- Search Menu
- Browse content in Arts and Humanities
- Browse content in Archaeology
- Anglo-Saxon and Medieval Archaeology
- Archaeological Methodology and Techniques
- Archaeology by Region
- Archaeology of Religion
- Archaeology of Trade and Exchange
- Biblical Archaeology
- Contemporary and Public Archaeology
- Environmental Archaeology
- Historical Archaeology
- History and Theory of Archaeology
- Industrial Archaeology
- Landscape Archaeology
- Mortuary Archaeology
- Prehistoric Archaeology
- Underwater Archaeology
- Urban Archaeology
- Zooarchaeology
- Browse content in Architecture
- Architectural Structure and Design
- History of Architecture
- Residential and Domestic Buildings
- Theory of Architecture
- Browse content in Art
- Art Subjects and Themes
- History of Art
- Industrial and Commercial Art
- Theory of Art
- Biographical Studies
- Byzantine Studies
- Browse content in Classical Studies
- Classical History
- Classical Philosophy
- Classical Mythology
- Classical Literature
- Classical Reception
- Classical Art and Architecture
- Classical Oratory and Rhetoric
- Greek and Roman Epigraphy
- Greek and Roman Law
- Greek and Roman Papyrology
- Greek and Roman Archaeology
- Late Antiquity
- Religion in the Ancient World
- Digital Humanities
- Browse content in History
- Colonialism and Imperialism
- Diplomatic History
- Environmental History
- Genealogy, Heraldry, Names, and Honours
- Genocide and Ethnic Cleansing
- Historical Geography
- History by Period
- History of Emotions
- History of Agriculture
- History of Education
- History of Gender and Sexuality
- Industrial History
- Intellectual History
- International History
- Labour History
- Legal and Constitutional History
- Local and Family History
- Maritime History
- Military History
- National Liberation and Post-Colonialism
- Oral History
- Political History
- Public History
- Regional and National History
- Revolutions and Rebellions
- Slavery and Abolition of Slavery
- Social and Cultural History
- Theory, Methods, and Historiography
- Urban History
- World History
- Browse content in Language Teaching and Learning
- Language Learning (Specific Skills)
- Language Teaching Theory and Methods
- Browse content in Linguistics
- Applied Linguistics
- Cognitive Linguistics
- Computational Linguistics
- Forensic Linguistics
- Grammar, Syntax and Morphology
- Historical and Diachronic Linguistics
- History of English
- Language Acquisition
- Language Evolution
- Language Reference
- Language Variation
- Language Families
- Lexicography
- Linguistic Anthropology
- Linguistic Theories
- Linguistic Typology
- Phonetics and Phonology
- Psycholinguistics
- Sociolinguistics
- Translation and Interpretation
- Writing Systems
- Browse content in Literature
- Bibliography
- Children's Literature Studies
- Literary Studies (Asian)
- Literary Studies (European)
- Literary Studies (Eco-criticism)
- Literary Studies (Romanticism)
- Literary Studies (American)
- Literary Studies (Modernism)
- Literary Studies - World
- Literary Studies (1500 to 1800)
- Literary Studies (19th Century)
- Literary Studies (20th Century onwards)
- Literary Studies (African American Literature)
- Literary Studies (British and Irish)
- Literary Studies (Early and Medieval)
- Literary Studies (Fiction, Novelists, and Prose Writers)
- Literary Studies (Gender Studies)
- Literary Studies (Graphic Novels)
- Literary Studies (History of the Book)
- Literary Studies (Plays and Playwrights)
- Literary Studies (Poetry and Poets)
- Literary Studies (Postcolonial Literature)
- Literary Studies (Queer Studies)
- Literary Studies (Science Fiction)
- Literary Studies (Travel Literature)
- Literary Studies (War Literature)
- Literary Studies (Women's Writing)
- Literary Theory and Cultural Studies
- Mythology and Folklore
- Shakespeare Studies and Criticism
- Browse content in Media Studies
- Browse content in Music
- Applied Music
- Dance and Music
- Ethics in Music
- Ethnomusicology
- Gender and Sexuality in Music
- Medicine and Music
- Music Cultures
- Music and Religion
- Music and Media
- Music and Culture
- Music Education and Pedagogy
- Music Theory and Analysis
- Musical Scores, Lyrics, and Libretti
- Musical Structures, Styles, and Techniques
- Musicology and Music History
- Performance Practice and Studies
- Race and Ethnicity in Music
- Sound Studies
- Browse content in Performing Arts
- Browse content in Philosophy
- Aesthetics and Philosophy of Art
- Epistemology
- Feminist Philosophy
- History of Western Philosophy
- Metaphysics
- Moral Philosophy
- Non-Western Philosophy
- Philosophy of Science
- Philosophy of Language
- Philosophy of Mind
- Philosophy of Perception
- Philosophy of Action
- Philosophy of Law
- Philosophy of Religion
- Philosophy of Mathematics and Logic
- Practical Ethics
- Social and Political Philosophy
- Browse content in Religion
- Biblical Studies
- Christianity
- East Asian Religions
- History of Religion
- Judaism and Jewish Studies
- Qumran Studies
- Religion and Education
- Religion and Health
- Religion and Politics
- Religion and Science
- Religion and Law
- Religion and Art, Literature, and Music
- Religious Studies
- Browse content in Society and Culture
- Cookery, Food, and Drink
- Cultural Studies
- Customs and Traditions
- Ethical Issues and Debates
- Hobbies, Games, Arts and Crafts
- Lifestyle, Home, and Garden
- Natural world, Country Life, and Pets
- Popular Beliefs and Controversial Knowledge
- Sports and Outdoor Recreation
- Technology and Society
- Travel and Holiday
- Visual Culture
- Browse content in Law
- Arbitration
- Browse content in Company and Commercial Law
- Commercial Law
- Company Law
- Browse content in Comparative Law
- Systems of Law
- Competition Law
- Browse content in Constitutional and Administrative Law
- Government Powers
- Judicial Review
- Local Government Law
- Military and Defence Law
- Parliamentary and Legislative Practice
- Construction Law
- Contract Law
- Browse content in Criminal Law
- Criminal Procedure
- Criminal Evidence Law
- Sentencing and Punishment
- Employment and Labour Law
- Environment and Energy Law
- Browse content in Financial Law
- Banking Law
- Insolvency Law
- History of Law
- Human Rights and Immigration
- Intellectual Property Law
- Browse content in International Law
- Private International Law and Conflict of Laws
- Public International Law
- IT and Communications Law
- Jurisprudence and Philosophy of Law
- Law and Politics
- Law and Society
- Browse content in Legal System and Practice
- Courts and Procedure
- Legal Skills and Practice
- Primary Sources of Law
- Regulation of Legal Profession
- Medical and Healthcare Law
- Browse content in Policing
- Criminal Investigation and Detection
- Police and Security Services
- Police Procedure and Law
- Police Regional Planning
- Browse content in Property Law
- Personal Property Law
- Study and Revision
- Terrorism and National Security Law
- Browse content in Trusts Law
- Wills and Probate or Succession
- Browse content in Medicine and Health
- Browse content in Allied Health Professions
- Arts Therapies
- Clinical Science
- Dietetics and Nutrition
- Occupational Therapy
- Operating Department Practice
- Physiotherapy
- Radiography
- Speech and Language Therapy
- Browse content in Anaesthetics
- General Anaesthesia
- Neuroanaesthesia
- Browse content in Clinical Medicine
- Acute Medicine
- Cardiovascular Medicine
- Clinical Genetics
- Clinical Pharmacology and Therapeutics
- Dermatology
- Endocrinology and Diabetes
- Gastroenterology
- Genito-urinary Medicine
- Geriatric Medicine
- Infectious Diseases
- Medical Toxicology
- Medical Oncology
- Pain Medicine
- Palliative Medicine
- Rehabilitation Medicine
- Respiratory Medicine and Pulmonology
- Rheumatology
- Sleep Medicine
- Sports and Exercise Medicine
- Clinical Neuroscience
- Community Medical Services
- Critical Care
- Emergency Medicine
- Forensic Medicine
- Haematology
- History of Medicine
- Browse content in Medical Dentistry
- Oral and Maxillofacial Surgery
- Paediatric Dentistry
- Restorative Dentistry and Orthodontics
- Surgical Dentistry
- Browse content in Medical Skills
- Clinical Skills
- Communication Skills
- Nursing Skills
- Surgical Skills
- Medical Ethics
- Medical Statistics and Methodology
- Browse content in Neurology
- Clinical Neurophysiology
- Neuropathology
- Nursing Studies
- Browse content in Obstetrics and Gynaecology
- Gynaecology
- Occupational Medicine
- Ophthalmology
- Otolaryngology (ENT)
- Browse content in Paediatrics
- Neonatology
- Browse content in Pathology
- Chemical Pathology
- Clinical Cytogenetics and Molecular Genetics
- Histopathology
- Medical Microbiology and Virology
- Patient Education and Information
- Browse content in Pharmacology
- Psychopharmacology
- Browse content in Popular Health
- Caring for Others
- Complementary and Alternative Medicine
- Self-help and Personal Development
- Browse content in Preclinical Medicine
- Cell Biology
- Molecular Biology and Genetics
- Reproduction, Growth and Development
- Primary Care
- Professional Development in Medicine
- Browse content in Psychiatry
- Addiction Medicine
- Child and Adolescent Psychiatry
- Forensic Psychiatry
- Learning Disabilities
- Old Age Psychiatry
- Psychotherapy
- Browse content in Public Health and Epidemiology
- Epidemiology
- Public Health
- Browse content in Radiology
- Clinical Radiology
- Interventional Radiology
- Nuclear Medicine
- Radiation Oncology
- Reproductive Medicine
- Browse content in Surgery
- Cardiothoracic Surgery
- Gastro-intestinal and Colorectal Surgery
- General Surgery
- Neurosurgery
- Paediatric Surgery
- Peri-operative Care
- Plastic and Reconstructive Surgery
- Surgical Oncology
- Transplant Surgery
- Trauma and Orthopaedic Surgery
- Vascular Surgery
- Browse content in Science and Mathematics
- Browse content in Biological Sciences
- Aquatic Biology
- Biochemistry
- Bioinformatics and Computational Biology
- Developmental Biology
- Ecology and Conservation
- Evolutionary Biology
- Genetics and Genomics
- Microbiology
- Molecular and Cell Biology
- Natural History
- Plant Sciences and Forestry
- Research Methods in Life Sciences
- Structural Biology
- Systems Biology
- Zoology and Animal Sciences
- Browse content in Chemistry
- Analytical Chemistry
- Computational Chemistry
- Crystallography
- Environmental Chemistry
- Industrial Chemistry
- Inorganic Chemistry
- Materials Chemistry
- Medicinal Chemistry
- Mineralogy and Gems
- Organic Chemistry
- Physical Chemistry
- Polymer Chemistry
- Study and Communication Skills in Chemistry
- Theoretical Chemistry
- Browse content in Computer Science
- Artificial Intelligence
- Computer Architecture and Logic Design
- Game Studies
- Human-Computer Interaction
- Mathematical Theory of Computation
- Programming Languages
- Software Engineering
- Systems Analysis and Design
- Virtual Reality
- Browse content in Computing
- Business Applications
- Computer Security
- Computer Games
- Computer Networking and Communications
- Digital Lifestyle
- Graphical and Digital Media Applications
- Operating Systems
- Browse content in Earth Sciences and Geography
- Atmospheric Sciences
- Environmental Geography
- Geology and the Lithosphere
- Maps and Map-making
- Meteorology and Climatology
- Oceanography and Hydrology
- Palaeontology
- Physical Geography and Topography
- Regional Geography
- Soil Science
- Urban Geography
- Browse content in Engineering and Technology
- Agriculture and Farming
- Biological Engineering
- Civil Engineering, Surveying, and Building
- Electronics and Communications Engineering
- Energy Technology
- Engineering (General)
- Environmental Science, Engineering, and Technology
- History of Engineering and Technology
- Mechanical Engineering and Materials
- Technology of Industrial Chemistry
- Transport Technology and Trades
- Browse content in Environmental Science
- Applied Ecology (Environmental Science)
- Conservation of the Environment (Environmental Science)
- Environmental Sustainability
- Environmentalist Thought and Ideology (Environmental Science)
- Management of Land and Natural Resources (Environmental Science)
- Natural Disasters (Environmental Science)
- Nuclear Issues (Environmental Science)
- Pollution and Threats to the Environment (Environmental Science)
- Social Impact of Environmental Issues (Environmental Science)
- History of Science and Technology
- Browse content in Materials Science
- Ceramics and Glasses
- Composite Materials
- Metals, Alloying, and Corrosion
- Nanotechnology
- Browse content in Mathematics
- Applied Mathematics
- Biomathematics and Statistics
- History of Mathematics
- Mathematical Education
- Mathematical Finance
- Mathematical Analysis
- Numerical and Computational Mathematics
- Probability and Statistics
- Pure Mathematics
- Browse content in Neuroscience
- Cognition and Behavioural Neuroscience
- Development of the Nervous System
- Disorders of the Nervous System
- History of Neuroscience
- Invertebrate Neurobiology
- Molecular and Cellular Systems
- Neuroendocrinology and Autonomic Nervous System
- Neuroscientific Techniques
- Sensory and Motor Systems
- Browse content in Physics
- Astronomy and Astrophysics
- Atomic, Molecular, and Optical Physics
- Biological and Medical Physics
- Classical Mechanics
- Computational Physics
- Condensed Matter Physics
- Electromagnetism, Optics, and Acoustics
- History of Physics
- Mathematical and Statistical Physics
- Measurement Science
- Nuclear Physics
- Particles and Fields
- Plasma Physics
- Quantum Physics
- Relativity and Gravitation
- Semiconductor and Mesoscopic Physics
- Browse content in Psychology
- Affective Sciences
- Clinical Psychology
- Cognitive Psychology
- Cognitive Neuroscience
- Criminal and Forensic Psychology
- Developmental Psychology
- Educational Psychology
- Evolutionary Psychology
- Health Psychology
- History and Systems in Psychology
- Music Psychology
- Neuropsychology
- Organizational Psychology
- Psychological Assessment and Testing
- Psychology of Human-Technology Interaction
- Psychology Professional Development and Training
- Research Methods in Psychology
- Social Psychology
- Browse content in Social Sciences
- Browse content in Anthropology
- Anthropology of Religion
- Human Evolution
- Medical Anthropology
- Physical Anthropology
- Regional Anthropology
- Social and Cultural Anthropology
- Theory and Practice of Anthropology
- Browse content in Business and Management
- Business Strategy
- Business Ethics
- Business History
- Business and Government
- Business and Technology
- Business and the Environment
- Comparative Management
- Corporate Governance
- Corporate Social Responsibility
- Entrepreneurship
- Health Management
- Human Resource Management
- Industrial and Employment Relations
- Industry Studies
- Information and Communication Technologies
- International Business
- Knowledge Management
- Management and Management Techniques
- Operations Management
- Organizational Theory and Behaviour
- Pensions and Pension Management
- Public and Nonprofit Management
- Strategic Management
- Supply Chain Management
- Browse content in Criminology and Criminal Justice
- Criminal Justice
- Criminology
- Forms of Crime
- International and Comparative Criminology
- Youth Violence and Juvenile Justice
- Development Studies
- Browse content in Economics
- Agricultural, Environmental, and Natural Resource Economics
- Asian Economics
- Behavioural Finance
- Behavioural Economics and Neuroeconomics
- Econometrics and Mathematical Economics
- Economic Systems
- Economic History
- Economic Methodology
- Economic Development and Growth
- Financial Markets
- Financial Institutions and Services
- General Economics and Teaching
- Health, Education, and Welfare
- History of Economic Thought
- International Economics
- Labour and Demographic Economics
- Law and Economics
- Macroeconomics and Monetary Economics
- Microeconomics
- Public Economics
- Urban, Rural, and Regional Economics
- Welfare Economics
- Browse content in Education
- Adult Education and Continuous Learning
- Care and Counselling of Students
- Early Childhood and Elementary Education
- Educational Equipment and Technology
- Educational Strategies and Policy
- Higher and Further Education
- Organization and Management of Education
- Philosophy and Theory of Education
- Schools Studies
- Secondary Education
- Teaching of a Specific Subject
- Teaching of Specific Groups and Special Educational Needs
- Teaching Skills and Techniques
- Browse content in Environment
- Applied Ecology (Social Science)
- Climate Change
- Conservation of the Environment (Social Science)
- Environmentalist Thought and Ideology (Social Science)
- Natural Disasters (Environment)
- Social Impact of Environmental Issues (Social Science)
- Browse content in Human Geography
- Cultural Geography
- Economic Geography
- Political Geography
- Browse content in Interdisciplinary Studies
- Communication Studies
- Museums, Libraries, and Information Sciences
- Browse content in Politics
- African Politics
- Asian Politics
- Chinese Politics
- Comparative Politics
- Conflict Politics
- Elections and Electoral Studies
- Environmental Politics
- European Union
- Foreign Policy
- Gender and Politics
- Human Rights and Politics
- Indian Politics
- International Relations
- International Organization (Politics)
- International Political Economy
- Irish Politics
- Latin American Politics
- Middle Eastern Politics
- Political Methodology
- Political Communication
- Political Philosophy
- Political Sociology
- Political Behaviour
- Political Economy
- Political Institutions
- Political Theory
- Politics and Law
- Public Administration
- Public Policy
- Quantitative Political Methodology
- Regional Political Studies
- Russian Politics
- Security Studies
- State and Local Government
- UK Politics
- US Politics
- Browse content in Regional and Area Studies
- African Studies
- Asian Studies
- East Asian Studies
- Japanese Studies
- Latin American Studies
- Middle Eastern Studies
- Native American Studies
- Scottish Studies
- Browse content in Research and Information
- Research Methods
- Browse content in Social Work
- Addictions and Substance Misuse
- Adoption and Fostering
- Care of the Elderly
- Child and Adolescent Social Work
- Couple and Family Social Work
- Developmental and Physical Disabilities Social Work
- Direct Practice and Clinical Social Work
- Emergency Services
- Human Behaviour and the Social Environment
- International and Global Issues in Social Work
- Mental and Behavioural Health
- Social Justice and Human Rights
- Social Policy and Advocacy
- Social Work and Crime and Justice
- Social Work Macro Practice
- Social Work Practice Settings
- Social Work Research and Evidence-based Practice
- Welfare and Benefit Systems
- Browse content in Sociology
- Childhood Studies
- Community Development
- Comparative and Historical Sociology
- Economic Sociology
- Gender and Sexuality
- Gerontology and Ageing
- Health, Illness, and Medicine
- Marriage and the Family
- Migration Studies
- Occupations, Professions, and Work
- Organizations
- Population and Demography
- Race and Ethnicity
- Social Theory
- Social Movements and Social Change
- Social Research and Statistics
- Social Stratification, Inequality, and Mobility
- Sociology of Religion
- Sociology of Education
- Sport and Leisure
- Urban and Rural Studies
- Browse content in Warfare and Defence
- Defence Strategy, Planning, and Research
- Land Forces and Warfare
- Military Administration
- Military Life and Institutions
- Naval Forces and Warfare
- Other Warfare and Defence Issues
- Peace Studies and Conflict Resolution
- Weapons and Equipment
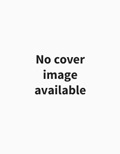
- < Previous chapter
- Next chapter >

4 Presenting Data
- Published: July 2009
- Cite Icon Cite
- Permissions Icon Permissions
Data presentation can greatly influence audiences. This chapter reviews principles and approaches for presenting data, focusing on whether data needs to be used. Data can presented using words alone (e.g., metaphors or narratives), numbers (e.g., tables), symbols (e.g., bar charts or line graphs), or some combination that integrates these methods. Although new software packages and advanced techniques are available, visual symbols that can most readily and effectively communicate public health data are pie charts, bar charts, line graphs, icons/icon arrays, visual scales, and maps. Perceptual cues, especially proximity, continuation, and closure, influence how people process information. Contextual cues help enhance meaning by providing sufficient context to help audiences better understand data. Effective data presentation depends upon articulating the purpose for communicating, understanding audiences and context, and developing storylines to be communicated, taking into account the need to present data ethically and in a manner easily understood.
Signed in as
Institutional accounts.
- GoogleCrawler [DO NOT DELETE]
- Google Scholar Indexing
Personal account
- Sign in with email/username & password
- Get email alerts
- Save searches
- Purchase content
- Activate your purchase/trial code
Institutional access
- Sign in with a library card Sign in with username/password Recommend to your librarian
- Institutional account management
- Get help with access
Access to content on Oxford Academic is often provided through institutional subscriptions and purchases. If you are a member of an institution with an active account, you may be able to access content in one of the following ways:
IP based access
Typically, access is provided across an institutional network to a range of IP addresses. This authentication occurs automatically, and it is not possible to sign out of an IP authenticated account.
Sign in through your institution
Choose this option to get remote access when outside your institution. Shibboleth/Open Athens technology is used to provide single sign-on between your institution’s website and Oxford Academic.
- Click Sign in through your institution.
- Select your institution from the list provided, which will take you to your institution's website to sign in.
- When on the institution site, please use the credentials provided by your institution. Do not use an Oxford Academic personal account.
- Following successful sign in, you will be returned to Oxford Academic.
If your institution is not listed or you cannot sign in to your institution’s website, please contact your librarian or administrator.
Sign in with a library card
Enter your library card number to sign in. If you cannot sign in, please contact your librarian.
Society Members
Society member access to a journal is achieved in one of the following ways:
Sign in through society site
Many societies offer single sign-on between the society website and Oxford Academic. If you see ‘Sign in through society site’ in the sign in pane within a journal:
- Click Sign in through society site.
- When on the society site, please use the credentials provided by that society. Do not use an Oxford Academic personal account.
If you do not have a society account or have forgotten your username or password, please contact your society.
Sign in using a personal account
Some societies use Oxford Academic personal accounts to provide access to their members. See below.
A personal account can be used to get email alerts, save searches, purchase content, and activate subscriptions.
Some societies use Oxford Academic personal accounts to provide access to their members.
Viewing your signed in accounts
Click the account icon in the top right to:
- View your signed in personal account and access account management features.
- View the institutional accounts that are providing access.
Signed in but can't access content
Oxford Academic is home to a wide variety of products. The institutional subscription may not cover the content that you are trying to access. If you believe you should have access to that content, please contact your librarian.
For librarians and administrators, your personal account also provides access to institutional account management. Here you will find options to view and activate subscriptions, manage institutional settings and access options, access usage statistics, and more.
Our books are available by subscription or purchase to libraries and institutions.
- About Oxford Academic
- Publish journals with us
- University press partners
- What we publish
- New features
- Open access
- Rights and permissions
- Accessibility
- Advertising
- Media enquiries
- Oxford University Press
- Oxford Languages
- University of Oxford
Oxford University Press is a department of the University of Oxford. It furthers the University's objective of excellence in research, scholarship, and education by publishing worldwide
- Copyright © 2024 Oxford University Press
- Cookie settings
- Cookie policy
- Privacy policy
- Legal notice
This Feature Is Available To Subscribers Only
Sign In or Create an Account
This PDF is available to Subscribers Only
For full access to this pdf, sign in to an existing account, or purchase an annual subscription.
Data Presentation
Josée Dupuis, PhD, Professor of Biostatistics, Boston University School of Public Health
Wayne LaMorte, MD, PhD, MPH, Professor of Epidemiology, Boston University School of Public Health
Introduction
While graphical summaries of data can certainly be powerful ways of communicating results clearly and unambiguously in a way that facilitates our ability to think about the information, poorly designed graphical displays can be ambiguous, confusing, and downright misleading. The keys to excellence in graphical design and communication are much like the keys to good writing. Adhere to fundamental principles of style and communicate as logically, accurately, and clearly as possible. Excellence in writing is generally achieved by avoiding unnecessary words and paragraphs; it is efficient. In a similar fashion, excellence in graphical presentation is generally achieved by efficient designs that avoid unnecessary ink.
Excellence in graphical presentation depends on:
- Choosing the best medium for presenting the information
- Designing the components of the graph in a way that communicates the information as clearly and accurately as possible.
Table or Graph?
- Tables are generally best if you want to be able to look up specific information or if the values must be reported precisely.
- Graphics are best for illustrating trends and making comparisons
The side by side illustrations below show the same information, first in table form and then in graphical form. While the information in the table is precise, the real goal is to compare a series of clinical outcomes in subjects taking either a drug or a placebo. The graphical presentation on the right makes it possible to quickly see that for each of the outcomes evaluated, the drug produced relief in a great proportion of subjects. Moreover, the viewer gets a clear sense of the magnitude of improvement, and the error bars provided a sense of the uncertainty in the data.
Principles for Table Display
- Sort table rows in a meaningful way
- Avoid alphabetical listing!
- Use rates, proportions or ratios in addition (or instead of) totals
- Show more than two time points if available
- Multiple time points may be better presented in a Figure
- Similar data should go down columns
- Highlight important comparisons
- Show the source of the data
Consider the data in the table below from http://www.cancer.gov/cancertopics/types/commoncancers
Our ability to quickly understand the relative frequency of these cancers is hampered by presenting them in alphabetical order. It is much easier for the reader to grasp the relative frequency by listing them from most frequent to least frequent as in the next table.
However, the same information might be presented more effectively with a dot plot, as shown below.

Data from http://www.cancer.gov/cancertopics/types/commoncancers
Principles of Graphical Excellence from E.R. Tufte
Pattern perception.
Pattern perception is done by
- Detection: recognition of geometry encoding physical values
- Assembly: grouping of detected symbol elements; discerning overall patterns in data
- Estimation: assessment of relative magnitudes of two physical values
Geographic Variation in Cancer
As an example, Tufte offers a series of maps that summarize the age-adjusted mortality rates for various types of cancer in the 3,056 counties in the United States. The maps showing the geographic variation in stomach cancer are shown below.
These maps summarize an enormous amount of information and present it efficiently, coherently, and effectively.in a way that invites the viewer to make comparisons and to think about the substance of the findings. Consider, for example, that the region to the west of the Great Lakes was settled largely by immigrants from Germany and Scand anavia, where traditional methods of preserving food included pickling and curing of fish by smoking. Could these methods be associated with an increased risk of stomach cancer?
John Snow's Spot Map of Cholera Cases
Consider also the spot map that John Snow presented after the cholera outbreak in the Broad Street section of London in September 1854. Snow ascertained the place of residence or work of the victims and represented them on a map of the area using a small black disk to represent each victim and stacking them when more than one occurred at a particular location. Snow reasoned that cholera was probably caused by something that was ingested, because of the intense diarrhea and vomiting of the victims, and he noted that the vast majority of cholera deaths occurred in people who lived or worked in the immediate vicinity of the broad street pump (shown with a red dot that we added for clarity). He further ascertained that most of the victims drank water from the Broad Street pump, and it was this evidence that persuaded the authorities to remove the handle from the pump in order to prevent more deaths.

Humans can readily perceive differences like this when presented effectively as in the two previous examples. However, humans are not good at estimating differences without directly seeing them (especially for steep curves), and we are particularly bad at perceiving relative angles (the principal perception task used in a pie chart).
The use of pie charts is generally discouraged. Consider the pie chart on the left below. It is difficult to accurately assess the relative size of the components in the pie chart, because the human eye has difficulty judging angles. The dot plot on the right shows the same data, but it is much easier to quickly assess the relative size of the components and how they changed from Fiscal Year 2000 to Fiscal Year 2007.
Consider the information in the two pie charts below (showing the same information).The 3-dimensional pie chart on the left distorts the relative proportions. In contrast the 2-dimensional pie chart on the right makes it much easier to compare the relative size of the varies components..
More Principles of Graphical Excellence
Exclude unneeded dimensions.
These 3-dimensional techniques distort the data and actually interfere with our ability to make accurate comparisons. The distortion caused by 3-dimensional elements can be particularly severe when the graphic is slanted at an angle or when the viewer tends to compare ends up unwittingly comparing the areas of the ink rather than the heights of the bars.
It is much easier to make comparisons with a chart like the one below.

Source: Huang, C, Guo C, Nichols C, Chen S, Martorell R. Elevated levels of protein in urine in adulthood after exposure to
the Chinese famine of 1959–61 during gestation and the early postnatal period. Int. J. Epidemiol. (2014) 43 (6): 1806-1814 .
Omit "Chart Junk"
Consider these two examples.
Here is a simple enumeration of the number of pets in a neighborhood. There is absolutely no reason to connect these counts with lines. This is, in fact, confusing and inappropriate and nothing more than "chart junk."

Source: http://www.go-education.com/free-graph-maker.html
Moiré Vibration
Moiré effects are sometimes used in modern art to produce the appearance of vibration and movement. However, when these effects are applied to statistical presentations, they are distracting and add clutter because the visual noise interferes with the interpretation of the data.
Tufte presents the example shown below from Instituto de Expansao Commercial, Brasil, Graphicos Estatisticas (Rio de Janeiro, 1929, p. 15).
While the intention is to present quantitative information about the textile industry, the moiré effects do not add anything, and they are distracting, if not visually annoying.
Present Data to Facilitate Comparisons
Here is an attempt to compare catches of cod fish and crab across regions and to relate the variation to changes in water temperature. The problem here is that the Y-axes are vastly different, making it hard to sort out what's really going on. Even the Y-axes for temperature are vastly different.

http://seananderson.ca/courses/11-multipanel/multipanel.pdf1
The ability to make comparisons is greatly facilitated by using the same scales for axes, as illustrated below.

Data source: Dawber TR, Meadors GF, Moore FE Jr. Epidemiological approaches to heart disease:
the Framingham Study. Am J Public Health Nations Health. 1951;41(3):279-81. PMID: 14819398
It is also important to avoid distorting the X-axis. Note in the example below that the space between 0.05 to 0.1 is the same as space between 0.1 and 0.2.

Source: Park JH, Gail MH, Weinberg CR, et al. Distribution of allele frequencies and effect sizes and
their interrelationships for common genetic susceptibility variants. Proc Natl Acad Sci U S A. 2011; 108:18026-31.
Consider the range of the Y-axis. In the examples below there is no relevant information below $40,000, so it is not necessary to begin the Y-axis at 0. The graph on the right makes more sense.
Also, consider using a log scale. this can be particularly useful when presenting ratios as in the example below.

Source: Broman KW, Murray JC, Sheffield VC, White RL, Weber JL (1998) Comprehensive human genetic maps:
Individual and sex-specific variation in recombination. American Journal of Human Genetics 63:861-869, Figure 1
We noted earlier that pie charts make it difficult to see differences within a single pie chart, but this is particularly difficult when data is presented with multiple pie charts, as in the example below.

Source: Bell ML, et al. (2007) Spatial and temporal variation in PM2.5 chemical composition in the United States
for health effects studies. Environmental Health Perspectives 115:989-995, Figure 3
When multiple comparisons are being made, it is essential to use colors and symbols in a consistent way, as in this example.

Source: Manning AK, LaValley M, Liu CT, et al. Meta-Analysis of Gene-Environment Interaction:
Joint Estimation of SNP and SNP x Environment Regression Coefficients. Genet Epidemiol 2011, 35(1):11-8.
Avoid putting too many lines on the same chart. In the example below, the only thing that is readily apparent is that 1980 was a very hot summer.

Data from National Weather Service Weather Forecast Office at
http://www.srh.noaa.gov/tsa/?n=climo_tulyeartemp
Make Efficient Use of Space
Reduce the ratio of ink to information.
This isn't efficient, because this graphic is totally uninformative.

Source: Mykland P, Tierney L, Yu B (1995) Regeneration in Markov chain samplers. Journal of the American Statistical Association 90:233-241, Figure 1
Bar graphs add ink without conveying any additional information, and they are distracting. The graph below on the left inappropriately uses bars which clutter the graph without adding anything. The graph on the right displays the same data, by does so more clearly and with less clutter.
Multiple Types of Information on the Same Figure
Choosing the best graph type, bar charts, error bars and dot plots.
As noted previously, bar charts can be problematic. Here is another one presenting means and error bars, but the error bars are misleading because they only extend in one direction. A better alternative would have been to to use full error bars with a scatter plot, as illustrated previously (right).
Consider the four graphs below presenting the incidence of cancer by type. The upper left graph unnecessary uses bars, which take up a lot of ink. This layout also ends up making the fonts for the types of cancer too small. Small font is also a problem for the dot plot at the upper right, and this one also has unnecessary grid lines across the entire width.
The graph at the lower left has more readable labels and uses a simple dot plot, but the rank order is difficult to figure out.
The graph at the lower right is clearly the best, since the labels are readable, the magnitude of incidence is shown clearly by the dot plots, and the cancers are sorted by frequency.
Single Continuous Numeric Variable
In this situation a cumulative distribution function conveys the most information and requires no grouping of the variable. A box plot will show selected quantiles effectively, and box plots are especially useful when stratifying by multiple categories of another variable.
Histograms are also possible. Consider the examples below.
Two Variables
The two graphs below summarize BMI (Body Mass Index) measurements in four categories, i.e., younger and older men and women. The graph on the left shows the means and 95% confidence interval for the mean in each of the four groups. This is easy to interpret, but the viewer cannot see that the data is actually quite skewed. The graph on the right shows the same information presented as a box plot. With this presentation method one gets a better understanding of the skewed distribution and how the groups compare.
The next example is a scatter plot with a superimposed smoothed line of prediction. The shaded region embracing the blue line is a representation of the 95% confidence limits for the estimated prediction. This was created using "ggplot" in the R programming language.

Source: Frank E. Harrell Jr. on graphics: http://biostat.mc.vanderbilt.edu/twiki/pub/Main/StatGraphCourse/graphscourse.pdf (page 121)
Multivariate Data
The example below shows the use of multiple panels.

Source: Cleveland S. The Elements of Graphing Data. Hobart Press, Summit, NJ, 1994.
Displaying Uncertainty
- Error bars showing confidence limits
- Confidence bands drawn using two lines
- Shaded confidence bands
- Bayesian credible intervals
- Bayesian posterior densities
Confidence Limits
Shaded Confidence Bands

Source: Frank E. Harrell Jr. on graphics: http://biostat.mc.vanderbilt.edu/twiki/pub/Main/StatGraphCourse/graphscourse.pdf

Source: Tweedie RL and Mengersen KL. (1992) Br. J. Cancer 66: 700-705
Forest Plot
This is a Forest plot summarizing 26 studies of cigarette smoke exposure on risk of lung cancer. The sizes of the black boxes indicating the estimated odds ratio are proportional to the sample size in each study.

Data from Tweedie RL and Mengersen KL. (1992) Br. J. Cancer 66: 700-705
Summary Recommendations
- In general, avoid bar plots
- Avoid chart junk and the use of too much ink relative to the information you are displaying. Keep it simple and clear.
- Avoid pie charts, because humans have difficulty perceiving relative angles.
- Pay attention to scale, and make scales consistent.
- Explore several ways to display the data!
12 Tips on How to Display Data Badly
Adapted from Wainer H. How to Display Data Badly. The American Statistician 1984; 38: 137-147.
- Show as few data as possible
- Hide what data you do show; minimize the data-ink ratio
- Ignore the visual metaphor altogether
- Only order matters
- Graph data out of context
- Change scales in mid-axis
- Emphasize the trivial; ignore the important
- Jiggle the baseline
- Alphabetize everything.
- Make your labels illegible, incomplete, incorrect, and ambiguous.
- More is murkier: use a lot of decimal places and make your graphs three dimensional whenever possible.
- If it has been done well in the past, think of another way to do it
Additional Resources
- Stephen Few: Designing Effective Tables and Graphs. http://www.perceptualedge.com/images/Effective_Chart_Design.pdf
- Gary Klaas: Presenting Data: Tabular and graphic display of social indicators. Illinois State University, 2002. http://lilt.ilstu.edu/gmklass/pos138/datadisplay/sections/goodcharts.htm (Note: The web site will be discontinued to be replaced by the Just Plain Data Analysis site).
- Help and information
- Dietetics and Nutrition
- Biochemistry
- Bioinformatics and Computational Biology
- Developmental Biology
- Ecology and Conservation
- Evolutionary Biology
- Genetics and Genomics
- Molecular and Cell Biology
- Plant Sciences and Forestry
- Research Methods in Life Sciences
- Study and Communication Skills in Life Sciences
- Zoology and Animal Sciences
- Analytical Chemistry
- Computational Chemistry
- Crystallography
- Environmental Chemistry
- Industrial Chemistry
- Inorganic Chemistry
- Materials Chemistry
- Medicinal Chemistry
- Organic Chemistry
- Organometallic Chemistry
- Physical Chemistry
- Polymer Chemistry
- Study and Communication Skills in Chemistry
- Theoretical Chemistry
- Clinical Genetics
- Endocrinology and Diabetes
- Infectious Diseases
- Medical Oncology
- Sports and Exercise Medicine
- Agriculture and Farming
- Applied Ecology
- Environmental Sustainability
- Environmentalist and Conservationist Organizations
- Management of Land and Natural Resources
- Haematology
- Biomathematics and Statistics
- Medical Skills
- Cognition and Behavioural Neuroscience
- Histopathology
- Medical Microbiology and Virology
- Biological and Medical Physics
- Cell Biology
- Public Health and Epidemiology
- Share This Facebook LinkedIn Twitter

Data Handling and Analysis (Second Edition)
- An introduction to the Fundamentals of Biomedical Science series
- 1. Information in biomedical science
- 2. Handling quantities: mass, volume, and concentration
- 3. Obtaining and verifying data
- 4. Presenting data in graphical form
- 5. Quality, audit, and good laboratory practice
- 6. Research 1: Setting the scene
- 7. Research 2: The analysis of modest data sets
- 8. Research 3: Large data sets
- 9. Communication
- Answers to end-of-chapter questions
- Answers to self-check questions
4. p. 54 Presenting data in graphical form
- Andrew D. Blann Andrew D. Blann PhD FRCPath FRCP(Ed) FIBMS CSci Institute of Biomedical Science Coldbath Square
- https://doi.org/10.1093/hesc/9780198812210.003.0004
- Published in print: 02 November 2018
- Published online: 31 August 2023
This chapter provides a background on the most common formats in which data can be presented visually, and how such data can be interpreted. It looks at methods of presenting both categorical and continuously variable data and reviews software packages that can produce excellent figures suitable for presentation and publication, limiting the requirement for hand-drawn artistic skills. It also explains how to interpret numerical data when they are presented graphically in the form of a normal and non-normal distribution, scatterplot, histogram, pie chart, dot plot and box plot and whisker plot, line plot, and graph. The chapter clarifies that continuously variable data with a normal distribution is represented by a bell-shaped curve, while data with a non-normal distribution is skewed to the left or to the right. It mentions data with a categorical distribution that is presented as a histogram.
- variable data
- software packages
- numerical data
- normal distribution
- non-normal distribution
- scatterplot
You do not currently have access to this article
Please login to access the full content.
Access to the full content requires a subscription
Printed from Oxford Science Trove. Under the terms of the licence agreement, an individual user may print out a single article for personal use (for details see Privacy Policy and Legal Notice).
date: 09 April 2024
- Cookie Policy
- Privacy Policy
- Legal Notice
- Accessibility
- [66.249.64.20|185.66.14.236]
- 185.66.14.236
Character limit 500 /500
Graph-Representation of Patient Data: a Systematic Literature Review
- Systems-Level Quality Improvement
- Open access
- Published: 12 March 2020
- Volume 44 , article number 86 , ( 2020 )
Cite this article
You have full access to this open access article
- Jens Schrodt ORCID: orcid.org/0000-0002-9768-4781 1 ,
- Aleksei Dudchenko ORCID: orcid.org/0000-0002-4717-2307 1 , 2 ,
- Petra Knaup-Gregori 1 &
- Matthias Ganzinger ORCID: orcid.org/0000-0002-2716-5425 1
12k Accesses
16 Citations
8 Altmetric
Explore all metrics
Graph theory is a well-established theory with many methods used in mathematics to study graph structures. In the field of medicine, electronic health records (EHR) are commonly used to store and analyze patient data. Consequently, it seems straight-forward to perform research on modeling EHR data as graphs. This systematic literature review aims to investigate the frontiers of the current research in the field of graphs representing and processing patient data. We want to show, which areas of research in this context need further investigation. The databases MEDLINE, Web of Science, IEEE Xplore and ACM digital library were queried by using the search terms health record , graph and related terms. Based on the “Preferred Reporting Items for Systematic Reviews and Meta-Analysis” (PRISMA) statement guidelines the articles were screened and evaluated using full-text analysis. Eleven out of 383 articles found in systematic literature review were finally included for analysis in this literature review . Most of them use graphs to represent temporal relations, often representing the connection among laboratory data points. Only two papers report that the graph data were further processed by comparing the patient graphs using similarity measurements. Graphs representing individual patients are hardly used in research context, only eleven papers considered such kind of graphs in their investigations. The potential of graph theoretical algorithms, which are already well established, could help increasing this research field, but currently there are too few papers to estimate how this area of research will develop. Altogether, the use of such patient graphs could be a promising technique to develop decision support systems for diagnosis, medication or therapy of patients using similarity measurements or different kinds of analysis.
Similar content being viewed by others
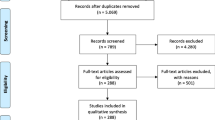
The role of artificial intelligence in healthcare: a structured literature review
Silvana Secinaro, Davide Calandra, … Paolo Biancone
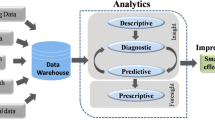
Big data in healthcare: management, analysis and future prospects
Sabyasachi Dash, Sushil Kumar Shakyawar, … Sandeep Kaushik
Big Data Analytics in Healthcare
Avoid common mistakes on your manuscript.

Introduction
Today, electronic health records (EHR) are the predominant way of documenting health care activities. While the establishment of EHR started decades ago, there are still lively research activities associated with it. Querying PubMed for the term “electronic health record” shows an increasing number of publications year by year until now (cf. Figure 1 ).
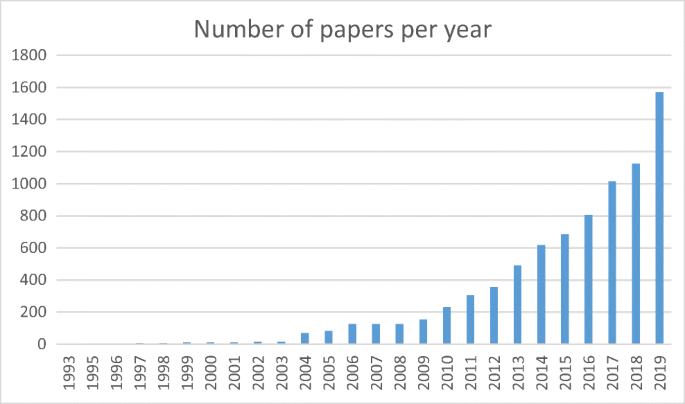
Development of papers per year using the keyword „electronic health record” until 2019
From an information technology perspective, the way how EHR data are persisted has also experienced some development over the years. One of the first approaches that was broadly applied was storing EHR data in relational databases [ 1 ]. Today, most hospital information systems are probably based on relational database management software and are accessed with the corresponding Structured Query Language (SQL) [ 2 , 3 ].
However, the development towards NoSQL database management systems also influenced EHR systems [ 4 , 5 ]. For instance, document oriented database management systems such as CouchDB [ 6 ] or MongoDB [ 7 , 8 ], no longer require the organization of data in tables. Instead, data are stored as documents written in data formats such as the JavaScript Object Notation (JSON) [ 9 ] or Extensible Markup Language (XML) [ 10 ]. Another type of NoSQL databases are graph databases [ 11 , 12 ]. In contrast to document databases, data are stored as properties in structures consisting of nodes and edges. Graph databases have many advantages in comparison to relational databases, for instance, graph databases are much easier to scale, are faster especially at highly connected data, and have a higher level of availability than common relational databases [ 13 ].
Graph databases are already in use in various social networks like Facebook and in other Internet companies like Amazon or Google [ 14 ]. In social networks, graph databases are very useful because of their property to store the relationships between different members of the social network directly and intuitively (for the user). This direct storage decreases computation time and makes it possible to create queries, which can access these relationships directly. To use these stored relationships of graph databases is also much easier than using the relational data model and its SQL statements for the same purpose. In the relational data model, complex join statements would be necessary to get the same effect and this complicates the creation of queries and increases their computation time compared to graph database queries [ 14 ]. And there are also many more fields of applications for graph databases apart from social networks e.g. biomolecular pathways [ 15 ], for integration of heterogeneous biological data [ 16 ] and for representing disease networks [ 17 ].
Graphs in context of graph theory are very clearly defined as a set of nodes connected by edges, which represent a relation between the connected nodes [ 18 ], this definition is used for the expression graph in the following chapters. Figure 2 shows the schematic representation of a graph. The dots are the vertices or nodes, the connections between the nodes represent the edges. Graph theory is a well-established area of mathematics that also covers methods to compare graphs. Apart from graph databases, these methods make the usage of graphs in medical context very interesting, for example to model patient data of EHR systems. With approaches like this, diagnoses, therapies and medications could be suggested on the basis of previous patients and the experiences made at treatment of these patients. Such a system could also be part of a decision support system for physicians in clinical context. For example, graphs are used for spatial description of cerebral anatomy [ 19 ] or for clustering of patients and for making a diagnosis [ 20 ]. Other approaches are closer related to EHR. Such projects focus for example on visualizing collaborative electronic health record usage with heart failure [ 11 ], modeling disease graphs [ 21 ] or to predicting knowledge graphs of unknown adverse drug reactions [ 22 ].
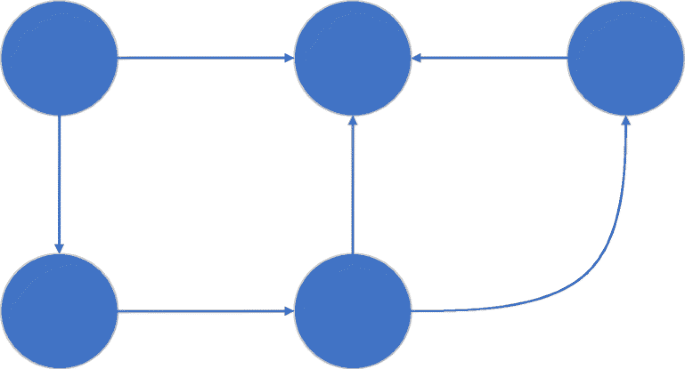
Schematic representation of a directed graph. The dots are called nodes, the connections between the nodes are called edges. The edges are directed, this is shown by the arrow, which points the edge in a direction.
The aim of this literature review is to investigate the frontiers of the current research in the field of graphs representing and processing patient data. We want to show, which areas of research require further investigation. Before planning research projects in this area we would like to get an overview of already established applications of graphs for individual patients on which, for example, similarity comparisons were performed or the temporal relationships in patient data were used for research projects. To compare patients, it seems to be necessary that patient data are represented by individual graphs or at least sub-graphs. The resulting main questions for the review were:
Which kinds of graph-based representations or graph database models of patient data are appropriate established for individual patients?
How is the patient data technologically processed after using the graph theoretical framework (e.g. by using graph databases or temporal modeling)?
Our systematic literature review is based on the guidelines of the Preferred Reporting Items for Systematic Reviews and Meta-Analyses (PRISMA) approach [ 23 ].
Search strategy
We used the keywords health records and graph with the synonyms medical record, patient record and all plural forms of the keywords for our database search in the databases MEDLINE, Web of Science, IEEE Xplore and ACM digital library. The fields, which were investigated with the search terms, were title and abstract. The keywords were included in the query syntax of each investigated database. The specific database queries are shown in Suppl. Fig. 1 .
Inclusion criteria
The investigated papers were screened against the following inclusion criteria. The first main criterion was the usage of the term graph in the sense of graph theory. This means that the graphs should contain nodes and edges, which is one main definition criterion for graphs of graph theory. Many papers used this word in another context, e.g. as some graphs used the term graph as a synonym for illustration and were therefore excluded. Articles were also excluded if they do not use graphs representing individual patients but for example for a set of patients. Further, only articles written in English or German were included. The database search was done at 20.03.2018 and therefore only articles published and indexed until this date were considered in the review.
Selection of articles
The articles retrieved from the database queries were screened by four reviewers according to the inclusion criteria based on their title and abstract. If there is no abstract, the full text of the article was used. Initially, all four reviewers tested the inclusion criteria on the same sample of ten articles independently. The results of this test review were discussed afterwards to reach a consensus understanding of the inclusion criteria.
To reduce the workload for the reviewers, the total number of articles was split into two halves, which were assigned to two teams of two reviewers. The members of each team assessed the articles assigned with their partners’ results blinded to ensure that each article received two independent votes. Reviewers marked each article as “included” or “excluded”. For excluded articles, a reason for the exclusion was documented. Articles, which were included by both reviewers, were selected for full-text investigation. Those articles, that were included by one reviewer and excluded by the other reviewer, were assessed by a third reviewer to reach a final decision. The third reviewer decided about inclusion or exclusion of the respective article.
Data extraction and synthesis
The articles included in the steps before were analyzed in full-text. Some articles still had to be excluded in this phase, because the fulfillment of inclusion criteria, which was recognized in the screening phase, could not be seconded by full-text analysis. To support full-text analysis, the computer-assisted qualitative data analysis software (CAQDAS) MAXQDA was used [ 24 , 25 ]. In MAXQDA we established a coding system, which was initially created using one article as basis. In a coding system, all central keywords of all investigated and included articles were collected as a hierarchical structure. Each keyword can be assigned to multiple articles and each article can be assigned to multiple keywords. The coding system was iteratively developed by investigating the further articles. Therefore, the papers were loaded in MAXQDA as PDF files to tag the information expressed by the codes in the coding system. Afterwards, cross article occurrences of the different codings were analyzed and main statements were extracted: the kinds of graphs used in the papers, the kinds of data sources, the node and edge contents as well as the processing methods used in the papers.
Overall results
By database search, we found 201 hits in MEDLINE, 107 hits in Web of Science, 58 hits in IEEE Xplore and 92 hits in ACM digital library. After eliminating duplicates, the total number of articles was 383. After assessing the inclusion criteria, the reviewers agreed on including 320 of 383 abstracts (84%). For 63 abstracts, they had contradicting opinions, which made the decision of a third reviewer necessary. Finally, 42 abstracts were included by the agreement of the first two reviewers; six further abstracts were added by the third reviewer checking the conflicting articles in abstract (and full-text if necessary). So, in total 48 articles (12.5%) were included, 335 (87.5%) were excluded. The main reasons for exclusion were
Papers did not use the term graph in a graph theoretical manner
Papers used graph theory, but the graphs did not represent individual patients (Supplementary Table 3 ).
After the step of full-text analysis of the 48 articles eleven of these articles finally remained (2.9%) [ 19 , 20 , 26 , 27 , 28 , 29 , 30 , 31 , 32 , 33 , 34 ] for analysis in MAXQDA by using a coding scheme.
Coding scheme
The coding scheme used in MAXQDA is shown in Suppl. Fig. 3 . The main categories after analyzing the eleven remaining articles were a) data source b) overall purpose / function c) graph properties d) investigated disease e) technical processing of graph. At first, the methods of representing and saving data in graphs and graph databases were investigated and this investigation can be aligned to the codings data source and graph properties . Theses codings define how the graphs are generated and what they contain as nodes and edges. The second main question that we investigated was the processing methods of these graphs and their contents. Thus, we investigated the processing methods of these graphs and their contents by using the codings overall purpose / function and especially technical processing of graph. This second step helps us to understand how currently existing investigations handle the processing of patient graphs and what kinds of goals these investigations would like to reach.
Graph properties
In Table 1 , all different kinds of node contents from the eleven articles included are shown. Six of the papers used laboratory data represented in nodes, five of the papers used medications and diagnosis. Functional nodes were used four times, whereas anatomic nodes and patient problems were used two times each. Procedures, vital signs and patient nodes (a node to identify the patient used in this graph) were used only one time in the papers.
In contrast to Table 1 , Table 2 shows the content of the edges used in the eleven papers. In two papers, the edges represent causal relations, so the nodes are connected in a causal context. In one paper, the edges represent anatomic-functional relations, whereas in two papers spatial relations were represented by the edges. In detail, the edges show the spatial relations of brain areas. Taxonomical relations and status and date are two more edge contents used, each in one paper. The edge content most often used by the included articles are temporal relations , which were used in six different papers.
Graph types
Table 3 shows all types of graphs used in the articles to represent electronic health records of a patient. Most of the remaining articles use a representation of electronic health records in a graph representing an individual patient in a temporal manner (temporal event data mining) [ 20 , 27 , 28 , 30 , 32 , 33 ]. In contrast to that, causal networking represents the causal context of patient data and was used in two papers for representing patient data [ 29 , 30 ]. Heterogeneous data mining was used by one paper and describes the representation of very different kinds of data of the patient in one graph [ 30 ] whereas database / data structural approaches were used in two papers. These papers demonstrate possible methods of saving patient data in a graph database or in a graph like structure [ 26 , 34 ]. Two further papers use the graphs for structural representation of tissue areas in the brain [ 19 , 31 ].
Data sources
We also investigated the different data sources for patient data used in the included articles as shown in Table 4 . Electronic health records are the biggest part of data sources used in the articles (62.5%). Some articles also use image-based information (12.5%) or data from a healthcare information system (12.5%). One article uses SNOMED CT clinical findings and another one uses a research database (each 6.25%).
Processing of Graphs
Table 5 shows the number of papers, which used the shown kinds of processing methods for patient graphs. Only one paper uses the model for prognosis issues [ 29 ]. Five papers investigate the storage of patient graphs in some kind, e.g. in graph databases. In only two papers, the authors are interested in similarity comparisons of the created patient graphs, whereas in nine papers the plain presentation of patient data in a graph plays a central role.
Goals and Content of the Articles
The research goals described in the different articles differ very much in detail, but an application often mentioned in the articles was personalized medicine, which was named by 6 of the 11 investigated papers. The other goals were quality improvement, information gaining, predictive modeling, disease diagnosis, patient segmentation (each used in 2 papers), population management, data mining, data warehouse and disease pattern (each used in one paper).
To reach these goals the papers follow very different strategies. Atif et al. used image-based information of brains to create a graph-based cerebral description of brain anatomy. This spatial graph is created manually for every patient and afterwards patients could be compared using these graphs [ 19 ]. In contrast, Campbell et al. used the SNOMED CT concept model in a graph database architecture because of the ontology character and polyhierarchy of SNOMED CT, which makes it difficult to implement electronic health records in relational databases [ 26 ]. The created graphs save SNOMED CT data in a specifically created graph format. Risk prediction is the main goal of Chen et al., so the authors developed a graph-based, semi-supervised learning algorithm to reach this goal [ 27 ]. By modelling the clinical evolution of an individual patient with kidney failure Esteban et al. wanted to develop the basis for a future clinical decision support system. This graph-based model contains thousands of events like laboratory results, ordered tests and diagnoses [ 28 ] and represents a patient in a graph. Hanzlicek et al. described MUDR EHR, a multimedia distributed health record for decision support. This electronic health record contains multiple medical concepts, which should help describing a patient in a structured way, apart from free text records [ 34 ]. Kaur et al. described a model, which combines different data stores of patient data. In this architecture the user creates his request at the interface and the architecture below translates this request into a query to get the data from the most suitable data store for this request [ 29 ]. The resulting graph of this paper helps to get the right information from the data stores. Liu et al. used longitudinal patient data to create so called temporal graphs. These graphs were clustered in different phenotypes, so that using these phenotypes helps improving diagnosis performance [ 20 ]. The resulting graphs represent a patient and his medical events in temporal context. The focus of Müller et al. was the lack of clinical context in other approaches. The authors solve this problem by creating a graph-grammar approach to design and implement a graph-oriented patient model, which allows the representation of the clinical context [ 30 ]. Puentes et al. also used (similar to Atif et al.) graphs to gain information out of image-based brain information to model spatial relationships of brain anatomical singularities of individual patients. This approach is especially used for spatial modelling of cerebral tumors [ 31 ]. Zhang et al. [ 32 ] created a convolutional neural network on heterogeneous attributes of a patient (e.g. diagnoses, procedures and medications) using a graph, which gains its data from electronic health records [ 32 ]. Zhang et al. [ 33 ] created a unified graph representation of the electronic health records of an individual patient in a temporal manner. Using this graph, in the second step a modified algorithm was used to create a temporal profile of each patient. This approach was used for risk prediction [ 33 ].
General Findings
Our literature review shows that there are many articles published in context of medical records and graphs, but only a small number of authors investigated graphs in the sense of graph theory or used graphs to represent individual patient data. The initial database query produced a sample size of almost 400 papers. Many of these papers had to be excluded because they used the term graph in a different context than for graph theory. But if the graph mentioned by the paper could be related to graph theory, the second most exclusion criterion often arises: Most of the papers, which use graphs, do not use these graphs to represent data of individual patients. Surprisingly, the exclusion resulted in only eleven papers remaining. From a theoretical point of view, the modeling of patient data as a graph could result in some advantages in analysis of this patient data because of the well-established tools and methods contents of graph theory. The usage of such already established algorithms could facilitate the development of new methods enormously. Research question 1 of this literature review corresponds to possible patient representations in graphs that were used in literature before. Table 1 and Table 2 show the different possibilities of using nodes and edges to represent patient data used in the investigated articles. Especially laboratory data as nodes and temporal relationships between nodes were most commonly used in the investigated papers. The graph type used most often was temporal event data mining, followed by causal networking, structure representation, database structural approaches and heterogeneous data mining. The focus on temporal relationships shows that most data in this field of research was investigated in context of temporal relationships but the low number of papers included in this literature review also shows that there is much more potential for further analysis. With Question 2 we wanted to get an overview of all different processing mechanisms used to investigate the patient graphs. The low number of papers found through the literature review made it very difficult to identify real tendencies, but the main results are shown in Table 5 – only two of the investigated papers do really process the patient data after setting it to a graph. In contrast to that, nine papers use the graphs only to represent the patient data and five papers also use the graphs to store the data in a specific form. This very low number of papers using graphs for representing individual patients and the even lower number of papers processing theses graphs raise different questions for further investigation: Is it reasonable to represent patient data in graphs and process them or is there any reason why this has not been done very often so far? What is the best the way to proof plausibility of such systems?
Limitations
In this study only the four databases MEDLINE, Web of Science, IEEE Xplore and ACM digital library were used, thus there might be some papers indexed in other databases, which were not found by our review. Apart from the databases, there also might be papers connected to this topic, which would have been captured by using another query. Also publication bias plays a role in literature reviews, especially in this context. The low number of papers using graph theory for representing patient data could also be caused by a high rate of unsuccessful papers in this field of research.
Our review shows the current state of research in context of patient graphs. The concentration of many of the eleven papers on the recent past might indicate, that this is a rather young research area, which could expand in the next few years, but currently the total number of papers connected to the research field is too low to make a clear statement. Altogether representing a patient in a graph is a very promising technique, which is already used in very different medical areas as shown by the content of the included papers. These different areas (brain tumors, kidney failures, patients in general and so on) show that there is much potential for further studies in this field of research. The possibilities with such systems are very broad and open new opportunities, for example in clinical context. We could imagine a system that helps analyzing patient graphs for finding differential diagnosis, the right medication, or even to get therapy proposals based on experiences made in previous patient cases.
Friedman, C., Hripcsak, G., Johnson, S. B. et al. A generalized relational Schema for an integrated clinical patient database. Proc Annu Symp Comput Appl med care: 335–339, 1990
Codd, E. F., A relational model of data for large shared data banks. Commun. ACM 13(6):377–387, 1970. https://doi.org/10.1145/362384.362685 .
Article Google Scholar
ISO/IEC Information technology—Database languages—SQL—Part 1: Framework (SQL/Framework)(ISO/IEC 9075–1:2016), 2016
Klein, J., Gorton, I., Ernst, N. et al., Performance evaluation of NoSQL databases. In: Singhal, R., Chahal, D. (Eds), Proceedings of the 1 st workshop on performance analysis of big data systems - PABS ‘15. New York: ACM Press, 2015, 5–10.
Google Scholar
Ercan, M. Z., Lane, M., Evaluation of NoSQL databases for EHR systems. In: 25 th Australasian conference on information systems, 2014
Rascovsky, S. J., Delgado, J. A., Sanz, A. et al., Informatics in radiology: Use of CouchDB for document-based storage of DICOM objects. Radiographics 32(3):913–927, 2012. https://doi.org/10.1148/rg.323115049 .
Article PubMed Google Scholar
Luo, L., Li, L., Hu, J., Wang, X., Hou, B., Zhang, T., and Zhao, L. P., A hybrid solution for extracting structured medical information from unstructured data in medical records via a double-reading/entry system. BMC Med Inform Decis Mak 16(1):114–114, 2016. https://doi.org/10.1186/s12911-016-0357-5 .
Article PubMed PubMed Central Google Scholar
Xu, W., Zhou, Z., Zhou, H., et al. MongoDB improves big data analysis performance on electric health record system. In: Ma S, Jia L, Li X et al. (eds) Life system modeling and simulation, vol 461. Springer Berlin Heidelberg, Berlin, Heidelberg, pp 350–357, 2014
Bray, T., The javascript object notation (json) data interchange format, 2017
Bray, T., Paoli, J., Sperberg-McQueen, C. M., et al. Extensible markup language (XML) 1.0, 2008
Soulakis, N. D., Carson, M. B., Lee, Y. J. et al., Visualizing collaborative electronic health record usage for hospitalized patients with heart failure. J Am Med Inform Assoc 22(2):299–311, 2015. https://doi.org/10.1093/jamia/ocu017 .
Zhou, X., Han, H., Chankai, I., et al. Approaches to text mining for clinical medical records. In: Haddad HM (ed) the 2006 ACM symposium, p 235, 2006
Nayak, A., Poriya, A., and Poojary, D., Type of NOSQL databases and its comparison with relational databases. International Journal of Applied Information Systems (IJAIS) 5(4):16–19, 2013.
Moniruzzaman, A. B. M., Hossain, S. A., NoSQL database: New era of databases for big data analytics -classification, characteristics and comparison. International Journal of Database Theory and Application 6(4), 2013
Fabregat, A., Korninger, F., Viteri, G., Sidiropoulos, K., Marin-Garcia, P., Ping, P., Wu, G., Stein, L., D'Eustachio, P., and Hermjakob, H., Reactome graph database: Efficient access to complex pathway data. PLoS Comput Biol 14(1):e1005968, 2018. https://doi.org/10.1371/journal.pcbi.1005968 .
Article CAS PubMed PubMed Central Google Scholar
Yoon, B.-H., Kim, S.-K., and Kim, S.-Y., Use of graph database for the integration of heterogeneous biological data. Genomics Inform 15(1):19–27, 2017. https://doi.org/10.5808/GI.2017.15.1.19 .
Lysenko, A., Roznovăţ, I. A., Saqi, M., Mazein, A., Rawlings, C. J., and Auffray, C., Representing and querying disease networks using graph databases. BioData Min 9(1):23–19, 2016. https://doi.org/10.1186/s13040-016-0102-8 .
Bollobás, B., Modern graph theory, 5. Print. Graduate texts in mathematics, vol 184. Springer, New York, NY, 2010
Atif, J., Hudelot, C., Nempont, O., et al. GRAFIP: A FRAMEWORK FOR THE REPRESENTATION OF HEALTHY AND PATHOLOGICAL CEREBRAL INFORMATION. In: 2007 4 th IEEE international symposium on biomedical imaging: From Nano to macro, pp 205–208, 2007
Liu, C., Wang, F., Hu, J., et al. Temporal Phenotyping from longitudinal electronic health records. In: Cao L, Zhang C, Joachims T et al. (eds) the 21th ACM SIGKDD international conference, pp 705–714, 2015
Yousefi, A., Mastouri, N., Sartipi, K., Scenario-oriented information extraction from electronic health records. In: 22 nd IEEE International Symposium on Computer-Based Medical Systems, 2009: CBMS 2009 ; date: 2–5 [i.e. 3–4] August 2009, Albuquerque, New Mexico, USA. IEEE, Piscataway, NJ, pp 1–5, 2009
Bean, D. M., Wu, H., Iqbal, E. et al., Knowledge graph prediction of unknown adverse drug reactions and validation in electronic health records. Sci Rep 7(1):1–11. https://doi.org/10.1038/s41598-017-16674-x .
Moher, D., Liberati, A., Tetzlaff, J., Altman, D. G., and PRISMA Group, Preferred reporting items for systematic reviews and meta-analyses: The PRISMA statement. BMJ 339:b2535, 2009. https://doi.org/10.1136/bmj.b2535 .
VERBI Software GmbH (2018) MAXQDA: Software for qualitative and mixed methods research. VERBI Software GmbH
Kuckartz U (2014) Qualitative text analysis: A guide to methods, practice & using software. Sage, Los Angeles, London, New Delhi, Singapore, Washington, DC
Campbell, W. S., Pedersen, J., McClay, J. C. et al., An alternative database approach for management of SNOMED CT and improved patient data queries. J Biomed Inform 57:350–357, 2015. https://doi.org/10.1016/j.jbi.2015.08.016 .
Chen, L., Li, X., Sheng, Q. Z. et al., Mining health examination records—A graph-based approach. IEEE Trans. Knowl. Data Eng. 28(9):2423–2437, 2016. https://doi.org/10.1109/TKDE.2016.2561278 .
Esteban, C., Schmidt, D., Krompass, D., et al. Predicting sequences of clinical events by using a personalized temporal latent embedding model. In: 2015 international conference on healthcare informatics (ICHI), pp 130–139, 2015
Kaur, K., and Rani, R., Managing data in healthcare information systems: Many models, one solution. Computer 48(3):52–59, 2015. https://doi.org/10.1109/MC.2015.77 .
Müller, R., Thews, O., Rohrbach, C., Sergl, M., and Pommerening, K., A graph-grammar approach to represent causal, temporal and other contexts in an oncological patient record. Methods Inf Med 35(2):127–141, 1996.
Puentes, J., Batrancourt, B., Atif, J., Angelini, E., Lecornu, L., Zemirline, A., Bloch, I., Coatrieux, G., and Roux, C., Integrated multimedia electronic patient record and graph-based image information for cerebral tumors. Comput Biol Med 38(4):425–437, 2008. https://doi.org/10.1016/j.compbiomed.2008.01.009 .
Zhang, J., Gong, J., Barnes, L., HCNN: Heterogeneous convolutional neural networks for comorbid risk prediction with electronic health records. In: 2017 IEEE/ACM international conference on connected health: Applications, systems and engineering technologies (CHASE), pp 214–221, 2017
Zhang, S., Liu, L., Li, H., et al. MTPGraph: A data-driven approach to predict medical risk based on temporal profile graph. In: 2016 IEEE Trustcom/BigDataSE/ISPA, pp 1174–1181, 2016
Hanzlicek, P., Spidlen, J., and Nagy, M., Universal electronic health record MUDR. Stud Health Technol Inform 105:190–201, 2004.
PubMed Google Scholar
Download references
Acknowledgements
The authors would like to thank Liwen Zhu for helping to screen the articles of this literature review.
Open Access funding provided by Projekt DEAL. This study was funded within the systems medicine project “Clinically-applicable, omics-based assessment of survival, side effects, and targets in multiple myeloma” (CLIOMMICS) by the German Federal Ministry of Education and Research (BMBF, grant id: 01ZX1609A) as part of the e:Med initiative.
Author information
Authors and affiliations.
Institute for Medical Biometry and Informatics, Heidelberg University, Im Neuenheimer Feld 130.3, 69120, Heidelberg, Germany
Jens Schrodt, Aleksei Dudchenko, Petra Knaup-Gregori & Matthias Ganzinger
School of Translational Information Technologies, ITMO University, Kronverksky Pr. 49, 197101, Saint-Petersburg, Russia
Aleksei Dudchenko
You can also search for this author in PubMed Google Scholar
Corresponding author
Correspondence to Matthias Ganzinger .
Ethics declarations
Conflict of interest.
All authors declare that they have no conflict of interest.
Ethical Approval
This article does not contain any studies with human participants or animals performed by any of the authors.
Additional information
Publisher’s note.
Springer Nature remains neutral with regard to jurisdictional claims in published maps and institutional affiliations.
This article is part of the Topical Collection on Systems-Level Quality Improvement
Electronic supplementary material
(PDF 82 kb)
(PDF 150 kb)
(PDF 89 kb)
(PDF 90.4 kb)
(PDF 1.19 mb)
(PDF 720 kb)
(PDF 1.03 mb)
Rights and permissions
Open Access This article is licensed under a Creative Commons Attribution 4.0 International License, which permits use, sharing, adaptation, distribution and reproduction in any medium or format, as long as you give appropriate credit to the original author(s) and the source, provide a link to the Creative Commons licence, and indicate if changes were made. The images or other third party material in this article are included in the article's Creative Commons licence, unless indicated otherwise in a credit line to the material. If material is not included in the article's Creative Commons licence and your intended use is not permitted by statutory regulation or exceeds the permitted use, you will need to obtain permission directly from the copyright holder. To view a copy of this licence, visit http://creativecommons.org/licenses/by/4.0/ .
Reprints and permissions
About this article
Schrodt, J., Dudchenko, A., Knaup-Gregori, P. et al. Graph-Representation of Patient Data: a Systematic Literature Review. J Med Syst 44 , 86 (2020). https://doi.org/10.1007/s10916-020-1538-4
Download citation
Received : 03 April 2019
Accepted : 07 February 2020
Published : 12 March 2020
DOI : https://doi.org/10.1007/s10916-020-1538-4
Share this article
Anyone you share the following link with will be able to read this content:
Sorry, a shareable link is not currently available for this article.
Provided by the Springer Nature SharedIt content-sharing initiative
- Graph theory
- Systematic literature review
- Electronic health record
- Temporal patient graph
Advertisement
- Find a journal
- Publish with us
- Track your research

An official website of the United States government
The .gov means it’s official. Federal government websites often end in .gov or .mil. Before sharing sensitive information, make sure you’re on a federal government site.
The site is secure. The https:// ensures that you are connecting to the official website and that any information you provide is encrypted and transmitted securely.
- Publications
- Account settings
Preview improvements coming to the PMC website in October 2024. Learn More or Try it out now .
- Advanced Search
- Journal List
- Int J Environ Res Public Health

A Systematic Review on Visualizations for Self-Generated Health Data for Daily Activities
Associated data.
Not applicable.
Due to the development of sensing technology people can easily track their health in various ways, and the interest in personal healthcare data is increasing. Individuals are interested in controlling their wellness, which requires self-awareness and an understanding of various health conditions. Self-generated health data are easily accessed through mobile devices, and data visualization is commonly used in applications. A systematic literature review was conducted to better understand the role of visualizations and learn how to develop effective ones. Thirteen papers were analyzed for types of data, characteristics of visualizations, and effectiveness for healthcare management. The papers were selected because they represented research on personal health data and visualization in a non-clinical setting, and included health data tracked in everyday life. This paper suggests six levels for categorizing the efficacy of visualizations that take into account cognitive and physical changes in users. Recommendations for future work on conducting evaluations are also identified. This work provides a foundation for personal healthcare data as more applications are developed for mobile and wearable devices.
1. Introduction
There are initiatives to return personal health data to patients because they can benefit from increased comprehension of their health status, engagement in care with caregivers, and motivation to choose positive health behaviors [ 1 ]. Personal health data allows individuals to become more proactive participants in their health and well-being [ 2 ]. Nonetheless, individuals are increasingly capable of generating and collecting their health data outside the clinical setting using mobile technologies. Due to the massive development of sensing technology, several applications support personal health management through smartphones or wearable devices. The data types collected during daily life can also be diverse; they include step counts from activity trackers, nutrition consumption, and sleep cycle data. These data are called self-generated health data [ 3 ]. Some data such as blood pressure and blood sugar level can be collected personally, however, may need professional medical knowledge to interpret. Along with this data, health history, symptoms, and treatment history data are called patient-generated data [ 4 ]. We distinguish the two and focus on self-generated data as they can be more generally used and understood by the general public.
The interest in personal data collection within the healthcare domain has widely increased. However, we are still in the early stage of designing effective methods of representing self-generated health data and fulfilling the diverse needs of users. Studying how people understand and interpret these self-generated results is important. Visualizations are commonly used to help individuals interpret and contextualize their health data [ 5 ]. Data visualizations are known to convey data intuitively by leveraging humans’ visual ability to perceive differences in sizes, colors, and spatial positions [ 6 ]. Although research exists on ways to make visualizations efficient and effective, research on self-generated health data is limited. In this study, we investigated how visualizations are used; what kind of data they use, how they impact users, and which factors should be considered for proper visualization design. Users can easily interpret and understand data that reflect their daily activity and lifestyle patterns. We conducted a systematic literature review on related studies of visualizations for self-generated data in the healthcare domain. We only included data collected in our daily life, which does not need medical knowledge to interpret.
When designing visualizations, it is important to define the actions that should be made by the users, determine what task should be supported, and whether the data and visualization were properly selected. To understand the various types of visualizations and how they impact the users, we analyze visualizations and different levels of efficacy such as physical and cognitive impacts. We hope our analysis of visualizations, different levels of efficacy of the applications, and understanding of individual differences will help further research on developing effective visualizations.
2. Related Works
2.1. self-tracking data.
Due to the pervasive ownership of mobile devices, extensive data-capture enables individuals to track their health data manually and passively. For example, 7 out of 10 United States adults track a health indicator (e.g., blood pressure, weight, food consumption, blood sugar, sleep) [ 7 , 8 ]. These self-generated data can be categorized in different ways. First is data collected for medical purposes with professional guidance, such as blood test results [ 9 ]. Users mostly collect these data in clinical settings to manage certain diseases like diabetes. Another type of data is collected automatically by tracking devices; examples include step counts [ 10 ] and sleep waves [ 11 ]. These data are used not only for patients but also by individuals who are interested in their health in daily activities. Lastly, some applications collect self-reported data such as personal mood through questionnaires [ 12 ]. These are mostly used for treating mental illness and rely on data that patients enter daily.
To understand the role and effectiveness of using personally generated health data, several systematic review studies have been conducted. The studies can largely be classified by studies in a clinical setting and in personal daily life. Eventually, the difference comes from how the patients could improve their health based on the data. First, in clinical practice, 21 studies showed that decision support was the main role for clinicians [ 13 ]. The authors were positive that data from patients will be part of the health care system, however, we need further study on understanding its impact on health outcomes, costs, and patient satisfaction. Additionally, Dinh-Le et al. conducted research on 10 start-up organizations that have developed wearable technology and enabled EHR integration for health systems [ 14 ]. The companies were still addressing the challenges of the meaningful use of device data. However, the majority of the companies acknowledged that technology enhances communications, self-constraint, and potential solutions to mitigate existing challenges.
On the other hand, there are also review studies on the use of personal health data in daily life. The first study focused on utilizing technology in diabetes self-management education and support services [ 15 ]. A total of 25 studies showed that technology supported healthy eating, being active and metabolic monitoring, and improvement in communication. At last, 13 studies were analyzed to explore the influence of mobile health applications on various dimensions of patient and healthcare provider relationships. The results showed that the use of mobile health applications influenced communication and relationship between the healthcare service providers and the patients [ 16 ].
2.2. Visualization
Well-designed visualizations have been shown to help fill the gap between low and high health literacy [ 17 ]. Visualizations include graphs, diagrams, and infographics, all of which present data with visual components (e.g., shape, color, and size). The rationale behind visualizations is that they attempt to leverage users’ visual skills while reducing cognitive demand for numeracy competencies.
To better understand visualizations, we categorized them into data visualization and infographics. Data visualization or information visualization is “the use of computer-supported, interactive, visual representations of abstract data to amplify cognition” [ 6 ]. These representations include simple graphs such as bar charts and new types of visualizations such as treemaps [ 18 ], which are not taught in the U.S. K-12 education system. Infographics often contain graphical elements drawn for a particular for a certain scenario or narrative. Data visualizations can be automatically generated even when the data changes, while infographics usually need manual graphical work for each case [ 19 ]. And as you see from the definition, interaction techniques such as filtering or zooming are essential for data visualizations.
Data visualization has played an important role in helping understand data, enhancing communication [ 20 ], or help the decision-making process to make better decisions [ 21 , 22 ]. Visualizations are also known to lower the cognitive load [ 23 ] which can allow users to glance through the data, but still getting a proper understanding while using mobile applications. Most self-tracking devices and their companion mobile apps provide different forms of data representation. However, due to the limited mobile display size, simple and static visualizations are commonly used (e.g., bar graphs in the FitBit app). There have several studies on evaluating the visualization on desktop environments [ 24 , 25 ]. To measure the effectiveness properly, selection of visualizations, task to be measured, expertise on the domain data and visualization, and measures for effectiveness should be carefully designed.
In June 2022, we searched scholarly databases such as PubMed, IEEE Xplore, ACM Digital Library, Scopus, and Google Scholar for articles pertaining to self-generated health data and visualizations. We selected these databases to capture various fields, including medicine, medical informatics, and computer science. Our search terms included a combination of terms such as self-generated health data, patient-generated health data, health data, data visualization, graph, daily activity, physical activity, daily habits, daily health, steps, sleep, and diet. We included studies written in English, studies of self-generated health data with applications of data visualization, and qualitative or quantitative experiments. We excluded studies completed in a clinical setting because we wanted to focus only on data that can be easily understood without medical knowledge. Domain knowledge is known to be important when interpreting visualizations and we wanted to minimize the effect of this, therefore, selected applications that utilize personal data that can be easily collected in our daily life. Additionally, we excluded research on mental health because most studies on mental health used survey questionnaires to collect data. The author also screened papers while reading titles and abstracts against the eligibility criteria. The screening process is shown in Figure 1 . Initially, we searched 1650 papers, and from the screening criteria, we eventually were left with 13 papers. For analysis, as the number of papers were only 13, a qualitative approach was applied to analyze the types of visualizations, the effectiveness of visualizations, and different level of efficacy for physical and cognitive levels.

Stages of the search process and number of selected studies at each stage.
In this section, we report the results of our analysis of 13 papers. First, we report the data that these studies collected and the visualization characteristics that they presented. This provides an overview of how visualization is utilized for personal health data within daily activities. We also analyze how the visualization was useful for the users. Based on the studies’ results regarding the efficacy of visualizations, we then suggest different levels of efficacy for users.
4.1. Visualization Characteristics
After reviewing 13 visualization studies about self-generated health data for daily activities, we determined that most of the studies related to lifestyle habits such as activity routines, as reflected by step counts, sleep cycles, and diet. We are largely able to divide visualizations into two categories: data visualization and infographics by representation. Data visualization was used to faithfully represent the collected data without visual decoration. When data included icons and metaphors, we classified them as infographics. Figure 2 includes examples of data visualizations and infographics that are similar to what has been reported in the literature. We categorized visualizations according to our definitions even when study authors specified their research topic as infographics. Data visualizations explicitly represent the numeric data in visual encoding such as height or length. On the other hand, infographics mostly have decorations using icons, additional visual aspects, or metaphors.

Table 1 reports types of data, representation type (i.e., data visualization and infographics), visualization type, and the presence of interaction techniques in the visualization for each paper. For the types of data, there were data that is collected through sensor devices such as step count, heart date, active calories, Body Mass Index (BMI), and sleep cycles. Other types of data needs to be entered by the user such as nutrition consumption, physical activities, or weight.
After analyzing the frequency of visualization representation use, we determined that six studies used only data visualization among 13 studies. Two studies only used infographics, and five used data visualization and infographics together. Of the 11 studies using data visualization, bar graphs were the most considered visualization type with nine cases. Four studies considered line graphs, and three considered stacked bar charts. Bar graphs are known to provide comparison easily and line graphs provide trend information to the users. As most of the data is collected continuously, timeline or calendar-based visualizations were also used to support the time series characteristics. Only two studies considered the user’s dynamic interaction with visualization results, such as touching and swiping. One of the studies was to evaluate commercial services that implemented a high level of interaction techniques [ 11 , 26 ]. Only one study conducted a specific experiment on the impact of visual aspects. Eikey et al. manipulated the color of an exercise progress bar and examined how the presentation of feedback impacts the users [ 27 ].
Among the studies about infographics, in the order of number line types, icon arrays, and metaphors of commonly known figures were used. Number line visualization is a visual representation that includes numerical information in an ordered manner. Based on the data, a tick shows the user’s position, and indicates whether the value is normal, healthy, or in a dangerous section. Icon array is a representation with one shape repeatedly which can be easily interpreted for counts. Several metaphors were used to help the users intuitively understand the data such as cloverleaf petals and flowers in the garden to show progress and achievement level, or glass metaphors for water consumption.
Regarding individual characteristics, two studies considered different levels of health literacy of users and developed personalized visualizations [ 28 , 29 ]. For different age groups, two studies investigated older adults as they could have different digital literacy levels and experiences with these types of digital devices [ 26 , 30 ].
Characteristics of visualizations including types of data presented, visualization type, and the presence of interaction technique.
1 Series of gender-specific body silhouettes corresponding to body mass index /waist circumference reference categories. 2 An adaptation of the “hGraph” [ 36 ]. Individual variables are plotted radially around a donut figure in which the figure represents optimal reference ranges.
4.2. Level of Efficacy
In this section, we analyzed the efficacy reported in the self-generated health data visualization studies. We found that there are different levels of utility and can be largely divided into physical impact and cognitive impact. Physical impact emphasizes the practical change of human behavior and cognitive impact emphasizes the effect on the human mind. A total of six efficacy levels were defined; four levels for cognitive impact and two levels for physical impact. In Table 2 , the bottom four rows refer to low-level impact and the top two rows reflect higher-level impacts.
This section defines each efficacy level and includes exemplar quotes or actions from related studies. First, the cognitive side has four levels: attract interest, enhance self-awareness, enhance motivation and self-efficacy, and gain insight. Attract interest refers to arousing curiosity, creating fun for users, and encouraging them to pay attention to their health conditions. In one stud of home monitoring systems that provided data on movements, participants cited the display as “providing entertainment” [ 32 ]. Enhance self-awareness refers to providing information so users can understand their health status and acquire relevant knowledge. Alrehiely et al. evaluated different types of visualizations of step count, heart rate, and active calories. A majority of the participants in their study were university students who stated that the visualizations were effective in “data comprehension and gaining knowledge (on personal data)” [ 10 ]. Ehn et al. investigated how seniors experience using activity monitors for physical activities in daily life. After using commercial apps with tablets for 9 days, one participant said, “Yes, I was positively surprised that I had taken so many steps. I hadn’t walked that much (laugh). I was positively surprised” [ 26 ]. Enhance motivation and self-efficacy refers to visualizations that encourage users to take appropriate actions based on visualized information about their health status. Schneider et al. conducted a study on promoting water consumption with visualizations and reported the following motivating effect, “I feel motivated to drink more water after looking at the visualization” [ 35 ]. Lastly, gain insight refers to visualizations providing users with meaningful interpretations and new perspectives, such as gaining a perspective on their lifestyle and reevaluating their life goals. The following statement describes this well, “Having capacity to visualize sleep data in clear and informative graphs could further help users easily understand their sleep patterns and facilitate meaningful interpretations of the data” [ 11 ].
For the physical side, promote behavioral change refers to visualizing personal health data that motivates users to increase or maintain activity. In one study, a participant using activity monitors mentioned that the visualization of physical activities helped them increase their activity: “I get pushed if I have been too lazy.” [ 26 ]. Promote effective self-care and community health refers to inducing effective self-management of individual behavioral changes and further promoting the community’s health. Arcial et al. used tailored infographics to promote community health and support community members’ comprehension of their health information [ 31 ]. The goal of these personal visualizations of health data is to help users understand their health status, provide feedback, and learn to be proactive in their health management by changing behaviors.
Among the various levels of utility, the most cited utility was “increasing an individual’s understanding of health status.” This is often individuals’ first step in engaging with their data, and it can eventually lead them to achieve higher levels of utility in visualizations. The next reported effect was “change in individual’s behavior” shown in the visualizations as a feedback mechanism. A majority of the research emphasized the positive effect of visualizations on users, however, Schneider et al. mentioned the negative effects of a poorly designed visualization, which can deter individuals from engaging with visualizations. This could come from a bad selection of the visualization, preference for certain visualizations, or personal differences in the ability to understand data or comprehend visualizations. Related work on data literacy and data visualization literacy is also becoming an important topic, especially as these abilities are critical when using visual interfaces [ 37 ].
Different levels of the efficacy of visualizations representing self-generated health data.
4.3. Impact of Individual Differences on Visualizations’ Effectiveness
Health data visualization can improve individuals’ understanding and level of health literacy, and research is being conducted to develop more intuitive and effective visualization [ 28 , 33 ]. However, the degree to which an individual understands visualized health data likely varies according to their health literacy, data literacy, digital literacy, and visualization literacy. In other words, an individual’s level of understanding of visualization may vary depending on their visualization literacy [ 37 ]. Data visualization literacy is the ability of a person to read, interpret, and construct data visualizations. To make sure that visualization is properly used or evaluated by users, service providers or researchers should take this factor into consideration and understand the level of literacy of their main audience. Researchers may need to administer visualization literacy tests to patients or users to properly understand their behavior.
5. Discussion
To increase the effectiveness of visualizations in self-generated health data, we suggest researchers and service providers consider the following key points. First, visualizations should be carefully designed by the selection of data to be shown, what type of visualization is effective, and what task should be supported by the visualization. We believe that Table 1 and Table 2 can guide the designers or developers to set the goal of the visualization and design accordingly understanding the variety of choices. Especially, as shown in Table 2 , the providers should define on what level the visualization and the app should support the user, and design the task that should be fulfilled with the visualization.
Additional factors also should be taken into consideration. As users will have varying visual literacy skills, researchers or service providers need to educate users so that they can comprehend data properly, and they should measure users’ visualization literacy level before introducing them to visualizations. Specific action guides for each visualization could be provided. Second, proper visualizations should be selected according to the data type and the main task that the developers indicated. Different visualizations support different tasks; bar charts are good for reading single data points and comparing items, while line charts help to read trends and changes over time [ 40 ]. Most data collected from tracking devices are time series data. However, several applications lack providing line charts or the ability to manipulate the time axis of visualization results. Visualizing data over time is also an important factor for comparing and providing feedback for behavioral changes shown in the data. Additionally, interactive visualizations are also important to help users engage with their data. Filtering dates or zooming actions with the finger can help users easily explore time series data.
We need a systematic way to evaluate visualizations to test their effectiveness and see whether certain visual interfaces can elicit different cognitive or physical changes. Currently, there are no consistent approaches for evaluating visualizations in the healthcare domain. Several factors can differ based on the type of data and the goal pursued, such as visualization selection, how to measure visualizations’ effectiveness, how long the study should take, personal literacy levels, and the need for education on the data or visualization. Even for a single visualization design, the selection of color, layouts, narrative texts, interactive elements, metaphor use, and number of visualizations shown in the display can all affect the readability or perceived usefulness to the user. Therefore, careful curation of these aspects is needed for proper experiments. If researchers conduct studies consistently and report them in similar formats, the results can be accumulated and eventually help to systematically approach different scenario usages.
For further research, we need to investigate the effectiveness of data visualizations compared to infographics. Even with the same data, different representations could affect curiosity, familiarity, and engagement levels. For data visualizations, additional research can be done on the effectiveness of visualizations related to certain data types in the mobile environment, which visualizations have a better impact on the behavioral changes, and the impact of longitudinal studies. For infographics, we can investigate the impact of artistic factors, the impact of narrative texts, and how users perceive metaphors in visualizations. When we have enough research in this field, we can come up with a theoretical framework, checklist, or design guidelines on visualization design for self-generated health data so that one can ensure that visualization would fulfill users’ needs and help them meet their goal.
6. Conclusions
Through a systematic literature review, we can understand that data visualizations can have a valuable impact on self-health management, empowering individuals to make cognitive and physical changes. To design better visualizations, we need to understand the characteristics of data visualizations, how these visualizations can affect users, and the individual differences among users. More attention is needed to develop and evaluate visualizations of certain data types. We find opportunities for more robust data collection and reporting and more systematic methods for evaluation that will help provide guidelines in this domain. Personal health data in nonclinical and clinical settings will continuously increase, and new digital health tools will undoubtedly be developed. We hope that this research will provide a foundation for future research.
Through this study we have analyzed the factors focused on the types of visualizations and the level of efficacy. However, to derive specific guidelines, we need to analyze specific task types, duration of the use of the service, integration level with clinical practice, and whether the applications are for only personal health or chronical management. Visualizations are sensitive and have the freedom of several design spaces. We believe that studies on several purposes will lead to effective visual interfaces.
Funding Statement
This research was supported by the MSIT (Ministry of Science and ICT), Korea, under the Grand Information Technology Research Center support program (IITP-2022-2020-0-01791) supervised by the IITP (Institute for Information & communications Technology Planning&Evaluation) and Basic Science Research Program through the National Research Foundation of Korea (NRF) funded by the Ministry if Education (2022R1F1A1066060116).
Institutional Review Board Statement
Informed consent statement, data availability statement, conflicts of interest.
The authors declare no conflict of interest.
Publisher’s Note: MDPI stays neutral with regard to jurisdictional claims in published maps and institutional affiliations.
Graph-Representation of Patient Data: a Systematic Literature Review
Affiliations.
- 1 Institute for Medical Biometry and Informatics, Heidelberg University, Im Neuenheimer Feld 130.3, 69120, Heidelberg, Germany.
- 2 School of Translational Information Technologies, ITMO University, Kronverksky Pr. 49, 197101, Saint-Petersburg, Russia.
- 3 Institute for Medical Biometry and Informatics, Heidelberg University, Im Neuenheimer Feld 130.3, 69120, Heidelberg, Germany. [email protected].
- PMID: 32166501
- PMCID: PMC7067737
- DOI: 10.1007/s10916-020-1538-4
Graph theory is a well-established theory with many methods used in mathematics to study graph structures. In the field of medicine, electronic health records (EHR) are commonly used to store and analyze patient data. Consequently, it seems straight-forward to perform research on modeling EHR data as graphs. This systematic literature review aims to investigate the frontiers of the current research in the field of graphs representing and processing patient data. We want to show, which areas of research in this context need further investigation. The databases MEDLINE, Web of Science, IEEE Xplore and ACM digital library were queried by using the search terms health record, graph and related terms. Based on the "Preferred Reporting Items for Systematic Reviews and Meta-Analysis" (PRISMA) statement guidelines the articles were screened and evaluated using full-text analysis. Eleven out of 383 articles found in systematic literature review were finally included for analysis in this literature review. Most of them use graphs to represent temporal relations, often representing the connection among laboratory data points. Only two papers report that the graph data were further processed by comparing the patient graphs using similarity measurements. Graphs representing individual patients are hardly used in research context, only eleven papers considered such kind of graphs in their investigations. The potential of graph theoretical algorithms, which are already well established, could help increasing this research field, but currently there are too few papers to estimate how this area of research will develop. Altogether, the use of such patient graphs could be a promising technique to develop decision support systems for diagnosis, medication or therapy of patients using similarity measurements or different kinds of analysis.
Keywords: Electronic health record; Graph theory; Systematic literature review; Temporal patient graph.
Publication types
- Systematic Review
- Computer Graphics*
- Electronic Data Processing
- Electronic Health Records*
Grants and funding
- 01ZX1609A/Bundesministerium für Bildung und Forschung

IMAGES
VIDEO
COMMENTS
Generally, data visualization involves representing data and information in various forms, such as graphs, charts, diagrams, and pictures [ 1 ]. These visualization techniques can provide healthcare providers with an easy way to identify and understand data trends, outliers, and patterns [ 2 ]. Visualization techniques have been essential in ...
Introduction. Visual learning is one of the primary forms of interpreting information, which has historically combined images such as charts and graphs (see Box 1) with reading text. 1 However, developments on learning styles have suggested splitting up the visual learning modality in order to recognize the distinction between text and images. 2 Technology has also enhanced visual presentation ...
Each kind of graphic has specific characteristics, suitable according with health data type. Health professionals must be aware of this visual data representations in order to use them correctly but also to avoid interpretation mistakes. Visual representations are fundamental in making prompt decisions and can be compiled into dashboards ...
This translates into a powerful technique for using big health care data, allowing a deep exploratory dive without an a priori hypothesis to identify data-driven trends and patterns. In this review, although we observed various applications of interactive visualization, we found limited evidence of its use in global health.
Oral Presentations. • Only include important results. • One report table might need to be broken down into as many as 8‐10 slides. • Don't paste huge tables onto slides and then say "sorry you can't read this"!! • Use large fonts and clear formatting. Table 1.
A large body of research about how people process information demonstrates that data selection and presentation influence comprehension, decision making, and behavior in health and other areas. 2-8 Conversely, poor data selection and presentation can lead to audience confusion, 9-12 medical errors, 13-15 flawed policy making, 10, 16, 17 ...
Encourage the eye to compare different pieces of data. Reveal the data at several levels of detail, from a broad overview to the fine structure. Serve a clear purpose: description, exploration, tabulation, or decoration. Be closely integrated with the statistical and verbal descriptions of the data set. From E. R. Tufte.
Visualization in healthcare involves the effective presentation of information through graphics, images, and videos. Big data systems handle a massive amount of information and require visualization techniques to present it in a comprehensible manner. The significance of visualization techniques in healthcare is not confined to healthcare ...
Visualization makes it easier for the audience to grasp and recall data. It should be presented in an attractive style to create interest in audience's mind; Data may be presented as a table or graphic. There are 3 types of tables: Formal (numbers), textural, and matrix; each one has its peculiarities and usefulness.
Numeracy and literacy may influence comprehension and compliance with screen designs or information flows; use of pictograms over tabular presentation of on-screen data may improve comprehension ...
3.2.4.3. Provide (public) access to information. The legitimation of political-administrative decision-making by means of data visualization through public health dashboards played a marginal role in the reviewed literature and, even more so, was not mentioned as a particular objective of information provision.
A graph or chart or diagram is a diagrammatical illustration of a set of data. Graphs are nothing but the chart hat helps us to convey the information and yes of course to make sense of the data ...
Several themes emerged from our review of the empirical literature specifically addressing the graphical presentation of PRO data to patients and clinicians, although we found few papers explicitly focused on this topic. ... The impact of the format of graphical presentation on health-related knowledge and treatment choices. Patient Educ. Couns ...
This chapter provides a background on the most common formats in which data can be presented visually, and how such data can be interpreted. It looks at methods of presenting both categorical and continuously variable data and reviews software packages that can produce excellent figures suitable for presentation and publication, limiting the requirement for hand-drawn artistic skills.
Data Interpretation Process. Data visualization is most commonly experienced as a static display, such as a bar graph, bubble chart or other type of graphic. The analytical process of how the data is transformed into a visualization, presented to an audience, and perceived by an audience is dynamic, as described below: Numbers or raw data is ...
Graph theory is a well-established theory with many methods used in mathematics to study graph structures. In the field of medicine, electronic health records (EHR) are commonly used to store and analyze patient data. Consequently, it seems straight-forward to perform research on modeling EHR data as graphs. This systematic literature review aims to investigate the frontiers of the current ...
Introduction 'Infographic' is an abbreviated term for an information graphic [].Infographics generally combine text, images and data visualisations to present information visually, increase the attention it receives, and to improve comprehension and recall [1-3].Infographics are becoming increasingly popular as a method for summarising research findings [3-5], although they often have ...
Abstract. Abstract: The purpose of this study was to identify the method of presentation (text, bar graphs, or pictographs) that best enhances the understanding of medical risk information among Japanese adults. We also investigated the effect of graphic format on understanding by differences in numeracy. This is the first study conducted in Japan to clarify understanding the risks and ...
DOI: 10.1016/j.pec.2008.07.023 Corpus ID: 43214606; The impact of the format of graphical presentation on health-related knowledge and treatment choices. @article{Hawley2008TheIO, title={The impact of the format of graphical presentation on health-related knowledge and treatment choices.}, author={Sarah T. Hawley and Brian J. Zikmund‐Fisher and Peter A Ubel and Aleksandra Jancovic and Todd ...
Health Sciences, Riyadh, SAU 4. ... visualization techniques, graphical presentation, big data, visualization ... presentation of healthcare data regarding different aspects, including disease ...
4.1. Visualization Characteristics. After reviewing 13 visualization studies about self-generated health data for daily activities, we determined that most of the studies related to lifestyle habits such as activity routines, as reflected by step counts, sleep cycles, and diet.
This systematic literature review aims to investigate the frontiers of the current research in the field of graphs representing and processing patient data. We want to show, which areas of research in this context need further investigation. The databases MEDLINE, Web of Science, IEEE Xplore and ACM digital library were queried by using the ...