- A-Z Publications

Annual Review of Statistics and Its Application
Volume 8, 2021, review article, modeling player and team performance in basketball.
- Zachary Terner 1 , and Alexander Franks 1
- View Affiliations Hide Affiliations Affiliations: Department of Statistics and Applied Probability, University of California, Santa Barbara, California 93106, USA; email: [email protected] [email protected]
- Vol. 8:1-23 (Volume publication date March 2021) https://doi.org/10.1146/annurev-statistics-040720-015536
- First published as a Review in Advance on October 09, 2020
- Copyright © 2021 by Annual Reviews. All rights reserved
In recent years, analytics has started to revolutionize the game of basketball: Quantitative analyses of the game inform team strategy; management of player health and fitness; and how teams draft, sign, and trade players. In this review, we focus on methods for quantifying and characterizing basketball gameplay. At the team level, we discuss methods for characterizing team strategy and performance, while at the player level, we take a deep look into a myriad of tools for player evaluation. This includes metrics for overall player value, defensive ability, and shot modeling, and methods for understanding performance over multiple seasons via player production curves. We conclude with a discussion on the future of basketball analytics and, in particular, highlight the need for causal inference in sports.
Article metrics loading...
Full text loading...
Literature Cited
- Alferink LA , Critchfield TS , Hitt JL , Higgins WJ 2009 . Generality of the matching law as a descriptor of shot selection in basketball. J. Appl. Behav. Anal. 42 : 595– 608 [Google Scholar]
- Arel B , Tomas MJ III 2012 . The NBA draft: a put option analogy. J. Sports Econ. 13 : 223– 49 [Google Scholar]
- Bar-Eli M , Avugos S , Raab M 2006 . Twenty years of hot hand research: review and critique. Psychol. Sport Exerc. 7 : 525– 53 [Google Scholar]
- Basketball Reference 2020 . Calculating individual offensive and defensive ratings. Basketball Reference https://www.basketball-reference.com/about/ratings.html [Google Scholar]
- Berri DJ. 1999 . Who is most valuable? Measuring the player's production of wins in the National Basketball Association. Manag. Decis. Econ. 20 : 411– 27 [Google Scholar]
- Berri DJ , Brook SL , Fenn AJ 2011 . From college to the pros: predicting the NBA amateur player draft. J. Product. Anal. 35 : 25– 35 [Google Scholar]
- Berry SM , Reese CS , Larkey PD 1999 . Bridging different eras in sports. J. Am. Stat. Assoc. 94 : 661– 76 [Google Scholar]
- Blei DM , Ng AY , Jordan MI 2003 . Latent Dirichlet allocation. J. Mach. Learn. Res. 3 : 993– 1022 [Google Scholar]
- Bornn L , Cervone D , Franks A , Miller A 2017 . Studying basketball through the lens of player tracking data. Handbook of Statistical Methods and Analyses in Sports J Albert, M Glickman, TB Swartz, RH Koning 261– 86 Boca Raton, FL: Chapman and Hall/CRC [Google Scholar]
- Bornn L , Daly-Grafstein D. 2019 . Using in-game shot trajectories to better understand defensive impact in the NBA. arXiv:1905.00822 [stat.AP]
- Brown M , Kvam P , Nemhauser G , Sokol J 2012 . Insights from the LRMC method for NCAA tournament predictions Paper presented at MIT Sloan Sports Analytics Conference, March 2–3 Boston, MA: [Google Scholar]
- Brown WO , Sauer RD. 1993 . Fundamentals or noise? Evidence from the professional basketball betting market. J. Finance 48 : 1193– 209 [Google Scholar]
- Cervone D , Bornn L , Goldsberry K 2016a . NBA court realty Paper presented at MIT Sloan Sports Analytics Conference, March 11–12 Boston, MA: [Google Scholar]
- Cervone D , D'Amour A , Bornn L , Goldsberry K 2014 . Pointwise: predicting points and valuing decisions in real time with NBA optical tracking data Paper presented at MIT Sloan Sports Analytics Conference, Feb. 28–March 1 Boston, MA: [Google Scholar]
- Cervone D , D'Amour A , Bornn L , Goldsberry K 2016b . A multiresolution stochastic process model for predicting basketball possession outcomes. J. Am. Stat. Assoc. 111 : 585– 99 [Google Scholar]
- Chang YH , Maheswaran R , Su J , Kwok S , Levy T et al. 2014 . Quantifying shot quality in the NBA Paper presented at MIT Sloan Sports Analytics Conference, Feb. 28–March 1 Boston, MA: [Google Scholar]
- D'Amour A , Cervone D , Bornn L , Goldsberry K 2015 . Move or die: how ball movement creates open shots in the NBA Paper presented at MIT Sloan Sports Analytics Conference, Feb. 27–28 Boston, MA: [Google Scholar]
- Daly-Grafstein D , Bornn L. 2019 . Rao-Blackwellizing field goal percentage. J. Quant. Anal. Sports 15 : 85– 95 [Google Scholar]
- Deshpande SK , Jensen ST. 2016 . Estimating an NBA players impact on his team's chances of winning. J. Quant. Anal. Sports 12 : 51– 72 [Google Scholar]
- DiFiori JP , Güllich A , Brenner JS , Côté J , Hainline B et al. 2018 . The NBA and youth basketball: recommendations for promoting a healthy and positive experience. Sports Med 48 : 2053– 65 [Google Scholar]
- Drakos MC , Domb B , Starkey C , Callahan L , Allen AA 2010 . Injury in the National Basketball Association: a 17-year overview. Sports Health 2 : 284– 90 [Google Scholar]
- Drazan JF , Loya AK , Horne BD , Eglash R 2017 . From sports to science: using basketball analytics to broaden the appeal of math and science among youth Paper presented at MIT Sloan Sports Analytics Conference, March 3–4 Boston, MA: [Google Scholar]
- Dutta S , Jacobson SH , Sauppe JJ 2017 . Identifying NCAA tournament upsets using balance optimization subset selection. J. Quant. Anal. Sports 13 : 79– 93 [Google Scholar]
- Efron B , Morris C. 1975 . Data analysis using Stein's estimator and its generalizations. J. Am. Stat. Assoc. 70 : 311– 19 [Google Scholar]
- Engelmann J. 2017 . Possession-based player performance analysis in basketball (adjusted +/– and related concepts). Handbook of Statistical Methods and Analyses in Sports J Albert, M Glickman, TB Swartz, RH Koning 231– 44 Boca Raton, FL: Chapman and Hall/CRC [Google Scholar]
- ESPN 2020 . 5-on-5: Can LeBron catch Giannis for MVP. ? ESPN Feb. 27. https://www.espn.com/nba/story/_/id/28787687/5-5-lebron-catch-giannis-mvp [Google Scholar]
- Fearnhead P , Taylor BM. 2011 . On estimating the ability of NBA players. J. Quant. Anal. Sports 7(3) : 11 [Google Scholar]
- Fewell JH , Armbruster D , Ingraham J , Petersen A , Waters JS 2012 . Basketball teams as strategic networks. PLOS ONE 7 : e47445 [Google Scholar]
- Franks A , Miller A , Bornn L , Goldsberry K 2015a . Characterizing the spatial structure of defensive skill in professional basketball. Ann. Appl. Stat. 9 : 94– 121 [Google Scholar]
- Franks A , Miller A , Bornn L , Goldsberry K 2015b . Counterpoints: advanced defensive metrics for NBA basketball Paper presented at MIT Sloan Sports Analytics Conference, Feb. 27–28 Boston, MA: [Google Scholar]
- Franks AM , D'Amour A , Cervone D , Bornn L 2016 . Meta-analytics: tools for understanding the statistical properties of sports metrics. J. Quant. Anal. Sports 12 : 151– 65 [Google Scholar]
- Gandar JM , Dare WH , Brown CR , Zuber RA 1998 . Informed traders and price variations in the betting market for professional basketball games. J. Finance 53 : 385– 401 [Google Scholar]
- Gauriot R , Page L. 2019 . Fooled by performance randomness: overrewarding luck. Rev. Econ. Stat. 101 : 658– 66 [Google Scholar]
- Gilovich T , Vallone R , Tversky A 1985 . The hot hand in basketball: on the misperception of random sequences. Cogn. Psychol. 17 : 295– 314 [Google Scholar]
- Goldman M , Rao JM. 2011 . Allocative and dynamic efficiency in NBA decision making Paper presented at MIT Sloan Sports Analytics Conference, March 4–5 Boston, MA: [Google Scholar]
- Goldman M , Rao JM. 2013 . Live by the three, die by the three? The price of risk in the NBA Paper presented at MIT Sloan Sports Analytics Conference, March 1–2 Boston, MA: [Google Scholar]
- Goldsberry K. 2012 . Courtvision: New visual and spatial analytics for the NBA Paper presented at MIT Sloan Sports Analytics Conference, March 2–3 Boston, MA: [Google Scholar]
- Goldsberry K. 2019 . Sprawlball: A Visual Tour of the New Era of the NBA Boston: Houghton Mifflin Harcourt [Google Scholar]
- Goldsberry K , Weiss E. 2013 . The Dwight effect: a new ensemble of interior defense analytics for the NBA Paper presented at MIT Sloan Sports Analytics Conference, March 1–2 Boston, MA: [Google Scholar]
- Gray KL , Schwertman NC. 2012 . Comparing team selection and seeding for the 2011 NCAA men's basketball tournament. J. Quant. Anal. Sports 8 : 1 https://doi.org/10.1515/1559-0410.1369 [Crossref] [Google Scholar]
- Groothuis PA , Hill JR , Perri TJ 2007 . Early entry in the NBA draft: the influence of unraveling, human capital, and option value. J. Sports Econ. 8 : 223– 43 [Google Scholar]
- Harmon M , Lucey P , Klabjan D 2017 . Predicting shot making in basketball using convolutional neural networks learnt from adversarial multiagent trajectories. arXiv:1609.04849 [stat.ML]
- Hofler RA , Payne JE. 2006 . Efficiency in the national basketball association: a stochastic frontier approach with panel data. Manag. Decis. Econ. 27 : 279– 85 [Google Scholar]
- Hollinger J. 2005a . PER: player efficiency rating explained. ESPN Aug. 17. https://www.espn.com/NBA/insider/columns/story?columnist=hollinger_john&id=2136379 [Google Scholar]
- Hollinger J. 2005b . Pro Basketball Forecast, 2005–06 Washington, DC: Potomac [Google Scholar]
- Hughes G. 2017 . How Europe changed the NBA game forever. Bleacher Report Sept. 6. https://bleacherreport.com/articles/1764154-how-europe-changed-the-nba-game-forever [Google Scholar]
- Jacobs J. 2017 . Deep dive on regularized adjusted plus-minus I: introductory example. Squared Statistics: Understanding Basketball Analytics Blog Sept. 18. https://squared2020.com/2017/09/18/deep-dive-on-regularized-adjusted-plus-minus-i-introductory-example/ [Google Scholar]
- James B. 1984 . The Bill James Baseball Abstract, 1984 New York: Ballantine [Google Scholar]
- James B. 1987 . The Bill James Baseball Abstract 1987 New York: Ballantine [Google Scholar]
- James B. 2010 . The New Bill James Historical Baseball Abstract New York: Simon and Schuster [Google Scholar]
- Keshri S , Oh M-H , Zhang S , Iyengar G 2019 . Automatic event detection in basketball using HMM with energy based defensive assignment. J. Quant. Anal. Sports 15 : 141– 53 [Google Scholar]
- Kubatko J , Oliver D , Pelton K , Rosenbaum DT 2007 . A starting point for analyzing basketball statistics. J. Quant. Anal. Sports 3 : 3 1 [Google Scholar]
- Lackritz J. 2017 . Probability models for streak shooting. Handbook of Statistical Methods and Analyses in Sports J Albert, M Glickman, TB Swartz, RH Koning 215– 30 Boca Raton, FL: Chapman and Hall/CRC [Google Scholar]
- Lamas L , Santana F , Heiner M , Ugrinowitsch C , Fellingham G 2015 . Modeling the offensive-defensive interaction and resulting outcomes in basketball. PLOS ONE 10 : e0144435 [Google Scholar]
- Le HM , Carr P , Yue Y , Lucey P 2017 . Data-driven ghosting using deep imitation learning Paper presented at MIT Sloan Sports Analytics Conference, March 3–4 Boston, MA: [Google Scholar]
- Lewis M. 2004 . Moneyball: The Art of Winning an Unfair Game New York: WW Norton [Google Scholar]
- Linou K. 2016 . NBA player movements. Software for Visualization of NBA Games https://github.com/linouk23/NBA-Player-Movements [Google Scholar]
- Lopez MJ. 2016 . Persuaded under pressure: evidence from the national football league. Econ. Inq. 54 : 1763– 73 [Google Scholar]
- Lopez MJ , Matthews GJ. 2015 . Building an NCAA men's basketball predictive model and quantifying its success. J. Quant. Anal. Sports 11 : 5– 12 [Google Scholar]
- Lucey P , Bialkowski A , Carr P , Yue Y , Matthews I 2014 . How to get an open shot: analyzing team movement in basketball using tracking data Paper presented at MIT Sloan Sports Analytics Conference, Feb. 28–March 1 Boston, MA: [Google Scholar]
- Malarranha J , Figueira B , Leite N , Sampaio J 2013 . Dynamic modeling of performance in basketball. Int. J. Perform. Anal. Sport 13 : 377– 87 [Google Scholar]
- Marty R. 2018 . High-resolution shot capture reveals systematic biases and an improved method for shooter evaluation Paper presented at MIT Sloan Sports Analytics Conference, Feb. 23–24 Boston, MA: [Google Scholar]
- Marty R , Lucey S. 2017 . A data-driven method for understanding and increasing 3-point shooting percentage Paper presented at MIT Sloan Sports Analytics Conference, March 3–4 Boston, MA: [Google Scholar]
- Masheswaran R , Chang Y , Su J , Kwok S , Levy T et al. 2014 . The three dimensions of rebounding Paper presented at MIT Sloan Sports Analytics Conference, Feb. 28–March 1 Boston, MA: [Google Scholar]
- McCann MA. 2004 . Illegal defense: the irrational economics of banning high school players from the NBA draft. SSRN https://papers.ssrn.com/sol3/papers.cfm?abstract_id=567745 [Google Scholar]
- McCarthy MM , Voos JE , Nguyen JT , Callahan L , Hannafin JA 2013 . Injury profile in elite female basketball athletes at the Women's National Basketball Association combine. Am. J. Sports Med. 41 : 645– 51 [Google Scholar]
- Melnick MJ. 2001 . Relationship between team assists and win-loss record in the National Basketball Association. Percept. Motor Skills 92 : 595– 602 [Google Scholar]
- Metulini R , Manisera M , Zuccolotto P 2018 . Modelling the dynamic pattern of surface area in basketball and its effects on team performance. J. Quant. Anal. Sports 14 : 117– 30 [Google Scholar]
- Miller A , Bornn L , Adams R , Goldsberry K 2013 . Factorized point process intensities: A spatial analysis of professional basketball. Proceedings of the 31st International Conference on Machine Learning EP Xing, T Jebara 235– 43 New York: ACM [Google Scholar]
- Miller AC , Bornn L. 2017 . Possession sketches: mapping NBA strategies. Paper presented at MIT Sloan Sports Analytics Conference, March 3–4, Boston, MA
- Miller J , Sanjurjo A. 2018a . Momentum isn't magic—vindicating the hot hand with the mathematics of streaks. Scientific American March 28. https://www.scientificamerican.com/article/momentum-isnt-magic-vindicating-the-hot-hand-with-the-mathematics-of-streaks/ [Google Scholar]
- Miller JB , Sanjurjo A. 2018b . A bridge from Monty Hall to the hot hand: restricted choice, selection bias, and empirical practice. OSF Preprints https://dx.doi.org/10.31219/osf.io/dmgtp [Crossref] [Google Scholar]
- Miller JB , Sanjurjo A. 2018c . Surprised by the hot hand fallacy? A truth in the law of small numbers. Econometrica 86 : 2019– 47 [Google Scholar]
- Myers D. 2020 . About box plus/minus (BPM). Basketball Reference https://www.basketball-reference.com/about/bpm2.html [Google Scholar]
- NBA (Natl. Basketb. Assoc.). 2020 . NBA Hackathon. NBA Media Ventures https://hackathon.nba.com/ [Google Scholar]
- Neiman T , Loewenstein Y. 2011 . Reinforcement learning in professional basketball players. Nat. Commun. 2 : 1– 8 [Google Scholar]
- Neudorfer A , Rosset S. 2018 . Predicting the NCAA basketball tournament using isotonic least squares pairwise comparison model. J. Quant. Anal. Sports 14 : 173– 83 [Google Scholar]
- NFL (Natl. Footb. Leag.) 2020 . Big Data Bowl. National Football League Operations https://operations.nfl.com/the-game/big-data-bowl/ [Google Scholar]
- Oliver D. 2004 . Basketball on Paper: Rules and Tools for Performance Analysis Washington, DC: Potomac [Google Scholar]
- Omidiran D. 2011 . A new look at adjusted plus/minus for basketball analysis Paper presented at MIT Sloan Sports Analytics Conference, March 4–5 Boston, MA: [Google Scholar]
- Page GL , Barney BJ , McGuire AT 2013 . Effect of position, usage rate, and per game minutes played on NBA player production curves. J. Quant. Anal. Sports 9 : 337– 45 [Google Scholar]
- Page GL , Fellingham GW , Reese CS 2007 . Using box-scores to determine a position's contribution to winning basketball games. J. Quant. Anal. Sports 3 : 4 1 [Google Scholar]
- Patton A. 2019 . How can we visualize a player's shooting gravity. ? Fansided/Nylon Calculus Blog July 22. https://fansided.com/2019/07/22/nylon-calculus-visualizing-NBA-shooting-gravity/ [Google Scholar]
- Pelton K. 2019 . The WARP rating system explained. SBNation http://sonicscentral.com/warp.html [Google Scholar]
- Pesca M. 2009 . The man who made baseball's box score a hit. NPR July 30. https://www.npr.org/templates/story/story.php?storyId=106891539 [Google Scholar]
- Piette J , Pham L , Anand S 2011 . Evaluating basketball player performance via statistical network modeling. Paper presented at MIT Sloan Sports Analytics Conference, March 4–5 Boston, MA:
- Poling A , Edwards TL , Weeden M , Foster TM 2011 . The matching law. Psychol. Record 61 : 313– 22 [Google Scholar]
- Prentice RL , Kalbfleisch JD. 1979 . Hazard rate models with covariates. Biometrics 35 : 25– 39 [Google Scholar]
- Price J , Wolfers J. 2010 . Racial discrimination among NBA referees. Q. J. Econ. 125 : 1859– 87 [Google Scholar]
- Reich BJ , Hodges JS , Carlin BP , Reich AM 2006 . A spatial analysis of basketball shot chart data. Am. Stat. 60 : 3– 12 [Google Scholar]
- Rosenbaum DT. 2004 . Measuring how NBA players help their teams win. 82games Apr. 30. http://www.82games.com/comm30.htm [Google Scholar]
- Ruiz FJ , Perez-Cruz F. 2015 . A generative model for predicting outcomes in college basketball. J. Quant. Anal. Sports 11 : 39– 52 [Google Scholar]
- Sampaio J , Drinkwater EJ , Leite NM 2010 . Effects of season period, team quality, and playing time on basketball players' game-related statistics. Eur. J. Sport Sci. 10 : 141– 49 [Google Scholar]
- Sandholtz N , Bornn L. 2020 . Markov decision processes with dynamic transition probabilities: an analysis of shooting strategies in basketball. Ann. Appl. Stat. 14(3) : 1122– 45 [Google Scholar]
- Sandholtz N , Mortensen J , Bornn L 2020 . Measuring spatial allocative efficiency in basketball. J. Quant. Anal. Sports 16(4) : 271– 89 [Google Scholar]
- Schwartz J. 2013 . The NBA loves stats. That's a problem. Slate Feb. 28. https://slate.com/culture/2013/02/nba-stats-gurus-cant-work-together-anymore-thats-a-problem.html [Google Scholar]
- Shah R , Romijnders R. 2016 . Applying deep learning to basketball trajectories. arXiv:1608.03793 [cs.NE]
- Shortridge A , Goldsberry K , Adams M 2014 . Creating space to shoot: quantifying spatial relative field goal efficiency in basketball. J. Quant. Anal. Sports 10 : 303– 13 [Google Scholar]
- Sicilia A , Pelechrinis K , Goldsberry K 2019 . Deephoops: evaluating micro-actions in basketball using deep feature representations of spatio-temporal data. arXiv:1902.08081 [stat.AP]
- Sill J. 2010 . Improved NBA adjusted plus-minus using regularization and out-of-sample testing. Paper presented at MIT Sloan Sports Analytics Conference, March 9, Boston, MA
- Silver N. 2012 . The Signal and the Noise: Why Most Predictions Fail—But Some Don't London: Penguin [Google Scholar]
- Silver N. 2015 . We're predicting the career of every NBA player. Here's how. FiveThirtyEight Blog Oct. 9. https://fivethirtyeight.com/features/how-were-predicting-NBA-player-career/ [Google Scholar]
- Silver N. 2019 . How our RAPTOR metric works. FiveThirtyEight Blog Oct. 10. https://fivethirtyeight.com/features/how-our-raptor-metric-works/ [Google Scholar]
- Simmons B. 2001 . Ewing theory 101. ESPN May 9. https://www.espn.com/espn/page2/story?page=simmons/010509a [Google Scholar]
- Skinner B. 2010 . The price of anarchy in basketball. J. Quant. Anal. Sports 6 : 1 1– 18 [Google Scholar]
- Skinner B , Goldman M. 2015 . Optimal strategy in basketball. arXiv:1512.05652 [physics.soc-ph]
- Skinner B , Guy SJ. 2015 . A method for using player tracking data in basketball to learn player skills and predict team performance. PLOS ONE 10 : e0136393 [Google Scholar]
- Smith C. 2018 . Kinexon's wearable sensor is changing the way NBA teams prep for success. Wareable Nov. 2. https://www.wareable.com/sport/kinexon-wearable-sensor-NBA-basketball-6679 [Google Scholar]
- Stone DF. 2012 . Measurement error and the hot hand. Am. Stat. 66 : 61– 66 [Google Scholar]
- Tu YK , Gunnell D , Gilthorpe MS 2008 . Simpson's paradox, Lord's paradox, and suppression effects are the same phenomenon—the reversal paradox. Emerg. Themes Epidemiol. 5 : 2 [Google Scholar]
- Vaci N , Cocić D , Gula B , Bilalić M 2019 . Large data and Bayesian modeling—aging curves of NBA players. Behav. Res. Methods 51 : 1544– 64 [Google Scholar]
- van Bommel M , Bornn L 2017 . Adjusting for scorekeeper bias in NBA box scores. Data Mining Knowl. Discov. 31 : 1622– 42 [Google Scholar]
- Vinué G , Epifanio I. 2019 . Forecasting basketball players' performance using sparse functional data. Stat. Anal. Data Mining 12 : 534– 47 [Google Scholar]
- Vinué G , Epifanio I , Alemany S 2015 . Archetypoids: a new approach to define representative archetypal data. Comput. Stat. Data Anal. 87 : 102– 15 [Google Scholar]
- Wakim A , Jin J. 2014 . Functional data analysis of aging curves in sports. arXiv:1403.7548 [stat.AP]
- Xin L , Zhu M , Chipman H 2017 . A continuous-time stochastic block model for basketball networks. Ann. Appl. Stat. 11 : 553– 97 [Google Scholar]
- Yaari G , Eisenmann S. 2011 . The hot (invisible?) hand: Can time sequence patterns of success/failure in sports be modeled as repeated random independent trials. ? PLOS ONE 6 : e24532 [Google Scholar]
- Yam DR , Lopez MJ. 2019 . What was lost? A causal estimate of fourth down behavior in the National Football League. J. Sports Anal. 5 : 153– 67 [Google Scholar]
- Yu SZ. 2010 . Hidden semi-Markov models. Artif. Intel. 174 : 215– 43 [Google Scholar]
- Yuan LH , Liu A , Yeh A , Kaufman A , Reece A et al. 2015 . A mixture-of-modelers approach to forecasting NCAA tournament outcomes. J. Quant. Anal. Sports 11 : 13– 27 [Google Scholar]
- Yue Y , Lucey P , Carr P , Bialkowski A , Matthews I 2014 . Learning fine-grained spatial models for dynamic sports play prediction. Proceedings of the 2014 IEEE International Conference on Data Mining 670– 79 Washington, DC: IEEE [Google Scholar]
- Ziv G , Lidor R , Arnon M 2010 . Predicting team rankings in basketball: the questionable use of on-court performance statistics. Int. J. Perform. Anal. Sport 10 : 103– 14 [Google Scholar]
Data & Media loading...
Supplementary Data
Download Supplemental Tables 1-2 (PDF).
- Article Type: Review Article
Most Read This Month
Most cited most cited rss feed, functional data analysis, probabilistic forecasting, bayesian computing with inla: a review, functional regression, topological data analysis, algorithmic fairness: choices, assumptions, and definitions, microbiome, metagenomics, and high-dimensional compositional data analysis, learning deep generative models, on p -values and bayes factors, q-learning: theory and applications.
ORIGINAL RESEARCH article
Long-term analysis of elite basketball players’ game-related statistics throughout their careers.
- 1 Sport Science Department, Universidad Politécnica de Madrid, Madrid, Spain
- 2 Institute of Sport Science and Innovations, Lithuanian Sports University, Kaunas, Lithuania
The aim of the present study was to analyze the changes of game-related statistics in expert players across their whole sports careers. From an initial sample including 252 professional basketball players competing in Spanish first division basketball league (ACB) in the 2017–2018 season, 22 met the inclusion criteria. The following game-related statistics were studied: average points, assist, rebounds (all normalized by minute played), 3-point field goals percentage, 2-point field goals percentage, and free throws percentage per season. Each variable was individually investigated with a customized excel spreadsheet assessing individual variations and career trends were calculated. The results showed a positive trend in most of the investigated players in assists (91% of cases) and free throw percentages (73% of cases). Similar percentages of positive and negative trends were observed for all the other game-related statistics (range: 41–59% for negative and positive, respectively). In conclusion, an increase in assist and free throw performance was shown in the investigated players across their playing career. This information is essential for basketball coaches suggesting the use of most experienced players in the final moments of the game.
Introduction
Basketball is a team sport characterized by the execution of series of skills in multiple situations occurring across the game. In particular, game-related statistics are fundamental and their level might depend on the players’ characteristics and training experience. Most of the game related statistics depends on multifactorial variables (i.e., offensive and defensive tactics) determining a complex dynamic system during games, which is difficult to control in its totality. The use of performance analysis in sport with the determination of the most important game related statistics during the game aims to improve the team performance, increasing the knowledge of the performance of each player. Specifically, game-related statistics are key tools for basketball coaches providing reliable information about teams’ performance such as those distinguishing between successful and unsuccessful teams. Previous investigations widely studied the game-related statistics mostly assessing team performance in order to determine the most valuable players and the importance of certain positions such as guards, forward and centers (e.g., Sampaio et al., 2006a ), to evaluate the impact of rule changes (e.g.; Gómez et al., 2006a ; Ibáñez et al., 2018 ), the effect of home advantage (e.g.; Carron et al., 2005 ; Pollard, 2008 ; Watkins, 2013 ), the importance of starters and bench players regarding their contribution to the game (e.g.; Sampaio et al., 2006b ), the scoring strategies differentiating between winning and losing teams in women’s basketball FIBA Eurobasket (e.g.; Conte and Lukonaitiene, 2018 ). It is important to note that in basketball several game related statistics have been used, while only some of them were deemed fundamental. Previous discriminant analyses quantitatively determined the team performance indicators (TPI), identified as a variable able to define the most important aspect of performance ( Hughes and Bartlett, 2002 ) and compare different leagues ( Sampaio and Leite, 2013 ), which most affect the game outcome ( Gomez et al., 2008 ; Ibánez et al., 2008 ). In particular, Yu et al. (2008) , established a list of the most influential TPI’s (Technical Performance Indices) such as points per game (PPG), field goals made (FGM), rebounds, assists, turnovers, blocks, fouls, and steals. Sampaio et al. (2013) included also free throws as an important technical performance indicator. The TPIs with the most impact on the outcome of a season in Spanish first division (ACB) teams were shooting percentage (both 2-point and 3-point percentage), assists and rebounds ( García et al., 2013 ; Gómez et al., 2008 ). However, to the best of our knowledge, no previous investigations assessed players’ individual game related statistics across a long period of time. Indeed, players’ experience might play a fundamental role in improving players’ game related statistics effectiveness. Therefore, studies addressing this topic are warranted.
The performance of a player across his career might play a fundamental role in distinguishing between elite and non-elite players. Indeed, acquiring playing experience, players could have a better performance due to the demand of basketball game to perform complex actions that require high anticipatory skills in difficult situations. Indeed, these high anticipatory skills can be translated into scoring and passing related variables concerning about game-related statistics ( Sampaio et al., 2015 ), and therefore they become an important variable deeming further analysis in basketball. In fact, elite players perceive better their environmental information and are capable of adapting their behavior accordingly and consequently perform better compared to other non-elite players ( Aglioti et al., 2008 ). Therefore, playing experience might be essential in increasing players’ anticipatory skills and consequently their game performance.
It has been previously showed that performance slowly decrease after reaching the peak period of the player career ( Baker et al., 2013 ). In basketball, Baker et al. (2013) , found that the typical basketball career lasts about 11 years, with the longest career studied being 23 years of playing at an elite level. However, it is not clear the performance changes across players career, and their trend (i.e., positive or negative) calling for further studies in this area. Therefore, the aim of this study was to descriptively analyze TPI changes throughout the career of expert basketball players, assessing the possible performance trend.
Materials and Methods
Participants.
From an initial sample of 252 professional basketball players competing in ACB, 22 players (9 backcourt and 13 frontcourt) were selected for this study based on the following inclusion criteria determined by a group of experts, who were identified according to Swann et al. (2015) guidelines: (a) male players, currently playing in the ACB league in the season 2017–2018; (b) to have a minimum playing experience of 10 years (including only season in which they effectively played) in the first division of any country with at least an average of 25% of number of games and minutes played per season; (c) to possess a minimum of 5 years playing experience in first division of any league amongst the top 30 countries in the FIBA Ranking (at February 28, 2018); (d) to have played at least 75% of their professional careers in any country’s first division league, consequently no years played in lower division leagues were analyzed. The aim of these criteria was to ensure the highest quality of the sample for expert players with a solid number of games and minutes played each season ( Swann et al., 2015 ).
The databases used to obtain the game related statistics of each season for the studied players were the ACB official web page 1 for any season played in the ACB league, and the RealGM website 2 , or the official ACB guide released by the Spanish Basketball Association for any season played outside Spain. These databases are normally used in studies related with basketball, and basketball statistics and are considered valid and reliable ( Gómez et al., 2018 ).
The following game-related statistics for each season were recorded and analyzed: average points, assist, rebounds, 3-point field goals percentage, 2-point field goals percentage and free throws percentage per season. The variable point, assists and rebounds were normalized by minute played with the following formula (example for points scored: mean seasonal points scored/mean seasonal minute played ∗ 40 min). All the data for these game-related statistics, for every season and every player included in this study were storage in a database and once they were used for the statistical analysis.
Statistical Analysis
All statistical analyses were performed with a customized excel spreadsheet specifically developed to monitor individual changes and trends in a rigorous quantitative way ( Hopkins, 2017 ). Recently, this excel spreadsheet has been adopted to assess individual changes in team sports ( Siahkouhian and Khodadadi, 2013 ; Loturco et al., 2017 ; Colyer et al., 2018 ; Hurst et al., 2018 ) and specifically in basketball ( Pliauga et al., 2018 ). This statistics approach could be used as a possible alternative to previously used methodologies such as the ANOVA factor ( Yu et al., 2008 ) or the Jonckheere–Terpstra test ( Leite and Sampaio, 2012 ). The individual trends across playing career for each investigated player were then quantified and the percentage of players documenting a positive, negative or steady (when the result is zero) slope were calculated using the following formula y = m⋅x+n. Figures 1 – 6 are an example of the individual points and trendlines obtained via the Hopkins spreadsheet and that were later analyzed.
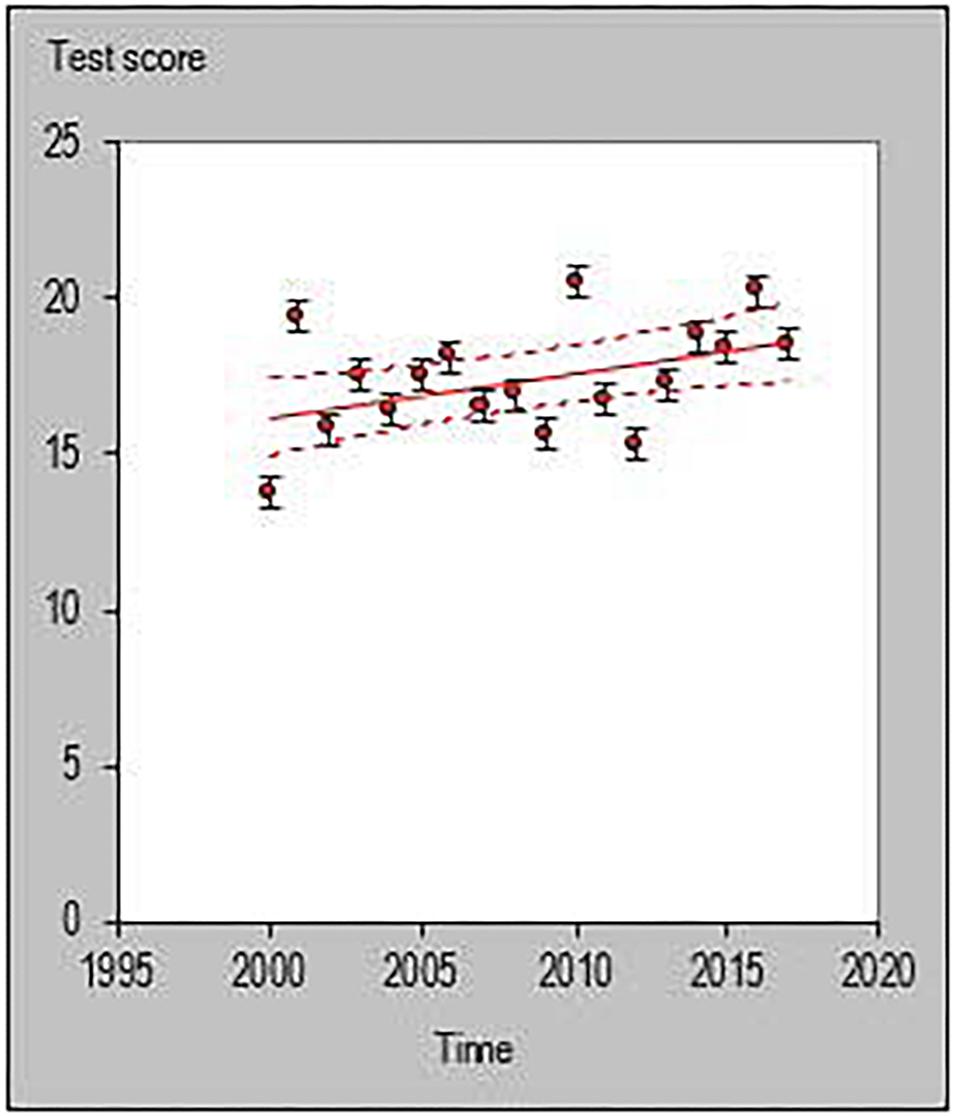
Figure 1. Individual trend of one participant for average points per season normalized by minute.
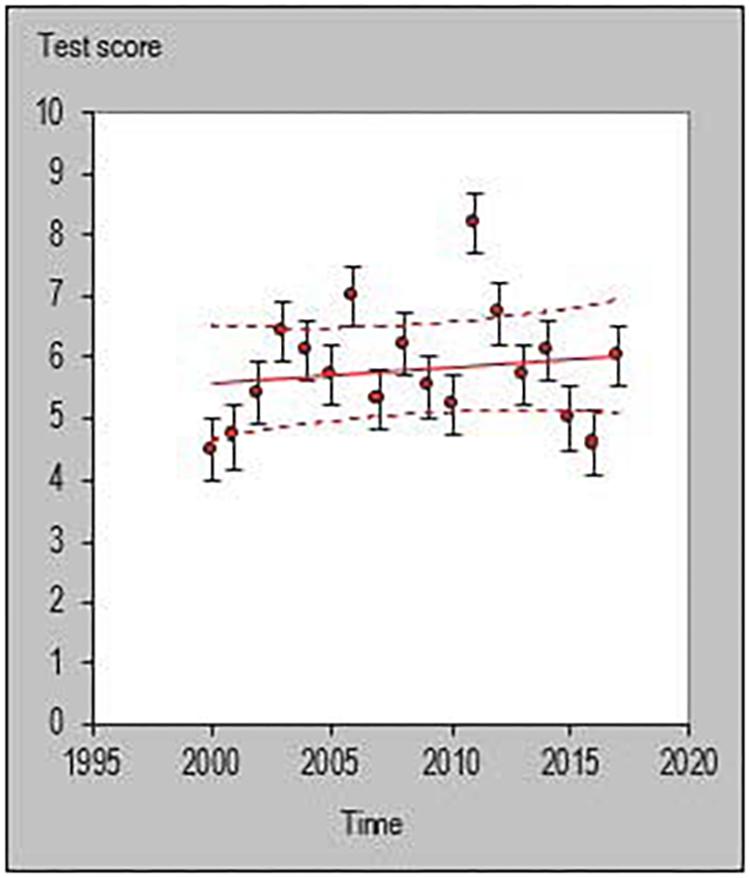
Figure 2. Individual trend of one participant for average rebounds per season normalized by minute.
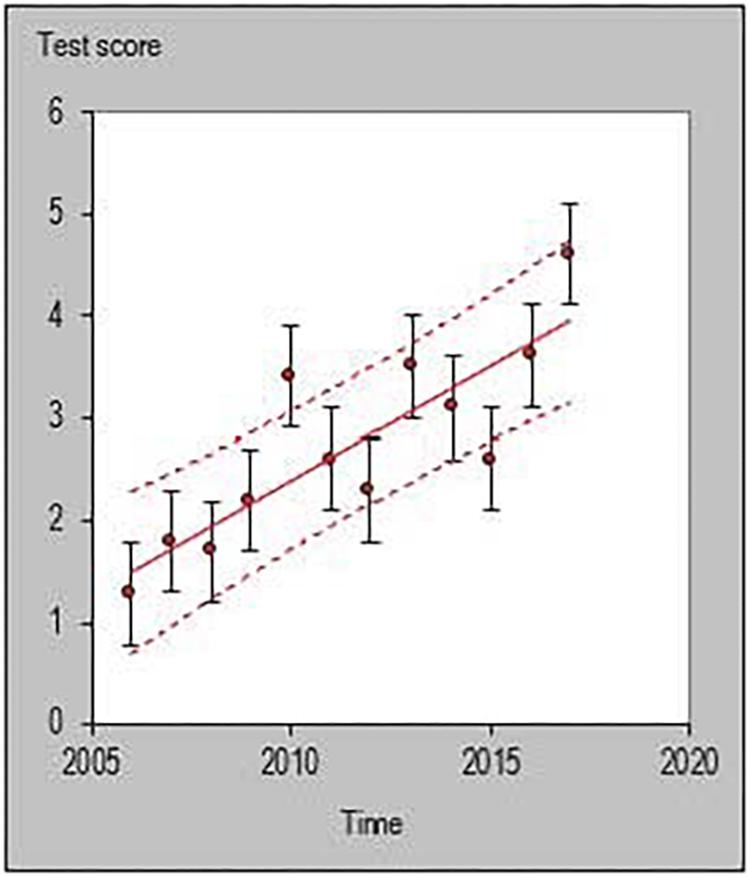
Figure 3. Individual trend of one participant for average assists per season normalized by minute.
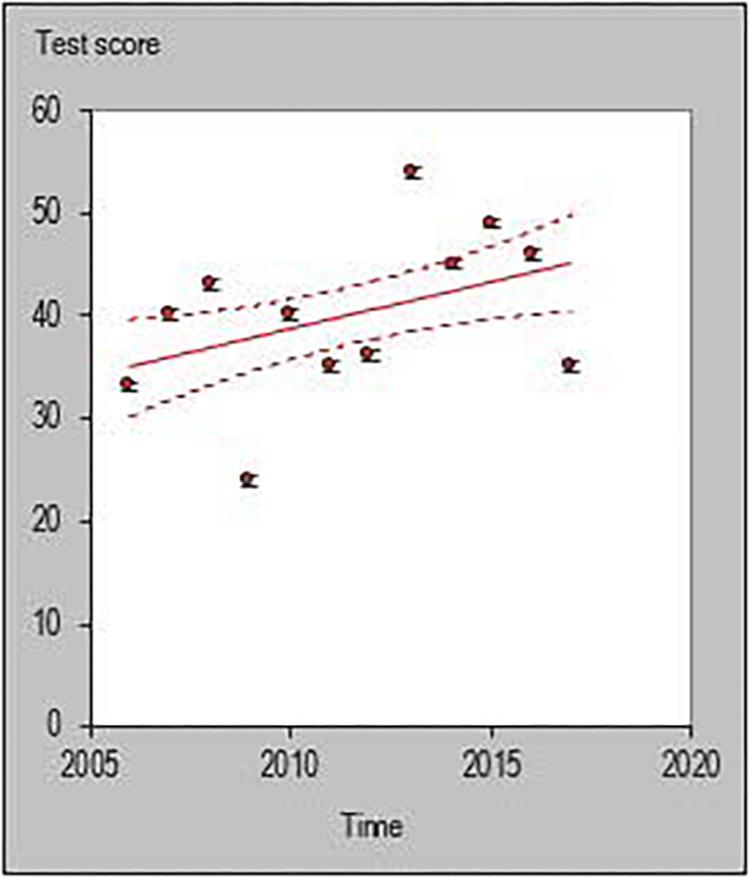
Figure 4. Individual trend of one participant for 3-point percentage per season.
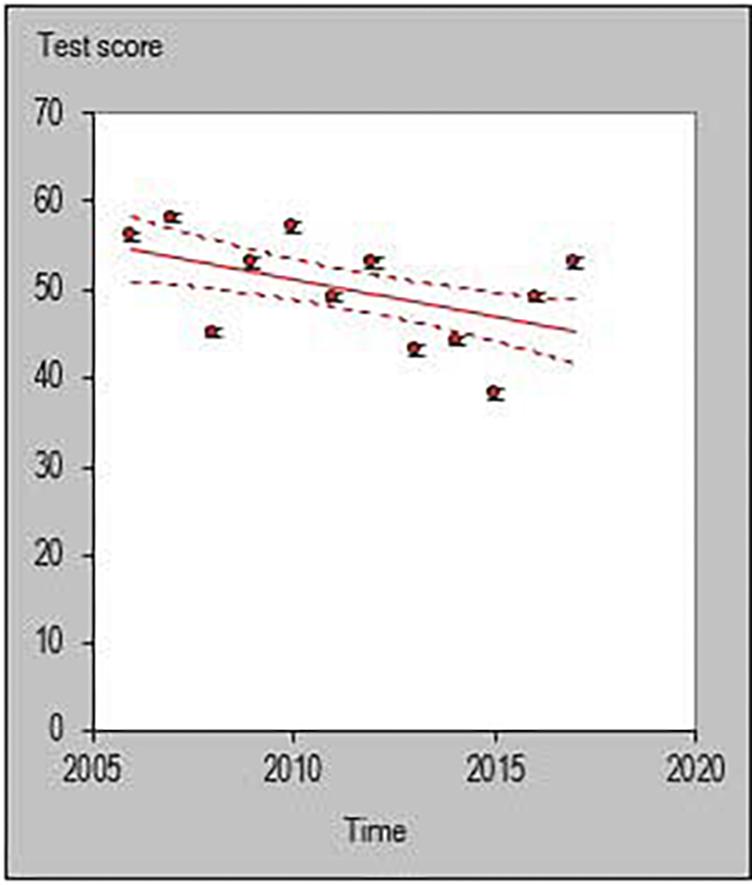
Figure 5. Individual trend of one participant for 2-point percentage per season.
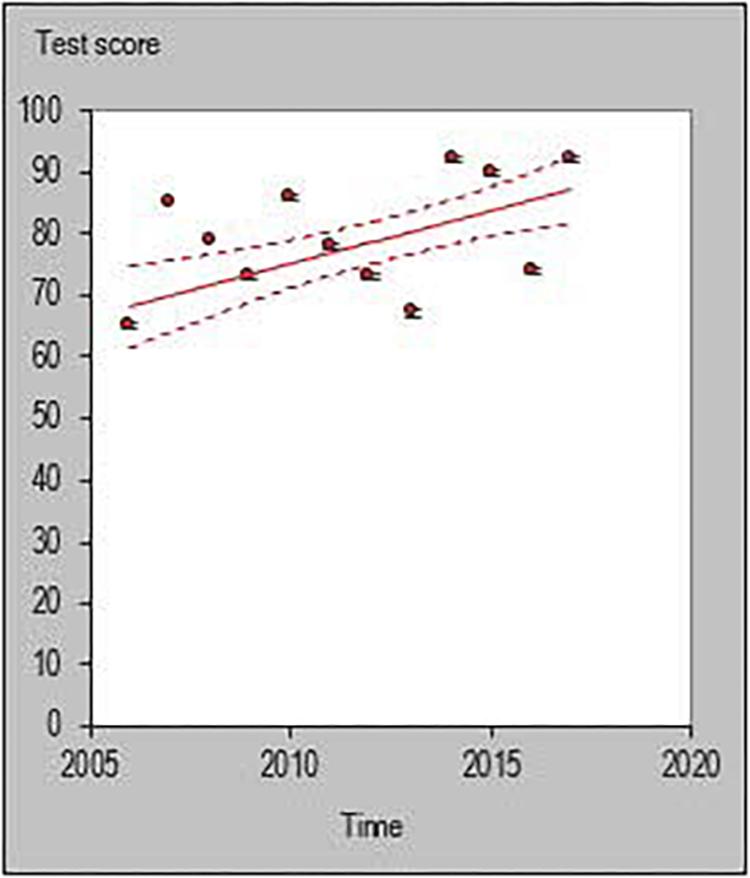
Figure 6. Individual trend of one participant for free throw percentage per season.
The mean slope for each performance indicator and the number of cases in which the slope was positive, steady, or negative are shown in Table 1 . Results revealed that most of the players have a positive trend in assists (91% of the cases) free throws (73% of the cases), and 3-point percentage although with a lower value (59%). Conversely, there were no differences of positive and negative trends reported for the other investigated parameters ( Table 1 ).
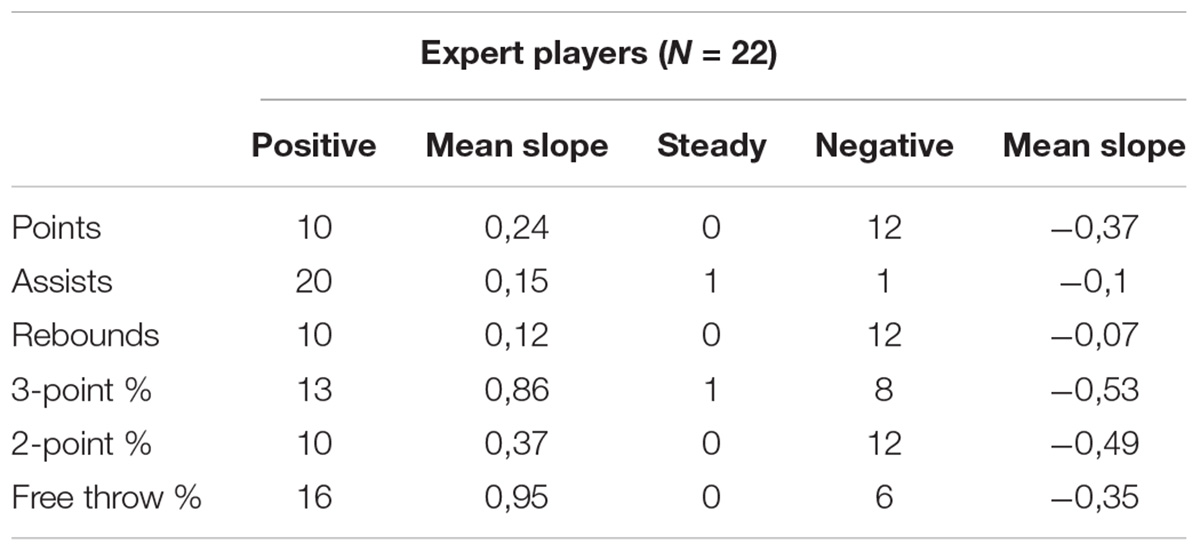
Table 1. Mean slope and number of cases of each variable.
The aim of this study was to analyze the trends TPIs throughout the career of expert basketball players. The results revealed that assists and free throws were the two TPIs mostly showing a positive trend during players’ careers. Specifically, the 91% of the studied players have a positive tendency in assists, with a mean slope of 0.15, and 73% of them have a positive tendency in free throws, with a mean slope of 0.95. Also, 59% of the players increase their 3-point percentage, but this result might have been influenced by the fact that more frontcourt than backcourt players met our inclusion criteria.
Basketball is a sport where situations change quickly and continuously as a result of the combination of factors such as the position of opponents in the field and their tactical behavior, the position of the ball and the timing of the offensive movements ( Altavilla and Raiola, 2014 ). Therefore, players are required to decide an appropriate response with a proper timing and executing it in a correct spacing. Often, players are subject to defensive pressure and the more skilled and experienced players might be able to anticipate events and perform unhurried actions as a result of their improved ability to “read the game” ( Sampaio et al., 2004 ). In this context, executing a successful pass (i.e., assist) assume a fundamental importance in basketball. Indeed, when analyzing the mechanism of this technical action, the assist requires a combination of good decision making in court, coordination, anticipation, timing, and a good execution ( Melnick, 2001 ; Gómez et al., 2006b ). Previous research demonstrated that assists and free throw percentage are two of the most factors to win a game ( Dias, 2007 ; Gómez and Lorenzo, 2007 ; Sampaio et al., 2015 ). Moreover, Sampaio et al. (2004) , suggested that assists are indicators of players’ maturity and experience, increasing in number as the player gets a better ability to read the game due to the years of playing experience. The results of our investigation highlighted supporting results, since most of the investigated players increased their assist performance across their playing career. This information seems essential for basketball coaches, who can rely on the performance of more experienced basketball players characterized by a better tactical awareness in order to execute successful passes and increase the scoring possibilities during the game. Indeed, Melnick (2001) showed a positive correlation between number of assists of a team and a better win-loss record through a season.
Free throws have also been demonstrated to be performance indicators differentiating between winning and losing teams in particular in close games ( Ibánez et al., 2008 ; Conte et al., 2018 ; Gómez et al., 2018 ). Therefore, it was expected that players increasing their experience and possibly assuming a leadership and fundamental role in their team would increase their free throw performance during their career. Accordingly, our results demonstrated an increased trend across players’ career for free throws and therefore possibly increasing their teams’ possibility to be successful. In this sense, experience accumulated in games and practices is the most crucial factor for developing expertise in one aspect ( Gómez et al., 2018 ). An increase in the percentages of free throws can be associated with the fact that players have already mastered the shooting during their years of training. Interestingly, a previous investigation showed that free throws shooting trajectories are more efficient and possess a lower variability in more experienced players compared to less experienced players ( Button et al., 2003 ). The practical application of our result is that coaches should favor the participation of most experienced players in last minutes of close games, when usually there are higher number of fouls generating free throws opportunities.
Other variables such as points, rebounds, and 2-point percentage did not show any trend increase across the players’ career. A possible reason for this finding is that these variables might be more influenced by physical factors (i.e., strength, power, and fitness), which showed a decrease during the lifespan ( Horton et al., 2008 ). Even though experienced players compensate this decrease in their physical abilities with a better understanding of the games’ tactical aspects, better timing and spacing and better decision-making abilities, it seems not enough to show a positive trend according to the results of our investigation.
Although this investigation provides basketball coaches with useful information, some limitations should be mentioned. Firstly, the results might have been influenced by some confounding factors such as injuries across the season, the playing status (i.e., starting vs. bench players), economical aspects such as players’ contracts and players and/or coaching staffs changing teams during investigated period. Therefore, future studies are warranted in order to overcome these limitations possibly controlling these factors. However, to the best of our knowledge, this investigation provides the first evidence about the individual trend in players’ performance across their playing career and notably increase the knowledge in this field. Moreover, further studies should be designed in order to assess players’ individual season-by-season changes across their playing career.
The results of this investigation suggest that as the players acquire years of experience in first division elite teams, their assists per game and free throw percentage increase. Conversely, other game-related statistics such as points, rebounds, 3-point percentage, and 2-point percentage showed both positive and negative trends in the investigated players resulting in a high between players variability. Finally, further research is required in this field using an individualized approach to increase the knowledge about players’ performance across their playing career.
Author Contributions
All authors listed have made a substantial, direct and intellectual contribution to the work, and approved it for publication.
Conflict of Interest Statement
The authors declare that the research was conducted in the absence of any commercial or financial relationships that could be construed as a potential conflict of interest.
- ^ http://www.acb.com
- ^ https://basketball.realgm.com
Aglioti, S., Cesari, P., Romani, M., and Urgesi, C. (2008). Action anticipation and motor resonance in elite basketball players. Nat. Neurosci. 11, 1109–1116. doi: 10.1038/nn.2182
PubMed Abstract | CrossRef Full Text | Google Scholar
Altavilla, G., and Raiola, G. (2014). Global vision to understand the game situations in modern basketball. J. Phys. Educ. Sport 14, 493–496.
Google Scholar
Baker, J., Koz, D., Kungl, A. M., Fraser-Thomas, J., and Schorer, J. (2013). Staying at the top: Playing position and performance affect career length in professional sport. High Ability Stud. 24, 63–76. doi: 10.1080/13598139.2012.738325
CrossRef Full Text | Google Scholar
Button, C., Macleod, M., Sanders, R., and Coleman, S. (2003). Examining movement variability in the basketball free-throw action at different skill levels. Res. Q. Exerc. Sport 74, 257–269. doi: 10.1080/02701367.2003.10609090
Carron, A. V., Loughhead, T. M., and Bray, S. R. (2005). The home advantage in sport competitions: Courneya and Carron’s (1992) conceptual framework a decade later. J. Sports Sci. 23, 395–407. doi: 10.1080/02640410400021542
Colyer, S. L., Stokes, K. A., Bilzon, J., Holdcroft, D., and Salo, A. I. T. (2018). The effect of altering loading distance on skeleton start performance: is higher pre-load velocity always beneficial? J. Sports Sci. 36, 1930–1936. doi: 10.1080/02640414.2018.1426352
Conte, D., and Lukonaitiene, I. (2018). Scoring Strategies Differentiating between Winning and Losing Teams during FIBA EuroBasket Women 2017. Sports 6:50. doi: 10.3390/sports6020050
Conte, D., Tessitore, A., Gjullin, A., Mackinnon, D., Lupo, C., and Favero, T. (2018). Investigating the game-related statistics and tactical profile in NCAA division I men’s basketball games. Biol. Sport 35, 137–143. doi: 10.5114/biolsport.2018.71602
Dias, J. M. (2007). The stats value for winning in the world basketball championship for men 2006. Fit. Perform. 6, 57–61. doi: 10.3900/fpj.6.1.57.e
García, J., Ibáñez, S. J., Martínez, R., Leite, N., and Sampaio, J. (2013). Identifying Basketball Performance Indicators in Regular Season and Playoff Games. J. Hum. Kinet. 36, 163–170. doi: 10.2478/hukin-2013-0016
Gómez, M. A., Avugos, S., Oñoro, M. A., Lorenzo, A., and Bar-Eli, M. (2018). Shaq is not alone: free-throws in the final moments of a basketball game. J. Hum. Kinet. 62, 135–144. doi: 10.1515/hukin-2017-0165
Gómez, M. A., and Lorenzo, A. (2007). Análisis discriminante de las estadísticas de juego entre bases, aleros y pívots en baloncesto masculino. Apunts 87, 86–92.
Gómez, M. A., Lorenzo, A., Barakat, R., Ortega, E., and Palao, J. M. (2008). Differencesin game-related statistics of basketball performance between men’s winning and losing teams according to game location. Percept. Mot. Skills 106, 98–107. doi: 10.2466/pms.106.1.43-50
Gómez, M. A., Lorenzo, A., Sampaio, J., and Ibáñez, S. J. (2006a). Differences in game-related statistics between winning and losing teams in women’s basketball. J. Hum. Mov. Stud. 51, 357–369.
Gómez, M. A., Tsamourtzis, E., and Lorenzo, A. (2006b). Defensive systems in basketball ball possessions. Int. J. Perform. Anal. Sport 6, 98–107. doi: 10.1080/02640414.2013.792942
Gomez, M. A., Lorenzo, A., Sampaio, J., Ibanez, S. J., and Ortega, E. (2008). Game related statistics that discriminated winning and losing teams from the Spanish men’s professional basketball teams. Coll. Antropol. 32, 451–456.
Hopkins, W. G. (2017). A Spreadsheet for Monitoring an Individual’s Changes and Trend. Sportscience 21, 5–9.
Horton, S., Baker, J., and Schorer, J. (2008). Expertise and aging: maintaining skills through the lifespan. Euro. Rev. Aging Phys. Act. 5, 89–96. doi: 10.1007/s11556-008-0034-5
Hughes, M. D., and Bartlett, R. M. (2002). The use of performance indicators in performance analysis. J. Sports Sci. 20, 739–754. doi: 10.1080/026404102320675602
Hurst, C., Batterham, A. M., Weston, K. L., and Weston, M. (2018). Short and long-term reliability of leg extensor power measurement in middle-aged and older adults. J. Sports Sci. 36, 970–977. doi: 10.1080/02640414.2017.1346820
Ibáñez, S. J., García-Rubio, J., Gómez, M. A., and González-Espinosa, S. (2018). The Impact of Rule Modifications on Elite Basketball Teams’ Performance. J. Hum. Kinet. 64, 181–193. doi: 10.1515/hukin-2017-0193
Ibánez, S. J., Sampaio, J., Feu, S., Lorenzo, A., Gómez, M. A., and Ortega, E. (2008). Basketball game related statistics that discriminate between team’s season-long success. Euro. J. Sport Sci. 8, 369–372. doi: 10.1080/17461390802261470
Leite, N. M. C., and Sampaio, J. E. (2012). Long-Term Athletic Development across Different Age Groups and Gender from Portuguese Basketball Players. Int. J. Sports Sci. Coach. 7, 285–300. doi: 10.1260/1747-9541.7.2.285
Loturco, I., Pereira, L. A., Kobal, R., Kitamura, K., Cal Abad, C. C., Marques, G., et al. (2017). Validity and Usability of a New System for Measuring and Monitoring Variations in Vertical Jump Performance. J. Strength Cond. Res. 31, 2579–2585. doi: 10.1519/JSC.0000000000002086
Melnick, M. J. (2001). Relationship between team assists and win-loss record in the National Basketball Association. Percept. Mot. Skills 92, 595–602. doi: 10.2466/pms.2001.92.2.595
Pliauga, V., Lukonaitiene, I., Kamandulis, S., Skurvydas, A., Sakalauskas, R., Scanlan, A., et al. (2018). The effect of block and traditional periodization training models on jump and sprint performance in collegiate basketball players. Biol. Sport 35, 373–382. doi: 10.5114/biolsport.2018.78058
Pollard, R. (2008). Home Advantage in football: a current review of an unsolved puzzle. Open Sports Sci. J. 1, 12–14. doi: 10.2174/1875399X00801010012
Sampaio, J., Ibáñez, S., and Lorenzo, A. (2013). “Basketball,” in Routledge Handbook of Sport Performance Analysis , eds T. McGarry, P. O’Donoghue, and J. Sampaio (London: Routledge), 357–366.
Sampaio, J., Ibáñez, S. J., and Feu, S. (2004). Discriminative power of basketball game-related statistics by level of competition and sex. Percept. Mot. Skills 99, 1231–1238. doi: 10.2466/pms.99.3f.1231-1238
Sampaio, J., Ibáñez, S., Lorenzo, A., and Gomez, M. (2006a). Discriminative game related statistics between basketball starters and nonstarters when related to team quality and game outcome. Percept. Mot. Skills 103, 486–494.
PubMed Abstract | Google Scholar
Sampaio, J., Janeira, M., Ibáñez, S., and Lorenzo, A. (2006b). Discriminant analysis of game-related statistics between basketball guards, forwards and centres in three professional leagues. Euro. J. Sport Sci. 6, 173–178. doi: 10.1080/17461390600676200
Sampaio, J., and Leite, N. (2013). “Performance Indicators in Game Sports,” in Routledge Handbook of Sports Performance Analysis (1st ed.) , eds T. McGarry, P. O’Donoghue, and J. Sampaio (New York, NY: Routledge Handbooks).
Sampaio, J., McGarry, T., Calleja-González, J., Jiménez Sáiz, S., Schelling i del Alcázar, X., and Balciunas, M. (2015). Exploring Game Performance in the National Basketball Association Using Player Tracking Data. PLoS One 10:e0132894. doi: 10.1371/journal.pone.0132894
Siahkouhian, M., and Khodadadi, D. (2013). Narita target heart rate equation underestimates the predicted adequate exercise level in sedentary young boys. Asian J. Sports Med. 4, 175–180. doi: 10.5812/asjsm.34255
Swann, C., Moran, A., and Piggott, D. (2015). Defining elite athletes: issues in the study of expert performance in sport psychology. Psychol. Sport Exerc. 16, 3–14. doi: 10.1016/J.PSYCHSPORT.2014.07.004
Watkins, P. (2013). Revisiting the Home Court Advantage in College Basketball. Int. J. Sport Soc. 3, 33–42. doi: 10.18848/2152-7857/CGP/v03i01/53892
Yu, K., Su, Z., and Zhuang, R. (2008). An Exploratory Study of Long-Term Performance Evaluation for Elite Basketball Players. Int. J. Sports Sci. Eng. 2, 195–203.
Keywords : evolution, statistics, tendencies, career, team sports, professional
Citation: Lorenzo J, Lorenzo A, Conte D and Giménez M (2019) Long-Term Analysis of Elite Basketball Players’ Game-Related Statistics Throughout Their Careers. Front. Psychol. 10:421. doi: 10.3389/fpsyg.2019.00421
Received: 30 November 2018; Accepted: 12 February 2019; Published: 27 February 2019.
Reviewed by:
Copyright © 2019 Lorenzo, Lorenzo, Conte and Giménez. This is an open-access article distributed under the terms of the Creative Commons Attribution License (CC BY) . The use, distribution or reproduction in other forums is permitted, provided the original author(s) and the copyright owner(s) are credited and that the original publication in this journal is cited, in accordance with accepted academic practice. No use, distribution or reproduction is permitted which does not comply with these terms.
*Correspondence: Jorge Lorenzo, [email protected]
Disclaimer: All claims expressed in this article are solely those of the authors and do not necessarily represent those of their affiliated organizations, or those of the publisher, the editors and the reviewers. Any product that may be evaluated in this article or claim that may be made by its manufacturer is not guaranteed or endorsed by the publisher.
Thank you for visiting nature.com. You are using a browser version with limited support for CSS. To obtain the best experience, we recommend you use a more up to date browser (or turn off compatibility mode in Internet Explorer). In the meantime, to ensure continued support, we are displaying the site without styles and JavaScript.
- View all journals
- Explore content
- About the journal
- Publish with us
- Sign up for alerts
- Open access
- Published: 25 January 2023
Research on visual search behaviors of basketball players at different levels of sports expertise
- Peng Jin 1 ,
- Zhigang Ge 1 &
- Tieming Fan 2
Scientific Reports volume 13 , Article number: 1406 ( 2023 ) Cite this article
4461 Accesses
5 Citations
1 Altmetric
Metrics details
- Health occupations
This study aims to examine visual search strategies of skilled basketball players in an anticipation task. This study selected 48 experienced and inexperienced basketball players. The participants were grouped into novice and expert groups based on their experience. The participants were asked to look at series of pictures of offensive patterns of play in a basketball game from a third person perspective and chose one of the three options: passing, shooting and break through. This study measured and recorded the response time, key pressing results, and eye movements. Eye movement data were recorded using the Tobii X-3 120 eye tracker. The results showed that, the expert group demonstrated superior anticipation performance, and were more accuracy. In addition, the results showed that participants in the expert group and faster predicted the offensive way faster than the novice group. The results also showed that experienced basketball players employed a simple and efficient visual search strategy including greater fixation counts and longer fixation duration on more informative areas than the novice group. Furthermore, the visualization metrics showed that the expert group had a more concise fixation trajectory and focused mainly on key information area. Generally, expert players exhibited a more efficient and effective visual search strategy demonstrating better performance on anticipation tasks.
Similar content being viewed by others

Viewing angle, skill level and task representativeness affect response times in basketball defence
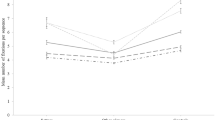
Decision-making and dynamics of eye movements in volleyball experts
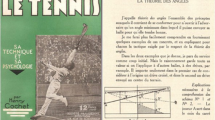
Henri Cochet's theory of angles in tennis (1933) reveals a new facet of anticipation
Introduction.
The ability to anticipate and make accurate decisions on time is fundamental to high performance in sports, particularly team ball games and racket sports 1 , 2 . This claim is supported by visual search studies that use eye registration techniques to examine where expert players direct their line of sight 3 , 4 . Visual search is one of the key factors behind anticipation. It is also one of the common methods used to gain insight into how athletes make decisions in complex and time-constrained situations.
Visual search, the process of finding a target from a set of irrelevant distractor items 5 , 6 , is an important way for people to obtain and process exterior information to interact with the environment effectively 7 . Regarding motor behavior, visual search ability is a key factor in determining the effectiveness of some professional work, including drivers 8 , beach lifeguards 9 , and many military tasks 10 . Visual search is also important in individual sports like judo 11 , cycling 12 , tennis 13 , and team sports like soccer 14 , volleyball 15 , baseball 16 , and handball 6 . Visual search can reveal how athletes process visual information. In addition, visual search can also reflect the cognitive abilities of athletes and aid in decision-making and judgment response which affect performance. Therefore, exploring the visual search characteristics of athletes helps select elite athletes and determine the most effective training that can improve special perceptual skills.
Sports scientists have devoted their efforts to investigating the visual search characteristics of athletes over the past years. Many studies have found differences in visual search strategies between athletes with different expertise in open sports such as soccer 4 , volleyball 17 , 18 , and futsal 19 . Few studies have indicated that experienced athletes demonstrate fewer but longer fixation to more important areas than novice athletes 20 , 21 , 22 . On the contrary, some authors have reported that experienced athletes have more fixations and shorter durations than novice athletes 23 , 24 . Several studies have demonstrated that visual search strategies are influenced by the type of response 25 , the number of players involved 26 , methods applied 27 , and task complexity 28 . These divergent results illustrate that experienced athletes use a different visual search strategy depending on various conditions. Many studies have focused on visual attention as a whole rather than the various parts of visual attention and their role and status in problem discovery. As a result, it is impossible to know what visual attention characteristics benefit experienced players. Therefore, this study aims to investigate the three independent areas of interest (AOI) that constitute the whole offensive situation and their corresponding eye movement characteristics.
Basketball requires players to have a strong ability to process the visual information of fast movement to make judgments and decisions within a short time 29 . During a basketball match, players must analyze a lot of visual information, including factors such as teammates, opponents, and offensive or defensive game patterns 30 . The visual search ability of basketball players directly affects the processing quality and speed, this means that effective visual search strategies help players have better match performances. The development of "small ball tactics" in basketball has gradually become mainstream 31 . The game has the characteristics of "strong confrontation" 32 , "fast rhythm" 33 , and "multi transformation" 34 , which requires athletes to have higher visual search abilities. Therefore, exploring the visual search strategy of experienced basketball players can help athletes improve their performance. In addition, it can help coaches and scientists understand how expert players make decisions and process information 35 .
Most visual search studies in basketball have been limited to closed skills like free throws 36 or jump shots 37 . As a result, many aspects of expert basketball players’ gaze behavior in open-play are still unknown. This study extended on the work by Maarseveen M V et al. 38 , which further analyze the visual search behaviors and decision-making of offensive ball handlers. This study used eye-movement registration techniques and an anticipation task, to compare the differences in visual search strategy between experts and novice athletes. The results reported in this study included key-press response time, response accuracy and gaze behavior. Visualization was added to make the results more intuitive. Participants were required to predict the offensive choice of the ball carrier in attack game scenarios (passing to the teammate, shooting at the basket or driving toward the basket). This study hypothesized that experience basketball players would exhibit a more efficient and effective visual search strategy than the novice group. It was assumed that experienced players have longer fixation duration and more fixation counts on critical AOI, more concise fixation trajectory and focus mainly on key information. In addition, it was assumed that experienced athletes could fixate on areas for longer periods and fewer periods on irrelevant areas. As a result, experienced athletes can make sports decisions with shorter response time and higher response accuracy.
Participants
The sample size was estimated using G*Power3.1.9 software. The sample size considerer the effect size of 0.80, an alpha level of 0.05, the power of 0.80, and two tails. In total, 48 male basketball players were recruited. The participants were divided into an expert group (n = 24, 12 guards and 12 forwards) and a novice group (n = 24, 11 guards and 13 forwards) according to their basketball experience and skills. The expert group comprised eight professional players from China Basketball Association (CBA) teams and 16 college players from China University Basketball Association (CUBA) teams. The average age of the participants was 20.36 years (SD = 2.72), On the other hand, the average years of training for the participants were 9.46 years (SD = 2.68). In addition, all the players were national first-level athletes. The novice group comprised 24 physical education undergraduate students from the Nanjing Sport Institute. The average age of the participants in the novice group was 21.65 years (SD = 2.19), The novice group had an average of 2.46 years of training experience (SD = 2.97). All participants reported normal or corrected-to-normal levels of visual function and were right-handed. In addition, the participants gave their written informed consent following the Declaration of Helsinki. The study was approved by the ethics committee of the Shanghai University of sport (NO.2015003SUS), the participants who completed this study were compensated for their time.
This study used the Tobii Pro X3-120 (Tobii Technology Danderyd, Sweden) eye tracker to measure the gaze behavior during the presentation of stimuli at a constant frame rate of 120 Hz per second utilizing the “corneal reaction techniques”. The data collected using the Tobii Pro X3-120 eye tracker was recorded using a high-performance Dell notebook computer. The numeric keys are also used to judge the response of keys. The study also used one Dell LCD monitor(23 inches, resolution of 1920 × 1080 pixels, refresh rate 120 Hz) to present experimental materials. The eye tracker device was placed under the Dell external monitor and 55-65 cm from the subjects to ensure the stability and high accuracy of eye tracking. Measures of gaze behaviors were calculated using the Tobii studio version 3.3.8 software.
Test stimuli
The test stimuli were based on Adobe Premiere Pro CC software from NBA games. The stimuli included a series of pictures of offensive patterns of play in a basketball game from a third person perspective. Highly experienced basketball coaches assessed the images before being used in this study. AdobePhotoShopCS6 software processed the selected pictures to ensure consistent brightness and saturation. In total, 21 pictures, including six practice pictures and 15 test pictures were included in this study. On the other hand, the clips composed of three types of offensive scenario diagrams; passing, breakthrough and shooting. All the visuals were made into JPG files and imported into Tobii Pro Lab software, which was also used to program and present the experiment. The participants were required to make judgments on the pictures and press the keys to complete the task.
Areas of Interest (AOIs) enable numerical and statistical analysis based on regions or objects of interest in stimuli. Researchers can divide and define AOI based on their experimental hypotheses and research objectives. The Tobii Pro Lab software allows the researcher to divide the AOI before or after the experiment. Therefore, and the size, shape and position of the AOI can be controlled according to the needs of the researcher. This study identified three AIOs based on the literature and the research goals (see Supplementary Figure S1 ). The three AOIs were determined by the coaches based on the evaluation of threat area during attack: K-AOI = key areas of interest(ball hander, ball hander defender, screener/roller, screener/roller defender, best choice teammate), R-AOI = related areas of interest(functional space, i.e., areas of free space in front of the defensive player), I-AOI = irrelevant areas of interest (the remaining space undefined) 3 , 39 .
The participants sat on a chair facing the monitor where the test film was presented. After adjusting the sitting position, the participants were asked to tap their right-hand index finger, middle finger, and ring finger on the keys numbered “1,2,3” on the notebook. The participants sat at a distance of 60 cm from the external monitor and a standard 9-point calibration procedure was performed. After calibration, the participants were show the instructions about the task (Instruction: Several pictures of the basketball game will appear on the screen. Each clip will present for a 5000 ms. Please observe carefully and make a keypress selection within 5000 ms. "1" represents pass, "2" represents shoot, and "3" represents breakthrough). After 10 s, the participants received six practice trials to ensure that they were familiar with the experiment procedure (all subjects performed more than 60 key presses to get familiar with the key position). Finally, if the participants had no questions, there was a reminder indicating the beginning of the experiment, and the experiment began after three seconds.
The study was conducted in trials, and each trial started with a cue picture displayed for 2000 ms. The only image on the screen was the position information of the basketball. The participants were required to only focus on the position of the basketball. The cue was also to inform the participants that the test starts immediately. After 2000 ms, the game scene film was displayed for 5000 ms, the participants were asked to respond as quickly and as accurately as possible to the filmed stimuli. During the presentation, participants were required to press the keystrokes to make a judgement. The next cue picture appeared if the participants failed to press any key within the given timeframe. The correct answers were assessed when all five coaches reached a consensus. The Tobii Pro X3-120 eye-tracker used in this experiment collected date on eye movement and recorded the reaction time and key pressing results of the participants. Participants completed 15 trials, and each test session was completed in approximately 10 min. (see Fig. 1 ).
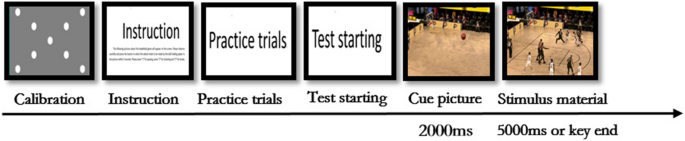
Illustration of the sequential phases included in a visual search task.
Ethics statement
The study was performed in accordance with the recommendations of the “ethics committee of the Shanghai University of sport” with written informed consent from all participants. The participants gave their written informed consent in accordance with the Declaration of Helsinki. The protocol was approved by the “ethics committee of the Shanghai University of sport (No. 2015003SUS)”.
Dependent variables and data analysis
Anticipation test.
Response time (RT) : the time taken for the participants to press the keystrokes to make a judgment. Response time was measured from when the image was displayed to when the key was pressed..
Response accuracy (RA) : the participants was measured using the number of accurately identified images.
Eye tracking data
Fixation: A fixation was defined as a condition in which the eye remained stationary within a tolerance of movements up to 3° for a period greater than 100ms 40 .
Fixation duration : The total duration of the fixations inside this area of interest during an interval (Tobii Prolab_UserManual).
Fixation count : The number of fixations occurring in this area of interest during an interval (Tobii Prolab_UserManual).
Visualization
Gaze plot : A gaze plot is a visualization tool for showing the location, order and time spent at locations on the stimulus. This study used a gaze plot to reveal the time sequence of looking or where and when the participants looked.
Heat map : A visualization tool for showing how looking is distributed over the stimulus. This is a visualization that can effectively reveal the focus of visual attention for dozens or even hundreds of participants at a time.
Data analysis
Data were analyzed using Tobii Pro Lab (version1.21.21571). The eye movement data collected in this experiment were sorted and analyzed using the Tobii Pro Lab software. The reaction time and key selection are automatically recorded by the Participant Events in the Analysis Module. The collected data was then exported to SPSS 23.0 as an EXCEL sheet. Statistical data analyses were done on SPSS 23.0, and the sampling rate of 85% was decided as the standard in this experiment. Each test film was divided into three AOIs for statistical analysis. The gaze plot and heat map are presented in the visualization module. This study performed t-tests to analyze response accuracy, response time, percentage of fixation duration in AOI, percentage of fixation counts in AOI according to expertise (experts versus novices). The standard level of significance was set as p < 0.05.
Response time (RT)
Figure 2 presents the means and standard deviations of the response time between experts and novices, means and standard deviations calculated as the average overall clips for each individual, as well as the grand average for the group. The K–S test was used, which presented normal distribution. On this basis, the independent sample T-test was performed on response time of the two groups. As shown in Fig. 2 , the experts group had a significantly faster response time than the novice group (t = − 3.35, p < 0.05; d = 0.96).
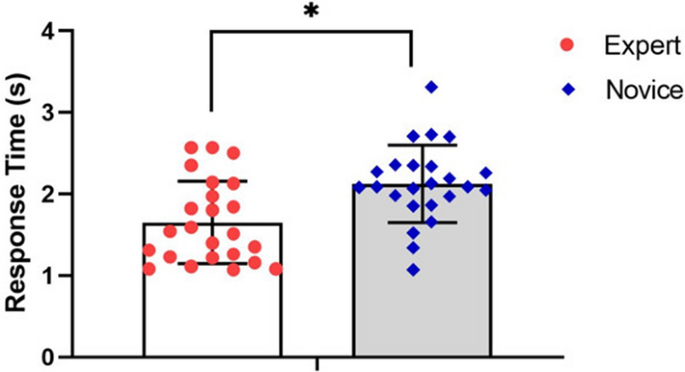
Comparison of the response time between groups.
Response accuracy (RA)
The difference in response accuracy between the two groups is shown in Fig. 3 . The difference in RA is presented as an average and standard deviation. This study used the K-S test, which presented normal distribution, and an independent sample t-test which compared the two groups. The results in Fig. 3 show that the response accuracy of expert players was greater than that of novice players (t = 3.63, p < 0.05; d = 0.89). This result indicates that expert players can make better judgments than novice payers in visual search tasks.
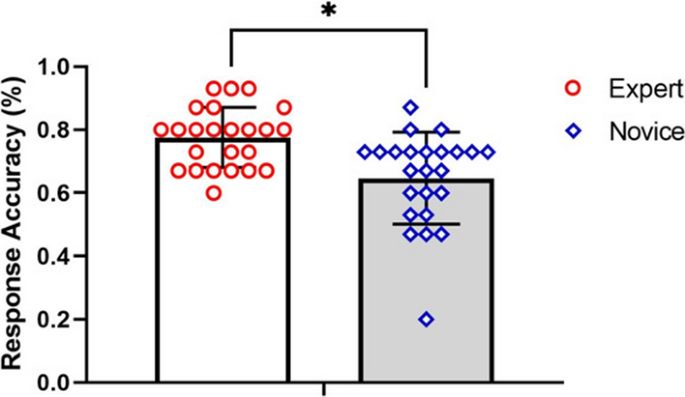
Comparison of the response accuracy between groups.
Percentage of fixation duration in AOI
The independent t-test results show that expert players and novice players spent more time fixated on K-AOI (M = 0.56 ± 0.10 for the expert group vs. M = 0.53 ± 0.14 for the novice group). However, there were no differences between the two groups (t = 0.75, p ˃0.05; d = 0.25). The study found that expert players demonstrated a greater percentage of fixation duration in R-AOI than novice players (M = 0.29 ± 0.11 vs. 0.17 ± 0.09, t = 4.30, p < 0.05; d = 1.19). Also, expert players spent a smaller percentage of fixation duration in I-AOI than novice players (M = 0.15 ± 0.07 vs. 0.30 ± 0.12, t = − 5.13, p < 0.05; d = 1.53) (see Fig. 4 ).
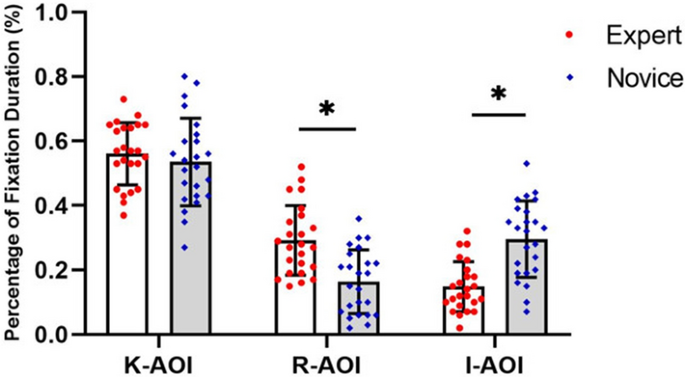
Comparison of the percentage of fixation duration in AOIs between groups.
Percentage of fixation counts in AOI
The results show that although the expert and novice groups spent more percentage of fixation counts in K-AOI (M = 0.54 ± 0.12 vs. M = 0.50 ± 0.10), there was no difference between the two groups in terms of the percentage of fixation counts in K-AOI (t = − 1.17, p > 0.05; d = 0.36). The results also show that the expert group had a greater percentage of fixation counts in R-AOI than novice players (M = 0.28 ± 0.11 vs. M = 0.19 ± 0.08, t = − 1.17, p < 0.05; d = 0.94). On the contrary, the novice group had a higher percentage of fixation counts in I-AOI than the expert group (M = 0.18 ± 0.11 vs. 0.31 ± 0.10, t = − 4.25, p < 0.05; d = 1.24) (see Fig. 5 ).
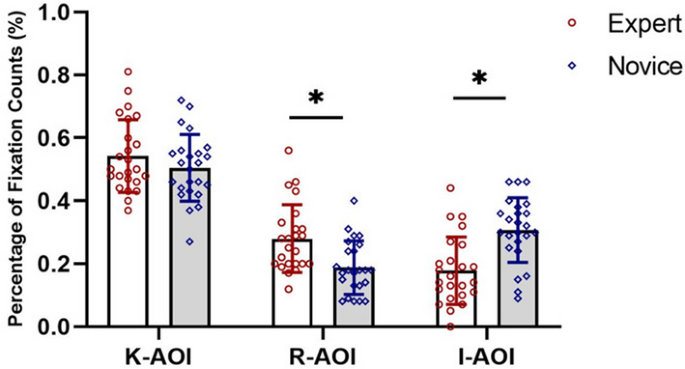
Comparison of the percentage of fixation counts in AOIs between groups.
The gaze plot illustrates that the gaze trajectories of the two groups are different. The expert group had fewer fixation counts, and the fixation (dot) was concentrated in the K-AOI and R-AOI. On the contrary, the fixation counts of the novice group were scattered and irregular (see Figs. 6 , 7 , 8 , and 9 ).

Aggregated Gaze Plot for experts.
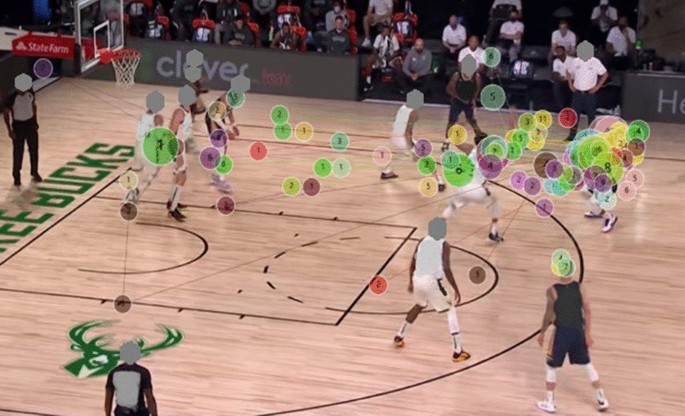
Aggregated Gaze Plot for novices.
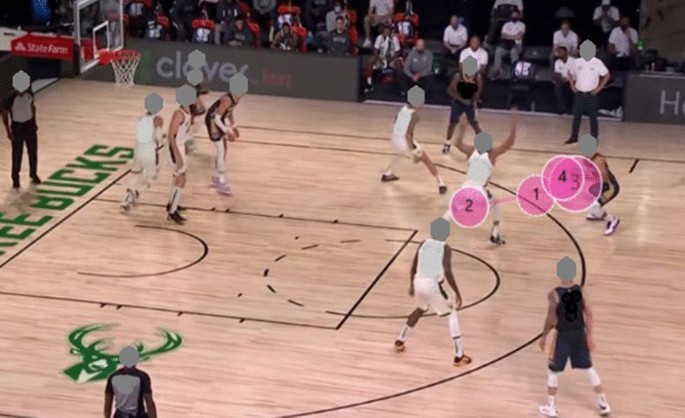
Example of Gaze Plot for experts.
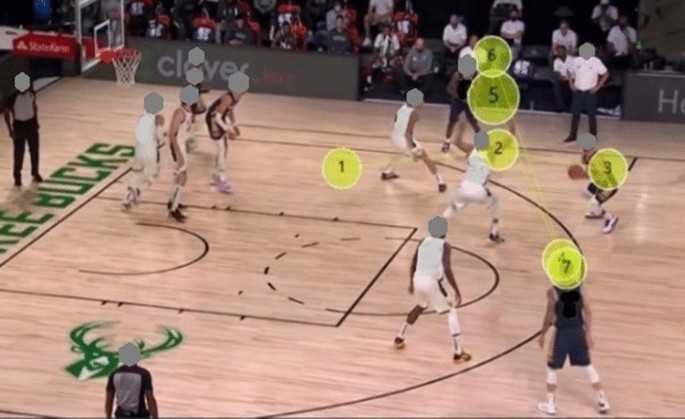
Example of Gaze Plot for novices.
The heat map shows that the attention of the expert groups was more focused than the attention of the novice group. The heat map also shows that the expert group pays more attention to the K-AOI and R-AOI, while the novice group not only pays attention to the K-AOI but also allocate more attention resources to the I-AOI (see Figs. 10 and 11 ).
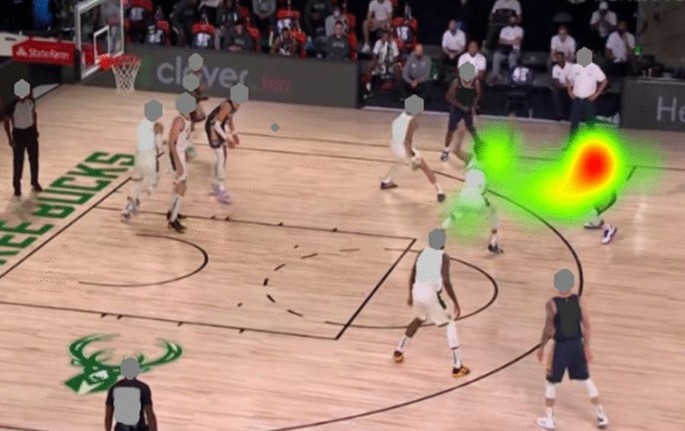
Heat map for experts.
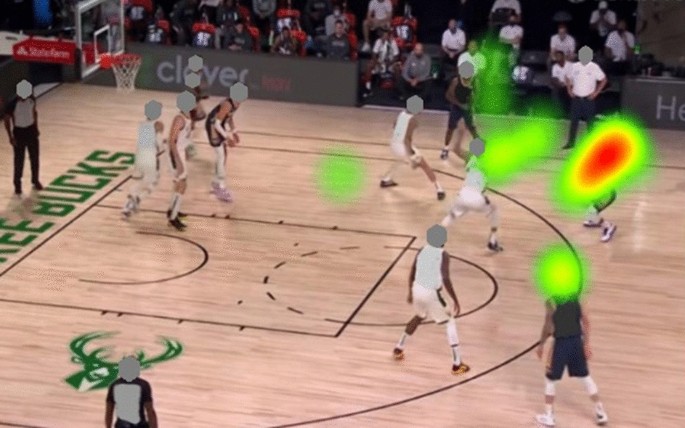
Heat map for novices.
The general aim of the current study was to analyze the difference in visual search strategies between expert and novice basketball players using film-based offensive scenarios of a basketball game. The results supported the hypothesis: expert basketball players have higher response accuracy and shorter response time. In addition, the results of the eye tracking date and visualization also showed that expert athletes have more advantages in judging and processing key information areas and more reasonable fixation distribution than novice players.
As predicted, the expert group was faster in response time and more accurate in predicting offensive ways than the novice group. This result is consistent with the previous research involving players with different skill levels 24 , 41 , 42 . This is likely because expert players have accumulated domain-specific knowledge and structural information through a lot of time spent in competition and training. Therefore, when presented with similar competitive scene, experts develop strong sense of identity and use fewer cognitive steps to make decisions resulting in a shorter response time, this concept was also supported by Dittrich’s research 43 . In contrast, less-skilled players cannot successfully anticipate because they lack episodic and task-related expertise. As a result, novice players took a lot of time to respond and had a low response accuracy. However, this study is inconsistent with previous findings, especially studies relating to research in volleyball and French boxing 44 , 45 . They failed to find a significant difference in response time between different groups, which could be attributed to the specific demands and sport type. In addition, analysis of the gaze behaviors can better explain the anticipation process when expert players respond in a short time.
Unlike previous researchers, who investigated the differences in gaze behaviors between different skilled-level players, most studies in this research field have reported that the expert groups employ more efficient strategies that involved more number of fixations and shorter fixation duration than their novice counterparts 14 , 17 , 24 , 26 . This research has illustrated that expert players exhibit superior performance on the film-based test. However, several studies in this area adopts a holistic research approach where the experimental materials are considered as a whole and not divided into regions. There needs to be more differentiated research on the role and status of the distinct region.
In the study, we were mainly interested in examining the difference between two groups (expert and novice) of basketball players on percentage of fixation duration and Percentage of fixation counts on AOIs. The results showed that the expert and novice groups had a 50% fixation duration on the K-AOI. This result illustrates that experienced and inexperienced players realized that the K-AOI is the essential location to supply the critical information to understand the scenario better. This result is consistent with previous studies that reported that players prefer to focus on the players controlling the ball and the defenders because these factors are the most important elements for the players to extract effective information 46 . This visual feature helps experienced athletes improve their visual processing efficiency and respond correctly.
This study found skilled-based differences in the percentage of fixation duration and fixation counts on R-AOI and I-AOI. The study found that experienced players prefer spending a higher percentage of fixation duration and percentage of fixation counts on R-AOI and a lower percentage on I-AOI than the novice groups. In addition, it has been established that experienced players make more fixations of longer durations on relevant AOIs. The work by other studies also support our results. Researchers assessed expertise in chess and found that the experienced chess players pay more attention to the middle of the board than their chess pieces. This result suggests that experts are better at selectively allocating their attention and ignoring irrelevant areas 47 , 48 . On the other hand, experienced basketball players utilize their experience and knowledge to direct their attention to the R-AOI because those locations contain the functional space between the ball hander, the corresponding teammate, and their defenders to analyze their offensive choices. On the contrary, novice players pay attention to all the visual stimuli on the field due to the possibility that lack of experience. As a result, novice players have invested more attention resources in I-AOI.
These results are consistent with the existing evidence that the players with higher percentage of correct anticipation distribute more attention towards the functional place, however, the players with lower percentage of correct anticipation prefer to look more on the space outside the players 38 . Similar results have also been reported in other team ball sports such as soccer and volleyball 46 , 49 . Nonetheless, these results support the information reduction hypothesis: experts should exhibit longer fixation duration and more fixation counts on task-relevant areas and shorter fixation durations and fewer fixation counts on task-irrelevant areas 50 . In addition, these findings support the guide search theory which states that experienced participants will systematically move their focus from one AOI to another, guided by experience or previous knowledge 51 . However, some studies have found an opposite results pattern. These studies have reported that experienced players made fewer fixations of shorter durations on the relevant region 52 , 53 . These results are supported by the long-term working memory theory 54 . Experienced players encode and retrieve information more rapidly from long-term working memory, contributing to their shorter fixation durations than novices. This is because experts use parafoveal regions to extract information from many visual cues 11 , 55 .
Heat maps create a more user-friendly pattern for visualizing eye-tracking metrics for multiple subjects. Results show that K-AOI received the same attention (50%) from all subjects. As a result, it can be concluded that K-AOI is the most important decision area. However, the biggest difference in attention allocation between the experts and the novices is the attention allocation on R-AOIs and I-AOIs. The expert players invest more attention to the R-AOIs, which may be responsible for the "fast and accurate" anticipation tasks. In contrast, the novice players pay more attention to the I-AOIs, indicating that the novice group focused on more scattered areas and could not obtain the most critical information in a short time.
Gaze plot can comprehensively and intuitively illustrate the spatio-temporal characteristics of the visual search of participants, and is useful in showing a user’s sequence of fixation. The gaze plot resluts show that the fixation of the expert players is mostly concentrated in the K-AOI and R-AOI, and the fixation trajectory is simple and clear. Therefore, researchers have concluded that expert players know where the critical and relevant cues for proper decision-making are in the game scenario. In contrast, novice players fixate more on the I-AOI and show complex fixation points, irregular and more looking back trajectories. The fixation strategy of novice athletes reduces the efficiency of visual information processing and wastes limited attention resources. This strategy has been attributed to why novice players have slower response time and lower accuracy when performing decision-making task 38 .
There were several limitations to the present study. The main shortcoming was that the stimulating materials could not be presented from a first-person perspective, and future research should take stimulating pictures from the perspective of players. Second, this study utilized only offensive situations, yet a real match situation involves not only offensive situations but also defensive situations. Therefore, this study encourages future studies to use defensive situations to understand visual search strategies better. Third, video-based tests were not used in our study. Dynamic stimulation materials are more in line with the reality of the game. As a result, this study encourages future studies to use dynamic contexts to improve the ecological validity of the experiment. Additionally, this study did not take into account the relationship between observable cues and decision-making. Therefore, future researchers should try to demonstrate this relationship. Meanwhile, further investigation should employ a broader range of metrics to enrich our understanding about characteristics of visual search strategies among expert players. Finally, the present study is not a Randomized Controlled trial, it is impossible for our paradigm to organize participants randomly, it is always possible that highly practiced basketball players had better visual search strategies to start with. Therefore, a longitudinal design is necessary to observe the process of the change in visual search abilities longitudinally in basketball players as time goes on in the future study.
In summary, the results of the present study indicate that experienced basketball players exhibit a more efficient and effective visual search strategy than novice players. They employed a higher fixation duration and greater fixation counts on more informative areas when looking at the game-situation pictures. These visual search characteristics illustrate that expert basketball players demonstrate a faster response time and greater response accuracy in a decision-making test.
Data availability
The datasets generated and analyzed during in this study are available from the corresponding author upon reasonable request.
Mann, D. L., Causer, J., Nakamoto, H. & Runswick, O. R. Visual search behaviors in expert perceptual judgements. In Anticipation and Decision Making in Sport , 59–78 (Routledge, 2019).
Murray, N. P. & Hunfalvay, M. A comparison of visual search strategies of elite and non-elite tennis players through cluster analysis. J. Sports Sci. 35 , 241–246. https://doi.org/10.1080/02640414.2016.1161215 (2017).
Article Google Scholar
Afonso, J., Garganta, J., Mcrobert, A., Williams, A. M. & Mesquita, I. The perceptual cognitive processes underpinning skilled performance in volleyball: Evidence from eye-movements and verbal reports of thinking involving an in situ representative task. J. Sports Sci. Med. 11 , 339 (2012).
Google Scholar
Natsuhara, T. et al. Decision-making while passing and visual search strategy during ball receiving in team sport play. Percept. Mot. Skills 127 , 468–489. https://doi.org/10.1177/0031512519900057 (2020).
Vromen, J. M. G., Becker, S. I., Baumann, O., Mattingley, J. B. & Remington, R. W. Flexible information coding in frontoparietal cortex across the functional stages of cognitive processing. bioRxiv https://doi.org/10.1101/246132 (2018).
Ribeiro, L. et al. Tactical knowledge and visual search analysis of female handball athletes from different age groups. J. Phys. Educ. Sport 21 , 948–955. https://doi.org/10.7752/jpes.2021.02118 (2021).
Eckstein, M. P. Visual search: A retrospective. J. Vis. 11 , 74–76. https://doi.org/10.1167/11.5.14 (2011).
Chapman, P., Underwood, G. & Roberts, K. Visual search patterns in trained and untrained novice drivers. Transp. Res. Part F: Psychol. Behav. 5 , 157–167. https://doi.org/10.1016/S1369-8478(02)00014-1 (2002).
Page, J., Bates, V., Long, G., Dawes, P. & Tipton, M. Beach lifeguards: visual search patterns, detection rates and the influence of experience. Ophthalmic Physiol. Opt. 31 , 216–224. https://doi.org/10.1111/j.1475-1313.2011.00824.x (2011).
Kramer, M. R., Porfido, C. L. & Mitroff, S. R. Evaluation of strategies to train visual search performance in professional populations. Curr. Opin. Psychol. 29 , 113–118. https://doi.org/10.1016/j.copsyc.2019.01.001 (2019).
Piras, A., Pierantozzi, E. & Squatrito, S. Visual search strategy in judo fighters during the execution of the first grip. Int. J. Sports Sci. Coach. 9 , 185–198. https://doi.org/10.1260/1747-9541.9.1.185 (2014).
Vansteenkiste, P. et al. Cycling around a curve: the effect of cycling speed on steering and gaze behavior. PLoS ONE 9 , e102792. https://doi.org/10.1371/journal.pone.0102792 (2014).
Article ADS CAS Google Scholar
Shim, J., Carlton, L. G., Chow, J. W. & Chae, W.-S. The use of anticipatory visual cues by highly skilled tennis players. J. Mot. Behav. 37 , 164–175. https://doi.org/10.3200/JMBR.37.2.164-175 (2005).
de Assis, J. V., González-Víllora, S., Clemente, F. M., Cardoso, F. & Teoldo, I. Do youth soccer players with different tactical behaviour also perform differently in decision-making and visual search strategies?. Int. J. Perform. Anal. Sport 20 , 1143–1156. https://doi.org/10.1080/24748668.2020.1838784 (2020).
Piras, A., Lobietti, R. & Squatrito, S. Response time, visual search strategy, and anticipatory skills in volleyball players. J. Ophthalmol. https://doi.org/10.1155/2014/189268 (2014).
Takeuchi, T. & Inomata, K. Visual search strategies and decision making in baseball batting. Percept. Mot. Skills 108 , 971-980E. https://doi.org/10.2466/PMS.108.3.971-980 (2009).
Afonso, J. & Mesquita, I. Skill-based differences in visual search behaviours and verbal reports in a representative film-based task in volleyball. Int. J. Perform. Anal. Sport 13 , 669–677. https://doi.org/10.1080/24748668.2013.11868679 (2013).
Castro, H. D. O., Praça, G. M., Costa, G. D. C. T., Pedrosa, G. F. & Greco, P. J. Visual behavior and the quality of decision-making on volleyball. Revista Brasileira de Cineantropometria & Desempenho Humano 18 , 638–647. https://doi.org/10.1590/1980-0037 (2016).
Corrêa, U. C., Oliveira, T. A. C. D., Clavijo, F. A. R., da Silva, S. L. & Zalla, S. Time of ball possession and visual search in the decision-making on shooting in the sport of futsal. Int. J. Perform. Anal. Sport 20 , 254–263. https://doi.org/10.1080/24748668.2020.1741916 (2020).
Gegenfurtner, A., Lehtinen, E. & Säljö, R. Expertise differences in the comprehension of visualizations: A meta-analysis of eye-tracking research in professional domains. Educ. Psychol. Rev. 23 , 523–552. https://doi.org/10.1007/s10648-011-9174-7 (2011).
Kredel, R., Vater, C., Klostermann, A. & Hossner, E.-J. Eye-tracking technology and the dynamics of natural gaze behavior in sports: A systematic review of 40 years of research. Front. Psychol. 8 , 1845. https://doi.org/10.3389/fpsyg.2017.01845 (2017).
Bertrand, C. & Thullier, F. Effects of player position task complexity in visual exploration behavior in soccer. Int. J. Sport Psychol. 40 , 306–323. https://doi.org/10.1016/j.humov.2008.12.002 (2009).
Williams, A. M. & Davids, K. Visual search strategy, selective attention, and expertise in soccer. Res. Q Exerc. Sport 69 , 111–128. https://doi.org/10.1080/02701367.1998.10607677 (1998).
Article CAS Google Scholar
Roca, A., Ford, P. R., McRobert, A. P. & Williams, A. M. Identifying the processes underpinning anticipation and decision-making in a dynamic time-constrained task. Cogn. Process. 12 , 301–310 (2011).
Klostermann, A., Vater, C., Kredel, R. & Hossner, E.-J. Perception and action in sports on the functionality of foveal and peripheral vision. Front. Sports Active Living 1 , 66. https://doi.org/10.3389/fspor.2019.00066 (2020).
Vaeyens, R., Lenoir, M., Williams, A. M., Mazyn, L. & Philippaerts, R. M. The effects of task constraints on visual search behavior and decision-making skill in youth soccer players. J. Sport Exerc. Psychol. 29 , 147–169. https://doi.org/10.1123/jsep.29.2.147 (2007).
Raab, M. & Johnson, J. G. Expertise-based differences in search and option-generation strategies. J. Exp. Psychol. Appl. 13 , 158. https://doi.org/10.1037/1076-898X.13.3.158 (2007).
Williams, A. M., Davids, K. & Williams, J. G. Visual Perception & Action in Sport. https://doi.org/10.1146/annurev.ps.29.020178.000335 (1999).
Jin, P. et al. Dynamic visual attention characteristics and their relationship to match performance in skilled basketball players. PeerJ 8 , e9803. https://doi.org/10.7717/peerj.9803 (2020).
Gil-Arias, A., Garcia-Gonzalez, L., Alvarez, F. D. V. & Gallego, D. I. Developing sport expertise in youth sport: A decision training program in basketball. PeerJ 7 , e7392. https://doi.org/10.7717/peerj.7392 (2019).
Zhang, S., Lorenzo, A., Woods, C. T., Leicht, A. S. & Gomez, M.-A. Evolution of game-play characteristics within-season for the National Basketball Association. Int. J. Sports Sci. Coach. 14 , 355–362. https://doi.org/10.1177/1747954119847171 (2019).
Zhu, Q. Classification and optimization of basketball players’ training effect based on particle swarm optimization. J. Healthc. Eng. https://doi.org/10.1155/2022/2120206 (2022).
Zuccolotto, P., Manisera, M. & Sandri, M. Big data analytics for modeling scoring probability in basketball: The effect of shooting under high-pressure conditions. Int. J. Sports Sci. Coach. 13 , 569–589. https://doi.org/10.1177/174795411773749 (2018).
Zhang, S. et al. Players’ technical and physical performance profiles and game-to-game variation in NBA. Int. J. Perform. Anal. Sport 17 , 466–483. https://doi.org/10.1080/24748668.2017.1352432 (2017).
Hüttermann, S., Noël, B. & Memmert, D. Eye tracking in high-performance sports: Evaluation of its application in expert athletes. Int. J. Comput. Sci. Sport 17 , 182–203. https://doi.org/10.2478/ijcss-2018-0011 (2018).
Uchida, Y., Mizuguchi, N., Honda, M. & Kanosue, K. Prediction of shot success for basketball free throws: Visual search strategy. Eur. J. Sport Sci. 14 , 426–432. https://doi.org/10.1080/17461391.2013.866166 (2014).
Van Maarseveen, M. J. & Oudejans, R. R. Motor and gaze behaviors of youth basketball players taking contested and uncontested jump shots. Front. Psychol. 9 , 706. https://doi.org/10.3389/fpsyg.2018.00706 (2018).
Van Maarseveen, M. J., Savelsbergh, G. J. & Oudejans, R. R. In situ examination of decision-making skills and gaze behaviour of basketball players. Hum. Mov. Sci. 57 , 205–216 (2018).
Tenenbaum, G. Expert athletes: An integrated approach to decision making. In Expert Performance in Sports: Advances in Research on Sport Expertise (eds Starkes, J. L., Ericsson, K. A.) 191–218. United States of America: Human Kinetics (2003).
Panchuk, D. & Vickers, J. N. Gaze behaviors of goaltenders under spatial–temporal constraints. Hum. Mov. Sci. 25 , 733–752. https://doi.org/10.1016/j.humov.2006.07.001 (2006).
North, J. S., Ward, P., Ericsson, A. & Williams, A. M. Mechanisms underlying skilled anticipation and recognition in a dynamic and temporally constrained domain. Memory 19 , 155–168. https://doi.org/10.1080/09658211.2010.541466 (2011).
Williams, M. A., Ward, J. D., Ward, P. & Smeeton, N. J. Domain specificity, task specificity, and expert performance. Res. Q. Exerc. Sport 79 , 428–433. https://doi.org/10.1080/02701367.2008.10599509 (2008).
Dittrich, W. H. Seeing biological motion - is there a role for cognitive strategies?. Lect. Notes Comput. Sci. 1739 , 3–22. https://doi.org/10.1007/3-540-46616-9_1 (1999).
Vansteenkiste, P., Vaeyens, R., Zeuwts, L., Philippaerts, R. & Lenoir, M. Cue usage in volleyball: A time course comparison of elite, intermediate and novice female players. Biol. Sport 31 , 295. https://doi.org/10.5604/20831862.1127288 (2014).
Ripoll, H., Kerlirzin, Y., Stein, J.-F. & Reine, B. Analysis of information processing, decision making, and visual strategies in complex problem solving sport situations. Hum. Mov. Sci. 14 , 325–349. https://doi.org/10.1016/0167-9457(95)00019-O (1995).
Williams, A. & Davids, K. Visual search strategy, selective attention, and expertise in soccer. Res. Q. Exerc. Sport 69 , 111–128 (1998).
Bilalić, M., Kiesel, A., Pohl, C., Erb, M. & Grodd, W. It takes two–skilled recognition of objects engages lateral areas in both hemispheres. PLoS ONE 6 , e16202. https://doi.org/10.1371/journal.pone.0016202 (2011).
Reingold, E. M., Charness, N., Pomplun, M. & Stampe, D. M. Visual span in expert chess players: Evidence from eye movements. Psychol. Sci. 12 , 48–55. https://doi.org/10.1111/1467-9280.00309 (2001).
Piras, A., Lobietti, R. & Squatrito, S. A study of saccadic eye movement dynamics in volleyball: Comparison between athletes and non-athletes. J. Sports Med. Phys. Fitness 50 , 99. https://doi.org/10.1080/14763141003690229 (2010).
Haider, H. & Frensch, P. A. Eye movement during skill acquisition: More evidence for the information-reduction hypothesis. J. Exp. Psychol. Learn. Mem. Cogn. 25 , 172. https://doi.org/10.1037/0278-7393.25.1.172 (1999).
Wolfe, J. M. Guided search 2.0 a revised model of visual search. Psychon. Bul. Rev. 1 , 202–238. https://doi.org/10.3758/BF03200774 (1994).
Wu, Y. et al. The role of visual perception in action anticipation in basketball athletes. Neuroscience 237 , 29–41. https://doi.org/10.1016/j.neuroscience.2013.01.048 (2013).
Campbell, M. J. & Moran, A. P. There is more to green reading than meets the eye! Exploring the gaze behaviours of expert golfers on a virtual golf putting task. Cogn. Process. 15 , 363–372. https://doi.org/10.1007/s10339-014-0608-2 (2014).
Ericsson, K. A. & Kintsch, W. Long-term working memory. Psychol. Rev. 102 , 211–245. https://doi.org/10.1037//0033-295X.102.2.211 (1995).
Piras, A. & Vickers, J. N. The effect of fixation transitions on quiet eye duration and performance in the soccer penalty kick: Instep versus inside kicks. Cogn. Process. 12 , 245–255. https://doi.org/10.1007/s10339-011-0406-z (2011).
Download references
Acknowledgements
The authors thank the engineer of Tobii Technology Inc, Suzhou Co,.Ltd for providing technical support. The authors also like to thank all the coaches and players for their endorsement and participation in the study.
Author information
Authors and affiliations.
Department of Physical Education, Southeast University, Nanjing, China
Peng Jin & Zhigang Ge
No. 9 Middle School, Nanjing, China
Tieming Fan
You can also search for this author in PubMed Google Scholar
Contributions
J.P. and F.T. contributed to conceptualization, data collection and processing, writing-review and editing, and supervision. G.Z. was involved in scenario filming and stimulus creation, and manuscript preparation. F.T. contributed to methodology and writing-original draft preparation. All authors contributed to the article and approved it for publication.
Corresponding author
Correspondence to Peng Jin .
Ethics declarations
Competing interests.
The authors declare no competing interests.
Additional information
Publisher's note.
Springer Nature remains neutral with regard to jurisdictional claims in published maps and institutional affiliations.
Supplementary Information
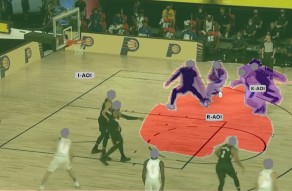
Supplementary Figure S1.
Supplementary information 2., supplementary information 3., supplementary information 4., rights and permissions.
Open Access This article is licensed under a Creative Commons Attribution 4.0 International License, which permits use, sharing, adaptation, distribution and reproduction in any medium or format, as long as you give appropriate credit to the original author(s) and the source, provide a link to the Creative Commons licence, and indicate if changes were made. The images or other third party material in this article are included in the article's Creative Commons licence, unless indicated otherwise in a credit line to the material. If material is not included in the article's Creative Commons licence and your intended use is not permitted by statutory regulation or exceeds the permitted use, you will need to obtain permission directly from the copyright holder. To view a copy of this licence, visit http://creativecommons.org/licenses/by/4.0/ .
Reprints and permissions
About this article
Cite this article.
Jin, P., Ge, Z. & Fan, T. Research on visual search behaviors of basketball players at different levels of sports expertise. Sci Rep 13 , 1406 (2023). https://doi.org/10.1038/s41598-023-28754-2
Download citation
Received : 19 June 2022
Accepted : 24 January 2023
Published : 25 January 2023
DOI : https://doi.org/10.1038/s41598-023-28754-2
Share this article
Anyone you share the following link with will be able to read this content:
Sorry, a shareable link is not currently available for this article.
Provided by the Springer Nature SharedIt content-sharing initiative
This article is cited by
Effects of object working memory load on visual search in basketball players: an eye movement study.
BMC Psychology (2023)
By submitting a comment you agree to abide by our Terms and Community Guidelines . If you find something abusive or that does not comply with our terms or guidelines please flag it as inappropriate.
Quick links
- Explore articles by subject
- Guide to authors
- Editorial policies
Sign up for the Nature Briefing newsletter — what matters in science, free to your inbox daily.


An official website of the United States government
The .gov means it’s official. Federal government websites often end in .gov or .mil. Before sharing sensitive information, make sure you’re on a federal government site.
The site is secure. The https:// ensures that you are connecting to the official website and that any information you provide is encrypted and transmitted securely.
- Publications
- Account settings
- My Bibliography
- Collections
- Citation manager
Save citation to file
Email citation, add to collections.
- Create a new collection
- Add to an existing collection
Add to My Bibliography
Your saved search, create a file for external citation management software, your rss feed.
- Search in PubMed
- Search in NLM Catalog
- Add to Search
Effect of core training on athletic and skill performance of basketball players: A systematic review
Affiliations.
- 1 Faculty of Physical Education and Art, Jiangxi University of Science and Technology, Jiangxi Province, China.
- 2 Faculty of Educational Studies, Department of Sports Studies, Universiti Putra Malaysia, Serdang, Malaysia.
- 3 School of Foreign Languages, Yuxi Normal University, Yuxi, China.
- 4 Faculty of Educational Studies, Department of Foundation of Education, Universiti Putra Malaysia, Serdang, Malaysia.
- 5 Faculty of Medicine and Health Sciences, Department of Nursing, Universiti Putra Malaysia, Serdang, Malaysia.
- 6 Faculty of Educational Studies, Department of Language and Humanities Education, Universiti Putra Malaysia, Serdang, Malaysia.
- PMID: 37347733
- PMCID: PMC10286970
- DOI: 10.1371/journal.pone.0287379
A limited number of studies focus on the effect of core training on basketball players' athletic performance and skills. This systematic reviewaimed to comprehensively and critically review the available studies in the literature that investigate the impact of core training on basketball players' physical and skill performance, and then offer valuable recommendations for both coaches and researchers. Thedata collection, selection, and analysis adhered to the PRISMA protocol. English databases, including Ebscohost, Scopus, PubMed, Web of Science, and Google Scholar,were searched until September 2022. A total of eight articles were included, with four studies comparing the effects of core training versus traditional strength training or usual basketball training. All studies investigated the impact of core training on athletic performance. The findings revealed that core training can help players improve their overall athletic and skill performance, particularly in the areas of strength, sprinting,jumping, balance, agility, shooting, dribbling, passing, rebounding, and stepping. In addition, core training, particularly on unstable surfaces,as well as combining static and dynamic core training,improvebasketball players' athletic and skill performance. Despite the relativelylittle evidence demonstrating the effect of core training on endurance, flexibility, and defensive skills, this review demonstrates that it should be incorporated into basketball training sessions.
Copyright: © 2023 Luo et al. This is an open access article distributed under the terms of the Creative Commons Attribution License, which permits unrestricted use, distribution, and reproduction in any medium, provided the original author and source are credited.
PubMed Disclaimer
Conflict of interest statement
The authors have declared that no competing interests exist.
Fig 1. The PRISMA flow chart for…
Fig 1. The PRISMA flow chart for the search, screening, and selection strategy for the…
Similar articles
- The effects of functional training on physical fitness and skill-related performance among basketball players: a systematic review. Cao S, Liu J, Wang Z, Geok SK. Cao S, et al. Front Physiol. 2024 May 9;15:1391394. doi: 10.3389/fphys.2024.1391394. eCollection 2024. Front Physiol. 2024. PMID: 38784117 Free PMC article.
- Effect of core strength training on the badminton player's performance: A systematic review & meta-analysis. Ma S, Soh KG, Japar SB, Liu C, Luo S, Mai Y, Wang X, Zhai M. Ma S, et al. PLoS One. 2024 Jun 12;19(6):e0305116. doi: 10.1371/journal.pone.0305116. eCollection 2024. PLoS One. 2024. PMID: 38865415 Free PMC article.
- Association Between Conditioning Capacities and Shooting Performance in Professional Basketball Players: An Analysis of Stationary and Dynamic Shooting Skills. Pojskic H, Sisic N, Separovic V, Sekulic D. Pojskic H, et al. J Strength Cond Res. 2018 Jul;32(7):1981-1992. doi: 10.1519/JSC.0000000000002100. J Strength Cond Res. 2018. PMID: 29939949
- The Jump Shot Performance in Youth Basketball: A Systematic Review. França C, Gomes BB, Gouveia ÉR, Ihle A, Coelho-E-Silva MJ. França C, et al. Int J Environ Res Public Health. 2021 Mar 22;18(6):3283. doi: 10.3390/ijerph18063283. Int J Environ Res Public Health. 2021. PMID: 33810053 Free PMC article. Review.
- The effects of plyometric jump training on physical fitness attributes in basketball players: A meta-analysis. Ramirez-Campillo R, García-Hermoso A, Moran J, Chaabene H, Negra Y, Scanlan AT. Ramirez-Campillo R, et al. J Sport Health Sci. 2022 Nov;11(6):656-670. doi: 10.1016/j.jshs.2020.12.005. Epub 2020 Dec 24. J Sport Health Sci. 2022. PMID: 33359798 Free PMC article. Review.
- The Effects of Core Stabilization Trunk Muscle Fatigue on Lower Limb Stiffness of Basketball Players. Tazji MK, Sadeghi H, Abbasi A, Aziminia M, Shahhosseini A, Marjani ME, Koumantakis GA. Tazji MK, et al. Sports (Basel). 2023 Oct 12;11(10):200. doi: 10.3390/sports11100200. Sports (Basel). 2023. PMID: 37888527 Free PMC article.
- Roman IR, Molinuevo JS, Quintana MS. The relationship between exercise intensity and performance in drills aimed at improving the proficiency, technical and tactical skills of basketball players. RICYDE Revista Internacional de Ciencias del Deporte. 2009;(14):1–10.
- Kostopoulos N, Bekris E, Apostolidis N, Kavroulakis E, Kostopoulos P. The effect of a balance and proprioception training program on amateur basketball players’ passing skills. Journal of Physical Education and Sport. 2012;12(3):316–23.
- Reed CA, Ford KR, Myer GD, Hewett TE. The effects of isolated and integrated ‘core stability’training on athletic performance measures. Sports medicine. 2012;42(8):697–706. - PMC - PubMed
- Montgomery PG, Pyne DB, Minahan CL. The physical and physiological demands of basketball training and competition. International journal of sports physiology and performance. 2010;5(1):75–86. doi: 10.1123/ijspp.5.1.75 - DOI - PubMed
- García F, Castellano J, Reche X, Vázquez-Guerrero J. Average Game Physical Demands and the Most Demanding Scenarios of Basketball Competition in Various Age Groups. Journal of Human Kinetics. 2021;79(1):165–74. doi: 10.2478/hukin-2021-0070 - DOI - PMC - PubMed
Publication types
- Search in MeSH
Grants and funding
Linkout - more resources, full text sources.
- Europe PubMed Central
- PubMed Central
- Public Library of Science
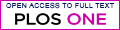
- Citation Manager
NCBI Literature Resources
MeSH PMC Bookshelf Disclaimer
The PubMed wordmark and PubMed logo are registered trademarks of the U.S. Department of Health and Human Services (HHS). Unauthorized use of these marks is strictly prohibited.
A Bayesian network to analyse basketball players’ performances: a multivariate copula-based approach
- Original Research
- Open access
- Published: 09 August 2022
- Volume 325 , pages 419–440, ( 2023 )
Cite this article
You have full access to this open access article
- Pierpalo D’Urso 1 ,
- Livia De Giovanni 2 &
- Vincenzina Vitale ORCID: orcid.org/0000-0001-9512-3609 1
3293 Accesses
2 Citations
Explore all metrics
Statistics in sports plays a key role in predicting winning strategies and providing objective performance indicators. Despite the growing interest in recent years in using statistical methodologies in this field, less emphasis has been given to the multivariate approach. This work aims at using the Bayesian networks to model the joint distribution of a set of indicators of players’ performances in basketball in order to discover the set of their probabilistic relationships as well as the main determinants affecting the player’s winning percentage. From a methodological point of view, the interest is to define a suitable model for non-Gaussian data, relaxing the strong assumption on normal distribution in favour of Gaussian copula. Through the estimated Bayesian network, we discovered many interesting dependence relationships, providing a scientific validation of some known results mainly based on experience. At last, some scenarios of interest have been simulated to understand the main determinants that contribute to rising in the number of won games by a player.
Similar content being viewed by others
Modelling Career Trajectories of Cricket Players Using Gaussian Processes
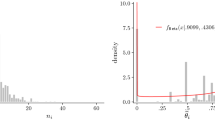
Hierarchical Bayes modelling of penalty conversion rates of Bundesliga players
Regimes in baseball players’ career data.
Avoid common mistakes on your manuscript.
1 Introduction
Sports analytics has experienced rapid growth both for teams and individual players in recent years. Statistics tools can be particularly useful to assess performances and define efficient gaming strategies. In this paper, we focus on basketball, a sports pioneer in using analytic tools, and data from the National Basketball Association (NBA).
As far as the literature on statistics in basketball is concerned, many of the proposed approaches can be traced back to the Bill James’s Sabermetrics approach in baseball (James, 1984 , 1987 ). The seminal work is, however, considered that of Oliver ( 2004 ): he identifies the so-called “Four Factors” that affect the game outcome with different weights and they are: the Effective Field Goal percentage, the Turnovers per Possession, the Offensive Rebounding percentage and the Free Throw Attempt rate, analysed later with more detail.
Kubatko et al. ( 2007 ) give a common starting point for research in basketball by providing a detailed overview and description of the main sources of data for basketball statistics; they also define the main basic variables used in what described as the “mainstream of basketball statistics”, firstly emerged outside the academy and due to Oliver ( 2004 ), Hollinger ( 2004 ), Hollinger and Hollinger ( 2005 ). In Nikolaidis ( 2015 ) one can find some indicative ideas for the statistical analysis of basketball data; he also shows that any basketball team can improve significantly its decision-making process if it relies on statistical support.
Yang et al. ( 2014 ) evaluate the efficiency of National Basketball Association (NBA) teams under a two-stage DEA (Data Envelopment Analysis) framework. Applying the additive efficiency approach, they decompose the overall team efficiency into first-stage wage efficiency and second-stage on-court efficiency, finding out the individual endogenous weights for each stage.
Other recent scientific proposals to model player and team performances make use of network models (Piette et al., 2011 ; Fewell et al., 2012 ; Skinner & Guy, 2015 ; Xin et al., 2017 ). In the class of models accounting for spatial features, Metulini et al. ( 2018 ) try to identify the pattern of surface area in basketball that improves team performance while Zuccolotto et al. ( 2019 ) propose a spatial statistical method based on classification trees to assess the scoring probability of teams and players in different areas of a court map.
Sandholtz et al. ( 2020 ) propose a new metric for spatial allocative efficiency: they estimate the player’s field goal percentage at every location in the offensive half court using a Bayesian hierarchical model. Then, comparing the estimated field goal percentage with the empirical field goal attempt rate, they identify the areas where the lineup exhibits inefficient shot allocation.
As far as the individual performances are concerned, Fearnhead and Taylor ( 2011 ) define a proper statistical methodology to estimating the ability of NBA players by comparing their team performance when they are on the court with respect to when they are off it. Lopez and Matthews ( 2015 ) propose an NCAA men’s basketball predictive model while is due to Cervone et al. ( 2016 ) a multiresolution stochastic process model for predicting basketball possession outcomes. Engelmann ( 2017 ) analyses the possession-based player performance too while Wu and Bornn ( 2018 ) model the offensive player movement in professional basketball.
Among the regression-based approach, Deshpande and Jensen ( 2016 ) propose a Bayesian linear regression model to estimate and evaluate the impact NBA players have on their teams’ chances of winning while Sill ( 2010 ) shows that the Adjusted Plus-Minus (often abbreviated APM, a basketball analytic used to predict the impact of an individual player on the scoring margin of a game after controlling for the strength of every teammate and every opponent during each minute he’s on the court) can be improved by using ridge regression.
Other alternatives based on Bayesian regression models are in Page et al. ( 2007 ) and Deshpande and Jensen ( 2016 ) while in the work of Casals and Martinez ( 2013 ), a mixed model is proposed and applied to model player performances.
In the field of neural networks, one can refer to the works of Loeffelholz et al. ( 2009 ), Blaikie et al. ( 2011 ), Wang and Zemel ( 2016 ), Shen et al. ( 2020 ), Babaee Khobdeh et al. ( 2021 ). For an extensive literature on the use of Statistics in basketball, an interesting review is provided by Terner and Franks ( 2021 ).
But, as stated by Baghal ( 2012 ), there is a lack of attention to applying multivariate analyses on basketball metrics even though it is well known that winning a game depends on a plurality of interrelated variables affecting the game outcome each with a different weight. To this aim, in the same work, Baghal ( 2012 ) uses a structural equation model to determine whether the Four Factors can be modelled as indicators of individual latent factors for the offensive and defensive quality. Team salaries are added to the model too in order to estimate the relationship between the cost and offensive and defensive quality.
With the same aim of enlarging the analysis by considering a multivariate approach, we propose the use of Bayesian networks (BNs) (Pearl, 1988 ), i.e. probabilistic expert systems able to identify complex dependence structures, such as that can exist among the set of performance indicators, and to carry out what-if analysis, at the same time.
Based on our knowledge, the use of BN in sports is rather limited to very few works (Razali et al., 2017 ; Constantinou et al., 2013 ), the latter using BNs with other scopes.
As deeply discussed later, the attractiveness of a BN mainly stems both from its pictorial representation of the dependency structure through the graph topology and from its inference engine; the latter makes a BN a decision support system since the reasoning (inference) is performed by setting some variables in known states and then updating the probabilities of the remaining variables conditioned by this evidence. The so-called belief updating can thus help to identify the most impactful variables and suggest the best strategies in different contexts.
From a methodological point of view, this proposal copes with the problem of handling non-Gaussian distributions, avoiding the bad practice of variables’ discretization. Therefore, a suitable extension to the Gaussian copula is considered to estimate the network structure under the above assumption, relaxing Guassianity. Specifically, the Non-Parametric BNs (NPBNs) proposed by Hanea et al. ( 2006 ) and Kurowicka and Cooke ( 2006 ) are applied in this paper.
Based on all these considerations, this work aims at introducing to the use of BNs in sports analytics; their versatility and their natural vocation to be used for prediction purposes could represent a valid instrument to predict team and player performance, to suggest strategies as well as a valid tool for decision-making in sports.
Specifically, we use BNs to identify the main determinants affecting the player winning percentage taking into account the most important performance indicators suggested in the literature. Moreover, we propose a model that combines information from data and experts which represents an important added value in this context.
Data used in this work have been downloaded from the NBA website and refer to 377 players who played at least 10 games and more than 6 min on the court in the regular season 2019–2020.
The paper is organized as follows. In Sect. 2 we provide a theoretical description of BNs and their structural learning methods followed by a brief discussion about the limits of the discretization; in Sect. 3 we introduce the class of Non-Parametric BNs followed by a little digression on the Nonparanormal estimator proposed by Liu et al. ( 2009 ) being the Gaussian copula a specific case of the class of Nonparanormal models. After the description of variables used in this study in Sect. 4 , we discuss the estimated model in Sect. 5 . The last section includes some comments and conclusions.
2 Basics on Bayesian networks
Bayesian Networks (Cowell et al., 1999 ; Pearl, 1988 ) are probabilistic graphical models used to deal with uncertainty in complex probabilistic domains. They bring together graph theory and probability theory to model multivariate dependence relationships among a large collection of random variables. It consists of a qualitative component, in the form of a directed acyclic graph (DAG), and of a quantitative component, in the form of conditional probabilities associated to each node of the DAG. Hence, they can be defined as multivariate statistical models satisfying sets of (conditional) independence statements encoded in a DAG.
More formally, given a random vector \(\mathbf {X}=\lbrace X_{1},\ldots , X_{k}\rbrace \) with distribution P , each node in the DAG corresponds to a random variable \(X_{i}\) , resulting in a graph with k vertices and a set of directed edges between pairs of nodes; hence, a DAG G is a pair \(G = (V, E)\) , where V is the set of vertices (or nodes) and E the set of directed edges. While each node corresponds to a random variable, a missing arrow between nodes imply (conditional) independence.
Specifically, the conditional independence properties of a joint distribution P can be read directly from the graph by applying the rules defined in the so-called d-separation criterion introduced by Pearl ( 1988 ) or the directed global Markov criterion due to Lauritzen et al. ( 1990 ), that is an equivalent criterion to that of the d-separation.
Based on Pearl’s criterion, given three disjoint subsets of nodes \(\mathbf {X}\) , \(\mathbf {Y}\) and \(\mathbf {Z}\) in a DAG G , \(\mathbf {Z}\) is said to d-separate \(\mathbf {X}\) from \(\mathbf {Y}\) , if for all paths between \(\mathbf {X}\) and \(\mathbf {Y}\) there is a vertex w such that either:
the connection is serial or diverging and \(w \in \mathbf {Z}\) (it is equivalent to say that w is instantiated);
the connection is converging, and neither w nor any of its descendants (i.e. the nodes that can be reached from w ) are in \(\mathbf {Z}\) .
A serial connection is of the type \(x \longrightarrow w \longrightarrow y\) (or \(x \longleftarrow w \longleftarrow y\) ), a diverging connection is \(x\longleftarrow w \longrightarrow y\) while a converging one is \(x\longrightarrow w \longleftarrow y\) . Clearly, if \(\mathbf {X}\) and \(\mathbf {Y}\) are not d-separated, they are d-connected.
In the graph in the Fig. 1 , for example, \(X_{3}\) d-separates \(X_{1}\) and \(X_{2}\) from \(X_{4}\) , implying the conditional independence among the same corresponding variables in P , i.e. \((X_{1},X_{2})\perp X_{4}| X_{3}\) .
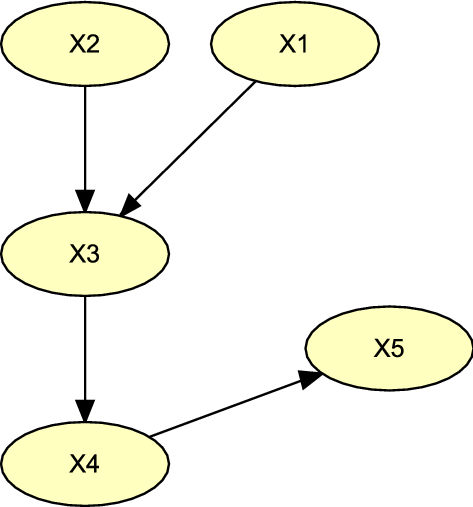
An example of DAG with 5 vertices
A directed edge from \(X_{i}\) to \(X_{j}\) states that \(X_{j}\) is influenced by \(X_{i}\) : using graph terminology, \(X_{j}\) is said the child of \(X_{i}\) that, in turn, is said the parent of \(X_{j}\) . The set of parents of \(X_{j}\) in the graph G is denoted by \(pa(X_{j})\) . In the DAG of Fig. 1 , for example, \(pa(X_{3})=\lbrace X_{1}, X_{2}\rbrace \) .
Moreover, with each variable \(X_{i}\) in the DAG, is associated a conditional probability \(Pr( X_{i}| pa(X_{i}))\) ; all set of these conditional probabilities constitute the quantitative part of the network.
Based on the local Markov property Footnote 1 and applying the chain rule, the joint distribution P over the random vector \(\mathbf {X}\) can be factorized as:
Besides its ability to decompose a multivariate distribution in a set of local computations, the power of Bayesian networks stems also from the availability of fast and efficient algorithms that perform probabilistic inference (Lauritzen & Spiegelhalter, 1988 ; Jensen et al., 1990 ). They update the marginal probability distributions of some variables when the information on one or more distributions of other variables is observed, thus allowing to carry out what-if analysis.
The structure of a BN ( i.e the DAG), as well as its parameters, can be defined by means of the expert knowledge or learned directly from data using structural learning techniques (Buntine, 1996 ; Neapolitan, 2003 ); in practice, the combination of both approaches is, however, recommended, introducing the prior information as constraints in the first learning phase. The next paragraph provides a little overview of the main methods proposed in the literature to learn the DAG structure.
2.1 Structural learning in BNs
The structural learning techniques for BNs fall into one of these two mainstream approaches: constraint-based (Spirtes et al., 1993 ; Cooper, 1997 ) and score-based (Heckerman, 1995 ; Cooper & Herskovits, 1992 ) algorithms.
A DAG learnt by means of a constraint-based algorithm entails all the marginal and conditional independence relationships inferred from independence tests: the most famous algorithm in this class is the PC algorithm due to Spirtes et al. ( 1993 ).
A DAG learnt by means of a score-based approach is the result of an optimization search strategy based on a scoring function. The graph structure is that which corresponds to the optimal score metric (the Penalised Likelihoods, AIC and BIC, are frequently used) among candidate structures, hence, identifying the better fitting network.
It must be noticed that hybrid methods have also been proposed in the literature, combining both constraint-based and score-based techniques. The two best-known members of this family are the Sparse Candidate algorithm (SC) by Friedman et al. ( 1999b ) and the Max-Min Hill-Climbing algorithm (MMHC) by Tsamardinos et al. ( 2006 ).
In this work, we apply a score-based algorithm known as the hill climbing algorithm, which is a heuristic optimization procedure given that the investigation of the space of all possible DAGs is infeasible in practice by growing exponentially with the number of nodes.
The hill climbing uses a greedy search strategy: generally starting from a network structure (more often the empty one), it iteratively performs small changes in the current candidate network by adding, deleting or reversing one arc at a time, stopping when any of changes improves the chosen score function.
In this study, specifically, the hill climbing with bootstrap resampling and model averaging is applied as proposed by Friedman et al. ( 1999a ) and implemented in the R package bnlearn . More robust to noise in the data, this technique consists of sampling data using bootstrap n times and applying the chosen learning algorithm to each bootstrap sample. Then, the resulting averaged network is a graph whose edges are those with a strength greater than a threshold value (see Scutari and Nagarajan ( 2011 ) for details about the computation of the strength significance threshold) where the strength of each arc is defined in terms of the number of times the arc appears over the n learned networks. Footnote 2
Despite the broad fields of applicability, in the parametric setting, most inference and structural learning methods work under the joint multinomial assumption for discrete data and the joint normality for continuous data. In the case of mixed variables, the conditional Gaussian distribution (CLGBNs; Lauritzen and Wermuth ( 1989 )) is assumed with the constraint that continuous nodes can not have discrete children.
Focusing on the continuous case, which is of interest in this work, in the Gaussian BNs (GBNs, Geiger and Heckerman ( 1994 )), a multivariate normal distribution is assumed for \(\mathbf {X}\) implying that each component \(X_{i}\) follows a univariate Gaussian distribution too. Therefore,
Hence, the parameters of the local distributions are the \(\beta \) coefficients of the linear regression model in ( 2 ). The influence of the parents on a child is translated in terms of these partial regression coefficients, meaning that their conditioning effect is given by the additive linear term in the mean without affecting the variance.
The main drawback associated with the use of the Gaussian BNs. relates to the joint normality which may be a very strong assumption, very often unrealistic; in the next paragraph, we shall examine in detail this issue.
2.2 The joint normality assumption and its limits
When the marginal distributions of the continuous variables of the domain are far from normal, the common practice consists in their discretization; very often, it results in loss of information and, in some cases, can destroy the true underlying dependence structure. To this aim, Nojavan et al. ( 2017 ) designed an experiment to evaluate the impact of the commonly used discretization methods for BNs showing that different methods result in differing future predictions. They state that “we would caution against decisions based on models for which the outputs vary by the choice (of discretization method) that does not have justifiable scientific basis” arguing that, in general, when possible, discretization of continuous variables should be avoided.
As highlighted by Rohmer ( 2020 ), a second drawback is computational since one cannot ignore that the size of conditional probability tables grows exponentially as the number of states of the involved nodes increases.
The class of Non-Parametric BNs (NPBNs) proposed in the literature by Kurowicka and Cooke ( 2006 ) and Hanea et al. ( 2006 ) and based on a D-vine copula approach can overcome these limits. Joint density is modelled using joint normal copula without requiring any parametric assumption on the marginals, as opposed to the parametric case, which justifies the use of the adjective “Non-Parametric”.
Other interesting works combining the distributional flexibility of pair-copula constructions (PCCs) with the parsimony of the conditional independence models associated with the DAG, can be found in Bauer et al. ( 2012 ), Hobæk Haff et al. ( 2016 ), Bauer and Czado ( 2016 ), Pircalabelu et al. ( 2017 ).
Is due to Harris and Drton ( 2013 ) the proposal of the Rank PC Algorithm for Nonparanormal Graphical Models; properly, they extend the well-known PC structural learning algorithm for Gaussian BNs (Spirtes et al., 1993 ) to the case of Gaussian copula.
Elidan ( 2010 ) proposes the Copula Bayesian Networks (CBNs): as in BNs, they make use of a graph to encode independencies but rely on local copula functions and an explicit globally shared parameterization of the univariate densities. Therefore, joint density is constructed via a composition of local copulas and marginal densities. A generalization of the CBNs to hybrid BNs can be found in Karra and Mili ( 2016 ).
In the class of the undirected graphical models, Liu et al. ( 2009 ) introduce the family of Nonparanormal distributions to relax the Gaussian assumption proposing two different methods of estimation for a nonparametric undirected graphical model.
In our work, under the assumption of a Gaussian Copula, we estimate a NPBN to identify the main determinants affecting the player winning percentage considering several important performance indicators in basketball. We use the theoretical framework of NPBNs proposed by Kurowicka and Cooke ( 2006 ) except for the structural learning phase, i.e the estimation of the DAG: to learn the graph, we apply the Hill Climbing algorithm to a suitable transformation of the original random variables in normal scores as suggested by Liu et al. ( 2009 ) and defined more in detail later.
In the next section, we introduce the theoretical framework of NPBNs.
3 Non-parametric Bayesian networks
The Non-Parametric Bayesian Networks, proposed in the literature by Hanea et al. ( 2006 ) and Kurowicka and Cooke ( 2006 ), are BNs whose dependence structure is modelled through a Gaussian Copula. The term “Non-Parametric” has been referred to the marginals since no distributional assumption is strictly required, i.e. the empirical distributions could be used.
For further interesting readings we refer to Hanea et al. ( 2010 ) and Kurowicka and Cooke ( 2010 ). Footnote 3
In a general setting, each node corresponds to a quantitative variable with an arbitrary invertible distribution function while the directed edges are associated with (conditional) parent–child rank correlations computed under the assumption of a joint normal copula. These (conditional) copulae, assigned to the arcs of the NPBN, depend on a (non-unique) ordering of the parent nodes. We highlight that the ordering of parent nodes can be assessed by the experts considering the decreasing strength of influence on the child, based on data availability or other qualitative considerations.
The main result arises from the following theorem (Hanea et al., 2006 ):
given k variables \(X_{1},\ldots ,X_{k}\) with invertible distribution functions \(F_{1},\ldots ,F_{k}\) ;
given the corresponding DAG with k nodes entailing all conditional independence relationships;
given a specification of the conditional rank correlations on the arcs of the NPBN;
given a copula realizing all correlations \([-1,1]\) satisfying the so-called zero-independence property Footnote 4 ;
the joint density over the k variables is (1) uniquely determined, (2) satisfies the BN factorization and (3) the conditional rank correlations are algebraically independent.
Moreover, the zero independence property implies that the conditional independence statements can be translated in the graph, assigning zero rank correlation to the corresponding arc, i.e. the arc can be removed.
The NPBNs specification also implies that they require only the definition of the one-dimensional marginal distributions and the arbitrary (conditional) rank correlations associated with the arcs of the BN to be quantified and well specified.
More important, the Gaussian copula has been chosen not only because it satisfies the zero independence property but also because it allows to perform conditioning analytically. This allows for extremely fast exact inference, therefore, speeding the probabilistic inference process in complex domains.
More specifically, let \(X_{1}\) and \(X_{2}\) be two continuous random variables, with invertible distribution functions \(F_{1}\) and \(F_{2}\) while \(Y_{1}\) and \(Y_{2}\) are the corresponding transformation to standard normal variables. The conditional distribution of \(X_{1}|X_{2}\) is obtained directly applying \(F_{1}^{-1}(\varPhi (Y_{1}|Y_{2}))\) .
This inference engine performing conditioning analytically is efficiently implemented in the UniNet software.
For sake of completeness, one needs to make a little digression on the validation procedure for NPBNs proposed by Hanea et al. ( 2006 ), Hanea et al. ( 2010 ) and Joe and Kurowicka ( 2011 ) to test the Gaussian Copula assumption.
To validate that the joint normal copula distribution is a valid model for the multivariate data, they defined a new measure of multivariate dependence equal to the determinant of the rank correlation matrix. Lying in [0, 1], it is equal to 1 if all variables are independent, to 0 in the case of multivariate linear dependence. All values in such interval take into account the different degree of dependence.
Two determinants have to be computed: the DER, the determinant of the empirical rank correlation matrix and the DNR, the determinant of the empirical normal rank correlation matrix computed on the transformed variables (that are standard normals) applying the inverse Pearson transformation such that \(\rho _{s}=\frac{6}{\pi } arcsin(\frac{\rho }{2})\) where \(\rho \) is the Pearson correlation coefficient while \(\rho _{s}\) the Spearman one.
This validation step is carried out by simulating the sampling distribution of DNR and checking whether DER is within the \(90\%\) central confidence band of DNR; if yes, the Gaussian Copula assumption cannot be rejected at the \(10\%\) significance level. This validation procedure is also used in this work.
The DAG selection in Uninet can be done by removing from the saturated graph those edges with a small conditional rank correlation Footnote 5 ; if the DNR falls within the 90% central confidence band of the determinant of the rank correlation matrix (computed under the assumption of joint normal copula) of the selected non-saturated BN, the model adequately approximates the complete graph and could be retained.
Since in the above model selection the arcs’ directions are assigned based on non-statistical considerations, such as subject-matter knowledge and time/logical ordering, in this paper, the structural learning phase is replaced by the following procedure: the hill climbing algorithm with bootstrap resampling and model averaging is applied to a suitable transformation in normal scores of the original variables as proposed by Liu et al. ( 2009 ) and defined in the next paragraph.
We point out that the parameters’ learning phase is based on the procedure described for the NPBNs, thus based on the conditional rank correlations.

3.1 The nonparanormal estimator proposed by Liu et al. ( 2009 )
Following Liu et al. ( 2009 ), the Nonparanormal models extend Gaussian models to semiparametric Gaussian copula models, with nonparametric marginals. Formally:
Definition 1
a random vector \(\mathbf {X}=(X_{1},\ldots ,X_{k})^{T}\) has a Nonparanormal distribution if there exist functions \({\lbrace {f_{j}\rbrace }}_{j=1}^{k}\) such that \(\mathbf {Z}\equiv f(\mathbf {X})\sim N({\varvec{\mu ,\Sigma }})\) , where \(f(\mathbf {X})=( f_1(X_1), \ldots , f_k(X_k))\) . Then, \(\mathbf {X} \sim NPN \sim N({\varvec{\mu ,\Sigma }},f)\) .
In another equivalent way, let \({\lbrace {f_{j}\rbrace }}_{j=1}^{k}\) be a set of monotone univariate functions and let \(\varvec{\Sigma }\) be a positive-definite correlation matrix. Then a k -dimensional random variable \(\mathbf {X}=(X_{1},\ldots ,X_{k})^{T}\) has a Nonparanormal distribution \(\mathbf {X} \sim NPN \sim N({\varvec{\mu ,\Sigma }},f)\) if \(f(\mathbf {X}):=( f_1(X_1), \ldots , f_k(X_k))\sim N_k(0,{\varvec{\Sigma }})\) .
Therefore, a random vector \(\mathbf {X}\) belongs to the Nonparanormal family if there exists a set of monotonically increasing transformations functions \({\lbrace {f_{j}\rbrace }}_{j=1}^{k}\) such that \(f(\mathbf {X})=( f_1(X_1), \ldots , f_k(X_k))^T\) is Gaussian.
Moreover, as a lemma, Liu et al. ( 2009 ) proved that the Nonparanormal distribution \(NPN({\varvec{\mu ,\Sigma }},f)\) is a Gaussian copula when the \(f_j\) ’s are monotone and differentiable.
A little digression is needed to remember that, in the classical Gaussian Graphical Models, one assumes that the observations have a multivariate Gaussian distribution with mean \(\varvec{\mu }\) , and covariance matrix \(\varvec{\Sigma }\) . More importantly, the conditional independence can be implied by the inverse covariance (concentration) matrix \(\varvec{\Omega }=\varvec{\Sigma }^{-1}\) . Indeed, if \(\Omega _{jk} = 0\) , then the i -th variable and j -th variable are conditional independent given all other variables. In other words, the graph G is encoded by the precision matrix.
The following Lemma (Liu et al., 2009 ) actually states that it also holds for the Nonparanormal graphical models.
If \(\mathbf {X} \sim NPN (\varvec{\mu },\varvec{\Sigma },f)\) is Nonparanormal and each \(f_{j}\) is differentiable, then \(X_{i} \perp \!\!\!\perp X_{j}|X_{\backslash \lbrace i, j\rbrace }\, \Leftrightarrow \Omega _{ij}=0, \quad where \quad \varvec{\Omega }=\varvec{\Sigma }^{-1}\) .
The important point to note is that, although the NPN identifies a more flexible and broader class of distributions than the parametric one, the independence relations among the variables are still encoded in the precision matrix \(\varvec{\Sigma }^{-1}\) .
For the scopes of Liu et al. ( 2009 ), the primary objective of the Nonparanormal model (NPN) is to estimate the underlying sample covariance matrix for a better recovery of the underlying undirected graph. Therefore, the random variable \(\mathbf {X}=(X_{1},\ldots ,X_{k})\) is replaced by the transformed random variable \(f(\mathbf {X})=( f_1(X_1), \ldots , f_k(X_k))\) assuming that \(f(\mathbf {X})\) is multivariate Gaussian.
Based on the above theoretical framework, the Nonparanormal estimator proposed by Liu et al. ( 2009 ) is based on a two-step procedure: (1) replace the observations, for each variable, by their respective normal scores, i.e. they estimate the transformation functions; (2) apply the graphical lasso to the transformed data to estimate the undirected graph.
In the step (1), as a Non-parametric estimator for the functions \(\lbrace {f_{j}\rbrace }\) , Liu et al. ( 2009 ) proposed the normal score-based Nonparanormal estimator, i.e. a truncated empirical distribution (the so called Winsorized estimator) whose computation is done by using the R package huge .
In detail, let \(x_{1},\ldots ,x_{n}\) be n observations of the X variable and let \(u_{1},\ldots ,u_{n}\) be the corresponding rank; denoting with \(\varPhi \) the normal CDF function, the truncated normal score \(f_{i}\) is estimated by:
where \(\hat{u_{i}}= \frac{u_{i}}{n+1}\) and \(\delta =\frac{1}{4n^{1/4} \sqrt{\pi log(n)}}\) .
For further mathematical details, see Liu et al. ( 2009 , 2012 ).
Following Liu et al. ( 2009 ), we replace the observations with their respective normal scores; in this way, we can apply to them the Hill climbing algorithm as for the Normal case.
In the next sections, we describe the variables used in the analysis and, then, apply the proposed learning strategy to them.
4 Data description
The variables used in this study were downloaded from the NBA website and listed in Table 1 . They refer to 377 players, all those who played at least 10 games and at least 6 min per game in the 2019–2020 regular season.
Thanks to Oliver’s studies (Oliver, 2004 ), in the Basketball, particular attention has been given to the so-called “Four Factors”: the effective Field Goal percentage, the Turnovers per Possession, the Offensive Rebounding percentage and the Free Throw Attempt rate.
These measures are considered good proxies for the overall offensive or defensive performance and are, in general, not all equivalent. Indeed, in his studies, Oliver assigned a weight to each factor so that the effective Field Goal percentage is the most important followed by the turnovers, the rebounding, and the free throws.
Because of their importance, they have included in our work. In detail, the effective field goal percentage (EFG) is the field goal percentage adjusting for made 3-point field goals, i.e. accounts for made three-pointers and serves to catch a player’s (or team’s) shooting efficiency from the field.
The turnovers per possession (To_Ratio) is the number of turnovers a player (or a team) averages per 100 possessions used.
The Offensive Rebounding percentage (OREB) is the percentage of available offensive rebounds a player obtains while on the floor; players, as well as teams, by obtaining an offensive rebound, can have one more attempt to make a basket that, in turn, can rise the winning probability.
The Free Throw Attempt rate (FTA_RATE) is the number of free throws made by a player in comparison to the number of field goal attempts; it expresses at the same time the player’s ability to get to the foul line and to make foul shots.
In addition, the Defensive Rebound percentage (DREB) is also considered, that is the percentage of available defensive rebounds a player obtains while on the floor.
We take into account the offensive and defensive ratings (OFFRTG and DEFRTG) too; they could be seen as measures of the player’s efficiency per possession. In particular, OFFRTG is the number of points per 100 possessions that the team scores while that individual player is on the court while DEFRTG is the number of points per 100 possessions that the team allows while that individual player is on the court.
We also include the Assist percentage (AST), the percentage of a team’s assists that a player has while on the court and the Assist Ratio (AST_RATIO), i.e. the number of assists a player averages per 100 possessions used.
Moreover, we take into account the age of the player (AGE), the Minutes played by a player (MIN) as well the number of possession per 48 min (PACE) and the usage percentage (USG), i.e. the percentage of team plays used by a player when they are on the floor. At last, the variable that refers to the wins over the played games (WIN) is also included.
5 The estimated network
Based on the previously described variables and 1.000 simulations, we cannot reject, at the 10 \(\%\) level, the hypothesis that data were generated from the joint normal copula since the determinant of the empirical rank correlation matrix (DER), being equal to 0.00179533, falls within the \(90\%\) central confidence interval for the determinant of the normal rank correlation matrix (DNR) [0.0014418, 0.0029218].
Under this assumption, the Bayesian network is estimated based on the model selection procedure described in the theoretical Sections, i.e., first of all, by transforming the variables into normal scores with Winsorized truncation and then running the Hill Climbing algorithm fixing the number of bootstrap samples at 500 and using the AIC criterion.
The threshold value is set to 0.70; in this way, only the arcs appearing at least the \(70\%\) of times are retained. This high value allows to define a parsimonious model avoiding that in the parameters’ learning phase too many arcs are associated with a conditional rank correlation close to 0. Footnote 6
We argue that the model selection procedure is mainly data-driven even if some arcs’ direction are forbidden based on logical constraints.
Given the DAG structure, the conditional correlations associated with the arcs are then estimated based on the Gaussian copula assumption through the Uninet software.
The estimated NPBN is shown in Fig. 2 a. The number associated with each arc is the conditional normal rank correlation, remembering that the rank correlation between the node and its first parent is unconditional, all the others are conditioned to the previous parents’ nodes. The ordering between parents is meaningful; hence, for each node, it has been set based on expert knowledge.
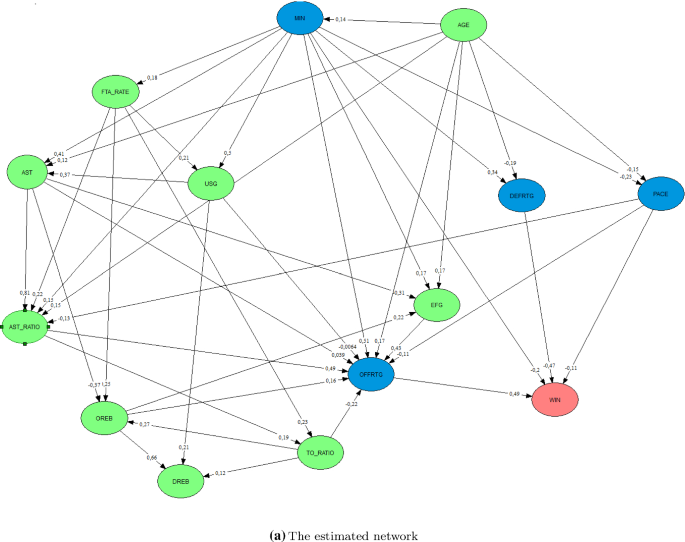
The estimated averaged BN under the assumption of Gaussian Copula, using the Uninet software representation
In the Fig. 2 b, the same network is reported whose nodes are replaced by monitors showing the associated empirical marginal distributions (with the indication of the mean and the standard deviation) estimated from data.
The estimated network describes the complexity of the multivariate dependence structure discovering the conditional independence relationships among the performance indicators.
In particular, the concept of the Markov blanket can be useful to identify the main variables that influence, for example, the player’s winning percentage. By definition, the Markov blanket of a node X , denoted by MB ( X ), includes all the parents, children and parents of children of X . It contains all information needed to infer a target variable X , making all variables not belonging to it redundant. Regarding the target node “WIN”, its Markov Blanket is composed of all nodes coloured blue in Fig. 2 .
In terms of conditional independence, this implies that the winning percentage and the green variables are conditionally independent, given the blue ones.
This means that the player winning percentage is completely determined by the player offensive and defensive ratings (OFFRTG and DEFRTG), with nearly the same rank correlation even if with opposite sign, Footnote 7 by the possession (PACE) and by the minutes staying on the floor (MIN). No other information is needed to predict winning percentage if those related to the above variables are available.
This is in accordance with the SEM model proposed by Baghal ( 2012 ), whose two latent factors affecting winning percentage were the offensive and defensive quality factors influenced, in turn, by the Four Factors. This is also verified in the BN since the Four Factors affect the winning percentage by means of indirect paths; indeed, unless FTA_RATE, all the other three are parent nodes of the OFFRTG. Moreover, from the estimated network, the important role of the effective field percentage is confirmed as the main factor influencing the offensive quality.
In general, all the discovered conditional relationships well match with those expected or defined in the literature.
In the following, in order to use the BN for inference purposes, we simulated the following scenario focusing on the importance of the individual Offensive and Defensive Rating, i.e. the player’s offensive production and the player’s defensive production. Taking into account an average player, who plays 26 min and is 27 years old, the winning percentage is 49.5% (see Fig. 3 a).
Suppose we have an OFFRTG of 10% higher than the mean, thus setting the value of the OFFRTG node at 121 (see Fig. 3 b), the mean value of the WIN node goes from 49.5 to \(69.2\%\) . Suppose now that we decrease the mean value of the DEFRTG node by 10%, fixing the value of the node at 99 (see Fig. 3 c), the mean value of the WIN node rises to \(61.7\%\) . It means that a 10% increase in offensive quality implies a 40% relative increase in the winning percentage while the same variation in terms of defensive quality implies a \(25\%\) relative increase in the winning percentage. Therefore, the quality of the player offensive production seems to have a stronger impact on the winning percentage.
By looking at the monitors, the effects on the whole distribution can be seen as they allow the visualization of both the original unconditional distribution (showed in grey) and the new conditional distribution (showed in black).
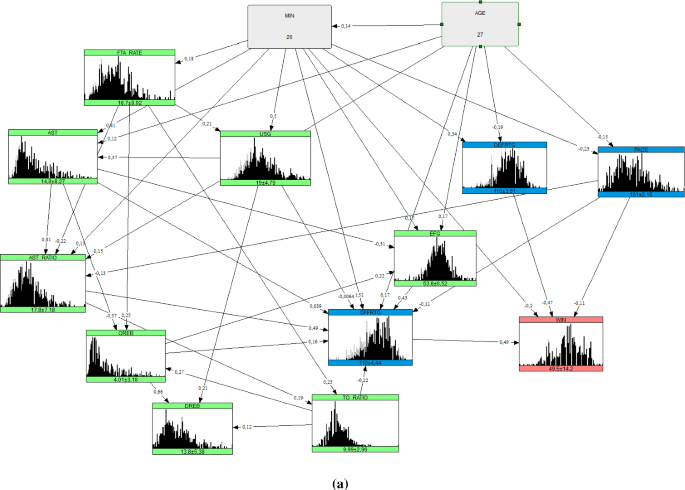
First simulated scenario
In the second scenario, we vary the mean value of the Four Factors by 10%, given the same average player. This combination leads to a relative increase in the winning percentage of \(7\%\) (see Fig. 4 compared to Fig. 3 a), all other factors being equal, the DEFRTG above all.
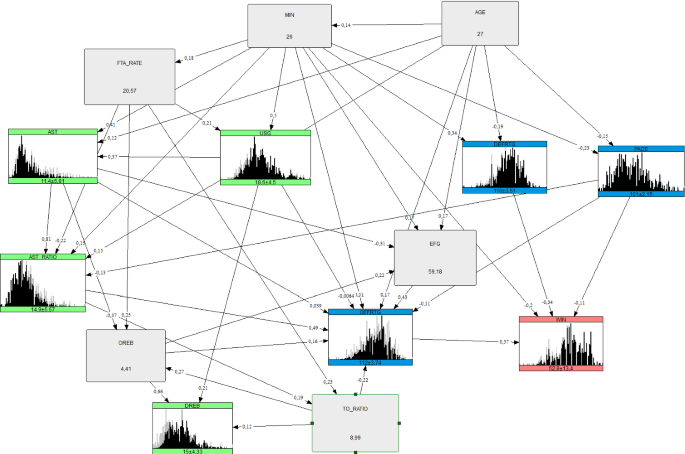
Second simulated scenario
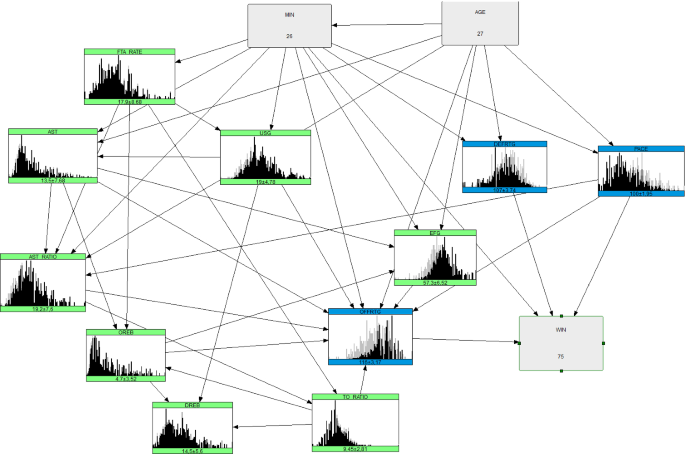
Third simulated scenario
More interesting could be the third simulated scenario in which we follow a bottom-up approach. This time, we fix the expected high level of winning percentage at \(75\%\) in order to know what are the minimum levels of the other factors that guarantee to reach the above percentage (see Fig. 5 compared with Fig. 3 a). Amongst them, the main evidence relates to the marked increase of Net Rating, i.e. the difference between OFFRTG and DEFRTG Obviously, the other indicators also change accordingly and can be investigated by looking at the change in their mean values (and in their distributions) compared to the baseline ones in Fig. 3 a.
In the fourth scenario we investigate the role of the PACE variable. Being equal the values of OFFRTG and DEFRTG (see Fig. 6 a), decreasing its value to 98 increases the mean value of the winning percentage from 50.8 to 52.7 (see Fig 6 b) while if it grows up to 105, the mean value of the winning percentage decreases to 48.6 (see Fig 6 c).
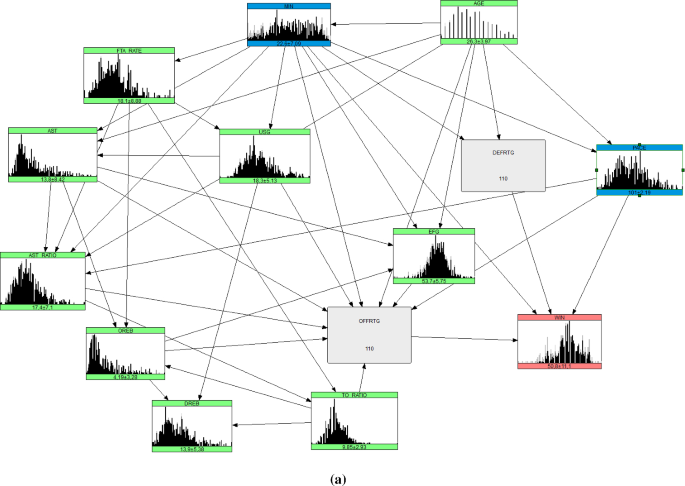
Fourth simulated scenario
At last, to investigate the influence of the player’s age, we simulate a fifth scenario. Being equal all other factors’ configuration, by increasing the age from 26 (Fig. 7 a) to 35 (Fig. 7 b), the mean value of the winning percentage goes from 53.7 to 59.4. This could be explained by the higher value of the OFFRTG which also corresponds to an increase in the AST_RATIO for the older player.
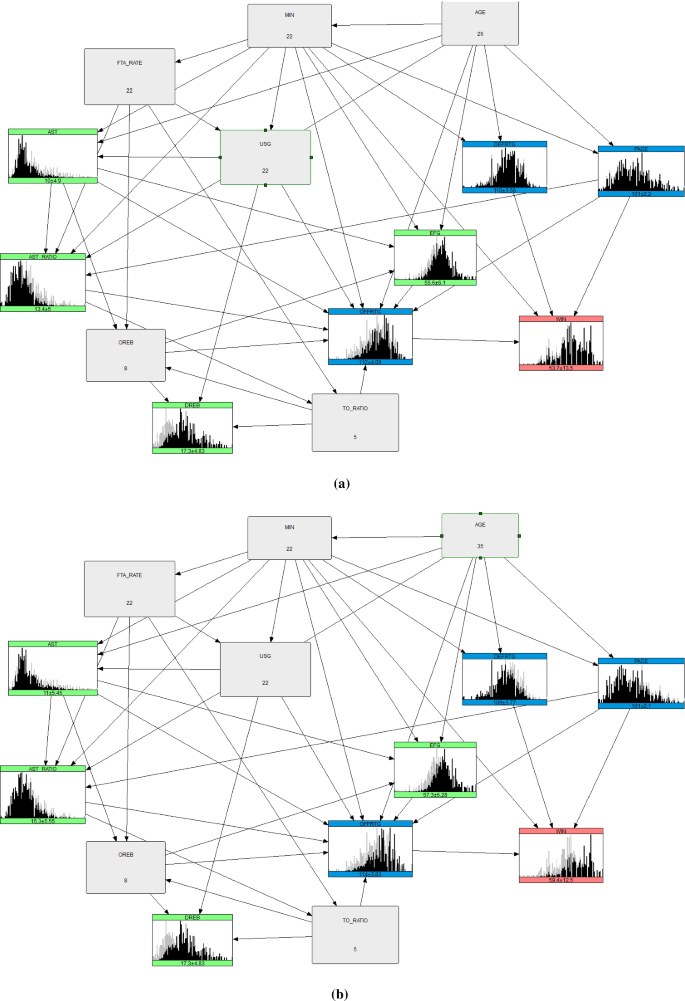
Fifth simulated scenario
By these simulations, we have shown the potential uses of BNs in this context, suggesting that any other scenario of interest could be investigated and deeply analyzed.
6 Conclusions
The aim of this work is to contribute to sports analytics by introducing a multivariate approach and, therefore, more complex statistical models, such as BNs, to deal with many performance indicators at the same time, studying their interrelationships rather than analysing them individually.
As shown through the what-if analysis, the links among different measures are evident. By means of the concept of Markov Blanket, we also identified the key determinants of the winning percentage. Moreover, the role of the Four Factors has been analysed: they affect the quality of the offensive and defensive production of a player that, in turn, directly affect the winning percentage. From a methodological point of view, the use of an approach overcoming the Gaussian assumption by means of a Gaussian Copula, is of interest in this field and, in general, in Statistics since it is well-known to allow great flexibility in the models. In the future, other statistical models based on BNs could be proposed that take into account the position of the player and many other features not yet included, such as the different seasons of the league.
The local Markov property states that each node \(X_{i}\) is conditionally independent of its non-descendants (e.g., nodes \(X_{j}\) for which there is no path from \(X_{i}\) to \(X_{j}\) ) given its parents.
The algorithm also estimates the strength of each arc’s directions conditional on the arc being present in the graph.
For interesting applications see Dalla Valle and Kenett ( 2015 ), Vitale et al. ( 2018 ), Marella et al. ( 2019 ).
If a chosen copula satisfies the so-called zero-independence property, a zero correlation implies the independent copula as well zero partial correlation implies conditional independence.
Another model selection procedure starts from a BN with no arcs, adding all the edges whose rank correlation (in the normal rank correlation matrix) is greater than the given threshold.
Moreover, this threshold provides almost the same model as the one based on the BIC criterion (with a lower threshold).
We argue that the rank correlation between OFFRTG and WIN is marginal, that between DEFRTG and WIN is conditional to OFFRTG. To this purpose, changing the ordering of these two parents nodes, we observed that the marginal rank correlation between DEFRTG and WIN is equal to \(-\,0.34\) while the conditional rank correlation between OFFRTG and WIN given DEFRTG, rises to 0.57.
Babaee Khobdeh, S., Yamaghani, M. R., & Khodaparast Sareshkeh, S. (2021). Clustering of basketball players using self-organizing map neural networks. Journal of Applied Research on Industrial Engineering, 8 (4), 412–428.
Google Scholar
Baghal, T. (2012). Are the “four factors” indicators of one factor? an application of structural equation modeling methodology to nba data in prediction of winning percentage. Journal of Quantitative Analysis in Sports , 8 (1).
Bauer, A., & Czado, C. (2016). Pair-copula Bayesian networks. Journal of Computational and Graphical Statistics, 25 (4), 1248–1271.
Article Google Scholar
Bauer, A., Czado, C., & Klein, T. (2012). Pair-copula constructions for non-Gaussian dag models. Canadian Journal of Statistics, 40 (1), 86–109.
Blaikie, A. D., Abud, G. J., David, J. A., Pasteur, R. D. (2011). “nfl & ncaa football prediction using artificial neural network”. In Proceedings of the midstates conference for undergraduate research in computer science and mathematics , Denison University, Granville, OH.
Buntine, W. (1996). A guide to the literature on learning probabilistic networks from data. IEEE Transactions on Knowledge and Data Engineering, 8 (2), 195–210.
Casals, M., & Martinez, A. J. (2013). Modelling player performance in basketball through mixed models. International Journal of Performance Analysis in Sport, 13 (1), 64–82.
Cervone, D., D’Amour, A., Bornn, L., & Goldsberry, K. (2016). A multiresolution stochastic process model for predicting basketball possession outcomes. Journal of the American Statistical Association, 111 (514), 585–599.
Constantinou, A. C., Fenton, N. E., & Neil, M. (2013). Profiting from an inefficient association football gambling market: Prediction, risk and uncertainty using Bayesian networks. Knowledge-Based Systems, 50, 60–86.
Cooper, G. F. (1997). A simple constraint-based algorithm for efficiently mining observational databases for causal relationships. Data Mining and Knowledge Discovery, 1 (2), 203–224.
Cooper, G. F., & Herskovits, E. (1992). A Bayesian method for the induction of probabilistic networks from data. Machine Learning, 9 (4), 309–347.
Cowell, R. G., Dawid, P., Lauritzen, S. L., & Spiegelhalter, D. J. (1999). Probabilistic networks and expert systems . Springer.
Dalla Valle, L., & Kenett, R. S. (2015). Official statistics data integration for enhanced information quality. Quality and Reliability Engineering International, 31 (7), 1281–1300. https://doi.org/10.1002/qre.1859 ( in Press ).
Deshpande, S. K., & Jensen, S. T. (2016). Estimating an nba player’s impact on his team’s chances of winning. Journal of Quantitative Analysis in Sports, 12 (2), 51–72.
Elidan, G. (2010). Copula Bayesian networks. Advances in Neural Information Processing Systems , 23 , 559–567.
Engelmann, J. (2017). Possession-based player performance analysis in basketball (adjusted+/–and related concepts). In Handbook of statistical methods and analyses in sports . Chapman and Hall (pp. 231–244).
Fearnhead, P., & Taylor, B. M. (2011). On estimating the ability of nba players. Journal of Quantitative analysis in sports , 7 (3)
Fewell, J. H., Armbruster, D., Ingraham, J., Petersen, A., & Waters, J. S. (2012). Basketball teams as strategic networks. PLoS ONE, 7 (11), e47445.
Friedman, N., Goldszmidt, M., & Wyner, A. (1999a). Data analysis with Bayesian networks: A bootstrap approach. In Proceedings of the 15th annual conference on uncertainty in artificial intelligence (pp. 196–201).
Friedman, N., Nachman, I., & Pe’er, D. (1999b). Learning Bayesian network structure from massive datasets: The “sparse candidate” algorithm. In M. Kaufmann (Ed.), Proceedings of 15th conference on uncertainty in artificial intelligence (pp. 206–221).
Geiger, D., & Heckerman, D. (1994). Learning gaussian networks. In Proceedings of the tenth international conference on uncertainty in artificial intelligence . Morgan Kaufmann Publishers Inc., San Francisco, CA, USA (pp. 235–243).
Hanea, A. M., Kurowicka, D., & Cooke, R. M. (2006). Hybrid method for quantifying and analyzing Bayesian belief nets. Quality and Reliability Engineering International, 22 (6), 613–729.
Hanea, A., Kurowicka, D., Cooke, R., & Ababei, D. (2010). Mining and visualising ordinal data with non-parametric continuous bbns. Computational Statistics and Data Analysis, 54 (3), 668–687.
Harris, N., & Drton, M. (2013). Pc algorithm for nonparanormal graphical models. Journal of Machine Learning Research , 14 (11), 3365–3383.
Heckerman, D. (1995). A tutorial on learning with Bayesian networks. In Technical report .
Hobæk Haff, I., Aas, K., Frigessi, A., & Lacal, V. (2016). Structure learning in Bayesian networks using regular vines. Computational Statistics and Data Analysis, 101 (C), 186–208.
Hollinger, J. (2004). Pro basketball forecast 2004–2005. Brassey’s.
Hollinger, J., & Hollinger, J. (2005). Pro basketball forecast, 2005–2006 . Potomac Books.
James, B. (1984). The bill James baseball abstract 1987 . Ballantine Books.
James, B. (1987). The bill James baseball abstract 1987 . Ballantine Books.
Jensen, F. V., Lauritzen, S. L., & Olesen, K. G. (1990). Bayesian updating in causal probabilistic networks by local computations. Computational Statistics Quarterly, 4, 269–282.
Joe, H., & Kurowicka, D. (2011). Dependence modeling: Vine copula handbook . World Scientific.
Karra, K., & Mili, L. (2016). Hybrid copula Bayesian networks. In Conference on probabilistic graphical models . PMLR (pp. 240–251).
Kubatko, J., Oliver, D., Pelton, K., & Rosenbaum, D. T. (2007). A starting point for analyzing basketball statistics. Journal of Quantitative Analysis in Sports , 3 (3), 1–24.
Kurowicka, D., & Cooke, R. (2006). Uncertainty analysis with high dimensional dependence modelling . Wiley.
Kurowicka, D., & Cooke, R. (2010). Vines and continuous non-parametric Bayesian belief nets with emphasis on model learning, vol Re-Thinking Risk Measurement and Reporting. Uncertainty, Bayesian Analysis and Expert Judgement, Risk Books, London, chap, 24, 295–329.
Lauritzen, S. L., Dawid, A. P., Larsen, B. N., & Leimer, H. G. (1990). Independence properties of directed Markov fields. Networks, 20 (5), 491–505.
Lauritzen, S. L., & Spiegelhalter, D. J. (1988). Local computations with probabilities on graphical structures and their application to expert systems. Journal of the Royal Statistical Society, Series B, 50 (2), 157–224.
Lauritzen, S. L., & Wermuth, N. (1989). Graphical models for associations between variables, some of which are qualitative and some quantitative. The annals of Statistics , 17 (1), 31–57.
Liu, H., Han, F., Yuan, M., Lafferty, J., Wasserman, L., et al. (2012). High-dimensional semiparametric gaussian copula graphical models. The Annals of Statistics, 40 (4), 2293–2326.
Liu, H., Lafferty, J., & Wasserman, L. (2009). The nonparanormal: Semiparametric estimation of high dimensional undirected graphs. Journal of Machine Learning Research , 10 (10), 2295–2328.
Loeffelholz, B., Bednar, E., & Bauer, K. W. (2009). Predicting nba games using neural networks. Journal of Quantitative Analysis in Sports , 5 (1), 1–17.
Lopez, M. J., & Matthews, G. J. (2015). Building an ncaa men’s basketball predictive model and quantifying its success. Journal of Quantitative Analysis in Sports, 11 (1), 5–12.
Marella, D., Vicard, P., Vitale, V., & Ababei, D. (2019). Measurement error correction by nonparametric Bayesian networks: Application and evaluation. In Statistical learning of complex data. CLADAG 2017. Studies in classification, data analysis, and knowledge organization (pp. 155–162). Springer.
Metulini, R., Manisera, M., & Zuccolotto, P. (2018). Modelling the dynamic pattern of surface area in basketball and its effects on team performance. Journal of Quantitative Analysis in Sports, 14 (3), 117–130.
Neapolitan, R. E. (2003). Learning Bayesian networks . Prentice-Hall Inc.
Nikolaidis, Y. (2015). Building a basketball game strategy through statistical analysis of data. Annals of Operations Research, 227 (1), 137–159.
Nojavan, F., Qian, S. S., & Stow, C. A. (2017). Comparative analysis of discretization methods in Bayesian networks. Environmental Modelling & Software, 87, 64–71.
Oliver, D. (2004). Basketball on paper: Rules and tools for performance analysis . Potomac Books, Inc.
Page, G. L., Fellingham, G. W., & Reese, C. S. (2007). Using box-scores to determine a position’s contribution to winning basketball games. Journal of Quantitative Analysis in Sports , 3 (4), 1–18.
Pearl, J. (1988). Probabilistic reasoning in intelligent systems: Networks of plausible inference . Morgan Kaufmann Publishers Inc.
Piette, J., Pham, L., & Anand, S. (2011). Evaluating basketball player performance via statistical network modeling. In The 5th MIT Sloan sports analytics conference .
Pircalabelu, E., Claeskens, G., & Gijbels, I. (2017). Copula directed acyclic graphs. Statistics and Computing, 27 (1), 55–78.
Razali, N., Mustapha, A., Yatim, F. A., & Ab Aziz, R. (2017). Predicting football matches results using Bayesian networks for English premier league (epl). In Iop conference series: Materials science and engineering (Vol. 226, p. 012099). IOP Publishing.
Rohmer, J. (2020). Uncertainties in conditional probability tables of discrete Bayesian belief networks: A comprehensive review. Engineering Applications of Artificial Intelligence, 88, 103384.
Sandholtz, N., Mortensen, J., & Bornn, L. (2020). Measuring spatial allocative efficiency in basketball. Journal of Quantitative Analysis in Sports, 16 (4), 271–289.
Scutari, M., & Nagarajan, R. (2011). On identifying significant edges in graphical models. In Proceedings of workshop on probabilistic problem solving in biomedicine (pp. 15–27). Springer.
Shen, J., Zhao, Y., Liu, J. K., & Wang, Y. (2020). Recognizing scoring in basketball game from AER sequence by spiking neural networks. In 2020 international joint conference on neural networks (IJCNN) (pp. 1–8). IEEE.
Sill J (2010) Improved nba adjusted+/-using regularization and out-of-sample testing. In Proceedings of the 2010 MIT Sloan sports analytics conference .
Skinner, B., & Guy, S. J. (2015). A method for using player tracking data in basketball to learn player skills and predict team performance. PLoS ONE, 10 (9), e0136393.
Spirtes, P., Glymour, C., & Scheines, R. (1993). Discovery algorithms for causally sufficient structures. In Causation, prediction, and search (pp. 103–162). Springer.
Terner, Z., & Franks, A. (2021). Modeling player and team performance in basketball. Annual Review of Statistics and Its Application, 8, 1–23.
Tsamardinos, I., Brown, L. E., & Aliferis, C. F. (2006). The max–min hill-climbing Bayesian network structure learning algorithm. Machine Learning, 65 (1), 31–78.
Vitale, V., Musella, F., Vicard, P., & Guizzi, V. (2018) Modelling an energy market with Bayesian networks for non-normal data. Computational Management Science , 1–18.
Wang, K. C., & Zemel, R. (2016). Classifying nba offensive plays using neural networks. In: Proceedings of MIT Sloan sports analytics conference (Vol. 4).
Wu, S., & Bornn, L. (2018). Modeling offensive player movement in professional basketball. The American Statistician, 72 (1), 72–79.
Xin, L., Zhu, M., & Chipman, H. (2017). A continuous-time stochastic block model for basketball networks. The Annals of Applied Statistics, 11 (2), 553–597.
Yang, C. H., Lin, H. Y., & Chen, C. P. (2014). Measuring the efficiency of nba teams: Additive efficiency decomposition in two-stage dea. Annals of Operations Research, 217 (1), 565–589.
Zuccolotto, P., Sandri, M., & Manisera, M. (2019). Spatial performance indicators and graphs in basketball. Social Indicators Research , 1–14.
Download references
Acknowledgements
We thanks Orso Maria Panaccione, semi-professional Basketball player, former champion of Italy with the youth team of Reyer Venezia, to have borrowed his experience and knowledge in this field.
Open access funding provided by Università degli Studi di Roma La Sapienza within the CRUI-CARE Agreement.
Author information
Authors and affiliations.
Department of Social Sciences and Economics, Sapienza University of Rome, P.za Aldo Moro 5, 00185, Rome, Italy
Pierpalo D’Urso & Vincenzina Vitale
Department of Political Sciences, Data Lab Luiss University, Viale Romania, 32, 00197, Rome, Italy
Livia De Giovanni
You can also search for this author in PubMed Google Scholar
Corresponding author
Correspondence to Vincenzina Vitale .
Additional information
Publisher's note.
Springer Nature remains neutral with regard to jurisdictional claims in published maps and institutional affiliations.
Rights and permissions
Open Access This article is licensed under a Creative Commons Attribution 4.0 International License, which permits use, sharing, adaptation, distribution and reproduction in any medium or format, as long as you give appropriate credit to the original author(s) and the source, provide a link to the Creative Commons licence, and indicate if changes were made. The images or other third party material in this article are included in the article’s Creative Commons licence, unless indicated otherwise in a credit line to the material. If material is not included in the article’s Creative Commons licence and your intended use is not permitted by statutory regulation or exceeds the permitted use, you will need to obtain permission directly from the copyright holder. To view a copy of this licence, visit http://creativecommons.org/licenses/by/4.0/ .
Reprints and permissions
About this article
D’Urso, P., De Giovanni, L. & Vitale, V. A Bayesian network to analyse basketball players’ performances: a multivariate copula-based approach. Ann Oper Res 325 , 419–440 (2023). https://doi.org/10.1007/s10479-022-04871-5
Download citation
Accepted : 27 June 2022
Published : 09 August 2022
Issue Date : June 2023
DOI : https://doi.org/10.1007/s10479-022-04871-5
Share this article
Anyone you share the following link with will be able to read this content:
Sorry, a shareable link is not currently available for this article.
Provided by the Springer Nature SharedIt content-sharing initiative
- Bayesian networks
- Gaussian copula
- Basketball data
- Four factors
- Multivariate dependence
- Find a journal
- Publish with us
- Track your research
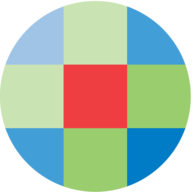
- Subscribe to journal Subscribe
- Get new issue alerts Get alerts
Secondary Logo
Journal logo.
Colleague's E-mail is Invalid
Your message has been successfully sent to your colleague.
Save my selection
Performance Changes in NBA Basketball Players Vary in Starters vs. Nonstarters Over a Competitive Season
Gonzalez, Adam M. 1 ; Hoffman, Jay R. 1 ; Rogowski, Joseph P. 2 ; Burgos, William 2 ; Manalo, Edwin 2 ; Weise, Keon 2 ; Fragala, Maren S. 1 ; Stout, Jeffrey R. 1
1 Sport and Exercise Science, University of Central Florida, Orlando, Florida
2 Orlando Magic Basketball Club, Orlando, Florida
Address correspondence to Dr. Jay R. Hoffman, [email protected] .
Gonzalez, AM, Hoffman, JR, Rogowski, JP, Burgos, W, Manalo, E, Weise, K, Fragala, MS, and Stout, JR. Performance changes in NBA basketball players vary in starters vs. nonstarters over a competitive season. J Strength Cond Res 27(3): 611–615, 2013—The purpose of this study was to compare starters (S) with nonstarters (NS), on their ability to maintain strength, power, and quickness during a competitive National Basketball Association (NBA) season. Twelve NBA players were assessed at the beginning and end of the competitive season. However, because of trades and injury, only 7 (S = 4, NS = 3) players (28.2 ± 3.4 years; 200.9 ± 9.4 cm; 104.7 ± 13.9 kg; 7.2 ± 1.9% body fat) participated in both testing sessions and underwent analysis. Anthropometric performance (repetitive vertical jump power [VJP], squat power [SQT power], and reaction time) and subjective feelings of energy, focus, alertness, and fatigue were recorded during each testing session. Results were interpreted using magnitude-based statistics to make inferences on true differences between starters and nonstarters using the unequal variances t -statistic. Starters played an average of 27.8 ± 6.9 minutes per game and nonstarters played an average of 11.3 ± 7.0 minutes per game. During the course of the season, changes in VJP indicated that starters were likely to increase VJP ([INCREMENT] = 77.3 ± 78.1 W) compared to nonstarters ([INCREMENT] = −160.0 ± 151.0 W). There also appeared to be a possible beneficial effect on maintaining reaction time in starters ([INCREMENT] = 0.005 ± 0.074 seconds) compared with nonstarters ([INCREMENT] = 0.047 ± 0.073 seconds). In addition, no clear differences in ΔSQT power were seen between starters ([INCREMENT] = 110.8 ± 141.4 W) and nonstarters ([INCREMENT] = 143.5 ± 24.7 W). Changes in subjective feelings of energy indicated that starters were very likely to maintain their energy over the course of a season. It also appeared possible that starters were able to have a more positive response to subjective measures of fatigue and alertness than nonstarters, with only trivial differences between starters and nonstarters in regards to maintaining focus. Results of this study suggest that NBA players may enhance lower-body power, repetitive jump ability, and reaction during a competitive season, which appear to be enhanced with the stimulus of playing time.
Introduction
A professional basketball season imposes a great amount of physiological stress on athletes. The typical competitive National Basketball Association (NBA) season consists of 82 regular season competitions over a span of 5.5 months (2–5 games per week). In addition, athletes also play a month of preseason games and practice and potentially up to 2 months of a postseason. On top of games, players are also required to participate in daily or twice-a-day practice sessions in preparation for competition. The training stress incurred over the long competitive NBA season may subject athletes to the risk of overtraining syndrome if appropriate adjustments are not made to training programs. Overtraining symptoms are often noticed when an athlete is unable to fully recover from the demands of a sport and often hinders the athlete's ability to maintain optimal performance ( 4,19,20 ). Assessing sport-specific performance characteristics of professional basketball players during the season may provide coaches and training staffs an ability to identify and make necessary adjustments to reduce the risk for overtraining.
There is limited research published pertaining to NBA athletes and their demanding season. However, there have been several studies examining the physiological changes during a season of competition in intercollegiate and European-level basketball players ( 2,8,16 ). Research has clearly indicated that basketball is predominantly an anaerobic sport ( 5,17 ), and several investigations on intercollegiate and European players have indicated that strength, power, agility, and speed can differentiate between different levels of competition or predict playing time ( 3,11,14 ). Several studies have indicated that performance measures can be maintained during a season of competition ( 9,10 ), whereas others have indicated that gains in strength can be achieved in previously untrained basketball players during a competitive season ( 8 ). However, none of these studies examined a season that was of similar length to that seen of an NBA team. Considering the length of the basketball season in the NBA, those athletes who are part of the regular rotation (starters) may experience a greater fatigue than those who are not playing consistently (nonstarters [NS]). Identification of players who experience decreases in performance may provide an opportunity for the coach to make appropriate adjustments to their training volume, training intensity, or even playing time to maximize potential as the season progresses toward the playoffs. Thus, the purpose of this study was to compare starters (S) with nonstarters, on the ability to maintain strength, power, and quickness during a competitive NBA season.
Experimental Approach to the Problem
Professional basketball players on an NBA team performed strength, power, and agility assessments during a competitive basketball season. During each testing period, players also completed questionnaires regarding their subjective feelings of energy, focus, fatigue, and alertness. These measures were part of a program designed to assess the physiological stresses of a competitive NBA season. Testing began before the start of the regular season and concluded a week before the end of the regular season. Testing occurred every month, but only the first and last testing periods were analyzed. All players participated in a regular weekly resistance training program during the competitive season. The frequency of resistance training was dependent upon travel schedule, but players did lift between 8–12 times per month. To determine the effect of playing time on performance changes, starters were compared with nonstarters.
Twelve players under contract to play for the NBA franchise Orlando Magic were assessed at the beginning of the competitive season. However, because of trades and injury, only 7 players (28.2 ± 3.4 years; 200.9 ± 9.4 cm; 104.7 ± 13.9 kg; 7.2 ± 1.9% body fat) participated in end of season testing. All analyses were performed with those players that participated in both pre- and postseason testing. All performance assessments were part of the athlete's normal training routine. Players gave their informed consent as part of their requirements as a team member, which is consistent with the policies of the University's Institutional Review Board for use of human subjects in research.
Performance Variables
All athletes performed anthropometric (height, body mass, and body composition), repetitive vertical jump power (VJP), squat power (SQT power), quickness, and reaction time. In addition, during each testing session, subjective feelings of energy, focus, alertness, and fatigue were recorded. The order of testing was consistent for all testing sessions. Upon reporting to the training facility, athletes dressed in their normal practice uniforms and underwent anthropometric assessments, completed the subjective questionnaires, and then performed the vertical jump test, reaction test and concluded with the squat test. Test-retest reliabilities for all assessments were R > 0.90. Total time played (total minutes), average minutes per game, games played, and games started were recorded from the team's official statistics.
Anthropometric Measures
Anthropometric assessments included height, body mass, and percent body fat (%BF). Body mass was measured to the nearest 0.1 kg. Body composition was assessed via skinfold analyses. Percent body fat was estimated via a 6-site skinfold test. The sites measured were the triceps, scapula, chest, iliac crest, anterior thigh, and abdomen, using methodology previously described ( 13 ). Body density was calculated using the equation of Jackson and Pollock ( 13 ), and %BF was calculated using the equation of Siri ( 18 ). The same research assistant performed all skinfold assessments.
Vertical Jump Power
Each player performed five consecutive countermovement jumps. During each jump, players stood with their hands on their waist at all times and were instructed to maximize the height of each jump while minimizing the contact time with the ground between jumps. Subjects wore a belt connected to a Tendo Power Output Unit (Tendo Sports Machines, Trencin, Slovak Republic). The velocity of each jump was calculated, and the mean power output (VJP) for each repetition was recorded and used for subsequent analysis.
Reaction Time
Lower-body reaction time was measured with a 20-second reaction test on the Quick Board (The Quick Board; LLC, Memphis, TN, USA) reaction timer. Subjects stood on a board of 5 circles, in a 2 × 1 × 2 pattern. Subjects straddled the middle circle and reacted to a visual stimulus located on a display box that depicted 1 of 5 potential lights that corresponded with the circles on the board. Upon activation of the light, the subject attempted to move the foot closest to the circle that corresponded to the visual stimulus. Upon a successful connection, the next stimulus would appear. The total number of successful attempts for the 20-second test and the average time between the activation of the light and the response to the corresponding circle was recorded.
Squat Power
Power output during the squat exercise was measured for each repetition with a Tendo Power Output Unit (Tendo Sports Machines,). The Tendo unit consists of a transducer attached to the end of the barbell, which measured linear displacement and time. Subsequently, bar velocity was calculated, and power was determined when barbell load was entered into the microcomputer. The mean power output (SQT power) was recorded for each repetition and used for subsequent analysis. Test-retest reliability for the Tendo unit in our laboratory has consistently shown R > 0.90. The squat test consisted of 2 sets of 5 repetitions at load approximating 80% of the athlete's 1 repetition maximum in the squat exercise.
Subjective Measures of Energy, Focus, Alertness, and Fatigue
Subjects were instructed to assess their subjective feelings of energy, focus, fatigue, and alertness using a 15-cm visual analog scale (VAS). The scale was anchored by the words “low” and “high” to represent extreme ratings where the greater measured value represented the greater feeling. Questions were structured as “My level of energy is,” ”My level of focus is,” “My level of alertness is,” and “My level of fatigue is.” The VAS was assessed at each test date, and subjects were asked to rate their feelings at that time by marking on the corresponding line. The validity and reliability of VAS in assessing fatigue and energy has been previously established ( 15 ).
Statistical Analyses
The effects of the NBA season were calculated as the change from pre- to postseason measurements among starters and nonstarters. Magnitude-based inferential analyses were used as an alternative to normal parametric statistics to account for the small sample size ( n = 7). Several studies have supported magnitude-based inferences as a complementary statistical tool to null hypothesis testing for reducing interpretation errors ( 1,12 ). The precision of the magnitude inference was set at 90% confidence limits, using a p value derived from an unpaired t -test, and the threshold values remained constant at ±0.2 for the small sample size. Inferences on true differences between starters and nonstarters were determined using the unequal variances t -statistic on a published spreadsheet ( 1 ). Inferences were calculated on whether the true population effect was substantially positive, negative, or trivial based on the range of the confidence interval relative to the value for the smallest clinical worthwhile effect. If the likely range substantially overlaps both positive and negative values, it is inferred that the outcome is unclear. The chance that the effect was positive or negative was evaluated by the following scale: <1%, almost certainly not; 1–5%, very unlikely; 5–25%, unlikely; 25–75%, possible; 75–95%, likely; 95–99% very likely; and >99% almost certain ( 12 ). Results were interpreted using magnitude-based statistics and reported as mean ± SD .
Starters ( n = 4) played an average of 1813 ± 639 total minutes (27.8 ± 6.9 minutes per game) and nonstarters ( n = 3) played an average of 543 ± 375 total minutes (11.3 ± 7.0 minutes per game) over the competitive season. Evaluation of magnitude inferences indicated a very likely difference in playing time between starters and nonstarters. Magnitude-based inferences on changes in performance and anthropometric measures are depicted in Table 1 . During the season, it appeared possible that starters maintained their body mass (0.5 ± 1.2 kg), whereas nonstarters lost their body mass (−0.9 ± 3.1 kg). Magnitude-based inferences on the Δchange in body composition indicated a possible beneficial effect of starters (0.025 ± 1.389%) on maintaining %BF compared with starters (0.833 ± 1.443%). The Δchanges in VJP indicated that starters were likely to increase VJP (77.3 ± 78.1 W) compared with nonstarters (−160.0 ± 151.0 W). Examining the individual responses of each player revealed that 3 of the 4 players who are starters increased VJP, whereas all 3 players who are nonstarters decreased VJP during the season (see Figure 1 ). Magnitude-based inferences indicated that a competitive NBA season had a possible beneficial effect on maintaining reaction time in starters (0.005 ± 0.074 seconds) compared with nonstarters (0.047 ± 0.073 seconds). In addition, there appeared to be no clear difference in ΔSQT power between starters (110.8 ± 141.4 W) and v (143.5 ± 24.7 W). Interestingly, all players (both starters and nonstarters) increased SQT power during the course of the basketball season.

Pre- to postseason changes in subjective measures of energy, fatigue, focus, and alertness can be observed in Figure 2 . Magnitude-based inferences on these changes can be seen in Table 2 . Changes in subjective feelings of energy indicated that the ability of starters to maintain their energy was very unlikely over the course of a season. It also appeared possible that starters were able to have a more positive response to subjective measures of fatigue and alertness than nonstarters. There appeared to be trivial differences between starters and nonstarters in regards to the ability to maintain focus.

The NBA season is a long arduous event that may pose a significant limitation to the athlete's ability to maintain performance levels. Although all players on the roster practice and travel, the athlete's who are part of the coaches regular playing rotation (get in the game on a consistent basis for extended period of time) may experience greater levels of fatigue and risk experiencing performance decrements if appropriate rest and recovery are not achieved ( 4,19 ). However, to our knowledge, this is the first study to attempt to quantify the magnitude of performance changes during an NBA basketball season. The results of this study indicated that starters were not only able to maintain their physical performance levels throughout the season but the greater playing time appeared to have provided a greater stimulus for enhancing VJP. In addition, starters appeared to maintain their body composition and reaction time better than nonstarters. Greater playing time also appeared to have positive effects on feelings of fatigue and alertness, whereas the only detriment associated with starters was a possible decrease in energy as the season progressed. The team was successful as reflected by a 52 to 30 regular season record and made the playoffs, but lost in the first round.
The measures used to assess performance changes in this study were consistent with what has been recommended for basketball players ( 5,9,17 ). Basketball is predominately an anaerobic sport ( 5,7,11,17 ), and studies have shown that lower-body strength and power performance are indicators of playing time in basketball players ( 11 ). As such, it appears imperative that strength and power measures should be maintained during a season. Interestingly, previous studies have demonstrated that this is attainable in National Collegiate Athletic Association Division I basketball players, as long as these players continue to lift through the season ( 8,10 ). Furthermore, strength gains can be achieved during the season, especially in novice or less experienced athletes ( 10 ). However, in contrast to the collegiate basketball season, the NBA season is nearly twice as long, possibly potentiating a greater cumulative fatigue in the NBA athlete. Despite this concern, the results of this study provide additional support for in-season strength and power improvements in NBA athletes. It appears that greater playing time acts as a stimulus for enhancing jump power performance. All players were required to perform a repetitive 5-jump test. This was intended to mimic the repetitive jumping experienced by players fighting for rebounds during a game. The greater jump power performance seen in starters compared with nonstarters suggests that the additional playing time, or the intensity of the jumps performed in an NBA game, provides a greater stimulus for eliciting improved repeated jump performance. This likely contributed to the greater reaction time observed in starters as well.
Other investigators examining European basketball athletes participating in longer competitive season than collegiate athletes, but less than NBA athletes, have reported that anabolic/catabolic balance is maintained during the season ( 16 ). This suggests that the physiological stress associated with that specific competitive scenario was well tolerated by those athletes. Although we did not measure hormonal changes, performance results of this study do suggest that the athletes were able to withstand the rigors of the NBA season. Still, fatigue can manifest itself in several different ways ( 4 ). Considering that energy levels were more affected in starters than nonstarters suggests that some negative results were seen toward the end of the season. Although speculative, we may have started to see the initial signs of an overtraining syndrome. Interestingly, fatigue appeared to manifest itself to a greater extent in nonstarters than starters. This may be related to dissatisfaction associated with insufficient playing time. A previous study has shown that the mood of professional basketball players, especially fatigue and tension, is influenced by personal factors related to the athlete's relationship to the coach or team administrators ( 6 ).
Practical Applications
The importance of monitoring elite basketball players' performance for overtraining is crucial not only to the athletes but also the success of teams. However, it is important to acknowledge that each athlete responds individually to the stresses of practice and games. Although the results of the team may be consistent, it is important for the strength and conditioning coach to examine individual player performance as well. When needed, specific adjustments to the athlete's daily routine (i.e., greater recovery, less time on the court) may prevent potential performance decrements that may not manifest as part of the team results. Results of this study suggest that NBA players may enhance lower-body power, repetitive jump ability, and reaction during a competitive season. This appears to be enhanced with the stimulus of competition.
- Cited Here |
- View Full Text | CrossRef
- View Full Text | PubMed | CrossRef
- PubMed | CrossRef
sport; overtraining; strength; reaction time; power
- + Favorites
- View in Gallery
Readers Of this Article Also Read
The relationship between running speed and measures of vertical jump in..., contribution of strength characteristics to change of direction and agility..., relationship between athletic performance tests and playing time in elite..., a comparison between the wingate anaerobic power test to both vertical jump and ..., physical fitness qualities of professional rugby league football players:....
Click through the PLOS taxonomy to find articles in your field.
For more information about PLOS Subject Areas, click here .
Loading metrics
Open Access
Peer-reviewed
Research Article
The effects of sport expertise and shot results on basketball players’ action anticipation
Roles Conceptualization, Funding acquisition, Investigation, Methodology, Validation, Writing – original draft
Affiliation Department of Sports, Physical Education College of Zhengzhou University, Zhengzhou, China
Roles Conceptualization, Data curation, Funding acquisition, Methodology, Project administration, Software, Validation, Visualization, Writing – review & editing
* E-mail: [email protected]
Affiliation Department of Social Sports, Physical Education College of Zhengzhou University, Zhengzhou, China

- Yawei Li,
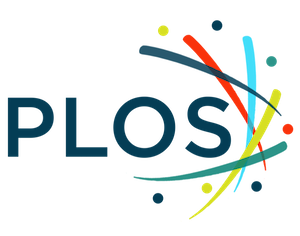
- Published: January 6, 2020
- https://doi.org/10.1371/journal.pone.0227521
- Reader Comments
The purpose of the present cross-sectional study was to clarify the effects of sport expertise and shot results on the action anticipation of basketball players. Eighty-eight male subjects participated in this study, namely, 30 collegiate basketball players, 28 recreational basketball players and 30 non-athletes. Each participant performed a shot anticipation task in which he watched the shooting phase, rising phase, high point and falling phase of a free throw and predicted the fate of the ball. The results showed that the collegiate players and recreational players demonstrated higher accuracy than the non-athletes for the falling phase but not for the other temporal conditions. Analysis of the shot results demonstrated that for made shots, the collegiate players and recreational players provided more accurate predictions than the non-athletes. These results suggested that the experienced players required a sufficient amount of information to be able to make accurate judgements and demonstrated that the experts’ judgement bias for made shots was independent of the temporal condition.
Citation: Li Y, Feng T (2020) The effects of sport expertise and shot results on basketball players’ action anticipation. PLoS ONE 15(1): e0227521. https://doi.org/10.1371/journal.pone.0227521
Editor: William Marshall Land, University of Texas at San Antonio, UNITED STATES
Received: July 28, 2019; Accepted: December 19, 2019; Published: January 6, 2020
Copyright: © 2020 Li, Feng. This is an open access article distributed under the terms of the Creative Commons Attribution License , which permits unrestricted use, distribution, and reproduction in any medium, provided the original author and source are credited.
Data Availability: All relevant data are within the paper and its Supporting Information files.
Funding: This work was supported by the National Social Science Found of China, grants No.19CTY013 awarded to Yawei Li and Tian Feng ( http://www.npopss-cn.gov.cn/ ). Humanities and social science research project of Henan Province Office of Education, grants No.2020-ZDJH-427 to Tian Feng ( http://www.haedu.gov.cn ). Research project of henan social science federation, grants SKL-2019-1955 to Yawei Li ( http://www.hnpopss.gov.cn ). The funders had no role in study design, data collection and analysis, decision to publish, or preparation of the manuscript.
Competing interests: The authors have declared that no competing interests exist.
Introduction
Action anticipation refers to the ability to predict the outcome of an event [ 1 ]. In contrast to racquet sports (such as tennis or badminton) in which a server usually decides the ball direction before starting his or her action, a basketball shooter can never be certain of the shot result until the final second. Thus, illustrating the development of shot anticipation ability in basketball is critical. Anticipating the outcome of an action, such as predicting whether a basketball will hit or miss the basket, directly affects the performance of an athlete. Accurate judgement helps a player plan his or her subsequent action (such as obtaining a rebound or playing defence) accordingly. Thus, the main goal of this study was to investigate the influence of sport expertise and shot results on the action anticipation of basketball players.
Studies have shown that sport expertise improves action anticipation ability. Aglioti and Cesari [ 2 ] asked basketball athletes, spectators (coaches or journalists) and novices to predict the success of free throws and found that the athletes responded earlier and more accurately than the other two groups of participants. Similarly, Uchida and Mizuguchi [ 3 ] compared the anticipation performance of experienced basketball players and novices observing a basketball free throw. The results demonstrated that the correct response rate among the experienced players was significantly greater than random chance (50%) and that of the novices, while the novices’ correct response rates were close to random chance. The distinct visual strategies used by athletes and novices may account for the observed differences. The researchers found that the basketball players focused more on body cues (i.e., the shooter’s knee, wrist and finger joint angles), while the novice group focused more on the ball [ 2 , 3 ].
Temporal information has been confirmed to play a necessary role in predicting outcomes, and the advantage of experts has been revealed in early time phases. Wu and Zeng [ 4 ] divided the time course of a free throw into three different phases with 11 sequential pictures as follows: the basketball leaving the model player’s hand (3 pictures), the basketball reaching the climax of its trajectory (6 pictures), and the basketball approaching the basket (9 pictures). The participants were required to predict the ball’s fate based on varying temporal information under different temporal conditions. The researchers found that when the participants observed 3 or 6 pictures, the athletes achieved higher prediction accuracy than the novices, indicating that experts utilize early cues better than novices when making predictions without complete information. Consistently, studies involving tennis or table tennis have shown that regional-level athletes use early valid information and achieve higher prediction accuracy than college-level and novice groups [ 5 , 6 ].
The result of a shot may be related to the anticipation advantage of elites, although most previous studies have concentrated only on the results of anticipation (i.e., correct or incorrect). The interaction between the actual results and anticipation yields the following four situations: true positive (correct prediction that a shot would be successful), false positive (incorrect prediction that a shot would be successful), true negative (correct prediction that a shot would be unsuccessful), and false negative (incorrect prediction that a shot was unsuccessful). Some studies have sought to determine the effect of shot results on anticipation at different visual angles. Cañal-Bruland and Balch [ 7 ] asked basketball players and observers to perform and observe shots. Both the players’ and observers’ vision was occluded at the time of the ball’s release, and they were asked to predict the ball’s fate. The authors found that the players were better at judging their own shots as “in” than judging others’ shots. To the best of our knowledge, only subjects who lack experience may have worse prediction accuracy than random chance (50%). Interestingly, a recent study by Maglott and Chiasson [ 8 ] found that for missed shots, collegiate shooters had worse prediction accuracy for their own shots than recreational shooters, and their prediction accuracy was significantly worse than random chance. Moreover, signal detection theory implies that collegiate players show a higher bias towards predicting that the shot result is “in”.
This judgement bias seems to be caused by the presence of the "regulatory fit" effect [ 9 ]. According to the theory of regulatory focus, two types of individual focus exist, namely, promotion focus and prevention focus [ 9 ]. Individuals with a promotion focus are eager to succeed and adopt more positive behavioural strategies, while individuals with a prevention focus are more inclined to avoid failure and adopt conservative behavioural strategies [ 10 ]. The effect emerges when the action strategy is consistent with one’s focus. Moreover, research conducted by Memmert and Unkelbach [ 11 ] shows that three-point shooting in basketball is more likely to be a task involving a promotion focus, and athletes exhibit better behavioural performance when matching with the action strategy. Therefore, predicting a shot as “in” is a positive strategy that may fit experts’ promotion focus, cause a regulatory fit, and thus improve their accuracy. In contrast, novices are more likely to have a prevention focus and exhibit superior performance when the ball is out.
Concerning the influence of the shot result, some important questions have been raised, but the answers remain unclear. Notably, experts and novices show different anticipation abilities in predicting the shot results of “in” and “out”. Will athletes exhibit an advantage in predicting successful shots when the shots are divided into varying time phases? Will the performance of athletes and novices change under different conditions of temporal occlusion? We sought to answer these questions by evaluating three groups of individuals with varying levels of basketball expertise (collegiate players, recreational players and non-athletes) and investigating the effect of shot results on action anticipation in different time phases. Both recreational players and non-athletes were included to determine whether a certain amount of expertise may confer a prediction advantage. We hypothesized that (1) compared to the non-athletes, the collegiate players and recreational players will show superior anticipation performance under the early temporal condition(s) and that the collegiate players will outperform the recreational players under the early temporal condition(s); (2) under the early temporal condition(s) of made shots, both collegiate players and recreational players will perform better than the non-athletes and that the collegiate players will be more accurate than the recreational players; and (3) for missed shots, the two experienced groups will provide more accurate predictions than the non-athletes under the early temporal conditions and that the collegiate players will outperform the recreational players.
Materials and methods
Ethical approval.
This study was carried out ethically and was approved by the Ethical Committee of Physical Education College of Zhengzhou University (No. 2019001). The individual in this manuscript has given written informed consent (as outlined in PLOS consent form) to publish these case details.
Participants
Eight-eight male subjects participated in this study, including 30 collegiate players (age: 21.53±0.97 years, height: 1.87±0.06 m, mass: 91.3±16.9 kg), 28 recreational players (age: 21.14±0.85 years, height: 1.79±0.04 m, mass: 77.6±14.7 kg) and 30 non-athletes (age: 21.00±1.15 years, height: 1.74±0.06 m, mass: 64.9±9.9 kg). The collegiate players participated on the basketball team of the Physical Education College of Zhengzhou University and practised 10.61±2.40 hours per week. The recreational players were collegiate track and field, boxing, rowing and judo athletes. These players participated in basketball games recreationally for 3.20±1.55 hours per week. The non-athletes were university students who had never participated in any sports training. The three groups did not vary in age, F (2, 83) = 2.23, p = 0.11, η p 2 = 0.51. Informed consent regarding the purpose and methods of the study and the obligations, responsibilities and rights of the subjects was obtained prior to the experiment.
Notably, we interrupted the complete sequence of each throw at one of four possible clip durations. We used continuous pictures rather than a video for the experimental stimuli since the selected pictures provided important information (for anticipation of the ball being “in” or “out”) that was more stable than information provided by a video. Two professional right-handed male athletes were required to shoot 60 free throws after warming up. All shots were recorded by a digital camera (Canon EOS 5D Mark IV, focal length of 3.5 mm). The camera height was 1.70 metres. Each shot was recorded at a speed of 6 frames per second from when the player held the ball to when the ball hit (or missed) the basket. Finally, at total of 40 free throws were selected according to the flight phase of the ball, including 20 made shots and 20 missed shots.
According to temporal information, the flight phase of a basketball and previous research results, each shot was divided into the following four temporal conditions: (1) the shooting phase, (2) the rising phase, (3) the high point, and (4) the falling phase. The final two pictures (pictures 11 and 12) were excluded from the experimental stimulus set to prevent the subjects from seeing the shot results. The exposure time for each picture was 167 ms, which was the same as the time required to take the pictures (6 pictures per second). Table 1 shows the characteristics of each temporal condition (the number of pictures, presentation time and ball position), and Fig 1 presents an example of the experimental stimuli.
- PPT PowerPoint slide
- PNG larger image
- TIFF original image
Continuous pictures of free-throw shooting as shown to the subjects. The pictures depict the time from when the player held the ball to the time when the ball hit (or missed) the basket. Each picture is presented for 167 ms, and the red line depicts when the shot stops under the 4 conditions of the temporal experimental stimuli.
https://doi.org/10.1371/journal.pone.0227521.g001
https://doi.org/10.1371/journal.pone.0227521.t001
The experiment was conducted in a quiet room at the Physical Education College of Zhengzhou University, and each participant was tested individually under the supervision of an experimenter. The participants completed a questionnaire regarding their individual information (i.e., sex, age, training year, height, mass, and training hours per week) and then participated in an action anticipation task. In the experiment, the participants were seated in front of a screen (23.8 inches) at a distance of 60 cm. A two-alternative forced choice task requiring the participants to predict the result of a free throw was conducted. The task began with a black cross-shaped fixation point on a white background displayed at the centre of the screen for 2,000 ms. Then, continuous pictures of a free throw were displayed, and each picture was presented for 167 ms at a resolution of 1024 x 682 pixels. After viewing all photos within each trial, the participants predicted the fate of the ball (made or missed by pressing the “F” key or the “J” key, respectively) quickly with the goal of being accurate. Responses submitted more than 3,000 ms after presentation of the stimulus were considered errors. The subsequent trial started immediately following completion of the previous trial. After reading the standardized task instructions, the subjects were asked to complete 15 practice trials with feedback (including 4, 6, 8 and 10 pictures). The pictures used in the practice trials were not used in the formal experiment. If a participant’s accuracy during the practice trials did not exceed 60%, i.e., the subject made more than 6 incorrect judgements, then he did not pass the practice session and was required to perform an additional practice session. Two collegiate players, two recreational players and five non-athletes required two practice sessions to meet the requirement. One collegiate player and one non-athlete required three practice sessions to meet the requirement. The experiment included 160 trials (40 shots×4 temporal conditions, 668 ms, 1002 ms, 1336 ms and 1670 ms), and the 40 shots included 20 successful shots and 20 missed shots under each temporal condition. All trials were divided into 8 blocks, and each block included 20 shots (both successful and missed) under one of the four conditions. The experiment was designed and displayed with E-prime 2.0 (Psychology Software Tools, Inc., Pennsylvania, USA). The response keys for the made and missed shots were counterbalanced across the subjects, and no feedback was provided during the experimental trials. A 30-second break was provided between each block. The entire experiment lasted approximately 50 min.
Statistical analyses
Assessing the reaction time of the participants is difficult because the participants were able to make their decisions before the option to press the key became available, and each prediction was made after the picture disappeared. Therefore, only the accuracy results were evaluated. The accuracies in the three groups under each temporal condition exhibited normal distributions ( z < .803, p >.539 in all instances). To test Hypotheses 1, 2 and 3 regarding the performance of the three groups under different temporal conditions and shot results, a mixed-design three-way analysis of variance (ANOVA) model with accuracy as the dependent variable, group (collegiate players, recreational players, and non-athletes) as the between-subjects factor, and temporal condition (668 ms, 1002 ms, 1336 ms and 1670 ms) and shot result (made shots and missed shots) as the within-subjects factors was calculated. Greenhouse-Geisser correction was applied when the assumption of sphericity was violated, and Bonferroni-corrected post hoc t -tests were used to identify the main effects and interactions.
The ANOVA of accuracy indicated significant main effects of the temporal condition, F (3, 255) = 65.20, p <0.001, η p 2 = 0.43, and shot result, F (1,85) = 59.15, p <0.001, η p 2 = 0.41, but not group, F (2, 85) = 1.40, p = 0.253, η p 2 = 0.03. The interaction between the temporal condition and group was significant, F (6, 255) = 5.69, p <0.001, η p 2 = 0.12. The post hoc tests of accuracy under the four temporal conditions per group revealed that under the 1670-ms condition, the collegiate players ( M = 0.71, SD = 0.11) showed better accuracy than the non-athletes ( M = 0.59, SD = 0.10, p <0.001), but the recreational players ( M = 0.65, SD = 0.11) did not differ from the other two groups of participants ( p >0.112 in all instances). No significant difference was found among the three groups under any other temporal conditions ( p >0.173 in all instances, see Fig 2 .).
https://doi.org/10.1371/journal.pone.0227521.g002
Additionally, a marginally significant interaction was found between the shot result and group, F (2, 85) = 3.02, p = 0.054, η p 2 = 0.07. Fig 3 shows the results of the post hoc tests. Under the made shot condition, the collegiate players were superior to the non-athletes (collegiate players: M = 0.69, SD = 0.13, non-athletes: M = 0.60, SD = 0.16, p <0.05), but the recreational players ( M = 0.64, SD = 0.11) did not differ from the other two groups of participants ( p >0.440 in all instances). For the missed shot condition, no significant differences between the groups were found (collegiate players: M = 0.42, SD = 0.12, recreational players: M = 0.44, SD = 0.11, non-athletes: M = 0.48, SD = 0.14, p >0.243 in all instances).
https://doi.org/10.1371/journal.pone.0227521.g003
The interaction between temporal condition and shot result was significant, F (3, 255) = 9.54, p <0.001, η p 2 = 0.10, and post hoc tests showed that all participants predicted the made shots ( M = 0.65, SD = 0.14) better than the missed shots ( M = 0.45, SD = 0.12) under every temporal condition ( p <0.001 in all instances). The three-way interaction was not significant, F (6, 255) = 0.94, p = 0.455, η p 2 = 0.02.
The present study is the first to clarify the effects of sport expertise and shot results on the action anticipation of basketball players. The performance of basketball players with varying skill levels (collegiate players and recreational players) and non-athletes was compared in an action anticipation task. Concerning the temporal conditions and shot results, the results demonstrated that the collegiate players and recreational players exhibited better accuracy than the non-athletes for the falling phase but not for the other temporal conditions. Additionally, all participants showed superior performance for made shots versus missed shots under every temporal condition. Furthermore, for the made shots, the collegiate players demonstrated higher prediction accuracy than the non-athletes. This study is the first to combine different temporal information with shot results to test the anticipation of basketball players with varying skill levels and non-athletes; thus, the results of this study may add important information to the present literature.
In the field of action anticipation, sport expertise and temporal information have attracted considerable interest. Studies focusing on the anticipation of basketball shooting found that when watching early (from 429 ms to 858 ms) and medium-term (from 426 ms to 852 ms) action phases during a shot, athletes provided more responses and predicted the shot result more accurately, but no difference between groups was found when they watched the late action phase of the shots [ 2 , 4 ]. However, the present study compared the accuracy of basketball players with varying levels of experience and non-athletes under different temporal conditions (0–668 ms, 0–1002 ms, 0–1336 ms and 0–1670 ms after the start of the shot) and demonstrated the advantage of collegiate players under the latest temporal condition (i.e., the phase when the ball approached the basket). This result contradicts the findings of previous studies and Hypothesis 1. This inconsistency may be caused by differences in experimental protocols. Our study required the subjects to make a two-alternative decision regarding whether a shot would be successful or missed, which is similar to actual situations in sports. Accordingly, as the information provided to a subject decreases, the accuracy should approach 50%. The studies conducted by Aglioti and Cesari [ 2 ] and Wu and Zeng [ 4 ] allowed the subjects to make an uncertain choice (i.e., “I do not know”), which may lead to different response strategies. In the early and medium-term action phases of the shots, the non-athletes were more inclined to choose uncertainty, but the athletes tended to make certain judgements and showed better performance. Furthermore, in the study conducted by Aglioti and Cesari [ 2 ], the non-athletes’ accuracy was approximately 80% when watching a shot for 1207 ms (similar to the high point phase in our study), while the present study found that the accuracies of the players and non-athletes were only 52% and 53%, respectively. The differences in the results may be explained by individual differences in the participants. Our results implied that the experienced players required a sufficient amount of information to be able to make accurate judgements.
In addition, other studies concerning anticipation in tennis or table tennis revealed that elite athletes were able to utilize early valid information to facilitate their prediction of the ball direction [ 5 , 6 ]. Zhao and Lu [ 5 ] found that when observing a video of table tennis serves with early kinematic cues and early flight cues, the regional-level group had higher prediction accuracy than the college-level and novice groups. To the best of our knowledge, a tennis server usually determines the ball’s direction before he or she serves. Thus, the server’s body posture presents some directional information that experienced players can use to make accurate judgements. In contrast to tennis serving, basketball shooting is a more uncertain task during which the shooter may think “I hope that the ball goes in, but I am not sure”; thus, early clues may not be present due to the uncertainty of the shot result.
According to previous studies, sport expertise seems to cause an anticipation bias towards made shots. Analysis of the shot results and groups revealed that basketball playing experience selectively influenced the collegiate players’ anticipation of a shot. For the made shots, the accuracy of the collegiate players was significantly higher than that of the non-athletes. Consistent with these results, Cañal-Bruland and Balch [ 7 ] and Maglott and Chiasson [ 8 ] demonstrated that players shooting a ball were better at judging their own shots as “in” than observers and recreational shooters. Although their experiment required the participants to shoot a ball themselves and then to make a judgement using both visual and proprioceptive information, our study required the participants to judge other people’s shots and showed that the collegiate players performed better than the other groups when presented with made shots, suggesting that experts can match the proprioception of their own shots to the visual information of others’ shots.
Additionally, regulatory focus theory suggests that performance improvement is affected by the "regulatory fit" [ 12 ]. Expert basketball players have higher motivation for achievement and must exert their best effort to win a match, conferring a self-regulatory focus, i.e., “focus on gains”, and a behavioural strategy, i.e., “search for a win”. Memmert and Unkelbach [ 11 ] found that the regulatory fit led to a broader scope of attention in a basketball shot task. When individuals with a “focus on gains” were required to predict whether positive results would occur (e.g., expert players were required to judge whether a shot would be a successful shot), the regulatory fit could help them improve their performance [ 13 ]. Regarding signal detection theory, the goal of “focus on gains” individuals is to ensure a “hit” and prevent a “miss” [ 12 ]. Consistent with this theory, the superior performance (e.g., more hits and fewer misses) of the collegiate players in the present study may be due to the effect of the regulatory fit. However, our results were not completely consistent with Hypothesis 2. The present study corroborated that the experts’ accuracy was higher than that of the other groups regardless of the temporal condition, indicating that the anticipation bias of experts existed in all phases of a shot.
By comparing the accuracy of the missed shots, the results showed an interesting trend such that the accuracy of the missed shot predictions gradually decreased as the skill level increased under all conditions. Similarly, Maglott and Chiasson [ 8 ] reported that for missed shots, collegiate shooters had poorer prediction accuracy regarding their own shots than recreational shooters, and their prediction accuracy was significantly worse than random chance. Adding a non-athlete group in the present study demonstrated the effect more specifically. The accuracy of the collegiate players and recreational players for missed shots was lower than that of the non-athletes. In addition, the differences between the accuracy in each group and random chance (50%) were compared according to a study conducted by Uchida and Mizuguchi [ 3 ]. The accuracy of the collegiate players and recreational players for missed shots was lower than random chance in the first three phases (collegiate players: t <-3.65, p <0.001 in all instances; recreational players: t <-2.26, p <0.05 in all instances), but no such difference was observed in the non-athlete group in any shot phase ( t >-1.13, p >0.12 in all instances). Consistent with this finding, studies have found that sometimes skilled athletes’ subconscious can inhibit objective visual information [ 14 ]. The subjective expectations of made shots in the present study appeared to represent this type of subconscious, which has also been called overconfidence or desirability bias [ 7 ]. Although some researchers may consider this bias a useful tool for experts to successfully shoot a free throw [ 15 ], it indeed impeded the experts’ prediction accuracy. At least, the poor performance of the experts reminded the collegiate players to not always think that a shot will be successful.
Some limitations existed in the present study. First, an anticipation error can be corrected by a rebound or reshot if the person who made the judgement realizes his or her mistake. Aglioti and Cesari [ 2 ] found that only experts have the ability to distinguish between error and correct judgements. However, the present study did not examine whether the participants could determine whether their judgements were correct or incorrect. Additionally, future studies should determine whether error realization can be affected by temporal information or shot results. Second, the present study required the subjects to respond after viewing 4, 6, 8 and 10 pictures. The experimental protocol was not totally consistent with an actual situation in the sport. In a basketball game, players can predict the ball’s fate at any time after the ball is released; thus, future designs of the anticipation task may use complete videos and allow subjects to make predictions at any time during the video. Finally, the recreational group in the present study included athletes from other sports, such as track and field, boxing, rowing and judo, and whether experience in other sports may transfer to action anticipation is unknown. Therefore, more studies are needed to compare players with different levels of basketball expertise only.
Conclusions
The present study considered the effects of sport expertise and shot results on the action anticipation of basketball players. When free throws were divided into four temporal conditions (i.e., the shooting phase, the rising phase, the high point and the falling phase), the collegiate players and recreational players demonstrated better accuracy than the non-athletes for the falling phase but not for the other temporal conditions. Concerning the accuracy of the shot results, the results showed that the collegiate players and recreational players had more accurate predictions than the non-athletes when judging made shots in the late time phase. Moreover, an analysis of the missed shots revealed worse performance by the collegiate players compared with the non-athletes in the middle time phase.
Supporting information
S1 file. raw data..
https://doi.org/10.1371/journal.pone.0227521.s001
S2 File. Ethical approval.
https://doi.org/10.1371/journal.pone.0227521.s002
- View Article
- PubMed/NCBI
- Google Scholar

An official website of the United States government
The .gov means it’s official. Federal government websites often end in .gov or .mil. Before sharing sensitive information, make sure you’re on a federal government site.
The site is secure. The https:// ensures that you are connecting to the official website and that any information you provide is encrypted and transmitted securely.
- Publications
- Account settings
Preview improvements coming to the PMC website in October 2024. Learn More or Try it out now .
- Advanced Search
- Journal List
- Front Psychol
- PMC10514490
Modeling the influence of basketball players’ offense roles on team performance
Ruobing chen.
1 China Basketball College, Beijing Sport University, Beijing, China
Mingxin Zhang
2 Athletic Performance College, Shanghai University of Sport, Shanghai, China
3 College of Physical Education, Dalian University, Dalian, China
Associated Data
The original contributions presented in the study are included in the article/ Supplementary material , further inquiries can be directed to the corresponding author.
This study aimed to (1) use the clustering method to build a classification model based on the play-type data of basketball players, to classify native and foreign players into different offensive roles; (2) use the clustered offensive role model to investigate how different offensive roles influence team performance. The sample was drawn from 20 teams spanning five seasons (2017–2021) in the Chinese Basketball Association, comprising 823 native and 228 foreign players. The clustering results obtained fourteen offensive roles for native players and five for foreign players. Subsequent analyses revealed that the offensive roles of two native player clusters, namely N6 Spot-up Wings who Attack (OR = 3.281, p < 0.05) and N13 Bigs who Cut to the Rim (OR = 4.272, p < 0.05), significantly influenced team performance. Conversely, no significant impact was observed for foreign players. The findings of this study offer novel insights into player dynamics and offer coaches a fresh perspective on team composition.
1. Introduction
The primary objective of sports performance analysis is to enhance the comprehension of sports and consequently guide decision-making processes for individuals aiming to improve their athletic performance ( O’donoghue, 2009 ). Moreover, the dynamic and intricate nature of sports requires a careful analysis through quantitative measurement techniques ( Hughes and Franks, 2007 ). In earlier years, the book ‘Moneyball’ discussed the use of advanced statistical analysis to evaluate baseball players’ performance and worth. This challenged established scouting strategies that typically depend on subjective judgment and outdated data metrics ( Lewis, 2004 ). Previous research has primarily concentrated on retrospective static analyses utilizing historical data and statistical methods to evaluate the performance of individual players or teams ( Gomez-Ruano et al., 2020 ). For instance, distinguishing between winning and losing teams ( Gómez et al., 2006 ; Çene, 2018 ), elucidating the significance of contributions made by starters and substitutes during games ( Gòmez et al., 2009 ), analyzing variances in physiological indicators among players in distinct positions ( Teramoto and Cross, 2018 ; Zarić et al., 2020 ), investigating gender disparities ( Gómez et al., 2013 ), and conducting cross-sectional comparisons of performance data across different leagues ( Sampaio et al., 2006 ).
However, over the past decade, researchers have increasingly adopted dynamic and intricate analysis techniques, especially in developing performance models ( Gomez-Ruano et al., 2020 ). These advanced techniques have proven valuable in processing and analyzing the wealth of available sports data, facilitating the construction of more accurate and reliable performance models ( Haghighat et al., 2013 ). For instance, decision trees have been utilized by researchers to identify patterns in player behavior and performance ( Willoughby and Kostuk, 2005 ; Morgan et al., 2013 ). Logistic regression has been employed to model the probability of specific game outcomes or player actions ( Zdravevski and Kulakov, 2009 ; Igiri and Nwachukwu, 2014 ). Support vector machines (SVM) have been used for tasks such as player classification or predicting player attributes ( Mustafa et al., 2017 ; Pai et al., 2017 ). Random forests have shown promise in capturing complex relationships within sports data and making accurate predictions ( Lin et al., 2014 ; Knoll and Stübinger, 2020 ). In addition to machine learning models, deep learning approaches, like neural networks, have gained popularity in sports analytics due to their ability to automatically learn and extract intricate patterns from large datasets ( Haghighat et al., 2013 ). These models have demonstrated success in tasks such as player trajectory prediction and play sequence analysis ( Kahn, 2003 ; Ivanković et al., 2010 ). Moreover, Ranking models, such as Elo Rating, TrueSkill scores, and PageRank algorithms, have been commonly used to assess player or team performance relative to their peers ( Barrow et al., 2013 ; Ibstedt et al., 2019 ; Kovalchik, 2020 ). Furthermore, sports research has extensively explored the application of both quantitative and qualitative models. Rule-based models offer interpretability and have been employed to derive decision-making strategies in various sports contexts ( Zdravevski and Kulakov, 2009 ; Trawiński, 2010 ). Time series models are often used to capture temporal dependencies in sports data, enabling trend analysis and forecasting player performance over time ( Yong et al., 2020 ). Clustering models have been utilized to group players or teams based on similar characteristics, facilitating player profiling and performance comparison ( Patel, 2017 ; Mateus et al., 2020 ). This combination of analytic techniques, encompassing both quantitative and qualitative variables, provides the foundation of data and information. Importantly, the synergy between these two types of data enables researchers to understand and predict athlete performance more comprehensively, offering valuable insight into the multifaceted drivers of sports performance ( O’Donoghue, 2014 ).
Assessing the critical performance indicators of players and teams is an equally important aspect of sports analysis ( Sarlis and Tjortjis, 2020 ). Previously, basketball data expert Dean Oliver presented a pioneering understanding of data analysis ( Oliver, 2004 ), prompting scholars to subsequently develop diverse metrics for evaluating the game from multiple viewpoints ( Cooper et al., 2009 ; Metulini and Gnecco, 2022 ). Given that the intricacy of match performance stems from the unique contextual setting in which each match takes place, situational elements like location, opponent, and stage of play exert behavioral influences on the performance of athletes and sports teams ( McGarry et al., 2013 ). Consequently, researchers must pay meticulous attention to the impact of contextual variables when constructing statistical models and investigating critical competition indicators within diverse competitive contexts and conditions, thereby establishing a standardized profile of technical performance during competitions ( Hughes et al., 2001 ; Zhang et al., 2017 ). Certain studies have consolidated performance indicators across various sports, encompassing game, tactical, technical, and biomechanical indicators on a macro level ( Hughes and Bartlett, 2002 ). Basketball scholars have refined these indicators by introducing Team Performance Indicators (TPI, Yu et al., 2008 ), which have been instrumental in investigating different facets of basketball.
Basketball is a team sport where success primarily hinges on integrating players with complementary skills ( Katzenbach and Smith, 2015 ). The study of team composition has consistently regarded player positions as a crucial factor, traditionally determined by height and weight considerations ( Deman et al., 2001 ). In particular, taller or more robust players are often stationed near the basket, while smaller players typically exhibit more significant activity on the perimeter ( Latin et al., 1994 ; Ostojic et al., 2006 ). The conventional approach categorizes players into five positions: Point Guard, Shooting Guard, Small Forward, Power Forward, and Center ( Trninić et al., 1999 ). However, as players develop physically and technically, they can assume multiple roles on the court. Consequently, the traditional method tends to oversimplify the skill sets of high-level players, resulting in suboptimal utilization of team resources ( Cheng, 2017 ). Scholars commonly employ cluster analysis in unsupervised learning to study player positions. Examples encompass hierarchical clustering ( Whitehead, 2017a ), topology ( Alagappan, 2012 ), K-means and model-based clustering ( Kalman and Bosch, 2020 ), and Gaussian mixture clustering ( Wang et al., 2022 ). Additionally, discriminant analysis (DAs) has demonstrated its suitability for predicting player positions ( Pion et al., 2018 ). However, the studies mentioned above primarily rely on basic statistics, such as box scores, which have inherent limitations ( Shea and Baker, 2013 ). For instance, high scoring can be attributed to a player’s shooting technique or the ability of teammates to create favorable shooting opportunities. Similarly, the frequency of defensive rebounds can be influenced by rebounding technique or the number of missed shots by opponents ( Remmert, 2003 ; Christmann et al., 2018 ). Certain researchers have investigated player behavior by considering interactive information, such as passing and pick-and-roll actions, consistently demonstrating that cooperative play between players yields superior results compared to individual actions ( Courel-Ibáñez et al., 2013 ; Gómez et al., 2015 ; Marmarinos et al., 2016 ). Consequently, the study of interactive information data can illuminate the technical and tactical aspects and is more favorable than traditional statistics for analyzing and evaluating athletic performance, as well as assisting coaches in decision-making ( Christmann et al., 2018 ).
Synergy, a sports data science company, offers play-type data and game footage access. They also provide advanced visualization tools for coaches and analysts to analyze opponents’ playing styles, monitor player development over time, and gain insights into player and team performance ( Božović, 2021 ; Fu and Stasko, 2022 ). There are 11 standardized play types in offense, such as spot-up, transition, isolation, off-screen, and so on ( Božović, 2021 ). Basketball offensive tactics are aimed at creating optimal shot selection within a 24-s period. The interplay of gaining separation from the defender(s) (e.g., to shoot or receive a pass) and gaining proximity (for a screen, handoff, cut, or drive) is characteristic of the basketball game ( Christmann et al., 2018 ). These patterns or actions are designed by the coaches, heavily practiced by the players, and then repeated in the game. Understanding how players and teams create successful scoring opportunities is, therefore, critical both practically and theoretically ( Matulaitis and Bietkis, 2021 ). For example, poor pick-and-roll use is recognized as a significant cause of team failure ( Vaquera et al., 2013 ); the most effective finishing moves in the Euroleague are isolation, pick-and-roll, spot-up, and cut ( Matulaitis and Bietkis, 2021 ), while the finishing actions that contribute the least to scoring are off-screen and hand-off ( Zukolo et al., 2019 ). The applicability of these data presents an excellent tool for detailed analysis and creating scout reports ( Božović, 2021 ). Scholars have utilized this data to investigate team styles, player roles, and the influence of various game types on team performance ( McBasketball, 2017 ; Božović, 2021 ), thereby providing basketball statisticians with a novel perspective for player observation. It is worth noting that player play type is a relatively understudied topic, with minimal research focused on it. Moreover, such studies are particularly scarce for high-level Asian leagues. Therefore, building on prior research, we postulate that classifying players’ play-type data will reveal contrasting offensive roles between native and foreign players, which, in turn, will have distinct impacts on team performance.
Given the above considerations, the aims of this study are as follows: (1) use the clustering method to build a classification model based on the play-type data of basketball players, to classify native and foreign players into different offensive roles; (2) use the clustered offensive role model to investigate how different offensive roles influence team performance.
2. Materials and methods
2.1. sample and variables.
The research data was obtained from the sports data website Synergy, 1 and we gained the license to use play-type related data and video. Table 1 presents the category of 11 play types in basketball, these indicators are considered standardized and cover all scoring attempts in the game of basketball, and they were able to translate the team’s tactical decisions into countable data ( McBasketball, 2017 ; Božović, 2021 ). Play-type data was collected for players from 20 teams during five CBA seasons, from 2017 to 2022, encompassing a total of 4,475 games including regular season, playoff qualifying rounds, and playoffs. The raw data consisted of 1,454 native players and 265 foreign players. To ensure the dependability of the clustering and subsequent analysis, players who had less than 3 % of the team’s total scoring attempts were excluded. Consequently, our final sample included 823 native players ( n = 823) and 228 foreign players ( n = 228).
K-means clustering-related play-type indicator.
Play-type | Description |
---|---|
Spot-up | When the possession-ending event is a catch-and-shoot or catch-and-drive play. |
Pick-and-roll ball-handler | A screen is set on the ball handler’s defender out on the perimeter. The offensive player can use the screen or go away from it, and as long as the play yields a possession-ending event, it is tagged as a pick and roll. |
Transition | When the possession-ending event comes before the defense sets following a possession change and a transition from one end of the court to the other. |
Cuts | An interior play where the finisher catches a pass while moving toward, parallel to, or slightly away from the basket. This will include the back screen, flash cuts, and times when the player is left open near the basket. |
Pick-and-roll man | When a screen is set for the ball handler, the setter receives the ball for a possession-ending event. This action can include: pick and rolls, pick and pops, and the screener slipping the pick. |
Post-up | When an offensive player receives the ball with their back to the basket and is less than 15 feet from the rim when the possession-ending event occurs. |
Off-screen | Identifies players coming off of screens (typically downs screens) going away from the basket toward the perimeter. This includes curl, fades, and flare. |
Putbacks | When the rebounder attempts to score before passing the ball or establishing themselves in another play type. |
Isolation | When the possession-ending event is created during a “one-on-one” match-up, the defender needs to be set and have all of his defensive options at the initiation of the play. |
Hand-off | The screen setter starts with the ball and hands the ball to a player cutting close by. This enables the player handing the ball off to effectively screen off a defender creating space for the player receiving the ball. |
Miscellaneous | When the action does not fit any of the other play types. This includes but is not limited to last-second full-court shots, fouls in the backcourt, and errant passes not out of a different play type. |
2.2. Statistical analysis
The initial step involved K-means clustering in categorizing native and foreign players into distinct groups based on their play type data ( Zhang et al., 2016 ; Patel, 2017 ). The elbow method was employed to determine the optimal number of clusters for the two groups. The clustering results were assessed using the silhouette coefficient ( Zhou and Gao, 2014 ), which ranges between-1 and 1. A higher value closer to 1 indicates superior clustering results, while a negative value indicates poor results. After the clustering results were obtained, the dataset was depersonalized using the t-SNE method, and the results were then visualized ( Van der Maaten and Hinton, 2008 ; Patel, 2017 ). The process above was implemented using the scikit-learn machine learning framework in Python.
In the second step, non-parametric tests were employed to examine the potential differences in the number of distinct offensive roles between teams that reached the semi-finals and those that did not. Effect sizes (ES) were calculated using Cohen’s d, and their interpretation followed the established criteria: 0.10 denoted a small effect, 0.30 indicated a medium effect, and 0.50 marked a large effect ( Volker, 2006 ). Additionally, we constructed a logistic regression model to investigate the impact of each offensive role on the team’s performance. The number of distinct offensive roles within the team served as the independent variable, while whether the team reached the semi-finals was the dependent variable. Based on their rankings in the season, all teams were categorized as either non-semi-finalists or semi-finalists (code: 0, 1). The threshold for determining statistical significance was set at α = 0.05. The above analyses were conducted using IBM SPSS Statistics for Mac, version 24.0 (Armonk, NY: IBM Corp.).
2.3. Reliability and validity of data
To establish the validity of the play type data obtained from Synergy, a random selection of five games per season was observed by three analysts with over 5 years of experience in basketball video analysis. This observed data was then combined with the information provided by Synergy to conduct an intra-group correlation analysis using the Two-Way Mixed-Effects Model ( Koo and Li, 2016 ), ultimately showing a high degree of consistency (ICC = 0.99). The local research institutional review board formally approved all procedures.
3.1. Players offensive role definition
Before clustering, the “elbow method” is employed to determine the optimal number of clusters. The primary concept behind this method involves identifying the inflection point where the sum of squared errors (SSE) within a cluster decline significantly. This inflection point represents the optimal number of clusters ( Zhang et al., 2016 ; Cui, 2020 ). According to Figure 1 , the optimal number of clusters for native players is three, while for foreign players, it is two.

The elbow method determines the optimal number of clusters for a player’s offensive role. Subfigure (A) demonstrates a declining inflection point in SSE for native players when the number of clusters is three; for foreign players, Subfigure (B) demonstrates a declining inflection point in SSE when the number of clusters is two.
Based on the play type percentages depicted in Figure 2 , the players can be macroscopically categorized as ball-handlers (C1, C4), wings (C0), and big men (C2, C3) ( Whitehead, 2017a ; Diambra, 2018 ). The specific definitions of these three types of roles are as follows:

Play-type percentage of different clusters (Macro offensive role).
Ball-Handlers: These roles have a high percentage of pick-and-roll ball-handlers and transition.
Wings: These roles have a high percentage of spot-up and transition.
Big men: These roles have a high percentage of cut, pick-and-roll man, post-up, and putbacks.
Nevertheless, in the context of basketball development, limiting the definition to only three roles deviates from the sport’s current developmental trend ( Bianchi et al., 2017 ; Whitehead, 2017a ). Consequently, we used K-means clustering to generate fourteen offensive roles (N0-N13) for native players and five (F0-F4) for foreign players. According to Figures 3 , ,4, 4 , the silhouette coefficient calculated for clustering native players is 0.20, indicating a moderate level of both cohesion and separation among the clusters. Put simply, the clusters formed for native players exhibit a certain level of internal similarity while maintaining reasonable distinction from each other. On the other hand, the silhouette coefficient for clustering foreign players is 0.24, indicating an enhanced clustering quality for this group. The clusters formed for foreign players demonstrate improved internal homogeneity and clearer boundaries between them ( Zhou and Gao, 2014 ).

Visualization of clustering results for native players. Subplot (A) shows that the silhouette coefficients of all clusters exceed the mean value. Subfigure (B) transforms eleven play-type variables into two dimensions by the t-SNE dimensionality reduction technique, and the numbers in Subfigure (B) correspond to the Cluster Label in Subplot (A) .

Visualization of clustering results for foreign players. Subplot (A) shows that the silhouette coefficients of all clusters exceed the mean value. Subfigure (B) transforms eleven play-type variables into two dimensions by the t-SNE dimensionality reduction technique, and the numbers in Subfigure (B) correspond to the Cluster Label in Subplot (A) .
Figure 5 displays the distribution of player clusters based on play type percentages. Similar to other studies that have analyzed player positions and roles using clustering methods, we identify players by observing how they are represented in different types of play data ( Lutz, 2012 ; Wang et al., 2022 ). The subsequent descriptions outline the offensive roles associated with each offensive role:

Play-type percentage of different clusters (Specific Offensive roles).
N0, “Primary Ball Handlers”: This group consists of native players with the highest percentage of pick-and-roll ball handlers.
N1, “Mobile Bigs who Cut”: This category comprises big men with exceptional mobility and a cutting style of scoring.
N2, “Impact Wings”: These players exhibit the highest percentage of participation in transition offenses.
N3, “Stationary Spot-up Wings”: This offensive role demonstrates the highest percentage of spot-up plays among all players.
N4, “Stationary Tall Wings”: These players exhibit a slightly lower percentage of spot-ups than N3 players but a higher percentage of roll-man plays and putbacks.
N5, “Ball Handlers who Share the Load”: This offensive role demonstrates a slightly lower percentage of pick-and-roll ball-handler than the N0 players.
N6, “Spot-up Wings who Attack”: These players possess a high spot-up percentage but primarily rely on cuts, roll-man, and putbacks to score.
N7, “Bigs who Roll to the Rim”: These players play a significant role in the pick-and-roll offense, frequently rolling to the basket to score after setting a screen.
N8, “Mobile Bigs who Post-up at the Rim”: These players exhibit the highest post-up percentage among native players and actively contribute to pick-and-roll plays and cuts for scoring opportunities.
N9, “Spot-up Wings who Transition”: This offensive role predominantly engages in spot-up and transition plays, while other play types are less prevalent.
N10, “Tall Wings who Pick-and-pop”: These players are characterized by a high spot-up and roll-man percentage, indicating their inclination toward catch-and-shoot plays following a pick.
N11, “Wings who Work Around Screen”: This offensive role excels at scoring by utilizing screens set by teammates on the court, leading to a high off-screen and handoff percentage.
N12, “Ball-handler with Off-ball Duties”: This offensive role exhibits a lower pick-and-roll ball-handler rate than N0 and N5 players but demonstrates a higher spot-up percentage.
N13, “Bigs who Cut to the Rim”: These players exhibit the highest percentage of cuts and putbacks while displaying the lowest percentage of spot-up plays.
F0, “Bigs with Skills Everywhere”: These skilled bigs employ a diverse array of plays to attempt scoring, including posting up inside, spotting up outside, and executing cuts or rolls to the basket.
F1, “Domineering Ball Handlers”: These players possess the highest percentage of pick-and-roll ball handlers and exhibit much isolation plays.
F2, “Wings who Initiate Offense”: These players demonstrate a particular percentage of pick-and-roll ball-handling and isolation plays. Despite not being the primary ball handlers on the court, they can initiate attacks with the ball.
F3, “Ball Stopping Mobile Bigs”: This offensive role is distinguished by a high percentage of spot-ups and isolation plays, often resulting in individual finishes for possessions.
F4, “Bigs with Skills in the Post”: This offensive role demonstrates the highest post-up percentage, indicating a greater emphasis on low-post offense.
3.2. Analysis of the influence of offensive roles on team performance
We initially examined if there existed differences in the diversity of offensive roles within the teams. Non-parametric test results ( Table 2 ) indicate that categories N10 Tall Wings who Pick-and-pop ( p < 0.01, ES = 0.84), N13 Bigs who Cut to the Rim ( p < 0.05, ES = 0.62), and F4 Bigs with Skills in the Post ( p < 0.05, ES = 0.67) exhibited significant disparities among the teams that advanced to the semi-finals and those that did not.
Non-parametric test of the number of different offensive roles.
Clusters | Whether to reach the semi-finals(M SD) | Mann–Whitney U | Mann–Whitney z | ES | ||
---|---|---|---|---|---|---|
No ( = 79) | Yes ( = 20) | |||||
N0 | 0.68 0.96 | 0.85 1.04 | 724 | −0.635 | 0.525 | 0.17 |
N1 | 0.51 0.64 | 0.60 0.68 | 731 | −0.582 | 0.56 | 0.14 |
N2 | 0.75 0.91 | 0.90 1.17 | 760 | −0.284 | 0.776 | 0.15 |
N3 | 0.51 0.71 | 0.60 0.75 | 735.5 | −0.544 | 0.586 | 0.13 |
N4 | 0.48 0.68 | 0.45 0.51 | 770.5 | −0.197 | 0.844 | 0.05 |
N5 | 1.00 0.97 | 0.80 0.62 | 733 | −0.528 | 0.597 | 0.25 |
N6 | 0.58 0.79 | 0.65 0.49 | 675 | −1.122 | 0.262 | 0.10 |
N7 | 0.35 0.64 | 0.40 0.50 | 712 | −0.853 | 0.394 | 0.08 |
N8 | 0.37 0.70 | 0.30 0.47 | 788 | −0.022 | 0.982 | 0.11 |
N9 | 0.87 0.76 | 1.00 0.80 | 727.5 | −0.59 | 0.555 | 0.16 |
N10 | 0.49 0.83 | 0.00 0.00 | 520 | −3.017 | 0.003 | 0.84 |
N11 | 0.49 0.68 | 0.20 0.41 | 616 | −1.794 | 0.073 | 0.53 |
N12 | 0.63 0.85 | 0.70 0.57 | 682.5 | −1.038 | 0.299 | 0.09 |
N13 | 0.54 0.68 | 1.05 0.95 | 552 | −2.286 | 0.022 | 0.62 |
F0 | 0.42 0.52 | 0.40 0.60 | 759 | −0.317 | 0.751 | 0.03 |
F1 | 0.51 0.60 | 0.55 0.69 | 776.5 | −0.134 | 0.894 | 0.07 |
F2 | 0.79 0.81 | 0.85 0.75 | 735 | −0.518 | 0.604 | 0.08 |
F3 | 0.34 0.53 | 0.30 0.57 | 745 | −0.49 | 0.624 | 0.08 |
F4 | 0.29 0.46 | 0.05 0.22 | 599.5 | −2.237 | 0.025 | 0.67 |
*Means p < 0.05, **means p < 0.001, ES, Effect Size.
Further, we constructed a logistic regression model using the number of offensive roles and the team’s performance. Table 3 displays the logistic regression model’s indicators. For native players, significantly positive effects were observed for N6 Spot-up Wings who Attack (OR = 3.281, p < 0.05) and N13 Bigs who Cut to the Rim (OR = 4.272, p < 0.05). The offensive roles of foreign players did not significantly impact the team’s quality.
Logistic regression analysis of players’ offensive roles.
Clusters | SE | score | Wald χ | Odds ratio | CI 95% | ||
---|---|---|---|---|---|---|---|
N0 | −0.123 | 0.403 | −0.305 | 0.093 | 0.761 | 0.884 | 0.401 ~ 1.950 |
N1 | 0.92 | 0.673 | 1.367 | 1.869 | 0.172 | 2.509 | 0.671 ~ 9.381 |
N2 | 0.248 | 0.423 | 0.586 | 0.343 | 0.558 | 1.281 | 0.559 ~ 2.934 |
N3 | −0.255 | 0.659 | −0.388 | 0.15 | 0.698 | 0.775 | 0.213 ~ 2.817 |
N4 | 0.399 | 0.721 | 0.553 | 0.306 | 0.58 | 1.49 | 0.363 ~ 6.125 |
N5 | −0.576 | 0.511 | −1.127 | 1.27 | 0.26 | 0.562 | 0.207 ~ 1.531 |
N6 | 1.188 | 0.586 | 2.029 | 4.116 | 0.042 | 3.281 | 1.041 ~ 10.342 |
N7 | 0.985 | 0.816 | 1.207 | 1.457 | 0.227 | 2.678 | 0.541 ~ 13.259 |
N8 | 1.026 | 0.969 | 1.058 | 1.12 | 0.29 | 2.789 | 0.417 ~ 18.635 |
N9 | 0.39 | 0.555 | 0.703 | 0.494 | 0.482 | 1.477 | 0.497 ~ 4.389 |
N10 | −1.675 | 0.579 | −1.957 | 3.571 | 0.997 | 0.383 | 0.027 ~ l.272 |
N11 | −1.416 | 0.945 | −1.498 | 2.244 | 0.134 | 0.243 | 0.038 ~ 1.547 |
N12 | −0.009 | 0.558 | −0.016 | 0.075 | 0.987 | 0.991 | 0.332 ~ 2.960 |
N13 | 1.452 | 0.629 | 2.31 | 5.335 | 0.021 | 4.272 | 1.246 ~ 14.649 |
F0 | −0.002 | 0.75 | −0.002 | 0.068 | 0.998 | 0.998 | 0.229 ~ 4.344 |
F1 | 0.669 | 0.782 | 0.855 | 0.731 | 0.393 | 1.952 | 0.421 ~ 9.044 |
F2 | 0.3 | 0.578 | 0.518 | 0.269 | 0.604 | 1.35 | 0.434 ~ 4.194 |
F3 | 0.315 | 0.803 | 0.393 | 0.154 | 0.695 | 1.371 | 0.284 ~ 6.610 |
F4 | −2.008 | 1.285 | −1.562 | 2.439 | 0.118 | 0.134 | 0.011 ~ 1.668 |
Nagelkerke R 2 = 0.468, β , Regression Coefficient; SE, Standard Error. *means p < 0.05.
4. Discussion
The aim of this study was to (1) use the clustering method to build a classification model based on the play-type data of basketball players, to classify native and foreign players into different offensive roles; (2) employ the classified offensive role model to examine the impact of diverse offensive roles on team performance.
Performance modeling is a valuable tool with diverse applications, including predicting game results ( Zdravevski and Kulakov, 2009 ), identifying tactical strategies ( Tian et al., 2019 ), aiding in player draft and recruitment processes ( Berri et al., 2011 ), and evaluating player and team performance ( Terner and Franks, 2021 ). Several studies have utilized this approach to categorize players’ positions and roles and forecast their performance ( Rangel et al., 2019 ; Wang et al., 2022 ). However, comparative studies between native and foreign players have rarely employed similar approaches. Most studies have consistently demonstrated the superior capabilities of foreign players compared to native players, suggesting their need for a more significant on-court role ( Guimarãe et al., 2018 ; Gasperi et al., 2020 ). Performance modeling helps mitigate these biases and preconceptions, enabling a fair and data-driven assessment of players from different backgrounds. This is especially crucial for foreign players, as their style and skill sets may differ from native players due to cultural, training, and developmental factors ( Ozmen, 2012 ). Nevertheless, it is essential to recognize the limitations of performance modeling. The reliability of predictions can be influenced by the quality, completeness, and accuracy of the data on which the models rely ( Fu and Stasko, 2022 ). Additionally, basketball is a complex and dynamic game influenced by various situational factors, teamwork, and coaching strategies, which the models may not fully capture. Caution should be exercised by coaches and researchers when interpreting and relying solely on predictions generated by performance modeling, acknowledging the critical role of human judgment and expertise in the decision-making process ( Remmert, 2003 ; Lamas et al., 2015 ).
To accurately reflect the contemporary dynamics of the sport, it is necessary to reassess the categorization of player roles in modern basketball. Conventional classifications, including point guard, shooting guard, small forward, power forward, and center, no longer encompass versatility and blurred positional boundaries ( Latin et al., 1994 ; Ostojic et al., 2006 ). Contemporary players possess a broader spectrum of skills and physical conditioning, allowing them to assume multiple roles on the court. This transformation is propelled by the emphasis on versatility, floor spacing, and the ability to create mismatches ( Bianchi et al., 2017 ; Whitehead, 2017b ). In their study of the Brazilian professional basketball league, Rangel et al. noted that versatility players showed an upward trend over nine seasons and presented a higher frequency at the power forward and point guard positions ( Rangel et al., 2019 ). Trninić et al. proposed multidimensional criteria for the various positions and roles of the game of basketball, and their findings showed that the ability to play multiple positions is crucial for players at positions 2 and 3, while players at position 1 are more expert players ( Trninić et al., 2000 ). In basketball, certain guards assume the role of ball handlers within their team, which forwards or centers may also take on. Conversely, some guards do not fulfill the ball handler role, and this principle can be extended to other positions on the court ( Sailofsky, 2018 ). This coincides with our findings: our clustering results yielded 8 categories of wings and 7 categories of big men, reflecting the importance of forward players playing multiple roles in the game; there were only 4 categories of ball-handlers, suggesting that combing the offense and creating scoring chances are their main responsibilities. In addition, the study by Rangel et al. noted that the roles of foreign players showed higher diversity ( Rangel et al., 2019 ). In our study, except for F1 players who are required to be the team’s primary ball-handler, the other types of players showed diversity in their scoring styles. Although the diversity of player roles is a growing trend in basketball, having more than three such “glue guys” proved to be detrimental to team performance in the study by Lutz (2012) . Therefore, coaches should try to balance the playing positions and tactical roles of players when constructing lineups.
Our study employed the elbow method to categorize players into three macro-level roles: ball handlers, wings, and big men. Previous studies have reported similar findings, corroborating and reinforcing our findings ( Bianchi et al., 2017 ; Whitehead, 2017a ). These findings offer valuable insights for analyzing player roles and conducting further research on the evolution of basketball tactics. Subsequent categorization revealed 14 offensive roles for native players and five for international players. Several previous studies have also created specific labels for basketball players’ roles in the game. For example, Alagappan et al. expanded the traditional five positions to 13 ( Alagappan, 2012 ), and Lutz et al. defined players into 10 different clusters ( Lutz, 2012 ). This categorization, however, fails to fully transcend the conventional positional constraints due to the substantial variation in statistical metrics across different player positions. For instance, guards tend to have higher counts of assists and successful three-point shots, whereas centers excel in rebounds ( Sampaio et al., 2006 ). There are also clusters of a few players, such as “one-of-a-kind,” which are individuals with outstanding talent and statistics, For example, James Harden and Russell Westbrook, who perform well in a variety of statistical data ( Alagappan, 2012 ; Lutz, 2012 ). While these players are not necessarily guaranteed to win ( Lutz, 2012 ), they are key players that every team pursues. Stated differently, these player clusters might lack representativeness as not all teams can boast of having such players. Consequently, the delineation of players according to roles, rather than rigid positions, facilitates adaptability and flexibility in response to team strategies and individual proficiencies ( Lorenzo et al., 2019 ; Zhang et al., 2019 ). In the course of a game, adept teams swiftly modify their successive offensive maneuvers in response to varying defensive shifts, whereas alterations in defensive strategies minimally affect offensive actions ( Christmann et al., 2018 ). Hence, characterizing player roles through play-type data will better mirror a player’s skill set and designated role.
An analysis of the offensive role composition in different teams demonstrated significant variations among three distinct offensive role categories, all falling within the classification of big players (N10, N13, F4). Prior studies on player classification consistently demonstrate the relatively low number of big men. Furthermore, the rarity of big men with specific skills like long-range shooting or proficiency in the low post hampers their availability on most teams ( Çene, 2018 ). Consequently, teams without such players must adjust their tactics or select alternative types of big men as substitutes ( Wang et al., 2022 ). The logistic regression results revealed a positive impact of the two offensive roles (N6 and N13) on a team’s advancement to the semi-finals. Consistent with previous studies on play types, successful teams were found to exhibit more cuts, low-post offense, spot-up shots, and free throws ( Zukolo et al., 2019 ; Matulaitis and Bietkis, 2021 ), which aligns with the characteristics of the two identified offensive roles. Furthermore, the significance of the off-ball movement was emphasized. Additionally, offensive rebounding emerged as a crucial aspect of performance. Offensive rebounding creates secondary scoring opportunities and diminishes the opponent’s chances of transitioning into offense ( Zukolo et al., 2019 ). Ball handlers play a pivotal role within the team, as over half of the set-offense are initiated through ball screens ( Gómez et al., 2015 ; Vaquera et al., 2016 ). However, research has indicated that lineups with more than two high-usage ball handlers tend to underperform, decreasing team efficiency ( Kalman and Bosch, 2020 ; Wang et al., 2022 ). The same finding was also seen in the study by Lutz et al. who noted that ball handlers have a negative impact on a team’s point differential ( Lutz, 2012 ). Transition offense, while efficient, does not exhibit significant correlations with game outcomes, likely due to its relatively small proportion within overall gameplay ( Selmanovi, 2015 ; Suárez-Cadenas et al., 2016 ; Matulaitis and Bietkis, 2021 ). Consequently, players specializing solely in transition offense and spot-up shooting are considered highly interchangeable. Moreover, players proficient in scoring from screens (N11) heavily rely on the team’s ability to create space for them and face heightened defensive pressure ( McIntyre et al., 2016 ). Notably, off-ball screens and hand-offs contribute the least to scoring; losing teams tend to have more attacks ( Suárez-Cadenas et al., 2016 ; Zukolo et al., 2019 ).
Finally, our study indicates that the offensive role of foreign players has no substantial impact on team performance. These findings align with prior research that found no significant difference in efficiency between foreign players of top teams and average (non-top) teams ( Ozmen, 2012 ; Wang et al., 2022 ). Conversely, native players of top teams are more efficient than native players of teams outside the top tier. Native players in the CBA league exhibit distinct offensive characteristics, with spot-up, transition, and cutting actions comprising a significant portion of their gameplay. These offensive strategies prioritize teamwork and have been proven effective in several studies ( Christmann et al., 2018 ; Zukolo et al., 2019 ; Matulaitis and Bietkis, 2021 ). However, they rely on teammate support for execution, making these offensive roles often perceived as scoring beneficiaries ( Whitehead, 2017b ; Zukolo et al., 2019 ). In contrast, pick-and-roll ball handlers, isolation, and post-ups were more prevalent among foreign players. While some studies disagree on the efficiency of these playing styles ( Selmanovi, 2015 ; Christmann et al., 2018 ; Zukolo et al., 2019 ), they tend to emphasize individual ability. Consequently, these offensive roles are regarded as scoring creators ( Whitehead, 2017b ; Zukolo et al., 2019 ), particularly in teams lacking strong scoring capabilities, where the role of these players becomes even more vital ( Arcidiacono et al., 2017 ). Conversely, foreign players have demonstrated superior talent to domestic players in the league ( Ozmen, 2012 ; Özmen, 2019 ; Gasperi et al., 2020 ). Due to the distinct playing time regulations concerning foreign players in the CBA league, teams are constrained to field a single foreign player during each quarter. This stipulation has led most teams to prioritize the scoring ability of these foreign recruits. Lutz et al. pointed out in their study that some player classifications such as aggressive bigs, big bodies, and perimeter scorers, have a negative impact on the game score difference ( Lutz, 2012 ). Also, it was mentioned in their study that big players are generally unfavorable to the outcome of the game ( Lutz, 2012 ), which diverges from our findings. Our explanation is that similar to the roles of native and foreign players in other leagues, native players play a secondary role in their team’s offense, relying on their teammates to create chances while focusing more on defense and other secondary responsibilities and playing a lesser role in the creative aspects of the game ( Guimarãe et al., 2018 ; Özmen, 2019 ); while some studies have also pointed out that big players who focus on defense have a positive impact on the outcome of the game ( Lutz, 2012 ; Wang et al., 2022 ), which support our research findings. These insights prompt coaches to recognize that with all teams having the funds to recruit talented foreign players, it is vital to develop native players, especially big men with a great sense of teamwork and defensive ability.
5. Limitations and future directions
This study has certain limitations. Firstly, similar to other studies employing clustering for player role classification, the final clustering results are inherently subjective, leading to the possibility of misclassifying players into different roles, which is an unavoidable issue in clustering studies. Secondly, basketball is a multifaceted game with various dimensions, where player roles can significantly fluctuate depending on team strategies, game situations, and opponent circumstances. This study solely analyzed players’ offensive types while neglecting relevant defensive metrics. Some players renowned for their defensive prowess may have been overlooked, potentially oversimplifying players’ roles and disregarding the diversity of their on-court contributions. Lastly, when studying the impact of offensive roles on team performance, we solely employed the number of players as the independent variable due to sample size limitations; other variables were not considered, such as players’ playing time and efficiency values across different play types, which constrained our ability to investigate further the influence of players’ roles on team performance.
Regarding future research directions, several areas warrant exploration. First, player roles evolve in response to coaching decisions, formation adjustments, and player development. Investigating the patterns and trends of these role changes is one of the areas we intend to explore. Second, incorporating a larger sample size and additional influential variables concerning the impact of player roles on team performance would enhance model stability, thereby facilitating the further investigation of the matter. Lastly, future research should integrate advanced machine learning techniques like deep or reinforcement learning to capture player roles’ intricate relationships and temporal dynamics. These approaches are adept at handling large-scale data, identifying complex patterns and dependencies, and yielding a more nuanced comprehension of offensive roles.
6. Conclusion
In this study, we utilize play-type data with interaction information to categorize native and foreign players in the CBA league into 14 and five distinct offensive roles, respectively, employing a clustering approach. These results provide a unique perspective for understanding and observing the player’s role. Further research found that big players made a difference in team composition. The offensive roles of two categories of native players, whose attributes emphasized both teamwork and off-ball movement, showed a significant influence on team performance. In contrast, no such impact was found for foreign players. Consequently, basketball coaches and managers should consider optimizing the composition of individual player clusters during the team-building process and prioritize the development of native players.
Data availability statement
Ethics statement.
Ethical review and approval was not required for the study on human participants in accordance with the local legislation and institutional requirements. Written informed consent from the patients/participants or patients/participants' legal guardian/next of kin was not required to participate in this study in accordance with the national legislation and the institutional requirements.
Author contributions
RC: Data curation, Software, Visualization, Writing – original draft. MZ: Conceptualization, Funding acquisition, Methodology, Supervision, Writing – review & editing. XX: Methodology, Software, Writing – review & editing.
The author(s) declare that no financial support was received for the research, authorship, and/or publication of this article.
Conflict of interest
The authors declare that the research was conducted in the absence of any commercial or financial relationships that could be construed as a potential conflict of interest.
Publisher’s note
All claims expressed in this article are solely those of the authors and do not necessarily represent those of their affiliated organizations, or those of the publisher, the editors and the reviewers. Any product that may be evaluated in this article, or claim that may be made by its manufacturer, is not guaranteed or endorsed by the publisher.
1 https://synergysports.com/sport/basketball/
Supplementary material
The Supplementary material for this article can be found online at: https://www.frontiersin.org/articles/10.3389/fpsyg.2023.1256796/full#supplementary-material
- Alagappan M. (2012). From 5 to 13: redefining the positions in basketball . in MIT sloan sports analytics conference, Boston, MA, USA. February, 2016. [ Google Scholar ]
- Arcidiacono P., Kinsler J., Price J. (2017). Productivity spillovers in team production: evidence from professional basketball . J. Labor Econ. 35 , 191–225. doi: 10.1086/687529 [ CrossRef ] [ Google Scholar ]
- Barrow D., Drayer I., Elliott P., Gaut G., Osting B. (2013). Ranking rankings: an empirical comparison of the predictive power of sports ranking methods . J. Quant. Analysis Sports 9 , 187–202. doi: 10.1515/jqas-2013-0013 [ CrossRef ] [ Google Scholar ]
- Berri D. J., Brook S. L., Fenn A. J. (2011). From college to the pros: predicting the NBA amateur player draft . J. Prod. Anal. 35 , 25–35. doi: 10.1007/s11123-010-0187-x [ CrossRef ] [ Google Scholar ]
- Bianchi F., Facchinetti T., Zuccolotto P. (2017). Role revolution: towards a new meaning of positions in basketball . Electronic J. App. Stat. Analysis 10 , 712–734. [ Google Scholar ]
- Božović B. (2021). The use of “synergy sports technology” for the collection of basketball game statistics . In Sinteza 2021-international scientific conference on information technology and data related research Belgrade: Singidunum University, 272–276. [ Google Scholar ]
- Çene E. (2018). What is the difference between a winning and a losing team: insights from Euroleague basketball . Int. J. Perform. Anal. Sport 18 , 55–68. doi: 10.1080/24748668.2018.1446234 [ CrossRef ] [ Google Scholar ]
- Cheng A. (2017). Using machine learning to find the 8 types of players in the NBA . Fastbreak Data . 9 . Available at: https://medium.com/fastbreak-data/classifying-the-modern-nba-player-with-machine-learning-539da03bb824 . [ Google Scholar ]
- Christmann J., Akamphuber M., Müllenbach A. L., Güllich A. (2018). Crunch time in the NBA–the effectiveness of different play types in the endgame of close matches in professional basketball . Int. J. Sports Sci. Coach. 13 , 1090–1099. doi: 10.1177/1747954118772485 [ CrossRef ] [ Google Scholar ]
- Cooper W. W., Ruiz J. L., Sirvent I. (2009). Selecting non-zero weights to evaluate effectiveness of basketball players with DEA . Eur. J. Oper. Res. 195 , 563–574. doi: 10.1016/j.ejor.2008.02.012 [ CrossRef ] [ Google Scholar ]
- Courel-Ibáñez J., Suárez E., Ortega Toro E., Piñar López M. I., Cárdenas Vélez D. (2013). Is the inside pass a performance indicator? Observational analysis of elite basketball teams . Revista de Psicología del Deporte 22 , 0191–0194. [ Google Scholar ]
- Cui M. (2020). Introduction to the k-means clustering algorithm based on the elbow method . Account. Audit. Finance 1 , 5–8. [ Google Scholar ]
- Deman B., Trnini S., Dizdar D. (2001). Expert model of decision-making system for efficient orientation of basketball players to positions and roles in the game–empirical verification . Coll. Antropol. 25 , 141–152. [ PubMed ] [ Google Scholar ]
- Diambra N. J. (2018). Using topological clustering to identify emerging positions and strategies in NCAA men’s basketball Master's Thesis, Knoxville, TN, University of Tennessee. [ Google Scholar ]
- Fu Y., Stasko J. (2022). Supporting data-driven basketball journalism through interactive visualization . in Proceedings of the 2022 CHI conference on human factors in computing systems, 1–17. [ Google Scholar ]
- Gasperi L., Conte D., Leicht A., Gómez-Ruano M.-Á. (2020). Game related statistics discriminate national and foreign players according to playing position and team ability in the Women’s basketball euro league . IJERPH 17 :5507. doi: 10.3390/ijerph17155507, PMID: [ PMC free article ] [ PubMed ] [ CrossRef ] [ Google Scholar ]
- Gómez M.-Á., Battaglia O., Lorenzo A., Lorenzo J., Jiménez S., Sampaio J. (2015). Effectiveness during ball screens in elite basketball games . J. Sports Sci. 33 , 1844–1852. doi: 10.1080/02640414.2015.1014829, PMID: [ PubMed ] [ CrossRef ] [ Google Scholar ]
- Gómez M.-A., Lorenzo A., Ibañez S.-J., Sampaio J. (2013). Ball possession effectiveness in men’s and women’s elite basketball according to situational variables in different game periods . J. Sports Sci. 31 , 1578–1587. doi: 10.1080/02640414.2013.792942, PMID: [ PubMed ] [ CrossRef ] [ Google Scholar ]
- Gòmez M.-Á., Lorenzo A., Ortega E., Sampaio J., Ibàñez S.-J. (2009). Game related statistics discriminating between starters and nonstarters players in Women’s National Basketball Association League (WNBA) . J. Sports Sci. Med. 8 , 278–283. PMID: [ PMC free article ] [ PubMed ] [ Google Scholar ]
- Gómez M. A., Lorenzo A., Sampaio J., Ibáñez S. J. (2006). Differences in game-related statistics between winning and losing teams in women’s basketball . J. Hum. Mov. Stud. 51 , 357–369. [ Google Scholar ]
- Gomez-Ruano M.-A., Ibáñez S. J., Leicht A. S. (2020). Performance analysis in sport . Lausanne: Frontiers Media SA [ PMC free article ] [ PubMed ] [ Google Scholar ]
- Guimarãe E., Santos A., Santos E., Tavares F., Janeira M. A. (2018). National players vs. foreign players: what distinguishes their game performances? A study in the portuguese basketball league. RICYDE . Revista Internacional de Ciencias del Deporte 14 , 374–381. [ Google Scholar ]
- Haghighat M., Rastegari H., Nourafza N., Branch N., Esfahan I. (2013). A review of data mining techniques for result prediction in sports . Advan. Comp. Sci. Int. J. 2 , 7–12. [ Google Scholar ]
- Hughes M. D., Bartlett R. M. (2002). The use of performance indicators in performance analysis . J. Sports Sci. 20 , 739–754. doi: 10.1080/026404102320675602 [ PubMed ] [ CrossRef ] [ Google Scholar ]
- Hughes M., Evans S., Wells J. (2001). Establishing normative profiles in performance analysis . Int. J. Perform. Anal. Sport 1 , 1–26. doi: 10.1080/24748668.2001.11868245 [ CrossRef ] [ Google Scholar ]
- Hughes M., Franks I. (2007). The essentials of performance analysis: an introduction . United Kingdom: Routledge. [ Google Scholar ]
- Ibstedt J., Rådahl E., Turesson E. (2019). Application and further development of trueskill™ ranking in sports .
- Igiri C. P., Nwachukwu E. O. (2014). An improved prediction system for football a match result . IOSR J. Engin. 4 , 2278–8719. [ Google Scholar ]
- Ivanković Z., Racković M., Markoski B., Radosav D., Ivković M. (2010). Analysis of basketball games using neural networks . 2010 11th international symposium on computational intelligence and informatics (CINTI) Manhattan: IEEE, 251–256. [ Google Scholar ]
- Kahn J. (2003). Neural network prediction of NFL football games . WWW. Electronic Pub. 9 :15. [ Google Scholar ]
- Kalman S., Bosch J. (2020). NBA lineup analysis on clustered player tendencies: a new approach to the positions of basketball & modeling lineup efficiency of soft lineup aggregates . in 14th annual MIT sloan sports analytics conference. [ Google Scholar ]
- Katzenbach J. R., Smith D. K. (2015). The wisdom of teams: creating the high-performance organization . Brighton: Harvard Business Review Press. [ Google Scholar ]
- Knoll J., Stübinger J. (2020). Machine-learning-based statistical arbitrage football betting . KI-Künstliche Intelligenz 34 , 69–80. doi: 10.1007/s13218-019-00610-4 [ CrossRef ] [ Google Scholar ]
- Koo T. K., Li M. Y. (2016). A guideline of selecting and reporting intraclass correlation coefficients for reliability research . J. Chiropr. Med. 15 , 155–163. doi: 10.1016/j.jcm.2016.02.012, PMID: [ PMC free article ] [ PubMed ] [ CrossRef ] [ Google Scholar ]
- Kovalchik S. (2020). Extension of the Elo rating system to margin of victory . Int. J. Forecast. 36 , 1329–1341. doi: 10.1016/j.ijforecast.2020.01.006 [ CrossRef ] [ Google Scholar ]
- Lamas L., Santana F., Heiner M., Ugrinowitsch C., Fellingham G. (2015). Modeling the offensive-defensive interaction and resulting outcomes in basketball . PLoS One 10 :e0144435. doi: 10.1371/journal.pone.0144435, PMID: [ PMC free article ] [ PubMed ] [ CrossRef ] [ Google Scholar ]
- Latin R. W., Berg K., Baechle T. (1994). Physical and performance characteristics of NCAA division I male basketball players . J. Strength Cond. Res. 8 , 214–218. [ Google Scholar ]
- Lewis M. (2004). Moneyball: the art of winning an unfair game . New York: WW Norton & Company. [ Google Scholar ]
- Lin J., Short L., Sundaresan V. (2014). Predicting national basketball association winners. CS 229 FINAL PROJECT, 1–5.
- Lorenzo J., Lorenzo A., Conte D., Giménez M. (2019). Long-term analysis of elite basketball players’ game-related statistics throughout their careers . Front. Psychol. 10 :421. doi: 10.3389/fpsyg.2019.00421, PMID: [ PMC free article ] [ PubMed ] [ CrossRef ] [ Google Scholar ]
- Lutz D. (2012). A cluster analysis of NBA players. In Proceedings of the MIT Sloan Sports Analytics Conference , Boston, MA, USA. Retrieved February, 2016.
- Marmarinos C., Apostolidis N., Kostopoulos N., Apostolidis A. (2016). Efficacy of the “pick and roll” offense in top level European basketball teams . J. Hum. Kinet. 51 , 121–129. doi: 10.1515/hukin-2015-0176, PMID: [ PMC free article ] [ PubMed ] [ CrossRef ] [ Google Scholar ]
- Mateus N., Esteves P., Gonçalves B., Torres I., Gomez M. A., Arede J., et al.. (2020). Clustering performance in the European basketball according to players’ characteristics and contextual variables . Int. J. Sports Sci. Coach. 15 , 405–411. doi: 10.1177/1747954120911308 [ CrossRef ] [ Google Scholar ]
- Matulaitis K., Bietkis T. (2021). Prediction of offensive possession ends in elite basketball teams . IJERPH 18 :1083. doi: 10.3390/ijerph18031083, PMID: [ PMC free article ] [ PubMed ] [ CrossRef ] [ Google Scholar ]
- McBasketball C. (2017). Nylon Calculus: How to understand synergy play type categories , 9.
- McGarry T., O’Donoghue P., de Eira Sampaio A. J., Sampaio J. (2013). Routledge handbook of sports performance analysis . Routledge, London UK. [ Google Scholar ]
- McIntyre A., Brooks J., Guttag J., Wiens J. (2016). Recognizing and analyzing ball screen defense in the NBA . Proceedings of the MIT sloan sports analytics conference, Boston, MA, USA, 11–12. [ Google Scholar ]
- Metulini R., Gnecco G. (2022). Measuring players’ importance in basketball using the generalized Shapley value . Ann. Oper. Res. 325 , 441–465. doi: 10.1007/s10479-022-04653-z [ CrossRef ] [ Google Scholar ]
- Morgan S., Williams M. D., Barnes C. (2013). Applying decision tree induction for identification of important attributes in one-versus-one player interactions: a hockey exemplar . J. Sports Sci. 31 , 1031–1037. doi: 10.1080/02640414.2013.770906, PMID: [ PubMed ] [ CrossRef ] [ Google Scholar ]
- Mustafa R. U., Nawaz M. S., Lali M. I. U., Zia T., Mehmood W. (2017). Predicting the cricket match outcome using crowd opinions on social networks: a comparative study of machine learning methods . Malays. J. Comput. Sci. 30 , 63–76. doi: 10.22452/mjcs.vol30no1.5 [ CrossRef ] [ Google Scholar ]
- O’donoghue P. (2009). Research methods for sports performance analysis . United Kingdom: Routledge. [ Google Scholar ]
- O’Donoghue P. (2014). An introduction to performance analysis of sport . United Kingdom: Routledge. [ Google Scholar ]
- Oliver D. (2004). Basketball on paper: Rules and tools for performance analysis . Dulles, VA: Potomac Books, Inc. [ Google Scholar ]
- Ostojic S. M., Mazic S., Dikic N. (2006). Profiling in basketball: physical and physiological characteristics of elite players . J. Strength Cond. Res. 20 , 740–744. doi: 10.1519/00124278-200611000-00003, PMID: [ PubMed ] [ CrossRef ] [ Google Scholar ]
- Ozmen M. U. (2012). Foreign player quota, experience and efficiency of basketball players . J. Quant. Analysis Sports 8 :1. doi: 10.1515/1559-0410.1370 [ CrossRef ] [ Google Scholar ]
- Özmen M. U. (2019). Short-term impact of a foreign player quota liberalisation policy on domestic player performance: evidence from a regression discontinuity design . Int. J. Sport Policy Politics 11 , 39–55. doi: 10.1080/19406940.2018.1488758 [ CrossRef ] [ Google Scholar ]
- Pai P.-F., Chang Liao L.-H., Lin K.-P. (2017). Analyzing basketball games by a support vector machines with decision tree model . Neural Comput. Applic. 28 , 4159–4167. doi: 10.1007/s00521-016-2321-9 [ CrossRef ] [ Google Scholar ]
- Patel R. (2017). Clustering professional basketball players by performance . University of California, Los Angeles. [ Google Scholar ]
- Pion J., Segers V., Stautemas J., Boone J., Lenoir M., Bourgois J. G. (2018). Position-specific performance profiles, using predictive classification models in senior basketball . Int. J. Sports Sci. Coach. 13 , 1072–1080. doi: 10.1177/1747954118765054 [ CrossRef ] [ Google Scholar ]
- Rangel W., Ugrinowitsch C., Lamas L. (2019). Basketball players’ versatility: assessing the diversity of tactical roles . Int. J. Sports Sci. Coach. 14 , 552–561. doi: 10.1177/1747954119859683 [ CrossRef ] [ Google Scholar ]
- Remmert H. (2003). Analysis of group-tactical offensive behavior in elite basketball on the basis of a process orientated model . Eur. J. Sport Sci. 3 , 1–12. doi: 10.1080/17461390300073311 [ CrossRef ] [ Google Scholar ]
- Sailofsky D. (2018). Drafting errors and decision making theory in the NBA draft .
- Sampaio J., Janeira M., Ibáñez S., Lorenzo A. (2006). Discriminant analysis of game-related statistics between basketball guards, forwards and centres in three professional leagues . Eur. J. Sport Sci. 6 , 173–178. doi: 10.1080/17461390600676200 [ CrossRef ] [ Google Scholar ]
- Sarlis V., Tjortjis C. (2020). Sports analytics—evaluation of basketball players and team performance . Inf. Syst. 93 :101562. doi: 10.1016/j.is.2020.101562 [ CrossRef ] [ Google Scholar ]
- Selmanovi A. (2015). Basic characteristics of offensive modalities in the Euroleague . Acta Kinesiologica 9 , 83–87. [ Google Scholar ]
- Shea S. M., Baker C. E. (2013). Basketball analytics: objective and efficient strategies for understanding how teams win . Scotts Valley, California: CreateSpace Independent Publishing Platform. Advanced Metrics. [ Google Scholar ]
- Suárez-Cadenas E., Courel-Ibáñez J., Cárdenas D., Perales J. C. (2016). Towards a decision quality model for shot selection in basketball: an exploratory study . Span. J. Psychol. 19 :E55. doi: 10.1017/sjp.2016.53, PMID: [ PubMed ] [ CrossRef ] [ Google Scholar ]
- Teramoto M., Cross C. L. (2018). Importance of team height to winning games in the National Basketball Association . Int. J. Sports Sci. Coach. 13 , 559–568. doi: 10.1177/1747954117730953 [ CrossRef ] [ Google Scholar ]
- Terner Z., Franks A. (2021). Modeling player and team performance in basketball . Annual Rev. Stat. Applicat. 8 , 1–23. doi: 10.1146/annurev-statistics-040720-015536 [ CrossRef ] [ Google Scholar ]
- Tian C., De Silva V., Caine M., Swanson S. (2019). Use of machine learning to automate the identification of basketball strategies using whole team player tracking data . Appl. Sci. 10 :24. doi: 10.3390/app10010024 [ CrossRef ] [ Google Scholar ]
- Trawiński K. (2010). A fuzzy classification system for prediction of the results of the basketball games . in International conference on fuzzy systems (IEEE; ), 1–7. [ Google Scholar ]
- Trninić S., Dizdar D., Dežman B. (2000). Empirical verification of the weighted system of criteria for the elite basketball players quality evaluation . Coll. Antropol. 24 , 443–465. PMID: [ PubMed ] [ Google Scholar ]
- Trninić S., Dizdar D., Jaklinović-Fressl Ž. (1999). Analysis of differences between guards, forwards and centres based on some anthropometric characteristics and indicators of playing performance in basketball . Kinesiology 31 , 28–34. [ Google Scholar ]
- Van der Maaten L., Hinton G. (2008). Visualizing data using t-SNE . J. Mach. Learn. Res. 9 , 2579–2605. [ Google Scholar ]
- Vaquera A., Cubillo R., García-Tormo J. V., Morante J. C. (2013). Validation of a tactical analysis methodology for the study of pick and roll in basketball . Revista de psicología del deporte 22 , 277–281. [ Google Scholar ]
- Vaquera A., García-Tormo J. V., Ruano M. G., Morante J. C. (2016). An exploration of ball screen effectiveness on elite basketball teams . Int. J. Perform. Anal. Sport 16 , 475–485. doi: 10.1080/24748668.2016.11868902 [ CrossRef ] [ Google Scholar ]
- Volker M. A. (2006). Reporting effect size estimates in school psychology research . Psychol. Sch. 43 , 653–672. doi: 10.1002/pits.20176 [ CrossRef ] [ Google Scholar ]
- Wang X., Han B., Zhang S., Zhang L., Lorenzo Calvo A., Gomez M.-Á. (2022). The differences in the performance profiles between native and foreign players in the Chinese basketball association . Front. Psychol. 12 :788498. doi: 10.3389/fpsyg.2021.788498, PMID: [ PMC free article ] [ PubMed ] [ CrossRef ] [ Google Scholar ]
- Whitehead T. (2017a). Nylon Calculus: Defining 23 offensive roles using the NBA’s play-type data. Available at: https://fansided.com/2018/10/15/nylon-calculus-defining-23-offensive-roles-nba-play-type-data/ .
- Whitehead T. (2017b). Nylon Calculus: Ranking the best and worst scorers in every offensive role. Available at: https://fansided.com/2017/08/09/nylon-calculus-ranking-best-worst-scorers-every-offensive-role/ .
- Willoughby K. A., Kostuk K. J. (2005). An analysis of a strategic decision in the sport of curling . Decis. Anal. 2 , 58–63. doi: 10.1287/deca.1050.0032 [ CrossRef ] [ Google Scholar ]
- Yong W., Lingyun P., Jia W. (2020). Statistical analysis and ARMA modeling for the big data of marathon score . Science. Sports 35 , 375–385. doi: 10.1016/j.scispo.2020.01.009 [ CrossRef ] [ Google Scholar ]
- Yu K., Su Z., Zhuang R. (2008). An exploratory study of long-term performance evaluation for elite basketball players . Int. J. Sports Sci. Engineer. 2 , 195–203. [ Google Scholar ]
- Zarić I., Kukić F., Jovićević N., Zarić M., Marković M., Toskić L., et al.. (2020). Body height of elite basketball players: do taller basketball teams rank better at the FIBA world cup? Int. J. Environ. Res. Public Health 17 :3141. doi: 10.3390/ijerph17093141, PMID: [ PMC free article ] [ PubMed ] [ CrossRef ] [ Google Scholar ]
- Zdravevski E., Kulakov A. (2009). System for prediction of the winner in a sports game . in International conference on ICT innovations (Heidelberg: Springer; ), 55–63. [ Google Scholar ]
- Zhang S., Lorenzo A., Gómez M.-A., Liu H., Gonçalves B., Sampaio J. (2017). Players’ technical and physical performance profiles and game-to-game variation in NBA . Int. J. Perform. Anal. Sport 17 , 466–483. doi: 10.1080/24748668.2017.1352432 [ CrossRef ] [ Google Scholar ]
- Zhang S., Lorenzo A., Zhou C., Cui Y., Gonçalves B., Angel Gómez M. (2019). Performance profiles and opposition interaction during game-play in elite basketball: evidences from National Basketball Association . Int. J. Perform. Anal. Sport 19 , 28–48. doi: 10.1080/24748668.2018.1555738 [ CrossRef ] [ Google Scholar ]
- Zhang L., Lu F., Liu A., Guo P., Liu C. (2016). Application of K-means clustering algorithm for classification of NBA guards . Int. J. Sci. Engineer. Applicat. 5 , 1–6. doi: 10.7753/IJSEA0501.1001 [ CrossRef ] [ Google Scholar ]
- Zhou H. B., Gao J. T. (2014). Automatic method for determining cluster number based on silhouette coefficient . Adv. Mater. Res. 951 , 227–230. doi: 10.4028/www.scientific.net/AMR.951.227 [ CrossRef ] [ Google Scholar ]
- Zukolo Z., Dizdar D., Selmanović A., Vidranski T. (2019). The role of finishing actions in the final result of the basketball match . Sport Sci. 12 , 90–95. [ Google Scholar ]
Information
- Author Services
Initiatives
You are accessing a machine-readable page. In order to be human-readable, please install an RSS reader.
All articles published by MDPI are made immediately available worldwide under an open access license. No special permission is required to reuse all or part of the article published by MDPI, including figures and tables. For articles published under an open access Creative Common CC BY license, any part of the article may be reused without permission provided that the original article is clearly cited. For more information, please refer to https://www.mdpi.com/openaccess .
Feature papers represent the most advanced research with significant potential for high impact in the field. A Feature Paper should be a substantial original Article that involves several techniques or approaches, provides an outlook for future research directions and describes possible research applications.
Feature papers are submitted upon individual invitation or recommendation by the scientific editors and must receive positive feedback from the reviewers.
Editor’s Choice articles are based on recommendations by the scientific editors of MDPI journals from around the world. Editors select a small number of articles recently published in the journal that they believe will be particularly interesting to readers, or important in the respective research area. The aim is to provide a snapshot of some of the most exciting work published in the various research areas of the journal.
Original Submission Date Received: .
- Active Journals
- Find a Journal
- Proceedings Series
- For Authors
- For Reviewers
- For Editors
- For Librarians
- For Publishers
- For Societies
- For Conference Organizers
- Open Access Policy
- Institutional Open Access Program
- Special Issues Guidelines
- Editorial Process
- Research and Publication Ethics
- Article Processing Charges
- Testimonials
- Preprints.org
- SciProfiles
- Encyclopedia
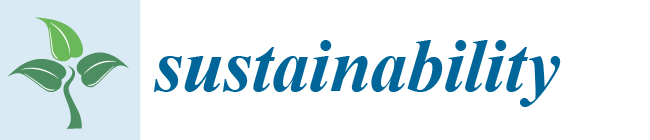
Article Menu
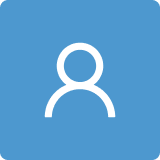
- Subscribe SciFeed
- Recommended Articles
- Google Scholar
- on Google Scholar
- Table of Contents
Find support for a specific problem in the support section of our website.
Please let us know what you think of our products and services.
Visit our dedicated information section to learn more about MDPI.
JSmol Viewer
Research on coordination of fresh supply chain considering supplier misreporting and consumer return.
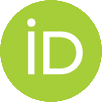
Share and Cite
Lin, Z.; Chen, R.; Luo, L.; Ren, H. Research on Coordination of Fresh Supply Chain Considering Supplier Misreporting and Consumer Return. Sustainability 2024 , 16 , 6225. https://doi.org/10.3390/su16146225
Lin Z, Chen R, Luo L, Ren H. Research on Coordination of Fresh Supply Chain Considering Supplier Misreporting and Consumer Return. Sustainability . 2024; 16(14):6225. https://doi.org/10.3390/su16146225
Lin, Zhijun, Rui Chen, Laijun Luo, and Haiping Ren. 2024. "Research on Coordination of Fresh Supply Chain Considering Supplier Misreporting and Consumer Return" Sustainability 16, no. 14: 6225. https://doi.org/10.3390/su16146225
Article Metrics
Article access statistics, further information, mdpi initiatives, follow mdpi.
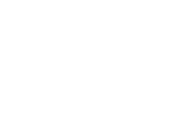
Subscribe to receive issue release notifications and newsletters from MDPI journals

IMAGES
VIDEO
COMMENTS
1. Introduction. Players' performance prediction by using current and past data has gained attention, particularly in basketball [1], [2].Sports analytics and forecasting through these data is a rapid growing field with many methods that can be implemented from a different perspective for each situation [3].In a team, and specifically for the technical staff and coaches, the knowledge of ...
Review articles, conference papers, book chapters, or unpublished articles. ... First, there is a dearth of researchon professional male basketball players. Second, research on other athletic and skill performances, such as endurance, flexibility, and basketball defensive skills, is lacking. Finally, the current review did not consider the ...
Training methods and evaluation of basketball players' agility quality: A systematic review ... non-English publications, and conference papers, a total of 463 articles were excluded. ... Although researchers have more and more training methods and means for basketball players' agility qualities, existing research primarily focuses on ...
In recent years, analytics has started to revolutionize the game of basketball: Quantitative analyses of the game inform team strategy; management of player health and fitness; and how teams draft, sign, and trade players. In this review, we focus on methods for quantifying and characterizing basketball gameplay. At the team level, we discuss methods for characterizing team strategy and ...
Introduction. Basketball is a popular sport that necessitates technical, tactical, psychological, and physiological abilities [].Physical fitness, including speed, strength, endurance, agility, and flexibility, as well as jumping, running, balance, and direction shifting, all affect basketball performance [2,3].The physical demands of basketball can be evaluated in terms of physiological ...
This research attempts to gather all the proper analytics used in sports as state-of-the-art performance indicators through sports data in decision making for basketball games, teams and players. Data mining is looking for unknown structure and data analytics [11], [12]. Hence, this could help decision-making and predict uncertain data [13].
1 Sport Science Department, Universidad Politécnica de Madrid, Madrid, Spain; 2 Institute of Sport Science and Innovations, Lithuanian Sports University, Kaunas, Lithuania; The aim of the present study was to analyze the changes of game-related statistics in expert players across their whole sports careers. From an initial sample including 252 professional basketball players competing in ...
Participants. The sample size was estimated using G*Power3.1.9 software. The sample size considerer the effect size of 0.80, an alpha level of 0.05, the power of 0.80, and two tails.
A limited number of studies focus on the effect of core training on basketball players' athletic performance and skills. This systematic reviewaimed to comprehensively and critically review the available studies in the literature that investigate the impact of core training on basketball players' physical and skill performance, and then offer valuable recommendations for both coaches and ...
In this paper, we focus on basketball, a sports pioneer in using analytic tools, and data from the National Basketball Association (NBA). As far as the literature on statistics in basketball is concerned, many of the proposed approaches can be traced back to the Bill James's Sabermetrics approach in baseball (James, 1984 , 1987 ).
Feature papers represent the most advanced research with significant potential for high impact in the field. ... In turn, basketball research has readily used game-related statistics (3% of PubMed studies in 2019) to describe player and team performance, which provide an expansive reservoir of data, usually publicly available, to link outcomes ...
(S) with nonstarters (NS), on their ability to maintain strength, power, and quickness during a competitive National Basketball Association (NBA) season. Twelve NBA players were assessed at the beginning and end of the competitive season. However, because of trades and injury, only 7 (S = 4, NS = 3) players (28.2 ± 3.4 years; 200.9 ± 9.4 cm; 104.7 ± 13.9 kg; 7.2 ± 1.9% body fat ...
This research paper examines Sports Analytics, focusing on injury patterns in the National Basketball Association (NBA) and their impact on players' performance. It employs a unique dataset to identify common NBA injuries, determine the most affected anatomical areas, and analyze how these injuries influence players' post-recovery performance. This study's novelty lies in its integrative ...
attempt to systematically amalgamate and appraise the scientific literature published in the XXI. Century, following a constraints-led approach (CLA). In particular, two underpinning factors of ...
The purpose of the present cross-sectional study was to clarify the effects of sport expertise and shot results on the action anticipation of basketball players. Eighty-eight male subjects participated in this study, namely, 30 collegiate basketball players, 28 recreational basketball players and 30 non-athletes. Each participant performed a shot anticipation task in which he watched the ...
Performance analysis and player profiling are essential components of understanding and improving athletic performance in basketball. In recent years, advancements in technology and data analytics have transformed the way performance data is collected, analyzed, and utilized in basketball. This research paper aims to contribute to the growing ...
Most research conducted in basketball has focused on athletic populations. For instance, a review of the 228 studies returned on PubMed for "basketball" in 2019 (up to August 9th) indicates over 25% of studies focused on the incidence, treatment, rehabilitation, or screening of injuries, while 11% of studies described physical, fitness, or functional attributes in competitive basketball ...
2.1. Sample and variables. The research data was obtained from the sports data website Synergy, 1 and we gained the license to use play-type related data and video. Table 1 presents the category of 11 play types in basketball, these indicators are considered standardized and cover all scoring attempts in the game of basketball, and they were able to translate the team's tactical decisions ...
OBJECTIVES: This research paper developed an Action Recognition technique for teaching basketball players using Big Data, and CapsNet called ARBIGNet METHODS: The technique uses a network that is ...
This research paper aims to statistically analyze data pertaining to the height and defensive and offensive performance of a sample population of NBA players from the 2022-2023 season to determine whether height truly ... stress, and concentration affect a basketball player's performance immensely. In a paper published by Hannibalsson (2018 ...
with seven of the eight original studies (88%) containing female basketball players. The immediate future of basketball research in high-performance settings is highlighted by issues faced in practice. Specifically, key players are missing games or being rested for "load management" in the NBA to reduce player injury risk, despite some ...
(1) Background: The objective of this systematic review was to collect relevant data in the available contemporary studies about sports injuries of basketball players and explain differences in sports injuries relative to gender, location, sport, and position on the court; (2) Methods: The papers were searched digitally using PubMed, MEDLINE, ERIC, Google Scholar, and ScienceDirect databases ...
according to various sports, in this paper we focus on basketball, in particular the U.S. National Basketball Association (NBA). The study is based on the analysis of the traditional box scores
A cross-sectional, descriptive research design was used whereby national- and state-level male adolescent basketball players (n = 24; 17.3 ± 0.5 years) completed a battery of power-related ...
Misreporting is prevalent in supply chain characterized by asymmetric information, and its impact on the supply chain is substantial and cannot be overlooked. In order to explore the impact of fresh supplier's misreporting decisions on fresh supply chain, this paper takes the fresh supply chain with a single fresh supplier and a single e-commerce enterprise as the research object, and ...