the students try to answer a few questions, pool their information as a class, and then move to practicing retrieval of the information?
Concrete examples
Providing supporting information can improve the learning of key ideas and concepts. Specifically, using concrete examples to supplement content that is more conceptual in nature can make the ideas easier to understand and remember. Concrete examples can provide several advantages to the learning process: (a) they can concisely convey information, (b) they can provide students with more concrete information that is easier to remember, and (c) they can take advantage of the superior memorability of pictures relative to words (see “Dual Coding”).
Words that are more concrete are both recognized and recalled better than abstract words (Gorman, 1961 ; e.g., “button” and “bound,” respectively). Furthermore, it has been demonstrated that information that is more concrete and imageable enhances the learning of associations, even with abstract content (Caplan & Madan, 2016 ; Madan, Glaholt, & Caplan, 2010 ; Paivio, 1971 ). Following from this, providing concrete examples during instruction should improve retention of related abstract concepts, rather than the concrete examples alone being remembered better. Concrete examples can be useful both during instruction and during practice problems. Having students actively explain how two examples are similar and encouraging them to extract the underlying structure on their own can also help with transfer. In a laboratory study, Berry ( 1983 ) demonstrated that students performed well when given concrete practice problems, regardless of the use of verbalization (akin to elaborative interrogation), but that verbalization helped students transfer understanding from concrete to abstract problems. One particularly important area of future research is determining how students can best make the link between concrete examples and abstract ideas.
Since abstract concepts are harder to grasp than concrete information (Paivio, Walsh, & Bons, 1994 ), it follows that teachers ought to illustrate abstract ideas with concrete examples. However, care must be taken when selecting the examples. LeFevre and Dixon ( 1986 ) provided students with both concrete examples and abstract instructions and found that when these were inconsistent, students followed the concrete examples rather than the abstract instructions, potentially constraining the application of the abstract concept being taught. Lew, Fukawa-Connelly, Mejí-Ramos, and Weber ( 2016 ) used an interview approach to examine why students may have difficulty understanding a lecture. Responses indicated that some issues were related to understanding the overarching topic rather than the component parts, and to the use of informal colloquialisms that did not clearly follow from the material being taught. Both of these issues could have potentially been addressed through the inclusion of a greater number of relevant concrete examples.
One concern with using concrete examples is that students might only remember the examples – especially if they are particularly memorable, such as fun or gimmicky examples – and will not be able to transfer their understanding from one example to another, or more broadly to the abstract concept. However, there does not seem to be any evidence that fun relevant examples actually hurt learning by harming memory for important information. Instead, fun examples and jokes tend to be more memorable, but this boost in memory for the joke does not seem to come at a cost to memory for the underlying concept (Baldassari & Kelley, 2012 ). However, two important caveats need to be highlighted. First, to the extent that the more memorable content is not relevant to the concepts of interest, learning of the target information can be compromised (Harp & Mayer, 1998 ). Thus, care must be taken to ensure that all examples and gimmicks are, in fact, related to the core concepts that the students need to acquire, and do not contain irrelevant perceptual features (Kaminski & Sloutsky, 2013 ).
The second issue is that novices often notice and remember the surface details of an example rather than the underlying structure. Experts, on the other hand, can extract the underlying structure from examples that have divergent surface features (Chi, Feltovich, & Glaser, 1981 ; see Fig. 5 for an example from physics). Gick and Holyoak ( 1983 ) tried to get students to apply a rule from one problem to another problem that appeared different on the surface, but was structurally similar. They found that providing multiple examples helped with this transfer process compared to only using one example – especially when the examples provided had different surface details. More work is also needed to determine how many examples are sufficient for generalization to occur (and this, of course, will vary with contextual factors and individual differences). Further research on the continuum between concrete/specific examples and more abstract concepts would also be informative. That is, if an example is not concrete enough, it may be too difficult to understand. On the other hand, if the example is too concrete, that could be detrimental to generalization to the more abstract concept (although a diverse set of very concrete examples may be able to help with this). In fact, in a controversial article, Kaminski, Sloutsky, and Heckler ( 2008 ) claimed that abstract examples were more effective than concrete examples. Later rebuttals of this paper contested whether the abstract versus concrete distinction was clearly defined in the original study (see Reed, 2008 , for a collection of letters on the subject). This ideal point along the concrete-abstract continuum might also interact with development.
Finding teacher blog posts on concrete examples proved to be more difficult than for the other strategies in this review. One optimistic possibility is that teachers frequently use concrete examples in their teaching, and thus do not think of this as a specific contribution from cognitive psychology; the one blog post we were able to find that discussed concrete examples suggests that this might be the case (Boulton, 2016 ). The idea of “linking abstract concepts with concrete examples” is also covered in 25% of teacher-training textbooks used in the US, according to the report by Pomerance et al. ( 2016 ); this is the second most frequently covered of the six strategies, after “posing probing questions” (i.e., elaborative interrogation). A useful direction for future research would be to establish how teachers are using concrete examples in their practice, and whether we can make any suggestions for improvement based on research into the science of learning. For example, if two examples are better than one (Bauernschmidt, 2017 ), are additional examples also needed, or are there diminishing returns from providing more examples? And, how can teachers best ensure that concrete examples are consistent with prior knowledge (Reed, 2008 )?
Dual coding
Both the memory literature and folk psychology support the notion of visual examples being beneficial—the adage of “a picture is worth a thousand words” (traced back to an advertising slogan from the 1920s; Meider, 1990 ). Indeed, it is well-understood that more information can be conveyed through a simple illustration than through several paragraphs of text (e.g., Barker & Manji, 1989 ; Mayer & Gallini, 1990 ). Illustrations can be particularly helpful when the described concept involves several parts or steps and is intended for individuals with low prior knowledge (Eitel & Scheiter, 2015 ; Mayer & Gallini, 1990 ). Figure 6 provides a concrete example of this, illustrating how information can flow through neurons and synapses.
In addition to being able to convey information more succinctly, pictures are also more memorable than words (Paivio & Csapo, 1969 , 1973 ). In the memory literature, this is referred to as the picture superiority effect , and dual coding theory was developed in part to explain this effect. Dual coding follows from the notion of text being accompanied by complementary visual information to enhance learning. Paivio ( 1971 , 1986 ) proposed dual coding theory as a mechanistic account for the integration of multiple information “codes” to process information. In this theory, a code corresponds to a modal or otherwise distinct representation of a concept—e.g., “mental images for ‘book’ have visual, tactual, and other perceptual qualities similar to those evoked by the referent objects on which the images are based” (Clark & Paivio, 1991 , p. 152). Aylwin ( 1990 ) provides a clear example of how the word “dog” can evoke verbal, visual, and enactive representations (see Fig. 7 for a similar example for the word “SPOON”, based on Aylwin, 1990 (Fig. 2 ) and Madan & Singhal, 2012a (Fig. 3 )). Codes can also correspond to emotional properties (Clark & Paivio, 1991 ; Paivio, 2013 ). Clark and Paivio ( 1991 ) provide a thorough review of dual coding theory and its relation to education, while Paivio ( 2007 ) provides a comprehensive treatise on dual coding theory. Broadly, dual coding theory suggests that providing multiple representations of the same information enhances learning and memory, and that information that more readily evokes additional representations (through automatic imagery processes) receives a similar benefit.
Paivio and Csapo ( 1973 ) suggest that verbal and imaginal codes have independent and additive effects on memory recall. Using visuals to improve learning and memory has been particularly applied to vocabulary learning (Danan, 1992 ; Sadoski, 2005 ), but has also shown success in other domains such as in health care (Hartland, Biddle, & Fallacaro, 2008 ). To take advantage of dual coding, verbal information should be accompanied by a visual representation when possible. However, while the studies discussed all indicate that the use of multiple representations of information is favorable, it is important to acknowledge that each representation also increases cognitive load and can lead to over-saturation (Mayer & Moreno, 2003 ).
Given that pictures are generally remembered better than words, it is important to ensure that the pictures students are provided with are helpful and relevant to the content they are expected to learn. McNeill, Uttal, Jarvin, and Sternberg ( 2009 ) found that providing visual examples decreased conceptual errors. However, McNeill et al. also found that when students were given visually rich examples, they performed more poorly than students who were not given any visual example, suggesting that the visual details can at times become a distraction and hinder performance. Thus, it is important to consider that images used in teaching are clear and not ambiguous in their meaning (Schwartz, 2007 ).
Further broadening the scope of dual coding theory, Engelkamp and Zimmer ( 1984 ) suggest that motor movements, such as “turning the handle,” can provide an additional motor code that can improve memory, linking studies of motor actions (enactment) with dual coding theory (Clark & Paivio, 1991 ; Engelkamp & Cohen, 1991 ; Madan & Singhal, 2012c ). Indeed, enactment effects appear to primarily occur during learning, rather than during retrieval (Peterson & Mulligan, 2010 ). Along similar lines, Wammes, Meade, and Fernandes ( 2016 ) demonstrated that generating drawings can provide memory benefits beyond what could otherwise be explained by visual imagery, picture superiority, and other memory enhancing effects. Providing convergent evidence, even when overt motor actions are not critical in themselves, words representing functional objects have been shown to enhance later memory (Madan & Singhal, 2012b ; Montefinese, Ambrosini, Fairfield, & Mammarella, 2013 ). This indicates that motoric processes can improve memory similarly to visual imagery, similar to memory differences for concrete vs. abstract words. Further research suggests that automatic motor simulation for functional objects is likely responsible for this memory benefit (Madan, Chen, & Singhal, 2016 ).
When teachers combine visuals and words in their educational practice, however, they may not always be taking advantage of dual coding – at least, not in the optimal manner. For example, a recent discussion on Twitter centered around one teacher’s decision to have 7 th Grade students replace certain words in their science laboratory report with a picture of that word (e.g., the instructions read “using a syringe …” and a picture of a syringe replaced the word; Turner, 2016a ). Other teachers argued that this was not dual coding (Beaven, 2016 ; Williams, 2016 ), because there were no longer two different representations of the information. The first teacher maintained that dual coding was preserved, because this laboratory report with pictures was to be used alongside the original, fully verbal report (Turner, 2016b ). This particular implementation – having students replace individual words with pictures – has not been examined in the cognitive literature, presumably because no benefit would be expected. In any case, we need to be clearer about implementations for dual coding, and more research is needed to clarify how teachers can make use of the benefits conferred by multiple representations and picture superiority.
Critically, dual coding theory is distinct from the notion of “learning styles,” which describe the idea that individuals benefit from instruction that matches their modality preference. While this idea is pervasive and individuals often subjectively feel that they have a preference, evidence indicates that the learning styles theory is not supported by empirical findings (e.g., Kavale, Hirshoren, & Forness, 1998 ; Pashler, McDaniel, Rohrer, & Bjork, 2008 ; Rohrer & Pashler, 2012 ). That is, there is no evidence that instructing students in their preferred learning style leads to an overall improvement in learning (the “meshing” hypothesis). Moreover, learning styles have come to be described as a myth or urban legend within psychology (Coffield, Moseley, Hall, & Ecclestone, 2004 ; Hattie & Yates, 2014 ; Kirschner & van Merriënboer, 2013 ; Kirschner, 2017 ); skepticism about learning styles is a common stance amongst evidence-informed teachers (e.g., Saunders, 2016 ). Providing evidence against the notion of learning styles, Kraemer, Rosenberg, and Thompson-Schill ( 2009 ) found that individuals who scored as “verbalizers” and “visualizers” did not perform any better on experimental trials matching their preference. Instead, it has recently been shown that learning through one’s preferred learning style is associated with elevated subjective judgements of learning, but not objective performance (Knoll, Otani, Skeel, & Van Horn, 2017 ). In contrast to learning styles, dual coding is based on providing additional, complementary forms of information to enhance learning, rather than tailoring instruction to individuals’ preferences.
Genuine educational environments present many opportunities for combining the strategies outlined above. Spacing can be particularly potent for learning if it is combined with retrieval practice. The additive benefits of retrieval practice and spacing can be gained by engaging in retrieval practice multiple times (also known as distributed practice; see Cepeda et al., 2006 ). Interleaving naturally entails spacing if students interleave old and new material. Concrete examples can be both verbal and visual, making use of dual coding. In addition, the strategies of elaboration, concrete examples, and dual coding all work best when used as part of retrieval practice. For example, in the concept-mapping studies mentioned above (Blunt & Karpicke, 2014 ; Karpicke, Blunt, et al., 2014 ), creating concept maps while looking at course materials (e.g., a textbook) was not as effective for later memory as creating concept maps from memory. When practicing elaborative interrogation, students can start off answering the “how” and “why” questions they pose for themselves using class materials, and work their way up to answering them from memory. And when interleaving different problem types, students should be practicing answering them rather than just looking over worked examples.
But while these ideas for strategy combinations have empirical bases, it has not yet been established whether the benefits of the strategies to learning are additive, super-additive, or, in some cases, incompatible. Thus, future research needs to (a) better formalize the definition of each strategy (particularly critical for elaboration and dual coding), (b) identify best practices for implementation in the classroom, (c) delineate the boundary conditions of each strategy, and (d) strategically investigate interactions between the six strategies we outlined in this manuscript.
Aleven, V. A., & Koedinger, K. R. (2002). An effective metacognitive strategy: learning by doing and explaining with a computer-based cognitive tutor. Cognitive Science, 26 , 147–179.
Article Google Scholar
Anderson, J. R. (1983). A spreading activation theory of memory. Journal of Verbal Learning and Verbal Behavior, 22 , 261–295.
Arnold, K. M., & McDermott, K. B. (2013). Test-potentiated learning: distinguishing between direct and indirect effects of tests. Journal of Experimental Psychology: Learning, Memory, and Cognition, 39 , 940–945.
PubMed Google Scholar
Aylwin, S. (1990). Imagery and affect: big questions, little answers. In P. J. Thompson, D. E. Marks, & J. T. E. Richardson (Eds.), Imagery: Current developments . New York: International Library of Psychology.
Google Scholar
Baldassari, M. J., & Kelley, M. (2012). Make’em laugh? The mnemonic effect of humor in a speech. Psi Chi Journal of Psychological Research, 17 , 2–9.
Barker, P. G., & Manji, K. A. (1989). Pictorial dialogue methods. International Journal of Man-Machine Studies, 31 , 323–347.
Bauernschmidt, A. (2017). GUEST POST: two examples are better than one. [Blog post]. The Learning Scientists Blog . Retrieved from http://www.learningscientists.org/blog/2017/5/30-1 . Accessed 25 Dec 2017.
Beaven, T. (2016). @doctorwhy @FurtherEdagogy @doc_kristy Right, I thought the whole point of dual coding was to use TWO codes: pics + words of the SAME info? [Tweet]. Retrieved from https://twitter.com/TitaBeaven/status/807504041341308929 . Accessed 25 Dec 2017.
Bellezza, F. S., Cheesman, F. L., & Reddy, B. G. (1977). Organization and semantic elaboration in free recall. Journal of Experimental Psychology: Human Learning and Memory, 3 , 539–550.
Benney, D. (2016). (Trying to apply) spacing in a content heavy subject [Blog post]. Retrieved from https://mrbenney.wordpress.com/2016/10/16/trying-to-apply-spacing-in-science/ . Accessed 25 Dec 2017.
Berry, D. C. (1983). Metacognitive experience and transfer of logical reasoning. Quarterly Journal of Experimental Psychology, 35A , 39–49.
Birnbaum, M. S., Kornell, N., Bjork, E. L., & Bjork, R. A. (2013). Why interleaving enhances inductive learning: the roles of discrimination and retrieval. Memory & Cognition, 41 , 392–402.
Bjork, R. A. (1999). Assessing our own competence: heuristics and illusions. In D. Gopher & A. Koriat (Eds.), Attention and peformance XVII. Cognitive regulation of performance: Interaction of theory and application (pp. 435–459). Cambridge, MA: MIT Press.
Bjork, R. A. (1994). Memory and metamemory considerations in the training of human beings. In J. Metcalfe & A. Shimamura (Eds.), Metacognition: Knowing about knowing (pp. 185–205). Cambridge, MA: MIT Press.
Bjork, R. A., & Bjork, E. L. (1992). A new theory of disuse and an old theory of stimulus fluctuation. From learning processes to cognitive processes: Essays in honor of William K. Estes, 2 , 35–67.
Bjork, E. L., & Bjork, R. A. (2011). Making things hard on yourself, but in a good way: creating desirable difficulties to enhance learning. Psychology and the real world: Essays illustrating fundamental contributions to society , 56–64.
Blunt, J. R., & Karpicke, J. D. (2014). Learning with retrieval-based concept mapping. Journal of Educational Psychology, 106 , 849–858.
Boulton, K. (2016). What does cognitive overload look like in the humanities? [Blog post]. Retrieved from https://educationechochamberuncut.wordpress.com/2016/03/05/what-does-cognitive-overload-look-like-in-the-humanities-kris-boulton-2/ . Accessed 25 Dec 2017.
Brown, P. C., Roediger, H. L., & McDaniel, M. A. (2014). Make it stick . Cambridge, MA: Harvard University Press.
Book Google Scholar
Butler, A. C. (2010). Repeated testing produces superior transfer of learning relative to repeated studying. Journal of Experimental Psychology: Learning, Memory, and Cognition, 36 , 1118–1133.
Caplan, J. B., & Madan, C. R. (2016). Word-imageability enhances association-memory by recruiting hippocampal activity. Journal of Cognitive Neuroscience, 28 , 1522–1538.
Article PubMed Google Scholar
Cepeda, N. J., Pashler, H., Vul, E., Wixted, J. T., & Rohrer, D. (2006). Distributed practice in verbal recall tasks: a review and quantitative synthesis. Psychological Bulletin, 132 , 354–380.
Cepeda, N. J., Vul, E., Rohrer, D., Wixted, J. T., & Pashler, H. (2008). Spacing effects in learning a temporal ridgeline of optimal retention. Psychological Science, 19 , 1095–1102.
Chi, M. T., De Leeuw, N., Chiu, M. H., & LaVancher, C. (1994). Eliciting self-explanations improves understanding. Cognitive Science, 18 , 439–477.
Chi, M. T., Feltovich, P. J., & Glaser, R. (1981). Categorization and representation of physics problems by experts and novices. Cognitive Science, 5 , 121–152.
CIFE. (2012). No January A level and other changes. Retrieved from http://www.cife.org.uk/cife-general-news/no-january-a-level-and-other-changes/ . Accessed 25 Dec 2017.
Clark, D. (2016). One book on learning that every teacher, lecturer & trainer should read (7 reasons) [Blog post]. Retrieved from http://donaldclarkplanb.blogspot.com/2016/03/one-book-on-learning-that-every-teacher.html . Accessed 25 Dec 2017.
Clark, J. M., & Paivio, A. (1991). Dual coding theory and education. Educational Psychology Review, 3 , 149–210.
Class Teaching. (2013). Deep questioning [Blog post]. Retrieved from https://classteaching.wordpress.com/2013/07/12/deep-questioning/ . Accessed 25 Dec 2017.
Clinton, V., Alibali, M. W., & Nathan, M. J. (2016). Learning about posterior probability: do diagrams and elaborative interrogation help? The Journal of Experimental Education, 84 , 579–599.
Coffield, F., Moseley, D., Hall, E., & Ecclestone, K. (2004). Learning styles and pedagogy in post-16 learning: a systematic and critical review . London: Learning & Skills Research Centre.
Cohen, R. L. (1981). On the generality of some memory laws. Scandinavian Journal of Psychology, 22 , 267–281.
Cooper, H. (1989). Synthesis of research on homework. Educational Leadership, 47 , 85–91.
Corbett, A. T., Reed, S. K., Hoffmann, R., MacLaren, B., & Wagner, A. (2010). Interleaving worked examples and cognitive tutor support for algebraic modeling of problem situations. In Proceedings of the Thirty-Second Annual Meeting of the Cognitive Science Society (pp. 2882–2887).
Cox, D. (2015). No stakes testing – not telling students their results [Blog post]. Retrieved from https://missdcoxblog.wordpress.com/2015/06/06/no-stakes-testing-not-telling-students-their-results/ . Accessed 25 Dec 2017.
Cox, D. (2016a). Ditch revision. Teach it well [Blog post]. Retrieved from https://missdcoxblog.wordpress.com/2016/01/09/ditch-revision-teach-it-well/ . Accessed 25 Dec 2017.
Cox, D. (2016b). ‘They need to remember this in three years time’: spacing & interleaving for the new GCSEs [Blog post]. Retrieved from https://missdcoxblog.wordpress.com/2016/03/25/they-need-to-remember-this-in-three-years-time-spacing-interleaving-for-the-new-gcses/ . Accessed 25 Dec 2017.
Craik, F. I. (2002). Levels of processing: past, present… future? Memory, 10 , 305–318.
Craik, F. I., & Lockhart, R. S. (1972). Levels of processing: a framework for memory research. Journal of Verbal Learning and Verbal Behavior, 11 , 671–684.
Danan, M. (1992). Reversed subtitling and dual coding theory: new directions for foreign language instruction. Language Learning, 42 , 497–527.
Dettmers, S., Trautwein, U., & Lüdtke, O. (2009). The relationship between homework time and achievement is not universal: evidence from multilevel analyses in 40 countries. School Effectiveness and School Improvement, 20 , 375–405.
Dirkx, K. J., Kester, L., & Kirschner, P. A. (2014). The testing effect for learning principles and procedures from texts. The Journal of Educational Research, 107 , 357–364.
Dunlosky, J. (2013). Strengthening the student toolbox: study strategies to boost learning. American Educator, 37 (3), 12–21.
Dunlosky, J., Rawson, K. A., Marsh, E. J., Nathan, M. J., & Willingham, D. T. (2013). Improving students’ learning with effective learning techniques: promising directions from cognitive and educational psychology. Psychological Science in the Public Interest, 14 , 4–58.
Ebbinghaus, H. (1913). Memory (HA Ruger & CE Bussenius, Trans.). New York: Columbia University, Teachers College. (Original work published 1885) . Retrieved from http://psychclassics.yorku.ca/Ebbinghaus/memory8.htm . Accessed 25 Dec 2017.
Eglington, L. G., & Kang, S. H. (2016). Retrieval practice benefits deductive inference. Educational Psychology Review , 1–14.
Eitel, A., & Scheiter, K. (2015). Picture or text first? Explaining sequential effects when learning with pictures and text. Educational Psychology Review, 27 , 153–180.
Engelkamp, J., & Cohen, R. L. (1991). Current issues in memory of action events. Psychological Research, 53 , 175–182.
Engelkamp, J., & Zimmer, H. D. (1984). Motor programme information as a separable memory unit. Psychological Research, 46 , 283–299.
Fawcett, D. (2013). Can I be that little better at……using cognitive science/psychology/neurology to plan learning? [Blog post]. Retrieved from http://reflectionsofmyteaching.blogspot.com/2013/09/can-i-be-that-little-better-atusing.html . Accessed 25 Dec 2017.
Fiechter, J. L., & Benjamin, A. S. (2017). Diminishing-cues retrieval practice: a memory-enhancing technique that works when regular testing doesn’t. Psychonomic Bulletin & Review , 1–9.
Firth, J. (2016). Spacing in teaching practice [Blog post]. Retrieved from http://www.learningscientists.org/blog/2016/4/12-1 . Accessed 25 Dec 2017.
Fordham, M. [mfordhamhistory]. (2016). Is there a meaningful distinction in psychology between ‘thinking’ & ‘critical thinking’? [Tweet]. Retrieved from https://twitter.com/mfordhamhistory/status/809525713623781377 . Accessed 25 Dec 2017.
Fritz, C. O., Morris, P. E., Nolan, D., & Singleton, J. (2007). Expanding retrieval practice: an effective aid to preschool children’s learning. The Quarterly Journal of Experimental Psychology, 60 , 991–1004.
Gates, A. I. (1917). Recitation as a factory in memorizing. Archives of Psychology, 6.
Gick, M. L., & Holyoak, K. J. (1983). Schema induction and analogical transfer. Cognitive Psychology, 15 , 1–38.
Gorman, A. M. (1961). Recognition memory for nouns as a function of abstractedness and frequency. Journal of Experimental Psychology, 61 , 23–39.
Hainselin, M., Picard, L., Manolli, P., Vankerkore-Candas, S., & Bourdin, B. (2017). Hey teacher, don’t leave them kids alone: action is better for memory than reading. Frontiers in Psychology , 8 .
Harp, S. F., & Mayer, R. E. (1998). How seductive details do their damage. Journal of Educational Psychology, 90 , 414–434.
Hartland, W., Biddle, C., & Fallacaro, M. (2008). Audiovisual facilitation of clinical knowledge: A paradigm for dispersed student education based on Paivio’s dual coding theory. AANA Journal, 76 , 194–198.
Hattie, J., & Yates, G. (2014). Visible learning and the science of how we learn . New York: Routledge.
Hausman, H., & Kornell, N. (2014). Mixing topics while studying does not enhance learning. Journal of Applied Research in Memory and Cognition, 3 , 153–160.
Hinze, S. R., & Rapp, D. N. (2014). Retrieval (sometimes) enhances learning: performance pressure reduces the benefits of retrieval practice. Applied Cognitive Psychology, 28 , 597–606.
Hirshman, E. (2001). Elaboration in memory. In N. J. Smelser & P. B. Baltes (Eds.), International encyclopedia of the social & behavioral sciences (pp. 4369–4374). Oxford: Pergamon.
Chapter Google Scholar
Hobbiss, M. (2016). Make it meaningful! Elaboration [Blog post]. Retrieved from https://hobbolog.wordpress.com/2016/06/09/make-it-meaningful-elaboration/ . Accessed 25 Dec 2017.
Jones, F. (2016). Homework – is it really that useless? [Blog post]. Retrieved from http://www.learningscientists.org/blog/2016/4/5-1 . Accessed 25 Dec 2017.
Kaminski, J. A., & Sloutsky, V. M. (2013). Extraneous perceptual information interferes with children’s acquisition of mathematical knowledge. Journal of Educational Psychology, 105 (2), 351–363.
Kaminski, J. A., Sloutsky, V. M., & Heckler, A. F. (2008). The advantage of abstract examples in learning math. Science, 320 , 454–455.
Kang, S. H. (2016). Spaced repetition promotes efficient and effective learning policy implications for instruction. Policy Insights from the Behavioral and Brain Sciences, 3 , 12–19.
Kang, S. H. K., McDermott, K. B., & Roediger, H. L. (2007). Test format and corrective feedback modify the effects of testing on long-term retention. European Journal of Cognitive Psychology, 19 , 528–558.
Karpicke, J. D., & Aue, W. R. (2015). The testing effect is alive and well with complex materials. Educational Psychology Review, 27 , 317–326.
Karpicke, J. D., Blunt, J. R., Smith, M. A., & Karpicke, S. S. (2014). Retrieval-based learning: The need for guided retrieval in elementary school children. Journal of Applied Research in Memory and Cognition, 3 , 198–206.
Karpicke, J. D., Lehman, M., & Aue, W. R. (2014). Retrieval-based learning: an episodic context account. In B. H. Ross (Ed.), Psychology of Learning and Motivation (Vol. 61, pp. 237–284). San Diego, CA: Elsevier Academic Press.
Karpicke, J. D., Blunt, J. R., & Smith, M. A. (2016). Retrieval-based learning: positive effects of retrieval practice in elementary school children. Frontiers in Psychology, 7 .
Kavale, K. A., Hirshoren, A., & Forness, S. R. (1998). Meta-analytic validation of the Dunn and Dunn model of learning-style preferences: a critique of what was Dunn. Learning Disabilities Research & Practice, 13 , 75–80.
Khanna, M. M. (2015). Ungraded pop quizzes: test-enhanced learning without all the anxiety. Teaching of Psychology, 42 , 174–178.
Kirby, J. (2014). One scientific insight for curriculum design [Blog post]. Retrieved from https://pragmaticreform.wordpress.com/2014/05/05/scientificcurriculumdesign/ . Accessed 25 Dec 2017.
Kirschner, P. A. (2017). Stop propagating the learning styles myth. Computers & Education, 106 , 166–171.
Kirschner, P. A., & van Merriënboer, J. J. G. (2013). Do learners really know best? Urban legends in education. Educational Psychologist, 48 , 169–183.
Knoll, A. R., Otani, H., Skeel, R. L., & Van Horn, K. R. (2017). Learning style, judgments of learning, and learning of verbal and visual information. British Journal of Psychology, 108 , 544-563.
Kornell, N., & Bjork, R. A. (2008). Learning concepts and categories is spacing the “enemy of induction”? Psychological Science, 19 , 585–592.
Kornell, N., & Finn, B. (2016). Self-regulated learning: an overview of theory and data. In J. Dunlosky & S. Tauber (Eds.), The Oxford Handbook of Metamemory (pp. 325–340). New York: Oxford University Press.
Kornell, N., Klein, P. J., & Rawson, K. A. (2015). Retrieval attempts enhance learning, but retrieval success (versus failure) does not matter. Journal of Experimental Psychology: Learning, Memory, and Cognition, 41 , 283–294.
Kraemer, D. J. M., Rosenberg, L. M., & Thompson-Schill, S. L. (2009). The neural correlates of visual and verbal cognitive styles. Journal of Neuroscience, 29 , 3792–3798.
Article PubMed PubMed Central Google Scholar
Kraft, N. (2015). Spaced practice and repercussions for teaching. Retrieved from http://nathankraft.blogspot.com/2015/08/spaced-practice-and-repercussions-for.html . Accessed 25 Dec 2017.
Learning Scientists. (2016a). Weekly Digest #3: How teachers implement interleaving in their curriculum [Blog post]. Retrieved from http://www.learningscientists.org/blog/2016/3/28/weekly-digest-3 . Accessed 25 Dec 2017.
Learning Scientists. (2016b). Weekly Digest #13: how teachers implement retrieval in their classrooms [Blog post]. Retrieved from http://www.learningscientists.org/blog/2016/6/5/weekly-digest-13 . Accessed 25 Dec 2017.
Learning Scientists. (2016c). Weekly Digest #40: teachers’ implementation of principles from “Make It Stick” [Blog post]. Retrieved from http://www.learningscientists.org/blog/2016/12/18-1 . Accessed 25 Dec 2017.
Learning Scientists. (2017). Weekly Digest #54: is there an app for that? Studying 2.0 [Blog post]. Retrieved from http://www.learningscientists.org/blog/2017/4/9/weekly-digest-54 . Accessed 25 Dec 2017.
LeFevre, J.-A., & Dixon, P. (1986). Do written instructions need examples? Cognition and Instruction, 3 , 1–30.
Lew, K., Fukawa-Connelly, T., Mejí-Ramos, J. P., & Weber, K. (2016). Lectures in advanced mathematics: Why students might not understand what the mathematics professor is trying to convey. Journal of Research in Mathematics Education, 47 , 162–198.
Lindsey, R. V., Shroyer, J. D., Pashler, H., & Mozer, M. C. (2014). Improving students’ long-term knowledge retention through personalized review. Psychological Science, 25 , 639–647.
Lipko-Speed, A., Dunlosky, J., & Rawson, K. A. (2014). Does testing with feedback help grade-school children learn key concepts in science? Journal of Applied Research in Memory and Cognition, 3 , 171–176.
Lockhart, R. S., & Craik, F. I. (1990). Levels of processing: a retrospective commentary on a framework for memory research. Canadian Journal of Psychology, 44 , 87–112.
Lovell, O. (2017). How do we know what to put on the quiz? [Blog Post]. Retrieved from http://www.ollielovell.com/olliesclassroom/know-put-quiz/ . Accessed 25 Dec 2017.
Luehmann, A. L. (2008). Using blogging in support of teacher professional identity development: a case study. The Journal of the Learning Sciences, 17 , 287–337.
Madan, C. R., Glaholt, M. G., & Caplan, J. B. (2010). The influence of item properties on association-memory. Journal of Memory and Language, 63 , 46–63.
Madan, C. R., & Singhal, A. (2012a). Motor imagery and higher-level cognition: four hurdles before research can sprint forward. Cognitive Processing, 13 , 211–229.
Madan, C. R., & Singhal, A. (2012b). Encoding the world around us: motor-related processing influences verbal memory. Consciousness and Cognition, 21 , 1563–1570.
Madan, C. R., & Singhal, A. (2012c). Using actions to enhance memory: effects of enactment, gestures, and exercise on human memory. Frontiers in Psychology, 3 .
Madan, C. R., Chen, Y. Y., & Singhal, A. (2016). ERPs differentially reflect automatic and deliberate processing of the functional manipulability of objects. Frontiers in Human Neuroscience, 10 .
Mandler, G. (1979). Organization and repetition: organizational principles with special reference to rote learning. In L. G. Nilsson (Ed.), Perspectives on Memory Research (pp. 293–327). New York: Academic Press.
Marsh, E. J., Fazio, L. K., & Goswick, A. E. (2012). Memorial consequences of testing school-aged children. Memory, 20 , 899–906.
Mayer, R. E., & Gallini, J. K. (1990). When is an illustration worth ten thousand words? Journal of Educational Psychology, 82 , 715–726.
Mayer, R. E., & Moreno, R. (2003). Nine ways to reduce cognitive load in multimedia learning. Educational Psychologist, 38 , 43–52.
McDaniel, M. A., & Donnelly, C. M. (1996). Learning with analogy and elaborative interrogation. Journal of Educational Psychology, 88 , 508–519.
McDaniel, M. A., Thomas, R. C., Agarwal, P. K., McDermott, K. B., & Roediger, H. L. (2013). Quizzing in middle-school science: successful transfer performance on classroom exams. Applied Cognitive Psychology, 27 , 360–372.
McDermott, K. B., Agarwal, P. K., D’Antonio, L., Roediger, H. L., & McDaniel, M. A. (2014). Both multiple-choice and short-answer quizzes enhance later exam performance in middle and high school classes. Journal of Experimental Psychology: Applied, 20 , 3–21.
McHugh, A. (2013). High-stakes tests: bad for students, teachers, and education in general [Blog post]. Retrieved from https://teacherbiz.wordpress.com/2013/07/01/high-stakes-tests-bad-for-students-teachers-and-education-in-general/ . Accessed 25 Dec 2017.
McNeill, N. M., Uttal, D. H., Jarvin, L., & Sternberg, R. J. (2009). Should you show me the money? Concrete objects both hurt and help performance on mathematics problems. Learning and Instruction, 19 , 171–184.
Meider, W. (1990). “A picture is worth a thousand words”: from advertising slogan to American proverb. Southern Folklore, 47 , 207–225.
Michaela Community School. (2014). Homework. Retrieved from http://mcsbrent.co.uk/homework-2/ . Accessed 25 Dec 2017.
Montefinese, M., Ambrosini, E., Fairfield, B., & Mammarella, N. (2013). The “subjective” pupil old/new effect: is the truth plain to see? International Journal of Psychophysiology, 89 , 48–56.
O’Neil, H. F., Chung, G. K., Kerr, D., Vendlinski, T. P., Buschang, R. E., & Mayer, R. E. (2014). Adding self-explanation prompts to an educational computer game. Computers In Human Behavior, 30 , 23–28.
Overoye, A. L., & Storm, B. C. (2015). Harnessing the power of uncertainty to enhance learning. Translational Issues in Psychological Science, 1 , 140–148.
Paivio, A. (1971). Imagery and verbal processes . New York: Holt, Rinehart and Winston.
Paivio, A. (1986). Mental representations: a dual coding approach . New York: Oxford University Press.
Paivio, A. (2007). Mind and its evolution: a dual coding theoretical approach . Mahwah: Erlbaum.
Paivio, A. (2013). Dual coding theory, word abstractness, and emotion: a critical review of Kousta et al. (2011). Journal of Experimental Psychology: General, 142 , 282–287.
Paivio, A., & Csapo, K. (1969). Concrete image and verbal memory codes. Journal of Experimental Psychology, 80 , 279–285.
Paivio, A., & Csapo, K. (1973). Picture superiority in free recall: imagery or dual coding? Cognitive Psychology, 5 , 176–206.
Paivio, A., Walsh, M., & Bons, T. (1994). Concreteness effects on memory: when and why? Journal of Experimental Psychology: Learning, Memory, and Cognition, 20 , 1196–1204.
Pashler, H., McDaniel, M., Rohrer, D., & Bjork, R. (2008). Learning styles: concepts and evidence. Psychological Science in the Public Interest, 9 , 105–119.
Pashler, H., Bain, P. M., Bottge, B. A., Graesser, A., Koedinger, K., McDaniel, M., & Metcalfe, J. (2007). Organizing instruction and study to improve student learning. IES practice guide. NCER 2007–2004. National Center for Education Research .
Patel, R., Liu, R., & Koedinger, K. (2016). When to block versus interleave practice? Evidence against teaching fraction addition before fraction multiplication. In Proceedings of the 38th Annual Meeting of the Cognitive Science Society, Philadelphia, PA .
Penfound, B. (2017). Journey to interleaved practice #2 [Blog Post]. Retrieved from https://fullstackcalculus.com/2017/02/03/journey-to-interleaved-practice-2/ . Accessed 25 Dec 2017.
Penfound, B. [BryanPenfound]. (2016). Does blocked practice/learning lessen cognitive load? Does interleaved practice/learning provide productive struggle? [Tweet]. Retrieved from https://twitter.com/BryanPenfound/status/808759362244087808 . Accessed 25 Dec 2017.
Peterson, D. J., & Mulligan, N. W. (2010). Enactment and retrieval. Memory & Cognition, 38 , 233–243.
Picciotto, H. (2009). Lagging homework [Blog post]. Retrieved from http://blog.mathedpage.org/2013/06/lagging-homework.html . Accessed 25 Dec 2017.
Pomerance, L., Greenberg, J., & Walsh, K. (2016). Learning about learning: what every teacher needs to know. Retrieved from http://www.nctq.org/dmsView/Learning_About_Learning_Report . Accessed 25 Dec 2017.
Postman, L. (1976). Methodology of human learning. In W. K. Estes (Ed.), Handbook of learning and cognitive processes (Vol. 3). Hillsdale: Erlbaum.
Pressley, M., McDaniel, M. A., Turnure, J. E., Wood, E., & Ahmad, M. (1987). Generation and precision of elaboration: effects on intentional and incidental learning. Journal of Experimental Psychology: Learning, Memory, and Cognition, 13 , 291–300.
Reed, S. K. (2008). Concrete examples must jibe with experience. Science, 322 , 1632–1633.
researchED. (2013). How it all began. Retrieved from http://www.researched.org.uk/about/our-story/ . Accessed 25 Dec 2017.
Ritchie, S. J., Della Sala, S., & McIntosh, R. D. (2013). Retrieval practice, with or without mind mapping, boosts fact learning in primary school children. PLoS One, 8 (11), e78976.
Rittle-Johnson, B. (2006). Promoting transfer: effects of self-explanation and direct instruction. Child Development, 77 , 1–15.
Roediger, H. L. (1985). Remembering Ebbinghaus. [Retrospective review of the book On Memory , by H. Ebbinghaus]. Contemporary Psychology, 30 , 519–523.
Roediger, H. L. (2013). Applying cognitive psychology to education translational educational science. Psychological Science in the Public Interest, 14 , 1–3.
Roediger, H. L., & Karpicke, J. D. (2006). The power of testing memory: basic research and implications for educational practice. Perspectives on Psychological Science, 1 , 181–210.
Roediger, H. L., Putnam, A. L., & Smith, M. A. (2011). Ten benefits of testing and their applications to educational practice. In J. Mester & B. Ross (Eds.), The psychology of learning and motivation: cognition in education (pp. 1–36). Oxford: Elsevier.
Roediger, H. L., Finn, B., & Weinstein, Y. (2012). Applications of cognitive science to education. In Della Sala, S., & Anderson, M. (Eds.), Neuroscience in education: the good, the bad, and the ugly . Oxford, UK: Oxford University Press.
Roelle, J., & Berthold, K. (2017). Effects of incorporating retrieval into learning tasks: the complexity of the tasks matters. Learning and Instruction, 49 , 142–156.
Rohrer, D. (2012). Interleaving helps students distinguish among similar concepts. Educational Psychology Review, 24(3), 355–367.
Rohrer, D., Dedrick, R. F., & Stershic, S. (2015). Interleaved practice improves mathematics learning. Journal of Educational Psychology, 107 , 900–908.
Rohrer, D., & Pashler, H. (2012). Learning styles: Where’s the evidence? Medical Education, 46 , 34–35.
Rohrer, D., & Taylor, K. (2007). The shuffling of mathematics problems improves learning. Instructional Science, 35 , 481–498.
Rose, N. (2014). Improving the effectiveness of homework [Blog post]. Retrieved from https://evidenceintopractice.wordpress.com/2014/03/20/improving-the-effectiveness-of-homework/ . Accessed 25 Dec 2017.
Sadoski, M. (2005). A dual coding view of vocabulary learning. Reading & Writing Quarterly, 21 , 221–238.
Saunders, K. (2016). It really is time we stopped talking about learning styles [Blog post]. Retrieved from http://martingsaunders.com/2016/10/it-really-is-time-we-stopped-talking-about-learning-styles/ . Accessed 25 Dec 2017.
Schwartz, D. (2007). If a picture is worth a thousand words, why are you reading this essay? Social Psychology Quarterly, 70 , 319–321.
Shumaker, H. (2016). Homework is wrecking our kids: the research is clear, let’s ban elementary homework. Salon. Retrieved from http://www.salon.com/2016/03/05/homework_is_wrecking_our_kids_the_research_is_clear_lets_ban_elementary_homework . Accessed 25 Dec 2017.
Smith, A. M., Floerke, V. A., & Thomas, A. K. (2016). Retrieval practice protects memory against acute stress. Science, 354 , 1046–1048.
Smith, M. A., Blunt, J. R., Whiffen, J. W., & Karpicke, J. D. (2016). Does providing prompts during retrieval practice improve learning? Applied Cognitive Psychology, 30 , 784–802.
Smith, M. A., & Karpicke, J. D. (2014). Retrieval practice with short-answer, multiple-choice, and hybrid formats. Memory, 22 , 784–802.
Smith, M. A., Roediger, H. L., & Karpicke, J. D. (2013). Covert retrieval practice benefits retention as much as overt retrieval practice. Journal of Experimental Psychology: Learning, Memory, and Cognition, 39 , 1712–1725.
Son, J. Y., & Rivas, M. J. (2016). Designing clicker questions to stimulate transfer. Scholarship of Teaching and Learning in Psychology, 2 , 193–207.
Szpunar, K. K., Khan, N. Y., & Schacter, D. L. (2013). Interpolated memory tests reduce mind wandering and improve learning of online lectures. Proceedings of the National Academy of Sciences, 110 , 6313–6317.
Thomson, R., & Mehring, J. (2016). Better vocabulary study strategies for long-term learning. Kwansei Gakuin University Humanities Review, 20 , 133–141.
Trafton, J. G., & Reiser, B. J. (1993). Studying examples and solving problems: contributions to skill acquisition . Technical report, Naval HCI Research Lab, Washington, DC, USA.
Tran, R., Rohrer, D., & Pashler, H. (2015). Retrieval practice: the lack of transfer to deductive inferences. Psychonomic Bulletin & Review, 22 , 135–140.
Turner, K. [doc_kristy]. (2016a). My dual coding (in red) and some y8 work @AceThatTest they really enjoyed practising the technique [Tweet]. Retrieved from https://twitter.com/doc_kristy/status/807220355395977216 . Accessed 25 Dec 2017.
Turner, K. [doc_kristy]. (2016b). @FurtherEdagogy @doctorwhy their work is revision work, they already have the words on a different page, to compliment not replace [Tweet]. Retrieved from https://twitter.com/doc_kristy/status/807360265100599301 . Accessed 25 Dec 2017.
Valle, A., Regueiro, B., Núñez, J. C., Rodríguez, S., Piñeiro, I., & Rosário, P. (2016). Academic goals, student homework engagement, and academic achievement in elementary school. Frontiers in Psychology, 7 .
Van Gog, T., & Sweller, J. (2015). Not new, but nearly forgotten: the testing effect decreases or even disappears as the complexity of learning materials increases. Educational Psychology Review, 27 , 247–264.
Wammes, J. D., Meade, M. E., & Fernandes, M. A. (2016). The drawing effect: evidence for reliable and robust memory benefits in free recall. Quarterly Journal of Experimental Psychology, 69 , 1752–1776.
Weinstein, Y., Gilmore, A. W., Szpunar, K. K., & McDermott, K. B. (2014). The role of test expectancy in the build-up of proactive interference in long-term memory. Journal of Experimental Psychology: Learning, Memory, and Cognition, 40 , 1039–1048.
Weinstein, Y., Nunes, L. D., & Karpicke, J. D. (2016). On the placement of practice questions during study. Journal of Experimental Psychology: Applied, 22 , 72–84.
Weinstein, Y., & Weinstein-Jones, F. (2017). Topic and quiz spacing spreadsheet: a planning tool for teachers [Blog Post]. Retrieved from http://www.learningscientists.org/blog/2017/5/11-1 . Accessed 25 Dec 2017.
Weinstein-Jones, F., & Weinstein, Y. (2017). Topic spacing spreadsheet for teachers [Excel macro]. Zenodo. http://doi.org/10.5281/zenodo.573764 . Accessed 25 Dec 2017.
Williams, D. [FurtherEdagogy]. (2016). @doctorwhy @doc_kristy word accompanying the visual? I’m unclear how removing words benefit? Would a flow chart better suit a scientific exp? [Tweet]. Retrieved from https://twitter.com/FurtherEdagogy/status/807356800509104128 . Accessed 25 Dec 2017.
Wood, B. (2017). And now for something a little bit different….[Blog post]. Retrieved from https://justateacherstandinginfrontofaclass.wordpress.com/2017/04/20/and-now-for-something-a-little-bit-different/ . Accessed 25 Dec 2017.
Wooldridge, C. L., Bugg, J. M., McDaniel, M. A., & Liu, Y. (2014). The testing effect with authentic educational materials: a cautionary note. Journal of Applied Research in Memory and Cognition, 3 , 214–221.
Young, C. (2016). Mini-tests. Retrieved from https://colleenyoung.wordpress.com/revision-activities/mini-tests/ . Accessed 25 Dec 2017.
Download references
Acknowledgements
Not applicable.
YW and MAS were partially supported by a grant from The IDEA Center.
Availability of data and materials
Author information, authors and affiliations.
Department of Psychology, University of Massachusetts Lowell, Lowell, MA, USA
Yana Weinstein
Department of Psychology, Boston College, Chestnut Hill, MA, USA
Christopher R. Madan
School of Psychology, University of Nottingham, Nottingham, UK
Department of Psychology, Rhode Island College, Providence, RI, USA
Megan A. Sumeracki
You can also search for this author in PubMed Google Scholar
Contributions
YW took the lead on writing the “Spaced practice”, “Interleaving”, and “Elaboration” sections. CRM took the lead on writing the “Concrete examples” and “Dual coding” sections. MAS took the lead on writing the “Retrieval practice” section. All authors edited each others’ sections. All authors were involved in the conception and writing of the manuscript. All authors gave approval of the final version.
Corresponding author
Correspondence to Yana Weinstein .
Ethics declarations
Ethics approval and consent to participate, consent for publication, competing interests.
YW and MAS run a blog, “The Learning Scientists Blog”, which is cited in the tutorial review. The blog does not make money. Free resources on the strategies described in this tutorial review are provided on the blog. Occasionally, YW and MAS are invited by schools/school districts to present research findings from cognitive psychology applied to education.
Publisher’s Note
Springer Nature remains neutral with regard to jurisdictional claims in published maps and institutional affiliations.
Rights and permissions
Open Access This article is distributed under the terms of the Creative Commons Attribution 4.0 International License ( http://creativecommons.org/licenses/by/4.0/ ), which permits unrestricted use, distribution, and reproduction in any medium, provided you give appropriate credit to the original author(s) and the source, provide a link to the Creative Commons license, and indicate if changes were made.
Reprints and permissions
About this article
Cite this article.
Weinstein, Y., Madan, C.R. & Sumeracki, M.A. Teaching the science of learning. Cogn. Research 3 , 2 (2018). https://doi.org/10.1186/s41235-017-0087-y
Download citation
Received : 20 December 2016
Accepted : 02 December 2017
Published : 24 January 2018
DOI : https://doi.org/10.1186/s41235-017-0087-y
Share this article
Anyone you share the following link with will be able to read this content:
Sorry, a shareable link is not currently available for this article.
Provided by the Springer Nature SharedIt content-sharing initiative
September 20, 2013
Is Teaching to a Student’s “Learning Style” a Bogus Idea?
Many researchers have suggested that differences in students’ learning styles may be as important as ability, but empirical evidence is thin
By Sophie Guterl
Ken Gibson was an advanced reader in elementary school and easily retained much of what he read. But when the teacher would stand him up in front of the class to read a report out loud, he floundered. His classmates, he noticed, also had their inconsistencies. Some relished oral presentations but took forever to read a passage on their own; others had a hard time following lectures. Gibson now explains these discrepancies as “learning styles” that differ from one student to the next. He founded a company, LearningRx, on the premise that these styles make a difference in how students learn.
The idea that learning styles vary among students has taken off in recent years. Many teachers, parents and students are adamant that they learn best visually or by hearing a lesson or by reading, and so forth. And some educators have advocated teaching methods that take advantage of differences in the way students learn. But some psychologists take issue with the idea that learning style makes any significant difference in the classroom.
There is no shortage of ideas in the professional literature. David Kolb of Case Western Reserve University posits that personality divides learners into categories based on how actively or observationally they learn and whether they thrive on abstract concepts or concrete ones. Another conjecture holds that sequential learners understand information best when it is presented one step at a time whereas holistic learners benefit more from seeing the big picture. Psychologists have published at least 71 different hypotheseson learning styles.
On supporting science journalism
If you're enjoying this article, consider supporting our award-winning journalism by subscribing . By purchasing a subscription you are helping to ensure the future of impactful stories about the discoveries and ideas shaping our world today.
Frank Coffield, professor of education at the University of London, set out to find commonalities among the many disparate ideas about learning style using a sample comprising 13 models. The findings, published in 2004, found that only three tests for learning styles met their criteria for both validity and reliability, meaning that the tests both measured what they intended to measure and yielded consistent results. Among the many competing ideas, Coffield and his colleagues found no sign pointing to an overarching model of learning styles.
In 2002 Gibson, after a brief career as a pediatric optometrist, started LearningRx, a nontraditional tutoring organization, based on the idea that different people rely on particular cognitive skills that are strongest. For instance, visual learners understand lessons best when they are presented via images or a slide show; auditory learners benefit more from lectures; kinesthetic learners prefer something concrete, such as building a diorama. “We have a natural tendency to use the skills that are strongest,” Gibson says. “That becomes our learning style.”
LearningRx trainers use cognitive skill assessments similar to IQ tests to identify a student’s areas of cognitive strengths and weaknesses—some people might be strong at memorizing written words or weak at doing mathematical computations in their heads. Then they administer “brain training” exercises designed to improve students' weakest skills. Such exercises might involve a trainer asking a student to quickly answer a series of math problems in his head.
Daniel Willingham, a professor of cognitive psychology at the University of Virginia and outspoken skeptic of learning styles, argues that Gibson and other cognitive psychologists are mistaken to equate cognitive strengths with learning styles. The two, Willingham says, are different: Whereas cognitive ability clearly affects the ability to learn, an individual’s style doesn’t. “You can have two basketball players, for example, with a different style. One is very conservative whereas the other is a real risk-taker and likes to take crazy shots and so forth, but they might be equivalent in ability.”
As Willingham points out, the idea that ability affects performance in the classroom is not particularly surprising. The more interesting question is whether learning styles, as opposed to abilities, make a difference in the classroom. Would educators be more effective if they identified their students’ individual styles and catered their lessons to them?
The premise should be testable, Willingham says. “The prediction is really straightforward: If you appeal to a person’s style versus going against his preferred style, that should make a difference for learning outcomes,” he says.
Harold Pashler of the University of California, San Diego, and his colleagues searched the research literature for exactly this kind of empirical evidence. They couldn't find any. One study they reviewed compared participants’ scores on the Verbalizer–Visualizer Questionnaire, a fifteen-item survey of true-or-false questions evaluating whether someone prefers auditory or optical information, with their scores on memory tests after presenting words via either pictures or verbal reading. On average, participants performed better on the free-recall test when they were shown images, regardless of their preferences.
Some studies claimed to have demonstrated the effectiveness of teaching to learning styles, although they had small sample sizes, selectively reported data or were methodologically flawed. Those that were methodologically sound found no relationship between learning styles and performance on assessments. Willingham and Pashler believe that learning styles is a myth perpetuated merely by sloppy research and confirmation bias.
Despite the lack of empirical evidence for learning styles, Gibson continues to think of ability and preference as being one and the same. Trainers at LearningRx ask their clients to describe their weaknesses, then measure their cognitive abilities using the Woodcock–Johnson Test . “Just by someone telling us what’s easy and hard for them, we can pretty well know where the deficiencies are,” he says. “Eighty-five to 90 percent of the time the symptoms and the test results are right on.”
When teachers wonder how to present a lesson to kids with a range of abilities, they may not find the answer in established learning style approaches. Instead, Willingham suggests keeping it simple. “It’s the material, not the differences among the students, that ought to be the determinant of how the teacher is going to present a lesson," he says. For example, if the goal is to teach students the geography of South America, the most effective way to do so across the board would be by looking at a map instead of verbally describing the shape and relative location of each country. “If there’s one terrific way that captures a concept for almost everybody, then you’re done.”
MINI REVIEW article
The modality-specific learning style hypothesis: a mini-review.
- 1 Department of Psychology, Faculty of Social and Educational Sciences, Norwegian University of Science and Technology, Trondheim, Norway
- 2 Department of Neuromedicine and Movement Science, Faculty of Medicine and Health Sciences, Norwegian University of Science and Technology, Trondheim, Norway
The impact on learning outcome of tailoring instruction and teaching toward modality-specific learning style preferences has been researched and debated for decades. Several topical reviews have concluded that there is no evidence to support the meshing hypothesis and that it represents a persistent neuromyth in education. The concept, however, is still utilized in educational practice and favored by many academics. This mini-review presents literature, which has applied explicit and rigorous methodological criteria, in relation to the meshing hypothesis. In order to demonstrate evidence for the meshing hypothesis, studies had to screen participants for their preferred learning style, assign participants to matched or non-matched conditions, and then provide the same test to assess learning for all participants, as well as presenting statistical crossover-interaction effects. Across studies that have applied these methodological criteria, the overall effect sizes were very low and non-significant, indicating that there is still no replicable statistical evidence for enhanced learning outcome by aligning instruction to modality-specific learning styles.
Introduction
The concept of matching instructional strategies to an individual’s learning style in order to enhance learning outcome and achieve better academic success is a well-known concept among educators and the general population ( Pashler et al., 2008 ; Dekker et al., 2012 ; Howard-Jones, 2014 ). Learning styles are considered to have an impact in any learning situation regardless of content and this “refers to the concept that individuals differ in regard to what mode of instruction or study is most effective to them” ( Pashler et al., 2008 ). The term learning styles first appeared in the literature many decades ago (e.g., Thelen, 1954 ) and has been the focus of extensive research for the past three decades, especially in Western Europe and the United States ( Coffield et al., 2004 ).
Amongst a plethora of concepts and perspectives on learning styles (see Coffield et al., 2004 , for a tour de force on learning style concepts), one of the most cited and well-known learning style perspectives concerns modality-specific preferences ( Coffield et al., 2004 ; Howard-Jones, 2014 ; Cuevas, 2015 ). The overall prediction is that if individuals are given instruction in their preferred modality (visual, auditory, or kinesthetic), they will experience enhanced learning outcomes. This has been termed the meshing hypothesis ( Pashler et al., 2008 ). A related perspective that offers basically the same prediction states that people who are “verbalizers” will perform better if they are given verbal instructions and that “visualizers” will perform better if instructions are presented visually ( Massa and Mayer, 2006 ; Kollöffel, 2012 ). In either perspective, the instructional method should mesh with the preferred modality-specific learning style. The learning style concept, in general, and the meshing hypothesis, in particular, have been subjects of tremendous scrutiny in the recent years that continues to the present. Several independent authors have advanced the view that the latter represents a neuromyth , a term applied to educational applications argued to be based upon popular perspectives of brain functioning ( Geake, 2008 ; Riener and Willingham, 2010 ; Dekker et al., 2012 ; Howard-Jones, 2014 ; Newton, 2015 ; Newton and Miah, 2017 ). Typically, the evidence for neuromyths does not correspond to the findings of studies from cognitive psychology and the neurosciences, and sometimes the scientific evidence contradicts the brain-based claims ( Geake, 2008 ). In terms of the meshing hypothesis, the implicit assumption is that the learning material delivered via one sensory modality (i.e., visual, auditory, or kinesthetic) is processed in the brain independently from material delivered via other sensory modalities. However, substantial scientific evidence shows support for cross-modal processing and interconnectivity that contradicts the meshing perspective and demonstrates that input modalities in the brain are always interlinked ( Calvert et al., 2000 ).
The overall claim for improving learning by matching the mode of instruction to modality-specific learning preferences independent of both ability and content ( Riener and Willingham, 2010 ), as reflected by the meshing hypothesis, has also been scrutinized in several literature reviews. At first sight, modality-specific instruction appears to be supported by a large body of empirical literature ( Rohrer and Pashler, 2012 ). However, upon closer inspection, few of these studies have been found to have an appropriate research design ( Pashler et al., 2008 ). First, subjects need to be divided according to their preferred learning style, e.g., visual or auditory learners, based upon some sort of learning style assessment. Second, studies with an appropriate design must then randomize subjects (regardless of their assessed learning style) to receive either instruction tailored to their style or instruction tailored for other learning styles. This asserts that some subjects were presented with the “correct” kind of instruction (i.e., aligned with their preferred learning modality) and some with the “incorrect” instruction. Finally, all participants must be administered the same test to assess learning, and the results would support the efficacy of the practice of aligning instruction with modality-specific learning style if, and only if, the test scores reveal that, e.g., visual learners do better if instruction is presented visually rather than auditorily, and likewise, auditory learners do better if instruction is presented auditorily rather than visually (crossover-interaction effects; Pashler et al., 2008 ). In previous reviews, it has been stated repeatedly that there is a lack of studies that employ this rigorous design and that the few available at the time have, overall, generated no evidence to support the meshing hypothesis ( Coffield et al., 2004 ; Kozhevnikov, 2007 ; Pashler et al., 2008 ; Willingham et al., 2015 ).
The disappointing outcome of all these empirical and theoretical endeavors and efforts is that the modality-specific learning style concept is, as stated by Newton (2015) , thriving across all levels of education. This is reflected in the findings of 89% of research papers published from 2013 to 2015 and located in ERIC and PubMed databases support the application of learning styles to instructional methodology ( Newton, 2015 ). Furthermore, a survey by Dekker et al. (2012) showed that 93% of United Kingdom primary and secondary school teachers assumed that “individuals learn better when they receive information in their preferred learning style.” Later studies have revealed similar findings in other countries, K-12 teachers responding positively to statements favoring modality-specific learning styles ( Howard-Jones, 2014 ; Gleichgerrcht et al., 2015 ; Ferrero et al., 2016 ). In addition, when faculty working in higher education in the United States were given the following question: Does teaching to a student’s learning style enhance learning? , approximately two-thirds answered in the affirmative ( Dandy and Bendersky, 2014 ). At the institutional level, Meyer and Murrell (2014) found that, across 39 educational institutions in the United States, more than 70% taught “learning style theory” as a topic in teacher education.
A recent study showed a downward trend for the general belief in learning styles among academics working in higher education in the United Kingdom ( n = 114), although 58% still report believing in the concept and about a third report using learning styles actively in their work ( Newton and Miah, 2017 ). Thus, there appears to be widespread acceptance among educators, students, and academics globally and across all levels of education that the concept of learning styles is an established, textbook principle. Indeed, texts used in teacher education courses present learning style theory as a way to differentiate instruction for students ( Cuevas, 2015 ).
The presented considerations demonstrate that there exists a substantial continuum of perspectives on the application of modality-specific learning styles, ranging from viewing the concept as a neuromyth that should be abandoned in pedagogical practice to those who speak in favor of the concept and might use it as part of their routine practices. The principal aim of this mini-review is to provide a contribution toward narrowing this gap in perspectives by providing an updated overview of the available empirical studies that have applied rigorous methodological criteria as outlined by Pashler et al. (2008) . To the best of the authors’ knowledge, although previous reviews touching upon modality-specific learning styles have been both thorough and in-depth, they have been mostly narrative and have not been accompanied by a focus on specific effect sizes. This latter approach can be important for disentangling divergences in results, as there might be disagreements among studies. Pooling methodological and conceptually similar studies that all involve a certain degree of error allows for deriving an estimate of overall effect size that considers contrasting results from different studies. Such an update seems timely, given that several studies with methodological rigor have been published since the previous reviews.
Scope of the Mini-Review: Selection Criteria for Reporting of Evidence
The aim of this mini-review was to present literature in relation to the meshing hypothesis. Consequently, the authors independently performed database searches in EBSCO (including ERIC, Academic Search Complete, Psychology and Behavioral Sciences Collection) and Ovid (including Medline, EMBASE, and PsychINFO) using combinations of the terms learning styles ∗ , visual ∗ , and auditory ∗ . The searches were conducted up to January 2018. The reference lists from previous reviews were also examined, as well as citation-based searches in Google Scholar. A total of 1215 records were initially scanned, and 10 studies ( Constantinidou and Baker, 2002 ; Massa and Mayer, 2006 ; Kassaian, 2007 ; Korenman and Peynircioglu, 2007 ; Slack and Norwich, 2007 ; Tight, 2010 ; Kollöffel, 2012 ; Hansen and Cottrell, 2013 ; Rogowsky et al., 2015 ; Papanagnou et al., 2016 ) were found that had applied the appropriate methodology according to the criteria by Pashler et al. (2008) .

Tailoring Instruction for Modality-Specific Preferences: No Statistical Evidence for the Meshing Hypothesis
Statistical evidence for the meshing hypothesis could potentially be found in crossover-interaction effects, i.e., visual learners demonstrate improved learning if instruction is visual rather than auditory, and likewise, auditory learners show improvements if instruction is auditory rather than visual. The 10 publications amounted to 13 experiments, from which it was possible to extract means (SD) for computation of effect sizes (Hedges’ g ) for 11 of them. Altogether, 22 effect sizes from post-test data representing the differences in scores between the matched groups and the mismatched groups were analyzed by a random effects model. This resulted in a small and non-significant effect size for visual matching ( g = -0.09, 95% CI [-0.74–0.58], p = 0.80, n = 484) as well as for auditory matching ( g = -0.27, 95% CI [-0.87–0.32], p = 0.37, n = 356). In the paper by Constantinidou and Baker (2002) , the authors did not report data that allow for the computation of Hedges’ g . The authors did state, however, that no significant correlation between learning style and experimental task performance was found. Similarly, Papanagnou et al. (2016) reported only mean values for matched/non-matched learning outcomes and stated that both matched and non-matched groups achieved similar learning outcomes. Based on these data, it thus appears that there is no replicable evidence for a statistical crossover-interaction effect where participants systematically show higher learning outcomes when they are in a condition in which their preferred learning style modality matches the instructional mode and a lower learning outcome when there is a mismatch.
The overall (non-significant) effect sizes obtained across studies appears to be, by any standard, too small to be interpreted as signifying any modality-matching effect on learning outcomes. Although the interpretation of effect sizes is not a straightforward scientific endeavor ( Cohen, 1992 ), the effect size cut-offs indicating a practically relevant effect provided in the literature represent a much more substantial magnitude. For example, Ferguson (2009) recommended that a minimum effect size representing a “practically” significant effect amounts to g ≥ 0.41, and Hattie (2009) has advanced the view that effect sizes ≥0.40 represent a “hinge-point” at which deliberate interventions provide relevant outcomes for teaching and learning. Adding to the overall interpretation of the effect sizes obtained in the current meta-analysis, the 95% confidence intervals demonstrate crossings of zero both for the overall effect size and in data from some individual studies. This latter finding is a strong indicator that the null hypothesis (no effect of modality matching) should not be rejected ( Wilkinson et al., 1999 ).
An often-stated problem in the learning style literature is the plethora of inventories designed and applied for both research and commercial purposes ( Coffield et al., 2004 ; Peterson et al., 2009 ; Scott, 2010 ; Armstrong et al., 2012 ). At first sight, this might appear as a methodological challenge toward the pooling of results across studies. In particular, the VAKT classification vs. the verbalizer–visualizer dimension have previously been advocated as different and non-comparable approaches toward learning styles; e.g., it has been claimed that the verbalizer–visualizer dimension should be defined as a cognitive style and not included among the “family” of learning styles ( Massa and Mayer, 2006 ; Kollöffel, 2012 ). However, the latter perspective involves modality-specific content. Written material is considered proper instruction for verbalizers, as it is processed as spoken words, and therefore, a verbalizer can be considered synonymous to an auditory learner ( Felder and Silverman, 1988 ). Based on these contentions, there are strong theoretical arguments for a comparison of studies applying inventories based upon either perspective. Furthermore, rarely is any theoretical or methodological argument for the inclusion of a specific inventory in studies given, and in addition, some authors advance the view that one should apply the inventories that are most used (or most popular) in order to generate comparable results ( Hansen and Cottrell, 2013 ).
There still appear to be relatively few studies adhering strictly to the methodological criteria outlined by Pashler et al. (2008) . In particular, the participants’ learning styles are not necessarily established before they are separated into groups (e.g., Korenman and Peynircioglu, 2007 ), and participants can be randomly assigned to either one ( Massa and Mayer, 2006 ; Rogowsky et al., 2015 ) or all conditions (e.g., Kassaian, 2007 ). The only study located through the systematic literature search across six different databases and the screening of more than a 1000 records that was totally aligned with Pashler’s criteria was Rogowsky et al. (2015) . These authors report no statistically significant relationship or crossover-interaction effect between modality-specific learning styles and modes of instruction. Here, the authors assessed the participants’ learning styles and randomly assigned participants to either listening to a digital audiobook or reading an e-text, and all participants completed the same achievement test. Interestingly, the effect sizes from this latter study (visual-matching: g = -0.11, auditory-matching: g = -0.256) were similar to the overall effect size across studies.
The experimental tasks applied in studies varied considerably. The pooling of such various approaches can be justified by the modality-specific learning style theory. Here, the basic contention is that modality matching introduces more efficient learning irrespective of content and contexts. Indeed, the concept of a modality-specific learning style has been featured in the literature as a hardwired and more or less inherited preference in the cognitive system that should be taken into consideration in any learning situation ( Coffield et al., 2004 ). One methodological concern, however, arises when examining learning tasks more closely. It appears that some tasks have a “built-in” stronger visual or auditory component, which could potentially introduce an additive bias in favor of both a particular instructional mode and a learning style ( Fiorina et al., 2007 ; Hansen and Cottrell, 2013 ; Willingham et al., 2015 ). Although this could potentially lead to inflated effect sizes, the overall pattern of results across studies suggested no statistical effect of modality matching.
As stated in the introduction, the modality-specific learning style hypothesis is still a favored concept amongst the general public, educators, and in the research literature ( Pashler et al., 2008 ; Dekker et al., 2012 ; Howard-Jones, 2014 ). In previous reviews, it has been systematically addressed that there is, in general, no evidence to support the application of the learning style concept ( Coffield et al., 2004 ; Desmedt and Valcke, 2004 ; Kozhevnikov, 2007 ; Pashler et al., 2008 ; Peterson et al., 2009 ; Cuevas, 2015 ; Willingham et al., 2015 ). The present study responds to a call from the much-cited review (>1,500 citations in Google Scholar) of Pashler et al. (2008) , who stated that, in order for the learning styles hypothesis to be supported, several well-designed studies would have to test, amongst other elements, the modality-matching hypothesis and show significant interaction effects. Although the total number of studies ( n = 10) with appropriate methodology is not large at this time, the pattern of results clearly leans toward showing that tailoring instruction/teaching toward preferred modality-specific learning styles has no effect on learning outcome/rate.
Concluding Remarks
This mini-review has demonstrated that, across studies that have applied equivalent quantitative empirical research designs, no overall improvement in learning outcome when applying modality-specific matching of instruction was found. This conclusion of the presented meta-analysis of an element of the modality-specific learning style literature appears to add to further evidence-based refutations of the meshing hypothesis. Interestingly, some early meta-analysis on other elements of learning styles presented similar conclusions ( Tamir, 1985 ; Kavale and Forness, 1987 ). This appears in contrast to the recent literature review by Newton (2015) , in which it was demonstrated that a considerable percent (89%) of published studies in the period from 2013 to 2015 was positive toward learning styles. It thus appears important to continue to critically scrutinize different aspects of the learning style literature and to conduct pattern-type explanations ( Derry, 1999 ) involving conceptual syntheses of insights emerging from diverse disciplines. For example, connections have been found between visual-spatial strengths and superior abilities in other cognitive domains ( O’Boyle et al., 2005 ; Root-Bernstein et al., 2008 ). This latter work is not typically connected with modality-specific learning styles in the academic literature and highlights the need for further work on the credibility of the meshing hypothesis in order to prevent potential misuse of what might appear to be a persistent neuromyth.
Author Contributions
All authors listed have made a substantial, direct and intellectual contribution to the work, and approved it for publication.
Conflict of Interest Statement
The authors declare that the research was conducted in the absence of any commercial or financial relationships that could be construed as a potential conflict of interest.
Armstrong, S. J., Peterson, E. R., and Rayner, S. G. (2012). Understanding and defining cognitive style and learning style: a Delphi study in the context of educational psychology. Educ. Stud. 38, 449–455. doi: 10.1080/03055698.2011.643110
CrossRef Full Text | Google Scholar
Calvert, G. A., Campbell, R., and Brammer, M. J. (2000). Evidence from functional magnetic resonance imaging of crossmodal binding in human heteromodal cortex. Curr. Biol. 10, 649–657. doi: 10.1016/S0960-9822(00)00513-3
Coffield, F., Moseley, D., Hall, E., and Ecclestone, K. (2004). Learning Styles and Pedagogy in Post-16 Learning: A Systematic and Critical Review. Available at: http://www.hull.ac.uk/php/edskas/learning
Google Scholar
Cohen, J. (1992). A power primer. Psychol. Bull. 112, 155–159. doi: 10.1037/0033-2909.112.1.155
Constantinidou, F., and Baker, S. (2002). Stimulus modality and verbal learning performance in normal aging. Brain Lang. 82, 296–311. doi: 10.1016/S0093-934X(02)00018-4
Cuevas, J. (2015). Is learning styles-based instruction effective? A comprehensive analysis of recent research on learning styles. Theory Res. Educ. 13, 308–333. doi: 10.1177/1477878515606621
Dandy, K., and Bendersky, K. (2014). Student and faculty beliefs about learning in higher education: implications for teaching. Int. J. Teach. Learn. High. Educ. 26, 358–380.
Dekker, S., Lee, N. C., Howard-Jones, P., and Jolles, J. (2012). Neuromyths in education: prevalence and predictors of misconceptions among teachers. Front. Psychol. 3:429. doi: 10.3389/fpsyg.2012.00429
PubMed Abstract | CrossRef Full Text | Google Scholar
Derry, G. N. (1999). What Science is and How it Works. Princeton, NJ: Princeton University Press.
Desmedt, E., and Valcke, M. (2004). Mapping the learning styles “jungle”: an overview of the literature based on citation analysis. Educ. Psychol. 24, 445–464. doi: 10.1080/0144341042000228843
Felder, R. M., and Silverman, L. K. (1988). Learning and teaching styles in engineering education. Eng. Educ. 78, 674–681.
Ferguson, C. J. (2009). An effect size primer: a guide for clinicians and researchers. Prof. Psychol. Res. Pr. 40, 532–538. doi: 10.1037/a0015808
Ferrero, M., Garaizar, P., and Vadillo, M. A. (2016). Neuromyths in education: prevalence among Spanish teachers and an exploration of cross-cultural variation. Front. Hum. Neurosci. 10:496. doi: 10.3389/fnhum.2016.00496
Fiorina, L., Antonietti, A., Colombo, B., and Bartolomeo, A. (2007). Thinking style, browsing primes and hypermedia navigation. Comput. Educ. 49, 916–941. doi: 10.1016/j.compedu.2005.12.005
Geake, J. (2008). Neuromythologies in Education. Educ. Res. 50, 123–133. doi: 10.1080/00131880802082518
Gleichgerrcht, E., Luttges, B. L., Salvarezza, F., and Campos, A. L. (2015). Educational neuromyths among teachers in Latin America. Mind Brain Educ. 9, 170–178. doi: 10.1111/mbe.12086
Hansen, L., and Cottrell, D. (2013). An evaluation of modality preference using a “Morse code” recall task. J. Exp. Educ. 81, 123–137. doi: 10.1080/00220973.2012.678408
Hattie, J. (2009). Visible Learning: A Synthesis of Over 800 Meta-Analyses Relating to Achievement. Abingdon: Routledge.
Howard-Jones, P. A. (2014). Neuroscience and education: myths and messages. Nat. Rev. Neurosci. 15, 817–824. doi: 10.1038/nrn3817
Kassaian, Z. (2007). Learning styles and lexical presentation modes. Estud. Lingüíst. Inglesa Apl. 7, 53–78.
Kavale, K. A., and Forness, S. R. (1987). Substance over style: assessing the efficacy of modality testing and teaching. Except. Child. 54, 228–239. doi: 10.1177/001440298705400305
Kollöffel, B. (2012). Exploring the relation between visualizer–verbalizer cognitive styles and performance with visual or verbal learning material. Comput. Educ. 58, 697–706. doi: 10.1016/j.compedu.2011.09.016
Korenman, L. M., and Peynircioglu, Z. F. (2007). Individual differences in learning and remembering music: auditory versus visual presentation. J. Res. Music Educ. 55:48. doi: 10.1177/002242940705500105
Kozhevnikov, M. (2007). Cognitive styles in the context of modern psychology: toward an integrated framework of cognitive style. Psychol. Bull. 133, 464–481. doi: 10.1037/0033-2909.133.3.464
Massa, L. J., and Mayer, R. E. (2006). Testing the ATI hypothesis: should multimedia instruction accommodate verbalizer-visualizer cognitive style? Learn. Individ. Dif. 16, 321–335. doi: 10.1016/j.lindif.2006.10.001
Meyer, K. A., and Murrell, V. S. (2014). A national study of theories and their importance for faculty development for online teaching. J. Distance Learn. Adm. Contents 17, 1–15. doi: 10.1111/j.1365-2923.2012.04350.x
Newton, P. M. (2015). The learning styles myth is thriving in higher education. Front. Psychol. 6:1908. doi: 10.3389/fpsyg.2015.01908
Newton, P. M., and Miah, M. (2017). Evidence-based higher education-Is the learning styles ‘myth’ important? Front. Psychol. 8:444. doi: 10.3389/fpsyg.2017.00444
O’Boyle, M. W., Cunnington, R., Silk, T. J., Vaughan, D., Jackson, G., Syngeniotis, A., et al. (2005). Mathematically gifted male adolescents activate a unique brain network during mental rotation. Cogn. Brain Res. 25, 583–587. doi: 10.1016/j.cogbrainres.2005.08.004
Papanagnou, D., Serrano, A., Barkley, K., Chandra, S., Governatori, N., Piela, N., et al. (2016). Does tailoring instructional style to a medical student’s self-perceived learning style improve performance when teaching intravenous catheter placement? A randomized controlled study. BMC Med. Educ. 16:205. doi: 10.1186/s12909-016-0720-3
Pashler, H., McDaniel, M., Rohrer, D., and Bjork, R. (2008). Learning styles concepts and evidence. Psychol. Sci. Public Interest 9, 105–119. doi: 10.1111/j.1539-6053.2009.01038.x
Peterson, E. R., Rayner, S. G., and Armstrong, S. J. (2009). Researching the psychology of cognitive style and learning style: is there really a future? Learn. Individ. Dif. 19, 518–523. doi: 10.1016/j.lindif.2009.06.003
Riener, C., and Willingham, D. (2010). The myth of learning styles. Change 42, 32–35. doi: 10.1080/00091383.2010.503139
Rogowsky, B. A., Calhoun, B. M., and Tallal, P. (2015). Matching learning style to instructional method: effects on comprehension. J. Educ. Psychol. 107, 64–78. doi: 10.1037/a0037478
Rohrer, D., and Pashler, H. (2012). Learning styles: where’s the evidence? Med. Educ. 46, 634–635.
Root-Bernstein, R., Allen, L., Beach, L., Bhadula, R., Fast, J., Hosey, C., et al. (2008). Arts foster scientific success: avocations of nobel, national academy, royal society, and sigma xi members. J. Psychol. Sci. Technol. 1, 51–63. doi: 10.1891/1939-7054.1.2.51
Scott, C. (2010). The enduring appeal of ‘learning styles’. Aust. J. Educ. 54, 5–17. doi: 10.1177/000494411005400102
Slack, N., and Norwich, B. (2007). Evaluating the reliability and validity of a learning styles inventory: a classroom-based study. Educ. Res. 49, 51–63. doi: 10.1080/00131880701200765
Tamir, P. (1985). Meta-analysis of cognitive preferences and learning. J. Res. Sci. Teach. 22, 1–17. doi: 10.1002/tea.3660220101
Thelen, H. A. (1954). Dynamics of Groups at Work. Chicago, IL: Univeristy of Chicago Press.
Tight, D. G. (2010). Perceptual learning style matching and L2 vocabulary acquisition. Lang. Learn. 60, 792–833. doi: 10.1111/j.1467-9922.2010.00572.x
Wilkinson, L., Task Force on Statistical Inference, American Psychological Association, and Science Directorate (1999). Statistical methods in psychology journals: guidelines and explanations. Am. Psychol. 54, 594–604. doi: 10.1037/0003-066X.54.8.594
Willingham, D. T., Hughes, E. M., and Dobolyi, D. G. (2015). The scientific status of learning styles theories. Teach. Psychol. 42, 266–271. doi: 10.1007/s10459-009-9202-2
Keywords : modality-specific, instruction, teaching, learning styles, meshing hypothesis, neuromyth
Citation: Aslaksen K and Lorås H (2018) The Modality-Specific Learning Style Hypothesis: A Mini-Review. Front. Psychol. 9:1538. doi: 10.3389/fpsyg.2018.01538
Received: 17 April 2018; Accepted: 02 August 2018; Published: 21 August 2018.
Reviewed by:
Copyright © 2018 Aslaksen and Lorås. This is an open-access article distributed under the terms of the Creative Commons Attribution License (CC BY) . The use, distribution or reproduction in other forums is permitted, provided the original author(s) and the copyright owner(s) are credited and that the original publication in this journal is cited, in accordance with accepted academic practice. No use, distribution or reproduction is permitted which does not comply with these terms.
*Correspondence: Håvard Lorås, [email protected]
Disclaimer: All claims expressed in this article are solely those of the authors and do not necessarily represent those of their affiliated organizations, or those of the publisher, the editors and the reviewers. Any product that may be evaluated in this article or claim that may be made by its manufacturer is not guaranteed or endorsed by the publisher.

An official website of the United States government
The .gov means it’s official. Federal government websites often end in .gov or .mil. Before sharing sensitive information, make sure you’re on a federal government site.
The site is secure. The https:// ensures that you are connecting to the official website and that any information you provide is encrypted and transmitted securely.
- Publications
- Account settings
Preview improvements coming to the PMC website in October 2024. Learn More or Try it out now .
- Advanced Search
- Journal List
- Front Psychol
Evidence-Based Higher Education – Is the Learning Styles ‘Myth’ Important?
Associated data.
The basic idea behind the use of ‘Learning Styles’ is that learners can be categorized into one or more ‘styles’ (e.g., Visual, Auditory, Converger) and that teaching students according to their style will result in improved learning. This idea has been repeatedly tested and there is currently no evidence to support it. Despite this, belief in the use of Learning Styles appears to be widespread amongst schoolteachers and persists in the research literature. This mismatch between evidence and practice has provoked controversy, and some have labeled Learning Styles a ‘myth.’ In this study, we used a survey of academics in UK Higher Education ( n = 114) to try and go beyond the controversy by quantifying belief and, crucially, actual use of Learning Styles. We also attempted to understand how academics view the potential harms associated with the use of Learning Styles. We found that general belief in the use of Learning Styles was high (58%), but lower than in similar previous studies, continuing an overall downward trend in recent years. Critically the percentage of respondents who reported actually using Learning Styles (33%) was much lower than those who reported believing in their use. Far more reported using a number of techniques that are demonstrably evidence-based. Academics agreed with all the posited weaknesses and harms of Learning Styles theory, agreeing most strongly that the basic theory of Learning Styles is conceptually flawed. However, a substantial number of participants (32%) stated that they would continue to use Learning Styles despite being presented with the lack of an evidence base to support them, suggesting that ‘debunking’ Learning Styles may not be effective. We argue that the interests of all may be better served by promoting evidence-based approaches to Higher Education.
Introduction
The use of so-called ‘Learning Styles’ in education has caused controversy. The basis for the use of Learning Styles is that individual difference between learners can supposedly be captured by diagnostic instruments which classify learners into ‘styles’ such as ‘visual,’ ‘kinaesthetic,’ ‘assimilator,’ etc. According to many, but not all, interpretations of Learning Styles theory, to teach individuals using methods which are matched to their ‘Learning Style’ will result in improved learning ( Pashler et al., 2008 ). This interpretation is fairly straightforward to test, and, although there are over 70 different instruments for classifying Learning Styles ( Coffield et al., 2004 ) the current status of the literature is that there is no evidence to support the use of Learning Styles in this way ( Pashler et al., 2008 ; Rohrer and Pashler, 2012 ). This has lead to Learning Styles being widely classified as a ‘myth’ ( Geake, 2008 ; Riener and Willingham, 2010 ; Lilienfeld et al., 2011 ; Dekker et al., 2012 ; Pasquinelli, 2012 ; Rato et al., 2013 ; Howard-Jones, 2014 ).
Despite this lack of evidence, it appears that belief in the use of Learning Styles is common amongst schoolteachers – A 2012 study demonstrated that 93% of schoolteachers in the UK agree with the statement “Individuals learn better when they receive information in their preferred Learning Style (e.g., auditory, visual, kinaesthetic) ( Dekker et al., 2012 ).” A 2014 survey reported that 76% of UK schoolteachers ‘used Learning Styles’ and most stated that to do so benefited their pupils in some way ( Simmonds, 2014 ). A study of Higher Education faculty in the USA showed that 64% agreed with the statement “Does teaching to a student’s learning style enhance learning?” ( Dandy and Bendersky, 2014 ). A recent study demonstrated that current research papers ‘about’ Learning Styles, in the higher education research literature, overwhelmingly endorsed their use despite the lack of evidence described above ( Newton, 2015 ). Most of this endorsement was implicit and most of the research did not actually test Learning Styles, rather proceeded on the assumption that their use was a ‘good thing.’ For example, researchers would ask a group of students to complete a Learning Styles questionnaire, and then make recommendations for curriculum reform based upon the results.
This mismatch between the empirical evidence and belief in Learning Styles, alongside the persistence of Learning Styles in the wider literature, has lead to tension and controversy. There have been numerous publications in the mainstream media attempting to explain the limitations of Learning Styles (e.g., Singal, 2015 ; Goldhill, 2016 ) and rebuttals from practitioners who believe that the theory of Learning Styles continues to offer something useful and/or that criticism of them is invalid (e.g., Black, 2016 ). Some of the original proponents of the concept have self-published their own defense of Learning Styles, e.g., ( Felder, 2010 ; Fleming, 2012 ).
The continued use of Learning Styles is, in theory, associated with a number of harms ( Pashler et al., 2008 ; Riener and Willingham, 2010 ; Dekker et al., 2012 ; Rohrer and Pashler, 2012 ; Dandy and Bendersky, 2014 ; Willingham et al., 2015 ). These include a ‘pigeonholing’ of learners according to invalid criteria, for example a ‘visual learner’ may be dissuaded from pursuing subjects which do not appear to match their diagnosed Learning Style (e.g., learning music), and/or may become overconfident in their ability to master subjects perceived as matching their Learning Style. Other proposed harms include wasting resources on an ineffective method, undermining the credibility of education research/practice and the creation of unrealistic expectations of teachers by students.
This study aimed at asking first whether academics in UK Higher Education also believe in Learning Styles. We then attempted to go beyond the controversy and ask whether academics actually use Learning Styles, and how seriously they rate the proposed harms associated with the use of Learning Styles, with the aim of understanding how best to address the persistence of Learning Styles in education. In addition, we compared belief in/use of Learning Styles to some educational techniques whose use is supported by good research evidence, to put the use of, and belief in, Learning Styles into context.
We found that belief in the use of Learning Styles was high (58% of participants), but that actual use of Learning Styles was much lower (33%) and lower than other techniques which are demonstrably effective. The most compelling weakness/harm associated with Learning Styles was a simple theoretical weakness; 90% of participants agreed that Learning Styles are conceptually flawed.
Materials and Methods
Data were collected using an online questionnaire distributed to Higher Education institutions in the UK. Ethical approval for the study was given by the local Research Ethics Committee at Swansea University with informed consent from all subjects.
Participants
The survey was distributed via email. Distribution was undertaken indirectly; emails were sent to individuals at eight different Higher Education institutions across the UK. Those persons were known to the corresponding author as colleagues in Higher Education but not through work related to Learning Styles. Those individuals were asked to send the survey on to internal email distribution lists of academics involved in Higher Education using the following invitation text (approved by the ethics committee) “You are invited to participate in a short anonymous survey about teaching methods in Higher Education. It will take approximately 10–15 min to complete. It is aimed at academics in Higher Education,” followed by a link to the survey which was entitled “Teaching Methods in Higher Education.” Thus the survey was not directly distributed by the authors and did not contain the phrase ‘Learning Styles’ anywhere in the title or introductory text. These strategies of indirect distribution, voluntary completion and deliberately not using the term ‘Learning Styles’ in the title were based upon similar strategies used in similar studies ( Dekker et al., 2012 ; Dandy and Bendersky, 2014 ) and were aimed at avoiding biasing and/or polarizing the participant pool, given the aforementioned controversy associated with the literature on Learning Styles. Although this inevitably results in a convenience sample (we do not know how many people the survey as sent to or how many responded), this was preferable to distributing a survey that was expressly about Learning Styles (which may have put off those who are already familiar with the concept). The survey remained open for 2 months (which included the end-of-year holiday period) and was closed once we had over 100 participants who had fully completed the survey, to ensure a sample size equivalent to similar studies ( Dekker et al., 2012 ; Dandy and Bendersky, 2014 ).
One hundred sixty-one participants started the survey, with 114 completing the survey up to the final (optional) question about demographics. This meant that 29% of participants did not complete, which is slightly better than the average dropout rate of 30% for online surveys ( Galesic, 2006 ). Question-by-question analysis revealed that the majority of these non-completers (79%) did not progress beyond the very first ranking question (ranking the effectiveness of teaching methods) and thus did not complete the majority of the survey, including answering those questions about Learning Styles. Participants had been teaching in Higher Education for an average of 11 years ( SD = 9.8). Participants were asked to self-report their academic discipline. Simple coding of these revealed that participants came from a wide variety of disciplines, including Life and Physical Sciences (26%), Arts, humanities and languages (24%), Healthcare professions (medicine, nursing, pharmacy, etc.) (16%), Social Sciences (10%), Business and Law (5%).
Materials and Procedure
The lack of an evidence base for Learning Styles has been described numerous times in the literature, and these papers have suggested that there may be harms associated with the use of Learning Styles ( Pashler et al., 2008 ; Riener and Willingham, 2010 ; Dekker et al., 2012 ; Rohrer and Pashler, 2012 ; Dandy and Bendersky, 2014 ; Willingham et al., 2015 ). We reviewed these publications to identify commonly posited harms. We then constructed a questionnaire using LimeSurvey TM . All the survey questions are available via the Supplementary Material. Key aspects of the structure and design are described below. The survey was piloted by five academics from Medical and Life Sciences, all of whom were aware of the lack of evidence regarding Learning Styles. They were asked to comment on general clarity and were specifically asked to comment on the section regarding the evidence for the use of Learning Styles and whether it would disengage participants (see below). Key concepts in the survey were addressed twice, from different approaches, so as to ensure the quality of data obtained.
Participants were first asked to confirm that they were academics in Higher Education. They were then asked about their use of five teaching methods, four of which are supported by research evidence [Worked Examples, Feedback, Microteaching and Peer Teaching ( Hattie, 2009 )] and Learning Styles. They were then asked to rank these methods by efficacy.
We then asked participants about their use of Learning Styles, both generally and the use of specific classifications (VARK, Kolb, Felder, Honey and Mumford). For each of these individual Learning Styles classifications we identified, in our question, the individual styles that result (e.g., active/reflective, etc., from Felder). Thus participants were fully oriented to what was meant by ‘Learning Styles’ before we went on to ask them about the efficacy of Learning Styles. To allow comparisons with existing literature, we used the same question as Dekker et al. (2012) “Rate your agreement with this statement ‘Individuals learn better when they receive information in their preferred Learning Style (e.g., auditory, visual, kinaesthetic).”’
We then explained to participants about the lack of an evidence base for the use of Learning Styles, including the work of Coffield et al. (2004) , Pashler et al. (2008) , Rohrer and Pashler (2012) , Willingham et al. (2015) . We explained the difference between learning preferences and Learning Style, and made it clear that there was specifically no evidence to support the ‘matching’ of teaching methods to individual Learning Styles. We explained that this fact may be surprising, and that participants would be free to enter any comments they had at the end of the survey. Those academics who piloted the initial survey were specifically asked to comment on this aspect of the survey to ensure that it was neutral and objective.
We then asked participants to rate their agreement with some of the proposed harms associated with the use of Learning Styles. Mixed into the questions about harms were some proposed reasons to use Learning Styles, regardless of the evidence. These questions were interspersed so as to avoid ‘acquiescence bias’ ( Sax et al., 2003 ). Agreement was measured on a 5-point Likert scale.
Finally, participants were asked for some basic demographic information and then offered the opportunity to provide free-text comments on the content of the survey.
Quantitative data were analyzed by non-parametric methods; specific tests are described in the results. Percentages of participants agreeing, or disagreeing, with a particular statement were calculated by collapsing the two relevant statements within the Likert scale (e.g., ‘Strongly Agree and Agree’ were collapsed into a single value). Qualitative data (free-text comments) were analyzed using a simple ground-up thematic analysis ( Braun and Clarke, 2006 ) to identify common themes. Both authors independently read and re-read the comments to identify their own common themes. The authors then met and discussed these, arriving at agreed common themes and quantifying the numbers of participants who had raised comments for each theme. Many participant comments were pertinent to more than one theme.
Belief vs. Use; Do Teachers in Higher Education Actually Use Learning Styles?
We addressed this question from two perspectives. Academics were asked to identify which teaching methods, from a list of 5, they had used in the last 12 months. Results are shown in Figure Figure1 1 . Thirty-three percent of participants reported having used Learning Styles in the last 12 months, but this was lower than the evidence-based techniques of formative assessment, worked examples, and peer teaching. Participants were then asked “have you ever administered a Learning Styles questionnaire to your students” and were given four specific examples along with the ‘styles’ identified by those examples. The examples chosen were those most commonly found in a recent study of the literature on Learning Styles ( Newton, 2015 ). Participants were also given the option to check ‘other’ and identify any other types of Learning Styles questionnaire that they might have used. 33.1% of participants had given their students any sort of Learning Styles Questionnaire, with the response for individual classifications being 18.5% (Honey and Mumford), 14.5% (Kolb), 12.9% (VARK), and 1.6% (Felder).

Use of various teaching methods in the last 12 months. Academics were asked which of the methods they had used in the last 12 months. Four of the methods were accompanied by a brief description: Formative Assessment (practice tests), Peer Teaching (students teaching each other), Learning Styles (matching teaching to student Learning Styles). Microteaching (peer review by educators using recorded teaching).
We subsequently asked two, more general, questions about Learning Styles. The first of these was the same as that used by Dekker et al. “Individuals learn better when they receive information in their preferred Learning Style (e.g., auditory, visual, kinaesthetic),” with which 58% agreed. The second was “I try to organize my teaching to accommodate different student Learning Styles (e.g., visual, kinaesthetic, assimilator/converger),” with which 64% of participants agreed. These data show a contrast between a general belief in the use of Learning Styles, which is much higher than actual use ( Figure Figure2 2 ).

Belief in use of Learning Styles. At different points throughout the survey, participants were asked to rate their agreement with the statements regarding their belief in, and their actual use of, Learning Styles. These questions were asked prior to informing participants about the lack of evidence for the use of Learning Styles. When asked if they believed in the use of Learning Styles 1,2 , approximately two thirds of participants agreed, whereas when asked specifically about actual use 3,4 , agreement dropped to one-third.
1 Rate your agreement with this statement: Individuals learn better when they receive information in their preferred Learning Style (Individuals learn better LS) .
2 Rate your agreement with the statement: I try to organize my teaching to accommodate different Learning Styles (Accomodate LS) .
3 Have you ever administered a Learning Styles questionnaire to your students? If so, please state which one (Given students a LSQ) .
4 Which of these teaching methods have you used in the last 12 months? (Used LS in year) .
Possible Harms Associated with the Use of Learning Styles
There was significant agreement with all the proposed difficulties associated with the use of Learning Styles, as shown in Figure Figure3 3 . However, compared to the other proposed harms, participants showed stronger agreement with the statement “The theory of Learning Styles is conceptually flawed” – it does not account for the complexity of ‘understanding.’ It is not possible to teach complex concepts such as mathematics or languages by presenting them in only one style. In addition, some information cannot be presented in a single style (e.g., teaching medical students to recognize heart sounds would be impossible using visual methods, whereas teaching them to recognize different skin rashes would be impossible using sounds). In this section of the survey we also included two questions that were not about proposed harms. Forty-six percent of participants agreed with the statement “Even though there is no ‘evidence base’ to support the use of Learning Styles, it is my experience that their use in my teaching benefits student learning,” while 70% agreed that “In my experience, students believe, rightly or wrongly, that they have a particular Learning Style.”

Participants were asked to rate their agreement with various difficulties that have been proposed to result from the use of Learning Styles. Participants agreed with all the proposed harms but there was a stronger agreement (compared to other options) with the idea that the use of Learning Styles is conceptually flawed. ∗ , significantly different from median of ‘3’ (1-sample Wilcoxon Signed Rank test). #, different from other statements (Kruskal–Wallis test).
Ranking of Proposed Harms
Having asked participants to rate their agreement (or not) with the various harms associated with the use of Learning Styles, we then asked participants to “Rank the aforementioned factors in terms of how compelling they are as reasons not to use Learning Styles” (1, most compelling, 6, least compelling) and to “only rank those factors which you agree with.” There is not universal agreement on the analysis of ranking data and so we analyzed these data in two simple, descriptive ways. The first was to determine how frequently each harm appeared as the top ranked reason. The second was to calculate a ranking score, such that the top ranked harm was scored 6, and the lowest ranked scored 1, and then to sum these across the participants. Both are shown in Table Table1 1 . Results from both methods were similar and agreed with the prior analysis ( Figure Figure3 3 ), with participants most concerned about the basic conceptual flaws associated with the use of Learning Styles, alongside a potential pigeonholing of learners into a particular style.
Ranking of proposed harms as compelling reasons not to use Learning Styles.
Ranking score | # Times top ranked | |
---|---|---|
Waste resources that could be used elsewhere | 302 (4) | 11 (4) |
Pigeonhole learners | 445 (2) | 34 (1) |
Understanding more complex than Learning Styles | 455 (1) | 33 (2) |
Profit motive of those selling Learning Styles instruments | 191 (6) | 7 (5=) |
Unrealistic expectations of teachers | 366 (3) | 14 (3) |
Credibility of education as a discipline | 257 (5) | 7 (5=) |
Continued Use of Learning Styles?
Toward the end of the questionnaire, we asked participants two question to determine whether the completion of the questionnaire had made any difference to their understanding of the evidence base for the use of Learning Styles. Participants were first asked to rate their agreement with the statement “Completing this questionnaire has helped me understand the lack of any evidence base to support the use of Learning Styles.” The 64% agreed while 9% disagreed and 27% neither agreed or disagreed.
Participants were then asked “In light of the information presented, rate your agreement with the following statement – ‘I plan to try and account for individual student Learning Styles in my teaching.”’ 31.6% agreed, 43.9% disagreed, and 23.6% neither agreed or disagreed. The results from this question were compared to those obtained before the evidence was presented, when participants were asked to rate their level of agreement with this statement “I try to organize my teaching to accommodate different student Learning Styles (e.g., visual, kinaesthetic, assimilator/converger).” The results, shown in Figure Figure4 4 , show a statistically significant difference in the two sets of responses suggesting that completion of the questionnaire improved participants understanding of the lack of an evidence base for the use of Learning Styles and thus they were unlikely to continue using them. However, almost one-third of participants still agreed with the statement; they intended to continue using Learning Styles.

The completion of the survey instrument associated with a change of participants views of Learning Styles. At the beginning of the study, participants were asked to rate their agreement with the statement “I try to organize my teaching to accommodate different student Learning Styles (e.g., visual, kinaesthetic, assimilator/converger),” and 64% agreed. At the end of the study, participants were asked “In light of the information presented, rate your agreement with the following statement – ‘I plan to try and account for individual student Learning Styles in my teaching,”’ and 32% agreed. ∗ , a Wilcoxon signed rank test revealed a statistically significant difference in the pattern of response ( P < 0.0001, W = -1977).
This then raised a series of interesting questions about why participants would persist in using Learning Styles despite having been presented with all the evidence showing that they are not effective (although participants were not specifically asked whether they would persist in the matching of instructional design to student Learning Style). The sample size here, although equivalent to previous studies, is modest and obviously the 32% are only a portion of that. Thus we were reluctant to undertake extensive post hoc analysis to identify relationships within the sample. However, in response to a reviewer’s suggestion we undertook a simple descriptive analysis of the profile of the 31.6% of participants who indicated that they would continue to account for Learning Styles and compare them to the 43.9% who said that they would not. When splitting the data into these two groups, we observed that almost all (94.4%) of those who said they would still use Learning Styles at the end of the survey had originally agreed with the question “I try to organize my teaching to accommodate different student Learning Styles (e.g., visual, kinaesthetic, assimilator/converger),” and no participants from that group had disagreed. In contrast, agreement was only 40% for the group that eventually said they would not use Learning Styles, while disagreement was 46%. A similar split was found for the question “Even though there is no ‘evidence base’ to support the use of Learning Styles, it is my experience that their use in my teaching benefits student learning”; for the group that would go on to say that they will still use Learning Styles, 89% agreed, while agreement was only 18% from the group that would go on to say they will not continue to use Learning Styles.
Educational Research Literature
Finally we asked participants to rate their agreement with the statement “my educational practice is informed by the education research literature.” Forty-eight percent of participants agreed with the statement. A Spearman Rank Correlation test revealed no correlation between responses on that question and on the ‘Dekker’ question “Individuals learn better when they receive information in their preferred Learning Style (e.g., auditory, visual, kinaesthetic)” r = 0.07508, P = 0.4.
Qualitative Comments
Forty-eight participants left free-text comments. The dominant common theme, raised by 23 participants was the need to use a variety of teaching methods in order to (for example) keep students engaged or to promote reflection. This theme was often stated in the context of ‘despite the evidence again showing a lack of effectiveness of Learning Styles.’ A related theme (13 participants) was that participants had a looser interpretation of ‘Learning Styles,’ for example that they referred simply to ‘styles of learning,’ while a second related theme from nine participants was they would still, despite the evidence, use Learning Styles and/or found them useful. Eight participants commented that they were aware of the lack of evidence base for the use of Learning Styles and eight participants also gave their own examples of why Learning Styles were conceptually flawed. Despite the careful piloting described above, a small number of participants (four) commented that the survey was biased against Learning Styles, while eight participants perceived some of the questions to be ‘leading.’ No specific ‘leading’ questions were identified but there was a substantial overlap between these two themes, with three of the comments about the survey being ‘biased against Learning Styles’ coming alongside, or as part of, a comment about questions being ‘leading,’ with an implied relationship between the two. An additional theme, from five participants, was thanks; for raising the issue and/or interesting content.
The first aim of this study was to determine how widespread belief in, and use of, Learning Styles is by academics in UK Higher Education. In a 2012 study, 93% of a sample of 137 UK school teachers agreed with the statement “Individuals learn better when they receive information in their preferred learning style (e.g., auditory, visual, kinesthetic).” In our sample of academics in UK Higher Education, 58% agreed with that same statement while 64% agreed with the similar, subsequent statement “I try to organize my teaching to accommodate different Learning Styles.” Thus a majority of academics in UK HE ‘believe’ in the use of Learning Styles although the figures are lower than in the 2012 study of schoolteachers. However, prior to asking these questions we asked some more direct questions about the actual use of Learning Styles instruments. Here the figures were much lower, with 33% of participants answering ‘yes’ to the statement “Have you ever administered a Learning Styles questionnaire to your students” and the same number stating that they had used ‘Learning Styles’ as a method in the last 12 months, where the method was defined as “matching teaching to individual student Learning Styles.” This value was lower than for a number of teaching methods that are evidence-based. Interestingly the most commonly used Learning Styles instrument was the Kolb Learning Styles Inventory; this is the Learning Styles classification that has been most frequently tested for evidence of such a ‘matching effect’ and where no evidence has been found ( Pashler et al., 2008 ).
The empirical evidence is clear that there is currently no evidence to support the use of Learning Styles instruments in this way ( Coffield et al., 2004 ; Pashler et al., 2008 ) and thus the fact that actual use of Learning Styles is lower than the use of demonstrably evidence-based methods could be considered reassuring, as could our finding that actual use is lower than ‘belief’ in the efficacy of Learning Styles. In addition, although we find that a majority of UK academics in Higher Education believe in the use of Learning Styles, the actual numbers observed are the lowest of any similar study. Studies examining belief in the use of Learning Styles have been carried out over the last few years in a number of different populations, and the overall trend is down, from 93% of UK schoolteachers in 2012 (Dekker), to 76% of UK schoolteachers in 2014 (Simmonds), 64% of HE academics in the US in 2014 (Dandy and Bendersky) to 58% here. There are obviously a number of caveats to consider before concluding that belief in the use of Learning Styles is declining; these studies have been conducted in different countries (US and UK), using teachers in different disciplines (school teachers and higher education). A follow-up, longitudinal study across different populations/contexts would be informative to address whether belief in the use of Learning Styles is truly declining, and to further understand whether actual use of Learning Styles is lower than ‘belief,’ as we have found here.
However, a more pessimistic interpretation of the data would be to focus on our finding that one-third of academics in UK higher education have, in the last year, used a method that was shown to be ineffective more than a decade earlier. The free-text comments give us some insight into the broader issue and perhaps a further hypothesis as to why the ‘myth’ of Learning Styles persists. The dominant theme was a stated need to use a diverse range of teaching methods. This is a separate issue to the use of Learning Styles and there was no suggestion in the survey that to not use Learning Styles was to advocate for all students to be taught the same way, and/or to use only one method of teaching. Neither of these approaches are advocated by the wider literature which seeks to ‘debunk’ Learning Styles, but it is clear from the abundance of comments on this theme that these two issues were related in the view of many of the participants. This is supported by the emergence of the related theme of ‘styles of learning rather than Learning Styles’; many participants had a looser definition of ‘Learning Styles’ than those introduced early in the survey. This finding leads us to urge caution and clarity in the continued ‘debunking’ of the ‘myth’ of Learning Styles. Learners obviously have preferences for how they learn. In addition, there is an obvious appeal to using a variety of teaching methods and in asking students to reflect on the ways in which they learn. However, these three concepts are unrelated to the (unsupported) idea that there is a benefit to learners from diagnosing their ‘Learning Style’ using one of the specific classifications ( Coffield et al., 2004 ) and attempting to match teaching to those styles. However, these concepts were clearly linked in the mind of many of our participants.
Participants agreed with many of the statements describing proposed harms or weaknesses of Learning Styles. Part of our intention here was to understand which are the most compelling of these; all have, at least, a face validity if not empirical evidence to support them. As we attempt to ‘spread the word’ about Learning Styles and promote alternate, evidence-based approaches, it is useful to know where perceived weaknesses are with Learning Styles. Thus our aim was not so much to observe absolute rates of agreement with individual harms/weaknesses (we would expect to see agreement, given that participants had just been told of the lack of evidence for Learning Styles), but to identify any differences in rates of agreement between the individual statements. There was strongest agreement with the conceptual weaknesses associated with Learning Style theory; that it is not possible to teach ‘understanding’ using a particular style, or to capture certain types of learning in all styles. Weakest agreement was with the statement that “The continued promotion of Learning Styles as a product is exploiting students and their teachers, for the financial gain of those companies which sell access to, and training in, the various Learning Style questionnaires.” The difference between the ‘conceptual weakness’ and other weaknesses/harms was statistically significant, suggesting that, where efforts are being made to ‘debunk’ the ‘myth’ of Learning Styles, then an appeal to the simple conceptual problems may be the most compelling approach. This would also seem to fit with the data described above re: ‘belief vs. use’; although it is tempting to believe that individual students have a Learning Style than can be utilized to benefit their education, the conceptual flaws inherent in the theory mean that actually putting them into practice may prove challenging.
Completion of the questionnaire, which highlighted all of the problems associated with the use of Learning Styles, was clearly associated with a group-shift in the stated likelihood that the participant group would use Learning Styles, although we must also consider that, having been presented with all the evidence that Learning Styles are not effective, it seems reasonable to assume that some participants may succumb to some form of social desirability bias, wherein participants respond in the way that they perceive the researchers desire or expect ( Nederhof, 1985 ). However, despite being presented with all the aforementioned evidence, approximately one-third of participants still agreed with the statement “In light of the information presented……‘I plan to try and account for individual student Learning Styles in my teaching.’” As described in the section “Introduction” there is an ongoing controversy, often played out via blogs and social media, about the use of Learning Styles, with some continuing to advocate for their use despite presentation of all the aforementioned evidence. It is even possible that to persist with a ‘myth debunking’ approach to Learning Styles may be counter-productive; the so-called ‘backfire effect’ describes a phenomenon wherein attempts to counter myths and misconceptions can result in a strengthening of belief in those myths. For example, 43% of the US population believe that the flu vaccine causes flu, and amongst that group are some who are very worried about the side effects of vaccines. Correcting the misconception that the vaccine causes flu is effective in reducing belief in the myth, yet reduces the likelihood that those who are concerned about vaccines will get vaccinated ( Nyhan and Reifler, 2015 ). We observed that almost all those who said they would still use Learning Styles after completing the survey had originally said that they try to account for Learning Styles in their teaching. An interesting question for further study may be to ask, of those who are currently using Learning Styles, whether being presented with the (lack of) evidence regarding their use makes it more likely that those academics will continue to use them? In addition, it may be informative to use an in-depth qualitative approach that would allow us to understand, in detail, what it is about Learning Styles that continues to appeal.
Instead of focusing on Learning Styles, it may be more productive for all, most importantly for students, to focus on the use of teaching and development activities which are demonstrably effective. For example, the use of microteaching, a simple, multi-peer review activity, the effectiveness of which has been repeatedly demonstrated in teacher-training settings ( Yeany and Padilla, 1986 ). Only 12% of survey participants here stated that they had used microteaching within the last 12 months, yet to do so would be relatively straightforward; it is little more than the application of a few more peers to an episode of peer-observation; something that is routinely undertaken by academics in UK Higher Education. This finding may be confounded by participants simply not being aware that ‘microteaching’ means, basically, ‘multi-peer observation and feedback,’ although this was explained twice in the survey itself.
Further support for an approach focused on raising awareness comes from our finding ( Figure Figure1 1 ) that, as a group, participants stated use of different teaching methods mapped directly on to their perceived usefulness (e.g., the most commonly used technique was formative assessment which was also perceived as the most effective). It seems reasonable to infer a causative relationship between these two observations, i.e., that participants use techniques which they consider to be effective, and thus if we can raise awareness of techniques which are demonstrably effective, then their use will increase.
There are some limitations to our study. A review of factors associated with dropouts from online surveys ( Galesic, 2006 ) observed that the average dropout rate amongst general-invitation online surveys (such as this one) is ∼30%, and so our dropout rate is entirely within expectations, although upon reflection we could perhaps have designed the instrument in a way that reduced dropout. A number of factors are associated with higher dropout rates, including the participant’s level of interest in the topic and the presence of ‘matrix questions.’ As described in the methods, we deliberately avoid entitling the survey as being about ‘Learning Styles’ to avoid biasing the responses, and a detailed analysis of the participation rate for each question revealed that the majority of dropouts occurred very early in the survey, after being asked to rank the effectiveness of the five teaching methods; a question potentially requiring higher effort than the others. An additional point reviewed by Galesic (2006) is the evidence that the quality of responses tails off for the items preceding the actual dropout point, thus the fact that participation rate remained steady after this early dropout is reassuring. It would also have been helpful to have a larger sample size. Although ours was equivalent to that in similar studies ( Dekker et al., 2012 ; Dandy and Bendersky, 2014 ) we may have been able to tease out more detail from the responses with a larger sample size, for example to determine whether ‘belief’ in Learning Styles was associated with any of the demographics factors (e.g., subject discipline, or age) to get a deeper understanding of why and where Learning Styles persist.
In summary, we found that 58% of academics in UK Higher Education believe that Learning Styles are effective, but only about a third actually use them, a lower percentage than use other, demonstrably evidence-based techniques. Ninety percent of academics agreed that there is a basic conceptual flaw with Learning Styles Theory. These data suggest that, although there is an ongoing controversy about Learning Styles, their actual use may be low, and further attempts to educate colleagues about this limitation might best focus on the fundamental conceptual limitations of Learning Styles theory. However, approximately one-third of academics stated that they would continue to use Learning Styles despite being presented with all the evidence. Thus it may be better still to focus on the promotion of techniques that are demonstrably effective.
Author Contributions
PN conceived the study, PN and MM designed the questionnaire, PN piloted and distributed the questionnaire, PN and MM analyzed the data, PN wrote the manuscript.
Conflict of Interest Statement
The authors declare that the research was conducted in the absence of any commercial or financial relationships that could be construed as a potential conflict of interest.
Acknowledgments
The authors would like to thank those colleagues who distributed the survey at their institutions, and Helen Davies from the Swansea Academy of Learning and Teaching for support with Limesurvey TM .
Supplementary Material
The Supplementary Material for this article can be found online at: http://journal.frontiersin.org/article/10.3389/fpsyg.2017.00444/full#supplementary-material
- Black C. (2016). Science/Fiction HOW LEARNING STYLES BECAME A MYTH . Available at: http://carolblack.org/science-fiction/ [ Google Scholar ]
- Braun V., Clarke V. (2006). Using thematic analysis in psychology. Qual. Res. Psychol. 3 77–101. 10.1191/1478088706qp063oa [ CrossRef ] [ Google Scholar ]
- Coffield F., Moseley D., Hall E., Ecclestone K. (2004). Learning Styles and Pedagogy in Post 16 Learning: A Systematic and Critical Review. The Learning and Skills Research Centre . Available at: http://localhost:8080/xmlui/handle/1/273 [ Google Scholar ]
- Dandy K., Bendersky K. (2014). Student and faculty beliefs about learning in higher education: implications for teaching. Int. J. Teach. Learn. High. Educ. 26 358–380. [ Google Scholar ]
- Dekker S., Lee N. C., Howard-Jones P., Jolles J. (2012). Neuromyths in education: prevalence and predictors of misconceptions among teachers. Front. Psychol. 3 : 429 10.3389/fpsyg.2012.00429 [ PMC free article ] [ PubMed ] [ CrossRef ] [ Google Scholar ]
- Felder R. M. (2010). ARE LEARNING STYLES INVALID? (HINT: NO!) . Available at: http://www4.ncsu.edu/unity/lockers/users/f/felder/public/Papers/LS_Validity%28On-Course%29.pdf [ Google Scholar ]
- Fleming N. D. (2012). The Case Against Learning Styles. Available at: http://vark-learn.com/wp-content/uploads/2014/08/The-Case-Against-Learning-Styles.pdf [ Google Scholar ]
- Galesic M. (2006). Dropouts on the web: effects of interest and burden experienced during an online survey. J. Off. Stat. 22 313–328. [ Google Scholar ]
- Geake J. (2008). Neuromythologies in education. Educ. Res. 50 123–133. 10.1080/00131880802082518 [ CrossRef ] [ Google Scholar ]
- Goldhill O. (2016). The Concept of Different “learning Styles” Is One of the Greatest Neuroscience Myths — Quartz. Available at: http://qz.com/585143/the-concept-of-different-learning-styles-is-one-of-the-greatest-neuroscience-myths/ [ Google Scholar ]
- Hattie J. A. C. (2009). Visible Learning: A Synthesis of over 800 Meta-Analyses Relating to Achievement. London: Routledge. [ Google Scholar ]
- Howard-Jones P. A. (2014). Neuroscience and education: myths and messages. Nat. Rev. Neurosci. 15 817–824. 10.1038/nrn3817 [ PubMed ] [ CrossRef ] [ Google Scholar ]
- Lilienfeld S. O., Lynn S. J., Ruscio J., Beyerstein B. L. (2011). 50 Great Myths of Popular Psychology: Shattering Widespread Misconceptions about Human Behavior. Hoboken, NJ: John Wiley & Sons. [ Google Scholar ]
- Nederhof A. J. (1985). Methods of coping with social desirability bias: a review. Eur. J. Soc. Psychol. 15 263–280. 10.1002/ejsp.2420150303 [ CrossRef ] [ Google Scholar ]
- Newton P. M. (2015). The learning styles myth is thriving in higher education. Educ. Psychol. 6 : 1908 10.3389/fpsyg.2015.01908 [ PMC free article ] [ PubMed ] [ CrossRef ] [ Google Scholar ]
- Nyhan B., Reifler J. (2015). Does correcting myths about the flu vaccine work? An experimental evaluation of the effects of corrective information. Vaccine 33 459–464. 10.1016/j.vaccine.2014.11.017 [ PubMed ] [ CrossRef ] [ Google Scholar ]
- Pashler H., McDaniel M., Rohrer D., Bjork R. (2008). Learning styles: concepts and evidence. Psychol. Sci. Public Interest 9 105–119. 10.1111/j.1539-6053.2009.01038.x [ PubMed ] [ CrossRef ] [ Google Scholar ]
- Pasquinelli E. (2012). Neuromyths: Why do they exist and persist? Mind Brain Educ. 6 89–96. 10.1111/j.1751-228X.2012.01141.x [ CrossRef ] [ Google Scholar ]
- Rato J. R., Abreu A. M., Castro-Caldas A. (2013). Neuromyths in education: What is fact and what is fiction for portuguese teachers? Educ. Res. 55 441–453. 10.1080/00131881.2013.844947 [ CrossRef ] [ Google Scholar ]
- Riener C., Willingham D. (2010). The myth of learning styles. Change 42 32–35. 10.1080/00091383.2010.503139 [ CrossRef ] [ Google Scholar ]
- Rohrer D., Pashler H. (2012). Learning styles: Where’s the evidence? Med. Educ. 46 634–635. 10.1111/j.1365-2923.2012.04273.x [ PubMed ] [ CrossRef ] [ Google Scholar ]
- Sax L. J., Gilmartin S. K., Bryant A. N. (2003). Assessing response rates and nonresponse bias in web and paper surveys. Res. High. Educ. 44 409–432. 10.1023/A:1024232915870 [ CrossRef ] [ Google Scholar ]
- Simmonds A. (2014). How Neuroscience Is Affecting Education: Report of Teacher and Parent Surveys. Available at: https://wellcome.ac.uk/sites/default/files/wtp055240.pdf [ Google Scholar ]
- Singal J. (2015). One Reason the “Learning Styles” Myth Persists. Available at: http://nymag.com/scienceofus/2015/12/one-reason-the-learning-styles-myth-persists.html [ Google Scholar ]
- Willingham D. T., Hughes E. M., Dobolyi D. G. (2015). The scientific status of learning styles theories. Teach. Psychol. 42 266–271. 10.1177/0098628315589505 [ CrossRef ] [ Google Scholar ]
- Yeany R. H., Padilla M. J. (1986). Training science teachers to utilize better teaching strategies: a research synthesis. J. Res. Sci. Teach. 23 85–95. 10.1002/tea.3660230202 [ CrossRef ] [ Google Scholar ]
7 types of learning styles and how you can to teach them
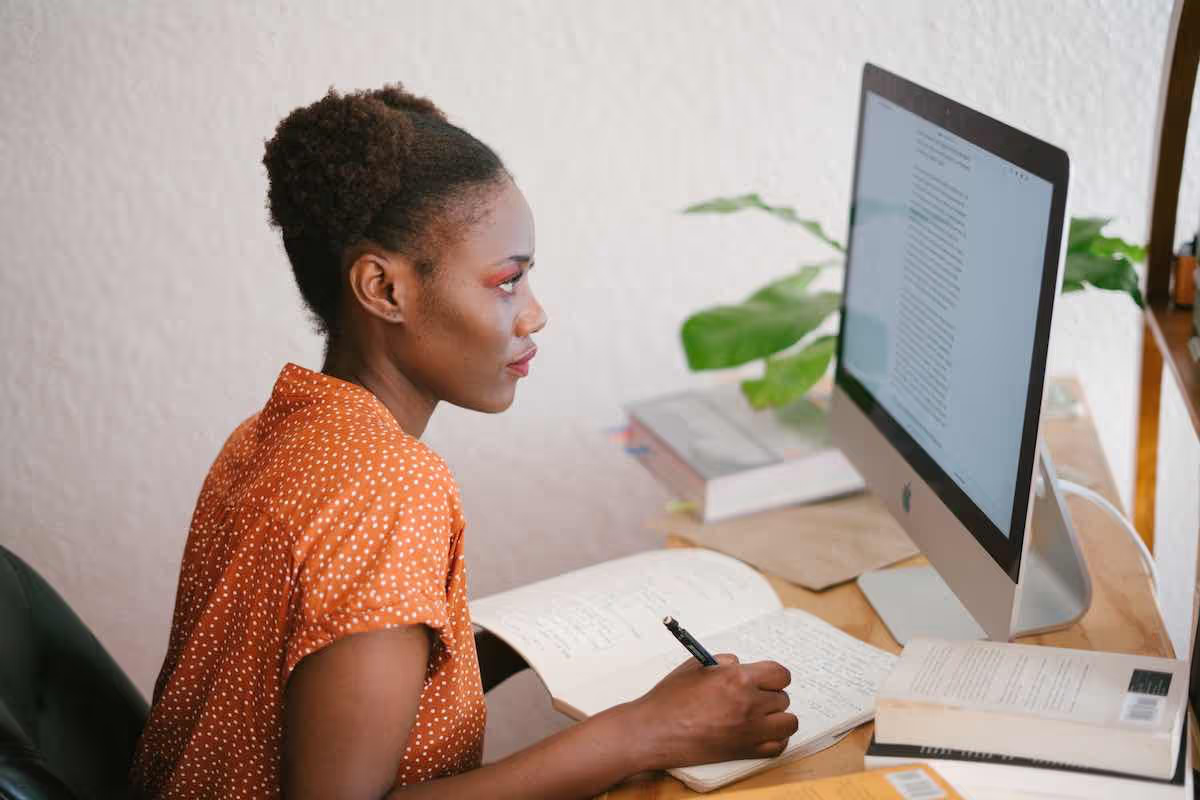
We all absorb and retain information in different ways. Some people learn faster and more efficiently when content includes visuals like charts, photos, or videos. Others prefer to read and write to retain information. Course creators that know the different types of learning styles can use them to improve student experiences and outcomes. If you know your students and the ways they learn, you can adapt your teaching styles to suit them better.
Creating courses with learning and teaching styles in mind will set you and your students up for success. We’ll go over each learning method and how to identify them. Plus, we’ll provide examples and tips on how to create courses, coaching, and other educational products for each learning style.
What are learning styles?
Learning styles are the methods that people use to understand and remember information. By identifying your students’ learning styles, you can create course materials that suit their preferences.
There is some debate over how many types of learning exist. Most agree that there are four to seven learning styles. We’ll go over each in detail below.
It’s also important to note that one person can have multiple styles. These are known as multimodal learners. They retain information and may thrive using more than one learning style.
The seven types of learning
New Zealand educator Neil Fleming developed the VARK model in 1987. It’s one of the most common methods to identify learning styles. Fleming proposed four primary learning preferences—visual, auditory, reading/writing, and kinesthetic. The first letter of each spells out the acronym (VARK).
We’ll go over the VARK learning styles and three others that researchers and educators have identified below.
1. Visual learning
When you create a course curriculum , consider how many and what type of visuals to include. A 2019 study claims that around 65% of people are visual learners . In other words, visual learners make up the majority of the population. You’ll likely have several in your courses, so keep that in mind when creating materials for it.
To learn best, visual learners need graphs, illustrations, diagrams, videos, and other visuals. You can also teach visual learners better by incorporating these into your lessons.
- Infographics
- Illustrations
- Photographs
- Flashcards with images
- Virtual whiteboards
Using all of these visuals at once will overwhelm your students. Instead, identify opportunities to display information as a visual and choose the best method for it.
Video courses are the best way to help visual learners. If you’re new to recording videos, you can take a course or watch a tutorial on making high-quality videos for your courses. You can also design and voiceover slideshows.
Visual learners also read and write like other students but may add images to notes, highlight sentences, or draw graphs. It can be helpful to provide them with downloadable versions of course materials so that they can take notes. If you use Teachable, you can easily add digital downloads to your website and courses.
Teachable creator Lauren Hom’s lettering course combines visual and other types of learning styles. Course lessons include videos, live drawing practice, and printable workbooks.
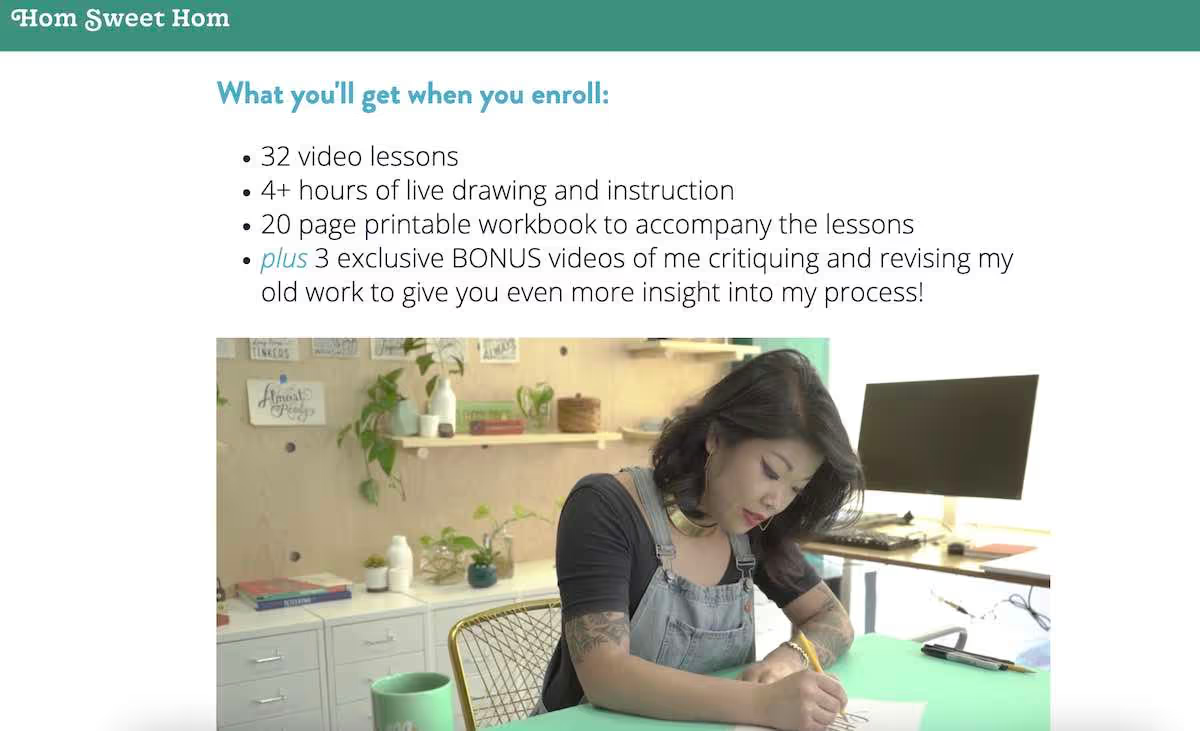
2. Auditory learning
In the same study, researchers found that around 30% of people are auditory learners. Auditory learners like to listen to absorb information. Auditory learners may listen to lectures, podcasts, music, and videos.
They also tend to read their notes aloud to help them understand and retain information or listen to music to study.
You can cater to auditory learners by:
- Using music and songs to remember information
- Providing audio versions of notes
- Encouraging discussions of learning materials
In addition to adjusting your teaching methods to different types of learners, you should also consider the subject.
For example, if you teach guitar online , it will naturally have an audio element. However, you may combine the sounds of different guitar strings with images and videos of them. When you combine different teaching methods, you can cater to multiple learning styles.
3. Reading and writing
Learners who prefer reading and writing thrive with traditional textbooks, handouts, and written assignments. Reading and writing learners are similar to visual learners because they like to see the information on a page.
To teach reading and writing learners, try to present information in one of these forms:
- Written instructions
- Written assignments
You could also consider creating an ebook to supplement your course material. So if you have a video course, add transcripts to your lessons so students can read along and take notes.
4. Kinesthetic
The kinesthetic learning style is learning by doing. And people who are kinesthetic learners learn better when they’re physically moving and getting hands-on experience.
Kinesthetic learners prefer playing games or doing puzzles as part of the learning process. They tend to enjoy problem-solving and trying new activities to build skills.
Many people associate kinesthetic learning with physical activities and in-person learning environments. However, you can still cater to kinesthetic learners when you create an online course .
For example, many developer courses include coding challenges, hackathons, and other activities where students learn by doing.
Here are some ideas to help you teach kinesthetic learners:
- Schedule short breaks for live courses longer than 30 minutes.
- Add real-life assignments. For example, a course about plants may add a practical element where students transplant and care for a houseplant.
- Create project briefs based on real-life scenarios, so students can practice.
- Add physical activity. Some online courses—meditation, yoga, and fitness—will naturally be more interactive.
If you want to add a more physical element, you can also include printables and supplies. Another option is to send materials to students in the mail.
There are many ways to teach kinesthetic learners. One example is the Hands-on Kids Activities Club (HOKA), a membership club for teachers. Every month, teachers get downloadable printables and other resources to create hands-on learning experiences. In one bundle, students learn about an artist and do an art project in that artist’s style.
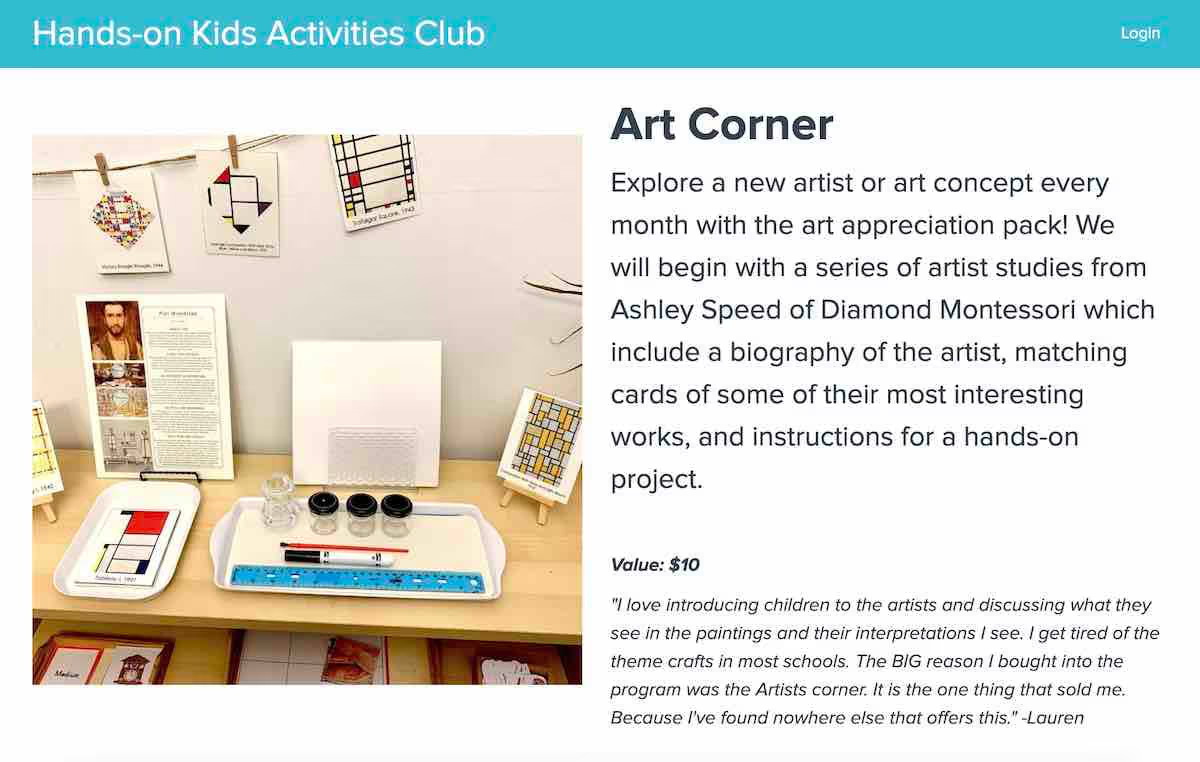
5. Verbal or linguistic learning
Verbal learners or linguistic learners retain information best by hearing and envisioning words. You may also hear this called verbal-linguistic learning. Similar to an auditory learner, a verbal learner speaks aloud to memorize information better. They tend to be avid readers and may be talented storytellers or poets.
Any of these can help a verbal learner:
- Presentations
- Flashcards with words
- Word games and puzzles
This type of learning is also common in language courses. If you teach students how to speak Spanish, English, French, or another language, verbal learning will come in handy. They’ll want to hear how you pronounce words and practice speaking them on their own.
6. Social or interpersonal learning
Some students learn better alone and others learn better while in groups. Social, also called interpersonal, learners thrive in group discussions and group coaching.
They enjoy speaking in front of groups and asking questions. A social learner will like to give and receive feedback from other students and bounce ideas off others.
Interpersonal learners prefer these types of activities:
- Group discussions and activities
- Public speaking—presenting their work
- Working with a partner
- Studying flashcards with a partner
- Team-building exercises
7. Solitary or intrapersonal learning
Solitary learners prefer to learn on their own rather than with groups of peers. The word intrapersonal is similar to introvert—they can feel drained from social activities.
These students don’t enjoy group work and would rather get a list of items to study and work independently. Instead of getting ideas and feedback from other students, solitary learners are more introspective. They can get lost in their work and are more hesitant to ask for feedback or ideas from others.
Here are some ideas to help teach solitary learners:
- Ask questions to build trust and learn more about them.
- Give them space to work independently.
- Explain the why behind projects. Solitary learners focus on the future and outcomes, so they like to know the importance of learning different concepts.
Solitary learners are self-starters, so they usually have the determination to complete a course. Even though they prefer learning independently, learning from others has many benefits too.
Sometimes getting a solitary learner to open up more, ask for feedback, and challenge themselves can improve their learning. You could also offer solitary learners coaching or feedback sessions with you to help them develop their learning in a one-on-one environment.
How to identify student learning styles
Most adults have a sense of their preferred learning style. You can ask students or coaching clients which methods they prefer via an intake form when they sign up for your courses or coaching.
To identify learning styles, you can:
- Include an intake form on your sign-up pages
- Ask new students about their preferred learning styles directly
- Observe your students throughout the course
- Use assessments to help students figure out the learning style they like best
You can also use an online quiz like the VARK questionnaire to understand new students better. Another option is to create your own assessment and tailor it to your teaching style and course topic. Some sample questions you can use to create a quiz or questionnaire to identify learning styles are:
- Do you prefer to work alone or in groups?
- Would diagrams and illustrations make it easier to understand a concept?
- Is it easier to remember something in words or images?
- To understand how a machine works, would you take the machine apart yourself?
- Do you remember facts and figures more by hearing them spoken or reading them?
Let students know this is the kind of quiz with no wrong answer. You’ll use the answers to understand what type of learning style they prefer and tailor your teaching to better suit them.
Note that this type of questionnaire works best with coaching or online courses that use cohorts with specific start dates. You can use it to fine-tune your course curriculum for each cohort or personalize coaching sessions.
How to teach different types of learning
As you plan your course, think about how you can accommodate each learning style. For example, auditory learners usually thrive on discussion. On the other hand, learners who prefer to read and write might struggle with group discussions or debates. Discussions can be harder for them because they like to write their thoughts down first before speaking.
To accommodate different types of learning styles, provide several options. In the example above, you could give your students a discussion prompt ahead of time. Reading and writing learners can write talking points down before and auditory learners get the benefits of learning through discussion.
The ui.dev online courses are perfect examples of how to consider different types of learning. Looking at their React coding course, you can see that they provide lessons in two forms—video and text. This way visual, auditory, and reading and writing learners can refer to the materials that they understand best. It also includes kinesthetic learning with practice coding activities and projects where students build real-world applications.
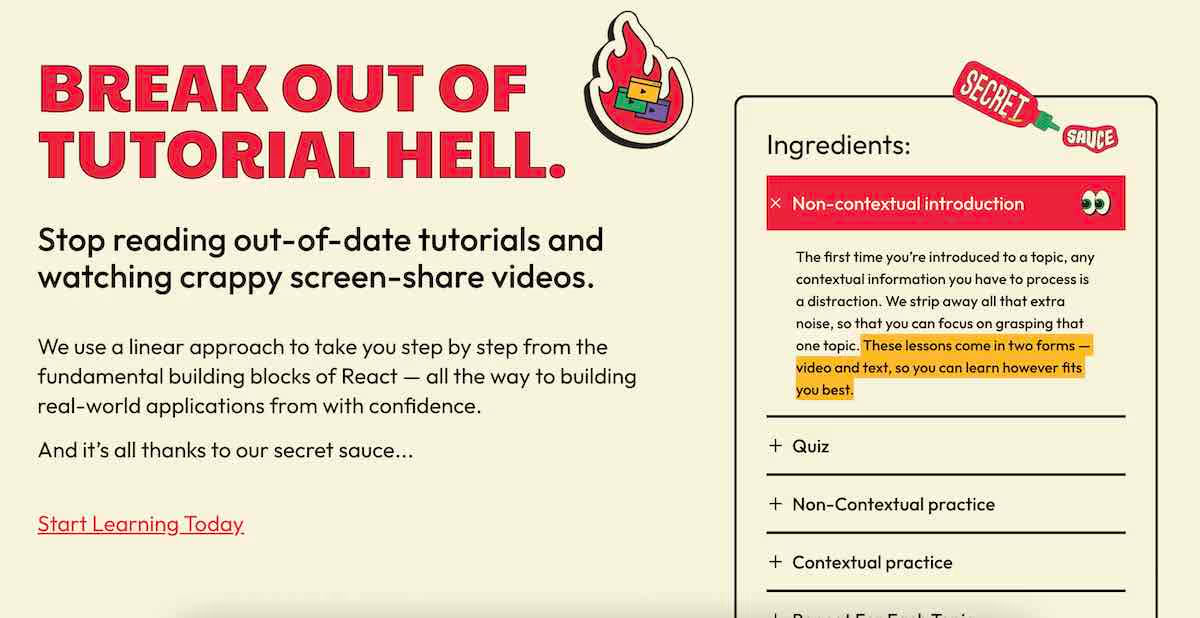
Share your knowledge online
No two students are exactly alike—a learning style that works for some students might not work for others. You can still offer your students or clients a meaningful learning experience.
Identifying how your students learn best helps you teach them in ways that will be the most successful. It also shows them that you care about their learning experience and outcomes. So by considering all the different learning styles, you’ll create an online course that appeals to a larger pool of people.
If you’re ready to share your knowledge with all types of learning styles, you can easily create a course on Teachable . And then you can create online courses, coaching services, and even digital downloads. To get started, sign up for free or choose from one of the paid plans .
More like this
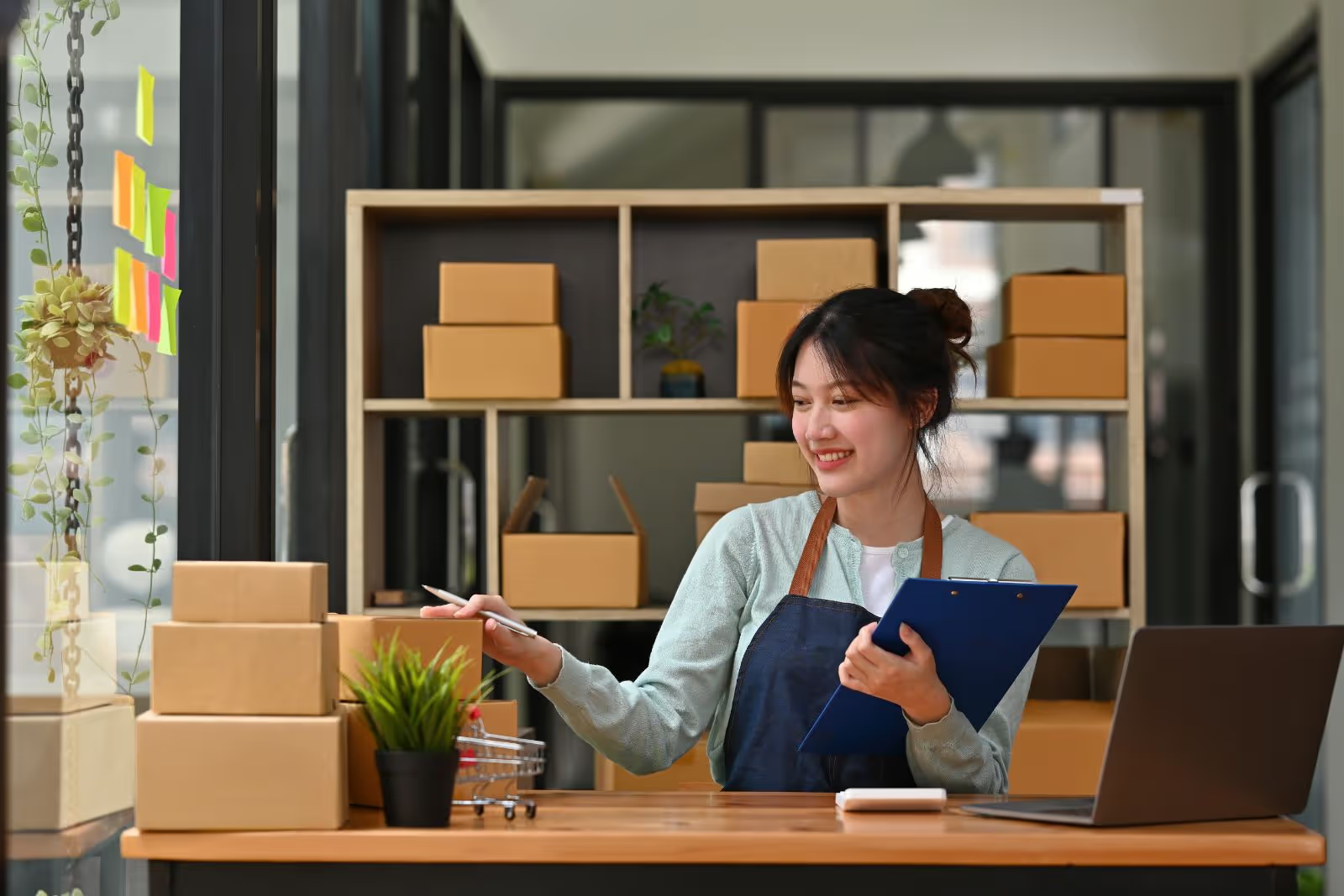
Your weekly dose of creative chat and Teachable updates. Get our weekly newsletter.

IMAGES
COMMENTS
learning styles assessments should NOT be incorporated into general education practice Learning Styles preferred ways of processing information; one learning theory model suggests visual, aural, read/write, and kinesthetic modes of learning -the concept that individuals differ in regard to what mode of instruction is most effective for them
Study with Quizlet and memorize flashcards containing terms like Learning Style is defined as an individual's unique approach to learning based on strengths, weaknesses, and preferences. Each of us is born with a preferred learning style. That learning style is the best method for how an individual takes in and processes information., People who learn best through visual stimulation are visual ...
Study with Quizlet and memorize flashcards containing terms like Current research on whether students can be identified has having specific "learning styles" suggests that teachers should: A. ask the students to say what their learning styles are and then allow them to choose activities to match. B. assess each student's learning style and provide learning activities that match it. C. adapt ...
The trustworthiness of the research findings is demonstrated by the confidence intervals derived from the meta-small analysis. A thorough examination of the Quizlet's effect on numerous outcome measures was made possible by the combination of experimental and quasi-experimental research. ... The use of Quizlet as a learning resource has also ...
The authors of the present review were charged with determining whether these practices are supported by scientific evidence. We concluded that any credible validation of learning-styles-based instruction requires robust documentation of a very particular type of experimental finding with several necessary criteria. First, students must be divided into groups on the basis of their learning ...
LEARNING STYLES INSTRUMENTS. As previously stated, several models and measures of learning styles have been described in the literature. Kolb proposed a model involving a 4-stage cyclic structure that begins with a concrete experience, which lends to a reflective observation and subsequently an abstract conceptualization that allows for active experimentation. 18 Kolb's model is associated ...
The learning-styles view has acquired great influence within the education field, and is frequently encountered at levels ranging from kindergarten to graduate school. There is a thriving industry devoted to publishing learning-styles tests and guidebooks for teachers, and many organizations offer professional development workshops for teachers ...
The term "learning styles" (also called learning modalities) generally refers to the idea that different students learn more effectively when information is presented in specific ways; however, many definitions of this concept exist, leading to a great deal of conceptual confusion (Pashler et al., 2009).According to Pashler et al., the origin of learning styles theories can be traced to a ...
28 June. The concept of "learning styles" has been overwhelmingly embraced by educators in the U.S. and worldwide. Studies show that an estimated 89% of teachers believe in matching instruction to a student's preferred learning style (Newton & Salvi, 2020). That's a problem—because research tells us that this approach doesn't work ...
Study with Quizlet and memorize flashcards containing terms like An understanding of learning styles.... A. enables a teacher to pinpoint individual differences that affect a person's learning B. assumes that all of us have a capacity to grow and develop C. allows informed students to become active participants in their own learning D. can help teachers tune into cultural differences without ...
Abstract. The term "learning styles" refers to the concept that individuals differ in regard to what mode of instruction or study is most effective for them. Proponents of learning-style ...
The term "learning styles" refers to the concept that individuals differ in regard to what mode of instruction or study is most effective for them. Proponents of learning-style assessment contend that optimal instruction requires diagnosing individuals' learning style and tailoring instruction accordingly. Assessments of learning style typically ask people to evaluate what sort of ...
In their review of research on learning styles for the Association for Psychological Science, Pashler, McDaniel, Rohrer, and Bjork (2008) came to a stark conclusion: "If classification of students' learning styles has practical utility, it remains to be demonstrated." (p. 117) Pashler et al pointed out that experiments designed to ...
The science of learning has made a considerable contribution to our understanding of effective teaching and learning strategies. However, few instructors outside of the field are privy to this research. In this tutorial review, we focus on six specific cognitive strategies that have received robust support from decades of research: spaced practice, interleaving, retrieval practice, elaboration ...
Over the last two decades, learning styles instruction has become ubiquitous in public education. It has gained influence and has enjoyed wide acceptance among educators at all levels, parents, and the general public (Pashler et al., 2009).It is prevalent in teacher education programs, adult education programs (Bishka, 2010), promoted in k-12 schools in many countries (Scott, 2010), and ...
The idea that learning styles vary among students has taken off in recent years. Many teachers, parents and students are adamant that they learn best visually or by hearing a lesson or by reading ...
An understanding of learning styles. - Enables a teacher to pinpoint individual differences that affect a person's learning. - Assumes that all of us have a capacity to grow and develop. - Allows informed students to become active participants in their own learning. - Can help teachers tune into cultural differences without stereotyping ...
Introduction. The concept of matching instructional strategies to an individual's learning style in order to enhance learning outcome and achieve better academic success is a well-known concept among educators and the general population (Pashler et al., 2008; Dekker et al., 2012; Howard-Jones, 2014).Learning styles are considered to have an impact in any learning situation regardless of ...
Cognitive style is described by Allport ( 1937) as an individual's typical or habitual mode of problem solving, thinking, perceiving and remembering, while the term learning style is adopted to reflect a concern with the application of cognitive style in a learning situation (Riding & Cheema, 1991 ).
Study with Quizlet and memorize flashcards containing terms like Which of the following is true about the scientific research on learning styles and academic performance?, Which of the following is an accurate statement about Roediger & Karpicke's experiment on the testing effect?, According to John Nestojko's research, published in the journal Memory and Cognition, students' learning was ...
Study with Quizlet and memorize flashcards containing terms like The reading outlines three requirements for evaluating the proposal that students learn best when teachers match their teaching style to students' individual learning styles. Which of the following statements reflect the current status of meeting these requirements., Cognitive tasks use either the left or right side of the brain ...
A recent study demonstrated that current research papers 'about' Learning Styles, ... Studies examining belief in the use of Learning Styles have been carried out over the last few years in a number of different populations, and the overall trend is down, from 93% of UK schoolteachers in 2012 (Dekker), to 76% of UK schoolteachers in 2014 ...
The seven types of learning. New Zealand educator Neil Fleming developed the VARK model in 1987. It's one of the most common methods to identify learning styles. Fleming proposed four primary learning preferences—visual, auditory, reading/writing, and kinesthetic. The first letter of each spells out the acronym (VARK).