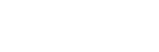

Qualitative Research: Observation
- Getting Started
- Focus Groups
- Observation
- Case Studies
- Data Collection
- Cleaning Text
- Analysis Tools
- Institutional Review
Participant Observation

Photo: https://slideplayer.com/slide/4599875/
Field Guide
- Participant Observation Field Guide
What is an observation?
A way to gather data by watching people, events, or noting physical characteristics in their natural setting. Observations can be overt (subjects know they are being observed) or covert (do not know they are being watched).
- Researcher becomes a participant in the culture or context being observed.
- Requires researcher to be accepted as part of culture being observed in order for success
Direct Observation
- Researcher strives to be as unobtrusive as possible so as not to bias the observations; more detached.
- Technology can be useful (i.e video, audiorecording).
Indirect Observation
- Results of an interaction, process or behavior are observed (for example, measuring the amount of plate waste left by students in a school cafeteria to determine whether a new food is acceptable to them).
Suggested Readings and Film
- Born into Brothels . (2004) Oscar winning documentary, an example of participatory observation, portrays the life of children born to prostitutes in Calcutta. New York-based photographer Zana Briski gave cameras to the children of prostitutes and taught them photography
- Davies, J. P., & Spencer, D. (2010). Emotions in the field: The psychology and anthropology of fieldwork experience . Stanford, CA: Stanford University Press.
- DeWalt, K. M., & DeWalt, B. R. (2011). Participant observation : A guide for fieldworkers . Lanham, Md: Rowman & Littlefield.
- Reinharz, S. (2011). Observing the observer: Understanding our selves in field research . NY: Oxford University Press.
- Schensul, J. J., & LeCompte, M. D. (2013). Essential ethnographic methods: A mixed methods approach . Lanham, MD: AltaMira Press.
- Skinner, J. (2012). The interview: An ethnographic approach . NY: Berg.
- << Previous: Focus Groups
- Next: Case Studies >>
- Last Updated: Mar 1, 2024 10:13 AM
- URL: https://guides.library.duke.edu/qualitative-research

Services for...
- Faculty & Instructors
- Graduate Students
- Undergraduate Students
- International Students
- Patrons with Disabilities

- Harmful Language Statement
- Re-use & Attribution / Privacy
- Support the Libraries


An official website of the United States government
The .gov means it’s official. Federal government websites often end in .gov or .mil. Before sharing sensitive information, make sure you’re on a federal government site.
The site is secure. The https:// ensures that you are connecting to the official website and that any information you provide is encrypted and transmitted securely.
- Publications
- Account settings
Preview improvements coming to the PMC website in October 2024. Learn More or Try it out now .
- Advanced Search
- Journal List
- J Basic Clin Pharm
- v.5(4); September 2014-November 2014
Qualitative research method-interviewing and observation
Shazia jamshed.
Department of Pharmacy Practice, Kulliyyah of Pharmacy, International Islamic University Malaysia, Kuantan Campus, Pahang, Malaysia
Buckley and Chiang define research methodology as “a strategy or architectural design by which the researcher maps out an approach to problem-finding or problem-solving.”[ 1 ] According to Crotty, research methodology is a comprehensive strategy ‘that silhouettes our choice and use of specific methods relating them to the anticipated outcomes,[ 2 ] but the choice of research methodology is based upon the type and features of the research problem.[ 3 ] According to Johnson et al . mixed method research is “a class of research where the researcher mixes or combines quantitative and qualitative research techniques, methods, approaches, theories and or language into a single study.[ 4 ] In order to have diverse opinions and views, qualitative findings need to be supplemented with quantitative results.[ 5 ] Therefore, these research methodologies are considered to be complementary to each other rather than incompatible to each other.[ 6 ]
Qualitative research methodology is considered to be suitable when the researcher or the investigator either investigates new field of study or intends to ascertain and theorize prominent issues.[ 6 , 7 ] There are many qualitative methods which are developed to have an in depth and extensive understanding of the issues by means of their textual interpretation and the most common types are interviewing and observation.[ 7 ]
Interviewing
This is the most common format of data collection in qualitative research. According to Oakley, qualitative interview is a type of framework in which the practices and standards be not only recorded, but also achieved, challenged and as well as reinforced.[ 8 ] As no research interview lacks structure[ 9 ] most of the qualitative research interviews are either semi-structured, lightly structured or in-depth.[ 9 ] Unstructured interviews are generally suggested in conducting long-term field work and allow respondents to let them express in their own ways and pace, with minimal hold on respondents’ responses.[ 10 ]
Pioneers of ethnography developed the use of unstructured interviews with local key informants that is., by collecting the data through observation and record field notes as well as to involve themselves with study participants. To be precise, unstructured interview resembles a conversation more than an interview and is always thought to be a “controlled conversation,” which is skewed towards the interests of the interviewer.[ 11 ] Non-directive interviews, form of unstructured interviews are aimed to gather in-depth information and usually do not have pre-planned set of questions.[ 11 ] Another type of the unstructured interview is the focused interview in which the interviewer is well aware of the respondent and in times of deviating away from the main issue the interviewer generally refocuses the respondent towards key subject.[ 11 ] Another type of the unstructured interview is an informal, conversational interview, based on unplanned set of questions that are generated instantaneously during the interview.[ 11 ]
In contrast, semi-structured interviews are those in-depth interviews where the respondents have to answer preset open-ended questions and thus are widely employed by different healthcare professionals in their research. Semi-structured, in-depth interviews are utilized extensively as interviewing format possibly with an individual or sometimes even with a group.[ 6 ] These types of interviews are conducted once only, with an individual or with a group and generally cover the duration of 30 min to more than an hour.[ 12 ] Semi-structured interviews are based on semi-structured interview guide, which is a schematic presentation of questions or topics and need to be explored by the interviewer.[ 12 ] To achieve optimum use of interview time, interview guides serve the useful purpose of exploring many respondents more systematically and comprehensively as well as to keep the interview focused on the desired line of action.[ 12 ] The questions in the interview guide comprise of the core question and many associated questions related to the central question, which in turn, improve further through pilot testing of the interview guide.[ 7 ] In order to have the interview data captured more effectively, recording of the interviews is considered an appropriate choice but sometimes a matter of controversy among the researcher and the respondent. Hand written notes during the interview are relatively unreliable, and the researcher might miss some key points. The recording of the interview makes it easier for the researcher to focus on the interview content and the verbal prompts and thus enables the transcriptionist to generate “verbatim transcript” of the interview.
Similarly, in focus groups, invited groups of people are interviewed in a discussion setting in the presence of the session moderator and generally these discussions last for 90 min.[ 7 ] Like every research technique having its own merits and demerits, group discussions have some intrinsic worth of expressing the opinions openly by the participants. On the contrary in these types of discussion settings, limited issues can be focused, and this may lead to the generation of fewer initiatives and suggestions about research topic.
Observation
Observation is a type of qualitative research method which not only included participant's observation, but also covered ethnography and research work in the field. In the observational research design, multiple study sites are involved. Observational data can be integrated as auxiliary or confirmatory research.[ 11 ]
Research can be visualized and perceived as painstaking methodical efforts to examine, investigate as well as restructure the realities, theories and applications. Research methods reflect the approach to tackling the research problem. Depending upon the need, research method could be either an amalgam of both qualitative and quantitative or qualitative or quantitative independently. By adopting qualitative methodology, a prospective researcher is going to fine-tune the pre-conceived notions as well as extrapolate the thought process, analyzing and estimating the issues from an in-depth perspective. This could be carried out by one-to-one interviews or as issue-directed discussions. Observational methods are, sometimes, supplemental means for corroborating research findings.
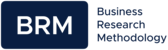
Observation
Observation, as the name implies, is a way of collecting data through observing. This data collection method is classified as a participatory study, because the researcher has to immerse herself in the setting where her respondents are, while taking notes and/or recording. Observation data collection method may involve watching, listening, reading, touching, and recording behavior and characteristics of phenomena.
Observation as a data collection method can be structured or unstructured. In structured or systematic observation, data collection is conducted using specific variables and according to a pre-defined schedule. Unstructured observation, on the other hand, is conducted in an open and free manner in a sense that there would be no pre-determined variables or objectives.
Moreover, this data collection method can be divided into overt or covert categories. In overt observation research subjects are aware that they are being observed. In covert observation, on the other hand, the observer is concealed and sample group members are not aware that they are being observed. Covert observation is considered to be more effective because in this case sample group members are likely to behave naturally with positive implications on the authenticity of research findings.
Advantages of observation data collection method include direct access to research phenomena, high levels of flexibility in terms of application and generating a permanent record of phenomena to be referred to later. At the same time, this method is disadvantaged with longer time requirements, high levels of observer bias, and impact of observer on primary data, in a way that presence of observer may influence the behaviour of sample group elements.
It is important to note that observation data collection method may be associated with certain ethical issues. As it is discussed further below in greater details, fully informed consent of research participant(s) is one of the basic ethical considerations to be adhered to by researchers. At the same time, the behaviour of sample group members may change with negative implications on the level of research validity if they are notified about the presence of the observer.
This delicate matter needs to be addressed by consulting with dissertation supervisor, and commencing the primary data collection process only after ethical aspects of the issue have been approved by the supervisor.
My e-book, The Ultimate Guide to Writing a Dissertation in Business Studies: a step by step assistance offers practical assistance to complete a dissertation with minimum or no stress. The e-book covers all stages of writing a dissertation starting from the selection to the research area to submitting the completed version of the work within the deadline.
John Dudovskiy
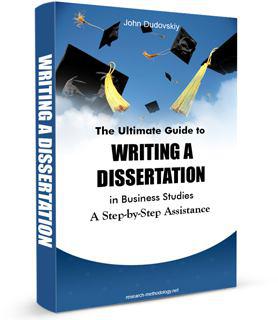
Observation Methods
- First Online: 14 December 2017
Cite this chapter
- Malgorzata Ciesielska 4 ,
- Katarzyna W. Boström 5 &
- Magnus Öhlander 6
9962 Accesses
49 Citations
Observation may be seen as the very foundation of everyday social interaction: as people participate in social life, they are diligent observers and commentators of others’ behavior. Observation is also one of the most important research methods in social sciences and at the same time one of the most complex. It may be the main method in the project or one of several complementary qualitative methods. As a scientific method it is has to be carried out systematically, with a focus on specific research questions. Therefore, we start with practical guide on clarifying research objectives, accessing the research field, selecting subjects, observer’s roles, and tips on documenting the data collected. The observation comprises several techniques and approaches that can be combined in a variety of ways. Observation can be either participant or not, direct or indirect. Further in this chapter, the main characteristics of three types of observations are outlined (the fourth type—direct non-participant—is discussed in the chapter on shadowing). While participant observation follows the ideal of a long-time immersion in a specific culture as a marginal member, researcher conducting non-participant observation takes position of an outsider and tries to distance him/herself from the taken-for-granted categorizations and evaluations. In the case of indirect observation, the researcher relies on observations of others (e.g. other researchers), various types of documentation, or self-observation. The chapter discusses the differences between those types of observation, shows inspirational examples from previous studies, and summarizes the method.
This is a preview of subscription content, log in via an institution to check access.
Access this chapter
Institutional subscriptions
Similar content being viewed by others
Participant Observation and Objectivity in Anthropology
Social Issues as a Focus of Community Studies
Participant Observation: The Personal Commitment in Native Life—A Problematic Methodological Topos
Adjam, M. (2017). Minnesspår. Hågkomstens rum och rörelse i skuggan av flykt . Höör: Brutus Östlings bokförlag Symposion.
Google Scholar
Arvastson, G., & Ehn, B. (2009). Etnografiska observationer . Lund: Studentlitteratur.
Bernard, H. R. (2000). Social Research Methods: Qualitative and Quantitative Approaches . Thousand Oaks: Sage.
Bernard, H. R. (2006). Research Methods in Anthropology. Qualitative and Quantitative Approaches . Thousand Oaks: Sage.
Bowden, A., & Ciesielska, M. (2016). Ecomuseums as Cross-Sector Partnerships: Similarities and Dissimilarities in their Governance, Strategy and Leadership. Public Money and Management, 36 (1), 23–30.
Article Google Scholar
Ciesielska, M. (2010). Hybrid Organisations. A Case of the Open Source-Business Setting . Frederiksberg: Copenhagen Business School Press.
Ciesielska, M., & Westenholz, A. (2016). Dilemmas Within Commercial Involvement in Open Source Software. Journal of Organizational Change Management, 29 (3), 344–360.
Ciesielska, M., Wolanik Boström, K., & Öhlander, M. (2012). Obserwacja. In D. Jemielniak (Ed.), Badania Jakościowe. Podręcznik akademicki. Tom I: Podejścia, teorie, problemy (pp. 41–67). Warszawa: PWN.
D’Eredita, M. A., & Barreto, C. (2006). How Does Tacit Knowledge Proliferate? An Episode-Based Perspective Organization Studies, 27 (12), 1821–1841.
Drake, D. H., & Harvey, J. (2014). Performing the Role of Ethnographer. Processing and Managing the Emotional Dimensions of Prison Research. International Journal of Social Research Methodology, 17 (5), 489–501.
Emerson, R. M., Fretz, R. I., & Shaw, L. L. (1995). Writing Ethnographic Fieldnotes . Chicago: University of Chicago Press.
Book Google Scholar
Emerson, R. M., Fretz, R. I., & Shaw, L. L. (2001). Participant Observation and Fieldnotes. In P. Atkinson (Ed.), Handbook of Ethnography . London: Sage.
Fangen, K. (2001). Pride and Power. A Sociological Study of the Norwegian Radical Nationalist Underground Movement . Oslo: Akademika.
Hammersley, M., & Atkinson, P. (2007). Ethnography. Principles in Practice . London: Routledge.
Hine, C. (2000). Virtual Ethnography . London: Sage.
Jauregui, B. (2013). Dirty Anthropology. Epistemologies of Violence and Ethical Entanglements in Police Ethnography. In W. Garriot (Ed.), Policing and Contemporary Governance. The Anthropology of Police in Practice . London: Palgrave and Macmillan.
Klintberg, B. (1986). Råttan i pizzan . Stockholm: Norstedts Förlag.
Kostera, M. (2007). Organizational Ethnography: Methods and Inspirations . London: Sage.
Kozinetz, R. V. (2015). Netnography. Redefined . Thousand oaks: Sage.
Nakumara, K. (2013). Making Sense of Sensory Ethnography. The Sensual and the Multisensory. American Anthropologist, 115 (1), 132–144.
Pink, S. (2015). Doing Sensory Ethnography (2nd ed.). Los Angeles/London: Sage.
Pripps, O., & Öhlander, M. (2011). Observation. In L. Kaijser & M. Öhlander (Eds.), Etnologiskt fältarbete . Lund: Studentlitteratur.
Rathje, W. (2001). Integrated Archaeology. A Garbage Paradigm. In V. Buchli & G. Lucas (Eds.), Archaeologies of the Contemporary Past . London: Routledge.
Rathje, W., & Murphy, C. (1992). Rubbish! The Archaeology of Garbage . New York: HarperCollins Publishers.
Silow Kallenberg, K. (2015). Smutsig etnografi. En metoddiskussion. Kulturella perspektiv, 24 (2), 2–12.
Silow Kallenberg, K. (2016). Gränsland. Svensk ungdomsvård mellan vård och straff . Huddinge: Södertörns högskola.
Sotirin, P. (1999). Bringing the Outside in. Ethnography in/beyond the Classroom . Annual Meeting of the National Communication Association, Chicago, 4–7.
Spradley, J. P. (1980). Participant Observation . New York: Holt, Rinehart and Winston.
Webb, E. J., Campbell, D. T., Schwartz, R. D., & Sechrest, L. (1966). Unobtrusive Measures: Nonreactive Research in the Social Sciences . Chicago: Rand McNally.
Wolanik Boström, K., & Öhlander, M. (2015). Mobile Physicians Making Sense of Culture(s). On Mobile Everyday Ethnography. Ethnologia Europaea, 45 (1), 7–24.
Download references
Author information
Authors and affiliations.
Teesside University Business School, Teesside University, Middlesbrough, UK
Malgorzata Ciesielska
Umeå University, Umea, Sweden
Katarzyna W. Boström
Stockholm University, Stockholm, Sweden
Magnus Öhlander
You can also search for this author in PubMed Google Scholar
Editor information
Editors and affiliations.
Teesside University Business School, Teesside University, Middlesbrough, United Kingdom
Akademia Leona Koźmińskiego, Warsaw, Poland
Dariusz Jemielniak
Rights and permissions
Reprints and permissions
Copyright information
© 2018 The Author(s)
About this chapter
Ciesielska, M., Boström, K.W., Öhlander, M. (2018). Observation Methods. In: Ciesielska, M., Jemielniak, D. (eds) Qualitative Methodologies in Organization Studies. Palgrave Macmillan, Cham. https://doi.org/10.1007/978-3-319-65442-3_2
Download citation
DOI : https://doi.org/10.1007/978-3-319-65442-3_2
Published : 14 December 2017
Publisher Name : Palgrave Macmillan, Cham
Print ISBN : 978-3-319-65441-6
Online ISBN : 978-3-319-65442-3
eBook Packages : Business and Management Business and Management (R0)
Share this chapter
Anyone you share the following link with will be able to read this content:
Sorry, a shareable link is not currently available for this article.
Provided by the Springer Nature SharedIt content-sharing initiative
- Publish with us
Policies and ethics
- Find a journal
- Track your research

10 Observational Research Examples
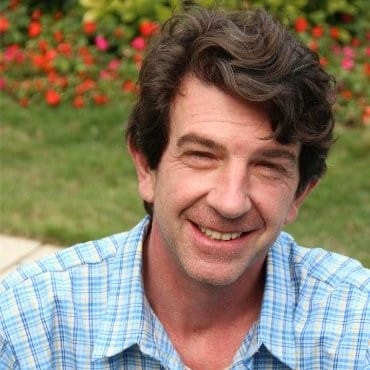
Dave Cornell (PhD)
Dr. Cornell has worked in education for more than 20 years. His work has involved designing teacher certification for Trinity College in London and in-service training for state governments in the United States. He has trained kindergarten teachers in 8 countries and helped businessmen and women open baby centers and kindergartens in 3 countries.
Learn about our Editorial Process
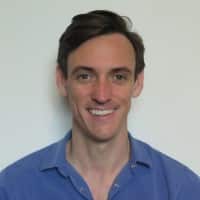
Chris Drew (PhD)
This article was peer-reviewed and edited by Chris Drew (PhD). The review process on Helpful Professor involves having a PhD level expert fact check, edit, and contribute to articles. Reviewers ensure all content reflects expert academic consensus and is backed up with reference to academic studies. Dr. Drew has published over 20 academic articles in scholarly journals. He is the former editor of the Journal of Learning Development in Higher Education and holds a PhD in Education from ACU.

Observational research involves observing the actions of people or animals, usually in their natural environments.
For example, Jane Goodall famously observed chimpanzees in the wild and reported on their group behaviors. Similarly, many educational researchers will conduct observations in classrooms to gain insights into how children learn.
Examples of Observational Research
1. jane goodall’s research.
Jane Goodall is famous for her discovery that chimpanzees use tools. It is one of the most remarkable findings in psychology and anthropology .
Her primary method of study involved simply entering the natural habitat of her research subjects, sitting down with pencil and paper, and making detailed notes of what she observed.
Those observations were later organized and transformed into research papers that provided the world with amazing insights into animal behavior.
When she first discovered that chimpanzees use twigs to “fish” for termites, it was absolutely stunning. The renowned Louis Leakey proclaimed: “we must now redefine tool, redefine man, or accept chimps as humans.”
2. Linguistic Development of Children
Answering a question like, “how do children learn to speak,” can only be answered by observing young children at home.
By the time kids get to first grade, their language skills have already become well-developed, with a vocabulary of thousands of words and the ability to use relatively complex sentences.
Therefore, a researcher has to conduct their study in the child’s home environment. This typically involves having a trained data collector sit in a corner of a room and take detailed notes about what and how parents speak to their child.
Those observations are later classified in a way that they can be converted into quantifiable measures for statistical analysis.
For example, the data might be coded in terms of how many words the parents spoke, degree of sentence complexity, or emotional dynamic of being encouraging or critical. When the data is analyzed, it might reveal how patterns of parental comments are linked to the child’s level of linguistic development.
Related Article: 15 Action Research Examples
3. Consumer Product Design
Before Apple releases a new product to the market, they conduct extensive analyses of how the product will be perceived and used by consumers.
The company wants to know what kind of experience the consumer will have when using the product. Is the interface user-friendly and smooth? Does it fit comfortably in a person’s hand?
Is the overall experience pleasant?
So, the company will arrange for groups of prospective customers come to the lab and simply use the next iteration of one of their great products. That lab will absolutely contain a two-way mirror and a team of trained observers sitting behind it, taking detailed notes of what the test groups are doing. The groups might even be video recorded so their behavior can be observed again and again.
That will be followed by a focus group discussion , maybe a survey or two, and possibly some one-on-one interviews.
4. Satellite Images of Walmart
Observational research can even make some people millions of dollars. For example, a report by NPR describes how stock market analysts observe Walmart parking lots to predict the company’s earnings.
The analysts purchase satellite images of selected parking lots across the country, maybe even worldwide. That data is combined with what they know about customer purchasing habits, broken down by time of day and geographic region.
Over time, a detailed set of calculations are performed that allows the analysts to predict the company’s earnings with a remarkable degree of accuracy .
This kind of observational research can result in substantial profits.
5. Spying on Farms
Similar to the example above, observational research can also be implemented to study agriculture and farming.
By using infrared imaging software from satellites, some companies can observe crops across the globe. The images provide measures of chlorophyll absorption and moisture content, which can then be used to predict yields. Those images also allow analysts to simply count the number of acres being planted for specific crops across the globe.
In commodities such as wheat and corn, that prediction can lead to huge profits in the futures markets.
It’s an interesting application of observational research with serious monetary implications.
6. Decision-making Group Dynamics
When large corporations make big decisions, it can have serious consequences to the company’s profitability, or even survival.
Therefore, having a deep understanding of decision-making processes is essential. Although most of us think that we are quite rational in how we process information and formulate a solution, as it turns out, that’s not entirely true.
Decades of psychological research has focused on the function of statements that people make to each other during meetings. For example, there are task-masters, harmonizers, jokers, and others that are not involved at all.
A typical study involves having professional, trained observers watch a meeting transpire, either from a two-way mirror, by sitting-in on the meeting at the side, or observing through CCTV.
By tracking who says what to whom, and the type of statements being made, researchers can identify weaknesses and inefficiencies in how a particular group engages the decision-making process.
See More: Decision-Making Examples
7. Case Studies
A case study is an in-depth examination of one particular person. It is a form of observational research that involves the researcher spending a great deal of time with a single individual to gain a very detailed understanding of their behavior.
The researcher may take extensive notes, conduct interviews with the individual, or take video recordings of behavior for further study.
Case studies give a level of detailed information that is not available when studying large groups of people. That level of detail can often provide insights into a phenomenon that could lead to the development of a new theory or help a researcher identify new areas of research.
Researchers sometimes have no choice but to conduct a case study in situations in which the phenomenon under study is “rare and unusual” (Lee & Saunders, 2017). Because the condition is so uncommon, it is impossible to find a large enough sample of cases to study with quantitative methods.
Go Deeper: Pros and Cons of Case Study Research
8. Infant Attachment
One of the first studies on infant attachment utilized an observational research methodology . Mary Ainsworth went to Uganda in 1954 to study maternal practices and mother/infant bonding.
Ainsworth visited the homes of 26 families on a bi-monthly basis for 2 years, taking detailed notes and interviewing the mothers regarding their parenting practices.
Her notes were then turned into academic papers and formed the basis for the Strange Situations test that she developed for the laboratory setting.
The Strange Situations test consists of 8 situations, each one lasting no more than a few minutes. Trained observers are stationed behind a two-way mirror and have been trained to make systematic observations of the baby’s actions in each situation.
9. Ethnographic Research
Ethnography is a type of observational research where the researcher becomes part of a particular group or society.
The researcher’s role as data collector is hidden and they attempt to immerse themselves in the community as a regular member of the group.
By being a part of the group and keeping one’s purpose hidden, the researcher can observe the natural behavior of the members up-close. The group will behave as they would naturally and treat the researcher as if they were just another member. This can lead to insights into the group dynamics , beliefs, customs and rituals that could never be studied otherwise.
10. Time and Motion Studies
Time and motion studies involve observing work processes in the work environment. The goal is to make procedures more efficient, which can involve reducing the number of movements needed to complete a task.
Reducing the movements necessary to complete a task increases efficiency, and therefore improves productivity. A time and motion study can also identify safety issues that may cause harm to workers, and thereby help create a safer work environment.
The two most famous early pioneers of this type of observational research are Frank and Lillian Gilbreth.
Lilian was a psychologist that began to study the bricklayers of her husband Frank’s construction company. Together, they figured out a way to reduce the number of movements needed to lay bricks from 18 to 4 (see original video footage here ).
The couple became quite famous for their work during the industrial revolution and
Lillian became the only psychologist to appear on a postage stamp (in 1884).
Why do Observational Research?
Psychologists and anthropologists employ this methodology because:
- Psychologists find that studying people in a laboratory setting is very artificial. People often change their behavior if they know it is going to be analyzed by a psychologist later.
- Anthropologists often study unique cultures and indigenous peoples that have little contact with modern society. They often live in remote regions of the world, so, observing their behavior in a natural setting may be the only option.
- In animal studies , there are lots of interesting phenomenon that simply cannot be observed in a laboratory, such as foraging behavior or mate selection. Therefore, observational research is the best and only option available.
Read Also: Difference Between Observation and Inference
Observational research is an incredibly useful way to collect data on a phenomenon that simply can’t be observed in a lab setting. This can provide insights into human behavior that could never be revealed in an experiment (see: experimental vs observational research ).
Researchers employ observational research methodologies when they travel to remote regions of the world to study indigenous people, try to understand how parental interactions affect a child’s language development, or how animals survive in their natural habitats.
On the business side, observational research is used to understand how products are perceived by customers, how groups make important decisions that affect profits, or make economic predictions that can lead to huge monetary gains.
Ainsworth, M. D. S. (1967). Infancy in Uganda . Baltimore: Johns Hopkins University Press.
Ainsworth, M. D. S., Blehar, M., Waters, E., & Wall, S. (1978). Patterns of attachment: A
psychological study of the Strange Situation. Hillsdale: Erlbaum.
Crowe, S., Cresswell, K., Robertson, A., Huby, G., Avery, A., & Sheikh, A. (2011). The case study approach. BMC Medical Research Methodology , 11 , 100. https://doi.org/10.1186/1471-2288-11-100
d’Apice, K., Latham, R., & Stumm, S. (2019). A naturalistic home observational approach to children’s language, cognition, and behavior. Developmental Psychology, 55 (7),1414-1427. https://doi.org/10.1037/dev0000733
Lee, B., & Saunders, M. N. K. (2017). Conducting Case Study Research for Business and Management Students. SAGE Publications.
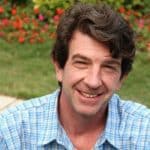
- Dave Cornell (PhD) https://helpfulprofessor.com/author/dave-cornell-phd/ 23 Achieved Status Examples
- Dave Cornell (PhD) https://helpfulprofessor.com/author/dave-cornell-phd/ 25 Defense Mechanisms Examples
- Dave Cornell (PhD) https://helpfulprofessor.com/author/dave-cornell-phd/ 15 Theory of Planned Behavior Examples
- Dave Cornell (PhD) https://helpfulprofessor.com/author/dave-cornell-phd/ 18 Adaptive Behavior Examples
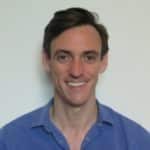
- Chris Drew (PhD) https://helpfulprofessor.com/author/chris-drew-phd/ 23 Achieved Status Examples
- Chris Drew (PhD) https://helpfulprofessor.com/author/chris-drew-phd/ 15 Ableism Examples
- Chris Drew (PhD) https://helpfulprofessor.com/author/chris-drew-phd/ 25 Defense Mechanisms Examples
- Chris Drew (PhD) https://helpfulprofessor.com/author/chris-drew-phd/ 15 Theory of Planned Behavior Examples
Leave a Comment Cancel Reply
Your email address will not be published. Required fields are marked *
Research Methods In Psychology
Saul McLeod, PhD
Editor-in-Chief for Simply Psychology
BSc (Hons) Psychology, MRes, PhD, University of Manchester
Saul McLeod, PhD., is a qualified psychology teacher with over 18 years of experience in further and higher education. He has been published in peer-reviewed journals, including the Journal of Clinical Psychology.
Learn about our Editorial Process
Olivia Guy-Evans, MSc
Associate Editor for Simply Psychology
BSc (Hons) Psychology, MSc Psychology of Education
Olivia Guy-Evans is a writer and associate editor for Simply Psychology. She has previously worked in healthcare and educational sectors.
Research methods in psychology are systematic procedures used to observe, describe, predict, and explain behavior and mental processes. They include experiments, surveys, case studies, and naturalistic observations, ensuring data collection is objective and reliable to understand and explain psychological phenomena.
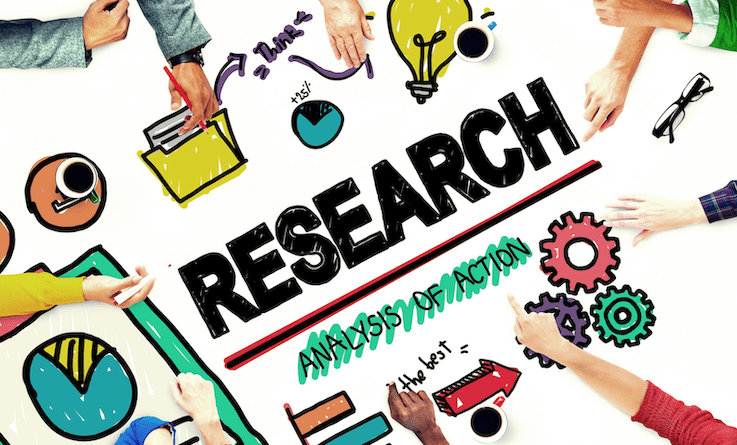
Hypotheses are statements about the prediction of the results, that can be verified or disproved by some investigation.
There are four types of hypotheses :
- Null Hypotheses (H0 ) – these predict that no difference will be found in the results between the conditions. Typically these are written ‘There will be no difference…’
- Alternative Hypotheses (Ha or H1) – these predict that there will be a significant difference in the results between the two conditions. This is also known as the experimental hypothesis.
- One-tailed (directional) hypotheses – these state the specific direction the researcher expects the results to move in, e.g. higher, lower, more, less. In a correlation study, the predicted direction of the correlation can be either positive or negative.
- Two-tailed (non-directional) hypotheses – these state that a difference will be found between the conditions of the independent variable but does not state the direction of a difference or relationship. Typically these are always written ‘There will be a difference ….’
All research has an alternative hypothesis (either a one-tailed or two-tailed) and a corresponding null hypothesis.
Once the research is conducted and results are found, psychologists must accept one hypothesis and reject the other.
So, if a difference is found, the Psychologist would accept the alternative hypothesis and reject the null. The opposite applies if no difference is found.
Sampling techniques
Sampling is the process of selecting a representative group from the population under study.
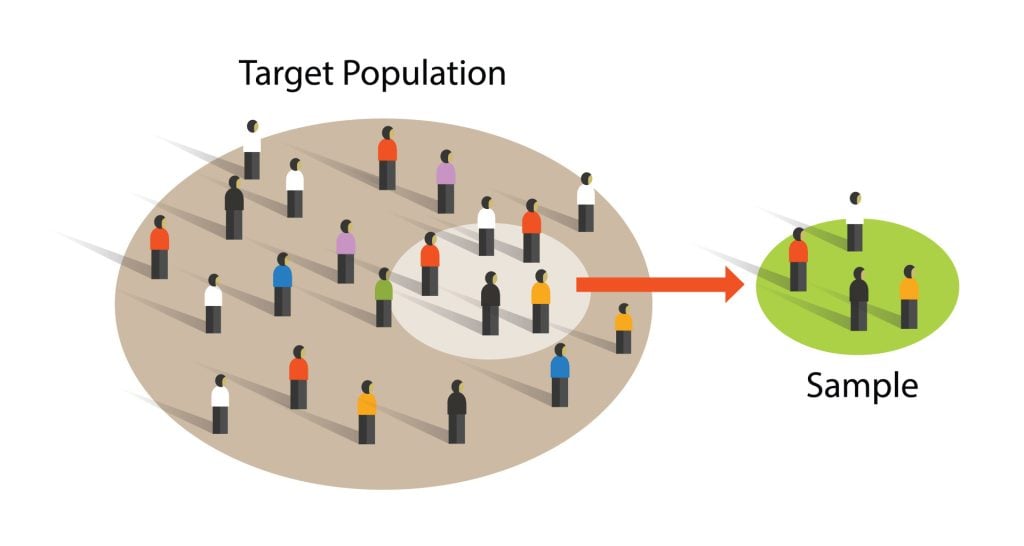
A sample is the participants you select from a target population (the group you are interested in) to make generalizations about.
Representative means the extent to which a sample mirrors a researcher’s target population and reflects its characteristics.
Generalisability means the extent to which their findings can be applied to the larger population of which their sample was a part.
- Volunteer sample : where participants pick themselves through newspaper adverts, noticeboards or online.
- Opportunity sampling : also known as convenience sampling , uses people who are available at the time the study is carried out and willing to take part. It is based on convenience.
- Random sampling : when every person in the target population has an equal chance of being selected. An example of random sampling would be picking names out of a hat.
- Systematic sampling : when a system is used to select participants. Picking every Nth person from all possible participants. N = the number of people in the research population / the number of people needed for the sample.
- Stratified sampling : when you identify the subgroups and select participants in proportion to their occurrences.
- Snowball sampling : when researchers find a few participants, and then ask them to find participants themselves and so on.
- Quota sampling : when researchers will be told to ensure the sample fits certain quotas, for example they might be told to find 90 participants, with 30 of them being unemployed.
Experiments always have an independent and dependent variable .
- The independent variable is the one the experimenter manipulates (the thing that changes between the conditions the participants are placed into). It is assumed to have a direct effect on the dependent variable.
- The dependent variable is the thing being measured, or the results of the experiment.
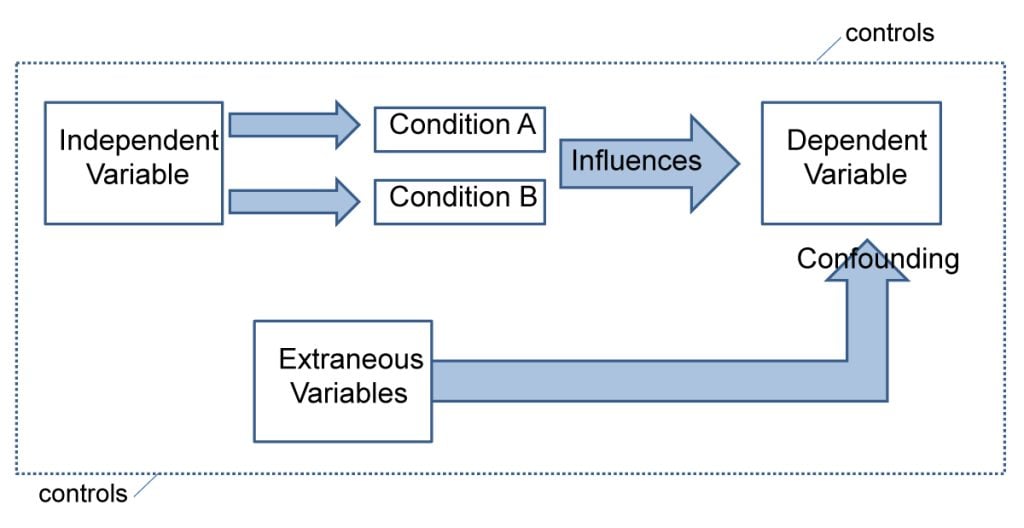
Operationalization of variables means making them measurable/quantifiable. We must use operationalization to ensure that variables are in a form that can be easily tested.
For instance, we can’t really measure ‘happiness’, but we can measure how many times a person smiles within a two-hour period.
By operationalizing variables, we make it easy for someone else to replicate our research. Remember, this is important because we can check if our findings are reliable.
Extraneous variables are all variables which are not independent variable but could affect the results of the experiment.
It can be a natural characteristic of the participant, such as intelligence levels, gender, or age for example, or it could be a situational feature of the environment such as lighting or noise.
Demand characteristics are a type of extraneous variable that occurs if the participants work out the aims of the research study, they may begin to behave in a certain way.
For example, in Milgram’s research , critics argued that participants worked out that the shocks were not real and they administered them as they thought this was what was required of them.
Extraneous variables must be controlled so that they do not affect (confound) the results.
Randomly allocating participants to their conditions or using a matched pairs experimental design can help to reduce participant variables.
Situational variables are controlled by using standardized procedures, ensuring every participant in a given condition is treated in the same way
Experimental Design
Experimental design refers to how participants are allocated to each condition of the independent variable, such as a control or experimental group.
- Independent design ( between-groups design ): each participant is selected for only one group. With the independent design, the most common way of deciding which participants go into which group is by means of randomization.
- Matched participants design : each participant is selected for only one group, but the participants in the two groups are matched for some relevant factor or factors (e.g. ability; sex; age).
- Repeated measures design ( within groups) : each participant appears in both groups, so that there are exactly the same participants in each group.
- The main problem with the repeated measures design is that there may well be order effects. Their experiences during the experiment may change the participants in various ways.
- They may perform better when they appear in the second group because they have gained useful information about the experiment or about the task. On the other hand, they may perform less well on the second occasion because of tiredness or boredom.
- Counterbalancing is the best way of preventing order effects from disrupting the findings of an experiment, and involves ensuring that each condition is equally likely to be used first and second by the participants.
If we wish to compare two groups with respect to a given independent variable, it is essential to make sure that the two groups do not differ in any other important way.
Experimental Methods
All experimental methods involve an iv (independent variable) and dv (dependent variable)..
The researcher decides where the experiment will take place, at what time, with which participants, in what circumstances, using a standardized procedure.
- Field experiments are conducted in the everyday (natural) environment of the participants. The experimenter still manipulates the IV, but in a real-life setting. It may be possible to control extraneous variables, though such control is more difficult than in a lab experiment.
- Natural experiments are when a naturally occurring IV is investigated that isn’t deliberately manipulated, it exists anyway. Participants are not randomly allocated, and the natural event may only occur rarely.
Case studies are in-depth investigations of a person, group, event, or community. It uses information from a range of sources, such as from the person concerned and also from their family and friends.
Many techniques may be used such as interviews, psychological tests, observations and experiments. Case studies are generally longitudinal: in other words, they follow the individual or group over an extended period of time.
Case studies are widely used in psychology and among the best-known ones carried out were by Sigmund Freud . He conducted very detailed investigations into the private lives of his patients in an attempt to both understand and help them overcome their illnesses.
Case studies provide rich qualitative data and have high levels of ecological validity. However, it is difficult to generalize from individual cases as each one has unique characteristics.
Correlational Studies
Correlation means association; it is a measure of the extent to which two variables are related. One of the variables can be regarded as the predictor variable with the other one as the outcome variable.
Correlational studies typically involve obtaining two different measures from a group of participants, and then assessing the degree of association between the measures.
The predictor variable can be seen as occurring before the outcome variable in some sense. It is called the predictor variable, because it forms the basis for predicting the value of the outcome variable.
Relationships between variables can be displayed on a graph or as a numerical score called a correlation coefficient.
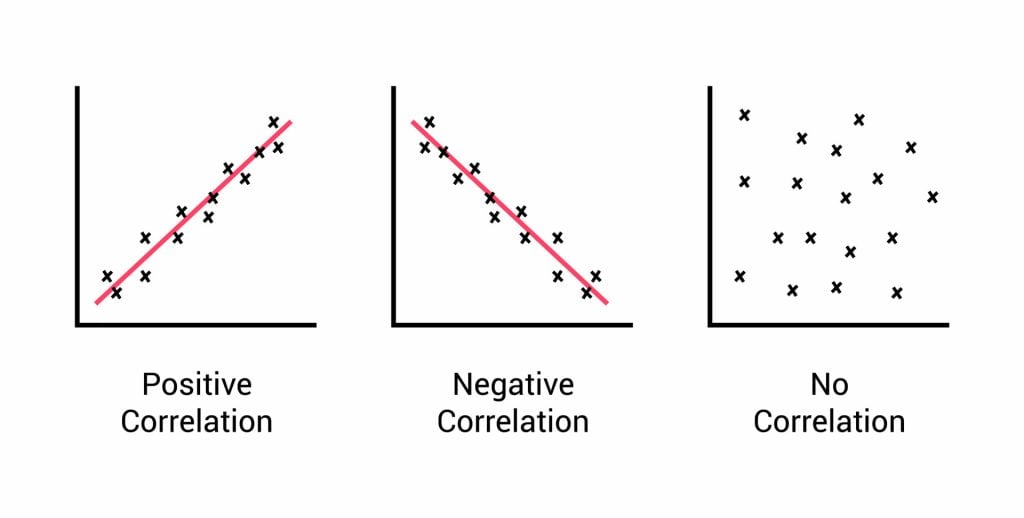
- If an increase in one variable tends to be associated with an increase in the other, then this is known as a positive correlation .
- If an increase in one variable tends to be associated with a decrease in the other, then this is known as a negative correlation .
- A zero correlation occurs when there is no relationship between variables.
After looking at the scattergraph, if we want to be sure that a significant relationship does exist between the two variables, a statistical test of correlation can be conducted, such as Spearman’s rho.
The test will give us a score, called a correlation coefficient . This is a value between 0 and 1, and the closer to 1 the score is, the stronger the relationship between the variables. This value can be both positive e.g. 0.63, or negative -0.63.
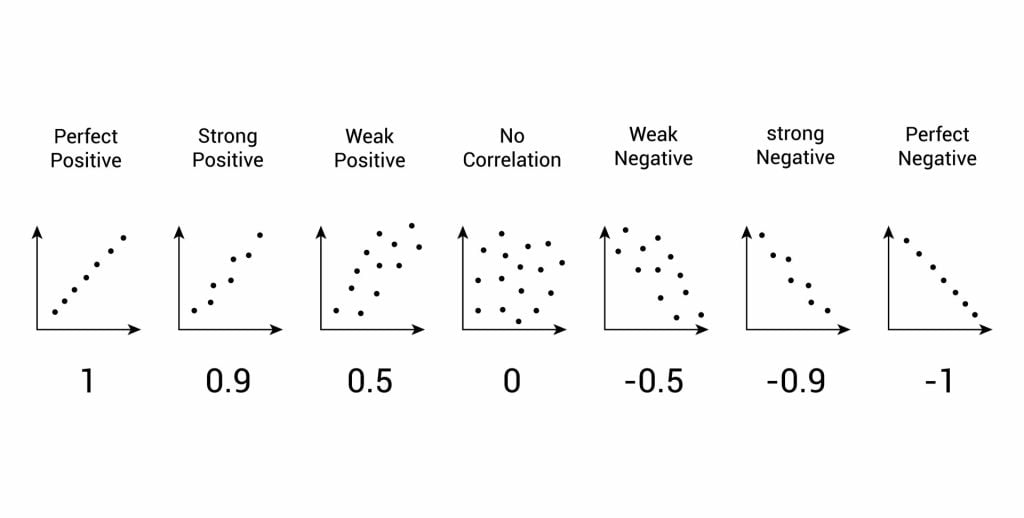
A correlation between variables, however, does not automatically mean that the change in one variable is the cause of the change in the values of the other variable. A correlation only shows if there is a relationship between variables.
Correlation does not always prove causation, as a third variable may be involved.
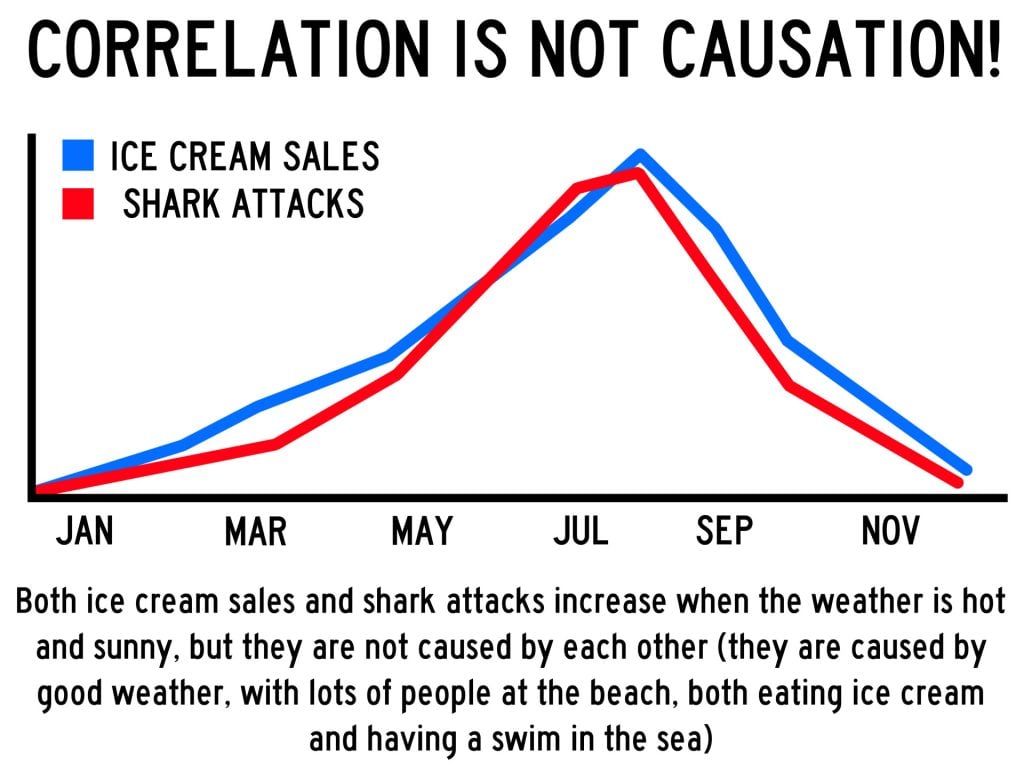
Interview Methods
Interviews are commonly divided into two types: structured and unstructured.
A fixed, predetermined set of questions is put to every participant in the same order and in the same way.
Responses are recorded on a questionnaire, and the researcher presets the order and wording of questions, and sometimes the range of alternative answers.
The interviewer stays within their role and maintains social distance from the interviewee.
There are no set questions, and the participant can raise whatever topics he/she feels are relevant and ask them in their own way. Questions are posed about participants’ answers to the subject
Unstructured interviews are most useful in qualitative research to analyze attitudes and values.
Though they rarely provide a valid basis for generalization, their main advantage is that they enable the researcher to probe social actors’ subjective point of view.
Questionnaire Method
Questionnaires can be thought of as a kind of written interview. They can be carried out face to face, by telephone, or post.
The choice of questions is important because of the need to avoid bias or ambiguity in the questions, ‘leading’ the respondent or causing offense.
- Open questions are designed to encourage a full, meaningful answer using the subject’s own knowledge and feelings. They provide insights into feelings, opinions, and understanding. Example: “How do you feel about that situation?”
- Closed questions can be answered with a simple “yes” or “no” or specific information, limiting the depth of response. They are useful for gathering specific facts or confirming details. Example: “Do you feel anxious in crowds?”
Its other practical advantages are that it is cheaper than face-to-face interviews and can be used to contact many respondents scattered over a wide area relatively quickly.
Observations
There are different types of observation methods :
- Covert observation is where the researcher doesn’t tell the participants they are being observed until after the study is complete. There could be ethical problems or deception and consent with this particular observation method.
- Overt observation is where a researcher tells the participants they are being observed and what they are being observed for.
- Controlled : behavior is observed under controlled laboratory conditions (e.g., Bandura’s Bobo doll study).
- Natural : Here, spontaneous behavior is recorded in a natural setting.
- Participant : Here, the observer has direct contact with the group of people they are observing. The researcher becomes a member of the group they are researching.
- Non-participant (aka “fly on the wall): The researcher does not have direct contact with the people being observed. The observation of participants’ behavior is from a distance
Pilot Study
A pilot study is a small scale preliminary study conducted in order to evaluate the feasibility of the key s teps in a future, full-scale project.
A pilot study is an initial run-through of the procedures to be used in an investigation; it involves selecting a few people and trying out the study on them. It is possible to save time, and in some cases, money, by identifying any flaws in the procedures designed by the researcher.
A pilot study can help the researcher spot any ambiguities (i.e. unusual things) or confusion in the information given to participants or problems with the task devised.
Sometimes the task is too hard, and the researcher may get a floor effect, because none of the participants can score at all or can complete the task – all performances are low.
The opposite effect is a ceiling effect, when the task is so easy that all achieve virtually full marks or top performances and are “hitting the ceiling”.
Research Design
In cross-sectional research , a researcher compares multiple segments of the population at the same time
Sometimes, we want to see how people change over time, as in studies of human development and lifespan. Longitudinal research is a research design in which data-gathering is administered repeatedly over an extended period of time.
In cohort studies , the participants must share a common factor or characteristic such as age, demographic, or occupation. A cohort study is a type of longitudinal study in which researchers monitor and observe a chosen population over an extended period.
Triangulation means using more than one research method to improve the study’s validity.
Reliability
Reliability is a measure of consistency, if a particular measurement is repeated and the same result is obtained then it is described as being reliable.
- Test-retest reliability : assessing the same person on two different occasions which shows the extent to which the test produces the same answers.
- Inter-observer reliability : the extent to which there is an agreement between two or more observers.
Meta-Analysis
Meta-analysis is a statistical procedure used to combine and synthesize findings from multiple independent studies to estimate the average effect size for a particular research question.
Meta-analysis goes beyond traditional narrative reviews by using statistical methods to integrate the results of several studies, leading to a more objective appraisal of the evidence.
This is done by looking through various databases, and then decisions are made about what studies are to be included/excluded.
- Strengths : Increases the conclusions’ validity as they’re based on a wider range.
- Weaknesses : Research designs in studies can vary, so they are not truly comparable.
Peer Review
A researcher submits an article to a journal. The choice of the journal may be determined by the journal’s audience or prestige.
The journal selects two or more appropriate experts (psychologists working in a similar field) to peer review the article without payment. The peer reviewers assess: the methods and designs used, originality of the findings, the validity of the original research findings and its content, structure and language.
Feedback from the reviewer determines whether the article is accepted. The article may be: Accepted as it is, accepted with revisions, sent back to the author to revise and re-submit or rejected without the possibility of submission.
The editor makes the final decision whether to accept or reject the research report based on the reviewers comments/ recommendations.
Peer review is important because it prevent faulty data from entering the public domain, it provides a way of checking the validity of findings and the quality of the methodology and is used to assess the research rating of university departments.
Peer reviews may be an ideal, whereas in practice there are lots of problems. For example, it slows publication down and may prevent unusual, new work being published. Some reviewers might use it as an opportunity to prevent competing researchers from publishing work.
Some people doubt whether peer review can really prevent the publication of fraudulent research.
The advent of the internet means that a lot of research and academic comment is being published without official peer reviews than before, though systems are evolving on the internet where everyone really has a chance to offer their opinions and police the quality of research.
Types of Data
- Quantitative data is numerical data e.g. reaction time or number of mistakes. It represents how much or how long, how many there are of something. A tally of behavioral categories and closed questions in a questionnaire collect quantitative data.
- Qualitative data is virtually any type of information that can be observed and recorded that is not numerical in nature and can be in the form of written or verbal communication. Open questions in questionnaires and accounts from observational studies collect qualitative data.
- Primary data is first-hand data collected for the purpose of the investigation.
- Secondary data is information that has been collected by someone other than the person who is conducting the research e.g. taken from journals, books or articles.
Validity means how well a piece of research actually measures what it sets out to, or how well it reflects the reality it claims to represent.
Validity is whether the observed effect is genuine and represents what is actually out there in the world.
- Concurrent validity is the extent to which a psychological measure relates to an existing similar measure and obtains close results. For example, a new intelligence test compared to an established test.
- Face validity : does the test measure what it’s supposed to measure ‘on the face of it’. This is done by ‘eyeballing’ the measuring or by passing it to an expert to check.
- Ecological validit y is the extent to which findings from a research study can be generalized to other settings / real life.
- Temporal validity is the extent to which findings from a research study can be generalized to other historical times.
Features of Science
- Paradigm – A set of shared assumptions and agreed methods within a scientific discipline.
- Paradigm shift – The result of the scientific revolution: a significant change in the dominant unifying theory within a scientific discipline.
- Objectivity – When all sources of personal bias are minimised so not to distort or influence the research process.
- Empirical method – Scientific approaches that are based on the gathering of evidence through direct observation and experience.
- Replicability – The extent to which scientific procedures and findings can be repeated by other researchers.
- Falsifiability – The principle that a theory cannot be considered scientific unless it admits the possibility of being proved untrue.
Statistical Testing
A significant result is one where there is a low probability that chance factors were responsible for any observed difference, correlation, or association in the variables tested.
If our test is significant, we can reject our null hypothesis and accept our alternative hypothesis.
If our test is not significant, we can accept our null hypothesis and reject our alternative hypothesis. A null hypothesis is a statement of no effect.
In Psychology, we use p < 0.05 (as it strikes a balance between making a type I and II error) but p < 0.01 is used in tests that could cause harm like introducing a new drug.
A type I error is when the null hypothesis is rejected when it should have been accepted (happens when a lenient significance level is used, an error of optimism).
A type II error is when the null hypothesis is accepted when it should have been rejected (happens when a stringent significance level is used, an error of pessimism).
Ethical Issues
- Informed consent is when participants are able to make an informed judgment about whether to take part. It causes them to guess the aims of the study and change their behavior.
- To deal with it, we can gain presumptive consent or ask them to formally indicate their agreement to participate but it may invalidate the purpose of the study and it is not guaranteed that the participants would understand.
- Deception should only be used when it is approved by an ethics committee, as it involves deliberately misleading or withholding information. Participants should be fully debriefed after the study but debriefing can’t turn the clock back.
- All participants should be informed at the beginning that they have the right to withdraw if they ever feel distressed or uncomfortable.
- It causes bias as the ones that stayed are obedient and some may not withdraw as they may have been given incentives or feel like they’re spoiling the study. Researchers can offer the right to withdraw data after participation.
- Participants should all have protection from harm . The researcher should avoid risks greater than those experienced in everyday life and they should stop the study if any harm is suspected. However, the harm may not be apparent at the time of the study.
- Confidentiality concerns the communication of personal information. The researchers should not record any names but use numbers or false names though it may not be possible as it is sometimes possible to work out who the researchers were.

- Privacy Policy

Home » Research Methods – Types, Examples and Guide
Research Methods – Types, Examples and Guide
Table of Contents

Research Methods
Definition:
Research Methods refer to the techniques, procedures, and processes used by researchers to collect , analyze, and interpret data in order to answer research questions or test hypotheses. The methods used in research can vary depending on the research questions, the type of data that is being collected, and the research design.
Types of Research Methods
Types of Research Methods are as follows:
Qualitative research Method
Qualitative research methods are used to collect and analyze non-numerical data. This type of research is useful when the objective is to explore the meaning of phenomena, understand the experiences of individuals, or gain insights into complex social processes. Qualitative research methods include interviews, focus groups, ethnography, and content analysis.
Quantitative Research Method
Quantitative research methods are used to collect and analyze numerical data. This type of research is useful when the objective is to test a hypothesis, determine cause-and-effect relationships, and measure the prevalence of certain phenomena. Quantitative research methods include surveys, experiments, and secondary data analysis.
Mixed Method Research
Mixed Method Research refers to the combination of both qualitative and quantitative research methods in a single study. This approach aims to overcome the limitations of each individual method and to provide a more comprehensive understanding of the research topic. This approach allows researchers to gather both quantitative data, which is often used to test hypotheses and make generalizations about a population, and qualitative data, which provides a more in-depth understanding of the experiences and perspectives of individuals.
Key Differences Between Research Methods
The following Table shows the key differences between Quantitative, Qualitative and Mixed Research Methods
Research Method | Quantitative | Qualitative | Mixed Methods |
---|---|---|---|
To measure and quantify variables | To understand the meaning and complexity of phenomena | To integrate both quantitative and qualitative approaches | |
Typically focused on testing hypotheses and determining cause and effect relationships | Typically exploratory and focused on understanding the subjective experiences and perspectives of participants | Can be either, depending on the research design | |
Usually involves standardized measures or surveys administered to large samples | Often involves in-depth interviews, observations, or analysis of texts or other forms of data | Usually involves a combination of quantitative and qualitative methods | |
Typically involves statistical analysis to identify patterns and relationships in the data | Typically involves thematic analysis or other qualitative methods to identify themes and patterns in the data | Usually involves both quantitative and qualitative analysis | |
Can provide precise, objective data that can be generalized to a larger population | Can provide rich, detailed data that can help understand complex phenomena in depth | Can combine the strengths of both quantitative and qualitative approaches | |
May not capture the full complexity of phenomena, and may be limited by the quality of the measures used | May be subjective and may not be generalizable to larger populations | Can be time-consuming and resource-intensive, and may require specialized skills | |
Typically focused on testing hypotheses and determining cause-and-effect relationships | Surveys, experiments, correlational studies | Interviews, focus groups, ethnography | Sequential explanatory design, convergent parallel design, explanatory sequential design |
Examples of Research Methods
Examples of Research Methods are as follows:
Qualitative Research Example:
A researcher wants to study the experience of cancer patients during their treatment. They conduct in-depth interviews with patients to gather data on their emotional state, coping mechanisms, and support systems.
Quantitative Research Example:
A company wants to determine the effectiveness of a new advertisement campaign. They survey a large group of people, asking them to rate their awareness of the product and their likelihood of purchasing it.
Mixed Research Example:
A university wants to evaluate the effectiveness of a new teaching method in improving student performance. They collect both quantitative data (such as test scores) and qualitative data (such as feedback from students and teachers) to get a complete picture of the impact of the new method.
Applications of Research Methods
Research methods are used in various fields to investigate, analyze, and answer research questions. Here are some examples of how research methods are applied in different fields:
- Psychology : Research methods are widely used in psychology to study human behavior, emotions, and mental processes. For example, researchers may use experiments, surveys, and observational studies to understand how people behave in different situations, how they respond to different stimuli, and how their brains process information.
- Sociology : Sociologists use research methods to study social phenomena, such as social inequality, social change, and social relationships. Researchers may use surveys, interviews, and observational studies to collect data on social attitudes, beliefs, and behaviors.
- Medicine : Research methods are essential in medical research to study diseases, test new treatments, and evaluate their effectiveness. Researchers may use clinical trials, case studies, and laboratory experiments to collect data on the efficacy and safety of different medical treatments.
- Education : Research methods are used in education to understand how students learn, how teachers teach, and how educational policies affect student outcomes. Researchers may use surveys, experiments, and observational studies to collect data on student performance, teacher effectiveness, and educational programs.
- Business : Research methods are used in business to understand consumer behavior, market trends, and business strategies. Researchers may use surveys, focus groups, and observational studies to collect data on consumer preferences, market trends, and industry competition.
- Environmental science : Research methods are used in environmental science to study the natural world and its ecosystems. Researchers may use field studies, laboratory experiments, and observational studies to collect data on environmental factors, such as air and water quality, and the impact of human activities on the environment.
- Political science : Research methods are used in political science to study political systems, institutions, and behavior. Researchers may use surveys, experiments, and observational studies to collect data on political attitudes, voting behavior, and the impact of policies on society.
Purpose of Research Methods
Research methods serve several purposes, including:
- Identify research problems: Research methods are used to identify research problems or questions that need to be addressed through empirical investigation.
- Develop hypotheses: Research methods help researchers develop hypotheses, which are tentative explanations for the observed phenomenon or relationship.
- Collect data: Research methods enable researchers to collect data in a systematic and objective way, which is necessary to test hypotheses and draw meaningful conclusions.
- Analyze data: Research methods provide tools and techniques for analyzing data, such as statistical analysis, content analysis, and discourse analysis.
- Test hypotheses: Research methods allow researchers to test hypotheses by examining the relationships between variables in a systematic and controlled manner.
- Draw conclusions : Research methods facilitate the drawing of conclusions based on empirical evidence and help researchers make generalizations about a population based on their sample data.
- Enhance understanding: Research methods contribute to the development of knowledge and enhance our understanding of various phenomena and relationships, which can inform policy, practice, and theory.
When to Use Research Methods
Research methods are used when you need to gather information or data to answer a question or to gain insights into a particular phenomenon.
Here are some situations when research methods may be appropriate:
- To investigate a problem : Research methods can be used to investigate a problem or a research question in a particular field. This can help in identifying the root cause of the problem and developing solutions.
- To gather data: Research methods can be used to collect data on a particular subject. This can be done through surveys, interviews, observations, experiments, and more.
- To evaluate programs : Research methods can be used to evaluate the effectiveness of a program, intervention, or policy. This can help in determining whether the program is meeting its goals and objectives.
- To explore new areas : Research methods can be used to explore new areas of inquiry or to test new hypotheses. This can help in advancing knowledge in a particular field.
- To make informed decisions : Research methods can be used to gather information and data to support informed decision-making. This can be useful in various fields such as healthcare, business, and education.
Advantages of Research Methods
Research methods provide several advantages, including:
- Objectivity : Research methods enable researchers to gather data in a systematic and objective manner, minimizing personal biases and subjectivity. This leads to more reliable and valid results.
- Replicability : A key advantage of research methods is that they allow for replication of studies by other researchers. This helps to confirm the validity of the findings and ensures that the results are not specific to the particular research team.
- Generalizability : Research methods enable researchers to gather data from a representative sample of the population, allowing for generalizability of the findings to a larger population. This increases the external validity of the research.
- Precision : Research methods enable researchers to gather data using standardized procedures, ensuring that the data is accurate and precise. This allows researchers to make accurate predictions and draw meaningful conclusions.
- Efficiency : Research methods enable researchers to gather data efficiently, saving time and resources. This is especially important when studying large populations or complex phenomena.
- Innovation : Research methods enable researchers to develop new techniques and tools for data collection and analysis, leading to innovation and advancement in the field.
About the author
Muhammad Hassan
Researcher, Academic Writer, Web developer
You may also like

Case Study – Methods, Examples and Guide

Conceptual Framework – Types, Methodology and...

Research Contribution – Thesis Guide

One-to-One Interview – Methods and Guide

Research Questions – Types, Examples and Writing...

Descriptive Research Design – Types, Methods and...
- Open access
- Published: 26 August 2024
Solid health care waste management practice in Ethiopia, a convergent mixed method study
- Yeshanew Ayele Tiruneh 1 ,
- L. M. Modiba 2 &
- S. M. Zuma 2
BMC Health Services Research volume 24 , Article number: 985 ( 2024 ) Cite this article
Metrics details
Introduction
Healthcare waste is any waste generated by healthcare facilities that is considered potentially hazardous to health. Solid healthcare waste is categorized into infectious and non-infectious wastes. Infectious waste is material suspected of containing pathogens and potentially causing disease. Non-infectious waste includes wastes that have not been in contact with infectious agents, hazardous chemicals, or radioactive substances, similar to household waste, i.e. plastic, papers and leftover foods.
This study aimed to investigate solid healthcare waste management practices and develop guidelines to improve solid healthcare waste management practices in Ethiopia. The setting was all health facilities found in Hossaena town.
A mixed-method study design was used. For the qualitative phase of this study, eight FGDs were conducted from 4 government health facilities, one FGD from each private health facility (which is 37 in number), and forty-five FGDs were conducted. Four FGDs were executed with cleaners; another four were only health care providers because using homogeneous groups promotes discussion. The remaining 37 FGDs in private health facilities were mixed from health professionals and cleaners because of the number of workers in the private facilities. For the quantitative phase, all health facilities and health facility workers who have direct contact with healthcare waste management practice participated in this study. Both qualitative and quantitative study participants were taken from the health facilities found in Hossaena town.
Seventeen (3.1%) health facility workers have hand washing facilities. Three hundred ninety-two (72.6%) of the participants agree on the availability of one or more personal protective equipment (PPE) in the facility ‘‘ the reason for the absence of some of the PPEs, like boots and goggles, and the shortage of disposable gloves owes to cost inflation from time to time and sometimes absent from the market’’ . The observational finding shows that colour-coded waste bins are available in 23 (9.6%) rooms. 90% of the sharp containers were reusable, and 100% of the waste storage bins were plastic buckets that were easily cleanable. In 40 (97.56%) health facilities, infectious wastes were collected daily from the waste generation areas to the final disposal points. Two hundred seventy-one (50.2%) of the respondents were satisfied or agreed that satisfactory procedures are available in case of an accident. Only 220 (40.8%) respondents were vaccinated for the Hepatitis B virus.
Hand washing facilities, personal protective equipment and preventive vaccinations are not readily available for health workers. Solid waste segregation practices are poor and showed that solid waste management practices (SWMP) are below the acceptable level.
Peer Review reports
Healthcare waste (HCW) encompasses all types of waste generated while providing health-related services, spanning activities such as diagnosis, immunization, treatment, and research. It constitutes a diverse array of materials, each presenting potential hazards to health and the environment. Within the realm of HCW, one finds secretions and excretions from humans, cultures, and waste containing a stock of infectious agents. Discarded plastic materials contaminated with blood or other bodily fluids, pathological wastes, and discarded medical equipment are classified as healthcare waste. Sharps, including needles, scalpels, and other waste materials generated during any healthcare service provision, are also considered potentially hazardous to health [ 1 ].
Healthcare waste in solid form (HCW) is commonly divided into two primary groups: infectious and non-infectious. The existence of pathogens in concentrations identifies infectious waste or amounts significant enough to induce diseases in vulnerable hosts [ 1 ] If healthcare facility waste is free from any combination with infectious agents, nearly 85% is categorized as non-hazardous waste, exhibiting characteristics similar to conventional solid waste found in households [ 2 ]. World Health Organization (WHO) recommends that appropriate colour-coded waste receptacles be available in all medical and other waste-producing areas [ 3 ].
Solid waste produced in the course of healthcare activities carries a higher potential for infection and injury than any other type of waste. Improper disposal of sharps waste increases the risk of disease transmission among health facility workers and general populations [ 1 ]. Inadequate and inappropriate handling of healthcare waste may have serious public health consequences and a significant environmental impact. The World Health Organization (2014) guidelines also include the following guidance for hand washing and the use of alcohol-based hand rubs: Wash hands before starting work, before entering an operating theatre, before eating, after touching contaminated objects, after using a toilet, and in all cases where hands are visibly soiled [ 4 ].
Among the infectious waste category, sharps waste is the most hazardous waste because of its ability to puncture the skin and cause infection [ 3 ]. Accidents or occurrences, such as near misses, spills, container damage, improper waste segregation, and incidents involving sharps, must be reported promptly to the waste management officer or an assigned representative [ 5 ].
Africa is facing a growing waste management crisis. While the volumes of waste generated in Africa are relatively small compared to developed regions, the mismanagement of waste in Africa already impacts human and environmental health. Infectious waste management has always remained a neglected public health problem in developing countries, resulting in a high burden of environmental pollution affecting the general masses. In Ethiopia, there is no updated separate regulation specific to healthcare waste management in the country to enforce the proper management of solid HCW [ 6 ].
In Ethiopia, like other developing countries, healthcare waste segregation practice was not given attention and did not meet the minimum HCWM standards, and it is still not jumped from paper. Previous study reveals that healthcare waste generation rates are significantly higher than the World Health Organization threshold, which ranges from 29.5–53.12% [ 7 , 8 ]. In Meneilk II Hospital, the proportion of infectious waste was 53.73%, and in the southern and northern parts of Ethiopia, it was 34.3 and 53%, respectively. Generally, this figure shows a value 3 to 4 times greater than the threshold value recommended by the World Health Organization [ 7 ].
Except for sharp wastes, segregation practice was poor, and all solid wastes were collected without respecting the colour-coded waste disposal system [ 9 ]. The median waste generation rate was found to vary from 0.361- 0.669 kg/patient/day, comprising 58.69% non-hazardous and 41.31% hazardous wastes. The amount of waste generated increased as the number of patients flow increased. Public hospitals generated a high proportion of total healthcare waste (59.22%) in comparison with private hospitals (40.48) [ 10 ]. The primary SHCW treatment and disposal mechanism was incineration, open burning, burring into unprotected pits and open dumping on municipal dumping sites as well as in the hospital backyard. Carelessness, negligence of the health workers, patients and cleaners, and poor commitment of the facility leaders were among the major causes of poor HCWM practice in Ethiopia [ 9 ]. This study aimed to investigate solid healthcare waste management practices and develop guidelines to improve solid healthcare waste management practices in Ethiopia.
The setting for this study was all health facilities found in Hossaena town, which is situated 232 kms from the capital city of Ethiopia, Addis Ababa, and 165 kms from the regional municipality of Hawasa. The health facilities found in the town were one university hospital, one private surgical centre, three government health centres, 17 medium clinics, and 19 small clinics were available in the city and; health facility workers who have direct contact with generating and disposal of HCW and those who are responsible as a manager of health facilities found in Hossaena town are the study settings. All health facilities except drug stores and health facility workers who have direct contact with healthcare waste generation participated in this study.
A mixed-method study design was used. For the quantitative part of this study, all healthcare workers who have direct contact with healthcare waste management practice participated in this study, and one focus group discussion from each health facility was used. Both of the study participants were taken from the same population. All health facility workers who have a role in healthcare waste management practice were included in the quantitative part of this study. The qualitative data collection phase used open-ended interviews, focus group discussions, and visual material analysis like posters and written materials. All FGDs were conducted by the principal investigator, one moderator, and one note-taker, and it took 50 to 75 min. 4–6 participants participated in each FGD.
According to Elizabeth (2018: 5), cited by Creswell and Plano (2007: 147), the mixed method is one of the research designs with philosophical assumptions as well as methods of inquiry. As a method, it focuses on collecting, analyzing, and mixing both quantitative and qualitative data in a single study. As a methodology, it involves philosophical assumptions guiding the direction of the collection and analysis and combining qualitative and quantitative approaches in many phases of the research project. The central premise is that using qualitative and quantitative approaches together provides a better understanding of the research problems than either approach alone.
The critical assumption of the concurrent mixed methods approach in this study is that quantitative and qualitative data provide different types of information, often detailed views of participants’ solid waste management practice qualitatively and scores on instruments quantitatively, and together, they yield results that should be the same. In this approach, the researcher collected quantitative and qualitative data almost simultaneously and analyzed them separately to cross-validate or compare whether the findings were similar or different between the qualitative and quantitative information. Concurrent approaches to the data collection process are less time-consuming than other types of mixed methods studies because both data collection processes are conducted on time and at the same visit to the field [ 11 ].
Data collection
The data collection involves collecting both quantitative and qualitative data simultaneously. The quantitative phase of this study assessed three components. Health care waste segregation practice, the availability of waste segregation equipment for HCW segregation, temporary storage facilities, transportation for final disposal, and disposal facilities data were collected using a structured questionnaire and observation of HCW generation. Recycling or re-using practice, waste treatment, the availability of the HCWM committee, and training data were collected.
Qualitative data collection
The qualitative phase of the data collection for this study was employed by using focus group discussions and semi-structured interviews about SHCWMP. Two focus group discussions (FGD) from each health facility were conducted in the government health facilities, one at the administrative level and one at the technical worker level, and one FGD was conducted for all private health facilities because of the number of available health facility workers. Each focus group has 4–6 individuals.
In this study, the qualitative and the quantitative data provide different information, and it is suitable for this study to compare and contrast the findings of the two results to obtain the best understanding of this research problem.
Quantitative data collection
The quantitative data were entered into Epi data version 3.1 to minimize the data entry mistakes and exported to the statistical package for social science SPSS window version 27.0 for analysis. A numeric value was assigned to each response in a database, cleaning the data, recoding, establishing a codebook, and visually inspecting the trends to check whether the data were typically distributed.
Data analysis
Data were analyzed quantitatively by using relevant statistical tools, such as SPSS. Descriptive statistics and the Pearson correlation test were used for the bivariate associations and analysis of variance (ANOVA) to compare the HCW generation rate between private and government health facilities and between clinics, health centres and hospitals in the town. Normality tests were performed to determine whether the sample data were drawn from a normally distributed population.
The Shapiro–Wilk normality tests were used to calculate a test statistic based on the sample data and compare it to critical values. The Shapiro–Wilk test is a statistical test used to assess whether a given sample comes from a normally distributed population. The P value greater than the significance level of 0.05 fails to reject the null hypothesis. It concludes that there is not enough evidence to suggest that the data does not follow the normal distribution. Visual inspection of a histogram, Q-Q plot, and P-P plot (probability-probability plot) was assessed.
Bivariate (correlation) analysis assessed the relationships between independent and dependent variables. Then, multiple linear regression analysis was used to establish the simple correlation matrices between different variables for investigating the strength relationships of the study variables in the analysis. In most variables, percentages and means were used to report the findings with a 95% confidence interval. Open-ended responses and focused group findings were undertaken by quantifying and coding the data to provide a thematic narrative explanation.
Appropriate and scientific care was taken to maintain the data quality before, during, and after data collection by preparing the proper data collection tools, pretesting the data collection tools, providing training for data collectors, and proper data entry practice. Data were cleaned on a daily basis during data collection practice, during data entry, and before analysis of its completeness and consistency.
Data analysis in a concurrent design consists of three phases. First, analyze the quantitative database in terms of statistical results. Second, analyze the qualitative database by coding the data and collapsing the codes into broad themes. Third comes the mixed-method data analysis. This is the analysis that consists of integrating the two databases. This integration consists of merging the results from both the qualitative and the quantitative findings.
Descriptive analysis was conducted to describe and summarise the data obtained from the samples used for this study. Reliability statistics for constructs, means and modes of each item, frequencies and percentage distributions, chi-square test of association, and correlations (Spearman rho) were used to portray the respondents’ responses.
All patient care-providing health facilities were included in this study, and the generation rate of healthcare waste and composition assessed the practice of segregation, collection, transportation, and disposal system was observed quantitatively using adopted and adapted structured questionnaires. To ensure representativeness, various levels of health facilities like hospitals, health centres, medium clinics, small clinics and surgical centres were considered from the town. All levels of health facilities are diagnosing, providing first aid services and treating patients accordingly.
The hospital and surgical centre found in the town provide advanced surgical service, inpatient service and food for the patients that other health facilities do not. The HCW generation rate was proportional to the number of patients who visited the health facilities and the type of service provided. The highest number of patients who visited the health facilities was in NEMMCSH; the service provided was diverse, and the waste generation rate was higher than that of other health facilities. About 272, 18, 15, 17, and 20 average patients visited the health facilities daily in NEMMCSH: government health centres, medium clinics, small clinics, and surgical centres. Paper and cardboard (141.65 kg), leftover food (81.71 kg), and contaminated gloves (42.96 kg) are the leading HCWs generated per day.
A total of 556 individual respondents from sampled health facilities were interviewed to complete the questionnaire. The total number of filled questionnaires was 540 (97.1) from individuals representing these 41 health facilities.
The principal investigator observed the availability of handwashing facilities near SHCW generation sites. 17(3.1%) of health facility workers had hand washing facilities near the health care waste generation and disposal site. Furthermore,10 (3.87%), 2 (2.1%), 2 (2.53%), 2 (2.1%), 1 (6.6%) of health facility workers had the facility of hand washing near the health care waste generation site in Nigist Eleni Mohamed Memorial Comprehensive Specialized Hospital (NEMMCSH), government health centres, medium clinics, small clinics, and surgical centre respectively. This finding was nearly the same as the study findings conducted in Myanmar; the availability of hand washing facilities near the solid health care waste generation was absent in all service areas [ 12 ]. The observational result was convergent with the response of facility workers’ response regarding the availabilities of hand washing facilities near to the solid health care waste generation sites.
The observational result was concurrent with the response of facility workers regarding the availability of hand-washing facilities near the solid health care waste generation sites.
The availability of personal protective equipment (PPE) was checked in this study. Three hundred ninety-two (72.6%) of the respondents agree on the facility’s availability of one or more personal protective equipment (PPE). The availability of PPEs in different levels of health facilities shows 392 (72.6%), 212 (82.2%), 56 (58.9%), 52 (65.8%), 60 (65.2%), 12 (75%) health facility workers in NEMMCSH, government health centres, medium clinics, small clinics, and surgical centres respectively agree to the presence of personal protective equipment in their department. The analysis further shows that the availability of masks for healthcare workers was above the mean in NEMMCSH and surgical centres.
Focus group participants indicated that health facilities did not volunteer to supply Personal protective equipment (PPEs) for the cleaning staff.
“We cannot purchase PPE by ourselves because of the salary paid for the cleaning staff.”
Cost inflation and the high cost of purchasing PPEs like gloves and boots are complained about by all (41) health facility owners.
“the reason for the absence of some of the PPEs like boots, goggles, and shortage of disposable gloves are owing to cost inflation from time to time and sometimes absent from the market is the reason why we do not supply PPE to our workers.”
Using essential personal protective equipment (PPEs) based on the risk (if the risk is a splash of blood or body fluid, use a mask and goggles; if the risk is on foot, use appropriate shoes) is recommended by the World Health Organization [ 13 ]. The mean availability of gloves in health facilities was 343 (63.5% (95% CI: 59.3–67.4). Private health institutions are better at providing gloves for their workers, 67.1%, 72.8%, and 62.5% in medium clinics, small clinics, and surgical centres, respectively, which is above the mean.
Research participants agree that.
‘‘ there is a shortage of gloves to give service in Nigist Eleni Mohamed Memorial Comprehensive Specialized Hospital (NEMMCSH) and government health centres .’’
Masks are the most available personal protective equipment for health facility workers compared to others. 65.4%, 55.6%, and 38% of the staff are available with gloves, plastic aprons and boots, respectively.
The mean availability of masks, heavy-duty gloves, boots, and aprons was 71.1%, 65.4%, 38%, and 44.4% in the study health facilities. Health facility workers were asked about the availability of different personal protective equipment, and 38% of the respondents agreed with the presence of boots in the facility. Still, the qualitative observational findings of this study show that all health facility workers have no shoes or footwear during solid health care waste management practice.
SHCW segregation practice was checked by observing the availability of SHCW collection bins in each patient care room. Only 4 (1.7%) of the room’s SHCW bins are collected segregated (non-infectious wastes segregated in black bins and infectious wastes segregated in yellow bins) based on the World Health Organization standard. Colour-coded waste bins, black for non-infectious and yellow for infectious wastes, were available in 23 (9.6%) rooms. 90% of the sharp containers were reusable, and 100% of the waste storage bins were plastic buckets that were easily cleanable. Only 6.7% of the waste bins were pedal operated and adequately covered, and the rest were fully opened, or a tiny hole was prepared on the container’s cover. All of the healthcare waste disposal bins in each health facility and at all service areas were away from the arm’s reach distance of the waste generation places, and this is contrary to World Health Organization SHCWM guidelines [ 13 ]. The observation result reveals that the reason for the above result was that medication trolleys were not used during medication or while healthcare providers provided any health services to patients.
Most medical wastes are incinerated. Burning solid and regulated medical waste generated by health care creates many problems. Medical waste incinerators emit toxic air pollutants and ash residues that are the primary source of environmental dioxins. Public concerns about incinerator emissions and the creation of federal regulations for medical waste incinerators are causing many healthcare facilities to rethink their choices in medical waste treatment. Health Care Without Harm [ 14 ], states that non-incineration treatment technologies are a growing and developing field. The U.S. National Academy of Science 2000 argued that the emission of pollutants during incineration is a potential risk to human health, and living or working near an incineration facility can have social, economic, and psychological effects [ 15 ].
The incineration of solid healthcare waste technology has been accepted and adopted as an effective method in Ethiopia. Incineration of healthcare waste can produce secondary waste and pollutants if the treatment facilities are not appropriately constructed, designed, and operated. It can be one of the significant sources of toxic substances, such as polychlorinated dibenzo-dioxins/dibenzofurans (PCDD/ PCDF), polyvinyl chloride (PVC), hexachlorobenzenes and polychlorinated biphenyls, and dioxins and furans that are known as hazardous pollutants. These pollutants may have undesirable environmental impacts on human and animal health, such as liver failure and cancer [ 15 , 16 ].
All government health facilities (4 in number) used incineration to dispose of solid waste. 88.4% and 100% of the wastes are incinerated in WUNEMMCSH and government health centres. This finding contradicts the study findings in the United States of America and Malaysia, in which 49–60% and 59–60 were incinerated, respectively, and the rest were treated using other technologies [ 15 , 16 ].
World Health Organization (2014:45) highlighted those critical elements of the appropriate operation of incinerators include effective waste reduction and waste segregation, placing incinerators away from populated areas, satisfactory engineered design, construction following appropriate dimensional plans, proper operation, periodic maintenance, and staff training and management are mandatory.
Solid waste collection times should be fixed and appropriate to the quantity of waste produced in each area of the health care facility. General waste should not be collected simultaneously or in the same trolley as infectious or hazardous wastes. The collection should be done daily for most wastes, with collection timed to match the pattern of waste generation during the day [ 13 ].
SHCW segregation practices were observed for 240 rooms in 41 health facilities that provide health services in the town. In government health centres, medium clinics, small clinics, and surgical centres, SHCW segregation practice was not based on the World Health Organization standard. All types of solid waste were collected in a single container near the generation area, and there were no colour-coded SHCW storage dust bins. Still, in NEMMCSH, in most of the service areas, colour-coded waste bins are available, and the segregation practice was not based on the standard. Only 3 (10%) of the dust bins collected the appropriate wastes according to the World Health Organization standard, and the rest were mixed with infectious and non-infectious SHCW.
Table 1 below shows health facility managers were asked about healthcare waste segregation practices, and 9 (22%) of the facility leaders responded that there is an appropriate solid healthcare waste segregation practice in their health facilities. Still, during observation, only 4 (1.7%) of the rooms in two (4.87%) of the facilities, SHCW bins collected the segregated wastes (non-infectious wastes segregated at the black bin and infectious wastes segregated at yellow bin) based on the world health organization standard. The findings of this study show there is a poor segregation practice, and all kinds of solid wastes are collected together.
In 40 (97.56%) health facilities, infectious wastes were collected daily from the waste generation areas to the final disposal points. During observation in one of the study health facilities, infectious wastes were not collected daily and left for days. Utility gloves, boots, and aprons are not available for cleaning staff to collect and transport solid healthcare wastes in all study health facilities. 29.26% of the facilities’ cleaning staff have a face mask, and 36.5% of the facilities remove waste bins from the service area when 3/4 full, and the rest were not removed or replaced with new ones. There is a separate container only in 2 health facilities for infectious and non-infectious waste segregation practice, and the rest were segregated and collected using single and non-colour coded containers.
At all of the facilities in the study area, SHCW was transported from the service areas to the disposal site were transported manually by carrying the collection container and there is no trolley for transportation. This finding was contrary to the study findings conducted in India, which show segregated waste from the generation site was being transported through the chute to the carts placed at various points on the hospital premises by skilled sanitary workers [ 17 ].
Only 2 out of 41 health facilities have temporary solid waste storage points at the facility. One of the temporary storage places was clean, and the other needed to be properly cleaned and unsightly. Two (100%) of the temporary storage areas are not fenced and have no restriction to an authorized person. Temporary storage areas are available only in two health facilities that are away from the service provision areas.
Observational findings revealed that pre-treatment of SHCW before disposal was not practised at all study health facilities. 95% of the facilities have no water supply for hand washing during and after solid healthcare waste generation, collection, and disposal.
The United States Agency estimated sharp injuries from medical wastes to health professionals and sanitary service personnel for toxic substances and disease registry. Most of the injuries are caused during the recapping of hypodermic needles before disposal into sharps containers [ 13 ]. Nearly half of the respondents, 245 (51.5%), are recapping needles after providing an injection to the patient. Recapping was more practised in NEMMCSH and surgical centres, which is 57.5% and 57.5%, respectively. In government health centres, medium clinics, and surgical centres, the recapping of used needles was practised below the mean, which is 47.9%, 48, and 43.8%, respectively. This finding was reasonable compared to the study findings of Doylo et al. [ 18 ] in western Ethiopia, where 91% of the health workers are recapping needles after injection [ 18 ]. The research finding shows that there is no significant association P-value of 0.82 between the training and recapping of needles after injection.
Focus group participants ’ response for appropriate SHCWMP regarding patients ’ and visitors ’ lack of knowledge on SHCW segregation practice
“The personal responsibilities of patients and visitors on solid HCW disposal should be explained to help appropriate safe waste management practice and maintain good hygiene .” “Providing waste management training and creating awareness are the two aspects of improving SHCW segregation practice.” “Training upgrades and creates awareness on hygiene for all workers.”
Sharp waste collection practices were observed in 240 rooms in the study health facilities, and 9.2% of the rooms used disposable sharp containers.
Sixty per cent (60%), 13.3%, 8.24%, and 15.71% of the sharps containers in NEMMCSH, government health centres, medium clinics, and small clinics, respectively, were using disposable sharps containers; sharps were disposed together with the sharps container, and surgical centre was using reusable sharp collection container. All disposable sharps containers in medium and small clinics used non-puncture-resistant or simple packaging carton boxes. 60% and 13.3% of the disposable sharps containers in NEMMCSH and the government health centre use purposefully manufactured disposable safety boxes.

Needle sticks injury reporting and occurrence
A total of 70 injuries were reported to the health facility manager in the last one year, and 44 of the injuries were reported by health professionals. The rest of the injuries were reported by supportive staff. These injuries were reported from 35 health facilities, and the remaining six health facilities did not report any cases of injury related to work; see Tables 2 and 3 below.
Accidents or incidents, including near misses, spillages, damaged containers, inappropriate segregation, and any incidents involving sharps, should be reported to the waste-management officer. Accidental contamination must be notified using a standard-format document. The cause of the accident or incident should be investigated by the waste-management officer (in case of waste) or another responsible officer, who should also take action to prevent a recurrence [ 13 ]. Two hundred seventy-one (50.2% (CI: 45.7–54.6) of the respondents agree that satisfactory procedures are available in case of an accident, while the remaining 269 (49.8%( CI: 45.4–54.3) of respondents do not agree on the availability of satisfactory procedures in case of an accident, see Table 4 below. The availability of satisfactory procedures in case of an accident is above the mean in medium clinics, which is 60.8%. 132(24.4%) of the staff are pricked by needle stick injury while providing health services. Nearly half of the respondents, 269 (49.8%), who have been exposed to needle stick injury do not get satisfactory procedures after being pricked by a needle, and those who have not been stung by a needle stick injury for the last year. 204 (37.8%) disagree with the presence of satisfactory procedures in the case of a needle stick injury. In NEMMCSH, 30.2% of the research participants were pricked by needle stick injury within one year of period, and 48.8% of those who were stung by needle stick injuries did not agree upon the presence of satisfactory procedures in case of needle stick injuries in the study hospital. 17.9% and 49.5%, 24.1% and 60.8%, 7.6% and 50% of the respondents are pricked by needle sticks, and they disagree on the availability of satisfactory procedures in case of accidents, respectively, in government health centres, medium clinics, small clinics, and surgical centre respectively.
One hundred seventy-seven (32.7% (CI:29.1–37) respondents were exposed to needle stick injury while working in the current health facilities. One hundred three (58.1%) and 26 (32.9%) needle stick injuries were reported from WUNEMMCSH and medium clinics, which is above the mean. One hundred thirty-two(24.7% (95%CI:20.7–28.1) of the respondents are exposed to needle stick injury within one year of the period. Seventy-eight(30.2%), 17 (17.9%), 19 (24.1%), 15 (16.3%), 3 (18.8%) of the staff are injured by needle sticks from NEMMCSH, government health centres, medium clinics, small clinics, and surgical centre staffs respectively within one year of service.
The mean availabilities of satisfactory procedures in case of accidents were 321 (59.4% (CI:55.4–63.7). Out of this, 13.7% of the staff is injured by needle sticks within one year before the survey. Except in NEMMCSH, the mean availabilities of satisfactory procedures were above the mean, which is 50%, 60%, 77.2%, 66.3%, and 81.3% in NEMMCSH, government health centres, medium clinics, small clinics, and surgical centres respectively.
Table 5 below shows that Hepatitis B, COVID-19, and tetanus toxoid vaccinations are the responses of the research participants to an open-ended question on which vaccine they took. The finding shows that 220 (40.8%) of the respondents were vaccinated to prevent themselves from health facility-acquired infection. One hundred fifty-six (70.9%) of the respondents are vaccinated to avoid themselves from Hep B infection. Fifty-nine (26%0.8) of the respondents were vaccinated to protect themselves from two diseases that are Hep B and COVID-19.
Appropriate health care waste management practice was assessed by using 12 questions: availability of colour-coded waste bins, foot-operated dust bins, elbow or foot-operated hand washing basin, personal protective equipment, training, role and responsibility of the worker, the presence of satisfactory procedures in case of an accident, incinerator, vaccination, guideline, onsite treatment, and the availability of poster. The mean of appropriate healthcare waste management practice was 55.58%. The mean of solid health care waste management practice based on the level of health facilities was summed and divided into 12 variables to get each health facility’s level of waste management practice. 64.9%, 45.58%, 49%, 46.9%, and 51.8% are the mean appropriate health care waste management practices in NEMMCSH, government health centres, medium clinics, small clinics, and surgical centres, respectively. In NEMMCSH, the practice of solid healthcare waste management shows above the mean, and the rest was below the mean of solid healthcare waste management practice.
Healthcare waste treatment and disposal practice
Solid waste treatment before disposal was not practised at all study health facilities. There is an incineration practice at all of the study health facilities, and the World Health Organization 2014 recommended three types of incineration practice for solid health care waste management: dual-chamber starved-air incinerators, multiple chamber incinerators, and rotary kilns incinerators. Single-chamber, drum, and brick incinerators do not meet the best available technique requirements of the Stockholm Convention guidelines [ 13 ]. The findings of this study show that none of the incinerators found in the study health facilities meet the minimum standards of solid healthcare waste incineration practice, and they need an air inlet to facilitate combustion. Eleven (26.82%) of the health facilities have an ash pit to dispose of burned SHCW; the majority, 30 (73.17%), dispose of the incinerated ash and burned needles in the municipal waste disposal site. In one out of 11 health facilities with an ash pit, one of the incinerators was built on the ash pit, and the incinerated ashes were disposed of in the ash pit directly. Pre-treatment of SHCW before disposal was not practised at all health facilities; see Table 6 below.
All government health facilities use incineration to dispose of solid waste. 88.4% and 100% of the solid wastes are incinerated in WUNEMMCS Hospital and government health centres, respectively. This finding was not similar to the other studies because other technologies like autoclave microwave and incineration were used for 59–60% of the waste [ 15 ]. Forty-one (100%) of the study facilities were using incinerators, and only 5 (12.19%) of the incinerators were constructed by using brick and more or less promising than others for incinerating the generated solid wastes without considering the emitting gases into the atmosphere and the residue chemicals and minerals in the ashes.
Research participants’ understanding of the environmental friendliness of health care waste management practice was assessed, and the result shows that more than half, 312(57%) of the research participants do not agree on the environmental friendliness of the waste disposal practices in the health facilities. The most disagreement regarding environmental friendliness was observed in NEMMCSH; 100 (38.8%) of the participants only agreed the practice was environmentally friendly of the service. Forty-four (46.3%), 37 (46.8%), 40 (43.5%), and 7 (43.8%) of the participants agree on the environmental friendliness of healthcare waste management practice in government health centres, medium clinics, small clinics, and surgical centres, respectively.
One hundred twenty-five (48.4%) and 39(42.4%) staff are trained in solid health care waste management practice in NEMMCSH and small clinic staff, respectively; this result shows above the mean. Twenty-seven (28.4%), 30 (38%), and 4 (25%) of the staff are trained in health care waste management practice in Government health centres, medium clinics, and surgical centres, respectively. The training has been significantly associated with needle stick injury, and the more trained staff are, the less exposed to needle stick injury. One hundred ninety-six (36.4%) of the participants answered yes to the question about the availability of trainers in the institution. 43.8% of the NEMMCSH staff agreed on the availability of trainers on solid health care waste management, which is above the mean, and 26.3%, 31.6%, 31.5%, and 25% for the government health centres, medium clinics, small clinics, and surgical centre respectively, which is below the mean.
Trained health professionals are more compliant with SHCWM standards, and the self-reported study findings of this study show that 41.7% (95%CI:37.7–46) of the research participants are trained in health care waste management practice. This finding was higher compared to the study findings of Sahiledengle in 2019 in the southeast of Ethiopia, shows 13.0% of healthcare workers received training related to HCWM in the past one year preceding the study period and significantly lower when compared to the study findings in Egypt which is 71% of the study participants were trained on SHCWM [ 8 , 19 , 20 ].
Three out of four government health facility leaders, 17 (45.94%) of private health facility leaders/owners of the clinic and 141 FGD participants complain about the absence of some PPEs like boots and aprons to protect themselves from infectious agents.
‘ ‘Masks, disposable gloves, and changing gowns are a critical shortage at all health facilities.’’
Cleaners in private health facilities are more exposed to infectious agents because of the absence of personal protective equipment. Except for the cleaning staff working in the private surgical centre, all cleaning staff 40 (97.56) of the health facilities complain about the absence of changing gowns and the fact that there are no boots in the facilities.
Cost inflation and the high cost of purchasing PPEs like gloves and boots are complained by all of (41) the health facility owners and the reason for the absence of some of the PPEs like boots, goggles, and shortage of disposable gloves. Sometimes, absence from the market is the reason why we do not supply PPE to our workers.
Thirty-four (82.92%) of the facility leaders are forwarded, and there is a high expense and even unavailability of some of the PPEs, which are the reasons for not providing PPEs for the workers.
‘‘Medical equipment and consumables importers and whole sellers are selective for importing health supplies, and because of a small number of importers in the country and specifically, in the locality, we can’t get materials used for health care waste management practice even disposable gloves. ’’
One of the facility leaders from a private clinic forwarded that before the advent of COVID-19 -19) personal protective equipment was more or less chip-and-get without difficulty. Still, after the advent of the first Japanese COVID-19 patient in Ethiopia, people outside the health facilities collect PPEs like gloves and masks and storing privately in their homes.
‘‘PPEs were getting expensive and unavailable in the market. Incinerator construction materials cost inflation, and the ownership of the facility building are other problems for private health facilities to construct standard incinerators.’’
For all of the focus group discussion participants except in NEMMCSH and two private health facilities, covered and foot-operated dust bins were absent or in a critical shortage compared to the needed ones.
‘‘ Waste bins are open and not colour-coded. The practice attracts flies and other insects. Empty waste bins are replaced without cleaning and disinfecting by using chlorine solution.’’ “HCW containers are not colour-coded, but we are trying to label infectious and non-infectious in Amharic languages.”
Another issue raised during focus group discussions is incineration is not the final disposal method. It needs additional disposal sites, lacks technology, is costly to construct a brick incinerator, lacks knowledge for health facility workers, shortage of man powers /cleaners, absence of environmental health professionals in health centres and all private clinics, and continues exposure to the staff for needle stick injury, foully smell, human scavengers, unsightly, fire hazard, and lack of water supply in the town are the major teams that FGD participants raise and forwarded the above issue as a problem to improve SHCWMP.
Focus group participants, during the discussion, raised issues that could be more comfortable managing SHCWs properly in their institution. Two of the 37 private health facilities are working in their own compound, and the remaining 35 are rented; because of this, they have difficulty constructing incinerators and ash removal pits and are not confident about investing in SHCWM systems. Staff negligence and involuntary abiding by the rules of the facilities were raised by four of the government health facilities, and it was difficult to punish those who violated the healthcare waste management rules because the health facility leaders were not giving appropriate attention to the problem.
Focus group participants forwarded recommendations on which interventions can improve the management of SHCW, and recommendations are summarised as follows:
“PPE should be available in quality and quantity for all health facility workers who have direct contact with SHCW.” “Scientific-based waste management technologies should be availed for health facilities.” “Continuous induction HCW management training should be provided to the workers. Law enforcement should be strengthened.” “Communal HCW management sites should be availed, especially for private health facilities.” “HCWM committee should be strengthened.” “Non-infectious wastes should be collected communally and transported to the municipal SHCW disposal places.” “Leaders should be knowledgeable on the SHCWM system and supervise the practice continuously.” “Patient and client should be oriented daily about HCW segregation practice.” “Regulatory bodies should supervise the health facilities before commencing and periodically between services .”
The above are the themes that FGD participants discussed and forwarded for the future improvements of SHAWMP in the study areas.

Lack of water supply in the town
Other issues raised during FGDs were health facilities’ lack of water supply. World Health Organization (2014: 89) highlights that water supply for the appropriate waste management system should be mandatory at any time in all health service delivery points.
Thirty-nine (95.12%) of the health facilities complain about the absence of water supply to improve HCW management practices and infection prevention and control practices in the facilities.
“We get water once per week, and most of the time, the water is available at night, and if we are not fetching as scheduled, we can’t get water the whole week”.
In this research, only those who have direct contact have participated in this study, and 434 (80.4%) of the respondents agree they have roles and responsibilities for appropriate solid health care waste management practice. The rest, 19.6%, do not agree with their commitment to manage health care wastes properly, even though they are responsible. Health facility workers in NEMMCSH and medium clinics know their responsibilities better than others, and their results show above the mean. 84.5%, 74.5%, 81%, 73.9% and 75% in NEMMCSH, Government health centres, medium clinics, small clinics, and surgical centres, respectively.
Establishing a policy and a legal framework, training personnel, and raising public awareness are essential elements of successful healthcare waste management. A policy can be viewed as a blueprint that drives decision-making at a political level and should mobilize government effort and resources to create the conditions to make changes in healthcare facilities. Three hundred and seventy-four (69.3%) of the respondents agree with the presence of any solid healthcare waste management policy in Ethiopia. The more knowledge above the mean (72.9%) on the presence of the policy is reported from NEMMCSH.
Self-reported level of knowledge on what to do in case of an accident revealed that 438 (81.1% CI: 77.6–84.3%) of the respondents knew what to do in case of an accident. Government health centre staff and medium clinic staff’s knowledge about what to do in case of an accident was above the mean (88.4% and 82.3%), respectively, and the rest were below the mean. The action performed after an occupational accident revealed that 56 (35.7%) of the respondents did nothing after any exposure to an accident. Out of 56 respondents who have done nothing after exposure, 47 (83.92%) of the respondents answered yes to their knowledge about what to do in case of an accident. Out of 157 respondents who have been exposed to occupational accidents, only 59 (37.6%) of the respondents performed the appropriate measures, 18 (11.5%), 9 (5.7%), 26 (16.6%), 6 (3.8%) of the respondents are taking prophylaxis, linked to the incident officer, consult the available doctors near to the department, and test the status of the patient (source of infection) respectively and the rest were not performing the scientific measures, that is only practising one of the following practices washing the affected part, squeezing the affected part to remove blood, cleaning the affected part with alcohol.
Health facility workers’ understanding of solid health care waste management practices was assessed by asking whether the current SHCWM practice needs improvement. Four hundred forty-nine (83.1%) health facility workers are unsatisfied with the current solid waste management practice at the different health facility levels, and they recommend changing it to a scientific one. 82.6%, 87.4%, 89.9%, 75%, and 81.3% of the respondents are uncomfortable or need to improve solid health care waste management practices in NEMMCSH, government health centres, medium clinics, small clinics, and surgical centres, respectively.
Lack of safety box, lack of colour-coded waste bins, lack of training, and no problems are the responses to the question problems encountered in managing SHCWMP. Two Hundred and Fifty (46.92%) and 232 (42.96%) of the respondents recommend the availability of safety boxes and training, respectively.
Four or 9.8% of the facilities have infection prevention and control (IPC) teams in the study health facilities. This finding differed from the study in Pakistan, where thirty per cent (30%) of the study hospitals had HCWM or infection control teams [ 21 ]. This study’s findings were similar to those conducted in Pakistan by Khan et al. [ 21 ], which confirmed that the teams were almost absent at the secondary and primary healthcare levels [ 20 ].
The availability of health care waste management policy report reveals that 69.3% (95% CI: 65.4–73) of the staff are aware of the presence of solid health care waste management policy in the institution. Availability of health care waste management policy was 188 (72.9%), 66 (69.5%), 53 (677.1%), 57 (62%), 10 (62.5%) in NEMMCSH, Government health centres, medium clinics, small clinics, and surgical centre respectively. Healthcare waste management policy availability was above the mean in NEMMCSH and government health centres; see Table 6 below.
Open-ended responses on the SHCWM practice of health facility workers were collected using the prepared interview guide, and the responses were analyzed using thematic analysis. All the answered questions were tallied on the paper and exported to Excel software for thematic analysis.
The study participants recommend.
“appropriate segregation practice at the point of generation” "health facility must avail all the necessary supplies that used for SHCWMP, punishment for those violating the rule of SHCWMP",
“waste management technologies should be included in solid waste management guidelines, and enforcement should be strengthened.”
The availability of written national or adopted/adapted SHCWM policies was observed at all study health facilities. Twenty eight (11.66%) of the rooms have either a poster or a written document of the national policy document. However, all staff working in the observed rooms have yet to see the inside content of the policy. The presence of the policy alone cannot bring change to SHCWMP. This finding shows that the presence of policy in the institution was reasonable compared to the study findings in Menelik II hospital in Addis Ababa, showing that HCWM regulations and any applicable facility-based policy and strategy were not found [ 22 ]. The findings of this study were less compared to the study findings in Pakistan; 41% of the health facilities had the policy document or internal rules for the HCWM [ 21 ].
Focus group participants have forwarded recommendations on which interventions can improve the management of SHCW, and recommendations are summarised as follows.
‘‘Supplies should be available in quality and quantity for all health facility workers with direct contact with SHCW. Scientific-based waste management technologies should be available for health facilities. Continues and induction health care waste management training should be provided to the workers. Law enforcement should be strengthened. Community healthcare waste management sites should be available, especially for private health facilities. HCWM committee should be strengthened. Non-infectious wastes should be collected communally and transported to the municipal SHCW disposal places. Leaders should be knowledgeable about the SHCWM system and supervise the practice continuously. Patients and clients should be oriented daily about health care waste segregation practices. Regulatory bodies should supervise the health facilities before commencing and periodically in between the service are the themes those FGD participants discussed and forward for the future improvements of SHCWMP in the study areas.’’
The availability of PPEs in different levels of health facilities shows 392 (72.6%), 212 (82.2%), 56 (58.9%), 52 (65.8%), 60 (65.2%), 12 (75%) health facility workers in NEMMCSH, government health centres, medium clinics, small clinics, and surgical centres respectively agree to the presence of personal protective equipment in their department. The availability of PPEs in this study was nearly two-fold when compared to the study findings in Myanmar, where 37.6% of the staff have PPEs [ 12 ].
The mean availability of masks, heavy-duty gloves, boots, and aprons was 71.1%, 65.4%, 38%, and 44.4% in the study health facilities. This finding shows masks are less available in the study health facilities compared to other studies. The availability of utility gloves, boots, and plastic aprons is good in this study compared to the study conducted by Banstola, D in Pokhara Sub-Metropolitan City [ 23 ].
The findings of this study show there is a poor segregation practice, and all kinds of solid wastes were collected together. This finding was similar to the study findings conducted in Addis Ababa, Ethiopia, by Debere et al. [ 24 ] and contrary to the study findings conducted in Nepal and India, which shows 50% and 65–75% of the surveyed health facilities were practising proper waste segregation systems at the point of generation without mixing general wastes with hazardous wastes respectively [ 9 , 17 ].
Ninety percent of private health facilities collect and transport SHCW generated in every service area and transport it to the disposal place by the collection container (no separate container to collect and transport the waste to the final disposal site). This finding was similar to the study findings of Debre Markos’s town [ 25 ]. At all of the facilities in the study area, SHCW was transported from the service areas to the disposal site manually by carrying the collection container, and there was no trolley for transportation. This finding was contrary to the study findings conducted in India, which show segregated waste from the generation site was being transported through the chute to the carts placed at various points on the hospital premises by skilled sanitary workers [ 17 ].
Observational findings revealed that pre-treatment of SHCW before disposal was not practised at all study health facilities. This study was contrary to the findings of Pullishery et al. [ 26 ], conducted in Mangalore, India, which depicted pre-treatment of the waste in 46% of the hospitals [ 26 ]. 95% of the facilities have no water supply for handwashing during and after solid healthcare waste generation, collection, and disposal. This finding was contrary to the study findings in Pakistan hospitals, which show all health facilities have an adequate water supply near the health care waste management sites [ 27 ].
Questionnaire data collection tools show that 129 (23.8%) of the staff needle stick injuries have occurred on health facility workers within one year of the period before the data collection. This finding was slightly smaller than the study findings of Deress et al. [ 25 ] in Debre Markos town, North East Ethiopia, where 30.9% of the workers had been exposed to needle stick injury one year prior to the study [ 25 ]. Reported and registered needle stick injuries in health facilities are less reported, and only 70 (54.2%) of the injuries are reported to the health facilities. This finding shows an underestimation of the risk and the problem, which was supported by the study conducted in Menilik II hospitals in Addis Ababa [ 22 ]. 50%, 33.4%, 48%, 52%, and 62.5% of needle stick injuries were not reported in NEMMCSH, Government health centres, medium clinics, small clinics, and surgical centres, respectively, to the health facility manager.
Nearly 1/3 (177 or 32.7%) of the staff are exposed to needle stick injuries. Needle stick injuries in health facilities are less reported, and only 73 (41.24%) of the injuries are reported to the health facilities within 12 months of the data collection. This finding is slightly higher than the study finding of Deress et al. [ 25 ] in Debere Markos, Ethiopia, in which 23.3% of the study participants had encountered needle stick/sharps injuries preceding 12 months of the data collection period [ 25 ].
Seventy-three injuries were reported to the health facility manager in the last one year, 44 of the injuries were reported by health professionals, and the rest were reported by supportive staff. These injuries were reported from 35(85.3%) health facilities; the remaining six have no report. These study findings were better than the findings of Khan et al. [ 21 ], in which one-third of the facilities had a reporting system for an incident, and almost the same percentage of the facilities had post-exposure procedures in both public and private sectors [ 21 ].
Within one year of the study period, 129 (23.88%) needle stick injuries occurred. However, needle stick injuries in health facilities are less reported, and only 70 (39.5%) of the injuries are reported to the health facilities. These findings were reasonable compared to the study findings of the southwest region of Cameroon, in which 50.9% (110/216) of all participants had at least one occupational exposure [ 28 , 29 ]. This result report shows a very high exposure to needle stick injury compared to the study findings in Brazil, which shows 6.1% of the research participants were injured [ 27 ].
The finding shows that 220 (40.8%) of the respondents were vaccinated to prevent themselves from health facility-acquired infection. One Hundred Fifty-six (70.9%) of the respondents are vaccinated in order to avoid themselves from Hep B infection. Fifty-nine (26%0.8) of the respondents were vaccinated to protect themselves from two diseases that are Hep B and COVID-19. This finding was nearly the same as the study findings of Deress et al. [ 7 ],in Ethiopia, 30.7% were vaccinated, and very low compared to the study findings of Qadir et al. [ 30 ] in Pakistan and Saha & Bhattacharjya India which is 66.67% and 66.17% respectively [ 25 , 30 , 31 ].
The incineration of solid healthcare waste technology has been accepted and adopted as an effective method in Ethiopia. These pollutants may have undesirable environmental impacts on human and animal health, such as liver failure and cancer [ 15 , 16 ]. All government health facilities use incineration to dispose of solid waste. 88.4% and 100% of the wastes are incinerated in WUNEMMCSH and government health centres, respectively. This finding contradicts the study findings in the United States of America and Malaysia, which are 49–60% and 59–60 are incinerated, respectively, and the rest are treated using other technologies [ 15 , 16 ].
All study health facilities used a brick or barrel type of incinerator. The incinerators found in the study health facilities need to meet the minimum standards of solid health care waste incineration practice. These findings were similar to the study findings of Nepal and Pakistan [ 32 ]. The health care waste treatment system in health facilities was found to be very unsystematic and unscientific, which cannot guarantee that there is no risk to the environment and public health, as well as safety for personnel involved in health care waste treatment. Most incinerators are not properly operated and maintained, resulting in poor performance.
All government health facilities use incineration to dispose of solid waste. All the generated sharp wastes are incinerated using brick or barrel incinerators, as shown in Fig. 1 above. This finding was consistent with the findings of Veilla and Samwel [ 33 ], who depicted that sharp waste generation is the same as sharps waste incinerated [ 33 ]. All brick incinerators were constructed without appropriate air inlets to facilitate combustion except in NEMMCSH, which is built at a 4-m height. These findings were similar to the findings of Tadese and Kumie at Addis Ababa [ 34 ].
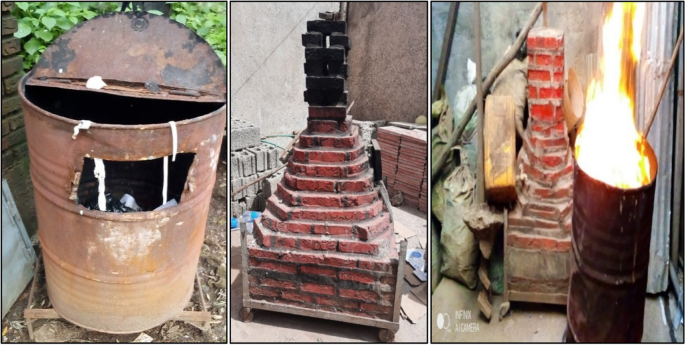
Barrel and brick incinerators used in private clinic
Strengths and limitations
This is a mixed-method study; both qualitative and quantitative study design, data collection and analysis techniques were used to understand the problem better. The setting for this study was one town, which is found in the southern part of the country. It only represents some of the country’s health facilities, and it is difficult to generalize the findings to other hospitals and health centres. Another limitation of this study was that private drug stores and private pharmacies were not incorporated.
Conclusions
In the study, health facilities’ foot-operated solid waste dust bins are not available for healthcare workers and patients to dispose of the generated wastes. Health facility managers in government and private health institutions should pay more attention to the availability of colour-coded dust bins. Most containers are opened, and insects and rodents can access them anytime. Some of them are even closed (not foot-operated), leading to contamination of hands when trying to open them.
Healthcare waste management training is mandatory for appropriate healthcare waste disposal. Healthcare-associated exposure should be appropriately managed, and infection prevention and control training should be provided to all staff working in the health facilities.
Availability of data and materials
The authors declare that data for this work are available upon request to the first author.
Chartier, Y et al. Safe management of wastes from health-care activities. 2nd ed. WHO; 2014.
Tesfahun E, et al. Developing models for the prediction of hospital healthcare waste generation rate. Waste Manag Res. 2014;34(1):75–80.
Manzoor J, Sharma M. Impact of Biomedical Waste on Environment and Human Health. Environmental Claims Journal. 2019;31(4):311–34.
Article Google Scholar
Yves C, Jorge E, Ute P, Annette P, et al. Safe management of wastes from health-care activities. WHO 2nd ed. 2014.
OSHA. Occupational Safety and Health Administration, Guidelines for Healthcare Waste Management. 2023.
Godfrey L, Ahmed M, et al. Solid waste management in Africa: governance failure or development opportunity?. Intech open. 2019.
Deress T, Jemal M, Girma M, Adane K. Knowledge, attitude, and practice of waste handlers about medical waste management in Debre Markos town healthcare facilities, northwest Ethiopia. BMC Res Notes. 2019;12(1):146.
Article PubMed PubMed Central Google Scholar
Sahiledengle B. Self-reported healthcare waste segregation practice and its correlate among healthcare workers in hospitals of Southeast Ethiopia. BMC Health Serv Res. 2019;19(1):591.
Debalkie D, Kume A. Healthcare Waste Management: The Current Issue in Menellik II Referral Hospital, Ethiopia. Curr World Environ. 2017;12(1):42–52.
Debere MK, Gelaye KA, Alamdo AG, Trifa ZM. Assessment of the health care waste generation rates and its management system in hospitals of Addis Ababa, Ethiopia, 2011. BMC Public Health. 2013;13(28).
Creswell JW. Research design qualitative, quantitative, & mixed method approach. 4th ed. SAGE Publications, Inc.; 2014.
Win EM, Saw YM, Oo KL, Than TM, Cho SM, Kariya T, et al. Healthcare waste management at primary health centres in Mon State, Myanmar: the comparisons between hospital and non-hospital type primary health centres. Nagoya J Med Sci. 2019;81(1):81–91.
PubMed PubMed Central Google Scholar
WHO. Safe management of wastes from health-care activities. 2nd ed. editor Chartier, Y et al. 2014.
Richard B, Ben A, Kristian S. Health care without harm climate-smart health care series green paper number one. 2019.
Khadem Ghasemi M, Mohd YR. Advantages and Disadvantages of Healthcare Waste Treatment and Disposal Alternatives: Malaysian Scenario. Pol J Environ Stud. 2016;25(1):17–25.
Mohseni-Bandpei A, Majlesi M, Rafiee M, Nojavan S, Nowrouz P, Zolfagharpour H. Polycyclic aromatic hydrocarbons (PAHs) formation during the fast pyrolysis of hazardous health-care waste. Chemosphere. 2019;227:277–88.
Article PubMed CAS Google Scholar
Pandey A, Ahuja S, Madan M, Asthana AK. Bio-Medical Waste Managment in a Tertiary Care Hospital: An Overview. J Clin Diagn Res. 2016;10(11):DC01-DC3.
Doylo T, Alemayehu T, Baraki N. Knowledge and Practice of Health Workers about Healthcare Waste Management in Public Health Facilities in Eastern Ethiopia. J Community Health. 2019;44(2):284–91.
Article PubMed Google Scholar
Hosny G, Samir S, Sharkawy R. An intervention significantly improve medical waste handling and management: A consequence of raising knowledge and practical skills of health care workers. Int J Health Sci.2018;12(4).
Khan EA, Sabeeh SM, Chaudhry MA, Yaqoob A, Kumar R. et al. Health care waste management in Pakistan: A situational analisis and way forward. Pak J Public Health. 2016;6(3).
Khan BA, Cheng L, Khan AA, Ahmed H. Healthcare waste management in Asian developing countries: A mini review. Waste Manag Res. 2019;37(9):863–75.
Debalkie D, Kumie A. Healthcare Waste Management: The Current Issue in Menellik II Referral Hospital. Ethiopia Current World Environment. 2017;12(1):42–52.
Banstola D, Banstola R, Nepal D, Baral P. Management of hospital solid wastes: A study in Pokhara sub metropolitan city. J Institute Med. 2017;31(1):68–74.
Debere MK, Gelaye KA, Alamdo AG, Trifa, ZM. Assessment of the HCW generation rates and its management system in hospitals of Addis Ababa, Ethiopia. BMC Public Health. 2014;13(28):1–9.
Deress T, Hassen F, Adane K, Tsegaye A. Assessment of Knowledge, Attitude, and Practice about Biomedical Waste Management and Associated Factors among the Healthcare Professionals at Debre Markos Town Healthcare Facilities. Northwest Ethiopia J Environ Public Health. 2018;2018:7672981.
PubMed Google Scholar
Pullishery F, Panchmal GS, Siddique S, Abraham A. Awareness, knowledge, and practices on bio-medical waste management among health care professionals in Mangalore- A cross sectional study. Integr Med. 2016;3(1):29–35.
Google Scholar
Ream PS, Tipple AF, Salgado TA, Souza AC, Souza SM, Galdino-Junior H, et al. Hospital housekeepers: Victims of ineffective hospital waste management. Arch Environ Occup Health. 2016;71(5):273–80.
Ngwa CH, Ngoh EA, Cumber SN. Assessment of the knowledge, attitude and practice of health care workers in Fako division on post exposure prophylaxis to blood borne viruses: a hospital based cross-sectional study. Pan Afr Med J. 2018;31.
Health care waste managemnt in pakistan. a situation analysis and way forward. Pakistan Journal of Public Health. 2016;6(3):35–45.
Qadir DM, Murad DR, Faraz DN. Hospital Waste Management; Tertiary Care Hospitals. The Professional Medical Journal. 2016;23(07):802–6.
Saha A, Bhattacharjya H. Health-Care Waste Management in Public Sector of Tripura, North-East India: An Observational Study. Indian J Community Med. 2019;44(4):368–72.
Pullishery F, Panchmal G, Siddique S, Abraham A. Awareness, knowledge, and practices on bio-medical waste management among health care professionals in Mangalore- A cross sectional study. Integr Med. 2016;3(1):29–35.
Veilla EM, Samwel VM. Assessment of sharps waste management practices in a referral hospital. Afr J Environ Sci Technol. 2016;10(3):86–95.
Tadesse ML, Kumie A. Healthcare waste generation and management practice in government health centers of Addis Ababa. Ethiopia BMC Public Health. 2014;14:1221.
Download references
Acknowledgements
The authors are grateful to the health facility leaders and ethical committees of the hospitals for their permission. The authors acknowledge the cooperation of the health facility workers who participated in this study.
This research did not receive any specific grant from funding agencies in the public, commercial, or not-for-profit sectors.
Author information
Authors and affiliations.
Wachemo University College of Medicine and public health, Hossana, Ethiopia
Yeshanew Ayele Tiruneh
Department of Public Health, University of South Africa, College of Human Science, Pretoria, South Africa
L. M. Modiba & S. M. Zuma
You can also search for this author in PubMed Google Scholar
Contributions
Dr. Yeshanew Ayele Tiruneh is a researcher of this study; the principal investigator does all the proposal preparation, methodology, data collection, result and discussion, and manuscript writing. Professor LM Modiba and Dr. SM Zuma are supervisors for this study. They participated in the topic selection and modification to the final manuscript preparation by commenting on and correcting the study. Finally, the three authors read and approved the final version of the manuscript and agreed to submit the manuscript for publication.
Corresponding author
Correspondence to Yeshanew Ayele Tiruneh .
Ethics declarations
Ethics approval and consent to participate.
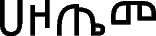
Consent for publication
Not applicable.
Competing interests
The authors declare no competing interests.
Additional information
Publisher’s note.
Springer Nature remains neutral with regard to jurisdictional claims in published maps and institutional affiliations.
Rights and permissions
Open Access This article is licensed under a Creative Commons Attribution-NonCommercial-NoDerivatives 4.0 International License, which permits any non-commercial use, sharing, distribution and reproduction in any medium or format, as long as you give appropriate credit to the original author(s) and the source, provide a link to the Creative Commons licence, and indicate if you modified the licensed material. You do not have permission under this licence to share adapted material derived from this article or parts of it. The images or other third party material in this article are included in the article’s Creative Commons licence, unless indicated otherwise in a credit line to the material. If material is not included in the article’s Creative Commons licence and your intended use is not permitted by statutory regulation or exceeds the permitted use, you will need to obtain permission directly from the copyright holder. To view a copy of this licence, visit http://creativecommons.org/licenses/by-nc-nd/4.0/ .
Reprints and permissions
About this article
Cite this article.
Tiruneh, Y.A., Modiba, L.M. & Zuma, S.M. Solid health care waste management practice in Ethiopia, a convergent mixed method study. BMC Health Serv Res 24 , 985 (2024). https://doi.org/10.1186/s12913-024-11444-8
Download citation
Received : 05 March 2023
Accepted : 14 August 2024
Published : 26 August 2024
DOI : https://doi.org/10.1186/s12913-024-11444-8
Share this article
Anyone you share the following link with will be able to read this content:
Sorry, a shareable link is not currently available for this article.
Provided by the Springer Nature SharedIt content-sharing initiative
- Health care waste
- Waste management
- Private health facilities
BMC Health Services Research
ISSN: 1472-6963
- General enquiries: [email protected]
‘This could change everything!’ Nous Research unveils new tool to train powerful AI models with 10,000x efficiency
- Share on Facebook
- Share on LinkedIn
Join our daily and weekly newsletters for the latest updates and exclusive content on industry-leading AI coverage. Learn More
Nous Research turned heads earlier this month with the release of its permissive, open-source Llama 3.1 variant Hermes 3 .
Now, the small research team dedicated to making “personalized, unrestricted AI” models has announced another seemingly massive breakthrough: DisTrO (Distributed Training Over-the-Internet), a new optimizer that reduces the amount of information that must be sent between various GPUs (graphics processing units) during each step of training an AI model.
Nous’s DisTrO optimizer means powerful AI models can now be trained outside of big companies, across the open web on consumer-grade connections, potentially by individuals or institutions working together from around the world.
DisTrO has already been tested and shown in a Nous Research technical paper to yield an 857 times efficiency increase compared to one popular existing training algorithm, All-Reduce , as well as a massive reduction in the amount of information transmitted during each step of the training process (86.8 megabytes compared to 74.4 gigabytes) while only suffering a slight loss in overall performance. See the results in the table below from the Nous Research technical paper:

Ultimately, the DisTrO method could open the door to many more people being able to train massively powerful AI models as they see fit.
As the firm wrote in a post on X yesterday : “Without relying on a single company to manage and control the training process, researchers and institutions can have more freedom to collaborate and experiment with new techniques, algorithms, and models. This increased competition fosters innovation, drives progress, and ultimately benefits society as a whole.”
What if you could use all the computing power in the world to train a shared, open source AI model? Preliminary report: https://t.co/b1XgJylsnV Nous Research is proud to release a preliminary report on DisTrO (Distributed Training Over-the-Internet) a family of… pic.twitter.com/h2gQJ4m7lB — Nous Research (@NousResearch) August 26, 2024
The problem with AI training: steep hardware requirements
As covered on VentureBeat previously, Nvidia’s GPUs in particular are in high demand in the generative AI era, as the expensive graphics cards’ powerful parallel processing capabilities are needed to train AI models efficiently and (relatively) quickly. This blog post at APNic describes the process well.
A big part of the AI training process relies on GPU clusters — multiple GPUs — exchanging information with one another about the model and the information “learned” within training data sets.
However, this “inter-GPU communication” requires that GPU clusters be architected, or set up, in a precise way in controlled conditions, minimizing latency and maximizing throughput. Hence why companies such as Elon Musk’s Tesla are investing heavily in setting up physical “superclusters” with many thousands (or hundreds of thousands) of GPUs sitting physically side-by-side in the same location — typically a massive airplane hangar-sized warehouse or facility.
Because of these requirements, training generative AI — especially the largest and most powerful models — is typically an extremely capital-heavy endeavor, one that only some of the most well-funded companies can engage in, such as Tesla, Meta, OpenAI, Microsoft, Google, and Anthropic.
The training process for each of these companies looks a little different, of course. But they all follow the same basic steps and use the same basic hardware components. Each of these companies tightly controls its own AI model training processes, and it can be difficult for incumbents, much less laypeople outside of them, to even think of competing by training their own similarly-sized (in terms of parameters, or the settings under the hood) models.
But Nous Research, whose whole approach is essentially the opposite — making the most powerful and capable AI it can on the cheap, openly, freely, for anyone to use and customize as they see fit without many guardrails — has found an alternative.
What DisTrO does differently
While traditional methods of AI training require synchronizing full gradients across all GPUs and rely on extremely high bandwidth connections, DisTrO reduces this communication overhead by four to five orders of magnitude.
The paper authors haven’t fully revealed how their algorithms reduce the amount of information at each step of training while retaining overall model performance, but plan to release more on this soon.
The reduction was achieved without relying on amortized analysis or compromising the convergence rate of the training, allowing large-scale models to be trained over much slower internet connections — 100Mbps download and 10Mbps upload, speeds available to many consumers around the world.
The authors tested DisTrO using the Meta Llama 2, 1.2 billion large language model (LLM) architecture and achieved comparable training performance to conventional methods with significantly less communication overhead.
They note that this is the smallest-size model that worked well with the DisTrO method, and they “do not yet know whether the ratio of bandwidth reduction scales up, down, or stays constant as model size increases.”
Yet, the authors also say that “our preliminary tests indicate that it is possible to get a bandwidth requirements reduction of up to 1000x to 3000x during the pre-training,” phase of LLMs, and “for post-training and fine-tuning, we can achieve up to 10000x without any noticeable degradation in loss.”
They further hypothesize that the research, while initially conducted on LLMs, could be used to train large diffusion models (LDMs) as well: think the Stable Diffusion open source image generation model and popular image generation services derived from it such as Midjourney .
Still need good GPUs
To be clear: DisTrO still relies on GPUs — only instead of clustering them all together in the same location, now they can be spread out across the world and communicate over the consumer internet.
Specifically, DisTrO was evaluated using 32x H100 GPUs, operating under the Distributed Data Parallelism (DDP) strategy, where each GPU had the entire model loaded in VRAM .
This setup allowed the team to rigorously test DisTrO’s capabilities and demonstrate that it can match the convergence rates of AdamW+All-Reduce despite drastically reduced communication requirements.
This result suggests that DisTrO can potentially replace existing training methods without sacrificing model quality, offering a scalable and efficient solution for large-scale distributed training.
By reducing the need for high-speed interconnects DisTrO could enable collaborative model training across decentralized networks, even with participants using consumer-grade internet connections.
The report also explores the implications of DisTrO for various applications, including federated learning and decentralized training.
Additionally, DisTrO’s efficiency could help mitigate the environmental impact of AI training by optimizing the use of existing infrastructure and reducing the need for massive data centers.
Moreover, the breakthroughs could lead to a shift in how large-scale models are trained, moving away from centralized, resource-intensive data centers towards more distributed, collaborative approaches that leverage diverse and geographically dispersed computing resources.
What’s next for the Nous Research team and DisTrO?
The research team invites others to join them in exploring the potential of DisTrO. The preliminary report and supporting materials are available on GitHub , and the team is actively seeking collaborators to help refine and expand this groundbreaking technology.
Already, some AI influencers such as @kimmonismus on X (aka chubby) have praised the research as a huge breakthrough in the field, writing, “This could change everything!”
Wow, amazing! This could change everything! https://t.co/2f0PDSaTSm — Chubby♨️ (@kimmonismus) August 27, 2024
With DisTrO, Nous Research is not only advancing the technical capabilities of AI training but also promoting a more inclusive and resilient research ecosystem that has the potential to unlock unprecedented advancements in AI.
Stay in the know! Get the latest news in your inbox daily
By subscribing, you agree to VentureBeat's Terms of Service.
Thanks for subscribing. Check out more VB newsletters here .
An error occured.
August 27, 2024
Queen’s Brian May Is a Champion for Badgers and Science
Queen guitarist Brian May has spent a decade studying the science of bovine tuberculosis, which can be carried by badgers, and has identified a new method of spread
By Elizabeth Gibney & Nature magazine
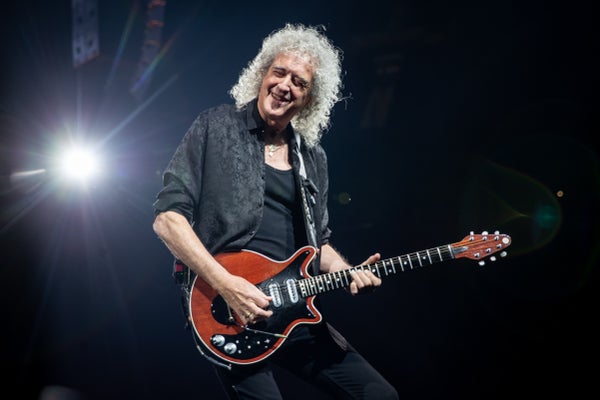
Brian May: “It kind of irks me that we don’t have a scientific paper out there.”
Miikka Skaffari/Getty Images
Brian May has many strings to his guitar. The musician, who is still touring with his rock band Queen, is also an astrophysicist, specializing in 3D stereoscopic images of distant bodies. And to the UK public, he’s also a passionate campaigner for animal rights.
After abandoning his PhD at Imperial College London in 1974 to follow his musical passions, May finally returned to complete his doctorate in 2007. Soon after, the rock star embroiled himself in a polarizing scientific row over whether the European badger ( Meles meles ) was causing mass infection of cattle with bovine tuberculosis (TB). Each year, the problem costs the UK government more than £100 million (US$130 million) and leads to the slaughter of more than 20,000 cows.
Some scientists initially backed the government’s policy of culling badgers — 230,000 have been killed since 2013 — although many now doubt the approach’s effectiveness. The past government had planned to phase out culling in favour of vaccination, but 20 culling licences were issued this year. The new Labour government has said that it plans to end culling, but these licences will continue.
On supporting science journalism
If you're enjoying this article, consider supporting our award-winning journalism by subscribing . By purchasing a subscription you are helping to ensure the future of impactful stories about the discoveries and ideas shaping our world today.
In a BBC documentary airing in the United Kingdom on 23 August, May describes his decade-long research project to understand what is behind bovine TB. Alongside him on the programme is Anne Brummer, chief executive of their co-founded wildlife charity, the Save Me Trust. “He lives and sleeps this,” says Brummer. “He hates injustice and is a very passionate, compassionate person. He just wants to solve these problems.”
May spoke to Nature about how his scientific skills have been essential to his work, and the “monstrous” findings made by his team, which includes Brummer, farmer Robert Reid and veterinary surgeon Dick Sibley.
What has the influence of your scientific background been on your work with animals? Do you think it gave you more confidence?
Absolutely. The scientific method is something precious, and you do learn it — the hard way — if you’re doing a PhD. Everything comes down to asking the right questions and keeping an open mind, and resisting the terrible inclination that scientists have, because they’re human beings, of finding what you expect to find. We’ve all been told that badgers are how the pathogen is spread, so we look for that pattern. And, sadly, I think that’s why the myth has perpetuated.
Were you convinced from the beginning that badgers were not causing the spread of TB in cattle?
I was always suspicious, but I didn’t have anything to justify my position. But I felt that even if they were responsible, it wasn’t their fault. I remember being at a Zoological Society meeting around 13 years ago, where I had the temerity to stand up and say, “Doesn’t anybody think this is morally wrong?”, and I felt like a child, because everybody looked at me with such scorn. I realized that the only way to get anywhere was to stop shouting, start listening and get into the science. Along the way I think we’ve made breakthroughs that I didn’t even dream of making.
You’ve spent the past 12 years as part of a research team on the Gatcombe farm in Devon, near the south coast of England, studying TB transmission. What did you find?
We developed a view on how the mycobacterium responsible for TB transmits from one animal to another. TB has classically been known as a respiratory disease, but our discovery is that a cow doesn’t contract TB by breathing in something, it contracts it by eating the pathogen from defecation from a neighbouring cow. It’s a monstrous discovery, because once you start understanding your enemy, then you can start to defeat it. Now we know that the thing is passed from cow to cow, because of poor hygiene.
How does testing contribute to the problem?
We also found that the [government-sanctioned] skin test for TB is as little as 50% accurate. That’s a terrible thing to discover, because you might as well toss a coin. We discovered that one cow had been through the skin test 30 times and pronounced healthy, and when it went for a postmortem, it was riddled with TB. So, the skin test is the villain of the piece, and the fact that farmers are relying on this incredibly inaccurate test to remove cows from their precious herds and take them off to slaughter is a scandal.
Do you have any plans to put your findings into a scientific paper?
Absolutely, yes. That’s definitely one of our next steps. It kind of irks me that we don’t have a scientific paper out there, but all in good time.
What makes you so convinced that badgers play no part in transmission?
On Robert Reid’s farm we, for some time, had a healthy herd with an infected population of badgers around it. And all through this period, almost 10 years, there’s never been a single infection from the cows that could graze in the fields, near where the badgers live. All have been in the sheds.
There is also a farmer in Tiverton who built an amazing fence five miles long around his beef herd, to keep the wildlife out. Eventually, he lost half his herd. How did that happen? It’s highly likely that a new bull — shown as healthy by the skin test — is the way this herd became destroyed. It’s likely that this is a pattern we’ve seen in many other places, as well. I would like farmers to see the documentary and think, OK, maybe we’re ready for a change. We need to change so many methods in cattle farming to solve this problem.
In the film, you say that speaking out against badger-culling has become as important to you as your music. Where does astrophysics fit in?
It’s right up there. I’m still doing astrophysics. I’m privileged to be part of a few teams of exploration in NASA, the European Space Agency and the Japanese Aerospace Exploration Agency. I have a great time doing that. What I contribute is stereoscopy and it’s been a lot of fun, because it gives you very human insights into the exploration of these wonderful places they’re visiting.
What does the bovine TB affair teach us about science and policymaking?
All I’d like to say is that it worries me that the peer-review process can embody flaws. If you get the people to peer review who are in the same clique, you’re not going to peruse the material thoroughly enough.
This article is reproduced with permission and was first published on August 22, 2024 .
Generate accurate APA citations for free
- Knowledge Base
- APA Style 7th edition
- How to write an APA methods section
How to Write an APA Methods Section | With Examples
Published on February 5, 2021 by Pritha Bhandari . Revised on June 22, 2023.
The methods section of an APA style paper is where you report in detail how you performed your study. Research papers in the social and natural sciences often follow APA style. This article focuses on reporting quantitative research methods .
In your APA methods section, you should report enough information to understand and replicate your study, including detailed information on the sample , measures, and procedures used.
Instantly correct all language mistakes in your text
Upload your document to correct all your mistakes in minutes
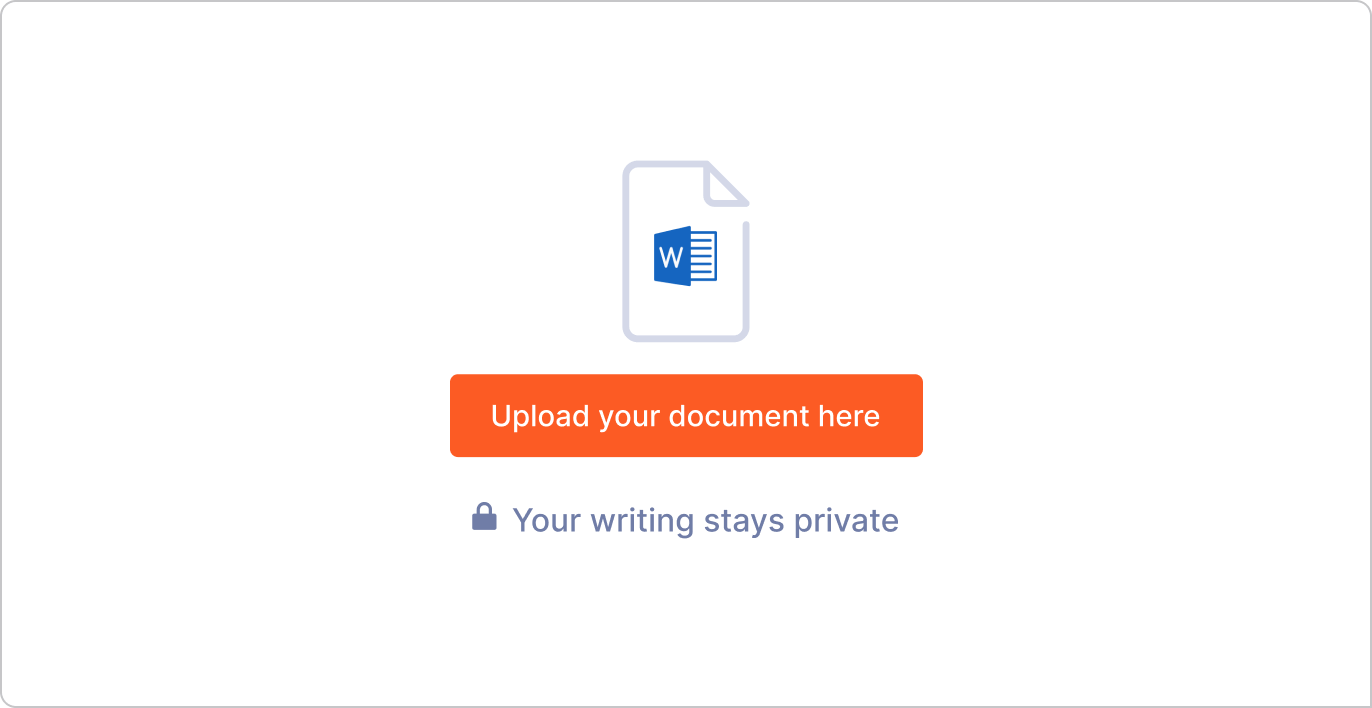
Table of contents
Structuring an apa methods section.
Participants
Example of an APA methods section
Other interesting articles, frequently asked questions about writing an apa methods section.
The main heading of “Methods” should be centered, boldfaced, and capitalized. Subheadings within this section are left-aligned, boldfaced, and in title case. You can also add lower level headings within these subsections, as long as they follow APA heading styles .
To structure your methods section, you can use the subheadings of “Participants,” “Materials,” and “Procedures.” These headings are not mandatory—aim to organize your methods section using subheadings that make sense for your specific study.
Heading | What to include |
---|---|
Participants | |
Materials | |
Procedure |
Note that not all of these topics will necessarily be relevant for your study. For example, if you didn’t need to consider outlier removal or ways of assigning participants to different conditions, you don’t have to report these steps.
The APA also provides specific reporting guidelines for different types of research design. These tell you exactly what you need to report for longitudinal designs , replication studies, experimental designs , and so on. If your study uses a combination design, consult APA guidelines for mixed methods studies.
Detailed descriptions of procedures that don’t fit into your main text can be placed in supplemental materials (for example, the exact instructions and tasks given to participants, the full analytical strategy including software code, or additional figures and tables).
Are your APA in-text citations flawless?
The AI-powered APA Citation Checker points out every error, tells you exactly what’s wrong, and explains how to fix it. Say goodbye to losing marks on your assignment!
Get started!
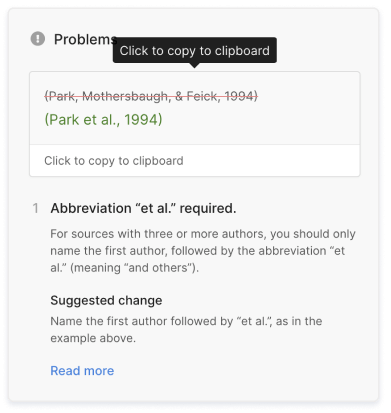
Begin the methods section by reporting sample characteristics, sampling procedures, and the sample size.
Participant or subject characteristics
When discussing people who participate in research, descriptive terms like “participants,” “subjects” and “respondents” can be used. For non-human animal research, “subjects” is more appropriate.
Specify all relevant demographic characteristics of your participants. This may include their age, sex, ethnic or racial group, gender identity, education level, and socioeconomic status. Depending on your study topic, other characteristics like educational or immigration status or language preference may also be relevant.
Be sure to report these characteristics as precisely as possible. This helps the reader understand how far your results may be generalized to other people.
The APA guidelines emphasize writing about participants using bias-free language , so it’s necessary to use inclusive and appropriate terms.
Sampling procedures
Outline how the participants were selected and all inclusion and exclusion criteria applied. Appropriately identify the sampling procedure used. For example, you should only label a sample as random if you had access to every member of the relevant population.
Of all the people invited to participate in your study, note the percentage that actually did (if you have this data). Additionally, report whether participants were self-selected, either by themselves or by their institutions (e.g., schools may submit student data for research purposes).
Identify any compensation (e.g., course credits or money) that was provided to participants, and mention any institutional review board approvals and ethical standards followed.
Sample size and power
Detail the sample size (per condition) and statistical power that you hoped to achieve, as well as any analyses you performed to determine these numbers.
It’s important to show that your study had enough statistical power to find effects if there were any to be found.
Additionally, state whether your final sample differed from the intended sample. Your interpretations of the study outcomes should be based only on your final sample rather than your intended sample.
Write up the tools and techniques that you used to measure relevant variables. Be as thorough as possible for a complete picture of your techniques.
Primary and secondary measures
Define the primary and secondary outcome measures that will help you answer your primary and secondary research questions.
Specify all instruments used in gathering these measurements and the construct that they measure. These instruments may include hardware, software, or tests, scales, and inventories.
- To cite hardware, indicate the model number and manufacturer.
- To cite common software (e.g., Qualtrics), state the full name along with the version number or the website URL .
- To cite tests, scales or inventories, reference its manual or the article it was published in. It’s also helpful to state the number of items and provide one or two example items.
Make sure to report the settings of (e.g., screen resolution) any specialized apparatus used.
For each instrument used, report measures of the following:
- Reliability : how consistently the method measures something, in terms of internal consistency or test-retest reliability.
- Validity : how precisely the method measures something, in terms of construct validity or criterion validity .
Giving an example item or two for tests, questionnaires , and interviews is also helpful.
Describe any covariates—these are any additional variables that may explain or predict the outcomes.
Quality of measurements
Review all methods you used to assure the quality of your measurements.
These may include:
- training researchers to collect data reliably,
- using multiple people to assess (e.g., observe or code) the data,
- translation and back-translation of research materials,
- using pilot studies to test your materials on unrelated samples.
For data that’s subjectively coded (for example, classifying open-ended responses), report interrater reliability scores. This tells the reader how similarly each response was rated by multiple raters.
Report all of the procedures applied for administering the study, processing the data, and for planned data analyses.
Data collection methods and research design
Data collection methods refers to the general mode of the instruments: surveys, interviews, observations, focus groups, neuroimaging, cognitive tests, and so on. Summarize exactly how you collected the necessary data.
Describe all procedures you applied in administering surveys, tests, physical recordings, or imaging devices, with enough detail so that someone else can replicate your techniques. If your procedures are very complicated and require long descriptions (e.g., in neuroimaging studies), place these details in supplementary materials.
To report research design, note your overall framework for data collection and analysis. State whether you used an experimental, quasi-experimental, descriptive (observational), correlational, and/or longitudinal design. Also note whether a between-subjects or a within-subjects design was used.
For multi-group studies, report the following design and procedural details as well:
- how participants were assigned to different conditions (e.g., randomization),
- instructions given to the participants in each group,
- interventions for each group,
- the setting and length of each session(s).
Describe whether any masking was used to hide the condition assignment (e.g., placebo or medication condition) from participants or research administrators. Using masking in a multi-group study ensures internal validity by reducing research bias . Explain how this masking was applied and whether its effectiveness was assessed.
Participants were randomly assigned to a control or experimental condition. The survey was administered using Qualtrics (https://www.qualtrics.com). To begin, all participants were given the AAI and a demographics questionnaire to complete, followed by an unrelated filler task. In the control condition , participants completed a short general knowledge test immediately after the filler task. In the experimental condition, participants were asked to visualize themselves taking the test for 3 minutes before they actually did. For more details on the exact instructions and tasks given, see supplementary materials.
Data diagnostics
Outline all steps taken to scrutinize or process the data after collection.
This includes the following:
- Procedures for identifying and removing outliers
- Data transformations to normalize distributions
- Compensation strategies for overcoming missing values
To ensure high validity, you should provide enough detail for your reader to understand how and why you processed or transformed your raw data in these specific ways.
Analytic strategies
The methods section is also where you describe your statistical analysis procedures, but not their outcomes. Their outcomes are reported in the results section.
These procedures should be stated for all primary, secondary, and exploratory hypotheses. While primary and secondary hypotheses are based on a theoretical framework or past studies, exploratory hypotheses are guided by the data you’ve just collected.
Scribbr Citation Checker New
The AI-powered Citation Checker helps you avoid common mistakes such as:
- Missing commas and periods
- Incorrect usage of “et al.”
- Ampersands (&) in narrative citations
- Missing reference entries
This annotated example reports methods for a descriptive correlational survey on the relationship between religiosity and trust in science in the US. Hover over each part for explanation of what is included.
The sample included 879 adults aged between 18 and 28. More than half of the participants were women (56%), and all participants had completed at least 12 years of education. Ethics approval was obtained from the university board before recruitment began. Participants were recruited online through Amazon Mechanical Turk (MTurk; www.mturk.com). We selected for a geographically diverse sample within the Midwest of the US through an initial screening survey. Participants were paid USD $5 upon completion of the study.
A sample size of at least 783 was deemed necessary for detecting a correlation coefficient of ±.1, with a power level of 80% and a significance level of .05, using a sample size calculator (www.sample-size.net/correlation-sample-size/).
The primary outcome measures were the levels of religiosity and trust in science. Religiosity refers to involvement and belief in religious traditions, while trust in science represents confidence in scientists and scientific research outcomes. The secondary outcome measures were gender and parental education levels of participants and whether these characteristics predicted religiosity levels.
Religiosity
Religiosity was measured using the Centrality of Religiosity scale (Huber, 2003). The Likert scale is made up of 15 questions with five subscales of ideology, experience, intellect, public practice, and private practice. An example item is “How often do you experience situations in which you have the feeling that God or something divine intervenes in your life?” Participants were asked to indicate frequency of occurrence by selecting a response ranging from 1 (very often) to 5 (never). The internal consistency of the instrument is .83 (Huber & Huber, 2012).
Trust in Science
Trust in science was assessed using the General Trust in Science index (McCright, Dentzman, Charters & Dietz, 2013). Four Likert scale items were assessed on a scale from 1 (completely distrust) to 5 (completely trust). An example question asks “How much do you distrust or trust scientists to create knowledge that is unbiased and accurate?” Internal consistency was .8.
Potential participants were invited to participate in the survey online using Qualtrics (www.qualtrics.com). The survey consisted of multiple choice questions regarding demographic characteristics, the Centrality of Religiosity scale, an unrelated filler anagram task, and finally the General Trust in Science index. The filler task was included to avoid priming or demand characteristics, and an attention check was embedded within the religiosity scale. For full instructions and details of tasks, see supplementary materials.
For this correlational study , we assessed our primary hypothesis of a relationship between religiosity and trust in science using Pearson moment correlation coefficient. The statistical significance of the correlation coefficient was assessed using a t test. To test our secondary hypothesis of parental education levels and gender as predictors of religiosity, multiple linear regression analysis was used.
If you want to know more about statistics , methodology , or research bias , make sure to check out some of our other articles with explanations and examples.
- Normal distribution
- Measures of central tendency
- Chi square tests
- Confidence interval
- Quartiles & Quantiles
Methodology
- Cluster sampling
- Stratified sampling
- Thematic analysis
- Cohort study
- Peer review
- Ethnography
Research bias
- Implicit bias
- Cognitive bias
- Conformity bias
- Hawthorne effect
- Availability heuristic
- Attrition bias
- Social desirability bias
In your APA methods section , you should report detailed information on the participants, materials, and procedures used.
- Describe all relevant participant or subject characteristics, the sampling procedures used and the sample size and power .
- Define all primary and secondary measures and discuss the quality of measurements.
- Specify the data collection methods, the research design and data analysis strategy, including any steps taken to transform the data and statistical analyses.
You should report methods using the past tense , even if you haven’t completed your study at the time of writing. That’s because the methods section is intended to describe completed actions or research.
In a scientific paper, the methodology always comes after the introduction and before the results , discussion and conclusion . The same basic structure also applies to a thesis, dissertation , or research proposal .
Depending on the length and type of document, you might also include a literature review or theoretical framework before the methodology.
Cite this Scribbr article
If you want to cite this source, you can copy and paste the citation or click the “Cite this Scribbr article” button to automatically add the citation to our free Citation Generator.
Bhandari, P. (2023, June 22). How to Write an APA Methods Section | With Examples. Scribbr. Retrieved August 26, 2024, from https://www.scribbr.com/apa-style/methods-section/
Is this article helpful?
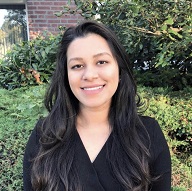
Pritha Bhandari
Other students also liked, how to write an apa results section, apa format for academic papers and essays, apa headings and subheadings, get unlimited documents corrected.
✔ Free APA citation check included ✔ Unlimited document corrections ✔ Specialized in correcting academic texts
Thank you for visiting nature.com. You are using a browser version with limited support for CSS. To obtain the best experience, we recommend you use a more up to date browser (or turn off compatibility mode in Internet Explorer). In the meantime, to ensure continued support, we are displaying the site without styles and JavaScript.
- View all journals
- Explore content
- About the journal
- Publish with us
- Sign up for alerts
- Open access
- Published: 22 July 2024
Neural general circulation models for weather and climate
- Dmitrii Kochkov ORCID: orcid.org/0000-0003-3846-4911 1 na1 ,
- Janni Yuval ORCID: orcid.org/0000-0001-7519-0118 1 na1 ,
- Ian Langmore 1 na1 ,
- Peter Norgaard 1 na1 ,
- Jamie Smith 1 na1 ,
- Griffin Mooers 1 ,
- Milan Klöwer 2 ,
- James Lottes 1 ,
- Stephan Rasp 1 ,
- Peter Düben ORCID: orcid.org/0000-0002-4610-3326 3 ,
- Sam Hatfield 3 ,
- Peter Battaglia 4 ,
- Alvaro Sanchez-Gonzalez 4 ,
- Matthew Willson ORCID: orcid.org/0000-0002-8730-1927 4 ,
- Michael P. Brenner 1 , 5 &
- Stephan Hoyer ORCID: orcid.org/0000-0002-5207-0380 1 na1
Nature ( 2024 ) Cite this article
53k Accesses
3 Citations
678 Altmetric
Metrics details
- Atmospheric dynamics
- Climate and Earth system modelling
- Computational science
General circulation models (GCMs) are the foundation of weather and climate prediction 1 , 2 . GCMs are physics-based simulators that combine a numerical solver for large-scale dynamics with tuned representations for small-scale processes such as cloud formation. Recently, machine-learning models trained on reanalysis data have achieved comparable or better skill than GCMs for deterministic weather forecasting 3 , 4 . However, these models have not demonstrated improved ensemble forecasts, or shown sufficient stability for long-term weather and climate simulations. Here we present a GCM that combines a differentiable solver for atmospheric dynamics with machine-learning components and show that it can generate forecasts of deterministic weather, ensemble weather and climate on par with the best machine-learning and physics-based methods. NeuralGCM is competitive with machine-learning models for one- to ten-day forecasts, and with the European Centre for Medium-Range Weather Forecasts ensemble prediction for one- to fifteen-day forecasts. With prescribed sea surface temperature, NeuralGCM can accurately track climate metrics for multiple decades, and climate forecasts with 140-kilometre resolution show emergent phenomena such as realistic frequency and trajectories of tropical cyclones. For both weather and climate, our approach offers orders of magnitude computational savings over conventional GCMs, although our model does not extrapolate to substantially different future climates. Our results show that end-to-end deep learning is compatible with tasks performed by conventional GCMs and can enhance the large-scale physical simulations that are essential for understanding and predicting the Earth system.
Similar content being viewed by others
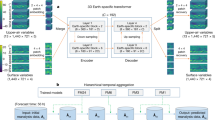
Accurate medium-range global weather forecasting with 3D neural networks
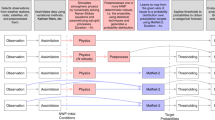
Deep learning for twelve hour precipitation forecasts
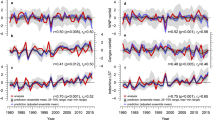
Skilful predictions of the Asian summer monsoon one year ahead
Solving the equations for Earth’s atmosphere with general circulation models (GCMs) is the basis of weather and climate prediction 1 , 2 . Over the past 70 years, GCMs have been steadily improved with better numerical methods and more detailed physical models, while exploiting faster computers to run at higher resolution. Inside GCMs, the unresolved physical processes such as clouds, radiation and precipitation are represented by semi-empirical parameterizations. Tuning GCMs to match historical data remains a manual process 5 , and GCMs retain many persistent errors and biases 6 , 7 , 8 . The difficulty of reducing uncertainty in long-term climate projections 9 and estimating distributions of extreme weather events 10 presents major challenges for climate mitigation and adaptation 11 .
Recent advances in machine learning have presented an alternative for weather forecasting 3 , 4 , 12 , 13 . These models rely solely on machine-learning techniques, using roughly 40 years of historical data from the European Center for Medium-Range Weather Forecasts (ECMWF) reanalysis v5 (ERA5) 14 for model training and forecast initialization. Machine-learning methods have been remarkably successful, demonstrating state-of-the-art deterministic forecasts for 1- to 10-day weather prediction at a fraction of the computational cost of traditional models 3 , 4 . Machine-learning atmospheric models also require considerably less code, for example GraphCast 3 has 5,417 lines versus 376,578 lines for the National Oceanic and Atmospheric Administration’s FV3 atmospheric model 15 (see Supplementary Information section A for details).
Nevertheless, machine-learning approaches have noteworthy limitations compared with GCMs. Existing machine-learning models have focused on deterministic prediction, and surpass deterministic numerical weather prediction in terms of the aggregate metrics for which they are trained 3 , 4 . However, they do not produce calibrated uncertainty estimates 4 , which is essential for useful weather forecasts 1 . Deterministic machine-learning models using a mean-squared-error loss are rewarded for averaging over uncertainty, producing unrealistically blurry predictions when optimized for multi-day forecasts 3 , 13 . Unlike physical models, machine-learning models misrepresent derived (diagnostic) variables such as geostrophic wind 16 . Furthermore, although there has been some success in using machine-learning approaches on longer timescales 17 , 18 , these models have not demonstrated the ability to outperform existing GCMs.
Hybrid models that combine GCMs with machine learning are appealing because they build on the interpretability, extensibility and successful track record of traditional atmospheric models 19 , 20 . In the hybrid model approach, a machine-learning component replaces or corrects the traditional physical parameterizations of a GCM. Until now, the machine-learning component in such models has been trained ‘offline’, by learning parameterizations independently of their interaction with dynamics. These components are then inserted into an existing GCM. The lack of coupling between machine-learning components and the governing equations during training potentially causes serious problems, such as instability and climate drift 21 . So far, hybrid models have mostly been limited to idealized scenarios such as aquaplanets 22 , 23 . Under realistic conditions, machine-learning corrections have reduced some biases of very coarse GCMs 24 , 25 , 26 , but performance remains considerably worse than state-of-the-art models.
Here we present NeuralGCM, a fully differentiable hybrid GCM of Earth’s atmosphere. NeuralGCM is trained on forecasting up to 5-day weather trajectories sampled from ERA5. Differentiability enables end-to-end ‘online training’ 27 , with machine-learning components optimized in the context of interactions with the governing equations for large-scale dynamics, which we find enables accurate and stable forecasts. NeuralGCM produces physically consistent forecasts with accuracy comparable to best-in-class models across a range of timescales, from 1- to 15-day weather to decadal climate prediction.
Neural GCMs
A schematic of NeuralGCM is shown in Fig. 1 . The two key components of NeuralGCM are a differentiable dynamical core for solving the discretized governing dynamical equations and a learned physics module that parameterizes physical processes with a neural network, described in full detail in Methods , Supplementary Information sections B and C , and Supplementary Table 1 . The dynamical core simulates large-scale fluid motion and thermodynamics under the influence of gravity and the Coriolis force. The learned physics module (Supplementary Fig. 1 ) predicts the effect of unresolved processes, such as cloud formation, radiative transport, precipitation and subgrid-scale dynamics, on the simulated fields using a neural network.
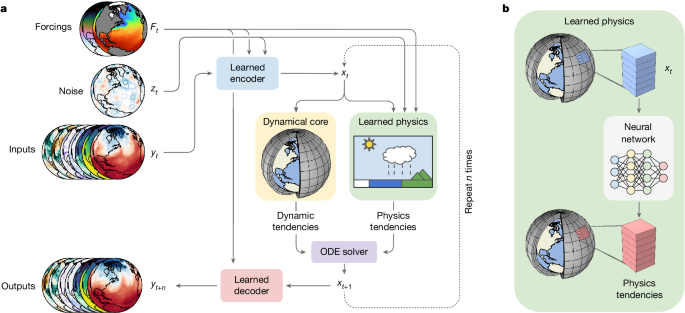
a , Overall model structure, showing how forcings F t , noise z t (for stochastic models) and inputs y t are encoded into the model state x t . The model state is fed into the dynamical core, and alongside forcings and noise into the learned physics module. This produces tendencies (rates of change) used by an implicit–explicit ordinary differential equation (ODE) solver to advance the state in time. The new model state x t +1 can then be fed back into another time step, or decoded into model predictions. b , The learned physics module, which feeds data for individual columns of the atmosphere into a neural network used to produce physics tendencies in that vertical column.
The differentiable dynamical core in NeuralGCM allows an end-to-end training approach, whereby we advance the model multiple time steps before employing stochastic gradient descent to minimize discrepancies between model predictions and reanalysis (Supplementary Information section G.2 ). We gradually increase the rollout length from 6 hours to 5 days (Supplementary Information section G and Supplementary Table 5 ), which we found to be critical because our models are not accurate for multi-day prediction or stable for long rollouts early in training (Supplementary Information section H.6.2 and Supplementary Fig. 23 ). The extended back-propagation through hundreds of simulation steps enables our neural networks to take into account interactions between the learned physics and the dynamical core. We train deterministic and stochastic NeuralGCM models, each of which uses a distinct training protocol, described in full detail in Methods and Supplementary Table 4 .
We train a range of NeuralGCM models at horizontal resolutions with grid spacing of 2.8°, 1.4° and 0.7° (Supplementary Fig. 7 ). We evaluate the performance of NeuralGCM at a range of timescales appropriate for weather forecasting and climate simulation. For weather, we compare against the best-in-class conventional physics-based weather models, ECMWF’s high-resolution model (ECMWF-HRES) and ensemble prediction system (ECMWF-ENS), and two of the recent machine-learning-based approaches, GraphCast 3 and Pangu 4 . For climate, we compare against a global cloud-resolving model and Atmospheric Model Intercomparison Project (AMIP) runs.
Medium-range weather forecasting
Our evaluation set-up focuses on quantifying accuracy and physical consistency, following WeatherBench2 12 . We regrid all forecasts to a 1.5° grid using conservative regridding, and average over all 732 forecasts made at noon and midnight UTC in the year 2020, which was held-out from training data for all machine-learning models. NeuralGCM, GraphCast and Pangu compare with ERA5 as the ground truth, whereas ECMWF-ENS and ECMWF-HRES compare with the ECMWF operational analysis (that is, HRES at 0-hour lead time), to avoid penalizing the operational forecasts for different biases than ERA5.
Model accuracy
We use ECMWF’s ensemble (ENS) model as a reference baseline as it achieves the best performance across the majority of lead times 12 . We assess accuracy using (1) root-mean-squared error (RMSE), (2) root-mean-squared bias (RMSB), (3) continuous ranked probability score (CRPS) and (4) spread-skill ratio, with the results shown in Fig. 2 . We provide more in-depth evaluations including scorecards, metrics for additional variables and levels and maps in Extended Data Figs. 1 and 2 , Supplementary Information section H and Supplementary Figs. 9 – 22 .
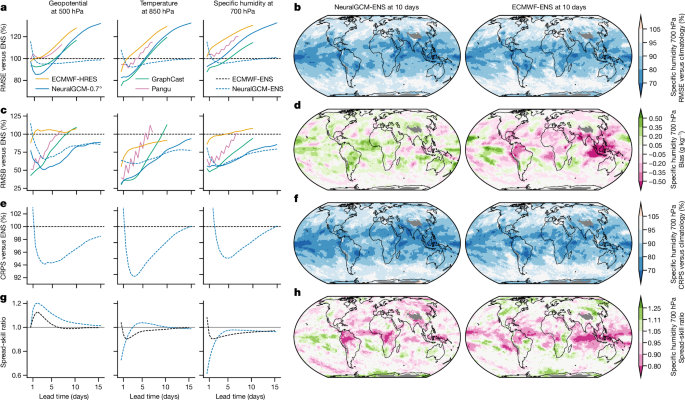
a , c , RMSE ( a ) and RMSB ( c ) for ECMWF-ENS, ECMWF-HRES, NeuralGCM-0.7°, NeuralGCM-ENS, GraphCast 3 and Pangu 4 on headline WeatherBench2 variables, as a percentage of the error of ECMWF-ENS. Deterministic and stochastic models are shown in solid and dashed lines respectively. e , g , CRPS relative to ECMWF-ENS ( e ) and spread-skill ratio for the ENS and NeuralGCM-ENS models ( g ). b , d , f , h , Spatial distributions of RMSE ( b ), bias ( d ), CRPS ( f ) and spread-skill ratio ( h ) for NeuralGCM-ENS and ECMWF-ENS models for 10-day forecasts of specific humidity at 700 hPa. Spatial plots of RMSE and CRPS show skill relative to a probabilistic climatology 12 with an ensemble member for each of the years 1990–2019. The grey areas indicate regions where climatological surface pressure on average is below 700 hPa.
Deterministic models that produce a single weather forecast for given initial conditions can be compared effectively using RMSE skill at short lead times. For the first 1–3 days, depending on the atmospheric variable, RMSE is minimized by forecasts that accurately track the evolution of weather patterns. At this timescale we find that NeuralGCM-0.7° and GraphCast achieve best results, with slight variations across different variables (Fig. 2a ). At longer lead times, RMSE rapidly increases owing to chaotic divergence of nearby weather trajectories, making RMSE less informative for deterministic models. RMSB calculates persistent errors over time, which provides an indication of how models would perform at much longer lead times. Here NeuralGCM models also compare favourably against previous approaches (Fig. 2c ), with notably much less bias for specific humidity in the tropics (Fig. 2d ).
Ensembles are essential for capturing intrinsic uncertainty of weather forecasts, especially at longer lead times. Beyond about 7 days, the ensemble means of ECMWF-ENS and NeuralGCM-ENS forecasts have considerably lower RMSE than the deterministic models, indicating that these models better capture the average of possible weather. A better metric for ensemble models is CRPS, which is a proper scoring rule that is sensitive to full marginal probability distributions 28 . Our stochastic model (NeuralGCM-ENS) running at 1.4° resolution has lower error compared with ECMWF-ENS across almost all variables, lead times and vertical levels for ensemble-mean RMSE, RSMB and CRPS (Fig. 2a,c,e and Supplementary Information section H ), with similar spatial patterns of skill (Fig. 2b,f ). Like ECMWF-ENS, NeuralGCM-ENS has a spread-skill ratio of approximately one (Fig. 2d ), which is a necessary condition for calibrated forecasts 29 .
An important characteristic of forecasts is their resemblance to realistic weather patterns. Figure 3 shows a case study that illustrates the performance of NeuralGCM on three types of important weather phenomenon: tropical cyclones, atmospheric rivers and the Intertropical Convergence Zone. Figure 3a shows that all the machine-learning models make significantly blurrier forecasts than the source data ERA5 and physics-based ECMWF-HRES forecast, but NeuralCGM-0.7° outperforms the pure machine-learning models, despite its coarser resolution (0.7° versus 0.25° for GraphCast and Pangu). Blurry forecasts correspond to physically inconsistent atmospheric conditions and misrepresent extreme weather. Similar trends hold for other derived variables of meteorological interest (Supplementary Information section H.2 ). Ensemble-mean predictions, from both NeuralGCM and ECMWF, are closer to ERA5 in an average sense, and thus are inherently smooth at long lead times. In contrast, as shown in Fig. 3 and in Supplementary Information section H.3 , individual realizations from the ECMWF and NeuralGCM ensembles remain sharp, even at long lead times. Like ECMWF-ENS, NeuralGCM-ENS produces a statistically representative range of future weather scenarios for each weather phenomenon, despite its eight-times-coarser resolution.
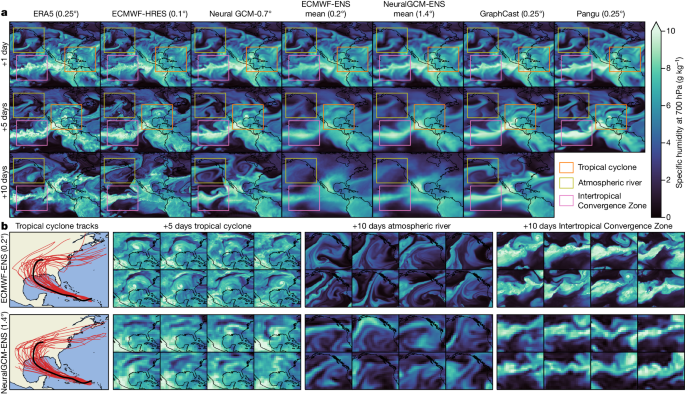
All forecasts are initialized at 2020-08-22T12z, chosen to highlight Hurricane Laura, the most damaging Atlantic hurricane of 2020. a , Specific humidity at 700 hPa for 1-day, 5-day and 10-day forecasts over North America and the Northeast Pacific Ocean from ERA5 14 , ECMWF-HRES, NeuralGCM-0.7°, ECMWF-ENS (mean), NeuralGCM-ENS (mean), GraphCast 3 and Pangu 4 . b , Forecasts from individual ensemble members from ECMWF-ENS and NeuralGCM-ENS over regions of interest, including predicted tracks of Hurricane Laura from each of the 50 ensemble members (Supplementary Information section I.2 ). The track from ERA5 is plotted in black.
We can quantify the blurriness of different forecast models via their power spectra. Supplementary Figs. 17 and 18 show that the power spectra of NeuralCGM-0.7° is consistently closer to ERA5 than the other machine-learning forecast methods, but is still blurrier than ECMWF’s physical forecasts. The spectra of NeuralGCM forecasts is also roughly constant over the forecast period, in stark contrast to GraphCast, which worsens with lead time. The spectrum of NeuralGCM becomes more accurate with increased resolution (Supplementary Fig. 22 ), which suggests the potential for further improvements of NeuralGCM models trained at higher resolutions.
Water budget
In NeuralGCM, advection is handled by the dynamical core, while the machine-learning parameterization models local processes within vertical columns of the atmosphere. Thus, unlike pure machine-learning methods, local sources and sinks can be isolated from tendencies owing to horizontal transport and other resolved dynamics (Supplementary Fig. 3 ). This makes our results more interpretable and facilitates the diagnosis of the water budget. Specifically, we diagnose precipitation minus evaporation (Supplementary Information section H.5 ) rather than directly predicting these as in machine-learning-based approaches 3 . For short weather forecasts, the mean of precipitation minus evaporation has a realistic spatial distribution that is very close to ERA5 data (Extended Data Fig. 4c–e ). The precipitation-minus-evaporation rate distribution of NeuralGCM-0.7° closely matches the ERA5 distribution in the extratropics (Extended Data Fig. 4b ), although it underestimates extreme events in the tropics (Extended Data Fig. 4a ). It is noted that the current version of NeuralGCM directly predicts tendencies for an atmospheric column, and thus cannot distinguish between precipitation and evaporation.
Geostrophic wind balance
We examined the extent to which NeuralGCM, GraphCast and ECMWF-HRES capture the geostrophic wind balance, the near-equilibrium between the dominant forces that drive large-scale dynamics in the mid-latitudes 30 . A recent study 16 highlighted that Pangu misrepresents the vertical structure of the geostrophic and ageostrophic winds and noted a deterioration at longer lead times. Similarly, we observe that GraphCast shows an error that worsens with lead time. In contrast, NeuralGCM more accurately depicts the vertical structure of the geostrophic and ageostrophic winds, as well as their ratio, compared with GraphCast across various rollouts, when compared against ERA5 data (Extended Data Fig. 3 ). However, ECMWF-HRES still shows a slightly closer alignment to ERA5 data than NeuralGCM does. Within NeuralGCM, the representation of the geostrophic wind’s vertical structure only slightly degrades in the initial few days, showing no noticeable changes thereafter, particularly beyond day 5.
Generalizing to unseen data
Physically consistent weather models should still perform well for weather conditions for which they were not trained. We expect that NeuralGCM may generalize better than machine-learning-only atmospheric models, because NeuralGCM employs neural networks that act locally in space, on individual vertical columns of the atmosphere. To explore this hypothesis, we compare versions of NeuralCGM-0.7° and GraphCast trained to 2017 on 5 years of weather forecasts beyond the training period (2018–2022) in Supplementary Fig. 36 . Unlike GraphCast, NeuralGCM does not show a clear trend of increasing error when initialized further into the future from the training data. To extend this test beyond 5 years, we trained a NeuralGCM-2.8° model using only data before 2000, and tested its skill for over 21 unseen years (Supplementary Fig. 35 ).
Climate simulations
Although our deterministic NeuralGCM models are trained to predict weather up to 3 days ahead, they are generally capable of simulating the atmosphere far beyond medium-range weather timescales. For extended climate simulations, we prescribe historical sea surface temperature (SST) and sea-ice concentration. These simulations feature many emergent phenomena of the atmosphere on timescales from months to decades.
For climate simulations with NeuralGCM, we use 2.8° and 1.4° deterministic models, which are relatively inexpensive to train (Supplementary Information section G.7 ) and allow us to explore a larger parameter space to find stable models. Previous studies found that running extended simulations with hybrid models is challenging due to numerical instabilities and climate drift 21 . To quantify stability in our selected models, we run multiple initial conditions and report how many of them finish without instability.
Seasonal cycle and emergent phenomena
To assess the capability of NeuralGCM to simulate various aspects of the seasonal cycle, we run 2-year simulations with NeuralGCM-1.4°. for 37 different initial conditions spaced every 10 days for the year 2019. Out of these 37 initial conditions, 35 successfully complete the full 2 years without instability; for case studies of instability, see Supplementary Information section H.7 , and Supplementary Figs. 26 and 27 . We compare results from NeuralGCM-1.4° for 2020 with ERA5 data and with outputs from the X-SHiELD global cloud-resolving model, which is coupled to an ocean model nudged towards reanalysis 31 . This X-SHiELD run has been used as a target for training machine-learning climate models 24 . For comparison, we evaluate models after regridding predictions to 1.4° resolution. This comparison slightly favours NeuralGCM because NeuralGCM was tuned to match ERA5, but the discrepancy between ERA5 and the actual atmosphere is small relative to model error.
Figure 4a shows the temporal variation of the global mean temperature to 2020, as captured by 35 simulations from NeuralGCM, in comparison with the ERA5 reanalysis and standard climatology benchmarks. The seasonality and variability of the global mean temperature from NeuralGCM are quantitatively similar to those observed in ERA5. The ensemble-mean temperature RMSE for NeuralGCM stands at 0.16 K when benchmarked against ERA5, which is a significant improvement over the climatology’s RMSE of 0.45 K. We find that NeuralGCM accurately simulates the seasonal cycle, as evidenced by metrics such as the annual cycle of the global precipitable water (Supplementary Fig. 30a ) and global total kinetic energy (Supplementary Fig. 30b ). Furthermore, the model captures essential atmospheric dynamics, including the Hadley circulation and the zonal-mean zonal wind (Supplementary Fig. 28 ), as well as the spatial patterns of eddy kinetic energy in different seasons (Supplementary Fig. 31 ), and the distinctive seasonal behaviours of monsoon circulation (Supplementary Fig. 29 ; additional details are provided in Supplementary Information section I.1 ).
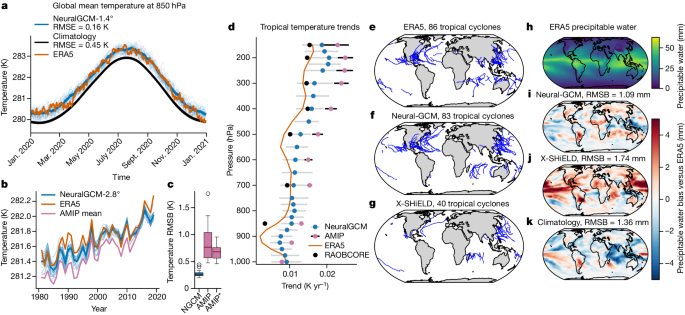
a , Global mean temperature for ERA5 14 (orange), 1990–2019 climatology (black) and NeuralGCM-1.4° (blue) for 2020 using 35 simulations initialized every 10 days during 2019 (thick line, ensemble mean; thin lines, different initial conditions). b , Yearly global mean temperature for ERA5 (orange), mean over 22 CMIP6 AMIP experiments 34 (violet; model details are in Supplementary Information section I.3 ) and NeuralGCM-2.8° for 22 AMIP-like simulations with prescribed SST initialized every 10 days during 1980 (thick line, ensemble mean; thin lines, different initial conditions). c , The RMSB of the 850-hPa temperature averaged between 1981 and 2014 for 22 NeuralGCM-2.8° AMIP runs (labelled NGCM), 22 CMIP6 AMIP experiments (labelled AMIP) and debiased 22 CMIP6 AMIP experiments (labelled AMIP*; bias was removed by removing the 850-hPa global temperature bias). In the box plots, the red line represents the median. The box delineates the first to third quartiles; the whiskers extend to 1.5 times the interquartile range (Q1 − 1.5IQR and Q3 + 1.5IQR), and outliers are shown as individual dots. d , Vertical profiles of tropical (20° S–20° N) temperature trends for 1981–2014. Orange, ERA5; black dots, Radiosonde Observation Correction using Reanalyses (RAOBCORE) 41 ; blue dots, mean trends for NeuralGCM; purple dots, mean trends from CMIP6 AMIP runs (grey and black whiskers, 25th and 75th percentiles for NeuralGCM and CMIP6 AMIP runs, respectively). e – g , Tropical cyclone tracks for ERA5 ( e ), NeuralGCM-1.4° ( f ) and X-SHiELD 31 ( g ). h – k , Mean precipitable water for ERA5 ( h ) and the precipitable water bias in NeuralGCM-1.4° ( i ), initialized 90 days before mid-January 2020 similarly to X-SHiELD, X-SHiELD ( j ) and climatology ( k ; averaged between 1990 and 2019). In d – i , quantities are calculated between mid-January 2020 and mid-January 2021 and all models were regridded to a 256 × 128 Gaussian grid before computation and tracking.
Next, we compare the annual biases of a single NeuralGCM realization with a single realization of X-SHiELD (the only one available), both initiated in mid-October 2019. We consider 19 January 2020 to 17 January 2021, the time frame for which X-SHiELD data are available. Global cloud-resolving models, such as X-SHiELD, are considered state of the art, especially for simulating the hydrological cycle, owing to their resolution being capable of resolving deep convection 32 . The annual bias in precipitable water for NeuralGCM (RMSE of 1.09 mm) is substantially smaller than the biases of both X-SHiELD (RMSE of 1.74 mm) and climatology (RMSE of 1.36 mm; Fig. 4i–k ). Moreover, NeuralGCM shows a lower temperature bias in the upper and lower troposphere than X-SHiELD (Extended Data Fig. 6 ). We also indirectly compare precipitation bias in X-SHiELD with precipitation-minus-evaporation bias in NeuralGCM-1.4°, which shows slightly larger bias and grid-scale artefacts for NeuralGCM (Extended Data Fig. 5 ).
Finally, to assess the capability of NeuralGCM to generate tropical cyclones in an annual model integration, we use the tropical cyclone tracker TempestExtremes 33 , as described in Supplementary Information section I.2 , Supplementary Fig. 34 and Supplementary Table 6 . Figure 4e–g shows that NeuralGCM, even at a coarse resolution of 1.4°, produces realistic trajectories and counts of tropical cyclone (83 versus 86 in ERA5 for the corresponding period), whereas X-SHiELD, when regridded to 1.4° resolution, substantially underestimates the tropical cyclone count (40). Additional statistical analyses of tropical cyclones can be found in Extended Data Figs. 7 and 8 .
Decadal simulations
To assess the capability of NeuralGCM to simulate historical temperature trends, we conduct AMIP-like simulations over a duration of 40 years with NeuralGCM-2.8°. Out of 37 different runs with initial conditions spaced every 10 days during the year 1980, 22 simulations were stable for the entire 40-year period, and our analysis focuses on these results. We compare with 22 simulations run with prescribed SST from the Coupled Model Intercomparison Project Phase 6 (CMIP6) 34 , listed in Supplementary Information section I.3 .
We find that all 40-year simulations of NeuralGCM, as well as the mean of the 22 AMIP runs, accurately capture the global warming trends observed in ERA5 data (Fig. 4b ). There is a strong correlation in the year-to-year temperature trends with ERA5 data, suggesting that NeuralGCM effectively captures the impact of SST forcing on climate. When comparing spatial biases averaged over 1981–2014, we find that all 22 NeuralGCM-2.8° runs have smaller bias than the CMIP6 AMIP runs, and this result remains even when removing the global temperature bias in CMIP6 AMIP runs (Fig. 4c and Supplementary Figs. 32 and 33 ).
Next, we investigated the vertical structure of tropical warming trends, which climate models tend to overestimate in the upper troposphere 35 . As shown in Fig. 4d , the trends, calculated by linear regression, of NeuralGCM are closer to ERA5 than those of AMIP runs. In particular, the bias in the upper troposphere is reduced. However, NeuralGCM does show a wider spread in its predictions than the AMIP runs, even at levels near the surface where temperatures are typically more constrained by prescribed SST.
Lastly, we evaluated NeuralGCM’s capability to generalize to unseen warmer climates by conducting AMIP simulations with increased SST (Supplementary Information section I.4.2 ). We find that NeuralGCM shows some of the robust features of climate warming response to modest SST increases (+1 K and +2 K); however, for more substantial SST increases (+4 K), NeuralGCM’s response diverges from expectations (Supplementary Fig. 37 ). In addition, AMIP simulations with increased SST show climate drift, underscoring NeuralGCM’s limitations in this context (Supplementary Fig. 38 ).
NeuralGCM is a differentiable hybrid atmospheric model that combines the strengths of traditional GCMs with machine learning for weather forecasting and climate simulation. To our knowledge, NeuralGCM is the first machine-learning-based model to make accurate ensemble weather forecasts, with better CRPS than state-of-the-art physics-based models. It is also, to our knowledge, the first hybrid model that achieves comparable spatial bias to global cloud-resolving models, can simulate realistic tropical cyclone tracks and can run AMIP-like simulations with realistic historical temperature trends. Overall, NeuralGCM demonstrates that incorporating machine learning is a viable alternative to building increasingly detailed physical models 32 for improving GCMs.
Compared with traditional GCMs with similar skill, NeuralGCM is computationally efficient and low complexity. NeuralGCM runs at 8- to 40-times-coarser horizontal resolution than ECMWF’s Integrated Forecasting System and global cloud-resolving models, which enables 3 to 5 orders of magnitude savings in computational resources. For example, NeuralGCM-1.4° simulates 70,000 simulation days in 24 hours using a single tensor-processing-unit versus 19 simulated days on 13,824 central-processing-unit cores with X-SHiELD (Extended Data Table 1 ). This can be leveraged for previously impractical tasks such as large ensemble forecasting. NeuralGCM’s dynamical core uses global spectral methods 36 , and learned physics is parameterized with fully connected neural networks acting on single vertical columns. Substantial headroom exists to pursue higher accuracy using advanced numerical methods and machine-learning architectures.
Our results provide strong evidence for the disputed hypothesis 37 , 38 , 39 that learning to predict short-term weather is an effective way to tune parameterizations for climate. NeuralGCM models trained on 72-hour forecasts are capable of realistic multi-year simulation. When provided with historical SSTs, they capture essential atmospheric dynamics such as seasonal circulation, monsoons and tropical cyclones. However, we will probably need alternative training strategies 38 , 39 to learn important processes for climate with subtle impacts on weather timescales, such as a cloud feedback.
The NeuralGCM approach is compatible with incorporating either more physics or more machine learning, as required for operational weather forecasts and climate simulations. For weather forecasting, we expect that end-to-end learning 40 with observational data will allow for better and more relevant predictions, including key variables such as precipitation. Such models could include neural networks acting as corrections to traditional data assimilation and model diagnostics. For climate projection, NeuralGCM will need to be reformulated to enable coupling with other Earth-system components (for example, ocean and land), and integrating data on the atmospheric chemical composition (for example, greenhouse gases and aerosols). There are also research challenges common to current machine-learning-based climate models 19 , including the capability to simulate unprecedented climates (that is, generalization), adhering to physical constraints, and resolving numerical instabilities and climate drift. NeuralGCM’s flexibility to incorporate physics-based models (for example, radiation) offers a promising avenue to address these challenges.
Models based on physical laws and empirical relationships are ubiquitous in science. We believe the differentiable hybrid modelling approach of NeuralGCM has the potential to transform simulation for a wide range of applications, such as materials discovery, protein folding and multiphysics engineering design.
Differentiable atmospheric model
NeuralGCM combines components of the numerical solver and flexible neural network parameterizations. Simulation in time is carried out in a coordinate system suitable for solving the dynamical equations of the atmosphere, describing large-scale fluid motion and thermodynamics under the influence of gravity and the Coriolis force.
Our differentiable dynamical core is implemented in JAX, a library for high-performance code in Python that supports automatic differentiation 42 . The dynamical core solves the hydrostatic primitive equations with moisture, using a horizontal pseudo-spectral discretization and vertical sigma coordinates 36 , 43 . We evolve seven prognostic variables: vorticity and divergence of horizontal wind, temperature, surface pressure, and three water species (specific humidity, and specific ice and liquid cloud water content).
Our learned physics module uses the single-column approach of GCMs 2 , whereby information from only a single atmospheric column is used to predict the impact of unresolved processes occurring within that column. These effects are predicted using a fully connected neural network with residual connections, with weights shared across all atmospheric columns (Supplementary Information section C.4 ).
The inputs to the neural network include the prognostic variables in the atmospheric column, total incident solar radiation, sea-ice concentration and SST (Supplementary Information section C.1 ). We also provide horizontal gradients of the prognostic variables, which we found improves performance 44 . All inputs are standardized to have zero mean and unit variance using statistics precomputed during model initialization. The outputs are the prognostic variable tendencies scaled by the fixed unconditional standard deviation of the target field (Supplementary Information section C.5 ).
To interface between ERA5 14 data stored in pressure coordinates and the sigma coordinate system of our dynamical core, we introduce encoder and decoder components (Supplementary Information section D ). These components perform linear interpolation between pressure levels and sigma coordinate levels. We additionally introduce learned corrections to both encoder and decoder steps (Supplementary Figs. 4–6 ), using the same column-based neural network architecture as the learned physics module. Importantly, the encoder enables us to eliminate the gravity waves from initialization shock 45 , which otherwise contaminate forecasts.
Figure 1a shows the sequence of steps that NeuralGCM takes to make a forecast. First, it encodes ERA5 data at t = t 0 on pressure levels to initial conditions on sigma coordinates. To perform a time step, the dynamical core and learned physics (Fig. 1b ) then compute tendencies, which are integrated in time using an implicit–explicit ordinary differential equation solver 46 (Supplementary Information section E and Supplementary Table 2 ). This is repeated to advance the model from t = t 0 to t = t final . Finally, the decoder converts predictions back to pressure levels.
The time-step size of the ODE solver (Supplementary Table 3 ) is limited by the Courant–Friedrichs–Lewy condition on dynamics, and can be small relative to the timescale of atmospheric change. Evaluating learned physics is approximately 1.5 times as expensive as a time step of the dynamical core. Accordingly, following the typical practice for GCMs, we hold learned physics tendencies constant for multiple ODE time steps to reduce computational expense, typically corresponding to 30 minutes of simulation time.
Deterministic and stochastic models
We train deterministic NeuralGCM models using a combination of three loss functions (Supplementary Information section G.4 ) to encourage accuracy and sharpness while penalizing bias. During the main training phase, all losses are defined in a spherical harmonics basis. We use a standard mean squared error loss for prompting accuracy, modified to progressively filter out contributions from higher total wavenumbers at longer lead times (Supplementary Fig. 8 ). This filtering approach tackles the ‘double penalty problem’ 47 as it prevents the model from being penalized for predicting high-wavenumber features in incorrect locations at later times, especially beyond the predictability horizon. A second loss term encourages the spectrum to match the training data using squared loss on the total wavenumber spectrum of prognostic variables. These first two losses are evaluated on both sigma and pressure levels. Finally, a third loss term discourages bias by adding mean squared error on the batch-averaged mean amplitude of each spherical harmonic coefficient. For analysis of the impact that various loss functions have, refer to Supplementary Information section H.6.1 , and Supplementary Figs. 23 and 24 . The combined action of the three training losses allow the resulting models trained on 3-day rollouts to remain stable during years-to-decades-long climate simulations. Before final evaluations, we perform additional fine-tuning of just the decoder component on short rollouts of 24 hours (Supplementary Information section G.5 ).
Stochastic NeuralGCM models incorporate inherent randomness in the form of additional random fields passed as inputs to neural network components. Our stochastic loss is based on the CRPS 28 , 48 , 49 . CRPS consists of mean absolute error that encourages accuracy, balanced by a similar term that encourages ensemble spread. For each variable we use a sum of CRPS in grid space and CRPS in the spherical harmonic basis below a maximum cut-off wavenumber (Supplementary Information section G.6 ). We compute CRPS on rollout lengths from 6 hours to 5 days. As illustrated in Fig. 1 , we inject noise to the learned encoder and the learned physics module by sampling from Gaussian random fields with learned spatial and temporal correlation (Supplementary Information section C.2 and Supplementary Fig. 2 ). For training, we generate two ensemble members per forecast, which suffices for an unbiased estimate of CRPS.
Data availability
For training and evaluating the NeuralGCM models, we used the publicly available ERA5 dataset 14 , originally downloaded from https://cds.climate.copernicus.eu/ and available via Google Cloud Storage in Zarr format at gs://gcp-public-data-arco-era5/ar/full_37-1h-0p25deg-chunk-1.zarr-v3. To compare NeuralGCM with operational and data-driven weather models, we used forecast datasets distributed as part of WeatherBench2 12 at https://weatherbench2.readthedocs.io/en/latest/data-guide.html , to which we have added NeuralGCM forecasts for 2020. To compare NeuralGCM with atmospheric models in climate settings, we used CMIP6 data available at https://catalog.pangeo.io/browse/master/climate/ , as well as X-SHiELD 24 outputs available on Google Cloud storage in a ‘requester pays’ bucket at gs://ai2cm-public-requester-pays/C3072-to-C384-res-diagnostics. The Radiosonde Observation Correction using Reanalyses (RAOBCORE) V1.9 that was used as reference tropical temperature trends was downloaded from https://webdata.wolke.img.univie.ac.at/haimberger/v1.9/ . Base maps use freely available data from https://www.naturalearthdata.com/downloads/ .
Code availability
The NeuralGCM code base is separated into two open source projects: Dinosaur and NeuralGCM, both publicly available on GitHub at https://github.com/google-research/dinosaur (ref. 50 ) and https://github.com/google-research/neuralgcm (ref. 51 ). The Dinosaur package implements a differentiable dynamical core used by NeuralGCM, whereas the NeuralGCM package provides machine-learning models and checkpoints of trained models. Evaluation code for NeuralGCM weather forecasts is included in WeatherBench2 12 , available at https://github.com/google-research/weatherbench2 (ref. 52 ).
Bauer, P., Thorpe, A. & Brunet, G. The quiet revolution of numerical weather prediction. Nature 525 , 47–55 (2015).
Article ADS CAS PubMed Google Scholar
Balaji, V. et al. Are general circulation models obsolete? Proc. Natl Acad. Sci. USA 119 , e2202075119 (2022).
Article CAS PubMed PubMed Central Google Scholar
Lam, R. et al. Learning skillful medium-range global weather forecasting. Science 382 , 1416–1421 (2023).
Article ADS MathSciNet CAS PubMed Google Scholar
Bi, K. et al. Accurate medium-range global weather forecasting with 3D neural networks. Nature 619 , 533–538 (2023).
Article ADS CAS PubMed PubMed Central Google Scholar
Hourdin, F. et al. The art and science of climate model tuning. Bull. Am. Meteorol. Soc. 98 , 589–602 (2017).
Article ADS Google Scholar
Bony, S. & Dufresne, J.-L. Marine boundary layer clouds at the heart of tropical cloud feedback uncertainties in climate models. Geophys. Res. Lett. 32 , L20806 (2005).
Webb, M. J., Lambert, F. H. & Gregory, J. M. Origins of differences in climate sensitivity, forcing and feedback in climate models. Clim. Dyn. 40 , 677–707 (2013).
Article Google Scholar
Sherwood, S. C., Bony, S. & Dufresne, J.-L. Spread in model climate sensitivity traced to atmospheric convective mixing. Nature 505 , 37–42 (2014).
Article ADS PubMed Google Scholar
Palmer, T. & Stevens, B. The scientific challenge of understanding and estimating climate change. Proc. Natl Acad. Sci. USA 116 , 24390–24395 (2019).
Fischer, E. M., Beyerle, U. & Knutti, R. Robust spatially aggregated projections of climate extremes. Nat. Clim. Change 3 , 1033–1038 (2013).
Field, C. B. Managing the Risks of Extreme Events and Disasters to Advance Climate Change Adaptation: Special Report of the Intergovernmental Panel on Climate Change (Cambridge Univ. Press, 2012).
Rasp, S. et al. WeatherBench 2: A benchmark for the next generation of data-driven global weather models. J. Adv. Model. Earth Syst. 16 , e2023MS004019 (2024).
Keisler, R. Forecasting global weather with graph neural networks. Preprint at https://arxiv.org/abs/2202.07575 (2022).
Hersbach, H. et al. The ERA5 global reanalysis. Q. J. R. Meteorol. Soc. 146 , 1999–2049 (2020).
Zhou, L. et al. Toward convective-scale prediction within the next generation global prediction system. Bull. Am. Meteorol. Soc. 100 , 1225–1243 (2019).
Bonavita, M. On some limitations of current machine learning weather prediction models. Geophys. Res. Lett. 51 , e2023GL107377 (2024).
Weyn, J. A., Durran, D. R. & Caruana, R. Improving data-driven global weather prediction using deep convolutional neural networks on a cubed sphere. J. Adv. Model. Earth Syst. 12 , e2020MS002109 (2020).
Watt-Meyer, O. et al. ACE: a fast, skillful learned global atmospheric model for climate prediction. Preprint at https://arxiv.org/abs/2310.02074 (2023).
Bretherton, C. S. Old dog, new trick: reservoir computing advances machine learning for climate modeling. Geophys. Res. Lett. 50 , e2023GL104174 (2023).
Reichstein, M. et al. Deep learning and process understanding for data-driven Earth system science. Nature 566 , 195–204 (2019).
Brenowitz, N. D. & Bretherton, C. S. Spatially extended tests of a neural network parametrization trained by coarse-graining. J. Adv. Model. Earth Syst. 11 , 2728–2744 (2019).
Rasp, S., Pritchard, M. S. & Gentine, P. Deep learning to represent subgrid processes in climate models. Proc. Natl Acad. Sci. USA 115 , 9684–9689 (2018).
Yuval, J. & O’Gorman, P. A. Stable machine-learning parameterization of subgrid processes for climate modeling at a range of resolutions. Nat. Commun. 11 , 3295 (2020).
Kwa, A. et al. Machine-learned climate model corrections from a global storm-resolving model: performance across the annual cycle. J. Adv. Model. Earth Syst. 15 , e2022MS003400 (2023).
Arcomano, T., Szunyogh, I., Wikner, A., Hunt, B. R. & Ott, E. A hybrid atmospheric model incorporating machine learning can capture dynamical processes not captured by its physics-based component. Geophys. Res. Lett. 50 , e2022GL102649 (2023).
Han, Y., Zhang, G. J. & Wang, Y. An ensemble of neural networks for moist physics processes, its generalizability and stable integration. J. Adv. Model. Earth Syst. 15 , e2022MS003508 (2023).
Gelbrecht, M., White, A., Bathiany, S. & Boers, N. Differentiable programming for Earth system modeling. Geosci. Model Dev. 16 , 3123–3135 (2023).
Gneiting, T. & Raftery, A. E. Strictly proper scoring rules, prediction, and estimation. J. Am. Stat. Assoc. 102 , 359–378 (2007).
Article MathSciNet CAS Google Scholar
Fortin, V., Abaza, M., Anctil, F. & Turcotte, R. Why should ensemble spread match the RMSE of the ensemble mean? J. Hydrometeorol. 15 , 1708–1713 (2014).
Holton, J. R. An introduction to Dynamic Meteorology 5th edn (Elsevier, 2004).
Cheng, K.-Y. et al. Impact of warmer sea surface temperature on the global pattern of intense convection: insights from a global storm resolving model. Geophys. Res. Lett. 49 , e2022GL099796 (2022).
Stevens, B. et al. DYAMOND: the dynamics of the atmospheric general circulation modeled on non-hydrostatic domains. Prog. Earth Planet. Sci. 6 , 61 (2019).
Ullrich, P. A. et al. TempestExtremes v2.1: a community framework for feature detection, tracking, and analysis in large datasets. Geosc. Model Dev. 14 , 5023–5048 (2021).
Eyring, V. et al. Overview of the Coupled Model Intercomparison Project Phase 6 (CMIP6) experimental design and organization. Geosci. Model Dev. 9 , 1937–1958 (2016).
Mitchell, D. M., Lo, Y. E., Seviour, W. J., Haimberger, L. & Polvani, L. M. The vertical profile of recent tropical temperature trends: persistent model biases in the context of internal variability. Environ. Res. Lett. 15 , 1040b4 (2020).
Bourke, W. A multi-level spectral model. I. Formulation and hemispheric integrations. Mon. Weather Rev. 102 , 687–701 (1974).
Ruiz, J. J., Pulido, M. & Miyoshi, T. Estimating model parameters with ensemble-based data assimilation: a review. J. Meteorol. Soc. Jpn Ser. II 91 , 79–99 (2013).
Schneider, T., Lan, S., Stuart, A. & Teixeira, J. Earth system modeling 2.0: a blueprint for models that learn from observations and targeted high-resolution simulations. Geophys. Res. Lett. 44 , 12–396 (2017).
Schneider, T., Leung, L. R. & Wills, R. C. J. Opinion: Optimizing climate models with process knowledge, resolution, and artificial intelligence. Atmos. Chem. Phys. 24 , 7041–7062 (2024).
Sutskever, I., Vinyals, O. & Le, Q. V. Sequence to sequence learning with neural networks. Adv. Neural Inf. Process. Syst. 27 , 3104–3112 (2014).
Haimberger, L., Tavolato, C. & Sperka, S. Toward elimination of the warm bias in historic radiosonde temperature records—some new results from a comprehensive intercomparison of upper-air data. J. Clim. 21 , 4587–4606 (2008).
Bradbury, J. et al. JAX: composable transformations of Python+NumPy programs. GitHub http://github.com/google/jax (2018).
Durran, D. R. Numerical Methods for Fluid Dynamics: With Applications to Geophysics Vol. 32, 2nd edn (Springer, 2010).
Wang, P., Yuval, J. & O’Gorman, P. A. Non-local parameterization of atmospheric subgrid processes with neural networks. J. Adv. Model. Earth Syst. 14 , e2022MS002984 (2022).
Daley, R. Normal mode initialization. Rev. Geophys. 19 , 450–468 (1981).
Whitaker, J. S. & Kar, S. K. Implicit–explicit Runge–Kutta methods for fast–slow wave problems. Mon. Weather Rev. 141 , 3426–3434 (2013).
Gilleland, E., Ahijevych, D., Brown, B. G., Casati, B. & Ebert, E. E. Intercomparison of spatial forecast verification methods. Weather Forecast. 24 , 1416–1430 (2009).
Rasp, S. & Lerch, S. Neural networks for postprocessing ensemble weather forecasts. Month. Weather Rev. 146 , 3885–3900 (2018).
Pacchiardi, L., Adewoyin, R., Dueben, P. & Dutta, R. Probabilistic forecasting with generative networks via scoring rule minimization. J. Mach. Learn. Res. 25 , 1–64 (2024).
Smith, J. A., Kochkov, D., Norgaard, P., Yuval, J. & Hoyer, S. google-research/dinosaur: 1.0.0. Zenodo https://doi.org/10.5281/zenodo.11376145 (2024).
Kochkov, D. et al. google-research/neuralgcm: 1.0.0. Zenodo https://doi.org/10.5281/zenodo.11376143 (2024).
Rasp, S. et al. google-research/weatherbench2: v0.2.0. Zenodo https://doi.org/10.5281/zenodo.11376271 (2023).
Download references
Acknowledgements
We thank A. Kwa, A. Merose and K. Shah for assistance with data acquisition and handling; L. Zepeda-Núñez for feedback on the paper; and J. Anderson, C. Van Arsdale, R. Chemke, G. Dresdner, J. Gilmer, J. Hickey, N. Lutsko, G. Nearing, A. Paszke, J. Platt, S. Ponda, M. Pritchard, D. Rothenberg, F. Sha, T. Schneider and O. Voicu for discussions.
Author information
These authors contributed equally: Dmitrii Kochkov, Janni Yuval, Ian Langmore, Peter Norgaard, Jamie Smith, Stephan Hoyer
Authors and Affiliations
Google Research, Mountain View, CA, USA
Dmitrii Kochkov, Janni Yuval, Ian Langmore, Peter Norgaard, Jamie Smith, Griffin Mooers, James Lottes, Stephan Rasp, Michael P. Brenner & Stephan Hoyer
Earth, Atmospheric and Planetary Sciences, Massachusetts Institute of Technology, Cambridge, MA, USA
Milan Klöwer
European Centre for Medium-Range Weather Forecasts, Reading, UK
Peter Düben & Sam Hatfield
Google DeepMind, London, UK
Peter Battaglia, Alvaro Sanchez-Gonzalez & Matthew Willson
School of Engineering and Applied Sciences, Harvard University, Cambridge, MA, USA
Michael P. Brenner
You can also search for this author in PubMed Google Scholar
Contributions
D.K., J.Y., I.L., P.N., J.S. and S. Hoyer contributed equally to this work. D.K., J.Y., I.L., P.N., J.S., G.M., J.L. and S. Hoyer wrote the code. D.K., J.Y., I.L., P.N., G.M. and S. Hoyer trained models and analysed the data. M.P.B. and S. Hoyer managed and oversaw the research project. M.K., S.R., P.D., S. Hatfield, P.B. and M.P.B. contributed technical advice and ideas. M.W. ran experiments with GraphCast for comparison with NeuralGCM. A.S.-G. assisted with data preparation. D.K., J.Y., I.L., P.N. and S. Hoyer wrote the paper. All authors gave feedback and contributed to editing the paper.
Corresponding authors
Correspondence to Dmitrii Kochkov , Janni Yuval or Stephan Hoyer .
Ethics declarations
Competing interests.
D.K., J.Y., I.L., P.N., J.S., J.L., S.R., P.B., A.S.-G., M.W., M.P.B. and S. Hoyer are employees of Google. S. Hoyer, D.K., I.L., J.Y., G.M., P.N., J.S. and M.B. have filed international patent application PCT/US2023/035420 in the name of Google LLC, currently pending, relating to neural general circulation models.
Peer review
Peer review information.
Nature thanks Karthik Kashinath and the other, anonymous, reviewer(s) for their contribution to the peer review of this work. Peer reviewer reports are available.
Additional information
Publisher’s note Springer Nature remains neutral with regard to jurisdictional claims in published maps and institutional affiliations.
Extended data figures and tables
Extended data fig. 1 maps of bias for neuralgcm-ens and ecmwf-ens forecasts..
Bias is averaged over all forecasts initialized in 2020.
Extended Data Fig. 2 Maps of spread-skill ratio for NeuralGCM-ENS and ECMWF-ENS forecasts.
Spread-skill ratio is averaged over all forecasts initialized in 2020.
Extended Data Fig. 3 Geostrophic balance in NeuralGCM, GraphCast 3 and ECMWF-HRES.
Vertical profiles of the extratropical intensity (averaged between latitude 30°–70° in both hemispheres) and over all forecasts initialized in 2020 of (a,d,g) geostrophic wind, (b,e,h) ageostrophic wind and (c,f,i) the ratio of the intensity of ageostrophic wind over geostrophic wind for ERA5 (black continuous line in all panels), (a,b,c) NeuralGCM-0.7°, (d,e,f) GraphCast and (g,h,i) ECMWF-HRES at lead times of 1 day, 5 days and 10 days.
Extended Data Fig. 4 Precipitation minus evaporation calculated from the third day of weather forecasts.
(a) Tropical (latitudes −20° to 20°) precipitation minus evaporation (P minus E) rate distribution, (b) Extratropical (latitudes 30° to 70° in both hemispheres) P minus E, (c) mean P minus E for 2020 ERA5 14 and (d) NeuralGCM-0.7° (calculated from the third day of forecasts and averaged over all forecasts initialized in 2020), (e) the bias between NeuralGCM-0.7° and ERA5, (f-g) Snapshot of daily precipitation minus evaporation for 2020-01-04 for (f) NeuralGCM-0.7° (forecast initialized on 2020-01-02) and (g) ERA5.
Extended Data Fig. 5 Indirect comparison between precipitation bias in X-SHiELD and precipitation minus evaporation bias in NeuralGCM-1.4°.
Mean precipitation calculated between 2020-01-19 and 2021-01-17 for (a) ERA5 14 (c) X-SHiELD 31 and the biases in (e) X-SHiELD and (g) climatology (ERA5 data averaged over 1990-2019). Mean precipitation minus evaporation calculated between 2020-01-19 and 2021-01-17 for (b) ERA5 (d) NeuralGCM-1.4° (initialized in October 18th 2019) and the biases in (f) NeuralGCM-1.4° and (h) climatology (data averaged over 1990–2019).
Extended Data Fig. 6 Yearly temperature bias for NeuralGCM and X-SHiELD 31 .
Mean temperature between 2020-01-19 to 2020-01-17 for (a) ERA5 at 200hPa and (b) 850hPa. (c,d) the bias in the temperature for NeuralGCM-1.4°, (e,f) the bias in X-SHiELD and (g,h) the bias in climatology (calculated from 1990–2019). NeuralGCM-1.4° was initialized in 18th of October (similar to X-SHiELD).
Extended Data Fig. 7 Tropical Cyclone densities and annual regional counts.
(a) Tropical Cyclone (TC) density from ERA5 14 data spanning 1987–2020. (b) TC density from NeuralGCM-1.4° for 2020, generated using 34 different initial conditions all initialized in 2019. (c) Box plot depicting the annual number of TCs across different regions, based on ERA5 data (1987–2020), NeuralGCM-1.4° for 2020 (34 initial conditions), and orange markers show ERA5 for 2020. In the box plots, the red line represents the median; the box delineates the first to third quartiles; the whiskers extend to 1.5 times the interquartile range (Q1 − 1.5IQR and Q3 + 1.5IQR), and outliers are shown as individual dots. Each year is defined from January 19th to January 17th of the following year, aligning with data availability from X-SHiELD. For NeuralGCM simulations, the 3 initial conditions starting in January 2019 exclude data for January 17th, 2021, as these runs spanned only two years.
Extended Data Fig. 8 Tropical Cyclone maximum wind distribution in NeuralGCM vs. ERA5 14 .
Number of Tropical Cyclones (TCs) as a function of maximum wind speed at 850hPa across different regions, based on ERA5 data (1987–2020; in orange), and NeuralGCM-1.4° for 2020 (34 initial conditions; in blue). Each year is defined from January 19th to January 17th of the following year, aligning with data availability from X-SHiELD. For NeuralGCM simulations, the 3 initial conditions starting in January 2019 exclude data for January 17th, 2021, as these runs spanned only two years.
Supplementary information
Supplementary information.
Supplementary Information (38 figures, 6 tables): (A) Lines of code in atmospheric models; (B) Dynamical core of NeuralGCM; (C) Learned physics of NeuralGCM; (D) Encoder and decoder of NeuralGCM; (E) Time integration; (F) Evaluation metrics; (G) Training; (H) Additional weather evaluations; (I) Additional climate evaluations.
Peer Review File
Rights and permissions.
Open Access This article is licensed under a Creative Commons Attribution 4.0 International License, which permits use, sharing, adaptation, distribution and reproduction in any medium or format, as long as you give appropriate credit to the original author(s) and the source, provide a link to the Creative Commons licence, and indicate if changes were made. The images or other third party material in this article are included in the article’s Creative Commons licence, unless indicated otherwise in a credit line to the material. If material is not included in the article’s Creative Commons licence and your intended use is not permitted by statutory regulation or exceeds the permitted use, you will need to obtain permission directly from the copyright holder. To view a copy of this licence, visit http://creativecommons.org/licenses/by/4.0/ .
Reprints and permissions
About this article
Cite this article.
Kochkov, D., Yuval, J., Langmore, I. et al. Neural general circulation models for weather and climate. Nature (2024). https://doi.org/10.1038/s41586-024-07744-y
Download citation
Received : 13 November 2023
Accepted : 15 June 2024
Published : 22 July 2024
DOI : https://doi.org/10.1038/s41586-024-07744-y
Share this article
Anyone you share the following link with will be able to read this content:
Sorry, a shareable link is not currently available for this article.
Provided by the Springer Nature SharedIt content-sharing initiative
This article is cited by
Google ai predicts long-term climate trends and weather — in minutes.
- Helena Kudiabor
Nature (2024)
Weather and climate predicted accurately — without using a supercomputer
- Oliver Watt-Meyer
By submitting a comment you agree to abide by our Terms and Community Guidelines . If you find something abusive or that does not comply with our terms or guidelines please flag it as inappropriate.
Quick links
- Explore articles by subject
- Guide to authors
- Editorial policies
Sign up for the Nature Briefing: AI and Robotics newsletter — what matters in AI and robotics research, free to your inbox weekly.


IMAGES
COMMENTS
Revised on June 22, 2023. An observational study is used to answer a research question based purely on what the researcher observes. There is no interference or manipulation of the research subjects, and no control and treatment groups. These studies are often qualitative in nature and can be used for both exploratory and explanatory research ...
2.1 Introduction. Observation is one of the most important research methods in social sci-. ences and at the same time one of the most diverse. e term includes. several types, techniques, and ...
Definition: Observation is the process of collecting and recording data by observing and noting events, behaviors, or phenomena in a systematic and objective manner. It is a fundamental method used in research, scientific inquiry, and everyday life to gain an understanding of the world around us.
Observation in qualitative research "is one of the oldest and most fundamental research methods approaches. This approach involves collecting data using one's senses, especially looking and listening in a systematic and meaningful way" (McKechnie, 2008, p. 573).Similarly, Adler and Adler (1994) characterized observations as the "fundamental base of all research methods" in the social ...
A way to gather data by watching people, events, or noting physical characteristics in their natural setting. Observations can be overt (subjects know they are being observed) or covert (do not know they are being watched). Participant Observation. Researcher becomes a participant in the culture or context being observed.
Health research study designs benefit from observations of behaviors and contexts. •. Direct observation methods have a long history in the social sciences. •. Social science approaches should be adapted for health researchers' unique needs. •. Health research observations should be feasible, well-defined and piloted.
Observation. Observation is a type of qualitative research method which not only included participant's observation, but also covered ethnography and research work in the field. In the observational research design, multiple study sites are involved. Observational data can be integrated as auxiliary or confirmatory research.
Observation. Observation, as the name implies, is a way of collecting data through observing. This data collection method is classified as a participatory study, because the researcher has to immerse herself in the setting where her respondents are, while taking notes and/or recording. Observation data collection method may involve watching ...
This paper explores the validity of qualitative observational research methods, specifically participant observation. Through an exploration of the relevant literature and a critical review of a ...
Revised on June 22, 2023. Naturalistic observation is a qualitative research method where you record the behaviors of your research subjects in real world settings. You avoid interfering with or influencing any variables in a naturalistic observation. You can think of naturalistic observation as "people watching" with a purpose.
2.2 Observational Research Design 2.2.1 Research Aims The choice of method must always be adapted to the initial research problem and the scientific context of the study. Observation can be either the main method in a project or one of several complementary qualita-tive methods. At the outset of a research project, it may give an inspira-
Participant observation is a common research method in social sciences, with findings often published in research reports used to inform policymakers or other stakeholders. Example: Rural community participant observation. You are studying the social dynamics of a small rural community located near where you grew up.
Formating your Observational Research Paper. Content and Formating Guidelines for your Observational Research Paper. The main components of your paper include: A Title page, Introduction, Method, Results, Conclusions (or Discussion), References, your diagram of the room layout and your observation notes/checklist.
Qualitative research methods. Each of the research approaches involve using one or more data collection methods.These are some of the most common qualitative methods: Observations: recording what you have seen, heard, or encountered in detailed field notes. Interviews: personally asking people questions in one-on-one conversations. Focus groups: asking questions and generating discussion among ...
BENEFITS OF OBSERVATION. tion method observation has a number of clear benefits:It can be used to study complex or new behaviours It allows the researchers to see what really happens It allows research in the '. atural setting' A lot of data can be collected quickly. They can be used to study 'wicked problems' (those which are di.
Examples of Observational Research. 1. Jane Goodall's Research. Jane Goodall is famous for her discovery that chimpanzees use tools. It is one of the most remarkable findings in psychology and anthropology. Her primary method of study involved simply entering the natural habitat of her research subjects, sitting down with pencil and paper ...
The paper concludes with five recommendations for using observation to advance the state of. research on creativity and education. Keywords: observation, methods, quantitative, qualititative ...
Olivia Guy-Evans, MSc. Research methods in psychology are systematic procedures used to observe, describe, predict, and explain behavior and mental processes. They include experiments, surveys, case studies, and naturalistic observations, ensuring data collection is objective and reliable to understand and explain psychological phenomena.
Quantitative research methods are used to collect and analyze numerical data. This type of research is useful when the objective is to test a hypothesis, determine cause-and-effect relationships, and measure the prevalence of certain phenomena. Quantitative research methods include surveys, experiments, and secondary data analysis.
The observational finding shows that colour-coded waste bins are available in 23 (9.6%) rooms. 90% of the sharp containers were reusable, and 100% of the waste storage bins were plastic buckets that were easily cleanable. ... similar to household waste, i.e. plastic, papers and leftover foods. This study aimed to investigate solid healthcare ...
DisTrO has already been tested and shown in a Nous Research technical paper to yield an 857 times efficiency increase compared to one popular existing training algorithm, All-Reduce, as well as a ...
Observation method is "a data collection m ethod in which a person (usually trained) observes. subjects of phenomena and records inf or mation a bout characteristics of the ph eno mena ...
Research methods are specific procedures for collecting and analyzing data. Developing your research methods is an integral part of your research design. When planning your methods, there are two key decisions you will make. First, decide how you will collect data. Your methods depend on what type of data you need to answer your research question:
Queen guitarist Brian May has spent a decade studying the science of bovine tuberculosis, which can be carried by badgers, and has identified a new method of spread
Research papers in the social and natural sciences often follow APA style. This article focuses on reporting quantitative research methods. In your APA methods section, you should report enough information to understand and replicate your study, including detailed information on the sample, measures, and procedures used.
General circulation models (GCMs) are the foundation of weather and climate prediction 1,2.GCMs are physics-based simulators that combine a numerical solver for large-scale dynamics with tuned ...